Chen_Accurate_and_Robust_2013_ICCV_paper
基于改进YOLOv5的皮革抓取点识别及定位
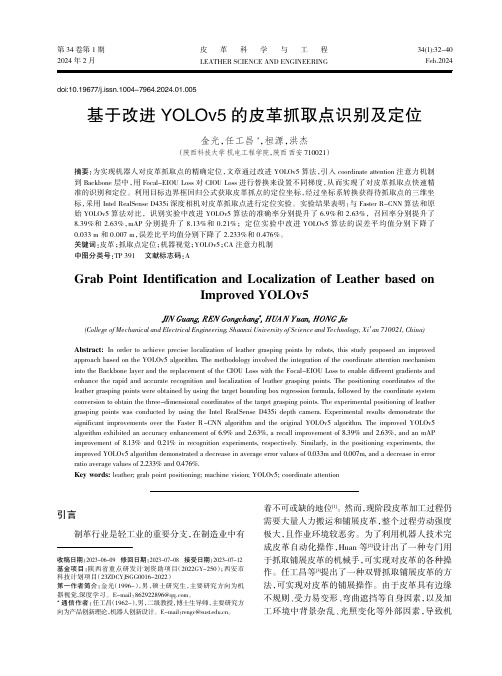
doi:10.19677/j.issn.1004-7964.2024.01.005基于改进YOLOv5的皮革抓取点识别及定位金光,任工昌*,桓源,洪杰(陕西科技大学机电工程学院,陕西西安710021)摘要:为实现机器人对皮革抓取点的精确定位,文章通过改进YOLOv5算法,引入coordinate attention 注意力机制到Backbone 层中,用Focal-EIOU Loss 对CIOU Loss 进行替换来设置不同梯度,从而实现了对皮革抓取点快速精准的识别和定位。
利用目标边界框回归公式获取皮革抓点的定位坐标,经过坐标系转换获得待抓取点的三维坐标,采用Intel RealSense D435i 深度相机对皮革抓取点进行定位实验。
实验结果表明:与Faster R-CNN 算法和原始YOLOv5算法对比,识别实验中改进YOLOv5算法的准确率分别提升了6.9%和2.63%,召回率分别提升了8.39%和2.63%,mAP 分别提升了8.13%和0.21%;定位实验中改进YOLOv5算法的误差平均值分别下降了0.033m 和0.007m,误差比平均值分别下降了2.233%和0.476%。
关键词:皮革;抓取点定位;机器视觉;YOLOv5;CA 注意力机制中图分类号:TP 391文献标志码:AGrab Point Identification and Localization of Leather based onImproved YOLOv5(College of Mechanical and Electrical Engineering,Shaanxi University of Science and Technology,Xi ’an 710021,China)Abstract:In order to achieve precise localization of leather grasping points by robots,this study proposed an improved approach based on the YOLOv5algorithm.The methodology involved the integration of the coordinate attention mechanism into the Backbone layer and the replacement of the CIOU Loss with the Focal-EIOU Loss to enable different gradients and enhance the rapid and accurate recognition and localization of leather grasping points.The positioning coordinates of the leather grasping points were obtained by using the target bounding box regression formula,followed by the coordinate system conversion to obtain the three-dimensional coordinates of the target grasping points.The experimental positioning of leather grasping points was conducted by using the Intel RealSense D435i depth camera.Experimental results demonstrate the significant improvements over the Faster R -CNN algorithm and the original YOLOv5algorithm.The improved YOLOv5algorithm exhibited an accuracy enhancement of 6.9%and 2.63%,a recall improvement of 8.39%and 2.63%,and an mAP improvement of 8.13%and 0.21%in recognition experiments,respectively.Similarly,in the positioning experiments,the improved YOLOv5algorithm demonstrated a decrease in average error values of 0.033m and 0.007m,and a decrease in error ratio average values of 2.233%and 0.476%.Key words:leather;grab point positioning;machine vision;YOLOv5;coordinate attention收稿日期:2023-06-09修回日期:2023-07-08接受日期:2023-07-12基金项目:陕西省重点研发计划资助项目(2022GY-250);西安市科技计划项目(23ZDCYJSGG0016-2022)第一作者简介:金光(1996-),男,硕士研究生,主要研究方向为机器视觉,深度学习。
[2015]Chen_Similarity_Learning_on_2015_CVPR_paper
![[2015]Chen_Similarity_Learning_on_2015_CVPR_paper](https://img.taocdn.com/s3/m/64685ed7aa00b52acfc7cabd.png)
person image via covariance descriptors that is robust to illumination change and background variation, while Zhao et al. [38] learn the distinct salience feature to distinguish the correct matched person from others. Farenzena et al. [9] further consider symmetric and asymmetric prior of human body, to integrate different local feature from different body parts. Cheng et al. [5] employ pre-learned pictorial structure model to more accurately localize the body parts. In contrast, methods that focus on similarity learning usually extract the features in a more straightforward way: most of them extract color or textural histograms from predefined image regions in a “block” shape or “strip” shape [36, 14, 17, 29, 20, 41]; some methods further encode the region descriptors to form high level images features [25, 21]. Our method is compatible with both region based features or encoded features. In the similarity measuring step, feature design based methods usually employ off-the-shelf distance metrics, such as Euclidean distance [9], Bhattacharyya distance [5], and covariance distance [24, 1], etc. Meanwhile, how to learn a proper similarity measurement is studied in different perspectives. Gray et al.[12] employ boosting to a select a subset of optimal features for matching. Prosser et al.[31] and Zheng et al.[41] stress the importance of loss function and describe the triplet relation between samples. They don’t compare the direct similarity score between correct matched and incorrect matched pairs, but are only interested in the rank of these scores that reflects how likely they match to a given query image. Recently, Mahalanobis distance learning has been applied for re-identification problem [28, 17, 14, 6], where the distance metrics are optimized in either a discriminative fashion [28, 6] or a generative fashion [17]. As Mahalanobis distance can implicitly model the transition in feature space between two camera views, these methods achieve better performance than the similarity functions directly learnt in the original feature space. Li et al. [21] further extend the metric learning. They proposed the Locally-Adaptive Decision Function (LADF) to jointly models a distance metric and a locally adaptive thresholding rule. In this paper, we focus on the second step and develop a new similarity function. The effectiveness of our similarity function stems from the feature representation, the explicit polynomial kernel feature map of concatenated descriptors of image pairs. The purpose of utilization explicit feature map is distinguished from existing explicit kernel work [33, 27]. They derive explicit feature maps to speed up nonlinear kernel machines, while we utilize the explicit polynomial kernel feature map to characterize the image pairs. With obtained feature, our method can be compared with methods based on patch matching [37, 38]. For each patch, these methods greedily search the corresponding patch in adjacent space and only keep the maximum matching score
遥感图像跨域语义分割的无监督域自适应对齐方法

㊀2023年12月A c t aG e o d a e t i c ae tC a r t o g r a p h i c aS i n i c a D e c e m b e r,2023㊀㊀第52卷㊀第12期测㊀绘㊀学㊀报V o l.52,N o.12引文格式:沈秭扬,倪欢,管海燕.遥感图像跨域语义分割的无监督域自适应对齐方法[J].测绘学报,2023,52(12):2115G2126.D O I:10.11947/j.A G C S.2023.20220483.S H E NZ i y a n g,N I H u a n,G U A N H a i y a n.U n s u p e r v i s e dd o m a i na d a p t a t i o na l i g n m e n t m e t h o df o rc r o s sGd o m a i ns e m a n t i c s e g m e n t a t i o no f r e m o t e s e n s i n g i m a g e s[J].A c t aG e o d a e t i c a e tC a r t o g r a p h i c aS i n i c a,2023,52(12):2115G2126.D O I:10.11947/ j.A G C S.2023.20220483.遥感图像跨域语义分割的无监督域自适应对齐方法沈秭扬,倪㊀欢,管海燕南京信息工程大学遥感与测绘工程学院,江苏南京210044U n s u p e r v i s e d d o m a i n a d a p t a t i o n a l i g n m e n t m e t h o d f o r c r o s sGd o m a i n s e m a n t i c s e g m e n t a t i o no f r e m o t e s e n s i n g i m a g e sS H E NZ i y a n g,N I H u a n,G U A NH a i y a nS c h o o l o fR e m o t eS e n s i n g&G e o m a t i c sE n g i n e e r i n g,N a n j i n g U n i v e r s i t y o f I n f o r m a t i o nS c i e n c e&T e c h n o l o g y, N a n j i n g210044,C h i n aA b s t r a c t:D e e p l e a r n i n g m o d e l s r e l y o na l a r g e n u m b e r o f h o m o g e n o u s l a b e l e d s a m p l e s,i.e.,l i m i t i n g t h e t r a i n i n g a n dt e s t i n g d a t at oo b e y t h es a m ed a t a d i s t r i b u t i o n.H o w e v e r,w h e nf a c i n g l a r g eGs c a l ea n d d i v e r s e r e m o t e s e n s i n g d a t a,i t i s d i f f i c u l t t o g u a r a n t e e t h e r e q u i r e m e n t o f h o m o g e n e o u s d i s t r i b u t i o n a m o n g d a t a,a n dt h es e g m e n t a t i o na c c u r a c y o fd e e p l e a r n i n g m o d e l sd e c r e a s e ss i g n i f i c a n t l y.T o a d d r e s s t h i s p r o b l e m,t h i s p a p e r p r o p o s e s a n u n s u p e r v i s e d d o m a i n a d a p t a t i o n(U D A)m e t h o d f o r s e m a n t i c s e g m e n t a t i o n o f r e m o t e s e n s i n g i m a g e s.W h e n t h e d i s t r i b u t i o n s o f t r a i n i n g d a t a(s o u r c e d o m a i n)a n d t e s t i n g d a t a(t a r g e t d o m a i n)a r ed i f f e r e n t,t h e p r o p o s e dm e t h o d i m p r o v e s t h ea c c u r a c y o f s e m a n t i c s e g m e n t a t i o n i n t h e t a r g e t d o m a i nb y t r a i n i n g d e e p l e a r n i n g m o d e l s u s i n g o n l y s o u r c eGd o m a i n l a b e l s.T h em e t h o d i n t r o d u c e so p t i m a l t r a n s p o r t t h e o r y a n d g l o b a la l i g n m e n t i n i m a g e,f e a t u r ea n do u t p u t s p a c e s t om i t i g a t e t h ed o m a i ns h i f t b e t w e e n t h es o u r c ea n dt a r g e td o m a i n s.T h ee x p e r i m e n t se m p l o y t h eP o t s d a m a n dV a i h i n g e nd a t a s e t s p r o v i d e db y t h e I n t e r n a t i o n a lS o c i e t y f o rP h o t o g r a m m e t r y a n dR e m o t eS e n s i n g(I S P R S)t ov a l i d a t et h e p e r f o r m a n c e.T h er e s u l t ss h o wt h a t t h e m e t h o di nt h i s p a p e ra c h i e v e sh i g h e ra c c u r a c y c o m p a r e d w i t h e x i s t i n g m e t h o d s.B a s e d o nt h e a b l a t i o ns t u d y,t h e e f f e c t i v e n e s so ft h e o p t i m a lt r a n s p o r tt h e o r y i s d e m o n s t r a t e d i n t h eU D A f r a m e w o r k f o r s e m a n t i c s e g m e n t a t i o nd r i v e nb y d e e p l e a r n i n g.K e y w o r d s:r e m o t e s e n s i n g i m a g e r y;s e m a n t i c s e g m e n t a t i o n;d o m a i na d a p t a t i o n;o p t i m a l t r a n s p o r tF o u n d a t i o ns u p p o r t:B e i j i n g K e y L a b o r a t o r y o f A d v a n c e d O p t i c a l R e m o t e S e n s i n g T e c h n o l o g y(N o.A O R S202310);T h eN a t i o n a l N a t u r a l S c i e n c e F o u n d a t i o n o f C h i n a(N o s.41801384;41971414);P o s t g r a d u a t e R e s e a r c h&P r a c t i c e I n n o v a t i o nP r o g r a mo f J i a n g s uP r o v i n c e(N o.K Y C X22_1214)摘㊀要:深度学习模型依赖于大量同源标记样本,即限定训练数据与测试数据服从同一分布.但是,面对大范围㊁多样化的遥感图像时,数据之间的同分布要求难以保证,深度学习模型的分割精度下降明显.针对这一问题,本文提出了一种面向遥感图像语义分割的无监督域自适应方法,在训练数据(源域)与测试数据(目标域)分布不同的情况下,仅利用源域标签训练深度学习模型,提高目标域语义分割精度.本文方法引入最优传输理论,在图像空间㊁特征空间和输出空间进行全局对齐,以减轻源域和目标域之间的域偏移.试验采用国际摄影测量与遥感学会(I S P R S)所提供的P o t s d a m和V a i h i n g e n数据集进行验证.结果表明,相比于现有方法,本文方法取得了更高的分割精度.此外,通过消融分析,在深度学习驱动的语义分割无监督域自适应框架下,证明了最优传输理论的有效性.关键词:遥感图像;语义分割;域自适应;最优传输中图分类号:P237㊀㊀㊀㊀文献标识码:A㊀㊀㊀㊀文章编号:1001G1595(2023)12G2115G12D e c e m b e r 2023V o l .52N o .12A G C Sh t t p :ʊx b .c h i n a s m p .c o m 基金项目:先进光学遥感技术北京市重点实验室开放基金(A O R S 202310);国家自然科学基金(41801384;41971414);江苏省研究生科研与实践创新计划(K Y C X 22_1214)㊀㊀地物分类(语义分割)是遥感地学分析的基础,得到了广泛研究.这些研究引入经典的机器学习方法和深度学习技术,推动了遥感图像语义分割的自动化和实用化.经典的机器学习方法,如支持向量机[1]㊁人工神经网络[2]㊁决策树[3]㊁随机森林[4]及自适应增强[5]等,难以建模深层特征空间的语义信息,难以在遥感图像语义分割任务中取得精度突破.深度学习方法,如卷积神经网络[6G7]㊁图卷积网络[8]㊁T r a n s f o r m e r [9]及多模态融合[10]等,有效建模高层次语义信息,进一步提高了遥感图像语义分割精度.但是,深度学习模型要求用于训练的源域数据与目标域数据间服从同一分布.在成像传感器和地理环境不同时,同分布要求无法满足,即源域和目标域之间存在域偏移,阻碍了深度学习模型的泛化能力.如图1所示,直接将训练好的模型应用于存在域偏移的目标域数据集上,难以取得预期结果[11].因此,如何将模型迁移到存在域偏移的目标域数据集上,是当前遥感领域需要解决的重要问题[12].图1㊀源域模型在源域和目标域的分割结果对比F i g .1㊀T h ec o m p a r i s o nb e t w e e ns e gm e n t a t i o nr e s u l t s p r o d u c e d b y s o u r c e Gd o m a i n m o d e li n s o u r c e a n d t a r ge t d o m a i n s 目前,无监督域自适应是解决域偏移问题的有效方法,仅利用源域标签进行训练,便可得到适用于目标域的语义分割模型.无监督域自适应方法分为两大类[13],即基于差异测度和基于生成对抗网络(ge n e r a t i v ea d v e r s a r i a ln e t s ,G A N )[14]的方法.基于差异测度的方法通过不同测度,如MM D (m a x i m u m m e a nd i s c r e p a n c y )[15G16]㊁C O R A L (c o r r e l a t i o n a l i gn m e n t )[17G18]及C M D (c e n t r a lm o m e n t d i s c r e p a n c y)[19]等,来衡量源域和目标域之间的差异,进而实现差异最小化.基于G A N 的方法根据应用方式的不同,又可分为两个子类.第1类利用G A N 的重构能力,如通过C y c l e G A N [20]㊁C o l o r M a p G A N [21]和R e s i D u a l G A N [22]等方法对源域图像进行风格转换,并对转换后的源域图像进行监督训练,从而缓解域偏移问题;第2类则使用G A N 在特征[23]或输出[24]空间进行对抗学习,并引入实例[25]和类别[26G27]信息,提取稳健的域不变特征.基于G A N 的方法在遥感图像语义分割域自适应任务中应用更为广泛,但由于对抗学习过程的复杂性,G A N 难以同时拓展到多个空间.基于此,本文舍弃G A N 思想,采用基于差异测度的方法,引入最优传输理论,从数学角度构建源域和目标域对齐途径,并充分利用图像㊁特征和输出空间信息.基于最优传输理论的域自适应思想通过减小域间的W a s s e r s t e i n 距离来对齐源域和目标域分布[28].该思想首先利用最优传输,根据目标域特征迁移源域图像,然后对迁移后的源域图像进行监督学习,并引入参考分布[29]㊁空间原型信息[30]和注意力机制[31],提高跨域泛化能力.通过最优传输与域自适应理论的结合,模型能够以一种合理的几何方式衡量源域和目标域的特征分布差异[32G33].但是,目前基于最优传输的域自适应方法主要面向自然图像分类任务,即每一张图像仅对应一个标签,尚无法充分顾及高分遥感图像语义分割任务需求.为弥补以上问题,本文基于最优传输理论,提出一种顾及多空间分布对齐的全局域自适应方法,以解决高分遥感图像语义分割的域偏移问题.本文方法的核心即在图像空间㊁特征空间和输出空间,利用最优传输理论来减轻源域和目标域的分布差异.本文的创新点如下:①将最优传输理论引入遥感图像语义分割域自适应任务,给出了整合最优传输与语义分割域自适应框架的具体方案;②构建了一种基于最优传输的全局域自适应模型,与现有方法相比,进一步减弱了域偏移影响,取得了更高精度.6112第12期沈秭扬,等:遥感图像跨域语义分割的无监督域自适应对齐方法1㊀基于最优传输的无监督域自适应方法本文方法具体分为3个部分:图像空间风格迁移㊁特征空间和输出空间对齐.方法的整体框架如图2所示,首先在图像空间计算源域图像和目标域图像之间的最优传输矩阵,利用最优传输矩阵将源域图像风格转换至目标域;其次将转换后的源域图像㊁目标域图像输入语义分割网络,同时获取源域和目标域的深度特征(对应特征空间)和模型预测(对应输出空间);再次计算源域和目标域特征空间W a s s e r s t e i n 距离(e a r t h m o v e rd i s t a n ce ,E M D ),作为特征空间损失;然后在输出空间计算源域和目标域之间的E M D ,作为输出空间损失;同时为保证模型稳定性,将源域输出空间结果进行上采样,作为源域预测结果,利用源域标签计算交叉熵损失,实现源域监督学习;最后将训练好的模型应用于目标域图像,以完成目标域语义分割.注:O T 为最优传输;L o s s f e a t u r e 为特征空间损失;L o s s o u t p u t 为输出空间损失;L o s s s e g 为语义分割损失.图2㊀本文方法框架F i g .2㊀F r a m e w o r ko f t h e p r o po s e dm e t h o d ㊀㊀本文采用基于R e s N e t 101[34]的D e e pL a b GV 2框架作为语义分割网络,并遵循文献[24]的做法,移除最后一个分类层,将最后两个卷积层的步长从2修改为1,使得输出特征的尺寸是输入图像的1/8;网络在最后两个卷积层中应用扩张卷积以增大感受野,其步长分别为2和4;在特征提取后,使用A S P P (a t r o u s s p a t i a l p y r a m i d p o o l i n g)[35]作为最终预测层.1.1㊀最优传输和域自适应最优传输[36]理论可以找到从一个分布d s (如源域分布)至另一个分布d t (如目标域分布)的最优映射方案.具体而言,最优传输在d s 和d t 之间搜索一个具有最小传输成本的概率耦合γɪΠ(d s ,d t ),如式(1)所示㊀T d s ,d t =i n f γɪΠ(μs ,μt)ʏR 2c (x s ,x t )d γ(x s ,x t )(1)式中,c 是成本函数,可以用来衡量源域样本x s 和目标域样本x t 之间的差异.T d s ,d t可以进一步定义d s 和d t 之间的p 阶Wa s s e r s t e i n 距离,具体为W p (d s ,d t )=i n f γɪΠ(E x s ~μs ,x t ~μtd (x s ,x t )p )1p{}(2)式中,d (x s ,x t )p是一种距离度量,对应式(1)中的成本函数c (x s ,x t ).W a s s e r s t e i n 距离在计算机视觉领域也被称为E M D [37].在本文的域自适应问题中,源域和目标域的分布d s 和d t 只能通过离散样本获取,故离散化后的最优传输公式为T d s ,d t=m i n γɪΠ(d s ,d t )‹γ,C ›F (3)式中,‹.,.›F 是Fr o b e n i u s 点积;C 表示是代价矩阵;c (x s ,x t )表示成对代价.该优化问题的最小值可以用作分布之间的距离,以上是本文研究的基础.1.2㊀图像空间最优传输基于最优传输的图像风格迁移将目标域图像空间的色彩风格迁移至源域,其中图像空间由图7112D e c e m b e r2023V o l.52N o.12A G C S h t t p:ʊx b.c h i n a s m p.c o m像平面及色彩通道的数值信息构成.假设源域和目标域图像空间色彩分布分别是N(μs,Σs)和N(μt,Σt),其中N(.,.)代表高斯分布.最优传输确定N(μs,Σs)和N(μt,Σt)之间的闭合形式映射,其满足N(x t)ɪN(T(x s)),即T(x s)=(x s-μs) A+μt(4)式中,A为传输矩阵;x s和x t分别表示源域和目标域样本.值得注意的是,可行传输矩阵A的结果不唯一,但最优可行解,即最优传输矩阵不存在多个解[38].通过最优传输可以找到一个最优的映射T来最小化源域和目标域分布之间的距离,即m i n TʏR2c(x s,T(x s))d z(x s)(5)式中,c的含义和式(1)中的含义一致,即成本函数,本文采用欧氏距离形式.式(5)对应的最优传输矩阵为A=Σ-12sΣ12sΣtΣ12s()Σ-12s(6)图像空间最优传输的具体步骤如下:(1)统计源域和目标域图像色彩空间分布直方图,获取源域和目标域色彩分布参数μs㊁Σs㊁μt㊁Σt;(2)根据式(6),计算最优传输矩阵A;(3)利用式(4)对源域图像x s进行转换,得到具备目标域色彩风格的源域图像T(x s),如图2图像空间部分所示.1.3㊀特征空间与输出空间最优传输文献[33]提出D e e p J D O T方法,最早将J D O T[32]引入深度学习域自适应任务.但是,该方法仅在特征空间进行最优传输,且仅可以应用于图像分类任务.在语义分割任务中,所需传输的样本数量远大于图像分类任务,直接将D e e p J D O T应用于语义分割任务是不现实的.若降低输入图像尺寸,则会增加特征空间匹配难度,导致源域和目标域特征错误匹配㊁传输,降低整体域自适应效果.针对上述问题,本文提出了以下解决方案:①在不降低输入图像尺寸的情况下,在特征空间进行下采样,进一步压缩需要匹配的样本数量,在保证特征能够成功匹配的情况下降低最优传输计算量;②在输出空间进行最优传输,通过输出空间类别边缘分布保证源域和目标域对齐.特征空间和输出空间的优化过程为m i nγ1,γ2ɪΠ(d s,d t),f,gðiðjγ1i j d(g(T(x s i)),g(x t j))+㊀γ2i j d(f(g(x s j)),f(g(x t j)))(7)式中,g是特征提取器(即D e e p L a bGV2特征提取部分);f是分类器(即A S P P);i㊁j分别表示每次从源域和目标域中提取的图像样本序号;d(.,.)是衡量源域与目标域差异的距离函数;γ1i j㊁γ2i j分别是特征空间和输出空间的最优传输矩阵.1.3.1㊀特征空间最优传输在特征空间,本文采用L2距离衡量源域和目标域特征之间的差异,即d(g(T(x s i)),g(x t j))=g(T(x s i))-g(x t j)2(8)进而,特征空间的损失函数为L o s s f e a t u r e=γ1i j g(T(x s i))-g(x t j)2(9)式中,源域特征g(T(x s i))和目标域特征g(x t j)皆被下采样;γ1i j为源域第i个图像和目标域第j个图像特征空间的最优传输矩阵.反向传播上述损失,即可减轻源域和目标域特征空间差异,进而生成域不变特征.1.3.2㊀输出空间最优传输输出空间包含重要的类别分布信息,通过输出空间对齐,可以减弱源域和目标域的类别分布差异.具体而言,本文采用L2距离衡量源域和目标域输出空间距离,即㊀㊀d(f(g(x s j)),f(g(x t j)))=㊀㊀㊀f(g(T(x s i)))-f(g(x t j))2(10)进而,输出空间的损失函数为L o s s o u t p u t=γ2i j f(g(T(x s i)))-f(g(x t j))2(11)式中,γ2i j为源域第i个图像和目标域第j个图像输出空间的最优传输矩阵.反向传播上述损失,可以减轻源域和目标域输出空间类别分布差异,进而增强模型域自适应能力.1.4㊀模型优化为了保证所提出方法的基础性能,本文添加源域监督学习过程,即根据源域图像的预测结果和其对应的标签信息,计算交叉熵损失,具体为㊀㊀L o s s s e g=-ði,h,wðkɪN y s(h,w)il n(S(f(g(T(x s i)))(h,w))(k))(12)式中,y s i是源域标签;h和w是标签图像高度和宽度;k是类别;S( )是s o f t m a x函数.结合特征空间与输出空间的损失函数,整体模型优化损失为L o s s=L o s s s e g+β1L o s s f e a t u r e+β2L o s s o u t p u t(13)8112第12期沈秭扬,等:遥感图像跨域语义分割的无监督域自适应对齐方法式中,β1㊁β2为特征空间和输出空间最优传输损失的控制参数.默认设置为β1=0.01,β2=0.01.需要说明的是,图像空间风格迁移,特征空间㊁输出空间最优传输,源域监督学习的损失函数计算可以在同一次训练中进行;即本文方法不需要单独训练源域模型,域自适应过程与源域监督学习可以同步进行,有效减少了人工干涉,缩短了训练时间,进一步提高了模型自动化能力.2㊀试㊀验2.1㊀试验数据与精度评价指标本文使用国际摄影测量与遥感学会(I S P R S )所提供的两个高分航空遥感数据集,即P o t s d a m 数据集和V a i h i n g e n 数据集.其中P o t s d a m 数据集由38张6000ˑ6000像素图像组成,分辨率为0.05m ,包括I R R G 和R G B 两种波段组合;涵盖6个常见地物类别,即不透水层㊁车辆㊁树木㊁低矮植被㊁建筑物和背景.V a i h i n g e n 数据集由33张大小不一的图像构成,图像平均大小为2000ˑ2000像素,分辨率为0.09m ,具备与P o t s d a m 数据集相同的地物类别体系,但仅有I R R G 波段组合.如图3所示,P o t s d a m 数据集和V a i h i n ge n 数据集在图像色彩㊁地物外观及尺度上均存在较大差异,这为跨域语义分割任务带来了挑战.为定量评估方法性能,本文使用当前主流的交并比(i n t e r s e c t i o no v e r u n i o n ,I o U )指数来评估各类别分割精度.同时,本文引入所有类别的I o U 精度平均值(m e a n i n t e r s e c t i o no v e r u n i o n ,m I o U ),以衡量模型的整体性能.图3㊀I S P R SP o t s d a m 数据集和V a i h i n ge n 数据集F i g .3㊀I S P R SP o t s d a md a t a s e t s a n dV a i h i n ge nd a t a s e t s 2.2㊀试验设置为了充分验证所提出方法有效性,本文对P o t s d a m 数据集I R R GңV a i h i n g e n 数据集I R R G ㊁V a i h i n g e n 数据集I R R GңP o t s d a m 数据集I R R G ㊁P o t s d a m 数据集R G BңV a i h i n g e n 数据集I R R G ㊁V a i h i n g e n 数据集I R R GңP o t s d a m 数据集R G B 这4组跨域场景进行试验.试验使用P yt o r c h 框架和单个N V I D I A G T X2080T i 显卡进行训练,并使用动量为0.9㊁权重衰减为5ˑ10-4的S G D 算法优化网络.试验初始学习率l r 设置为5ˑ10-4,并以0.9的幂进行多项式衰减l r i t e r =l r ˑ1-i t e r m a x _i t e r æèçöø÷0.9(14)式中,i t e r 为迭代次数;m a x _i t e r 是最大迭代次数;m a x _i t e r 设置为50000.训练时,模型随机裁切源域图像为1000ˑ1000像素的图像块进行训练,并随机进行图像竖直翻转和水平翻转等增强处理;测试时使用1000ˑ1000像素的滑动窗口进行整幅图像预测.关于超参数β1和β2的设置,本文通过P o t s d a m 数据集I R R GңV a i h i n g e n 数据集I R R G 的试验进行了验证.β1和β2代表特征空间和输出空间最优传输在整个训练过程中的影响权重,数值越大,模型在训练过程中对域迁移关注度越高.β1和β2数值为0.0100时,本文方法取得最高精度(表1和表2).在逐步增大β1和β2过程中,模型精度略有下降,这是由于模型过度关注源域和目标域分布对齐,而忽略源域语义分割监督训练的结果;在逐步减小β1和β2的过程中,模型精度也缓慢下降,这说明特征空间和输出空间域迁移对精度提升的积极作用.因此,本文将β1和β2的默认值设置为0.0100.表1㊀超参数β1的选择T a b .1㊀T h e s e l e c t i o no f h y p e r p a r a m e t e r β1表2㊀超参数β2的选择T a b .2㊀T h e s e l e c t i o no f h y p e r p a r a m e t e r29112D e c e m b e r2023V o l.52N o.12A G C S h t t p:ʊx b.c h i n a s m p.c o m㊀㊀为说明本文方法的优势,本文与5种代表性域自适应方法进行了对比.这些方法包括C y c l e G A N[20]㊁A d a p t S e g N e t[24]㊁S I M(s t u f f i n s t a n c e m a t c h i n g)[25]㊁C a G A N(c l a s sGa w a r e g e n e r a t i v e a d v e r s a r i a l n e t w o r k)[26]和U D A方法[27].这些方法的语义分割模型均为基于R e s N e t101的D e e p L a bGV2.此外,本文加入了 仅源域 (即仅在源域进行监督训练,直接用于目标域预测),并将仅源域训练精度作为几组试验的基线精度.2.3㊀试验结果与分析2.3.1㊀精度对比与分析试验精度结果见表3 表6,其中仅源域训练精度最低,这说明不同域之间存在分布偏差,单纯源域训练所得到的模型难以在目标域上取得较高精度.此外,如表3和表4㊁表5和表6的精度差异所示,即便训练任务中源域和目标域存在相同的域偏移,但由于迁移顺序的差异,仍会带来不同的精度结果,且图像数量较多的源域具备更加多样化的特征分布,可以在迁移至目标域时取得更高精度.C y c l e G A N方法在V a i h i n g e n数据集ңP o t s d a m数据集迁移任务中,即表4和表6中,较好地减弱了低矮植被与树木两个类别的域偏移问题,但在其他地物类别上精度较低,如表4的不透水层和表6的车辆,相对于仅源域训练的精度有所下降,且C y c l e G A N方法的m I o U指数提升并不明显,这表明单一的图像空间风格迁移并不能较好地解决域偏移问题.A d a p t S e g N e t方法在多组试验中的表现相对较好,但由于缺乏图像空间色彩分布和特征空间高维特征分布对齐,其在复杂的跨域任务V a i h i n g e n数据集I R R GңP o t s d a m数据集R G B中表现较差,建筑物类别精度相对于仅源域训练精度有所下降;引入实例和类别信息的S I M㊁C a G A N和U D A(C h e n)方法,进一步缓解了域偏移问题,保证了各类别精度的稳步提升.相比于其他方法,本文方法通过结合多个空间最优传输优势,在仅源域训练的精度基础上,取得了显著的精度提升(表3 表6),m I o U指数分别提高了17.39%㊁22.02%㊁16.91%㊁17.84%,且高于其他方法,这表明多空间最优传输相结合可以有效提高模型总体的域自适应能力.表3㊀P o t s d a m数据集I R R GңV a i h i n g e n数据集I R R G精度结果T a b.3㊀T h e a c c u r a c y r e s u l t s o fP o t s d a mI R R GңV a i h i n g e n I R R G(%)方法不透水层建筑物低矮植被树木车辆m I o U仅源域36.2052.9316.3156.0920.6636.44C y c l e G A N[20]59.1658.3629.3443.9728.6243.89A d a p t S e g N e t[24]56.1164.2334.9956.4032.7548.90S I M[25]57.1469.2338.4756.6329.2350.14C a G A N[26]58.9171.6137.4256.1832.8651.40U D A(C h e n)[27]57.2670.3838.6555.8830.5350.54本文方法60.8770.2136.8759.6641.5453.83表4㊀V a i h i n g e n数据集I R R GңP o t s d a m数据集I R R G精度结果T a b.4㊀T h e a c c u r a c y r e s u l t s o fV a i h i n g e n I R R GңP o t s d a mI R R G(%)方法不透水层建筑物低矮植被树木车辆m I o U仅源域44.9441.5436.524.3222.8830.04C y c l e G A N[20]40.9442.7643.8834.6346.1641.67A d a p t S e g N e t[24]53.9050.5344.9821.4643.6942.91S I M[25]55.6954.7244.9627.1051.1746.73C a G A N[26]53.3147.0042.8624.8134.2840.45U D A(C h e n)[27]54.9456.3143.5630.3848.6646.77本文方法63.9869.2446.5240.7239.8352.062.3.2㊀可视化结果与分析可视化结果如图4 图7所示.在所有测试方法中,仅源域训练的结果最差,在目标域图像场景复杂度较高时(如图6(c)和图7(c)所示),地物的边界完全模糊,预测类别混乱,仅在少量结果中可以看到建筑物的大致轮廓.C y c l e G A N能够较好解决因色彩差异而导致的域偏移问题,但由于缺少高维特征分布对齐,地物边界存在模糊不清现象,背景类与其他类别混淆严重.A d a p t S e g N e t方法相比于C y c l e G A N方法具备一定优势,但在源0212第12期沈秭扬,等:遥感图像跨域语义分割的无监督域自适应对齐方法域和目标域图像波段组合不同时,如图6(e)㊁图7(e )所示,建筑物㊁低矮植被与背景的分类结果混淆,部分区域存在明显误判现象.C a G A N 在输出空间对抗训练的基础上添加了类别信息,进一步缓解了模型在部分类别中的错分问题,但地物边界仍然模糊,且由于高维类别特征分布差异较大,简单的类别特征分布对齐反而带来了负迁移问题,即未能找到源域和目标域分布的合理对齐方式.如图4(a )和图6(d )结果所示,C a G A N 将建筑物错分为背景,可视化结果不及A d a p t S e gN e t ;S I M 和U D A (C h e n )方法也存在诸多误判现象,但它们分别采用实例对齐和判别器逐类判别过程,部分解决了遥感图像复杂的类内差异所引起的迁移困难问题.表5㊀P o t s d a m 数据集R G B ңV a i h i n g e n 数据集I R R G 精度结果T a b .5㊀T h e a c c u r a c y r e s u l t s o fP o t s d a m R G B ңV a i h i n ge n I R R G (%)方法不透水层建筑物低矮植被树木车辆m I o U仅源域42.9645.9314.4346.0812.8632.45C yc l e G A N [20]53.3843.8619.5740.9334.4438.44Ad a p t Se gN e t [24]59.7958.6629.2641.9631.9044.31S I M [25]56.3160.6328.9344.7930.6744.27C a G A N[26]60.1548.5425.8354.2430.2943.81U D A (C h e n)[27]56.9062.6829.8345.5830.5945.12本文方法51.5473.6823.8955.1442.5349.36表6㊀V a i h i n g e n 数据集I R R G ңP o t s d a m 数据集R G B 精度结果T a b .6㊀T h e a c c u r a c y r e s u l t s o fV a i h i n ge n I R R G ңP o t s d a m R G B (%)方法不透水层建筑物低矮植被树木车辆m I o U仅源域32.5838.6628.731.0331.8326.57C y c l e G A N [20]38.3145.3538.4030.1919.6434.38A d a p t S e g N e t [24]45.4136.5528.795.8638.0630.93S I M[25]48.5151.4238.9720.1746.7141.15C a G A N [26]45.9946.2833.7418.4841.7437.24U D A (C h e n)[27]50.4346.2837.0216.3141.8238.37本文方法54.5958.3538.8635.8234.4144.41图4㊀P o t s d a m 数据集I R R GңV a i h i n g e n 数据集I R R G 可视化结果F i g .4㊀T h e v i s u a l r e s u l t s o fP o t s d a mI R R GңV a i h i n ge n I R R G 1212D e c e m b e r 2023V o l .52N o .12A G C Sh t t p :ʊx b .c h i n a s m p .c om 图5㊀V a i h i n g e n 数据集I R R GңP o t s d a m 数据集I R R G 可视化结果F i g .5㊀T h e v i s u a l r e s u l t s o fV a i h i n ge n I R R GңP o t s d a mI R RG 图6㊀P o t s d a m 数据集R G B ңV a i h i n g e n 数据集I R R G 可视化结果F i g .6㊀T h e v i s u a l r e s u l t s o fP o t s d a m R G B ңV a i h i n ge n I R R G ㊀㊀本文提出多空间结合的最优传输域自适应方法,能够有效结合多空间最优传输优势,在保持地物边界的同时有效区分纹理和色调相近的地物,提高了模型在目标域上的分割效果.如图4(c )所示,本文方法较好地分类了低矮植被,未出现其他方法中常见的低矮植被与背景的混淆问题,这缘于最优传输可以在分布间差异较大情况下,提供具备完备几何意义的距离度量,这对遥感图像复杂场景的分割任务是至关重要的.此外,如图6㊁图7所示,即便在复杂迁移任务中,本文方法也能够清晰界定地物轮廓,内部噪声较少,相对准确地识别复杂形态地物(如树木).2.3.3㊀模型复杂度分析为了定量评估模型效率,本文采用参数量和计算量(f l o a t i n g Gp o i n to p e r a t i o n s p e rs e c o n d ,F L O P s )两个指标,在输入图像尺寸(512ˑ512像2212第12期沈秭扬,等:遥感图像跨域语义分割的无监督域自适应对齐方法素)相同情况下,测试模型运算的复杂度,具体结果见表7.其中,C yc l e G A N 的参数量和F L O P s 值显著高于其他方法;本文方法的参数量和F L O P s 值最小.这表明,相对于采用G A N 的域自适应方法,包括C y c l e G A N ,A d a p t S e gN e t ,S I M ,C a G A N 和U D A (C h e n )方法,本文方法的模型复杂度更小,训练更加便捷.图7㊀V a i h i n g e n 数据集I R R GңP o t s d a m 数据集R G B 可视化结果F i g .7㊀T h e v i s u a l r e s u l t s o fV a i h i n ge n I R R GңP o t s d a m R G B 表7㊀测试模型的参数量和F L O P sT a b .7㊀P a r a m e t e r s a n dF L O P s o f a l l t e s t e dm o d e l s方法参数量/M F L O P s /G BC yc l e G A N 5606.51588Ad a p t Se g N e t 2506.36200S I M 2473.97198C a G A N2474.36193U D A (C h e n )2540.05332本文方法2441.971842.4㊀消融试验与分析为验证本文方法各模块的有效性,本文在P o t s d a m 数据集I R R GңV a i h i n g e n 数据集I R R G 迁移任务上进行了消融试验,表8和图8显示了每个模块及其不同组合的作用和可视化效果.在单空间对齐测试中,输出空间最优传输的测试精度最高(m I o U 指数达到46.88%),这缘于输出空间同时包含几何和类别信息.同时,即便特征空间维度较高,最优传输理论仍然可以充分考虑特征中隐含的几何结构,因此,特征空间最优传输也能取得精度提升(m I o U 指数达到42.11%).此外,在图像空间最优传输和输出空间最优传输的可视化结果中,地物类别更加准确,而在特征空间最优传输的可视化结果中,地物边界的界定则更加清晰(如上方建筑物).表8㊀消融试验精度分析T a b .8㊀T h e q u a n t i t a t i v e a n a l y s i s o f a b l a t i o n s t u d y仅源域图像空间最优传输特征空间最优传输输出空间最优传输m I o U/(%)ɿ36.44ɿɿ45.68ɿɿ42.11ɿɿ46.88ɿɿɿ50.32ɿɿɿ48.85ɿɿɿ51.23ɿɿɿɿ53.83在多空间组合对齐测试中,精度普遍高于单空间对齐,这说明多空间最优传输可以有效提高跨域语义分割精度.将图像空间与特征空间或输出空间最优传输进行结合(即图像空间+特征空间最优传输,图像空间+输出空间最优传输),可获取相对完整的预测结果,地物边界相对清晰,类别错分现象有所减少,有效消除单输出空间或特征空间最优传输结果中出现的过分割现象.将图3212D e c e m b e r2023V o l.52N o.12A G C S h t t p:ʊx b.c h i n a s m p.c o m像空间㊁特征空间和输出空间最优传输相结合(即本文方法),能够获取清晰准确的地物边界,地物内部缺失问题得到改善;并且,图中右侧部分车辆和树木细节的分割结果也较好.这与表8的定量化精度结果相呼应,图像空间㊁特征空间和输出空间最优传输相结合所取得的精度最高(m I o U指数达到53.83%).这说明基于最优传输构建的单空间对齐模块可以简单而有效的结合在一起,充分发挥各个模块的优势,提高整体域自适应性能.图8㊀消融试验可视化结果F i g.8㊀T h e v i s u a l r e s u l t s o f a b l a t i o n s t u d y3㊀总㊀结本文提出了一种基于最优传输理论的无监督域自适应方法,用于解决遥感图像跨域语义分割时普遍存在的域偏移问题.首先,本文利用最优传输理论构建了一种更为简单的色彩映射方法,在图像空间进行风格迁移,减弱图像空间域偏移影响;然后,将最优传输引入语义分割无监督域自适应框架,分别在特征空间和输出空间使用最优传输理论计算损失,减轻数据分布差异,提升了模型的跨域语义分割性能.试验引入P o t s d a m数据集和V a i h i n g e n数据集,利用I o U指数,对本文方法进行测试.结果表明,相对于其他单一空间域自适应方法,本文方法能够有效结合高维特征空间㊁输出空间与图像空间域自适应方法优势;在不同域迁移任务中,本文方法皆表现出较为明显的优势,得到了更高的跨域语义分割精度.本文方法尚未充分研究并细化源域和目标域潜在的类间关系,在后续研究中,将对该问题进行深入研究,寻求突破.参考文献:[1]㊀陈杰,邓敏,肖鹏峰,等.结合支持向量机与粒度计算的高分辨率遥感影像面向对象分类[J].测绘学报,2011,40(2):135G141,147.C H E NJ i e,DE N G M i n,X I A O P e n g f e n g,e ta l.O b j e c tGo r i e n t e d c l a s s i f i c a t i o n o f h i g h r e s o l u t i o n i m a g e r yc o m b i n i n g s u p p o r t v e c t o r m a c h i n e w i t h g r a n u l a rc o m p u t i n g[J].A c t a G e od ae t i c ae tC a r t o g r a p h i c aS i n i c a,2011,40(2):135G141,147.[2]㊀A W A D M,C H E H D IK,N A S R IA.M u l t i c o m p o n e n t i m a g es e g m e n t a t i o nu s i n g a g e n e t i c a l g o r i t h ma n d a r t i f i c i a l n e u r a ln e t w o r k[J].I E E E G e o s c i e n c e a n d R e m o t e S e n s i n gL e t t e r s,2007,4(4):571G575.[3]㊀L A L I B E R T EAS,F R E D R I C K S O NEL,R A N G OA.C o mGb i n i n g d ec i s i o n t r e e s w i t h h i e r a r c h i c a l o b j e c tGo r i e n t e di m a g ea n a l y s i sf o r m a p p i n g a r i dr a n g e l a n d s[J].P h o t oGg r a mm e t r i c E n g i n e e r i n g&R e m o t e S e n s i n g,2007,73(2):197G207.[4]㊀王猛,张新长,王家耀,等.结合随机森林面向对象的森林资源分类[J].测绘学报,2020,49(2):235G244.D O I:10.11947/j.A G C S.2020.20190272.WA N G M e n g,Z H A N G X i n c h a n g,WA N GJ i a y a o,e t a l.F o r e s t r e s o u r c ec l a s s i f i c a t i o nb a s e do nr a n d o mf o r e s ta n do b j e c to r i e n t e d m e t h o d[J].A c t a G e o d a e t i c a e t C a r t oGg r a p h i c aS i n i c a,2020,49(2):235G244.D O I:10.11947/j.A G C S.2020.20190272.[5]㊀D O UP e n g,C H E N Y a n g b o,Y U E H a i y u n.R e m o t eGs e n s i n gi m a g e r y c l a s s i f i c a t i o n u s i n g m u l t i p l ec l a s s i f i c a t i o n a l g oG4212。
基于特征匹配滤波的自适应模板跟踪算法

K y od :e pa p a ; enl e syet t n mensi ;bet akn e w r s t leud t kred ni i i ; a f o j cig m t e t sma o ht ct r
1 引言
Me ns i 算法是一种快速搜 索算法 , 有特征 a hf t 具 稳定、鲁棒性强 、实时性好等诸多优势【 4 11 _ 。但是 ,当 场景 中 目标外观变化较大或非 目标干扰较为严 重时 ,
t n m ac i g fl r h s me o e i e n t e s e ii ie s ta e d t e u dae n t mp ae i th n it ,t i t d d cd so p cfc p x l h tn e o b p td i e lt .Th o e h h e
AnAu o Ad p ieM o e d t a k n g rt m i gFe t r a c i gF l r t・ a t d l v Up a eTr c i gAlo i h Usn a u eM t h n i e t
Z HOU e - e , Ch n Ch n HUANG a g W ANG a - i g Ch n , Xi o M n
e pr e tl eut so a tea o tm i a f c v lt nt te o t gh a n b c bok x ei n a rsl w t th l rh nef t es ui ti edadoj t lc m t sh h gi s e i o o o h r an e
Ab t a t Th sp p rpr p s san v l e lt d p i eu d t g srt g sp r fM e n S itfa e r ih i sr c: i a e o o e o e mp ae a a tv p a i tae y a ato a h f rm wo k whc s t n b s d o e t r ac i g fl r i h s e a o o e d ta k n .I a c r a c t e c lr s a e a e n f au e m th n t n t e c n r f h a rc i g n c o d n e wih t o o p c i e i h
基于掩码矩阵
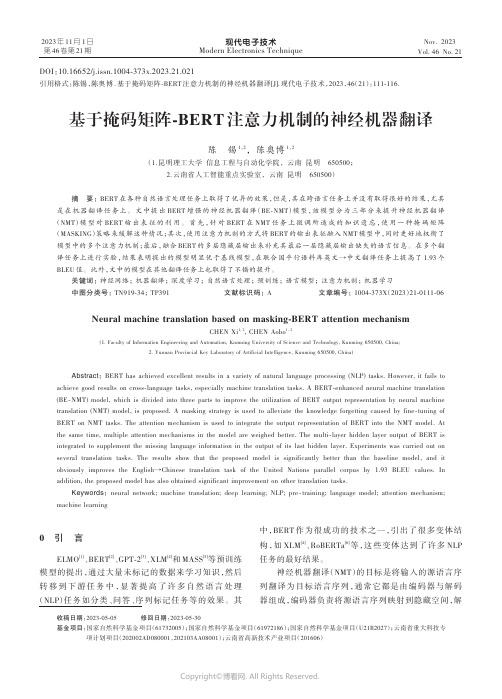
现代电子技术Modern Electronics Technique2023年11月1日第46卷第21期Nov. 2023Vol. 46 No. 210 引 言ELMO [1]、BERT [2]、GPT⁃2[3]、XLM [4]和MASS [5]等预训练模型的提出,通过大量未标记的数据来学习知识,然后转移到下游任务中,显著提高了许多自然语言处理(NLP )任务如分类、问答、序列标记任务等的效果。
其中,BERT 作为很成功的技术之一,引出了很多变体结构,如XLM [4]、RoBERTa [6]等,这些变体达到了许多NLP 任务的最好结果。
神经机器翻译(NMT )的目标是将输入的源语言序列翻译为目标语言序列,通常它都是由编码器与解码器组成,编码器负责将源语言序列映射到隐藏空间,解基于掩码矩阵⁃BERT 注意力机制的神经机器翻译陈 锡1,2, 陈奥博1,2(1.昆明理工大学 信息工程与自动化学院, 云南 昆明 650500;2.云南省人工智能重点实验室, 云南 昆明 650500)摘 要: BERT 在各种自然语言处理任务上取得了优异的效果,但是,其在跨语言任务上并没有取得很好的结果,尤其是在机器翻译任务上。
文中提出BERT 增强的神经机器翻译(BE⁃NMT )模型,该模型分为三部分来提升神经机器翻译(NMT )模型对BERT 输出表征的利用。
首先,针对BERT 在NMT 任务上微调所造成的知识遗忘,使用一种掩码矩阵(MASKING )策略来缓解这种情况;其次,使用注意力机制的方式将BERT 的输出表征融入NMT 模型中,同时更好地权衡了模型中的多个注意力机制;最后,融合BERT 的多层隐藏层输出来补充其最后一层隐藏层输出缺失的语言信息。
在多个翻译任务上进行实验,结果表明提出的模型明显优于基线模型,在联合国平行语料库英文→中文翻译任务上提高了1.93个BLEU 值。
此外,文中的模型在其他翻译任务上也取得了不错的提升。
基于人工智能的多模态雷达自适应抗干扰优化算法
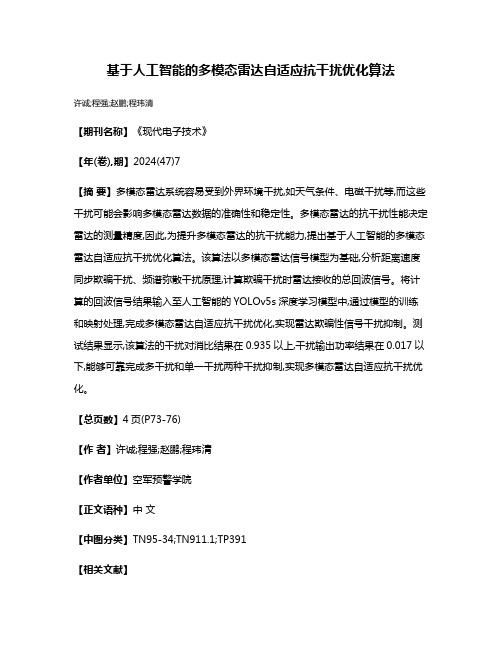
基于人工智能的多模态雷达自适应抗干扰优化算法
许诚;程强;赵鹏;程玮清
【期刊名称】《现代电子技术》
【年(卷),期】2024(47)7
【摘要】多模态雷达系统容易受到外界环境干扰,如天气条件、电磁干扰等,而这些干扰可能会影响多模态雷达数据的准确性和稳定性。
多模态雷达的抗干扰性能决定雷达的测量精度,因此,为提升多模态雷达的抗干扰能力,提出基于人工智能的多模态雷达自适应抗干扰优化算法。
该算法以多模态雷达信号模型为基础,分析距离速度同步欺骗干扰、频谱弥散干扰原理,计算欺骗干扰时雷达接收的总回波信号。
将计算的回波信号结果输入至人工智能的YOLOv5s深度学习模型中,通过模型的训练和映射处理,完成多模态雷达自适应抗干扰优化,实现雷达欺骗性信号干扰抑制。
测试结果显示,该算法的干扰对消比结果在0.935以上,干扰输出功率结果在0.017以下,能够可靠完成多干扰和单一干扰两种干扰抑制,实现多模态雷达自适应抗干扰优化。
【总页数】4页(P73-76)
【作者】许诚;程强;赵鹏;程玮清
【作者单位】空军预警学院
【正文语种】中文
【中图分类】TN95-34;TN911.1;TP391
【相关文献】
1.基于相控阵雷达自适应波束形成的抗干扰技术
2.基于AA的多通道雷达自适应抗干扰方法
3.基于AA的多通道雷达自适应抗干扰方法
4.一种基于自适应搜索的多模态多目标优化算法
5.基于鲸鱼优化算法的变分模态分解和改进的自适应加权融合算法的管道泄漏检测与定位方法
因版权原因,仅展示原文概要,查看原文内容请购买。
基于随机森林与粒子群算法的隧道掘进机操作参数地质类型自适应决策

第 54 卷第 4 期2023 年 4 月中南大学学报(自然科学版)Journal of Central South University (Science and Technology)V ol.54 No.4Apr. 2023基于随机森林与粒子群算法的隧道掘进机操作参数地质类型自适应决策刘明阳,陶建峰,覃程锦,余宏淦,刘成良(上海交通大学 机械与动力工程学院,上海,200240)摘要:考虑到隧道掘进机的性能对地质条件比较敏感且其操作依赖于司机经验,提出基于随机森林和粒子群算法的隧道掘进机操作参数地质条件自适应决策方法。
利用随机森林(RF)分别建立地质类型、操作参数与推进速度、刀盘转矩的映射关系模型;结合映射关系模型,构建以盾构机推进速度最大为目标,以刀盘转速、螺旋输送机转速、总推力、土仓压力4个操作参数为控制变量的优化方程;利用粒子群算法(PSO)求解各地质类型地层中的最优操作参数决策结果。
通过新加坡某地铁工程施工数据验证所提方法的有效性和优越性。
研究结果表明:建立的随机森林模型中推进速度和刀盘转矩预测的决定系数R 2分别达到0.936和0.961,均大于adaboost 、多元线性回归、岭回归、支持向量回归和深度神经网络模型中相应的R 2;基于粒子群算法的操作参数决策方法能够准确求解操作参数最优解,寻优用时均比遗传算法、蚁群算法和穷举法的短。
本文所提决策方法使隧道掘进机在该施工段的福康宁卵石地层、句容地层IV 、句容地层V 、海洋黏土地层中的推进速度分别提升了67.2%、41.8%、53.6%和15.0%。
关键词:隧道掘进机;操作参数决策;随机森林;粒子群优化中图分类号:TH17;TU62 文献标志码:A 文章编号:1672-7207(2023)04-1311-14Geological adaptive TBM operation parameter decision based onrandom forest and particle swarm optimizationLIU Mingyang, TAO Jianfeng, QIN Chengjin, YU Honggan, LIU Chengliang(School of Mechanical Engineering, Shanghai Jiaotong University, Shanghai 200240, China)Abstract: Considering that the performance of TBM is affected by geological condition and driver experience, a geological adaptive TBM operation parameter decision based on random forest(RF) and particle swarm optimization algorithm(PSO) was proposed. RF was used to establish the mapping relation model between geological types, operating parameters and thrust speed, cutter head torque. An optimization equation was established using the mapping relationship model in which the maximum TBM thrust speed was taken as the target, and cutterhead speed, screw conveyor speed, total thrust and earth pressure were taken as control variables.收稿日期: 2022 −06 −19; 修回日期: 2022 −08 −21基金项目(Foundation item):国家重点研发计划项目(2018YFB1702503) (Project(2018YFB1702503) supported by the National KeyR&D Program of China)通信作者:陶建峰,博士,教授,从事机械电子工程研究;E-mail :**************.cnDOI: 10.11817/j.issn.1672-7207.2023.04.010引用格式: 刘明阳, 陶建峰, 覃程锦, 等. 基于随机森林与粒子群算法的隧道掘进机操作参数地质类型自适应决策[J]. 中南大学学报(自然科学版), 2023, 54(4): 1311−1324.Citation: LIU Mingyang, TAO Jianfeng, QIN Chengjin, et al. Geological adaptive TBM operation parameter decision based on random forest and particle swarm optimization[J]. Journal of Central South University(Science and Technology), 2023, 54(4): 1311−1324.第 54 卷中南大学学报(自然科学版)PSO was used to solve the optimal combination of operating parameters for each geological type. The validity and superiority of the proposed method were verified by the construction data of a subway project in Singapore. The results show that the R2 of the driving speed and cutter head torque predicted by random forest model reaches 0.936 and 0.961, which are greater than those of adaboost, multiple linear regression, ridge regression, SVR and DNN. PSO can accurately solve the optimal solution of operating parameters, and the time consumption is shorter than that of genetic algorithm, ant colony algorithm and exhaustive algorithm. By using the proposed method, the TBM thrust speed increases by 67.2%, 41.8%, 53.6%, 15.0% in the strata of Fokonnen Pebble Formation, Jurong Formation IV, Jurong Formation V and Marine Clay Formation in this construction section, respectively.Key words: tunnel boring machine; operating parameter decision; random forest; particle swarm optimization隧道掘进机是一种大型隧道掘进装备,具有开挖速度快、自动化程度高、施工质量好的优点,广泛地被应用于地铁、铁路、公路等隧道工程中[1]。
一种改进的高斯频率域压缩感知稀疏反演方法(英文)
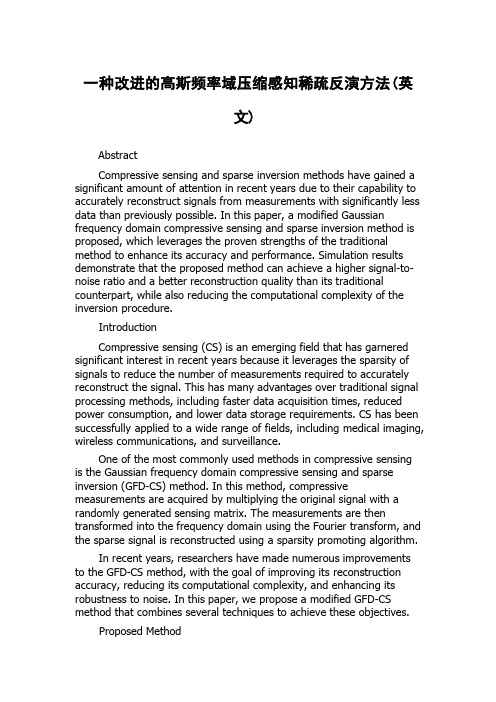
AbstractCompressive sensing and sparse inversion methods have gained a significant amount of attention in recent years due to their capability to accurately reconstruct signals from measurements with significantly less data than previously possible. In this paper, a modified Gaussian frequency domain compressive sensing and sparse inversion method is proposed, which leverages the proven strengths of the traditional method to enhance its accuracy and performance. Simulation results demonstrate that the proposed method can achieve a higher signal-to- noise ratio and a better reconstruction quality than its traditional counterpart, while also reducing the computational complexity of the inversion procedure.IntroductionCompressive sensing (CS) is an emerging field that has garnered significant interest in recent years because it leverages the sparsity of signals to reduce the number of measurements required to accurately reconstruct the signal. This has many advantages over traditional signal processing methods, including faster data acquisition times, reduced power consumption, and lower data storage requirements. CS has been successfully applied to a wide range of fields, including medical imaging, wireless communications, and surveillance.One of the most commonly used methods in compressive sensing is the Gaussian frequency domain compressive sensing and sparse inversion (GFD-CS) method. In this method, compressive measurements are acquired by multiplying the original signal with a randomly generated sensing matrix. The measurements are then transformed into the frequency domain using the Fourier transform, and the sparse signal is reconstructed using a sparsity promoting algorithm.In recent years, researchers have made numerous improvementsto the GFD-CS method, with the goal of improving its reconstruction accuracy, reducing its computational complexity, and enhancing its robustness to noise. In this paper, we propose a modified GFD-CS method that combines several techniques to achieve these objectives.Proposed MethodThe proposed method builds upon the well-established GFD-CS method, with several key modifications. The first modification is the use of a hierarchical sparsity-promoting algorithm, which promotes sparsity at both the signal level and the transform level. This is achieved by applying the hierarchical thresholding technique to the coefficients corresponding to the higher frequency components of the transformed signal.The second modification is the use of a novel error feedback mechanism, which reduces the impact of measurement noise on the reconstructed signal. Specifically, the proposed method utilizes an iterative algorithm that updates the measurement error based on the difference between the reconstructed signal and the measured signal. This feedback mechanism effectively increases the signal-to-noise ratio of the reconstructed signal, improving its accuracy and robustness to noise.The third modification is the use of a low-rank approximation method, which reduces the computational complexity of the inversion algorithm while maintaining reconstruction accuracy. This is achieved by decomposing the sensing matrix into a product of two lower dimensional matrices, which can be subsequently inverted using a more efficient algorithm.Simulation ResultsTo evaluate the effectiveness of the proposed method, we conducted simulations using synthetic data sets. Three different signal types were considered: a sinusoidal signal, a pulse signal, and an image signal. The results of the simulations were compared to those obtained using the traditional GFD-CS method.The simulation results demonstrate that the proposed method outperforms the traditional GFD-CS method in terms of signal-to-noise ratio and reconstruction quality. Specifically, the proposed method achieves a higher signal-to-noise ratio and lower mean squared error for all three types of signals considered. Furthermore, the proposed method achieves these results with a reduced computational complexity compared to the traditional method.ConclusionThe results of our simulations demonstrate the effectiveness of the proposed method in enhancing the accuracy and performance of the GFD-CS method. The combination of sparsity promotion, error feedback, and low-rank approximation techniques significantly improves the signal-to-noise ratio and reconstruction quality, while reducing thecomputational complexity of the inversion procedure. Our proposed method has potential applications in a wide range of fields, including medical imaging, wireless communications, and surveillance.。
用户评论方面级情感分析研究

用户评论方面级情感分析研究陈虹,杨燕+,杜圣东西南交通大学信息科学与技术学院,成都611756+通信作者E-mail:***************.cn 摘要:方面级情感分析是自然语言处理的热门研究方向之一,相比于传统的情感分析技术,基于方面的情感分析是细粒度的,能够判断句子中多个目标的情感倾向,能更加准确地挖掘用户对目标的情感极性。
针对以往研究忽略目标单独建模的问题,提出了一种基于双向长短期记忆神经网络(BiLSTM )的交互注意力神经网络模型(Bi-IAN )。
该模型通过BiLSTM 对目标和上下文分别进行建模,获得目标和上下文的隐藏表示,提取其中的语义信息。
接下来利用交互注意模块学习上下文和目标之间的注意力,分别生成目标和上下文的表示,捕捉目标和上下文之内和之间的相关性,并重构评价对象和上下文的表示,最终通过非线性层得到分类结果。
在数据集SemEval 2014任务4和Chinese review datasets 上的实验训练显示,在正确率和F 1-score 上,比现有的基准情感分析模型有更好的效果。
关键词:方面级情感分析;深度学习;循环神经网络(RNN );注意力机制文献标志码:A中图分类号:TP391.1Research on Aspect-Level Sentiment Analysis of User ReviewsCHEN Hong,YANG Yan +,DU ShengdongSchool of Information Science and Technology,Southwest Jiaotong University,Chengdu 611756,ChinaAbstract:Aspect-based sentiment analysis has become one of the hot research directions of natural language pared with the traditional sentiment analysis technology,aspect-based sentiment analysis is aimed at specific targets in sentences,and can judge the sentiment tendency of multiple targets in a sentence,and more accurately mine the sentiment polarity of the target.It is a fine-grained sentiment analysis technology.Aiming at the fact that the previous research ignored the problem of separate modeling of targets,an interactive attention network model based on bidirectional long short-term memory (Bi-IAN)is proposed.The model uses bidirectional long short-term memory (BiLSTM)to model the targets and the context respectively,to obtain hidden representation and extract the semantic information.Next,the attention vector between the context and the targets is learnt through interactive learning,and then the representation of the target and the context are generated.The relevance within and between the target and the context is captured,the representation of the target and context is reconstructed,and finally the model gets the classification result through the non-linear layer.Experimental training on the dataset SemEval 2014task 4and Chinese review datasets shows that the model proposed has better results than the existing benchmark sentiment analysis model in terms of accuracy and F 1-score.Key words:aspect-level sentiment analysis;deep learning;recurrent neural network (RNN);attention mechanism计算机科学与探索1673-9418/2021/15(03)-0478-08doi:10.3778/j.issn.1673-9418.2007011基金项目:国家自然科学基金(61976247);国家科技支撑计划(2015BAH19F02)。
一种高性能SAR图像边缘点特征匹配方法
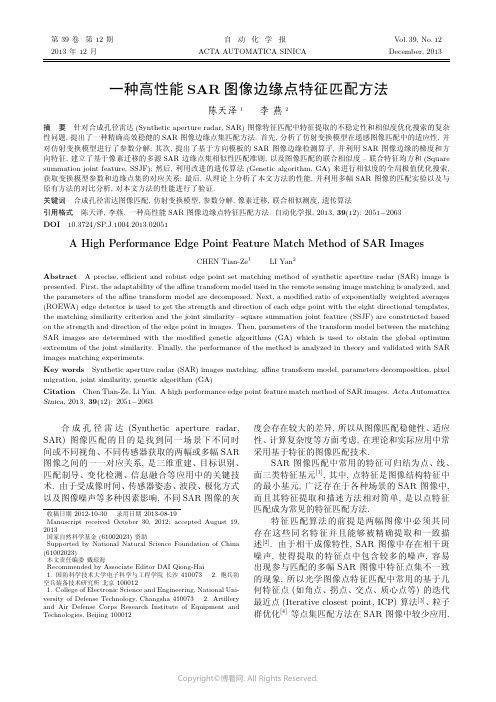
第39卷第12期自动化学报Vol.39,No.12 2013年12月ACTA AUTOMATICA SINICA December,2013一种高性能SAR图像边缘点特征匹配方法陈天泽1李燕2摘要针对合成孔径雷达(Synthetic aperture radar,SAR)图像特征匹配中特征提取的不稳定性和相似度优化搜索的复杂性问题,提出了一种精确高效稳健的SAR图像边缘点集匹配方法.首先,分析了仿射变换模型在遥感图像匹配中的适应性,并对仿射变换模型进行了参数分解;其次,提出了基于方向模板的SAR图像边缘检测算子,并利用SAR图像边缘的梯度和方向特征,建立了基于像素迁移的多源SAR边缘点集相似性匹配准则,以及图像匹配的联合相似度–联合特征均方和(Square summation joint feature,SSJF);然后,利用改进的遗传算法(Genetic algorithm,GA)来进行相似度的全局极值优化搜索,获取变换模型参数和边缘点集的对应关系;最后,从理论上分析了本文方法的性能,并利用多幅SAR图像的匹配实验以及与原有方法的对比分析,对本文方法的性能进行了验证.关键词合成孔径雷达图像匹配,仿射变换模型,参数分解,像素迁移,联合相似测度,遗传算法引用格式陈天泽,李燕.一种高性能SAR图像边缘点特征匹配方法.自动化学报,2013,39(12):2051−2063DOI10.3724/SP.J.1004.2013.02051A High Performance Edge Point Feature Match Method of SAR ImagesCHEN Tian-Ze1LI Yan2Abstract A precise,efficient and robust edge point set matching method of synthetic aperture radar(SAR)image is presented.First,the adaptability of the affine transform model used in the remote sensing image matching is analyzed,and the parameters of the affine transform model are decomposed.Next,a modified ratio of exponentially weighted averages (ROEWA)edge detector is used to get the strength and direction of each edge point with the eight directional templates, the matching similarity criterion and the joint similarity–square summation joint feature(SSJF)are constructed based on the strength and direction of the edge point in images.Then,parameters of the transform model between the matching SAR images are determined with the modified genetic algorithms(GA)which is used to obtain the global optimum extremum of the joint similarity.Finally,the performance of the method is analyzed in theory and validated with SAR images matching experiments.Key words Synthetic aperture radar(SAR)images matching,affine transform model,parameters decomposition,pixel migration,joint similarity,genetic algorithm(GA)Citation Chen Tian-Ze,Li Yan.A high performance edge point feature match method of SAR images.Acta Automatica Sinica,2013,39(12):2051−2063合成孔径雷达(Synthetic aperture radar, SAR)图像匹配的目的是找到同一场景下不同时间或不同视角、不同传感器获取的两幅或多幅SAR 图像之间的一一对应关系,是三维重建、目标识别、匹配制导、变化检测、信息融合等应用中的关键技术.由于受成像时间、传感器姿态、波段、极化方式以及图像噪声等多种因素影响,不同SAR图像的灰收稿日期2012-10-30录用日期2013-08-19Manuscript received October30,2012;accepted August19, 2013国家自然科学基金(61002023)资助Supported by National Natural Science Foundation of China (61002023)本文责任编委戴琼海Recommended by Associate Editor DAI Qiong-Hai1.国防科学技术大学电子科学与工程学院长沙4100732.炮兵防空兵装备技术研究所北京1000121.College of Electronic Science and Engineering,National Uni-versity of Defense Technology,Changsha4100732.Artillery and Air Defense Corps Research Institute of Equipment and Technologies,Beijing100012度会存在较大的差异,所以从图像匹配稳健性、适应性、计算复杂度等方面考虑,在理论和实际应用中常采用基于特征的图像匹配技术.SAR图像匹配中常用的特征可归结为点、线、面三类特征基元[1],其中,点特征是图像结构特征中的最小基元,广泛存在于各种场景的SAR图像中,而且其特征提取和描述方法相对简单,是以点特征匹配成为常见的特征匹配方法.特征匹配算法的前提是两幅图像中必须共同存在这些同名特征并且能够被精确提取和一致描述[2].由于相干成像特性,SAR图像中存在相干斑噪声,使得提取的特征点中包含较多的噪声,容易出现参与匹配的多幅SAR图像中特征点集不一致的现象,所以光学图像点特征匹配中常用的基于几何特征点(如角点、拐点、交点、质心点等)的迭代最近点(Iterative closest point,ICP)算法[3]、粒子群优化[4]等点集匹配方法在SAR图像中较少应用.2052自动化学报39卷目前,SAR图像点特征匹配中应用较多的是几何不变特征匹配方法.该类方法一般采用具有几何不变性的特征提取方法(如SIFT[5−8]、SURF[9])来提取几何不变特征点,或采用具有几何不变性的描述方法来对特征进行描述,如根据点特征之间的相互关系建立不变三角网[9−10]、通过几何哈希方法建立特征描述子[11]等,以消除几何变换对特征匹配的影响来获取点集间的匹配关系.但前者对于服从乘性噪声分布模型的SAR图像难以取得理想效果[6],而且SIFT特征提取方法也难以在异侧成像的SAR图像中寻找到稳健的同名点[5],后者需要在特征提取的基础上,利用三角网组网和直方图建立等特征编组方法来进行特征描述,过程较为复杂,对SAR图像中的斑点噪声比较敏感,容易出现同一场景的不同SAR图像中特征点集的描述结果不同的现象,影响特征匹配的准确性,导致误配、错配甚至匹配不成功的情况出现.所以稳健而普适的图像特征匹配需要采用一种SAR图像中普遍存在、且提取和描述方法都较为简单的特征.以边缘点为基础表示和描述的结构特征,既是图像中的主体信息,相同场景中两幅图像的边缘特征,又代表了共同场景中存在的物理结构,存在某种意义上的相似,所以可以通过提取包含图像结构特征的边缘点集,构建相似性准则,来实现图像匹配.基于边缘点的图像匹配,可以通过建立边缘直方图来进行直方图匹配[12],亦可利用形状描述算子来进行形状匹配[13−14],以建立特征之间的对应关系.但这些方法需在边缘特征的基础上进行复杂的特征编组等处理,同样难以保证匹配特征的一致性,导致这些方法的稳定性和适用性存在不足.为了简化特征提取与描述的过程,Keller等[15]和Yao等[16]、苏泽群等[17]、Wang等[18]基于边缘特征的隐含相似性,利用像素迁移的思想,构建了梯度均方和(Square summation gradient,SSG)相似度,实现了多传感器图像的配准.与其他特征匹配方法相比,像素迁移方法最大的优点在于获取图像特征描述子的过程大为简化,只需进行边缘特征提取即可,而且各类场景和目标图像中基本都存在边缘特征,是以具有较好的普适性.其难点在于,与其他特征匹配方法不同,该方法中待匹配的两幅图像的单对同名点之间没有明确的相似关系,单幅图像中边缘点之间也无明确的关联属性,无法建立待匹配的两幅图像中边缘点集之间的对应关系(如几何关系、统计关系、拓扑关系),同名特征匹配时不能利用特征之间的相互关系来直接进行参数解算或缩小参数搜索范围,而必须通过模型参数全局空间内的优化搜索来实现最优匹配,是以将图像匹配问题转化成了一个数学优化问题[18].而且,文献[15−18]中的方法只利用梯度和来建立相似度准则函数,没有利用更多的边缘点信息来构建匹配准则,使得模型参数解算的收敛速度非常慢,循环时间过长,循环迭代中也只利用SSG参量来进行判决,其循环迭代结束的判决条件不独立,不能对匹配结果从另一个方面进行验证,从而容易陷入局部最优而难以找到全局最优.而且,该方法中仿射变换模型的6个参数不具有明确的几何意义,使得在优化和解算过程中,无法有依据地确定优化搜索空间范围和参数取值分辨率等,导致图像匹配的优化搜索难以收敛或收敛太慢,运行效率过低,也不便于在后续处理中对匹配参数进行分析和理解.针对这些问题,本文提出了基于仿射变换参数分解模型和联合测度的SAR图像边缘特征匹配方法,来增强图像匹配的适应性,提高匹配精度和匹配效率.首先,对仿射变换模型进行参数分解,使得仿射变换的6个参数具有具体的几何意义,据此来有针对性地确定和限制参数范围;其次,利用基于模板的方向ROEWA算子提取SAR图像边缘的强度和方向信息;然后,基于像素迁移思想,建立了多源SAR图像边缘点集相似性匹配准则,以及图像匹配的联合相似度–联合特征均方和(Square summation joint feature,SSJF);最后,采用改进后的遗传算法来进行优化搜索,以快速达到全局最优.1仿射变换模型及参数分解图像之间的变换模型主要包括:刚体变换、相似变换、仿射变换、投影变换和多项式变换等.文献[19]从多视角成像模型的角度,证明仿射变换适合成像平台离场景很远且场景平坦的两幅图像之间的变换.对于遥感图像,地面相对于传感器的距离较远,当地形起伏不大时,可近似认为各同名点的对应Z轴坐标或深度的比值为常数,即近似满足仿射变换,所以可以用仿射变换模型来表示.1.1仿射变换模型仿射变换模型除了考虑两幅图像之间的平移、旋转和缩放外,还考虑了图像切变(即沿对角线的压缩)的几何变化,其变换关系可表达如下:x2=a1x1+b1y1+c1y2=a2x1+b2y1+c2(1)其中,(x1,y1)为待匹配图像坐标,(x2,y2)为参考图像像素坐标,a1,b1,c1,a2,b2,c2为模型参数.6个参数中,c1,c2分别表示图像在X轴和Y轴之间的平移,但a1,b1,a2,b2的具体几何意义不明确,在图像匹配中,无法根据具体情况确定各参数的值域范围和参数取值分辨率,为了避免出现匹配失败的情12期陈天泽等:一种高性能SAR图像边缘点特征匹配方法2053况,一般采取扩大参数值域范围的方法,从而导致搜索时间过长.1.2模型参数分解为了明确a1,b1,a2,b24个参数的几何意义,可以将仿射变换过程分解成缩放、旋转、切变、平移四个步骤[20],具体变换过程如图1所示.图示中的虚线表示变换前图形,实线表示变换后图形,前一幅图中的变换结果为后一幅图中变换的原图.图1仿射变换过程示意图Fig.1Sketch map of the affine transformation procedure若分别令水平放缩尺度、垂直放缩尺度、旋转角度、切变尺度、水平平移和垂直平移参数为s x,s y,θ,r,d x,d y,则式(1)中各参数可如式(2)表示.其中,m=r+√2−r22,n=r−√2−r22.所以式(1)中a1,b1,a2,b24个不具有具体几何意义的参数,经过分解后,可由s x,s y,θ,r这4个具有明确几何意义的参数来进行计算,亦可明确其具体的值域范围.其中水平放缩尺度、垂直放缩尺度s x,s y可根据两幅待匹配图像的分辨率的实际情况进行预估.实际的遥感图像中,水平分辨率和垂直分辨率一般相同,所以水平和垂直放缩尺度也一般相等,即s x=s y.θ的值域范围为[0,360),切变尺度r的值域范围为(0,√2).水平平移和垂直平移d x,d y的值域可根据具体情况确定.a1=s x(m cosθ+n sinθ)a2=s x(n cosθ−m sinθ)b1=s y(n cosθ+m sinθ)b2=s y(m cosθ−n sinθ)c1=d xc2=d y(2)2SAR图像边缘强度和方向特征提取由于SAR图像相干斑噪声一般服从乘性噪声模型而非加性噪声模型,所以传统的差分梯度边缘提取算法在SAR图像中难于取得理想的效果,文献[21]从理论上证明了差分梯度边缘检测算子不适于SAR图像边缘检测.目前,ROA(Ratio of aver-age)算子[22]以及ROEWA(Ratio of exponentially weighted averages)算子[23]是SAR图像中阶跃边缘检测的主要方法.其中,ROEWA算子采用的是多边缘模型,更接近于实际的SAR图像,实用性更强.而且ROEWA算子与梯度算子有很多共性,最重要的就是在边缘处取得极大值,在非边缘处取值较小且变化较小,所以可将ROEWA算子作用后的强度图像认定为梯度图像.ROEWA算子具有恒虚警特性,边缘定位准、虚假边缘少、抗断裂性好,非常适用于检测SAR图像中线状目标的边缘,但不能得到边缘方向.目前用于SAR图像边缘点方向检测的方法很多,文献[24]提出了利用Gabor滤波器计算边缘方向的方法,但整幅图像只能提取四个方向.本文采用改进的ROEWA算法,在计算边缘强度的同时,利用方向模板和二次曲线进行方向估计,得到了边缘方向,具体方法和步骤参见文献[25].该方法的边缘提取结果如图2所示.2054自动化学报39卷图2SAR图像边缘强度和方向特征提取结果Fig.2Extraction of edge strength and direction fromSAR image3基于像素迁移的SAR图像匹配一般来说,特征匹配中常用的几何特征(如点、线、形状、轮廓、骨架等)本质上是梯度较大的边缘点特征.这些梯度较大的边缘点集,可看作是涵盖了大量结构特征和少量无关因素(如孤点大噪声等)的综合.对于具有相同场景的不同图像,这些点集存在着某种意义上的相似.像素迁移,即是从一幅图像中提取部分梯度较大的边缘点构成初始点集,将其各点坐标按照变换模型变换到另一幅图像中,其对应点集即为目的点集[18],通过构建与之适应的相似性测度,然后采取合适的参数优化方法,来实现多幅图像的边缘点集匹配.3.1SAR图像匹配初始点集选择光电图像像素迁移方法中选择的梯度极值一般为道路、河岸、海岸线等线状目标的连续边缘,具有较好的稳定性.但由于电磁散射机理的原因,SAR 图像中的梯度(或强度)极值区域可能包含了部分具有强散射结构、大介电常数材质或粗糙表面的物体(如建筑物、电线杆、金属目标等)对应的强散射图像,这些强散射区域在SAR图像中表现为高亮度和高强度,其边界会掩盖场景中真正的边缘特征,导致边缘不连续,而且会随着SAR图像成像参数的改变而变化,不是边缘特征匹配中所需要的稳定结构特征.所以本文在选取初始边缘点集时,在ROEWA 算子边缘强度特征提取的基础上,选择灰度和边缘强度靠前的连续边缘特征,剔除亮度较高且边缘强度较大的边缘点,如此虽然会去掉一部分强边缘特征,但提高了选取的结构特征的稳定性,可以满足图像匹配的需要.3.2基于像素迁移的相似性匹配准则一般而言,图像匹配可以归结为一个对应关系求解的过程.从匹配准则的角度来说,其数学模型可以描述为[16]minf,T(J)=k[I1(x(k))−f(I2(T(x(k))))]2(3)其中,T为变换模型,I1,I2是图像信息,x(k)代表坐标点集,f表示对图像信息的某种映射,J为准则函数.根据I1,I2中的相互关系确定相似测度,即可获取I1,I2中的对应关系.但基于像素迁移的图像匹配方法中准则函数构造和参数解算与上述方法不同.在准则函数构建中,由于同一区域不同图像的相似特征隐含在梯度最大的点集当中,所以以隐含相似结构特征的梯度最大点集为初始点集,通过坐标变换转换到另一幅图像的目的点集,当不同变换模型中目的点集的梯度值最大时,则该次转换最为有效.因此像素迁移匹配方法中的相似性准则为几何变换后目的点集的SSG 最大,其数学模型为maxT(J)=S2∈I2|I1(T(S2))|2(4)其中,I1,I2表示梯度模值图,S2为I2中最大梯度点集.由于每次迁移对应一个SSG值和一组变换模型参数,因此变换模型可以通过对相似度的迭代优化方法来获得,即当SSG取得最大值时,相似度最大,其对应的变换模型参数即为所求的匹配解.但上述方法在构建相似度时只考虑了边缘的梯度特征.实际上,图像边缘点的方向是边缘点的梯度方向,图像中直线结构特征的边缘点方向相同,是以边缘点方向特征同样也能描述场景结构,图像边缘方向提取实验(如图2(c)所示)证明了这一结论.全局匹配中,两幅待匹配图像中不同同名特征点的方向差应该为一个常数,即两幅图像之间的旋转角度.所以,将一幅图像中边缘点集通过空间变换迁移到另一幅图像的边缘点集中,每次迁移亦对应一组变12期陈天泽等:一种高性能SAR 图像边缘点特征匹配方法2055换参数和一个方向差分均方和(Square summationdifference direction,SSDD)值.当两个边缘点集中对应边缘点的方向差最接近于旋转角度时,两个点集最相似,SSDD 取得最小.该次迁移最为有效,对应的参数即为所求匹配解,根据式(3),则其数学模型为min T(J )=k[D (x 1(k ))−D (x 2(k ))−θ]2(5)其中,D (x 1(k ))和D (x 2(k ))为x 1(k )和x 2(k )的方向值,θ为待匹配的两幅图像之间的旋转角度.所以,为了提高图像匹配中模型参数解算的精度,可以增加隐含特征点集方向关系的约束条件.当两幅图像精确匹配时,应该满足SSG 最大且SSDD 最小(理论上,当图像方向特征提取完全精确时,SSDD 为0),综合两者考虑,将两幅图像的隐含特征点集的SSDD 与SSG 测度进行综合,建立基于联合特征均方和(SSJF)的联合相似测度.3.3联合相似度构建参照文献[16]的方法,我们首先构建了SSG 和SSDD 两个独立相似度:F 1(S 1(P ))=(x i ,y i )∈S 1(P )|I 1(x i ,y i )|2(6)F 2(S 1(P ))=(x i ,y i )∈S 1(P )|∆D (x i ,y i )|2(7)∆D (x i ,y i )=D 1(x i ,y i )−D 2(x i ,y i )−θ(8)其中,设I 1,I 2,D 1,D 2分别为两幅图像的边缘强度图和方向图,P 是模型变换的参数矢量,本文中采用仿射变换模型,θ为两幅匹配图像之间的旋转角度.S 2为图像I 2中强度值在所有强度中靠前的坐标点集,S 1(P )为S 2进行P 变换后迁移到图像I 1中的点集.在参数解算的循环迭代过程中,希望SSG 单调递增,SSDD 单调递减,即当两幅图像精确匹配时,应该满足SSG 最大、SSDD 最小.据此本文定义了基于SSG 和SSDD 的联合测度SSJF:F (S 1(P ))=|αF 1(S 1(P ))−(1−α)F 2(S 1(P ))|(9)其中,α为SSG 测度在联合测度中的权重,其值依据SSG 和SSDD 两个独立测度在联合测度中的相对作用确定.由于像素迁移匹配方法是通过优化解算方法求取SSJF 在图像I 1中的极值,所以α的取值不会影响测度最终的收敛方向和参数解算的精度,但会影响参数解算优化搜索的速度.联合测度中,收敛速度越快的独立测度,其权重应该越大.文中的两个独立测度,各自的收敛速度取决于图像重叠区域中梯度图和方向图的结构性的强弱程度.结构性越强,收敛速度越快.常规场景的图像中,一般较少有显著的直线边缘特征,但大多会有不规则形状的曲线边缘特征,组成这些曲线的边缘点一般具有近似相等的梯度,但其方向变化较大,梯度图的结构性一般强于方向图的结构性,所以在一般情况下,SSG 测度的权重要大于SSDD 测度的权重.此外,在实际应用中,SAR 图像边缘点强度的值域范围与边缘点方向差的值域范围不相等,所以还需要进行规格化,即将两者的值域归化到同一范围.将式(6)和式(7)代入式(9),则:F 1(S 1(P ))=(x i ,y i )∈S 1(P )α|I 1(x i ,y i ) 2−(1−α)|∆D (x i ,y i ) 2(10)每次迁移对应一组变换参数和一个SSJF 值.因此,联合测度值最大时,其相似度也为最大,该次迁移最为有效,对应的参数即为所求匹配解.其数学模型可以写为max P(J )=F (S 1(P ))(11)联合测度的建立,提供了边缘点梯度特征和方向特征两个方面的约束,在极值求解的优化解算中,通过两个独立测度的相互印证,可避免单一测度时陷入搜索空间某一局部范围“陷阱”的情况,加快联合测度的优化迭代效率,并可通过设置边缘点方向误差的阈值来计算相应的SSDD 阈值,作为循环迭代结束的判读依据.4准则函数的优化求解4.1优化解算分析准则函数(11)的优化搜索实质是一个多元函数的优化问题,对于图像匹配来说,该优化问题的数学结构十分清楚,但维数高、空间大、多极值、环境复杂.待求的SSJF 随P 的变化而变得非常复杂,并且存在大量密集局部极值点,因此容易陷入局部最优,对求取全局最优也将非常困难,依靠传统优化算法(包括Powell 、牛顿法、粒子群优化(Particle swarm optimization,PSO)等[15])难以求解.遗传算法(Genetic algorithms,GA)是一种借鉴生物界自然选择和进化机制发展起来的高度并行、随机、自适应搜索算法[26],本文利用遗传算法空间全局搜索能力较强的特点,直接以SSJF 作为个体的适应值,在可行解空间中搜寻最适应个体,也即全局最优解.2056自动化学报39卷4.2遗传算法优化寻优方法的改进现有的遗传算法中,各参数范围以及对应染色体的长度一直保持不变,即各参数的分辨率和可选个数一直不变.这样虽然保证能取得正确匹配解,但在整个循环迭代过程中,收敛速度较慢,而且在设置各参数对应染色体的长度时,为了取得优化效率和解算精度的折中,难以设置范围和高分辨率的染色体长度,使得优化解算难以取得高精度的结果.根据遗传算法优化解算的基本原理分析可知:在循环优化的初始阶段,主要目的是快速稳定地搜寻到匹配解算的初始值,所以需要参数范围大,但取值分辨率可稍低,而且该阶段SSJF极值较小,容易找到最新的SSJF极值,收敛速度较快;在循环优化的后期,主要目的是确定匹配解算的精确值,所以需要取值分辨率较高,但参数范围可压缩,而且该阶段SSJF极值相对较大,难以较快找到新的极值,收敛速度较慢.所以,本文在收敛迭代过程中,基于优化解算逐渐精确的各参数的解,逐步压缩各参数范围来提高收敛速度,同时逐步扩展各参数的染色体长度和种群大小,提高匹配定位的精度.为了保证正确匹配值在修正后的参数范围内,以优化解算的各参数值为中心,重新计算参数范围.对于长度增加的各参数染色体值,为了不改变该次循环中各参数染色体的值,根据格雷码编码规则,对各参数染色体增加的长度以补0方式处理.基于改进的GA的SSJF极值求解步骤如下:步骤1.提取待匹配图像I2中边缘强度值在所有强度中梯度较大的坐标点集,作为每次迁移的原象.步骤2.确定模型变换参数矢量P、设置控制参数(交换率P c、突变率P m、种群大小N s),构建适应度函数,按预定规模进行种群初始化.步骤3.遗传算法优化迭代.a)依适应值最优策略进行后代选择;b)按参数模型变换到I1强度图和方向图中,计算SSDD、SSG和SSJF以及适应值;c)寻找渐优的SSJF极值,否则返回a);d)修正各参数的取值范围和染色体长度以及相应增加的种群大小;e)SSDD小于阈值或者达到最大迭代次数,结束循环,否则返回a).5性能分析图像匹配方法的性能主要包括精度、效率和稳健性三个方面.5.1匹配精度由图像之间的变换模型可知,理论上,基于几何变换的图像匹配精度由变换参数的取值精度和待匹配图像图幅大小决定,参数取值精度由参数取值分辨率衡量.本文方法中,计算量许可的条件下,参数s x,s y, r的取值分辨率可达10−2数量级,参数d x,d y,θ的取值分辨率可达10−1数量级,则sinθ、cosθ的取值分辨率精度可达10−3数量级,所以仿射变换模型系数a1,b1,a2,b2的取值精度的数量级可达10−2×10−1×10−3,即10−6,c1,c2可达10−1.设图像尺寸的数量级为10x,则最终匹配精度数量级为max(10x−6,10−1).SSG方法中,计算量相等(即6个参数染色体总长度相等)的条件下,参数a1,b1的取值精度的数量级可达10−3,c1,c2的取值精度数量级仍为10−1,所以最终精度数量级为max(10x−3,10−1).遥感图像的图幅尺寸一般为103到104数量级,则本文方法匹配精度可达10−1数量级,SSG方法匹配精度为100到101数量级.由此可以看出,在遥感图像匹配中,本文方法的匹配精度比SSG方法的匹配精度可高出1至2个数量级.而且,要想进一步提高匹配精度,本文方法只需提高d x,d y即c1,c2的取值精度即可,但SSG方法还需提高a1,b1,a2,b2的取值精度,如此则计算量会大大增加.5.2解算效率利用本文方法的关键在于参数空间范围内找到SSJF的最优,所以匹配效率主要取决于参数循环的计算量,而计算量由运行代数和每代循环的计算量共同决定.利用本文方法可以大大减少计算量,较好地提高搜索效率.首先,SSG方法中,因为大部分参数不具有具体的几何意义,实际匹配中无法确定相对精确的参数范围,所以参数的值域范围无法确定,为了确保匹配成功,只得扩大值域范围,使得遗传算法中的染色体长度增加,种群大小变大,运行代数也需相应增加,所以增加了计算量.本文方法中的各参数具有具体的几何意义,各参数的初始范围可以根据实际情况较好地确定,能大大减少运行代数和每次循环的计算量.其次,原来方法中的6个参数是直接得到的,而本文的参数需要经过进一步的乘法运算后才能得到仿射变换参数,所以为了得到相同的参数取值精度,本文方法中染色体长度大大减小,可大大减少计算量.此外,SSG方法中,只利用SSG特征来进行相似性评估,没有其他条件进行约束,所以需要大量的匹配点才能保证不出现错匹配情况.本文方法利用联合测度来进行相似性计算,可以从边缘。
基于激光雷达的无人驾驶3D多目标跟踪
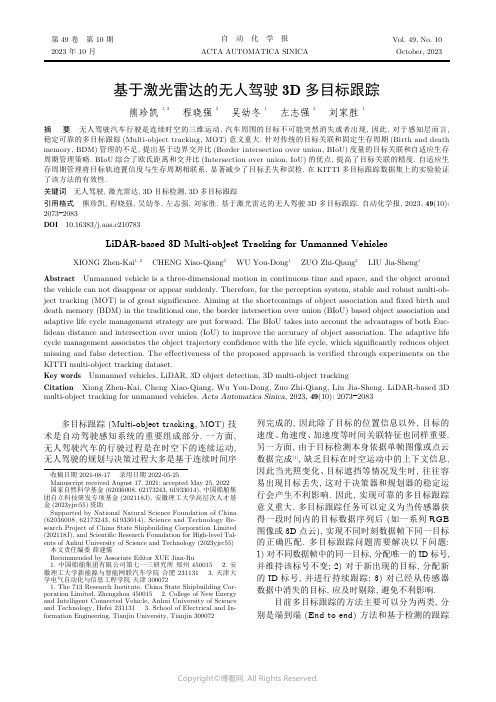
基于激光雷达的无人驾驶3D 多目标跟踪熊珍凯 1, 2程晓强 3吴幼冬 1左志强 3刘家胜1摘 要 无人驾驶汽车行驶是连续时空的三维运动, 汽车周围的目标不可能突然消失或者出现, 因此, 对于感知层而言,稳定可靠的多目标跟踪(Multi-object tracking, MOT)意义重大. 针对传统的目标关联和固定生存周期(Birth and death memory, BDM)管理的不足, 提出基于边界交并比(Border intersection over union, BIoU)度量的目标关联和自适应生存周期管理策略. BIoU 综合了欧氏距离和交并比(Intersection over union, IoU)的优点, 提高了目标关联的精度. 自适应生存周期管理将目标轨迹置信度与生存周期相联系, 显著减少了目标丢失和误检. 在KITTI 多目标跟踪数据集上的实验验证了该方法的有效性.关键词 无人驾驶, 激光雷达, 3D 目标检测, 3D 多目标跟踪引用格式 熊珍凯, 程晓强, 吴幼冬, 左志强, 刘家胜. 基于激光雷达的无人驾驶3D 多目标跟踪. 自动化学报, 2023, 49(10):2073−2083DOI 10.16383/j.aas.c210783LiDAR-based 3D Multi-object Tracking for Unmanned VehiclesXIONG Zhen-Kai 1, 2 CHENG Xiao-Qiang 3 WU You-Dong 1 ZUO Zhi-Qiang 3 LIU Jia-Sheng 1Abstract Unmanned vehicle is a three-dimensional motion in continuous time and space, and the object around the vehicle can not disappear or appear suddenly. Therefore, for the perception system, stable and robust multi-ob-ject tracking (MOT) is of great significance. Aiming at the shortcomings of object association and fixed birth and death memory (BDM) in the traditional one, the border intersection over union (BIoU) based object association and adaptive life cycle management strategy are put forward. The BIoU takes into account the advantages of both Euc-lidean distance and intersection over union (IoU) to improve the accuracy of object association. The adaptive life cycle management associates the object trajectory confidence with the life cycle, which significantly reduces object missing and false detection. The effectiveness of the proposed approach is verified through experiments on the KITTI multi-object tracking dataset.Key words Unmanned vehicles, LiDAR, 3D object detection, 3D multi-object trackingCitation Xiong Zhen-Kai, Cheng Xiao-Qiang, Wu You-Dong, Zuo Zhi-Qiang, Liu Jia-Sheng. LiDAR-based 3D multi-object tracking for unmanned vehicles. Acta Automatica Sinica , 2023, 49(10): 2073−2083多目标跟踪 (Multi-object tracking, MOT) 技术是自动驾驶感知系统的重要组成部分. 一方面,无人驾驶汽车的行驶过程是在时空下的连续运动,无人驾驶的规划与决策过程大多是基于连续时间序列完成的, 因此除了目标的位置信息以外, 目标的速度、角速度、加速度等时间关联特征也同样重要.另一方面, 由于目标检测本身依据单帧图像或点云数据完成[1], 缺乏目标在时空运动中的上下文信息,因此当光照变化、目标遮挡等情况发生时, 往往容易出现目标丢失, 这对于决策器和规划器的稳定运行会产生不利影响. 因此, 实现可靠的多目标跟踪意义重大. 多目标跟踪任务可以定义为当传感器获得一段时间内的目标数据序列后 (如一系列RGB 图像或3D 点云), 实现不同时刻数据帧下同一目标的正确匹配. 多目标跟踪问题需要解决以下问题:1) 对不同数据帧中的同一目标, 分配唯一的ID 标号,并维持该标号不变; 2) 对于新出现的目标, 分配新的ID 标号, 并进行持续跟踪; 3) 对已经从传感器数据中消失的目标, 应及时剔除, 避免不利影响.目前多目标跟踪的方法主要可以分为两类, 分别是端到端 (End to end) 方法和基于检测的跟踪收稿日期 2021-08-17 录用日期 2022-05-25Manuscript received August 17, 2021; accepted May 25, 2022国家自然科学基金(62036008, 62173243, 61933014), 中国船舶集团自立科技研发专项基金(202118J), 安徽理工大学高层次人才基金(2023yjrc55)资助Supported by National Natural Science Foundation of China (62036008, 62173243, 61933014), Science and Technology Re-search Project of China State Shipbuilding Corporation Limited (202118J), and Scientific Research Foundation for High-level Tal-ents of Anhui University of Science and Technology (2023yjrc55)本文责任编委 薛建儒Recommended by Associate Editor XUE Jian-Ru1. 中国船舶集团有限公司第七一三研究所 郑州 4500152. 安徽理工大学新能源与智能网联汽车学院 合肥 2311313. 天津大学电气自动化与信息工程学院 天津 3000721. The 713 Research Institute, China State Shipbuilding Cor-poration Limited, Zhengzhou 4500152. College of New Energy and Intelligent Connected Vehicle, Anhui University of Science and Technology, Hefei 2311313. School of Electrical and In-formation Engineering, Tianjin University, Tianjin 300072第 49 卷 第 10 期自 动 化 学 报Vol. 49, No. 102023 年 10 月ACTA AUTOMATICA SINICAOctober, 2023(Tracking by detection) 方法. 前者将目标检测与跟踪视为一个统一的过程, 输入单帧图像或点云数据, 直接输出带有唯一ID标号的目标检测框信息;后者则将检测与跟踪视为递进过程, 首先使用目标检测网络如Complex-YOLO[2], PointRCNN[3] 获得检测框位置, 然后再使用目标在多帧数据中的时空联系性进行关联, 获得跟踪结果. 代表方法包括SORT (Simple online and real time tracking)[4]、Deep-SORT (SORT with a deep association metric)[5]和AB3DMOT (A baseline for 3D multi-object tracking)[6]. 其中AB3DMOT将2D多目标跟踪问题扩展到3D多目标跟踪任务, 提出了一种简洁高效的实时多目标跟踪框架, 并在KITTI数据集上验证了出色效果, 实时性达到了200帧/s, 是3D多目标跟踪领域的经典之作.本文在分析AB3DMOT跟踪算法的基础上,针对原算法中的以下两点问题进行了研究: 1) 目标关联度计算在基于检测的跟踪方法中起到了突出作用, 原AB3DMOT算法使用传统的交并比 (Inter-section over union, IoU) 作为度量指标, 因此存在当两个检测框不相交时IoU = 0的问题[7−8], 造成匹配失败; 2) 目前的MOT算法中大多都会用到生存周期 (Birth and death memory, BDM) 策略, 用于降低漏检率、获得更好的跟踪效果, 但多采用固定生存周期, 对所有目标进行无差别处理, 并未考虑检测结果本身置信度对跟踪的影响. 针对上述问题,本文提出了一种基于边界交并比 (Border intersec-tion over union, BIoU) 度量的自适应多目标跟踪算法, 主要创新点包括:1) 提出了BIoU度量方法用于计算检测结果的关联性矩阵, 相比于单一使用欧氏距离或传统IoU 度量的方法, BIoU可以有效解决无交集和奇点问题, 获得更可靠的多目标跟踪结果;2) 提出了一种自适应的生存周期管理策略, 将检测结果本身的置信度与生存周期关联起来, 可以有效避免由于遮挡导致的跟踪失败和由于误检导致的错误跟踪的情况.基于KITTI多目标跟踪数据集[9]进行的实验表明, 本文提出的基于BIoU和自适应生存周期管理的多目标跟踪算法, 相比于原算法可以有效地提高跟踪的准确性和鲁棒性.1 研究现状1.1 2D/3D多目标跟踪任务多目标跟踪问题按照跟踪目标的状态种类可以分为2D多目标跟踪和3D多目标跟踪. 其中2D多目标跟踪主要用于图像领域的目标跟踪任务, 如安防监控、军事侦察、自然观测等领域[10]. DeepSORT[5]算法引入马氏距离度量和级联匹配用于提高跟踪精度. Leal-Taixé 等[11]介绍了一种两阶段深度学习跟踪算法: 使用局部时空编码聚合像素和光流信息,通过梯度增强分类器将图像上下文特征与CNN输出相结合. 孟琭等[12]详细说明了光流法、相关滤波法和深度学习方法在目标跟踪领域的应用效果. 与2D多目标跟踪相比, 基于点云数据的3D多目标跟踪具有较为准确的深度估计, 此类方法大多基于运动学模型. Azim等[13]利用卡尔曼滤波器对获得的相邻两帧激光点云基于八叉树的占据栅格地图分析其不一致信息, 从而检测动态物体; 再使用全局最近邻的方法进行数据关联; 最后基于卡尔曼滤波跟踪动态物体的中心点. Song等[14]采用一种多任务的稀疏学习算法来选取最佳的候补对象, 提高了复杂环境下的跟踪效果. 为了验证有效的深度估计对目标跟踪性能的影响, Sharma等[15]使用基于3D 点云校准的2D图像进行目标跟踪, 考虑了三维空间信息, 有效缓解了基于RGB图像中的深度估计不准确和遮挡问题, 实现了较好的跟踪效果. 2020年, Weng等[6]将2D跟踪算法SORT的思想迁移到3D点云中, 提出AB3DMOT算法, 无需GPU训练即可实现优秀的跟踪性能.1.2 关联度量目标匹配是多目标跟踪中的重要环节, 有效度量预测目标与检测目标之间的关联性, 是获得可靠匹配结果的关键. 常用的匹配方法包括基于外观显著性特征的匹配方法[16−17]和基于空间位置相关性的匹配方法[18−19]. 与2D图像相比, 3D点云数据更为稀疏, 外观特征不明显, 因此更常采用空间位置的相关性进行匹配. IoU和目标间距离是常用的两种度量方法. SORT[4]和AB3DMOT[6]算法中均使用预测框与检测框的IoU值作为关联度量, 再利用匈牙利算法进行匹配. 使用原始IoU进行关联度量存在两个问题: 1) 当预测框与检测框无交集时IoU = 0,此时无法获得有效的度量信息; 2) 多个预测框可能与检测框具有相同的IoU值, 如图1(a)中的情况.另一种方法是使用目标间距离作为度量指标, 如预测框与检测框中心点之间的欧氏距离[19]. 但使用距离度量同样存在不同的预测框与目标框的距离相同的情况. 如图1(b), 虽然蓝色和红色表示的两个预测框差异很大, 但它们与检测框中心的欧氏距离是相同的. 近年来, 使用深度网络来学习目标关联性2074自 动 化 学 报49 卷特征的方法也得到了诸多研究, 如Wu 等[18] 将Point-RCNN 检测器得到的特征也作为关联度量的要素加入度量过程, 从而获得更可靠的度量结果.1.3 生存周期管理在现有的多目标跟踪算法中, 会使用生存周期管理策略[5−6, 18]. 一方面, 当出现因为遮挡造成的目标丢失情况时, 生存周期管理策略可以保证在一段时间内仍然可以持续跟踪目标而不会立刻丢失; 另一方面, 当出现误检情况时, 由于生存周期管理策略要求目标连续检测多帧, 所以可以有效过滤掉单帧误检的目标. 目前通常使用的是固定周期的管理策略, 即对所有目标进行相同时长跟踪用于确定目标或删除目标. 而在实际应用中, 考虑到目标检测单元会输出检测框的置信度, 用于表征检测结果的可靠性, 因此, 可以根据置信度对不同目标进行自适应生存周期管理, 即: 对置信度较高的目标可以保持更长时间以解决遮挡造成的目标漏检; 对置信度较低的目标在发生误检后应尽快删除.2 基于BIoU 的3D 多目标跟踪2.1 问题描述z z 多目标跟踪的主要任务是在给定一个图像序列的前提下, 找到图像序列中待检测目标, 并将不同帧中检测到的目标进行关联, 得到目标的运动信息,给每一个运动目标一个固定准确的ID 标号. 对于3D 目标检测, 一方面其天然克服了基于RGB 图像中的2D 目标检测的遮挡与目标位置估计不准确的问题, 使得基于运动估计的在线跟踪算法易于应用;另一方面, 对于3D 目标检测的点云数据而言, 缺乏类似RGB 图像那样丰富的语义特征, 导致难以使用特征描述的方式进行跟踪. 因此, AB3DMOT [6]算法仅使用简单朴素的跟踪策略就实现了高效实时的目标跟踪效果. 但是, 该方法在匈牙利匹配环节中使用原始的3D IoU 作为成本度量, 而无人驾驶汽车的多目标跟踪本质仍然是一种近似的2D 平面运动, 其在 轴方向上变化较小, 导致目标检测在 轴方向上的估计将对跟踪性能产生较大影响, 同时由于IoU 度量的局限性, 本文提出BIoU 作为一种新的成本度量融入到匈牙利匹配之中. 目标的生存周期管理是目标跟踪的重要环节, 生存周期设置过短在目标检测不稳定时会造成较为频繁的ID 切换,生存周期过长容易增加错误跟踪和目标误检. 因此,通过对跟踪轨迹的置信度打分, 本文设计了自适应的生存周期管理机制, 动态地调整目标的生存周期减少了ID 切换和目标误检, 实现了较好的跟踪性能.如图2所示, 本文所提出的3D 多目标跟踪的整体流程可以分为以下几个部分:1) 使用3D 检测器获得目标检测框;2) 使用3D 卡尔曼滤波器获得上一帧的目标预测框;3) 基于BIoU 计算检测框与预测框的关联度,使用匈牙利匹配算法获得最优匹配结果;4) 使用3D 卡尔曼滤波对所有匹配目标进行状态更新;5) 对未匹配成功的目标进行生存周期管理;6) 输出具有唯一ID 标号的目标边框.2.2 卡尔曼滤波卡尔曼滤波[20]是目前状态估计应用最为广泛的估计优化算法, 它能够根据过去的信号信息, 利用统计计算的原理, 优化最小均方误差, 从而预测出未来的状态量. 卡尔曼滤波是对时间参数估计的yyx(a) 不同预测框和检测框具有相同 IoU(a) Different predicted boxes have same IoUs withthe detected box(b) 不同预测框和检测框具有相同欧氏距离(b) Different predicted boxes have same Euclideandistances with the detected boxxOO检测框检测框2预测框2预测框1预测框1预测框图 1 IoU 度量和欧氏距离度量失效情况Fig. 1 Invalid cases about IoU metrics andEuclidean distance metrics10 期熊珍凯等: 基于激光雷达的无人驾驶3D 多目标跟踪2075最小二乘逼近, 能够建立起状态变量随时间变化的方程, 从而估计出今后某个时刻的状态.卡尔曼滤波算法的核心包括如下几个方程:1) 描述预测过程中, 系统的状态向量预测方程A B U kk ˆXk k X k −1k −1W k −1k −1其中, 为状态转移矩阵, 为控制输入矩阵, 表示 时刻的控制输入向量, 表示 时刻状态的预测值, 表示 时刻的状态输出值, 为 时刻的状态转移过程中的随机干扰噪声, 表现为均值为零的高斯白噪声.2) 描述先验估计协方差矩阵的更新过程Q W k ˆPk k 其中, 为状态转移过程噪声 的协方差矩阵, 为时刻的先验估计协方差矩阵的预测值.3) 描述观测模型中由系统观测值得到系统观测向量的过程H Z k k V k 其中, 为状态观测矩阵, 为 时刻状态变量的观测值, 为状态观测过程中的随机干扰噪声向量, 表现为均值为零的高斯白噪声.4) 卡尔曼滤波增益方程 (权重)K k k R V k 其中, 表示 时刻的卡尔曼增益, 为观测噪声 的协方差矩阵.k 5) 卡尔曼滤波估计方程 ( 时刻的最优状态估计)X k k 其中, 表示 时刻状态向量经过卡尔曼滤波后的最优估计, 是系统的实际输出值, 表现为在预测值的基础上按照卡尔曼滤波增益叠加一个预测误差项.k 6) 卡尔曼滤波协方差估计方程( 时刻的最优协方差估计)P k k 其中, 为 时刻卡尔曼滤波的后验估计协方差矩阵的预测值. 该方程用来描述状态向量协方差矩阵的变化过程, 正是这种不断更新的机制才能保证卡尔曼滤波不断克服随机噪声的影响.卡尔曼滤波算法能够在不准确的测量数据基础上尽可能地消除噪声的影响, 对真实值做出预测. 目标跟踪算法把跟踪目标的属性作为状态变量, 利用卡尔曼滤波器对属性进行线性滤波, 得到更好的预测值.2.2.1 状态预测为了预测上一帧到当前帧的目标状态, 这里使用恒定速度模型估计对象间的帧间位移, 将目标状态表示成一个11维向量, 即x y z l wh θs v x v y v z x y z 其中, , 和 分别表示目标的中心点坐标, , 和 分别表示目标的3D 边框尺度, 为目标边框的航向角, 表示当前轨迹的置信度分数, , 和 分别为目标在三维空间中沿 , 和 轴方向上的运动速度.k −1第 帧数据中的所有目标状态构成的集合表示为ξik −1k−1i m k −1k −1k −1k 其中, 表示在 时刻的第 个目标状态, 表示在 时刻存在的目标数量. 通过 时刻的目标状态, 根据恒定速度模型可以估计出第 帧的目标状态. 目标的预测位置为k −1ξik −1k ξik 对于 时刻的每个目标状态 都可以给出 时刻的预测状态 .新出现的轨迹与目标丢失的轨迹完成匹配的目标预测目标基于 BIoU 度量的目标关联自适应生存周期管理具有唯一ID 的目标检测目标匹配失败的跟踪目标轨迹关联原始点云3D 目标检测3D 卡尔曼滤波T kT k − 1匹配失败的检测目标图 2 基于BIoU 和自适应生存周期的3D 多目标跟踪Fig. 2 3D multi-object tracking based on BIoU and adaptive birth and death memory2076自 动 化 学 报49 卷2.2.2 状态更新根据数据关联的结果, 可以将跟踪目标与检测目标分为4类: 跟踪匹配成功、检测匹配成功、跟踪匹配失败和检测匹配失败. 它们各自的具体形式为T match D match w k T unmatch D unmatch m k −1n k D match 其中, 和 表示匹配成功的跟踪目标与检测目标, 表示当前时刻匹配成功的数量,和 分别表示匹配失败的跟踪目标和检测目标, 表示上一时刻的所有跟踪目标数量, 表示当前时刻的检测目标数量. 完成匹配之后, 使用匹配成功的目标 根据贝叶斯规则更新对应的目标状态.2.3 基于BIoU 的匈牙利匹配算法为了解决传统IoU 度量或距离度量的失效问题, 本文设计了一种结合欧氏距离与IoU 的综合度量指标, 即BIoU 度量, 它由原始IoU 和边界距离惩罚项共同组成, 具体形式为IoU (B 1,B 2)R BIoU γp lt 1,p rb 1,p lt 2,p rb 2ρ(·)C max (B 1,B 2)IoU (B 1,B 2)ρ(p lt 1,p lt 2)ρ(p rb 1,p rb 2)C max (B 1,B 2)其中, 表示一般意义下的两个边界框的交并比, 为基于边界欧氏距离的惩罚项, 是惩罚项因子, 分别表示两个边界框最小外接框的左上顶点和右下顶点, 函数 为两个顶点间的欧氏距离, 表示两个边界框最小外接框的最大对角线距离, 用于对边界距离进行归一化处理. 需要说明的是, 采用最小外接框的方法可以弱化旋转对边界距离的影响, 便于计算边界距离.图3(a)展示了2D BIoU 的计算方法. 绿色和蓝色实线框代表两个不同的边界框, 虚线框为它们各自的最小外接框, 灰色区域表示 ,红色线段表示边界距离 和 ,黄色线段表示最大对角线距离 .对于3D 多目标跟踪, 本文将上述2D BIoU 的定义扩展到3D 坐标系下, 如图3(b)所示. 3D BIoU 的计算式为IoU 3D (V 1,V 2)V 1V 2R BIoU 3D p lft 1,p lft 2,p rrb 1,p rrb 2ρ(p lft 1,p lft 2)ρ(p rrb 1,p rrb 2)C max (V 1,V 2)BIoU thres BIoU 3D <BIoU thres 其中, 表示两个3D 边界框 和 的体积交并比 (图中的灰色区域), 惩罚项 与边界距离相关, 分别是两个3D 边界框最小外接框的左−前−上顶点和右−后−下顶点, 和 分别是对应边界距离 (图中的红色线段), 则表示两个最小外接框所有顶点间的最大对角线距离 (图中的黄色线段). 在给定阈值 的情况下, 当 时,则认为两个3D 边界框匹配失败, 即两个3D 边界框分别属于独立的两个不同目标.2.4 自适应生存周期管理策略F min F max 在多目标跟踪中, 现有目标会离开视野, 也可能有新目标进入视野, 因此需要一个模块来管理轨迹的产生和删除. 生存周期管理是常见的做法: 将所有未成功匹配的检测目标视为潜在的新轨迹, 为了避免跟踪误报, 只有在该目标连续检测到 帧后才认为是一个新的目标轨迹; 将所有未成功匹配的跟踪目标结果视为即将离开视野的轨迹, 为了避免误删轨迹, 只有该目标未成功匹配 帧后才视为目标丢失并将其删除. 理想情况下, 该策略可以保留因为单帧漏检未能成功匹配的目标轨迹, 并仅删除已经离开视野的目标轨迹. 但在实际中, 3D 目p lft 1p lt 1p lt 2p lft 2p rrb 1p rrb 2p rb 1p rb 2m a x (V 1, V 2)ma x(B1, B 2)IoU 3D (V 1, V 2 )IoU (B 1, B 2 )V 2B 2B 1V 1r (p rrb 1, p rrb 2)r (pr b1, pr b2)r (p l f t 1, p l f t 2)r (pl t 1, pl t 2)(a) 2D 边界交并比(a) 2D BIoU (b) 3D 边界交并比(b) 3D BIoU图 3 边界交并比示意图Fig. 3 Schematic diagram of BIoU10 期熊珍凯等: 基于激光雷达的无人驾驶3D 多目标跟踪2077标检测器的误检和漏检普遍存在, 采用固定的生存周期管理策略, 将出现错误的跟踪轨迹. 原因主要是固定的生存周期管理策略未有效利用检测目标的置信度信息, 而对所有目标均进行相同周期的检查操作, 从而导致检测置信度较低的目标 (往往为误检目标) 也需要跟踪多帧后才会被删除, 而检测置信度较高的目标一旦被多帧遮挡 (往往出现漏检)也可能被删除.因此, 本文提出一种自适应生存周期管理策略,根据目标检测结果的置信度, 动态调整最大生存周期, 具体为score αβσ(·)F max F Amax αβF max =3,α=0.5,β=−5其中, 为当前目标的检测置信度, 和 为尺度系数和偏移系数, 表示Sigmoid 非线性函数, 为最大生存周期, 为根据目标检测置信度计算后的生存周期. 通过选取合适的 和 , 实现更好的跟踪效果. 图4给出了当 时生存周期与检测置信度之间的关系. 利用Sig-moid 函数的S 型曲线特性, 检测目标的置信度越高, 该目标的生存周期将会越长, 从而实现生存周期动态调整.32F A m a x10010置信度−102030图 4 自适应生存周期Fig. 4 Adaptive birth and death memory3 实验结果与分析3.1 数据集与评价指标介绍本实验基于KITTI 数据集的多目标跟踪基准进行评估, 该基准由21个训练序列和29个测试序列组成. 对于每个序列都提供了LiDAR 点云、RGB 图像以及校准文件. 用于训练和测试的数据帧数分别为8 008和11 095. 对于测试集数据, KITTI 不向用户提供任何标签, 而是在服务器上保留标签以进行MOT 评估. 对于训练集数据, 含有30 601个目标和636条轨迹, 同样包括Car 、Pedestrian 和Cyclist 类别. 由于本文使用的目标跟踪系统是基于卡尔曼滤波算法的, 不需要进行深度学习训练, 因此将所有21个训练序列作为验证集进行验证. 实验对比了Car 、Pedestrian 和Cyclist 全部3个类别上的多目标跟踪效果.对于多目标跟踪方法, 理想的评价指标应当能够同时满足3个要求: 1) 所有出现的目标都能够及时被发现; 2) 找到的目标位置应当尽可能和真实的目标位置一致; 3) 应当保持目标跟踪的一致性, 避免目标标志的频繁切换. 根据上述要求, 传统的多目标跟踪将评价指标设置为以下内容: 多目标跟踪准确度 (Multi-object tracking accuracy, MOTA)用于确定目标的个数以及跟踪过程中的误差累计,多目标跟踪精度 (Multi-object tracking precision,MOTP) 用于衡量目标位置上的精确度, 主要跟踪轨迹命中率 (Mostly tracked, MT), 主要丢失轨迹丢失率 (Mostly lost, ML), 身份切换次数 (ID swit-ch, IDS), 跟踪打断次数 (Fragment, FRAG) 等.3.2 实验结果实验流程如图5所示, 其中3D 目标检测器使用与A B 3D M O T 算法相同的、已经训练好的PointRCNN 模型. 在目标匹配阶段, 使用本文提出的BIoU 计算预测框与目标框之间的关联度, 然后使用匈牙利匹配算法进行目标匹配. 成功匹配的目标送入卡尔曼滤波器进行状态更新, 未成功匹配的检测目标和预测目标均送入自适应周期管理模块进行判定. 自适应周期管理模块根据目标的置信度分数自适应调整目标的最大生存周期, 删除已达到最大生存周期而仍未匹配成功的目标, 最终得出具备唯一ID 标号的目标轨迹. BIoU 和自适应生存周期管理中涉及的参数通过整定得到, 最终模型使用的参数如表1所示.为了验证本文所提出的基于BIoU 度量和自适应生存周期管理多目标跟踪算法的性能, 本文与基准AB3DMOT 算法在KITTI 多目标跟踪数据集的3类目标上进行了对比; 同时在Car 类别上还与另外两种端到端的深度学习算法FANTrack [21] 和DiTNet [22] 进行了比较. 实验结果如表2所示.表2的结果表明, 本文提出的基于BIoU 度量和自适应生存周期管理策略的多目标跟踪算法相比于基准算法在3类目标上均获得了更高的MT 值,意味着本文算法在对目标的长时间跟踪性能上明显优于基准算法; 在Pedestrian 和Cyclist 两个类别上, 跟踪准确度MOTA 较基准算法有显著提升, 而在Car 类别上与基准算法基本一致. 最值得注意的是, 本文算法在命中率MT 和丢失率ML 这两项指标上均有显著提升, 说明了本文算法在长时间稳定2078自 动 化 学 报49 卷跟踪目标这个问题上具有明显优势. 另外, 在Ped-estrian和Cyclist两类目标的跟踪任务上获得更好的效果, 也说明了基于BIoU的目标跟踪可以有效提高对小目标的跟踪性能. 分析其原因, 可以发现小目标更易于出现检测框与预测框交集较少或无交集的情况, 这也正是BIoU相较于传统IoU最大的优点, 即解决IoU相等或IoU = 0时的关联度量问题.图6和图7分别展示在出现误检和漏检情况时, 本文所提出改进跟踪算法和基准算法的跟踪结果. 在图6的第2行中, 两类算法均出现了误检的情况 (用红色圆圈标记), 但相较于基准算法(图6(a)的第1行), 由于引入了自适应生存周期管理,因此在下一帧数据中, 改进跟踪算法及时将误检目标删除, 而基准跟踪算法仍会对误检目标进行持续跟踪, 直到生存周期结束. 上述结果表明基于本文改进的跟踪算法可以明显减小误检目标的生存周期, 降低误检次数.同样, 对于目标漏检的情况, 本文算法也能给出更好的结果. 图7 (a)的第3行中, 由于红色圆圈标记的目标被其他物体遮挡而导致漏检, 在下一帧中, 该目标再次被检测到时, 已被标记为新的目标(这一点从对比图7(a)的第2行和图7(a)的第4行中检测框颜色不一致可以看出). 而使用本文提出的改进算法, 即使在图7(b)的第3行中出现了遮挡,因为生存周期并未结束, 因此目标仍然在持续跟踪, 也未发生身份切换的问题. 这说明, 在由于目标遮挡等问题出现漏检时, 本文提出的改进跟踪算法可以有效克服漏检问题, 保持目标持续跟踪与ID 恒定.3.3 消融实验对比F max=5F maxF AmaxF Amax为了进一步说明BIoU度量和自适应生存周期管理策略的实际效果, 本文在KITTI验证集上进行了消融实验. 为了便于比较, 本文设置最大生存周期; 对于固定生存周期策略, 生存周期即为; 对于自适应生存周期策略, 采用式 (13) 描述的关系自适应计算生存周期. 消融实验的结果如表3所示, 其中不使用BIoU和的跟踪方法即为原始AB3DMOT算法.首先, 对BIoU进行性能分析. 在表3中, 每类目标的第2行数据与第1行相比, 区别在于使用BIoU表 1 模型参数Table 1 Model parameters参数值说明γ0.05BIoU惩罚因子α0.5生存周期尺度系数β4生存周期偏移系数F max3 (Car)5 (Others)最大生存周期对Car目标为3对其他类别目标为5F min3目标轨迹的最小跟踪周期该值与AB3DMOT相同BIoU thres−0.01BIoU阈值小于阈值认为匹配失败具有唯一 ID 标号的跟踪结果跟踪结果自适应生存周期管理基于 BIoU 的目标匹配卡尔曼滤波器匹配失败检测目标检测目标3D 目标检测网络匹配失败预测目标预测目标匹配目标上一时刻轨迹当前时刻轨迹轨迹关联新加入轨迹与需要丢弃轨迹32FAmax1010置信度−102030p ltp ltB21X^k = AX k − 1k −+ BU k + WP^k = AP k − 1A T + QZk= HX k+V kKk= P^k H T(HP^k H T + R)−1Xk= X^k + K k(Z k−HX^k)Pk= (I−K k H)P^k B1d1d2max(B1, B2)p rbp rb图 5 基于激光雷达的3D多目标跟踪整体流程Fig. 5 Overall pipeline for LiDAR-based 3D multi-object tracking10 期熊珍凯等: 基于激光雷达的无人驾驶3D多目标跟踪2079。
基于交点的新层次聚类算法

ITNS主题专栏:人工智能技术研究•Artificial Intelligence technology research基于交点的新层次聚类算法李青旭,陈天鹰,胡波(华北计算机系统工程研究所,北京100083)摘要:介绍了一种新的分层聚类算法,该聚类算法的主要目的是利用交点提供更好的聚类质量和更高的准确性$为了验证该聚类算法,对基准数据集进行了几次实验,并与其他五种广泛使用的聚类算法进行对比$使用纯度作为外部标准来评估聚类算法的性能,并计算了由聚类算法得出的每个聚类的紧密度,以评估聚类算法的有效性$实验结果表明,在大多数情况下,该算法的错误率低于研究中使用的其他聚类算法$关键词:数据挖掘;无监督学习;聚类分析;聚类算法;分层聚类中图分类号:TP393文献标识码:6DOI:10.19358/j.issn.2096-5133.2020.10.004引用格式:李青旭,陈天鹰,胡波•基于交点的新层次聚类算法[J].信息技术与网络安全,2020,39(10):18-22.New hierarchical clustering algorithm based on intersectionLi Qingxu,Chen Tianying,Hu Bo(National Computer System Engineering Research Institute of China,Beijing100083,China)Abstract:This paper introduces a new hierarchical clustering algorithm.The main purpose of this clustering algorithm is to provide better clustering quality and higher accuracy by using intersections.In order to verify this clustering algorithm, we conducted several experiments on the benchmark data set.In addition to the algorithm we proposed,five well-known clustering algorithms were also used.The purity was used as an eVternal standard to evaluate the performance of the clustering algorithm ,and the tightness of each cluster obtained by the clustering algorithm was also calculated to evaluate the effectiveness of the clustering algorithm.Finally,the experimental results show that in most cases,the error rate of the proposed algorithm is lower than other clustering algorithms used in this study.Key words:data mining;unsupervised learning;cluster analysis;clustering algorithm;hierarchical clustering0引言由于处理的数据量每天都在增加,因此能够检测数据结构并识别数据集中的子集的方法变得越来越重要"聚类是这些方法中的一种"聚类或聚类分析是一项无监督的归纳学习任务,它基于各个点之间的相似性将数据组织到同质的组中"聚类是机器学习,是数据挖掘和统计中已研究的基本问题之一[1-3]"聚类方法可以产生与分类方法相同的结果,但是不存在预定义的类,因此也可以视为无监督分类[4-']"聚类算法的性能可以通过其发现数据集中某些或所有隐藏模式的能力来衡量,可以通过测量数据点之间的相似性(不相似性)来发现隐藏的模式"相似度表示在明确定义的意义上测得的数学相似度,通常使用距离函数进行定义,根据聚类算法的规则,可以测量数据点本身之间或数据点与某个特殊点之间的距离"同时,随着数据的划分,同一群集中的数据点应尽可能相似,而不同群集中的数据点应尽可能不相似[6-+]"多年来,已经开发岀多种不同的聚类方法。
APPARATUS AND METHOD FOR DIFFERENTIAL BEAMFORMING
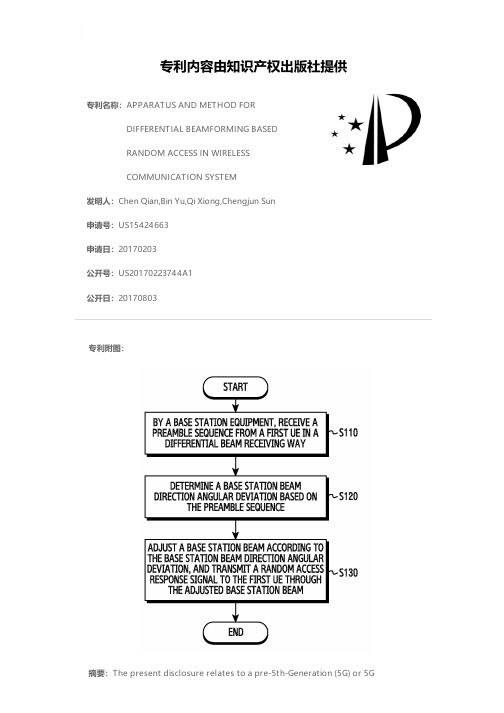
专利名称:APPARATUS AND METHOD FORDIFFERENTIAL BEAMFORMING BASEDRANDOM ACCESS IN WIRELESSCOMMUNICATION SYSTEM发明人:Chen Qian,Bin Yu,Qi Xiong,Chengjun Sun申请号:US15424663申请日:20170203公开号:US20170223744A1公开日:20170803专利内容由知识产权出版社提供专利附图:摘要:The present disclosure relates to a pre-5th-Generation (5G) or 5Gcommunication system to be provided for supporting higher data rates beyond 4th-Generation (4G) communication system such as Long Term Evolution (LTE). The present disclosure provides a differential beamforming based random access method, base station, and user equipment, wherein the differential beamforming based random access method comprises, by a base station: receiving a preamble sequence from a first terminal in a differential beamforming receiving mode; determining a base station beam direction angular deviation based on the preamble sequence; and adjusting a base station beam according to the base station beam direction angular deviation, and transmitting a random access response signal to the first terminal through the adjusted base station beam. In the present disclosure, by detecting a base station beam direction angular deviation in a differential beamforming receiving mode, a base station receiving beam can be adjusted to an optimal beam faster than a beam polling way of the prior art, thereby improving the performance of a random access procedure.申请人:Samsung Electronics Co., Ltd地址:Gyeonggi-do KR国籍:KR更多信息请下载全文后查看。
基于核稀疏保持投影的SAR目标特征提取方法研究
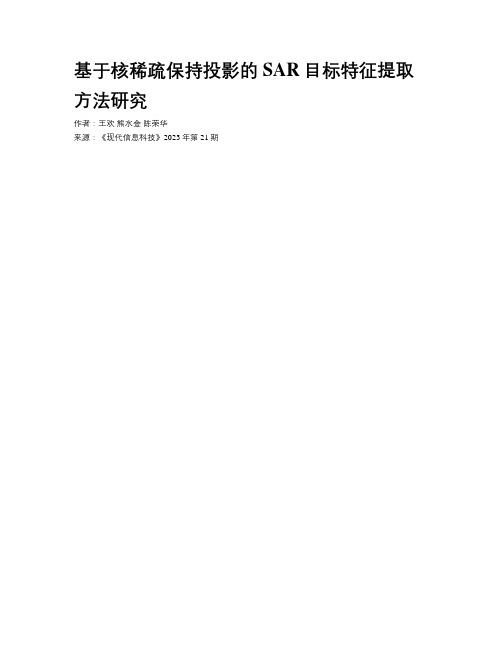
基于核稀疏保持投影的SAR目标特征提取方法研究作者:王欢熊水金陈荣华来源:《现代信息科技》2023年第21期收稿日期:2023-04-12基金项目:江西省教育厅科学技术研究项目(GJJ2204914)DOI:10.19850/ki.2096-4706.2023.21.005摘要:文章提出一种新的特征提取方法,将核稀疏保持投影(KSPP)方法运用到合成孔径雷达(SAR)目标识别中。
该方法将原始目标函数投影到高维特征空间,在高维特征空间求得样本的稀疏系数,将所有样本的稀疏系数组成稀疏重构矩阵,利用稀疏重构矩阵构造目标函数求得样本的特征向量,最后利用SVM分类器对目标进行分类识别。
基于MSTAR提供的实测SAR数据对方法进行验证,结果表明该方法能够有效地提高目标识别结果,且对目标的方位角不敏感,是一种有效的SAR目标特征提取方法。
关键词:核稀疏保持投影;特征提取;SAR;SVM分类器;MSTAR中图分类号:TN957.52 文献标识码:A 文章编号:2096-4706(2023)21-0020-05Research on SAR Target Feature Extraction Method Based On Kernel Sparsity Preserving ProjectionsWANG Huan, XIONG Shuijin, CHEN Ronghua(Jiangxi Vocational College of Finance and Economics, Jiujiang 332000, China)Abstract: This paper proposes a new feature extraction method, which applies Kernal Sparsity Preserving Projections (KSPP) to Sythentic Aperture Radar (SAR) target recognition. In this method, the original objective function is projected into the high-dimensional feature space, and the sparse coefficients of the samples are obtained in the high-dimensional feature space. The sparse coefficients of all samples are formed into the sparse reconstruction matrix, and the sparse reconstruction matrix is used to construct the objective function to obtain the feature vector of the samples. Finally, the SVM classifier is used to classify and recognize the targets. Based on the measured SAR data provided by MSTAR, the proposed method is verified. The results show that this method can effectively improve the target recognition results and is insensitive to the target azimuth angle, so it is an effective method for SAR target feature extraction.Keywords: KSPP; feature extraction; SAR; SVM classifier; MSTAR0 引言合成孔徑雷达(Sythentic Aperture Radar, SAR)自动目标识别(Automatic Target Recognition, ATR)技术在现代战场中有很重要的作用,利用雷达技术可以实现对目标有效的识别。
基于分割的自然场景下文本检测方法与应用

0引言视觉图像是人们获取外界信息的主要来源,文本则是对事物的一种凝练描述,人通过眼睛捕获文本获取信息,机器设备的眼睛则是冰冷的摄像头。
如何让机器设备从拍照获取的图像中准确检测识别文本信息逐渐为各界学者关注。
现代文本检测方法多为基于深度学习的方法,主要分为基于候选框和基于像素分割的两种形式。
本文选择基于像素分割的深度学习模型作为文本检测识别的主要研究方向,能够同时满足对自然场景文本的精确检测,又能保证后续设备功能(如语义分析等功能)的拓展。
1基于像素分割的文本检测方法1.1PixelLink 算法原理PixelLink [1]算法训练FCN [2]预测两种分类:文本与非文本像素、像素间连接关系。
数据集中的文本框内像素整体作为文本像素,其他为非文本像素。
与九宫格类似,每个像素的周围有8个相邻的像素,对应有8种连接关系。
文本与非文本像素之间的值为负,文本与文本像素之间的值为正,非文本像素之间的值为零。
将值为正的像素与相邻8个像素之间的连接关系连通成一片分区,∗基金项目:国家自然科学基金(61601202);江苏省自然科学基金(BK20140571);江苏大学高级专业人才科研启动基金(14JDG038)基于分割的自然场景下文本检测方法与应用∗陈小顺,王良君(江苏大学计算机科学与通信工程学院,江苏镇江212013)摘要:自然场景文本检测识别在智能设备中应用广泛,而对文本识别的第一步则是对文本进行精确的定位检测。
对于现有像素分割方法PixelLink 中存在的弯曲文本定位包含过多背景信息、检测图像后处理不足两个主要问题提出改进。
引入特征通道注意力机制,关注生成特征图中特征通道间的权重关系,提升检测方法的鲁棒性。
接着改变公开数据集标注形式,将坐标点表示为一串带有方向的序列形式,在LSTM 模型中进行多边形框的学习与框定。
最后在公开数据集和自建数据集上进行文本检测测试。
实验表明,改进的检测方法在各数据集中表现优于原方法,与当前领先方法精度相近,能够在各个环境中完成对文本的检测功能。
基于交叉累计剩余熵的多光谱图像配准方法

基于交叉累计剩余熵的多光谱图像配准方法李超,陈钱,钱惟贤(南京理工大学江苏省光谱成像与智能感知重点实验室,江苏南京210094)摘要:针对传统互信息图像配准容易产生局部极值的问题,提出将双边滤波器和交叉累计剩余熵结合作为匹配算法,进行多光谱图像的配准。
在这种配准算法中,首先针对多光谱图像特点,提出基于概率密度的双边滤波器边缘提取方法,其次采用交叉累计剩余熵代替互信息作为测度函数将参考图像与待匹配图像的边缘进行匹配。
双边滤波器的特性是去噪保边,而累计剩余熵比香农熵更具一般性,且该函数可以有效地避免局部极值,去除噪声。
实验证明,该方法鲁棒性好,配准效果明显。
关键词:多光谱配准;双边滤波器;概率密度函数;交叉累计剩余熵中图分类号:TP751文献标志码:A文章编号:1007-2276(2013)07-1866-05Registration algorithm of multispectral images based on crosscumulative residual entropyLi Chao,Chen Qian,Qian Weixian(Jiangsu Key Laboratory of Spectral Imaging &Intelligent Sense,Nanjing University of Scienceand Technology,Nanjing 210094,China)Abstract:In order to solve the problem that classical mutual information images registration may lead to local extremum,a new matching algorithm combining the bilateral filter and cross accumulated residual entropy combination was proposed in multispectral image registration.In this algorithm,firstly,according to multispectral images characteristics,bilateral filter edge extraction algorithm was put forward based on the probability density.Secondly,cross cumulative residual entropy (CCRE)was used as the similarity measure to match the reference images and transformed images effectively.Bilateral filter is an edge 鄄preserving and noise reducing smoothing filter,and CCRE is more general than Shannon Entropy.This function can effectively avoid the emergence of the local extremum,overcome noise influence on the the local extremum.Experimental results proved that the registration had good robustness,the effect was obvious.Key words:multispectral registration;bilateral filter;probability density function;cross cumulative residual entropy收稿日期:2012-11-02;修订日期:2012-12-08基金项目:国防装备预先研究项目;“光谱成像技术与信息处理”教育部长江学者创新团队(IRT0733);光谱成像与智能感知江苏省重点实验室,南京理工大学自主科研(2011XQTR01;2011YBXM74)作者简介:李超(1984-),男,博士生,主要从事光电探测与图像处理方面的研究。
可见光海面目标检测的结构随机森林方法

可见光海面目标检测的结构随机森林方法雷琴;施朝健;陈婷婷【摘要】For the influence of some complex sea states such as coastal scenery and surface ripple in sea images, target detection based on the visible light image is a technical difficult problem of the current. This paper presents a method of structured random forests for target detection in sea images. The method first constructs random decision forest based on image block, applies structured learning strategy to the forecast output spatial of the constructed random decision forest, and then trains the random decision forest in the sample space, and finally classifies the testing image blocks as the target region and the background region through random decision forest. The experimental results show that compared with the Canny operator, the Threshold-Segment operator,and the Salience_ROI operator, the method of this paper has significant advantages in the aspects of sea image target detection and uses low computation cost.%由于受到海岸景物和海面波纹等复杂海况的影响,基于可见光海面图像目标检测是一个技术难点。
基于平滑阈值函数的小波图像去噪
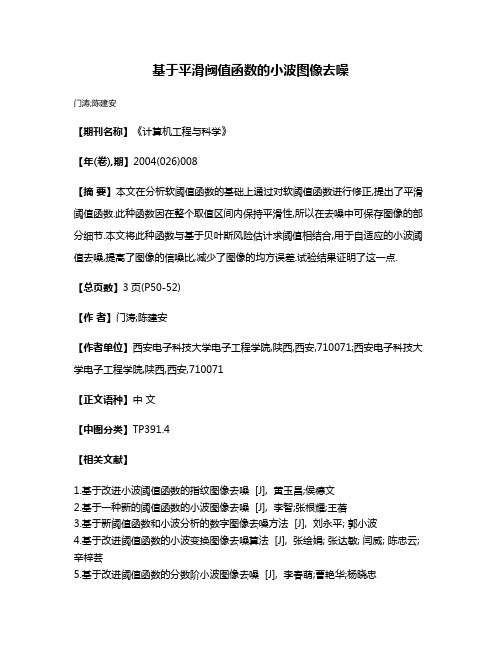
基于平滑阈值函数的小波图像去噪
门涛;陈建安
【期刊名称】《计算机工程与科学》
【年(卷),期】2004(026)008
【摘要】本文在分析软阈值函数的基础上通过对软阈值函数进行修正,提出了平滑阈值函数.此种函数因在整个取值区间内保持平滑性,所以在去噪中可保存图像的部分细节.本文将此种函数与基于贝叶斯风险估计求阈值相结合,用于自适应的小波阈值去噪,提高了图像的信噪比,减少了图像的均方误差.试验结果证明了这一点.【总页数】3页(P50-52)
【作者】门涛;陈建安
【作者单位】西安电子科技大学电子工程学院,陕西,西安,710071;西安电子科技大学电子工程学院,陕西,西安,710071
【正文语种】中文
【中图分类】TP391.4
【相关文献】
1.基于改进小波阈值函数的指纹图像去噪 [J], 黄玉昌;侯德文
2.基于一种新的阈值函数的小波图像去噪 [J], 李智;张根耀;王蓓
3.基于新阈值函数和小波分析的数字图像去噪方法 [J], 刘永平; 郭小波
4.基于改进阈值函数的小波变换图像去噪算法 [J], 张绘娟; 张达敏; 闫威; 陈忠云; 辛梓芸
5.基于改进阈值函数的分数阶小波图像去噪 [J], 李春萌;曹艳华;杨晓忠
因版权原因,仅展示原文概要,查看原文内容请购买。
基于深度强化学习的多目标跟踪技术研究

基于深度强化学习的多目标跟踪技术研究
杨麒霖;刘俊;管坚;莫倩倩;陈华杰;谷雨;石义芳
【期刊名称】《无线电通信技术》
【年(卷),期】2024(50)1
【摘要】在随机有限集多目标跟踪过程中,由于跟踪问题的复杂性,会耗费大量的计算成本,特别是在目标和杂波密集的复杂情况中,计算成本呈指数增长。
随机有限集中通常采用的分配算法——例如Murty算法的时间复杂度为滤波器生成的代价矩阵规模的三次方。
为了减少跟踪耗时,结合组合优化的思想,将代价矩阵重定义为二分图,采用了一种基于深度强化学习的二分图匹配算法,取代传统随机有限集中的分配算法,并通过仿真实验验证了所提方法的可行性。
实验表明,所提方法在保证跟踪性能的前提下减少了跟踪耗时,提升了跟踪实时性。
【总页数】6页(P187-192)
【作者】杨麒霖;刘俊;管坚;莫倩倩;陈华杰;谷雨;石义芳
【作者单位】杭州电子科技大学通信信息传输与融合技术国防重点学科实验室【正文语种】中文
【中图分类】TN953
【相关文献】
1.基于值分解的多目标多智能体深度强化学习方法
2.基于深度强化学习的造纸废水处理过程多目标优化
3.基于深度强化学习的多目标边缘任务调度研究
4.基于深度强化学习的车辆多目标协同巡航决策控制系统设计
因版权原因,仅展示原文概要,查看原文内容请购买。
- 1、下载文档前请自行甄别文档内容的完整性,平台不提供额外的编辑、内容补充、找答案等附加服务。
- 2、"仅部分预览"的文档,不可在线预览部分如存在完整性等问题,可反馈申请退款(可完整预览的文档不适用该条件!)。
- 3、如文档侵犯您的权益,请联系客服反馈,我们会尽快为您处理(人工客服工作时间:9:00-18:30)。
Accurate and Robust3D Facial Capture Using a Single RGBD CameraYen-Lin Chen Texas A&M UniversityHsiang-Tao WuMicrosoft Research AsiaFuhao ShiTexas A&M UniversityXin Tong Microsoft Research AsiaJinxiang Chai Texas A&M UniversityAbstractThis paper presents an automatic and robust approach that accurately captures high-quality3D facial perfor-mances using a single RGBD camera.The key of our ap-proach is to combine the power of automatic facial feature detection and image-based3D nonrigid registration tech-niques for3D facial reconstruction.In particular,we de-velop a robust and accurate image-based nonrigid regis-tration algorithm that incrementally deforms a3D template mesh model to best match observed depth image data and important facial features detected from single RGBD im-ages.The whole process is fully automatic and robust be-cause it is based on single frame facial registration frame-work.The system isflexible because it does not require any strong3D facial priors such as blendshape models.We demonstrate the power of our approach by capturing a wide range of3D facial expressions using a single RGBD camera and achieve state-of-the-art accuracy by comparing against alternative methods.1.IntroductionThe ability to accurately capture3D facial perfor-mances has many applications including animation,gam-ing,human-computer interaction,security,and telepres-ence.This problem has been partially solved by com-mercially available marker-based motion capture equipment (e.g.,[18]),but this solution is far too expensive for com-mon use.It is also cumbersome,requiring the user to wear more than60carefully positioned retro-reflective markers on the face.This paper presents an alternative to solving this problem:reconstructing the user’s3D facial performances using a single RGBD camera.The main contribution of this paper is a novel3D fa-cial modeling process that accurately reconstructs3D facial expression models from single RGBD images.We focus on single frame facial reconstruction because it ensures the process is fully automatic and does not suffer from drift-ing errors.At the core of our system lies a3D facial de-formation registration process that incrementally deforms a template face model to best match observed depth data.We model3D facial deformation in a reduced subspace through embedded deformation[16]and extend model-based opti-calflow formulation to depth image data.This allows us to formulate the3D nonrigid registration process in the Lucas-Kanade registration framework[1]and use linear system solvers to incrementally deform the template face model to match observed depth images.Our image-based3D nonrigid registration process,like any other iterative registration processess[1],requires a good initialization.The system often produces poor reg-istration results when facial deformations are far from the template face model.In addition,it does not take into ac-count perceptually significant facial features such as nose tip and mouth corners,thereby resulting in misalignments in those perceptually important facial regions.We address the challenges by complementing our image-based nonrigid registration process with automatic facial feature detection process.Our experiment shows that incorporating impor-tant facial features into the nonrigid registration process sig-nificantly improves the accuracy and robustness of the re-construction process.We demonstrate the power of our facial reconstruction system by modeling a wide range of facial expressions using a single Kinect(see Figure1).We evaluate the performance of the system by comparing against alterna-tive methods including marker-based motion capture[18],“faceshift”system[20],Microsoft face SDK[11],and non-rigid facial registration using Iterative Closest Points(ICP).2.BackgroundOur system accurately captures high-quality3D facial performances using a single RGBD camera.Therefore,we will focus our discussion on methods and systems devel-oped for acquiring3D facial performances.One of most successful approaches for3D facial per-formance capture is based on marker-based motion cap-2013 IEEE International Conference on Computer VisionFigure 1.Accurate and robust facial performance capture using a single Kinect :(top)reference image data;(bottom)the reconstructed facial performances.ture [9],which can robustly and accurately track a sparse set of markers attached on the face.Recent effort in this area (e.g.,[2,10])has been focused on complementing marker-based systems with other capturing types of devices such as video cameras and/or 3D scanners to improve the resolution and details of captured facial geometry.Marker-based mo-tion capture,however,is not practical for random users tar-geted by this paper as they are expensive and cumbersome for 3D facial performance capture.Marker-less motion capture provides an appealing al-ternative to facial performance capture because it is non-intrusive and does not impede the subject’s ability to per-form facial expressions.One solution to marker-less fa-cial capture is the use of depth and/or color data obtained from structured light systems [22,13,12,21].For exam-ple,Zhang and his colleagues [22]captured 3D facial ge-ometry and texture over time and built the correspondences across all the facial geometries by deforming a generic face template to fit the acquired depth data using optical flow computed from image sequences.Recently,Li and his col-leagues [12]captured dynamic depth maps with their real-time structured light system and fit a smooth template to the captured depth maps.The minimal requirement of a single camera for facial performance capture is particularly appealing,as it offers the lowest cost and a simplified setup.However,previ-ous single RGB camera systems for facial capture [7,6,14]are often vulnerable to ambiguity caused by a lack of dis-tinctive features on face and uncontrolled lighting environ-ments.One way to address the issue is to use 3D prior models to reduce the ambiguity of image-based facial de-formations (e.g.,[3,19]).More recent research [5,4,20]has been focused on modeling 3D facial deformation usinga single RGBD camera such as Microsoft Kinect or time-of-flight (TOF)cameras.For example,Cai and colleagues [5]explored how to use a linear deformable model constructed by an artist and Iterative Closest Points (ICP)techniques to fit deformable model from depth data.Breidt et al.[4]con-structed 3D identity and expression morphable models from a large corpus of prerecorded 3D facial scans and used them to fit depth data obtained from a ToF camera via similar ICP techniques.Among all the systems,our work is most closely related to Weise et al.[20],which uses RGBD image data captured by a single Kinect and a template,along with a set of prede-fined blend shape models,to track facial deformations over time.Our system shares a similar perspective as theirs be-cause both are targeting low-cost and portable facial capture accessible to random users.Our goal,however,is differ-ent from theirs in that we focus on authentic reconstruc-tion of 3D facial performances rather than performance-based facial retargeting and animation.Our method for fa-cial capture is also significantly different from theirs.Their approach utilizes a set of predefined blend shape models and closest points measurement to sequentially track facial performances in a Maximum A Posteriori (MAP)frame-work.In contrast,our approach focuses on single frame facial reconstruction and combines image-based registra-tion techniques with automatic facial detection in the Lucas-Kanade registration framework.Another difference is that we model deformation using embedded deformation rather than blendshape representation and therefore do not require any predefined blendshape models,which significantly re-duces overhead costs for 3D facial stly,as shown in our comparison experiment,our system achieves much more accurate results than Weise et al.[20].(a)(b)(c)(d)Figure2.Accurate and robust facial performance capture using a single Kinect:(a)the input depth image;(b)the input color image;(c)the detected facial features superimposed on the color image;(d)the reconstructed3D facial model using both the depth image and detected facial features.3.OverviewOur system acquires high-quality3D facial models fromsingle RGBD images recorded by a single Kinect.A Kinectcan simultaneously capture depth maps with a resolution of320×240and color images with a resolution of640×480at30frames per second based on infrared projection.Ourfacial modeling process leverages automatic facial featuredetection and image-based nonrigid registration for3D fa-cial expression modeling(Figure2).We start the process by automatically locating importantfacial features such as the nose tip and the mouth corners insingle RGBD images(Figure2(c)).Briefly,we formulatefeature detection as a per-pixel classification problem andapply randomized trees to associate each pixel with proba-bility scores of being a particular feature.The detected fea-tures are often noisy and frequently corrupted by outliersdue to classification errors.To handle this challenge,weemploy geometric hashing to robustly search closest exam-ples in a training set of labeled images,where all the key fa-cial features are labeled,and remove misclassified featuresinconsistent with the closest example.In thefinal step,werefine feature locations by utilizing active appearance mod-els(AAM)and2D facial priors embedded in K closest ex-amples of the detected features.For more details of ourfeature detection process,please refer to[15].We model3D facial deformation using embedded defor-mation[16]and this allows us to constrain the solution to bein a reduced subspace.We introduce a model-based depthflow algorithm to incrementally deform the face template tobest match observed depth data as well as detected facialfeatures.We formulate the problem in the Lucas-Kanadeimage registration framework and incrementally estimatesboth rigid transformations(ρ)and nonrigid deformation(g)via linear system solvers.In addition,we introduce extraterms into the registration framework to further improve therobustness and accuracy of our system.4.Image-based Nonrigid Facial RegistrationThis section focuses on automatic3D facial modeling us-ing single RGBD images,which is achieved by deforminga template mesh model,s0,to best match observed imagedata.In our implementation,we obtain the template meshmodel s0by scanning facial geometry of the subject undera neutral expression.4.1.Facial Configuration SpaceWe model nonrigid deformation g using embedded de-formation representation developed by Sumner et al.[16].Embedded deformation builds a space deformation repre-sented by a collection of affine transformations organizedin a graph structure.One affine transformation is associatedwith each node and induces a deformation on the nearbyspace.The influence of nearby nodes is blended by the em-bedded deformation algorithm in order to deform the ver-tices or the graph nodes themselves.We choose embeddeddeformation because it allows us to model the deformationin a reduced subspace,thereby significantly reducing theambiguity for3D facial modeling.In embedded deformation,the affine transformation foran individual node is defined by a3-by-3matrix A j anda3-by-1translation vector t j.In this way,the collec-tion of all per-node affine transformations,denoted as g={A j,t j}j=1,...,M,where M is the total number of the nodes,expresses a non-rigid deformation of the template meshmodel in a reduced deformation space.Specifically,the de-formed position˜v i of each shape vertex v i is a weightedsum of its positions after application of the affine transfor-mations associated with the k closest nodes to the vertex:˜v i=kj=1w j(v i)[A j(v i−n j)+n j+t j](1)where{A j,t j}j=1,...,k are the affine transformations asso-ciated with the k closest nodes of the vertex v i and n j is thenode position for the j-th node on the template mesh.Theweights w j(v i),j=1,...,k,are spatially varying and thusdepend on the vertex position.The weights for each vertexare precomputed in a way similar to[16].In our experiment,graph nodes are chosen by uniformly sampling vertices ofthe template mesh model in the frontal facial region.Wehave found M=250graph nodes are often sufficient tomodel facial details captured by a Kinect.We also experi-mentally set k to4.We represent rigid transformations of the face using a6-by-1vectorρ,which stacks the translation and rotationvectors.The state of our facial modeling process can thusbe defined by q=[ρ,g].We denote the deformed meshmodel as s=s0⊕q,where the operator⊕represents the application of both rigid transformations and embedded de-formations to the template mesh s0.Let¯p=[¯x,¯y,¯z]be the barycentric coordinates of apoint on the surface of the template mesh s0.The globalcoordinates of the corresponding point on the surface ofthe deforming mesh s are defined by a forward kinematicsfunction h for mesh deformation:p=h(q;¯p,s0),which computes the global position(p)of a surface point from the model state(q)given the local coordinates(p0)of a sur-face point on the template mesh(s0).We model the rela-tionship between the global coordinates of a surface point and its corresponding pixel on image plane using a pro-jection transformation function obtained from Kinect SDK: x=f(p),where x=[u,v]represents the2D coordinates of the corresponding pixel in depth image.4.2.Objective FunctionWe adopt an“analysis-by-synthesis”strategy to mea-sure how well the transformed and deformed face templatemodelfits observed RGBD image data.Our image-basednonrigid facial registration process aims to minimize thefollowing objective function:minqE data+α1E rot+α2E reg(2) where thefirst term is the datafitting term,which measures how well the deformed template model matches the ob-served RGBD data.The second term E rot ensures that lo-cal graph nodes deform as rigidly as possible(i.e.,A T j A j= I3×3).The third term E reg serves as a regularizer for the deformation by indicating that the affine transformations of adjacent graph nodes should agree with one another.The weightsα1andα2control the importance of the second and third terms.In our experiment,we set the weightsα1 andα2to1.0and0.5,respectively.Here we focus our dis-cussion on thefirst term.For details about the second and third term,please refer to the original work of embedded deformation[16].We define the datafitting term as a weighted combina-tion of three terms:αdepth E depth+αfeature E feature+αboundary E boundary(3)where thefirst term E depth is the depth image term which minimizes the difference between the“observed”and the “hypothesized”depth data.The second term E feature is the facial feature term which ensures the“hypothesized”facial features are consistent with the“detected”facial fea-tures in observed data.The third term is the boundary term which stabilizes the registration process by penalizing the misalignments of boundary points between the“hypothe-sized”and“observed”face models.In our experiment,we set the weightsαfeature,αdepth,andαboundary to0.001, 0.1and5,respectively.This requires minimizing a sum of squared nonlinear function values.Our idea is to extend the Lucas-Kanade algorithm[1]to solve the above non-linear least squares problem.Lucas-Kanade algorithm,which is a Gauss-Newton gradient descent non-linear optimization algorithm, assumes that a current estimate of q is known and then it-eratively solves for increments to the parametersδq using linear system solvers.4.2.1Depth Image TermThis section introduces a novel model-based depthflow al-gorithm for incrementally estimating rigid transformation (ρ)and nonrigid transformation(g)of the template mesh (s0)to best match the observed depth image D.Assume the movement(δp)between the two frames to be small,the depth image constraint at D(x,t)is defined as follows: D(x(p),t)+δz=D(x(p+δp),t+1).(4) Intuitively,when a3D surface point(p)has a delta move-ment(δp)in3D space,its projected pixel x(p)on image plane will have the corresponding movementδx=(δu,δv). However,unlike color image registration via opticalflow, the depth value of a pixel is not constant.Instead,it will produce a corresponding small changeδz along the depth axis.This is due to reparameterization of3D point p on2D image space.Similar to the opticalflow formulation,we derive the depthflow formulation by approximating the right side of Equation(4)with a Taylor series expansion.We have(∂D∂x∂x∂p−∂z∂p)δp+∂D∂t=0,(5)where partial derivatives∂D/∂x are gradients of the depth image at pixel x.The derivatives∂x/∂p can be evaluated by the projection function x=f(p).The temporal derivative ∂D/∂t simply measures the pixel difference of two consec-utive depth images.The partial derivatives∂z∂pis simply a row vector[0,0,1].We adopt an“analysis-by-synthesis”strategy to incre-mentally register the deforming template mesh with the ob-served depth image via depthflow.More specifically,werender a depth image based on the current model state(q) and then estimate an optimal update of the model state(δq) by minimizing the inconsistency between the“observed”and“rendered”depth images.To register the deforming template face with the observed depth image via depthflow, we associate the delta movement of a point(δp)with the delta change of the model state(δq):δp=∂h(q;p0,s0)∂qδq,(6)where the vector-valued function h is the forward kinemat-ics function for mesh deformation[17].After combing Equation(5)with Equation(6)using chain rules,we have(∂D∂x∂x∂p−∂z∂p)∂h∂qδq+∂D∂t=0.(7)The above equation shows how to optimally update the model state(δq)based on spatial and temporal derivatives of the“rendered”depth image D(u,v).In the model-based depthflow formulation,we evaluate the spatial derivatives based on the gradients of the“rendered”depth image.Thetemporal derivatives∂D∂t are evaluated by the difference be-tween the“observed”and“rendered”depth images.An op-timal update of the model state can be achieved by summing over the contributions of individual depth pixels associated with the template face.A remaining issue for the depth image term evaluation is to determine which pixels in the“rendered”depth image should be included for evaluation.We stabilize the model-based depthflow estimation process by excluding the pixels outside the border of outer boundary of the face.Corre-sponding vertices on the template mesh are automatically marked by back projecting the border pixels of the rendered depth image.Similarly,we remove the pixels that are in-side the border of inner boundary of the face,in particular the mouth and eyes.The inner boundary of the face is de-fined by the close regions of the detected facial features of the mouth and eyes.4.2.2Facial Feature TermDepth data alone is often not sufficient to model accurate facial deformation because it does not take into account perceptually significant facial features such as the nose tip and the mouth corners,thereby resulting in misalignments in those perceptually important facial regions.We address the challenge by including the facial feature term into the objective function.In our implementation,we choose to define the facial feature term based on a combination of2D and3D facial points obtained from detection process.In preprocessing step,we annotate the locations of fa-cial features on the template mesh model by identifying the barycentric coordinates(¯p i)of facial features on the tem-plate mesh.The facial feature term minimizes the inconsis-tency between the“hypothesized”and“observed”features in either2D or3D space:iωi f(h(q;¯p i,s0))−x i 2+(1−ωi) h(q;¯p i,s0)−p i 2where the vector x i and p i are2D and3D coordinates of the i-th detected facial features.The weightωi is a binary value,which returns“1”if depth information is missing, otherwise“0”.Note that only facial features around impor-tant regions,including the mouth and nose,eyes,and eye-brows,are included for facial feature term evaluation.This is because facial features located on outer contour are often not very stable.4.2.3Boundary TermDepth data from a Kinect is often very noisy and fre-quently contains missing data along the face boundary.This inevitably results in noisy geometry reconstruction around the face boundary.We introduce the boundary term to sta-bilize the registration along the boundary.To handle noisy depth data around the outer boundary of the face,wefirst estimate the rigid-body transformationρthat aligns the template mesh with observed data(see Sec-tion4.3).During nonrigid registration process,we stabilize the outer boundary of the deforming face by penalizing the deviation from the transformed template s0⊕ρ.Vertices on the outer boundary of the template/deforming mesh are au-tomatically marked by back projecting outer boundary pix-els of the“rendered”depth image.We define the boundary term in3D position space by minimizing the sum of the squares of the distances between the boundary vertices of the deforming mesh(s0⊕ρ⊕g)and their target3D posi-tions obtained from the transformed mesh(s0⊕ρ).4.3.Registration OptimizationOur3D facial modeling requires minimizing a sum of squared nonlinear function values defined in Equation(2). Our idea is to extend the Lucas-Kanade framework[1]to solve the non-linear least squares problem.Lucas-Kanade algorithm assumes that a current estimate of q is known and then iteratively solves for increments to the parametersδq using linear system solvers.In our implementation,we start with the template mesh and iteratively transform and de-form the template mesh until the change of the state q is smaller than a specified threshold.We have observed that a direct estimation of rigid trans-formations and embedded deformation is prone to local minima and often produces poor results.We thus decouple rigid transformations from nonrigid deformation and solvethem in two sequential steps.In thefirst step,we drop off the boundary term from the objective function defined in Equation(3)and estimate the rigid transformationρusing iterative linear solvers.We stabilize the rigid alignment by using a pre-segmented template that excludes the chin re-gion from the registration as this part of the face typically exhibits the strongest nonrigid deformations.In the second step,we keep the computed rigid transformation constant and iteratively estimate embedded deformation g based on the objective function defined in Equation(2).This requires minimizing a sum of squared nonlinear function values.Our idea is to extend the Lucas-Kanade al-gorithm[1]to solve the above non-linear least squares prob-lem using iterative linear system solvers.In our implemen-tation,we analytically evaluate the Jacobian terms of the objective function.The fact that each step in the registration algorithm can be executed in parallel allows implementinga fast solver on modern graphics hardware.5.Algorithm EvaluationOur evaluation consists of two parts.Thefirst part com-pares our approach with alternative methods.The second part validates the proposed approach by evaluating the im-portance of each key component of our process.We have evaluated the effectiveness of our algorithm based on both synthetic and real data.Our results are best seen in the accompanying video.We also show sample frames of the evaluation results in our supplementary PDFfile.Evaluation on synthetic data.We evaluate our system on synthetic RGBD image data generated by high-fidelity 3D facial data captured by Huang and colleagues[10].The whole testing sequence consists of1388frames.Wefirst synthesize a sequence of color and depth images based on a RGBD camera(i.e.,Kinect)setting similar to what occurs in the real world.The resolutions of image and depth data, therefore,are set to640×480and320×240,respectively, with24-bit RGB color values and13-bit integer depth val-ues in millimeters.The face models are placed at a distance to approximate real world capturing scenarios.We test our algorithm as well as alternative methods on synthetic RGBD images and obtain the quantitative er-ror of the algorithm by comparing its reconstruction data against ground truth data.In particular,we compute the av-erage correspondence/reconstruction error between our re-constructed models and the ground truth data across the en-tire sequence.We evaluate the reconstruction error by mea-suring the sum of distances between vertex position on the reconstruction mesh and its corresponding position on the ground truth mesh.Note that we know the correspondences between the two meshes because the reconstruction meshes are deformed from thefirst mesh of ground truth data.Evaluation on real data.We further evaluate the per-formance of our system on real data by comparing againstOur Method Nonrigid ICPx(2D image plane)0.64997pixel2.5890pixely(2D image plane)0.85933pixel4.0202pixel x(3D space)1.2112mm4.7829mmy(3D space)1.5817mm7.5349mmz(3D space)2.1866mm4.8383mm Table1.Quantitative evaluation of our algorithm and Nonrigid ICP registration using ground truth data scaptured with a full marker set in a twelve-camera Vicon system[18].ground truth facial data acquired by an optical motion cap-ture system[18].We placed62retro-reflective markers(4 mm diameter hemispheres)on the subject’s face and set up a twelve-camera Vicon motion capture system[18]to record dynamic facial movements at240frames per second acqui-sition rate.We synchronize a Kinect camera with the optical motion capture system to record the corresponding RGBD image data.The whole test sequence consists of838frames corresponding a wide range of facial expressions.We test our algorithm as well as alternative methods on recorded RGBD image data.We use the3D trajectories of62mark-ers captured by Vicon as ground truth data to evaluate the performance of the algorithm.The captured marker posi-tions and observed RGBD image data are from different co-ordinate systems and therefore we need to transform them into the same coordinate system.To achieve this goal,we use the video images from Kinect as a reference to manually label marker positions in the Kinect coordinate system and use them to estimate an optimal4×4transformation matrix to transform markers from the motion capture coordinate system to the Kinect coordinate system.parisons Against Alternative MethodsWe have evaluated the performance of our system by comparing against alternative methods.Comparison against Vicon system.In this experiment, we quantitatively assess the quality of the captured motion by comparing with ground truth motion data captured with a full marker set in a twelve-camera Vicon system[18].The average reconstruction error,which is computed as average 3D marker position discrepancy between the reconstructed facial models and the ground truth mocap data,was reported in Table1.The accompanying video also shows a side-by-side comparison between our result and the result obtained by Vicon.The computed quantitative errors provide us an upper bound on the actual errors because of reconstruction errors from the Vicon mocap system and imperfect align-ments of the Vicon markers with the Kinect data.Note that we attached the mocap markers on the subject’s face,which makes the markers deviate from the actual surface of the face.Comparison against nonrigid ICP techniques.We。