Fast Monte Carlo calculation of scatter correctio
Monte Carlo方法简介
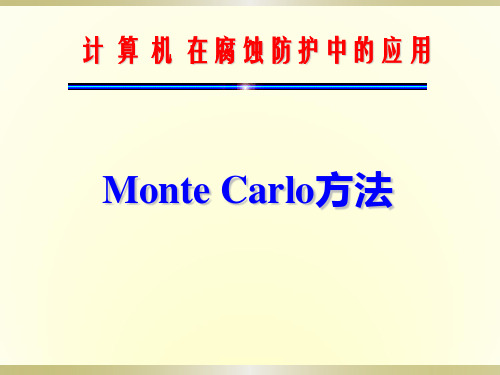
Monte Carlo方法
Modelling water adsorption on Au(210) surfaces: II. Monte Carlo simulations
Monte Carlo方法
高分子构象的Monte Carlo模拟
Monte Carlo方法
Adsorption Mechanism and Dynamic Behavior of Water and Ethanol Molecules Inside Au Nanotubes
统计系统的热力学性质及其他物理量
No
统计性 质不变?
打印结果,结束
Monte Carlo方法
微正则系综蒙特卡罗方法 巨正则系综蒙特卡罗方法 正则系综蒙特卡罗方法 等温等压蒙特卡罗方法
MC 就是一种通过重要性抽样的方法计算统计平均值的 一种随机方法。 它基于统计力学,通过 微观可观测量的系 综平均来求算其宏观性质,
1、数学:本身已形成计算数学的一个分支; 2、粒子物理:输运问题、屏蔽问题、核武器试验分析等; 3、统计物理、化学,材料、工程各领域; 4、其它:疾病传播与免疫、系统工程与管理优化等等。
Monte Carlo方法
1% 49 %
Nicholas Metropolis (1915-1999)
49 % 1%
•分子模拟的两种主要方法:
⑴ ⑵ 分子动力学法 (MD,Molecular Dynamics) 基于粒子运动的经典轨迹 Monte Carlo法 (MC) 基于概率和统计力学
Monte Carlo方法
1.2 Monte Carlo方法的发展历史
Monte Carlo 原为地中海沿岸Monaco(摩纳哥)的一个城市 的地名, 是世界闻名的大赌场,Monte Carlo方法的随机抽样特 征在它的命名上得到了反映。
使用Monte Carlo方法观察受限状态下嵌段聚合物自组装结构-高分子物理-实验5-05

实验五使用Monte Carlo方法观察受限状态下嵌段聚合物自组装结构一、实验目的1.了解Monte Carlo方法模拟聚合物自组装的基本原理2.观察在受限状态下,聚合物自组装过程二、实验原理嵌段共聚物是由化学性质不同的、两个或两个以上的链段,通过化学键相连接而组成的高分子体系。
不同链段之间由于性质不同而相互排斥,导致体系在熔融状态下或者溶液中发生相分离。
由于各嵌段之间由共价键相连,体系的相分离只能发生在微观的链段尺度上。
这种微观尺度的相分离形成的自组装结构尺寸在10 ~ 100纳米之间,它们可以应用于各种纳米器件的制备如微反应器、磁性介质存储等领域,有着广泛的应用前景。
通过细致的研究,人们已经得出这样的结论:这些纳米结构的形成主要是依赖于嵌段共聚物的各种分子参数,例如:分子链内各组分的化学物理特性,不同嵌段间的相互作用,以及分子链的结构性质。
此外,人们通过研究还发现,自组装体系的环境通过对自组装过程的限制可以影响聚合物体系最终的自组装结构。
这一现象表明,人们或许可以通过调控外界环境从而制备新型的纳米结构材料。
在受限状态下,嵌段聚合物与环境界面间的相互作用、环境限制的几何形状和尺度都会影响聚合物自组装的过程。
例如,对称的二嵌段共聚物在本体熔融状态下会自组装形成层状结构。
当这种对称的二嵌段共聚物在硬质平行板间进行自组装时,如果板间距与聚合物自身的层状周期不相容,聚合物的周期结构就会发生改变,从而偏离本体时的稳定结构。
研究者们发现在这一过程中,如果平行板对不同的嵌段有不同的作用,体系就会出现板壁诱导形成的独特结构。
Monte Carlo方法在数学上称其为随机模拟(random simulation)方法,随机抽样(random sampling)技术或统计实验(statistical testing)方法。
它的基本思想是:为了求解数学、物理、几何、化学等问题,建立一个概率模型或随机过程,使它的参数等于问题的解;当所解的问题本身属随机性问题时,则可采用直接模拟法,即根据实际物理情况的概率法来构造Monte Carlo模型;然后通过对模型,或过程的观察,或抽样实验来计算所求参数的统计特征,最后给出所求解的近似值。
蒙特卡洛法与有限元结合搜索边坡临界滑动面
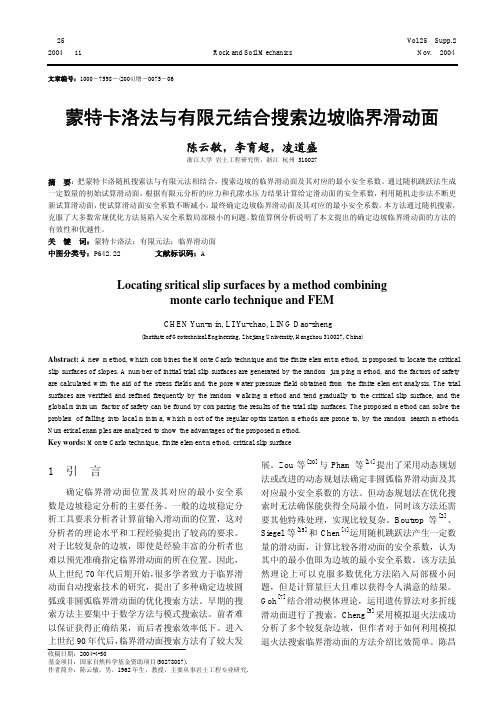
2004年11月 Rock and Soil Mechanics Nov. 2004收稿日期:2004-4-30基金项目:国家自然科学基金资助项目(50278087).作者简介:陈云敏,男,1962年生,教授,主要从事岩土工程专业研究.文章编号:1000-7598-(2004)增-0075-06蒙特卡洛法与有限元结合搜索边坡临界滑动面陈云敏,李育超,凌道盛( 浙江大学 岩土工程研究所,浙江 杭州 310027 )摘 要:把蒙特卡洛随机搜索法与有限元法相结合,搜索边坡的临界滑动面及其对应的最小安全系数。
通过随机跳跃法生成一定数量的初始试算滑动面,根据有限元分析的应力和孔隙水压力结果计算给定滑动面的安全系数,利用随机走步法不断更新试算滑动面,使试算滑动面安全系数不断减小,最终确定边坡临界滑动面及其对应的最小安全系数。
本方法通过随机搜索,克服了大多数常规优化方法易陷入安全系数局部极小的问题。
数值算例分析说明了本文提出的确定边坡临界滑动面的方法的有效性和优越性。
关 键 词:蒙特卡洛法;有限元法;临界滑动面 中图分类号:P642.22 文献标识码:ALocating sritical slip surfaces by a method combiningmonte carlo technique and FEMCHEN Yun-min, LI Yu-chao, LING Dao-sheng(Institute of Geotechnical Engineering, Zhejiang University, Hangzhou 310027, China)Abstract: A new method, which combines the Monte Carlo technique and the finite element method, is proposed to locate the critical slip surfaces of slopes. A number of initial trial slip surfaces are generated by the random jumping method, and the factors of safety are calculated with the aid of the stress fields and the pore water pressure field obtained from the finite element analysis. The trial surfaces are verified and refined frequently by the random walking method and tend gradually to the critical slip surface, and the global minimum factor of safety can be found by comparing the results of the trial slip surfaces. The proposed method can solve the problem of falling into local minima, which most of the regular optimization methods are prone to, by the random search methods. Numerical examples are analyzed to show the advantages of the proposed method. Key words: Monte Carlo technique, finite element method, critical slip surface1 引 言确定临界滑动面位置及其对应的最小安全系数是边坡稳定分析的主要任务。
210975402_高比例新能源电力系统静态电压稳定裕度在线概率评估

第51卷第5期电力系统保护与控制Vol.51 No.5 2023年3月1日Power System Protection and Control Mar. 1, 2023 DOI: 10.19783/ki.pspc.220677高比例新能源电力系统静态电压稳定裕度在线概率评估齐金山1,姚良忠1,廖思阳1,刘运鑫1,蒲天骄2,李 健2,王新迎2(1.武汉大学电气与自动化学院,湖北 武汉 430072;2.中国电力科学研究院有限公司,北京 100192)摘要:新能源的随机性、波动性及弱调节特性给电力系统静态电压的安全及稳定性带来了挑战。
针对此问题,提出一种考虑源荷双侧不确定性的高比例新能源电力系统静态电压稳定裕度在线概率评估方法。
首先,基于新能源无功调节特性与传统机组的差异,分析了大量新能源替代传统机组对稳定裕度的影响。
然后,分析了新能源出力不确定性对稳定裕度分布范围的影响,并建立源荷不确定性模型以生成典型场景。
最后,为了应对新能源快速波动性给稳定裕度带来的影响,提出基于优化ELM-KDE的稳定裕度在线概率评估方法。
利用优化极限学习机(extreme learning machine, ELM)预测典型场景稳定裕度并通过核密度估计(kernel density estimation, KDE)准确获得其概率分布函数。
构建了静态电压稳定期望裕度和静态电压稳定风险度两个指标对结果进行表征。
分别在New England 39和IEEE300节点系统进行了仿真测试,并将结果与传统蒙特卡洛方法计算结果对比,验证了所提方法的有效性。
关键词:高比例新能源;静态电压稳定裕度;不确定模型;概率评估;极限学习机Online probabilistic assessment of static voltage stability margin for power systemswith a high proportion of renewable energyQI Jinshan1, YAO Liangzhong1, LIAO Siyang1, LIU Yunxin1, PU Tianjiao2, LI Jian2, WANG Xinying2(1. School of Electrical Engineering and Automation, Wuhan University, Wuhan 430072, China;2. China Electric Power Research Institute, Beijing 100192, China)Abstract: The randomness, volatility and weak regulation characteristics of renewable energy have brought new challenges to the static voltage safety and stability of a power system. In view of this, an online probability evaluation method of static voltage stability margin of a power system with a high proportion of renewable energy considering the bilateral uncertainties of source and load is proposed. First, the influence of a large number of renewable energies replacing traditional units on static voltage stability margin is analyzed based on the difference of reactive power regulation characteristics between them. Then the influence of renewable energy output uncertainty on the distribution range of the stability margin is analyzed, and source and load uncertainty models are established to generate typical scenarios. Finally, in order to deal with the rapid fluctuation of stability margin brought by renewable energy, an online probability assessment method of stability margin based on optimized ELM-KDE is proposed. The stability margin of typical scenarios is predicted by an optimized extreme learning machine (ELM), and its probability distribution function is accurately obtained by kernel density estimation (KDE). The expected margin of static voltage stability and the risk of static voltage stability are constructed to characterize the results. Simulation tests are carried out on the New England 39 and IEEE 300 node systems, and the results are compared with the traditional Monte Carlo calculation results to verify the effectiveness of the proposed method.This work is supported by the National Key Research and Development Program of China (No. 2020YFB0905900).Key words: high proportional renewable energy; static voltage stability margin; uncertainty model; probabilistic assessment; extreme learning machine0 引言近年来,世界范围内相继发生多起停电事故,基金项目:国家重点研发计划项目资助(2020YFB0905900);国家电网有限公司总部科技项目资助:电力物联网关键技术 再次为电力系统的安全稳定敲响了警钟[1-4]。
MonteCarlo方法在放射治疗剂量分布计算中的应用_王春燕

在调强放射治疗技术中 , 由 于准直器叶片几何 形状的复 杂性和传送的时间依赖性 , 其剂量计算问题一直困扰着放射医 学界 。P J K e all ,J V Siebe r s[7]等人应用 M o nte Ca r lo 方法对 这一问题进行了研究 。对于两种能量光子及多叶准直器模型的 辐射场剂量分布进行了计算 , 并与实验测量结果进行比较 , 在 实验误差允许的范围内 , 结果是一致的 。同时 ,他们还发现对于 患者调强放射治疗辐射场 , 应用 M o nte C ar lo 方法所得到的结 果比其它计算方法(笔形束模型等)更接近实验测量值 。
M CN P 程序 全名为 M o nte C ar lo N ew tr o n a nd P h o to T r a nspo r t C o le , 它是美国洛斯阿拉莫斯(L A N L)国家实验室 的众多科学家和 M C 专家在一系列程序工作基础上 , 集中编制 的一个当前最高水平的通用的科学计算程序 , 用于处理连续能 量 、时间相关 、 三维几何的中子 -光子 -电子辐射输运问题 。 M CN P 从 1977 年 6 月产生第一个版本后 , 不断更新 , 到 1997 年 3 月产生了最新版本 M C N P -4B , 可以在各类计算机上运 行。
EG S 4 计算程序在国际上有着广泛的应用 , 在辐射物理方 面 , 主要计算加速器产生的辐射剂量分布 , 设计屏蔽系统 , 研制 剂量测量仪等 。在医学物理方面 , 模拟计算射线在人体中产生 的剂量分布及衰减 , 进而确定治疗方案等 , 在西欧和北美的一 些国家 , 已被广泛应用于医院 , 用于放射治疗及诊断中的剂量 计算 , 自从 1979 年公布以来 , 已有近千篇相关论文发表 , 其可 靠性得到了国际社会的认可 。
直接使用CAD几何的蒙特卡罗粒子输运方法研究

摘要:传统的蒙特卡罗程序通常使用构造实体几何(CSG)的方法进行几何建模$然而对于某些复杂的
高阶曲面使用CSG进行精确建模非常困难且会耗费大量的时间$为解决这一问题,国际上提出了一种 直接使用CAD几何建模的蒙特卡罗(DAGMC)方法来进行粒子输运计பைடு நூலகம்$本文基于反应堆蒙特卡罗
本文基于堆用蒙特卡罗程序RMC⑺开发 使用DAGMC几何的DAG-RMC程序。通过 对方块组合体、燃料棒和犹他州茶壶3种模型 分别使用不同几何建模方法得到的临界计算结 果分析,验证DAG-RMC程序临界计算功能的 正确性。
1 RMC中DAGMC的实现
将RMC中原有的Cell类扩展为CSGCell 和DAGMCCell两类9 Surface类扩展为CSGSurface 和 DAGMCSurface 两类,并在 RMC 源 代码中调用DAGMC第3方库中的几何处理 函数。首先,利用DAGMC库函数将CAD几 何文件(h5m)所包含的栅元、曲面和材料信息 读取并转换为RMC的栅元、曲面和材料信息, 并把材料分配给对应的栅元,给各曲面定义对 应的边界条件。DAGMC几何的材料分配有两 种方式:一种是读取RMC输入文件的材料卡 信息;另一种是将材料信息嵌入到DAGMC文 件(h5m)中,使用UWUW模式读取材料信息$
然后,利用DAGMC库函数实现粒子定 位、计算粒子到边界距离、穿面后栅元判断等功 能,从而使RMC可基于CAD几何进行粒子输 运$添加DAGMC几何后的DAG-RMC程序
粒子输运模拟流程如图1所示,相关库函数的 作用列于表1$
图1 DAG-RMC粒子输运模拟流程
Fig. 1 DAG-RMC particle transport simulation process
腱绳驱动仿人灵巧手运动分析

NEW PRODUCT NEW TECHNOLOGY0 引言伴随着工业及科技领域的蓬勃发展,中国对航空航天、核工业等战略性产业愈加重视。
在这些工业场景中,工作人员通常需要在极端、危险的条件下进行作业,对人身体健康会产生较大危害。
为此,可替代技术人员进入此类极端工业场景完成复杂工作任务的智能机器人应运而生,其末端执行器的选择直接影响了机器人的工作效率。
作为结合了仿生学新型末端执行器[1,2]的灵巧手,拥有灵巧性高、适应性强、可完成多种不同类型的复杂操作等优点,成为近年来机器人领域研究的热点。
仿人灵巧手可分为全驱动仿人灵巧手和欠驱动仿人灵巧手[3-7]2类。
针对欠驱动灵巧手的发展和研究,美国宇航局(NASA)对在航天领域应用的空间机器人研制了早期最为经典的一种欠驱动灵巧手Robonaut Hand[8],此后又与通用汽车合作对上一代灵巧手进行优化研制了第二代Robonaut Hand。
Catalano M G等[9]研制了PISA/IIT SoftHand,这种手仅用单一舵机驱动整个具有19个自由度的灵巧手,其很好地利用了腱绳驱动方式的优势,为腱绳驱动灵巧手提供了思路。
徐昱琳等[10]研制的SHU-Ⅱ采用轻质腱绳驱动并将6个直流电动机内置于手掌,其中5个电动机分别控制各手指弯曲运动,余下的1个电动机控制拇指侧摆,具有较大的运动抓取空间。
基于此,本文设计了一种腱绳驱动仿人灵巧手,先对其进行运动学分析,再求出其雅克比矩阵,分析手指动力学性能和运动空间,为仿人灵巧手提供控制依据和理论。
1 仿人灵巧手的总体设计本文灵巧手依据人手作为仿生对象,根据人体手部腱绳驱动仿人灵巧手运动分析王峥宇1 张 立1 陈耀轩1 梅 杰1,2 陈定方1,21武汉理工大学交通与物流工程学院 武汉 430063 2武汉理工大学智能制造与控制研究所 武汉 430063摘 要:针对在极端环境下代替人工进行作业的需求,文中设计了一种由腱绳驱动的灵巧手作为高效机器人末端执行器。
MonteCarlo方法研究低能电子束曝光沉积能分布规律
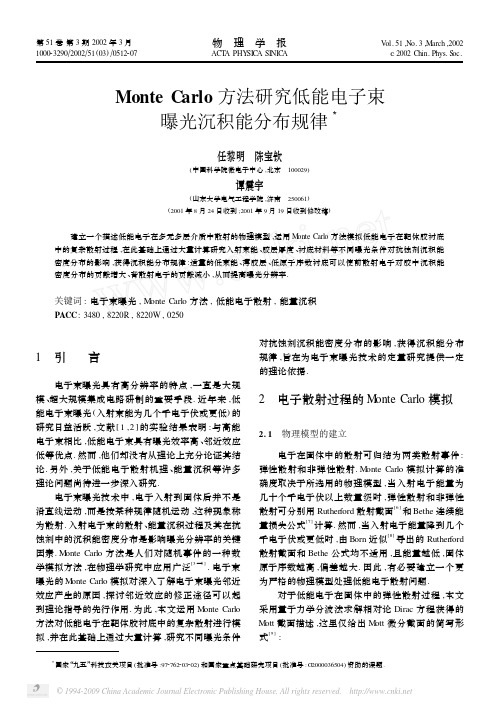
Monte C arlo 方法研究低能电子束曝光沉积能分布规律3任黎明 陈宝钦(中国科学院微电子中心,北京 100029)谭震宇(山东大学电气工程学院,济南 250061)(2001年8月24日收到;2001年9月19日收到修改稿) 建立一个描述低能电子在多元多层介质中散射的物理模型,运用M onte Carlo 方法模拟低能电子在靶体胶衬底中的复杂散射过程,在此基础上通过大量计算研究入射束能、胶层厚度、衬底材料等不同曝光条件对抗蚀剂沉积能密度分布的影响,获得沉积能分布规律:适量的低束能、薄胶层、低原子序数衬底可以使前散射电子对胶中沉积能密度分布的贡献增大、背散射电子的贡献减小,从而提高曝光分辨率.3国家“九五”科技攻关项目(批准号:972762203202)和国家重点基础研究项目(批准号:G 2000036504)资助的课题.关键词:电子束曝光,M onte Carlo 方法,低能电子散射,能量沉积PACC :3480,8220R ,8220W ,02501 引言电子束曝光具有高分辨率的特点,一直是大规模、超大规模集成电路研制的重要手段.近年来,低能电子束曝光(入射束能为几个千电子伏或更低)的研究日益活跃,文献[1,2]的实验结果表明:与高能电子束相比,低能电子束具有曝光效率高、邻近效应低等优点.然而,他们却没有从理论上充分论证其结论.另外,关于低能电子散射机理、能量沉积等许多理论问题尚待进一步深入研究.电子束曝光技术中,电子入射到固体后并不是沿直线运动,而是按某种规律随机运动,这种现象称为散射.入射电子束的散射、能量沉积过程及其在抗蚀剂中的沉积能密度分布是影响曝光分辨率的关键因素.M onte Carlo 方法是人们对随机事件的一种数学模拟方法,在物理学研究中应用广泛[3—5].电子束曝光的M onte Carlo 模拟对深入了解电子束曝光邻近效应产生的原因,探讨邻近效应的修正途径可以起到理论指导的先行作用.为此,本文运用M onte Carlo 方法对低能电子在靶体胶衬底中的复杂散射进行模拟,并在此基础上通过大量计算,研究不同曝光条件对抗蚀剂沉积能密度分布的影响,获得沉积能分布规律,旨在为电子束曝光技术的定量研究提供一定的理论依据.2 电子散射过程的M onte Carlo 模拟2.1 物理模型的建立电子在固体中的散射可归结为两类散射事件:弹性散射和非弹性散射.M onte Carlo 模拟计算的准确度取决于所选用的物理模型,当入射电子能量为几十个千电子伏以上数量级时,弹性散射和非弹性散射可分别用Rutherford 散射截面[6]和Bethe 连续能量损失公式[7]计算.然而,当入射电子能量降到几个千电子伏或更低时,由Born 近似[8]导出的Rutherford 散射截面和Bethe 公式均不适用,且能量越低,固体原子序数越高,偏差越大.因此,有必要建立一个更为严格的物理模型处理低能电子散射问题.对于低能电子在固体中的弹性散射过程,本文采用量子力学分波法求解相对论Dirac 方程获得的M ott 截面描述,这里仅给出M ott 微分截面的简写形式[9]:第51卷第3期2002年3月100023290Π2002Π51(03)Π0512207物 理 学 报ACT A PHY SIC A SI NIC AV ol.51,N o.3,March ,2002ν2002Chin.Phys.S oc.d σ(θ)d Ω=|f (θ)|2+|g (θ)|2,(1)其中f (θ)和g (θ)为利用量子力学分波法求解相对论Dirac 方程获得的入射波和散射波函数.低能电子非弹性散射平均能量损失率的计算,采用Joy 修正的Bethe 公式[10]:d E d S =-7.85×104ρZ A E ln 1.166(E +kJ )J(keV Πcm ),(2)其中ρ为介质密度,Z 为原子序数,A 为原子量,k 为修正系数.对于模拟中所用到的光刻胶PM MA 、衬底Si 和Au ,本文将k 值分别取为0.757,0.822和0.851.2.2 Monte C arlo 模拟方法电子入射固体后,要发生多次散射.电子每次散射行为由4个变量决定:前次散射终点处的能量E n 、散射角θn 、散射方位角<n 、散射步长Λn .电子在多元介质中散射,本文采用概率随机抽样方法[11]确定散射中心.确定散射中心后,计算弹性散射总截面σt ,则电子散射步长Λ、散射角θ、散射方位角<均可由随机抽样方法产生:Λ=-A ln R 1Π(ρN 0σt ),(3)R 2=∫θd σ(θ)d Ωsin θd θ∫πd σ(θ)d Ωsin θd θ,(4)<=2πR 3,(5)其中R 1,R 2,R 3均为[0,1]内均匀分布的随机数,A 为原子量,ρ为介质密度,N 0为阿伏伽德罗常数,σt 为弹性散射总截面.电子第n 次散射终点处的能量E n +1通过下式计算:E n +1=E n -d E d sEnΛn ,(6)其中E n 为电子第n -1次散射终点处的能量,Λn 为第n 次的散射步长,|d E Πd s |En为第n 次非弹性散射平均能量损失率,由(2)式计算.M onte Carlo 方法模拟电子散射过程就是依据一定的物理模型,通过计算上述各量,对每个电子的每次散射行为进行模拟,进而模拟出大量电子在固体中的运动轨迹.图1为模拟得到的不同入射束能的低能电子在靶体PM MA 2Si 中的散射轨迹图.其中PMMA 厚度取为66nm (这是实验中已制成的较小厚度),模拟电子数为20000.图1 不同入射束能电子束在PM M A 2S i 中的散射轨迹图3 沉积能密度计算电子束曝光技术中,胶中沉积能密度是研究沉积能分布规律的一个重要参数.一般而言,沉积能密度分布越陡峭,邻近效应越低,线条分辨率越高.研究沉积能密度分布,通常将电子散射效应简化为两种:前(向)散射与背(向)散射,如图2所示.相应地,将发生前散射和背散射的电子分别称为前散射电子和背散射电子.由图2可以看出:背散射使电子束变宽的程度比前散射大得多.返回胶中的背散射电子将参与对胶层的曝光作用,致使不需要曝光的区域被曝光,从而使显影出来的图形比预期的要宽,导致邻近效应加重.在分辨率要求较高的情况下,背散射是曝光精度的最大限制.由于前散射电子与背散射电子均会引起能量沉积,通常将其沉积于胶中的能量分别加以记录.图2 电子的散射效应对垂直入射的电子束,由于电子在胶中的散射关于入射中心轴对称,为了计算胶中的能量沉积,可做如下划分:如图3所示,将胶层沿电子束入射中心方向分为若干非常薄的子层,子层的厚度记为ΔZ .在每一子层上,以z 轴为中心,将胶层沿径向分为若干同心圆环,圆环半径增量记为Δr ,ΔZ 和Δr 足够3153期任黎明等:M onte Carlo 方法研究低能电子束曝光沉积能分布规律小.这样,就将胶层划分为若干个小单元.图3 能量沉积单元示意图利用M onte Carlo 方法模拟每个电子在胶中散射过程的同时,记录沉积在任一单元Ω中的能量.对大量电子进行模拟,并将所有入射电子在Ω中沉积的能量相加,便可计算出任一小单元中沉积的能量.沉积能分布主要受入射束能、胶层厚度、衬底材料等曝光条件影响.由于低能电子束曝光工艺中使用薄胶层,为便于比较不同曝光条件对胶中沉积能密度的影响,可以用沉积能面密度(即每个电子单位面积上沉积的能量)代替沉积能体密度来表示横向沉积能密度分布.按照上述能量沉积单元划分方法,沉积能面密度A (r )等于沿深度方向,胶层中对应于同一半径圆环的所有子层沉积能总和E (r )与该圆环面积及入射电子总数之比为A (r )=E (r )Π(ΔS ・N 0),(7)其中N 0为入射电子总数,ΔS 为圆环面积,ΔS =π(r +Δr )2-πr 2.3.1 入射束能的影响入射束能E 0的大小直接影响电子散射过程,对沉积能密度分布的作用较大.图4为不同入射束能电子束在Si 衬底上PM MA 胶中横向沉积能密度分布.可见入射束能越低,电子在胶中作用范围越小,沉积能密度分布曲线越陡峭,从而邻近效应越低,这正是低能电子束曝光具有极高分辨率的主要原因.表1为不同入射束能的电子在Si 衬底上66nm PM MA 胶中的纵向沉积能密度分布,其中E P ΠE T 为胶中沉积的能量E P 占沉积在胶和衬底中的总能量E T 的百分比,可以看出:电子束入射束能越低,沉积于胶中的能量占总沉积能的比例越大.因此,适量的图4 不同入射束能电子束在S i 衬底上PM M A 胶中的横向沉积能密度分布低能电子束曝光可以将大部分能量沉积于胶中,使用较小的曝光剂量即可达到充分曝光的目的,这就使低能电子束曝光具有曝光效率高的优点.另外,胶层厚度一定,电子作用深度大于胶层厚度时,入射束能E 0越低,电子与衬底的最大作用深度(Z max )越小,对衬底的损伤越轻.表1不同入射束能电子在S i 衬底上P M M A 胶中的纵向沉积能密度分布E 0ΠkeV 5432 1.5Z max Πnm42930620412084(E P ΠE T )Π%10.2515.9328.8261.1493.41 为比较前散射电子和背散射电子对胶中沉积能的影响,本文进一步计算了不同入射束能下前散射电子与背散射电子对胶中沉积能密度分布的贡献,如图5(a )和(b )所示.由图5可见:当E 0=5keV 时,除了入射点附近,背散射电子的贡献比前散射电子大得多,而且背散射电子较前散射电子作用范围大得多,胶中沉积能密度分布主要由背散射电子决定;而当E 0=1.5keV 时,背散射电子对胶中沉积能的贡献远较前散射电子的贡献小(约低两个数量级),作用范围也小,胶中沉积能密度分布取决于前散射电子.对此本文作如下分析:由能量损失率公式(2)可知,入射束能较高时,电子能量损失率较低,在胶中损失的能量较少,电子散射行程较长,因而大量电子穿越界面进入衬底,这就使得返回胶中的背散射电子数目较多,且能量较高,从而在胶中沉积较多的能量,作用范围也较宽,因此,背散射电子对胶中沉积能密度分布起决定作用.但在入射点附近,背散射电子经过的概率却较小,对沉积能密度分布贡献不大;415物 理 学 报51卷当入射电子束能量较低时,由于能量损失率较高,电子行程短,许多电子未进入衬底就已经耗尽能量,终止在胶中,这样由衬底返回胶中的背散射电子较少,且能量较低,对胶中沉积能的贡献较小.由此可以得出一个结论:适量的低能电子束曝光,可以使前散射电子比背散射电子的贡献大得多,对胶中沉积能密度分布起主导作用,从而降低邻近效应,提高分辨率.图5 不同入射束能下前散射电子与背散射电子对胶中沉积能密度分布的贡献 ———为前散射电子,……为背散射电子312 胶层厚度的影响胶膜的厚度T f 对沉积能密度分布也有重要影响.图6为入射束能为3keV 的电子在三种厚度胶层中的沉积能密度分布.可见胶层越薄,胶中沉积能密度分布范围越小,沉积能密度分布越陡峭.这就说明低能电子束曝光中,薄胶层有利于降低邻近效应,提高曝光分辨率.图6 不同厚度胶膜中沉积能密度分布另外,为了比较不同厚度胶膜情况下,前散射电子和背散射电子对胶中沉积能密度分布的影响,本文进一步计算了E 0=3keV 时,前散射电子与背散射电子在4种不同厚度胶膜中的沉积能密度分布,如图7(a )和(b )所示.可以看出:1)胶厚越薄,前散射电子与背散射电子的沉积能密度分布范围越小,但主要影响前散射电子,对于背散射电子,此趋势不明显,沉积能密度分布曲线几乎重合;2)入射点附近前散射电子对胶中沉积能的贡献较背散射电子的贡献大一个数量级,沉积能密度主要取决于前散射电子.对此本文分析如下:对前散射电子而言,由于胶层越薄,入射电子进入衬底前在胶中散射的次数越少,因而前散射电子的分布范围越小,且能量主要集中于入射点附近;而对背散射电子而言,由于低能电子在胶层中能量损失率较低,因而胶层厚度的小范围变化(由于低能电子束曝光工艺中使用薄胶层,模拟计算中胶层厚度不可能变化很大)对进入衬底的电子能量影响不大,从而对背散射电子的数量及其对沉积能密度分布的贡献影响较小.3.3 衬底材料的影响衬底材料的性质可以决定从衬底返回胶中背散射电子的数量、能谱分布等,因此对胶中沉积能密度分布也有重要影响.衬底材料对胶中沉积能密度分布的影响与入射束能有关,图8(a )和(b )给出不同束能的电子在Si 衬底和Au 衬底上66nm PM MA 胶中的横向沉积能密度分布.由图8可见,当E 0=3keV 时,两种衬底上胶中沉积能密度分布在入射点附近表现出明显差异,原子序数较低的Si 衬底,沉积能密度分布略微陡峭一些;而当E 0=1.5keV 时,不同衬底上胶中沉积能密度分布曲线几乎重合.从而表明:一定胶层厚度,入射束能较低时,衬底材料对胶中沉积能密度分布影响不大.5153期任黎明等:M onte Carlo 方法研究低能电子束曝光沉积能分布规律图7 E 0=3keV 时前散射电子(a )与背散射电子(b )对不同厚度PM M A胶中沉积能密度分布的贡献图8 不同衬底胶中沉积能密度分布 ———为S i 衬底,……为Au 衬底 为研究不同衬底材料对前散射电子与背散射电子在胶中沉积能密度分布的影响,本文进一步计算了上述两种入射束能下,Si 衬底和Au 衬底上前散射电子与背散射电子在66nm 胶中的沉积能密度分布,如图9(a )和(b )和图10(a )和(b )所示.由图9和图10可以看出:前散射电子的沉积能密度分布几乎不受衬底材料影响,衬底材料主要影响背散射电子的沉积能密度分布,而且入射束能越高,对背散射电子沉积能密度分布的影响越大.当E 0=3keV 时,由图9(a )和(b )可知,在入射点附近,对Si 衬底而言,胶中沉积能密度主要由前散射电子决定,背散射电子对沉积能的贡献比前散射电子的贡献小得多;而对Au 衬底而言,背散射电子对胶中沉积能的贡献与前散射电子的贡献差别不大(具有相同的数量级).因此,在入射点附近,Au 衬底上胶中沉积能密度要大一些.但在离入射点较远处,两种衬底上胶中背散射电子对沉积能的贡献相差不大,因而沉积能密度分布差别较小.这样就出现了图8(a )所示结果;当E 0=1.5keV 时,由图10(a )和(b )可知,背散射电子对Au 和Si 两种衬底上胶中沉积能贡献虽然稍有差异,但由于两种衬底上前散射电子对胶中沉积能的贡献相当,而前散射电子较背散射电子的贡献大得多(几乎大两个数量级),因此总而言之,两种衬底上胶中沉积能密度分布差别较小,出现了图8(b )所示结果.对此本文分析如下:由于前散射电子几乎不受衬底影响,因此无论入射束能如何变化,不同衬底几乎不影响前散射电子的沉积能密度分布,但是,入射束能E 0的大小却影响背散射电子的沉积能密度分布.当E 0较高时,进入衬底的电子数量及能量均较大,由能量损失公式(2)知:原子序数较高的衬底,其能量损失率相对较低,电子在衬底中散射所损失的能量较少,由衬底返回胶中的背散射电子数量及能量均较大;而当入射束能E 0较低时,由于进入衬底的电子的数量及能量均较小,不同衬底材料所产生的背散射电子的数目及能量差别不大,因此入射束能较低时,衬底材料变化对背散射电子沉积能密度分布的影响较小.615物 理 学 报51卷图9 E 0=3keV 时前散射电子(a )与背散射电子(b )对不同衬底胶中沉积能密度分布的贡献 图注同图8图10 E 0=115keV 时前散射电子(a )与背散射电子(b )对不同衬底胶中沉积能密度分布的贡献 图注同图84 结语由沉积能密度分布的M onte Carlo 模拟计算结果,本文通过分析和归纳,总结出沉积能的分布规律:适量的低束能、薄胶层、低原子序数衬底,可以使前散射电子对胶中沉积能密度分布的贡献增大、背 散射电子的贡献减小,从而提高曝光分辨率.当然,实际电子束曝光中,还应该兼顾其他因素,合理地选用曝光条件.例如,低束能可以提高曝光效率、降低邻近效应、减轻对衬底的损伤程度.但是,受工艺中所能达到的胶膜厚度的限制,入射束能也不可太低,否则部分光刻胶将得不到充分曝光,显影后在胶与衬底界面残留下胶膜,从而影响刻蚀精度.[1]Lee Y H ,Browning R ,M alu f N ,Owen G and Pease R F W 1992J .Vac .Sci .Technol .B 103094[2]S tark TJ ,Eden feld KM ,G riffis D P ,Radzimski ZJ and Russell P E 1993J .Vac .Sci .Technol .B 112367[3]Mu W B ,Chen P X 2001Acta Phys .Sin .50189(in Chinese )[牟维兵、陈盘训2001物理学报50189][4]W ei H L ,Liu Z L and Y ao KL 2000Acta Phys .Sin .49791(in Chinese )[魏合林、刘祖黎、姚凯伦2000物理学报49791][5]Zhao H ,W ang Y S ,Xu Z and Xu X R 1999Acta Phys .Sin .48533(in Chinese )[赵 辉、王永生、徐 征、徐叙 1999物理学报48533][6]Murata R 1974J .Appl .Phys .454410[7]Bethe H A 1933Handbook o f Physics (Berlin :S pringer )v ol 24p273[8]Murata K,K awata H and Nagam i K1987J .Vac .Sci .Technol .B 51247153期任黎明等:M onte Carlo 方法研究低能电子束曝光沉积能分布规律[9]M ott N F and M assy H S W1949The Theory o f Atomic Collision(Ox ford:Clarendon)p243[10]Joy D C and Luo S1989Scanning11176[11]Chen Y Q and M ao YJ1984Acta Phys.Sin.33621(in Chinese)[陈永祺、毛允静1984物理学报33621]Studies of energy dissipation distribution in low2energy electron beam lithographyby Monte C arlo method3Ren Li2M ing Chen Bao2Qin(Microelectronics R and D Center,Chinese Academy o f Sciences,Beijing 100029,China)T an Zhen2Y u(Electric Engineering College,Shandong Univer sity,Jinan 250061,China)(Received24August2001;revised manuscript received19September2001)AbstractA physical m odel describing the scattering processes of low2energy electrons is proposed.The M onte Carlo method was ap2 plied to simulate the com plex scattering processes of G aussian2distribution low2energy electrons in the resist substrate target.And on this basis,the in fluences of different exposure conditions such as incident beam energy,resist thickness and substrate material on energy dissipation density were investigated to obtain the regularity of energy dissipation distribution.It is indicated that ap2 propriately low beam energy,thin resist and low atom ic number substrate can increase the contribution of forward scattering elec2 trons to energy dissipation density distribution in the resist and reduce the contribution of backscattering electrons and thus im2 prove the exposure resolution.K eyw ords:electron beam lithography,M onte Carlo method,low2energy electron scattering,energy dissipation distribution PACC:3480,8220R,8220W,02503Project supported by the S pecial Funds for National Ninth Five2Y ear Science and T echnology Program of China(G rant N o.972762203202),and the S tate K ey Program of Basic Research of China(G rant N o.G2000036504).815物 理 学 报51卷。
马尔科夫链蒙特卡罗方法(MCMC)

马尔科夫链蒙特卡罗⽅法(MCMC)⼀.蒙特卡罗法的缺陷通常的蒙特卡罗⽅法可以模拟⽣成满⾜某个分布的随机向量,但是蒙特卡罗⽅法的缺陷就是难以对⾼维分布进⾏模拟。
对于⾼维分布的模拟,最受欢迎的算法当属马尔科夫链蒙特卡罗算法(MCMC),他通过构造⼀条马尔科夫链来分步⽣成随机向量来逼近制定的分布,以达到减⼩运算量的⽬的。
⼆.马尔科夫链⽅法概要马尔科夫链蒙特卡罗⽅法的基本思路就是想办法构造⼀个马尔科夫链,使得其平稳分布是给定的某分布,再逐步⽣模拟该马尔科夫链产⽣随机向量序列。
其基本思路如下。
就像是普通的蒙特卡罗⽅法本质上依赖于概率论中的⼤数定理,蒙特卡罗⽅法的理论⽀撑是具有遍历性的马尔科夫链的⼤数定理。
马尔科夫链蒙特卡罗⽅法的⼤体思路如下:(1)给定某个分布p(x), 构造某个马尔科夫链\lbrace X_{t}\rbrace_{t\in\mathbb{N}}使得p是其平稳分布,且满⾜⼀定的特殊条件;(2)从⼀点x_{0}出发,依照马尔科夫链\lbrace X_{t}\rbrace_{t\in\mathbb{N}}随机⽣成向量序列x_{0},x_{1},...;(3)蒙特卡罗积分估计:计算E_{p}(f)\approx\sum_{t=1}^{N}f(x_{t})三.MCMC的数学基础——马尔科夫链的遍历性,⼤数定理MCMC为什么可以近似计算积分? 其实在数学上这是不太平凡的,下⾯简要介绍⼀下其数学理论依据。
3.1 马尔科夫链与其遍历性, 马尔科夫链的⼤数定理:所谓马尔科夫链通俗的说就是⼀个随机过程,其满⾜,t时刻的状态和t-1之前的状态⽆关。
我们⽤严格的测度论语⾔说就是:定义3.1:定义于概率空间(\Omega,\mathcal{G},P), 取值于\mathcal{Y}\in\mathbb{R}^{K}的随机向量序列\lbraceX_{t}\rbrace_{t\in\mathbb{N}}称为离散时间马尔科夫链(Markov Chain of discrete time)如果其满⾜:对于任意\mathcal{Y}的Borel集B\in \mathcal{B}_{\mathcal{Y}}P(X_{t+1}^{-1}(B)\mid X_{t},...,X_{1})=P(X_{t+1}^{-1}(B)\mid X_{t})进⼀步的,如果\lbrace X_{t}\rbrace_{t\in\mathbb{N}}还满⾜:\begin{equation}P(X_{t+1}^{-1}(B)\mid X_{t})=P(X_{1}^{-1}(B)\mid X_{0})\end{equation}我们称马尔科夫链\lbrace X_{t}\rbrace_{t\in\mathbb{N}}为时间齐次(time homogeneous)的,这时我们定义该马尔科夫链的转移核(transition kernel)$P_{t}: \mathbb{N}\times\mathcal{B}_{\mathcal{Y}}\longrightarrow [0,1]:$P_{t}(y,A)\triangleq P(X_{t}\in A\mid X_{0}=y),对任意t\in\mathbb{N}, 并且我们直接简记P(y,A)=P_{1}(y,A), 对y\in\mathcal{Y}, A\in\mathcal{B}_{\mathcal{Y}}。
VR中英文对照
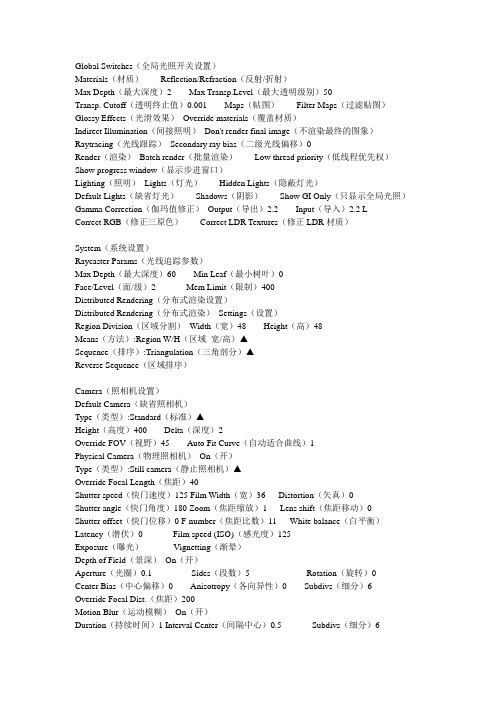
Global Switches(全局光照开关设置)Materials(材质)Reflection/Refraction(反射/折射)Max Depth(最大深度)2 Max Transp.Level(最大透明级别)50Transp. Cutoff(透明终止值)0.001 Maps(帖图)Filter Maps(过滤贴图)Glossy Effects(光滑效果)Override materials(覆盖材质)Indirect Illumination(间接照明)Don't render final image(不渲染最终的图象)Raytracing(光线跟踪)Secondary ray bias(二级光线偏移)0Render(渲染)Batch render(批量渲染)Low thread priority(低线程优先权)Show progress window(显示步进窗口)Lighting(照明)Lights(灯光)Hidden Lights(隐蔽灯光)Default Lights(缺省灯光)Shadows(阴影)Show GI Only(只显示全局光照)Gamma Correction(伽玛值修正)Output(导出)2.2 Input(导入)2.2 LCorrect RGB(修正三原色)Correct LDR Textures(修正LDR材质)System(系统设置)Raycaster Params(光线追踪参数)Max Depth(最大深度)60 Min Leaf(最小树叶)0Face/Level(面/级)2 Mem Limit(限制)400Distributed Rendering(分布式渲染设置)Distributed Rendering(分布式渲染)Settings(设置)Region Division(区域分割)Width(宽)48 Height(高)48Means(方法):Region W/H(区域宽/高)▲Sequence(排序):Triangulation(三角剖分)▲Reverse Sequence(区域排序)Camera(照相机设置)Default Camera(缺省照相机)Type(类型):Standard(标准)▲Height(高度)400 Delta(深度)2Override FOV(视野)45 Auto Fit Curve(自动适合曲线)1Physical Camera(物理照相机)On(开)Type(类型):Still camera(静止照相机)▲Override Focal Length(焦距)40Shutter speed(快门速度)125 Film Width(宽)36 Distortion(矢真)0Shutter angle(快门角度)180 Zoom(焦距缩放)1 Lens shift(焦距移动)0Shutter offset(快门位移)0 F-number(焦距比数)11 White balance(白平衡)Latency(潜伏)0 Film speed (ISO)(感光度)125Exposure(曝光)V ignetting(渐晕)Depth of Field(景深)On(开)Aperture(光圈)0.1 Sides(段数)5 Rotation(旋转)0 Center Bias(中心偏移)0 Anisotropy(各向异性)0 Subdivs(细分)6 Override Focal Dist.(焦距)200Motion Blur(运动模糊)On(开)Duration(持续时间)1 Interval Center(间隔中心)0.5 Subdivs(细分)6Bias(偏移)0 Geometry samples(几何结构采样)2Output(导出设置)Output Size(导出大小)Override Viewport(替代视窗)Width(宽)320 640x480 / 1024x768 / 1600x1200Height(高)240 800x600 / 1280x960 / 2048x1536Image Aspect(图像比率)1.3333 L Pixel Aspect(像素)1 LRender Output(渲染导出)Save file(保存文件)V-Ray Raw Image File(VRay专用RA W格式图像文件)Render to VRImage(渲染到VRay图像)Animation(动画)On(开)Frame Rate(框架率)NTSC / PAL / Film(电影)/ Custom(自定义)FPS(帧)30Environment(环境设置)GI (Skylight)(全局光照(天空光))1 M Reflection(反射)1 mBackground(背景)1 M Refraction(折射)1 mImage Sampler(图像采样设置)Image Sampler(图像采样)Fixed Rate(固定细分)■Subdivs(细分)1Adaptive QMC(自适应准蒙特卡罗)■Min Subdivs(最小细分)1 Max Subdivs(最大细分)16Adaptive Subdivision(自适应细分)■Min Rate(最小比率)-1 Max Rate(最大比率)2 Threshold(极限值)0.1 Normaks (法线)0.1Antialiasing filter(边缘抗齿锯过滤)On(开)Area(面积):Size(大小)1.5▲QMC Sampler(准蒙特卡罗采样设置)QMC Sampler(准蒙特卡罗采样)Adaptive Amount(自适应数量)1 Min Samples(最小采样值)8Noise Threshold(噪波极限值)0.01 Subdiv Mult(细分倍增)1Path Sampler(路径采样器):Randomized Halton(使随机化)▲Color Mapping(颜色映射设置)Color Mapping(颜色映射)Type(类型):Reinhard()▲Multiplier(倍增)1 Burn V alue(曝光值)0.8 Affect Background(影响背景)Clamp Output(加强输出)Sub-pixel(子像素贴图)VFB Channels(VFB通道设置)VFB Channels(VFB通道):Atmosphere(空气)▲Diffuse(漫反射)Shadow(阴影)Lighting(照明)GI(全局光照)Caustics(散焦)Raw GI(RA W全局光照)Raw Shadow(RA W阴影)Z-Depth(Z轴深度)Normals(法向)Background (背景)Displacement(置换设置)Displacement(置换)Edge Length(pix)(边界长度)4 Max Subdivs(最大细分)256 Amount(数量)1 Relative to bbox(相对边界盒)V iew-Dependent(依靠视图)Tight Bounds(紧密跳跃`)Indirect Illumination(间接照明设置)GI(全局光照)On(开)Reflect Caustics(反射)Refract Caustics(折射)Post-Processing(布置数据处理)Saturation(饱和度)1 Contrast Base(基本对比度)0.5Contrast(对比度)1 Save maps per frame(保存每帖贴图)Primary Engine(首次反弹)Multiplier(倍增)1 Quasi Monte-Carlo(准蒙特卡罗算法)■▲Secondary Engine(二次反弹)Multiplier(倍增)1 Light Cache(灯光缓冲)■▲Quasi-Monte Carlo GI(准蒙特卡罗全局光照设置)■QMC GI(准蒙特卡罗全局光照)Subdivs(细分)8 Secondary Bounces(二次反弹)3Light Cache(灯光缓冲设置)■Calculation Parameters(计算参数)Subdivs(细分)1000 Scale(比例):Screen(屏幕)▲Sample Size(采样大小)0.02 Num.Phases(进程数量)4Store Direct Light(存储直接灯光)Show Calc.Phase(显示计算相位)Adaptive(自适应)Reconstruction Parameters(重建参数)Pre-filter(预滤器)10 Use For Glossy Rays(使用灯光缓冲光滑光线)Filter(过滤):Nearest(接近)▲Interp.Samples(插补采样)5Mode(方式)Single Frame(单帧)Fly Through(通过)Path Tracing(路径跟踪)From File(来自文件)Current Map(当前贴图)Save(保存)Reset(清除)Post Render(渲染后)Don't Delete(不删除)Auto Save(自动保存)Irradiance Map(发光贴图)■Basic Parameters(基本参数)Min Rate(最小比率)-3 Max Rate(最大比率)0 Color Threshold(色彩极限值)0.3 HSph.Subdivs(半球细分)50 Samples(采样)20 Normal Threshold(法线极限值)0.1Distance Threshold(距离极限值)0.1Basic Options(基本选项)Show Calculation Phase(显示计算相位)Show Samples(显示采样点)Show Direct Light(存储直接灯光)Detail enhancement(细节增强)On(开)Scale(比例):Screen(屏幕)▲Radius(半径)60 Subdiv mult(细分倍增)0.3 Advanced Options(高级选项)Interpolation Type(插值类型):Least Squares Fit(最小平方适应)▲Sample Lookup Type(采样查找类型):Density Based(基于密度)▲Calc Samples(计算采样)15 Multipass(多重预计算)Randomize Samples(随机采样)Check Sample Visibility(检查样本可见性)Mode(方式)Single Frame(单帧)Incremental add to current map(添加方式增加到当前贴图)Bucket Mode(块模式)From File(来自文件)Current Map(当前贴图)Save(保存)Reset(清除)Post Render(渲染后)Don't Delete(不删除)Auto Save(自动保存)Photon Map(光子贴图)■Basic Parameters(基本参数)Bounces(反弹)10 Max Photons(最大光子)30Search Distance(搜寻距离)20 Multiplier(倍增)1Retrace Threshold(反射极限值)0 Max Density(最大密度)0Retrace Bounces(反射反弹数)10 Interp. Samples(插值采样)10Convex Hull Estimate(凸起表面区域评估)Store Direct Light(存储直接灯光)Mode(方式)New Map(新贴图)From File(来自文件)Current Map(当前贴图)Save(保存)Reset(清除)0 samples(采样)Post Render(渲染后)Don't Delete(不删除)Auto Save(自动保存)Caustics(散焦设置)Caustics(散焦)On(开)Max Photons(最大光子)50 Multiplier(倍增)1Max Density(最大密度)0 Search Distance(搜索距离)20Mode(方式)New Map(新贴图)From File(来自文件)Current Map(当前贴图)Save(保存)Reset(清除)0 samples(采样)Post Render(渲染后)Don't Delete(不删除)Auto Save(自动保存)Material Editor(材质编辑)Material Preview(材质预览)Update Preview(更新查阅)Material Workspace(材质预览)Scene Materials(场材质)Default_VRay_Material(默认材质)Add material(增加材质)*Add V rayMld(增加VRay专用材质)*Add VRay2SidedMld(增加VRat双面材质)[ Front(正面)Back(背面)Color(颜色)] *Add VRaySKp2SidedMld(增加VRaySKp双面材质)[ Front(正面)Back(背面)] Import new material(导入新材质)Purge unused materials(清除不用的材质)Rename(改名)Remove(移除)Duplicate(副本复制)Import(导入)Export(导出)Select objects by material(选择对象到材质)Apply material to object(s)(应用到物体)Apply material to layer(s)(应用到层)Add new layer(增加新的层)Emissive(发光)Color(颜色)Intensity(强度)1 Transparency(透明度)Reflection(反射)Reflection(反射)Filter(过滤)Highlight Glossiness(高光光泽)1 Reflection Glossiness(反射光泽)1Subdivs(细分)8 Anisotropy(各向异性)0Shader Type(阴影类型):Blinn(材质)▲Rotation(旋转)0Diffuse(漫反射)Color(颜色)Transparency(透明度)Refraction(折射)Refraction(折射)Transparency(透明度)Glossiness(光泽度)1 IOR(折射率)1.55 Subdivs(细分)8Translucency(透明)Translucent(半透明)Fog Color(雾色)Thickness(厚度)1000 Fog Multiplier(雾强度)1Scatter coeff(扩散系数)0 Affect Shadows(影响阴影)Fwd/bck coeff(前向/后向系数)1 Affect Alpha(影响通道)Options(选项)Trace Reflections(追踪反射)Double-Sided(双面)Trace Refractions(追踪折射)Reflect on Backside(内表面反射)Cutoff(终止)0.001 Disable V olume Fog(禁止体积雾)Maps(贴图)Bump(凹凸)Background(背景)Reflection(反射)Displacement(置换)GI(全局光照明)Refraction(折射)Keep continuity(保持连续性)Spotlight(聚光灯)Intensity(强度)On(开)Color(颜色)Multiplier(倍增)1Options(选项)Decay(衰退):Linear(线状的)▲Hardness(坚硬)0.5Sampling(采样)Photon Subdivs(光子细分)500 Caustic Subdivs(腐蚀细分)1000 Shadows(阴影)Enabled(开启)Bias(偏移)0 Radius(半径)0 Subdivs(细分)8Point light(点光源)Intensity(强度)On(开)Color(颜色)Multiplier(倍增)1Options(选项)Decay(衰退):Linear(线状的)▲Sampling(采样)Photon Subdivs(光子细分)500 Caustic Subdivs(腐蚀细分)1000 Shadows(阴影)Enabled(开启)Bias(偏移)0 Radius(半径)0 Subdivs(细分)8Directional light(平行光)Intensity(强度)On(开)Color(颜色)Multiplier(倍增)1Sampling(采样)Photon Subdivs(光子细分)500 Caustic Subdivs(腐蚀细分)1000 Shadows(阴影)Enabled(开启)Bias(偏移)0 Radius(半径)0 Subdivs(细分)8Rectangular light(区域光)Intensity(强度)On(开)Color(颜色)Multiplier(倍增)1Options(选项)Light Portal(光线入口)Invisible(不可见)Double Sided(双面)No Decay(不衰减)Store with Irradiance Map(存储发光贴图)Ignore Light Normals(忽略灯光法向)Sampling(采样)Subdivs(细分)8 Photon Subdivs(光子细分)500 Caustic Subdivs (腐蚀细分)1000Shadows(阴影)Enabled(开启)Bias(偏移)0Linear light(管状光)Color(颜色)On(开)Shadows intensity(阴影厚度)100 Spotlight hardness(聚光灯锐利度)100Displacement(置换)On(开)Advanced controls(高级控制器)Texture(材质)Mapping channel(映射通道)1Displacement(置换)Black point(黑点)0.00 White point(白点)1.00 Ignore creases (忽略皱痕)Subdivision(细分)Subdivide(细分)Contrast(对比度)20% Max steps(最大步幅)4。
20世纪十大数值算法

二十世纪十大数值算法这份算法名单是CiSE (Computing in Science & Engineering) 的两位编辑在 2000 年评出的,基本已经得到大家的认可了。
按时间顺序列出:1. 1946: the Metropolis algorithm, also known as the Monte Carlo method.蒙特卡罗算法, 一年级的时候接触到这个名词. 忘记是"对且很可能快"还是"快且很可能快"了....去随机性ms已经成为很重要的问题....费了半天劲搞出了随机性, 又要处心积虑的去随机化......2. 1947: the simplex method for linear programming. 不懂, 貌似是线性规划方面的3. 1950: Krylov subspace iteration methods. 不懂4. 1951: Formalization of the decompositional approach to matrix computations.好像是矩阵处理方面的东东, 包括LU分解之类的. 如此看来此算法无愧于10大算法. 矩阵在符号计算方面的重要性不言而喻嘛5. 1957: Development of the Fortran optimizing compiler. 即使现在, 科学计算领域还有一席之地6. 1959–61: QR algorithm. 计算矩阵特征值.7. 1962: Quick sort. 快速排序8. 1965: FFT (Fast Fourier Transform) . 快速傅立叶变换....,9. 1977: Integer relation detection algorithm. 不懂.10. 1987: Fast multipole algorithm. 依然不懂...........The Top Ten Algorithms of the CenturyJack Dongarra and Francis Sullivan published a list of "The Top Ten Algorithms of the Century." Their list included:1.The Monte Carlo method or Metropolis algorithm, devised by John von Neumann, Stanislaw Ulam,and Nicholas Metropolis;2.The simplex method of linear programming, developed by George Dantzig;3.The Krylov Subspace Iteration method, developed by Magnus Hestenes, Eduard Stiefel, andCornelius Lanczos;4.The Householder matrix decomposition, developed by Alston Householder;5.The Fortran compiler, developed by a team lead by John Backus;6.The QR algorithm for eigenvalue calculation, developed by J Francis;7.The Quicksort algorithm, developed by Anthony Hoare;8.The Fast Fourier Transform, developed by James Cooley and John Tukey;9.The Integer Relation Detection Algorithm, developed by Helaman Ferguson and Rodney Forcade;(given N real values X I, is there a nontrivial set of integer coefficients A I so that sum ( 1 <= I <= N )A I * X I = 0?10.The fast Multipole algorithm, developed by Leslie Greengard and Vladimir Rokhlin; (to calculategravitational forces in an N-body problem normally requires N^2 calculations. The fast multipole method uses order N calculations, by approximating the effects of groups of distant particles using multipole expansions)Reference 1:Dongarra and Sullivan,Top Ten Algorithms of the Century,Computing in Science and Engineering,January/February 2000.Reference 2:Barry Cipra,The Best of the 20th Century: Editors Name Top 10 AlgorithmsSIAM News,Volume 33, Number 4, May 2000, page 1.。
计算材料学概述 之 蒙特卡洛方法.详解
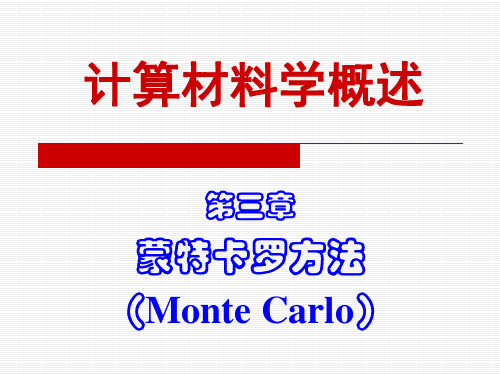
随机数产生的办法
关于随机数的几点注意
注1 由于均匀分布的随机数的产生总是采用某个确定 的模型进行的,从理论上讲,总会有周期现象出现的。 初值确定后,所有随机数也随之确定,并不满足真正 随机数的要求。因此通常把由数学方法产生的随机数 成为伪随机数。 但其周期又相当长,在实际应用中几乎不可能出 现。因此,这种由计算机产生的伪随机数可以当作真 正的随机数来处理。 注2 应对所产生的伪随机数作各种统计检验,如独 立性检验,分布检验,功率谱检验等等。
考虑平面上的一个边长为1的正方形及其内部的一个形状不规 则的“图形”,如何求出这个“图形”的面积呢?Monte Carlo方法是这样一种“随机化”的方法:向该正方形“随机 地”投掷N个点,若有M个点落于“图形”内,则该“图形” 的面积近似为M/N。
用该方法计算π的基本思路是: 1 、根据圆面积的公式: s=πR^2 ,当R=1时,
11
面积的计算
辛普逊方法
蒙特-卡洛方法
在长方形中均匀投N0组(x,y) 如 y<f(x), 则 N=N+1
I = ΣSn
f (x)
Hale Waihona Puke I =(N/N0)×S0f (x)
S0
S
x x
MC 的优点 MC与传统数学方法相比,具有直观性强,简便易行的优点,该方
法能处理一些其他方法无法解决的负责问题,并且容易在计算机 上实现,在很大程度上可以代替许多大型、难以实现的复杂实验 和社会行为。无污染、无危险、能摆脱实验误差。
Monte Carlo方法之随机数的产生
许多计算机系统都有随机数生成函数 F90: call random_seed
call random_number(a) 2、ISEED=RTC()
Monte_Carlo cadence蒙特卡洛教程

Purpose
To describe the recommended methodology for process and device modeling, including process and mismatch parameter variations, to be used in conjunction with the Monte Carlo analysis capability of the Virtuoso® Spectre® circuit simulator.
Recommended Monte Carlo Modeling Methodology for Virtuoso Spectre Circuit Simulator Application Note
Product Version 5.0 November 2003
ห้องสมุดไป่ตู้
2004 Cadence Design Systems, Inc. All rights reserved. Printed in the United States of America. Cadence Design Systems, Inc., 555 River Oaks Parkway, San Jose, CA 95134, USA Trademarks: Trademarks and service marks of Cadence Design Systems, Inc. (Cadence) contained in this document are attributed to Cadence with the appropriate symbol. For queries regarding Cadence’s trademarks, contact the corporate legal department at the address shown above or call 800.862.4522. All other trademarks are the property of their respective holders. Restricted Print Permission: This publication is protected by copyright and any unauthorized use of this publication may violate copyright, trademark, and other laws. Except as specified in this permission statement, this publication may not be copied, reproduced, modified, published, uploaded, posted, transmitted, or distributed in any way, without prior written permission from Cadence. This statement grants you permission to print one (1) hard copy of this publication subject to the following conditions: 1. The publication may be used solely for personal, informational, and noncommercial purposes; 2. The publication may not be modified in any way; 3. Any copy of the publication or portion thereof must include all original copyright, trademark, and other proprietary notices and this permission statement; and 4. Cadence reserves the right to revoke this authorization at any time, and any such use shall be discontinued immediately upon written notice from Cadence. Disclaimer: Information in this publication is subject to change without notice and does not represent a commitment on the part of Cadence. Except as may be explicitly set forth in such agreement, Cadence does not make, and expressly disclaims, any representations or warranties as to the completeness, accuracy or usefulness of the information contained in this document. Cadence does not warrant that use of such information will not infringe any third party rights, nor does Cadence assume any liability for damages or costs of any kind that may result from use of such information. Restricted Rights: Use, duplication, or disclosure by the Government is subject to restrictions as set forth in FAR52.227-14 and DFAR252.227-7013 et seq. or its successor.
蒙特卡罗方法计算三重积分
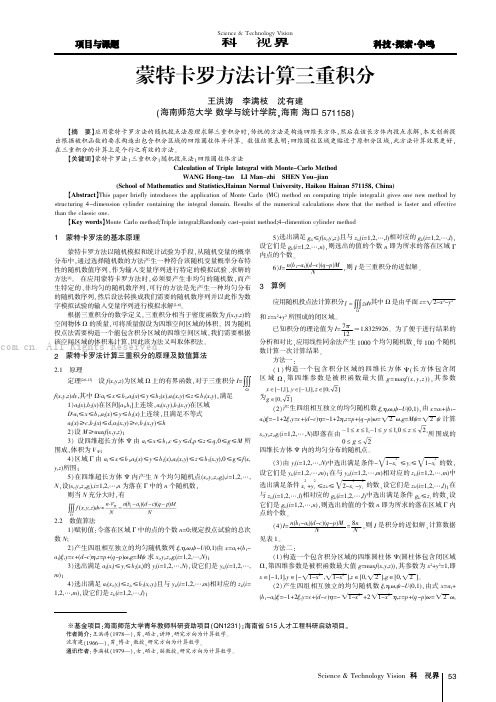
Science &Technology Vision 科技视界1蒙特卡罗法的基本原理蒙特卡罗方法以随机模拟和统计试验为手段,从随机变量的概率分布中,通过选择随机数的方法产生一种符合该随机变量概率分布特性的随机数值序列,作为输入变量序列进行特定的模拟试验、求解的方法[1]。
在应用蒙特卡罗方法时,必须要产生非均匀的随机数,而产生特定的、非均匀的随机数序列,可行的方法是先产生一种均匀分布的随机数序列,然后设法转换成我们需要的随机数序列并以此作为数字模拟试验的输入变量序列进行模拟求解[2-6]。
根据三重积分的数学定义,三重积分相当于密度函数为f (x ,y ,z )的空间物体Ω的质量,可将质量假设为四维空间区域的体积。
因为随机投点法需要构造一个能包含积分区域的四维空间区域,我们需要根据该空间区域的体积来计算,因此该方法又叫取体积法。
2蒙特卡罗法计算三重积分的原理及数值算法2.1原理定理[2,6-13]设f (x ,y ,z )为区域Ω上的有界函数,对于三重积分I=f (x ,y ,z )dv ,其中Ω∶a 1≤x ≤b 1,a 2(x )≤y ≤b 2(x ),a 3(x ,y )≤z ≤b 3(x ,y ),满足1)a 2(x ),b 2(x )在区间[a 1,b 1]上连续,a 3(x ,y ),b 3(x ,y )在区域D ∶a 1≤x ≤b 1,a 2(x )≤y ≤b 2(x )上连续,且满足不等式a 2(x )≥c ,b 2(x )≤d,a 3(x ,y )≥e,b 3(x ,y )≤h 2)设M ≥max f (x ,y ,z );3)设四维超长方体Ψ由a 1≤x ≤b 1,c ≤y ≤d,p ≤z ≤q,0≤g ≤M 所围成,体积为V Ψ;4)区域Γ由a 1≤x ≤b 1,a 2(x )≤y ≤b 2(x ),a 3(x ,y )≤z ≤b 3(x ,y ),0≤g≤f (x,y,z )所围;5)在四维超长方体Ψ内产生N 个均匀随机点(x i ,y i ,z i ,g i ),i =1,2,…,N ,设(x i ,y i ,z i ,g i ),i =1,2,…,n 为落在Γ中的n 个随机数,则当N 充分大时,有2.2数值算法1)赋初值:令落在区域Γ中的点的个数n =0;规定投点试验的总次数N ;2)产生四组相互独立的均匀随机数列ξ,η,ω,ψ~U (0,1)由x =a 1+(b 1-a 1)ξ,y =c +(d-c )η,z =p +(q-p )ω,g =Mψ求x i ,y i ,z i ,g i (i =1,2,…,N );3)选出满足a 2(x i )≤y i ≤b 2(x i )的y i (i =1,2,…,N ),设它们是y ki (i =1,2,…,m );4)选出满足a 3(x i ,y i )≤z ki ≤b 3(x i ,y i )且与y ki (i =1,2,…,m )相对应的z ki (i =1,2,…,m ),设它们是z ki (i =1,2,…,l );5)选出满足g ki ≤f (x i ,y i ,z i )且与z ki (i =1,2,…,l )相对应的g ki (i =1,2,…,l ),设它们是g ki (i =1,2,…,n ),则选出的值的个数n 即为所求的落在区域Γ内点的个数。
外国对定积分的计算文献
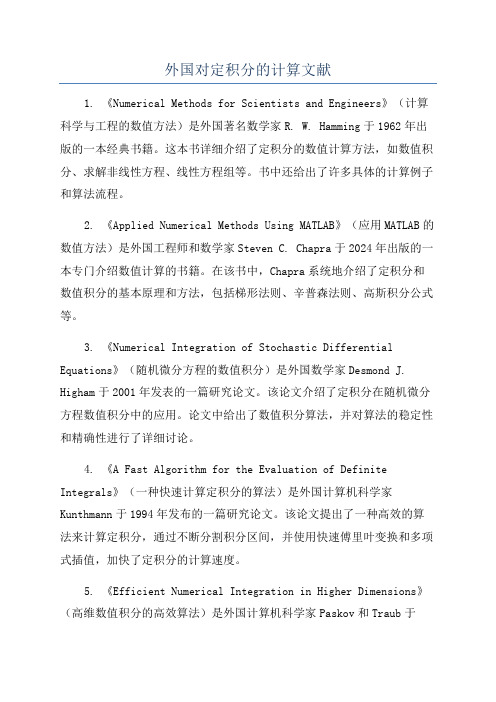
外国对定积分的计算文献1. 《Numerical Methods for Scientists and Engineers》(计算科学与工程的数值方法)是外国著名数学家R. W. Hamming于1962年出版的一本经典书籍。
这本书详细介绍了定积分的数值计算方法,如数值积分、求解非线性方程、线性方程组等。
书中还给出了许多具体的计算例子和算法流程。
2. 《Applied Numerical Methods Using MATLAB》(应用MATLAB的数值方法)是外国工程师和数学家Steven C. Chapra于2024年出版的一本专门介绍数值计算的书籍。
在该书中,Chapra系统地介绍了定积分和数值积分的基本原理和方法,包括梯形法则、辛普森法则、高斯积分公式等。
3. 《Numerical Integration of Stochastic Differential Equations》(随机微分方程的数值积分)是外国数学家Desmond J. Higham于2001年发表的一篇研究论文。
该论文介绍了定积分在随机微分方程数值积分中的应用。
论文中给出了数值积分算法,并对算法的稳定性和精确性进行了详细讨论。
4. 《A Fast Algorithm for the Evaluation of Definite Integrals》(一种快速计算定积分的算法)是外国计算机科学家Kunthmann于1994年发布的一篇研究论文。
该论文提出了一种高效的算法来计算定积分,通过不断分割积分区间,并使用快速傅里叶变换和多项式插值,加快了定积分的计算速度。
5. 《Efficient Numerical Integration in Higher Dimensions》(高维数值积分的高效算法)是外国计算机科学家Paskov和Traub于1995年发表的文章。
该文章介绍了一种高效的算法来计算高维定积分,通过利用Monte Carlo方法和复合辛普森法则,提高了高维定积分的计算效率。
《工程数值计算Python教程》第9章 Monte Carlo模拟

count = np.sum(a < 0.5)
print('a: ', a)
print('count: ', count)
【例9-2】随机产生圆 2 + 2 = 1中均匀分布的500个随机点,并画图展示。
有三种不同思路求解该问题。
(1) 由于圆位于矩形−1 ≤ ≤ 1,−1 ≤ ≤ 1
(3) normal方法用于产生正态(高斯)分布的随机数,如果一个随机变量符合期望值
(均值)为、方差为 2 的正态分布,则其概率密度函数为:
1
−
=
exp −
2 2
2
Байду номын сангаас
2
正态分布通常记为 , 2 ,当 = 0, = 1时称为标准正态分布。normal用法为:
normal(loc=0.0, scale=1.0, size=None)
参数seed用于设置随机数发生器的种子,默认值为None,此时算法会由操作系统产生
新的种子。但有时需要产生完全相同的随机数序列,可将seed设置为某一固定的整数
值即可。
default_rng方法返回一个随机数发生器Generator的实例,随机数发生器包含许多方法
以产生各种分布的随机数,主要包括:
plt.xlabel('x')
plt.ylabel('y')
plt.show()
(2) 第(1)种方法会产生一些最终被丢弃的点。
rng = np.random.default_rng()
其实,当确定后,的取值范围就确定了,
n = 500
如果先随机产生,再按的取值范围随机产
蒙特卡洛计算

蒙特卡洛计算
蒙特卡洛计算(Monte Carlo calculation)是一种基于随机数的
数值计算方法,在很多领域中被广泛应用。
它最早由美国物理学家Nicholas Metropolis、Stanislaw Ulam和John von Neumann在1940年代发展起来,是以蒙特卡洛赌场而得名。
蒙特卡洛计算的基本思想是利用统计学上的随机抽样方法,通过生成大量的随机数来近似求解问题。
它通常包括以下步骤:
1. 定义问题:将实际问题转化为数学模型,并确定需要计算的目标。
2. 生成随机数:根据需要生成符合特定分布或规律的随机数。
这些随机数可以用来模拟系统状态的变化或者参与概率计算。
3. 运行模拟:根据问题模型和生成的随机数,进行一系列的模拟实验。
这些实验可能是基于概率的取样、随机游走、随机漫步等方法。
4. 统计分析:对模拟的结果进行统计分析,得到对问题求解的近似值。
常见的统计分析方法包括平均值、方差、置信区间等。
蒙特卡洛计算在众多领域中都有应用,如物理学、金融学、工程学、计算机科学等。
它可以用于求解复杂的数学问题,模拟物理系统的行为,进行风险分析和优化等。
蒙特卡洛计算的优点是灵活性高,适用于处理各种复杂、难以
精确求解的问题,其结果也能够提供一定的近似精度。
但它也存在着计算量大、计算速度较慢的问题,并且结果的准确性和稳定性可能受到随机数生成的影响。
蒙特卡罗方法计算高纯锗探测器的全能峰效率
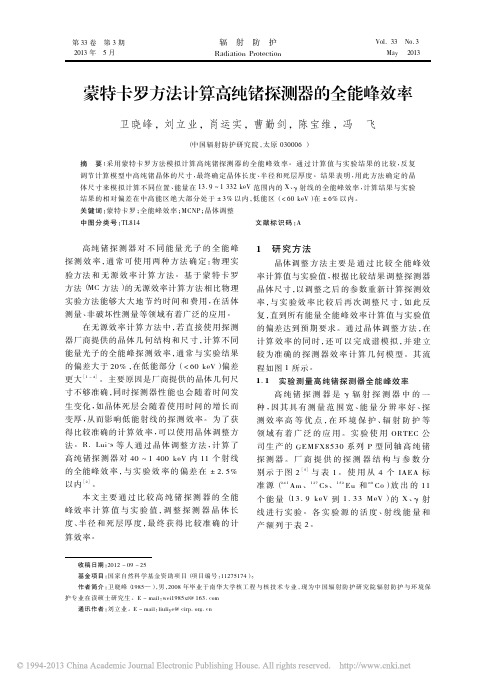
关键词: 蒙特卡罗; 全能峰效率; MCNP; 晶体调整
中图分类号: TL814
文献标识码: A
高纯 锗 探 测 器 对 不 同 能 量 光 子 的 全 能 峰 探测 效 率,通 常 可 使 用 两 种 方 法 确 定: 物 理 实 验方法 和 无 源 效 率 计 算 方 法。 基 于 蒙 特 卡 罗 方法( MC 方法) 的无源效率计算方法相比物理 实验方法能够大 大 地 节 约 时 间 和 费 用,在 活 体 测 量 、非 破 坏 性 测 量 等 领 域 有 着 广 泛 的 应 用 。
核素
活度1) ( kBq) 能量( keV)Leabharlann 241 Am 137 Cs
332. 9 211. 1
13. 9 17. 5 21. 0 26. 3 59. 5 661. 7
1) 各核素活度为实验时的活度。
产额( % )
16. 2 16. 1 3. 3 2. 4 35. 9 84. 7
核素 152 Eu 60 Co
通 过 Gamma Vision 测 量 软 件 记 录 并 分 析
测量结 果。 当 得 到 不 同 测 量 位 置 处 不 同 能 量
射 线 的 全 能 峰 净 计 数 率 后,通 过 公 式 ε =
净计数率 C 活度 A × 产额
η
即
可
得
到
全
能
峰
效
率
的
实
验值。
1. 2 蒙特卡罗模拟计算
MCNP 是由美国洛斯阿拉莫斯国家实验室
表 1 厂商提供的探测器主要参数 Tab. 1 Specification of detector provided by manufacture
- 1、下载文档前请自行甄别文档内容的完整性,平台不提供额外的编辑、内容补充、找答案等附加服务。
- 2、"仅部分预览"的文档,不可在线预览部分如存在完整性等问题,可反馈申请退款(可完整预览的文档不适用该条件!)。
- 3、如文档侵犯您的权益,请联系客服反馈,我们会尽快为您处理(人工客服工作时间:9:00-18:30)。
Fast Monte Carlo calculation of scatter corrections for CBCT imagesErnesto Mainegra-Hing and Iwan KawrakowIonizing Radiation Standards,National Research Council of Canada,Ottawa K1A0R6,CanadaE-mail:mainegra@irs.phy.nrc.caAbstract.A very fast Monte Carlo algorithm for the calculation of the scatter contributionin cone beam computed tomography,implemented within the EGSnrc framework,is presented.Based on the combination of several variance reduction techniques,an efficiency improvement ofthree orders of magnitude over an analog simulation is obtained.A denoising algorithm appliedto the computed scatter distribution is shown to further increase the efficiency of the calculationby about a factor of10.An iterative scatter correction algorithm is proposed and its feasibilityis demonstrated on three different phantoms.1.IntroductionIn cone beam computed tomography(CBCT)scatter corrections are commonly performed experimentally by increasing the object-detector air-gap,using anti-scatter grids,or combining both.These methods can reduce the scatter in some cases,but also reduce the primary signal intensity.Beam stop arrays(BSA)techniques have been shown to effectively reduce the scatter-primary ratio by a factor of14[1].However,BSA techniques are not practical since two acquisitions per angle are required increasing the patient dose and acquisition time.Algorithmic methods using analytical models have been also used which are fast,but are of limited use when complex geometries or heterogenous media are involved.An attractive alternative is to use Monte Carlo(MC)simulations to obtain a more accurate estimate of the scatter distribution.However,previous attempts in the literature report extremely long calculation times[2].The aim of the algorithm introduced in this paper is to correct a CBCT scan taken under full scatter conditions without previous correction.Extensive use of variance reduction techniques(VRT)for efficient photon transport,denoising the scatter distribution,and a new approach for removing the scatter from the measured scan,bring this type of approach closer to on-line clinical applicability.2.Methods2.1.DefinitionsA CBCT scan consists of a set of projections onto a2D detector array around the volume of interest in a180o or360o orbit.If R i denotes the reading in pixel i for a given projection and S i the corresponding scatter contribution to the signal,then the quantitya i=lnb iA i=lnb iR i−S i=µ(l)dl(1)is the attenuation along a line connecting the x-ray source with the detecting element i .The blank scan b i is the signal at pixel i without the phantom and µis the attenuation coefficient at position l along the line.If the scatter contribution S i is known,then the scatter-free scan a i (or A i )can be computed according to Eq.(1)and given to an image reconstruction algorithm to obtain the 3D distribution of attenuation coefficients in the phantom.The quantity actually measured by the scanner is r i =ln b i R i.(2)In what follows s i =S i /b i is a short hand notation and symbols with a tilde denote MC computed quantities,e.g.˜r i is the real scan obtained from the MC simulation,˜Si the scatter,etc.Because the main purpose of this investigation is to assess VRT in the MC simulation and the iterative scatter correction algorithm described in the next section,only scans generated by a MC simulation are used in this paper,a very simple x-ray source is employed (a monoenergetic 60keV point source)and the detector response is assumed to be given by e of measured scans,a realistic photon source,and a realistic detector response is left for future investigations.2.2.Iterative scatter correction algorithmThe proposed iterative scatter correction algorithm works as follows:(i)Set a i =r i(ii)Reconstruct the phantom using the current estimate of a i .A filtered back-projectionalgorithm due to Feldkamp,Davis and Kress[3]is used in this step.(iii)Compute the scatter-free scan ˜Ai and the scatter ˜S i corresponding to this phantom (iv)Perform a scatter correction.The first approach considered isa i =(1+α)r i −α˜r i −ln ˜A i ˜Ai +˜S i (3)with αa relaxation parameter.The second approach is defined via a i =r i −ln 1−˜s i exp (βr i +(1−β)˜r i )(4)with βa free parameter.(v)Go to step (ii).The iteration is stopped when a convergence has been reached,with a suitable termination criterion currently under investigation.In all examples presented in this paper a manual inspection after each iteration is used.Eq.(3)is easily obtained from Eq.(1)by replacing A i with ˜A i ·R i /˜R i ,assuming that major differences in R i and ˜Ri are given by differences in their attenuation properties.Finally a relaxation term α·(r i −˜r i )is introduced to compensate for errors introduced by this assumption.Eq.(4)is Eq.(1)rewritten as a function of r i ,where s i has been replaced by ˜s i and a relaxation term β·(r i −˜r i )added to the exponent.It is easy to see that when the computed quantities approach the real ones,both methods recover the actual scatter-free scan given in Eq.(1).The advantage of Eq.(3)is that it is numerically stable,its disadvantage is a relatively slow convergence.The advantage of Eq.(4)is a very fast convergence,its main disadvantage is the fact that small errors in the scatter estimate lead to large errors in a i in regions with large attenuation (rge r i )as seen from the following equation (β=1is used for the sake of simplicity):∆a i =x 1−x ∆˜S i ˜Si ,x =˜s i exp(r i )(5)and from the fact that x approaches unity for large r i .pixel/AU s c a t t e r (A U )Figure parison between the scatter distribution from cal-culations with a negligible uncer-tainty (black line),a large statisti-cal uncertainty obtained in 15sec-onds of CPU time (red symbols)and the fast calculation after de-noising (blue line)for the phantom described in section 3.1.2.3.Fast Monte Carlo simulation of photon transportpixels / AU µ / c m -1pixels / AUµ / c m -1Figure 2.Profile across the middle slice of the reconstructed attenuation image of a water cylinder (10cm radius,20cm length).The scatter correction algorithm defined in Eq.(3)is used.The left panel shows the result without,the right panel with,denoising.A new EGSnrc [4]user code,egs cbct ,is developed for the purposes of this investigation.egs cbct is written in C++and uses the EGSnrc C++class library [5].Air-kerma at the detector plane is scored efficiently using a track-length estimation,whereby all photons crossing the scoring plane contribute to the kerma.This has been shown elsewhere [6]to be about 20times more efficient than computing kerma by switching offelectron transport and collecting the energy deposited by electrons on the spot.To further improve the efficiency of the kerma estimator,use is made of a VRT known as forced detection.Every time a photon direction is aimed at the scoring field,its contribution from the current position is scored,including the effect of attenuation through the geometry.Interaction splitting combined with Russian Roulette (RR)is employed to increase the number of photons directed towards the detector array while reducing the time spent following photons moving away from the scoring field.Woodcock tracing (a.k.a.delta transport)is also implemented to speed up the transport of those few photons aimed away from the scoring plane that survived RR.Since the forced detection technique introduces large fluctuations in the weights of the photons reaching the scoring plane,a sophisticated scheme is devised,which consist of path-length biasing combined with position dependent splitting numbers to ensure nearly constant weights of the photons contributing to the score.It is worthnoting that all these techniques,to be described in more detail in a forthcoming publication,are true VRT,i.e.they don’t change the result.To ensure their correct implementation,comparisons with analog simulations were performed and no statistically significant differences were found. The combined efficiency gain is about a factor of200compared to track-length estimation.To further decrease the simulation time,the scatter scan˜S i is subjected to denoising using a2D version of the locally-adaptive Savitzky-Goley(LASG)filter presented in Ref.[7].Due to the fact that in general the scatter distribution is a“well behaved”function without sharp discontinuities,the denoising algorithm performs very well as illustrated in Fig.1.Figure 3.A30cm3water phantom with 5bone inserts as modeled with the EGSnrc C++class library.pixels / AU12345678ln(blank/scan)ideal scanreal scaniteration 1iteration 5iteration 0Figure4.Iterative correction shown for a profile across middle slice(64×64×64,0.5 cm voxels)for60keV x-ray beam on30cm3 water phantom.Reconstruction done with72 projections.3.Results3.1.A water cylinderAs afirst test of the proposed scatter correction algorithm a simple homogeneous water cylinder (20cm diameter×20cm length)is considered with the x-ray source rotating around the symmetry axis.Since the phantom is cylindrically symmetric,it is sufficient to compute the0o projection only,obtaining all other projections by simply copying the0o scan(128projections are used in this example).In thisfirst test the correction method given in Eq.(3)withα=0 is employed.Figure2(left panel)shows a profile through the middle slice of the reconstructed attenuation image after the0’th iteration(ing the uncorrected scans r i),1’st and5’th iterations.One can see how with each iteration step,the values of the attenuation coefficient approach the correct value ofµ(60keV)=0.21cm−1.The largefluctuations observed in the middle of the profile are due to the strong correlation when re-using the0o projection for all 128projections.The central points along the rotation axis are the most affected since exactly the same points are used in the reconstruction for this region.If one uses the LASG denoising algorithm to reduce the statistical noise,a much smoother profile is obtained and a faster convergence to the correct value of the attenuation coefficient is observed,as can be seen in the right panel offigure2.(a)(b)(c)(d)Figure 5.0o scans of a water cube with 5bone inserts with a 64×64detector.(a)Initial uncorrected scan with full scatter.(b)Scatter-free scan obtained from direct simulation which is the goal of the scatter corrections.(c)Result of correcting the scatter without smoothing.(d)A smoothing procedure is used with 10times less histories as when not smoothing.3.2.Water cube with 5bone rodsA more challenging arrangement is a 30cm 3water cube with five bone inserts as shown in figure3.In this case the correction algorithm given in Eq.(3)with α=0converges too ing α=1initially improves the convergence rate,but after 6iteration the change in each subsequent iteration is very small.Fig.4shows a comparison between the scatter-free scan (black line)and the estimated scatter-free scans after 0,1and 5iterations along a line in the 0o scan.The measured scan (used to obtain the phantom in the 0’th iteration)is also shown with the blue line.The influence of denoising is illustrated in Fig.5,which shows the full-scatter scan (a),scatter-free scan (b),and reconstructed scatter-free scans after 5iterations without (c)and with (d)denoising.The image in Fig.5d is obtained with 10times fewer particle histories compared to Fig.5c,thus indicating that denoising brings another factor of 10improvement in simulation speed.pixels / AU 0.200.220.240.260.280.300.320.340.360.380.40µ / c m -1actual phantom reconstructed phantom Figure 6.The middle slice of the reconstructed attenuation image (left panel)and a profile through the center (right panel)of the phantom described in section 3.3.3.3.Water sphere with spherical inserts of different compositionThe FDK reconstruction algorithm employed in this study introduces significant artifacts near sharp edges.As a consequence,the actual phantom composition in these regions is not correctly reproduced.This in turn introduces errors in the estimated scatter distributions which are exponentially amplified in regions with significant attenuation according to equation 5.Therefore,a geometry with smooth boundaries,which is also more representative for practical applications than the water box with bone inserts from the previous section,is considered as a final example:a15cm radius water sphere with four spherical inserts of varying composition (ρ=1.1,1.5,1.6,2.0g/cm3).The scatter correction algorithm defined in Eq.(4)withβ=0.5 is employed.However,it is is found that the scatter distribution computed from a voxelized representation of the phantom must be corrected before use in Eq.(4).The correction is a simple shift determined from the requirement that the computed scan reproduces the measured scan in regions with no attenuation(i.e.regions that are not behind the scanned object)Figure 6shows a profile across a central slice of the reconstructed sphere after the third iteration.In this case the algorithm converges faster and is also capable of faithfully reproducing the inserts of different density.4.ConclusionsThe proposed approach to correct CBCT images for scatter using only the results of a Monte Carlo simulation is shown to correctly reproduce the attenuation coefficients.A new EGSnrc code named egs cbct for performing CBCT scatter computations is developed.The set of variance reduction techniques implemented in egs cbct increases the efficiency of the simulation by a factor of4000compared to a fully analog e of denoising for the computed scatter distribution reduces the simulation time by another factor of10.To put this in perspective,if one were to repeat the calculations described by Jarry et al[2]using egs cbct,the calculation time would be39seconds instead of the430hours reported.Due to the limitations of the FDK reconstruction algorithm,objects with round boundaries can be better reproduced than objects with sharp edges.The investigations presented in this paper show that using the signal beyond the objects dimensions as representative of the actual scatter to shift the computed scatter distribution,together with the correction method defined in Eq.(4)produces the best convergence and contrast.Since results reported here can be considered only preliminary, extensive benchmarking of the algorithm with real CBCT images,and a better model of the x-ray source and detector array is needed.AcknowledgmentsWe would like to thank Dr.Glenn Wells of the Ottawa Heart Institute for kindly providing the FDK image reconstruction algorithm used in our study.References[1]Ning R,Tang X and Conover D2004Med.Phys.311195–1202[2]Jarry G,Graham S A,Moseley D J,Jaffray D A,Siewerdsen J H and Verhaegen F2006Med.Phys.334320–4329[3]Feldkamp L A,Davis L C and Kress J W1984J.Opt.Soc.Am.A1612–619[4]Kawrakow I2000Med.Phys.27485–498[5]Kawrakow I2005EGSnrc C++class library Technical Report PIRS–898National ResearchCouncil of Canada Ottawa,Canada[6]Mainegra-Hing E and Kawrakow I2006Med.Phys.333340–3347[7]Kawrakow I2002Phys.Med.Biol.473087–3104。