Evaluating Affective Computing Environments Using Physiological Measures
考研英语阅读理解外刊原文经济学人
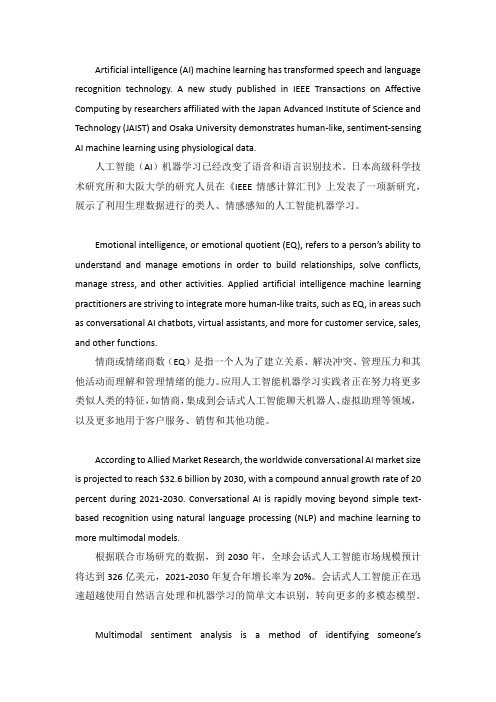
Artificial intelligence (AI) machine learning has transformed speech and language recognition technology. A new study published in IEEE Transactions on Affective Computing by researchers affiliated with the Japan Advanced Institute of Science and Technology (JAIST) and Osaka University demonstrates human-like, sentiment-sensing AI machine learning using physiological data.人工智能(AI)机器学习已经改变了语音和语言识别技术。
日本高级科学技术研究所和大阪大学的研究人员在《IEEE情感计算汇刊》上发表了一项新研究,展示了利用生理数据进行的类人、情感感知的人工智能机器学习。
Emotional intelligence, or emotional quotient (EQ), refers to a person’s ability to understand and manage emotions in order to build relationships, solve conflicts, manage stress, and other activities. Applied artificial intelligence machine learning practitioners are striving to integrate more human-like traits, such as EQ, in areas such as conversational AI chatbots, virtual assistants, and more for customer service, sales, and other functions.情商或情绪商数(EQ)是指一个人为了建立关系、解决冲突、管理压力和其他活动而理解和管理情绪的能力。
2021全国甲卷英语阅读词汇

2021全国甲卷英语阅读词汇2021年全国甲卷英语阅读部分涉及的词汇量相对较大,涵盖了各个领域的词汇。
以下是一些较为常见和重要的词汇,供您参考:1. Technology and Science(科技与科学):- innovation 创新- technological 技术的- artificial intelligence 人工智能- digital 数字的- virtual 虚拟的- algorithm 算法- software 软件- hardware 硬件- robotic 机器人的- engineering 工程学2. Environment and Nature(环境与自然):- pollution 污染- conservation 保护- biodiversity 生物多样性- ecosystem 生态系统- climate change 气候变化- renewable 可再生的- deforestation 森林砍伐- sustainable 可持续的- habitat 栖息地- renewable energy 可再生能源3. Society and Culture(社会与文化):- globalization 全球化- diversity 多样性- multicultural 多元文化的- heritage 遗产- tradition 传统- customs 风俗习惯- cultural exchange 文化交流- values 价值观- generation gap 代沟- social media 社交媒体4. Economics and Business(经济与商业):- inflation 通货膨胀- recession 经济衰退- investment 投资- unemployment 失业- entrepreneur 企业家- market market 市场经济- supply and demand 供求关系- consumer 消费者- profit 利润- competition 竞争5. Education and Learning(教育与学习):- curriculum 课程- assessment 评估- literacy 识字能力- numeracy 数字能力- intellectual property 知识产权- critical thinking 批判性思维- lifelong learning 终身学习- research 研究- distance learning 远程教育- academic 学术的以上是一些常见的词汇,将其运用于阅读理解中可以更好地理解和分析文章,并帮助提高英语阅读能力。
关于人工智能运用于气象的想法 英语作文
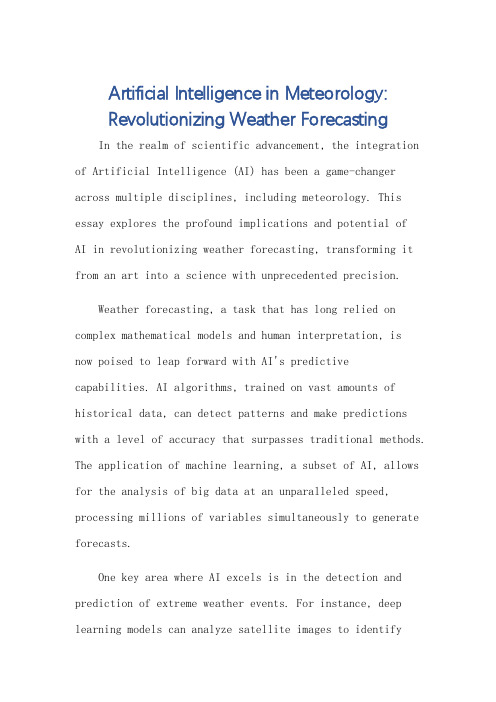
Artificial Intelligence in Meteorology:Revolutionizing Weather ForecastingIn the realm of scientific advancement, the integration of Artificial Intelligence (AI) has been a game-changer across multiple disciplines, including meteorology. This essay explores the profound implications and potential ofAI in revolutionizing weather forecasting, transforming it from an art into a science with unprecedented precision.Weather forecasting, a task that has long relied on complex mathematical models and human interpretation, isnow poised to leap forward with AI's predictivecapabilities. AI algorithms, trained on vast amounts of historical data, can detect patterns and make predictions with a level of accuracy that surpasses traditional methods. The application of machine learning, a subset of AI, allows for the analysis of big data at an unparalleled speed, processing millions of variables simultaneously to generate forecasts.One key area where AI excels is in the detection and prediction of extreme weather events. For instance, deep learning models can analyze satellite images to identifystorm systems and predict their trajectory, intensity, and potential impact. This early warning system can save lives and minimize property damage by providing sufficient time for evacuation and preparation. AI can also enhance our understanding of climate change by analyzing long-term weather patterns, detecting anomalies, and predictingfuture scenarios with more accuracy.Moreover, AI-powered drones and sensors are augmenting traditional weather monitoring. These devices can collect real-time data from remote or hazardous locations, providing a comprehensive view of atmospheric conditions. AI algorithms then process this data, creating a more accurate and detailed picture of weather patterns. In addition, AI can predict localized weather phenomena like microbursts or flash floods, which traditional models often miss due to their broad scope.However, the integration of AI in meteorology is not without challenges. The sheer volume of data generated requires robust infrastructure and sophisticated algorithms to handle. Moreover, ensuring the reliability and transparency of AI predictions is crucial, as errors couldlead to severe consequences. There's also the ethical consideration of AI's role in decision-making processes, particularly in situations where lives are at stake.Despite these challenges, the potential benefits of AIin meteorology are immense. It could lead to the development of personalized weather forecasts, tailored to individual needs and locations. It could also improve the efficiency of industries heavily dependent on weather conditions, such as agriculture, aviation, and energy production.In conclusion, the marriage of AI and meteorology is a testament to the transformative power of technology in science. As we continue to refine and integrate AI into weather forecasting, we are not just enhancing our abilityto predict the weather but also our capacity to understand and mitigate the impacts of our ever-changing climate. The future of meteorology, fueled by AI, promises a world where weather forecasts are more accurate, timely, and actionable, ultimately contributing to a safer and more resilient society.This exploration of "Artificial Intelligence in Meteorology: Revolutionizing Weather Forecasting" underscores the potential of AI in transforming traditional practices and highlights the need for continued research and development in this field. As we delve deeper into the intersection of AI and meteorology, we open up new possibilities for understanding and interacting with the forces that shape our planet.。
高考英语创新环境方面作文

高考英语创新环境方面作文Title: Embracing Innovation in the English Classroom: A Path to a Richer Learning EnvironmentIn the dynamic world of education, the integration of innovation is crucial for fostering a vibrant and engaging learning environment. This integration becomes especially significant when it comes to teaching and learning English, a language that is both global and ever-evolving. Embedding innovation into the English classroom not only enhances student engagement but also prepares them for the challenges and opportunities of the 21st century.Firstly, technology plays a pivotal role in bringing innovation to the English classroom. The use of digital tools like interactive whiteboards, online databases, and learning platforms provide students with a more immersive and interactive learning experience. These tools allow teachers to present content in a dynamic and engaging manner, while also encouraging students to explore and experiment with the language beyond the traditional textbook.Moreover, innovation in the English classroom extends beyond the use of technology. Teachers can foster a culture of creativity and criticalthinking by encouraging students to engage with real-world problems through projects and case studies. This approach not only makes the learning process more relevant and meaningful but also helps students develop the problem-solving skills that are essential for success in today's world.Furthermore, promoting collaborative learning is another innovative strategy that can transform the English classroom. By working in groups, students have the opportunity to share ideas, perspectives, and knowledge, thereby enriching their understanding of the subject matter. Collaborative learning also cultivates important social skills like communication, teamwork, and conflict resolution.In conclusion, innovation is essential for creating a vibrant and engaging learning environment in the English classroom. By harnessing the power of technology, fostering creativity and critical thinking, and promoting collaborative learning, teachers can transform the learning process into a dynamic and meaningful experience for their students. As we move forward in the age of technology and globalization, it is imperative that we continue to innovate and adapt our teaching methods to meet the changing needs of our students and society.。
计算机专业词汇英语翻译
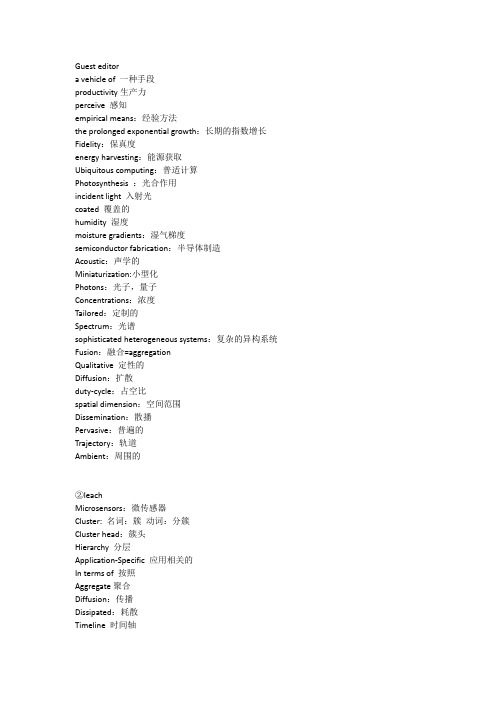
Guest editora vehicle of 一种手段productivity生产力perceive 感知empirical means:经验方法the prolonged exponential growth:长期的指数增长Fidelity:保真度energy harvesting:能源获取Ubiquitous computing:普适计算Photosynthesis :光合作用incident light 入射光coated 覆盖的humidity 湿度moisture gradients:湿气梯度semiconductor fabrication:半导体制造Acoustic:声学的Miniaturization:小型化Photons:光子,量子Concentrations:浓度Tailored:定制的Spectrum:光谱sophisticated heterogeneous systems:复杂的异构系统Fusion:融合=aggregationQualitative 定性的Diffusion:扩散duty-cycle:占空比spatial dimension:空间范围Dissemination:散播Pervasive:普遍的Trajectory:轨道Ambient:周围的②leachMicrosensors:微传感器Cluster: 名词:簇动词:分簇Cluster head:簇头Hierarchy 分层Application-Specific 应用相关的In terms of 按照Aggregate聚合Diffusion:传播Dissipated:耗散Timeline 时间轴Backs off:后退Dissipation:耗散spread-spectrum:扩频intra-cluster:簇内Outperform:胜过③pegasisHomogeneous:同质的fusion :融合aggregationFuse:v. 融合Humidity:湿度Beacon:信标timestamp 时间戳in terms of :就...而言greedy approach:贪婪算法truncated chain:截断链Critical:关键的propagation delays:传播延迟Dissipate:v.发散SNR:信噪比Joules:焦耳The upper bound:上限tier:等级token :令牌,象征Dense:密集的Sparse:稀疏的Heuristic:启发式Outperforms:胜过Preliminary:初步的Exponential:指数的traveling salesman problem 旅行商问题tradeoff 代价④z-macLatency:时间延迟Robust:鲁棒性slot assignment:时隙分配multiple access control:多址接入控制Aggregate:聚合duty cycle:占空比the overhead of:开销Vendors:厂商surface-mount:表面贴装hand-soldering:手工焊接Predetermined:预定的Stochastic:随机的Explicit Contention Notification:明确竞争通知Unicast:单播Congestion:拥塞Benchmark:基准Preamble:头部⑤A building。
人工智能与环境科学:智能环境监测与保护
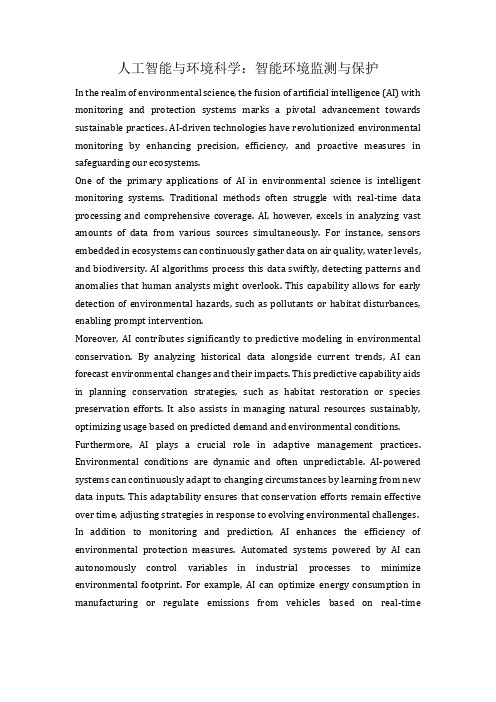
人工智能与环境科学:智能环境监测与保护In the realm of environmental science, the fusion of artificial intelligence (AI) with monitoring and protection systems marks a pivotal advancement towards sustainable practices. AI-driven technologies have revolutionized environmental monitoring by enhancing precision, efficiency, and proactive measures in safeguarding our ecosystems.One of the primary applications of AI in environmental science is intelligent monitoring systems. Traditional methods often struggle with real-time data processing and comprehensive coverage. AI, however, excels in analyzing vast amounts of data from various sources simultaneously. For instance, sensors embedded in ecosystems can continuously gather data on air quality, water levels, and biodiversity. AI algorithms process this data swiftly, detecting patterns and anomalies that human analysts might overlook. This capability allows for early detection of environmental hazards, such as pollutants or habitat disturbances, enabling prompt intervention.Moreover, AI contributes significantly to predictive modeling in environmental conservation. By analyzing historical data alongside current trends, AI can forecast environmental changes and their impacts. This predictive capability aids in planning conservation strategies, such as habitat restoration or species preservation efforts. It also assists in managing natural resources sustainably, optimizing usage based on predicted demand and environmental conditions. Furthermore, AI plays a crucial role in adaptive management practices. Environmental conditions are dynamic and often unpredictable. AI-powered systems can continuously adapt to changing circumstances by learning from new data inputs. This adaptability ensures that conservation efforts remain effective over time, adjusting strategies in response to evolving environmental challenges. In addition to monitoring and prediction, AI enhances the efficiency of environmental protection measures. Automated systems powered by AI can autonomously control variables in industrial processes to minimize environmental footprint. For example, AI can optimize energy consumption in manufacturing or regulate emissions from vehicles based on real-timeenvironmental conditions. Such applications not only reduce ecological impact but also contribute to achieving sustainability goals more effectively.In conclusion, the integration of artificial intelligence with environmental science represents a paradigm shift in how we perceive and protect our natural world. By leveraging AI's capabilities in monitoring, prediction, and adaptive management, we can forge a path towards sustainable development and ecological resilience. As technology continues to advance, so too does our ability to safeguard the environment for future generations.。
在信息时代培养有效的信息辨别能力英语范文
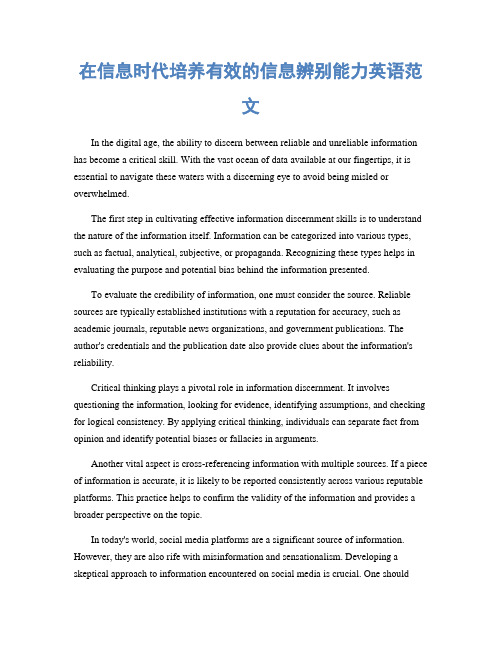
在信息时代培养有效的信息辨别能力英语范文In the digital age, the ability to discern between reliable and unreliable information has become a critical skill. With the vast ocean of data available at our fingertips, it is essential to navigate these waters with a discerning eye to avoid being misled or overwhelmed.The first step in cultivating effective information discernment skills is to understand the nature of the information itself. Information can be categorized into various types, such as factual, analytical, subjective, or propaganda. Recognizing these types helps in evaluating the purpose and potential bias behind the information presented.To evaluate the credibility of information, one must consider the source. Reliable sources are typically established institutions with a reputation for accuracy, such as academic journals, reputable news organizations, and government publications. The author's credentials and the publication date also provide clues about the information's reliability.Critical thinking plays a pivotal role in information discernment. It involves questioning the information, looking for evidence, identifying assumptions, and checking for logical consistency. By applying critical thinking, individuals can separate fact from opinion and identify potential biases or fallacies in arguments.Another vital aspect is cross-referencing information with multiple sources. If a piece of information is accurate, it is likely to be reported consistently across various reputable platforms. This practice helps to confirm the validity of the information and provides a broader perspective on the topic.In today's world, social media platforms are a significant source of information. However, they are also rife with misinformation and sensationalism. Developing a skeptical approach to information encountered on social media is crucial. One shouldalways verify the information with credible sources before accepting it as true or sharing it with others.Digital literacy is also an essential component of information discernment. Being digitally literate means having the skills to search for, locate, evaluate, and use information effectively. It includes understanding how search engines work, using advanced search techniques, and being aware of the algorithms that might affect the visibility of certain information.In conclusion, cultivating effective information discernment skills in the information age is not just beneficial; it is necessary. It empowers individuals to make informed decisions, engage in intelligent discourse, and contribute positively to society. By honing these skills, we can all become more responsible consumers and disseminators of information in an increasingly connected world. 。
2018年高考英语作文范文
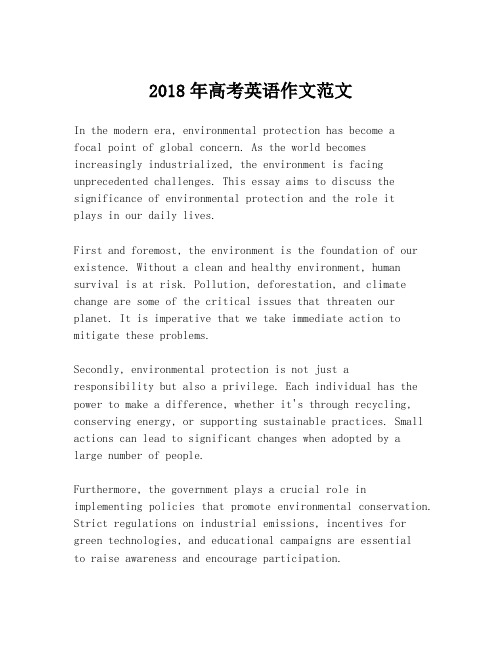
2018年高考英语作文范文In the modern era, environmental protection has become afocal point of global concern. As the world becomes increasingly industrialized, the environment is facing unprecedented challenges. This essay aims to discuss the significance of environmental protection and the role itplays in our daily lives.First and foremost, the environment is the foundation of our existence. Without a clean and healthy environment, human survival is at risk. Pollution, deforestation, and climate change are some of the critical issues that threaten our planet. It is imperative that we take immediate action to mitigate these problems.Secondly, environmental protection is not just aresponsibility but also a privilege. Each individual has the power to make a difference, whether it's through recycling, conserving energy, or supporting sustainable practices. Small actions can lead to significant changes when adopted by alarge number of people.Furthermore, the government plays a crucial role in implementing policies that promote environmental conservation. Strict regulations on industrial emissions, incentives for green technologies, and educational campaigns are essentialto raise awareness and encourage participation.In conclusion, environmental protection is a collectiveeffort that requires the commitment of every citizen and organization. It is our duty to ensure that future generations inherit a world that is as vibrant and diverse as the one we have today. By taking proactive steps, we can preserve our environment and secure a sustainable future for all.。
人脸表情识别英文参考资料
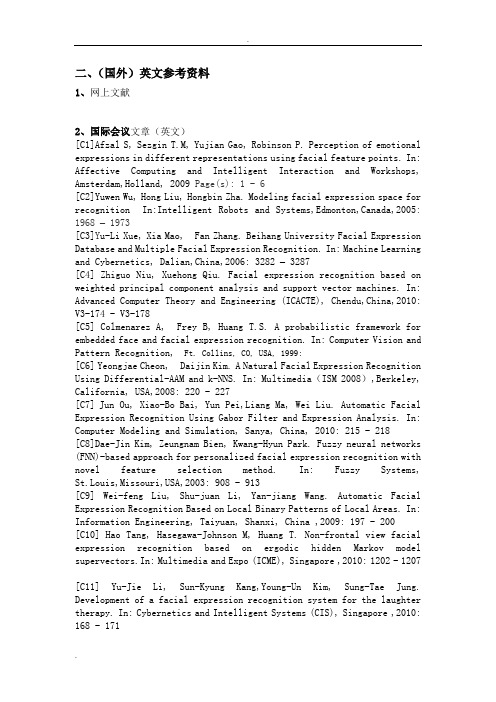
二、(国外)英文参考资料1、网上文献2、国际会议文章(英文)[C1]Afzal S, Sezgin T.M, Yujian Gao, Robinson P. Perception of emotional expressions in different representations using facial feature points. In: Affective Computing and Intelligent Interaction and Workshops, Amsterdam,Holland, 2009 Page(s): 1 - 6[C2]Yuwen Wu, Hong Liu, Hongbin Zha. Modeling facial expression space for recognition In:Intelligent Robots and Systems,Edmonton,Canada,2005: 1968 – 1973[C3]Y u-Li Xue, Xia Mao, Fan Zhang. Beihang University Facial Expression Database and Multiple Facial Expression Recognition. In: Machine Learning and Cybernetics, Dalian,China,2006: 3282 – 3287[C4] Zhiguo Niu, Xuehong Qiu. Facial expression recognition based on weighted principal component analysis and support vector machines. In: Advanced Computer Theory and Engineering (ICACTE), Chendu,China,2010: V3-174 - V3-178[C5] Colmenarez A, Frey B, Huang T.S. A probabilistic framework for embedded face and facial expression recognition. In: Computer Vision and Pattern Recognition, Ft. Collins, CO, USA, 1999:[C6] Yeongjae Cheon, Daijin Kim. A Natural Facial Expression Recognition Using Differential-AAM and k-NNS. In: Multimedia(ISM 2008),Berkeley, California, USA,2008: 220 - 227[C7]Jun Ou, Xiao-Bo Bai, Yun Pei,Liang Ma, Wei Liu. Automatic Facial Expression Recognition Using Gabor Filter and Expression Analysis. In: Computer Modeling and Simulation, Sanya, China, 2010: 215 - 218[C8]Dae-Jin Kim, Zeungnam Bien, Kwang-Hyun Park. Fuzzy neural networks (FNN)-based approach for personalized facial expression recognition with novel feature selection method. In: Fuzzy Systems, St.Louis,Missouri,USA,2003: 908 - 913[C9] Wei-feng Liu, Shu-juan Li, Yan-jiang Wang. Automatic Facial Expression Recognition Based on Local Binary Patterns of Local Areas. In: Information Engineering, Taiyuan, Shanxi, China ,2009: 197 - 200[C10] Hao Tang, Hasegawa-Johnson M, Huang T. Non-frontal view facial expression recognition based on ergodic hidden Markov model supervectors.In: Multimedia and Expo (ICME), Singapore ,2010: 1202 - 1207[C11] Yu-Jie Li, Sun-Kyung Kang,Young-Un Kim, Sung-Tae Jung. Development of a facial expression recognition system for the laughter therapy. In: Cybernetics and Intelligent Systems (CIS), Singapore ,2010: 168 - 171[C12] Wei Feng Liu, ZengFu Wang. Facial Expression Recognition Based on Fusion of Multiple Gabor Features. In: Pattern Recognition, HongKong, China, 2006: 536 - 539[C13] Chen Feng-jun, Wang Zhi-liang, Xu Zheng-guang, Xiao Jiang. Facial Expression Recognition Based on Wavelet Energy Distribution Feature and Neural Network Ensemble. In: Intelligent Systems, XiaMen, China, 2009: 122 - 126[C14] P. Kakumanu, N. Bourbakis. A Local-Global Graph Approach for Facial Expression Recognition. In: Tools with Artificial Intelligence, Arlington, Virginia, USA,2006: 685 - 692[C15] Mingwei Huang, Zhen Wang, Zilu Ying. Facial expression recognition using Stochastic Neighbor Embedding and SVMs. In: System Science and Engineering (ICSSE), Macao, China, 2011: 671 - 674[C16] Junhua Li, Li Peng. Feature difference matrix and QNNs for facial expression recognition. In: Control and Decision Conference, Yantai, China, 2008: 3445 - 3449[C17] Yuxiao Hu, Zhihong Zeng, Lijun Yin,Xiaozhou Wei, Jilin Tu, Huang, T.S. A study of non-frontal-view facial expressions recognition. In: Pattern Recognition, Tampa, FL, USA,2008: 1 - 4[C18] Balasubramani A, Kalaivanan K, Karpagalakshmi R.C, Monikandan R. Automatic facial expression recognition system. In: Computing, Communication and Networking, St. Thomas,USA, 2008: 1 - 5[C19] Hui Zhao, Zhiliang Wang, Jihui Men. Facial Complex Expression Recognition Based on Fuzzy Kernel Clustering and Support Vector Machines. In: Natural Computation, Haikou,Hainan,China,2007: 562 - 566[C20] Khanam A, Shafiq M.Z, Akram M.U. Fuzzy Based Facial Expression Recognition. In: Image and Signal Processing, Sanya, Hainan, China,2008: 598 - 602[C21] Sako H, Smith A.V.W. Real-time facial expression recognition based on features' positions and dimensions. In: Pattern Recognition, Vienna,Austria, 1996: 643 - 648 vol.3[C22] Huang M.W, Wang Z.W, Ying Z.L. A novel method of facial expression recognition based on GPLVM Plus SVM. In: Signal Processing (ICSP), Beijing, China, 2010: 916 - 919[C23] Xianxing Wu; Jieyu Zhao; Curvelet feature extraction for face recognition and facial expression recognition. In: Natural Computation (ICNC), Yantai, China, 2010: 1212 - 1216[C24]Xu Q Z, Zhang P Z, Yang L X, et al.A facial expression recognition approach based on novel support vector machine tree. In Proceedings of the 4th International Symposium on Neural Networks, Nanjing, China, 2007: 374-381.[C25] Wang Y B, Ai H Z, Wu B, et al. Real time facial expression recognition with adaboost.In: Proceedings of the 17th International Conference on Pattern Recognition , Cambridge,UK, 2004: 926-929.[C26] Guo G, Dyer C R. Simultaneous feature selection and classifier training via linear programming: a case study for face expression recognition. In: Proceedings of the 2003 IEEE Computer Society Conference on Computer Vision and Pattern Recognition, Madison, W isconsin, USA, 2003,1: 346-352.[C27] Bourel F, Chibelushi C C, Low A A. Robust facial expression recognition using a state-based model of spatially-localised facial dynamics. In: Proceedings of the 5th IEEE International Conference on Automatic Face and Gesture Recognition, Washington, DC, USA, 2002: 113-118·[C28] Buciu I, Kotsia I, Pitas I. Facial expression analysis under partial occlusion. In: Proceedings of the 2005 IEEE International Conference on Acoustics, Speech, and Signal Processing, Philadelphia, PA, USA, 2005,V: 453-456.[C29] ZHAN Yong-zhao,YE Jing-fu,NIU De-jiao,et al.Facial expression recognition based on Gabor wavelet transformation and elastic templates matching. Proc of the 3rd International Conference on Image and Graphics.Washington DC, USA,2004:254-257.[C30] PRASEEDA L V,KUMAR S,VIDYADHARAN D S,et al.Analysis of facial expressions using PCA on half and full faces. Proc of ICALIP2008.2008:1379-1383.[C31]LEE J J,UDDIN M Z,KIM T S.Spatiotemporal human facial expression recognition using Fisher independent component analysis and Hidden Markov model[C]//Proc of the 30th Annual International Conference of IEEE Engineering in Medicine and Biology Society.2008:2546-2549.[C32] LITTLEWORT G,BARTLETT M,FASELL. Dynamics of facial expression extracted automatically from video. In: Proceedings of IEEE Conference on Computer Vision and Pattern Recognition, Workshop on Face Processing inVideo, Washington DC,USA,2006:80-81.[C33] Kotsia I, Nikolaidis N, Pitas I. Facial Expression Recognition in Videos using a Novel Multi-Class Support Vector Machines Variant. In: Acoustics, Speech and Signal Processing, Honolulu, Hawaii, USA, 2007: II-585 - II-588[C34] Ruo Du, Qiang Wu, Xiangjian He, Wenjing Jia, Daming Wei. Facial expression recognition using histogram variances faces. In: Applications of Computer Vision (WACV), Snowbird, Utah, USA, 2009: 1 - 7[C35] Kobayashi H, Tange K, Hara F. Real-time recognition of six basic facial expressions. In: Robot and Human Communication, Tokyo , Japan,1995: 179 - 186[C36] Hao Tang, Huang T.S. 3D facial expression recognition based on properties of line segments connecting facial feature points. In: Automatic Face & Gesture Recognition, Amsterdam, The Netherlands, 2008: 1 - 6[C37] Fengjun Chen, Zhiliang Wang, Zhengguang Xu, Donglin Wang. Research on a method of facial expression recognition.in: Electronic Measurement & Instruments, Beijing,China, 2009: 1-225 - 1-229[C38] Hui Zhao, Tingting Xue, Linfeng Han. Facial complex expression recognition based on Latent DirichletAllocation. In: Natural Computation (ICNC), Yantai, Shandong, China, 2010: 1958 - 1960[C39] Qinzhen Xu, Pinzheng Zhang, Wenjiang Pei, Luxi Yang, Zhenya He. An Automatic Facial Expression Recognition Approach Based on Confusion-Crossed Support Vector Machine Tree. In: Acoustics, Speech and Signal Processing, Honolulu, Hawaii, USA, 2007: I-625 - I-628[C40] Sung Uk Jung, Do Hyoung Kim, Kwang Ho An, Myung Jin Chung. Efficient rectangle feature extraction for real-time facial expression recognition based on AdaBoost.In: Intelligent Robots and Systems, Edmonton,Canada, 2005: 1941 - 1946[C41] Patil K.K, Giripunje S.D, Bajaj P.R. Facial Expression Recognition and Head Tracking in Video Using Gabor Filter .In: Emerging Trends in Engineering and Technology (ICETET), Goa, India, 2010: 152 - 157[C42] Jun Wang, Lijun Yin, Xiaozhou Wei, Yi Sun. 3D Facial Expression Recognition Based on Primitive Surface Feature Distribution.In: Computer Vision and Pattern Recognition, New York, USA,2006: 1399 - 1406[C43] Shi Dongcheng, Jiang Jieqing. The method of facial expression recognition based on DWT-PCA/LDA.IN: Image and Signal Processing (CISP), Yantai,China, 2010: 1970 - 1974[C44] Asthana A, Saragih J, Wagner M, Goecke R. Evaluating AAM fitting methods for facial expression recognition. In: Affective Computing and Intelligent Interaction and Workshops, Amsterdam,Holland, 2009:1-8[C45] Geng Xue, Zhang Youwei. Facial Expression Recognition Based on the Difference of Statistical Features.In: Signal Processing, Guilin, China, 2006[C46] Metaxas D. Facial Features Tracking for Gross Head Movement analysis and Expression Recognition.In: Multimedia Signal Processing, Chania,Crete,GREECE, 2007:2[C47] Xia Mao, YuLi Xue, Zheng Li, Kang Huang, ShanWei Lv. Robust facial expression recognition based on RPCA and AdaBoost.In: Image Analysis for Multimedia Interactive Services, London, UK, 2009: 113 - 116[C48] Kun Lu, Xin Zhang. Facial Expression Recognition from Image Sequences Based on Feature Points and Canonical Correlations.In: Artificial Intelligence and Computational Intelligence (AICI), Sanya,China, 2010: 219 - 223[C49] Peng Zhao-yi, Wen Zhi-qiang, Zhou Yu. Application of Mean Shift Algorithm in Real-Time Facial Expression Recognition.In: Computer Network and Multimedia Technology, Wuhan,China, 2009: 1 - 4[C50] Xu Chao, Feng Zhiyong, Facial Expression Recognition and Synthesis on Affective Emotions Composition.In: Future BioMedical InformationEngineering, Wuhan,China, 2008: 144 - 147[C51] Zi-lu Ying, Lin-bo Cai. Facial Expression Recognition with Marginal Fisher Analysis on Local Binary Patterns.In: Information Science and Engineering (ICISE), Nanjing,China, 2009: 1250 - 1253[C52] Chuang Yu, Yuning Hua, Kun Zhao. The Method of Human Facial Expression Recognition Based on Wavelet Transformation Reducing the Dimension and Improved Fisher Discrimination.In: Intelligent Networks and Intelligent Systems (ICINIS), Shenyang,China, 2010: 43 - 47[C53] Stratou G, Ghosh A, Debevec P, Morency L.-P. Effect of illumination on automatic expression recognition: A novel 3D relightable facial database .In: Automatic Face & Gesture Recognition and Workshops (FG 2011), Santa Barbara, California,USA, 2011: 611 - 618[C54] Jung-Wei Hong, Kai-Tai Song. Facial expression recognition under illumination variation.In: Advanced Robotics and Its Social Impacts, Hsinchu, Taiwan,2007: 1 - 6[C55] Ryan A, Cohn J.F, Lucey S, Saragih J, Lucey P, De la Torre F, Rossi A. Automated Facial Expression Recognition System.In: Security Technology, Zurich, Switzerland, 2009: 172 - 177[C56] Gokturk S.B, Bouguet J.-Y, Tomasi C, Girod B. Model-based face tracking for view-independent facial expression recognition.In: Automatic Face and Gesture Recognition, Washington, D.C., USA, 2002: 287 - 293[C57] Guo S.M, Pan Y.A, Liao Y.C, Hsu C.Y, Tsai J.S.H, Chang C.I. A Key Frame Selection-Based Facial Expression Recognition System.In: Innovative Computing, Information and Control, Beijing,China, 2006: 341 - 344[C58] Ying Zilu, Li Jingwen, Zhang Youwei. Facial expression recognition based on two dimensional feature extraction.In: Signal Processing, Leipzig, Germany, 2008: 1440 - 1444[C59] Fengjun Chen, Zhiliang Wang, Zhengguang Xu, Jiang Xiao, Guojiang Wang. Facial Expression Recognition Using Wavelet Transform and Neural Network Ensemble.In: Intelligent Information Technology Application, Shanghai,China,2008: 871 - 875[C60] Chuan-Yu Chang, Yan-Chiang Huang, Chi-Lu Yang. Personalized Facial Expression Recognition in Color Image.In: Innovative Computing, Information and Control (ICICIC), Kaohsiung,Taiwan, 2009: 1164 - 1167 [C61] Bourel F, Chibelushi C.C, Low A.A. Robust facial expression recognition using a state-based model of spatially-localised facial dynamics. In: Automatic Face and Gesture Recognition, Washington, D.C., USA, 2002: 106 - 111[C62] Chen Juanjuan, Zhao Zheng, Sun Han, Zhang Gang. Facial expression recognition based on PCA reconstruction.In: Computer Science and Education (ICCSE), Hefei,China, 2010: 195 - 198[C63] Guotai Jiang, Xuemin Song, Fuhui Zheng, Peipei Wang, Omer A.M.Facial Expression Recognition Using Thermal Image.In: Engineering in Medicine and Biology Society, Shanghai,China, 2005: 631 - 633[C64] Zhan Yong-zhao, Ye Jing-fu, Niu De-jiao, Cao Peng. Facial expression recognition based on Gabor wavelet transformation and elastic templates matching.In: Image and Graphics, Hongkong,China, 2004: 254 - 257[C65] Ying Zilu, Zhang Guoyi. Facial Expression Recognition Based on NMF and SVM. In: Information Technology and Applications, Chengdu,China, 2009: 612 - 615[C66] Xinghua Sun, Hongxia Xu, Chunxia Zhao, Jingyu Yang. Facial expression recognition based on histogram sequence of local Gabor binary patterns. In: Cybernetics and Intelligent Systems, Chengdu,China, 2008: 158 - 163[C67] Zisheng Li, Jun-ichi Imai, Kaneko M. Facial-component-based bag of words and PHOG descriptor for facial expression recognition.In: Systems, Man and Cybernetics, San Antonio,TX,USA,2009: 1353 - 1358[C68] Chuan-Yu Chang, Yan-Chiang Huang. Personalized facial expression recognition in indoor environments.In: Neural Networks (IJCNN), Barcelona, Spain, 2010: 1 - 8[C69] Ying Zilu, Fang Xieyan. Combining LBP and Adaboost for facial expression recognition.In: Signal Processing, Leipzig, Germany, 2008: 1461 - 1464[C70] Peng Yang, Qingshan Liu, Metaxas, D.N. RankBoost with l1 regularization for facial expression recognition and intensity estimation.In: Computer Vision, Kyoto,Japan, 2009: 1018 - 1025[C71] Patil R.A, Sahula V, Mandal A.S. Automatic recognition of facial expressions in image sequences: A review.In: Industrial and Information Systems (ICIIS), Mangalore, India, 2010: 408 - 413[C72] Iraj Hosseini, Nasim Shams, Pooyan Amini, Mohammad S. Sadri, Masih Rahmaty, Sara Rahmaty. Facial Expression Recognition using Wavelet-Based Salient Points and Subspace Analysis Methods.In: Electrical and Computer Engineering, Ottawa, Canada, 2006: 1992 - 1995[C73][C74][C75][C76][C77][C78][C79]3、英文期刊文章[J1] Aleksic P.S., Katsaggelos A.K. Automatic facial expression recognition using facial animation parameters and multistream HMMs.IEEE Transactions on Information Forensics and Security, 2006, 1(1):3-11 [J2] Kotsia I,Pitas I. Facial Expression Recognition in Image Sequences Using Geometric Deformation Features and Support Vector Machines. IEEE Transactions on Image Processing, 2007, 16(1):172 – 187[J3] Mpiperis I, Malassiotis S, Strintzis M.G. Bilinear Models for 3-D Face and Facial Expression Recognition. IEEE Transactions on Information Forensics and Security, 2008,3(3) : 498 - 511[J4] Sung J, Kim D. Pose-Robust Facial Expression Recognition Using View-Based 2D+3D AAM. IEEE Transactions on Systems and Humans, 2008 , 38 (4): 852 - 866[J5]Yeasin M, Bullot B, Sharma R. Recognition of facial expressions and measurement of levels of interest from video. IEEE Transactions on Multimedia, 2006, 8(3): 500 - 508[J6] Wenming Zheng, Xiaoyan Zhou, Cairong Zou, Li Zhao. Facial expression recognition using kernel canonical correlation analysis (KCCA). IEEE Transactions on Neural Networks, 2006, 17(1): 233 - 238 [J7]Pantic M, Patras I. Dynamics of facial expression: recognition of facial actions and their temporal segments from face profile image sequences. IEEE Transactions on Systems, Man, and Cybernetics, Part B: Cybernetics, 2006, 36(2): 433 - 449[J8] Mingli Song, Dacheng Tao, Zicheng Liu, Xuelong Li, Mengchu Zhou. Image Ratio Features for Facial Expression Recognition Application. IEEE Transactions on Systems, Man, and Cybernetics, Part B: Cybernetics, 2010, 40(3): 779 - 788[J9] Dae Jin Kim, Zeungnam Bien. Design of “Personalized” Classifier Using Soft Computing Techniques for “Personalized” Facial Expression Recognition. IEEE Transactions on Fuzzy Systems, 2008, 16(4): 874 - 885 [J10] Uddin M.Z, Lee J.J, Kim T.-S. An enhanced independent component-based human facial expression recognition from video. IEEE Transactions on Consumer Electronics, 2009, 55(4): 2216 - 2224[J11] Ruicong Zhi, Flierl M, Ruan Q, Kleijn W.B. Graph-Preserving Sparse Nonnegative Matrix Factorization With Application to Facial Expression Recognition. IEEE Transactions on Systems, Man, and Cybernetics, Part B:Cybernetics, 2011, 41(1): 38 - 52[J12] Chibelushi C.C, Bourel F. Hierarchical multistream recognition of facial expressions. IEE Proceedings - Vision, Image and Signal Processing, 2004, 151(4): 307 - 313[J13] Yongsheng Gao, Leung M.K.H, Siu Cheung Hui, Tananda M.W. Facial expression recognition from line-based caricatures. IEEE Transactions on Systems, Man and Cybernetics, Part A: Systems and Humans, 2003, 33(3): 407 - 412[J14] Ma L, Khorasani K. Facial expression recognition using constructive feedforward neural networks. IEEE Transactions on Systems, Man, and Cybernetics, Part B: Cybernetics, 2004, 34(3): 1588 - 1595[J15] Essa I.A, Pentland A.P. Coding, analysis, interpretation, and recognition of facial expressions. IEEE Transactions on Pattern Analysis and Machine Intelligence, 1997, 19(7): 757 - 763[J16] Anderson K, McOwan P.W. A real-time automated system for the recognition of human facial expressions. IEEE Transactions on Systems, Man, and Cybernetics, Part B: Cybernetics, 2006, 36(1): 96 - 105[J17] Soyel H, Demirel H. Facial expression recognition based on discriminative scale invariant feature transform. Electronics Letters 2010, 46(5): 343 - 345[J18] Fei Cheng, Jiangsheng Yu, Huilin Xiong. Facial Expression Recognition in JAFFE Dataset Based on Gaussian Process Classification. IEEE Transactions on Neural Networks, 2010, 21(10): 1685 – 1690[J19] Shangfei Wang, Zhilei Liu, Siliang Lv, Yanpeng Lv, Guobing Wu, Peng Peng, Fei Chen, Xufa Wang. A Natural Visible and Infrared Facial Expression Database for Expression Recognition and Emotion Inference. IEEE Transactions on Multimedia, 2010, 12(7): 682 - 691[J20] Lajevardi S.M, Hussain Z.M. Novel higher-order local autocorrelation-like feature extraction methodology for facial expression recognition. IET Image Processing, 2010, 4(2): 114 - 119[J21] Yizhen Huang, Ying Li, Na Fan. Robust Symbolic Dual-View Facial Expression Recognition With Skin Wrinkles: Local Versus Global Approach. IEEE Transactions on Multimedia, 2010, 12(6): 536 - 543[J22] Lu H.-C, Huang Y.-J, Chen Y.-W. Real-time facial expression recognition based on pixel-pattern-based texture feature. Electronics Letters 2007, 43(17): 916 - 918[J23]Zhang L, Tjondronegoro D. Facial Expression Recognition Using Facial Movement Features. IEEE Transactions on Affective Computing, 2011, pp(99): 1[J24] Zafeiriou S, Pitas I. Discriminant Graph Structures for Facial Expression Recognition. Multimedia, IEEE Transactions on 2008,10(8): 1528 - 1540[J25]Oliveira L, Mansano M, Koerich A, de Souza Britto Jr. A. Selecting 2DPCA Coefficients for Face and Facial Expression Recognition. Computingin Science & Engineering, 2011, pp(99): 1[J26] Chang K.I, Bowyer W, Flynn P.J. Multiple Nose Region Matching for 3D Face Recognition under Varying Facial Expression. Pattern Analysis and Machine Intelligence, IEEE Transactions on2006, 28(10): 1695 - 1700 [J27] Kakadiaris I.A, Passalis G, Toderici G, Murtuza M.N, Yunliang Lu, Karampatziakis N, Theoharis T. Three-Dimensional Face Recognition in the Presence of Facial Expressions: An Annotated Deformable Model Approach.IEEE Transactions on Pattern Analysis and Machine Intelligence, 2007, 29(4): 640 - 649[J28] Guoying Zhao, Pietikainen M. Dynamic Texture Recognition Using Local Binary Patterns with an Application to Facial Expressions. IEEE Transactions on Pattern Analysis and Machine Intelligence, 2007, 29(6): 915 - 928[J29] Chakraborty A, Konar A, Chakraborty U.K, Chatterjee A. Emotion Recognition From Facial Expressions and Its Control Using Fuzzy Logic. IEEE Transactions on Systems, Man and Cybernetics, Part A: Systems and Humans, 2009, 39(4): 726 - 743[J30] Pantic M, RothkrantzL J.M. Facial action recognition for facial expression analysis from static face images. IEEE Transactions on Systems, Man, and Cybernetics, Part B: Cybernetics, 2004, 34(3): 1449 - 1461 [J31] Calix R.A, Mallepudi S.A, Bin Chen, Knapp G.M. Emotion Recognition in Text for 3-D Facial Expression Rendering. IEEE Transactions on Multimedia, 2010, 12(6): 544 - 551[J32]Kotsia I, Pitas I, Zafeiriou S, Zafeiriou S. Novel Multiclass Classifiers Based on the Minimization of the Within-Class Variance. IEEE Transactions on Neural Networks, 2009, 20(1): 14 - 34[J33]Cohen I, Cozman F.G, Sebe N, Cirelo M.C, Huang T.S. Semisupervised learning of classifiers: theory, algorithms, and their application to human-computer interaction. IEEE Transactions on Pattern Analysis and Machine Intelligence, 2004, 26(12): 1553 - 1566[J34] Zafeiriou S. Discriminant Nonnegative Tensor Factorization Algorithms. IEEE Transactions on Neural Networks, 2009, 20(2): 217 - 235 [J35] Zafeiriou S, Petrou M. Nonlinear Non-Negative Component Analysis Algorithms. IEEE Transactions on Image Processing, 2010, 19(4): 1050 - 1066[J36] Kotsia I, Zafeiriou S, Pitas I. A Novel Discriminant Non-Negative Matrix Factorization Algorithm With Applications to Facial Image Characterization Problems. IEEE Transactions on Information Forensics and Security, 2007, 2(3): 588 - 595[J37] Irene Kotsia, Stefanos Zafeiriou, Ioannis Pitas. Texture and shape information fusion for facial expression and facial action unit recognition. Pattern Recognition, 2008, 41(3): 833-851[J38]Wenfei Gu, Cheng Xiang, Y.V. Venkatesh, Dong Huang, Hai Lin. Facial expression recognition using radial encoding of local Gabor features andclassifier synthesis.Pattern Recognition, In Press, Corrected Proof, Available online 27 May 2011[J39] F Dornaika, E Lazkano, B Sierra. Improving dynamic facial expression recognition with feature subset selection.Pattern Recognition Letters, 2011, 32(5): 740-748[J40] Te-Hsun Wang, Jenn-Jier James Lien. Facial expression recognition system based on rigid and non-rigid motion separation and 3D pose estimation. Pattern Recognition, 2009, 42(5): 962-977[J41] Hyung-Soo Lee, Daijin Kim.Expression-invariant face recognition by facial expression transformations.Pattern Recognition Letters, 2008, 29(13): 1797-1805[J42] Guoying Zhao, Matti Pietikäinen. Boosted multi-resolution spatiotemporal descriptors for facial expression recognition. Pattern Recognition Letters, 2009, 30(12): 1117-1127[J43] Xudong Xie, Kin-Man Lam. Facial expression recognition based on shape and texture. Pattern Recognition, 2009, 42(5):1003-1011[J44] Peng Yang, Qingshan Liu, Dimitris N. Metaxas Boosting encoded dynamic features for facial expression recognition. Pattern Recognition Letters, 2009,30(2): 132-139[J45] Sungsoo Park, Daijin Kim. Subtle facial expression recognition using motion magnification. Pattern Recognition Letters, 2009, 30(7): 708-716[J46] Chathura R. De Silva, Surendra Ranganath, Liyanage C. De Silva. Cloud basis function neural network: A modified RBF network architecture for holistic facial expression recognition.Pattern Recognition, 2008, 41(4): 1241-1253[J47] Do Hyoung Kim, Sung Uk Jung, Myung Jin Chung. Extension of cascaded simple feature based face detection to facial expression recognition.Pattern Recognition Letters, 2008, 29(11): 1621-1631[J48] Y. Zhu, L.C. De Silva, C.C. Ko. Using moment invariants and HMM in facial expression recognition. Pattern Recognition Letters, 2002, 23(1-3): 83-91[J49] Jun Wang, Lijun Yin. Static topographic modeling for facial expression recognition and puter Vision and Image Understanding, 2007, 108(1-2): 19-34[J50] Caifeng Shan, Shaogang Gong, Peter W. McOwan. Facial expression recognition based on Local Binary Patterns: A comprehensive study. Image and Vision Computing, 2009, 27(6): 803-816[J51] Xue-wen Chen, Thomas Huang. Facial expression recognition: A clustering-based approach. Pattern Recognition Letters, 2003, 24(9-10): 1295-1302[J52] Irene Kotsia, Ioan Buciu, Ioannis Pitas.An analysis of facial expression recognition under partial facial image occlusion. Image and Vision Computing, 2008, 26(7): 1052-1067[J53] Shuai Liu, Qiuqi Ruan. Orthogonal Tensor Neighborhood Preserving Embedding for facial expression recognition.Pattern Recognition, 2011, 44(7):1497-1513[J54] Eszter Székely, Henning Tiemeier, Lidia R. Arends, Vincent W.V. Jaddoe, Albert Hofman, Frank C. Verhulst, Catherine M. Herba. Recognition of Facial Expressions of Emotions by 3-Year-Olds. Emotion, 2011, 11(2): 425-435[J55] Kathleen M. Corcoran, Sheila R. Woody, David F. Tolin.Recognition of facial expressions in obsessive–compulsive disorder. Journal of Anxiety Disorders, 2008, 22(1): 56-66[J56] Bouchra Abboud, Franck Davoine, MôDang. Facial expression recognition and synthesis based on an appearance model. Signal Processing: Image Communication, 2004, 19(8): 723-740[J57] Teng Sha, Mingli Song, Jiajun Bu, Chun Chen, Dacheng Tao. Feature level analysis for 3D facial expression recognition. Neurocomputing, 2011, 74(12-13) :2135-2141[J58] S. Moore, R. Bowden. Local binary patterns for multi-view facial expression recognition. Computer Vision and Image Understanding, 2011, 15(4):541-558[J59] Rui Xiao, Qijun Zhao, David Zhang, Pengfei Shi. Facial expression recognition on multiple manifolds.Pattern Recognition, 2011, 44(1):107-116[J60] Shyi-Chyi Cheng, Ming-Yao Chen, Hong-Yi Chang, Tzu-Chuan Chou. Semantic-based facial expression recognition using analytical hierarchy process.Expert Systems with Applications, 2007, 33(1): 86-95[J71] Carlos E. Thomaz, Duncan F. Gillies, Raul Q. Feitosa. Using mixture covariance matrices to improve face and facial expression recognitions. Pattern Recognition Letters, 2003, 24(13): 2159-2165[J72]Wen G,Bo C,Shan Shi-guang,et al. The CAS-PEAL large-scale Chinese face database and baseline evaluations.IEEE Transactions on Systems,Man and Cybernetics,part A:Systems and Hu-mans,2008,38(1):149-161. [J73] Yongsheng Gao,Leung ,M.K.H. Face recognition using line edge map.IEEE Transactions on Pattern Analysis and Machine Intelligence,2002,24:764-779.[J74] Hanouz M,Kittler J,Kamarainen J K,et al. Feature-based affine-invariant localization of faces.IEEE Transactions on Pat-tern Analysis and Machine Intelligence,2005,27:1490-1495.[J75] WISKOTT L,FELLOUS J M,KRUGER N,et al.Face recognition by elastic bunch graph matching.IEEE Trans on Pattern Analysis and Machine Intelligence,1997,19(7):775-779.[J76] Belhumeur P.N, Hespanha J.P, Kriegman D.J. Eigenfaces vs. fischerfaces: recognition using class specific linear projection.IEEE Trans on Pattern Analysis and Machine Intelligence,1997,15(7):711-720 [J77] MA L,KHORASANI K.Facial Expression Recognition Using ConstructiveFeedforward Neural Networks. IEEE Transactions on Systems, Man and Cybernetics, Part B,2007,34(3):1588-1595.[J78][J79][J80][J81][J82][J83][J84][J85][J86][J87][J88][J89][J90]。
大学的变化英语作文

In recent years,universities have undergone significant transformations,reflecting the evolving needs of society and advancements in technology.Here are some of the key changes that have shaped the modern university landscape:1.Technological Integration:The use of technology in classrooms has become ubiquitous. Lectures are often supplemented with online resources,and students can access course materials through digital platforms.Interactive tools and software have become integral to the learning process.2.Diversification of Learning Methods:Traditional lectures are no longer the only method of instruction.Universities now offer a variety of learning experiences,including seminars,workshops,and online courses.This diversity caters to different learning styles and preferences.3.Globalization:Universities have become more international,with an increase in the number of international students and faculty.This has led to a more diverse campus environment and a greater emphasis on global perspectives in teaching and research.4.Research Focus:There has been a shift towards researchintensive education. Universities are now not just places of learning but also hubs for innovation and research, often collaborating with industries and governments to address global challenges.5.Flexibility in Study:With the rise of online and distance learning,students can now pursue higher education at their own pace and from anywhere in the world.This flexibility has made education more accessible to a wider range of people.6.Emphasis on Employability:Universities are increasingly focusing on preparing students for the job market.This includes offering internships,career guidance,and courses that are aligned with industry needs.7.Sustainability:There is a growing awareness of the need for sustainable practices. Many universities are adopting green initiatives,such as reducing waste,conserving energy,and integrating environmental studies into their curriculum.8.Student Services:The range of services offered to students has expanded to include mental health support,career counseling,and extracurricular activities that promote personal development and wellbeing.9.Financial Models:The cost of higher education has risen,leading to debates about affordability and the value of a university degree.Some institutions are exploringalternative financial models,such as income share agreements.10.Campus Life:The social aspect of university life has also evolved,with a greater focus on community engagement,studentled initiatives,and the development of campus facilities that promote interaction and collaboration.These changes reflect the dynamic nature of higher education and its ability to adapt to the changing world.Universities are not just adapting to these changes but are also driving them,shaping the future of education and research.。
2014英语一text2翻译 -回复
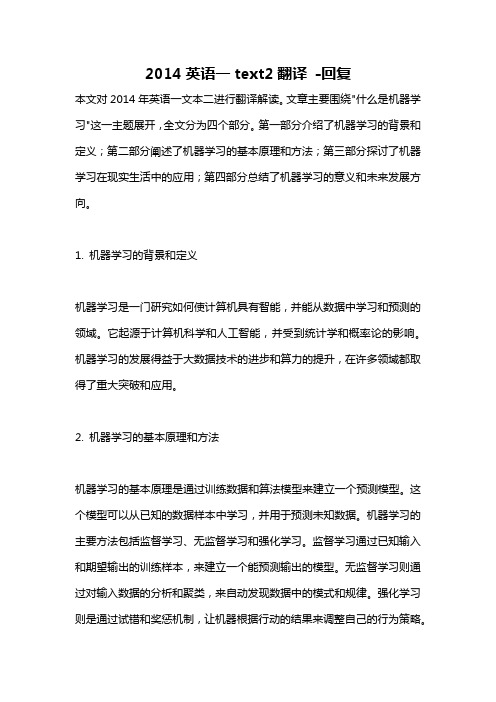
2014英语一text2翻译-回复本文对2014年英语一文本二进行翻译解读。
文章主要围绕"什么是机器学习"这一主题展开,全文分为四个部分。
第一部分介绍了机器学习的背景和定义;第二部分阐述了机器学习的基本原理和方法;第三部分探讨了机器学习在现实生活中的应用;第四部分总结了机器学习的意义和未来发展方向。
1. 机器学习的背景和定义机器学习是一门研究如何使计算机具有智能,并能从数据中学习和预测的领域。
它起源于计算机科学和人工智能,并受到统计学和概率论的影响。
机器学习的发展得益于大数据技术的进步和算力的提升,在许多领域都取得了重大突破和应用。
2. 机器学习的基本原理和方法机器学习的基本原理是通过训练数据和算法模型来建立一个预测模型。
这个模型可以从已知的数据样本中学习,并用于预测未知数据。
机器学习的主要方法包括监督学习、无监督学习和强化学习。
监督学习通过已知输入和期望输出的训练样本,来建立一个能预测输出的模型。
无监督学习则通过对输入数据的分析和聚类,来自动发现数据中的模式和规律。
强化学习则是通过试错和奖惩机制,让机器根据行动的结果来调整自己的行为策略。
3. 机器学习在现实生活中的应用机器学习在现实生活中的应用非常广泛。
在医疗领域,机器学习可以通过分析患者病历和医疗数据,来辅助医生进行疾病诊断和治疗方案的制定。
在金融领域,机器学习可以通过分析市场数据和交易模式,来预测股票和商品的价格走势。
在交通领域,机器学习可以通过分析交通流量和交通规则,来优化交通信号和路线规划,提高交通效率和安全性。
在社交网络和推荐系统中,机器学习可以通过分析用户的行为和兴趣,来推荐相关的信息和产品。
4. 机器学习的意义和未来发展方向机器学习的意义在于它能够使计算机具备自动学习和预测的能力,不再需要人工干预和编程。
这将极大地提高计算机在各个领域的智能和效率。
未来,机器学习还有许多发展方向,如深度学习、增强学习、迁移学习等。
信息时代理性面对作文
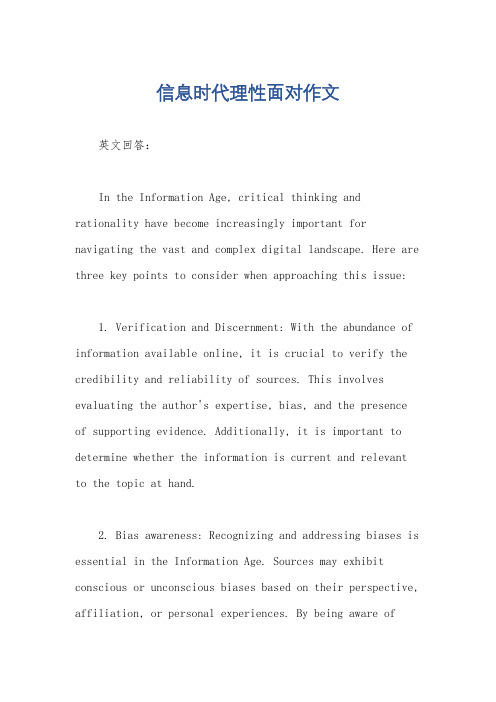
信息时代理性面对作文英文回答:In the Information Age, critical thinking andrationality have become increasingly important for navigating the vast and complex digital landscape. Here are three key points to consider when approaching this issue:1. Verification and Discernment: With the abundance of information available online, it is crucial to verify the credibility and reliability of sources. This involves evaluating the author's expertise, bias, and the presence of supporting evidence. Additionally, it is important to determine whether the information is current and relevant to the topic at hand.2. Bias awareness: Recognizing and addressing biases is essential in the Information Age. Sources may exhibit conscious or unconscious biases based on their perspective, affiliation, or personal experiences. By being aware ofthese potential biases, individuals can mitigate their influence and seek a more balanced and objective viewpoint.3. Logical reasoning: The ability to think critically and apply logical reasoning is fundamental for evaluating information. This involves identifying the claims, evidence, and arguments presented and assessing their validity and relevance. It also includes recognizing logical fallacies and common cognitive biases that can lead to erroneous conclusions.中文回答:信息时代理性面对。
大学应该禁止学生ai去完成作业吗英语作文

大学应该禁止学生ai去完成作业吗英语作文Title: Should Universities Ban Students from Using AI to Complete Assignments?In the rapidly evolving landscape of technology, artificial intelligence (AI) has become a ubiquitous presence in our daily lives. Its integration into various fields, including education, has sparked debates about its ethical and practical implications. One such debate centers on whether universities should prohibit students from utilizing AI to complete their assignments. This essay explores this question, weighing the arguments for and against such a ban.On the one hand, those advocating for a ban argue that allowing AI-assisted assignments undermines the fundamental purpose of education. The primary objective of academic institutions is to foster critical thinking, analytical skills, and a deep understanding of subject matter. By relying on AI to complete assignments, students may be bypassing the learning process, which involves grappling with complex ideas, making mistakes, and ultimatelyarriving at insights through their own efforts. AI'sability to rapidly generate answers and solutions can encourage a culture of laziness and superficial learning, stripping away the essence of academic rigor.Moreover, the use of AI in assignments raises concerns about plagiarism and authenticity. When students rely on AI to generate content, it becomes difficult to ascertain the level of their original thought and contribution. This blurring of authorship can lead to a dilution ofintellectual property and the integrity of academic work. Universities strive to maintain high standards of academic honesty, and the unchecked use of AI threatens to undermine these standards.However, those opposed to a ban argue that AI represents a powerful tool that can enhance learning rather than replace it. In the modern world, technologicalliteracy is increasingly vital. Understanding how to effectively use AI can be a valuable skill in itself, particularly in fields like computer science, data analytics, and engineering. By incorporating AI into thelearning process, students are better prepared to navigate the technological landscape of their future careers.Furthermore, AI can serve as a supplementary resource, aiding students in overcoming challenges and exploring new ideas. It can provide insights and perspectives that might not be immediately apparent to a student working alone. Used responsibly, AI can act as a catalyst for deeper understanding and innovation.Additionally, it is important to recognize that banning AI may not be a practical or enforceable solution. Technology is constantly evolving, and attempts to prohibit its use may be futile. Instead, universities should focus on educating students about the ethical and responsible use of AI. This includes discussing the limitations of AI, the importance of critical thinking, and the value of original work. By fostering a culture of informed decision-making, universities can help students navigate the complexities of using AI in their academic pursuits.In conclusion, the question of whether universities should ban students from using AI to complete assignments is a complex one. While there are valid concerns about thepotential negative impacts of AI on learning and academic integrity, it is also important to recognize the potential benefits of this technology. Instead of banning AI, universities should strive to strike a balance, encouraging responsible and informed use while fostering a learning environment that nurtures critical thinking andintellectual growth. This approach can help students harness the power of AI to enhance their learning experiences while maintaining the integrity and rigor of academic pursuits.。
2023年新高考英语重点词汇

2023年新高考英语重点词汇2023年新高考英语重点词汇一、人类社会与科技1. Artificial intelligence(人工智能)2. Virtual reality(虚拟现实)3. Quantum computing(量子计算)4. Automation(自动化)5. Biotechnology(生物技术)6. Robotics(机器人技术)7. Internet of things(物联网)随着科技的迅猛发展,这些词汇已经慢慢进入了我们生活的方方面面。
在新高考中,与科学技术领域相关的词汇将会被重点考查。
不仅仅是表面上的简单理解,而需要在学科知识的基础上深入掌握。
二、环境与气候1. Biodiversity(生物多样性)2. Climate change(气候变化)3. Carbon footprint(碳足迹)4. Renewable energy(可再生能源)5. Deforestation(森林砍伐)6. Greenhouse effect(温室效应)7. Acid rain(酸雨)环境污染和气候变化已经成为了全球共同面对的问题。
在英语考试中,环境与气候的话题同样是考察重点。
考生不仅需要熟悉相关词汇,还需要理解这些问题的背后涉及到的原理和措施。
三、政治与经济1. Globalization(全球化)2. Capitalism(资本主义)3. Democracy(民主主义)4. Socialism(社会主义)5. Populism(民粹主义)6. Protectionism(保护主义)7. Multilateralism(多边主义)政治与经济是英语考试中经常涉及的话题,新高考也不例外。
这些词汇与国家发展和国际关系息息相关,需要考生们在掌握词汇的基础上了解相关政策和实践。
四、文化与艺术1. Heritage(文化遗产)2. Literature(文学)3. Art(艺术)4. Film(电影)5. Music(音乐)6. Theater(戏剧)7. Painting(绘画)文化与艺术作为人类精神生活的重要组成部分,同样也是新高考英语考试的重点之一。
2018年英语四级作文第二套

2018年英语四级作文第二套英文回答:In the ever-evolving technological landscape, the emergence of artificial intelligence (AI) has sparked a transformative era, igniting both excitement and trepidation. As we navigate this uncharted territory, it is essential to delve into the profound implications of AI on our economy, society, and the very nature of humanity.The advent of AI presents unprecedented opportunities for economic growth. Automation and machine learning enable businesses to enhance efficiency, reduce costs, and develop innovative products and services. AI-driven technologies can optimize production processes, predict market trends, and provide personalized customer experiences. By leveraging AI's capabilities, industries can unlock new frontiers of innovation and create novel employment opportunities.However, AI also poses significant challenges to the workforce. As machines assume tasks previously performed by humans, concerns arise about job displacement and the widening gap between skilled and unskilled labor. Governments and educational institutions must proactively address these challenges by investing in reskilling and upskilling programs that prepare workers for the AI-driven economy.Beyond the economic realm, AI has profound societal implications. AI-powered algorithms influence decision-making processes, from loan approvals to criminal sentencing. The potential for bias and discrimination in these algorithms raises ethical concerns that demandcareful consideration. Additionally, AI's ability tocollect and analyze vast amounts of personal data raises questions about privacy and the preservation of individual autonomy. Striking a balance between the benefits of AI and the protection of human rights and values will require ongoing scrutiny and public discourse.Furthermore, AI raises philosophical questions aboutthe nature of humanity. As AI systems become more sophisticated, the boundaries between humans and machines blur. The pursuit of artificial general intelligence, orthe ability of machines to think and reason like humans, prompts us to confront the limits of our own cognitive abilities and the significance of consciousness.The transformative power of AI mandates responsible development and ethical deployment. Governments, businesses, and individuals must collaborate to ensure that AI is harnessed for the greater good. Establishing clearregulatory frameworks, promoting transparency and accountability, and involving diverse stakeholders in the decision-making process are crucial steps towards maximizing the benefits and mitigating the risks of AI.As we venture into the future, the relationship between humans and AI will continue to evolve. By embracing a multidisciplinary approach that combines technological advancement with ethical and social considerations, we can harness the potential of AI to build a society that is both prosperous and equitable.中文回答:随着人工智能(AI)的出现,一场变革性的时代已经到来,它既令人兴奋,又令人担忧。
南昌大学学术英语期末考试课文翻译
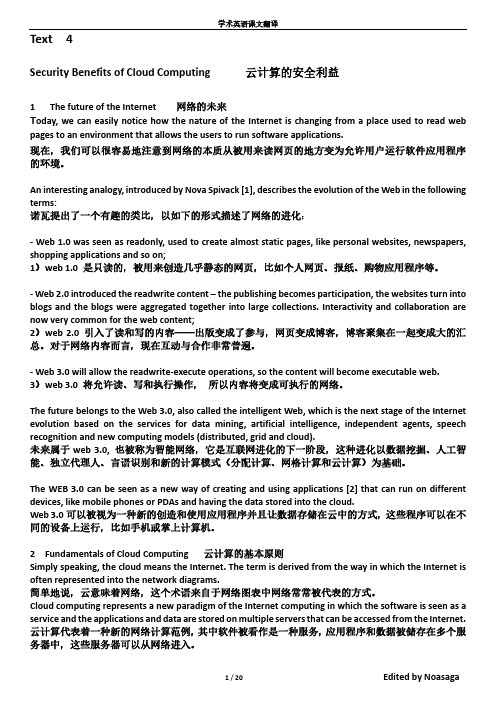
Text 4Security Benefits of Cloud Computing 云计算的安全利益1The future of the Internet 网络的未来T oday, we can easily notice how the nature of the Internet is changing from a place used to read web pages to an environment that allows the users to run software applications.现在,我们可以很容易地注意到网络的本质从被用来读网页的地方变为允许用户运行软件应用程序的环境。
An interesting analogy, introduced by Nova Spivack [1], describes the evolution of the Web in the following terms:诺瓦提出了一个有趣的类比,以如下的形式描述了网络的进化:- Web 1.0 was seen as readonly, used to create almost static pages, like personal websites, newspapers, shopping applications and so on;1)web 1.0 是只读的,被用来创造几乎静态的网页,比如个人网页、报纸、购物应用程序等。
- Web 2.0 introduced the readwrite content – the publishing becomes participation, the websites turn into blogs and the blogs were aggregated together into large collections. Interactivity and collaboration are now very common for the web content;2)web 2.0 引入了读和写的内容——出版变成了参与,网页变成博客,博客聚集在一起变成大的汇总。
人工智能对环境影响英语作文
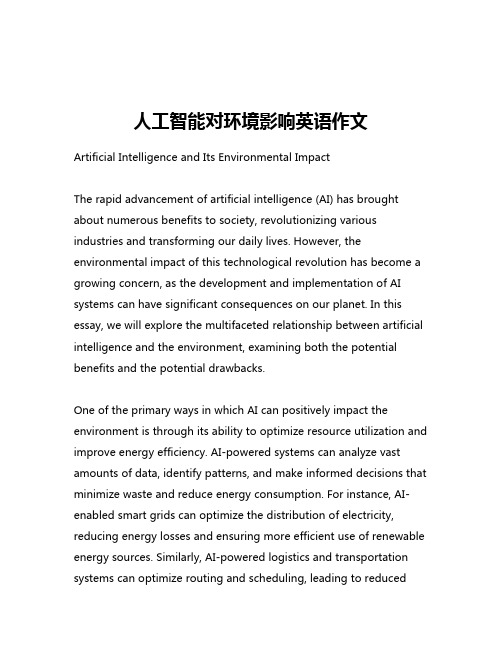
人工智能对环境影响英语作文Artificial Intelligence and Its Environmental ImpactThe rapid advancement of artificial intelligence (AI) has brought about numerous benefits to society, revolutionizing various industries and transforming our daily lives. However, the environmental impact of this technological revolution has become a growing concern, as the development and implementation of AI systems can have significant consequences on our planet. In this essay, we will explore the multifaceted relationship between artificial intelligence and the environment, examining both the potential benefits and the potential drawbacks.One of the primary ways in which AI can positively impact the environment is through its ability to optimize resource utilization and improve energy efficiency. AI-powered systems can analyze vast amounts of data, identify patterns, and make informed decisions that minimize waste and reduce energy consumption. For instance, AI-enabled smart grids can optimize the distribution of electricity, reducing energy losses and ensuring more efficient use of renewable energy sources. Similarly, AI-powered logistics and transportation systems can optimize routing and scheduling, leading to reducedfuel consumption and lower carbon emissions.Furthermore, AI can play a crucial role in environmental monitoring and conservation efforts. AI-powered sensors and satellite imagery can be used to detect and track environmental changes, such as deforestation, habitat loss, and the spread of invasive species. This information can then be used by policymakers and conservation organizations to implement targeted interventions and develop more effective strategies for protecting the environment. Additionally, AI-powered simulations and predictive models can help researchers and decision-makers better understand complex environmental systems and make more informed decisions.However, the environmental impact of AI is not limited to its potential benefits. The development and deployment of AI systems can also have significant negative consequences, particularly in terms of energy consumption and resource usage. The training and operation of AI models, especially those based on deep learning, can be highly energy-intensive, requiring vast amounts of computing power and generating significant greenhouse gas emissions. As the demand for AI-powered applications continues to grow, the energy footprint of these systems could become a significant contributor to global climate change.Moreover, the manufacture and disposal of the hardware requiredfor AI systems can also have a significant environmental impact. The extraction of raw materials, the production of electronic components, and the disposal of e-waste can all contribute to environmental degradation, pollution, and the depletion of natural resources. This issue is particularly pressing as the rapid pace of technological change often leads to the premature obsolescence of AI hardware, further exacerbating the problem of e-waste.To mitigate the environmental impact of artificial intelligence, a multifaceted approach is necessary. Researchers and developers must prioritize the development of energy-efficient AI systems, exploring ways to reduce the energy consumption of training and deployment processes. This may involve the use of more efficient hardware, the optimization of algorithms, and the incorporation of renewable energy sources into the infrastructure supporting AI systems.Additionally, the life cycle of AI hardware must be addressed, with a focus on sustainable design, responsible sourcing of materials, and the implementation of comprehensive recycling and disposal programs. Governments and policymakers can play a crucial role in this regard, by implementing regulations and incentives that encourage the development of environmentally-friendly AI technologies and the responsible management of AI-related waste.Furthermore, the integration of AI with other emerging technologies, such as renewable energy, smart city infrastructure, and sustainable agriculture, can amplify the positive environmental impact of artificial intelligence. By leveraging the power of AI to optimize these systems, we can unlock new opportunities for environmental conservation and sustainable development.In conclusion, the relationship between artificial intelligence and the environment is a complex and multifaceted one. While AI has the potential to significantly contribute to environmental protection and sustainability, its development and deployment must be carefully managed to mitigate the potential negative consequences. By prioritizing energy efficiency, responsible hardware management, and the strategic integration of AI with other sustainable technologies, we can harness the power of artificial intelligence to create a more environmentally-conscious future. As we continue to advance in the field of AI, it is crucial that we remain mindful of its environmental impact and work towards creating a harmonious balance between technological progress and environmental stewardship.。
Affective Computing英汉对照版

Affective ComputingR.W.PicardAbstractRecent neurological studies indicate that the role of emotion in human cognition is essential emotions are not a luxury. Instead, emotions play a critical role in rational decision-making, in perception, in human interaction, and in human intelligence. These facts, combined with abilities computers are acquiring in expressing and recognizing affect, open new areas for research. This paper defines key issues in “”affective computing,”computing that relates to arises from or deliberately influences emotions. New models are suggested for computer recognition of human emotion, and both theoretical and practical applications are described for learning, human computer interaction, perceptual information retrieval, creative arts and entertainment, human health,and machine intelligence. Significant potential advances in emotion and cognition theory hinge on the development of affective computing, especially in the form of wearable computers. This paper establishes challenges and future directions for this emerging field.摘要最近一项神经学研究表明情感在人类的认知中扮演着重要的角色,情感不是一个奢侈品。
Affective emotion expression and communication Survey

Mechanism-level models
• To emulate high-level or low-level aspects of the mechanism involved in emotional processing
- heart rate variability, brainwaves, respiration, muscle tension, blood pressure and temperature
• Input behaviors: emotional mouse • Gestures
Computational Models of Affect
• Appraisal: OCC model • Copping: expression
Expression types:
• Verbal: speech, text • Non-verbal: facial expression, motion, gesture, actions, etc.
Emotion Modeling and Expression Language
United Kingdom:
• U. of Cambridge, U. of Birmingham, U. of York, etc.
Others:
• Switzerland, Finland, Netherlands, Canada, Austria, etc.
Emotion Perception
英语作文未来的理想
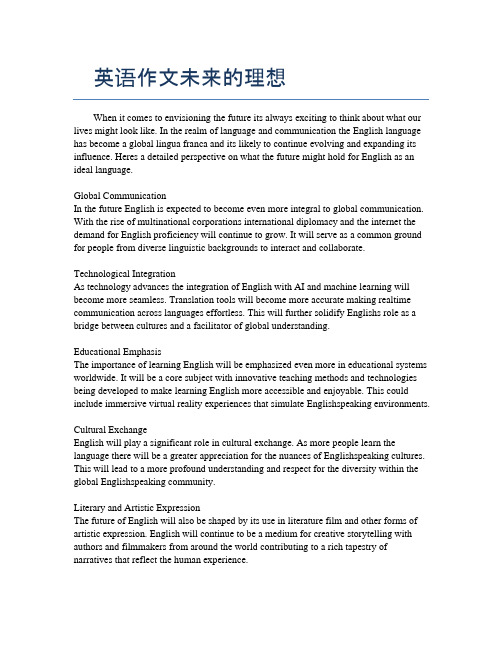
英语作文未来的理想When it comes to envisioning the future its always exciting to think about what our lives might look like. In the realm of language and communication the English language has become a global lingua franca and its likely to continue evolving and expanding its influence. Heres a detailed perspective on what the future might hold for English as an ideal language.Global CommunicationIn the future English is expected to become even more integral to global communication. With the rise of multinational corporations international diplomacy and the internet the demand for English proficiency will continue to grow. It will serve as a common ground for people from diverse linguistic backgrounds to interact and collaborate. Technological IntegrationAs technology advances the integration of English with AI and machine learning will become more seamless. Translation tools will become more accurate making realtime communication across languages effortless. This will further solidify Englishs role as a bridge between cultures and a facilitator of global understanding.Educational EmphasisThe importance of learning English will be emphasized even more in educational systems worldwide. It will be a core subject with innovative teaching methods and technologies being developed to make learning English more accessible and enjoyable. This could include immersive virtual reality experiences that simulate Englishspeaking environments. Cultural ExchangeEnglish will play a significant role in cultural exchange. As more people learn the language there will be a greater appreciation for the nuances of Englishspeaking cultures. This will lead to a more profound understanding and respect for the diversity within the global Englishspeaking community.Literary and Artistic ExpressionThe future of English will also be shaped by its use in literature film and other forms of artistic expression. English will continue to be a medium for creative storytelling with authors and filmmakers from around the world contributing to a rich tapestry of narratives that reflect the human experience.Language Variation and EvolutionWhile English will remain a unifying language it will also continue to evolve and adapt to local contexts giving rise to new dialects and forms of English. This will enrich the language and make it even more versatile and expressive.Inclusivity and AccessibilityEfforts will be made to make English more inclusive and accessible to people with different learning needs and abilities. This includes developing resources for English language learners with disabilities and creating platforms that cater to a wide range of learning styles.Environmental ConsiderationsAs the world becomes more environmentally conscious the future of English will also reflect this trend. There will be a focus on sustainable practices in language learning and teaching such as digital resources that reduce paper waste.Global CitizenshipLearning English will be seen as a pathway to becoming a global citizen. It will be a tool for understanding global issues participating in international dialogues and contributing to solutions that benefit the entire planet.In conclusion the future of English as an ideal language is bright and full of potential. It will continue to be a powerful tool for communication cultural exchange and global understanding adapting and evolving to meet the needs of an increasingly interconnected world.。
- 1、下载文档前请自行甄别文档内容的完整性,平台不提供额外的编辑、内容补充、找答案等附加服务。
- 2、"仅部分预览"的文档,不可在线预览部分如存在完整性等问题,可反馈申请退款(可完整预览的文档不适用该条件!)。
- 3、如文档侵犯您的权益,请联系客服反馈,我们会尽快为您处理(人工客服工作时间:9:00-18:30)。
Evaluating Affective Computing Environments UsingPhysiological MeasuresRegan Lee MandrykSimon Fraser UniversityBurnaby, BC, V5A 1S6rlmandry@cs.sfu.cawww.sfu.ca/~rlmandryABSTRACTEmerging technologies offer exciting new ways of using entertainment technology to create fantastic play experiences and foster interactions between players. Evaluating collaborative play technology is challenging because success isn’t defined in terms of productivity and performance, but in terms of enjoyment and interaction. Current subjective methods of evaluating entertainment technology aren’t sufficiently robust. Our research project aims to test the efficacy of physiological measures as evaluators of collaborative user experience with play technologies. We found evidence that there is a different physiological response in the body when playing against a computer versus playing against a friend. These physiological results are mirrored in the subjective reports provided by the participants. This research provides an initial step towards using physiological responses to objectively evaluate a user’s experience with collaborative play technology.INTRODUCTIONEmerging technologies in ubiquitous computing and ambient intelligence offer exciting new interface opportunities for co-located play technology, as evidenced in a recent growth in the number of conference workshops and research articles devoted to this topic [1, 2, 7]. Our research team is interested in employing these new technologies to foster interactions between users in co-located, collaborative play environments. We want technology not only to enable fun, compelling experiences, but also to enhance the interaction and communication between players. These goals are not the traditional goals of productivity enhancement, usually seen in HCI research. We are more concerned with the affective component of computing technologies [12], or generating an emotional response to a play environment. For example, we recently created two novel collaborative play environments [9, 10] with the goal of enhancing interaction between players and to create a compelling experience. Other researchers have used emerging technologies to create entertainment environments with the same goal in mind [1, 5, 7]. However, evaluating the success of these new interaction techniques and environments is an open research challenge. Traditionally, human-computer interaction research (HCI) has been rooted in the cognitive sciences of psychology and human factors, and in the applied sciences of engineering, and computer science [11]. Although the study of human cognition has made significant progress in the last decade, the notion of emotion is equally important to design [11], especially when the primary goals are to challenge and entertain the user. This approach presents a shift in focus from usability analysis to user experience analysis. Traditional objective measures used for productivity environments, such as time and accuracy, are not relevant to collaboration or play.ISSUES AND CHALLENGESThe first issue prohibiting good evaluation of collaborative play technologies is the inability to define what makes a system successful. We are not interested in traditional performance measures, but are more interested in whether our environment fosters interaction and communication between the players, creates an engaging experience, and is fun. A successful interaction technique should provide seamless access to the game environment and be a source of fun in itself. Although traditional usability issues may still be relevant, they are subordinate to the actual playing experience as defined by challenge, engagement, and fun.Once a definition of success has been determined, we need to resolve how to measure the chosen variables. Unlike performance measures, such as speed or accuracy, the measures of success for collaborative play technologies are more elusive. We want to increase interaction, enhance engagement, and create a fun experience. The current research problem lies in what metrics to use to measure engagement, interaction, fun, and collaboration.We have previously used both subjective reports and video coding as methods of evaluating our new technologies although there is no control environment with which to make comparisons [9, 10, 13]. Subjective reporting through questionnaires and interviews is generalizable and convenient, but misses complex patterns. Using video to code gestures, body language, and verbalizations is a rich source of data, but is also a lengthy and rigorous process.Research in Human Factors has used physiological measures as an indicator of mental effort and stress [14, 15]. Psychologists have been using physiological measures as unique identifiers of human emotions such as anger, grief, and sadness [4]. Physiological data have not been employed to identify human experience states ofenjoyment, fun, and interaction. My doctoral research focuses on using physiological data as objective indicators of challenge, fun, boredom, and engagement in electronic entertainment environments.In our research, we record users’ physiological, verbal and facial reactions to game technology, and apply post-processing techniques to correlate an individual’s physiological data with their subjective reported experience and events in the game. Our ultimate goal is to create a methodology for the objective evaluation of collaborative play technology, as rigorous as currentmethods for productivity systems.Figure 1: Quadrant display: screen capture of biometrics, video of player’s face, video of controller, andscreen capture of game.OUR RESULTSWe have conducted a number of experiments to further our research goal. In our first experiment, we manipulated the difficulty of a game environment, hoping to elicit varying levels of boredom, challenge, frustration, and fun. We analysed both the subjective results and the mean physiological results individually, and also correlated the two data types for each individual.Strong correlations between subjective ratings and the mean of many physiological measures were present in all players, but these correlations weren’t consistent across individuals. One problem was that the subjects enjoyed playing in all of the conditions, even if the difficulty level didn’t match their experience (fun median=3.0 for all conditions). The players also created challenges for themselves in the easier levels, changing the nature of the difficulty conditions, confounding the results.The main challenge with analyzing this experiment was relating single point data (subjective ratings) to time series data (physiology). To match these two types of data, we converted the time series data to a single point through averaging (e.g. mean) or integrating (e.g. HRV) the time series. Although this method has been used in other domains, it erases the variance within each condition. Game design employs variance and reward, thus this approach may not be appropriate. In the second experiment, to better understand how body responses can be used to create an objective evaluation methodology, we observed pairs of participants playing a computer game. Because this methodology is a novel approach to measure collaboration and engagement, and the results from Experiment One were ambiguous, we used an experimental manipulation designed to maximize the difference in the experience for the participant, so much that they would not be able to compensate with meta gaming activities. They played in two conditions: against another co-located player, and against the computer. We chose these conditions because we have previously observed pairs (and groups) of participants playing together under a variety of collaborative conditions [3, 6, 9, 13]. Our previous observations revealed that players seem to be more engaged with a game when another co-located player is involved.The results of the second experiment are described in full in a paper at CSCW 2004 [8]. To summarize, we found different mean physiological responses in the body and different subjective reports when playing against a friend versus playing against a computer. Participants found it significantly more fun, engaging, and exciting, and less boring to play against a friend than against a computer. In addition, mean galvanic skin response (GSR), and mean electromyography of the jaw (EMG) were significantly higher when playing against a friend.Although these results are an encouraging progression towards user experience analysis for collaborative play technologies, they have the same disadvantage as subjective results. They are single points of data representing an entire condition, however, unlike subjective reporting, they represent an objective measure of user experience. Used in concert, these two methods can provide a more detailed and accurate representation of the player’s experience.In order to correlate subjective and physiological responses, we needed to normalize the data. Physiological data has very large individual differences, thus individual baselines have to be taken into account. In order to perform a group analysis, we transformed both the physiological and subjective results into dimensionless numbers between zero and one. For each player, the difference between the conditions was divided by the span of that individual’s results. A correlation of the normalized differences would show that the amount by which subjects increased their subjective rating when playing against a friend is proportional to the amount that the physiological measure increased in that condition. We found that normalized GSR was correlated with normalized fun and inversely correlated with normalized frustration. We also found that normalized respiratory amplitude was correlated with normalized challenge.In addition to comparing and correlating the means from the two conditions, we investigated GSR responses for small windows of time surrounding game events. The raised mean GSR signals when playing against a friendreveal that players are more aroused when playing against a friend than when playing against a computer. However, we do not know whether this elevated result can be attributed to a higher tonic level or more phasic responses. One of the advantages of using physiological data to create evaluation metrics is that they provide high-resolution, continuous, contextual data. GSR is a highly responsive body signal, it provides a fast-response time-series, reactive to events in the game (see Figure 2).Figure 2: Example participant’s GSR response to scoring a goal against a friend and against the computertwice. Note the much larger response whenscoring against a friend. Data were windowed 10seconds prior to the goals and 15 seconds after. Using methods like the time-window analysis that we conducted, provides continuous objective data that can be used to evaluate the player experience, yielding salient information that can discriminate between experiences with greater resolution than averages alone. In this paper, we graphically represented continuous responses to different game events, and looked at the magnitude of the response using the span of the physiological measure. In our current work, we are taking advantage of the high-resolution, contextual nature of physiological data to provide an objective, continuous measure of player experience.CURRENT DIRECTIONSOur initial experiments were designed to correlate physiological data streams with a player’s subjective experience. Our current research aims to correlate the physiological data with the actual experience, determined objectively. To do this, we have annotated video data, collected while participants played NHL 2003™ against the computer, against a friend, and against a stranger. We coded not only for game events (e.g. goals for, goals against, hits given, and hits received), but also for interpersonal interactions (e.g. talk, laugh, trash talk).In parallel, we extracted certain mathematical features from the physiological time series (e.g. local maxima, local minima, saddle points). We then determined the relationship between the objective video annotation data and the physiological time series data. This approach completes the triangulation of data sources (subjective data relates to physiological data relates to video annotation data), but also provides an automatic continuous representation of user experience with play technologies. However, this approach is limited by examining each physiological signal (e.g. GSR, EMG) separately.In our future work, we plan to examine how the combined information provided by the signals can reveal unique emotional responses to play technologies. By using a fuzzy logic model, combined with our previous results, we hope to generate a strong tool for evaluating play technologies. This approach is strong as it is grounded in the data itself, yielding meaningful results. CONCLUSIONSThe evaluation of computing environments devoted to entertainment and play is ripe for advancement. Subjective data yield valuable quantitative and qualitative results. However, when used alone, they do not provide sufficient information. Physiological measures have previously been used to evaluate productivity systems, especially to reflect a user’s stress or mental effort. The application of physiological measurement and analysis to collaborative leisure technology has exciting potential. Although we do not currently understand how the body physically responds to enhanced interaction, or increased enjoyment, our research project aims to ultimately provide researchers with a methodology for objectively evaluating user experience with collaborative play technologies. We foresee that objective evaluation, combined with current subjective techniques will provide researchers with techniques as rigorous and valuable as current methods of evaluating user performance with productivity systems. In addition, our results can be used to create a powerful tool, used by designers and developers of entertainment technologies. REFERENCES[1] Björk, S., Falk, J., Hansson, R., and Ljungstrand,P. (2001). Pirates! Using the Physical World as aGame Board. In Proceedings of Interact 2001.Tokyo, Japan.[2] Björk, S., Holopainen, J., Ljungstrand, P., andMandryk, R.L. (2002). Introduction to SpecialIssue on Ubiquitous Games.Personal andUbiquitous Computing, 6: p. 358–361.[3] Danesh, A., Inkpen, K.M., Lau, F., Shu, K., andBooth, K.S. (2001). Geney: Designing acollaborative activity for the Palm handheldcomputer. In Proceedings of Conference onHuman Factors in Computing Systems(CHI2001). Seattle, WA, USA: ACM Press. p. 388-395.[4] Ekman, P., Levenson, R.W., and Friesen, W.V.(1983). Autonomic Nervous System ActivityDistinguishes among Emotions.Science,221(4616): p. 1208-1210.[5] Holmquist, L.E., Falk, J., and Wigström, J.(1999). Supporting Group Collaboration withInter-Personal Awareness Devices.Journal ofPersonal Technologies, 3(1-2).[6] Inkpen, K., Booth, K.S., Klawe, M., and Upitis,R. (1995). Playing Together Beats PlayingApart, Especially for Girls. In Proceedings ofComputer Supported Collaborative Learning(CSCL '95).[7] Magerkurth, C., Stenzel, R., and Prante, T.(2003). STARS - A Ubiquitous ComputingPlatform for Computer Augmented TabletopGames. In Proceedings of Video Track ofUbiquitous Computing (UBICOMP’03). Seattle,Washington, USA.[8] Mandryk, R.L. and Inkpen, K. (2004).Physiological Indicators for the Evaluation ofCo-located Collaborative Play. In Proceedings ofComputer Supported Cooperative Work(CSCW2004). Chicago, IL, USA.[9] Mandryk, R.L., Inkpen, K.M., Bilezikjian, M.,Klemmer, S.R., and Landay, J.A. (2001).Supporting Children's Collaboration AcrossHandheld Computers. In Conference Supplementto Conference on Human Factors in ComputingSystems(CHI 2001). Seattle, WA, USA. p. 255-256.[10] Mandryk, R.L., Maranan, D.S., and Inkpen,K.M. (2002). False Prophets: Exploring HybridBoard/Video Games. In Conference Supplementto Conference on Human Factors in ComputingSystems(CHI 2002). p. 640-641.[11] Norman, D.A. (2002). Emotion and Design:Attractive things work better. Interactions, 9 (4).[12] Picard, R.W., (1997). Affective Computing.Cambridge, MA: MIT Press.[13] Scott, S.D., Mandryk, R.L., and Inkpen, K.M.(2003). Understanding Children's CollaborativeInteractions in Shared Environments.Journal ofComputer Assisted Learning, 19(2): p. 220-228.[14] Vicente, K.J., Thornton, D.C., and Moray, N.(1987). Spectral Analysis of Sinus Arrhythmia:A Measure of Mental Effort.Human Factors,29(2): p. 171-182.[15] Wilson, G.M. (2001). PsychophysiologicalIndicators of the Impact of Media Quality onUsers. In Proceedings of CHI 2001 DoctoralConsortium. Seattle, WA, USA.: ACM Press. p.95-96.。