2019 Why Do Adversarial Attacks Transfer Explaining Transferability of Evasion and Poisoning Attacks
多模态翻译理论与实践研究读书笔记
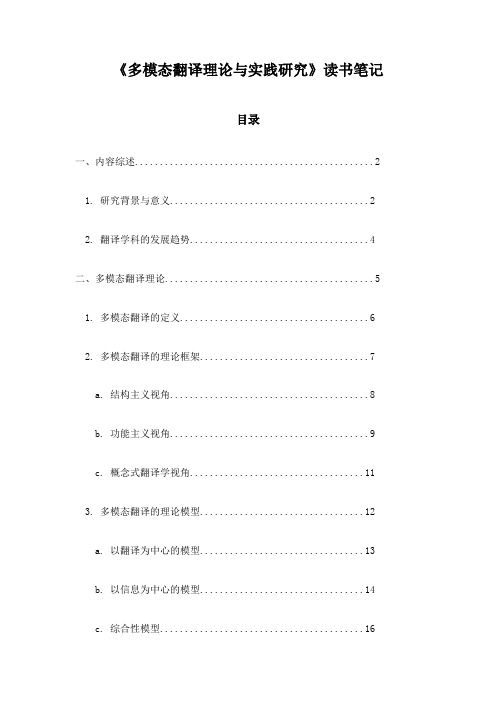
《多模态翻译理论与实践研究》读书笔记目录一、内容综述 (2)1. 研究背景与意义 (2)2. 翻译学科的发展趋势 (4)二、多模态翻译理论 (5)1. 多模态翻译的定义 (6)2. 多模态翻译的理论框架 (7)a. 结构主义视角 (8)b. 功能主义视角 (9)c. 概念式翻译学视角 (11)3. 多模态翻译的理论模型 (12)a. 以翻译为中心的模型 (13)b. 以信息为中心的模型 (14)c. 综合性模型 (16)三、多模态翻译实践 (17)1. 多模态翻译的应用领域 (18)a. 文化交流与传播 (20)b. 语言教学 (20)c. 人工智能辅助翻译 (22)2. 多模态翻译的案例分析 (23)a. 跨文化交际中的多模态翻译 (25)b. 语言教学中的多模态翻译实践 (26)c. 人工智能辅助翻译系统的设计与应用 (27)四、多模态翻译的挑战与对策 (28)1. 技术挑战 (29)a. 自然语言处理技术 (31)b. 计算机辅助翻译工具 (32)2. 理论挑战 (33)a. 翻译理论的创新与发展 (34)b. 多模态翻译的哲学思考 (36)3. 实践挑战 (37)a. 教育培训的需求分析 (38)b. 企业跨文化交流与合作 (39)五、结论 (40)1. 研究成果总结 (41)2. 研究展望与建议 (42)一、内容综述《多模态翻译理论与实践研究》是一本深入探讨多模态翻译理论和实践的学术著作。
本书通过对多模态翻译的全面分析,揭示了其在跨文化交流中的重要作用和广阔的应用前景。
在内容综述部分,作者首先回顾了多模态翻译的基本概念和理论框架,包括多模态翻译的定义、特点、分类以及多模态翻译的理论基础等。
作者详细介绍了多模态翻译在不同领域的应用实践,如翻译学术文献、广告语、影视作品等,并分析了多模态翻译所面临的挑战和问题,如跨文化交际障碍、语言结构差异等。
作者还探讨了多模态翻译与语义翻译、交际翻译等翻译理论的关系,强调了多模态翻译在促进跨文化交流、增进不同文化理解和尊重方面的重要作用。
交通流
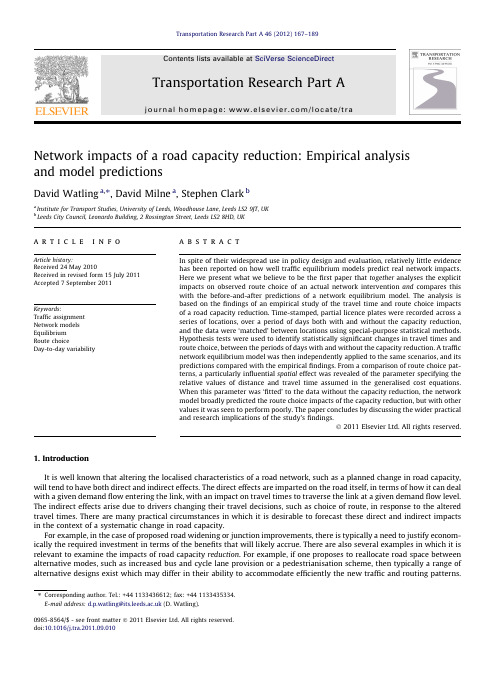
Network impacts of a road capacity reduction:Empirical analysisand model predictionsDavid Watling a ,⇑,David Milne a ,Stephen Clark baInstitute for Transport Studies,University of Leeds,Woodhouse Lane,Leeds LS29JT,UK b Leeds City Council,Leonardo Building,2Rossington Street,Leeds LS28HD,UKa r t i c l e i n f o Article history:Received 24May 2010Received in revised form 15July 2011Accepted 7September 2011Keywords:Traffic assignment Network models Equilibrium Route choice Day-to-day variabilitya b s t r a c tIn spite of their widespread use in policy design and evaluation,relatively little evidencehas been reported on how well traffic equilibrium models predict real network impacts.Here we present what we believe to be the first paper that together analyses the explicitimpacts on observed route choice of an actual network intervention and compares thiswith the before-and-after predictions of a network equilibrium model.The analysis isbased on the findings of an empirical study of the travel time and route choice impactsof a road capacity reduction.Time-stamped,partial licence plates were recorded across aseries of locations,over a period of days both with and without the capacity reduction,and the data were ‘matched’between locations using special-purpose statistical methods.Hypothesis tests were used to identify statistically significant changes in travel times androute choice,between the periods of days with and without the capacity reduction.A trafficnetwork equilibrium model was then independently applied to the same scenarios,and itspredictions compared with the empirical findings.From a comparison of route choice pat-terns,a particularly influential spatial effect was revealed of the parameter specifying therelative values of distance and travel time assumed in the generalised cost equations.When this parameter was ‘fitted’to the data without the capacity reduction,the networkmodel broadly predicted the route choice impacts of the capacity reduction,but with othervalues it was seen to perform poorly.The paper concludes by discussing the wider practicaland research implications of the study’s findings.Ó2011Elsevier Ltd.All rights reserved.1.IntroductionIt is well known that altering the localised characteristics of a road network,such as a planned change in road capacity,will tend to have both direct and indirect effects.The direct effects are imparted on the road itself,in terms of how it can deal with a given demand flow entering the link,with an impact on travel times to traverse the link at a given demand flow level.The indirect effects arise due to drivers changing their travel decisions,such as choice of route,in response to the altered travel times.There are many practical circumstances in which it is desirable to forecast these direct and indirect impacts in the context of a systematic change in road capacity.For example,in the case of proposed road widening or junction improvements,there is typically a need to justify econom-ically the required investment in terms of the benefits that will likely accrue.There are also several examples in which it is relevant to examine the impacts of road capacity reduction .For example,if one proposes to reallocate road space between alternative modes,such as increased bus and cycle lane provision or a pedestrianisation scheme,then typically a range of alternative designs exist which may differ in their ability to accommodate efficiently the new traffic and routing patterns.0965-8564/$-see front matter Ó2011Elsevier Ltd.All rights reserved.doi:10.1016/j.tra.2011.09.010⇑Corresponding author.Tel.:+441133436612;fax:+441133435334.E-mail address:d.p.watling@ (D.Watling).168 D.Watling et al./Transportation Research Part A46(2012)167–189Through mathematical modelling,the alternative designs may be tested in a simulated environment and the most efficient selected for implementation.Even after a particular design is selected,mathematical models may be used to adjust signal timings to optimise the use of the transport system.Road capacity may also be affected periodically by maintenance to essential services(e.g.water,electricity)or to the road itself,and often this can lead to restricted access over a period of days and weeks.In such cases,planning authorities may use modelling to devise suitable diversionary advice for drivers,and to plan any temporary changes to traffic signals or priorities.Berdica(2002)and Taylor et al.(2006)suggest more of a pro-ac-tive approach,proposing that models should be used to test networks for potential vulnerability,before any reduction mate-rialises,identifying links which if reduced in capacity over an extended period1would have a substantial impact on system performance.There are therefore practical requirements for a suitable network model of travel time and route choice impacts of capac-ity changes.The dominant method that has emerged for this purpose over the last decades is clearly the network equilibrium approach,as proposed by Beckmann et al.(1956)and developed in several directions since.The basis of using this approach is the proposition of what are believed to be‘rational’models of behaviour and other system components(e.g.link perfor-mance functions),with site-specific data used to tailor such models to particular case studies.Cross-sectional forecasts of network performance at specific road capacity states may then be made,such that at the time of any‘snapshot’forecast, drivers’route choices are in some kind of individually-optimum state.In this state,drivers cannot improve their route selec-tion by a unilateral change of route,at the snapshot travel time levels.The accepted practice is to‘validate’such models on a case-by-case basis,by ensuring that the model—when supplied with a particular set of parameters,input network data and input origin–destination demand data—reproduces current mea-sured mean link trafficflows and mean journey times,on a sample of links,to some degree of accuracy(see for example,the practical guidelines in TMIP(1997)and Highways Agency(2002)).This kind of aggregate level,cross-sectional validation to existing conditions persists across a range of network modelling paradigms,ranging from static and dynamic equilibrium (Florian and Nguyen,1976;Leonard and Tough,1979;Stephenson and Teply,1984;Matzoros et al.,1987;Janson et al., 1986;Janson,1991)to micro-simulation approaches(Laird et al.,1999;Ben-Akiva et al.,2000;Keenan,2005).While such an approach is plausible,it leaves many questions unanswered,and we would particularly highlight two: 1.The process of calibration and validation of a network equilibrium model may typically occur in a cycle.That is to say,having initially calibrated a model using the base data sources,if the subsequent validation reveals substantial discrep-ancies in some part of the network,it is then natural to adjust the model parameters(including perhaps even the OD matrix elements)until the model outputs better reflect the validation data.2In this process,then,we allow the adjustment of potentially a large number of network parameters and input data in order to replicate the validation data,yet these data themselves are highly aggregate,existing only at the link level.To be clear here,we are talking about a level of coarseness even greater than that in aggregate choice models,since we cannot even infer from link-level data the aggregate shares on alternative routes or OD movements.The question that arises is then:how many different combinations of parameters and input data values might lead to a similar link-level validation,and even if we knew the answer to this question,how might we choose between these alternative combinations?In practice,this issue is typically neglected,meaning that the‘valida-tion’is a rather weak test of the model.2.Since the data are cross-sectional in time(i.e.the aim is to reproduce current base conditions in equilibrium),then in spiteof the large efforts required in data collection,no empirical evidence is routinely collected regarding the model’s main purpose,namely its ability to predict changes in behaviour and network performance under changes to the network/ demand.This issue is exacerbated by the aggregation concerns in point1:the‘ambiguity’in choosing appropriate param-eter values to satisfy the aggregate,link-level,base validation strengthens the need to independently verify that,with the selected parameter values,the model responds reliably to changes.Although such problems–offitting equilibrium models to cross-sectional data–have long been recognised by practitioners and academics(see,e.g.,Goodwin,1998), the approach described above remains the state-of-practice.Having identified these two problems,how might we go about addressing them?One approach to thefirst problem would be to return to the underlying formulation of the network model,and instead require a model definition that permits analysis by statistical inference techniques(see for example,Nakayama et al.,2009).In this way,we may potentially exploit more information in the variability of the link-level data,with well-defined notions(such as maximum likelihood)allowing a systematic basis for selection between alternative parameter value combinations.However,this approach is still using rather limited data and it is natural not just to question the model but also the data that we use to calibrate and validate it.Yet this is not altogether straightforward to resolve.As Mahmassani and Jou(2000) remarked:‘A major difficulty...is obtaining observations of actual trip-maker behaviour,at the desired level of richness, simultaneously with measurements of prevailing conditions’.For this reason,several authors have turned to simulated gaming environments and/or stated preference techniques to elicit information on drivers’route choice behaviour(e.g. 1Clearly,more sporadic and less predictable reductions in capacity may also occur,such as in the case of breakdowns and accidents,and environmental factors such as severe weather,floods or landslides(see for example,Iida,1999),but the responses to such cases are outside the scope of the present paper. 2Some authors have suggested more systematic,bi-level type optimization processes for thisfitting process(e.g.Xu et al.,2004),but this has no material effect on the essential points above.D.Watling et al./Transportation Research Part A46(2012)167–189169 Mahmassani and Herman,1990;Iida et al.,1992;Khattak et al.,1993;Vaughn et al.,1995;Wardman et al.,1997;Jou,2001; Chen et al.,2001).This provides potentially rich information for calibrating complex behavioural models,but has the obvious limitation that it is based on imagined rather than real route choice situations.Aside from its common focus on hypothetical decision situations,this latter body of work also signifies a subtle change of emphasis in the treatment of the overall network calibration problem.Rather than viewing the network equilibrium calibra-tion process as a whole,the focus is on particular components of the model;in the cases above,the focus is on that compo-nent concerned with how drivers make route decisions.If we are prepared to make such a component-wise analysis,then certainly there exists abundant empirical evidence in the literature,with a history across a number of decades of research into issues such as the factors affecting drivers’route choice(e.g.Wachs,1967;Huchingson et al.,1977;Abu-Eisheh and Mannering,1987;Duffell and Kalombaris,1988;Antonisse et al.,1989;Bekhor et al.,2002;Liu et al.,2004),the nature of travel time variability(e.g.Smeed and Jeffcoate,1971;Montgomery and May,1987;May et al.,1989;McLeod et al., 1993),and the factors affecting trafficflow variability(Bonsall et al.,1984;Huff and Hanson,1986;Ribeiro,1994;Rakha and Van Aerde,1995;Fox et al.,1998).While these works provide useful evidence for the network equilibrium calibration problem,they do not provide a frame-work in which we can judge the overall‘fit’of a particular network model in the light of uncertainty,ambient variation and systematic changes in network attributes,be they related to the OD demand,the route choice process,travel times or the network data.Moreover,such data does nothing to address the second point made above,namely the question of how to validate the model forecasts under systematic changes to its inputs.The studies of Mannering et al.(1994)and Emmerink et al.(1996)are distinctive in this context in that they address some of the empirical concerns expressed in the context of travel information impacts,but their work stops at the stage of the empirical analysis,without a link being made to net-work prediction models.The focus of the present paper therefore is both to present thefindings of an empirical study and to link this empirical evidence to network forecasting models.More recently,Zhu et al.(2010)analysed several sources of data for evidence of the traffic and behavioural impacts of the I-35W bridge collapse in Minneapolis.Most pertinent to the present paper is their location-specific analysis of linkflows at 24locations;by computing the root mean square difference inflows between successive weeks,and comparing the trend for 2006with that for2007(the latter with the bridge collapse),they observed an apparent transient impact of the bridge col-lapse.They also showed there was no statistically-significant evidence of a difference in the pattern offlows in the period September–November2007(a period starting6weeks after the bridge collapse),when compared with the corresponding period in2006.They suggested that this was indicative of the length of a‘re-equilibration process’in a conceptual sense, though did not explicitly compare their empiricalfindings with those of a network equilibrium model.The structure of the remainder of the paper is as follows.In Section2we describe the process of selecting the real-life problem to analyse,together with the details and rationale behind the survey design.Following this,Section3describes the statistical techniques used to extract information on travel times and routing patterns from the survey data.Statistical inference is then considered in Section4,with the aim of detecting statistically significant explanatory factors.In Section5 comparisons are made between the observed network data and those predicted by a network equilibrium model.Finally,in Section6the conclusions of the study are highlighted,and recommendations made for both practice and future research.2.Experimental designThe ultimate objective of the study was to compare actual data with the output of a traffic network equilibrium model, specifically in terms of how well the equilibrium model was able to correctly forecast the impact of a systematic change ap-plied to the network.While a wealth of surveillance data on linkflows and travel times is routinely collected by many local and national agencies,we did not believe that such data would be sufficiently informative for our purposes.The reason is that while such data can often be disaggregated down to small time step resolutions,the data remains aggregate in terms of what it informs about driver response,since it does not provide the opportunity to explicitly trace vehicles(even in aggre-gate form)across more than one location.This has the effect that observed differences in linkflows might be attributed to many potential causes:it is especially difficult to separate out,say,ambient daily variation in the trip demand matrix from systematic changes in route choice,since both may give rise to similar impacts on observed linkflow patterns across re-corded sites.While methods do exist for reconstructing OD and network route patterns from observed link data(e.g.Yang et al.,1994),these are typically based on the premise of a valid network equilibrium model:in this case then,the data would not be able to give independent information on the validity of the network equilibrium approach.For these reasons it was decided to design and implement a purpose-built survey.However,it would not be efficient to extensively monitor a network in order to wait for something to happen,and therefore we required advance notification of some planned intervention.For this reason we chose to study the impact of urban maintenance work affecting the roads,which UK local government authorities organise on an annual basis as part of their‘Local Transport Plan’.The city council of York,a historic city in the north of England,agreed to inform us of their plans and to assist in the subsequent data collection exercise.Based on the interventions planned by York CC,the list of candidate studies was narrowed by considering factors such as its propensity to induce significant re-routing and its impact on the peak periods.Effectively the motivation here was to identify interventions that were likely to have a large impact on delays,since route choice impacts would then likely be more significant and more easily distinguished from ambient variability.This was notably at odds with the objectives of York CC,170 D.Watling et al./Transportation Research Part A46(2012)167–189in that they wished to minimise disruption,and so where possible York CC planned interventions to take place at times of day and of the year where impacts were minimised;therefore our own requirement greatly reduced the candidate set of studies to monitor.A further consideration in study selection was its timing in the year for scheduling before/after surveys so to avoid confounding effects of known significant‘seasonal’demand changes,e.g.the impact of the change between school semesters and holidays.A further consideration was York’s role as a major tourist attraction,which is also known to have a seasonal trend.However,the impact on car traffic is relatively small due to the strong promotion of public trans-port and restrictions on car travel and parking in the historic centre.We felt that we further mitigated such impacts by sub-sequently choosing to survey in the morning peak,at a time before most tourist attractions are open.Aside from the question of which intervention to survey was the issue of what data to collect.Within the resources of the project,we considered several options.We rejected stated preference survey methods as,although they provide a link to personal/socio-economic drivers,we wanted to compare actual behaviour with a network model;if the stated preference data conflicted with the network model,it would not be clear which we should question most.For revealed preference data, options considered included(i)self-completion diaries(Mahmassani and Jou,2000),(ii)automatic tracking through GPS(Jan et al.,2000;Quiroga et al.,2000;Taylor et al.,2000),and(iii)licence plate surveys(Schaefer,1988).Regarding self-comple-tion surveys,from our own interview experiments with self-completion questionnaires it was evident that travellersfind it relatively difficult to recall and describe complex choice options such as a route through an urban network,giving the po-tential for significant errors to be introduced.The automatic tracking option was believed to be the most attractive in this respect,in its potential to accurately map a given individual’s journey,but the negative side would be the potential sample size,as we would need to purchase/hire and distribute the devices;even with a large budget,it is not straightforward to identify in advance the target users,nor to guarantee their cooperation.Licence plate surveys,it was believed,offered the potential for compromise between sample size and data resolution: while we could not track routes to the same resolution as GPS,by judicious location of surveyors we had the opportunity to track vehicles across more than one location,thus providing route-like information.With time-stamped licence plates, the matched data would also provide journey time information.The negative side of this approach is the well-known poten-tial for significant recording errors if large sample rates are required.Our aim was to avoid this by recording only partial licence plates,and employing statistical methods to remove the impact of‘spurious matches’,i.e.where two different vehi-cles with the same partial licence plate occur at different locations.Moreover,extensive simulation experiments(Watling,1994)had previously shown that these latter statistical methods were effective in recovering the underlying movements and travel times,even if only a relatively small part of the licence plate were recorded,in spite of giving a large potential for spurious matching.We believed that such an approach reduced the opportunity for recorder error to such a level to suggest that a100%sample rate of vehicles passing may be feasible.This was tested in a pilot study conducted by the project team,with dictaphones used to record a100%sample of time-stamped, partial licence plates.Independent,duplicate observers were employed at the same location to compare error rates;the same study was also conducted with full licence plates.The study indicated that100%surveys with dictaphones would be feasible in moderate trafficflow,but only if partial licence plate data were used in order to control observation errors; for higherflow rates or to obtain full number plate data,video surveys should be considered.Other important practical les-sons learned from the pilot included the need for clarity in terms of vehicle types to survey(e.g.whether to include motor-cycles and taxis),and of the phonetic alphabet used by surveyors to avoid transcription ambiguities.Based on the twin considerations above of planned interventions and survey approach,several candidate studies were identified.For a candidate study,detailed design issues involved identifying:likely affected movements and alternative routes(using local knowledge of York CC,together with an existing network model of the city),in order to determine the number and location of survey sites;feasible viewpoints,based on site visits;the timing of surveys,e.g.visibility issues in the dark,winter evening peak period;the peak duration from automatic trafficflow data;and specific survey days,in view of public/school holidays.Our budget led us to survey the majority of licence plate sites manually(partial plates by audio-tape or,in lowflows,pen and paper),with video surveys limited to a small number of high-flow sites.From this combination of techniques,100%sampling rate was feasible at each site.Surveys took place in the morning peak due both to visibility considerations and to minimise conflicts with tourist/special event traffic.From automatic traffic count data it was decided to survey the period7:45–9:15as the main morning peak period.This design process led to the identification of two studies:2.1.Lendal Bridge study(Fig.1)Lendal Bridge,a critical part of York’s inner ring road,was scheduled to be closed for maintenance from September2000 for a duration of several weeks.To avoid school holidays,the‘before’surveys were scheduled for June and early September.It was decided to focus on investigating a significant southwest-to-northeast movement of traffic,the river providing a natural barrier which suggested surveying the six river crossing points(C,J,H,K,L,M in Fig.1).In total,13locations were identified for survey,in an attempt to capture traffic on both sides of the river as well as a crossing.2.2.Fishergate study(Fig.2)The partial closure(capacity reduction)of the street known as Fishergate,again part of York’s inner ring road,was scheduled for July2001to allow repairs to a collapsed sewer.Survey locations were chosen in order to intercept clockwiseFig.1.Intervention and survey locations for Lendal Bridge study.around the inner ring road,this being the direction of the partial closure.A particular aim wasFulford Road(site E in Fig.2),the main radial affected,with F and K monitoring local diversion I,J to capture wider-area diversion.studies,the plan was to survey the selected locations in the morning peak over a period of approximately covering the three periods before,during and after the intervention,with the days selected so holidays or special events.Fig.2.Intervention and survey locations for Fishergate study.In the Lendal Bridge study,while the‘before’surveys proceeded as planned,the bridge’s actualfirst day of closure on Sep-tember11th2000also marked the beginning of the UK fuel protests(BBC,2000a;Lyons and Chaterjee,2002).Trafficflows were considerably affected by the scarcity of fuel,with congestion extremely low in thefirst week of closure,to the extent that any changes could not be attributed to the bridge closure;neither had our design anticipated how to survey the impacts of the fuel shortages.We thus re-arranged our surveys to monitor more closely the planned re-opening of the bridge.Unfor-tunately these surveys were hampered by a second unanticipated event,namely the wettest autumn in the UK for270years and the highest level offlooding in York since records began(BBC,2000b).Theflooding closed much of the centre of York to road traffic,including our study area,as the roads were impassable,and therefore we abandoned the planned‘after’surveys. As a result of these events,the useable data we had(not affected by the fuel protests orflooding)consisted offive‘before’days and one‘during’day.In the Fishergate study,fortunately no extreme events occurred,allowing six‘before’and seven‘during’days to be sur-veyed,together with one additional day in the‘during’period when the works were temporarily removed.However,the works over-ran into the long summer school holidays,when it is well-known that there is a substantial seasonal effect of much lowerflows and congestion levels.We did not believe it possible to meaningfully isolate the impact of the link fully re-opening while controlling for such an effect,and so our plans for‘after re-opening’surveys were abandoned.3.Estimation of vehicle movements and travel timesThe data resulting from the surveys described in Section2is in the form of(for each day and each study)a set of time-stamped,partial licence plates,observed at a number of locations across the network.Since the data include only partial plates,they cannot simply be matched across observation points to yield reliable estimates of vehicle movements,since there is ambiguity in whether the same partial plate observed at different locations was truly caused by the same vehicle. Indeed,since the observed system is‘open’—in the sense that not all points of entry,exit,generation and attraction are mon-itored—the question is not just which of several potential matches to accept,but also whether there is any match at all.That is to say,an apparent match between data at two observation points could be caused by two separate vehicles that passed no other observation point.Thefirst stage of analysis therefore applied a series of specially-designed statistical techniques to reconstruct the vehicle movements and point-to-point travel time distributions from the observed data,allowing for all such ambiguities in the data.Although the detailed derivations of each method are not given here,since they may be found in the references provided,it is necessary to understand some of the characteristics of each method in order to interpret the results subsequently provided.Furthermore,since some of the basic techniques required modification relative to the published descriptions,then in order to explain these adaptations it is necessary to understand some of the theoretical basis.3.1.Graphical method for estimating point-to-point travel time distributionsThe preliminary technique applied to each data set was the graphical method described in Watling and Maher(1988).This method is derived for analysing partial registration plate data for unidirectional movement between a pair of observation stations(referred to as an‘origin’and a‘destination’).Thus in the data study here,it must be independently applied to given pairs of observation stations,without regard for the interdependencies between observation station pairs.On the other hand, it makes no assumption that the system is‘closed’;there may be vehicles that pass the origin that do not pass the destina-tion,and vice versa.While limited in considering only two-point surveys,the attraction of the graphical technique is that it is a non-parametric method,with no assumptions made about the arrival time distributions at the observation points(they may be non-uniform in particular),and no assumptions made about the journey time probability density.It is therefore very suitable as afirst means of investigative analysis for such data.The method begins by forming all pairs of possible matches in the data,of which some will be genuine matches(the pair of observations were due to a single vehicle)and the remainder spurious matches.Thus, for example,if there are three origin observations and two destination observations of a particular partial registration num-ber,then six possible matches may be formed,of which clearly no more than two can be genuine(and possibly only one or zero are genuine).A scatter plot may then be drawn for each possible match of the observation time at the origin versus that at the destination.The characteristic pattern of such a plot is as that shown in Fig.4a,with a dense‘line’of points(which will primarily be the genuine matches)superimposed upon a scatter of points over the whole region(which will primarily be the spurious matches).If we were to assume uniform arrival rates at the observation stations,then the spurious matches would be uniformly distributed over this plot;however,we shall avoid making such a restrictive assumption.The method begins by making a coarse estimate of the total number of genuine matches across the whole of this plot.As part of this analysis we then assume knowledge of,for any randomly selected vehicle,the probabilities:h k¼Prðvehicle is of the k th type of partial registration plateÞðk¼1;2;...;mÞwhereX m k¼1h k¼1172 D.Watling et al./Transportation Research Part A46(2012)167–189。
对抗学习在翻译任务中的应用
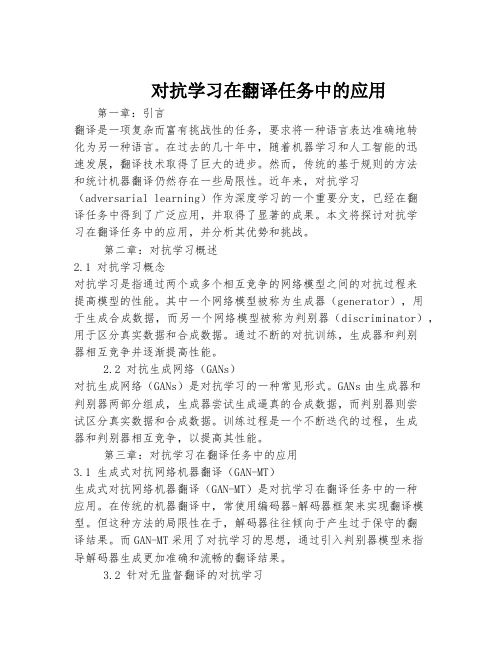
对抗学习在翻译任务中的应用第一章:引言翻译是一项复杂而富有挑战性的任务,要求将一种语言表达准确地转化为另一种语言。
在过去的几十年中,随着机器学习和人工智能的迅速发展,翻译技术取得了巨大的进步。
然而,传统的基于规则的方法和统计机器翻译仍然存在一些局限性。
近年来,对抗学习(adversarial learning)作为深度学习的一个重要分支,已经在翻译任务中得到了广泛应用,并取得了显著的成果。
本文将探讨对抗学习在翻译任务中的应用,并分析其优势和挑战。
第二章:对抗学习概述2.1 对抗学习概念对抗学习是指通过两个或多个相互竞争的网络模型之间的对抗过程来提高模型的性能。
其中一个网络模型被称为生成器(generator),用于生成合成数据,而另一个网络模型被称为判别器(discriminator),用于区分真实数据和合成数据。
通过不断的对抗训练,生成器和判别器相互竞争并逐渐提高性能。
2.2 对抗生成网络(GANs)对抗生成网络(GANs)是对抗学习的一种常见形式。
GANs由生成器和判别器两部分组成,生成器尝试生成逼真的合成数据,而判别器则尝试区分真实数据和合成数据。
训练过程是一个不断迭代的过程,生成器和判别器相互竞争,以提高其性能。
第三章:对抗学习在翻译任务中的应用3.1 生成式对抗网络机器翻译(GAN-MT)生成式对抗网络机器翻译(GAN-MT)是对抗学习在翻译任务中的一种应用。
在传统的机器翻译中,常使用编码器-解码器框架来实现翻译模型。
但这种方法的局限性在于,解码器往往倾向于产生过于保守的翻译结果。
而GAN-MT采用了对抗学习的思想,通过引入判别器模型来指导解码器生成更加准确和流畅的翻译结果。
3.2 针对无监督翻译的对抗学习无监督翻译是指在没有双语语料的情况下进行翻译任务。
对抗学习在无监督翻译中的应用取得了令人瞩目的成果。
通过构建对抗学习框架,使用同一语义空间中的两种语言进行学习,可以实现无监督翻译。
这种方法不依赖于人工标注的数据,大大降低了翻译任务的成本。
黑龙江省龙东十校2024-2025学年高二上学期开学考试英语试题

黑龙江省龙东十校2024-2025学年高二上学期开学考试英语试题一、阅读理解We are currently seeking a qualified and experienced individual to join our team as a marketing manager. As a key leadership position within our organization, the marketing manager will play a crucial role in driving the department’s success and contributing to our overall business objectives.Qualifications:*Bachelor’s degree in business administration, management or related fields.*Proven experience in a marketing role, with a track record of successfully leading teams and achieving results.*Strong communication, interpersonal and problem-solving skills.*Proficiency in relevant software apps.Responsibilities:*Overseeing daily operations of the department, including staff management, budgeting and goal setting.*Developing and implementing strategies to meet departmental targets and enhance efficiency.*Cooperating with cross-functional teams to ensure seamless coordination and communication.*Providing leadership and guidance to team members to develop a positive work culture and professional growth.If you are a motivated and enthusiastic leader with a passion for driving organizational success, we invite you to apply for the position. Join us in shaping the future of our department and making a lasting impact on our company.To apply, please submit your resume and a cover letter detailing your relevant experience and why you are the ideal candidate for this role. We look forward to welcoming a dedicated and ************************************************************.1.What is a must to apply for the position?A.A master’s degree.B.A strong body.C.The related work experience.D.The ability to develop software.2.What does the marketing manager have to do?A.Communicate with consumers regularly.B.Assist the leaders to develop annual plans.C.Achieve the overall goal of the industry.D.Help co-workers develop their business. 3.How can you get in touch with the interviewer?A.By filling out a form.B.By sending an email.C.By writing a letter.D.By making a phone call.Over the weekend, a Pennsylvania man risked his life to save his neighbors from a burning home, as captured in dramatic video footage.Oscar Rivera was playing with his children in his backyard on April 14 when he heard a loud boom (响声). Rushing to the front yard, he saw that the house across the street was engulfed in flames, with his neighbors trapped inside.According to WFMZ-TV, Rivera didn’t hesitate. He climbed up the three-story building, which was already covered in flames. On a narrow ledge (窗台) at the burning home, a woman was trying to pull a man out of an attic window as smoke poured out. The man was calling for help.Rivera and a neighbor quickly grabbed a ladder and rushed to assist. “I just started jumping, jumping and jumping,” Rivera told WFMZ-TV. He managed to reach the top floor and pulled the man from the window, who had yelled, “Help me. I can’t walk.”Eyewitness Janeen Huth, who recorded the rescue, praised Rivera for risking his life while his young children watched from the door. “Come on! Come on!” Huth can be heard shouting in the video, as other onlookers and firefighters worked to assist. “Rivera is a true hero,” she told The Morning Call. Rivera managed to get the victim onto the ledge, and firefighters then took over, bringing the man to safety.The rescued man sustained critical burns and was taken to the hospital, according to WFMZ-TV. The fire damaged six homes and led to the evacuation (疏散) of 20 people. The cause of the fire is currently under investigation. “I hope he’s okay,” Rivera said about the man he saved.4.What does the text describe?A.An investigation into a fire.B.A man’s heroic rescue.C.A fire department’s response.D.A fire that destroyed several homes. 5.What made Rivera rush to the front yard?A.Hearing a loud boom.B.Seeing the flame.C.Hearing a call for help.D.Being informed about the fire.6.What did Rivera do in the rescue?A.He provided first aid.B.He helped guide firefighters.C.He called emergency services.D.He pulled the man out.7.How did Janeen Huth react to Rivera’s action?A.She was indifferent.B.She admired his bravery.C.She criticized him for risking his life.D.She thought he should wait for firefighters.The immune (免疫的) system has special defense and attack strategies reserved for viruses (病毒). These involve tagging the viruses with antibodies and killing cells infected (使感染) by the virus. A global health crisis can bring the world to its knees, highlighting the huge impact a virus can have. This tiny organism, invisible under a normal microscope, can wreak havoc on worldwide.There are more viruses on Earth than stars in the universe, so why haven’t we always been maintaining social distance? Primarily because not all viruses can infect us, and for those that do, the body handles them quite well. To put it simply, viruses are extremely small parasites that infect all sorts of life, from the smallest bacteria to the largest mammals; they are considered parasites because they can’t survive by themselves. Viruses infect health y host cells and use their cellular “tools” to make more copies of themselves.Viruses are everywhere—in the air we breathe, the water we drink, and the land on which we walk. Yet, even after being bombarded with viruses, our body still manages to stay strong. This is because of our immune system and its clever strategies to fight back against viral attacks! Fortunately, with our modern healthcare advancements, we have extra help to fight viruses. These include vaccines, antiviral drugs, and other health technologies to keep us going in this never-ending war.As time goes by and our bodies encounter more viruses, we develop better immunological memory. This is the body’s way of remembering past infections, making it better prepared for future ones.8.Which can replace the underlined phrase “wreak havoc on” in paragraph 1?A.Depend heavily on.B.Have a bad effect on.C.Take the side effect off.D.Cut the whole power off.9.Why do we always fail to maintain social distance despite lots of viruses?A.Not all viruses infect humans.B.Not all viruses are small.C.Viruses need host cells.D.Viruses infect all life forms.10.What is one of the strategies our body uses to fight viral attacks?A.Reducing the number of viruses in the environment.B.Avoiding contacting with infected individuals.C.Taking advantage of the immune system.D.Increasing exposure to viruses.11.What can be the best title for the text?A.What is the definition of unusual viruses?B.How to know well the immune system?C.How does our body fight viruses?D.How to break down the viruses?A major tech company has expanded options for keeping personal information from online searches. The company stated earlier this week that it would allow people to request the removal of more types of content, such as personal contact information like phone numbers, email addresses, and physical addresses, from search results. The new policy also permits the removal of other information that may pose a risk for identity theft, such as confidential (机密的) login accounts.In a statement, the company said that open access to information is vital. “But so is empowering (授权) people with the tools they need to protect themselves and keep their sensitive, personally identifiable information private,” the statement continued. “Privacy and online safety go hand in hand. And when you’re using the Internet, it’s important to have control over how your sensitive, personally identifiable information can be found.”Previously, the company had allowed people to request the removal of highly personalcontent that could cause direct harm. This included information such as bank account or credit card numbers that could be used for fraud (欺诈).“However, as information increasingly appears in unexpected places and is used in new ways, policies need to evolve,” the company said. Having personal contact information openly available online can also pose a threat, and the company reported receiving requests to remove such content as well. It states that when it receives such requests, it will review all the content on the web page to avoid limiting the availability of useful information or content on public record on government or other official websites. “It’s important to remember that removing content from our search results won’t remove it from the Internet, which is why you may wish to contact the hosting site directly, if you’re comfortable doing so,” the company advised.12.What can we learn from the company’s statement?A.Keeping online private information safe is a must.B.Improving the net environment for citizens is a dream.C.The exposure of public information causes a discussion.D.Making a balanced approach to online safety is a fashion.13.Why does the company review web page content before removing personal information?A.To obey data privacy laws.B.To take down the entire website.C.To increase online personal information.D.To avoid removing useful or publicinformation.14.What is the main idea of the text?A.Personal information can be removed from online searches.B.Tech companies can find more images and information.C.Privacy risks arise from publishing private information.D.Tech companies know what you’ve done over the years.15.What is the text likely to be?A.A research paper on online privacy.B.A new approach to using a search engine.C.An advertisement for a data privacy service.D.A news report about privacy protection.Do you know what carpet cleaning is and how it works? This article will discuss it with you.Carpet cleaning is a service to remove the dirt, stains and other things from carpets and make them clean. People hire different carpet cleaning services to make their carpets look cleaner, more appealing and away from harmful infections. According to experts, people must clean their carpets once or twice a year. 16 .According to carpet manufacturers, hot water extraction is one of the most professional and recommended ways of cleaning carpets. 17Dry cleaning is also used for cleaning the carpets but this is far easier than wet cleaning.18 However, it is still used for cleaning the carpets which are not that dirty.Vacuum (真空) cleaning is mostly used in offices and houses by people themselves. People use a vacuum cleaner to clean the carpets and it is an effective method.19 Therefore, carpet cleaning must be done minimum 1-2 times a year to prevent yourself from infections, allergies, and so on. Another benefit is that it saves you a lot of money. If you don’t clean them on a regular basis, you have to purchase them.You have understood a lot about carpet cleaning. 20 Do not regard this as a trivial (琐碎的) matter. It concerns the health of you and your family.A.It is time for you to take action.B.Prepare yourself before you begin.C.It is not that better than wet cleaning.D.Dirty carpets damage your health a lot.E.Dry cleaning is more effective than wet cleaning.F.Now, the question is what methods are used to clean them.G.It can remove the dirt and other things deep from the carpets.二、完形填空My husband and I had been married for ten years when I got Stevens-Johnson syndrome (综合征). With painful blisters (水疱) all over my body, I, who had been independent, rapidly became 21 .My husband, Scott, was occupied taking care of kids and cooking dinners. He also became my personal caretaker, applying the 22 to all of my blisters because my hands couldn’t dothe job. I was 23 at total reliance on someone other than myself. At one point, I mentally and physically hit 24 , thinking I was a weak person. And that 25 me.I recovered from my illness, but I couldn’t recover from the negative 26 . This loss of27 affected the rest of my recovery. Fortunately, things 28 . Recently Scott and I went ona long bike ride. At one point with sharp pain 29 in my tired legs, I couldn’t go any further. Seeing me 30 , Scott stopped in front of me, saying, “Stay close behind me.” Scott was 31 me along. My legs quit burning 32 as my cycling became easier, and I was able to catch my breath.I now 33 that love between us is powerful. True love is forged (锻造) by the fire of late nights with sick family and days of 34 to make ends meet. I also believe that during these 35 times, love has the power to make us become stronger. 21.A.worthless B.helpless C.fearless D.homeless 22.A.medicine B.disease C.mission D.strategy 23.A.shocked B.amused C.embarrassed D.puzzled 24.A.goal B.bottom C.future D.desire 25.A.taught B.forgave C.inspired D.troubled 26.A.thought B.plan C.comment D.aspect 27.A.direction B.confidence C.dream D.success 28.A.took over B.showed off C.turned around D.stood out 29.A.extending B.reducing C.escaping D.disappearing 30.A.relieve B.complain C.leave D.struggle 31.A.walking B.sending C.pulling D.bringing 32.A.frequently B.initially C.strangely D.quickly 33.A.hope B.argue C.permit D.believe 34.A.trying B.daring C.choosing D.promising 35.A.common B.difficult C.pleasant D.primary三、语法填空阅读下面短文,在空白处填入1个适当的单词或括号内单词的正确形式。
《2024年负荷模式下同传工作记忆策略研究》范文

《负荷模式下同传工作记忆策略研究》篇一一、引言随着全球化的快速发展,同声传译(简称同传)作为国际交流的重要桥梁,其工作效率与质量受到了广泛关注。
在负荷模式下,同传工作需要处理大量的信息,而工作记忆则成为同传过程中的关键因素。
本篇论文旨在研究负荷模式下同传工作的记忆策略,以提高同传的效率和准确性。
二、研究背景及意义同声传译是一项高强度的脑力劳动,要求译员在听取源语发言的同时,迅速理解其含义,并将其转化为目标语输出。
在负荷模式下,译员需在短时间内处理大量的信息,这要求他们具备良好的工作记忆能力。
工作记忆是同传过程中不可或缺的认知能力,它直接影响着译员的翻译质量和效率。
因此,研究负荷模式下同传工作的记忆策略具有重要的理论和实践意义。
三、文献综述前人对同传工作记忆的研究主要集中在记忆的容量、存储和提取等方面。
研究表明,同传工作记忆需要处理语音、语义、语法等多个层面的信息,而这些信息的处理需要依赖有效的记忆策略。
目前,常见的同传工作记忆策略包括笔记法、语言转换策略、注意力分配策略等。
然而,这些策略在负荷模式下的应用效果及相互关系仍需进一步研究。
四、研究方法本研究采用实证研究方法,通过实验和问卷调查收集数据。
首先,设计同传实验,模拟负荷模式下的同传工作环境,记录译员的翻译过程和表现。
其次,通过问卷调查收集译员关于工作记忆策略的使用情况和主观感受。
最后,运用统计分析方法,探讨不同记忆策略对同传工作的影响。
五、实验结果与分析1. 实验结果通过同传实验和问卷调查,我们收集了大量关于同传工作记忆策略的数据。
实验结果显示,不同的译员在使用记忆策略上存在差异,这些差异影响了他们的翻译质量和效率。
此外,我们还发现,某些记忆策略在负荷模式下表现出更好的效果。
2. 分析与讨论通过对实验结果的分析,我们发现笔记法在负荷模式下具有重要作用。
笔记不仅可以帮助译员记录关键信息,还可以辅助其回忆和理解源语含义。
然而,过度依赖笔记也会分散译员的注意力,影响翻译质量。
scale-invariant attack method -回复
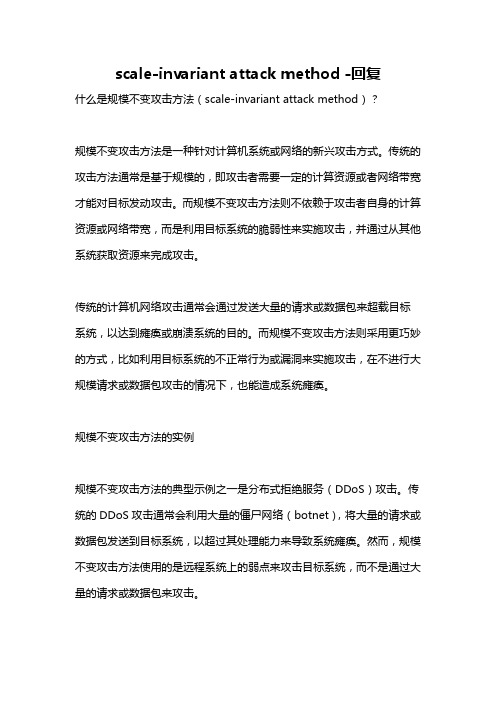
scale-invariant attack method -回复什么是规模不变攻击方法(scale-invariant attack method)?规模不变攻击方法是一种针对计算机系统或网络的新兴攻击方式。
传统的攻击方法通常是基于规模的,即攻击者需要一定的计算资源或者网络带宽才能对目标发动攻击。
而规模不变攻击方法则不依赖于攻击者自身的计算资源或网络带宽,而是利用目标系统的脆弱性来实施攻击,并通过从其他系统获取资源来完成攻击。
传统的计算机网络攻击通常会通过发送大量的请求或数据包来超载目标系统,以达到瘫痪或崩溃系统的目的。
而规模不变攻击方法则采用更巧妙的方式,比如利用目标系统的不正常行为或漏洞来实施攻击,在不进行大规模请求或数据包攻击的情况下,也能造成系统瘫痪。
规模不变攻击方法的实例规模不变攻击方法的典型示例之一是分布式拒绝服务(DDoS)攻击。
传统的DDoS攻击通常会利用大量的僵尸网络(botnet),将大量的请求或数据包发送到目标系统,以超过其处理能力来导致系统瘫痪。
然而,规模不变攻击方法使用的是远程系统上的弱点来攻击目标系统,而不是通过大量的请求或数据包来攻击。
一个具体的例子是使用规模不变攻击方法来攻击一个基于云计算的网络服务。
在这种攻击中,攻击者可以利用目标系统云计算环境中的某个虚拟机的漏洞,通过该虚拟机获得对运行其他虚拟机的物理服务器的控制权。
然后,攻击者可以在其他虚拟机上执行恶意代码,从而瘫痪整个云计算环境,即使目标系统本身并没有受到大量请求或数据包的攻击。
规模不变攻击方法的特点规模不变攻击方法有几个显著的特点。
首先,它们能够利用目标系统的特定脆弱性来实施攻击,并不依赖于攻击者自身的计算和网络资源。
其次,规模不变攻击方法相对隐蔽,因为它们通常不会引起目标系统运营商的警觉,这使得攻击更难被检测和阻止。
此外,规模不变攻击方法通常难以完全规避,因为它们利用的是目标系统本身的漏洞或异常行为。
对抗样本攻击原理

对抗样本攻击原理Adversarial attacks refer to the phenomenon where slight perturbations are introduced to input data, leading to misclassification by machine learning models. These attacks pose a significant threat to the security and robustness of AI systems. Adversarial attacks exploit the vulnerabilities of neural networks, causing them to misclassify inputs that are almost indistinguishable from the original data. These attacks can have serious consequences in real-world applications, such as autonomous vehicles, image recognition, and cybersecurity systems.对抗样本攻击是指对输入数据进行微小扰动,导致机器学习模型误分类的现象。
这些攻击对人工智能系统的安全性和稳健性构成了重大威胁。
对抗攻击利用神经网络的漏洞,导致它们对几乎无法与原始数据区分的输入数据进行错误分类。
这些攻击可能在现实世界的应用中产生严重后果,例如自动驾驶车辆、图像识别和网络安全系统。
One of the primary reasons for the success of adversarial attacks is the lack of robustness in deep learning models. Neural networks are highly susceptible to these attacks due to their inherent sensitivity tosmall changes in input data. The linear nature of neural networks allows for the exploitation of their vulnerabilities through carefully crafted adversarial examples. These adversarial examples are designed to circumvent the decision boundaries of the neural network and force misclassification.对抗样本攻击成功的一个主要原因是深度学习模型缺乏鲁棒性。
对抗性样本的生成机制及防御方法思考
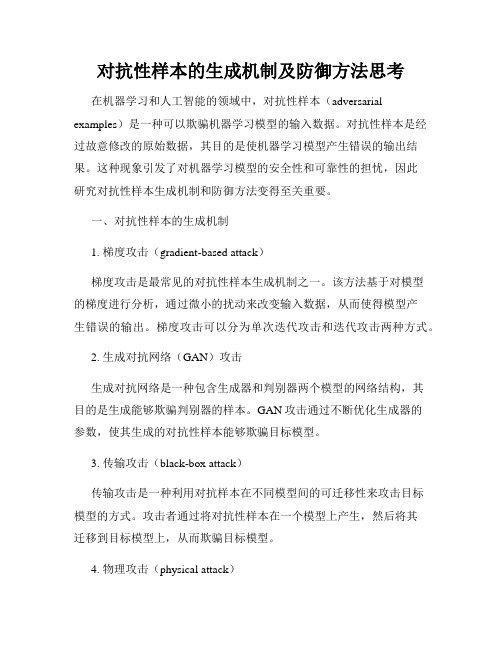
对抗性样本的生成机制及防御方法思考在机器学习和人工智能的领域中,对抗性样本(adversarial examples)是一种可以欺骗机器学习模型的输入数据。
对抗性样本是经过故意修改的原始数据,其目的是使机器学习模型产生错误的输出结果。
这种现象引发了对机器学习模型的安全性和可靠性的担忧,因此研究对抗性样本生成机制和防御方法变得至关重要。
一、对抗性样本的生成机制1. 梯度攻击(gradient-based attack)梯度攻击是最常见的对抗性样本生成机制之一。
该方法基于对模型的梯度进行分析,通过微小的扰动来改变输入数据,从而使得模型产生错误的输出。
梯度攻击可以分为单次迭代攻击和迭代攻击两种方式。
2. 生成对抗网络(GAN)攻击生成对抗网络是一种包含生成器和判别器两个模型的网络结构,其目的是生成能够欺骗判别器的样本。
GAN攻击通过不断优化生成器的参数,使其生成的对抗性样本能够欺骗目标模型。
3. 传输攻击(black-box attack)传输攻击是一种利用对抗样本在不同模型间的可迁移性来攻击目标模型的方式。
攻击者通过将对抗性样本在一个模型上产生,然后将其迁移到目标模型上,从而欺骗目标模型。
4. 物理攻击(physical attack)物理攻击是一种通过对输入样本进行物理改变来欺骗机器学习模型的方法。
例如,在图像分类任务中,攻击者可以通过粘贴贴纸或添加噪声等方式改变输入图像,从而使得模型产生错误的输出。
二、对抗性样本的防御方法1. 对抗性训练(adversarial training)对抗性训练是一种通过在训练过程中引入对抗性样本来提高模型的鲁棒性的方法。
在对抗性训练中,模型会在原始数据和对抗性样本上进行训练,使其学会抵抗对抗性样本的攻击。
2. 防御性降噪(defensive distillation)防御性降噪是一种通过对输入数据进行噪声的添加或滤波来抵御对抗性样本的攻击的方法。
降噪可以使对抗性样本的扰动被抑制,从而减少对模型输出的影响。
对抗性样本的生成机制及防御方法思考
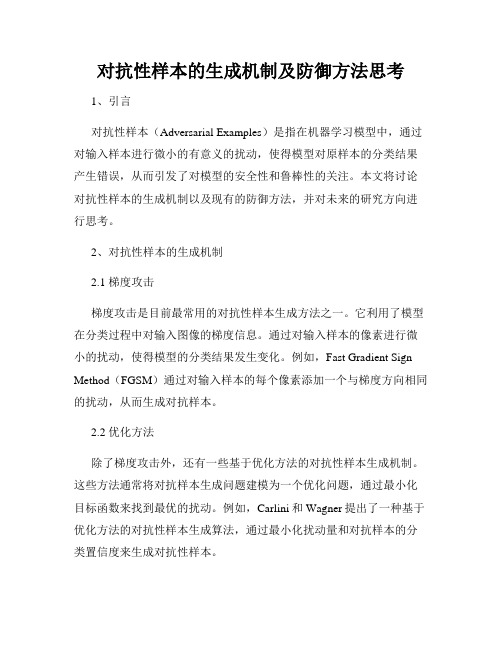
对抗性样本的生成机制及防御方法思考1、引言对抗性样本(Adversarial Examples)是指在机器学习模型中,通过对输入样本进行微小的有意义的扰动,使得模型对原样本的分类结果产生错误,从而引发了对模型的安全性和鲁棒性的关注。
本文将讨论对抗性样本的生成机制以及现有的防御方法,并对未来的研究方向进行思考。
2、对抗性样本的生成机制2.1 梯度攻击梯度攻击是目前最常用的对抗性样本生成方法之一。
它利用了模型在分类过程中对输入图像的梯度信息。
通过对输入样本的像素进行微小的扰动,使得模型的分类结果发生变化。
例如,Fast Gradient Sign Method(FGSM)通过对输入样本的每个像素添加一个与梯度方向相同的扰动,从而生成对抗样本。
2.2 优化方法除了梯度攻击外,还有一些基于优化方法的对抗性样本生成机制。
这些方法通常将对抗样本生成问题建模为一个优化问题,通过最小化目标函数来找到最优的扰动。
例如,Carlini和Wagner提出了一种基于优化方法的对抗性样本生成算法,通过最小化扰动量和对抗样本的分类置信度来生成对抗性样本。
3、对抗性样本的防御方法3.1 对抗训练对抗训练是一种常见的对抗性样本防御方法。
它在训练过程中引入了对抗样本,使得模型能够对这些样本进行学习和适应,从而提高模型的鲁棒性。
对抗训练通常通过在训练时对输入样本进行扰动,并将扰动后的样本作为额外的训练数据,从而提高模型对对抗性样本的鲁棒性。
3.2 集成学习集成学习是另一种有效的对抗性样本防御方法。
它通过结合多个不同的分类器的输出来进行决策,从而提高模型的鲁棒性。
对于对抗性样本来说,不同的分类器可能会对同一个样本产生不同的分类结果。
通过集成这些不同的结果,可以减弱对抗样本的干扰,并提高最终的分类准确率。
3.3 模型修正模型修正是一种基于模型本身的对抗性样本防御方法。
它通过改善模型的结构和参数,使得模型能够更好地适应对抗性样本。
例如,通过增加模型的复杂度和深度,可以提高模型对对抗性样本的鲁棒性。
对抗学习与模型防御

对抗攻击的分类与实例
▪ 对抗攻击的实例
1.对抗补丁是一种常见的对抗攻击方式,攻击者通过在输入图像上添加一些小的扰 动,使得模型将其误分类为其他类别。例如,通过在一张熊猫图片上添加一些小的 扰动,可以使得模型将其误分类为长臂猿。 2.水印攻击是一种通过在数字图像中嵌入一些不可见的水印信息,从而欺骗模型的 方法。这些水印信息可以被用来篡改模型的输出,从而实现对模型的攻击。 3.对抗训练是一种提高模型鲁棒性的方法,通过在训练数据中添加一些对抗样本, 使得模型能够更好地抵御对抗攻击的干扰。例如,在训练图像分类模型时,可以在 训练数据中添加一些被故意修改的图像,以提高模型的鲁棒性。
对抗学习概述与基本概念
▪ 对抗学习的定义与分类
1.对抗学习是一种研究如何在存在恶意攻击的情况下,提高模 型鲁棒性的学习方法。 2.对抗攻击可以分为白盒攻击和黑盒攻击两类,分别对应攻击 者对不同信息的掌握程度。 3.对抗学习可以应用于各种深度学习模型,包括图像识别、语 音识别、自然语言处理等。
▪ 对抗攻击的原理与技术
▪ 基于深度学习的异常检测技术
1.技术原理:基于深度学习模型的异常检测技术,通过学习正常数据的分布,识别 出与正常数据分布差异较大的异常数据。 2.实验结果:在多个数据集上进行了实验,准确率均超过了90%,证明了该技术的 有效性。 3.优点与局限:该技术具有较高的准确率和较低的误报率,但对于复杂的攻击可能 存在一定的局限。
未来研究方向与展望
可解释性对抗学习
1.研究如何使对抗学习模型更具可解释性,以便更好地理解其工作原理。 2.探索如何通过可视化技术展示对抗攻击和防御的效果。 3.研究可解释性对抗学习在解决实际问题中的应用,提高模型的信任度。
隐私保护对抗学习
对抗训练的公式范文
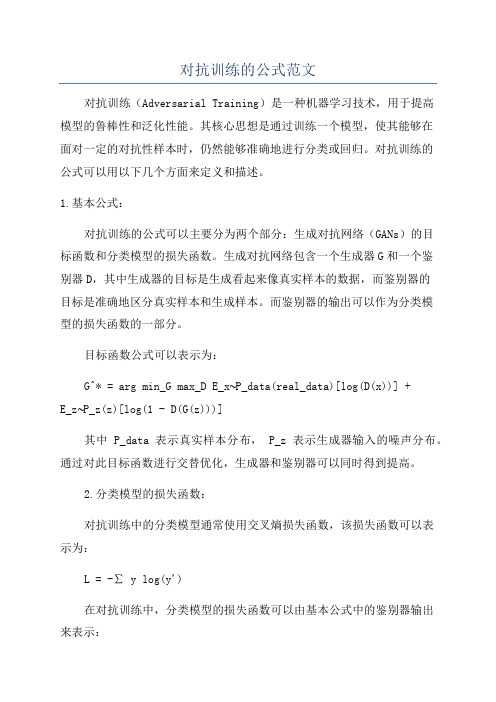
对抗训练的公式范文对抗训练(Adversarial Training)是一种机器学习技术,用于提高模型的鲁棒性和泛化性能。
其核心思想是通过训练一个模型,使其能够在面对一定的对抗性样本时,仍然能够准确地进行分类或回归。
对抗训练的公式可以用以下几个方面来定义和描述。
1.基本公式:对抗训练的公式可以主要分为两个部分:生成对抗网络(GANs)的目标函数和分类模型的损失函数。
生成对抗网络包含一个生成器G和一个鉴别器D,其中生成器的目标是生成看起来像真实样本的数据,而鉴别器的目标是准确地区分真实样本和生成样本。
而鉴别器的输出可以作为分类模型的损失函数的一部分。
目标函数公式可以表示为:G^* = arg min_G max_D E_x∼P_data(real_data)[log(D(x))] +E_z∼P_z(z)[log(1 - D(G(z)))]其中 P_data 表示真实样本分布, P_z 表示生成器输入的噪声分布。
通过对此目标函数进行交替优化,生成器和鉴别器可以同时得到提高。
2.分类模型的损失函数:对抗训练中的分类模型通常使用交叉熵损失函数,该损失函数可以表示为:L = -∑ y log(y')在对抗训练中,分类模型的损失函数可以由基本公式中的鉴别器输出来表示:L = -log(D(x) + log(1 - D(G(z))))其中D(x)表示鉴别器对真实样本的预测输出,D(G(z))表示鉴别器对生成样本的预测输出。
通过最小化此损失函数,分类模型可以学习到更鲁棒的特征表示以区分真实和生成样本。
3.对抗训练的具体步骤:具体的对抗训练过程可以分为以下几个步骤:-初始化生成器和鉴别器的参数;-通过最大化目标函数,更新鉴别器的参数;-通过最小化目标函数,更新生成器的参数;-重复上述两步直到达到预设的迭代次数或达到一定的性能指标。
在每次更新生成器和鉴别器的参数之前,都要先固定另一部分的参数。
这样做是为了确保在每次更新时都能够传递准确的梯度信息。
对抗学习在文本生成任务中的应用
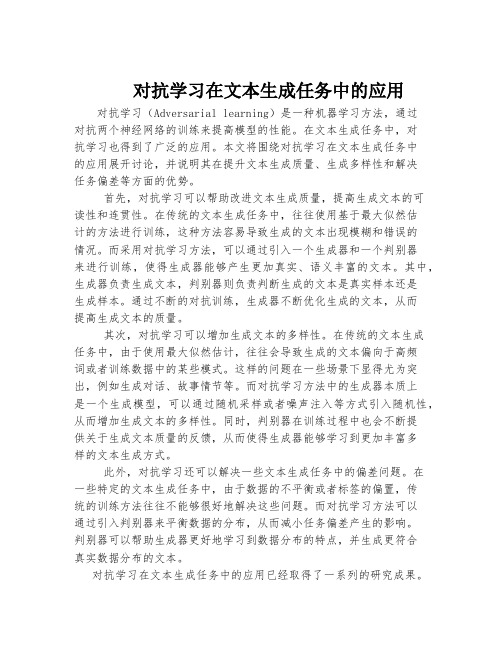
对抗学习在文本生成任务中的应用对抗学习(Adversarial learning)是一种机器学习方法,通过对抗两个神经网络的训练来提高模型的性能。
在文本生成任务中,对抗学习也得到了广泛的应用。
本文将围绕对抗学习在文本生成任务中的应用展开讨论,并说明其在提升文本生成质量、生成多样性和解决任务偏差等方面的优势。
首先,对抗学习可以帮助改进文本生成质量,提高生成文本的可读性和连贯性。
在传统的文本生成任务中,往往使用基于最大似然估计的方法进行训练,这种方法容易导致生成的文本出现模糊和错误的情况。
而采用对抗学习方法,可以通过引入一个生成器和一个判别器来进行训练,使得生成器能够产生更加真实、语义丰富的文本。
其中,生成器负责生成文本,判别器则负责判断生成的文本是真实样本还是生成样本。
通过不断的对抗训练,生成器不断优化生成的文本,从而提高生成文本的质量。
其次,对抗学习可以增加生成文本的多样性。
在传统的文本生成任务中,由于使用最大似然估计,往往会导致生成的文本偏向于高频词或者训练数据中的某些模式。
这样的问题在一些场景下显得尤为突出,例如生成对话、故事情节等。
而对抗学习方法中的生成器本质上是一个生成模型,可以通过随机采样或者噪声注入等方式引入随机性,从而增加生成文本的多样性。
同时,判别器在训练过程中也会不断提供关于生成文本质量的反馈,从而使得生成器能够学习到更加丰富多样的文本生成方式。
此外,对抗学习还可以解决一些文本生成任务中的偏差问题。
在一些特定的文本生成任务中,由于数据的不平衡或者标签的偏置,传统的训练方法往往不能够很好地解决这些问题。
而对抗学习方法可以通过引入判别器来平衡数据的分布,从而减小任务偏差产生的影响。
判别器可以帮助生成器更好地学习到数据分布的特点,并生成更符合真实数据分布的文本。
对抗学习在文本生成任务中的应用已经取得了一系列的研究成果。
例如,在机器翻译任务中,对抗学习可以帮助提高翻译质量,生成更流利和准确的翻译结果。
对抗样本的生成和防御技术进展与比较

对抗样本的生成和防御技术进展与比较对抗样本(Adversarial Examples)是指经过有意设计的微小扰动,能够使深度学习模型产生错误分类结果的输入样本。
对抗样本的生成与防御技术是保障深度学习模型鲁棒性的重要研究方向。
本文将对对抗样本的生成与防御技术的进展与比较进行探讨。
对抗样本生成技术的发展一直是研究的热点之一。
目前,主要的方法有基于梯度的生成方法和基于优化的生成方法。
基于梯度的生成方法通过计算损失函数对输入样本的梯度信息进行修正,从而生成对抗样本。
例如,Fast Gradient Sign Method (FGSM)是一种常用的基于梯度的对抗样本生成方法,它通过沿着分类器梯度的方向对输入样本进行微小扰动,从而改变模型的预测结果。
但是,这种方法的缺点是生成的对抗样本可能与原始样本相似度较低。
为了解决这个问题,一些研究者提出了基于优化的生成方法,如基于进化算法和基于生成对抗网络(GAN)的方法。
这些方法能够生成更具迷惑性且与原始样本更相似的对抗样本。
另一方面,对抗样本防御技术是保护深度学习模型免受对抗样本攻击的关键。
为了提高模型的鲁棒性,研究者们提出了许多防御方法。
具体而言,对抗训练是一种常用的防御技术,它在训练模型时,针对对抗样本进行增强的训练。
对抗训练主要通过向训练集中添加对抗样本,并在训练过程中使用对抗样本进行模型训练。
此外,一些研究者还提出了一些基于隐秘表征的防御方法,例如,在网络中添加额外的随机噪声,或者对输入进行平滑化处理。
这些方法能够增加对抗样本的难度,从而提高模型对对抗攻击的鲁棒性。
与对抗样本的生成技术相比,对抗样本的防御技术仍然存在很多挑战。
首先,对抗样本生成技术在不断发展,可以生成更加隐蔽的对抗样本。
其次,对抗样本防御技术的有效性和鲁棒性仍然缺乏理论保证。
此外,现有的防御方法在一些特定情况下可能会受到绕过攻击的影响。
因此,研究者们需要进一步探索更加有效和鲁棒的对抗样本防御技术。
对抗学习中的稳定训练方法
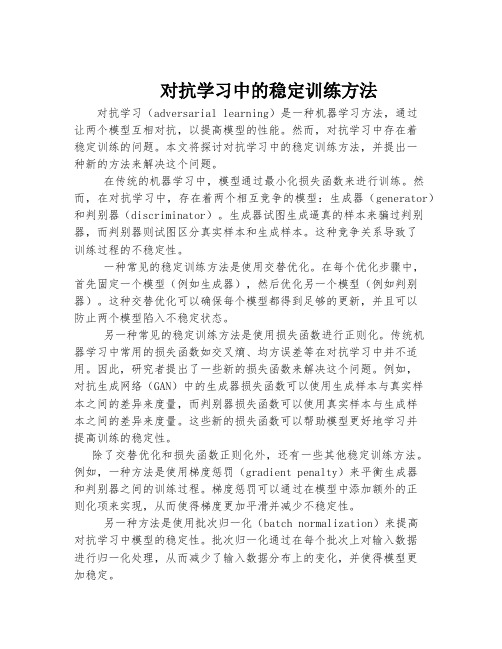
对抗学习中的稳定训练方法对抗学习(adversarial learning)是一种机器学习方法,通过让两个模型互相对抗,以提高模型的性能。
然而,对抗学习中存在着稳定训练的问题。
本文将探讨对抗学习中的稳定训练方法,并提出一种新的方法来解决这个问题。
在传统的机器学习中,模型通过最小化损失函数来进行训练。
然而,在对抗学习中,存在着两个相互竞争的模型:生成器(generator)和判别器(discriminator)。
生成器试图生成逼真的样本来骗过判别器,而判别器则试图区分真实样本和生成样本。
这种竞争关系导致了训练过程的不稳定性。
一种常见的稳定训练方法是使用交替优化。
在每个优化步骤中,首先固定一个模型(例如生成器),然后优化另一个模型(例如判别器)。
这种交替优化可以确保每个模型都得到足够的更新,并且可以防止两个模型陷入不稳定状态。
另一种常见的稳定训练方法是使用损失函数进行正则化。
传统机器学习中常用的损失函数如交叉熵、均方误差等在对抗学习中并不适用。
因此,研究者提出了一些新的损失函数来解决这个问题。
例如,对抗生成网络(GAN)中的生成器损失函数可以使用生成样本与真实样本之间的差异来度量,而判别器损失函数可以使用真实样本与生成样本之间的差异来度量。
这些新的损失函数可以帮助模型更好地学习并提高训练的稳定性。
除了交替优化和损失函数正则化外,还有一些其他稳定训练方法。
例如,一种方法是使用梯度惩罚(gradient penalty)来平衡生成器和判别器之间的训练过程。
梯度惩罚可以通过在模型中添加额外的正则化项来实现,从而使得梯度更加平滑并减少不稳定性。
另一种方法是使用批次归一化(batch normalization)来提高对抗学习中模型的稳定性。
批次归一化通过在每个批次上对输入数据进行归一化处理,从而减少了输入数据分布上的变化,并使得模型更加稳定。
除了上述方法外,还有很多其他方法被提出用于对抗学习中的稳定训练。
例如,一些研究者提出使用多个生成器和判别器来提高稳定性。
对抗样本产生原理及应对策略思考
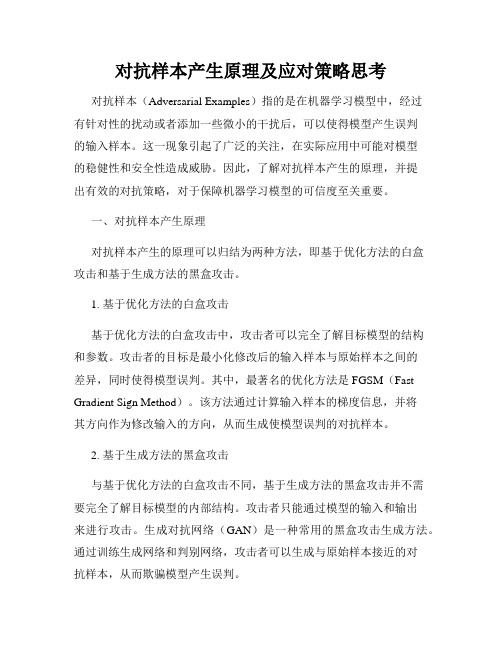
对抗样本产生原理及应对策略思考对抗样本(Adversarial Examples)指的是在机器学习模型中,经过有针对性的扰动或者添加一些微小的干扰后,可以使得模型产生误判的输入样本。
这一现象引起了广泛的关注,在实际应用中可能对模型的稳健性和安全性造成威胁。
因此,了解对抗样本产生的原理,并提出有效的对抗策略,对于保障机器学习模型的可信度至关重要。
一、对抗样本产生原理对抗样本产生的原理可以归结为两种方法,即基于优化方法的白盒攻击和基于生成方法的黑盒攻击。
1. 基于优化方法的白盒攻击基于优化方法的白盒攻击中,攻击者可以完全了解目标模型的结构和参数。
攻击者的目标是最小化修改后的输入样本与原始样本之间的差异,同时使得模型误判。
其中,最著名的优化方法是FGSM(Fast Gradient Sign Method)。
该方法通过计算输入样本的梯度信息,并将其方向作为修改输入的方向,从而生成使模型误判的对抗样本。
2. 基于生成方法的黑盒攻击与基于优化方法的白盒攻击不同,基于生成方法的黑盒攻击并不需要完全了解目标模型的内部结构。
攻击者只能通过模型的输入和输出来进行攻击。
生成对抗网络(GAN)是一种常用的黑盒攻击生成方法。
通过训练生成网络和判别网络,攻击者可以生成与原始样本接近的对抗样本,从而欺骗模型产生误判。
二、应对策略思考对于对抗样本的产生,一方面,我们需要寻找有效的方法来减少对抗样本造成的影响;另一方面,我们也需要思考如何提高模型的鲁棒性,从源头上减少对抗样本的产生。
1. 对抗样本防御方法(1)防御方法一:添加随机性通过在训练数据中引入随机性,可以增加模型对扰动的容忍度。
例如,可以采用Dropout、数据增强等方法来增加模型的鲁棒性。
(2)防御方法二:输入检测对输入样本进行检测,通过判断样本是否为对抗样本,可以有选择地对样本进行处理。
例如,可以使用输入空间的异常检测方法,对于异常的样本进行过滤或处理。
(3)防御方法三:提高模型复杂度提高模型的复杂度,增加模型的层数或参数量,能够增加攻击者生成对抗样本的难度。
《2024年可添加量不受限的对抗样本》范文
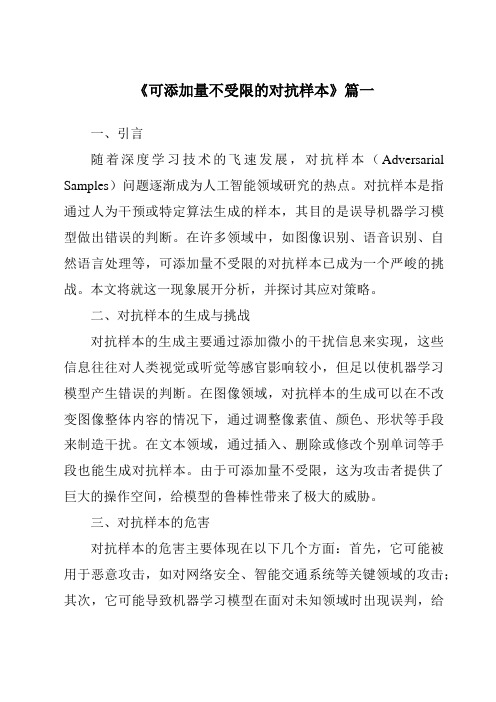
《可添加量不受限的对抗样本》篇一一、引言随着深度学习技术的飞速发展,对抗样本(Adversarial Samples)问题逐渐成为人工智能领域研究的热点。
对抗样本是指通过人为干预或特定算法生成的样本,其目的是误导机器学习模型做出错误的判断。
在许多领域中,如图像识别、语音识别、自然语言处理等,可添加量不受限的对抗样本已成为一个严峻的挑战。
本文将就这一现象展开分析,并探讨其应对策略。
二、对抗样本的生成与挑战对抗样本的生成主要通过添加微小的干扰信息来实现,这些信息往往对人类视觉或听觉等感官影响较小,但足以使机器学习模型产生错误的判断。
在图像领域,对抗样本的生成可以在不改变图像整体内容的情况下,通过调整像素值、颜色、形状等手段来制造干扰。
在文本领域,通过插入、删除或修改个别单词等手段也能生成对抗样本。
由于可添加量不受限,这为攻击者提供了巨大的操作空间,给模型的鲁棒性带来了极大的威胁。
三、对抗样本的危害对抗样本的危害主要体现在以下几个方面:首先,它可能被用于恶意攻击,如对网络安全、智能交通系统等关键领域的攻击;其次,它可能导致机器学习模型在面对未知领域时出现误判,给实际应用带来风险;最后,它可能被用于欺诈、伪造等不法行为,对社会造成不良影响。
四、应对策略针对可添加量不受限的对抗样本问题,我们提出以下应对策略:1. 增强模型的鲁棒性:通过优化模型结构、提高模型泛化能力等方法,降低模型对对抗样本的敏感性。
例如,采用对抗训练(Adversarial Training)技术,使模型能够在面对对抗样本时仍能保持较高的准确率。
2. 完善数据集:增加不同领域的训练数据,提高模型的适应性。
同时,对已知的对抗样本进行收集和标注,以便在训练过程中对模型进行针对性的优化。
3. 引入人类知识:结合人类专家的判断力,对机器学习模型的输出进行二次验证。
例如,在医疗诊断领域,可以借助医生的专业知识对模型的诊断结果进行校验。
4. 法律法规约束:加强对抗样本问题的法律监管力度,明确使用对抗样本进行恶意攻击的违法性质和处罚措施。
对抗样本产生原理及应对策略思考

对抗样本产生原理及应对策略思考近年来,随着深度学习和人工智能技术的快速发展,对抗样本攻击也逐渐成为一个备受关注的问题。
对抗样本是指通过对输入数据进行微小的扰动,使得深度学习模型在处理这些扰动后的样本时产生错误的分类结果。
这种攻击方式引发了对深度学习模型的可靠性和安全性的担忧。
本文将探讨对抗样本的产生原理,并提出一些应对策略的思考。
**1. 对抗样本的产生原理**对抗样本的产生原理可以分为两种类型:基于梯度信息的攻击和非基于梯度信息的攻击。
**基于梯度信息的攻击**基于梯度信息的攻击是指攻击者通过分析目标模型的梯度信息,针对输入样本进行扰动,以达到改变分类结果的目的。
其中,最为常见的攻击算法包括快速梯度符号方法(FGSM)、基于迭代的方法(如PGD)等。
FGSM攻击是一种简单而有效的对抗样本产生方法。
其基本思想是在输入样本上添加一个综合利用模型的梯度符号信息的小扰动,使模型错误地对样本进行分类。
PGD则是对FGSM的改进,在每一步迭代中增加随机扰动,增强了攻击效果。
**非基于梯度信息的攻击**非基于梯度信息的攻击方式不依赖模型的梯度信息,在攻击过程中仅使用样本的输入、输出信息。
这类攻击方法中,最著名的是黑盒攻击算法,其主要目标是通过在目标模型上产生的一系列输入输出样本来近似目标模型。
**2. 应对对抗样本的策略思考**虽然对抗样本攻击具有一定的威胁性,但研究者们也提出了一些有效的应对策略,以提高深度学习模型的鲁棒性。
**模型训练策略**一种常见的应对策略是通过对模型的训练过程进行改进,以增强对抗样本攻击的鲁棒性。
例如,在训练过程中引入对抗样本,通过与原始样本一同训练,使模型学习到对抗样本的特征,提高鲁棒性。
同时,正则化方法(如L2正则化)也可以用来限制模型在输入空间中的变化,减小对抗样本攻击的效果。
**防御性预处理**防御性预处理是指在输入样本进入深度学习模型之前对其进行处理,以减弱或抵御对抗样本攻击。
面向对抗攻击的机器学习研究

面向对抗攻击的机器学习研究机器学习是一种强大的工具,能够快速地解决各种问题。
但是,机器学习模型也很容易受到对抗攻击的影响。
对抗攻击是指有意地修改数据,使机器学习算法产生错误的结果。
这种攻击可能在自动驾驶、语音识别、金融交易等领域中造成严重后果。
因此,对抗攻击的机器学习研究变得至关重要。
对抗样本的生成是对抗攻击研究的核心问题。
目前,最常见的对抗攻击方法是PGD攻击。
PGD攻击是一种迭代攻击方法,它通过多次对模型输入数据进行微小的扰动,并不断地反复输入到模型中,以达到最终的攻击目的。
这种攻击方法的优点是不会降低攻击成功率,缺点是计算成本较高,容易被检测到。
为了提高对抗攻击中模型的鲁棒性,近年来的研究集中在以下几个方面:第一,对抗训练。
对抗训练是指在训练数据中添加针对PGD攻击的对抗样本,以提高模型的鲁棒性。
对抗训练的优点是实现简单,缺点是不能保证模型的鲁棒性,因为攻击者可以使用其他类型的攻击进行攻击。
第二,正则化方法。
正则化方法是指在损失函数中添加正则化项,以对抗攻击增加惩罚项。
与对抗训练相比,正则化方法可以增加模型的鲁棒性,并且不需要添加附加的对抗数据。
常用的正则化方法包括:对抗性训练。
对抗训练通过添加人工生成的对抗样本来提高模型的鲁棒性;对抗性正则化。
对抗性正则化通过对损失函数进行扩展,来加入正则化项,增强模型的鲁棒性;基于梯度投影的对抗性正则化。
第三,敌对训练。
敌对训练是指一种以深度强化学习为基础的算法,该算法设计了一组针对模型的不同类型攻击的敌对代理。
相比于对抗训练和正则化方法,敌对训练可以解决对抗攻击造成的强制性特征受损问题,产生的模型具有更好的鲁棒性。
对抗攻击是机器学习中的一项重要问题,因此,研究对抗攻击对机器学习模型鲁棒性的影响,以及提高对抗攻击中模型的鲁棒性是近年来的研究重点。
由于对抗攻击的复杂性,未来的对抗攻击研究可能集中于自动化对抗攻击检测和防御技术的开发。
Adversarial Examples(对抗样本)

3
Fig. 1: Example of attacks on deep learning models with ‘universal adversarial perturbations’ : The attacks are shown for the CaffeNet, VGG-F network and GoogLeNet. All the networks recognized the original clean images correctly with high confidence. After small perturbations were added to the images, the networks predicted wrong labels with similar high confidence. Notice that the perturbations are hardly perceptible for human vision system, however their effects on the deep learning models are catastrophic. 2018/5/15
4
Definition of terms
• Adversarial example/image is a modified version of a clean
image that is intentionally perturbed (e.g. by adding noise) to confuse/fool a machine learning technique, such as deep neural networks. • Adversarial perturbation is the noise that is added to the clean image to make it an adversarial example. • Adversarial training uses adversarial images besides the clean
adversarial 名词

adversarial 名词
Adversarial是一个形容词,表示对立的、敌对的、对抗性的。
在机器学习中,Adversarial通常用来形容针对模型的攻击或测试,即对抗样本(Adversarial examples)。
与Adversarial相关的一些词汇和概念包括:
1. Adversarial attack:对抗攻击,即针对机器学习模型的攻击,旨在改变模型的输出。
2. Adversarial example:对抗样本,经过特定的扰动处理后,能够欺骗机器学习模型产生错误分类结果的样本。
3. Adversarial training:对抗训练,是一种训练模型的方法,通过引入对抗样本来提高模型的鲁棒性。
4. Adversarial defense:对抗防御,是一种针对对抗攻击的防御方法,如检测对抗样本或对抗训练等。
5. Gradient descent:梯度下降,是一种优化算法,通过计算损失函数的梯度来更新模型参数,从而使损失函数最小化。
针对Adversarial攻击,有一些常见的防御方法,包括对抗训练、对抗样本检测、加噪声等。
此外,加强模型的鲁棒性、提高模型的泛化能力也是防御对抗攻击的有效手段。
在学习英语时,除了掌握相关的词汇和概念外,还需要多加练习和实践。
通过阅读相关文献、参与讨论、实践编程等方式,加深理解和掌握Adversarial相关的知识和技能。
此外,还可以参考英语学习的一些技巧,如多听多说、背单词、做阅读练习等,提高英语水平。
- 1、下载文档前请自行甄别文档内容的完整性,平台不提供额外的编辑、内容补充、找答案等附加服务。
- 2、"仅部分预览"的文档,不可在线预览部分如存在完整性等问题,可反馈申请退款(可完整预览的文档不适用该条件!)。
- 3、如文档侵犯您的权益,请联系客服反馈,我们会尽快为您处理(人工客服工作时间:9:00-18:30)。
Creating poisoning and evasion attack points is not a trivial task, particularly when many online services avoid disclosing information about their machine learning algorithms. As a result, attackers are forced to craft their attacks in black-box settings, against a surrogate model instead of the real model used by the service, hoping that the attack will be effective on the real model. The transferability property of an attack is satisfied when an attack developed for a particular machine learning model (i.e., a surrogate model) is also effective against the target model. Attack transferability was observed in early studies on adversarial examples [13, 41] and has gained a lot more interest in recent years with the advancement of machine learning cloud services. Previous work has reported empirical findings about the transferability of evasion attacks [3, 12, 13, 19, 24, 30, 31, 41, 42, 45] and, only recently, also on the transferability of poisoning integrity attacks [40]. In spite of these efforts, the question of when and why do adversarial points transfer remains largely unanswered.
Marco Melis
DIEE, University of Cagliari, Italy
Maura Pintor
DIEE, University of Cagliari, Italy
Matthew Jagielski
Northeastern University, Boston, MA
Battista Biggio
1 INTRODUCTION
The wide adoption of machine learning (ML) and deep learning algorithms in many critical applications introduces strong incentives for motivated adversaries to manipulate the results and models generated by these algorithms. Attacks against machine learning systems can happen during multiple stages in the learning pipeline. For instance, in many settings training data is collected online and thus can not be fully trusted. In poisoning availability attacks, the attacker controls a certain amount of training data, thus influencing the trained model and ultimately the predictions at testing time on most points in testing set [4, 16, 18, 26–28, 33, 35, 40, 46]. Poisoning integrity attacks have the goal of modifying predictions on a few targeted points by manipulating the training process [18, 40].
On the other hand, evasion attacks involve small manipulations of testing data points that results in misprediction at testing time on those points [3, 7, 9, 13, 30, 37, 41, 44, 47].
DIEE, University of Cagliari, Italy Pluribus One
ABSTRACT
Transferability captures the ability of an attack against a machinelearning model to be effective against a different, potentially unknown, model. Studying transferability of attacks has gained interest in the last years due to deployment of cyber-attack detection services based on machine learning. For these applications of machine learning, service providers avoid disclosing information about their machine-learning algorithms. As a result, attackers trying to bypass detection are forced to craft their attacks against a surrogate model instead of the actual target model used by the service. While previous work has shown that finding test-time transferable attack samples is possible, it is not well understood how an attacker may construct adversarial examples that are likely to transfer against different models, in particular in the case of training-time poisoning attacks. In this paper, we present the first empirical analysis aimed to investigate the transferability of both test-time evasion and training-time poisoning attacks. We provide a unifying, formal definition of transferability of such attacks and show how it relates to the input gradients of the surrogate and of the target classification models. We assess to which extent some of the most well-known machine-learning systems are vulnerable to transfer attacks, and explain why such attacks succeed (or not) across different models. To this end, we leverage some interesting connections highlighted in this work among the adversarial vulnerability of machine-learning models, their regularization hyperparameters and input gradients.