doc-hf4zqe80k7qgq11
XP安装序列号
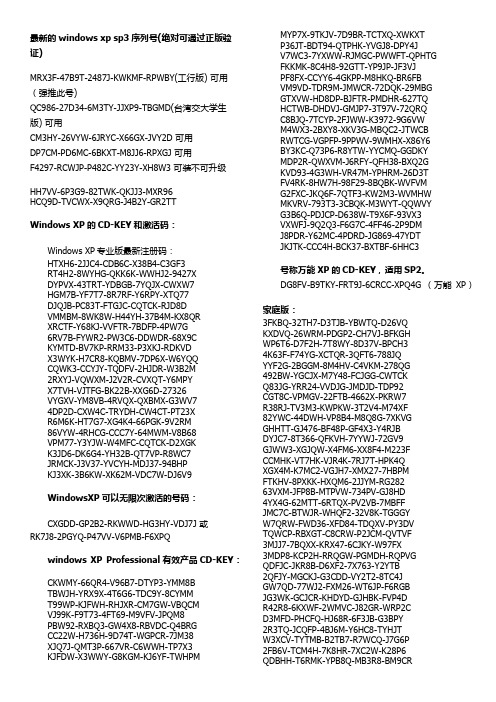
最新的windows xp sp3序列号(绝对可通过正版验证)MRX3F-47B9T-2487J-KWKMF-RPWBY(工行版) 可用(强推此号)QC986-27D34-6M3TY-JJXP9-TBGMD(台湾交大学生版) 可用CM3HY-26VYW-6JRYC-X66GX-JVY2D 可用DP7CM-PD6MC-6BKXT-M8JJ6-RPXGJ 可用F4297-RCWJP-P482C-YY23Y-XH8W3 可装不可升级HH7VV-6P3G9-82TWK-QKJJ3-MXR96HCQ9D-TVCWX-X9QRG-J4B2Y-GR2TTWindows XP的CD-KEY和激活码:Windows XP专业版最新注册码:HTXH6-2JJC4-CDB6C-X38B4-C3GF3RT4H2-8WYHG-QKK6K-WWHJ2-9427XDYPVX-43TRT-YDBGB-7YQJX-CWXW7HGM7B-YF7T7-8R7RF-Y6RPY-XTQ77DJQJB-PC83T-FTGJC-CQTCK-RJD8DVMMBM-8WK8W-H44YH-37B4M-KX8QRXRCTF-Y68KJ-VVFTR-7BDFP-4PW7G6RV7B-FYWR2-PW3C6-DDWDR-68X9CKYMTD-BV7KP-RRM33-P3XKJ-RDKVDX3WYK-H7CR8-KQBMV-7DP6X-W6YQQCQWK3-CCYJY-TQDFV-2HJDR-W3B2M2RXYJ-VQWXM-J2V2R-CVXQT-Y6MPYX7TVH-VJTFG-BK22B-XXG6D-27326VYGXV-YM8VB-4RVQX-QXBMX-G3WV74DP2D-CXW4C-TRYDH-CW4CT-PT23XR6M6K-HT7G7-XG4K4-66PGK-9V2RM86VYW-4RHCG-CCC7Y-64MWM-V8B68VPM77-Y3YJW-W4MFC-CQTCK-D2XGKK3JD6-DK6G4-YH32B-QT7VP-R8WC7JRMCK-J3V37-YVCYH-MDJ37-94BHPKJ3XK-3B6KW-XK62M-VDC7W-DJ6V9WindowsXP可以无限次激活的号码:CXGDD-GP2B2-RKWWD-HG3HY-VDJ7J 或RK7J8-2PGYQ-P47VV-V6PMB-F6XPQwindows XP Professional有效产品CD-KEY:CKWMY-66QR4-V96B7-DTYP3-YMM8BTBWJH-YRX9X-4T6G6-TDC9Y-8CYMMT99WP-KJFWH-RHJXR-CM7GW-VBQCMVJ99K-F9T73-4FT69-M9VFV-JPQM8PBW92-RXBQ3-GW4X8-RBVDC-Q4BRGCC22W-H736H-9D74T-WGPCR-7JM38XJQ7J-QMT3P-667VR-C6WWH-TP7X3KJFDW-X3WWY-G8KGM-KJ6YF-TWHPMMYP7X-9TKJV-7D9BR-TCTXQ-XWKXTP36JT-BDT94-QTPHK-YVGJ8-DPY4JV7WC3-7YXWW-RJMGC-PWWFT-QPHTGFKKMK-8C4H8-92GTT-YP9JP-JF3VJPF8FX-CCYY6-4GKPP-M8HKQ-BR6FBVM9VD-TDR9M-JMWCR-72DQK-29MBGGTXVW-HD8DP-BJFTR-PMDHR-627TQHCTWB-DHDVJ-GMJP7-3T97V-72QRQC8BJQ-7TCYP-2FJWW-K3972-9G6VWM4WX3-2BXY8-XKV3G-MBQC2-JTWCBRWTCG-VGPFP-9PPWV-9WMHX-X86Y6BY3KC-Q73P6-R8YTW-YYCMQ-GGDKYMDP2R-QWXVM-J6RFY-QFH38-BXQ2GKVD93-4G3WH-VR47M-YPHRM-26D3TFV4RK-8HW7H-98F29-8BQBK-WVFVMG2FXC-JKQ6F-7QTF3-KW2M3-WVMHWMKVRV-793T3-3CBQK-M3WYT-QQWVYG3B6Q-PDJCP-D638W-T9X6F-93VX3VXWFJ-9Q2Q3-F6G7C-4FF46-2P9DMJ8PDR-Y62MC-4PDRD-JG869-47YDTJKJTK-CCC4H-BCK37-BXTBF-6HHC3号称万能XP的CD-KEY,适用SP2。
wn7激活码
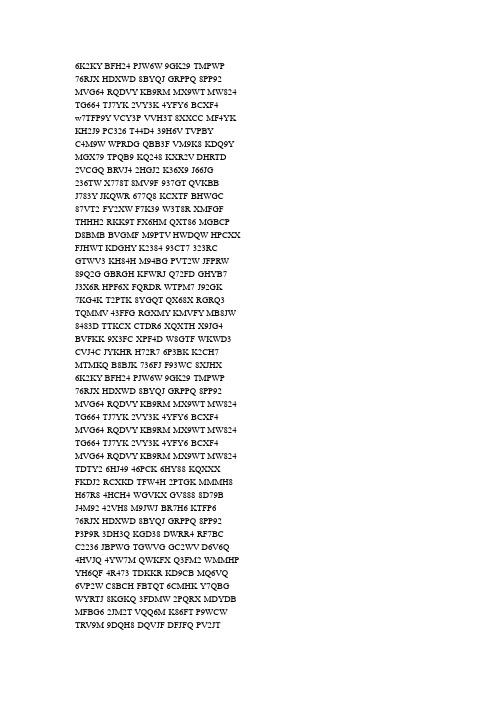
6K2KY-BFH24-PJW6W-9GK29-TMPWP76RJX-HDXWD-8BYQJ-GRPPQ-8PP92 MVG64-RQDVY-KB9RM-MX9WT-MW824 TG664-TJ7YK-2VY3K-4YFY6-BCXF4w7TFP9Y-VCY3P-VVH3T-8XXCC-MF4YK KH2J9-PC326-T44D4-39H6V-TVPBYC4M9W-WPRDG-QBB3F-VM9K8-KDQ9Y MGX79-TPQB9-KQ248-KXR2V-DHRTD2VCGQ-BRVJ4-2HGJ2-K36X9-J66JG236TW-X778T-8MV9F-937GT-QVKBBJ783Y-JKQWR-677Q8-KCXTF-BHWGC87VT2-FY2XW-F7K39-W3T8R-XMFGF THHH2-RKK9T-FX6HM-QXT86-MGBCPD8BMB-BVGMF-M9PTV-HWDQW-HPCXX FJHWT-KDGHY-K2384-93CT7-323RC GTWV3-KH84H-M94BG-PVT2W-JFPRW 89Q2G-GBRGH-KFWRJ-Q72FD-GHYB7J3X6R-HPF6X-FQRDR-WTPM7-J92GK7KG4K-T2PTK-8YGQT-QX68X-RGRQ3 TQMMV-43FFG-RGXMY-KMVFY-MB8JW 8483D-TTKCX-CTDR6-XQXTH-X9JG4 BVFKK-9X3FC-XPF4D-W8GTF-WKWD3 CVJ4C-JYKHR-H72R7-6P3BK-K2CH7 MTMKQ-B8BJK-736FJ-F93WC-8XJHX6K2KY-BFH24-PJW6W-9GK29-TMPWP76RJX-HDXWD-8BYQJ-GRPPQ-8PP92 MVG64-RQDVY-KB9RM-MX9WT-MW824 TG664-TJ7YK-2VY3K-4YFY6-BCXF4 MVG64-RQDVY-KB9RM-MX9WT-MW824 TG664-TJ7YK-2VY3K-4YFY6-BCXF4 MVG64-RQDVY-KB9RM-MX9WT-MW824 TDTY2-6HJ49-46PCK-6HY88-KQXXX FKDJ2-RCXKD-TFW4H-2PTGK-MMMH8 H67R8-4HCH4-WGVKX-GV888-8D79BJ4M92-42VH8-M9JWJ-BR7H6-KTFP676RJX-HDXWD-8BYQJ-GRPPQ-8PP92P3P9R-3DH3Q-KGD38-DWRR4-RF7BCC2236-JBPWG-TGWVG-GC2WV-D6V6Q4HVJQ-4YW7M-QWKFX-Q3FM2-WMMHP YH6QF-4R473-TDKKR-KD9CB-MQ6VQ6VP2W-C8BCH-FBTQT-6CMHK-Y7QBG WYRTJ-8KGKQ-3FDMW-2PQRX-MDYDB MFBG6-2JM2T-VQQ6M-K86FT-P9WCW TRV9M-9DQH8-DQVJF-DFJFQ-PV2JTJ783Y-JKQWR-677Q8-KCXTF-BHWGCC4M9W-WPRDG-QBB3F-VM9K8-KDQ9Y 2VCGQ-BRVJ4-2HGJ2-K36X9-J66JGMGX79-TPQB9-KQ248-KXR2V-DHRTD FJHWT-KDGHY-K2384-93CT7-323RC THHH2-RKK9T-FX6HM-QXT86-MGBCP KH2J9-PC326-T44D4-39H6V-TVPBYD8BMB-BVGMF-M9PTV-HWDQW-HPCXX TFP9Y-VCY3P-VVH3T-8XXCC-MF4YK TG664-TJ7YK-2VY3K-4YFY6-BCXF4 MVG64-RQDVY-KB9RM-MX9WT-MW824 TDTY2-6HJ49-46PCK-6HY88-KQXXX FKDJ2-RCXKD-TFW4H-2PTGK-MMMH8 H67R8-4HCH4-WGVKX-GV888-8D79BJ4M92-42VH8-M9JWJ-BR7H6-KTFP676RJX-HDXWD-8BYQJ-GRPPQ-8PP92P3P9R-3DH3Q-KGD38-DWRR4-RF7BCC2236-JBPWG-TGWVG-GC2WV-D6V6Q4HVJQ-4YW7M-QWKFX-Q3FM2-WMMHP YH6QF-4R473-TDKKR-KD9CB-MQ6VQ6VP2W-C8BCH-FBTQT-6CMHK-Y7QBG WYRTJ-8KGKQ-3FDMW-2PQRX-MDYDB MFBG6-2JM2T-VQQ6M-K86FT-P9WCW TRV9M-9DQH8-DQVJF-DFJFQ-PV2JTBB3K3-MMTHM-WFWJK-PCC8G-3DRGQ J6QGR-6CFJQ-C4HKH-RJPVP-7V83XTC48D-Y44RV-34R62-VQRK8-64VYGCTT6T-PWYJD-327V6-W2BWW-RJ29TBP8HR-QV3B8-WG24X-RQ3H3-DK67M TFP9Y-VCY3P-VVH3T-8XXCC-MF4YK87VT2-FY2XW-F7K39-W3T8R-XMFGF 236TW-X778T-8MV9F-937GT-QVKBBBB3K3-MMTHM-WFWJK-PCC8G-3DRGQ J6QGR-6CFJQ-C4HKH-RJPVP-7V83XTC48D-Y44RV-34R62-VQRK8-64VYG76RJX-HDXWD-8BYQJ-GRPPQ-8PP92 MVG64-RQDVY-KB9RM-MX9WT-MW824 CTT6T-PWYJD-327V6-W2BWW-RJ29TBP8HR-QV3B8-WG24X-RQ3H3-DK67M TDTY2-6HJ49-46PCK-6HY88-KQXXX6VP2W-C8BCH-FBTQT-6CMHK-Y7QBG FKDJ2-RCXKD-TFW4H-2PTGK-MMMH8 H67R8-4HCH4-WGVKX-GV888-8D79BJ4M92-42VH8-M9JWJ-BR7H6-KTFP676RJX-HDXWD-8BYQJ-GRPPQ-8PP926K2KY-BFH24-PJW6W-9GK29-TMPWPP3P9R-3DH3Q-KGD38-DWRR4-RF7BCC2236-JBPWG-TGWVG-GC2WV-D6V6Q4HVJQ-4YW7M-QWKFX-Q3FM2-WMMHP YH6QF-4R473-TDKKR-KD9CB-MQ6VQ6VP2W-C8BCH-FBTQT-6CMHK-Y7QBG WYRTJ-8KGKQ-3FDMW-2PQRX-MDYDB MFBG6-2JM2T-VQQ6M-K86FT-P9WCW TRV9M-9DQH8-DQVJF-DFJFQ-PV2JTBB3K3-MMTHM-WFWJK-PCC8G-3DRGQ J6QGR-6CFJQ-C4HKH-RJPVP-7V83XTC48D-Y44RV-34R62-VQRK8-64VYGCTT6T-PWYJD-327V6-W2BWW-RJ29TBP8HR-QV3B8-WG24X-RQ3H3-DK67M6JKV2-QPB8H-RQ893-FW7TM-PBJ73TQ32R-WFBDM-GFHD2-QGVMH-3P9GC GG4MQ-MGK72-HVXFW-KHCRF-KW6KY 4HJRK-X6Q28-HWRFY-WDYHJ-K8HDH 76RJX-HDXWD-8BYQJ-GRPPQ-8PP92 QXV7B-K78W2-QGPR6-9FWH9-KGMM7 236TW-X778T-8MV9F-937GT-QVKBBJ783Y-JKQWR-677Q8-KCXTF-BHWGC87VT2-FY2XW-F7K39-W3T8R-XMFGF THHH2-RKK9T-FX6HM-QXT86-MGBCPD8BMB-BVGMF-M9PTV-HWDQW-HPCXX FJHWT-KDGHY-K2384-93CT7-323RC GTWV3-KH84H-M94BG-PVT2W-JFPRW 89Q2G-GBRGH-KFWRJ-Q72FD-GHYB7J3X6R-HPF6X-FQRDR-WTPM7-J92GK7KG4K-T2PTK-8YGQT-QX68X-RGRQ3 TQMMV-43FFG-RGXMY-KMVFY-MB8JW 8483D-TTKCX-CTDR6-XQXTH-X9JG4 BVFKK-9X3FC-XPF4D-W8GTF-WKWD3 CVJ4C-JYKHR-H72R7-6P3BK-K2CH7 MTMKQ-B8BJK-736FJ-F93WC-8XJHXTG664-TJ7YK-2VY3K-4YFY6-BCXF4 MVG64-RQDVY-KB9RM-MX9WT-MW824 TDTY2-6HJ49-46PCK-6HY88-KQXXX FKDJ2-RCXKD-TFW4H-2PTGK-MMMH8 H67R8-4HCH4-WGVKX-GV888-8D79BJ4M92-42VH8-M9JWJ-BR7H6-KTFP676RJX-HDXWD-8BYQJ-GRPPQ-8PP92P3P9R-3DH3Q-KGD38-DWRR4-RF7BCC2236-JBPWG-TGWVG-GC2WV-D6V6Q4HVJQ-4YW7M-QWKFX-Q3FM2-WMMHP YH6QF-4R473-TDKKR-KD9CB-MQ6VQ6VP2W-C8BCH-FBTQT-6CMHK-Y7QBG WYRTJ-8KGKQ-3FDMW-2PQRX-MDYDB MFBG6-2JM2T-VQQ6M-K86FT-P9WCW TRV9M-9DQH8-DQVJF-DFJFQ-PV2JTBB3K3-MMTHM-WFWJK-PCC8G-3DRGQ J6QGR-6CFJQ-C4HKH-RJPVP-7V83XTC48D-Y44RV-34R62-VQRK8-64VYG6VP2W-C8BCH-FBTQT-6CMHK-Y7QBG CTT6T-PWYJD-327V6-W2BWW-RJ29TBP8HR-QV3B8-WG24X-RQ3H3-DK67M TG664-TJ7YK-2VY3K-4YFY6-BCXF4 MVG64-RQDVY-KB9RM-MX9WT-MW824 TDTY2-6HJ49-46PCK-6HY88-KQXXX FKDJ2-RCXKD-TFW4H-2PTGK-MMMH8 H67R8-4HCH4-WGVKX-GV888-8D79B76RJX-HDXWD-8BYQJ-GRPPQ-8PP92J4M92-42VH8-M9JWJ-BR7H6-KTFP676RJX-HDXWD-8BYQJ-GRPPQ-8PP92P3P9R-3DH3Q-KGD38-DWRR4-RF7BCC2236-JBPWG-TGWVG-GC2WV-D6V6Q4HVJQ-4YW7M-QWKFX-Q3FM2-WMMHP YH6QF-4R473-TDKKR-KD9CB-MQ6VQ6VP2W-C8BCH-FBTQT-6CMHK-Y7QBG WYRTJ-8KGKQ-3FDMW-2PQRX-MDYDB MFBG6-2JM2T-VQQ6M-K86FT-P9WCW TRV9M-9DQH8-DQVJF-DFJFQ-PV2JTBB3K3-MMTHM-WFWJK-PCC8G-3DRGQ J6QGR-6CFJQ-C4HKH-RJPVP-7V83XTC48D-Y44RV-34R62-VQRK8-64VYG CTT6T-PWYJD-327V6-W2BWW-RJ29TBP8HR-QV3B8-WG24X-RQ3H3-DK67M TFP9Y-VCY3P-VVH3T-8XXCC-MF4YK KH2J9-PC326-T44D4-39H6V-TVPBY236TW-X778T-8MV9F-937GT-QVKBB87VT2-FY2XW-F7K39-W3T8R-XMFGFJ783Y-JKQWR-677Q8-KCXTF-BHWGCC4M9W-WPRDG-QBB3F-VM9K8-KDQ9Y 2VCGQ-BRVJ4-2HGJ2-K36X9-J66JG76RJX-HDXWD-8BYQJ-GRPPQ-8PP92 MGX79-TPQB9-KQ248-KXR2V-DHRTD FJHWT-KDGHY-K2384-93CT7-323RCTHHH2-RKK9T-FX6HM-QXT86-MGBCP KH2J9-PC326-T44D4-39H6V-TVPBYD8BMB-BVGMF-M9PTV-HWDQW-HPCXX TFP9Y-VCY3P-VVH3T-8XXCC-MF4YK TG664-TJ7YK-2VY3K-4YFY6-BCXF4 MVG64-RQDVY-KB9RM-MX9WT-MW824 TDTY2-6HJ49-46PCK-6HY88-KQXXX FKDJ2-RCXKD-TFW4H-2PTGK-MMMH8 H67R8-4HCH4-WGVKX-GV888-8D79BJ4M92-42VH8-M9JWJ-BR7H6-KTFP676RJX-HDXWD-8BYQJ-GRPPQ-8PP92P3P9R-3DH3Q-KGD38-DWRR4-RF7BCC2236-JBPWG-TGWVG-GC2WV-D6V6Q4HVJQ-4YW7M-QWKFX-Q3FM2-WMMHP 236TW-X778T-8MV9F-937GT-QVKBB87VT2-FY2XW-F7K39-W3T8R-XMFGFJ783Y-JKQWR-677Q8-KCXTF-BHWGC76RJX-HDXWD-8BYQJ-GRPPQ-8PP92 MVG64-RQDVY-KB9RM-MX9WT-MW824 C4M9W-WPRDG-QBB3F-VM9K8-KDQ9Y 2VCGQ-BRVJ4-2HGJ2-K36X9-J66JGMGX79-TPQB9-KQ248-KXR2V-DHRTD 236TW-X778T-8MV9F-937GT-QVKBB87VT2-FY2XW-F7K39-W3T8R-XMFGFKH2J9-PC326-T44D4-39H6V-TVPBY6VP2W-C8BCH-FBTQT-6CMHK-Y7QBG TFP9Y-VCY3P-VVH3T-8XXCC-MF4YK76RJX-HDXWD-8BYQJ-GRPPQ-8PP92 MVG64-RQDVY-KB9RM-MX9WT-MW824 6K2KY-BFH24-PJW6W-9GK29-TMPWP6K2KY-BFH24-PJW6W-9GK29-TMPWP76RJX-HDXWD-8BYQJ-GRPPQ-8PP92 MVG64-RQDVY-KB9RM-MX9WT-MW824 TG664-TJ7YK-2VY3K-4YFY6-BCXF4 MVG64-RQDVY-KB9RM-MX9WT-MW824 TG664-TJ7YK-2VY3K-4YFY6-BCXF4 MVG64-RQDVY-KB9RM-MX9WT-MW824 TDTY2-6HJ49-46PCK-6HY88-KQXXX FKDJ2-RCXKD-TFW4H-2PTGK-MMMH8 H67R8-4HCH4-WGVKX-GV888-8D79BJ4M92-42VH8-M9JWJ-BR7H6-KTFP676RJX-HDXWD-8BYQJ-GRPPQ-8PP92P3P9R-3DH3Q-KGD38-DWRR4-RF7BCC2236-JBPWG-TGWVG-GC2WV-D6V6Q4HVJQ-4YW7M-QWKFX-Q3FM2-WMMHP YH6QF-4R473-TDKKR-KD9CB-MQ6VQ6VP2W-C8BCH-FBTQT-6CMHK-Y7QBG WYRTJ-8KGKQ-3FDMW-2PQRX-MDYDB MFBG6-2JM2T-VQQ6M-K86FT-P9WCW TRV9M-9DQH8-DQVJF-DFJFQ-PV2JTBB3K3-MMTHM-WFWJK-PCC8G-3DRGQ J6QGR-6CFJQ-C4HKH-RJPVP-7V83XTC48D-Y44RV-34R62-VQRK8-64VYG76RJX-HDXWD-8BYQJ-GRPPQ-8PP92 MVG64-RQDVY-KB9RM-MX9WT-MW824 CTT6T-PWYJD-327V6-W2BWW-RJ29TBP8HR-QV3B8-WG24X-RQ3H3-DK67M TDTY2-6HJ49-46PCK-6HY88-KQXXX6VP2W-C8BCH-FBTQT-6CMHK-Y7QBG FKDJ2-RCXKD-TFW4H-2PTGK-MMMH8 H67R8-4HCH4-WGVKX-GV888-8D79BJ4M92-42VH8-M9JWJ-BR7H6-KTFP676RJX-HDXWD-8BYQJ-GRPPQ-8PP926K2KY-BFH24-PJW6W-9GK29-TMPWPP3P9R-3DH3Q-KGD38-DWRR4-RF7BCC2236-JBPWG-TGWVG-GC2WV-D6V6Q4HVJQ-4YW7M-QWKFX-Q3FM2-WMMHP YH6QF-4R473-TDKKR-KD9CB-MQ6VQ6VP2W-C8BCH-FBTQT-6CMHK-Y7QBG WYRTJ-8KGKQ-3FDMW-2PQRX-MDYDB MFBG6-2JM2T-VQQ6M-K86FT-P9WCW TRV9M-9DQH8-DQVJF-DFJFQ-PV2JTBB3K3-MMTHM-WFWJK-PCC8G-3DRGQ J6QGR-6CFJQ-C4HKH-RJPVP-7V83XTC48D-Y44RV-34R62-VQRK8-64VYGCTT6T-PWYJD-327V6-W2BWW-RJ29TBP8HR-QV3B8-WG24X-RQ3H3-DK67M6JKV2-QPB8H-RQ893-FW7TM-PBJ73TQ32R-WFBDM-GFHD2-QGVMH-3P9GC GG4MQ-MGK72-HVXFW-KHCRF-KW6KY 4HJRK-X6Q28-HWRFY-WDYHJ-K8HDH 76RJX-HDXWD-8BYQJ-GRPPQ-8PP92 QXV7B-K78W2-QGPR6-9FWH9-KGMM7 236TW-X778T-8MV9F-937GT-QVKBBJ783Y-JKQWR-677Q8-KCXTF-BHWGC87VT2-FY2XW-F7K39-W3T8R-XMFGF THHH2-RKK9T-FX6HM-QXT86-MGBCPD8BMB-BVGMF-M9PTV-HWDQW-HPCXXFJHWT-KDGHY-K2384-93CT7-323RC GTWV3-KH84H-M94BG-PVT2W-JFPRW 89Q2G-GBRGH-KFWRJ-Q72FD-GHYB7J3X6R-HPF6X-FQRDR-WTPM7-J92GK7KG4K-T2PTK-8YGQT-QX68X-RGRQ3 TQMMV-43FFG-RGXMY-KMVFY-MB8JW 8483D-TTKCX-CTDR6-XQXTH-X9JG4 BVFKK-9X3FC-XPF4D-W8GTF-WKWD3 CVJ4C-JYKHR-H72R7-6P3BK-K2CH7 MTMKQ-B8BJK-736FJ-F93WC-8XJHXTG664-TJ7YK-2VY3K-4YFY6-BCXF4 MVG64-RQDVY-KB9RM-MX9WT-MW824 TDTY2-6HJ49-46PCK-6HY88-KQXXX FKDJ2-RCXKD-TFW4H-2PTGK-MMMH8 H67R8-4HCH4-WGVKX-GV888-8D79BJ4M92-42VH8-M9JWJ-BR7H6-KTFP676RJX-HDXWD-8BYQJ-GRPPQ-8PP92P3P9R-3DH3Q-KGD38-DWRR4-RF7BCC2236-JBPWG-TGWVG-GC2WV-D6V6Q4HVJQ-4YW7M-QWKFX-Q3FM2-WMMHP YH6QF-4R473-TDKKR-KD9CB-MQ6VQ6K2KY-BFH24-PJW6W-9GK29-TMPWP6VP2W-C8BCH-FBTQT-6CMHK-Y7QBG WYRTJ-8KGKQ-3FDMW-2PQRX-MDYDB MFBG6-2JM2T-VQQ6M-K86FT-P9WCW TRV9M-9DQH8-DQVJF-DFJFQ-PV2JTBB3K3-MMTHM-WFWJK-PCC8G-3DRGQ J6QGR-6CFJQ-C4HKH-RJPVP-7V83XTC48D-Y44RV-34R62-VQRK8-64VYG6VP2W-C8BCH-FBTQT-6CMHK-Y7QBG CTT6T-PWYJD-327V6-W2BWW-RJ29TBP8HR-QV3B8-WG24X-RQ3H3-DK67M TG664-TJ7YK-2VY3K-4YFY6-BCXF4 MVG64-RQDVY-KB9RM-MX9WT-MW824 TDTY2-6HJ49-46PCK-6HY88-KQXXX FKDJ2-RCXKD-TFW4H-2PTGK-MMMH8 H67R8-4HCH4-WGVKX-GV888-8D79B76RJX-HDXWD-8BYQJ-GRPPQ-8PP92J4M92-42VH8-M9JWJ-BR7H6-KTFP676RJX-HDXWD-8BYQJ-GRPPQ-8PP92P3P9R-3DH3Q-KGD38-DWRR4-RF7BCC2236-JBPWG-TGWVG-GC2WV-D6V6Q4HVJQ-4YW7M-QWKFX-Q3FM2-WMMHP YH6QF-4R473-TDKKR-KD9CB-MQ6VQ6VP2W-C8BCH-FBTQT-6CMHK-Y7QBGWYRTJ-8KGKQ-3FDMW-2PQRX-MDYDB 6K2KY-BFH24-PJW6W-9GK29-TMPWP MFBG6-2JM2T-VQQ6M-K86FT-P9WCW TRV9M-9DQH8-DQVJF-DFJFQ-PV2JTBB3K3-MMTHM-WFWJK-PCC8G-3DRGQ J6QGR-6CFJQ-C4HKH-RJPVP-7V83X TC48D-Y44RV-34R62-VQRK8-64VYG CTT6T-PWYJD-327V6-W2BWW-RJ29TBP8HR-QV3B8-WG24X-RQ3H3-DK67M TFP9Y-VCY3P-VVH3T-8XXCC-MF4YK KH2J9-PC326-T44D4-39H6V-TVPBY 236TW-X778T-8MV9F-937GT-QVKBB87VT2-FY2XW-F7K39-W3T8R-XMFGF J783Y-JKQWR-677Q8-KCXTF-BHWGCC4M9W-WPRDG-QBB3F-VM9K8-KDQ9Y 2VCGQ-BRVJ4-2HGJ2-K36X9-J66JG76RJX-HDXWD-8BYQJ-GRPPQ-8PP92 MGX79-TPQB9-KQ248-KXR2V-DHRTD FJHWT-KDGHY-K2384-93CT7-323RC THHH2-RKK9T-FX6HM-QXT86-MGBCP KH2J9-PC326-T44D4-39H6V-TVPBY D8BMB-BVGMF-M9PTV-HWDQW-HPCXX TFP9Y-VCY3P-VVH3T-8XXCC-MF4YK TG664-TJ7YK-2VY3K-4YFY6-BCXF4 MVG64-RQDVY-KB9RM-MX9WT-MW824 TDTY2-6HJ49-46PCK-6HY88-KQXXX 6K2KY-BFH24-PJW6W-9GK29-TMPWP FKDJ2-RCXKD-TFW4H-2PTGK-MMMH8 H67R8-4HCH4-WGVKX-GV888-8D79BJ4M92-42VH8-M9JWJ-BR7H6-KTFP676RJX-HDXWD-8BYQJ-GRPPQ-8PP92P3P9R-3DH3Q-KGD38-DWRR4-RF7BCC2236-JBPWG-TGWVG-GC2WV-D6V6Q4HVJQ-4YW7M-QWKFX-Q3FM2-WMMHP236TW-X778T-8MV9F-937GT-QVKBB87VT2-FY2XW-F7K39-W3T8R-XMFGFJ783Y-JKQWR-677Q8-KCXTF-BHWGC76RJX-HDXWD-8BYQJ-GRPPQ-8PP92MVG64-RQDVY-KB9RM-MX9WT-MW824C4M9W-WPRDG-QBB3F-VM9K8-KDQ9Y2VCGQ-BRVJ4-2HGJ2-K36X9-J66JGMGX79-TPQB9-KQ248-KXR2V-DHRTD6K2KY-BFH24-PJW6W-9GK29-TMPWP236TW-X778T-8MV9F-937GT-QVKBB87VT2-FY2XW-F7K39-W3T8R-XMFGFKH2J9-PC326-T44D4-39H6V-TVPBY6VP2W-C8BCH-FBTQT-6CMHK-Y7QBGTFP9Y-VCY3P-VVH3T-8XXCC-MF4YK76RJX-HDXWD-8BYQJ-GRPPQ-8PP92MVG64-RQDVY-KB9RM-MX9WT-MW8246K2KY-BFH24-PJW6W-9GK29-TMPWP。
office产品密钥

Microsoft Office 家庭与学生版 2007 中文版产品密钥
JX8J3-8VWYT-3WMBD-FVDKB-K7H23
V2XPX-HBBRX-7VJBD-86FX4-XM8XJ
PD9FC-HM9C2-Q3H34-DY3F3-WF726
Microsoft Office Visio 标准版 2007 中文版产品密钥
PY4FB-WV7V9-F7W7H-GH6Y4-QWJPQ
TRFQP-JVQFJ-98J9R-VT8RQ-XBCCQ
Microsoft Office Visio 标准版 2007英文版产品密钥
KWDBY-7XRCJ-TTC93-RHBYR-2VHTD
WFH6D-MYWGM-C3RJM-PKYCH-YFKJ3
WMQMJ-P3PHT-GGXHG-FHW3T-9B8PQ
Microsoft Office Visio 专业版 2007 中文版产品密钥
GXVD9-6MQ22-X98WF-BJBQG-VPTW3
BY9XR-GHW74-GV7MF-873WC-33VTG
QKCD2-GP6BK-WKFV8-V63GF-9HYGG
Microsoft Office Publisher 2007英文版产品密钥
JJB7M-HYTGH-WQ8TR-C27TV-49WPW
H4J9V-RCWYR-QPQD2-GVW7C-RF7TJ
特殊的版本(例如企业版、繁体版等)产品密钥 :
P2KDC-9HMXH-9QFVK-PMQCB-V2XMM
BMV8D-G272X-MHMXW-4DY9G-M8YTQ
A correspondence between maximal complete bipartite subgraphs and closed patterns

A Correspondence Between Maximal Complete Bipartite SubgraphsAnd Closed PatternsJinyan Li,Haiquan Li,Donny Soh,Limsoon WongInstitute for Infocomm Research21Heng Mui Keng Terrace,Singapore119613Email:jinyan,haiquan,studonny,limsoon@.sgAbstractFor an undirected graph without self-loop,we prove:(i)that the number of closed patterns in the adjacency matrix of is even;(ii)that the number of the closed patterns is precisely double the number of maximal complete bipartite subgraphs of;(iii)that for every maximal complete bipartite subgraph,there always exists a unique and distinct pair of closed patterns that matches the two vertex sets of the subgraph.Therefore,we can efficiently enumerate all maximal complete bipartite subgraphs by using algorithms for mining closed patterns which have been extensively studied in the data miningfield.1IntroductionInterest in graphs and their applications has grown exponentially in the past two decades(Gross&Yellen,2004;Makino &Uno,2004),largely due to the usefulness of graphs as models in many areas such as mathematical research,electrical engineering,computer programming,business administration,sociology,economics,marketing,biology,and networking and communications.In particular,many problems can be modelled with maximal complete bipartite subgraphs(see the definition below)formed by grouping two non-overlapping subsets of vertices of a certain graph that show a kind of full connectivity between them.We consider two examples.Suppose there are customers in a mobile communication network.Some people have a wide range of contact,while others have few.Which groups of customers(with a maximal number)have a full interaction with another group of customers,a problem similar to one(Murata,2004)studied in web mining?This situation can be modelled by a graph where a mobile phone customer is a node and a communication is an edge.Thus,a maximal bipartite subgraph of this graph corresponds to two groups of customers between whom there exist a full communication.Our second example is about proteins’interaction in a cell.There are usually thousands of proteins in a cell that interact with one another.This situation again can be modelled by a graph,where a protein is a node and a pair of interacting proteins forms an edge.Then, listing all maximal complete bipartite subgraphs from this graph can answer questions such as which two protein groups have a full interaction,which is a problem studied in biology(Reiss&Schwikowski,2004;Tong et al.,2002).Listing all maximal complete bipartite subgraphs is studied theoretically in(Eppstein,1994).The result is that all maximal complete bipartite subgraphs of a graph can be enumerated in time,where is the arboricity of the graph and is the number of vertices of the graph.Even though the algorithm has a linear complexity,it is not practical for large graphs due to the large constant overhead(can easily be around10-20in practice)(Zaki&Ogihara,1998).In this paper, we study this problem from data mining perspective:We use a heuristics data mining algorithm to efficiently enumerate all maximal complete bipartite subgraphs from a large graph.A main concept of the data mining algorithm is called closed patterns.There are many recent algorithms and implementations devoted to the mining of closed patterns from the so-called1transactional databases(Bastide et al.,2000;Goethals&Zaki,2003;Grahne&Zhu,2003;Uno et al.,2004;Pan et al., 2003;Pasquier et al.,1999;Pei et al.,2000;Wang et al.,2003;Zaki&Hsiao,2002).The data structures are efficient and the mining speed is tremendously fast.Our main contribution here is the observation that the mining of closed patterns from the adjacency matrix of a graph,termed a special transactional database,is equivalent to the problem of enumerating all maximal complete bipartite subgraphs of this graph.The rest of this short paper is organized as follows:Sections2and3provide basic definitions and propositions on graphs and closed patterns.In Section4we prove that there is a one-to-one correspondence between closed pattern pairs and maximal complete bipartite subgraphs for any simple graph.In Section5,we present our experimental results on a proteins’interaction graph.Section6discusses some other related work and then concludes this paper.2Maximal Complete Bipartite SubgraphsA graph is comprised of a set of vertices and a set of edges.We often omit the superscripts in,and other places when the context is clear.Throughout this paper,we assume is an undirected graph without any self-loops.In other words,we assume that(i)there is no edge and(ii)for every, can be replaced by—that is,is an unordered pair.A graph is a subgraph of a graph if and.A graph is bipartite if can be partitioned into two non-empty and non-intersecting subsets and such that.This bipartite graph is usually denoted by.Note that there is no edge in that joins two vertices within or.is complete bipartite if .Two vertices of a graph are said to be adjacent if—that is,there is an edge in that connects them. The neighborhood of a vertex of a graph is the set of all vertices in that are adjacent to—that is, or.The neighborhood for a non-empty subset of vertices of a graph is the set of common neighborhood of the vertices in—that is,.Note that for any subset of vertices of a graph such that and are both non-empty,it is the case thatis a complete bipartite subgraph of.Note also it is possible for a vertex of to be adjacent to every vertex of.In this case,the subset can be expanded by adding the vertex,while maintaining the same neighborhood.Where to stop the expansion?We use the following definition of maximal complete bipartite subgraphs.Definition2.1A graph is a maximal complete bipartite subgraph of if is a complete bipartite subgraph of such that and.Not all maximal complete bipartite subgraphs are equally interesting.Recall our earlier motivating example involving the customers in a mobile communication network.We would probably not be very interested in two groups of customers between whom there exist a full communication,if the groups both comprise a single person.In contrast,we would probably be considerably more interested if one of the group is large,or both of the groups are large.Hence,we can introduce the notion of density on maximal complete bipartite subgraphs.Definition2.2A maximal complete bipartite subgraph of a graph is said to be-dense ifor is at least,and the other is at least.A complete bipartite subgraph of such that and is maximal in the sense that there is no other complete bipartite subgraph of with and such thatand.To appreciate this notion of maximality,we prove the proposition below.2Proposition2.3Let and be two maximal complete bipartite subgraphs of such that and.Then.Proof:Suppose and are two maximal complete bipartite subgraphs of such that and.Since and,we have ing the definition of maximal complete bipartite subgraphs,we derive and.Then.Thus as desired.3Closed Patterns of an Adjacency MatrixThe adjacency matrix of a graph is important in this study.Let be a graph with.The adjacency matrix of is the matrix defined byifotherwiseRecall that our graphs do not have self-loop and are undirected.Thus is a symmetric matrix and every entry on the main diagonal is0.Also,.The adjacency matrix of a graph can be interpreted into a transactional database(),which is a concept used very often in the data mining community.To define a,wefirst define a transaction.Let be a set of items.Then a transaction is defined as a subset of.For example,assume to be all items in a supermarket,a transaction by a customer is the items that the customer bought.A is a non-empty set of transactions.Each transaction in a is assigned a unique identity .A pattern is defined as a non-empty set1of items of.A pattern may be or may not be contained in a transaction. Given a and a pattern,the number of transactions in containing is called the support of,denoted. In this paper,unless mentioned otherwise,we consider only patterns that appear in a given transactional database.In fact,for data mining,we are often interested only in patterns that appear sufficiently frequent.That is,we consider only patterns satisfying,for a threshold.Unless mentioned otherwise,we set in this paper. Let be a graph with.If each vertex in is defined as an item,then the neighborhood of is a transaction.Thus,is a.Such a special is denoted by.The identity of a transaction in is defined as the vertex itself—that is,.Note that has the same number of items and transactions.Note also that since we assume to be an undirected graph without self-loop.can be represented as a binary square matrix.This binary matrix is defined byifotherwiseSince iff,it can be seen that.So,“a pattern of”is equivalent to“a pattern of the adjacency matrix of”.Closed patterns are a type of interesting patterns in a.In the last few years,the problem of efficiently mining closed patterns from a large has attracted a lot of researchers in the data mining community(Bastide et al.,2000;Goethals &Zaki,2003;Grahne&Zhu,2003;Uno et al.,2004;Pan et al.,2003;Pasquier et al.,1999;Pei et al.,2000;Wang et al., 2003;Zaki&Hsiao,2002).Let be a set of items,and be a transactional database defined on.For a pattern,let —that is,are all transactions in containing the pattern.For a set of transactions ,let—that is,the set of items which are shared by all transactions ing thesetwo functions,we can define the notion of closed patterns.For a pattern,is called the closure of .A pattern is said to be closed with respect to a transactional database iff.We define the occurrence set of a pattern in as. It is straightforward to see that iff.There is a tight connection between the notions of neighbourhood in a graph and occurrence in the corresponding transactional database.Proposition3.1Given a graph and a(non-empty)pattern that occurs at least times in.Then .Note that we do not require to occur at least times in.Proof:If,then is adjacent to every vertex in.Therefore,for each.That is,If,then is adjacent to every vertex in.So,.Therefore,is a transaction of containing. So,.There is also a nice connection between the notions of neighborhood in a graph and that of closure of patterns in the corresponding transactional database.Proposition3.2Given a graph and a(non-empty)pattern that occurs at least times in.Then .Thus is a closure operation on patterns that occur at least times in.Proof:By construction,.We discuss in the next section deeper relationships between the closed patterns of and the maximal complete bipartite subgraphs of.4ResultsThe occurrence set of a closed pattern in plays a key role in the maximal complete bipartite subgraphs of.We introduce below some of its key properties.Proposition4.1Let be a graph.Let and be closed patterns that appear at least times in.Theniff.Proof:The left-to-right direction is trivial.To prove the right-to-left direction,let us suppose that.It is straightforward to see that iff.Then we get from.Since and are closed patterns of,it follows that,andfinishes the proposition.Proposition4.2Let be a graph and a closed pattern that occurs at least times in.Then and its occurrence set has empty intersection.That is,.Proof:Let.Then is adjacent to every vertex in.Since we assume is a graph without self-loop,. Therefore,.In fact this proposition holds for any pattern,not necessarily a closed pattern.4Lemma4.3Let be a graph.Let be a closed pattern that occurs at least times in.Then.Proof:As is a closed pattern,by definition,then are all and only items contained in every transaction of that contains.This is equivalent to that are all and only vertices of that are adjacent to every vertex in.This implies that are all and only transactions that contain.In other words, .Proposition4.4Let be a graph and a closed pattern that occurs at least times in.Then is a closed pattern of.Proof:By Lemma4.3,.So.Thus is a closed pattern.The three propositions above give rise to a couple of interesting corollaries below.Corollary4.5Let be a graph.Then the number of closed patterns that appear at least once in is even. Proof:Suppose there are closed patterns that appear at least once in,denoted as,,...,.As per Proposi-tion4.4,,,...,are all closed patterns of.As per Proposition4.1,is different from iff is different from.So every closed pattern can be paired with a distinct closed pattern by in a bijective manner.Furthermore,as per Proposition4.2,no closed pattern is paired with itself.This is possible only when the number is even.Corollary4.6Let be a graph.Then the number of closed patterns,such that both and appear at least times in,is even.Proof:As seen from the proof of Corollary4.5,every closed pattern of can be paired with,and the entire set of closed patterns can be partitioned into such pairs.So a pair of closed patterns and either satisfy or do not satisfy the condition that both and appear at least times in.Therefore,the number of closed patterns,such that both and appear at least times in,is even.Note that this corollary does not imply the number of closed patterns that appear at least times in is even.A counter example is given below.Example4.7Consider a given by the following matrix:0100110110010We list its closed patterns,their support,and their counterpart patterns below:support of close pattern2115Suppose we take.Then there are only3closed patterns—an odd number—that occur at least times,viz.,,and.Finally,we demonstrate our main result on the relationship with closed patterns and maximal complete bipartite subgraphs. In particular,we discover that every pair of a closed pattern and its occurrence set yields a distinct maximal complete bipartite subgraph of.Theorem4.8Let be an undirected graph without self-loop.Let be a closed pattern of.Then the graphis a maximal complete bipartite subgraph of.Proof:By assumption,is non-empty and has a non-zero support in.Therefore,is non-empty.By Proposition4.2,.Furthermore,,is adjacent to every vertex of.So, ,and every edge of connects a vertex of and a vertex of.Thus,is a complete bipartite subgraph of. By Proposition3.1,we have.By Proposition3.2,.By Proposition3.1,we derive .So is maximal.Thisfinishes the theorem.Theorem4.9Let be an undirected graph without self-loop.Let graph be a maximal complete bipartite subgraph of.Then,and are both a closed pattern of,and. Proof:Since is a maximal complete bipartite subgraph of,then and.By Proposition3.2,.So,is a closed pattern.Similarly,we can get is a closed pattern.By Proposition3.1,and,as required.The above two theorems say that maximal complete bipartite subgraphs of are all in the form of,where and are both a closed pattern of.Also,for every closed pattern of,the graphis a maximal complete bipartite subgraph of.So,there is a one-to-one correspondence between maximal complete bipartite subgraphs and closed pattern pairs.We can also derive a corollary linking support threshold of to the density of maximal complete bipartite subgraphs of .Corollary4.10Let be an undirected graph without self-loop.Then is a-dense maximal complete bipartite subgraph of iff is a closed pattern such that occurs at least times in and occur at least times in.The corollary above has the following important implication.Theorem4.11Let be an undirected graph without self-loop.Then is a-dense maximal complete bipartite subgraph of iff is a closed pattern such that occurs at least times in and .Proof:Suppose is a-dense maximal complete bipartite subgraph of. By Theorem4.9,.By definition of,.Substitute this into Corollary4.10,we get is a-dense maximal complete bipartite subgraph of iff is a closed pattern such that occurs at least times in and as desired.Theorems4.8and4.9show that algorithms for mining closed patterns can be used to extract maximal complete bipartite subgraphs of undirected graphs without self-loop.Such data mining algorithms are usually significantly more efficient at higher support threshold.Thus Theorem4.11suggests an important optimization for mining-dense maximal complete bipartite subgraphs.To wit,assuming,it suffices to mine closed patterns at support threshold, and then get the answer byfiltering out those patterns of length less than.6Table1:Close patterns in a yeast protein interaction network.support threshold#of qualified close patterns1121314117895 2.73439592094781 1.765560038664290.937715800362230.3989406174260.17111291380.0785Experimental ResultsWe use an example to demonstrate the efficiency of listing all maximal complete bipartite subgraphs by using an algorithm for mining closed patterns.The graph is a protein interaction network with proteins as vertices and interactions as edges. As there are many physical protein interaction networks corresponding to different species,here we take the simplest and most comprehensive yeast physical and genetic interaction network(Breitkreutz et al.,2003)as an example.This graph consists of4904vertices and17440edges(after removing185self loops and1413redundant edges from the original 19038interactions).Therefore,the adjacency matrix is a transactional database with4904items and4904transactions.On average,the number of items in a transaction is3.56.That is,the average size of the neighborhood of a protein is3.56.We use FPclose*(Grahne&Zhu,2003),a state-of-the-art algorithm for mining closed pattern,for enumerating the maximal complete bipartite subgraphs.Our machine is a PC with a CPU clock rate3.2GHz and2GB of memory.The results are reported in Table1,where the second column shows the total number of frequent close patterns whose support level is at least the threshold number in the column one.The third column of this table shows the number of close patterns whose cardinality and support are both at least the support threshold;all such closed patterns are termed qualified closed patterns. Only these qualified closed patterns can be used to form maximal complete bipartite subgraphs such that both of and are at least a threshold.From the table,we can see:The number of all closed patterns(corresponding to those with the support threshold of1)is even.Moreover,the number of qualified close patterns with cardinality no less than any support level is also even,as expected from Corollary4.6.The algorithm is efficient—The algorithm takes less than4seconds to complete the program for all situations reported here.This indicates that enumerating all maximal complete bipartite subgraphs from a large graph can be efficiently solved by using algorithms for mining closed patterns.A so-called“many-few”property(Maslov&Sneppen,2002)of protein interactions is observed again in our exper-iment results.The“many-few”property says that:a protein that interacts with lots of other proteins tends not to interact with another protein that interacts with lots of other proteins(Maslov&Sneppen,2002).This is most clearly seen in Table1at the higher support thresholds.For example,at the support threshold11,there are12402protein groups that have full interactions with at least11proteins.But there are only two groups that each contain at least11 proteins and that have full mutual interaction.76Discussion and ConclusionThere are two recent research results related to our work.The problem of enumerating all maximal complete bipartite subgraphs(called maximal bipartite cliques there)from a bipartite graph has been investigated by(Makino&Uno,2004).The difference is that our work is to enumerate all the subgraphs from any graphs(without self loops and undirected),butMakino and Uno’s work is limited to enumerating from only bipartite graphs.So,our method is more general.Zaki(Zaki& Ogihara,1998)observed that a transactional database can be represented by a bipartite graph,and also a relation thatclosed patterns(wrongly stated as maximal patterns in(Zaki&Ogihara,1998))of one-to-one correspond to maximal complete bipartite subgraphs(called maximal bipartite clique there)of.However,our work is to convert a graph, including bipartite graphs,into a special transactional database,and then to discover all closed patterns forenumerating all maximal complete bipartite subgraphs of.Furthermore,the occurrence set of a closed pattern of Zaki’s work may not be a closed pattern,but that of ours is always a closed pattern.Finally,let’s summarize the results achieved in this paper.We have studied the problem of listing all maximal complete bipartite subgraphs from a graph.We proved that this problem is equivalent to the mining of all closed patterns from the adjacency matrix of this graph.Experimental results on a large protein interactions’data show that our method is efficient and the listing is fast.ReferencesBastide,Y.,Pasquier,N.,Taouil,R.,Stumme,G.,&Lakhal,L.(2000).Mining minimal non-redundant association rules using frequent closed putational Logic,972–986.Breitkreutz,B.J.,Stark,C.,&Tyers,M.(2003).The grid:The general repository for interaction datasets.Genome Biology, 4,R23.Eppstein,D.(1994).Arboricity and bipartite subgraph listing rmation Processing Letters,51,207–211. Goethals,B.,&Zaki,M.J.(2003).Fimi’03:Workshop on frequent itemset mining implementations.Third IEEE Interna-tional Conference on Data Mining Workshop on Frequent Itemset Mining Implementations(pp.1–13).Grahne,G.,&Zhu,J.(2003).Efficiently using prefix-trees in mining frequent itemsets.Proceedings of FIMI’03:Workshop on Frequent Itemset Mining Implementations.Gross,J.L.,&Yellen,J.(2004).Handbook of graph theory.CRC Press.Makino,K.,&Uno,T.(2004).New algorithms for enumerating all maximal cliques.Proceedings of the9th Scandinavian Workshop on Algorithm Theory(SWAT2004)(pp.260–272).Springer-Verlag.Maslov,S.,&Sneppen,K.(2002).Specificity and stability in topology of protein networks.Science,296,910–913. Murata,T.(2004).Discovery of user communities from web audience measurement data.Proceedings of The2004 IEEE/WIC/ACM International Conference on Web Intelligence(WI2004)(pp.673–676).Pan,F.,Cong,G.,Tung,A.K.H.,Yang,J.,&Zaki,M.J.(2003).CARPENTER:Finding closed patterns in long biological datasets.Proceedings of the Ninth ACM SIGKDD International Conference on Knowledge Discovery and Data Mining (pp.637–642).Pasquier,N.,Bastide,Y.,Taouil,R.,&Lakhal,L.(1999).Discovering frequent closed itemsets for association rules. Proceedings of the7th International Conference on Database Theory(ICDT)(pp.398–416).Pei,J.,Han,J.,&Mao,R.(2000).CLOSET:An efficient algorithm for mining frequent closed itemsets.Proceedings of the2000ACM SIGMOD Workshop on Research Issues in Data Mining and Knowledge Discovery(pp.21–30).8Reiss,D.J.,&Schwikowski,B.(2004).Predicting protein-peptide interactions via a network-based motif sampler.Bioin-formatics(ISMB2004Proceedings),20(suppl.),i274–i282.Tong,A.H.,Drees,B.,Nardelli,G.,Bader,G.D.,Brannetti,B.,Castagnoli,L.,Evangelista,M.,Ferracuti,S.,Nelson, B.,Paoluzi,S.,Quondam,M.,Zucconi,A.,Hogue,C.W.,Fields,S.,Boone,C.,&Cesareni,G.(2002).A combined experimental and computational strategy to define protein interaction networks for peptide recognition modules.Science, 295,321–324.Uno,T.,Kiyomi,M.,&Arimura,H.(2004).Lcm ver.2:Efficient mining algorithms for frequent/closed/maximal itemsets. IEEE ICDM’04Workshop FIMI’04(International Conference on Data Mining,Frequent Itemset Mining Implementa-tions).Wang,J.,Han,J.,&Pei,J.(2003).CLOSET+:Searching for the best strategies for mining frequent closed itemsets. Proceedings of the Ninth ACM SIGKDD International Conference on Knowledge Discovery and Data Mining(KDD’03), Washington,DC,USA(pp.236–245).Zaki,M.J.,&Hsiao,C.-J.(2002).CHARM:An efficient algorithm for closed itemset mining.Proceedings of the Second SIAM International Conference on Data Mining.Zaki,M.J.,&Ogihara,M.(1998).Theoretical foundations of association rules.Proc.3rd SIGMOD Workshop on Research Issues in Data Mining and Knowledge Discovery.9。
xp序列号——精选推荐
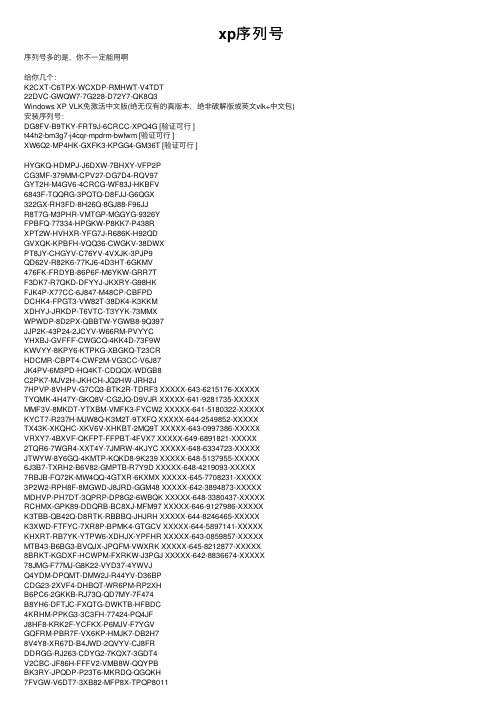
xp序列号序列号多的是,你不⼀定能⽤啊给你⼏个:K2CXT-C6TPX-WCXDP-RMHWT-V4TDT22DVC-GWQW7-7G228-D72Y7-QK8Q3Windows XP VLK免激活中⽂版(绝⽆仅有的真版本,绝⾮破解版或英⽂vlk+中⽂包) 安装序列号:DG8FV-B9TKY-FRT9J-6CRCC-XPQ4G [验证可⾏ ]t44h2-bm3g7-j4cqr-mpdrm-bwfwm [验证可⾏ ]XW6Q2-MP4HK-GXFK3-KPGG4-GM36T [验证可⾏ ]HYGKQ-HDMPJ-J6DXW-7BHXY-VFP2PCG3MF-379MM-CPV27-DG7D4-RQV97GYT2H-M4GV6-4CRCG-WF83J-HKBFV6843F-TQQRG-3PQTQ-D8FJJ-G6QGX322GX-RH3FD-8H26Q-8GJ88-F96JJR8T7G-M3PHR-VMTGP-MGGYG-9326YFPBFQ-77334-HPGKW-P8KK7-P438RXPT2W-HVHXR-YFG7J-R686K-H92QDGVXQK-KPBFH-VQQ36-CWGKV-38DWXPT8JY-CHGYV-C76YV-4VXJK-3PJP9QD62V-R82K6-77KJ6-4D3HT-6GKMV476FK-FRDYB-86P6F-M6YKW-GRR7TF3DK7-R7QKD-DFYYJ-JKXRY-G98HKFJK4P-X77CC-6J847-M48CP-CBFPDDCHK4-FPGT3-VW82T-38DK4-K3KKMXDHYJ-JRKDP-T6VTC-T3YYK-73MMXWPWDP-8D2PX-QBBTW-YGWB8-9Q397JJP2K-43P24-2JCYV-W66RM-PVYYCYHXBJ-GVFFF-CWGCQ-4KK4D-73F9WKWVYY-8KPY6-KTPKG-XBGKQ-T23CRHDCMR-CBPT4-CWF2M-VG3CC-V6J87JK4PV-6M3PD-HQ4KT-CDQQX-WDGB8C2PK7-MJV2H-JKHCH-JQ2HW-JRH2J7HPVP-8VHPV-G7CQ3-BTK2R-TDRF3 XXXXX-643-6215176-XXXXX TYQMK-4H47Y-GKQ8V-CG2JQ-D9VJR XXXXX-641-9281735-XXXXXMMF3V-8MKDT-YTXBM-VMFK3-FYCW2 XXXXX-641-5180322-XXXXXKYCT7-R237H-MJW8Q-K3M2T-9TXFQ XXXXX-644-2549852-XXXXXTX43K-XKQHC-XKV6V-XHKBT-2MQ9T XXXXX-643-0997386-XXXXXVRXY7-4BXVF-QKFPT-FFPBT-4FVX7 XXXXX-649-6891821-XXXXX2TQR6-7WGR4-XXT4Y-7JMRW-4KJYC XXXXX-648-6334723-XXXXX JTWYW-8Y6GQ-4KMTP-KQKD8-9K239 XXXXX-648-5137955-XXXXX6J3B7-TXRH2-B6V82-GMPTB-R7Y9D XXXXX-648-4219093-XXXXX7RBJB-FQ72K-MW4QQ-4GTXR-6KXMX XXXXX-645-7708231-XXXXX3P2W2-RPH8F-8MGWD-J8JRD-GGM48 XXXXX-642-3894873-XXXXX MDHVP-PH7DT-3QPRP-DP8G2-6WBQK XXXXX-648-3380437-XXXXX RCHMX-GPK89-DDQRB-BC8XJ-MFM97 XXXXX-646-9127986-XXXXXK3TBB-QB42Q-D8RTK-RBBBQ-JHJRH XXXXX-644-8246465-XXXXXK3XWD-FTFYC-7XR8P-BPMK4-GTGCV XXXXX-644-5897141-XXXXX KHXRT-RB7YK-YTPW6-XDHJX-YPFHR XXXXX-643-0859857-XXXXXMTB43-B6BG3-BVQJX-JPQFM-VWXRK XXXXX-645-8212877-XXXXX8BRKT-KGDXF-HCWPM-FXRKW-J3PGJ XXXXX-642-8836674-XXXXX78JMG-F77MJ-G8K22-VYD37-4YWVJQ4YDM-DPQMT-DMW2J-R44YV-D36BPCDG23-2XVF4-DHBQT-WR6PM-RP2XHB6PC6-2GKKB-RJ73Q-QD7MY-7F474B8YH6-DFTJC-FXQTG-DWKTB-HFBDC4KRHM-PPKG3-3C3FH-77424-PQ4JFJ8HF8-KRK2F-YCFKX-P6MJV-F7YGVGQFRM-PBR7F-VX6KP-HMJK7-DB2H78V4Y8-XR67D-B4JWD-2QVYV-CJ8FRDDRGG-RJ263-CDYG2-7KQX7-3GDT4V2CBC-JF86H-FFFV2-VMB8W-QQYPBBK3RY-JPQDP-P23T6-MKRDQ-QGQKH7FVGW-V6DT7-3XB82-MFP8X-TPQP8011WRGHR-CH8DG-2WDM2-DXXDW-RDVFY010 B8CHG-TT3T2-BXVRJ-GGBV4-RWQHH011V422J-M3W2R-HXD32-FFMTJ-WXK96015T2KKK-J6B64-PFVTP-X2DR3-HWX2J014 VTGYH-4B6HW-QFX72-GX6DB-CRK69010 HDHXD-HGHF8-FWYYQ-HV768-66PV2004M6J7C-84GM8-T242T-K3VWV-G36F7013 BBXJT-YK7P4-KH73D-XCRHB-XDPTH011 QPRGK-GWX2B-VHV6P-GDWF6-YGTGM010 KJR82-RQJK3-Q82JM-4R3C7-YHQK401133RDD-Q8WPH-CG8TF-8WX4V-JWCM4011 38VQV-D23BB-T42Q8-H4JGC-X8M66013GG2VV-4CBR8-8Y42V-4DKHP-BGJ4T007G7PHM-TFM4Y-CWM7P-RG4PQ-QJQ7Y013 8DY3Q-WJYT2-22QXJ-4MXXH-CYQC8015 JMJ62-PQ6BB-JHKJK-VWRQ6-KXHP60057YQBF-RPB7C-TR7JR-HB7MC-7QM9V007 XYYXQ-M8QFP-D7Q8T-HD2WC-2MX49013K3PXY-2RWBJ-GQWK8-764F4-JJTMQ012 BX6HT-MDJKW-H2J4X-BX67W-TVVFG 005 CCC64-69Q48-Y3KWW-8V9GV-TVKRM 005 MMX38-HJ2PJ-MMCDB-TBRWH-DGPJD008 FXVKV-BM7JQ-K6KCP-RDJCH-MD8XR001 TRCDH-VQMQV-XC64G-RCDX8-BJ6P4008 TWJXX-3CXFD-DYFFW-CB6CJ-FTJ3G01548VMC-D47YK-TXJQF-MMDKY-W34R8019 MKW7J-6FFYX-GHBPG-PYT2V-MC6KR003 VR2DK-BYD3F-3YJ4T-28JF2-RHDQR0058MQ33-W4TMG-QJGJT-342YD-G29CK013 YJBXQ-KBHKR-76DRM-VQK87-WCKMT003 VMGWJ-Q73GV-QFC7H-DPQHF-427QD0124X7BQ-MG2TM-Q4YYF-6DCCJ-86MWW017 T4HMT-VXFPB-4WGVC-GXFY3-W4F6M018 R86XR-TF7C7-DW3P6-BPFWM-B8FTJ0034MQY7-GD6JG-VWRYF-RDGGQ-X3KTW017 QRJ3Q-J7R23-47XHY-C7TMY-MFGMW003 JRY8X-8JMKF-XHYP6-VGH67-BDR4B005 JRY8X-8JMKF-XHYP6-VGH67-BDR4B005FB3KD-WHK73-GPJ4X-2R3R4-7RGT4020 BMQT7-7JV4W-3QHYY-KM83W-YBYX7018 MYYV6-WJRGX-7MRR2-PQQ7F-6FWCC006 36B4B-7T68F-Q2V2J-WD4WR-X3FPT009 GJVFB-GMJHV-VDWGX-XKPKW-XD293017 WFHYK-F7TG4-3B8RF-MR4J3-QG6P60186WF8K-KHFGM-MXMWD-RX2MY-Y2KMD011 3Q674-JPYY6-FHB7Y-WWC3Q-63BY4013 MFDJ7-2RW34-D4VJK-CV8RD-4PDC2015 XBD4K-3RWQK-QBYK6-KHXXR-QKYT2016 GVQC6-M47K4-FGPP2-PHMHC-PD3Y4013 YRB7C-Y283Y-JWFMH-BH6HX-VGKRJ006 KYTRG-7RVFF-7KGMY-7B7V3-V38X6014 KJM3R-72HRB-3PDFQ-G7QY4-XQVJX019 BRPFX-G4KMG-F278B-QPFGX-DYP4C015 CWKVH-WQ38Y-HTYQR-PJKW7-JDGT3009 MKYPK-CR6PM-RGRWR-X2GMY-JQYF8011 QDKWP-XC8HY-67BCP-JTJHM-JVPP7009C8QCQ-H8M7Y-FYC2B-4CBDF-8D3K3013 FC8VQ-KXXHG-FBG6R-R628H-C6TQP003 WYJYB-QJTC6-XMWWW-3YJK7-CX93Y016 FJD2V-BQHKH-FPVT4-BYV3F-WR66K005 VQ6VM-Q8M6X-FT8P7-7QQKG-T222G013 XP4P4-WK3MT-K36KR-W4RY3-YPMCD010 JC2BY-TWX7R-2TRVK-WJCVV-HP94J014P8HY8-82HTT-RH6GK-JVCQD-7722G013 DWFRT-3WWPX-7BF4W-WBJ2W-93QK9008 KPHJV-FFPT6-3WCGF-4HBKQ-BC9RK0122JHRT-8PTQ6-YG3TH-MJ3RP-XR4YF005 GWYY7-YYQ7J-HD6XB-MK4TX-39K4M010DHMPC-G8WR4-GPMQQ-PK474-GWXH3012 3XP3F-4JGHD-6WTBR-GM6RC-QVXH9012 PRHDX-KRYRJ-TB76X-J7D4C-RKHJY007 JWQ6K-PBRFB-XYXYM-34JQQ-CJJY7016 VMHWD-4PMHQ-HVFC2-88M3J-KDVH6008 VXXT3-TRW8C-T7HFD-264B7-4YJW60042PBMV-BV8CH-XHHG3-3H37P-2QVGG013 4DPYJ-XJY7B-CPYMK-8BJJR-RHB67017 QFQVR-WMKHV-RQ3WV-FF268-DV89D005 4XR87-KGBW4-TWYPG-MXX3B-FVH9Q006 JDX7K-DD3PQ-GDVR3-CV7J8-QWGX4007 MMBYK-HVXKF-42DHY-6DKM6-VQ9F2004 MJV26-DJHXC-DBDX2-J3682-62VGK004 VVRH8-6HVMV-RFPCY-H6WBH-6CH22016 QGVHJ-DGV4V-RMT48-BXDP2-DMT43003 随便⽤。
Windows序列通过正版验证
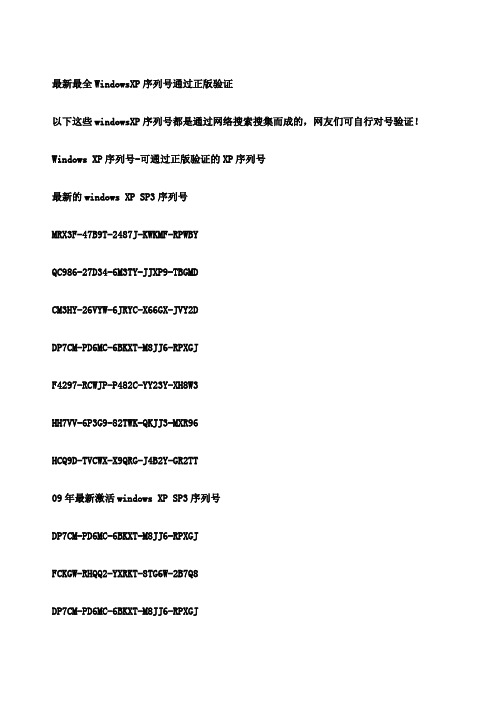
最新最全WindowsXP序列号通过正版验证以下这些windowsXP序列号都是通过网络搜索搜集而成的,网友们可自行对号验证!Windows XP序列号-可通过正版验证的XP序列号最新的windows XP SP3序列号MRX3F-47B9T-2487J-KWKMF-RPWBYQC986-27D34-6M3TY-JJXP9-TBGMDCM3HY-26VYW-6JRYC-X66GX-JVY2DDP7CM-PD6MC-6BKXT-M8JJ6-RPXGJF4297-RCWJP-P482C-YY23Y-XH8W3HH7VV-6P3G9-82TWK-QKJJ3-MXR96HCQ9D-TVCWX-X9QRG-J4B2Y-GR2TT09年最新激活windows XP SP3序列号DP7CM-PD6MC-6BKXT-M8JJ6-RPXGJFCKGW-RHQQ2-YXRKT-8TG6W-2B7Q8DP7CM-PD6MC-6BKXT-M8JJ6-RPXGJMRX3F-47B9T-2487J-KWKMF-RPWBYWindows XP序列号,分专业版和家庭版,请选择适合您的版本。
家庭版:3FKBQ-32TH7-D3TJB-YBWTQ-D26VQKXDVQ-26WRM-PDGP2-CH7VJ-BFKGHWP6T6-D7F2H-7T8WY-8D37V-BPCH34K63F-F74YG-XCTQR-3QFT6-788JQYYF2G-2BGGM-8M4HV-C4VKM-278QG492BW-YGCJX-M7Y48-FCJGG-CWTCKQ83JG-YRR24-VVDJG-JMDJD-TDP92CGT8C-VPMGV-22FTB-4662X-PKRW7R38RJ-TV3M3-KWPKW-3T2V4-M74XF82YWC-44DWH-VP8B4-M8Q8G-7XKVGGHHTT-GJ476-BF48P-GF4X3-Y4RJBDYJC7-8T366-QFKVH-7YYWJ-72GV9GJWW3-XGJQW-X4FM6-XX8F4-M223F CCMHK-VT7HK-VJR4K-7RJ7T-HPK4Q XGX4M-K7MC2-VGJH7-XMX27-7HBPM FTKHV-8PXKK-HXQM6-2JJYM-RG282 63VXM-JFP8B-MTPVW-734PV-GJ8HD 4YX4G-62MTT-6RTQX-PV2VB-7MBFF JMC7C-BTWJR-WHQF2-32V8K-TGGGY W7QRW-FWD36-XFD84-TDQXV-PY3DV TQWCP-RBXGT-C8CRW-P2JCM-QVTVF 3MJJ7-7BQXX-KRX47-6CJKY-W97FX 3MDP8-KCP2H-RRQGW-PGMDH-RQPVG QDFJC-JKR8B-D6XF2-7X763-Y2YTB 2QFJY-MGCKJ-G3CDD-VY2T2-8TC4J GW7QD-77WJ2-FXM26-WT6JP-F6RGB JG3WK-GCJCR-KHDYD-GJHBK-FVP4DR42R8-6KXWF-2WMVC-J82GR-WRP2C D3MFD-PHCFQ-HJ68R-6F3JB-G3BPY 2R3TQ-JCQFP-4BJ6M-Y6HC8-TYHJT W3XCV-TYTMB-B2TB7-R7WCQ-J7G6P 2FB6V-TCM4H-7K8HR-7XC2W-K28P6 QDBHH-T6RMK-YPB8Q-MB3R8-BM9CR 4CHYB-FRHR6-J8QFQ-B6HVF-VGJPF GDQPQ-FWJMD-MCRYR-74DGR-MJYVJ 4RFKJ-GGJVV-Y3TXH-XXB6R-JYGGD 7TJPG-4TRPT-C8PRJ-H338J-GV876 43BFW-KFJHY-QPMD4-738QV-8833G 2YH22-WPYXK-R8M32-42J4X-6RJPD YBPRD-Q76CB-FV8PK-XV8GB-FGQFG KRGKM-2QTBV-GKQC6-762J3-Q3GTP 2PCBJ-JKPPG-4FG8Q-7HPJH-87X8RMX2WW-4MHYT-Y47QG-4XRB8-WRKFJ J7F7Y-8CPRT-8Q4KH-4KWV8-YWBFX 8F3XP-MKXY3-2R6TR-BKPYW-WJCK8 PTMHT-JB72F-2WKW4-WRGY6-K84KV B6FJQ-8GFCV-YMFQ8-3BPX4-VDM6X VMG8Q-FF4BK-8CQD3-MDK88-YK9R9 V28QQ-Q862G-QVK6W-KJHYT-769M3 M4QWF-VYPPQ-M4DKK-WX2K7-2V29Q 8QXTR-RY36X-2C3T4-CT2QJ-YT9B7 JRWBM-FXQP7-83WF7-7RRDX-DD82Y X8TVX-4J2MR-X3TK4-7FRWX-T88TR VXFKK-FYTP6-8VKV7-P74PJ-6TF9H 8W8GB-FGB4Y-KFWCG-JFWDK-WMRTP FDWTM-34B7D-7X4H4-VCRW4-F8J8H Q8JJY-V34V6-23HBK-JPG2C-KPDPPMWF2H-JVJF2-PRMFY-3V44D-PR37T WW7B6-QPRQJ-F37PX-8GCVX-TVBCM YWDK6-PGWBD-CR62C-76FBB-MGXMG WQMD2-RJK68-26648-GFMGR-929V2 P6GKF-GPP2F-6TCGD-XCP4J-PYX2K 8WFC3-M23WH-MHJTH-DQCX7-MPWBQ DFHQG-H3Y3W-Y3PM6-GXCD4-RB9H2 MVP7X-2MXWF-CWBMF-KV866-3KJ8D FYFF3-XRKKC-2PYGD-H2TR7-DMF7F 27WC6-6XKFG-6K3DD-D3F23-8HHFQ TQ4W3-VTW4X-X4XTF-W4TXG-HVKYR 2VTVG-7MYXM-RPXD7-BP8DH-CF87J BBK3F-BYD63-HXWTP-CFW3Q-J8G9M MHW86-DRMYB-BCYKP-YB4QP-PK8T7 VWPRH-PBJKY-TWQTY-GKQCC-RD3QRH68PX-YVX8Q-XXJJ8-RPPX3-FJFW9 3PJ2X-8W2YY-YQFKC-JCTY3-3D7Y2 GBDQR-C47MH-VW8GH-8W3FX-QY3RV 6F67C-WKF86-CPXV2-YBRBD-JVTMX 8G6RP-GXPMW-BTHTJ-4MV3G-V297K 4QQTF-8RX4D-88VHR-V3WH8-43TRP C7JDR-8F6FB-2YQC7-46VBH-GPBK8 F3PXR-QYKTW-PT6RV-F2DWR-QCVF9 42BK8-28H73-HHYFY-YBYPM-R4MY9 7BCCB-R4KW6-PCDK6-QTX8R-X2F76 MW8V8-83TR2-R3YGT-2TTTJ-PJDTX 2BR4M-P4JBV-2MQBG-BW72M-GKFK4 GKXHH-7PW74-8JVTX-6YFV3-M4328 3VRV4-M8P3H-HTKJ8-WPCQP-4JFFP CHGBG-7HRR2-BDYJD-YRQPF-46V2KX7PKB-82CW6-6BCDF-786DB-X97P4 6GXYV-MDJJG-GX8RM-MGVK8-PK294 H3PPY-YVDJM-PTYKH-CRWJV-77FCP CQPWP-HXQHD-MHPG4-C74FX-TDCM2 RDPMY-Q8VYP-D4TKT-BJQTM-DTHTX 8C3V7-67RYX-PK2JP-TMVYC-JBD6C RXHQ2-6447R-RQCWC-3QGRM-KW7KC 438QK-XTRK4-2XJPC-MBB8T-QHF44 8RPX2-WMRFB-3H4TM-76Q2R-6DDRX 2CY7B-K3QT8-44V2X-37FWY-6MCQB MYDGX-CXTB4-XGJWX-BWPKV-R9YTF 84BXM-QYJPJ-JJHJT-J7CW8-BDGP8 BTW27-RKBRX-2VX6H-TT34J-XBPJM CMJ8D-F2RXW-XCQC7-HHHJ2-C9MXF 8CRV2-HB2F8-34TJC-GWGJ3-KFX6YQK2HX-XQTHB-RY82Q-DRCKP-WM2CR 24DFT-36QKX-3RJYB-QKR6V-3PW7H 6F67H-JCM78-MX3DP-T6WVQ-G8BJ3 DDQXH-Y7BHK-XJMY3-QHYKT-G33DC RPGTB-KQBRT-2GVVX-QJVFV-9W234 TDR4M-WDY3H-7YPDF-C4JJ3-BWQXF 8M2MQ-R3FGJ-T38HW-66RJ4-D9G6Q CKQ4C-8YHDG-MPW6Y-D3BRD-T8CBV KJ27M-HTVT6-JJWV2-PTGVJ-TRBV4 W77QW-QGX7H-4FMMW-TF6FB-WGG2B 7TFDP-GPCCD-R37TY-WRQVK-Y2PVG YKXDH-3VBMM-RYYTW-H2JP3-YQVJD 3TFWH-VP64T-J7XYG-HWRQ4-KKY99 BTR2C-XFTJK-46Y4C-6DKFH-7KTDQ 7DHFC-8W788-PJCWX-CJDY4-92RHY3GH6Y-JJQDX-MXC8J-8V3TG-FGYX6 48DF2-CPCMX-8W6J8-MWHDQ-4GPY8 VTXV3-74TJ7-3WDDV-V7832-PD4YP V8PTP-KG7DR-J8CQ3-XX7HM-J9QBM。
NDC3(N、GV)-40(11、11S) 50(11、11S) 65(11、11S) 80(11、

文件名称DocumentName使用说明书User Manual文件编号Document No.NDT2920281产品型号及名称ProductModel andNameNDC3(N、GV)-40(11、11S)/50(11、11S)/65(11、11S)/80(11、11S)/95(11、11S)交流接触器NDC3(N、GV)-40(11、11S)/50(11、11S)/65(11、11S)/80(11、11S)/95(11、11S)AC Contactor版 次Version1实施日期Implementation Date20190628编制/日期Preparedby/date刘晓鹏/20190628Liu Xiaopeng/20190628审核/日期Reviewedby/date王兴逢/20190628Wang Xingfeng/20190628批准/日期Approvedby/date雒国瑞/20190628Luo Guorui/20190628修订记录Revision History版次修订内容修订日期修订人员0 新增2019/5/19 刘晓鹏11.修改吸合功耗2.修改外形尺寸3.删除浪涌抑制模块内容2019/6/28 刘晓鹏1.适用范围与用途1. Application Scope and PurposeNDC3(N、GV)-40(11、11S)/50(11、11S)/65(11、11S)/8011(11、11S)/95(11、11S)交流接触器主要用于交流50/60Hz,额定绝缘电压为1000V,在AC-3使用类别下额定电压为415V时额定工作电流为40~95A的交流电路中,用于频繁启动和控制交流电动机;可逆接触器可以控制电动机的正反转和反接制动;并可以加装适当的过载继电器组成电磁起动器、以保护可能发生的过负荷电路。
The NDC3(N、GV)-40(11、11S)/50(11、11S)/65(11、11S)/8011(11、11S)/95(11、11S)AC contactors have the AC 50/60Hz and the rated insulation voltage of 1000V, and are mainly used for the AC circuit with the rated voltage of 415A and the rated working current of 40~95A as well as the AC-3 utilization category for frequently starting & controlling AC motors. Reversible contactors can control the forward and reverse braking of the motors; can be installed with the appropriate overload relays to form the magnetic starters, to protect the circuit in which overload may occur.2.应用范围2. Scope of Application(1)、适用环境(1)、Applicable environment标准使用环境温度:-25℃~+60℃;Standard operating ambient temperature: -25℃~+60℃;极限使用环境温度:-40℃~+70℃;Ultimate operating ambient temperature: -40℃~+70℃;存储温度:-60℃~+80℃;Storage temperature: -60℃~+80℃;正常使用海拔高度:≤3000米;Normal operating altitude: ≤3,000m;极限使用海拔高度:≤5000米;Ultimate operating altitude: ≤5,000m;环境湿度要求:Environmental humidity requirements:最高温度为+40℃时,空气的相对湿度不超过50%,在较低的温度下可以允许有较高的相对温度,对由于温度变化偶尔产生的凝露应采取措施。
单流阀
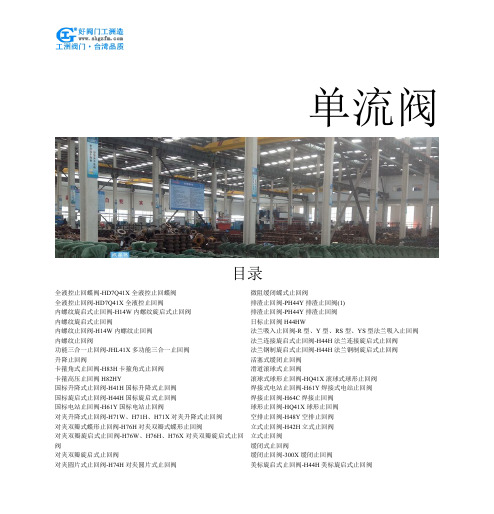
3
1
4
1
4
1
4
1
4
1
4
1
4
1
4
1
止回阀>>蝶式止回阀>>对夹式蝶型止回阀 产品详细信息
产品名称: 对夹式蝶型止回阀 产品型号: H71H 产品口径: DN15~DN300 产品压力: 1.0-25.0Mpa 产品材质: 铸钢、不锈钢、合金钢等
生产标准:国家标准 GB、机械标准 JB、化工标准 HG、美标 API、ANSI、德标 DIN、日本 JIS、JPI、 英标 BS 生产。阀体材质:铜、铸铁、铸钢、碳钢、 WCB、WC6、WC9、20#、25#、锻钢、A105、F11、 产品概括:F22、不锈钢、304、304L、316、316L、铬钼钢、 低温钢、钛合金钢等。工作压力 1.0Mpa-50.0Mpa。 工作温度:-196℃-650℃。连接方式:内螺纹、外螺 纹、法兰、焊接、对焊、承插焊、卡套、卡箍。驱 动方式:手动、气动、液动、电动。
二、执行标准 设计制造:JB/T8937 结构长度:JB/T8937 配管法兰:JB/T74 试验与检验:JB/T9092-1999
三、主要技术参数
试验压力
壳体试验 密封试验 阀体材料 工作温度 适用介质
1.6 2.4 1.8 WCB(C) ≤425 水,蒸气、油品
公称压力 PN(MPa) 2.5 3.8 2.8
标准规范:
设计制造标准:JB/T 8937 结构长度标准:GB/T 12221-2005 连接法兰标准:GB/T 9113 压力温度等级:GB/T 12224-2005 试验检验标准:GB/T 13927-2008
流体力学性能:
公称通径
DN
window server 2003 安装序列号02

win2003 Enterprise Server:QW32K-48T2T-3D2PJ-DXBWY-C6WRJ
win2003 Standard Server:M6RJ9-TBJH3-9DDXM-4VX9Q-K8M8M
PWIN98 OEM(第二版) S/N:BBH2G-D2VK9-QD4M9-F63XB-43C33
PWIN98 2A 2222版S/N:QY7TT-VJ7VG-7QPHY-QXHD3-B838Q
PWIN98 2A 2222版S/N:MB9HY-M4JGJ-B3RV2-FPH8D-FP8KY
PWIN98 2A 2222版S/N:WHWGP-XDR8Y-GR9X3-863RP-67J2T
Windows 98 Second Edition sn:QY7TT-VJ7VG-7QPHY-QXHD3-B838Q
windows2003序列号:cky24-q8qrh-x3kmr-c6bcy-t847y
win2003 Enterprise Server:QW32K-48T2T-3D2PJ-DXBWY-C6WRJ
win2003 Standard Server:M6RJ9-TBJH3-9DDXM-4VX9Q-K8M8M
win2003 Web Server:D42X8-7MWXD-M4B76-MKYP7-CW9FD
Windows2003服务器版的序列号:JB88F-WT2Q3-DPXTT-Y8GHG-7YYQY
PC-Cillin 2002 9.00 Build 1159序列号: PCTU-9991-9655-5350-4555
PC-Cillin 2002-Code:PCTU-9991-9655-5350-4555
- 1、下载文档前请自行甄别文档内容的完整性,平台不提供额外的编辑、内容补充、找答案等附加服务。
- 2、"仅部分预览"的文档,不可在线预览部分如存在完整性等问题,可反馈申请退款(可完整预览的文档不适用该条件!)。
- 3、如文档侵犯您的权益,请联系客服反馈,我们会尽快为您处理(人工客服工作时间:9:00-18:30)。
注意:
(1)植物体有六大器官是对绿色 开花植物而言;但海带,松、柏等 就没有。 (2)植物体的六大器官并不是都同 时存在。
花
茎
果实
叶
根
种子 油菜植株
小组讨论:
每一种器官的主要功能是什么?
根
输送水分和无机盐等物质
茎 学科网
叶
进行光合作用制造有机物
花 果实 种子
繁殖后代 吸收水分和无机盐
可以把植物的六大器官分成两类
6、下列各项中属于植物器官的是( C)
输导组织
包括在根、茎、叶处运输水 分和无机盐的导管和运输有 机物的筛管。
输 导 组 织 贯 穿 植 物 全 身
机械组织
在茎、叶柄、叶片、花柄、 果皮、种皮等处都有,其构 成细胞的细胞壁增厚,对植 物体有支撑和保护的作用。
分生组织
细胞小,细胞壁薄,细胞核大, 细胞质浓,具有很强的分裂能力, 能不断分裂产生新细胞,再由这 些细胞分化形成组织。主要分布 在茎尖、根尖。
导管运输水和无机盐,筛管运 输有机物。
支撑和保护作用。
茎尖、芽尖 分生组织
分裂产生新细胞。
保护组织
具有保护内部柔软部 分的功能,由根、茎、 叶表面的表皮细胞构 成。
营养组织
有储藏营养物质的功能,根、茎、 叶、花、果实、种子中都含有,细 胞壁薄,液泡大,有储藏营养物质 的功能。含有叶绿体的营养组织还 能进行光合作用。
花 生
殖
叶
种子
器 官
营 养茎
果实
器
官 根
学以致用,联系生活
说一说,我们吃的这些食物属于植物的什么器官。
[合作探究]
1、红薯、苹果、番茄、红豆、胡萝卜、 黄花菜 、马铃薯、白菜、花菜、菠菜、藕、绿豆将这些生
物材料进行分类,填写表格。
根 茎 叶 花 果实 种子
红薯、胡萝卜 马铃薯、藕 白菜、菠菜、 黄花菜、花菜 苹果、番茄 红豆、绿豆
1)、剥开桔子,由外而内进行观察果皮、 果肉、及黄白色的筋络,分别是什么组织?它 们分别有什么功能呢?
2)、撕开白菜,找出白菜中的各个组织并 了解其功能。
2、完成导学案“合作探究 3”
导学案“合作探究 3”
名称
桔皮 桔瓣 桔络
甘蔗皮
主要组织
保护组织
功能
保护内部幼嫩部分。
营养组织
储藏营养物质。
输导组织 机械组织
构点 层
机械 组织
次
无_系统_,由_器_官直接 由_系统_构成动物体。
构成植物体。
相 由受精卵 分裂、分化发育而来。由 细胞构
同 点
成
组织,由不同 组织构成
器官。
课堂检测
当你吃甘蔗时,首先你要把甘蔗茎 坚韧的皮剥去;咀嚼甘蔗茎时会有很多 的甜汁;那些咀嚼之后剩下的渣滓被吐 掉。试从组织构成器官的角度,说一说 甘蔗茎是由哪些组织构成的?
层皮属于( A )
A.保护组织 B.输导组织 C.营养组织 D.机械组织
4. 蓝莓果实因营养丰富,有“水果皇后的美称.从
生物体的结构层次分析,蓝莓的果实属于( C )
A.细胞 B.组织 C.器官 D.系统
5、下列哪项是营养组织的主要功能(C )
A.对细胞起支持作用 B.输导营养供给其他组织 C.贮藏营养物质供细胞利用 D.供给保护组织营养
植物体是由器官直接构成的,没有系统。
课堂延伸: 讨论交流:为什么植物体是一个统一的整体?
(1)从结构看,任何一个植物体都是由细胞构成的.细胞构成组织,组织构 成器官,器官进一步构成植物体.一个植物体内的各个细胞,不是孤立存在的, 而是与周围的细胞互相联系着.植物体内的输导组织,就像人体里的血管,它 们贯穿在植物体中,延伸到各个器官,起着运输水和无机盐,以及有机物的作 用,将植物体联系成为一个整体. (2)从功能看,植物体六种器官互相配合,共同完成生命活动.例如,根从土壤 中吸收水分和无机盐,通过茎中的输导组织运输到叶;叶利用根吸收的水分 和空气中的二氧化碳,通过光合作用制造有机物,通过茎中的输导组织运输到 茎和根,供其他部位的细胞利用.由此也可以看出,植物体的各个部分相互依 存,构成一个统一整体。
植物体的结构层次
霞浦一中 苏喜俊
组织 器官 系统 人体
受精卵
豌豆射手作为绿色开花植物, 它的结构层次又是怎样的呢?
第三节 植物体的结构层次
学习目标
• 能够说出绿色开花植物的六大器官; • 知道植物体的各种组织是由细胞分裂、分
化形成; • 认识植物的五大主要组织,知道植物的各
种组织均由分生组织分化形成; • 知道植物体的结构层次:细胞、组织、器
课堂小结
1、植物体的结构层次(从微观到宏观)
分生组织 细 组 保护组织 胞 织 营养组织
输导组织 机械组织
根
茎
器 官
叶
花
果实 种子
绿色开 花植物
2、动物体、植物体的结构层次比较:
(植物)
(动物)
_分_生组织
_ 上皮_组织
_保_护组织
_ 肌肉_组织
不
_营_养组织
_ 结缔_组织
结同
_输_导组织
_ 神经_组织
构成甘蔗茎的组织有保护组织、营养组织、输导 组织、机械组织等。
1、下列植物器官中,属于生殖器官的是( D)
A根 B.茎 C.叶 D.花
2、绿色开花植物体的构成是
(C )
A.细胞→器官→组织→植物体
B.组织→器官→细胞→植物体
C.细胞→组织→器官→植物体
D.器官→组织→细胞→植物体
3、用开水烫一下番茄,会在其表面撕下一层皮,这
根与茎如何区别?
植物体的器官又是由哪些结构 构成的?是否也像动物一样,由 各种不同的组织构成呢?
二、植物的几种主要组织
学生完成“合作探究2” 植物体内的主要组织有哪几种?
(参考课本64页)
植物体的主要组织
保护 组织
输导 组织
分生 组织
营养 组织
机械 组织
完成下面任务:
1、动手操作,解剖“桔子和白菜”:
桔子
桔皮——— 保护组织 桔瓣——— 营养组织 桔络——— 输导组织
白菜
上、下表皮— 保护组织
叶肉————— 营养组织 。
显
微 镜 下
桔皮 (保护组织)
观
察
各 组 织
桔络 (输导组织)
细
胞
特
桔瓣
点
(营养组织)
受精卵
组织 器官 系统 人体 组织 器官 植物体
官、个体。
一、绿色开花植物的六大器官
绿色开花植物体通常都是 由 根、茎 、 叶 、
花 、 果实 、 种子 六 大器官组成。
认真观察下面的绿 色开花植物都有什么器 官?
是不是所有的植物都由六大器官组成的? 植物体的六大器官是不是都同时存在?
不是
苔鲜
海带
只有绿色开花植物才由六大器官组成