地震相分析和层位解释的深度学习方法研究
层序地层学-第2章 地震层序与地震相分析-中国地质大学(北京)
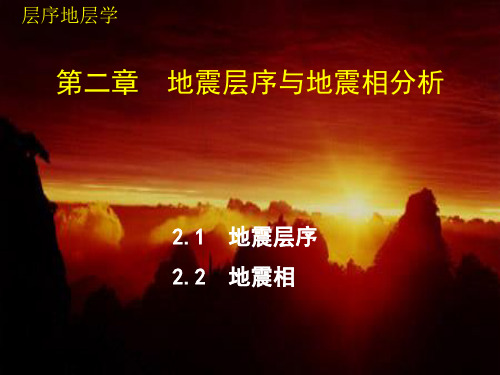
(2) 视削截界面
• 其下同相轴呈切线向下倾方向逐渐终止于该界
面上,且地层单元很快侧向尖灭。往往与最大
水进期的沉积饥饿面相对应。
• 此外因海平面下降而造成的陆棚边缘的削截也
可形成视削截界面,在顺侵蚀峡谷走向的地震 剖面中较常见。
开阔台地
开阔海陆棚
前斜坡
斜坡脚
停滞缺氧盆地
陆棚坡折
(3) 顶超界面
T8
复 合 不 整 合 面
Tg3
Tg5 Tg5-1 Tg8
塔西南隆起
塔中隆起 满加尔凹陷 塔北隆起
Z40线,按T8(第三系底界)拉平。示Tg复合不整合面,剖面近南北向
l300_t8
T3 T6 T2
T8
T82’ Tg
复合不整合面
Tg3
Tg5-1
Tg5
Tg8
L300线,按T8(第三系底界)拉平。示Tg与上覆、下伏众多不整合面在塔东地区组成的复 合不整合面,剖面近北东东向,从塔西南到塔东北
•
3、七十年代
以数字地震仪为主,资料质量显著提高,并可以获得丰富的各种参数,产生了地震地层学、 岩性地震学、烃类检测技术和储层参数估计技术。
•
4、八十年代
高分辨率地震勘探技术、交互式人机联作解释技术和地震反演技术取得重大进展,地震与 地质结合得更为紧密,学科朝宏观和微观发展,分别产生了层序地层学和储层地震学,走 向综合。
三、学习方法和要求
思路和技能
• 脑袋 • 手足
2.1 地震层序分析
• 2.1.1 地震反射界面的追踪对比方法 • 2.1.2 地质界面的类型和特征 • 2.1.3 地震反射界面的类型、成因及区分 • 2.1.4 地震反射界面的地层学意义
地球物理探测中的数据处理与解释研究

地球物理探测中的数据处理与解释研究地球物理探测是地球科学中最基础、最重要的研究领域之一。
通过对地球内部不同物理量(如重力、磁场、地震波等)的探测和观测,可以揭示地球内部的结构和性质,研究地球各种自然现象的形成机制和演化规律,推测出地球历史上的演化历程,为各种工程和资源勘探提供重要的基础数据和技术支持。
然而,地球物理探测的数据处理和解释研究却是一个十分复杂和难以突破的领域。
一般来说,地球物理探测中得到的数据包含了成千上万个参数,这些参数之间的关联性极其复杂,而且每种物理量的数据分布也往往存在各种噪声和偏差。
因此,如何从这些复杂数据中提取出有用的物理信息,成为了地球物理探测方法中必须攻克的重要问题。
一种重要的地球物理探测方法是磁法探测。
这种方法利用地球磁场的变化,探测地下矿床和岩层的磁化性质,从而推断出地下的结构和成分。
由于磁场常常受到工业和人类活动的影响,因此磁法探测中的数据处理和解释研究尤为重要。
目前,磁法探测中常用的数据处理方法有卷积、反演、平滑等,这些方法可以从原始数据中提取出不同空间分辨率和信噪比的信息。
但是,由于地下物质翻译中的非线性和非均匀性,以及地球磁场的非稳定性和非旋转性等因素的影响,磁法探测中的数据处理与解释仍存在许多挑战。
例如,磁法探测中通常采用的正则化反演方法,存在无法有效利用信息的缺陷;而采用热力学反演方法的成本十分高昂,并且不适用于大规模分布的数据处理。
除了磁法探测,地球物理探测还有电法、重力、地震等多种方法。
在这些方法中,数据处理和解释的研究重点不同,但也面临着类似的难题。
例如,在电法探测中,数据处理和解释的关键是如何处理大量观测数据,以获取可靠的电阻率分布;而在重力探测中,数据处理和解释则需要通过分析重力势场差异,推断出地下物质的密度分布和体积结构。
近年来,随着计算机和数据处理技术的快速发展,人们能够利用更高效的数值算法和数据处理工具来解决地球物理探测中的复杂问题。
例如,利用神经网络、数据挖掘、机器学习等先进技术,人们正在寻找一些能够应用于大规模数据处理的新方法和新框架。
基于深度学习的建筑物抗震能力评价模型
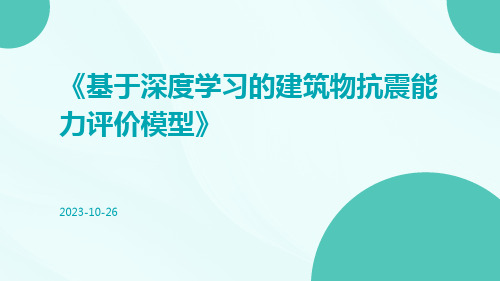
近年来,数值模拟和有限元分析等方法逐 渐应用于建筑物抗震能力评价,但仍然存 在计算量大、模型精度不高的问题。
建筑物抗震能力评价研究涉及土木 工程、地震工程、计算机科学等多 个领域,不同领域的研究人员从不 同的角度开展研究。
深度学习在建筑结构领域的应用现状
深度学习作为一种新型的机器学习技术,已经在建 筑结构领域得到广泛应用。
详细描述
训练过程中的优化策略对模型的训练速度和收敛速度具有重要影响。通过采用梯度下降算法、学习率衰减等优 化策略,可以加快模型的训练速度和收敛速度,从而缩短模型的训练时间,提高模型的预测效率。同时,还可 以采用早停法、验证集评估等手段防止过拟合现象的发生。
05
模型应用与验证
模型在真实数据上的应用
传统的建筑物抗震能力评价方法主要基于经验和 简单的计算模型,难以准确预测和评估建筑物的 抗震性能。
建筑物在地震中常常受到损坏甚至倒塌,对人民 生命财产造成严重威胁。
随着人工智能和深度学习技术的发展,为建筑物 抗震能力评价提供了新的解决方案。
研究意义
通过建立基于深度学习的建筑物抗震 能力评价模型,能够实现对建筑物抗 震能力的准确预测和评估,为地震灾 害的预防和减灾提供科学依据和技术 支持。
调整模型参数
根据训练效果,调整模型参数,如 学习率、隐藏层数、节点数等,以 提高模型的预测精度。
模型优化
采用多种优化算法,如梯度下降法 、随机梯度下降法等,对模型进行 优化,以提高其性能。
模型测试
使用测试集对训练好的模型进行测 试,评估模型的性能和预测精度。
模型评估指标
准确率
评估模型预测结果的准确程度。
数据驱动方法的优势
该研究进一步证明了数据驱动方法在解决复杂的工程问题如建筑 物抗震能力评价方面的优势。
智能地震相预测:教你用深度学习进行工区地震相预测

智能地震相预测:教你用深度学习进行工区地震相预测(附程序)地震相是储层表征的方法之一,通过对目标区域有利层序内地震相的研究,可以确定储层的沉积相及横向的分布范围,从而为储层的综合预测奠定基础。
01 地震相介绍地震相是指沉积相在地震资料上的响应,地震相一词来源于沉积相,可以理解为沉积相在地震剖面上表现的总和。
地震相包括在地震剖面上的响应,在水平切片或层振幅平面图上的响应,在反演速度剖面上或正演模型上的响应等。
地震相分析的方法就是识别每个层序内独特的地震反射波组特征及其形态组合,并将其赋予一定的地质含义,进而进行沉积相的解释,这一过程称为地震相分析。
地震相分类是储层表征的步骤之一,三维地震和地震属性等油气储层表征技术的进步丰富了专家对地下的描述。
然而,对这一巨大数据量的分析却成了一项复杂的任务,人工分析已经不能适应工程上的需要。
本文后续将带领大家探索使用深度学习在地震相分类中的应用。
02 项目实战1.实验数据介绍本次实战项目使用荷兰F3的数据。
荷兰北海区域富含碳氢化合物沉积物,北海大陆架位于荷兰海岸附近,被划分为不同的地理区域,用字母表的不同字母来描述;在这些区域内是标有数字的较小区域。
其中一个区域是尺寸为16km x 24km的矩形,称为F3区块,见图。
根据区块的层位和断层解释情况,对该区块进行了三维建模,最终的3D地质模型如图所示:2.深度学习实现地震相预测数据构建:我们用来训练和测试网络模型的最终地震模型并不是F3的全部区域。
为了满足网络运算,我们还从目标训练数据切割小块数据进行训练。
对于非常大的地震体数据,这种方法比使用整个剖面进行训练更可行。
在训练时,从训练集的inlines和crosslines线中随机抽取地震数据块及其相关标签。
在测试阶段,模型在inlines和crosslines方向上对重叠的小块数据进行采样,并对结果进行平均,以生成inlines或crosslines的二维标记测试集。
地震构造解释技术方法
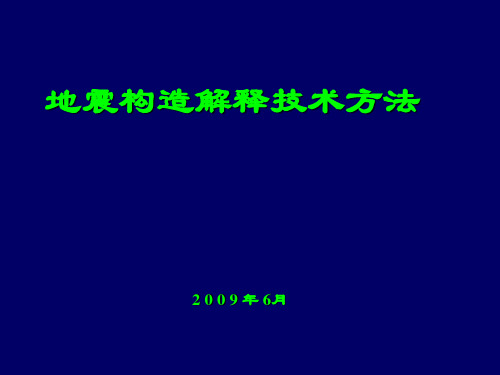
地震精细构造解释技术方法
一、地震地质综合标定 二、地震反射特征分析 三、地震地质层位解释 四、断层解释 五、时间切片生成及应用 六、相干体技术 七、断层平面组合及空间组合 八、地震地质相互结合、相互校验 九、地震属性分析 十、全三维解释技术
利用块移动工具分析同沉积断层
渤海盆地第三系拉张式构造模式
中国西部挤压式构造模式
要对地震剖面上的构造和断裂作出合理可靠的解释,在一定程 度上还决定于解释人员对工区有关褶皱、断裂等构造模式的掌 握程度。如我国东部渤海盆地第三系拉张式构造模式,由于受 拉张应力的作用,断裂通常表现为正断层。在我国西部,一般 表现为挤压式构造模式,由于构造受挤压作用,断裂通常表现 为逆断层。
高精度合成地震记录的检查之二——与工区内平均速度对比法
某工区内井的时深关系与平均速度(红色)对比图
合成地震记录提取其时间-深度对,与工区的平均速度曲 线相对比,应比较一致。
不同时深关系控制下的合成记录对比
时深关系畸变 错误时深关系
正确时深关系
不同时深关系曲线对比
高精度合成地震记录的检查之三——剩余记录法
地震资料解释可分为三个阶段:
构造解释—20世纪70年代以前主要以地震资料的构 造解释为主,即利用反射波旅行时、速度等信息,查明 地下地层的构造形态、埋藏深度、接触关系等。
地层岩性解释—出现在70年代后期,这一阶段包括两 部分内容:一是地震地层学解释,即根据地震剖面特征、 结构来划分沉积层序,分析沉积岩相和沉积环境,进一 步预测沉积盆地的有利油气聚集带;二是地震岩性学解 释,它是采用各种有效的地震技术,提取一系列地震属 性参数,并综合利用地质、钻井、测井资料,研究特定 地层的岩性、厚度分布、孔隙度、流体性质等。
地震平面岩相解释方法的研究及应用
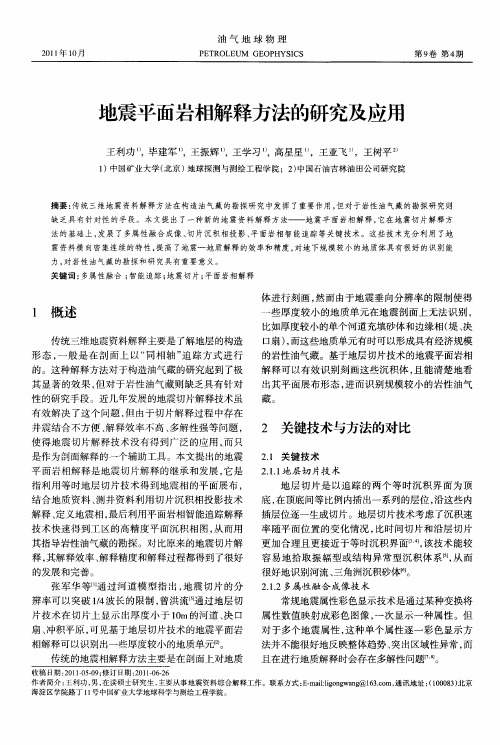
释方式等方面与传统剖面解释都不 同。表 2 是其与 传统剖面解释的对 比。
表 1地震平面岩相解释和地震切片解释对 比
表 2地震平面岩相解释与传统剖面解释对比
实施 的具体步骤主要体现在以下几方面 : ①根
3 流程 及应用
31 基 本 流 程 .
据钻井资料 、 录井资料和测井资料分析得出工 区所
使得 地 震 切 片解 释技 术 没 有得 到 广 泛 的应 用 , 只 而 是作 为剖 面解 释 的一个辅 助 工具 。本 文提 出的地 震 平 面岩 相 解 释是 地震 切 片解 释 的继 承 和发 展 , 是 它
21 关键 技 术 .
211 ..地层 切 片技 术
2 关键技术与方法的对比
密, 因而具有较高的可信度。 22 方法 对 比 .
221 ..地震 平 面 岩相 解释 与 切 片解释 的 比较 地震 平 面岩相 解 释是地 震切 片解 释 的继 承 和发
用计算机采用一定算法智能追踪平面地震相 , 并在 地质沉积模式 的指导下 , 根据井点切片沉积相来定 义平面地震相 , 这样就可以高效准确地得到整个工 区的平面岩相分布图。相对于原来的进行切片地震 相 解 释时所 采用 纯手 工勾 画解 释 , 技术 更加 精细 、 该
处 的沉 积 环境 及 沉积 模 式 , 后在 此 基 础上 确 定单 然
基 于地 层切 片技 术 的地 震平 面岩 相解 释有 两个
井相 ; ②由单井相进行联井分析, 进而建立联井层序 格架 ; ③在层序格架模型的基础上生成地层切片 , 然
后进行属性 的提取 、 优选 、 融合成像 ; ④利用切片沉 积相投影技术实现地震相的标定 , 从而在沉积模式 的指导下将地震 相得平 面展 布转化成平 面岩相分 布。 其流程 图如图3 所示 。
地震相解释和构造解释
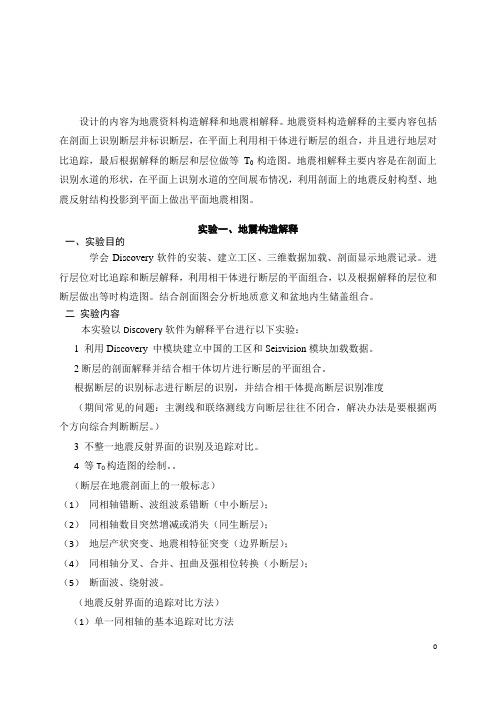
设计的内容为地震资料构造解释和地震相解释。
地震资料构造解释的主要内容包括在剖面上识别断层并标识断层,在平面上利用相干体进行断层的组合,并且进行地层对比追踪,最后根据解释的断层和层位做等T0构造图。
地震相解释主要内容是在剖面上识别水道的形状,在平面上识别水道的空间展布情况,利用剖面上的地震反射构型、地震反射结构投影到平面上做出平面地震相图。
实验一、地震构造解释一、实验目的学会Discovery软件的安装、建立工区、三维数据加载、剖面显示地震记录。
进行层位对比追踪和断层解释,利用相干体进行断层的平面组合,以及根据解释的层位和断层做出等时构造图。
结合剖面图会分析地质意义和盆地内生储盖组合。
二实验内容本实验以Discovery软件为解释平台进行以下实验:1 利用Discovery 中模块建立中国的工区和Seisvision模块加载数据。
2断层的剖面解释并结合相干体切片进行断层的平面组合。
根据断层的识别标志进行断层的识别,并结合相干体提高断层识别准度(期间常见的问题:主测线和联络测线方向断层往往不闭合,解决办法是要根据两个方向综合判断断层。
)3 不整一地震反射界面的识别及追踪对比。
4 等T0构造图的绘制。
(断层在地震剖面上的一般标志)(1)同相轴错断、波组波系错断(中小断层);(2)同相轴数目突然增减或消失(同生断层);(3)地层产状突变、地震相特征突变(边界断层);(4)同相轴分叉、合并、扭曲及强相位转换(小断层);(5)断面波、绕射波。
(地震反射界面的追踪对比方法)(1)单一同相轴的基本追踪对比方法★反射波同相轴具线状廷伸特征,相邻记录道的同一同相轴应为一连续的曲线,相邻界面的同相轴应大体平行。
★相邻记录道同一界面反射波同相轴波形特征相似,即振幅、周期、相位数等相似,它们在空间上是逐渐地变化的。
(2)根据波组或波系进行地震反射界面对比★波组是相邻若干个界面形成的多个强反射同相轴的组合。
波组之间是一些振幅比较弱的同相轴,★多个波组组成一个波系。
地震震源深度定位研究的现状与展望
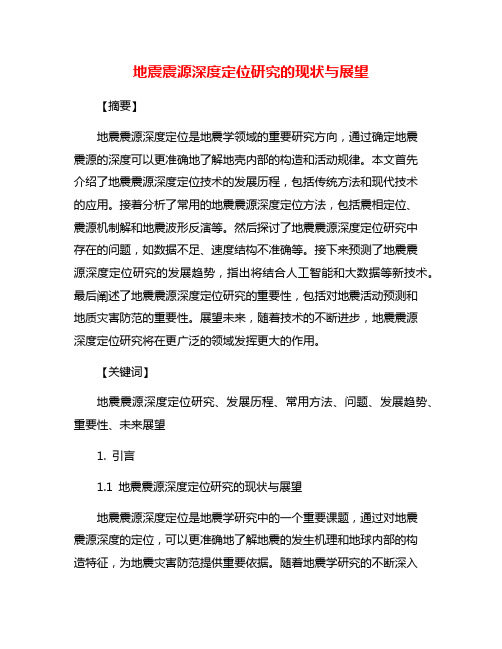
地震震源深度定位研究的现状与展望【摘要】地震震源深度定位是地震学领域的重要研究方向,通过确定地震震源的深度可以更准确地了解地壳内部的构造和活动规律。
本文首先介绍了地震震源深度定位技术的发展历程,包括传统方法和现代技术的应用。
接着分析了常用的地震震源深度定位方法,包括震相定位、震源机制解和地震波形反演等。
然后探讨了地震震源深度定位研究中存在的问题,如数据不足、速度结构不准确等。
接下来预测了地震震源深度定位研究的发展趋势,指出将结合人工智能和大数据等新技术。
最后阐述了地震震源深度定位研究的重要性,包括对地震活动预测和地质灾害防范的重要性。
展望未来,随着技术的不断进步,地震震源深度定位研究将在更广泛的领域发挥更大的作用。
【关键词】地震震源深度定位研究、发展历程、常用方法、问题、发展趋势、重要性、未来展望1. 引言1.1 地震震源深度定位研究的现状与展望地震震源深度定位是地震学研究中的一个重要课题,通过对地震震源深度的定位,可以更准确地了解地震的发生机理和地球内部的构造特征,为地震灾害防范提供重要依据。
随着地震学研究的不断深入和地震监测技术的不断发展,地震震源深度定位技术也得到了长足的发展。
在当前的地震震源深度定位研究中,各种不同的方法和技术被广泛应用。
从传统的地震波形分析到现代的全波形反演技术,地震学家们在地震震源深度定位方面取得了一系列重要成果。
在研究中还存在一些问题,比如地壳介质的复杂性、数据质量的提升等方面仍然需要进一步研究和改进。
未来,随着地震学研究的深入和技术的不断创新,地震震源深度定位研究将迎来更大的发展机遇。
我们可以通过结合多种技术手段,提高数据处理和模型计算的精度,进一步完善地震震源深度定位方法,为地震监测和预警系统的建设提供更可靠的支持。
地震震源深度定位研究的未来将更加重要和有意义。
2. 正文2.1 地震震源深度定位技术的发展历程地震震源深度定位技术的发展历程可以追溯到20世纪初。
最初,科学家主要依靠地面观测台网来确定地震的震源位置和深度。
地震复合属性-地震属性提取与解释新方法
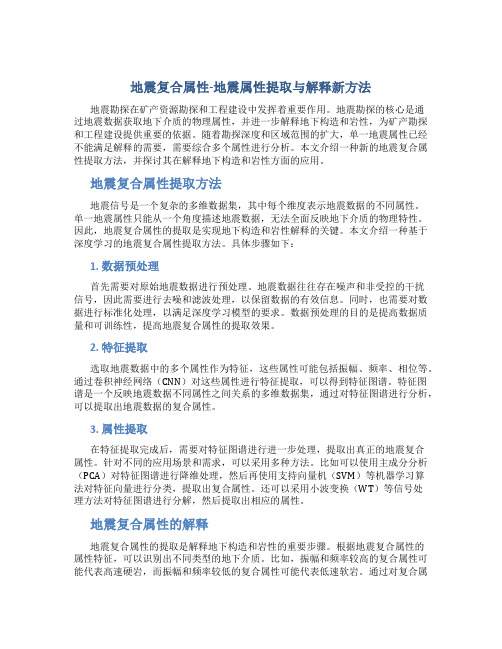
地震复合属性-地震属性提取与解释新方法地震勘探在矿产资源勘探和工程建设中发挥着重要作用。
地震勘探的核心是通过地震数据获取地下介质的物理属性,并进一步解释地下构造和岩性,为矿产勘探和工程建设提供重要的依据。
随着勘探深度和区域范围的扩大,单一地震属性已经不能满足解释的需要,需要综合多个属性进行分析。
本文介绍一种新的地震复合属性提取方法,并探讨其在解释地下构造和岩性方面的应用。
地震复合属性提取方法地震信号是一个复杂的多维数据集,其中每个维度表示地震数据的不同属性。
单一地震属性只能从一个角度描述地震数据,无法全面反映地下介质的物理特性。
因此,地震复合属性的提取是实现地下构造和岩性解释的关键。
本文介绍一种基于深度学习的地震复合属性提取方法。
具体步骤如下:1. 数据预处理首先需要对原始地震数据进行预处理。
地震数据往往存在噪声和非受控的干扰信号,因此需要进行去噪和滤波处理,以保留数据的有效信息。
同时,也需要对数据进行标准化处理,以满足深度学习模型的要求。
数据预处理的目的是提高数据质量和可训练性,提高地震复合属性的提取效果。
2. 特征提取选取地震数据中的多个属性作为特征,这些属性可能包括振幅、频率、相位等。
通过卷积神经网络(CNN)对这些属性进行特征提取,可以得到特征图谱。
特征图谱是一个反映地震数据不同属性之间关系的多维数据集,通过对特征图谱进行分析,可以提取出地震数据的复合属性。
3. 属性提取在特征提取完成后,需要对特征图谱进行进一步处理,提取出真正的地震复合属性。
针对不同的应用场景和需求,可以采用多种方法。
比如可以使用主成分分析(PCA)对特征图谱进行降维处理,然后再使用支持向量机(SVM)等机器学习算法对特征向量进行分类,提取出复合属性。
还可以采用小波变换(WT)等信号处理方法对特征图谱进行分解,然后提取出相应的属性。
地震复合属性的解释地震复合属性的提取是解释地下构造和岩性的重要步骤。
根据地震复合属性的属性特征,可以识别出不同类型的地下介质。
地震预测算法及模型的研究
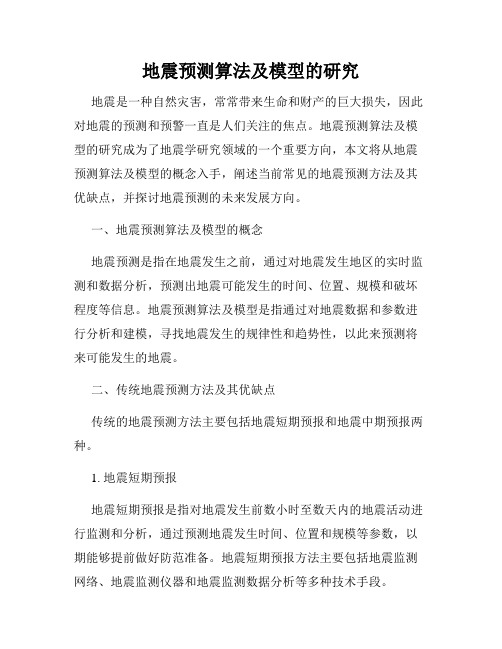
地震预测算法及模型的研究地震是一种自然灾害,常常带来生命和财产的巨大损失,因此对地震的预测和预警一直是人们关注的焦点。
地震预测算法及模型的研究成为了地震学研究领域的一个重要方向,本文将从地震预测算法及模型的概念入手,阐述当前常见的地震预测方法及其优缺点,并探讨地震预测的未来发展方向。
一、地震预测算法及模型的概念地震预测是指在地震发生之前,通过对地震发生地区的实时监测和数据分析,预测出地震可能发生的时间、位置、规模和破坏程度等信息。
地震预测算法及模型是指通过对地震数据和参数进行分析和建模,寻找地震发生的规律性和趋势性,以此来预测将来可能发生的地震。
二、传统地震预测方法及其优缺点传统的地震预测方法主要包括地震短期预报和地震中期预报两种。
1. 地震短期预报地震短期预报是指对地震发生前数小时至数天内的地震活动进行监测和分析,通过预测地震发生时间、位置和规模等参数,以期能够提前做好防范准备。
地震短期预报方法主要包括地震监测网络、地震监测仪器和地震监测数据分析等多种技术手段。
优点:地震短期预报能够在地震发生前的几个小时或几天预测地震的发生时间和位置,为防范措施的制定和实施提供充分的时间。
缺点:地震短期预报方法的预测准确率不高,同时也存在虚假报警的可能,会造成不必要的社会恐慌和经济损失。
2. 地震中期预报地震中期预报是指对地震发生前数日至数月的地震活动进行监测和分析,通过预测地震发生的时间、位置和规模等参数,为预防和减轻地震灾害提供科学依据和技术支撑。
优点:地震中期预报能够给出在未来数月内地震可能发生的时间和位置,并可以对震级做出一定的预测。
对于一些具有较高严重性的地震事件,地震中期预报可以为防灾救灾提供重要的决策依据。
缺点:地震中期预报的预测精度受到很多因素的影响,如地震活动的复杂性,预测时间跨度的限制等。
因此,地震中期预报尚不能对地震的发生时间和规模作出准确的预测和预报。
三、新型地震预测方法及其优势1. 基于机器学习的地震预测方法机器学习技术基于数据挖掘和人工智能的理论,可以从大量的地震数据中学习地震发生规律和趋势,以此来预测未来地震的发生概率和特征。
利用井震结合精细标定层位王硕1张琼2

利用井震结合精细标定层位王硕1 张琼2发布时间:2021-11-04T02:33:29.008Z 来源:基层建设2021年第23期作者:王硕1 张琼2[导读] 准确地标定层位是构造精细解释的基础,通过层位标定建立钻井揭示的地层与地震反射同相轴之间的对应关系,为地震层位的追踪解释和地震相分析提供依据,提高层位标定的准确性1,中国石油渤海钻探工程公司;2,中国石油大港油田分公司摘要:准确地标定层位是构造精细解释的基础,通过层位标定建立钻井揭示的地层与地震反射同相轴之间的对应关系,为地震层位的追踪解释和地震相分析提供依据,提高层位标定的准确性。
选择多井标定,能够大大提高最终标定结果的可靠性。
由于井震精度的差异,必然会造成两者的闭合误差,为了有效地消除或减小这种误差带来的影响,采用了闭合差技术制作合成记录,实现井震标定统一。
关键词:井震结合精细标定层位研究一、测井曲线标准化由于测井仪器的差异、采集年代的不同,或采集条件的影响,必然会造成测井数据的误差和各井之间同一标准层段的数据不统一,造成井震的闭合和各井之间的标定特征误差。
测井曲线标准化就是对测井曲线特别是声波时差曲线,通过调整基值和数据变化范围,使数据达成统一标准,消除系统误差,达到提高标定精度和解释预测精度的目的。
二、层位标定的技术方法—闭合差技术在层位标定的过程中,人工合成地震记录的产生是关键的一步,利用井的声波时差曲线来产生波阻抗,地震波在岩石中的传播速度与介质的弹性性质参数有严格的定量关系。
通过合成地震记录与地震剖面进行对比,从而标定目的层在剖而上位置。
目前,利用地震资料进行油气藏的描述与监控已成为地震勘探技术的主要内容。
由于地面地震与声波测井野外工作方法的不同,使得声波测井所获得的地层速度与地面地震以及VSP技术所获得的速度并不一致,地震资料与声波测井资料存在一定差异。
这突出地表现在由声波测井资料制作的合成记录与实际地震道之间存在一定的相位差。
大地电磁信号处理理论及方法研究
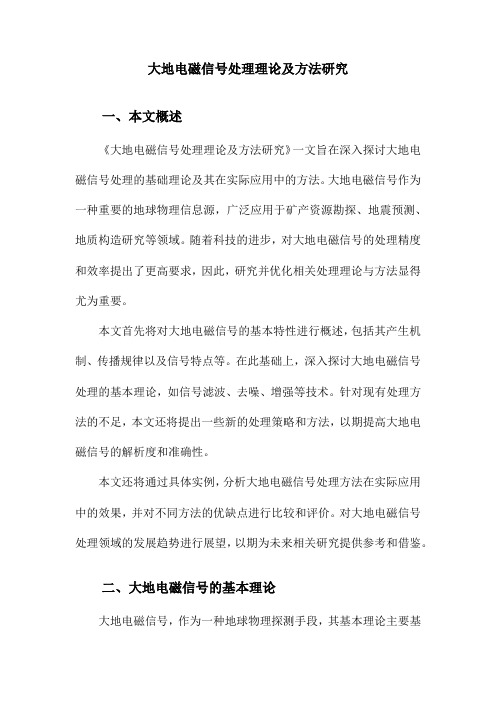
大地电磁信号处理理论及方法研究一、本文概述《大地电磁信号处理理论及方法研究》一文旨在深入探讨大地电磁信号处理的基础理论及其在实际应用中的方法。
大地电磁信号作为一种重要的地球物理信息源,广泛应用于矿产资源勘探、地震预测、地质构造研究等领域。
随着科技的进步,对大地电磁信号的处理精度和效率提出了更高要求,因此,研究并优化相关处理理论与方法显得尤为重要。
本文首先将对大地电磁信号的基本特性进行概述,包括其产生机制、传播规律以及信号特点等。
在此基础上,深入探讨大地电磁信号处理的基本理论,如信号滤波、去噪、增强等技术。
针对现有处理方法的不足,本文还将提出一些新的处理策略和方法,以期提高大地电磁信号的解析度和准确性。
本文还将通过具体实例,分析大地电磁信号处理方法在实际应用中的效果,并对不同方法的优缺点进行比较和评价。
对大地电磁信号处理领域的发展趋势进行展望,以期为未来相关研究提供参考和借鉴。
二、大地电磁信号的基本理论大地电磁信号,作为一种地球物理探测手段,其基本理论主要基于电磁场的基本原理和地球的电导性质。
电磁场理论是物理学中的一个重要领域,描述了电荷和电流在空间中产生的电场和磁场的规律。
大地电磁信号处理,就是对这些由地球内部电流系统产生的电磁场信号进行接收、分析和解释的过程。
大地电磁信号主要来源于地球内部的电流系统,这些电流系统由地壳和地幔中的岩石电导性差异、地热活动、构造运动等多种因素产生。
电流在地球介质中流动时,会产生电场和磁场,这些场在地表可以被接收并测量。
通过测量和分析这些电磁场信号,我们可以获取地球内部的结构和性质信息。
大地电磁信号的测量通常使用大地电磁测深(Magnetotellurics, MT)方法进行。
MT方法通过在地表布置多个电极和磁场传感器,测量电场和磁场的分量,从而得到大地的电阻率和相位信息。
这些信息反映了地球内部电流系统的分布和性质,可以进一步推断出地下的地质构造、岩石分布、地热活动等信息。
地震震源深度定位研究的现状与展望

地震震源深度定位研究的现状与展望【摘要】地震震源深度定位研究是地震学领域的重要研究方向,通过对地震波的传播特征进行分析,可以确定地震发生时的震源深度,为地震预警和灾害减轻提供重要依据。
本文首先介绍了地震震源深度定位的方法与技术,包括地震波形拾取、波速模型建立等内容。
其次回顾了震源深度定位研究的发展历程,探讨了在地震预警中的应用和面临的挑战。
接着讨论了当前研究面临的挑战及解决方案,并展望了未来地震震源深度定位研究的发展方向。
最后强调了地震震源深度定位研究的重要性,展望了未来的发展,并总结了现有研究成果及问题。
通过本文的介绍和讨论,希望能够进一步推动地震震源深度定位研究的发展,为地震防灾减灾工作提供更有力的支持。
【关键词】地震、震源深度、定位、研究、方法、技术、发展历程、地震预警、应用、挑战、解决方案、发展方向、重要性、未来发展、成果、问题。
1. 引言1.1 地震震源深度定位研究的现状与展望地震震源深度定位是地震学领域的重要研究内容,其对于准确评估地震危险性、提高地震预警效率、深化地震机理研究等具有重要意义。
随着地震监测技术的发展和数据处理方法的不断优化,地震震源深度定位的精度和可靠性也在不断提高。
目前,地震震源深度定位的方法与技术主要包括台站定位法、双差定位法、倾斜矩阵法等多种算法。
这些方法结合地震波的传播特性和观测数据,可以有效地对地震震源进行深度定位,并提供重要的地震参数信息。
随着地震震源深度定位研究的不断深入,其在地震预警中的应用也日益广泛。
精准的震源深度定位可以有效提高地震预警的准确性和及时性,为相关部门和公众提供更有效的应急响应措施。
地震震源深度定位仍面临诸多挑战,如地震波在地球内部传播路径复杂、观测台站分布不均匀等问题。
为解决这些挑战,未来的研究可探索深度学习算法、地震波形反演技术等新方法,进一步提升地震震源深度定位的精度和稳定性。
在未来的发展中,地震震源深度定位研究将继续深化,为地震灾害防范和地震预警体系的建设做出更大贡献。
地震预测中的机器学习算法分析
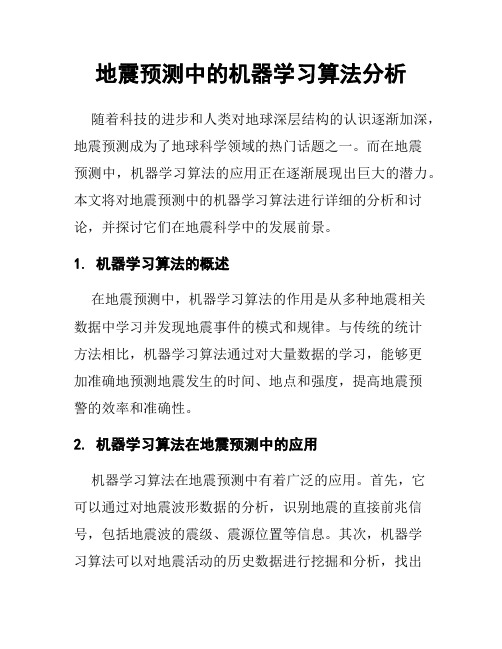
地震预测中的机器学习算法分析随着科技的进步和人类对地球深层结构的认识逐渐加深,地震预测成为了地球科学领域的热门话题之一。
而在地震预测中,机器学习算法的应用正在逐渐展现出巨大的潜力。
本文将对地震预测中的机器学习算法进行详细的分析和讨论,并探讨它们在地震科学中的发展前景。
1. 机器学习算法的概述在地震预测中,机器学习算法的作用是从多种地震相关数据中学习并发现地震事件的模式和规律。
与传统的统计方法相比,机器学习算法通过对大量数据的学习,能够更加准确地预测地震发生的时间、地点和强度,提高地震预警的效率和准确性。
2. 机器学习算法在地震预测中的应用机器学习算法在地震预测中有着广泛的应用。
首先,它可以通过对地震波形数据的分析,识别地震的直接前兆信号,包括地震波的震级、震源位置等信息。
其次,机器学习算法可以对地震活动的历史数据进行挖掘和分析,找出与地震发生相关的因素,例如地壳应力变化、地表形变等。
此外,机器学习还可以通过对地震相关数据的综合分析,建立地震预测模型,预测未来地震的可能性和发生时间。
3. 常用的机器学习算法在地震预测中的应用在地震预测中,常用的机器学习算法包括支持向量机(SVM)、决策树和深度学习等。
支持向量机通过寻找一个最优的超平面,将不同类别的地震事件分隔开来,从而进行地震事件的分类和预测。
决策树算法通过构建一个由决策节点和叶节点构成的树状结构,根据特征属性进行判断和预测。
深度学习算法则通过构建多层神经网络,从地震数据中学习并提取地震发生的规律,实现地震的预测和预警。
4. 特征选择和数据处理在地震预测中,特征选择和数据处理是机器学习算法的关键步骤。
特征选择是指从大量的地震相关数据中选择出最具代表性和预测性的特征,以提高模型的准确性和效率。
数据处理包括数据清洗、数据标准化和特征归一化等步骤,以确保模型的训练和预测结果的可靠性和稳定性。
5. 算法的性能评估和验证在地震预测中,对机器学习算法的性能进行评估和验证是非常重要的。
基于深度学习算法的地震损害预测模型
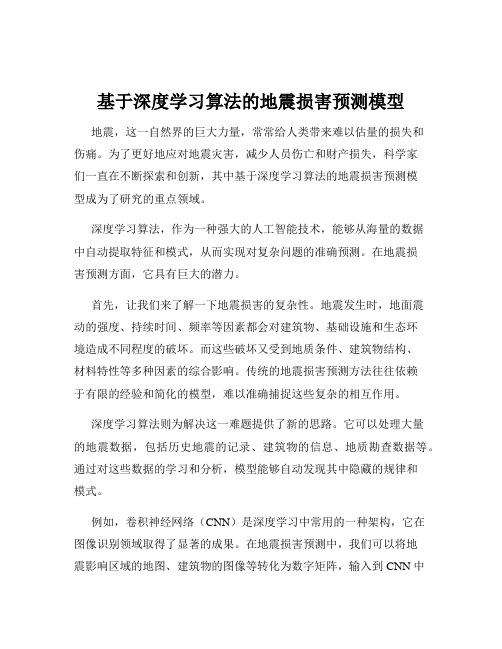
基于深度学习算法的地震损害预测模型地震,这一自然界的巨大力量,常常给人类带来难以估量的损失和伤痛。
为了更好地应对地震灾害,减少人员伤亡和财产损失,科学家们一直在不断探索和创新,其中基于深度学习算法的地震损害预测模型成为了研究的重点领域。
深度学习算法,作为一种强大的人工智能技术,能够从海量的数据中自动提取特征和模式,从而实现对复杂问题的准确预测。
在地震损害预测方面,它具有巨大的潜力。
首先,让我们来了解一下地震损害的复杂性。
地震发生时,地面震动的强度、持续时间、频率等因素都会对建筑物、基础设施和生态环境造成不同程度的破坏。
而这些破坏又受到地质条件、建筑物结构、材料特性等多种因素的综合影响。
传统的地震损害预测方法往往依赖于有限的经验和简化的模型,难以准确捕捉这些复杂的相互作用。
深度学习算法则为解决这一难题提供了新的思路。
它可以处理大量的地震数据,包括历史地震的记录、建筑物的信息、地质勘查数据等。
通过对这些数据的学习和分析,模型能够自动发现其中隐藏的规律和模式。
例如,卷积神经网络(CNN)是深度学习中常用的一种架构,它在图像识别领域取得了显著的成果。
在地震损害预测中,我们可以将地震影响区域的地图、建筑物的图像等转化为数字矩阵,输入到 CNN 中进行训练。
CNN 能够自动提取图像中的特征,如建筑物的形状、布局、结构等,从而预测它们在地震中的受损程度。
循环神经网络(RNN)及其变体,如长短期记忆网络(LSTM)和门控循环单元(GRU),则适用于处理时间序列数据。
地震震动的过程是一个随时间变化的动态过程,RNN 系列的算法可以很好地捕捉这种时间上的依赖关系。
例如,通过输入地震波的时间序列数据,模型可以预测建筑物在不同时刻的响应和可能的损害情况。
为了构建一个有效的地震损害预测模型,数据的收集和预处理是至关重要的第一步。
我们需要收集各种与地震和建筑物相关的数据,包括地震的震级、震源深度、震中位置、建筑物的类型、年龄、高度、结构材料、基础类型等。
“地震资料地质解释”课程实践教学平台设计和构建
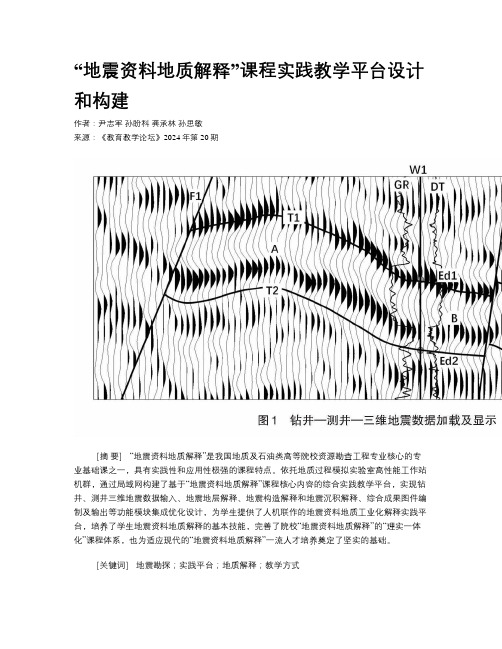
“地震资料地质解释”课程实践教学平台设计和构建作者:尹志军孙盼科龚承林孙思敏来源:《教育教学论坛》2024年第20期[摘要]“地震资料地质解释”是我国地质及石油类高等院校资源勘查工程专业核心的专业基础课之一,具有实践性和应用性极强的课程特点。
依托地质过程模拟实验室高性能工作站机群,通过局域网构建了基于“地震资料地质解释”课程核心内容的综合实践教学平台,实现钻井、测井三维地震数据输入、地震地层解释、地震构造解释和地震沉积解释、综合成果图件编制及输出等功能模块集成优化设计,为学生提供了人机联作的地震资料地质工业化解释实践平台,培养了学生地震资料地质解释的基本技能,完善了院校“地震资料地质解释”的“理实一体化”课程体系,也为适应现代的“地震资料地质解释”一流人才培养奠定了坚实的基础。
[关键词]地震勘探;实践平台;地质解释;教学方式[基金项目] 2021年度中国石油大学(北京)一流课程建设项目“‘地震资料地质解释’实践教学平台建设”(XM10720210164)[作者简介]尹志军(1971—),男,四川武胜人,博士,中国石油大学(北京)地球科学学院副教授,主要从事油气田开发地质研究。
[中图分类号] G642.0 [文献标识码] A [文章编号] 1674-9324(2024)20-0018-04 [收稿日期] 2023-04-20引言地震勘探是利用地下岩层弹性和密度的差异,通过接收和处理地面人工激发地震波在地层中传播的运动学和波动学参数,推断地下岩层的性质和形态的地球物理勘探方法。
地震资料地质解释就是基于地震勘探获取的地震波信息,系统开展地层层序、构造断裂系统、沉积储层的综合解释,是确定有利的油气成藏条件和远景富集区的关键技术[1],是油田勘探开发技术人员必须熟练掌握的专业技能,因此国内外地质及石油高等院校一直将“地震资料地质解释”设置为地质工程、资源勘查工程等专业本科生的必修专业基础课。
“地震资料地质解释”课程的教学内容决定了其应用性和实践性强的特点[2],国内石油、地质院校的众多教师对于“地震资料地质解释”课程教学内容、教学方式进行了深入探索[2-7],创建了地震资料地质解释实践教学平台,对于学生加深地震资料地质解释原理的理解、培养学生地震资料地质解释的基本技能至关重要[8]。
地震相分析
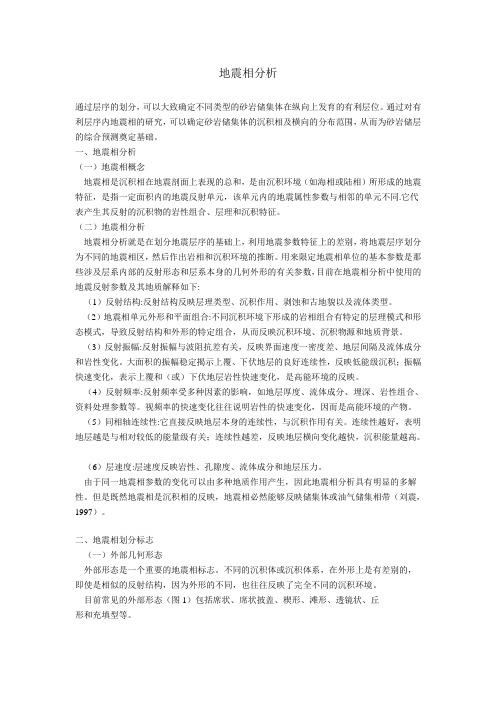
地震相分析通过层序的划分,可以大致确定不同类型的砂岩储集体在纵向上发育的有利层位。
通过对有利层序内地震相的研究,可以确定砂岩储集体的沉积相及横向的分布范围,从而为砂岩储层的综合预测奠定基础。
一、地震相分析(一)地震相概念地震相是沉积相在地震剖面上表现的总和,是由沉积环境(如海相或陆相)所形成的地震特征,是指一定面积内的地震反射单元,该单元内的地震属性参数与相邻的单元不同.它代表产生其反射的沉积物的岩性组合、层理和沉积特征。
(二)地震相分析地震相分析就是在划分地震层序的基础上,利用地震参数特征上的差别,将地震层序划分为不同的地震相区,然后作出岩相和沉积环境的推断。
用来限定地震相单位的基本参数是那些涉及层系内部的反射形态和层系本身的几何外形的有关参数,目前在地震相分析中使用的地震反射参数及其地质解释如下:(1)反射结构:反射结构反映层理类型、沉积作用、剥蚀和古地貌以及流体类型。
(2)地震相单元外形和平面组合:不同沉积环境下形成的岩相组合有特定的层理模式和形态模式,导致反射结构和外形的特定组合,从而反映沉积环境、沉积物源和地质背景。
(3)反射振幅:反射振幅与波阻抗差有关,反映界面速度一密度差、地层间隔及流体成分和岩性变化。
大面积的振幅稳定揭示上覆、下伏地层的良好连续性,反映低能级沉积;振幅快速变化,表示上覆和(或)下伏地层岩性快速变化,是高能环境的反映。
(4)反射频率:反射频率受多种因素的影响,如地层厚度、流体成分、埋深、岩性组合、资料处理参数等。
视频率的快速变化往往说明岩性的快速变化,因而是高能环境的产物。
(5)同相轴连续性:它直接反映地层本身的连续性,与沉积作用有关。
连续性越好,表明地层越是与相对较低的能量级有关;连续性越差,反映地层横向变化越快,沉积能量越高。
(6)层速度:层速度反映岩性、孔隙度、流体成分和地层压力。
由于同一地震相参数的变化可以由多种地质作用产生,因此地震相分析具有明显的多解性。
三维地震精细构造解释
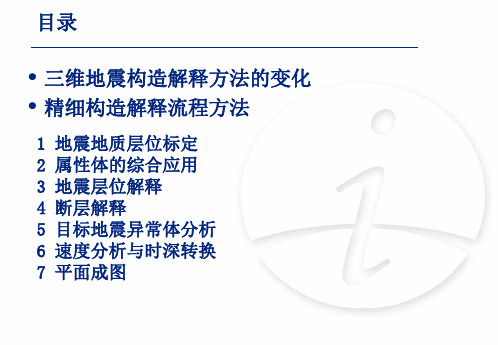
三维地震构造解释方法的变化 精细构造解释流程方法
1 地震地质层位标定 2 属性体的综合应用 3 地震层位解释 4 断层解释 5 目标地震异常体分析 6 速度分析与时深转换 7 平面成图
以地震资料为主的油藏描述需求
在勘探油藏描述过程中,油藏的圈闭形态、储层及油气层平面及空间展布 特征、属性特征都是以地震资料为主进行描述的,地震资料起了重要作用。
Half Window半时窗长度,指在半自动、自动拾取层位时,互
相关所用的半时窗长度,单位为毫秒,缺省值为20毫秒。
Landmark自动追踪方法
振幅追踪器--选取振幅作为追踪属性,是最常用
的自动追踪方式 ▪ 振幅的属性类型由用户确定。它包括:最小(波谷)、
零点、最大(波峰)、0(+/-)和0(-/+)。
性 测试 平面图
多井处理
可分辨 单薄层
顶底时差 振幅调谐
砂岩速度
砂岩 厚度
薄互层 微相顶底 砂泥速度
砂含量
地震参数提取
参数综合判别 地震反演参数
分布边界 有利范围
特征参数体层切片 特征参数平剖面图
油藏描述框图(续)
油
气 水
录井 显示
描述 测试
结果
油藏 地质 模型
储量
计算
高压物性
流体性质 油水边界
原油比重 体积系数
地震正演 模型
开发阶段对地震解释的要求
相应于开发阶段的生产需求,地震解释任务要求发生了
以下变化: ▪ 从以地震为主(井辅助地震)到井解释为主(地震辅助井) ▪ 从宏观到微观,研究对象从储集体到储集层、从大层到小层、
从油层组到油层
▪ 逐步精化,从相到微相、从层序到旋回, ▪ 从静态到动态,研究构造、储层到研究流体、剩余油分布
地震波形识别技术的研究与应用

地震波形识别技术的研究与应用地震波形识别是地震学领域中的一个重要研究方向,也是震源机制研究、地震预报、震源定位等方面的基础工作。
地震波形识别技术的研究旨在将地震波形数据转换为有用的信息,提高地震预测和监测的准确性和可靠性。
一、地震波形的特征地震波形是指地震产生的波形信号。
在地震波形中,包含了前、中、后三个阶段的信息。
前阶段的波形为短周期振动,发生于地震瞬间,又称为地震初动;中阶段的波形为长周期振动,称为主震波;后阶段的波形为长周期振动,称为余震波。
地震波形的特征可以从频域和时域两个方面来描述。
频域特征包括频率、频谱、谱宽等;时域特征包括振幅、振幅谱、相位等。
在实际应用中,主要从时域特征入手,确定地震波形的类型和参数。
二、地震波形识别技术的研究地震波形识别技术的研究是针对地震波形进行类别识别和参数分析,以便更好地理解地震波形产生的物理过程和地震的发生过程。
1. 类别识别地震波形的分类包括地震类型识别、地震震级识别、地震深度识别等。
常见的分类算法包括传统的统计分类算法和人工神经网络算法等。
2. 参数分析地震波形的参数分析主要包括振幅、振幅谱、相位等参数的计算和分析。
参数分析包括时域分析、频域分析、小波分析等。
3. 研究方向未来地震波形识别技术的研究方向包括深度学习方法、多模态数据融合、大数据分析等。
其中,深度学习方法是近年来新兴的一种分类方法,它可以自动提取特征并进行分类。
三、地震波形识别技术的应用地震波形识别技术的应用广泛,主要包括地震预报、震源机制研究、地震监测和震源定位等方面。
1. 地震预报地震波形识别技术可以通过识别地震波形的类型和参数,帮助预测地震发生的可能性,提高地震预测的准确性。
同时,地震波形识别技术还可以为地震预报提供更为直接的证据。
2. 震源机制研究地震波形识别技术可以帮助确定地震的震源机制,为地震机理研究提供可靠数据。
在这方面,地震波形识别技术可以结合地震烈度和地形等其他因素,并分析地震波形特征,对震源机制进行判断和分析。
- 1、下载文档前请自行甄别文档内容的完整性,平台不提供额外的编辑、内容补充、找答案等附加服务。
- 2、"仅部分预览"的文档,不可在线预览部分如存在完整性等问题,可反馈申请退款(可完整预览的文档不适用该条件!)。
- 3、如文档侵犯您的权益,请联系客服反馈,我们会尽快为您处理(人工客服工作时间:9:00-18:30)。
地震相分析和层位解释的深度学习方法研究地震相分析和层位解释的深度学习方法研究摘要地震相分析和层位解释是地球物理学研究中的重要课题,对于地质构造解释和油气勘探具有重要的意义。
本文提出了一种基于深度学习的地震相分析和层位解释方法,结合卷积神经网络和循环神经网络实现了从地震道数据中自动提取特征和解释地层信息。
首先,提取地震道数据的时间序列特征;其次,使用卷积神经网络进行特征提取,得到抽象的高层次特征表示;然后,使用循环神经网络对特征进行序列建模,进一步提高准确性和鲁棒性。
通过在公开数据集上的测试,验证了本方法的有效性,与传统方法相比,本方法能够更加准确地识别地震相,提升层位解释的精度和效率。
关键词: 地震相分析,层位解释,深度学习,卷积神经网络,循环神经网络AbstractSeismic phase analysis and stratigraphic interpretation are important topics in geophysical research and have significant implications forgeological structure interpretation and oil and gas exploration. This paper proposes a deep learning-based method for seismic phase analysis and stratigraphic interpretation, which combines convolutional neural networks and recurrent neural networks to automatically extract features from seismic data and interpret stratigraphic information. First, the time series features of seismic data are extracted. Second, convolutional neural networks are used for feature extraction to obtain abstract high-level feature representations. Then, the recurrent neural network is used for sequence modeling of features to further improve accuracy and robustness. Through tests on public datasets, the effectiveness of this method has been validated, and compared with traditional methods, this approach can more accurately identify seismic phases and improve the precision and efficiency of stratigraphic interpretation.Keywords: Seismic phase analysis, Stratigraphic interpretation, Deep learning, Convolutional neural networks, Recurrent neural networks。
Seismic phase analysis is a critical component of seismic data processing, which involves identifying different seismic phases from recorded data to understand the geological structure of the subsurface.However, this task can be challenging due to the complexity of the data and the presence of noise and other factors that may interfere with the signals. To address these challenges, researchers have explored various methods of analyzing seismic phases, including traditional techniques such as manual interpretation and hand-crafted feature extraction, as well as more advanced machine learning approaches.Recently, deep learning techniques, such as convolutional neural networks (CNNs) and recurrent neural networks (RNNs), have shown promise for improving the accuracy and efficiency of seismic phase analysis. CNNs are commonly used for image recognition, but they can also be applied to the analysis of time-series data such as seismic signals. By using a series of convolutional layers to automatically learnfeatures from the data, CNNs can effectively identify different seismic phases and improve the accuracy of seismic interpretation.RNNs, on the other hand, are specialized for the analysis of sequential data, making them well-suitedfor sequence modeling in seismic analysis. Byanalyzing the temporal relationships among different features, RNNs can improve the accuracy of seismic phase identification and enable more precisestratigraphic interpretation.Through tests on public datasets, researchers have demonstrated the effectiveness of deep learning for seismic phase analysis and stratigraphic interpretation. Compared to traditional techniques, deep learning approaches have shown higher accuracyand better performance in identifying seismic phases and interpreting stratigraphic data. As a result,these methods have the potential to significantly improve the efficiency and accuracy of seismic data analysis, making it easier to extract valuableinsights from these complex datasets。
Furthermore, deep learning methods have also been applied to seismic waveform inversion, a key component of subsurface imaging and reservoir characterization. By training a neural network to learn the relationship between input waveforms and the corresponding subsurface properties, waveform inversion can becarried out much more efficiently and accurately than traditional methods such as full waveform inversion. This has the potential to greatly reduce computation time and increase the reliability of subsurface models, leading to better decision making in oil and gas exploration and production.In addition, deep learning approaches have also been used for fault detection and prediction. Faults are geological structures that can lead to oil and gas traps, but can also pose hazards for drilling and production operations. By analyzing seismic data for patterns that are indicative of fault zones, deep learning algorithms can help identify potential locations of faults and assess their likelihood of being active or inactive. This can greatly assist in optimizing drilling locations and minimizing the risk of drilling into hazardous zones.Overall, the application of deep learning in the field of seismic data analysis holds great promise for improving our understanding of the subsurface and making more informed decisions in the exploration and production of oil and gas resources. However, there are still challenges that need to be addressed, such as the need for large amounts of high-quality training data and the potential for overfitting. Nevertheless, as technology continues to advance and more data becomes available, it is likely that deep learning methods will become increasingly important in the oil and gas industry。