wangG11acta-Mean-field statistical simulation of grain coarsening in the presence of particles
利用加速度计数据在轨标定重力卫星质心偏差

第 63 卷第 2 期2024 年 3 月Vol.63 No.2Mar.2024中山大学学报(自然科学版)(中英文)ACTA SCIENTIARUM NATURALIUM UNIVERSITATIS SUNYATSENI利用加速度计数据在轨标定重力卫星质心偏差*刘超群1,谷德峰1,2,黄志勇3,王傲明1,刘道平21. 中山大学人工智能学院,广东珠海 5190822. “天琴计划”教育部重点实验室/天琴前沿科学中心/国家航天局引力波研究中心,广东珠海 5190823. 地理信息工程国家重点实验室,陕西西安 710054摘要:针对重力卫星质心偏差在轨标定的问题,提出了一种仅使用加速度计数据进行质心在轨标定的方法:以1A级加速度计数据作为输入,采用Butterworth滤波去除数据中的噪声,提取质心标定机动产生的线性加速度和角加速度信号,最后结合最小二乘原理对质心偏差进行标定。
利用GRACE-FO C星的加速度计数据对提出的质心标定方法进行验证,估计了C星发射至今的质心偏差。
结果显示,三轴的标定精度均优于10 μm,与利用姿态数据计算的质心偏差在三轴方向上的互对比差异(RMS)为[9.6,9.3,7.9]μm,与喷气推进实验室(JPL)公布结果的RMS为[7.4,3.8,4.7]μm。
与传统方法不同,由于仅使用了加速度计测量数据,该方法可用于卫星姿态缺失情况下的质心在轨标定。
关键词:GRACE-FO;重力卫星;加速度计;质心标定中图分类号:V19 文献标志码:A 文章编号:2097 - 0137(2024)02 - 0123 - 08Calibrating of the in-orbit center-of-mass offset of gravity satelliteby accelerometer dataLIU Chaoqun1, GU Defeng1,2, HUANG Zhiyong3, WANG Aoming1, LIU Daoping21. School of Artificial Intelligence, Sun Yat-sen University, Zhuhai 519082, China2. MOE Key Laboratory of TianQin Mission / Frontiers Science Center for TianQin /CNSA Research Center for Gravitational Waves,Sun Yat-sen University,Zhuhai 519082, China3. State Key Laboratory of Geo-Information Engineering, Xi'an 710054, ChinaAbstract:A method for calibration of the in-orbit center-of-mass offset only on accelerometer data is proposed in order to address the issue of calibration of the in-orbit center-of-mass offset of gravity satel‐lite. With ACC1A data as input, and the Butterworth filter is utilized to remove noise from the data. The linear acceleration and angular acceleration signals caused by the calibration maneuver of center-of-mass are extracted, and finally, the center-of-mass offset is calibrated using the least squares estima‐tion. The method for calibration of the in-orbit center-of-mass proposed in this paper is validated using the accelerometer data from the GRACE-FO C satellite, and the center-of-mass offset since the satel‐lite's launch is estimated. The results show that the calibration accuracy of all three axes is better than10 μm. The RMS differences in the comparison of the center-of-mass offset results in the three-axis di‐rection, obtained by using the proposed method and by calculating with attitude data, are respectivelyDOI:10.13471/ki.acta.snus.ZR20230018*收稿日期:2023 − 11 − 21 录用日期:2023 − 12 − 07 网络首发日期:2024 − 01 − 05基金项目:国家自然科学基金(41874028);中央高校基本科研业务费专项资金(23xkjc001)作者简介:刘超群(1998年生),女;研究方向:智能感知与信息处理;E-mail:*******************通信作者:谷德峰(1980年生),男;研究方向:GNSS精密定轨与定位、卫星试验评估与应用等;E-mail:******************第 63 卷中山大学学报(自然科学版)(中英文)[9.6,9.3,7.9]μm, and the RMS of comparison with the center-of-mass offset published by JPL are re‐spectively [7.4,3.8,4.7]μm. Different from the traditional method, because only accelerometer data is used, the proposed method can be used for calibration of the in-orbit center-of-mass in the case of da‐ta failure of gyroscope and star camera.Key words:GRACE-FO; gravity satellite; accelerometer; center-of-mass calibrationGRACE型重力卫星的星载加速度计用于测量卫星所受非保守力(Tapley et al.,2004; Flury et al.,2008; Christophe et al.,2015)。
独立成分分析的数学模型-四
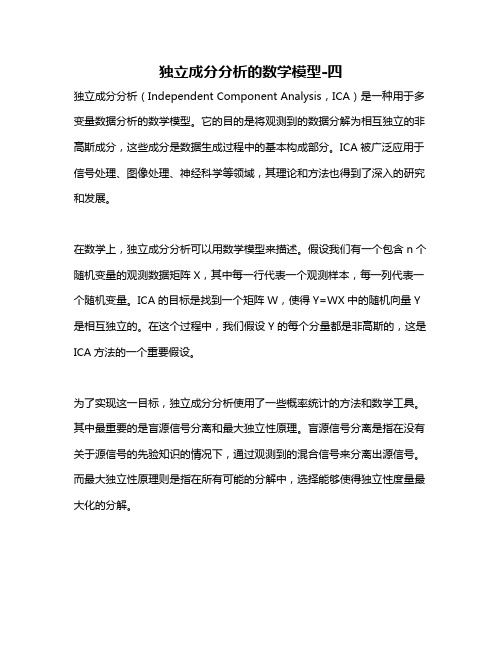
独立成分分析的数学模型-四独立成分分析(Independent Component Analysis,ICA)是一种用于多变量数据分析的数学模型。
它的目的是将观测到的数据分解为相互独立的非高斯成分,这些成分是数据生成过程中的基本构成部分。
ICA被广泛应用于信号处理、图像处理、神经科学等领域,其理论和方法也得到了深入的研究和发展。
在数学上,独立成分分析可以用数学模型来描述。
假设我们有一个包含n个随机变量的观测数据矩阵X,其中每一行代表一个观测样本,每一列代表一个随机变量。
ICA的目标是找到一个矩阵W,使得Y=WX中的随机向量Y 是相互独立的。
在这个过程中,我们假设Y的每个分量都是非高斯的,这是ICA方法的一个重要假设。
为了实现这一目标,独立成分分析使用了一些概率统计的方法和数学工具。
其中最重要的是盲源信号分离和最大独立性原理。
盲源信号分离是指在没有关于源信号的先验知识的情况下,通过观测到的混合信号来分离出源信号。
而最大独立性原理则是指在所有可能的分解中,选择能够使得独立性度量最大化的分解。
在数学上,独立成分分析可以用最大化独立性度量的方法来实现。
常用的独立性度量有信息熵、互信息、高阶统计量等。
通过最大化这些度量,可以得到最优的分解矩阵W,从而实现对观测数据的独立成分分析。
除了数学模型,独立成分分析还涉及到一些计算方法和算法。
其中最常用的是基于梯度下降的方法,通过迭代更新矩阵W的元素,使得独立性度量逐渐增大。
此外,还有一些基于信息熵、最小化互信息等原理的算法,它们都可以用来实现独立成分分析。
独立成分分析的数学模型和方法在实际应用中具有广泛的意义。
在信号处理领域,ICA可以用来从混合信号中分离出各个独立的信号成分,比如语音信号处理中的语音分离、图像处理中的盲源分离等。
在神经科学领域,ICA可以用来研究大脑活动的独立成分,从而更好地理解神经元的工作机制。
在金融领域,ICA可以用来分析金融时间序列数据,发现其中的独立成分,为金融风险管理和交易决策提供依据。
基于循环神经网络的欧亚中高纬夏季极端高温年代际预测模型研究

基于循环神经网络的欧亚中高纬夏季极端高温年代际预测模型研究作者:索朗多旦黄艳艳陈雨豪王会军来源:《大气科学学报》2024年第02期摘要近幾十年来频繁发生的极端高温事件严重威胁着自然生态系统、社会经济发展和人类生命安全。
针对生态环境脆弱的欧亚中高纬地区,首先评估了当前主流动力模式(CMIP6 DCPP)对于该地区夏季极端高温的年代际预测水平,并构建了基于循环神经网络(Recurrent Neural Networks,RNN)的年代际预测模型。
多模式集合平均(Multi-Model Ensemble,MME)的评估结果显示,得益于大样本和初始化的贡献,当前动力模式对于60°N以南区域(South Eurasia,SEA)展现了预测技巧,准确预测出了其线性增长趋势和1968—2008年间主要的年代际变率,然而模式对于60°N以北区域(North Eurasia,NEA)极端高温的年代际变率几乎没有任何预测技巧,仅预测出比观测低的线性增长趋势。
基于86个初始场的动力模式大样本预测结果,RNN将2008—2020年间NEA和SEA极端高温的年代际变率预测技巧显著提高,距平相关系数技巧从MME中的-0.61和-0.03,提升至0.86和0.83,均方差技巧评分从MME中的-1.10和-0.94,提升至0.37和0.52。
RNN的实时预测结果表明,在2021—2026年,SEA区域的极端高温将持续增加,2026年很可能发生突破历史极值的极端高温事件,NEA区域在2022年异常偏低,而后将呈现波动上升。
关键词极端高温;DCPP;年代际预测;循环神经网络在全球气候变暖的背景下,自20世纪50年代以来,全球大部分地区极端高温事件频率增加,持续时间延长(Alexander et al.,2006;孙建奇等,2011),呈现出明显的非线性增长,并且事件越极端,其发生频率的增长百分比越大(IPCC,2021)。
改进的DNLS图像分割算法
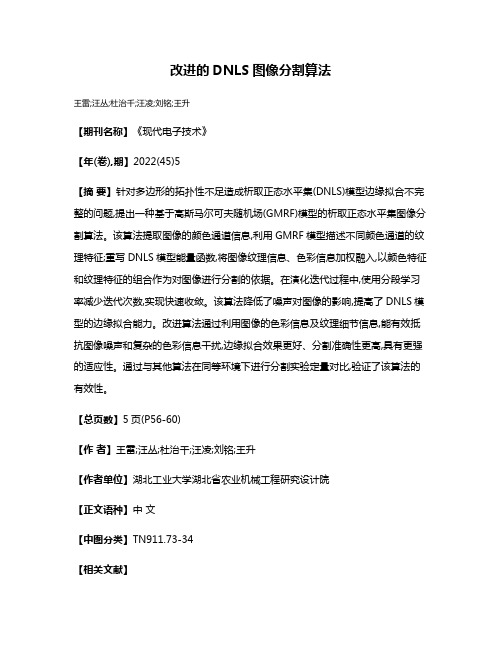
改进的DNLS图像分割算法
王雷;汪丛;杜治千;汪凌;刘铭;王升
【期刊名称】《现代电子技术》
【年(卷),期】2022(45)5
【摘要】针对多边形的拓扑性不足造成析取正态水平集(DNLS)模型边缘拟合不完整的问题,提出一种基于高斯马尔可夫随机场(GMRF)模型的析取正态水平集图像分割算法。
该算法提取图像的颜色通道信息,利用GMRF模型描述不同颜色通道的纹理特征;重写DNLS模型能量函数,将图像纹理信息、色彩信息加权融入,以颜色特征和纹理特征的组合作为对图像进行分割的依据。
在演化迭代过程中,使用分段学习率减少迭代次数,实现快速收敛。
该算法降低了噪声对图像的影响,提高了DNLS模型的边缘拟合能力。
改进算法通过利用图像的色彩信息及纹理细节信息,能有效抵抗图像噪声和复杂的色彩信息干扰,边缘拟合效果更好、分割准确性更高,具有更强的适应性。
通过与其他算法在同等环境下进行分割实验定量对比,验证了该算法的有效性。
【总页数】5页(P56-60)
【作者】王雷;汪丛;杜治千;汪凌;刘铭;王升
【作者单位】湖北工业大学湖北省农业机械工程研究设计院
【正文语种】中文
【中图分类】TN911.73-34
【相关文献】
1.基于小波图像融合算法和改进FCM聚类的MR脑部图像分割算法
2.三种图像分割算法的对比及图像分割方法的改进
3.基于改进遗传算法的otsu图像分割算法
4.基于改进分水岭算法的图像分割算法
5.改进乌鸦算法的二维Tsallis熵多阈值图像分割算法
因版权原因,仅展示原文概要,查看原文内容请购买。
直接使用CAD几何的蒙特卡罗粒子输运方法研究

摘要:传统的蒙特卡罗程序通常使用构造实体几何(CSG)的方法进行几何建模$然而对于某些复杂的
高阶曲面使用CSG进行精确建模非常困难且会耗费大量的时间$为解决这一问题,国际上提出了一种 直接使用CAD几何建模的蒙特卡罗(DAGMC)方法来进行粒子输运计பைடு நூலகம்$本文基于反应堆蒙特卡罗
本文基于堆用蒙特卡罗程序RMC⑺开发 使用DAGMC几何的DAG-RMC程序。通过 对方块组合体、燃料棒和犹他州茶壶3种模型 分别使用不同几何建模方法得到的临界计算结 果分析,验证DAG-RMC程序临界计算功能的 正确性。
1 RMC中DAGMC的实现
将RMC中原有的Cell类扩展为CSGCell 和DAGMCCell两类9 Surface类扩展为CSGSurface 和 DAGMCSurface 两类,并在 RMC 源 代码中调用DAGMC第3方库中的几何处理 函数。首先,利用DAGMC库函数将CAD几 何文件(h5m)所包含的栅元、曲面和材料信息 读取并转换为RMC的栅元、曲面和材料信息, 并把材料分配给对应的栅元,给各曲面定义对 应的边界条件。DAGMC几何的材料分配有两 种方式:一种是读取RMC输入文件的材料卡 信息;另一种是将材料信息嵌入到DAGMC文 件(h5m)中,使用UWUW模式读取材料信息$
然后,利用DAGMC库函数实现粒子定 位、计算粒子到边界距离、穿面后栅元判断等功 能,从而使RMC可基于CAD几何进行粒子输 运$添加DAGMC几何后的DAG-RMC程序
粒子输运模拟流程如图1所示,相关库函数的 作用列于表1$
图1 DAG-RMC粒子输运模拟流程
Fig. 1 DAG-RMC particle transport simulation process
基于机器学习的前列腺癌骨转移预测模型的建立及验证

基于机器学习的前列腺癌骨转移预测模型的建立及验证基于机器学习的前列腺癌骨转移预测模型的建立及验证前列腺癌是男性常见的恶性肿瘤之一,也是导致男性死亡的主要原因之一。
骨转移是前列腺癌的常见转移途径,且随着疾病进展,患者的预后也变得更加严峻。
因此,建立一个能够准确预测前列腺癌骨转移的模型对于早期干预和治疗具有重要意义。
本文旨在利用机器学习方法,建立一个可靠的前列腺癌骨转移预测模型,并对其进行有效验证。
首先,我们需要收集一定数量的前列腺癌患者的临床数据作为训练集,这些数据应包含患者的年龄、血清前列腺特异抗原(PSA)值、肿瘤分级、肿瘤大小、淋巴结转移情况等重要指标。
然后,利用这些数据,我们可以运用机器学习算法进行特征选择,以找出与前列腺癌骨转移相关的最重要特征。
常用的特征选择方法包括过滤法、包装法和嵌入法等。
接下来,通过使用机器学习算法如支持向量机(SVM)、随机森林(Random Forest)等进行模型训练和测试,以建立前列腺癌骨转移预测模型。
在模型训练过程中,我们需要将数据集划分为训练集和测试集,其中训练集用于模型的学习,测试集用于评估模型的性能。
模型的性能通常通过准确率、召回率、F1值等指标进行评估。
建立好模型后,我们需要对其进行验证,以验证其在未知样本上的预测能力。
为此,我们可以收集另一部分前列腺癌患者的数据作为验证集,在该验证集上测试模型的预测效果。
同时,我们可以与其他常见的前列腺癌骨转移预测方法进行比较,如传统的统计方法等。
通过比较验证结果,可以评估模型的准确性和稳定性。
在验证过程中,若模型的预测效果不佳,我们可以尝试调整机器学习算法的参数或者增加训练样本的数量,以提高模型的性能。
此外,还可以考虑引入更多的临床特征和生物标志物,以提高模型的预测能力。
总之,基于机器学习的前列腺癌骨转移预测模型的建立及验证是一个复杂而重要的过程。
通过充分利用机器学习算法和临床数据,我们可以建立一个准确预测前列腺癌骨转移的模型,并通过验证确保其在临床应用中的可靠性。
薛定谔—麦克斯韦尔方程径向解的存在性和多重性(英文)
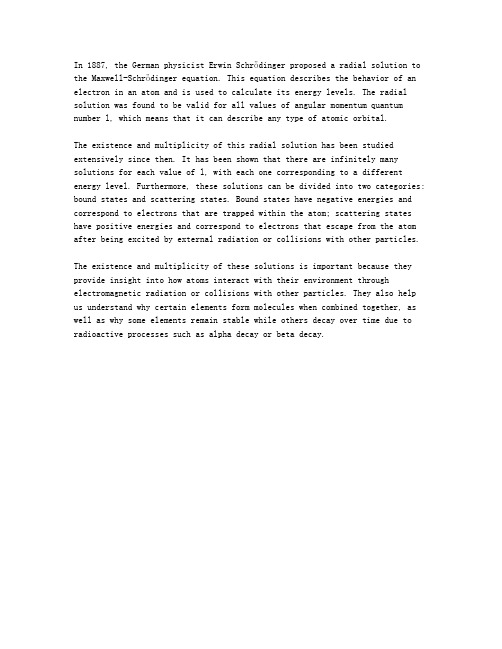
In 1887, the German physicist Erwin Schrödinger proposed a radial solution to the Maxwell-Schrödinger equation. This equation describes the behavior of an electron in an atom and is used to calculate its energy levels. The radial solution was found to be valid for all values of angular momentum quantum number l, which means that it can describe any type of atomic orbital.The existence and multiplicity of this radial solution has been studied extensively since then. It has been shown that there are infinitely many solutions for each value of l, with each one corresponding to a different energy level. Furthermore, these solutions can be divided into two categories: bound states and scattering states. Bound states have negative energies and correspond to electrons that are trapped within the atom; scattering states have positive energies and correspond to electrons that escape from the atom after being excited by external radiation or collisions with other particles.The existence and multiplicity of these solutions is important because they provide insight into how atoms interact with their environment through electromagnetic radiation or collisions with other particles. They also help us understand why certain elements form molecules when combined together, as well as why some elements remain stable while others decay over time due to radioactive processes such as alpha decay or beta decay.。
GDF11_通过AKT_通路抑制骨骼肌细胞分化

2023 ,43(2) : 092J.SHANXI AGRIC, UNIV . ( N atural Science Edition )学报(自然科学版)04185GDF11通过AKT 通路抑制骨骼肌细胞分化张鹏翔,吴佳豪,冀云燕,薛霖莉,董亚洁,宫泽恩,郝晓静,曹校瑞,赫晓燕*(山西农业大学 动物医学学院,山西 晋中 030801)摘要:[目的]探究骨骼肌GDF11(生长分化因子11,Growth and differentiation factor 11)在生长发育过程中的表达情况及其作用,明确GDF11在骨骼肌细胞中激活的非SMAD 调控通路,为抵抗骨骼肌衰老、促进骨骼肌损伤修复,探究肌肉发育调控的相关机制提供理论参考。
[方法]以不同发育阶段小鼠和C2C12细胞为材料,取不同发育阶段小鼠腓肠肌、使用分化培养基诱导细胞分化、使用D⁃半乳糖诱导C2C12细胞衰老,分别探究GDF11在个体层面和细胞层面骨骼肌生长发育和衰老中的表现和作用;通过构建GDF11的过表达载体和siRNA 载体,观察GDF11对C2C12细胞成肌分化的影响,并检测了在这一过程中细胞内相关基因的变化,探究GDF11在骨骼肌细胞发育中影响;通过对AKT 信号通路的抑制,明确GDF11在骨骼肌细胞分化过程中激活的非SMAD 调控通路。
[结果](1)在小鼠腓肠肌中,GDF11蛋白表达量随着年龄的增长呈现出先下降后上升的趋势。
(2)在细胞分化前期GDF11表达量逐渐升高,并在3 d 时细胞开始分化时达到最高,随后逐渐降低,7 d 时恢复到与初始相似的水平。
(3)随着D⁃半乳糖浓度的增加,细胞衰老程度逐渐增加,同时细胞GDF11表达量逐渐增加。
(4)过表达GDF11使细胞分化进程推迟,但抑制GDF11的表达对细胞分化进程并没有影响。
过表达GDF11上调了AKT 的磷酸化水平。
(5)过表达GDF11的C2C12细胞中阻断AKT 通路能够逆转GDF11造成的分化抑制。
数据通信原理实验指导书
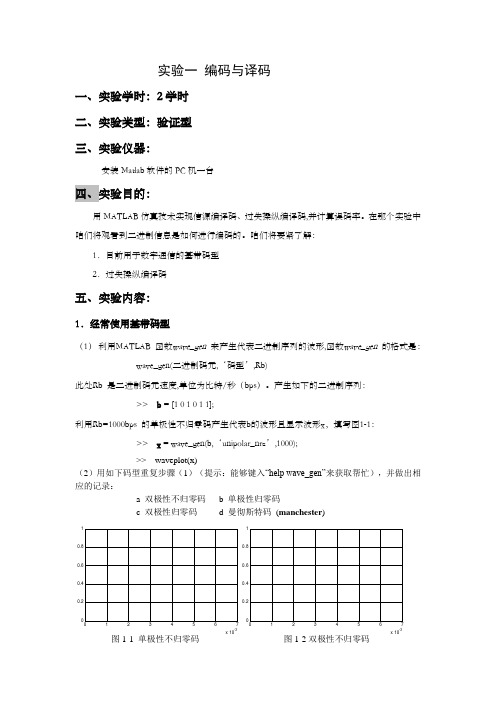
实验一编码与译码一、实验学时:2学时二、实验类型:验证型三、实验仪器:安装Matlab软件的PC机一台四、实验目的:用MATLAB仿真技术实现信源编译码、过失操纵编译码,并计算误码率。
在那个实验中咱们将观看到二进制信息是如何进行编码的。
咱们将要紧了解:1.目前用于数字通信的基带码型2.过失操纵编译码五、实验内容:1.经常使用基带码型(1)利用MATLAB 函数wave_gen 来产生代表二进制序列的波形,函数wave_gen 的格式是:wave_gen(二进制码元,‘码型’,Rb)此处Rb 是二进制码元速度,单位为比特/秒(bps)。
产生如下的二进制序列:>> b = [1 0 1 0 1 1];利用Rb=1000bps 的单极性不归零码产生代表b的波形且显示波形x,填写图1-1:>> x = wave_gen(b,‘unipolar_nrz’,1000);>> waveplot(x)(2)用如下码型重复步骤(1)(提示:能够键入“help wave_gen”来获取帮忙),并做出相应的记录:a 双极性不归零码b 单极性归零码c 双极性归零码d 曼彻斯特码(manchester)x 10-3x 10-3图1-1 单极性不归零码图1-2双极性不归零码x 10-3x 10-32.过失操纵编译码(1) 利用MATLAB 函数encode 来对二进制序列进行过失操纵编码, 函数encode 的格式是:A .code = encode(msg,n,k,'linear/fmt',genmat)B .code = encode(msg,n,k,'cyclic/fmt',genpoly)C .code = encode(msg,n,k,'hamming/fmt',prim_poly)其中A .用于产生线性分组码,B .用于产生循环码,C .用于产生hamming 码,msg 为待编码二进制序列,n 为码字长度,k 为分组msg 长度,genmat 为生成矩阵,维数为k*n ,genpoly 为生成多项式,缺省情形下为cyclpoly(n,k)。
一种稀疏度自适应广义正交匹配追踪算法

一种稀疏度自适应广义正交匹配追踪算法姚万业;姚吉行【摘要】针对压缩感知(Compressive Sensing,CS)在信号重构时稀疏度往往未知,导致过估计及重构误差变大、复杂度高等问题,通过分析重构残差与支撑集原子数目之间的变化关系,提出一种稀疏度自适应广义正交匹配追踪算法,该算法无需原始信号稀疏度的先验知识.首先,算法采用分阶段变步长的方式扩充支撑集原子数,然后在迭代后期重构残差变化缓慢时改变原子搜索策略精确估计稀疏度,完成原始信号的重构.仿真实验将完成重构所需迭代的次数作为算法复杂度衡量标准,将重构精度、准确重构率及重构运行时间作为评判算法性能的指标.结果表明,该算法重构概率远高于传统的OMP、gOMP算法,重构图像视觉效果更佳,且运算时间低于同类盲稀疏度算法.【期刊名称】《仪器仪表用户》【年(卷),期】2018(025)008【总页数】5页(P16-20)【关键词】压缩感知;信号重构;稀疏表示;自适应;广义正交匹配【作者】姚万业;姚吉行【作者单位】华北电力大学控制与计算机工程学院,河北保定071003;华北电力大学控制与计算机工程学院,河北保定071003【正文语种】中文【中图分类】TN911.230 引言压缩感知(Compressive Sensing, CS)是Donoho、Candes及Tao[1-3]等基于稀疏信号和逼近理论提出的一种新的信息获取方法。
对于具有稀疏性或在其变换域内是稀疏的信号,可通过低维空间、欠Nyquist采样数据的非自适应测量来完成对高维原始信号的重构,大大提高了信号的获取效率,便于数据的存储及传输。
CS理论一经提出就备受关注,并逐渐在图像处理[4],核磁共振[5]等工程领域得到广泛应用。
作为CS理论的核心之一,原始信号的重构一直是近些年的研究重点。
目前基于CS理论的重建算法主要分成3类[6]:凸优化算法,贪婪匹配追踪算法和组合算法。
其中由于贪婪算法复杂度低,重构用时短而备受关注[7]。
基于组合凸线性感知器的文本分类模型

第43卷第11期 2017年11月北京工业大学学报JOURNAL OF BEIJING UNIVERSITY OF TECHNOLOGYVol.43 No.11Nov. 2017基于组合凸线性感知器的文本分类模型李玉鑑,王曼丽,刘兆英(北京工业大学信息学部,北京100124)摘要:针对文本分类问题,从分片线性学习的角度出发,提出了一种文本分类的组合凸线性感知器模型.首先,对文本样本集进行预处理,包括特征选择、特征项赋权等;然后,分别利用生长支持组合凸线性感知器算法(growing support multiconlitron algorithm,GSM A)和支持组合凸线性感知器算法(support multiconlitron algorithm,SM A)构造组 合凸线性感知器,对样本集进行分类.该模型基于支持向量机的最大间隔思想,通过集成线性分类器,实现了对2类数据的划分,具有计算简单、适应能力强的优点.在标准文本数据集上的实验结果表明:该模型所构造的分类器 具有良好的文本分类性能,与其他典型文本分类方法的对比也说明了该方法的有效性.关键词:分片线性分类器;组合凸线性感知器;文本分类中图分类号:TP181 文献标志码: A 文章编号:0254 -0037(2017)11 -1681 -09d o i:10.11936/bjutxb2016070005Text Classification Model Based on MulticonlitronLI Y ujian,WANG M anli,LIU Zhaoying(Faculty of Information Technology,Beijing University of Technology,Beijing 100124,China)A b s tra c t:To deal with the problem of text classification,a text categorization method was proposed based on multiconlitron from the perspective of piecewise learning.First,text sample preprocessing including feature selection and feature weighting was performed.Then,the multiconlitron was constructed by using growing support multiconlitron algorithm (GSM A)and support multiconlitron algorithm (SM A) respectively for text classification.Inspired by the idea of maximum interval of support vector machine,the classification of two kinds of data by integrating the linear classifier was achieved by this model,which had the advantages of small computation cost and strong adaptive ability.Experiments on standard text data sets show that the proposed method has a good performance on text classification and the comparison results with some other typical text classification methods also verifies the effectiveness of the proposed method.K ey w o rd s:piecewise linear classifier;multiconlitron;text classification文本分类是指在给定分类体系下,使用计算机 自动地标记文本类别的过程.它在信息检索、数据 挖掘和舆情分析等领域中具有重要应用,已经成为 现代信息处理的研究热点之一.其中涉及文本表示、特征选择、分类模型和评价方法等关键技术[1].目前,比较成熟的文本分类器有K-最近邻(K-nearest neighbor,KNN)、朴素贝叶斯(naive Bayes,NB)、支 持向量机(support vector machine,SVM)、神经网收稿日期:2016-07-12基金项目:国家自然科学基金资助项目(61175004);髙等学校博士学科点专项科研基金资助项目(20121103110029);北京市 自然科学基金资助项目(4112009)作者简介:李玉鑑(1968—),男,教授,主要从事模式识别、图像处理、机器学习、数据挖掘方面的研究,E-mail:liyujian@bjut.1682北京工业大学学报2017 年络[2]等.K N N性能较稳定,但时空效率较低;N B分类速度最快,但分类精度最低;神经网络能充分逼近复杂的非线性关系,但需要大量的参数,学习时间过长;S V M分类精度最高,在解决小样本、非线性及高维模式识别中表现出许多特有的优势[3],已经成为文本分类领域的研究热点.支持向量机的基本思想是假定2类数据线性可分,通过寻找最大间隔分类超平面,使其能够正确地划分2类数据.现有的支持向量机又可分为线性支 持向量机和非线性支持向量机.前者用于解决线性 可分的情况,但是只能产生线性分类面,不足以处理比较复杂的线性不可分样本的分类问题.后者使用 核技巧可以解决非线性可分情况,但是核函数的选 择缺乏指导,并且计算资源需求较大,不利于求解大规模数据.分片线性分类器(piecew ise linear c la ssifie r,P L C)是一种特殊的非线性分类器.它可以直接在 原输入空间构造,分开任意复杂的2类数据,避免核函数的选取困难.同时,由于分类器的决策面是由 若干个超平面构成的,与一般超曲面相比,不仅简单,易于实现,计算量小,又能逼近各种形状的超曲 面,具有很强的适应能力[4].目前,分片线性分类器已经引起人们广泛的关 注,许多设计分片线性分类器的方法被提出,如线性规划方法[5]、局部训练方法[6]、决策树方法[7],甚至经典的K N N算法也可看作一种简单的分片线性分 类器.然而,绝大多数分片线性分类器是基于经验 并富有试探性的,缺乏统一的理论框架,而且对数据的空间分布结构依赖性较大,训练时间很长,分类器性能较差,甚至一些方法需要预先指定超平面的数 量,描述线性函数如何组织,这些缺点限制了其在文本分类领域的应用.针对上述情况,本文从分片线性学习的角度出 发,提出了文本分类的组合凸线性感知器模型.组 合凸线性感知器[8]是L i等在凸可分、叠可分和凸线性感知器的概念基础上提出的构造分片线性分类器的一个通用理论框架.该框架吸收了S V M的优点,并且采用了S V M的最大间隔思想,但无需使用核函数,避免了核函数的选取困难.它是线性支持向量 机和非线性支持向量机的一种折中处理,所构造的分片线性分类器性能一般介于二者之间.为将组合凸线性感知器(m ultipl e convex linear perceptrG n,m u ltico n litro n)应用于文本分类领域,研究并评测其性能,本文首先将文本用向量的形式表示,然后用主成分分析方法对文本向量进行降维,其次构造组合凸线性感知器,完成分类过程,最后评测组合凸线性感知器在文本分类领域的性能.为叙述简洁,在下文中,将凸线性感知器(con vex linear perceptron,conlitron)简称为凸线器,组合凸线性感 知器简称为组合凸线器.1组合凸线器的基本概念组合凸线器框架是以凸可分、凸线器等概念为基础的.因此,本节将从数据集凸包和可分性的关 系出发,详细阐述凸线器、组合凸线器等核心概念,下面首先给出凸包的定义[8]:定义1对于上的任意一个有限集X,它的凸包定义为CH(X) = {x x=移琢i,移琢i沂X,琢i逸0 }1臆i臆I X I1臆i臆1X17下面给出3个可分性定义.定义2 线性可分(lin early separable):对于2个有限集X,Y哿R n,如果X、Y的凸包不相交,即CH(X)nCH(Y) =0,则称它们是线性可分的.定义3 凸可分(con vexly separable):对于2个有限集X,Y哿R n,如果V j沂Y,j埸CH(X),则称X 相对Y是凸可分的.如果X相对Y是凸可分的,或者Y相对X是凸可分的,则称X与Y是凸可分的.定义 4 叠可分(co m m o n ly separable):对于2个有限集X,Y哿,如果X n Y= 0,即二者之间无重合样本,则称它们是叠可分的.图1分别给出了3种可分情况的示例.图1数据集的可分性说明Fig. 1Separability description of datasets线性判别函数可表示为f(x) =^^+ 6,并且对于正类的任意一个样本,f(x) >0;对于负类的任意 一个样本,f(x) <0;特别地,f(x) =0为一个超 平面.凸线性感知器是指一组线性函数的集合,它可以正确分开2类凸可分数据集,当训练方为X到Y第11期李玉鑑,等:基于组合凸线性感知器的文本分类模型1683时,可表示为C LP= \fi(x)=w^x+b l,(w l,b l)e E"x R,1臆/臆L}满足以下条件:|V x e X,V1臆l臆L,fl(x) = w t'x+b l逸0\y y e Y,31臆l臆L,fi(y) =w t-y+b t<0 ^)决策函数定义为+ 1,V1臆l臆L,f l(x)>0CLP(x) = {-1,埚1臆l臆L,f l(x)<0(2)其中L是指线性函数的个数.凸线器具有2个特点:第一是局部分类间隔最大特性,这是由于凸线器的每一个片段对应硬间隔SVMs.第二是方向性,当X对Y是凸可分时,可以构造从X到Y的凸线器,反之,当Y对X是凸可分时,可以得到从Y到X的凸线器,只是这2个凸线器的结构不同.组合凸线性感知器是指一组凸线器的集合,它可以将2类叠可分数据集正确分开,可表示为M C L P= \CLP k,1臆k臆K}满足以下条件:V x e X,埚1 臆k臆K,CLPk(x) = +1(V.y e Y,V1臆k臆K,CLPk(y) = -1决策函数定义为+ 1,埚1臆k臆K,CLPk(x)= MCLP(x) = {-1,V1臆k臆K,CLPk(x)=+1-1(4)式中K是指线性函数的个数.组合凸线器也具有局 部最大间隔特性和方向性2个特点.从不同方向构 造的2个组合凸线器具有不同的结构,并以包含更 少线性函数的组合凸线器为最终的分类模型.凸线 器和组合凸线器的示例见图2.2组合凸线器的构造方法组合凸线器的构造方法包括基本构造方法和生 长构造方法.其中,前者提出了构造3类分类器的 相应算法,后者则进一'步提高了分类精度.2.1基本构造方法对于线性可分的2类样本X和Y,其分类超平 面可以等价为求解它们凸包之间的最近点对问题,也就是求解其硬间隔SV M,优化目标具体可表示为[9]min 椰x- J 椰s.t.x e C H(X),j e CH(Y)(5)如果(x*,,)是经式(5)得到的最近点对,则 Y和Y的分类超平面可表示为(x*,,)的垂直平 分面f(x) = w**x+ b*(6)式中:w*=x* -J*;b* =( II,II2- ||x*||2)/2.交叉距离最小化算法(cross distance minimization algorithm,⑶M A)算法是以式(1)为优化目标的典 型凸包间最近点求解算法,图3给出了 C D M A中找 最近点对x*、_y*的几何释义.如果x:e C H(X)不 是C H(Y)到C H(X)的最近点,那么必然存在点 z*e C H(X),使距离差值小于精度参数,即以尤1- 力)-d(x** )〈着成立.若姿逸1,则z*=x2;若0 <姿 <1,则z*=x: + A(x2 - x j,且z*是_y*到线段C H k,x2}的垂点.图3 CDMA的几何解释Fig. 3 Geometric interpretation of CDMACDM A(X,Y,着)表示以2个有限集X、Y,精度着 为输入,得到的X和Y的硬间隔支持向量机f(x)= w**x+ b*.对于x,y哿『,如果X相对y是凸可分的,可 使用支持凸线性感知器算法[8] (support conlitron algorithm,S C A)构造一个方向从X到Y的凸线器将 X和Y正确分开.如图4所示,S C A算法首先从集 合Y选择一个距离CH(X)最近的点',利用⑶MA 算法构造一个线性函数f1(x) =CD M A(X,\心},着),作为凸线器的第1个组件,切掉数据集Y所有 满足乂(^)臆f j y j的点后,再从新的Y集合中,找 到距离C H(X)另一个最近点',再计算得到第2个 线性函数f2(x) = CDM A(X,\'},着),切掉Y中满 足f2(W臆f2(')的点,不断重复此过程直至Y= 0.最终得到一个X相对Y的凸线器.当精度参数1684北京工业大学学报2017 年为着时,可表示为S C A(X,Y,着).对于叠可分的2类样本X和中的每一个点^相对Y都是凸可分的,可使用支持组合凸线性 感知器算法(support multiconlitron algorithm,SM A)构造一个组合凸线器将X、Y正确分开.如图 5所示,S M A算法首先从集合X中选择距离Y最近 的一个点x p,构造第1个支持凸线器CLPi = SCA (U P},Y,着),它切掉了 X中满足条件C L P j x)= + 1的点,剩余的点仍记为X.然后,再从X中找到 距离Y的另一个最近点 ',计算得到第2个支持凸 线器CLP2 = S C A({X q},Y,着),又切掉了 X中满足 条件CLP1 (x)= + 1的点.重复此过程,直到 X= 0.最终,得到由若干个凸线器组成的一个组合凸 线器MCLP.当算法精度参数为着时,该组合凸线器 可表示为SM A(X,Y,着).2.2生长构造方法组合凸线器的生长构造方法是构造组合凸线器 的一种新方法,能够有效提高分类精度并简化分类 模型.该方法由挤压(SQUEEZE )和膨胀(INFLATE)两个基本操作组成,对于凸可分情况,使用挤压操作将初始训练得到的分类边界推向内部 凸区域,使其能够更好地拟合数据分布;对于叠可分 情况,使用膨胀操作调整初始训练得到的分类边界,使其移动到更加合理的位置.假设由S C A训练得到初始凸线器CLP={f;(x)= wr x+b,1臆1臆L},将集合Y分成L个相互重叠的 子集,即Y= U Y I,赘={ Yt,1臆/臆L}(7)式中,Yi = {少丨/心)<0,J沂Y},e CL P,是指Y中所有能够被f l(x)正确分类的样本点集合.显然,存 在i屹j使得I和Y.重叠,即Y i疑Y.屹0.如图6所示,挤压操作每次均从Y中选择包含 样本数量最多的子集Yp,通过CD M A训练得到线性 函数g i(x),切掉赘中所有能够被它正确分类的子 集,重复此过程,直到赘=0.描述如下:操作1:挤压操作SQUEEZE(C L P,X,Y,着)输入:初始凸线器CLP= /i(x),1臆1臆L}X= {X i,1臆i臆N},Y= {y.,1臆/臆M},精度参数着1-Yi = {J丨/(y) <0,y e Y},/i e CLP2. /饮1,赘饮{Y i,1臆i臆丨C L P丨=L}3.p= arg maxi j 丨Y i丨,Y i沂赘I4. g i(x)=⑶M A(X,Yp,着)5. 赘={ Yi丨Yi沂赘-{ Yp|,B j e Y i,g i(.y) >0}6.如果鬃屹0,那么k饮一k+ 1,并转到步骤3返回:C L P= {^(尤),1臆i臆图6挤压操作的几何解释Fig. 6Geometric interpretation of SQUEEZE采用挤压操作进行凸线器生长的算法称为生长 支持凸线性感知器算法[10](grow ing support co n litro n algorithm,GSCA).它能够产生更加合理的分类边 界,有效减少凸线器中线性函数数量,一定程度上防 止了过拟合情况的发生.GSCA中挤压次数可以设 定为多次,GSCA(Xp,Y,着,T)表示输入为2个有限 集X、Y和精度参数着构造的X相对Y挤压T次的 凸线器CLP.由于往往初次挤压能够有效调整分类 边界,本文建议进行1次挤压即可.假设由SMA训练得到的初始组合凸线器为MCLP= {CLP k(x),1臆k臆K},此组合凸线器包含K 个凸线器,将集合X分成K个相互重叠的子集,即X=U X k,鬃={ X k,1臆k臆K I(8)第11期李玉鑑,等:基于组合凸线性感知器的文本分类模型1685式中:U l C L P k(x) = +1,x e X},为X 中所有 能够被CLPk(x)正确分类的样本点集合,C L P k沂M CLP;鬃为被不同凸线器划分的X中点子集的集 合.显然,存在i屹/使得足和X.是重叠的,具体示例 如图7(a)所示.膨胀操作每次均从鬃中选择包含样本数量最 多的子集,在该子集与Y间通过G S C A训练得到凸 线器,切掉鬃所有能够被它正确分类的子集,重复 此过程,直至鬃=0.操作如下:操作 2:膨胀操作 INFLATE(M CLP,X,Y,着,T)输入:初始组合凸线器M C L P= {CLPk,l臆k臆K},X= j x;,1臆i臆N},Y= {'.,1臆j臆M},精度参数着,挤压次数T1.X k= {x lCLPk(x)= +1,x e X},CLPk e MCLP2.k饮1,鬃饮{Xi,1臆i臆IMCLPI = K}3. p= arg maxi{1X i1,X i沂鬃}4.CLPk=GSCA(Xp,Y,着,T)5. 鬃={ Xi I Xi 沂鬃-{ Xp},B x 沂X i,CLPk(x)= -1}6.如果鬃屹0,那么k饮一k+ 1,并转到步骤3返回:M C LP= {CLP;,1臆i臆k}如图7(b)所示,经过膨胀后原2个凸线器合并 成1个,且整体分类边界向外扩张.显然,膨胀操作 也可进行多次,并且当调用GSCA算法时,如果其中 挤压次数设置不为0,则意味着膨胀中包含挤压,此 时膨胀不仅单纯地从内部向外扩张,还会受到外部 挤压的作用,将分类边界最终定位于2个作用相对 均衡的位置.生长支持组合凸线性感知器算法[10] (gro w in g support m u lticon litron algorithm,G SM A)采用膨胀操作,实现了组合凸线器的生长,能够得到更 加合理的分类边界,并且简化凸线器的数量,在一定 程度上提升分类器的泛化能力.3文本预处理为了将组合凸线器应用于文本分类中,首先需 要用空间向量模型(vector space m odel,VSM)对文 本进行统一表示,具体过程涉及文本的特征提取和 特征赋权,然后进行组合凸线器的构造与应用.本 节主要介绍文本预处理过程,具体包括特征提取、特 征项赋权方法及主成分分析(principal co m p o n en t analysis,PCA)降维.3.1特征提取特征提取是指从预处理后的文本特征集合中,选择能够代表文本主要特征的特征子集的过程.这 些经过选择得到的特征子集,往往能够很好地区分 文本的类别,同时也能较好地突出本类别的特性. 目前,常用的特征提取方法有基于文本频率DF、基 于卡方检测(chi-square test,CHI)统计、信息增益 等.本节采用的是基于CHI统计的的文本特征提取 方法.C H I统计首先通过假设特征词t和文本类别^ 之间符合一维自由度的/分布,获取特征项t对于 ^的C H I值,然后将C H I值从大到小排序,选取前n 个特征项构成最终的特征子集合.其中,t对于^的C H I值计算公式为X2( t,C i)N x(AD-B C)2(9)(A + C)(B+ D)(A + B)(C+ D)()式中:N为训练语料中的文档总数;q为某一特定类 别;t为特定的词项;A为属于^类且包含t的文档 频数;B为不属于^类但是包含t的文档频数;C为 属于^类但是不包含t的文档频数;D为既不属于 ^也不包含t的文档频数.C H I统计考虑了特征项与类别的正相关和特征项与类别的反相关对特征项重要性的影响.如果特 征项t和类别q正相关,说明含有特征项t的文档 属于C i的概率更大;如果特征项t和类别C i反相关,说明含有特征项t的文档不属于q的概率更大.3.2特征项赋权对文本进行分类之前,需要将文本表示成统一 格式以便于计算机处理.本文采用空间向量模型[11]表示文本,它是目前使用频率最多且效果较好 的常用模型之一.在该模型中,每个文档被表示成 一个权值向量,若选择了 n个特征项^,那么就有相 应的n个特征权值wk,文本d可表示为向量d= (棕1,棕2,…,棕…)•本文中特征项权值的计算采用TF•RF方法,它1686北京工业大学学报2017 年是一种综合分类效果较好的特征赋权方法.其中:T F表示词频(term frequency); R F表示相关频率[12].对于词项^ ,令文本d关于t k的权值为tk,产生文本d的向量表示d=(棕1,棕2,…,棕…),权值棕k 的计算公式为棕k=TFk-RFk(10)式中:TFk表示词项tk在文档d中的出现频率;RFk 值的计算公式为R F k=lo g2(2+ —)(11)max(1,Ck)式中:《k为含T F k的正类文本总数;Ck为含T F k的负 类文本总数.3.3 PCA降维在实际文本分类应用中,无需区分数据集的可 分性,因为线性分类器和凸线器都是为组合凸线器 服务,充当组合凸线器的主要功能模块.因此,在本 文提出的文本分类的组合凸线器模型中,只需要分 别使用SM A、G S M A算法构造组合凸线器进行实验. 只是组合凸线器处理大规模数据时,参数寻优时间 过长,若直接对原始文本进行处理,时间性能较差,因此,本文在利用组合凸线器进行文本分类之前,采 用主成分分析(principal component analysis, P C A)[13]的方法对所有文本数据进行降维.P C A降 维是一个成熟通用的降维模型,能有效控制信息损 失,提取文本特征,大量地研究也表明P C A降维效 果好、实用性能强[14].此外,针对组合凸线器在文 本分类中的应用,本文只考虑了 2类文本分类问题, 显然,多类问题在理论上也可以做类似处理.4实验结果为了更好地研究组合凸线器在文本分类中的性 能,分别将其与基于传统的K近邻方法、支持向量 机方法进行了对比实验.在实验过程中,本文通过 一对多方法(one-versus-rest)将数据集中多类文本 转换成2类文本进行处理,也就是每次分类时,指定 一类样本为正类,其余所有类为负类.分类的性能 主要采用正确率A、准确率P、召回率R和^作为评价指标.正确率是指分类器正确分类的样本与总 样本数之比.准确率是指分类器正确分类的正样本 数与分类器分为正类的总样本数之比.召回率是指 分类器正确分类的正样本数与实际样本数之比.A是准确率与召回率之间的综合指标,定义如下:F=2 伊P伊R(12)F1= P+ R(12)此夕卜,还将利用训练时间(training tim e)、测试 时间(testing tim e)等作为指标,对有关分类器的时 间性能进行评价.4.1实验数据集简介本节的实验采用Reuters-21578[15]和R CV1_ 4[16]作为数据集.R euters作为常用的文本数据集, 选取其文本数目最多的前10类进行实验.R C V1_4 则是文本数据集R CV1[16]语料库的一个子集,包含 4类文本(C C A T、E C A T、G CA T、M CAT),每类文本维 度高达29 992维.实验数据的详细描述见表1.表1实验数据集描述Table 1 Descriptions of data sets used in the experimentsle 数据集训练集样本数测试集特征样本数维数正类负类Acq1 488 5 086 2 084Corn1596 415818Crude3496 2251 621Earn 2 709 3 8651 658 Reuters Grain3946180 2 5341 472Interest2896 2851 057Money-fx46061141 540Ship1916 3831 040Trade3376 2371 634Wheat1986376920CCAT1516 5 701ECATRCV1 41558 5 669 2 40829 992GCAT1175 5 042MCAT1978 5 2394郾2实验结果本文的实验平台是一台联想微机,处理器15-2400 (3. 10 G H z),内存4 G,操作系统为32位 Windows.实验内容包括2部分:第一部分是在Reuters数据集上S M A与K N N的对比实验;第二部分 是在RCV1_4上GSMA、S M A与KNN、SV M s的对比实 验.实验过程中,SMA、GSM A算法的所有精度参数设 置为着=10-3,K N N的K值通过十折交叉验证从{2, 3,…,20}中选取,SV M使用2种类型,带参数C的线 性SV M(SVM.lin)和带参数(C,酌)的高斯核SVM (SVM.rbf),并分别使用 LIBLINEAR[17]和 LIBSVM[18]来执行测试.在实验中,对于带参数C的线性SVM ,第11期李玉鑑,等:基于组合凸线性感知器的文本分类模型1687因为LIBLINEAR对C值并不敏感,直接设置参数C 为默认值.而对于带参数(C,g)的高斯核SVM, LIBSVM参数的值通过十折交叉验证来选取,C和g 的候选集均为|101= -6, -5,…,5,6}.4.2. 1S M A与S V M的对比实验首先,将Reuters数据集上的所有数据利用PCA 降维,选定25维进行实验,然后,分别利用S M A和 K N N进行实验.其中,K N N无需训练分类器,可以 直接对文本进行分类,而S M A需要训练分类模型. 实验记录了 K N N和S M A分类过程(训练时间与测 试时间)的耗时.实验结果见表2.表2 S M A(K N N)在R eu ters上的实验结果Table 2 Experimental results of SM A ( K N N) on Reuters正类A P R F t/sAcq0. 926 6(0. 926 2)0. 947 0(0.9136)0.955 1(0. 995 2)0. 951 0(0. 952 7)330. 30(546) Corn0. 958 6(0. 981 1)0. 987 3(0. 981 1)0. 970 2(1.000 0)0. 978 7(0. 990 4)39. 440(530) Crude0. 970 0(0. 953 4)0. 989 8(0. 953 7)0.978 1(0. 998 7)0. 983 9(0. 975 7)69. 831(534) Earn0. 951 5(0. 654 7)0. 974 3(0. 7824)0. 942 3(0. 571 8)0. 958 0(0. 660 7)342. 70(516) Grain0. 942 0(0. 948 3)0. 991 7(0. 955 4)0. 946 7(0. 9917)0. 968 7(0. 973 2)104. 09(522) Interest0. 962 1(0. 960 9)0. 985 9(0. 962 4)0. 974 5(0. 998 4)0. 980 2(0. 980 0)54. 125(530) Money-fx0. 954 2(0. 950 3)0. 986 8(0. 951 1)0. 964 5(0. 998 7)0. 975 5(0. 974 3)80. 056(581) Ship0. 969 2(0. 538 3)0. 988 9(0. 996 1)0. 979 2(0. 524 3)0. 984 0(0. 687 0)54. 962(543) Trade0.965 3(0.901 3)0. 985 4(0. 984 8)0. 978 1(0.9108)0.981 8(0.946 4)78. 950(525) Wheat0. 959 4(0. 97 4)0. 994 6(0. 974 0)0. 963 5(1.000 0)0. 978 8(0. 986 8)37. 078(556)平均值0.955 1(0. 878 9)0. 983 2(0. 945 5)0. 965 2(0. 899 0)0.974 1(0.912 7)119. 154(538. 3)由表2可以看出,S M A的平均分类正确率、平 均分类准确率、平均分类召回率和平均值均明显高于KN N,且S M A进行文本分类所需的总时间也 远远少于KNN.因此,相比KN N,S M A花费更少的 计算时间,得到了性能更好的分类模型.4郾2郾2 G S M A与SM A、SVMs、K N N的对比实验 通过R C V1_4评估组合凸线器生长构造方法G S M A的性能,以SV M、K N N和原有算法S M A为 基准.GSMA-T1-T2表示通过G S M A构造生长组合 凸线器过程中使用了 (取值1 ~4)次膨胀,每次 膨胀包含K(取值为1)次挤压.实验过程中,该数 据集上所有数据降维至20维,并且记录了所有算 法的训练时间与测试时间,具体实验结果见表3〜8.表3 G S M A和SM A、SVM. lin、SVM. rb f、K N N在分类正确率上的对比Table 3 Comparison of G SM A,SM A, SVM. lin, SVM. rb f,K N N on classification accuracies正类SMA GSMA-1-1GSMA-2-1GSMA-3-1GSMA-4-1SVM. lin SVM. rbf KNN CCAT0. 980 10. 985 50. 985 50. 984 20.981 70.95100. 983 80. 976 7 ECAT0. 964 70. 964 70. 971 30. 964 70. 960 10. 874 20. 964 70. 961 3 GCAT0. 971 30. 975 10. 976 70. 978 40. 975 10. 966 00. 978 80. 966 3 MCAT0. 973 40. 975 10. 975 10. 970 90. 976 70.91240. 975 90. 968 9Table 4表4 G S M A和SM A、SV M.lin、S V M.rb f、K N N在分类准确率上的对比 Comparison of G SM A, S M A, SVM. lin, SVM. r b f, KNN on classification precision正类SMA GSMA-1-1GSMA-2-1GSMA-3-1GSMA-4-1SVM. lin SVM. rbf KNN CCAT0. 954 40. 975 80.981 60.98150. 965 70. 884 90. 975 60. 976 7 ECAT0. 921 70.92170.931 10. 931 10. 932 60. 680 80. 942 50.981 9 GCAT0. 941 00. 946 40. 952 70. 959 10. 942 00. 937 50. 964 20. 936 2 MCAT0. 954 30. 967 50. 972 50. 974 40. 972 50. 797 40. 957 40. 939 31688北京工业大学学报2017 年表5G S M A和SM A、SV M.Iin、S V M.rb f、K N N在分类召回率上的对比Table 5 Comparison of G SM A,SM A, SVM. lin, SVM. rb f, KNN on classification recall 正类SMA GSMA-1-1GSMA-2-1GSMA-3-1GSMA^-1SVM. lin SVM. rbf KNN CCAT0. 950 60. 954 50. 943 60. 942 60. 946 60. 881 40. 946 60. 911 1 ECAT0. 912 80.91280. 924 40. 946 40. 934 40. 777 10. 889 50. 899 2 GCAT0. 965 60. 972 50. 971 10. 969 70. 974 30. 950 40. 965 60. 971 1 MCAT0. 948 50. 939 40. 947 60. 948 00. 947 60.91210. 954 50. 938 0表6G S M A和SM A、S V M.丨in、SVM. rb f、K N N在分类上的对比Table 6Comparison of G SM A,SM A, SVM. lin, SVM. rb f, KNN on classification F x正类SMA GSMA-1-1GSMA-2-1GSMA-3-1GSMA-4-1SVM. lin SVM. rbf KNN CCAT0. 952 50. 965 00. 962 20. 961 70. 958 10. 883 20. 960 90. 942 7 ECAT0. 917 20.91720. 927 70. 938 80. 943 90. 725 80. 915 30. 908 9 GCAT0. 953 10. 959 20. 961 80. 964 40. 957 90. 943 90. 964 90. 953 3 MCAT0. 95140. 953 30. 959 90. 961 00. 959 90. 850 80. 956 00. 938 6表7 G S M A和SM A、SV M.lin、S V M.rb f、K N N在训练时间上的对比Table 7 Comparison of G SM A, S M A, SVM. lin, SVM. r b f, KNN on training time s 正类SMA GSMA-1-1GSMA-2-1GSMA-3-1GSMA-4-1SVM. lin SVM. rbf KNN CCAT29. 418879. 262 5 543. 70113 585. 2128 667. 60. 20 2 785. 95—ECAT46. 8151 409. 9008 542. 90020 846. 8048 389. 70. 18 3 147. 67—GCAT40. 9891 249. 4307 569. 68218 448. 6027 432. 40. 21 3 146. 66—MCAT56. 7941 077. 4466 724. 30013 371. 7024 231. 30. 19 3 368. 20—表8G S M A和SM A、SVM. lin、SVM. rb f、K N N在测试时间上的对比Table 8Comparison of G SM A,SM A, SVM. lin, SVM. rb f,K N N on testing time 正类SMA GSMA-1-1GSMA-2-1GSMA-3-1GSMA-4-1SVM. lin SVM. rbf KNN CCAT0. 2650. 1600. 1350. 1100. 0860. 280. 32538 ECAT0. 3830. 2970. 2210. 1720. 1330. 100. 42557 GCAT0. 2650. 1890. 1690. 1560. 0920. 090. 27575 MCAT0. 4680. 3330. 2780. 2340. 1480. 090. 31581由表3〜8可知,G S M A四个指标的表现整体高 于SM A,甚至部分指标超过高斯核SV M,同时,SMA 的4个指标明显高于线性SV M,甚至召回率高出高 斯核SVM 1.7%,其余3个指标则非常接近高斯核 SVM.另外,GSMA、S M A的全部指标均高于KNN.需要注意的是,当G S M A的膨胀次数7\ =1时,挤压 次数T2 =1,G S M A在数据上表现出比较显著的性能 提升,达到了高斯核S V M的分类性能,且整体花费 的时间也远远低于高斯核SV M,只是随着膨胀次数 的增加,测试时间有所降低,训练时间花费更长.5结论1)本文提出基于组合凸线器的文本分类模型该模型分别利用SM A、G S M A算法构造组合凸线器对文本进行分类,并通过标准文本数据集上的实验,验证了本文提出的分类模型的有效性.2)与利用K N N进行文本分类的方法相比,用GSMA、S M A构造组合凸线器进行文本分类的方 法,在总体分类性能上具有明显优势.3)相对于S V M文本分类方法,GSMA、S M A 的第11期李玉鑑,等:基于组合凸线性感知器的文本分类模型1689分类正确率和运行时间基本上介于线性S V M与高 斯核S V M之间.此外,GSMA、S M A无需参数寻优,应用比高斯核S V M简单,甚至G SM A在部分数据集 上的分类性能超过高斯核SVM.参考文献:[1] DEVASENA C L, HEMALATHA M. Automatic textcategorization and summarization using rule reduction [C]椅 Proc. of IEEE Conference Advances in Engineering,Science and Management (ICA ESM). New York: IEEEComputer Society, 2012: 594-598.[2] SEBASTIANI F. Machine learning in automated textcategorization [J].ACM Computing Surveys, 2002, 34(I):147.[3 ] VAPNIK V N. Statistical learning theory [ J ].Encyclopedia of the Sciences of Learning, 2010, 41(4):3185-3188.[4] WEBB D. Efficient piecewise linear classifiers andapplications [J].Data Classification, 2011, 28(2): 215216.[5] HERMAN G T, YEUNG K T D. On piecewise linearclassifi cation [J].IEEE Transactions on Pattern Analysisand Machine Intelligence, 1992, 14(7): 782-786.[6] TENMOTO H, KUDO M, SHIMBO M. Piecewise linearclassifiers with an appropriate number of hyper planes [J].Pattern Recognition, 1998, 31(11): 1627-1634.[ 7] KOSTIN A. A simple and fast multi-class piecewise linear pattern classifier [ J].Pattern Recognition, 2006, 39(I I): 1949-1962.[8] LI Y J, LIU B, YANG X W, et al. Multiconlitron: ageneral piecewise linear classifier [J].IEEE Transactionson Neural Networks, 2011, 22(2) : 276-289.[9] KEERTHI S S, SHEVADE S K, BHATTACHARYYA C,et al. A fast iterative nearest point algorithm for supportvector machine classifier design [J].IEEE Transactions onNeural Networks, 2000, 11(1): 124-136.[10] LI Y, LENG Q, FU Y, et al. Growing construction ofconlitron and multiconlitron [ J ] . Knowledge-BasedSystems, 2014, 65: 12-20.[11] SALTON G, MCGILL M J. Introduction to moderninformation retrieval[M]. New York: McDraw-Hill C o.,1983: 30-42.[12] MAN L, CHEW LIM T, JIAN S, et al. Supervised andtraditional term weighting methods for automatic textcategorization. [ J ] . IEEE Transactions on PatternAnalysis & Machine Intelligence, 2008, 31 (4):721735.[ 13 ] JOLLIFFE I T. Principal component analysis and factor analysis[ M]椅Principal Component Analysis. New York:Springer, 1986: 115-128.[14] WANG L W. Is two-dimensional PCA a new technique[J].AAS, 2005, 31 (5) : 782-787.[15] BACHE K, LICHMAN M. UCI machine learningrepository[EB/O L]. [2013-04-20]. http:椅 archive.ics. uci. edu/mL/datasets. html.[16] LEWIS D D, YANG Y, ROSET, et al. RCV1: a newbenchmark collection for text categorization research [J].Journal of Machine Learning Research Archire, 2004, 5:361-397.[17] FAN R E, CHANG K W, HSIEH C J, et al.LIBLINEAR: a library for large linear classification [J].Journal of Machine Learning Research, 2010, 9(12):1871-1874.[18] CHANG C C, LIN C J. LIBSVM: a library for supportvector machines [ J ] . ACM Transactions on IntelligentSystems and Technology, 2011, 2(3):27-1-27-27.(责任编辑梁洁)。
自注意力机制在时间序列预测中的应用探索
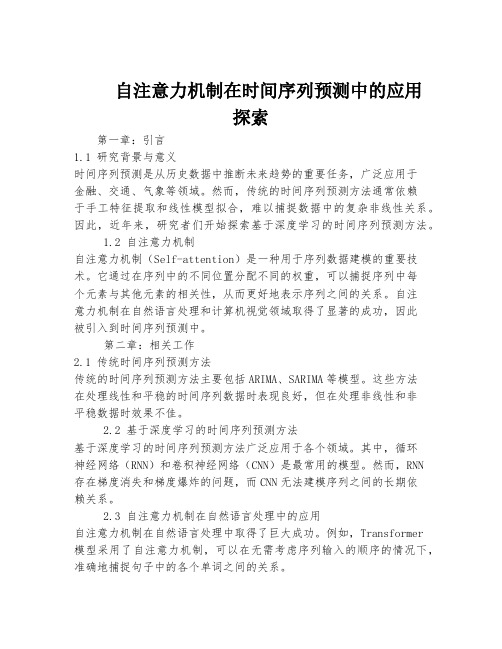
自注意力机制在时间序列预测中的应用探索第一章:引言1.1 研究背景与意义时间序列预测是从历史数据中推断未来趋势的重要任务,广泛应用于金融、交通、气象等领域。
然而,传统的时间序列预测方法通常依赖于手工特征提取和线性模型拟合,难以捕捉数据中的复杂非线性关系。
因此,近年来,研究者们开始探索基于深度学习的时间序列预测方法。
1.2 自注意力机制自注意力机制(Self-attention)是一种用于序列数据建模的重要技术。
它通过在序列中的不同位置分配不同的权重,可以捕捉序列中每个元素与其他元素的相关性,从而更好地表示序列之间的关系。
自注意力机制在自然语言处理和计算机视觉领域取得了显著的成功,因此被引入到时间序列预测中。
第二章:相关工作2.1 传统时间序列预测方法传统的时间序列预测方法主要包括ARIMA、SARIMA等模型。
这些方法在处理线性和平稳的时间序列数据时表现良好,但在处理非线性和非平稳数据时效果不佳。
2.2 基于深度学习的时间序列预测方法基于深度学习的时间序列预测方法广泛应用于各个领域。
其中,循环神经网络(RNN)和卷积神经网络(CNN)是最常用的模型。
然而,RNN存在梯度消失和梯度爆炸的问题,而CNN无法建模序列之间的长期依赖关系。
2.3 自注意力机制在自然语言处理中的应用自注意力机制在自然语言处理中取得了巨大成功。
例如,Transformer模型采用了自注意力机制,可以在无需考虑序列输入的顺序的情况下,准确地捕捉句子中的各个单词之间的关系。
第三章:自注意力机制在时间序列预测中的应用3.1 自注意力机制的敏感性分析为了验证自注意力机制在时间序列预测中的效果,我们对不同权重分配方式进行了敏感性分析。
实验结果表明,自注意力机制可以有效地捕捉序列中的关系,并提供更准确的预测结果。
3.2 基于自注意力机制的时间序列预测模型在本章中,我们提出了一种基于自注意力机制的时间序列预测模型。
该模型首先使用自注意力机制对输入序列进行特征提取,然后通过全连接层进行预测。
多尺度迭代光流估计
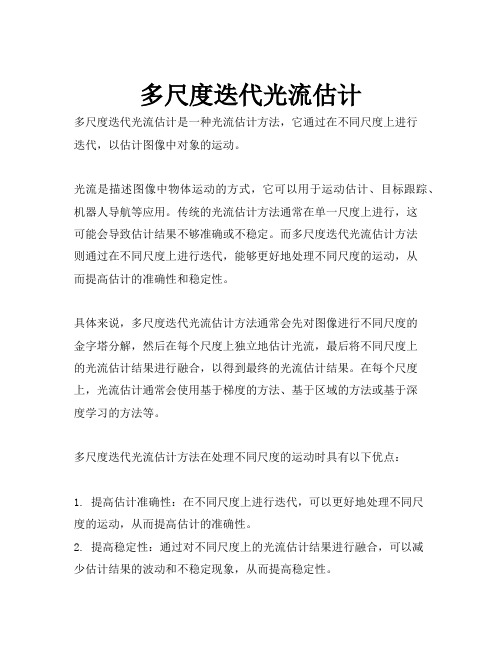
多尺度迭代光流估计
多尺度迭代光流估计是一种光流估计方法,它通过在不同尺度上进行
迭代,以估计图像中对象的运动。
光流是描述图像中物体运动的方式,它可以用于运动估计、目标跟踪、机器人导航等应用。
传统的光流估计方法通常在单一尺度上进行,这
可能会导致估计结果不够准确或不稳定。
而多尺度迭代光流估计方法
则通过在不同尺度上进行迭代,能够更好地处理不同尺度的运动,从
而提高估计的准确性和稳定性。
具体来说,多尺度迭代光流估计方法通常会先对图像进行不同尺度的
金字塔分解,然后在每个尺度上独立地估计光流,最后将不同尺度上
的光流估计结果进行融合,以得到最终的光流估计结果。
在每个尺度上,光流估计通常会使用基于梯度的方法、基于区域的方法或基于深
度学习的方法等。
多尺度迭代光流估计方法在处理不同尺度的运动时具有以下优点:
1. 提高估计准确性:在不同尺度上进行迭代,可以更好地处理不同尺
度的运动,从而提高估计的准确性。
2. 提高稳定性:通过对不同尺度上的光流估计结果进行融合,可以减
少估计结果的波动和不稳定现象,从而提高稳定性。
3. 适应性强:对于不同的应用场景和不同的数据集,可以通过调整不同尺度上的光流估计方法和融合策略,以适应不同的需求。
PDS在天体测量中的应用技术——Ⅳ.子集测量方法及总结
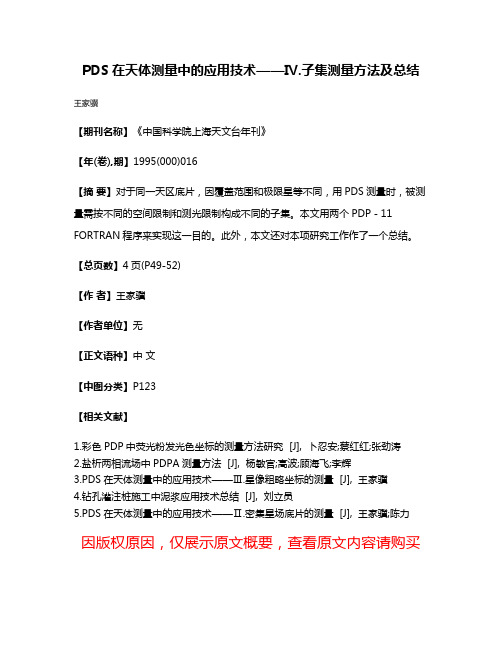
PDS在天体测量中的应用技术——Ⅳ.子集测量方法及总结王家骥
【期刊名称】《中国科学院上海天文台年刊》
【年(卷),期】1995(000)016
【摘要】对于同一天区底片,因覆盖范围和极限星等不同,用PDS测量时,被测量需按不同的空间限制和测光限制构成不同的子集。
本文用两个PDP-11 FORTRAN程序来实现这一目的。
此外,本文还对本项研究工作作了一个总结。
【总页数】4页(P49-52)
【作者】王家骥
【作者单位】无
【正文语种】中文
【中图分类】P123
【相关文献】
1.彩色PDP中荧光粉发光色坐标的测量方法研究 [J], 卜忍安;蔡红红;张劲涛
2.盐析两相流场中PDPA测量方法 [J], 杨敏官;高波;顾海飞;李辉
3.PDS在天体测量中的应用技术——Ⅲ.星像粗略坐标的测量 [J], 王家骥
4.钻孔灌注桩施工中泥浆应用技术总结 [J], 刘立员
5.PDS在天体测量中的应用技术——Ⅱ.密集星场底片的测量 [J], 王家骥;陈力因版权原因,仅展示原文概要,查看原文内容请购买。
自适应网格编码量化在图像压缩中的应用

自适应网格编码量化在图像压缩中的应用
王珏;王嘉
【期刊名称】《信息技术》
【年(卷),期】2010(000)004
【摘要】提出了一种对网格编码量化(TCQ)算法进行改进的方法-自适应网格编码量化算法(ADTCQ),并将其应用于JPEG2000图像压缩.自适应网格编码量化算法(ADTCQ)采用了多级TCQ的结构,并且通过利用已处理的数据对网格结构做出自适应调整.实验结果显示,应用于图像压缩时自适应网格编码量化(ADTCQ)算法的性能要明显优于标准TCQ算法.
【总页数】4页(P26-29)
【作者】王珏;王嘉
【作者单位】上海交通大学,图像通信与信息处理研究所电子工程系,上海,200240;上海交通大学,上海数字媒体处理与传输重点实验室,上海,200240;上海交通大学,图像通信与信息处理研究所电子工程系,上海,200240;上海交通大学,上海数字媒体处理与传输重点实验室,上海,200240
【正文语种】中文
【中图分类】TN919.81
【相关文献】
1.小波零树量化编码及其在图像压缩中的应用 [J], 肖晓玲;张翔
2.基于记忆和预测机制的自适应矢量量化及其在图像压缩编码中的应用 [J], 郑文
星;全子一
3.矢量量化在图像压缩编码中的应用进展 [J], 张霞;万国金;吴建华
4.算术编码方法在图像压缩编码中的应用 [J], 贾铸
5.子带编码在图像压缩编码中的应用 [J], 许海霞
因版权原因,仅展示原文概要,查看原文内容请购买。
基于高阶累积量的最大似然估计方法及其应用
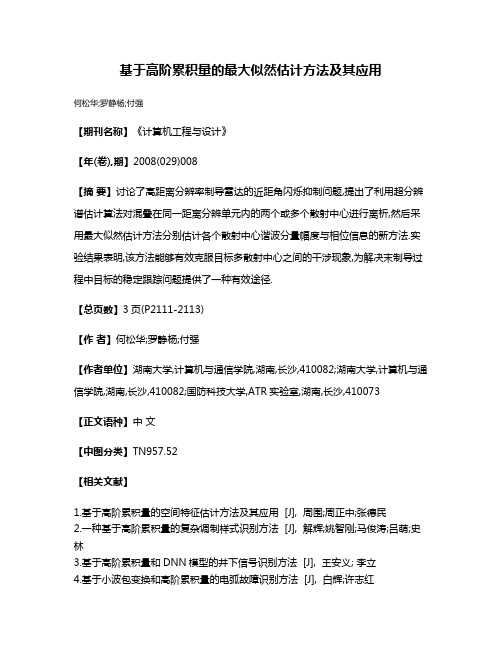
基于高阶累积量的最大似然估计方法及其应用
何松华;罗静杨;付强
【期刊名称】《计算机工程与设计》
【年(卷),期】2008(029)008
【摘要】讨论了高距离分辨率制导雷达的近距角闪烁抑制问题,提出了利用超分辨谱估计算法对混叠在同一距离分辨单元内的两个或多个散射中心进行离析,然后采用最大似然估计方法分别估计各个散射中心谐波分量幅度与相位信息的新方法.实验结果表明,该方法能够有效克服目标多散射中心之间的干涉现象,为解决末制导过程中目标的稳定跟踪问题提供了一种有效途径.
【总页数】3页(P2111-2113)
【作者】何松华;罗静杨;付强
【作者单位】湖南大学,计算机与通信学院,湖南,长沙,410082;湖南大学,计算机与通信学院,湖南,长沙,410082;国防科技大学,ATR实验室,湖南,长沙,410073
【正文语种】中文
【中图分类】TN957.52
【相关文献】
1.基于高阶累积量的空间特征估计方法及其应用 [J], 周围;周正中;张德民
2.一种基于高阶累积量的复杂调制样式识别方法 [J], 解辉;姚智刚;马俊涛;吕萌;史林
3.基于高阶累积量和DNN模型的井下信号识别方法 [J], 王安义; 李立
4.基于小波包变换和高阶累积量的电弧故障识别方法 [J], 白辉;许志红
5.基于高阶累积量与ESPRIT算法的功率振荡检测方法 [J], 杨宏宇;吕万;关雅静;陈曦;吴熙
因版权原因,仅展示原文概要,查看原文内容请购买。
用角相关函数识别随机粗糙面上散射目标的有限元解法

用角相关函数识别随机粗糙面上散射目标的有限元解法
金亚秋;李刚
【期刊名称】《电波科学学报》
【年(卷),期】1998(013)003
【摘要】研究随机粗糙面上有散射目标时散射场求解的有限元数值方法,并提出用散射场角相关函数在非记忆线上的增强,来识别粗糙面上散射目标的存在。
给出了随机粗糙面上无散射目标和有效射目标时散射场角有关函数的特征性变化。
【总页数】5页(P227-231)
【作者】金亚秋;李刚
【作者单位】复旦大学波散射和遥感中心;复旦大学波散射和遥感中心
【正文语种】中文
【中图分类】TN011
【相关文献】
1.随机粗糙面上电磁散射的高效迭代IEM计算 [J], 张晓燕;李子;江代力;刘志伟
2.随机粗糙面上目标散射差值计算的快速互耦迭代方法 [J], 叶红霞;金亚秋
3.粗糙体目标激光束散射场的互相关函数统计特征 [J], 王明军;柯熙政;华雪侠;董群锋;宫彦军
4.随机粗糙面上解决低频崩溃问题的电磁散射研究 [J], 高正平;郭靖锋
5.粗糙地面上复杂目标的电磁散射特性 [J], 付松;黄秀琼;周龙建;何思远;陈茹萌因版权原因,仅展示原文概要,查看原文内容请购买。
基于形状空间的克隆选择算法用于多峰值函数的优化
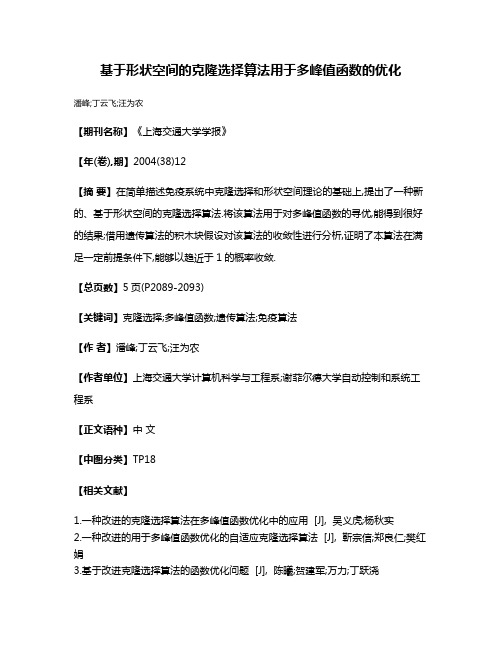
基于形状空间的克隆选择算法用于多峰值函数的优化
潘峰;丁云飞;汪为农
【期刊名称】《上海交通大学学报》
【年(卷),期】2004(38)12
【摘要】在简单描述免疫系统中克隆选择和形状空间理论的基础上,提出了一种新的、基于形状空间的克隆选择算法.将该算法用于对多峰值函数的寻优,能得到很好的结果;借用遗传算法的积木块假设对该算法的收敛性进行分析,证明了本算法在满足一定前提条件下,能够以趋近于1的概率收敛.
【总页数】5页(P2089-2093)
【关键词】克隆选择;多峰值函数;遗传算法;免疫算法
【作者】潘峰;丁云飞;汪为农
【作者单位】上海交通大学计算机科学与工程系;谢菲尔德大学自动控制和系统工程系
【正文语种】中文
【中图分类】TP18
【相关文献】
1.一种改进的克隆选择算法在多峰值函数优化中的应用 [J], 吴义虎;杨秋实
2.一种改进的用于多峰值函数优化的自适应克隆选择算法 [J], 靳宗信;郑良仁;樊红娟
3.基于改进克隆选择算法的函数优化问题 [J], 陈曦;贺建军;万力;丁跃浇
4.用于多峰值寻优的人工免疫克隆选择算法 [J], 徐静波
5.多峰值函数优化的改进克隆选择算法 [J], 李小勇;黄汉明;覃邦余;时丽娜
因版权原因,仅展示原文概要,查看原文内容请购买。
- 1、下载文档前请自行甄别文档内容的完整性,平台不提供额外的编辑、内容补充、找答案等附加服务。
- 2、"仅部分预览"的文档,不可在线预览部分如存在完整性等问题,可反馈申请退款(可完整预览的文档不适用该条件!)。
- 3、如文档侵犯您的权益,请联系客服反馈,我们会尽快为您处理(人工客服工作时间:9:00-18:30)。
Mean-field statistical simulation of grain coarsening in the presenceof stable and unstable pinning particlesG.Wang a ,b ,1,D.S.Xu a ,⇑,E.J.Payton b ,2,N.Ma b ,3,R.Yang a ,ls b ,Y.Wang b ,⇑aInstitute of Metal Research,Chinese Academy of Sciences,72Wenhua Road,Shenyang 110016,People’s Republic of China bDepartment of Materials Science and Engineering,The Ohio State University,2041College Road,Columbus,OH 43210,USAReceived 4October 2010;received in revised form 9February 2011;accepted 1April 2011Available online 22April 2011AbstractGrain coarsening in the presence of second-phase particles is investigated using a mean-field statistical model.With any given initial grain size distribution and average size and volume fraction of pinning particles as input,the model predicts grain growth kinetics and temporal evolution of the grain size distribution.Simulations are performed for both stable and unstable particle populations with sev-eral different initial grain size distributions.It is shown that the kinetics of grain coarsening is sensitive to the initial grain size distribution and pinning force variation as a function of time.Under a constant pinning force (stable particles),the final mean grain size does not vary significantly with different initial grain size distributions,though the kinetics of grain growth differs.On the other hand,a pinning force that decreases with time (associated with,for example,particle dissolution)could induce temporarily abnormal grain coarsening and a wider initial grain size distribution results in an earlier onset of the abnormal grain coarsening and a smaller final grain size.Ó2011Acta Materialia Inc.Published by Elsevier Ltd.All rights reserved.Keywords:Grain growth;Zener pinning;Time-dependent drag force;Abnormal grain coarsening;Computer modeling1.IntroductionMany engineering alloys are strengthened by second-phase particles.These particles not only interact with dislo-cations,they can also hinder the motion of grain bound-aries,inhibit grain growth and ultimately lead to a fine grain microstructure.The latter phenomenon is often referred to as “Zener pinning ”and has been studied exten-sively for the case of a stable population of pinning parti-cles [1,2].Second-phase particles may,however,become unstable during certain heat treatment schedules,and theircoarsening or dissolution will have an effect on grain growth behavior.It is therefore desirable to predict grain growth behavior in the presence of time-dependent Zener drag forces.Parallel to the treatment of precipitate growth,coarsen-ing and/or dissolution,there are two approaches to grain growth modeling:(i)mean-field models [3–10]based on Hillert’s treatment [11];and (ii)direct computer simula-tions,e.g.by the Monte Carlo method [12,13],the finite-element method [14]and the phase field method [15–17].The major advantage of the latter techniques is that mini-mal assumptions are needed concerning the detailed micro-structural information about both grains and pinning particles such as their shape,size and spatial distribution,or the changes of these characteristics with time.A serious drawback of the latter group,the direct simulation tech-niques,is that they require massive computer resources,especially in the case of three-dimensional (3-D)simula-tions.A detailed comparison of the strengths and limita-tions of three direct simulation methods for modeling1359-6454/$36.00Ó2011Acta Materialia Inc.Published by Elsevier Ltd.All rights reserved.doi:10.1016/j.actamat.2011.04.004⇑Corresponding authors.Tel.:+862423971946;fax:+862423891320.E-mail addresses:dsxu@ (D.S.Xu),wang.363@ (Y.Wang).1Present address:South China University of Technology,Guangzhou 510640,People’s Republic of China.2Present address:Institute for Materials,Ruhr-Universita ¨t Bochum,Bochum 44801,Germany.3Present address:ExxonMobil Research &Engineering Company,Annandale,NJ 08801,USA./locate/actamatActa Materialia 59(2011)4587–4594Zener pinning was made by Harun et al.[18].However,to the best of our knowledge,there have not yet been any published3-D simulations of grain growth in the presence of dissolving and/or coarsening populations of pinning particles using direct methods,though a2-D phase-field study on Zener pinning with evolving particles has been reported[19].In contrast,mean-field models have the abil-ity to make rapid predictions of grain growth kinetics using a desktop computer,and hence have been widely adopted in industrial applications.In previously published work, however,constant drag force was usually assumed and lit-tle attention has been paid to the case of time-dependent pinning forces thus far.In addition,the influence of the shape of the initial grain size distribution(GSD)on the grain growth behavior in the presence of pinning particles has not been fully investigated,especially for the case of time-dependent pinning forces.This paper investigates grain coarsening in the presence of different populations of pinning particles using a mean-field statistical model while maintaining a specific focus on the effect of time-dependent drag forces and differing initial GSDs on the grain growth behavior.The incorporation of drag forces into a3-D mean-field statistical model of grain growth is introduced in Section2,and the simulation meth-odology and critical parameters are discussed in Section3. Simulation results showing the effects of different initial GSDs and variable pinning forces are presented in Section4 and discussed in Section5.The majorfindings are summa-rized in Section6.2.Incorporation of particle pinning into the mean-field statistical grain growth modelIf grain growth is described in terms of elementary mat-ter exchanges between pairs of interacting grains and it is assumed that(i)grain boundary properties(energy and mobility)are isotropic,(ii)grain boundary velocity is pro-portional to the pressure difference caused by curvature and(iii)the average curvature of grains can be calculated from their volume-equivalent spheres(an assumption that is well founded experimentally according to Patterson and Liu[20]),the following kinetic equation for grain coarsening in single-phase polycrystalline materials may be derived[7–10,21]:dV i dt ¼M cXjA ij2R jÀ2R ið1Þwhere V i and R i are the volume and radius of grain i, respectively,t is time,M is grain boundary mobility,c is grain boundary energy,and A ij is the contact area between grain i and an adjacent grain j.Since the contact area between pairs of adjacent grains must always be equal, i.e.A ij=A ji,Eq.(1)automatically satisfies the volume con-servation condition of the whole system.It should be noted that Eq.(1)is sufficiently general since A ij can be chosen to account for any microstructure in the form of correlations between grain sizes.In the present work,the following for-mulation of A ij has been adopted:A ij¼4p R2iR2jXkR2k,ð2Þwhich can be derived from the assumption of a random ar-ray of grains[17].Following Hillert’s treatment[11],one can obtain the rate of volume change of a grain in a parti-cle-containing material by inserting into Eq.(1)a term to account for the drag of particles on moving grain boundaries:dV idt¼M cXjA ij2R jÀ2R iÆz:ð3ÞHere the drag term,z,due to second-phase particles can be evaluated following Sun et al.[22]or estimated from the classical Zener approximation[1,2]:z¼3f=2rð4Þwhere f and r are the volume fraction and average radius of the pinning particles,respectively.If the pinning particles are assumed to have no effect on the contact area between grains,then the grain growth rate can be described as: dR idt¼M cXjR2j2R jÀ2R iÆzXjR2jð5ÞIt is worth emphasizing that the sign for the z term in Eqs.(3)and(5)must be chosen in each case such that the pinning force is acting against the movement of the grain boundaries.The negative sign holds when1/R i<1/ R jÀz/2and the positive sign holds when1/R i>1/R j+ z/2.In the case that1/R i P1/R jÀz/2or1/R i61/R j+ z/2,dR i/dt must be zero.In this mean-field model the pin-ning force z simply imposes a uniform friction force on grain boundary migration.Grain boundaries can move only when local driving forces exceed this friction force.If z=0,Eq.(5)reduces to the classical Hillert equation[11]:dR idt¼2M cXjR j,XjR2jÀ1R i!¼2M c1R crÀ1R ið6Þand the critical grain size R cr¼R2=R agrees with the result obtained by Lu¨cke et al.[23],where the relationship is de-rived from a two-parameter statistical theory.3.Simulation methodology3.1.Numerical solution of the mean-field statistical grain growth equationTo solve Eq.(5)numerically,instead of using continu-ous distributions,grains are divided into discrete classesof size D R and those having sizes from R ciÀD R=2toR ciþD R=2belong to the i th class centered at R ci.The number of grains in class i can be calculated from the size distribution function(SDF)/i,which represents the4588G.Wang et al./Acta Materialia59(2011)4587–4594statistical frequency of occurrence of grains system.Thus,the following relationshipsX i /i¼1X i /iR ci¼ R:Inserting Eqs.(7)and(8)into Eq.(5) equation for grains in class i that reads:dR ci dt ¼M cXj/jðR cjÞ22R cjÀ2R ciÆz!"#,XjThe SDF must also obey the continuity@/ @t ¼À@@R/dRdt:Eq.(10)may be numerically integrated by the following forms:D/i D t ¼À1D R/idR cidtÀ/iÀ1dR c iÀ1dtdR cidtD/i D t ¼À1D R/idR cidtÀ/iþ1dR c iþ1dtdR cidtwhere D t is time step,D/i is the change of/ithe growth rate dR ci =dt is calculated from Eq.poral evolution of GSD may then beEq.(11),and the average grain radius any time through Eq.(8).3.2.Initial conditions and simulationIt is well accepted that the GSD is often log-normal in experimental observations[24] that the probability density function ofbefitted to:fðRÞ¼1R rffiffiffiffiffiffi2pp expÀðln RÀlÞ22r2"#;where R is the grain radius and thethe mean and standard deviation of ln R,respectively.In this paper,three log-normal GSDs are generated as model input,and l and r are chosen such that each case has the same initial mean grain size but a different distribution.Ta-ble1and Fig.1show the parameters and shapes of the three GSDs,respectively.The bin size D R of the GSD his-togram was set to0.2l m in all simulations.Since the value of M c in most alloys is in the range of0.1–10l m2s–1[25],M c=1l m2s–1was adopted in the present work.The time step was set to D t=0.01s,and the total annealing time that was simulated in each case was3600s. Because the GSD shifts from left to right during grain growth,the bin number was set to be400in order to avoid overflow of the maximum grain size from the right boundary of the GSD.In the case of constant pinning force simulations,the effects of drag term with values of z=0.1,0.2and0.3l mÀ1were investigated.For simulations of unstable particle populations,five decreasing pinning forces are assumed,each of which decrease from1.0to 0.01l mÀ1at a different rate.The shapes of the decreasing pinning force curves are given in Fig.2,where L1refers to linear decrease,L2and L3to the initially relatively slow and then rather rapid linear decreases,and L4and L5to the initially relatively fast and then rather slow linear decreases.4.Results4.1.Grain growth with constant pinning forceThe grain growth behavior of the three systems having GSDs shown in Fig.1with the same initial mean grain size1.The initial log-normal GSDs with the same mean grain size usedto the model.2.Five different shapes of decreasing pinning forces examined in present study.G.Wang et al./Acta Materialia59(2011)4587–45944589which indicates that a larger pinning force results in a rower GSD and a correspondingly larger peak value.3.Grain growth kinetics for the three different initial GSDs constant pinning force z =0.1l m À1.4.Temporal evolution of GSD2with constant pinning 0.1l m À1.5.Grain size distributions at t =3600s for the three initial GSDs constant pinning force z =0.1l m À1.6.Grain growth kinetics of GSD2at different levels of constant pinning force.7.Grain size distributions of GSD2at t =3600s with different constant pinning forces.A small kink exists after the peak evolved GSD.This kink is located where changes sign.This sign switching causes a in the distribution evolution equation,but on the overall grain growth kinetics.4.2.Grain growth with decreasing pinning In most commercial alloys,the pinning not always be stable.For example,a 2are two important types of precipitates in and they may dissolve and/or coarsen at different temperatures.According to Eq.lution and coarsening of these particles decreasing the pinning force in the system,ferent conditions the pinning force may ent rates.The case of constant decreasing rate Fig.8for each of the three initial GSDs It can be seen that starting from the same size,the one having the widest distribution initial growth rate,but the growth slows stages;eventually the system ends up with grain size than those having initially tions.The initial jump of GSD3could be the fact that there are more grains with pairwise stagnation criteria (1/R j +z R j Àz /2)in the case of GSD3than in the The later stage of the growth is different constant pinning forces (shown in Fig.3),initial distribution results in the coarsest ilar behaviors are also found in the cases of the results are not shown here.The growth rates for L1and L4increase smoothly as the pinning force decreases,irrespective to the sudden rate change in the case of L4.It is interesting,though,that a wavy feature is exhibited in the growth kinetics of case L5.In this case,the grain size initially holds constant because the driving force for growth cannot overcome the large pinning force.The average grain size then jumps rap-idly due to the rapid reduction in the pinning force.Finally a sharp rate change in the reduction of the pinning force results in a dramatic rate change in grain growth.Fig.10shows the GSDs at t =3600s with GSD1as ini-tial distribution,obtained for the five cases with decreasing pinning force.It can be seen that the larger initial pinning forces in L2and L3lead to narrower distributions and higher peak values of the SDF than for L4and L5,where the pinning force decreased dramatically at the beginning of the simulation.5.DiscussionIn this paper,grain growth in particle-containing mate-rials was simulated using a mean-field statistical model8.Grain growth kinetics for three different initial GSDs with linearly decreasing pinning force L1.9.Grain growth kinetics of GSD1with different decreasing pinning forces.10.Grain size distributions of GSD1at t =3600s with different decreasing pinning forces.G.based on the pair interactions of neighboring -pared with the early mean-field models [6–9],the model used here can capture more accurately the contributions from surrounding grains to the growth pressure because it does not rely on the calculation of a critical grain size,and it can be applied to cases with either constant or vari-able pinning forces.Although three different log-normal distributions with the same initial mean grain size are assumed as input here,the model may in principle accept any GSD as input.For simplification,the properties of all boundaries are set to be isotropic in the paper,but it is possible to extend the model to the cases with anisotropic boundary properties [21]or non-monotonic annealing temperatures.The kinetic equation in the present model is only an approximate solution for the evolution of the GSD.Recently,a rigorous and specific formula for the growth rate (volume change)of a grain in a 3-D microstructure was presented based upon the von Neumann relation [26]:dV dt ¼À2p M c L ðD ÞÀ16X ni ¼1e i ðD Þ !;ð13Þwhere L (D )is a natural measure of the linear size of do-main D and P ni ¼1e i ðD Þis the summation of the length of triple line e i over all n triple lines of D .For a spherical grain,the mean width of D ,L (D ),is twice its diameter [26],thus Eq.(13)can be written as:dR dt ¼M c 112R X ni ¼1e i ðD ÞÀ2R !:ð14ÞComparing the above equation with Eq.(5),one can see that both equations can be reconciled if the following expression holds:X n k ¼1e k ðD i Þ¼12R 2i 2X R j =XR 2j Æz :ð15ÞSpherical grains cannot fully fill space,and thus cannot have triple lines or fulfill any other topological constraints of a 3-D space-filling network.Therefore,the present sta-tistical model may be regarded as an approximation of the exact formula given by Eq.(13)based on two assump-tions:(i)the polycrystalline microstructure is described by arrays of spherical grains,and (ii)the equivalent value of P nk ¼1e k ðD i Þof spherical grains can be calculated by Eq.(15).One can also see that this value is affected by both the neighboring grain size and the mean-field pinning force.However,it should be mentioned that when the pinning force is zero,this mean-field model reduces to the classical Hillert grain growth model [11]and yields the Hillert distri-bution that tends to disagree with experimental observations.The grain growth processes of the three different initial GSDs considered here exhibit different kinetic features although they have the same initial grain size.Under con-stant pinning forces,the average grain sizes of all GSDs increase with time but the rates of increase decline towardzero with time (Figs.3and 4)when the pinning force even-tually balances the driving force in Eq.(3).The simulation results also indicate that with identical pinning force and initial average grain size,wider GSDs produce larger grain sizes (Fig.3).This effect arises because a wider distribution has a larger grain size discrepancy and therefore a larger average value of |1/R j À1/R i |.It can be seen from Eq.(5)that the driving force for grain growth is a function of GSD,so should be the final grain size.Thus,the Zener equation should only be regarded as a zero-order estima-tion of the stagnant grain size.The Zener size,R Z =4r /3f =2/z =20l m,is close to our calculated values shown in Fig.3.The distribution shapes converge as the grain size stag-nates (Figs.4and 5).This is not surprising because the local driving forces (local grain size differences)in all the cases are balanced by the same constant and uniform pin-ning force at stagnation.For a given initial GSD,the sim-ulation results show that a larger pinning force leads to a slower growth rate (Fig.6)and a narrower final GSD (Fig.7).In the case of a large pinning force,boundary motion is only possible between pairs of grains that are at both sides of the distribution.Since the absolute value of the driving force is much higher for small grains,the dis-tribution narrows as the large grains approach the stagnant size,at which point there is little driving force for their con-tinued growth.However,the GSD is still wider than the initial one,which means that when the drag force is large,fewer grains are outside the stagnation range for a given starting GSD and hence limited coarsening occurs to alter the GSD.The situation becomes more complicated when the pin-ning force changes with time.It can be seen from Fig.8that the three initial GSDs lead to different growth kinetics.In contrast to the cases of constant pinning force,where the growth rates decrease continuously (Fig.3),the growth rates increase continuously within the simulated annealing time as the pining force decreases monotonically.More interestingly,the narrower distribution GSD1has a more sluggish growth initially but grows more rapidly at later stages,ultimately yielding coarser grains.The sluggish ini-tial growth rate is due to the limited number of grains with high enough driving force for growth,i.e.the pairs of grains at the both sides of the distributions and outside the bounds set by the Zener drag.When growth is not stag-nant and the coarsening is most prominent at the tails of the distribution,abnormal grain coarsening may occur until a new balance is reached between the driving force and the drag force.When pinning forces with non-constant decreasing rates are assumed,the kinetic curves exhibit some interesting characteristics,such as a flat region during the first stage due to the excessively large pinning force and a rapid jump at some later time when the pinning force is reduced sud-denly (Fig.9).It can be observed from Figs.8–10that both the pinning force in the system and the initial size distribu-tion influence the shape of the GSD.In particular,Fig.84592G.Wang et al./Acta Materialia 59(2011)4587–4594shows that the narrower initial GSD(e.g.the largerfinal grain size when the decreasingis applied.In the case of GSD1,as the decreases,there is a relatively sharp increaseof grains with sizes outside the stagnationgrains are unleashed)at certain given2100s),and hence the grain growth ratetwo cases from then on.This is illustratedwhich the comparison of GSDs at t=2100sferent initial GSDs is given.Novikov[6,7]studied grain growthdecreasing pinning force and analyticallyreduction in pinning force can lead toof large grains.In order to check thean amplified growth during the processeswe calculate Y¼RdR max=dtÀR max dR=dt.growth rate of the largest grain is higher thanof average size,indicating amplified growth of large grains,which is related to abnormal(secondary recrystallization type)grain growth[27].Fig.12shows the change in Y as a function of time for the systems with GSD1and GSD3 subject to decreasing pinning forces L1,L2and L5(only the positive part of Y is shown here).It can be seen that amplified growth of large grains does takes place temporar-ily when the pinning force decreases,and this temporary abnormal grain coarsening is induced at an earlier time in the case of a wider initial distribution(GSD3in Fig.12).This is a result of the interplay between the char-acter of the initial GSD and the decreasing rate of the pin-ning force.For example,a wider distribution contains a larger number fraction of grains having sizes far from the critical grain size.Grains that are much smaller and larger than the critical grain size and are adjacent to each other higher the possibility for abnormal grain coarsening.Both the pinning force and the initial GSD(and its temporal evolution)determine the character of abnormal grain coarsening(i.e.the intensity and the time at which it occurs)during the heat treatment process.Ultimately,this results in different growth kinetics for different initial GSDs when growth occurs in the presence of an unstable popula-tion of second-phase particles.While the parameter Y does indicate that abnormal grain growth is possible for a few cases,it occurs only over a short time and with a small value of Y.This is related to the fact that the GSDs shown in Fig.10do not contain any truly abnormal grains that are typically an order of magni-tude larger than the average.On the other hand,the pin-ning force is distributed uniformly throughout the system in the mean-field model.Under such a circumstance,a true abnormal grain growth may never occur.Dennis et al.[28] studied abnormal grain growth in an Al–3.5wt.%Cu alloy and found that the fraction of pinning phase was much lower at abnormal grain boundaries than at normal bound-aries in thefine-grained matrix into which the abnormal grains were growing.Since the mean-field model can be applied easily in cases with more complicated variation of the pinning force,three additional types of unstable particles that could be encoun-tered in practice were considered.Thefirst is coarsening in which the volume fractions of particles are constant and their mean size is proportional to the cube root of time; the second is size-dependent dissolution,where the volume fractions of particles follow f=f0exp(Àkt n)(where f0is the initial volume fraction and k and n are constants)and the average particle radius can be estimated by r¼ffiffiffiffiffiffiffiffiffiffiffiffiffiffir2Àatp(where r0is the initial radius and a is con-stant)[29];and the third considers multiple populations of precipitates,where one population disappears com-pletely,leading to a step function in f/r.For real systems, the detailed expressions of f and r can be obtained byfitting the experimental measurements and the change of z with time can be calculated by Eq.(4).The change in drag force11.Grain size distributions at t=2100s for three different initial GSDs with linearly decreasing pinning force L1.12.Plots of Y¼RdR max=dtÀR max dR=dt as a function of time for systems having GSD1and GSD3with decreasing pinning force,L1, L5shown in Fig.2(only the positive axis of Y is shown here).G.with time is plotted in Fig.13,and the GSDs at t=3600s for the system initially having GSD1are given in Fig.14.It is shown that different decreasing rates of pinning force lead to different grain growth kinetics.Therefore,our mean-field model can deal with complex cases,and should therefore be of practical help to metallurgists.6.ConclusionsA mean-field statistical model of grain growth was developed to predict the growth kinetics and evolution of GSDs in systems with both stable and unstable populations of pinning particles.The model is able to consider any arbi-trary variation of pinning particle population as function of time during grain coarsening.For systems with stable particles and hence constant pinning force,the results dem-onstrate that larger pinning forces result infiner grain sizes and narrower GSDs,while for systems with unstable populations of pinning particles,the decreasing pinning forces result in complicated grain growth kinetics deter-mined by the interplay between the character of the initial GSD and the decreasing rate of the pinning force.Abnor-mal grain coarsening was observed temporarily after a rapid reduction in the pinning force,but the uniform distri-bution of pinning force among all moving boundaries assumed in the mean-field model prevented the prediction of extended abnormal grain growth as seen in secondary recrystallization.AcknowledgementsThe support of the Ministry of Science and Technology of China under Grant No.2006CB605104and2011CB606404, the National Natural Science Foundation of China under Grant No.50631030(G.W.,D.S.X.and R.Y.),GE Aviation (E.J.P.,M.J.M.and Y.W.),the US Air Force Office of Scientific Research under Grant No.FA9550-09-1-0014 (M.J.M.and Y.W.)and the US National Science Founda-tion under Grant No.CMMI-0728069and Grant No. DMR1008349(Y.W.)is gratefully acknowledged. References[1]Smith CS.Trans AIME1948;175:15.[2]Manohar PA,Ferry M,Chandra T.ISIJ Int1998;38:913.[3]Anderson I,GrongØ,Ryum N.Acta Metall1995;43:2689.[4]Rios PR.Acta Mater1997;45:1785.[5]Humphreys FJ.Acta Mater1997;45:5031.[6]Novikov VYu.Scripta Mater1997;37:463.[7]Novikov VYu.Scripta Mater1999;40:91.[8]Di Nunzio PE.Acta Mater2001;49:3635.[9]Maazi N,Rouag N.J Crys Growth2002;243:361.[10]Di Nunzio PE.Phys Rev B2003;68:115432.[11]Hillert M.Acta Metall1965;13:227.[12]Miodownik M,Holm EA,Hassold GN.Scripta Mater2000;42:1173.[13]Zollner D,Streitenberger P.Scripta Mater2006;54:1697.[14]Couturier G,Doherty R,Maurice C,Fortunier R.Acta Mater2005;53:977.[15]Suwa Y,Saito Y,Onodera H.Scripta Mater2006;55:407.[16]Moelans N,Blanpain B,Wollants P.Acta Mater2006;54:1175.[17]Moelans N,Blanpain B,Wollants P.Acta Mater2007;55:2173.[18]Harun A,Holm EA,Clode MP,Miodownik MA.Acta Mater2006;54:3261.[19]Fan D,Chen LQ,Chen SP.J Am Ceram Soc1998;81:526.[20]Patterson BR,Liu Y.Metall Trans A1992;23:2481.[21]Abbruzzese G,Lu¨cke K.Acta Metall1986;34:905.[22]Sun N,Patterson BR,Suni JP,Weiland H,Allard LF.Acta Mater2006;54:4091.[23]Lu¨cke K,Brandt R,Abbruzzese G.Mater Sci Forum1995;204–206:19.[24]Underwood EE.In:DeHoffRT,Rhines RF,editors.Quantitativemicroscopy.New York:McGraw-Hill;1968.p.149.[25]Feppon JM,Hutchinson WB.Acta Mater2002;50:3293.[26]MacPherson RD,Srolovitz DJ.Nature2007;446:1053.[27]Thompson CV,Frost HJ,Spaepen F.Acta Metall1987;35:887.[28]Dennis J,Bate PS,Humphreys FJ.Acta Mater2009;57:4539.[29]Wang G,Xu DS,Ma N,Zhou N,Payton EJ,Yang R,et al.ActaMater2009;57:316.13.Drag force z as a function of time for three different typesunstable particles:coarsening particles,size-dependent dissolvingand mixture of dissolving and stable particles.14.Grain size distributions at t=3600s of GSD1for three differenttypes of unstable particles:coarsening particles,size-dependent dissolvingparticles,and mixture of dissolving and stable particles.59(2011)4587–4594。