song_Smart Aggregate-Based Damage Detection of Circular RC columns
The_electronic_properties_of_graphene

The electronic properties of grapheneA.H.Castro NetoDepartment of Physics,Boston University,590Commonwealth Avenue,Boston,Massachusetts02215,USAF.GuineaInstituto de Ciencia de Materiales de Madrid,CSIC,Cantoblanco,E-28049Madrid,SpainN.M.R.PeresCenter of Physics and Department of Physics,Universidade do Minho,P-4710-057,Braga,PortugalK.S.Novoselov and A.K.GeimDepartment of Physics and Astronomy,University of Manchester,Manchester,M139PL,United Kingdom͑Published14January2009͒This article reviews the basic theoretical aspects of graphene,a one-atom-thick allotrope of carbon, with unusual two-dimensional Dirac-like electronic excitations.The Dirac electrons can be controlled by application of external electric and magneticfields,or by altering sample geometry and/or topology.The Dirac electrons behave in unusual ways in tunneling,confinement,and the integer quantum Hall effect.The electronic properties of graphene stacks are discussed and vary with stacking order and number of layers.Edge͑surface͒states in graphene depend on the edge termination͑zigzag or armchair͒and affect the physical properties of nanoribbons.Different types of disorder modify the Dirac equation leading to unusual spectroscopic and transport properties.The effects of electron-electron and electron-phonon interactions in single layer and multilayer graphene are also presented.DOI:10.1103/RevModPhys.81.109PACS number͑s͒:81.05.Uw,73.20.Ϫr,03.65.Pm,82.45.MpCONTENTSI.Introduction110II.Elementary Electronic Properties of Graphene112A.Single layer:Tight-binding approach1121.Cyclotron mass1132.Density of states114B.Dirac fermions1141.Chiral tunneling and Klein paradox1152.Confinement and Zitterbewegung117C.Bilayer graphene:Tight-binding approach118D.Epitaxial graphene119E.Graphene stacks1201.Electronic structure of bulk graphite121F.Surface states in graphene122G.Surface states in graphene stacks124H.The spectrum of graphene nanoribbons1241.Zigzag nanoribbons1252.Armchair nanoribbons126I.Dirac fermions in a magneticfield126J.The anomalous integer quantum Hall effect128 K.Tight-binding model in a magneticfield128 ndau levels in graphene stacks130 M.Diamagnetism130 N.Spin-orbit coupling131 III.Flexural Phonons,Elasticity,and Crumpling132 IV.Disorder in Graphene134A.Ripples135B.Topological lattice defects136C.Impurity states137D.Localized states near edges,cracks,and voids137E.Self-doping138F.Vector potential and gaugefield disorder1391.Gaugefield induced by curvature1402.Elastic strain1403.Random gaugefields141G.Coupling to magnetic impurities141H.Weak and strong localization142I.Transport near the Dirac point143J.Boltzmann equation description of dc transport indoped graphene144 K.Magnetotransport and universal conductivity1451.The full self-consistent Born approximation͑FSBA͒146 V.Many-Body Effects148A.Electron-phonon interactions148B.Electron-electron interactions1501.Screening in graphene stacks152C.Short-range interactions1521.Bilayer graphene:Exchange1532.Bilayer graphene:Short-range interactions154D.Interactions in high magneticfields154VI.Conclusions154 Acknowledgments155 References155REVIEWS OF MODERN PHYSICS,VOLUME81,JANUARY–MARCH20090034-6861/2009/81͑1͒/109͑54͒©2009The American Physical Society109I.INTRODUCTIONCarbon is the materia prima for life and the basis of all organic chemistry.Because of the flexibility of its bond-ing,carbon-based systems show an unlimited number of different structures with an equally large variety of physical properties.These physical properties are,in great part,the result of the dimensionality of these structures.Among systems with only carbon atoms,graphene—a two-dimensional ͑2D ͒allotrope of carbon—plays an important role since it is the basis for the understanding of the electronic properties in other allotropes.Graphene is made out of carbon atoms ar-ranged on a honeycomb structure made out of hexagons ͑see Fig.1͒,and can be thought of as composed of ben-zene rings stripped out from their hydrogen atoms ͑Pauling,1972͒.Fullerenes ͑Andreoni,2000͒are mol-ecules where carbon atoms are arranged spherically,and hence,from the physical point of view,are zero-dimensional objects with discrete energy states.Fullerenes can be obtained from graphene with the in-troduction of pentagons ͑that create positive curvature defects ͒,and hence,fullerenes can be thought as wrapped-up graphene.Carbon nanotubes ͑Saito et al.,1998;Charlier et al.,2007͒are obtained by rolling graphene along a given direction and reconnecting the carbon bonds.Hence carbon nanotubes have only hexa-gons and can be thought of as one-dimensional ͑1D ͒ob-jects.Graphite,a three dimensional ͑3D ͒allotrope of carbon,became widely known after the invention of the pencil in 1564͑Petroski,1989͒,and its usefulness as an instrument for writing comes from the fact that graphite is made out of stacks of graphene layers that are weakly coupled by van der Waals forces.Hence,when one presses a pencil against a sheet of paper,one is actually producing graphene stacks and,somewhere among them,there could be individual graphene layers.Al-though graphene is the mother for all these different allotropes and has been presumably produced every time someone writes with a pencil,it was only isolated 440years after its invention ͑Novoselov et al.,2004͒.The reason is that,first,no one actually expected graphene to exist in the free state and,second,even with the ben-efit of hindsight,no experimental tools existed to search for one-atom-thick flakes among the pencil debris cov-ering macroscopic areas ͑Geim and MacDonald,2007͒.Graphene was eventually spotted due to the subtle op-tical effect it creates on top of a chosen SiO 2substrate ͑Novoselov et al.,2004͒that allows its observation with an ordinary optical microscope ͑Abergel et al.,2007;Blake et al.,2007;Casiraghi et al.,2007͒.Hence,graphene is relatively straightforward to make,but not so easy to find.The structural flexibility of graphene is reflected in its electronic properties.The sp 2hybridization between one s orbital and two p orbitals leads to a trigonal planar structure with a formation of a bond between carbon atoms that are separated by 1.42Å.The band is re-sponsible for the robustness of the lattice structure in all allotropes.Due to the Pauli principle,these bands have a filled shell and,hence,form a deep valence band.The unaffected p orbital,which is perpendicular to the pla-nar structure,can bind covalently with neighboring car-bon atoms,leading to the formation of a band.Since each p orbital has one extra electron,the band is half filled.Half-filled bands in transition elements have played an important role in the physics of strongly correlated systems since,due to their strong tight-binding charac-ter,the Coulomb energies are large,leading to strong collective effects,magnetism,and insulating behavior due to correlation gaps or Mottness ͑Phillips,2006͒.In fact,Linus Pauling proposed in the 1950s that,on the basis of the electronic properties of benzene,graphene should be a resonant valence bond ͑RVB ͒structure ͑Pauling,1972͒.RVB states have become popular in the literature of transition-metal oxides,and particularly in studies of cuprate-oxide superconductors ͑Maple,1998͒.This point of view should be contrasted with contempo-raneous band-structure studies of graphene ͑Wallace,1947͒that found it to be a semimetal with unusual lin-early dispersing electronic excitations called Dirac elec-trons.While most current experimental data in graphene support the band structure point of view,the role of electron-electron interactions in graphene is a subject of intense research.It was P .R.Wallace in 1946who first wrote on the band structure of graphene and showed the unusual semimetallic behavior in this material ͑Wallace,1947͒.At that time,the thought of a purely 2D structure was not reality and Wallace’s studies of graphene served him as a starting point to study graphite,an important mate-rial for nuclear reactors in the post–World War II era.During the following years,the study of graphite culmi-nated with the Slonczewski-Weiss-McClure ͑SWM ͒band structure of graphite,which provided a description of the electronic properties in this material ͑McClure,1957;Slonczewski and Weiss,1958͒and was successful in de-scribing the experimental data ͑Boyle and Nozières 1958;McClure,1958;Spry and Scherer,1960;Soule et al.,1964;Williamson et al.,1965;Dillon et al.,1977͒.From 1957to 1968,the assignment of the electron and hole states within the SWM model were oppositetoFIG.1.͑Color online ͒Graphene ͑top left ͒is a honeycomb lattice of carbon atoms.Graphite ͑top right ͒can be viewed as a stack of graphene layers.Carbon nanotubes are rolled-up cylinders of graphene ͑bottom left ͒.Fullerenes ͑C 60͒are mol-ecules consisting of wrapped graphene by the introduction of pentagons on the hexagonal lattice.From Castro Neto et al.,2006a .110Castro Neto et al.:The electronic properties of grapheneRev.Mod.Phys.,V ol.81,No.1,January–March 2009what is accepted today.In1968,Schroeder et al.͑Schroeder et al.,1968͒established the currently ac-cepted location of electron and hole pockets͑McClure, 1971͒.The SWM model has been revisited in recent years because of its inability to describe the van der Waals–like interactions between graphene planes,a problem that requires the understanding of many-body effects that go beyond the band-structure description ͑Rydberg et al.,2003͒.These issues,however,do not arise in the context of a single graphene crystal but they show up when graphene layers are stacked on top of each other,as in the case,for instance,of the bilayer graphene.Stacking can change the electronic properties considerably and the layering structure can be used in order to control the electronic properties.One of the most interesting aspects of the graphene problem is that its low-energy excitations are massless, chiral,Dirac fermions.In neutral graphene,the chemical potential crosses exactly the Dirac point.This particular dispersion,that is only valid at low energies,mimics the physics of quantum electrodynamics͑QED͒for massless fermions except for the fact that in graphene the Dirac fermions move with a speed v F,which is300times smaller than the speed of light c.Hence,many of the unusual properties of QED can show up in graphene but at much smaller speeds͑Castro Neto et al.,2006a; Katsnelson et al.,2006;Katsnelson and Novoselov, 2007͒.Dirac fermions behave in unusual ways when compared to ordinary electrons if subjected to magnetic fields,leading to new physical phenomena͑Gusynin and Sharapov,2005;Peres,Guinea,and Castro Neto,2006a͒such as the anomalous integer quantum Hall effect ͑IQHE͒measured experimentally͑Novoselov,Geim, Morozov,et al.,2005a;Zhang et al.,2005͒.Besides being qualitatively different from the IQHE observed in Si and GaAlAs͑heterostructures͒devices͑Stone,1992͒, the IQHE in graphene can be observed at room tem-perature because of the large cyclotron energies for “relativistic”electrons͑Novoselov et al.,2007͒.In fact, the anomalous IQHE is the trademark of Dirac fermion behavior.Another interesting feature of Dirac fermions is their insensitivity to external electrostatic potentials due to the so-called Klein paradox,that is,the fact that Dirac fermions can be transmitted with probability1through a classically forbidden region͑Calogeracos and Dombey, 1999;Itzykson and Zuber,2006͒.In fact,Dirac fermions behave in an unusual way in the presence of confining potentials,leading to the phenomenon of Zitter-bewegung,or jittery motion of the wave function͑Itzyk-son and Zuber,2006͒.In graphene,these electrostatic potentials can be easily generated by disorder.Since dis-order is unavoidable in any material,there has been a great deal of interest in trying to understand how disor-der affects the physics of electrons in graphene and its transport properties.In fact,under certain conditions, Dirac fermions are immune to localization effects ob-served in ordinary electrons͑Lee and Ramakrishnan, 1985͒and it has been established experimentally that electrons can propagate without scattering over large distances of the order of micrometers in graphene͑No-voselov et al.,2004͒.The sources of disorder in graphene are many and can vary from ordinary effects commonly found in semiconductors,such as ionized impurities in the Si substrate,to adatoms and various molecules ad-sorbed in the graphene surface,to more unusual defects such as ripples associated with the soft structure of graphene͑Meyer,Geim,Katsnelson,Novoselov,Booth, et al.,2007a͒.In fact,graphene is unique in the sense that it shares properties of soft membranes͑Nelson et al.,2004͒and at the same time it behaves in a metallic way,so that the Dirac fermions propagate on a locally curved space.Here analogies with problems of quantum gravity become apparent͑Fauser et al.,2007͒.The soft-ness of graphene is related with the fact that it has out-of-plane vibrational modes͑phonons͒that cannot be found in3D solids.Theseflexural modes,responsible for the bending properties of graphene,also account for the lack of long range structural order in soft mem-branes leading to the phenomenon of crumpling͑Nelson et al.,2004͒.Nevertheless,the presence of a substrate or scaffolds that hold graphene in place can stabilize a cer-tain degree of order in graphene but leaves behind the so-called ripples͑which can be viewed as frozenflexural modes͒.It was realized early on that graphene should also present unusual mesoscopic effects͑Peres,Castro Neto, and Guinea,2006a;Katsnelson,2007a͒.These effects have their origin in the boundary conditions required for the wave functions in mesoscopic samples with various types of edges graphene can have͑Nakada et al.,1996; Wakabayashi et al.,1999;Peres,Guinea,and Castro Neto,2006a;Akhmerov and Beenakker,2008͒.The most studied edges,zigzag and armchair,have drastically different electronic properties.Zigzag edges can sustain edge͑surface͒states and resonances that are not present in the armchair case.Moreover,when coupled to con-ducting leads,the boundary conditions for a graphene ribbon strongly affect its conductance,and the chiral Dirac nature of fermions in graphene can be used for applications where one can control the valleyflavor of the electrons besides its charge,the so-called valleytron-ics͑Rycerz et al.,2007͒.Furthermore,when supercon-ducting contacts are attached to graphene,they lead to the development of supercurrentflow and Andreev pro-cesses characteristic of the superconducting proximity effect͑Heersche et al.,2007͒.The fact that Cooper pairs can propagate so well in graphene attests to the robust electronic coherence in this material.In fact,quantum interference phenomena such as weak localization,uni-versal conductancefluctuations͑Morozov et al.,2006͒, and the Aharonov-Bohm effect in graphene rings have already been observed experimentally͑Recher et al., 2007;Russo,2007͒.The ballistic electronic propagation in graphene can be used forfield-effect devices such as p-n͑Cheianov and Fal’ko,2006;Cheianov,Fal’ko,and Altshuler,2007;Huard et al.,2007;Lemme et al.,2007; Tworzydlo et al.,2007;Williams et al.,2007;Fogler, Glazman,Novikov,et al.,2008;Zhang and Fogler,2008͒and p-n-p͑Ossipov et al.,2007͒junctions,and as“neu-111Castro Neto et al.:The electronic properties of graphene Rev.Mod.Phys.,V ol.81,No.1,January–March2009trino”billiards ͑Berry and Modragon,1987;Miao et al.,2007͒.It has also been suggested that Coulomb interac-tions are considerably enhanced in smaller geometries,such as graphene quantum dots ͑Milton Pereira et al.,2007͒,leading to unusual Coulomb blockade effects ͑Geim and Novoselov,2007͒and perhaps to magnetic phenomena such as the Kondo effect.The transport properties of graphene allow for their use in a plethora of applications ranging from single molecule detection ͑Schedin et al.,2007;Wehling et al.,2008͒to spin injec-tion ͑Cho et al.,2007;Hill et al.,2007;Ohishi et al.,2007;Tombros et al.,2007͒.Because of its unusual structural and electronic flex-ibility,graphene can be tailored chemically and/or struc-turally in many different ways:deposition of metal at-oms ͑Calandra and Mauri,2007;Uchoa et al.,2008͒or molecules ͑Schedin et al.,2007;Leenaerts et al.,2008;Wehling et al.,2008͒on top;intercalation ͓as done in graphite intercalated compounds ͑Dresselhaus et al.,1983;Tanuma and Kamimura,1985;Dresselhaus and Dresselhaus,2002͔͒;incorporation of nitrogen and/or boron in its structure ͑Martins et al.,2007;Peres,Klironomos,Tsai,et al.,2007͓͒in analogy with what has been done in nanotubes ͑Stephan et al.,1994͔͒;and using different substrates that modify the electronic structure ͑Calizo et al.,2007;Giovannetti et al.,2007;Varchon et al.,2007;Zhou et al.,2007;Das et al.,2008;Faugeras et al.,2008͒.The control of graphene properties can be extended in new directions allowing for the creation of graphene-based systems with magnetic and supercon-ducting properties ͑Uchoa and Castro Neto,2007͒that are unique in their 2D properties.Although the graphene field is still in its infancy,the scientific and technological possibilities of this new material seem to be unlimited.The understanding and control of this ma-terial’s properties can open doors for a new frontier in electronics.As the current status of the experiment and potential applications have recently been reviewed ͑Geim and Novoselov,2007͒,in this paper we concen-trate on the theory and more technical aspects of elec-tronic properties with this exciting new material.II.ELEMENTARY ELECTRONIC PROPERTIES OF GRAPHENEA.Single layer:Tight-binding approachGraphene is made out of carbon atoms arranged in hexagonal structure,as shown in Fig.2.The structure can be seen as a triangular lattice with a basis of two atoms per unit cell.The lattice vectors can be written asa 1=a 2͑3,ͱ3͒,a 2=a2͑3,−ͱ3͒,͑1͒where a Ϸ1.42Åis the carbon-carbon distance.Thereciprocal-lattice vectors are given byb 1=23a͑1,ͱ3͒,b 2=23a͑1,−ͱ3͒.͑2͒Of particular importance for the physics of graphene are the two points K and K Јat the corners of the graphene Brillouin zone ͑BZ ͒.These are named Dirac points for reasons that will become clear later.Their positions in momentum space are given byK =ͩ23a ,23ͱ3aͪ,K Ј=ͩ23a ,−23ͱ3aͪ.͑3͒The three nearest-neighbor vectors in real space are given by␦1=a 2͑1,ͱ3͒␦2=a 2͑1,−ͱ3͒␦3=−a ͑1,0͒͑4͒while the six second-nearest neighbors are located at ␦1Ј=±a 1,␦2Ј=±a 2,␦3Ј=±͑a 2−a 1͒.The tight-binding Hamiltonian for electrons in graphene considering that electrons can hop to both nearest-and next-nearest-neighbor atoms has the form ͑we use units such that ប=1͒H =−t͚͗i ,j ͘,͑a ,i †b ,j +H.c.͒−t Ј͚͗͗i ,j ͘͘,͑a ,i †a ,j +b ,i †b ,j +H.c.͒,͑5͒where a i ,͑a i ,†͒annihilates ͑creates ͒an electron with spin ͑=↑,↓͒on site R i on sublattice A ͑an equiva-lent definition is used for sublattice B ͒,t ͑Ϸ2.8eV ͒is the nearest-neighbor hopping energy ͑hopping between dif-ferent sublattices ͒,and t Јis the next nearest-neighbor hopping energy 1͑hopping in the same sublattice ͒.The energy bands derived from this Hamiltonian have the form ͑Wallace,1947͒E ±͑k ͒=±t ͱ3+f ͑k ͒−t Јf ͑k ͒,1The value of t Јis not well known but ab initio calculations ͑Reich et al.,2002͒find 0.02t Շt ЈՇ0.2t depending on the tight-binding parametrization.These calculations also include the effect of a third-nearest-neighbors hopping,which has a value of around 0.07eV.A tight-binding fit to cyclotron resonance experiments ͑Deacon et al.,2007͒finds t ЈϷ0.1eV.FIG.2.͑Color online ͒Honeycomb lattice and its Brillouin zone.Left:lattice structure of graphene,made out of two in-terpenetrating triangular lattices ͑a 1and a 2are the lattice unit vectors,and ␦i ,i =1,2,3are the nearest-neighbor vectors ͒.Right:corresponding Brillouin zone.The Dirac cones are lo-cated at the K and K Јpoints.112Castro Neto et al.:The electronic properties of grapheneRev.Mod.Phys.,V ol.81,No.1,January–March 2009f ͑k ͒=2cos ͑ͱ3k y a ͒+4cosͩͱ32k y a ͪcosͩ32k x a ͪ,͑6͒where the plus sign applies to the upper ͑*͒and the minus sign the lower ͑͒band.It is clear from Eq.͑6͒that the spectrum is symmetric around zero energy if t Ј=0.For finite values of t Ј,the electron-hole symmetry is broken and the and *bands become asymmetric.In Fig.3,we show the full band structure of graphene with both t and t Ј.In the same figure,we also show a zoom in of the band structure close to one of the Dirac points ͑at the K or K Јpoint in the BZ ͒.This dispersion can be obtained by expanding the full band structure,Eq.͑6͒,close to the K ͑or K Ј͒vector,Eq.͑3͒,as k =K +q ,with ͉q ͉Ӷ͉K ͉͑Wallace,1947͒,E ±͑q ͒Ϸ±vF ͉q ͉+O ͓͑q /K ͒2͔,͑7͒where q is the momentum measured relatively to the Dirac points and v F is the Fermi velocity,given by v F =3ta /2,with a value v F Ӎ1ϫ106m/s.This result was first obtained by Wallace ͑1947͒.The most striking difference between this result and the usual case,⑀͑q ͒=q 2/͑2m ͒,where m is the electron mass,is that the Fermi velocity in Eq.͑7͒does not de-pend on the energy or momentum:in the usual case we have v =k /m =ͱ2E /m and hence the velocity changes substantially with energy.The expansion of the spectrum around the Dirac point including t Јup to second order in q /K is given byE ±͑q ͒Ӎ3t Ј±vF ͉q ͉−ͩ9t Јa 24±3ta 28sin ͑3q ͉͒ͪq ͉2,͑8͒whereq =arctanͩq x q yͪ͑9͒is the angle in momentum space.Hence,the presence of t Јshifts in energy the position of the Dirac point and breaks electron-hole symmetry.Note that up to order ͑q /K ͒2the dispersion depends on the direction in mo-mentum space and has a threefold symmetry.This is the so-called trigonal warping of the electronic spectrum ͑Ando et al.,1998,Dresselhaus and Dresselhaus,2002͒.1.Cyclotron massThe energy dispersion ͑7͒resembles the energy of ul-trarelativistic particles;these particles are quantum me-chanically described by the massless Dirac equation ͑see Sec.II.B for more on this analogy ͒.An immediate con-sequence of this massless Dirac-like dispersion is a cy-clotron mass that depends on the electronic density as its square root ͑Novoselov,Geim,Morozov,et al.,2005;Zhang et al.,2005͒.The cyclotron mass is defined,within the semiclassical approximation ͑Ashcroft and Mermin,1976͒,asm *=12ͫץA ͑E ͒ץEͬE =E F,͑10͒with A ͑E ͒the area in k space enclosed by the orbit andgiven byA ͑E ͒=q ͑E ͒2=E 2v F2.͑11͒Using Eq.͑11͒in Eq.͑10͒,one obtainsm *=E Fv F2=k Fv F.͑12͒The electronic density n is related to the Fermi momen-tum k F as k F2/=n ͑with contributions from the two Dirac points K and K Јand spin included ͒,which leads tom *=ͱv Fͱn .͑13͒Fitting Eq.͑13͒to the experimental data ͑see Fig.4͒provides an estimation for the Fermi velocity andtheFIG.3.͑Color online ͒Electronic dispersion in the honeycomb lattice.Left:energy spectrum ͑in units of t ͒for finite values of t and t Ј,with t =2.7eV and t Ј=−0.2t .Right:zoom in of the energy bands close to one of the Diracpoints.FIG.4.͑Color online ͒Cyclotron mass of charge carriers in graphene as a function of their concentration n .Positive and negative n correspond to electrons and holes,respectively.Symbols are the experimental data extracted from the tem-perature dependence of the SdH oscillations;solid curves are the best fit by Eq.͑13͒.m 0is the free-electron mass.Adapted from Novoselov,Geim,Morozov,et al.,2005.113Castro Neto et al.:The electronic properties of grapheneRev.Mod.Phys.,V ol.81,No.1,January–March 2009hopping parameter as v F Ϸ106ms −1and t Ϸ3eV,respec-tively.Experimental observation of the ͱn dependence on the cyclotron mass provides evidence for the exis-tence of massless Dirac quasiparticles in graphene ͑No-voselov,Geim,Morozov,et al.,2005;Zhang et al.,2005;Deacon et al.,2007;Jiang,Henriksen,Tung,et al.,2007͒—the usual parabolic ͑Schrödinger ͒dispersion im-plies a constant cyclotron mass.2.Density of statesThe density of states per unit cell,derived from Eq.͑5͒,is given in Fig.5for both t Ј=0and t Ј 0,showing in both cases semimetallic behavior ͑Wallace,1947;Bena and Kivelson,2005͒.For t Ј=0,it is possible to derive an analytical expression for the density of states per unit cell,which has the form ͑Hobson and Nierenberg,1953͒͑E ͒=42͉E ͉t 21ͱZ 0F ͩ2,ͱZ 1Z 0ͪ,Z 0=Άͩ1+ͯE t ͯͪ2−͓͑E /t ͒2−1͔24,−t ഛE ഛt4ͯE t ͯ,−3t ഛE ഛ−t ∨t ഛE ഛ3t ,Z 1=Ά4ͯE t ͯ,−t ഛE ഛtͩ1+ͯE tͯͪ2−͓͑E /t ͒2−1͔24,−3t ഛE ഛ−t ∨t ഛE ഛ3t ,͑14͒where F ͑/2,x ͒is the complete elliptic integral of thefirst kind.Close to the Dirac point,the dispersion is ap-proximated by Eq.͑7͒and the density of states per unit cell is given by ͑with a degeneracy of 4included ͒͑E ͒=2A c ͉E ͉v F2,͑15͒where A c is the unit cell area given by A c =3ͱ3a 2/2.It is worth noting that the density of states for graphene is different from the density of states of carbon nanotubes ͑Saito et al.,1992a ,1992b ͒.The latter shows 1/ͱE singu-larities due to the 1D nature of their electronic spec-trum,which occurs due to the quantization of the mo-mentum in the direction perpendicular to the tube axis.From this perspective,graphene nanoribbons,which also have momentum quantization perpendicular to the ribbon length,have properties similar to carbon nano-tubes.B.Dirac fermionsWe consider the Hamiltonian ͑5͒with t Ј=0and theFourier transform of the electron operators,a n =1ͱN c͚ke −i k ·R na ͑k ͒,͑16͒where N c is the number of unit ing this transfor-mation,we write the field a n as a sum of two terms,coming from expanding the Fourier sum around K Јand K .This produces an approximation for the representa-tion of the field a n as a sum of two new fields,written asa n Ӎe −i K ·R n a 1,n +e −i K Ј·R n a 2,n ,b n Ӎe −i K ·R n b 1,n +e −i K Ј·R n b 2,n ,͑17͒ρ(ε)ε/tρ(ε)ε/tFIG.5.Density of states per unit cell as a function of energy ͑in units of t ͒computed from the energy dispersion ͑5͒,t Ј=0.2t ͑top ͒and t Ј=0͑bottom ͒.Also shown is a zoom-in of the density of states close to the neutrality point of one electron per site.For the case t Ј=0,the electron-hole nature of the spectrum is apparent and the density of states close to the neutrality point can be approximated by ͑⑀͒ϰ͉⑀͉.114Castro Neto et al.:The electronic properties of grapheneRev.Mod.Phys.,V ol.81,No.1,January–March 2009where the index i =1͑i =2͒refers to the K ͑K Ј͒point.These new fields,a i ,n and b i ,n ,are assumed to vary slowly over the unit cell.The procedure for deriving a theory that is valid close to the Dirac point con-sists in using this representation in the tight-binding Hamiltonian and expanding the opera-tors up to a linear order in ␦.In the derivation,one uses the fact that ͚␦e ±i K ·␦=͚␦e ±i K Ј·␦=0.After some straightforward algebra,we arrive at ͑Semenoff,1984͒H Ӎ−t͵dxdy ⌿ˆ1†͑r ͒ͫͩ3a ͑1−i ͱ3͒/4−3a ͑1+i ͱ3͒/4ͪץx +ͩ3a ͑−i −ͱ3͒/4−3a ͑i −ͱ3͒/4ͪץy ͬ⌿ˆ1͑r ͒+⌿ˆ2†͑r ͒ͫͩ3a ͑1+i ͱ3͒/4−3a ͑1−i ͱ3͒/4ͪץx +ͩ3a ͑i −ͱ3͒/4−3a ͑−i −ͱ3͒/4ͪץy ͬ⌿ˆ2͑r ͒=−i v F͵dxdy ͓⌿ˆ1†͑r ͒·ٌ⌿ˆ1͑r ͒+⌿ˆ2†͑r ͒*·ٌ⌿ˆ2͑r ͔͒,͑18͒with Pauli matrices =͑x ,y ͒,*=͑x ,−y ͒,and ⌿ˆi†=͑a i †,b i †͒͑i =1,2͒.It is clear that the effective Hamil-tonian ͑18͒is made of two copies of the massless Dirac-like Hamiltonian,one holding for p around K and the other for p around K Ј.Note that,in first quantized lan-guage,the two-component electron wave function ͑r ͒,close to the K point,obeys the 2D Dirac equation,−i v F ·ٌ͑r ͒=E ͑r ͒.͑19͒The wave function,in momentum space,for the mo-mentum around K has the form±,K ͑k ͒=1ͱ2ͩe −i k /2±e i k /2ͪ͑20͒for H K =v F ·k ,where the Ϯsigns correspond to the eigenenergies E =±v F k ,that is,for the *and bands,respectively,and k is given by Eq.͑9͒.The wave func-tion for the momentum around K Јhas the form±,K Ј͑k ͒=1ͱ2ͩe i k /2±e −i k /2ͪ͑21͒for H K Ј=v F *·k .Note that the wave functions at K and K Јare related by time-reversal symmetry:if we set the origin of coordinates in momentum space in the M point of the BZ ͑see Fig.2͒,time reversal becomes equivalent to a reflection along the k x axis,that is,͑k x ,k y ͒→͑k x ,−k y ͒.Also note that if the phase is rotated by 2,the wave function changes sign indicating a phase of ͑in the literature this is commonly called a Berry’s phase ͒.This change of phase by under rotation is char-acteristic of spinors.In fact,the wave function is a two-component spinor.A relevant quantity used to characterize the eigen-functions is their helicity defined as the projection of the momentum operator along the ͑pseudo ͒spin direction.The quantum-mechanical operator for the helicity has the formhˆ=12·p ͉p ͉.͑22͒It is clear from the definition of h ˆthat the states K͑r ͒and K Ј͑r ͒are also eigenstates of h ˆ,h ˆK ͑r ͒=±12K͑r ͒,͑23͒and an equivalent equation for K Ј͑r ͒with inverted sign.Therefore,electrons ͑holes ͒have a positive ͑negative ͒helicity.Equation ͑23͒implies that has its two eigen-values either in the direction of ͑⇑͒or against ͑⇓͒the momentum p .This property says that the states of the system close to the Dirac point have well defined chiral-ity or helicity.Note that chirality is not defined in regard to the real spin of the electron ͑that has not yet ap-peared in the problem ͒but to a pseudospin variable as-sociated with the two components of the wave function.The helicity values are good quantum numbers as long as the Hamiltonian ͑18͒is valid.Therefore,the existence of helicity quantum numbers holds only as an asymptotic property,which is well defined close to the Dirac points K and K Ј.Either at larger energies or due to the presence of a finite t Ј,the helicity stops being a good quantum number.1.Chiral tunneling and Klein paradoxIn this section,we address the scattering of chiral elec-trons in two dimensions by a square barrier ͑Katsnelson et al.,2006;Katsnelson,2007b ͒.The one-dimensional scattering of chiral electrons was discussed earlier in the context on nanotubes ͑Ando et al.,1998;McEuen et al.,1999͒.We start by noting that by a gauge transformation the wave function ͑20͒can be written as115Castro Neto et al.:The electronic properties of grapheneRev.Mod.Phys.,V ol.81,No.1,January–March 2009。
Sennheiser IE 100 PRO Wireless产品说明书

with Bluetooth® connectorFEATURES• Dynamic full-range transducer for high-resolution,powerful monitoring sound• Reduces acoustic stress factors through natural anddistortion-free reproduction• 2 in 1 bundle: Bluetooth® module for wireless connec-tion to mobile devices, PCs or tablets, with a built-inmicrophone for calls or standard 3,5 mm jack-plugcable• Excellent shielding through optimized earpiece shapeand flexible silicone and foam attachmentsFor the stage. For massive sound. For the road.Developed for high expectations on live stages, the specially designed driver of the IE 100 PRO creates precise audio cla-rity for musicians in live sessions. Typical for the new type of membrane is a powerful, high-resolution and warm monito-ring sound. With the included Bluetooth® module, the in-ears become comfortable everyday companions for your mobile phone, PC or tablet. With the built-in mic, phone calls or Webcasts are also possible.Musicians and DJs choose the IE 100 PRO wireless set for its exceptional sound and high wearing comfort. Not only for live sessions, but also for producing on the road or as an everyday companion.The in-ears come with 4 earpiece adapters that optimize the fit for every ear size and shape. The setup is stage-safe from the connection to the cable conduit.Sophisticated monitoring sound for mixing on live stages, producing in the studio and everywhere in between.DELIVERY INCLUDES• IE 100 PRO (BLACK, CLEAR or RED)• Bluetooth connector• black cable for IE 100 PRO• USB-A to USB-C cable• soft pouch• cleaning tool• foam and silicone ear adapters• quick guide• safety guide• compliance sheetwith Bluetooth® connectorPRODUCT VARIANTSIE 100 PRO WIRELESS BLACKArt. no. 509171IE 100 PRO WIRELESS CLEAR Art. no. 509172IE 100 PRO WIRELESS RED Art. no. 509173SPECIFICATIONS IE 100 PROFrequency response 20 - 18,000 Hz Impedance20 ΩSound pressure level (SPL)115 dB (1 kHz / 1 V rms )Total harmonic distortion (THD)< 0.1 % (1 kHz, 94 dB)Noise attenuation < 26 dB Magnetized field strength 1.63 mT Operating temperature Storage temperature –5 °C to +50 °C (23 °F to 122 °F)–20 °C to +70 °C (–4 °F to 158 °F)Relative humidity< 95 %Bluetooth ® ConnectorWearing style Bluetooth® neckband cable Microphone principle MEMS Microphone frequency response100 - 8,000 HzMicrophone sensitivity -42 dBV/Pa (ITU-T P.79)Microphone pick-up pattern (speech audio)omni-directional Power supply - built-in rechargeable lithium- polymer battery 3.7 V ⎓, 100 mAhUSB charging 5 V ⎓, 100 mA max.Operating time10 h (music playback via SBC) with rechargeable battery;240 h in standby mode Charging time ofrechargeable batteries approx. 2.5 hOperating temperature Charging temperature Storage temperature +5 °C to +40 °C ± 5 °C (41 °F to 104 °F ± 9 °F)+10 °C to +40 °C ± 5 °C (50 °F to 104 °F ± 9 °F)–20 °C to +70 °C (–4 °F to 158 °F)Relative humidity Operation: Storage:10 - 80 %, non-condensing 10 - 90 %Magnetized field strength1.63 mT (with IE 100 PRO)0.23 mT (without headphone)Weight approx. 13 gBluetooth®VersionBluetooth 5.0 compatible,class 1, BLETransmission frequency 2,402 - 2,480 MHz Modulation GFSK, π/4 DQPSK, 8DPSK Profiles HSP, HFP, AVRCP, A2DP Output power 10 mW (max)CodecSBC, aptX®, aptX LL®, AACThe Bluetooth® word mark and logos are registered trade-marks owned by Bluetooth SIG, Inc. and any use of such marks by Sennheiser electronic GmbH & Co. KG is under license.with Bluetooth® connectorSennheiser electronic GmbH & Co. KG · Am Labor 1 · 30900 Wedemark · Germany · ACCESSORIESIE PRO Bluetooth Connector Art. no. 508943IE PRO Mono cable Art. no. 508944Twisted cable Art. no. 507478Black straight cableArt. no. 508584。
基于压电波动法的混凝土损伤检测
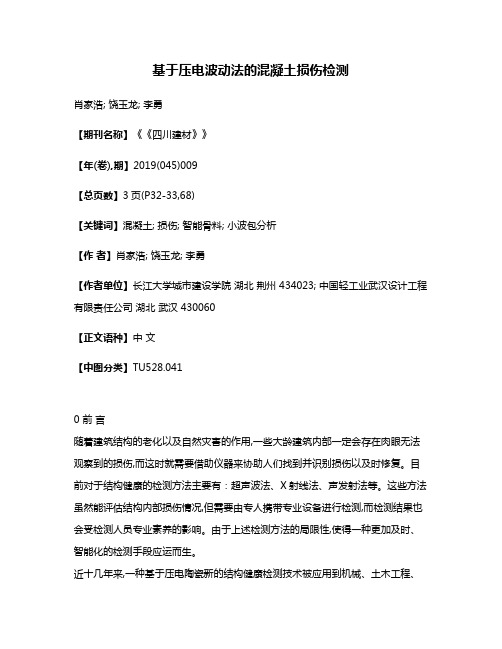
基于压电波动法的混凝土损伤检测肖家浩; 饶玉龙; 李勇【期刊名称】《《四川建材》》【年(卷),期】2019(045)009【总页数】3页(P32-33,68)【关键词】混凝土; 损伤; 智能骨料; 小波包分析【作者】肖家浩; 饶玉龙; 李勇【作者单位】长江大学城市建设学院湖北荆州 434023; 中国轻工业武汉设计工程有限责任公司湖北武汉 430060【正文语种】中文【中图分类】TU528.0410 前言随着建筑结构的老化以及自然灾害的作用,一些大龄建筑内部一定会存在肉眼无法观察到的损伤,而这时就需要借助仪器来协助人们找到并识别损伤以及时修复。
目前对于结构健康的检测方法主要有:超声波法、X射线法、声发射法等。
这些方法虽然能评估结构内部损伤情况,但需要由专人携带专业设备进行检测,而检测结果也会受检测人员专业素养的影响。
由于上述检测方法的局限性,使得一种更加及时、智能化的检测手段应运而生。
近十几年来,一种基于压电陶瓷新的结构健康检测技术被应用到机械、土木工程、航天航空等领域。
相对于其他检测技术而言,它具有体积小,响应灵敏,造价低等优点[2]。
在土木工程领域,它在混凝土结构[3-4]、钢管混凝土结构[5]、土壤[6-8]、管道[9-10]以及木结构[11-13]上都得到运用并取得很好的效果。
本文基于波动法对混凝土结构损伤进行检测并基于小波包分析的方法提取收集到的压电陶瓷信号的小波包能量。
对比得到的信号,建立混凝土健康状况与小波包能量指数之间的关系。
1 基本原理1.1 波动法原理应用压电陶瓷进行健康检测的方法主要分为主动法和被动法两类,而主动法中又有波动法和阻抗法两种方法。
由于压电陶瓷具有正逆压电效应,因此,可以同时被用作信号驱动器和信号接收器。
在波动法中一般使用两个或者多个压电智能骨料作为传感器,根据使用方法的不同分为驱动器和接收器,驱动器在高频信号的激励下产生应力波并传递给收集器,而在传播过程中信号的衰减程度主要取决于介质的特性,通过分析信号的衰减,可以识别结构内部的裂缝的存在甚至评估其损伤程度。
Installation Recharging Instruction说明书

邮编:516121As shown in picture one:1. If you would like to wear the body-pack at your waist, please first fix one side of the hook into one of the holes in the middle of the system, stretch the hook to the width of the body-pack and then fix the other side of the hook into the corresponding hole. Take care that the body-pack is not scratched by the hook.2. For recharging, please plug the adaptor into power supply and insert DC port to one of the DC female socket of the charger. The indicator of the charger will light, which means the charger is working. The body-pack can be put inside the charger for recharging.3. As shown in the picture, the body-pack hook should be placed inside the gap of the charger. Make sure you have put them in correct direction and gently press down to make sure they are well connected. The indicator lights red when recharging and turns green when it is fully recharged.4. If several units need recharging at the same time, please connect the charger units by metal connecting sheets and screws, and connect the chargers with DC connecting bars. 10 groups of chargers (20pcs of body- pack units) can be recharged at the same time.pic 1Transmitter Receiver①. Power on/off Switch:Turn on the power supply, the power indicator LED ② will light. The system willautomatically set up the same frequency as last operating channel for the transmitter or receiver.②. Power Supply and Charging Indicator:When the transmitter or receiver battery power is full, it will turn green; When the power is lower than one segment, it will turn red; When there’s no signal for receiver, the green light will flicker;When there’s normal signal for receiver, the indicator lights green permanently; When recharging, it will light red;When battery is fully charged, the red light will turn green.(Remark: It should be recharged while the system is powered off)③. SET Setting Buttons:A. Mute: The microphone signal mute for transmitter(short press the button for mute or cancelling mute).Function Buttons Instructiontime and do not recharge for a long time.Do not set the system in the charger for a long period of time (over one month), as there may affect the service life of charger and system.If the system is not in use for a long time, please remember to maintain thebattery at regular period. Generally, please recharge the battery at least once per two months so as to avoid battery damage.For any technical question regarding operation, service or accessories, please contact your local distributor.The recharging time is about 4 hours. After recharging, please shut off power in Frequency Range:50Hz-12KHzTransmit Frequency Response:780-850MHZ Number of Channels: 100Operating range:≥100m(the maximum in open area can be 300m)Transmit Power:≤10dBmReceiving Sensitivity:-120dBm S/N Ratio:≥60dBPlaytime: Approx. 12hrsRecharging Period: Approx. 4hrsBattery Specification: 3.7V/950mAh polymer lithium-ion batteryTROUBLE No soundPower indicator does not light Extreme loudsound or sound distortionLoud noise or no signal Transmitter/Receiverautomatically power off-no signalPOSSIBLE REASONThe microphone or headset is not plugged completelyThe battery of transmitter is running out of power or in low power The receiver is set at low volumeThe battery is running out ofpower or in low powerVolume of input sound is too highVolume of headphone is too highWeak signal or interferenceLow battery powerSOLUTIONPull out the microphone or headset and replugReplace or recharge thebattery Volume up the receiver Replace or recharge the batteryAdjust the distance between microphone sound capturing and sound sourceAdjust the headphone volume to a proper levelDo not get too far away from transmitter(<100m) or adjust the channelReplace or recharge the batteryTroubleshooting Dear Valued Cus omer:t Thank you for purchasing Takstar WTG-900 wireless tour guide system. In order to achieve best performance of this system, please read the instruction manual carefully.Takstar WTG-900 supports wireless talkback function, the mode of tour guide or talkback can be switched according to your using requirement. It is widely used for travelling,meeting, museum visiting, coaching, education and other wireless synchronous translation.● ● 10 sets of systems can be used at the same time without mutual interference ● More than 100m long operation range, the maximum in open area can be 300m ● Supports wireless talkback function, switch the mode of tour guide or talkback according to your using requirements● LCD-display indicates the battery level, receiving signal strength as well as the current channel to control the working state anytime● Adopts intelligent AGC audio signal processing technology for high sound capture capability and high quality sound reproduction● Powered by high capacity lithium-ion battery, playtime is more than 12hrs ● Light and durable aluminum alloy construction● Supplied with special charger and portable aluminum case for convenient outdoor using780-850MHz UHF frequency band guarantees interference-free reception Product FeaturesCG-12 Charger 1pc DC Connecting Bar 1pc Aluminum Case 1pc Metal Connecting Sheet 1pc CG-12 Charger 10pcs Screw1pcPower Adaptor 1pc WTG-900 Transmitter 1set WTG-900 Receiver19setsDC Connecting Bar9pcsAluminum CaseCharger Package: Kraft BoxWTG-900R Receiver1pc Single-side Clip-on Headset (L)1pc Single-side Clip-on Headset (R)1pc Sponge Earpads 5pcs Li-ion Battery (inlay)1pc Hanging Strap 1pc Bodypack Hook1pcWTG-900T Transmitter 1pc Headworn Microphone1pc Single-side Clip-on Headset (L)1pc Single-side Clip-on Headset (R)1pcSponge Earpads 5pcs Windscreen5pcs Lavalier Microphone1pc Li-ion Battery (inlay) 1pc Bodypack Hook 1pcHanging Strap 1pc User Manual1pcTransmitterSingle-side Clip-on headsetReceiverHeadworn Microphone Package: Kraft Box Package: Kraft BoxAB356785678A BC599756C4656789A B8107三、充电操作方法:将配备的电源适配器插入AC电源插座,再把DC头插入充电座或充电箱,此时,充电座或充电箱电源指示灯会常亮,说明工作正常。
【放射化学系列】铀、镎、钚的放射化学
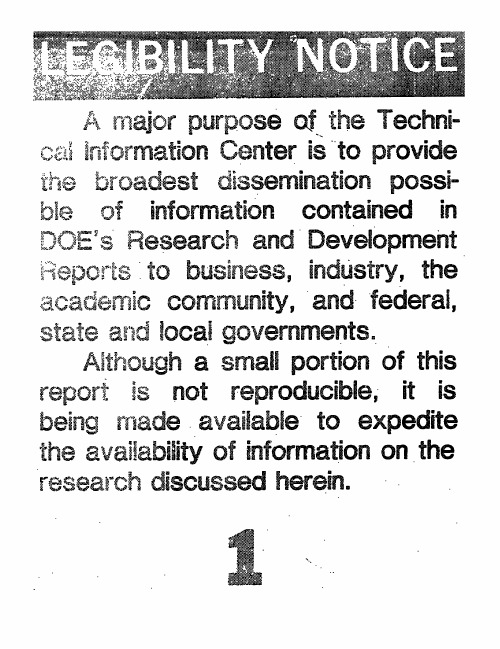
~.
. . . . . . . . . . . . . . . . . . . . . . . . . . . . . . . . .... . . . . . . . . . . . . . . . . . . ...~i~ .
~."
1.
11.
Iii.
.... ... .. .. ... ... .... . ... .. . ... .. .. ..". . .. .. . . .. . . . . . . ..iv
Ratiochemical Determination of Plutonium in Marine Samples by Extraction.Chrmatography:.............”.44 The Determination of Plutonim in EnVir~ntal Samples by Extraction with Tridodecylamine ................46 .
8.
Determination Ikanlum in NaturalMaters of
Afte=Aaion-Exchange Sepa.ration. ..........................26 Uranium kalyais by Liquid Scfnttl18tlon Counting .........28
VI.
~
Introduction.................................. ....” . .‘h ...........28 “’. \
Discussionoft heProcedures. ..................................38 Procedures:
IEEE_Spectrum_
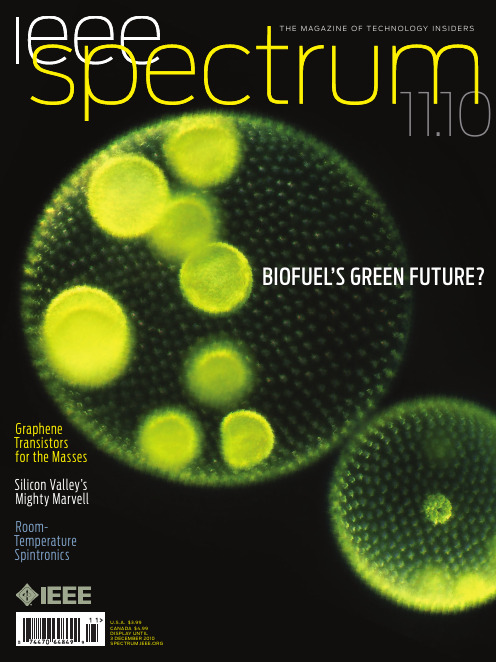
/theinstitute
available 5 November
34
40 opiNioN
10 SPECTRAL LINES tech is key to a modern economy, but it’s usually a lousy bet for an investor. By Philip E. Ross
12 FORUM For catching a fibber in the act, a technique called voice stress analysis might be better than functional mrI.
n Engage Students Using Interactive Modeling and Simulation Tools Sponsored by MathWorks http://spectrum.ieee. org/webinar/1686148
n Sponsored white paper: Requirements Engineering for the Automotive Industry Sponsored by IBM http://spectrum.ieee. org/whitepapers
have the most valuable patents in batteries, clean coal, fuel cells, and five other categories. To see where your company ranks, dive deep into our interactive charts, created by the Dutch firm Information Design Studio, and discuss the rankings in our new commenting system.
AE94.3A燃气涡轮机说明书
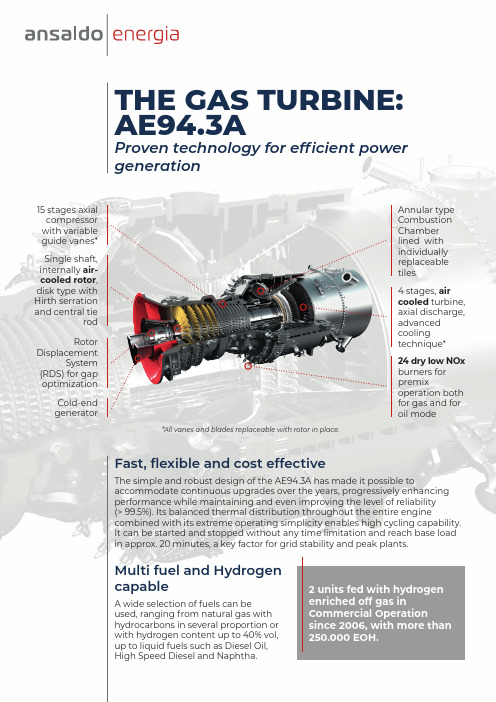
THE GAS TURBINE: AE94.3AProven technology for efficient power generationFast, flexible and cost effectiveThe simple and robust design of the AE94.3A has made it possible toaccommodate continuous upgrades over the years, progressively enhancing performance while maintaining and even improving the level of reliability (> 99.5%). Its balanced thermal distribution throughout the entire enginecombined with its extreme operating simplicity enables high cycling capability. It can be started and stopped without any time limitation and reach base load in approx. 20 minutes, a key factor for grid stability and peak plants.Single shaft, internally air-cooled rotor , disk type with Hirth serration and central tierod 15 stages axial compressor with variable guide vanes*Annular type Combustion Chamber lined with individually replaceable tilesCold-end generator24 dry low NOx burners for premixoperation both for gas and for oil modeRotorDisplacementSystem (RDS) for gap optimization2 units fed with hydrogen enriched off gas inCommercial Operationsince 2006, with more than 250.000 EOH.4 stages, air cooled turbine, axial discharge, advanced cooling technique*Multi fuel and Hydrogen capableA wide selection of fuels can beused, ranging from natural gas with hydrocarbons in several proportion or with hydrogen content up to 40% vol, up to liquid fuels such as Diesel Oil, High Speed Diesel and Naphtha.*All vanes and blades replaceable with rotor in place.Via N. Lorenzi, 8 - 16152 Genoa - Italy Tel: +39 010 6551***********************Environmental sustainableNOx level down to 15 ppm in dry gas mode and 60 ppm in dry oil mode (with possibility to reach 25 ppm with small water amount .Smart maintenance approachCustomized service agreements, including upgrading packages, allow Customers to choose the best solution to fit their needs.• Extended time between majoroverhauls (up to 5 years, depending on operating conditions)• High durability of hot gas path parts •Quick on-site activitiesGeneral note: Performance data are calculated with 100% methane (LHV) at ISO conditions, direct cooling.495992CC Net Output (MW)6060.3CC Net Efficiency (%)5,9955,970CC Net Heat Rate (kJ/kWh)4525Plant Turndown Minimum load (%)40.3Efficiency (%)755Exhaust Mass Flow (Kg/s)593Exhaust Temperature (°C)40GT minimum load (%)340Power output (MW)total > 4 millions EOHReferences:Ansaldo Energia, all rights reserved. Trademarks mentioned in this document are the property of Ansaldo Energia, its affiliates, or their respective owners in the scope of registration. The information contained in this document is merely indicative. No representation or warranty is provided, nor should be relied on, that such information is complete or correct or will apply to any particular project. This will depend on the technical and commercial circumstances. Said information is provided without liability and is subject to change without notice. Reproduction, use or disclosure to third parties, without express written authority, is strictly prohibited.。
锂离子电池在不同区间下的衰退影响因素分析及任意区间的老化趋势预测
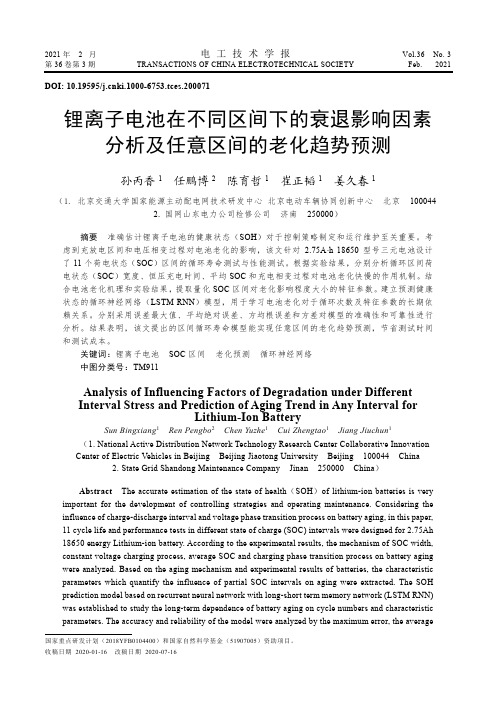
2021年2月电工技术学报Vol.36 No. 3 第36卷第3期TRANSACTIONS OF CHINA ELECTROTECHNICAL SOCIETY Feb. 2021 DOI: 10.19595/ki.1000-6753.tces.200071锂离子电池在不同区间下的衰退影响因素分析及任意区间的老化趋势预测孙丙香1任鹏博2陈育哲1崔正韬1姜久春1(1. 北京交通大学国家能源主动配电网技术研发中心北京电动车辆协同创新中心北京1000442. 国网山东电力公司检修公司济南 250000)摘要准确估计锂离子电池的健康状态(SOH)对于控制策略制定和运行维护至关重要。
考虑到充放电区间和电压相变过程对电池老化的影响,该文针对 2.75A⋅h 18650型号三元电池设计了11个荷电状态(SOC)区间的循环寿命测试与性能测试。
根据实验结果,分别分析循环区间荷电状态(SOC)宽度、恒压充电时间、平均SOC和充电相变过程对电池老化快慢的作用机制。
结合电池老化机理和实验结果,提取量化SOC区间对老化影响程度大小的特征参数。
建立预测健康状态的循环神经网络(LSTM RNN)模型,用于学习电池老化对于循环次数及特征参数的长期依赖关系。
分别采用误差最大值、平均绝对误差、方均根误差和方差对模型的准确性和可靠性进行分析。
结果表明,该文提出的区间循环寿命模型能实现任意区间的老化趋势预测,节省测试时间和测试成本。
关键词:锂离子电池SOC区间老化预测循环神经网络中图分类号:TM911Analysis of Influencing Factors of Degradation under DifferentInterval Stress and Prediction of Aging Trend in Any Interval forLithium-Ion BatterySun Bingxiang1 Ren Pengbo2 Chen Yuzhe1 Cui Zhengtao1 Jiang Jiuchun1(1. National Active Distribution Network Technology Research Center Collaborative InnovationCenter of Electric Vehicles in Beijing Beijing Jiaotong University Beijing 100044 China2. State Grid Shandong Maintenance Company Jinan 250000 China)Abstract The accurate estimation of the state of health(SOH)of lithium-ion batteries is very important for the development of controlling strategies and operating maintenance. Considering the influence of charge-discharge interval and voltage phase transition process on battery aging, in this paper,11 cycle life and performance tests in different state of charge (SOC) intervals were designed for 2.75Ah18650 energy Lithium-ion battery. According to the experimental results, the mechanism of SOC width, constant voltage charging process, average SOC and charging phase transition process on battery aging were analyzed. Based on the aging mechanism and experimental results of batteries, the characteristic parameters which quantify the influence of partial SOC intervals on aging were extracted. The SOH prediction model based on recurrent neural network with long-short term memory network (LSTM RNN) was established to study the long-term dependence of battery aging on cycle numbers and characteristic parameters. The accuracy and reliability of the model were analyzed by the maximum error, the average国家重点研发计划(2018YFB0104400)和国家自然科学基金(51907005)资助项目。
余彦教授凝胶电解质英文文章

余彦教授凝胶电解质英文文章English: Professor Yu Yan's research on gel electrolytes has made significant contributions to the development of advanced energy storage devices. Gel electrolytes, as a type of solid-state electrolyte, have the potential to improve the safety and performance of batteries. Professor Yu's team has focused on designing and synthesizing polymer gel electrolytes with high ionic conductivity and mechanical strength. This involves the use of various polymers, solvents, and additives to create a stable and efficient electrolyte that can be used in lithium-ion batteries, supercapacitors, and other energy storage systems. Their work has also involved studying the electrochemical and thermal properties of gel electrolytes, as well as optimizing their performance in different operating conditions. Overall, Professor Yu's research has advanced our understanding of gel electrolytes and paved the way for their practical application in diverse energy storage technologies.中文翻译: 余彦教授关于凝胶电解质的研究对先进能源存储设备的发展做出了重要贡献。
大面积钙钛矿组件 界面 能量损失

大面积钙钛矿组件界面能量损失英文回答:Large-area perovskite solar cells have gainedsignificant attention in recent years due to theirpotential for high power conversion efficiency and low-cost fabrication. However, one of the major challenges inscaling up perovskite solar cells is the issue of energy losses at the interfaces within the device.There are several factors that contribute to energy losses in large-area perovskite solar cells. One of themain sources of energy loss is the recombination of charge carriers at the interfaces between different layers in the device. For example, at the perovskite/electron transport layer interface, there can be a significant loss of energy due to charge recombination. This can occur when the charge carriers (electrons) in the perovskite layer recombine with the charge carriers (holes) in the electron transport layer, resulting in a loss of energy.Another source of energy loss in large-area perovskite solar cells is the reflection of light at the interfaces. When light hits the surface of the solar cell, a portion of it is reflected back instead of being absorbed andconverted into electricity. This can lead to a decrease in the overall efficiency of the device.In addition, energy losses can also occur during the charge extraction process in large-area perovskite solar cells. When the charge carriers are extracted from the device, there can be losses due to resistance in the electrical contacts or due to inefficient charge collection. These losses can further reduce the overall efficiency of the solar cell.To mitigate these energy losses, researchers are exploring various strategies. One approach is to optimize the interfaces between different layers in the device to minimize charge recombination. This can be achieved byusing suitable interface materials or engineering the interfaces to improve charge extraction and reducerecombination.Another strategy is to incorporate light-trapping structures or anti-reflection coatings to minimize light reflection at the interfaces. These structures can help increase the absorption of light and improve the overall efficiency of the solar cell.Furthermore, improving the charge extraction process is also crucial in reducing energy losses. This can be achieved by optimizing the electrical contacts and charge collection pathways within the device. For example, using materials with high conductivity and low resistance can help improve charge extraction and reduce energy losses.In conclusion, large-area perovskite solar cells face challenges in minimizing energy losses at the interfaces within the device. However, through optimization of the interfaces, incorporation of light-trapping structures, and improvement of the charge extraction process, researchers are making significant progress in reducing these losses and improving the overall efficiency of perovskite solarcells.中文回答:大面积钙钛矿太阳能电池由于其高转换效率和低成本制造的潜力,在近年来引起了广泛关注。
蓝色LED指示灯说明书
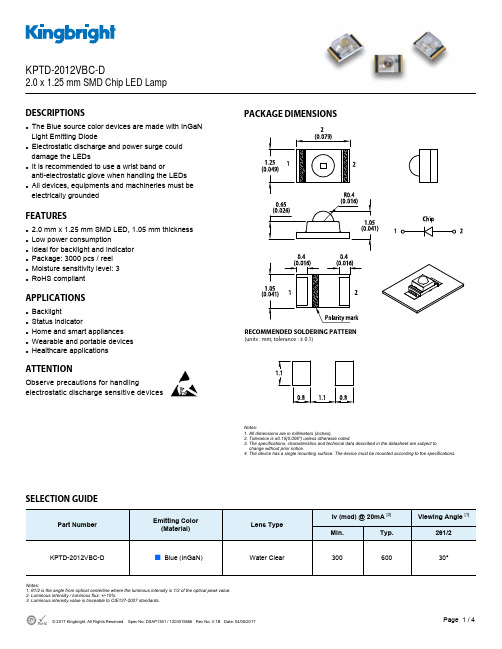
DESCRIPTIONSThe Blue source color devices are made with InGaN Light Emitting DiodeElectrostatic discharge and power surge could damage the LEDsIt is recommended to use a wrist band oranti-electrostatic glove when handling the LEDs All devices, equipments and machineries must be electrically groundedFEATURES2.0 mm x 1.25 mm SMD LED, 1.05 mm thickness Low power consumptionIdeal for backlight and indicator Package: 3000 pcs / reel Moisture sensitivity level: 3RoHS compliantAPPLICATIONSBacklightStatus indicatorHome and smart appliances Wearable and portable devicesHealthcare applicationsATTENTIONObserve precautions for handlingelectrostatic discharge sensitive devicesPACKAGE DIMENSIONSSELECTION GUIDENotes:1. θ1/2 is the angle from optical centerline where the luminous intensity is 1/2 of the optical peak value.2. Luminous intensity / luminous flux: +/-15%.3. Luminous intensity value is traceable to CIE127-2007 standards.Part NumberEmitting Color (Material)Lens TypeIv (mcd) @ 20mA [2] Viewing Angle [1]Min.Typ.2θ1/2KPTD-2012VBC-DWater Clear ■ Blue (InGaN)300 600 30°KPTD-2012VBC-D2.0 x 1.25 mm SMD Chip LED LampRECOMMENDED SOLDERING PATTERN(units : mm; tolerance : ± 0.1)Notes:1. All dimensions are in millimeters (inches).2. Tolerance is ±0.15(0.006") unless otherwise noted.3. The specifications, characteristics and technical data described in the datasheet are subject to change without prior notice.4. The device has a single mounting surface. The device must be mounted according to the specifications.ABSOLUTE MAXIMUM RATINGS at T A =25°CELECTRICAL / OPTICAL CHARACTERISTICS at T A =25°CParameterSymbolEmitting ColorValue UnitTyp. Max. Wavelength at Peak Emission I F = 20mA λpeak Blue 465 - nm Dominant Wavelength I F = 20mA λdom [1] Blue470-nmSpectral Bandwidth at 50% Φ REL MAX I F = 20mA ΔλBlue 22 - nmCapacitanceC Blue 100 - pFForward Voltage I F = 20mA V F [2]Blue 3.3 4 VReverse Current (V R = 5V) I R Blue - 50 uATemperature Coefficient of λpeak I F = 20mA, -10°C ≤ T ≤ 85°C TC λpeak Blue 0.04 - nm/°CTemperature Coefficient of λdom I F = 20mA, -10°C ≤ T ≤ 85°C TC λdom Blue 0.03 - nm/°CTemperature Coefficient of V F I F = 20mA, -10°C ≤ T ≤ 85°CTC V Blue -2.9 - mV/°CParameterSymbolUnit Power Dissipation P D 120 mW Reverse Voltage V R 5 V Junction Temperature T j 115 °C Operating Temperature T op -40 to +85 °C Storage Temperature T stg -40 to +85°C DC Forward Current I F 30 mA Peak Forward CurrentI FM [1]100 mA Thermal Resistance (Junction / Ambient) R th JA [2] 470 °C/W Thermal Resistance (Junction / Solder point)R th JS [2]360°C/WValue Electrostatic Discharge Threshold (HBM) -250 V Notes:1. The dominant wavelength (λd) above is the setup value of the sorting machine. (Tolerance λd : ±1nm. )2. Forward voltage: ±0.1V.3. Wavelength value is traceable to CIE127-2007 standards.4. Excess driving current and / or operating temperature higher than recommended conditions may result in severe light degradation or premature failure.Notes:1. 1/10 Duty Cycle, 0.1ms Pulse Width.2. R t h JA ,R t h JS Results from mounting on PC board FR4 (pad size ≥ 16 mm 2 per pad).3. Relative humidity levels maintained between 40% and 60% in production area are recommended to avoid the build-up of static electricity – Ref JEDEC/JESD625-A and JEDEC/J-STD-033.TECHNICAL DATABLUETAPE SPECIFICATIONS (units : mm)REEL DIMENSION (units : mm)REFLOW SOLDERING PROFILE for LEAD-FREE SMD PROCESSNotes:1. Don't cause stress to the LEDs while it is exposed to high temperature.2. The maximum number of reflow soldering passes is 2 times.3. Reflow soldering is recommended. Other soldering methods are not recommended as they mightcause damage to the product.PACKING & LABEL SPECIFICATIONSPRECAUTIONARY NOTES1. The information included in this document reflects representative usage scenarios and is intended for technical reference only.2. The part number, type, and specifications mentioned in this document are subject to future change and improvement without notice. Before production usage customer should refer tothe latest datasheet for the updated specifications.3. When using the products referenced in this document, please make sure the product is being operated within the environmental and electrical limits specified in the datasheet. Ifcustomer usage exceeds the specified limits, Kingbright will not be responsible for any subsequent issues.4. The information in this document applies to typical usage in consumer electronics applications. If customer's application has special reliability requirements or have life-threateningliabilities, such as automotive or medical usage, please consult with Kingbright representative for further assistance.5. The contents and information of this document may not be reproduced or re-transmitted without permission by Kingbright.6. All design applications should refer to Kingbright application notes available at /application notes。
Battery Management Systems
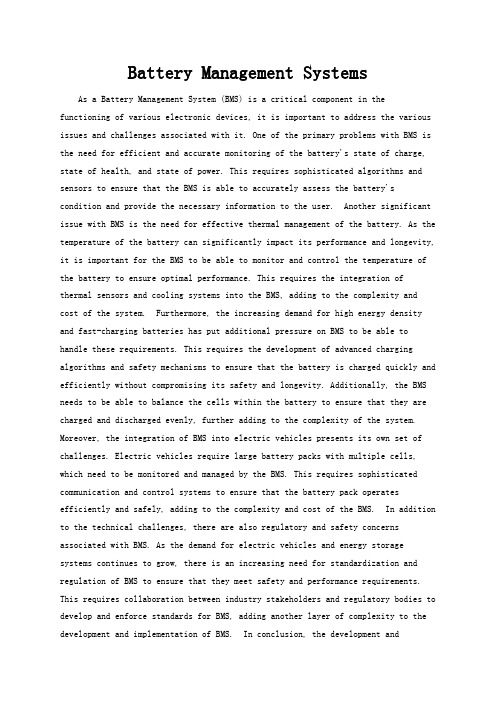
Battery Management Systems As a Battery Management System (BMS) is a critical component in thefunctioning of various electronic devices, it is important to address the various issues and challenges associated with it. One of the primary problems with BMS is the need for efficient and accurate monitoring of the battery's state of charge, state of health, and state of power. This requires sophisticated algorithms and sensors to ensure that the BMS is able to accurately assess the battery's condition and provide the necessary information to the user. Another significant issue with BMS is the need for effective thermal management of the battery. As the temperature of the battery can significantly impact its performance and longevity, it is important for the BMS to be able to monitor and control the temperature of the battery to ensure optimal performance. This requires the integration of thermal sensors and cooling systems into the BMS, adding to the complexity and cost of the system. Furthermore, the increasing demand for high energy density and fast-charging batteries has put additional pressure on BMS to be able to handle these requirements. This requires the development of advanced charging algorithms and safety mechanisms to ensure that the battery is charged quickly and efficiently without compromising its safety and longevity. Additionally, the BMS needs to be able to balance the cells within the battery to ensure that they are charged and discharged evenly, further adding to the complexity of the system. Moreover, the integration of BMS into electric vehicles presents its own set of challenges. Electric vehicles require large battery packs with multiple cells, which need to be monitored and managed by the BMS. This requires sophisticated communication and control systems to ensure that the battery pack operates efficiently and safely, adding to the complexity and cost of the BMS. In addition to the technical challenges, there are also regulatory and safety concerns associated with BMS. As the demand for electric vehicles and energy storage systems continues to grow, there is an increasing need for standardization and regulation of BMS to ensure that they meet safety and performance requirements. This requires collaboration between industry stakeholders and regulatory bodies to develop and enforce standards for BMS, adding another layer of complexity to the development and implementation of BMS. In conclusion, the development andimplementation of BMS present a multitude of challenges, ranging from technical and regulatory to safety and cost-related issues. Addressing these challenges requires collaboration between industry stakeholders, regulatory bodies, and research institutions to develop advanced technologies and standards for BMS. While these challenges may seem daunting, they also present opportunities for innovation and growth in the field of battery management systems, ultimately leading to safer, more efficient, and higher-performing batteries for various applications.。
Microstructure evolution and mechanical properties of
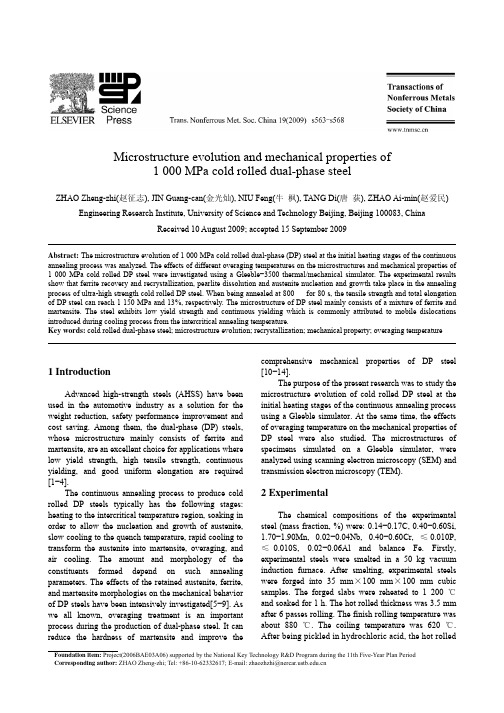
Microstructure evolution and mechanical properties of1 000 MPa cold rolled dual-phase steelZHAO Zheng-zhi(赵征志), JIN Guang-can(金光灿), NIU Feng(牛枫), TANG Di(唐荻), ZHAO Ai-min(赵爱民) Engineering Research Institute, University of Science and Technology Beijing, Beijing 100083, ChinaReceived 10 August 2009; accepted 15 September 2009Abstract: The microstructure evolution of 1 000 MPa cold rolled dual-phase (DP) steel at the initial heating stages of the continuous annealing process was analyzed. The effects of different overaging temperatures on the microstructures and mechanical properties of 1 000 MPa cold rolled DP steel were investigated using a Gleeble−3500 thermal/mechanical simulator. The experimental results show that ferrite recovery and recrystallization, pearlite dissolution and austenite nucleation and growth take place in the annealing process of ultra-high strength cold rolled DP steel. When being annealed at 800 ℃ for 80 s, the tensile strength and total elongation of DP steel can reach 1 150 MPa and 13%, respectively. The microstructure of DP steel mainly consists of a mixture of ferrite and martensite. The steel exhibits low yield strength and continuous yielding which is commonly attributed to mobile dislocations introduced during cooling process from the intercritical annealing temperature.Key words: cold rolled dual-phase steel; microstructure evolution; recrystallization; mechanical property; overaging temperature1 IntroductionAdvanced high-strength steels (AHSS) have been used in the automotive industry as a solution for the weight reduction, safety performance improvement and cost saving. Among them, the dual-phase (DP) steels, whose microstructure mainly consists of ferrite and martensite, are an excellent choice for applications where low yield strength, high tensile strength, continuous yielding, and good uniform elongation are required [1−4].The continuous annealing process to produce cold rolled DP steels typically has the following stages: heating to the intercritical temperature region, soaking in order to allow the nucleation and growth of austenite, slow cooling to the quench temperature, rapid cooling to transform the austenite into martensite, overaging, and air cooling. The amount and morphology of the constituents formed depend on such annealing parameters. The effects of the retained austenite, ferrite, and martensite morphologies on the mechanical behavior of DP steels have been intensively investigated[5−9]. As we all known, overaging treatment is an important process during the production of dual-phase steel. It can reduce the hardness of martensite and improve the comprehensive mechanical properties of DP steel [10−14].The purpose of the present research was to study the microstructure evolution of cold rolled DP steel at the initial heating stages of the continuous annealing process using a Gleeble simulator. At the same time, the effects of overaging temperature on the mechanical properties of DP steel were also studied. The microstructures of specimens simulated on a Gleeble simulator, were analyzed using scanning electron microscopy (SEM) and transmission electron microscopy (TEM).2 ExperimentalThe chemical compositions of the experimental steel (mass fraction, %) were: 0.14−0.17C, 0.40−0.60Si, 1.70−1.90Mn, 0.02−0.04Nb, 0.40−0.60Cr, ≤0.010P, ≤0.010S, 0.02−0.06Al and balance Fe. Firstly, experimental steels were smelted in a 50 kg vacuum induction furnace. After smelting, experimental steels were forged into 35 mm×100 mm×100 mm cubic samples. The forged slabs were reheated to 1 200 ℃and soaked for 1 h. The hot rolled thickness was 3.5 mm after 6 passes rolling. The finish rolling temperature was about 880 ℃. The coiling temperature was 620 ℃. After being pickled in hydrochloric acid, the hot rolledFoundation item: Project(2006BAE03A06) supported by the National Key Technology R&D Program during the 11th Five-Year Plan Period Corresponding author: ZHAO Zheng-zhi; Tel: +86-10-62332617; E-mail: zhaozhzhi@ZHAO Zheng-zhi, et al/Trans. Nonferrous Met. Soc. China 19(2009) s563−s568 s564bands were cold rolled to the final thickness of 1.0 mm, and the reduction was about 70%. Finally, the cold rolled sheets were cut into the samples for the simulation of continuous annealing experiment.The microstructure evolution at the initial steps of the continuous annealing process was studied using a Gleeble 1500 simulator. The steel was heated at 10 ℃/sto the different heating temperatures (550, 630, 670, 710, 730, 750 and 780 ℃) and held for 20 s followed by water-quenching. The effects of different overaging temperatures on the microstructures and mechanical properties of DP steel were investigated using a Gleeble 3500 simulator. The processing schedules and parameters used are shown in Fig.1. The soaking temperature of intercritical region was set at 800 ℃, soaking time is 80 s; after a slow cooling, the samples were rapidly cooled to 240, 280, 320 and 360 ℃, respectively and soaked for 300 s; at last, the samples were air cooled to the room temperature.Fig.1 Continuous annealing process of DP steelAfter heat treatment, the steel sheet would be cut into standard tensile specimens (length 200 mm, gauge length 50 mm). The tensile test was performed with CMT4105-type tensile test machine to test mechanical properties. The longitudinal cold rolling plane sections of samples after annealing were prepared and etched with 4% natal. The microstructure was analyzed by scanning electron microscopy (SEM). Some samples were analyzed using transmission electron microscopy (TEM).3 Results and discussion3.1 Mechanical properties and microstructures ofsamples after hot-rolling and continuousannealingTable 1 shows the tensile test data for the two samples after hot-rolling and continuous annealing in terms of yield strength, ultimate tensile strength and total elongation. When the annealing temperature is 800 ℃and soaking time is 60 s, the tensile strength reaches 1 110 MPa and the total elongation reaches 12%. Compared with the hot-rolled samples, the yield strength and total elongation of sample after annealing are similar, but the tensile strength increases by about 450 MPa. The yield ratio decreases obviously. The engineering uniaxial tensile stress—strain curve of the sample after continuous annealing is characterized by very uniform plastic flow until necking. There is no physical yield point and yield point extension, that is, the steel exhibits continuous yielding which is commonly attributed to mobile dislocations introduced during cooling from the intercritical annealing temperature. Many dislocation sources come into action at low strain and plastic flow begins simultaneously through the specimen, thereby suppressing discontinuous yielding[15].Table 1 Mechanical properties of samples after hot rolling and annealingConditionYieldstrength/MPaTensilestrength/MPaYieldratio*Totalelongation/% Hot rolling555 665 0.83 16 Annealing540 1110 0.49 12* Yield ratio is defined as the ratio of yield strength to tensile strength.The microstructures of the hot-rolled and cold-rolled samples are shown in Fig.2. It can be observed that hot rolled steel features a band microstructure, i.e. pearlite band in a ferrite grain matrix. The ferrite grain size is measured to be 5.0−9.0 µm. After cold rolling, the microstructure consists of elongated grains of ferrite and deformed colonies of pearlite (Fig.2(b)). After cold-rolling, there is an increase in the stored energy of the steel due to the high dislocation density and this provides the driving pressure for the ferrite recrystallization during annealing process. The total ferrite grain boundary area increases and the cementite laminar structure in pearlite is broken down. The latter has been shown to promote spheroidization of cementite during subsequent annealing process.The SEM micrograph of the sample after annealing is given in Fig.3(a). The microstructure of DP steel consists of a mixture of ferrite, martensite, martensite/austenite constituent. There is also some bainite in the microstructure. The martensite islands are homogeneously distributed in ferrite matrix. The DP steel has finer grain size and the size of ferrite grain and martensite island are about 1.0−2.0 µm. Some martensite islands have a bright white circle around the edge, and the center of martensite is of irregular black structure.ZHAO Zheng-zhi, et al/Trans. Nonferrous Met. Soc. China 19(2009) s563−s568 s565Fig.2 Microstructures of steel after hot rolling (a) and cold rolling (b)Fig.3 SEM images (a) and TEM micrograph (b) of steel after continuous annealingThe main reason is the manganese partitioning will occur during the continuous annealing process. During the heating process, a high-Mn side lap forms around austenite, which makes the hardenability of austenite island edge higher than that of the center. So, it makes high-Mn side lap form around martensite in the cooling process. The volume fraction of martensite is about 40%, which is the main reason for DP steel with a higher strength. After the continuous annealing process, band structure is significantly improved, which plays an important role in improving the performance of DP steel.The fine structures of martensite and ferrite are shown in Fig.3(b) by the TEM observation. The lath martensite is fine, and is relatively clean; at the same time, a very high density of dislocations can be observed in the ferrite grain adjacent to martensite. These dislocations are generated in order to accommodate transformation induced strain built between martensite transformed by quenching and retained ferrite. In addition, they are known to be mobile and play an important role on rapid, extensive strain hardening of DP steel from the onset of its plastic deformation.3.2 Microstructure evolution at initial steps ofcontinuous annealing processThe microstructure evolution at the initial stages of the continuous annealing process is very important for producing the ultra-high strength DP steel. During the annealing process of high strength DP steel, ferrite recovery and recrystallization, pearlite dissolution and austenite nucleation and growth will occur. When the sample is heated to 550 , the℃microstructure has no visible change as compared with the cold rolled sample. The ferrite grain is stretched along the rolling direction significantly; lamellar pearlite is stretched along the rolling direction too. At the same time, there are some carbide particles in the ferrite matrix, as shown in Fig.4(a). At this temperature, the recrystallization nucleus was not found in the structure. So, at this stage the sample is still at the recovery stage. When the heating temperature is 630 , the℃recrystallization nucleus begins to appear in the microstructure. The nucleus of crystal appears mainly nearby the large deformation ferrite (Fig.4(b)). The recrystallization nucleus is fine and equiaxed. Large deformation storage power is present in the large deformation region. So, recrystallization nucleus forms in this region firstly. With the heating temperature increasing, the recrystallization nucleus begins to grow. Therefore, the size of recrystallization is uneven at this stage, as shown in Fig.4(c). When the heating temperature is 670 ℃, the deformation structure still exists in the microstructure. With the temperature increasing, the deformed ferrite grains are replaced by recrystallization ferrite grains. When the heating temperature is 710 , the d℃eformation structure has already vanished, which is replaced by theZHAO Zheng-zhi, et al/Trans. Nonferrous Met. Soc. China 19(2009) s563−s568 s566equiaxed recrystallization grain. So, the process of recrystallization completes basically. In the ferrite recrystallization process, the pearlite transforms to granular from lamellar gradually.When the heating temperature is 730 ,℃it begins to enter the two-phase region; and the ferrite and spheroidised carbides begin to transform to austenite. A small amount of austenite nucleates in the original pearlite region, as shown in Fig.4(e). Austenite nucleates mainly in the ferrite and pearlite grain boundary; and a part of austenite also nucleates in the carbide particles of ferrite. After austenite nucleation, it begins to grow rapidly. At this stage, the pearlite dissolves rapidly. When the temperature reaches 750 , the austenite℃transformation occurs obviously. The bright white particle which distributes in the ferrite matrix is the martensite island. The martensite transforms from austenite during the rapid cooling process. At the same time, a small amount of martensite particles can also be observed in ferrite; and there are still some non-dissolved carbide particles in the ferrite matrix. The initial austenite growing-up is mainly controlled by the carbon Fig.4Microstructure evolutions duringcontinuous heating process: (a) 550 ℃; (b)630 ℃; (c) 670 ℃; (d) 710 ℃; (e) 730 ℃; (f)750 ℃; (g) 780 ℃ZHAO Zheng-zhi, et al/Trans. Nonferrous Met. Soc. China 19(2009) s563−s568 s567diffusion in the austenite, and the diffusion path is along the pearlite/austenite interface. When the annealing temperature is 780 , the austenite volume increase℃s, and the number of carbide particles is reduced gradually. There is only a very small amount of carbide particles distributing in ferrite matrix.3.3 Effect of overaging temperature onmicrostructure and mechanical properties ofDP steelThe overaging is a temper treatment to harden martensite in the dual-phase steel, reduce the hardness of martensite and improve the comprehensive mechanical properties[16]. Fig.5 shows the effect of overaging temperature on the mechanical properties of dual-phase steel. All the samples are intercritically annealed at 800℃ with different overaging temperatures. As can be seen from Fig.5, the highest tensile strength is achieved in the sample overaged at 280 ℃. The yield strength is 560 MPa, the tensile strength is 1 150 MPa, and the total elongation reaches 13%. The good combination of high strength and toughness properties is obtained. And then, with the increase of overaging temperature, the yield strength and tensile strength of samples decrease, while the total elongation increases. When the overaging temperature reaches 360 ℃, the tensile strength decreases, the yield strength does not change significantly. The mechanical properties of sample cannot meet the necessary requirements of CR980DP. At the same time, the stress—strain curve of the steel shows discontinuous yielding behaviour and develops yield plateaus.Fig.6 shows the SEM microstructures with different overaging temperatures. It can be seen that the microstructure mainly consists of dark grey ferrite grains and white martensite. When the overaging temperature is 360 ℃, the martensite boundary is fuzzier than that of sample overaged at 320 ℃, and there are more carbides, which is due to the effects of tempering on the martensite, such as the volume contraction of martensite during the tempering, the changes of the martensite strength and additional carbon clustering or precipitation near the ferrite and martensite interfaces.Fig.5 Effects of different overaging temperatures on mechanical propertiesFig.6 SEM images of microstructures of DP steel overaged at different temperatures: (a) 240 ℃; (b) 280 ℃; (c) 320 ℃; (d) 360 ℃ZHAO Zheng-zhi, et al/Trans. Nonferrous Met. Soc. China 19(2009) s563−s568 s5684 Conclusions1) When the DP steel is annealed at 800 ℃ for 80 s and overaged at 280 ℃, the tensile strength and total elongation of ultra-high strength dual-phase steel can reach 1 150 MPa and 13%, respectively.2) The microstructure of DP steel consists of a mixture of ferrite, martensite, martensite/austenite constituent. There are also some bainites in the microstructure. The martensite islands are homogeneously distributed in ferrite matrix. The ferrite and martensite island grain size are about 1.0−2.0 µm. When the overaging temperature reaches 360 ℃, the tensile strength decreases, the yield strength does not change significantly. The mechanical properties of sample cannot meet the necessary requirements of CR980DP. At the same time, the steel shows discontinuous yielding behaviour and develops yield plateaus.References[1]KANG Yong-lin. Quality control and formability of the mordernMotor plate [M]. Beijing: Metallurgical Industry Press, 1999.[2]LIU Peng, JIN Xian-zhe. The development and research ofautomobile steel plate [J]. Shanxi Metallurgy, 1997(2): 32−33.[3]MA Ming-tu, WU Bao-rong. Dual-phase steel-the physical andmechanical metallurgy [M]. Beijing: Metallurgical Industry Press,1988.[4]LLEWELLYN D T,HILLS D J. Dual phase steels [J]. Ironmakingand Steelmaking, 1996(6): 471−478.[5]SARKAR P P. Microstructural influence on the electrochemicalcorrosion behaviour of dual phase steels in 3.5% NaCl solution [J].Materials Letters, 2005(59): 2488−2491. [6]ROCHA R O, MELO T M F, PERELOMA E V, SANTOS D B.Microstructural evolution at the initial stages of continuous annealingof cold rolled dual-phase steel [J]. Materials Science and EngineeringA, 2005, 391: 296−304.[7]MA C, CHEN D L, BHOLE S D, BOUDREAU G, LEE A, BIRO E.Microstructure and fracture characteristics of spot-welded DP600 steel [J]. Materials Science and Engineering A, 2008, 485: 334−346.[8]SUN Shou-jin, Martin P. Manganese partitioning in dual-phase steelduring annealing [J]. Materials Science and Engineering A, 2000, 276: 167−174.[9]ZHU Xiao-dong, WANG Li. Effect of the continuous annealingparameters on the mechanical properties of cold rolled Si-Mn dualphase steel [C]//CSM 2003 Annual Meeting Proceedings, 2003: 684−688.[10]MOHAMMAD R A, EKRAMI A. Effect of ferrite volume fractionon work hardening behavior of high bainite dual phase (DP) steels [J].Materials Science and Engineering A, 2008, 477: 306−310.[11]HA VV A K Z, CEYLAN K, HUSEYIN A. Investigation of dual phasetransformation of commercial low alloy steels: Effect of holding timeat low inter-critical annealing temperatures [J]. Materials Letters, 2008, 62: 2651−2653.[12]DOU Ting-ting, KANG Yong-lin, YU Hao, KUANG Shuang, LIURen-dong, YAN Ling. Microstructural evolution of cold rolled dualphase steel during initial stages of continuous annealing [J]. Heat Treatment of Metal, 2008, 33(3): 31−35.[13]CHEN Hui-feng, ZHANG Qing-fen, AN Jia-shen. Recrystallizationcharacteristic of IF steel during rapid heating [J]. Journal of East China University of Metallurgy, 1999, 16(1): 21−23.[14]YANG D Z, BROWNEL E L, MATLOCK D K, et al. Ferriterecrystallization and austenite formation in cold rolled intercriticallyannealed steel [J]. Metallurgical Transactions A, 1985, 16A: 1385−391.[15]SULEYMAN G. Static strain ageing behaviour of dual phase steels[J]. Materials Science and Engineering A, 2008, 486: 63−71.[16]KUANG Shuang, KANG Yong-lin, YU Hao, LIU Ren-dong, YANLing. Experimental study on microstructure evolution in continuousannealing of cold-rolled dual phase steels [J]. Iron and Steel, 2007,42(11): 65−73.(Edited by CHEN Ai-hua)。
改善混凝土剪力墙结构的智能材料-土木工程专业毕业设计外文资料翻译
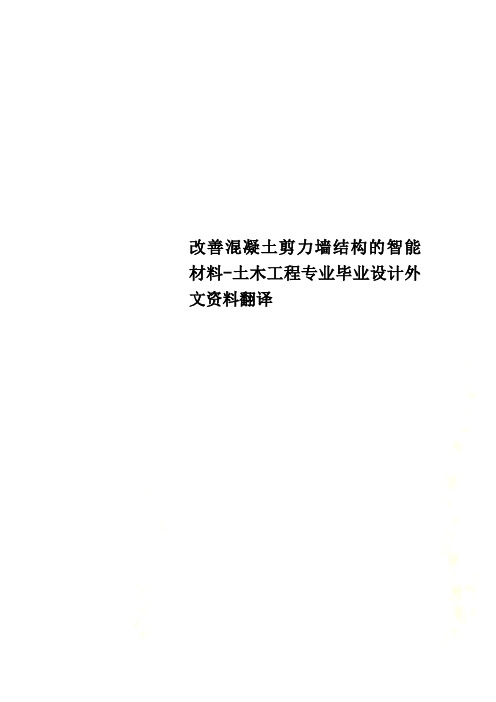
改善混凝土剪力墙结构的智能材料-土木工程专业毕业设计外文资料翻译毕业设计外文资料翻译题目改善混凝土剪力墙结构的智能材料学院土木建筑学院专业土木工程专业班级土木1105班学生 xxx学号 20110622198指导教师 xxx二〇一五年三月二十四日Open Journal of Civil Engineering, 2012, 2, 87-95改善混凝土剪力墙结构的智能材料Mehdi Ghassemieh 1* , Mohammad Reza Bahaari 1 , Seyed Mohyeddin Ghodratian1 , Seyed Ali Nojoumi 21 School of Civil Engineering, University of Tehran, Tehran, Iran2 Civil & Environmental Engineering Department, University of California, Los Angeles, USA文摘近几年土木工程许多领域都采用了智能材料。
这些材料之一里亚尔是形状记忆合金(SMA),它超弹性和形状等表现出一些独特的特征记忆效应。
由于这些特点,研究工作一直延伸到使用SMA在控制民用建筑。
探讨SMA增援的有效性提高剪力墙的行为,特别是在地震波作用的情况下。
两个普通剪力墙和耦合建模参考结构,介绍了有限元分析软件。
为提高剪力墙的地震响应,提出了在每一个连系梁结构和耦合的剪力墙体系的高度内垂直SMA钢筋实现向传统钢铁增援。
其中一维超弹性模型SMA材料中实现计算机软件使用FORTRAN语言代码。
埃尔森- 1 -莱蒙评价使用SMA限位减少甲板的响应在多跨简支梁桥[ 6 ]效率。
益田和努里基于形状记忆合金的超弹性阻尼装置[ 7 ]滞回特性优化研究。
DesRoches等人通过实验评估的属性是评估在抗地震设计和改造[8]中超弹性Ni-Ti形状记忆合金在循环荷载下其潜在的应用。
信号稀疏分解降噪在DOA估计中的应用
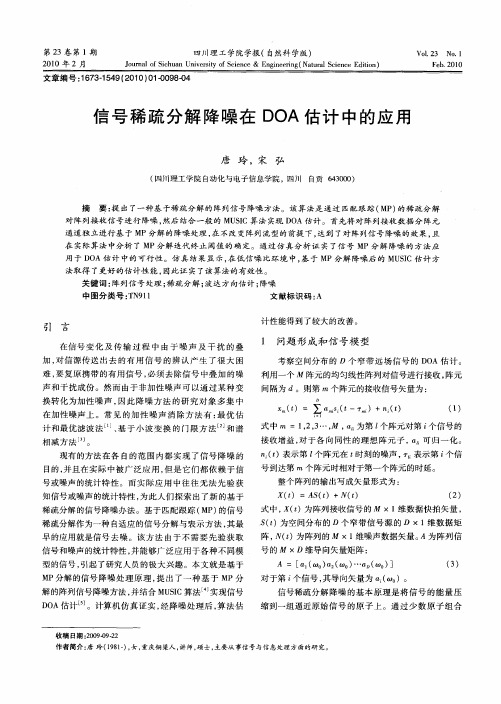
Vo. No 123 .1 Fe . 01 b2 0
文章 编号 :6 314 (0 0 0 .0 80 17 ・ 9 2 1 )10 9 - 5 - 4
信 号 稀疏 分 解 降噪在 D OA估 计 中 的应 用
唐 玲 ,宋 弘
( 四川理 工学院 自动化 与电子信息学 院 , 四川 自贡 6 30 ) 4 0 0
号 的 M ×D 维 导 向矢量 矩 阵 :
信号和噪声的统计特性 , 并能够广泛应 用于各种不 同模
型 的信 号 , 引起 了研 究人 员 的极 大 兴 趣 。本 文 就 是 基 于
A =[ 1∞ ) 20 ) D 0 ] 0 ( 0 0(9 …a ( ) 0
() 3
M P分解 的信号 降噪处理原 理 , 出了一种基 于 M 提 P分
中图分 类 号 :N 1 T91 文献 标 识码 : A
引 言
在 信 号 变 化 及 传 输 过 程 中 由 于 噪 声 及 干 扰 的 叠
计性能得到 了较大的改善。
1 问题 形 成 和信 号模 型
考察 空 间分 布 的 D 个 窄 带 远 场 信 号 的 D A 估 计 。 O 利用 一个 M 阵元 的均匀 线 性 阵列 对信 号进 行 接 收 , 元 阵
相 减方 法 。
间隔为 d。则第 m个阵元 的接收信号矢量为 :
D
X( =∑i s £ 7 ) r £ r£ a) X 一 +t ) m( i i (
() 1
式 中 m =12 3 M , , ,…, a 为第 z 个阵元对第 i 个信号的
接 收 增益 , 于 各 向 同性 的 理 想 阵 元 子 ,a 对 可 归 一 化 。
gsensor 跌落算法 -回复

gsensor 跌落算法-回复什么是gsensor跌落算法?Gsnesor跌落算法是一种用于检测设备跌落的算法。
Gsnesor是指G-Sensor,全称为Gravity Sensor,也称为accelerometer(加速度计)。
它是一种用于测量物体加速度的传感器。
Gsnesor跌落算法通过利用Gsnesor设备内部的加速度计传感器,可以检测设备是否发生了跌落,并根据不同情况采取相应的措施,比如自动关闭设备或产生警报。
为什么需要gsensor跌落算法?在现代社会,人们越来越依赖各种便携式电子设备,比如智能手机、平板电脑和笔记本电脑。
然而,由于这些设备的便携性,它们常常会在使用过程中发生跌落,导致设备损坏或数据丢失。
因此,为了提高设备的稳定性和安全性,有必要使用gsensor跌落算法。
Gsnesor跌落算法的原理是什么?Gsnesor跌落算法主要基于Gsnesor内部的加速度计传感器工作原理。
加速度计能够测量设备在不同方向上的加速度变化情况。
当设备发生跌落时,由于重力和冲击力的作用,设备的加速度将会发生巨大变化。
Gsnesor 跌落算法通过监测加速度的变化来判断设备是否发生了跌落。
Gsnesor跌落算法的工作流程是怎样的?1. 采集数据:Gsnesor跌落算法首先需要采集Gsnesor传感器的数据。
该数据包括设备在不同方向上的加速度值。
2. 分析数据:通过分析采集到的数据,Gsnesor跌落算法可以计算出设备的加速度变化情况。
例如,可以通过比较当前加速度值与之前的加速度值,来判断设备的加速度是否发生了突变。
3. 判断跌落:Gsnesor跌落算法通过设定一定的阈值来判断设备是否发生了跌落。
当设备的加速度变化超过设定的阈值时,算法会认为设备发生了跌落。
4. 执行相应措施:一旦Gsnesor跌落算法判断设备发生了跌落,它可以执行相应的措施。
例如,当设备跌落时,算法可以触发自动关闭设备的功能,以避免进一步损坏;或者可以触发警报功能,以提醒用户设备的安全问题。
聚合物固态电解质机械抑制枝晶生长
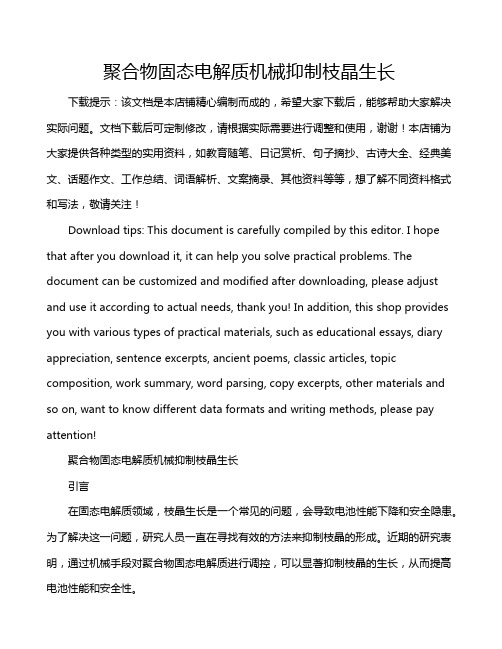
聚合物固态电解质机械抑制枝晶生长下载提示:该文档是本店铺精心编制而成的,希望大家下载后,能够帮助大家解决实际问题。
文档下载后可定制修改,请根据实际需要进行调整和使用,谢谢!本店铺为大家提供各种类型的实用资料,如教育随笔、日记赏析、句子摘抄、古诗大全、经典美文、话题作文、工作总结、词语解析、文案摘录、其他资料等等,想了解不同资料格式和写法,敬请关注!Download tips: This document is carefully compiled by this editor. I hope that after you download it, it can help you solve practical problems. The document can be customized and modified after downloading, please adjust and use it according to actual needs, thank you! In addition, this shop provides you with various types of practical materials, such as educational essays, diary appreciation, sentence excerpts, ancient poems, classic articles, topic composition, work summary, word parsing, copy excerpts, other materials and so on, want to know different data formats and writing methods, please pay attention!聚合物固态电解质机械抑制枝晶生长引言在固态电解质领域,枝晶生长是一个常见的问题,会导致电池性能下降和安全隐患。
HSMOTE-AdaBoost

第13卷㊀第7期Vol.13No.7㊀㊀智㊀能㊀计㊀算㊀机㊀与㊀应㊀用IntelligentComputerandApplications㊀㊀2023年7月㊀Jul.2023㊀㊀㊀㊀㊀㊀文章编号:2095-2163(2023)07-0007-08中图分类号:TP181文献标志码:AHSMOTE-AdaBoost:改进混合边界重采样集成分类算法李㊀静,刘㊀姜,倪㊀枫,李笑语(上海理工大学管理学院,上海200093)摘㊀要:处理类不平衡问题时,已有的采样方法存在易受噪声影响和忽略边界样本的问题,尤其是忽略多数类样本的类内差异,位于边界的样本实例非常容易被错分,而这些样本对划分决策边界具有重要作用㊂将SMOTE过采样和RUS随机欠采样方法结合并进行改进,提出混合边界重采样算法(HSMOTE-AdaBoost)㊂HSMOTE-AdaBoost算法首先对少数类运用SMOTE过采样,提高数据的平衡度;再使用K近邻算法清除噪声和采样方法产生的重叠实例;同时,基于与少数类样本的平均欧氏距离识别并保留边界多数类样本,然后对剩余的数据进行随机欠采样;最后,利用AdaBoost算法的优势,对平衡后的数据集进行多次迭代训练得到最终的分类模型㊂仿真实验结果表明,与传统的SMOTE-Boost㊁RUS-Boost㊁PC-Boost及改进后的算法KSMOTE-AdaBoost相比,该分类模型在不平衡数据集上的所有性能指标F-measure,G-mean,AUC值分别最高提升了22.97%,13.88%和10.03%,具有更优的分类效果㊂关键词:类不平衡;SMOTE过采样;AdaBoost算法;噪声样本;边界样本HSMOTE-AdaBoost:AnintegratedclassificationalgorithmbasedonimprovedmixedboundaryresamplingLIJing,LIUJiang,NIFeng,LIXiaoyu(BusinessSchool,UniversityofShanghaiforScienceandTechnology,Shanghai200093,China)ʌAbstractɔWhendealingwiththeclassimbalanceproblem,existingsamplingmethodshavetheissuesofbeingsusceptibletonoiseandignoringboundarysamples,especiallymajorityclassboundarysamples,makingtheboundarysampleinstances,whichplayanimportantroleindeterminingthedecisionboundary,easilybemisclassified.ByimprovingthecombinationofSMOTEoversamplingandrandomundersampling(RUS),ahybridboundaryresamplingalgorithm(HSMOTE-AdaBoost)isproposed.TheHSMOTE-AdaBoostalgorithmfirstlyperformsSMOTEoversamplingontheminoritysamplestoimprovedatabalanceandeffectiveness.Then,thepaperusestheKnearestneighboralgorithmtoremovenoiseandoverlappinginstancesgeneratedbythesamplingmethod.Meanwhile,thepaperrecognizesandretainstheboundarymajorityclasssamplesbasedontheaverageEuclideandistancetotheminoritysamples.Afterthat,theremainingdataisrandomlyundersampled.Finally,bymakinguseoftheadvantagesofAdaBoostalgorithm,thebalanceddatasetistrainedformultipleiterationstoobtainthefinalclassificationmodel.Theexperimentalresultsshowthat,comparingwiththetraditionalSMOTE-Boost,RUS-Boost,PC-BoostandtheimprovedalgorithmKSMOTE-AdaBoost,theincreaseoftheF-measure,G-meanandAUCvaluesofHSMOTE-AdaBoostcouldreach22.97%,13.88%and10.03%respectively,implyingabetterperformanceofHSMOTE-AdaBoost.ʌKeywordsɔclassimbalance;SMOTEoversampling;AdaBoostalgorithm;noisesample;boundarysample基金项目:国家自然科学基金(11701370);上海市 系统科学 高峰学科建设项目㊂作者简介:李㊀静(1998-),女,硕士研究生,主要研究方向:机器学习㊁数据挖掘;刘㊀姜(1983-),女,博士,副教授,硕士生导师,主要研究方向:符号计算㊁机器学习;倪㊀枫(1982-),男,博士,副教授,硕士生导师,主要研究方向:系统分析与集成;李笑语(2000-),女,本科生,主要研究方向:机器学习㊂通讯作者:刘㊀姜㊀㊀Email:jliu113@126.com收稿日期:2022-07-270㊀引㊀言在实际生活中,大部分的数据集都是不平衡的,即某些类会比其他类具有更多的实例,在这种情况下少数类的信息得不到充分的利用[1]㊂类不平衡问题给标准的分类学习算法带来了巨大的挑战,在不平衡的数据集上,大多数分类器倾向于对少数实例进行错误分类,而不是对多数实例进行错误分类[2-4]㊂但在现实生活中,少数类的错分代价会远高于多数类㊂类不平衡问题普遍存在于许多领域,Copyright ©博看网. All Rights Reserved.如网络入侵检测[5]㊁情感分析[6-7]㊁欺诈检测[8-9]㊁医疗疾病诊断检测[10]和故障诊断[11]等领域㊂大多数标准分类算法往往表现出对多数类的偏倚,因此类的不平衡性往往会损害标准分类器的性能,尤其是对少数类的分类性能[12-13]㊂目前,对于不平衡数据集分类问题的解决方案可以分为:数据级㊁算法级和集成层面[14-15]㊂其中,数据级解决方案包括许多不同形式的重采样技术,如随机过采样(ROS)和随机欠采样(RUS)[16-17]㊂其中,具有代表性的有Chawla等学者[18]提出了一新型过采样方法,SMOTE在少数类样本与其k近邻的连线上随机生成新的样本㊂He等学者[19]开发了一种自适应合成采样(ADASYN),使用密度分布来计算出每个少数样本需要合成的新样本数量㊂此外,还有几种混合的采样技术,其中一些方法就是将过采样与数据清理技术相结合,以减少过采样方法引入的重叠㊂典型的例子是SMOTE-Tomek[20]和SMOTE-ENN[21]㊂这些数据级方法虽然简单易用,但采样方法难以修改数据的分布和偏好相关联,一方面,欠采样会使数据集中一些有价值的数据信息未能得到利用;另一方面,过采样会生成多余数据,尤其是产生噪声数据㊂算法级的解决方案旨在开发新的算法或修改现有的算法,加强对少数类分类算法的学习㊂研究中,最受欢迎的分支是代价敏感学习,例如,Ting[22]提出了一种实例加权方法来裁剪代价敏感树以提高不平衡数据集的分类效果㊂Zhang等学者[23]通过对目标函数中的多数类和少数类设置不同的代价,提出了一种代价敏感神经网络算法,使得少数类样本尽可能地被识别㊂其他基于原有算法的改进方案通常是对现有分类算法的改进,文献[24-26]通过引入权重参数来调整分类决策函数,使其向少数类样本偏倚㊂然而算法的应用具有特定的情形,大多数的分类模型一旦被确定,则不会动态地调整相应的模型结构及其参数,使得这些算法级改进方法无法动态地学习新增的样本㊂集成层面解决方案就是将多个弱分类模型通过一定方式进行组合,得到一个新的㊁泛化性能更好的强分类器㊂Freund等学者[27]提出的自适应增强(AdaptiveBoosting,AdaBoost)算法是Boosting族中的代表算法㊂与其他分类器相比,AdaBoost能够有效地避免过拟合㊂文献[28-29]分别将AdaBoost与过采样和欠采样结合,提出SMOTEBoost和RUSBoost算法㊂SMOTEBoost运用SMOTE进行少数类样本的合成,经过多次迭代得到最终的强分类器㊂但SMOTEBoost算法在训练过程中生成的噪声数据会对分类性能产生影响㊂RUSBoost则采用欠采样方法,先随机删除一些多数类样本,随后使用删除后的数据构造弱分类器㊂文献[30]提出一种新的学习算法PCBoost,该算法考虑了属性特征,对少数类进行随机过采样,接着使用信息增益率来构造弱分类器,训练中错误分类的样本会被删除㊂上述研究中,虽然有不少基于采样方法与AdaBoost进行结合,但在采样方法上还需做进一步探讨㊂由于集成学习在分类性能上的优越性以及过采样方法使用的普遍性,本文重点关注采样方法与集成学习结合构建分类器㊂现有的过采样方法容易引入影响分类性能的噪声样本,而欠采样则会丢失有用的多数类样本的特征信息,尤其是位于分类边界的多数类样本㊂针对以上数据级改进方案中采样方法存在易受噪声影响和丢失多数类样本特征信息的问题,本文考虑了多数类样本的类内差异,并保留边界多数类样本;对少数类和多数类采取融合SMOTE过采样和RUS欠采样的混合采样策略,并结合集成技术AdaBoost,提出了HSMOTE-AdaBoost算法㊂首先,针对少数类样本中数据缺少和噪声干扰的问题,引入经典的SMOTE采样算法和K近邻噪声清除算法㊂其次,由于现有的大多数算法尚未考虑到多数类样本之间的类内差异,本文基于平均欧式距离识别并保留边界多数类样本,再对剩下的样本进行随机欠采样㊂最后,运用以J48为基分类器的AdaBoost算法进行分类㊂结果表明,本文所提出的HSMOTE-AdaBoost算法与传统的SMOTE-Boost,RUS-Boost,PC-Boost算法及改进后的算法KSMOTE-AdaBoost[31]相比,具有更好的分类效果㊂1㊀理论基础1.1㊀SMOTE算法Chawla等学者[18]于2002年提出了少数类样本合成技术,即SMOTE㊂该算法的流程步骤如下:原始训练样本为Tra,少数类样本是P,多数类样本是NP={p1,p2, ,ppnum},N={n1,n2, ,nnmum},这里pnum,nmum分别表示少数类样本个数和多数类样本个数㊂(1)计算少数类中的每个样本点pi与所有的少数类样本P的欧式距离,得到样本点的k近邻㊂(2)依据样本的采样倍率U,在k近邻之间进行线性插值㊂8智㊀能㊀计㊀算㊀机㊀与㊀应㊀用㊀㊀㊀㊀㊀㊀㊀㊀㊀㊀㊀㊀㊀㊀第13卷㊀Copyright©博看网. All Rights Reserved.(3)合成新的少数类样本:syntheticj=pi+rjˑdj(1)㊀㊀其中,dj是少数类样本点与其近邻的距离;rj是介于0到1之间的随机数㊂(4)新合成的少数类样本与原始训练样本Tra进行合并,得到新的训练样本㊂1.2㊀AdaBoost算法AdaBoost算法是经典的Boosting算法,通过迭代的方式不断提高被误判样本的权值,从而进行样本权值的更新,分类器在下一次分类会把重心放在那些被误分的样本上,以此来达到正确分类所有样本的目的㊂算法的训练过程如下:输入㊀Tra={(X1,Y1),(X2,Y2), ,(Xn,Yn)}为训练数据集,Xi表示样本实例,Yi为类标签集合,Yiɪ{+1,-1},i=1,2, ,n;迭代循环次数为M输出㊀最终分类器G(X)1:训练数据的权值分布初始化:D1=(w1,1, ,w1,i, ,w1,n),w1,i=1n,i=1,2, ,n2:form=1toM:2.1:使用具有权值分布Dm的训练数据集进行学习,得到基本分类器Gm(X)2.2:算出Gm(X)在训练数据集上的分类误差率:em=ðni=1wmiI(Gm(Xi)ʂYi)(2)㊀㊀这里,I为指示函数:2.3:计算Gm(X)的系数:αm=12ln1-emem(3)㊀㊀2.4:更新训练数据集的权值分布:Dm+1=(wm+1,1, ,wm+1,i, ,wm+1,n)(4)wm+1,i=wm,iZmexp(-αmYiGm(Xi))(5)㊀㊀其中,Zm为规范化因子,计算公式为:Zm=ðni=1wm,iexp(-αmYiGm(Xi))(6)㊀㊀3:构建基本分类器的线性组合:f(X)=ðMm=1αmGm(X)(7)㊀㊀4:得到最终的分类器:G(X)=sign(ðMm=1αmGm(X))(8)2㊀HSMOTE-AdaBoost算法针对不平衡数据的二分类问题,过采样会增加多余数据引入噪声样本,而欠采样会丢失一些有用信息,这2个问题极大地影响了算法的分类性能㊂HSMOTE-AdaBoost算法考虑了边界多数类样本特征信息的价值,将SMOTE算法和RUS算法进行了结合,并针对RUS算法中存在随机删除边界多数类样本的问题进行了改进㊂位于2个类边界上的实例是该分类样本的必要实例,在确定决策边界时至关重要,将其删除会降低分类器的性能㊂因此,本文提出的HSMOTE-AdaBoost算法考虑了边界多数类样本,并试图保持必要的多数类实例,分类模型图如图1所示㊂首先,使用SMOTE过采样合成少数类实例,以平衡数据集;接着,删除原始数据中的一些噪声数据,提高合成样本的质量;然后,识别多数类的边界实例,并将其添加到输出数据集中㊂同时,对剩余的数据进行随机欠采样㊂最后,使用AdaBoost集成算法生成强分类器,实验结果表明该分类器的性能得到了有效的提升㊂2.1㊀算法HSMOTE-AdaBoost的训练过程(1)合成少数类样本㊂将合成少数类过采样(SMOTE)预处理技术应用到数据集Timbal中,在少数类中引入人工实例,生成数据集Tɵimbal㊂具体步骤详述如下㊂①原始训练样本是Timbal,少数类样本为P,多数类是N,P=(p1,p2, ,pn),N=(n1,n2, ,nm),n,m分别表示少数类样本个数及多数类样本个数㊂计算少数类样本点pj与少数类P的欧式距离,得到该样本点的k近邻㊂②依据采样倍率U在k近邻中进行线性插值㊂③合成新的少数类样本:synthetic=pj+riˑdi(9)㊀㊀其中,di表示少数类样本点与其近邻的距离,ri是介于0到1之间的随机数㊂㊀㊀④新合成的少数类样本和原始训练样本进行合并,从而得到新的训练样本Tɵimbal㊂(2)识别和删除噪声㊂若少数类样本点在k近邻中有kᶄ个多数类样本,显然0ɤkᶄɤk,若kᶄɤ23k,则为噪声㊂(3)识别多数类的边界样本Nborder,并将其放入输出数据集Tbal中㊂具体步骤分述如下㊂①算出各多数类样本点ni(i=1,2, ,m)到少数类样本点pj(j=1,2, ,n)的距离之和为ðnj=1d(ni,pj)㊂9第7期李静,等:HSMOTE-AdaBoost:改进混合边界重采样集成分类算法Copyright ©博看网. All Rights Reserved.边界多数类样本非边界多数类样本随机欠抽样S M O T E 过采样噪声处理少数类集多数类集训练集原始数据集测试集分类更新样本权值重复T 次计算分类器的权重值最终分类器基分类器平衡数据集图1㊀分类模型图Fig.1㊀Classificationmodeldiagram㊀㊀②求平均距离:d-=ðmi=1ðnj=1d(ni,pj)m(10)㊀㊀③当多数类样本ni满足ðnj=1d(ni,pj)<d-时,将其划分到Tbal中㊂(4)对数据集E应用随机欠采样,将随机选取的样本推送到输出数据集Tbal,并推得:E=Tɵimbal-Nborder(11)㊀㊀(5)将处理后的平衡数据集作为模型的输入,使用AdaBoost集成技术得到最终的分类结果㊂2.2㊀算法HSMOTE-AdaBoost的详细步骤输入㊀D为数据集,K为过采样算法中选择的最近邻个数,M为迭代的次数输出㊀集成分类器1:将数据集D分成10份,其中2份为测试集TestData,剩下即训练集TrainData2:运用SMOTE过采样算法生成新的少数类样本3:用k近邻算法得到少数类样本的最近邻集合,依据判别条件对噪声样本进行识别及删除㊂判别条件为:若少数类样本中k近邻超过2/3的样本是多数类,则为噪声样本4:基于所有多数类样本点对各个少数类样本点的平均欧式距离识别边界多数类样本,并将其单独放在NewTrainData中㊂识别条件为:若该多数类样本点到所有少数类样本点的欧式距离之和小于平均距离,则该样本点为边界多数类样本点5:对剩余的数据进行随机欠抽样得到NewTrainData6:赋予NewTrainData中每个实例相同的权重㊂7:form=1toM8:㊀㊀根据实例的权值有放回地抽样得到Dm9:㊀㊀由Dm得到基分类器Gm(X)10:㊀㊀使用Gm(X)对NewTrainData中的实例进行分类,根据式(12)计算Gm(X)的错误率em:em=ðGm(Xi)ʂYiwm,i(12)㊀㊀其中,wm,i表示第m轮中第i个实例Xi的权值;Gm(Xi)ʂYi表示实例Xi被错分;Yi为类标签㊂11:㊀㊀ifem>0.5then转步骤712:㊀㊀endif13:㊀㊀for对正确分类的每个实例执行如下步骤:14:㊀㊀更新权值:实例的权值乘以em(1-em)15:㊀㊀归一化所有实例的权值16:㊀㊀endfor17:㊀㊀㊀将每个类的权值赋予0,对TestData的所有实例执行以下步骤18:㊀㊀fori=1toM:19:㊀㊀㊀根据式(13)计算Gi(X)对测试集中实例Xi的权值Wi:Wi=-logei1-ei(13)㊀㊀20:㊀㊀㊀㊀将Wi加到Gi(X)所预测的类上,若Xi被正确分类,则其值为0,否则为1㊂21:㊀㊀endfor22:㊀㊀返回权值最大的类,即为Xi的类别23:endfor01智㊀能㊀计㊀算㊀机㊀与㊀应㊀用㊀㊀㊀㊀㊀㊀㊀㊀㊀㊀㊀㊀㊀㊀第13卷㊀Copyright ©博看网. All Rights Reserved.3㊀仿真实验3.1㊀实验数据集实验使用来自KEEL公开数据集的6组数据集,考虑了数据集的样本数㊁特征数及不平衡率㊂实验之前对数据集进行预处理,将训练集和测试集归一化到[0,1]区间,表1展示了实验数据集的基本信息㊂表1㊀实验数据集Tab.1㊀Experimentaldataset序号数据集样本总数正类样本数负类样本数特征数不平衡率1ecoli23365228475.462yeast11484429105582.463glass12147613891.824glass62142918596.385ecoli33363530178.606ecoli13367725973.363.2㊀性能评价指标在对数据集进行二分类时,不能简单地采用整体精确度的高低来评价分类器性能的优劣㊂因为即使分类器对少数类样本的识别完全错误,总体的精确度也会比较高,所以仅靠这个单一评价指标并没有参考价值㊂为了全面体现分类性能,本文采用了综合评价指标F-measure,G-mean和AUC,F-measure,G-mean和AUC取值均在0到1之间,且值越大,说明分类器的分类性能越好㊂在介绍这些指标前,先引入混淆矩阵㊂混淆矩阵[32]可以将预测分类结果和实际分类结果以矩阵的形式直观地表示出来㊂混淆矩阵见表2㊂表2㊀混淆矩阵Tab.2㊀Confusionmatrix预测为正类预测为负类实际为正类真正类(TP)假负类(FN)实际为负类假正类(FP)真负类(TN)㊀㊀根据表2可得到以下评价指标㊂(1)召回率(Recall)㊂指真少数类占所有少数类的比例㊂可由如下公式来求值:Recall=TP/(TP+FN)(14)㊀㊀(2)精确率(Precision)㊂指真少数类占所有被预测为少数类的比例㊂可由如下公式来求值:Precision=TP/(TP+FP)(15)㊀㊀(3)F-measure[33],又称F-Score,兼顾精确度和召回率㊂可由如下公式来求值:F-measure=α2+1()Recall∗Precisionα2Recall+Precision(16)㊀㊀其中,α是取值为1的比例系数㊂(4)G-mean[34]㊂是一种有效衡量不平衡数据分类方法的指标,只有正类㊁负类两者召回率都高时,G-mean值才会高,表明分类器的分类性能较优㊂可由如下公式来求值:G-mean=TPTP+FNˑTNTN+FP(17)㊀㊀(5)AUC[35]㊂ROC曲线以假正率(FPrate)和真正率(TPrate)为轴,以可视化的方式展现正确分类的收益和误分类的代价之间的关联㊂ROC曲线下方的面积称为AUC(AreaUnderCurve),AUC可以量化分类器的预测性能,AUC的取值范围为0到1,AUC值越高,模型的预测性能越好㊂3.3㊀实验设置为了验证本文所提出的算法的优越性,将本文算法与传统的SMOTE-Boost,RUS-Boost,PC-Boost及改进后的KSMOTE-AdaBoost算法进行了比较㊂本文使用Python语言,Spyder开发环境对各种算法进行仿真实验㊂与以往文献[36]一致,SMOTE算法的k近邻数设为5时所合成的少数类样本质量较高㊂实验选用J48决策树作为基分类器,调用Weka软件中的C4.5函数包实现J48分类器㊂实验中将数据集的20%作为测试集,80%作为训练集,采用10次五折交叉验证的平均值作为最终的评价结果㊂3.4㊀实验结果分析图2 图4及表3 表5列出了使用本文算法和其他4种不同采样方法与集成学习技术结合算法在6个不同数据集上的F-measure㊁G-mean值及AUC的比较结果和具体数值,其中加粗部分为在该数据集上的最优结果㊂从图2 图4可以看出,由本文算法所得到的F-measure㊁G-mean及AUC值总体上优于其他4种对比算法㊂从表3 表5可以得出本文的分类模型在F-measure㊁G-mean及AUC值上均得到了提升,尤其是对比RUS-Boost算法㊂在数据集ecoli2㊁yeast1㊁glass6㊁ecoli3上,其分类评估指标均优于其他对比算法,F-measure的提升值高达22.97%,G-mean的提升值为13.88%,AUC的最高提升值为10.03%㊂从表3的F-measure值可看出,HSMOTE-AdaBoost算法除了在数据集ecoli1略低于SMOTE-Boost之外,在其他5个数据集上均有最佳表现㊂从表4 表5得出,在数据集glass1上,G-mean值稍逊于KSMOTE-AdaBoost算法,AUC指标略小于11第7期李静,等:HSMOTE-AdaBoost:改进混合边界重采样集成分类算法Copyright©博看网. All Rights Reserved.SMOTE-Boost算法,但其F-measure的提升值为3.99%㊂原因在于其不平衡比率较小,边界样本的重要性未得到充分体现㊂虽然在部分数据集中的AUC值与其他4种算法相差不大,但是随着不平衡率的提升,性能提升百分比在不断增大,在不平衡比率最大的数据集ecoli3上,相对于其他4种算法,性能提升了10.03%㊂此外,在部分数据集㊁如yeast1,ecoli2中,使用HSMOTE-AdaBoost算法优于RUS-Boost算法,这是因为在使用随机欠采样后有可能会删除一些潜在㊁有价值的多数类样本,而本文所提出的算法有效解决了这个问题,尽可能地保留了必要的实例从而提升了分类性能㊂S M O T E -B o o s t R U S -B o o s tP C -B o o s tK S M O T E -A d a B o o s tH S M O T E -A d a B o o s t1.00.90.80.70.6y e a s t 1g l a s s 1e c o l i 3g l a s s 6e c o l i 2e c o l i 1数据集F -m e a s u r e图2㊀不同算法的F-measure对比图Fig.2㊀F-measurecomparisonofdifferentalgorithmsS M O T E -B o o s t R U S -B o o s tP C -B o o s tK S M O T E -A d a B o o s t H S M O T E -A d a B o o s ty e a s t 1g l a s s 1e c o l i 3g l a s s 2e c o l i 1g l a s s 61.00.90.80.70.60.5G -m e a n数据集图3㊀不同算法的G-mean对比图Fig.3㊀G-meancomparisonofdifferentalgorithmsS M O T E -B o o s t R U S -B o o s t P C -B o o s tK S M O T E -A d a B o o s t H S M O T E -A d a B o o s tg l a s s 1y e a s t 1e c o l i 1g l a s s 6e c o l i 2e c o l i 31.00.90.80.70.6A U C数据集图4㊀不同算法的AUC对比图Fig.4㊀AUCcomparisonofdifferentalgorithms表3㊀不同算法的F-measureTab.3㊀F-measureofdifferentalgorithms数据集SMOTE-BoostRUS-BoostPC-BoostKSMOTE-AdaBoostHSMOTE-AdaBoostecoli20.9150.7530.9180.9210.926yeast10.6890.6030.6520.6390.714glass10.7510.6520.6890.7260.755glass60.9070.8790.9140.8850.922ecoli30.8460.8010.8870.8640.905ecoli10.9430.8990.9030.9070.928表4㊀不同算法的G-meanTab.4㊀G-meanofdifferentalgorithms数据集SMOTE-BoostRUS-BoostPC-BoostKSMOTE-AdaBoostHSMOTE-AdaBoostecoli20.8260.6750.8550.8820.901yeast10.6330.5780.6050.6780.689glass10.6540.6290.6890.7900.779glass60.9120.7650.9220.8900.931ecoli30.8740.7980.8540.8690.884ecoli10.9020.7890.8990.9050.91621智㊀能㊀计㊀算㊀机㊀与㊀应㊀用㊀㊀㊀㊀㊀㊀㊀㊀㊀㊀㊀㊀㊀㊀第13卷㊀Copyright ©博看网. All Rights Reserved.表5㊀不同算法的AUCTab.5㊀AUCofdifferentalgorithms数据集SMOTE-BoostRUS-BoostPC-BoostKSMOTE-AdaBoostHSMOTE-AdaBoostecoli20.9330.8560.9420.9410.951yeast10.7900.7520.7830.7920.798glass10.7590.6390.7450.7080.758glass60.9300.8950.8590.9320.946ecoli30.9120.8670.8760.9250.954ecoli10.9220.9070.9370.9340.9434㊀结束语针对二分类问题中不平衡数据集造成分类器分类性能低下的问题,本文考虑了多数类样本的类内差异,并保留了必要的边界多数类样本,将SMOTE过采样与随机欠采样组合运用及改进,并与AdaBoost相结合提出了一种解决类不平衡问题的HSMOTE-AdaBoost算法㊂与传统的SMOTE-Boost㊁RUS-Boost㊁PC-Boost及改进后的算法KSMOTE-AdaBoost相比,实验表明该算法训练的分类器能有效地处理类不平衡问题,分类性能更优㊂此外,本文的改进算法也存在进一步研究的价值,接下来可以结合其他经典的集成算法研究其分类性能㊂其次,从实验结果可以发现,在类不平衡比率越大的数据集上的分类效果越好,亟需从理论上对该结果做进一步的探讨和验证㊂最后,本文所提出的算法对二分类问题的分类性能提升比较明显,然而在现实生活中的大多数数据都是多类别的,未来将着重研究如何提高多类别分类问题的分类性能㊂参考文献[1]WUJianxin,BRUBAKERSC,MULLINMD,etal.Fastasymmetriclearningforcascadefacedetection[J].IEEETransactionsonPatternAnalysisandMachineIntelligence,2008,30(3):369-382.[2]GUOHaixiang,LIYijing,JENNIFERS,etal.Learningfromclass-imbalanceddata:Reviewofmethodsandapplications[J].ExpertSystemswithApplications,2017,73:220-239.[3]JOHNSONJM,KHOSHGOFTAARTM.Surveyondeeplearningwithclassimbalance[J].JournalofBigData,2019,6(1):27.[4]S EZJA,LUENGOJ,STEFANOWSKIJ.SMOTE-IPF:Addressingthenoisyandborderlineexamplesprobleminimbalancedclassificationbyare-samplingmethodwithfiltering[J].InformationSciences:AnInternationalJournal,2015,291:184-203.[5]ZHOUChenfeng,LECKIEC,KARUNASEKERAS.Asurveyofcoordinatedattacksandcollaborativeintrusiondetection[J].Computers&Security,2009,29(1):124-140.[6]NIRO,GILADK,BRACHAS,etal.Sentimentanalysisintranscribedutterances[M]//CAOT,LIME,ZHOUZH,Ho,etal.AdvancesinKnowledgeDiscoveryandDataMining.PAKDD2015.LectureNotesinComputerScience().Cham:Springer,2015,9078:27-38.[7]GOPALAKRISHNANV,RAMASWAMYC.Sentimentlearningfromimbalanceddataset:Anensemblebasedmethod[J].InternationalJournalofArtificialIntelligence,2014,12(2):75-87.[8]SIDNEYT,KOHYS,GILLIAND.Detectingonlineauctionshillingfraudsusingsupervisedlearning[J].ExpertSystemswithApplications,2014,41(6):3027-3040.[9]WEIWei,LIJinjiu,CAOLongbing,etal.Effectivedetectionofsophisticatedonlinebankingfraudonextremelyimbalanceddata[J].WorldWideWeb,2013,16(4):449-475.[10]AKRAMV,SAEEDJ.C-PUGP:Acluster-basedpositiveunlabeledlearningmethodfordiseasegenepredictionandprioritization[J].ComputationalBiologyandChemistry,2018,76:23-31.[11]KIMJH.Timefrequencyimageandartificialneuralnetworkbasedclassificationofimpactnoiseformachinefaultdiagnosis[J].InternationalJournalofPrecisionEngineeringandManufacturing,2018,19(6):821-827.[12]JLA,QZA,QWA,etal.AnoveloversamplingtechniqueforclassimbalancedlearningbasedonSMOTEandnaturalneighbors[J].InformationSciences,2021,565:438-455.[13]YANYuanting,LIURuiqing,DINGZihan,etal.AParameter-freeCleaningMethodforSMOTEinImbalancedClassification[J].IEEEAccess,2019:23537-23548.[14]PAULAB,LUIST,RIBEIROR.Asurveyofpredictivemodelingonimbalanceddomains[J].ACMComputingSurveys,2016,49(2):351-400.[15]李艳霞,柴毅,胡友强,等.不平衡数据分类方法综述[J].控制与决策,2019,34(04):673-688.[16]孟东霞,李玉鑑.基于特征边界欠采样的不平衡数据处理方法[J].统计与决策,2021,37(11):30-33.[17]AREFEENMA,NIMIST,RAHMANMS.NeuralNetwork-BasedUndersamplingTechniques[J].arXivpreprintarXiv:1908.16487,2019.[18]CHAWLANV,BOWYERKW,HALLLO.SMOTE:Syntheticminorityover-samplingtechnique[J].JournalofArtificialIntelligenceResearch,2002,16:1076-9757.[19]HEHaibo,YANGBai,GARCIAEA.ADASYN:adaptivesyntheticsamplingapproachforimbalancedlearning[J].ProceedingsofIEEEInternationalJointConferenceonNeural31第7期李静,等:HSMOTE-AdaBoost:改进混合边界重采样集成分类算法Copyright ©博看网. All Rights Reserved.Networks,2008,12(5):1322-1328.[20]KANGQi,CHENXiaoshuang,LISisi,etal.ANoise-filteredunder-samplingschemeforimbalancedclassification[J].IEEETransactionsonCybernetics,2017,47(12):4263-4274.[21]JIANGKun,JINGLu,XIAKuiliang.AnovelalgorithmforimbalancedataclassificationbasedongeneticalgorithmimprovedSMOTE[J].ArabianJournalforScience&Engineering,2016,41(8):3255-3266.[22]TINGKM.Aninstance-weightingmethodtoinducecost-sensitivetrees[J].IEEETransactionsonKnowledgeandDataEngineering,2002,14(3):659-665.[23]ZHANGChong,TANKC,LIHaizhou,etal.Acost-sensitivedeepbeliefnetworkforimbalancedclassification[J].IEEETransactionsonNeuralNetworksandLearningSystems,2018,30(1):109-122.[24]IMAMT,TINGKM,KAMRUZZAMANJ.Z-SVM:Ansvmforimprovedclassificationofimbalanceddata[M]//SATTARA,KANGBH.AdvancesinArtificialIntelligence.AI2006.LectureNotesinComputerScience().Berlin/Heidelberg:Springer,2006,4304:264-273.[25]王伟,薛安荣,刘峰.改进的SVM解决背景知识数据中的类不平衡[J].计算机应用研究,2011,28(08):2902-2904,2908.[26]杨扬,李善平.基于实例重要性的SVM解不平衡数据分类[J].模式识别与人工智能,2009,22(06):913-918.[27]FREUNDY,SCHAPIRERE.Adecision-theoreticgeneralizationofon-linelearningandanapplicationtoBoosting[J].JournalofComputerandSystemSciences,1997,55(1):119-139.[28]CHAWLANV,LAZAREVICA,HALLLO,etal.SMOTEBoost:improvingpredictionoftheminorityclassinboosting[J].LectureNotesinComputerScience,2003,2838(1):107-119.[29]CHRISS,KHOAHFOTAARTM,JASONVH,etal.RUSBoost:Ahybridapproachtoalleviatingclassimbalance[J].IEEETransactionsonSystems,Man,andCybernetics,PartA:SystemsandHumans,2010,40(1):185-197.[30]LIUCL,HSIEHPY.Model-basedsyntheticsamplingforimbalanceddata[J].IEEETransactionsonKnowledgeandDa-taEngineering,2020,32(8):1543-1556.[31]王忠震,黄勃,方志军,等.改进SMOTE的不平衡数据集成分类算法[J].计算机应用,2019,39(09):2591-2596.[32]DENGXinyang,LIUQi,DENGYong,etal.Animprovedmethodtoconstructbasicprobabilityassignmentbasedontheconfusionmatrixforclassificationproblem[J].InformationSciences,2016,340:250-261.[33]LIPTONZC,ELKANC,NARYANASWAMYB.OptimalthresholdingofclassifierstomaximizeF1measure[J].MachineLearningKnowledgeDiscoveryDatabases,2014,8725:225-239.[34]KUBATM,HOLTER,MATWINS.Learningwhennegativeexamplesabound[J].LectureNotesinComputerScience,1997,1224(1):146-153.[35]FLACHPA.ThegeometryofROCspace:UnderstandingmachinelearningmetricsthroughROCisometrics[C]//ProceedingsoftheTwentiethInternationalConferenceonInternationalConferenceonMachineLearning(ICMLᶄ03).Washington,DCUSA:ACM,2003:194-201.[36]石洪波,陈雨文,陈鑫.SMOTE过采样及其改进算法研究综述[J].智能系统学报,2019,14(06):1073-1083.(上接第6页)参考文献[1]MIKOLOVT,CHENKai,CORRADOG,etal.Efficientestimationofwordrepresentationsinvectorspace[C]//Proceedingsoft-heInternationalConferenceonLearningRepresentations.Scottsdale,USA:UMASS,2013:1-12.[2]PENNINGTONJ,SOCHERR,MANNINGC,etal.Glove:globalvectorsforwordrepresentation[C]//Proceedingsofthe2014ConferenceofEmpiricalMethodsinNaturalLanguageProcessing.Stroudsburg,PA:ACL,2014:1532-1543.[3]袁磊.基于改进CHI特征选择的情感文本分类研究[J].传感器与微系统,2017,36(05):47-51.[4]宋呈祥,陈秀宏,牛强.文本分类中基于CHI改进的特征选择方法[J].传感器与微系统,2019,38(02):37-40.[5]DEVLINJ,CHANGMW,LEEK,etal.BERT:pre-trainingofdeepbidirectionaltransformersforlanguageunderstanding[C]//Proceedingsofthe2019ConferenceoftheNorthAmericanChapteroftheAssociationforComputationalLinguistics:HumanLanguageTechnologies.Minneapolis,Minnesota:ACL,2019:4171-4186.[6]YANGZhilin,DAIZihang,YANGYiming,etal.XLNet:Generalizedautoregressivepretrainingforlanguageunderstanding[C]//NeuralInformationProcessingSystems.Canada:NIPSFoundation,2019:5754-5764.[7]ADHIKARIA,RAMA,TANGR,etal.Rethinkingcomplexneuralnetworkarchitecturesfordocumentclassification[C]//ProceedingsoftheConferenceoftheNorthAmericanChapteroftheAssociationforComputationalLinguistics:HumanLanguageTechnologies.Minneapolis,Minnesota:ACL,2019:4046-4051.[8]陈立潮,秦杰,陆望东,等.自注意力机制的短文本分类方法[J].计算机工程与设计,2022,43(03):728-734.[9]杨青,张亚文,朱丽,等.基于注意力机制和BiGRU融合的文本情感分析[J].计算机科学,2021,48(11):307-311.[10]孙红,陈强越.融合BERT词嵌入和注意力机制的中文文本分类[J].小型微型计算机系统,2022,43(01):22-26.[11]梁淑蓉,谢晓兰,陈基漓,等.基于XLNet的情感分析模型[J].科学技术与工程,2021,21(17):7200-7207.[12]搜狗实验室.搜狐新闻数据(SogouCS)[EB/OL].[2020-01-13].http://www.sogou.com/labs/resource/cs.php.[13]CUIYiming,CHEWanxiang,LIUTing,etal.Revisitingpre-trainedmodelsforchinesenaturalla-nguageprocessing[EB/OL].[2020].http://arxiv.org/abs/2004.13922.[14]陶志勇,李小兵,刘影,等.基于双向长短时记忆网络的改进注意力短文本分类方法[J].数据分析与知识发现,2019,3(12):21-29.[15]滕金保,孔韦韦,田乔鑫,等.基于CNN和LSTM的多通道注意力机制文本分类模型[J].计算机工程与应用,2021,57(23):154-162.41智㊀能㊀计㊀算㊀机㊀与㊀应㊀用㊀㊀㊀㊀㊀㊀㊀㊀㊀㊀㊀㊀㊀㊀第13卷㊀Copyright©博看网. All Rights Reserved.。
光触发自愈和形状记忆聚合物
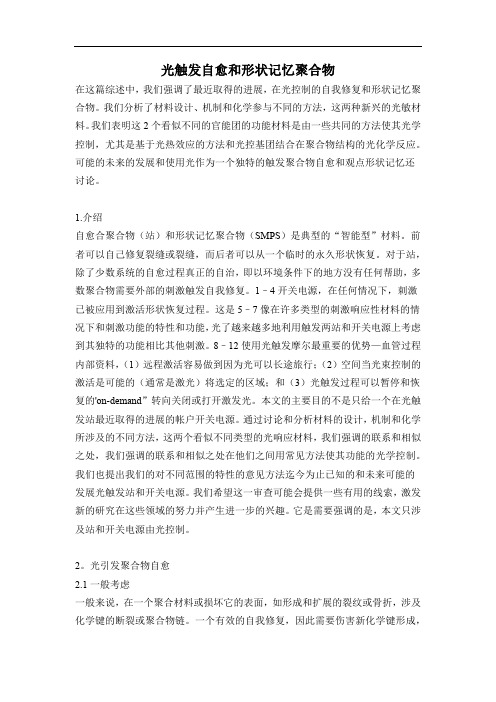
光触发自愈和形状记忆聚合物在这篇综述中,我们强调了最近取得的进展,在光控制的自我修复和形状记忆聚合物。
我们分析了材料设计、机制和化学参与不同的方法,这两种新兴的光敏材料。
我们表明这2个看似不同的官能团的功能材料是由一些共同的方法使其光学控制,尤其是基于光热效应的方法和光控基团结合在聚合物结构的光化学反应。
可能的未来的发展和使用光作为一个独特的触发聚合物自愈和观点形状记忆还讨论。
1.介绍自愈合聚合物(站)和形状记忆聚合物(SMPS)是典型的“智能型”材料。
前者可以自己修复裂缝或裂缝,而后者可以从一个临时的永久形状恢复。
对于站,除了少数系统的自愈过程真正的自治,即以环境条件下的地方没有任何帮助,多数聚合物需要外部的刺激触发自我修复。
1–4开关电源,在任何情况下,刺激已被应用到激活形状恢复过程。
这是5–7像在许多类型的刺激响应性材料的情况下和刺激功能的特性和功能,光了越来越多地利用触发两站和开关电源上考虑到其独特的功能相比其他刺激。
8–12使用光触发摩尔最重要的优势—血管过程内部资料,(1)远程激活容易做到因为光可以长途旅行;(2)空间当光束控制的激活是可能的(通常是激光)将选定的区域;和(3)光触发过程可以暂停和恢复的'on-demand”转向关闭或打开激发光。
本文的主要目的不是只给一个在光触发站最近取得的进展的帐户开关电源。
通过讨论和分析材料的设计,机制和化学所涉及的不同方法,这两个看似不同类型的光响应材料,我们强调的联系和相似之处,我们强调的联系和相似之处在他们之间用常见方法使其功能的光学控制。
我们也提出我们的对不同范围的特性的意见方法迄今为止已知的和未来可能的发展光触发站和开关电源。
我们希望这一审查可能会提供一些有用的线索,激发新的研究在这些领域的努力并产生进一步的兴趣。
它是需要强调的是,本文只涉及站和开关电源由光控制。
2。
光引发聚合物自愈2.1一般考虑一般来说,在一个聚合材料或损坏它的表面,如形成和扩展的裂纹或骨折,涉及化学键的断裂或聚合物链。
- 1、下载文档前请自行甄别文档内容的完整性,平台不提供额外的编辑、内容补充、找答案等附加服务。
- 2、"仅部分预览"的文档,不可在线预览部分如存在完整性等问题,可反馈申请退款(可完整预览的文档不适用该条件!)。
- 3、如文档侵犯您的权益,请联系客服反馈,我们会尽快为您处理(人工客服工作时间:9:00-18:30)。
Smart Aggregate-Based Damage Detection of Circular RC columnsunder Cyclic Combined LoadingYashar Moslehy1, Haichang Gu2, Abdeljalil Belarbi 1 , Y.L. Mo 1 and Gangbing Song2 1Department of Civil and Environmental Engineering2Department of Mechanical EngineeringUniversity of Houston4800 Calhoun, Houston, TX, 77004ABSTRACTStructural health monitoring is an important issue for the maintenance of large-scale civil infrastructure, especially for bridge columns. In this paper, an innovative piezoceramic-based approach is developed for the structural health monitoring of reinforced concrete columns. An innovative piezoceramic-based device, smart aggregate, is utilized as a transducer for health monitoring purposes. To investigate the seismic behavior of RC bridge columns, structural health monitoring tests were performed on two bridge columns under combined reversed cyclic loading at Missouri University of Science and Technology. Experimental results show that the proposed smart aggregate-based approach successfully evaluated the health status of concrete columns during the loading procedure.INTRODUCTIONConcrete structures are the most popular civil structures. Natural disasters, such as earthquakes, will cause severe damage to concrete structures. It is imperative to quickly assess the severity of the damage and health status of a concrete structure, especially for large-scale infrastructure, in real time or near real time after an earthquake to provide vital structural safety information for decision makers. It is desirable to have an automated and distributed system to perform structural health monitoring for large-scale infrastructure.In this paper, innovative piezoceramic-based devices, called smart aggregates, are used as transducers for structural health monitoring of reinforced concrete columns under a cyclic loading procedure. The proposed smart aggregates are low-cost, piezoceramic-based multi-functional devices, capable of performing comprehensive monitoring of concrete structures, including early-age strength monitoring (Gu et al. 2006), impact detection and evaluation (Song at al. 2007a), and structural health monitoring (Song at al. 2007b, Song et al. 2008). For structural health monitoring purposes, a smart aggregate-based active sensing system was developed. In the proposed active sensing system, one smart aggregate is used as an actuator to generate a sweep sine signal, while the other smart aggregates are used as sensors todetect the signal response. The propagation energy of the waves will be attenuated bycracks in the concrete structure. By analyzing the sensor signal, the health status ofthe concrete structure is evaluated. In the proposed health monitoring approach,wavelet packet analysis is used as a signal-processing tool. Based on the waveletpacket analysis results, a damage index was formed to evaluate the severity of thedamage. To extract the time-history and location information of damage, a Sensor-History Damage Index Matrix was developed.To verify the effectiveness of the proposed health monitoring approach, structuralhealth monitoring tests were performed on two reinforced concrete columns atMissouri University of Science and Technology (MUST). Reverse cyclic loadingprotocols were used to gradually damage the columns to failure while the proposedsmart aggregates were used as transducers to perform the structural healthmonitoring. 3-dimensional smart aggregates were also implemented to study theeffect of the orientation of the transducer in the health monitoring procedure.2. Test SetupIn this paper, the smart aggregates (SAs), shown in Figure 1, were formed byembedding a water-proof coated piezoceramic patch with lead wires into a smallconcrete block before casting. Three-dimensional smart aggregates, as shown inFigure 2, were also developed as transducers for health monitoring purpose. Tworeinforced concrete columns (columns A and B) were fabricated as the testing objectsat the Missouri University of Science and Technology (MUST). Eight smartaggregates and one 3-dimensional smart aggregate were installed at the pre-determined distributive locations in each column before casting. The location of smartaggregates in Column A is shown in Figure 3 and Figure 4. The locations of theembedded smart aggregates in column B is the same of those in column A, but of thedifferent labels. The labels of smart aggregates in columns A and B are shown inTable 1.Figure 1. Illustration of a smart aggregate3D-5Figure 2. Illustration of a three dimensional smart aggregate (NO.2)Water-proof coatingElectric wiresPiezoceramic patchFigure 3. Location of smart aggregates in column AFigure 4. Location of smart aggregates in column ATable 1 sensor labels for smart aggregates at the same distributed locations atcolumns A and B.Column A Column B Column A Column BSA-1 SA-9 SA-5 SA-13SA-2 SA-10 SA-6 SA-14SA-3 SA-11 SA-7 SA-15SA-4 SA-12 SA-8 SA-163D-1 3D-2TEST PROCEDUREThe reinforced concrete columns were gradually failed under combined reversed cyclic loading using the experimental setup shown in Figure 5. Reversed cyclic loading protocols as shown in Figure 6(a) and Figure 6(b), were applied on the concrete columns A and B, respectively, to gradually fail the concrete structures. The torsion/moment ratio for loading protocol of column A is 0.2; while torsion/moment ratio for loading protocol of column B is 0.6. Pictures of the damaged concrete beam A at ductility level 1 and 3 are shown in Figures 7and 8Error! Reference source not found., respectively. During the loading procedure, the proposed piezoceramic-based smart aggregates were used as transducers to perform health monitoring to evaluate the damage status of the reinforced concrete columns.Figure 5. Experimental Setup(a) Loading protocol for column A(b) Loading Protocol for column B Figure 6. Loading ProtocolFigure 7. Damage status at ductilitylevel 1 Figure 8. Damage status at ductility level 3PRINCIPLE OF THE PROPOSED SMART AGGREGATE-BASED STRUCTURAL HEALTH MONITORINGMotivationIn recent years, there has been an increasing demand for structural health monitoring of large-scale structures to reduce maintenance costs and to enhance safety. Traditional health monitoring methods (x-ray, C-scan, etc.) are expensive and sometimes ineffective for large-scale structures with limited or no accessibility. Piezoelectric transducers have emerged as new tools for the health monitoring of large-scale structures due to their advantages of active sensing, low cost, quick response, availability in different shapes, and simplicity of implementation. Literature reviewDamage detection and failure analysis of concrete structures have been studied for many years (Otani and Sozen 1972, Abrams and Sozen 1979, Saiidi et al. 1981, Kreger and Sozen 1983, Park et al. 1985, Hassan and Sozen 1997, Wang and Wen 2000, Nojavan and Yuan 2006). Various sensors and methods have been developed for damage detection and health monitoring. Fiber optical sensors (FOS), especially Fiber Bragg Grating (FBG) sensors, are now used for the health monitoring of various RC structures (Zhang et al. 2006, Ren et al. 2006, Lu and Xie 2006, Chen and Ansari, 2000). However, FOSs are fragile, expensive, and offer only local measurements, limiting their applications. Piezoelectric-based approaches have provided an innovative approach for the structural health monitoring of civil structures with the advantages of structural simplicity, low cost, quick response, and high reliability. In general, there are three major piezoelectric-based health monitoring approaches.The impedance-based health monitoring approach is a real-time, qualitative damage detection method (Hey et al. 2006, Naidu and Bhalla 2003, Tseng and Wang, 2004). The operating principle is based on the electromechanical coupling property of piezoelectric materials. The impedance-based approach has been applied to the health monitoring of trusses (Sun et al. 1995), aluminum specimens (Tseng and Naidu 2002), RC bridges (Soh et al. 2000), RC specimens (Bhalla and Soh 2004, Park et al. 2006), RC shear walls (Zhao and Li, 2006) and composite reinforced concrete/masonry walls (Raju et al. 1999). Normally, impedance-based approaches require a sampling rate of several hundred KHz, which is beyond the capacity of most microprocessors and wireless transmitters and may not be suitable for distributive deployment.The vibration-characteristic approach utilizes piezoelectric actuators to generate certain waves which propagate within the structure and compares the structural vibration-characteristic parameters (modal shape, model frequency) (Okafor et al.1996), vibration-characteristic response curves (sweep sine response (Song et al. 2007b), or transfer function (Saafi et al. 2001) with those of the healthy state to detect damage. Delamination between the reinforcing bar and concrete has also been studied using this approach (Miller et al. 2002, Na et al. 2003). This approach works with a wide spectrum of excitation frequencies, ranging from several hundred Hz to several KHz, and is adopted in this research with the help of innovative damage index matrices, to be defined later.The Lamb wave-based health monitoring approach is used with PZT (an acronym for Lead Zirconate Titanate, a commonly used piezoceramic material due to its strong piezoelectricity) patches either surface bonded to (Lim et al. 2006) or embedded in (Song et al. 2007b) the structure to detect structural damage by generating a Lamb wave and monitoring its reflections. However, this approach is not suitable for concrete structures, which have complex geometries and are usually not thin-shell structures.In this study, a piezoceramic-based vibration-characteristic approach was applied to perform the structural health monitoring of a reinforced concrete column under reversed cyclic loading. The wave response detected by the proposed piezoceramic-based smart aggregates was used to be analyzed to evaluate the health status of the concrete structure during the cyclic loading.PRINCIPLEIn this research, a smart aggregate-based active sensing system, as shown in Figure 9, was developed for the health monitoring of concrete columns. The piezoelectric transducer in one smart aggregate was used as an actuator to excite desired guided waves. The piezoelectric transducers in the other distributed smart aggregates were used as sensors to detect wave responses. Cracks or damage inside the concrete column acts as a stress relief in the wave propagation path. The amplitude of the wave and the transmission energy will decrease due to the existence of cracks. The value of the transmission energy drop will be correlated with the degree of the damage inside.Figure 7. Block diagram of piezoelectric-based active sensing systemIn the proposed structural health monitoring approach, wavelet packet analysis wasused as a signal-processing tool to analyze the sensor signal. A wavelet is a waveformof effectively limited duration that has an average value of zero. The advantage ofwavelet packet analysis is that it enables the inspection of relatively narrow frequencybands over a relatively short time window. In the proposed structural healthmonitoring algorithm, the sensor signal S is decomposed by an n -level wavelet packetdecomposition into 2n signal sets },,{221n X X X L . X j is expressed as],,[,2,1,m j j j j x x x X L =, (1)where m is the amount of sampling data. The energy of the decomposed signal isdefined as 2,22,21,22,m j j j j j i x x x X E +++==L , (2). where i is the time index and j is the frequency band index (j =1…2n ). The energyvector at time index i is defined as],,,[2,2,1,n i i i i E E E E L =. (3).Various kinds of damage indices have been developed for the health monitoring ofreinforced concrete structures in recent years. Root-mean-square deviation (RMSD) isa suitable damage index to compare the difference between the signatures of thehealthy state and the damaged state. Tseng and Naidu (2002) presented the damageindex by calculating the RMSD between the impedance of the PZT transducermounted on aluminum specimens. Soh et al. (2000) successfully conducted RMSDbetween PZT transducers signatures to form the damage index for health monitoringof a reinforced concrete (RC) bridge. In the proposed approach, a wavelet-baseddamage index is formed by calculating the Root Mean Square Deviation (RMSD)between the energy vectors of the healthy state and the damaged state. The energyvector for healthy state is defined as ],,,[2,2,1,n h h h h E E E E L =. The energy vectori E for the damaged state at time index i is defined as ],,,[2,2,1,n i i i i E E E E L =. Theproposed damage index at time i is defined as ∑∑==−=nn j j h j j h j i E E E I 212,212,,)(. (4) The proposed damage index represents the transmission energy loss portion causedby structural damage. When the damage index is close to 0, the concrete structure isin a healthy state. When the damage index is larger than a certain threshold value,damage has appeared in the concrete structure. In this case, the greater the index, themore serious the damage.To demonstrate the health status at different locations of the concrete structure, aSensor-History Damage Index Matrix (SHDIM) n m M ×(Song et al. 2008) is defined as[]n m j i n m I M ××=,, n j and m i L L ,1,1== (5)where the matrix element at the i th row and the j th column, j i I ,, is the damage indexof the i th smart aggregate at the time of the j th test (i.e. i is the sensor index, j is thetime index); m is the total number of smart aggregates and n is the total number oftests. The damage status at different locations of the concrete specimen at differenttest times can be described by a 3-dimensional damage index matrix plot. TheSHDIM is useful in monitoring the damage evolution process to predict the failure ofa structure.EXPERIMENTAL RESULTSExperimental Results of RC Column AA reversed cyclic loading protocol shown was implemented to gradually fail theconcrete column A. During the loading procedure, the proposed smart aggregate-based health monitoring approach was implemented at the positive loading peaks,zero loading points and negative loading peaks of the cyclic loading protocol asshown in Figure 10. From the ductility level of positive testing points shown inFigure 11, concrete column A yields at test point 16. From the actual ductility levelsshown in Table 2, the ductility value reaches 1 at fourth loading cycle and sixthloading cycle. This means that the concrete column yielded after fourth loading cycle and the damage status would be worse after fourth cycle.Figure 8 Loading protocol with health monitoring test pointsTable 2 Loading Protocol S. No Positive Cycles During Testing Actual DuctilityLevels-PositiveNegative Cycles During Testing Actual Ductility Levels-Negative 1 20% Vy 25% Vy20% Vy 25% Vy 2 40% Vy 50% Vy40% Vy 50% Vy 3 60% Vy 75% Vy60% Vy 75% Vy 4 80% Vy 100% Vy80% Vy 100% Vy 5 90% Vy 75% Vy90% Vy 75% Vy 6 100% Vy 100% Vy100% Vy 100% Vy 7 Ductility 1.5 Ductility 1.53Ductility 1.5 Ductility 1.53 8 Ductility 3.0 Ductility 3.2Ductility 3.0 Ductility 3.2 9 Ductility 4.5 Ductility 4.74Ductility 4.5 Ductility 4.74 10 Ductility 6.0 Ductility 6.20Ductility 6.0 Ductility 6.20 11 Ductility 8.0 Ductility 8.15Ductility 8.0 Ductility 8.15 12 Ductility 10.0 Ductility 10.2 Ductility 10.0 Ductility 10.2In the health monitoring test, PZT3 is used as an actuator to excite a sweep sine waveand other smart aggregates are used as sensors to detect the wave responses. Waveletpacket analysis is used to form a sensor history damage index matrix (SHDIM) toevaluate the damage status. From SHDIM results for positive loading peaks as shownin Figure 11, at point 10 (corresponding to the fourth loading cycle), there aredramatic increments in damage index values for different sensors; this indicates that asevere damage status occurred at this loading cycle. From the SHDIM results at zeroloading points as shown in Figure 12, damage index values reach to a critical valueafter point 11. From the SHDIM results at negative loading peaks as shown in Figure13, the damage index values reach to a critical value after point 12. Point 10, point 11and point 12 are the health monitoring testing points at fourth loading cycle and theSHDIM results demonstrate that the damage index values reach to a critical valueafter fourth loading cycle. Therefore it could be concluded that the concrete columnyield at point 10 of the fourth loading cycle.In addition to the detection of critical damage status, the proposed smart aggregateapproach is capable to extract the damage location information. In the SHDIM resultsfor positive loading, zero loading and negative loading, PZT 2 PZT4, PZT5 and PZT6in section A-A has greater damage index values than those of PZT7 in section B-Band PZT8 in section C-C. This means damage situation around in section A-A weremore severe that those of section B-B and C-C. Section A-A was located at the basearea of the column and has the greatest value of bending moment, thus the damagesituation at section A-A should be more severe than that of other locations whichfurther verifies the SHDIM results.D a m a g e i n d e x v a l u e Figure 9. Damage index matrix plot for positive loading peaksD a m a g e i n d e x v a l u eD a m a g e i n d e x v a l u eFigure 10. Damage index matrix plotfor zero loading points Figure 11. Damage index matrix plotfor negative loading peaksAttenuation of sensor energy is directly relative with the damage situation and the analysis of transmission energy attenuation could be used for the evaluation of the damages inside. Due to the mismatch of the acoustic impedance at the crack area, the crack area attenuates the transmission energy of the propagation waves during the loading procedure. The attenuated portion of transmission energy is proportion to the severity of the damages inside. As shown from the time response of PZT2 in Figure 14, the response amplitude greatly decreased during the reversed cyclic loading procedure. The sensor energy of PZT2 at positive, negative loading peaks and zero loading points as shown in Figure 15 further demonstrated that the existence of cracks block wave propagation and that sensor voltage could be used to extract damage information.To further demonstrate the sensor energy attenuation at different locations, a 3-D normalized sensor energy plot is drawn for each sensor. For comparison purpose, the maximum senor energy value is normalized to 1. Sensor energy plot for positive loading, zero loading and negative loading are shown in Figure 16, Figure 17 and Figure 18. From the normalized sensor energy plots, it can be seen that the sensor energy level dropped dramatically after the fourth cycle, which corresponds to points 10, 11 and 12. From the attenuation of the transmission energy, it can be derived that the concrete column yield around fourth loading cycle. The sensor energy results further verify the effectiveness of the wavelet packet-based SHDIM.Time (S)S e n s o r v o l t a g e (V )S e n s o r e n e r g yPoint numberFigure 12. Time response comparison of PZT2 (PZT3 is used as an actuator) Figure 13. Energy plot of PZT2 (PZT3is used as an actuator)N o r m a i l i z e d s e n s o r e n e r g yN o r m a i l i z e d s e n s o r e n e r g yFigure 14 Normalized sensor energyfor positive loadingFigure 15 Normalized sensor energyfor zero loadingN o r m a i l i z e d s e n s o r e n e r g yFigure 16 Normalized sensor energy for negative loadingIn addition to the ordinary smart aggregate, a 3-D smart aggregate with threepiezoceramic patches at different orientation were also implemented in the health monitoring test to study the effect of sensor orientation. From the normalized sensor energy shown in Figure 19, the sensor energy from three piezoceramic patches dropped dramatically after point 10 (fourth cyclic loading) and the attenuation portion was similar for these three piezoceramic patches. From the time responses shown in Figure 20, the second piezoceramic patch in the 3-D SA has much greater response amplitude than those of the other two piezoceramic patches. From these experimental results of 3-D SA, it can be concluded that the orientation of piezoceramic patch affects the wave response amplitude but it will not affect the attenuation portion of the transmission wave too much.Sensor numberN o r m a i l i z e d s e n s o r e n e r g yS e n s o r v o l t a g e (v )Time(s)Figure 17 Normalized sensor energy for positive loading of 3-D SA Figure 18 Time responses of three piezoceramic patches in the 3-D SAHealth Monitoring Results of RC Column BFor the health monitoring of column B, the developed smart aggregate-based health monitoring approach was performed at the end of each negative loading peak or positive loading peak in the reversed cyclic loading protocol. The health monitoring test number is marked the load-displacement curve for the peak values of cyclic loading as shown in Figure 21. Traditional health monitoring test is also performed to draw the load-displacement curve for comparison. From the load-displacement curve, it can be seen that the load-displacement is not linear after point 7 and the load cannot increase after point 17, which means the column yields after point 7 and it has a structural failure around point 17. From the ductility level of the testing points at positive loading peaks shown in Figure 22. Testing point 7 has the ductility value of 1, which represents the yielding of the concrete column.Figure 19 Load- Displacement curveFigure 20 Ductility level of the testingpoint at positive loading peaksFrom the SHDIM under positive loading condition as shown in Figure 23, the damage index value for each sensor increased gradually and had a sudden increase after testing point 7. Point 7 can be identified as a critical point to predict the structure damage which matches the results from the load-displacement curve. From the SHDIM under negative loading condition as shown in Figure 24, the damage index values have an increment before point 8 and then reduce, increasing afterwards to the critical point. The reason for this could be that during the reversed loading procedure,under positive loading, the column deforms and the crack width gradually increases on one side; however under negative loading, the column deforms to the opposite direction to close some cracks or even compact the density at some locations.Point numberD a m a g e i n d e x v a l u ePoint numberD a m a g e i n d e x v a l u eFigure 21 SHDIM under positive loading condition (SA15 as anactuator)Figure 22 SHDIM under negative loading condition (SA15 as an actuator)Figure 25 and Figure 26 show the sensor energy history of SA16 with SA15 as an actuator under positive and negative loading, respectively. As shown in Figure 25, the sensor energy under positive loading decreased with increasing load and energy dramatically decreased after point 7. This can be viewed as a critical point to predict structural damage. As shown in Figure 26, the sensor energy increased at test points 2, 4, and 6 and dramatically dropped after test point 6. Under negative loading, cracks generated during positive loading closed and the transmission energy may increase due to this reason. Also the level of sensor energy under negative loading was larger than the sensor energy under positive loading. This means it is possible that the proposed SAs could be used to detect the loading direction under reversed cyclic testing. As indicated in the energy history plot shown in Figures 25 and 26, after test point 7, the sensor energy has a dramatic drop under both positive and negative loading. This means at point 7, there has been a severe damage status. Figure 27 and Figure 28 show the 3-D normalized sensor energy of each sensor under positive and negative loading, respectively. For comparison purposes, the maximum value of the sensor energy is normalized to 1. It can be seen that the energy of each sensor has a dramatic drop after point 7, verifying that around point 7 is a critical point for health status of the concrete column. From the damage index matrix results, sensor history plot and 3-D normalized sensor energy plot, it can be seen that the critical failure point is at point 7 which matches the traditional health monitoring results.Point numberS e n s o r e n e r g yPoint number S e n s o r e n e r g yFigure 23. Sensor energy history plot of SA16 under positive loading (SA15 as an actuator)Figure 24 Sensor energy history plot of SA16 under negative loading (SA15as an actuator)N o r m a l i z e d E n e r g ySensor No.N o r m a l i z e d E n e r g yFigure 25. 3-D normalized sensor energy plot under positive loading(SA15 as an actuator) Figure 26. 3-D normalized sensor energy plot under negative loading(SA15 as an actuator)In this test, a three-dimensional (3-D) smart aggregate was used for structural health monitoring purposes. There are three piezoceramic patches located at different orientations inside the 3-D smart aggregate. Figure 29 and Figure 30 show the sensor energy of three different piezoceramic patches with different orientation under positive and negative loading, respectively. From the experimental results, the sensor energy of 3D-5 was the greatest among three sensors and the sensor energy of 3D-6 was the smallest among three sensors. SA15 as an actuator was in the vertical direction and sensors 3D-5 and 3D-6 were in the horizontal and vertical direction, respectively. The experimental results for the 3-D smart aggregate have shown that the response amplitude from 3-D smart aggregate are related with the orientation of the sensors. From the 3-D normalized sensor energy plots in Figure 27 and Figure 28, the attenuation trend of the transmission energy for three piezoceramic patches(3D-4, 3D-5 and 3D-6) in 3-D smart aggregate is similar. The experimental results of the 3-D smart aggregates in column B further verify that the orientation of piezoceramic patches affects the response amplitude; however the attenuation trend of transmission wave is not affected by the orientation of piezoceramic sensors.Figure 27. Sensor energy of threepiezoceramic patches in the 3-D smartaggregate under positive loading (SA15as an actuator)Figure 28. Sensor energy of threepiezoceramic patches in 3-D smartaggregate under negative loading(SA15 as an actuator)CONCLUSIONIn this study, innovative piezoceramic-based smart aggregates were used as transducers for the structural health monitoring of two reinforced concrete columns under reverse cyclic loading protocols with different torsion/moment ratio. The experimental results of sensor energy and 3-D normalized sensor energy plot demonstrated that the damages inside attenuated the transmitted energy during the loading procedure. A wavelet-packet-based damage index and a sensor-history damage index matrix were developed to evaluate the damage status based on the attenuation of the transmitted wave. The experimental results have shown that the proposed damage index matrix has extracted useful damage development information, such as a precautious point of failure and damage location information. The structural health monitoring results from the piezoceramic-based approach are verified by the ductility value in the loading protocol, normalized sensor energy plot and the load-displacements results from the traditional health monitoring approach. Three-dimensional smart aggregates were also implemented in the health monitoring tests and the experimental results show that the orientation of piezoceramic patch affects the response amplitude but the orientation does not affect the attenuation trend of the transmission energy. The proposed smart-aggregate-based approach has the potential to be applied to the health monitoring of large-scale concrete structures.ACKNOWLEDGEMENTSThe research study described herein was sponsored by the National Science Foundation under the Award No. CMMI-0724190. The opinions expressed in this study are those of the authors and do not necessarily reflect the views of the sponsor. In addition, the effort from team in Missouri University of Science and Technology at Rolla is greatly appreciated for the progress of this project.。