Practical, Distributed Network Coordinates
人工智能领域中英文专有名词汇总
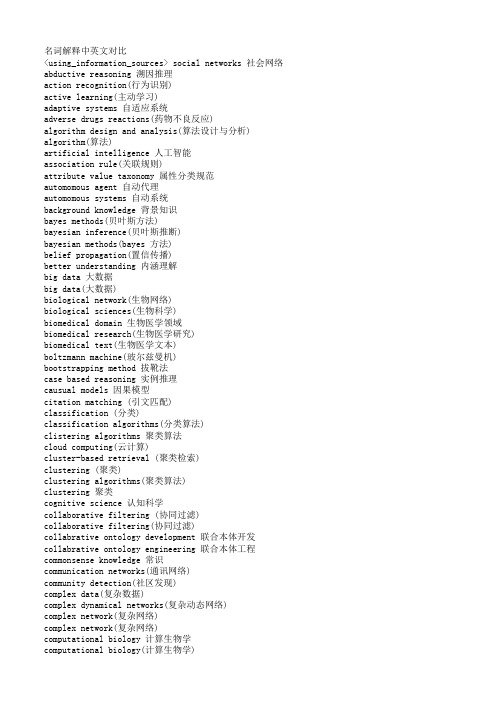
名词解释中英文对比<using_information_sources> social networks 社会网络abductive reasoning 溯因推理action recognition(行为识别)active learning(主动学习)adaptive systems 自适应系统adverse drugs reactions(药物不良反应)algorithm design and analysis(算法设计与分析) algorithm(算法)artificial intelligence 人工智能association rule(关联规则)attribute value taxonomy 属性分类规范automomous agent 自动代理automomous systems 自动系统background knowledge 背景知识bayes methods(贝叶斯方法)bayesian inference(贝叶斯推断)bayesian methods(bayes 方法)belief propagation(置信传播)better understanding 内涵理解big data 大数据big data(大数据)biological network(生物网络)biological sciences(生物科学)biomedical domain 生物医学领域biomedical research(生物医学研究)biomedical text(生物医学文本)boltzmann machine(玻尔兹曼机)bootstrapping method 拔靴法case based reasoning 实例推理causual models 因果模型citation matching (引文匹配)classification (分类)classification algorithms(分类算法)clistering algorithms 聚类算法cloud computing(云计算)cluster-based retrieval (聚类检索)clustering (聚类)clustering algorithms(聚类算法)clustering 聚类cognitive science 认知科学collaborative filtering (协同过滤)collaborative filtering(协同过滤)collabrative ontology development 联合本体开发collabrative ontology engineering 联合本体工程commonsense knowledge 常识communication networks(通讯网络)community detection(社区发现)complex data(复杂数据)complex dynamical networks(复杂动态网络)complex network(复杂网络)complex network(复杂网络)computational biology 计算生物学computational biology(计算生物学)computational complexity(计算复杂性) computational intelligence 智能计算computational modeling(计算模型)computer animation(计算机动画)computer networks(计算机网络)computer science 计算机科学concept clustering 概念聚类concept formation 概念形成concept learning 概念学习concept map 概念图concept model 概念模型concept modelling 概念模型conceptual model 概念模型conditional random field(条件随机场模型) conjunctive quries 合取查询constrained least squares (约束最小二乘) convex programming(凸规划)convolutional neural networks(卷积神经网络) customer relationship management(客户关系管理) data analysis(数据分析)data analysis(数据分析)data center(数据中心)data clustering (数据聚类)data compression(数据压缩)data envelopment analysis (数据包络分析)data fusion 数据融合data generation(数据生成)data handling(数据处理)data hierarchy (数据层次)data integration(数据整合)data integrity 数据完整性data intensive computing(数据密集型计算)data management 数据管理data management(数据管理)data management(数据管理)data miningdata mining 数据挖掘data model 数据模型data models(数据模型)data partitioning 数据划分data point(数据点)data privacy(数据隐私)data security(数据安全)data stream(数据流)data streams(数据流)data structure( 数据结构)data structure(数据结构)data visualisation(数据可视化)data visualization 数据可视化data visualization(数据可视化)data warehouse(数据仓库)data warehouses(数据仓库)data warehousing(数据仓库)database management systems(数据库管理系统)database management(数据库管理)date interlinking 日期互联date linking 日期链接Decision analysis(决策分析)decision maker 决策者decision making (决策)decision models 决策模型decision models 决策模型decision rule 决策规则decision support system 决策支持系统decision support systems (决策支持系统) decision tree(决策树)decission tree 决策树deep belief network(深度信念网络)deep learning(深度学习)defult reasoning 默认推理density estimation(密度估计)design methodology 设计方法论dimension reduction(降维) dimensionality reduction(降维)directed graph(有向图)disaster management 灾害管理disastrous event(灾难性事件)discovery(知识发现)dissimilarity (相异性)distributed databases 分布式数据库distributed databases(分布式数据库) distributed query 分布式查询document clustering (文档聚类)domain experts 领域专家domain knowledge 领域知识domain specific language 领域专用语言dynamic databases(动态数据库)dynamic logic 动态逻辑dynamic network(动态网络)dynamic system(动态系统)earth mover's distance(EMD 距离) education 教育efficient algorithm(有效算法)electric commerce 电子商务electronic health records(电子健康档案) entity disambiguation 实体消歧entity recognition 实体识别entity recognition(实体识别)entity resolution 实体解析event detection 事件检测event detection(事件检测)event extraction 事件抽取event identificaton 事件识别exhaustive indexing 完整索引expert system 专家系统expert systems(专家系统)explanation based learning 解释学习factor graph(因子图)feature extraction 特征提取feature extraction(特征提取)feature extraction(特征提取)feature selection (特征选择)feature selection 特征选择feature selection(特征选择)feature space 特征空间first order logic 一阶逻辑formal logic 形式逻辑formal meaning prepresentation 形式意义表示formal semantics 形式语义formal specification 形式描述frame based system 框为本的系统frequent itemsets(频繁项目集)frequent pattern(频繁模式)fuzzy clustering (模糊聚类)fuzzy clustering (模糊聚类)fuzzy clustering (模糊聚类)fuzzy data mining(模糊数据挖掘)fuzzy logic 模糊逻辑fuzzy set theory(模糊集合论)fuzzy set(模糊集)fuzzy sets 模糊集合fuzzy systems 模糊系统gaussian processes(高斯过程)gene expression data 基因表达数据gene expression(基因表达)generative model(生成模型)generative model(生成模型)genetic algorithm 遗传算法genome wide association study(全基因组关联分析) graph classification(图分类)graph classification(图分类)graph clustering(图聚类)graph data(图数据)graph data(图形数据)graph database 图数据库graph database(图数据库)graph mining(图挖掘)graph mining(图挖掘)graph partitioning 图划分graph query 图查询graph structure(图结构)graph theory(图论)graph theory(图论)graph theory(图论)graph theroy 图论graph visualization(图形可视化)graphical user interface 图形用户界面graphical user interfaces(图形用户界面)health care 卫生保健health care(卫生保健)heterogeneous data source 异构数据源heterogeneous data(异构数据)heterogeneous database 异构数据库heterogeneous information network(异构信息网络) heterogeneous network(异构网络)heterogenous ontology 异构本体heuristic rule 启发式规则hidden markov model(隐马尔可夫模型)hidden markov model(隐马尔可夫模型)hidden markov models(隐马尔可夫模型) hierarchical clustering (层次聚类) homogeneous network(同构网络)human centered computing 人机交互技术human computer interaction 人机交互human interaction 人机交互human robot interaction 人机交互image classification(图像分类)image clustering (图像聚类)image mining( 图像挖掘)image reconstruction(图像重建)image retrieval (图像检索)image segmentation(图像分割)inconsistent ontology 本体不一致incremental learning(增量学习)inductive learning (归纳学习)inference mechanisms 推理机制inference mechanisms(推理机制)inference rule 推理规则information cascades(信息追随)information diffusion(信息扩散)information extraction 信息提取information filtering(信息过滤)information filtering(信息过滤)information integration(信息集成)information network analysis(信息网络分析) information network mining(信息网络挖掘) information network(信息网络)information processing 信息处理information processing 信息处理information resource management (信息资源管理) information retrieval models(信息检索模型) information retrieval 信息检索information retrieval(信息检索)information retrieval(信息检索)information science 情报科学information sources 信息源information system( 信息系统)information system(信息系统)information technology(信息技术)information visualization(信息可视化)instance matching 实例匹配intelligent assistant 智能辅助intelligent systems 智能系统interaction network(交互网络)interactive visualization(交互式可视化)kernel function(核函数)kernel operator (核算子)keyword search(关键字检索)knowledege reuse 知识再利用knowledgeknowledgeknowledge acquisitionknowledge base 知识库knowledge based system 知识系统knowledge building 知识建构knowledge capture 知识获取knowledge construction 知识建构knowledge discovery(知识发现)knowledge extraction 知识提取knowledge fusion 知识融合knowledge integrationknowledge management systems 知识管理系统knowledge management 知识管理knowledge management(知识管理)knowledge model 知识模型knowledge reasoningknowledge representationknowledge representation(知识表达) knowledge sharing 知识共享knowledge storageknowledge technology 知识技术knowledge verification 知识验证language model(语言模型)language modeling approach(语言模型方法) large graph(大图)large graph(大图)learning(无监督学习)life science 生命科学linear programming(线性规划)link analysis (链接分析)link prediction(链接预测)link prediction(链接预测)link prediction(链接预测)linked data(关联数据)location based service(基于位置的服务) loclation based services(基于位置的服务) logic programming 逻辑编程logical implication 逻辑蕴涵logistic regression(logistic 回归)machine learning 机器学习machine translation(机器翻译)management system(管理系统)management( 知识管理)manifold learning(流形学习)markov chains 马尔可夫链markov processes(马尔可夫过程)matching function 匹配函数matrix decomposition(矩阵分解)matrix decomposition(矩阵分解)maximum likelihood estimation(最大似然估计)medical research(医学研究)mixture of gaussians(混合高斯模型)mobile computing(移动计算)multi agnet systems 多智能体系统multiagent systems 多智能体系统multimedia 多媒体natural language processing 自然语言处理natural language processing(自然语言处理) nearest neighbor (近邻)network analysis( 网络分析)network analysis(网络分析)network analysis(网络分析)network formation(组网)network structure(网络结构)network theory(网络理论)network topology(网络拓扑)network visualization(网络可视化)neural network(神经网络)neural networks (神经网络)neural networks(神经网络)nonlinear dynamics(非线性动力学)nonmonotonic reasoning 非单调推理nonnegative matrix factorization (非负矩阵分解) nonnegative matrix factorization(非负矩阵分解) object detection(目标检测)object oriented 面向对象object recognition(目标识别)object recognition(目标识别)online community(网络社区)online social network(在线社交网络)online social networks(在线社交网络)ontology alignment 本体映射ontology development 本体开发ontology engineering 本体工程ontology evolution 本体演化ontology extraction 本体抽取ontology interoperablity 互用性本体ontology language 本体语言ontology mapping 本体映射ontology matching 本体匹配ontology versioning 本体版本ontology 本体论open government data 政府公开数据opinion analysis(舆情分析)opinion mining(意见挖掘)opinion mining(意见挖掘)outlier detection(孤立点检测)parallel processing(并行处理)patient care(病人医疗护理)pattern classification(模式分类)pattern matching(模式匹配)pattern mining(模式挖掘)pattern recognition 模式识别pattern recognition(模式识别)pattern recognition(模式识别)personal data(个人数据)prediction algorithms(预测算法)predictive model 预测模型predictive models(预测模型)privacy preservation(隐私保护)probabilistic logic(概率逻辑)probabilistic logic(概率逻辑)probabilistic model(概率模型)probabilistic model(概率模型)probability distribution(概率分布)probability distribution(概率分布)project management(项目管理)pruning technique(修剪技术)quality management 质量管理query expansion(查询扩展)query language 查询语言query language(查询语言)query processing(查询处理)query rewrite 查询重写question answering system 问答系统random forest(随机森林)random graph(随机图)random processes(随机过程)random walk(随机游走)range query(范围查询)RDF database 资源描述框架数据库RDF query 资源描述框架查询RDF repository 资源描述框架存储库RDF storge 资源描述框架存储real time(实时)recommender system(推荐系统)recommender system(推荐系统)recommender systems 推荐系统recommender systems(推荐系统)record linkage 记录链接recurrent neural network(递归神经网络) regression(回归)reinforcement learning 强化学习reinforcement learning(强化学习)relation extraction 关系抽取relational database 关系数据库relational learning 关系学习relevance feedback (相关反馈)resource description framework 资源描述框架restricted boltzmann machines(受限玻尔兹曼机) retrieval models(检索模型)rough set theroy 粗糙集理论rough set 粗糙集rule based system 基于规则系统rule based 基于规则rule induction (规则归纳)rule learning (规则学习)rule learning 规则学习schema mapping 模式映射schema matching 模式匹配scientific domain 科学域search problems(搜索问题)semantic (web) technology 语义技术semantic analysis 语义分析semantic annotation 语义标注semantic computing 语义计算semantic integration 语义集成semantic interpretation 语义解释semantic model 语义模型semantic network 语义网络semantic relatedness 语义相关性semantic relation learning 语义关系学习semantic search 语义检索semantic similarity 语义相似度semantic similarity(语义相似度)semantic web rule language 语义网规则语言semantic web 语义网semantic web(语义网)semantic workflow 语义工作流semi supervised learning(半监督学习)sensor data(传感器数据)sensor networks(传感器网络)sentiment analysis(情感分析)sentiment analysis(情感分析)sequential pattern(序列模式)service oriented architecture 面向服务的体系结构shortest path(最短路径)similar kernel function(相似核函数)similarity measure(相似性度量)similarity relationship (相似关系)similarity search(相似搜索)similarity(相似性)situation aware 情境感知social behavior(社交行为)social influence(社会影响)social interaction(社交互动)social interaction(社交互动)social learning(社会学习)social life networks(社交生活网络)social machine 社交机器social media(社交媒体)social media(社交媒体)social media(社交媒体)social network analysis 社会网络分析social network analysis(社交网络分析)social network(社交网络)social network(社交网络)social science(社会科学)social tagging system(社交标签系统)social tagging(社交标签)social web(社交网页)sparse coding(稀疏编码)sparse matrices(稀疏矩阵)sparse representation(稀疏表示)spatial database(空间数据库)spatial reasoning 空间推理statistical analysis(统计分析)statistical model 统计模型string matching(串匹配)structural risk minimization (结构风险最小化) structured data 结构化数据subgraph matching 子图匹配subspace clustering(子空间聚类)supervised learning( 有support vector machine 支持向量机support vector machines(支持向量机)system dynamics(系统动力学)tag recommendation(标签推荐)taxonmy induction 感应规范temporal logic 时态逻辑temporal reasoning 时序推理text analysis(文本分析)text anaylsis 文本分析text classification (文本分类)text data(文本数据)text mining technique(文本挖掘技术)text mining 文本挖掘text mining(文本挖掘)text summarization(文本摘要)thesaurus alignment 同义对齐time frequency analysis(时频分析)time series analysis( 时time series data(时间序列数据)time series data(时间序列数据)time series(时间序列)topic model(主题模型)topic modeling(主题模型)transfer learning 迁移学习triple store 三元组存储uncertainty reasoning 不精确推理undirected graph(无向图)unified modeling language 统一建模语言unsupervisedupper bound(上界)user behavior(用户行为)user generated content(用户生成内容)utility mining(效用挖掘)visual analytics(可视化分析)visual content(视觉内容)visual representation(视觉表征)visualisation(可视化)visualization technique(可视化技术) visualization tool(可视化工具)web 2.0(网络2.0)web forum(web 论坛)web mining(网络挖掘)web of data 数据网web ontology lanuage 网络本体语言web pages(web 页面)web resource 网络资源web science 万维科学web search (网络检索)web usage mining(web 使用挖掘)wireless networks 无线网络world knowledge 世界知识world wide web 万维网world wide web(万维网)xml database 可扩展标志语言数据库附录 2 Data Mining 知识图谱(共包含二级节点15 个,三级节点93 个)间序列分析)监督学习)领域 二级分类 三级分类。
swarm learning精读
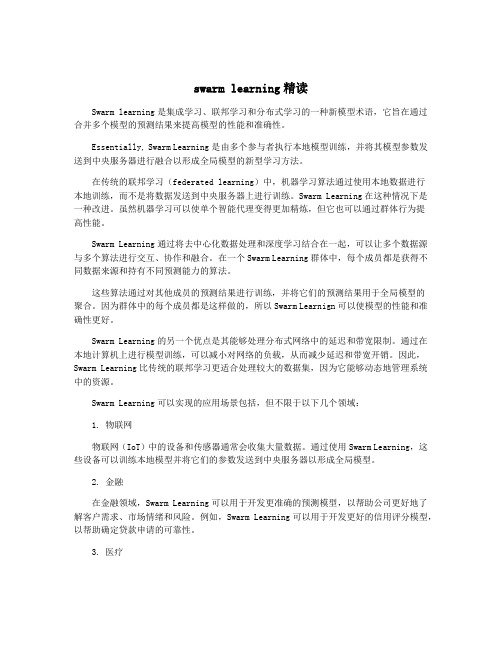
swarm learning精读Swarm learning是集成学习、联邦学习和分布式学习的一种新模型术语,它旨在通过合并多个模型的预测结果来提高模型的性能和准确性。
Essentially, Swarm Learning是由多个参与者执行本地模型训练,并将其模型参数发送到中央服务器进行融合以形成全局模型的新型学习方法。
在传统的联邦学习(federated learning)中,机器学习算法通过使用本地数据进行本地训练,而不是将数据发送到中央服务器上进行训练。
Swarm Learning在这种情况下是一种改进。
虽然机器学习可以使单个智能代理变得更加精炼,但它也可以通过群体行为提高性能。
Swarm Learning通过将去中心化数据处理和深度学习结合在一起,可以让多个数据源与多个算法进行交互、协作和融合。
在一个Swarm Learning群体中,每个成员都是获得不同数据来源和持有不同预测能力的算法。
这些算法通过对其他成员的预测结果进行训练,并将它们的预测结果用于全局模型的聚合。
因为群体中的每个成员都是这样做的,所以Swarm Learnign可以使模型的性能和准确性更好。
Swarm Learning的另一个优点是其能够处理分布式网络中的延迟和带宽限制。
通过在本地计算机上进行模型训练,可以减小对网络的负载,从而减少延迟和带宽开销。
因此,Swarm Learning比传统的联邦学习更适合处理较大的数据集,因为它能够动态地管理系统中的资源。
Swarm Learning可以实现的应用场景包括,但不限于以下几个领域:1. 物联网物联网(IoT)中的设备和传感器通常会收集大量数据。
通过使用Swarm Learning,这些设备可以训练本地模型并将它们的参数发送到中央服务器以形成全局模型。
2. 金融在金融领域,Swarm Learning可以用于开发更准确的预测模型,以帮助公司更好地了解客户需求、市场情绪和风险。
集成学习方法
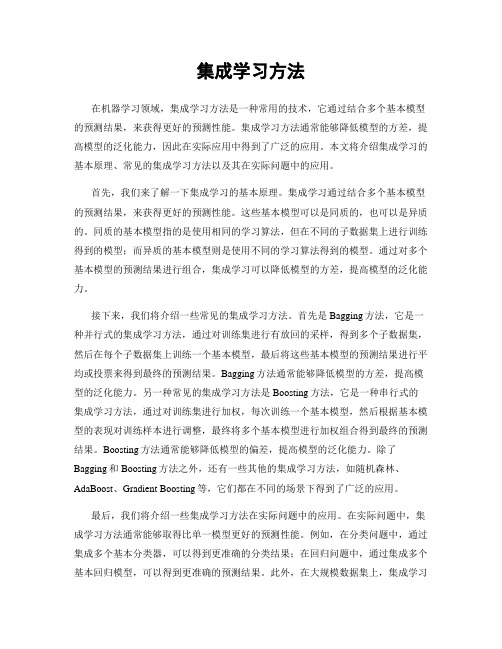
集成学习方法在机器学习领域,集成学习方法是一种常用的技术,它通过结合多个基本模型的预测结果,来获得更好的预测性能。
集成学习方法通常能够降低模型的方差,提高模型的泛化能力,因此在实际应用中得到了广泛的应用。
本文将介绍集成学习的基本原理、常见的集成学习方法以及其在实际问题中的应用。
首先,我们来了解一下集成学习的基本原理。
集成学习通过结合多个基本模型的预测结果,来获得更好的预测性能。
这些基本模型可以是同质的,也可以是异质的。
同质的基本模型指的是使用相同的学习算法,但在不同的子数据集上进行训练得到的模型;而异质的基本模型则是使用不同的学习算法得到的模型。
通过对多个基本模型的预测结果进行组合,集成学习可以降低模型的方差,提高模型的泛化能力。
接下来,我们将介绍一些常见的集成学习方法。
首先是Bagging方法,它是一种并行式的集成学习方法,通过对训练集进行有放回的采样,得到多个子数据集,然后在每个子数据集上训练一个基本模型,最后将这些基本模型的预测结果进行平均或投票来得到最终的预测结果。
Bagging方法通常能够降低模型的方差,提高模型的泛化能力。
另一种常见的集成学习方法是Boosting方法,它是一种串行式的集成学习方法,通过对训练集进行加权,每次训练一个基本模型,然后根据基本模型的表现对训练样本进行调整,最终将多个基本模型进行加权组合得到最终的预测结果。
Boosting方法通常能够降低模型的偏差,提高模型的泛化能力。
除了Bagging和Boosting方法之外,还有一些其他的集成学习方法,如随机森林、AdaBoost、Gradient Boosting等,它们都在不同的场景下得到了广泛的应用。
最后,我们将介绍一些集成学习方法在实际问题中的应用。
在实际问题中,集成学习方法通常能够取得比单一模型更好的预测性能。
例如,在分类问题中,通过集成多个基本分类器,可以得到更准确的分类结果;在回归问题中,通过集成多个基本回归模型,可以得到更准确的预测结果。
机器学习与人工智能领域中常用的英语词汇
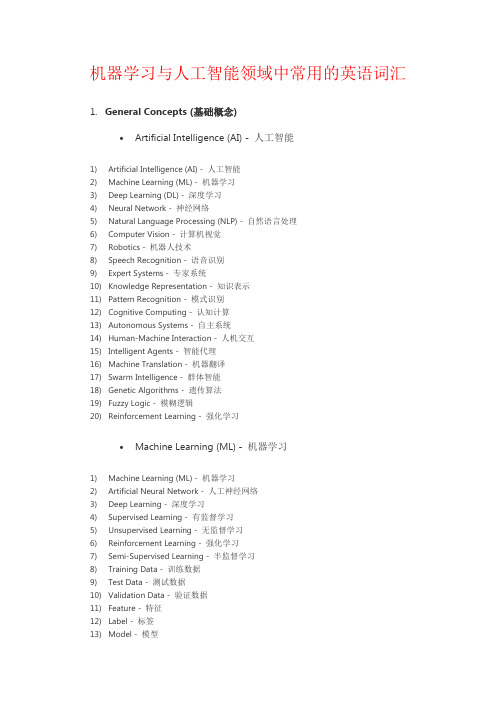
机器学习与人工智能领域中常用的英语词汇1.General Concepts (基础概念)•Artificial Intelligence (AI) - 人工智能1)Artificial Intelligence (AI) - 人工智能2)Machine Learning (ML) - 机器学习3)Deep Learning (DL) - 深度学习4)Neural Network - 神经网络5)Natural Language Processing (NLP) - 自然语言处理6)Computer Vision - 计算机视觉7)Robotics - 机器人技术8)Speech Recognition - 语音识别9)Expert Systems - 专家系统10)Knowledge Representation - 知识表示11)Pattern Recognition - 模式识别12)Cognitive Computing - 认知计算13)Autonomous Systems - 自主系统14)Human-Machine Interaction - 人机交互15)Intelligent Agents - 智能代理16)Machine Translation - 机器翻译17)Swarm Intelligence - 群体智能18)Genetic Algorithms - 遗传算法19)Fuzzy Logic - 模糊逻辑20)Reinforcement Learning - 强化学习•Machine Learning (ML) - 机器学习1)Machine Learning (ML) - 机器学习2)Artificial Neural Network - 人工神经网络3)Deep Learning - 深度学习4)Supervised Learning - 有监督学习5)Unsupervised Learning - 无监督学习6)Reinforcement Learning - 强化学习7)Semi-Supervised Learning - 半监督学习8)Training Data - 训练数据9)Test Data - 测试数据10)Validation Data - 验证数据11)Feature - 特征12)Label - 标签13)Model - 模型14)Algorithm - 算法15)Regression - 回归16)Classification - 分类17)Clustering - 聚类18)Dimensionality Reduction - 降维19)Overfitting - 过拟合20)Underfitting - 欠拟合•Deep Learning (DL) - 深度学习1)Deep Learning - 深度学习2)Neural Network - 神经网络3)Artificial Neural Network (ANN) - 人工神经网络4)Convolutional Neural Network (CNN) - 卷积神经网络5)Recurrent Neural Network (RNN) - 循环神经网络6)Long Short-Term Memory (LSTM) - 长短期记忆网络7)Gated Recurrent Unit (GRU) - 门控循环单元8)Autoencoder - 自编码器9)Generative Adversarial Network (GAN) - 生成对抗网络10)Transfer Learning - 迁移学习11)Pre-trained Model - 预训练模型12)Fine-tuning - 微调13)Feature Extraction - 特征提取14)Activation Function - 激活函数15)Loss Function - 损失函数16)Gradient Descent - 梯度下降17)Backpropagation - 反向传播18)Epoch - 训练周期19)Batch Size - 批量大小20)Dropout - 丢弃法•Neural Network - 神经网络1)Neural Network - 神经网络2)Artificial Neural Network (ANN) - 人工神经网络3)Deep Neural Network (DNN) - 深度神经网络4)Convolutional Neural Network (CNN) - 卷积神经网络5)Recurrent Neural Network (RNN) - 循环神经网络6)Long Short-Term Memory (LSTM) - 长短期记忆网络7)Gated Recurrent Unit (GRU) - 门控循环单元8)Feedforward Neural Network - 前馈神经网络9)Multi-layer Perceptron (MLP) - 多层感知器10)Radial Basis Function Network (RBFN) - 径向基函数网络11)Hopfield Network - 霍普菲尔德网络12)Boltzmann Machine - 玻尔兹曼机13)Autoencoder - 自编码器14)Spiking Neural Network (SNN) - 脉冲神经网络15)Self-organizing Map (SOM) - 自组织映射16)Restricted Boltzmann Machine (RBM) - 受限玻尔兹曼机17)Hebbian Learning - 海比安学习18)Competitive Learning - 竞争学习19)Neuroevolutionary - 神经进化20)Neuron - 神经元•Algorithm - 算法1)Algorithm - 算法2)Supervised Learning Algorithm - 有监督学习算法3)Unsupervised Learning Algorithm - 无监督学习算法4)Reinforcement Learning Algorithm - 强化学习算法5)Classification Algorithm - 分类算法6)Regression Algorithm - 回归算法7)Clustering Algorithm - 聚类算法8)Dimensionality Reduction Algorithm - 降维算法9)Decision Tree Algorithm - 决策树算法10)Random Forest Algorithm - 随机森林算法11)Support Vector Machine (SVM) Algorithm - 支持向量机算法12)K-Nearest Neighbors (KNN) Algorithm - K近邻算法13)Naive Bayes Algorithm - 朴素贝叶斯算法14)Gradient Descent Algorithm - 梯度下降算法15)Genetic Algorithm - 遗传算法16)Neural Network Algorithm - 神经网络算法17)Deep Learning Algorithm - 深度学习算法18)Ensemble Learning Algorithm - 集成学习算法19)Reinforcement Learning Algorithm - 强化学习算法20)Metaheuristic Algorithm - 元启发式算法•Model - 模型1)Model - 模型2)Machine Learning Model - 机器学习模型3)Artificial Intelligence Model - 人工智能模型4)Predictive Model - 预测模型5)Classification Model - 分类模型6)Regression Model - 回归模型7)Generative Model - 生成模型8)Discriminative Model - 判别模型9)Probabilistic Model - 概率模型10)Statistical Model - 统计模型11)Neural Network Model - 神经网络模型12)Deep Learning Model - 深度学习模型13)Ensemble Model - 集成模型14)Reinforcement Learning Model - 强化学习模型15)Support Vector Machine (SVM) Model - 支持向量机模型16)Decision Tree Model - 决策树模型17)Random Forest Model - 随机森林模型18)Naive Bayes Model - 朴素贝叶斯模型19)Autoencoder Model - 自编码器模型20)Convolutional Neural Network (CNN) Model - 卷积神经网络模型•Dataset - 数据集1)Dataset - 数据集2)Training Dataset - 训练数据集3)Test Dataset - 测试数据集4)Validation Dataset - 验证数据集5)Balanced Dataset - 平衡数据集6)Imbalanced Dataset - 不平衡数据集7)Synthetic Dataset - 合成数据集8)Benchmark Dataset - 基准数据集9)Open Dataset - 开放数据集10)Labeled Dataset - 标记数据集11)Unlabeled Dataset - 未标记数据集12)Semi-Supervised Dataset - 半监督数据集13)Multiclass Dataset - 多分类数据集14)Feature Set - 特征集15)Data Augmentation - 数据增强16)Data Preprocessing - 数据预处理17)Missing Data - 缺失数据18)Outlier Detection - 异常值检测19)Data Imputation - 数据插补20)Metadata - 元数据•Training - 训练1)Training - 训练2)Training Data - 训练数据3)Training Phase - 训练阶段4)Training Set - 训练集5)Training Examples - 训练样本6)Training Instance - 训练实例7)Training Algorithm - 训练算法8)Training Model - 训练模型9)Training Process - 训练过程10)Training Loss - 训练损失11)Training Epoch - 训练周期12)Training Batch - 训练批次13)Online Training - 在线训练14)Offline Training - 离线训练15)Continuous Training - 连续训练16)Transfer Learning - 迁移学习17)Fine-Tuning - 微调18)Curriculum Learning - 课程学习19)Self-Supervised Learning - 自监督学习20)Active Learning - 主动学习•Testing - 测试1)Testing - 测试2)Test Data - 测试数据3)Test Set - 测试集4)Test Examples - 测试样本5)Test Instance - 测试实例6)Test Phase - 测试阶段7)Test Accuracy - 测试准确率8)Test Loss - 测试损失9)Test Error - 测试错误10)Test Metrics - 测试指标11)Test Suite - 测试套件12)Test Case - 测试用例13)Test Coverage - 测试覆盖率14)Cross-Validation - 交叉验证15)Holdout Validation - 留出验证16)K-Fold Cross-Validation - K折交叉验证17)Stratified Cross-Validation - 分层交叉验证18)Test Driven Development (TDD) - 测试驱动开发19)A/B Testing - A/B 测试20)Model Evaluation - 模型评估•Validation - 验证1)Validation - 验证2)Validation Data - 验证数据3)Validation Set - 验证集4)Validation Examples - 验证样本5)Validation Instance - 验证实例6)Validation Phase - 验证阶段7)Validation Accuracy - 验证准确率8)Validation Loss - 验证损失9)Validation Error - 验证错误10)Validation Metrics - 验证指标11)Cross-Validation - 交叉验证12)Holdout Validation - 留出验证13)K-Fold Cross-Validation - K折交叉验证14)Stratified Cross-Validation - 分层交叉验证15)Leave-One-Out Cross-Validation - 留一法交叉验证16)Validation Curve - 验证曲线17)Hyperparameter Validation - 超参数验证18)Model Validation - 模型验证19)Early Stopping - 提前停止20)Validation Strategy - 验证策略•Supervised Learning - 有监督学习1)Supervised Learning - 有监督学习2)Label - 标签3)Feature - 特征4)Target - 目标5)Training Labels - 训练标签6)Training Features - 训练特征7)Training Targets - 训练目标8)Training Examples - 训练样本9)Training Instance - 训练实例10)Regression - 回归11)Classification - 分类12)Predictor - 预测器13)Regression Model - 回归模型14)Classifier - 分类器15)Decision Tree - 决策树16)Support Vector Machine (SVM) - 支持向量机17)Neural Network - 神经网络18)Feature Engineering - 特征工程19)Model Evaluation - 模型评估20)Overfitting - 过拟合21)Underfitting - 欠拟合22)Bias-Variance Tradeoff - 偏差-方差权衡•Unsupervised Learning - 无监督学习1)Unsupervised Learning - 无监督学习2)Clustering - 聚类3)Dimensionality Reduction - 降维4)Anomaly Detection - 异常检测5)Association Rule Learning - 关联规则学习6)Feature Extraction - 特征提取7)Feature Selection - 特征选择8)K-Means - K均值9)Hierarchical Clustering - 层次聚类10)Density-Based Clustering - 基于密度的聚类11)Principal Component Analysis (PCA) - 主成分分析12)Independent Component Analysis (ICA) - 独立成分分析13)T-distributed Stochastic Neighbor Embedding (t-SNE) - t分布随机邻居嵌入14)Gaussian Mixture Model (GMM) - 高斯混合模型15)Self-Organizing Maps (SOM) - 自组织映射16)Autoencoder - 自动编码器17)Latent Variable - 潜变量18)Data Preprocessing - 数据预处理19)Outlier Detection - 异常值检测20)Clustering Algorithm - 聚类算法•Reinforcement Learning - 强化学习1)Reinforcement Learning - 强化学习2)Agent - 代理3)Environment - 环境4)State - 状态5)Action - 动作6)Reward - 奖励7)Policy - 策略8)Value Function - 值函数9)Q-Learning - Q学习10)Deep Q-Network (DQN) - 深度Q网络11)Policy Gradient - 策略梯度12)Actor-Critic - 演员-评论家13)Exploration - 探索14)Exploitation - 开发15)Temporal Difference (TD) - 时间差分16)Markov Decision Process (MDP) - 马尔可夫决策过程17)State-Action-Reward-State-Action (SARSA) - 状态-动作-奖励-状态-动作18)Policy Iteration - 策略迭代19)Value Iteration - 值迭代20)Monte Carlo Methods - 蒙特卡洛方法•Semi-Supervised Learning - 半监督学习1)Semi-Supervised Learning - 半监督学习2)Labeled Data - 有标签数据3)Unlabeled Data - 无标签数据4)Label Propagation - 标签传播5)Self-Training - 自训练6)Co-Training - 协同训练7)Transudative Learning - 传导学习8)Inductive Learning - 归纳学习9)Manifold Regularization - 流形正则化10)Graph-based Methods - 基于图的方法11)Cluster Assumption - 聚类假设12)Low-Density Separation - 低密度分离13)Semi-Supervised Support Vector Machines (S3VM) - 半监督支持向量机14)Expectation-Maximization (EM) - 期望最大化15)Co-EM - 协同期望最大化16)Entropy-Regularized EM - 熵正则化EM17)Mean Teacher - 平均教师18)Virtual Adversarial Training - 虚拟对抗训练19)Tri-training - 三重训练20)Mix Match - 混合匹配•Feature - 特征1)Feature - 特征2)Feature Engineering - 特征工程3)Feature Extraction - 特征提取4)Feature Selection - 特征选择5)Input Features - 输入特征6)Output Features - 输出特征7)Feature Vector - 特征向量8)Feature Space - 特征空间9)Feature Representation - 特征表示10)Feature Transformation - 特征转换11)Feature Importance - 特征重要性12)Feature Scaling - 特征缩放13)Feature Normalization - 特征归一化14)Feature Encoding - 特征编码15)Feature Fusion - 特征融合16)Feature Dimensionality Reduction - 特征维度减少17)Continuous Feature - 连续特征18)Categorical Feature - 分类特征19)Nominal Feature - 名义特征20)Ordinal Feature - 有序特征•Label - 标签1)Label - 标签2)Labeling - 标注3)Ground Truth - 地面真值4)Class Label - 类别标签5)Target Variable - 目标变量6)Labeling Scheme - 标注方案7)Multi-class Labeling - 多类别标注8)Binary Labeling - 二分类标注9)Label Noise - 标签噪声10)Labeling Error - 标注错误11)Label Propagation - 标签传播12)Unlabeled Data - 无标签数据13)Labeled Data - 有标签数据14)Semi-supervised Learning - 半监督学习15)Active Learning - 主动学习16)Weakly Supervised Learning - 弱监督学习17)Noisy Label Learning - 噪声标签学习18)Self-training - 自训练19)Crowdsourcing Labeling - 众包标注20)Label Smoothing - 标签平滑化•Prediction - 预测1)Prediction - 预测2)Forecasting - 预测3)Regression - 回归4)Classification - 分类5)Time Series Prediction - 时间序列预测6)Forecast Accuracy - 预测准确性7)Predictive Modeling - 预测建模8)Predictive Analytics - 预测分析9)Forecasting Method - 预测方法10)Predictive Performance - 预测性能11)Predictive Power - 预测能力12)Prediction Error - 预测误差13)Prediction Interval - 预测区间14)Prediction Model - 预测模型15)Predictive Uncertainty - 预测不确定性16)Forecast Horizon - 预测时间跨度17)Predictive Maintenance - 预测性维护18)Predictive Policing - 预测式警务19)Predictive Healthcare - 预测性医疗20)Predictive Maintenance - 预测性维护•Classification - 分类1)Classification - 分类2)Classifier - 分类器3)Class - 类别4)Classify - 对数据进行分类5)Class Label - 类别标签6)Binary Classification - 二元分类7)Multiclass Classification - 多类分类8)Class Probability - 类别概率9)Decision Boundary - 决策边界10)Decision Tree - 决策树11)Support Vector Machine (SVM) - 支持向量机12)K-Nearest Neighbors (KNN) - K最近邻算法13)Naive Bayes - 朴素贝叶斯14)Logistic Regression - 逻辑回归15)Random Forest - 随机森林16)Neural Network - 神经网络17)SoftMax Function - SoftMax函数18)One-vs-All (One-vs-Rest) - 一对多(一对剩余)19)Ensemble Learning - 集成学习20)Confusion Matrix - 混淆矩阵•Regression - 回归1)Regression Analysis - 回归分析2)Linear Regression - 线性回归3)Multiple Regression - 多元回归4)Polynomial Regression - 多项式回归5)Logistic Regression - 逻辑回归6)Ridge Regression - 岭回归7)Lasso Regression - Lasso回归8)Elastic Net Regression - 弹性网络回归9)Regression Coefficients - 回归系数10)Residuals - 残差11)Ordinary Least Squares (OLS) - 普通最小二乘法12)Ridge Regression Coefficient - 岭回归系数13)Lasso Regression Coefficient - Lasso回归系数14)Elastic Net Regression Coefficient - 弹性网络回归系数15)Regression Line - 回归线16)Prediction Error - 预测误差17)Regression Model - 回归模型18)Nonlinear Regression - 非线性回归19)Generalized Linear Models (GLM) - 广义线性模型20)Coefficient of Determination (R-squared) - 决定系数21)F-test - F检验22)Homoscedasticity - 同方差性23)Heteroscedasticity - 异方差性24)Autocorrelation - 自相关25)Multicollinearity - 多重共线性26)Outliers - 异常值27)Cross-validation - 交叉验证28)Feature Selection - 特征选择29)Feature Engineering - 特征工程30)Regularization - 正则化2.Neural Networks and Deep Learning (神经网络与深度学习)•Convolutional Neural Network (CNN) - 卷积神经网络1)Convolutional Neural Network (CNN) - 卷积神经网络2)Convolution Layer - 卷积层3)Feature Map - 特征图4)Convolution Operation - 卷积操作5)Stride - 步幅6)Padding - 填充7)Pooling Layer - 池化层8)Max Pooling - 最大池化9)Average Pooling - 平均池化10)Fully Connected Layer - 全连接层11)Activation Function - 激活函数12)Rectified Linear Unit (ReLU) - 线性修正单元13)Dropout - 随机失活14)Batch Normalization - 批量归一化15)Transfer Learning - 迁移学习16)Fine-Tuning - 微调17)Image Classification - 图像分类18)Object Detection - 物体检测19)Semantic Segmentation - 语义分割20)Instance Segmentation - 实例分割21)Generative Adversarial Network (GAN) - 生成对抗网络22)Image Generation - 图像生成23)Style Transfer - 风格迁移24)Convolutional Autoencoder - 卷积自编码器25)Recurrent Neural Network (RNN) - 循环神经网络•Recurrent Neural Network (RNN) - 循环神经网络1)Recurrent Neural Network (RNN) - 循环神经网络2)Long Short-Term Memory (LSTM) - 长短期记忆网络3)Gated Recurrent Unit (GRU) - 门控循环单元4)Sequence Modeling - 序列建模5)Time Series Prediction - 时间序列预测6)Natural Language Processing (NLP) - 自然语言处理7)Text Generation - 文本生成8)Sentiment Analysis - 情感分析9)Named Entity Recognition (NER) - 命名实体识别10)Part-of-Speech Tagging (POS Tagging) - 词性标注11)Sequence-to-Sequence (Seq2Seq) - 序列到序列12)Attention Mechanism - 注意力机制13)Encoder-Decoder Architecture - 编码器-解码器架构14)Bidirectional RNN - 双向循环神经网络15)Teacher Forcing - 强制教师法16)Backpropagation Through Time (BPTT) - 通过时间的反向传播17)Vanishing Gradient Problem - 梯度消失问题18)Exploding Gradient Problem - 梯度爆炸问题19)Language Modeling - 语言建模20)Speech Recognition - 语音识别•Long Short-Term Memory (LSTM) - 长短期记忆网络1)Long Short-Term Memory (LSTM) - 长短期记忆网络2)Cell State - 细胞状态3)Hidden State - 隐藏状态4)Forget Gate - 遗忘门5)Input Gate - 输入门6)Output Gate - 输出门7)Peephole Connections - 窥视孔连接8)Gated Recurrent Unit (GRU) - 门控循环单元9)Vanishing Gradient Problem - 梯度消失问题10)Exploding Gradient Problem - 梯度爆炸问题11)Sequence Modeling - 序列建模12)Time Series Prediction - 时间序列预测13)Natural Language Processing (NLP) - 自然语言处理14)Text Generation - 文本生成15)Sentiment Analysis - 情感分析16)Named Entity Recognition (NER) - 命名实体识别17)Part-of-Speech Tagging (POS Tagging) - 词性标注18)Attention Mechanism - 注意力机制19)Encoder-Decoder Architecture - 编码器-解码器架构20)Bidirectional LSTM - 双向长短期记忆网络•Attention Mechanism - 注意力机制1)Attention Mechanism - 注意力机制2)Self-Attention - 自注意力3)Multi-Head Attention - 多头注意力4)Transformer - 变换器5)Query - 查询6)Key - 键7)Value - 值8)Query-Value Attention - 查询-值注意力9)Dot-Product Attention - 点积注意力10)Scaled Dot-Product Attention - 缩放点积注意力11)Additive Attention - 加性注意力12)Context Vector - 上下文向量13)Attention Score - 注意力分数14)SoftMax Function - SoftMax函数15)Attention Weight - 注意力权重16)Global Attention - 全局注意力17)Local Attention - 局部注意力18)Positional Encoding - 位置编码19)Encoder-Decoder Attention - 编码器-解码器注意力20)Cross-Modal Attention - 跨模态注意力•Generative Adversarial Network (GAN) - 生成对抗网络1)Generative Adversarial Network (GAN) - 生成对抗网络2)Generator - 生成器3)Discriminator - 判别器4)Adversarial Training - 对抗训练5)Minimax Game - 极小极大博弈6)Nash Equilibrium - 纳什均衡7)Mode Collapse - 模式崩溃8)Training Stability - 训练稳定性9)Loss Function - 损失函数10)Discriminative Loss - 判别损失11)Generative Loss - 生成损失12)Wasserstein GAN (WGAN) - Wasserstein GAN(WGAN)13)Deep Convolutional GAN (DCGAN) - 深度卷积生成对抗网络(DCGAN)14)Conditional GAN (c GAN) - 条件生成对抗网络(c GAN)15)Style GAN - 风格生成对抗网络16)Cycle GAN - 循环生成对抗网络17)Progressive Growing GAN (PGGAN) - 渐进式增长生成对抗网络(PGGAN)18)Self-Attention GAN (SAGAN) - 自注意力生成对抗网络(SAGAN)19)Big GAN - 大规模生成对抗网络20)Adversarial Examples - 对抗样本•Encoder-Decoder - 编码器-解码器1)Encoder-Decoder Architecture - 编码器-解码器架构2)Encoder - 编码器3)Decoder - 解码器4)Sequence-to-Sequence Model (Seq2Seq) - 序列到序列模型5)State Vector - 状态向量6)Context Vector - 上下文向量7)Hidden State - 隐藏状态8)Attention Mechanism - 注意力机制9)Teacher Forcing - 强制教师法10)Beam Search - 束搜索11)Recurrent Neural Network (RNN) - 循环神经网络12)Long Short-Term Memory (LSTM) - 长短期记忆网络13)Gated Recurrent Unit (GRU) - 门控循环单元14)Bidirectional Encoder - 双向编码器15)Greedy Decoding - 贪婪解码16)Masking - 遮盖17)Dropout - 随机失活18)Embedding Layer - 嵌入层19)Cross-Entropy Loss - 交叉熵损失20)Tokenization - 令牌化•Transfer Learning - 迁移学习1)Transfer Learning - 迁移学习2)Source Domain - 源领域3)Target Domain - 目标领域4)Fine-Tuning - 微调5)Domain Adaptation - 领域自适应6)Pre-Trained Model - 预训练模型7)Feature Extraction - 特征提取8)Knowledge Transfer - 知识迁移9)Unsupervised Domain Adaptation - 无监督领域自适应10)Semi-Supervised Domain Adaptation - 半监督领域自适应11)Multi-Task Learning - 多任务学习12)Data Augmentation - 数据增强13)Task Transfer - 任务迁移14)Model Agnostic Meta-Learning (MAML) - 与模型无关的元学习(MAML)15)One-Shot Learning - 单样本学习16)Zero-Shot Learning - 零样本学习17)Few-Shot Learning - 少样本学习18)Knowledge Distillation - 知识蒸馏19)Representation Learning - 表征学习20)Adversarial Transfer Learning - 对抗迁移学习•Pre-trained Models - 预训练模型1)Pre-trained Model - 预训练模型2)Transfer Learning - 迁移学习3)Fine-Tuning - 微调4)Knowledge Transfer - 知识迁移5)Domain Adaptation - 领域自适应6)Feature Extraction - 特征提取7)Representation Learning - 表征学习8)Language Model - 语言模型9)Bidirectional Encoder Representations from Transformers (BERT) - 双向编码器结构转换器10)Generative Pre-trained Transformer (GPT) - 生成式预训练转换器11)Transformer-based Models - 基于转换器的模型12)Masked Language Model (MLM) - 掩蔽语言模型13)Cloze Task - 填空任务14)Tokenization - 令牌化15)Word Embeddings - 词嵌入16)Sentence Embeddings - 句子嵌入17)Contextual Embeddings - 上下文嵌入18)Self-Supervised Learning - 自监督学习19)Large-Scale Pre-trained Models - 大规模预训练模型•Loss Function - 损失函数1)Loss Function - 损失函数2)Mean Squared Error (MSE) - 均方误差3)Mean Absolute Error (MAE) - 平均绝对误差4)Cross-Entropy Loss - 交叉熵损失5)Binary Cross-Entropy Loss - 二元交叉熵损失6)Categorical Cross-Entropy Loss - 分类交叉熵损失7)Hinge Loss - 合页损失8)Huber Loss - Huber损失9)Wasserstein Distance - Wasserstein距离10)Triplet Loss - 三元组损失11)Contrastive Loss - 对比损失12)Dice Loss - Dice损失13)Focal Loss - 焦点损失14)GAN Loss - GAN损失15)Adversarial Loss - 对抗损失16)L1 Loss - L1损失17)L2 Loss - L2损失18)Huber Loss - Huber损失19)Quantile Loss - 分位数损失•Activation Function - 激活函数1)Activation Function - 激活函数2)Sigmoid Function - Sigmoid函数3)Hyperbolic Tangent Function (Tanh) - 双曲正切函数4)Rectified Linear Unit (Re LU) - 矩形线性单元5)Parametric Re LU (P Re LU) - 参数化Re LU6)Exponential Linear Unit (ELU) - 指数线性单元7)Swish Function - Swish函数8)Softplus Function - Soft plus函数9)Softmax Function - SoftMax函数10)Hard Tanh Function - 硬双曲正切函数11)Softsign Function - Softsign函数12)GELU (Gaussian Error Linear Unit) - GELU(高斯误差线性单元)13)Mish Function - Mish函数14)CELU (Continuous Exponential Linear Unit) - CELU(连续指数线性单元)15)Bent Identity Function - 弯曲恒等函数16)Gaussian Error Linear Units (GELUs) - 高斯误差线性单元17)Adaptive Piecewise Linear (APL) - 自适应分段线性函数18)Radial Basis Function (RBF) - 径向基函数•Backpropagation - 反向传播1)Backpropagation - 反向传播2)Gradient Descent - 梯度下降3)Partial Derivative - 偏导数4)Chain Rule - 链式法则5)Forward Pass - 前向传播6)Backward Pass - 反向传播7)Computational Graph - 计算图8)Neural Network - 神经网络9)Loss Function - 损失函数10)Gradient Calculation - 梯度计算11)Weight Update - 权重更新12)Activation Function - 激活函数13)Optimizer - 优化器14)Learning Rate - 学习率15)Mini-Batch Gradient Descent - 小批量梯度下降16)Stochastic Gradient Descent (SGD) - 随机梯度下降17)Batch Gradient Descent - 批量梯度下降18)Momentum - 动量19)Adam Optimizer - Adam优化器20)Learning Rate Decay - 学习率衰减•Gradient Descent - 梯度下降1)Gradient Descent - 梯度下降2)Stochastic Gradient Descent (SGD) - 随机梯度下降3)Mini-Batch Gradient Descent - 小批量梯度下降4)Batch Gradient Descent - 批量梯度下降5)Learning Rate - 学习率6)Momentum - 动量7)Adaptive Moment Estimation (Adam) - 自适应矩估计8)RMSprop - 均方根传播9)Learning Rate Schedule - 学习率调度10)Convergence - 收敛11)Divergence - 发散12)Adagrad - 自适应学习速率方法13)Adadelta - 自适应增量学习率方法14)Adamax - 自适应矩估计的扩展版本15)Nadam - Nesterov Accelerated Adaptive Moment Estimation16)Learning Rate Decay - 学习率衰减17)Step Size - 步长18)Conjugate Gradient Descent - 共轭梯度下降19)Line Search - 线搜索20)Newton's Method - 牛顿法•Learning Rate - 学习率1)Learning Rate - 学习率2)Adaptive Learning Rate - 自适应学习率3)Learning Rate Decay - 学习率衰减4)Initial Learning Rate - 初始学习率5)Step Size - 步长6)Momentum - 动量7)Exponential Decay - 指数衰减8)Annealing - 退火9)Cyclical Learning Rate - 循环学习率10)Learning Rate Schedule - 学习率调度11)Warm-up - 预热12)Learning Rate Policy - 学习率策略13)Learning Rate Annealing - 学习率退火14)Cosine Annealing - 余弦退火15)Gradient Clipping - 梯度裁剪16)Adapting Learning Rate - 适应学习率17)Learning Rate Multiplier - 学习率倍增器18)Learning Rate Reduction - 学习率降低19)Learning Rate Update - 学习率更新20)Scheduled Learning Rate - 定期学习率•Batch Size - 批量大小1)Batch Size - 批量大小2)Mini-Batch - 小批量3)Batch Gradient Descent - 批量梯度下降4)Stochastic Gradient Descent (SGD) - 随机梯度下降5)Mini-Batch Gradient Descent - 小批量梯度下降6)Online Learning - 在线学习7)Full-Batch - 全批量8)Data Batch - 数据批次9)Training Batch - 训练批次10)Batch Normalization - 批量归一化11)Batch-wise Optimization - 批量优化12)Batch Processing - 批量处理13)Batch Sampling - 批量采样14)Adaptive Batch Size - 自适应批量大小15)Batch Splitting - 批量分割16)Dynamic Batch Size - 动态批量大小17)Fixed Batch Size - 固定批量大小18)Batch-wise Inference - 批量推理19)Batch-wise Training - 批量训练20)Batch Shuffling - 批量洗牌•Epoch - 训练周期1)Training Epoch - 训练周期2)Epoch Size - 周期大小3)Early Stopping - 提前停止4)Validation Set - 验证集5)Training Set - 训练集6)Test Set - 测试集7)Overfitting - 过拟合8)Underfitting - 欠拟合9)Model Evaluation - 模型评估10)Model Selection - 模型选择11)Hyperparameter Tuning - 超参数调优12)Cross-Validation - 交叉验证13)K-fold Cross-Validation - K折交叉验证14)Stratified Cross-Validation - 分层交叉验证15)Leave-One-Out Cross-Validation (LOOCV) - 留一法交叉验证16)Grid Search - 网格搜索17)Random Search - 随机搜索18)Model Complexity - 模型复杂度19)Learning Curve - 学习曲线20)Convergence - 收敛3.Machine Learning Techniques and Algorithms (机器学习技术与算法)•Decision Tree - 决策树1)Decision Tree - 决策树2)Node - 节点3)Root Node - 根节点4)Leaf Node - 叶节点5)Internal Node - 内部节点6)Splitting Criterion - 分裂准则7)Gini Impurity - 基尼不纯度8)Entropy - 熵9)Information Gain - 信息增益10)Gain Ratio - 增益率11)Pruning - 剪枝12)Recursive Partitioning - 递归分割13)CART (Classification and Regression Trees) - 分类回归树14)ID3 (Iterative Dichotomiser 3) - 迭代二叉树315)C4.5 (successor of ID3) - C4.5(ID3的后继者)16)C5.0 (successor of C4.5) - C5.0(C4.5的后继者)17)Split Point - 分裂点18)Decision Boundary - 决策边界19)Pruned Tree - 剪枝后的树20)Decision Tree Ensemble - 决策树集成•Random Forest - 随机森林1)Random Forest - 随机森林2)Ensemble Learning - 集成学习3)Bootstrap Sampling - 自助采样4)Bagging (Bootstrap Aggregating) - 装袋法5)Out-of-Bag (OOB) Error - 袋外误差6)Feature Subset - 特征子集7)Decision Tree - 决策树8)Base Estimator - 基础估计器9)Tree Depth - 树深度10)Randomization - 随机化11)Majority Voting - 多数投票12)Feature Importance - 特征重要性13)OOB Score - 袋外得分14)Forest Size - 森林大小15)Max Features - 最大特征数16)Min Samples Split - 最小分裂样本数17)Min Samples Leaf - 最小叶节点样本数18)Gini Impurity - 基尼不纯度19)Entropy - 熵20)Variable Importance - 变量重要性•Support Vector Machine (SVM) - 支持向量机1)Support Vector Machine (SVM) - 支持向量机2)Hyperplane - 超平面3)Kernel Trick - 核技巧4)Kernel Function - 核函数5)Margin - 间隔6)Support Vectors - 支持向量7)Decision Boundary - 决策边界8)Maximum Margin Classifier - 最大间隔分类器9)Soft Margin Classifier - 软间隔分类器10) C Parameter - C参数11)Radial Basis Function (RBF) Kernel - 径向基函数核12)Polynomial Kernel - 多项式核13)Linear Kernel - 线性核14)Quadratic Kernel - 二次核15)Gaussian Kernel - 高斯核16)Regularization - 正则化17)Dual Problem - 对偶问题18)Primal Problem - 原始问题19)Kernelized SVM - 核化支持向量机20)Multiclass SVM - 多类支持向量机•K-Nearest Neighbors (KNN) - K-最近邻1)K-Nearest Neighbors (KNN) - K-最近邻2)Nearest Neighbor - 最近邻3)Distance Metric - 距离度量4)Euclidean Distance - 欧氏距离5)Manhattan Distance - 曼哈顿距离6)Minkowski Distance - 闵可夫斯基距离7)Cosine Similarity - 余弦相似度8)K Value - K值9)Majority Voting - 多数投票10)Weighted KNN - 加权KNN11)Radius Neighbors - 半径邻居12)Ball Tree - 球树13)KD Tree - KD树14)Locality-Sensitive Hashing (LSH) - 局部敏感哈希15)Curse of Dimensionality - 维度灾难16)Class Label - 类标签17)Training Set - 训练集18)Test Set - 测试集19)Validation Set - 验证集20)Cross-Validation - 交叉验证•Naive Bayes - 朴素贝叶斯1)Naive Bayes - 朴素贝叶斯2)Bayes' Theorem - 贝叶斯定理3)Prior Probability - 先验概率4)Posterior Probability - 后验概率5)Likelihood - 似然6)Class Conditional Probability - 类条件概率7)Feature Independence Assumption - 特征独立假设8)Multinomial Naive Bayes - 多项式朴素贝叶斯9)Gaussian Naive Bayes - 高斯朴素贝叶斯10)Bernoulli Naive Bayes - 伯努利朴素贝叶斯11)Laplace Smoothing - 拉普拉斯平滑12)Add-One Smoothing - 加一平滑13)Maximum A Posteriori (MAP) - 最大后验概率14)Maximum Likelihood Estimation (MLE) - 最大似然估计15)Classification - 分类16)Feature Vectors - 特征向量17)Training Set - 训练集18)Test Set - 测试集19)Class Label - 类标签20)Confusion Matrix - 混淆矩阵•Clustering - 聚类1)Clustering - 聚类2)Centroid - 质心3)Cluster Analysis - 聚类分析4)Partitioning Clustering - 划分式聚类5)Hierarchical Clustering - 层次聚类6)Density-Based Clustering - 基于密度的聚类7)K-Means Clustering - K均值聚类8)K-Medoids Clustering - K中心点聚类9)DBSCAN (Density-Based Spatial Clustering of Applications with Noise) - 基于密度的空间聚类算法10)Agglomerative Clustering - 聚合式聚类11)Dendrogram - 系统树图12)Silhouette Score - 轮廓系数13)Elbow Method - 肘部法则14)Clustering Validation - 聚类验证15)Intra-cluster Distance - 类内距离16)Inter-cluster Distance - 类间距离17)Cluster Cohesion - 类内连贯性18)Cluster Separation - 类间分离度19)Cluster Assignment - 聚类分配20)Cluster Label - 聚类标签•K-Means - K-均值1)K-Means - K-均值2)Centroid - 质心3)Cluster - 聚类4)Cluster Center - 聚类中心5)Cluster Assignment - 聚类分配6)Cluster Analysis - 聚类分析7)K Value - K值8)Elbow Method - 肘部法则9)Inertia - 惯性10)Silhouette Score - 轮廓系数11)Convergence - 收敛12)Initialization - 初始化13)Euclidean Distance - 欧氏距离14)Manhattan Distance - 曼哈顿距离15)Distance Metric - 距离度量16)Cluster Radius - 聚类半径17)Within-Cluster Variation - 类内变异18)Cluster Quality - 聚类质量19)Clustering Algorithm - 聚类算法20)Clustering Validation - 聚类验证•Dimensionality Reduction - 降维1)Dimensionality Reduction - 降维2)Feature Extraction - 特征提取3)Feature Selection - 特征选择4)Principal Component Analysis (PCA) - 主成分分析5)Singular Value Decomposition (SVD) - 奇异值分解6)Linear Discriminant Analysis (LDA) - 线性判别分析7)t-Distributed Stochastic Neighbor Embedding (t-SNE) - t-分布随机邻域嵌入8)Autoencoder - 自编码器9)Manifold Learning - 流形学习10)Locally Linear Embedding (LLE) - 局部线性嵌入11)Isomap - 等度量映射12)Uniform Manifold Approximation and Projection (UMAP) - 均匀流形逼近与投影13)Kernel PCA - 核主成分分析14)Non-negative Matrix Factorization (NMF) - 非负矩阵分解15)Independent Component Analysis (ICA) - 独立成分分析16)Variational Autoencoder (VAE) - 变分自编码器17)Sparse Coding - 稀疏编码18)Random Projection - 随机投影19)Neighborhood Preserving Embedding (NPE) - 保持邻域结构的嵌入20)Curvilinear Component Analysis (CCA) - 曲线成分分析•Principal Component Analysis (PCA) - 主成分分析1)Principal Component Analysis (PCA) - 主成分分析2)Eigenvector - 特征向量3)Eigenvalue - 特征值4)Covariance Matrix - 协方差矩阵。
计算机专业词汇英语翻译
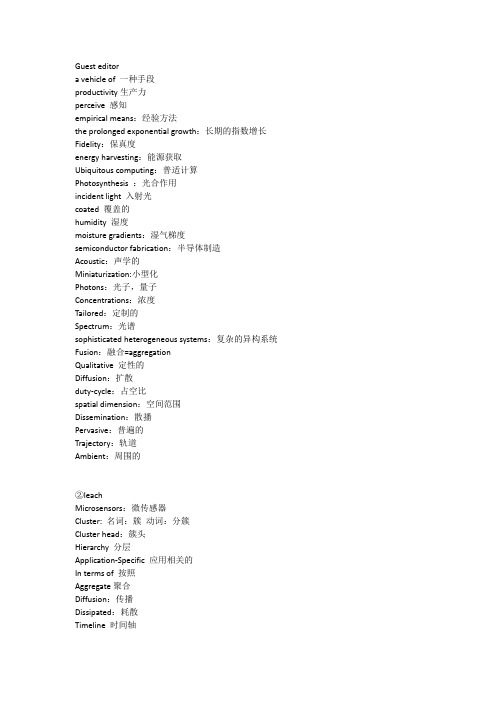
Guest editora vehicle of 一种手段productivity生产力perceive 感知empirical means:经验方法the prolonged exponential growth:长期的指数增长Fidelity:保真度energy harvesting:能源获取Ubiquitous computing:普适计算Photosynthesis :光合作用incident light 入射光coated 覆盖的humidity 湿度moisture gradients:湿气梯度semiconductor fabrication:半导体制造Acoustic:声学的Miniaturization:小型化Photons:光子,量子Concentrations:浓度Tailored:定制的Spectrum:光谱sophisticated heterogeneous systems:复杂的异构系统Fusion:融合=aggregationQualitative 定性的Diffusion:扩散duty-cycle:占空比spatial dimension:空间范围Dissemination:散播Pervasive:普遍的Trajectory:轨道Ambient:周围的②leachMicrosensors:微传感器Cluster: 名词:簇动词:分簇Cluster head:簇头Hierarchy 分层Application-Specific 应用相关的In terms of 按照Aggregate聚合Diffusion:传播Dissipated:耗散Timeline 时间轴Backs off:后退Dissipation:耗散spread-spectrum:扩频intra-cluster:簇内Outperform:胜过③pegasisHomogeneous:同质的fusion :融合aggregationFuse:v. 融合Humidity:湿度Beacon:信标timestamp 时间戳in terms of :就...而言greedy approach:贪婪算法truncated chain:截断链Critical:关键的propagation delays:传播延迟Dissipate:v.发散SNR:信噪比Joules:焦耳The upper bound:上限tier:等级token :令牌,象征Dense:密集的Sparse:稀疏的Heuristic:启发式Outperforms:胜过Preliminary:初步的Exponential:指数的traveling salesman problem 旅行商问题tradeoff 代价④z-macLatency:时间延迟Robust:鲁棒性slot assignment:时隙分配multiple access control:多址接入控制Aggregate:聚合duty cycle:占空比the overhead of:开销Vendors:厂商surface-mount:表面贴装hand-soldering:手工焊接Predetermined:预定的Stochastic:随机的Explicit Contention Notification:明确竞争通知Unicast:单播Congestion:拥塞Benchmark:基准Preamble:头部⑤A building。
win7服务详解及设置参考
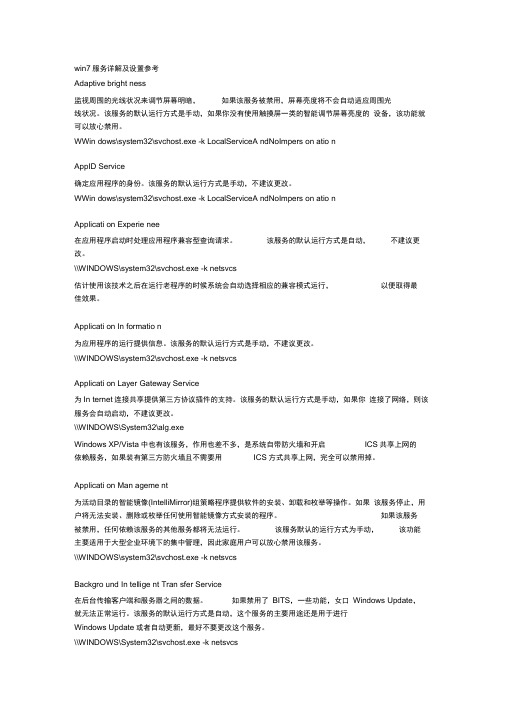
win7服务详解及设置参考Adaptive bright ness监视周围的光线状况来调节屏幕明暗,如果该服务被禁用,屏幕亮度将不会自动适应周围光线状况。
该服务的默认运行方式是手动,如果你没有使用触摸屏一类的智能调节屏幕亮度的设备,该功能就可以放心禁用。
WWin dows\system32\svchost.exe -k LocalServiceA ndNoImpers on atio nAppID Service确定应用程序的身份。
该服务的默认运行方式是手动,不建议更改。
WWin dows\system32\svchost.exe -k LocalServiceA ndNoImpers on atio nApplicati on Experie nee在应用程序启动时处理应用程序兼容型查询请求。
该服务的默认运行方式是自动,不建议更改。
\\WINDOWS\system32\svchost.exe -k netsvcs估计使用该技术之后在运行老程序的时候系统会自动选择相应的兼容模式运行,以便取得最佳效果。
Applicati on In formatio n为应用程序的运行提供信息。
该服务的默认运行方式是手动,不建议更改。
\\WINDOWS\system32\svchost.exe -k netsvcsApplicati on Layer Gateway Service为In ternet连接共享提供第三方协议插件的支持。
该服务的默认运行方式是手动,如果你连接了网络,则该服务会自动启动,不建议更改。
\\WINDOWS\System32\alg.exeWindows XP/Vista中也有该服务,作用也差不多,是系统自带防火墙和开启ICS共享上网的依赖服务,如果装有第三方防火墙且不需要用ICS方式共享上网,完全可以禁用掉。
Applicati on Man ageme nt为活动目录的智能镜像(IntelliMirror)组策略程序提供软件的安装、卸载和枚举等操作。
课程名称中英文对照参考表
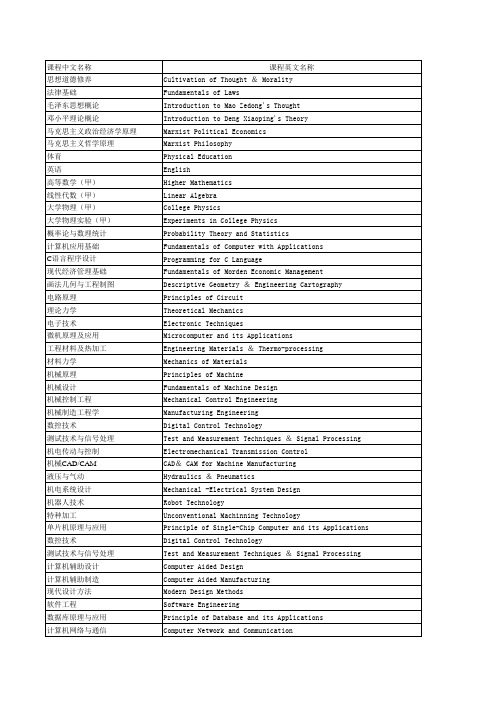
外国文学作选读Selected Reading of Foreign Literature现代企业管理概论Introduction to Modern Enterprise Managerment电力电子技术课设计Power Electronics Technology Design计算机动画设计3D Animation Design中国革命史China’s Revolutionary History中国社会主义建设China Socialist Construction集散控制DCS Distributed Control计算机控制实现技术Computer Control Realization Technology计算机网络与通讯Computer Network and CommunicationERP/WEB应用开发Application & Development of ERP/WEB数据仓库与挖掘Data Warehouse and Data Mining物流及供应链管理Substance and Supply Chain Management成功心理与潜能开发Success Psychology & Potential Development信息安全技术Technology of Information Security图像通信Image Communication金属材料及热加工Engineering Materials & Thermo-processing机械原理课程设计Course Design for Principles of Machine机械设计课程设计Course Design for Mechanical Design机电系统课程设计Course Design for Mechanical and Electrical System 创新成果Creative Achievements课外教育Extracurricular education。
人工智能专用名词
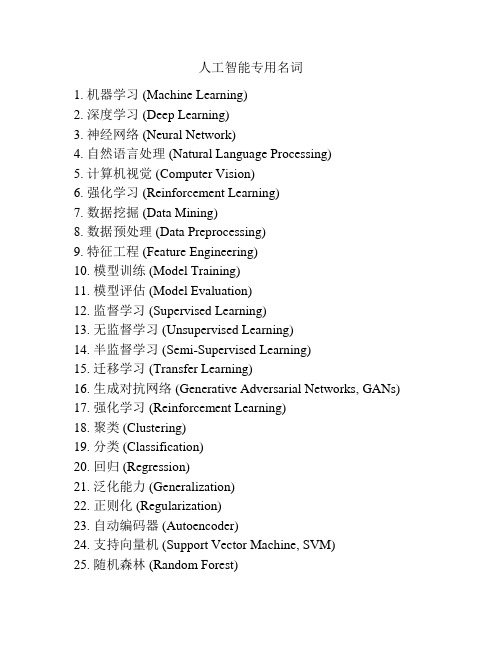
人工智能专用名词1. 机器学习 (Machine Learning)2. 深度学习 (Deep Learning)3. 神经网络 (Neural Network)4. 自然语言处理 (Natural Language Processing)5. 计算机视觉 (Computer Vision)6. 强化学习 (Reinforcement Learning)7. 数据挖掘 (Data Mining)8. 数据预处理 (Data Preprocessing)9. 特征工程 (Feature Engineering)10. 模型训练 (Model Training)11. 模型评估 (Model Evaluation)12. 监督学习 (Supervised Learning)13. 无监督学习 (Unsupervised Learning)14. 半监督学习 (Semi-Supervised Learning)15. 迁移学习 (Transfer Learning)16. 生成对抗网络 (Generative Adversarial Networks, GANs)17. 强化学习 (Reinforcement Learning)18. 聚类 (Clustering)19. 分类 (Classification)20. 回归 (Regression)21. 泛化能力 (Generalization)22. 正则化 (Regularization)23. 自动编码器 (Autoencoder)24. 支持向量机 (Support Vector Machine, SVM)25. 随机森林 (Random Forest)26. 梯度下降 (Gradient Descent)27. 前向传播 (Forward Propagation)28. 反向传播 (Backpropagation)29. 混淆矩阵 (Confusion Matrix)30. ROC曲线 (Receiver Operating Characteristic Curve, ROC Curve)31. AUC指标 (Area Under Curve, AUC)32. 噪声 (Noise)33. 过拟合 (Overfitting)34. 欠拟合 (Underfitting)35. 超参数 (Hyperparameters)36. 网格搜索 (Grid Search)37. 交叉验证 (Cross Validation)38. 降维 (Dimensionality Reduction)39. 卷积神经网络 (Convolutional Neural Network, CNN)40. 循环神经网络 (Recurrent Neural Network, RNN)。
组合学习和归属学习
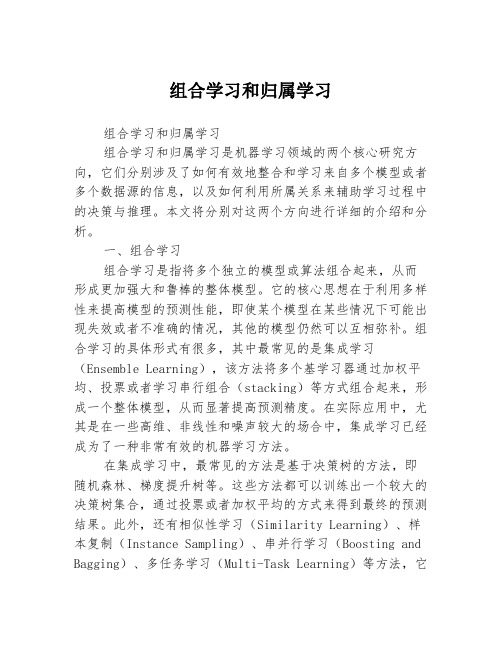
组合学习和归属学习组合学习和归属学习组合学习和归属学习是机器学习领域的两个核心研究方向,它们分别涉及了如何有效地整合和学习来自多个模型或者多个数据源的信息,以及如何利用所属关系来辅助学习过程中的决策与推理。
本文将分别对这两个方向进行详细的介绍和分析。
一、组合学习组合学习是指将多个独立的模型或算法组合起来,从而形成更加强大和鲁棒的整体模型。
它的核心思想在于利用多样性来提高模型的预测性能,即使某个模型在某些情况下可能出现失效或者不准确的情况,其他的模型仍然可以互相弥补。
组合学习的具体形式有很多,其中最常见的是集成学习(Ensemble Learning),该方法将多个基学习器通过加权平均、投票或者学习串行组合(stacking)等方式组合起来,形成一个整体模型,从而显著提高预测精度。
在实际应用中,尤其是在一些高维、非线性和噪声较大的场合中,集成学习已经成为了一种非常有效的机器学习方法。
在集成学习中,最常见的方法是基于决策树的方法,即随机森林、梯度提升树等。
这些方法都可以训练出一个较大的决策树集合,通过投票或者加权平均的方式来得到最终的预测结果。
此外,还有相似性学习(Similarity Learning)、样本复制(Instance Sampling)、串并行学习(Boosting and Bagging)、多任务学习(Multi-Task Learning)等方法,它们都是组合学习的经典方法。
在实际应用中,组合学习的优势主要表现在以下几个方面:1. 可以融合多个异构模型,克服了单一模型存在的欠拟合或过拟合问题。
2. 可以有效利用不同模型之间的互补性,提高了预测性能的可靠性和鲁棒性。
3. 可以通过分布式计算来加快模型训练和推理的速度和效率。
4. 可以应对大规模、高维度、复杂和多模态数据的挑战。
但是,组合学习也存在一些问题,例如模型间的差异性可能导致预测结果的偏差或过拟合,在处理小规模或无噪声数据时可能会降低模型的灵敏度和精度,另外也需要考虑模型的可解释性和模型的鲁棒性等问题。
一种向量划分的网络社团发现方法
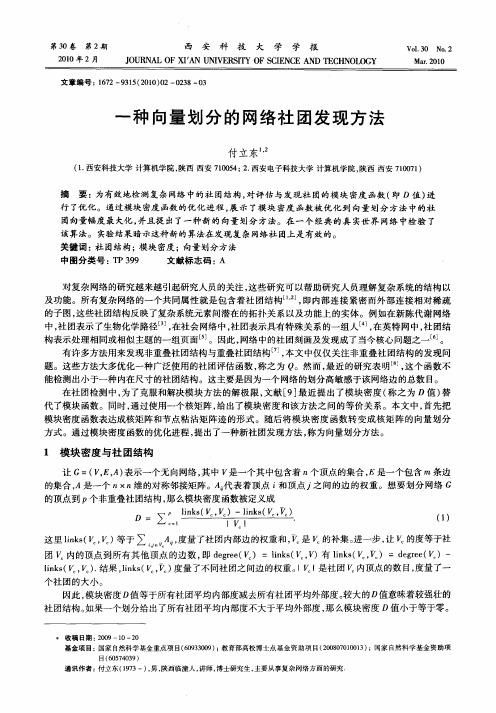
1 模 块 密 度 与社 团结 构
让 G=( E, 表 示一个 无 向网络 , 中 是一个 其 中包 含着 n个顶 点的集 合 , 一个包 含 m条边 , ) 其 E是
的集合 , 一个 /x凡维 的对称邻 接矩 阵 。A 代 表着 顶 点 i 顶点 之 间 的边 的 权重 。想 要划 分 网络 G 是 z 和 的顶 点到 P个 非重叠 社 团结 构 , 么模 块密度 函数被 定义成 那
基金项 目:国家 自然科学基金重点项 目( 03 09) 教育部高校博士点基金 资助项 目(0 8 7 10 3 ;国家 自然科学基金资助项 69 3 0 ; 2 00 0 0 1 )
目(0 70 9 6 54 3 )
通讯作者 : 付立东 (93一)男 , 17 , 陕西临潼人 , , 讲师 博士研究生 , 主要从事复杂网络方面的研究.
个 社 团的大 小 。
因此 , 块 密度 D值等 于所有 社 团平均 内部 度减 去所有 社 团平 均外部 度 。 大 的 D值 意味着 较强壮 的 模 较
社 团结构。 如果一个划分给出了所有社 团平均内部度不大于平均外部度, 那么模块密度பைடு நூலகம்D值小于等于零。
收 稿 日期 : 0 9—1 20 0—2 0
l k ( ,c i s I )一l k ( , c n , i s I) n , ,、 1
———
r—~
’
这里lk( , 等于 ∑ , 度量了社团内部边的权重和, 是 的补集。 i s ) n 进一步, l的度等于 让/ c 社
团 内的顶点 到 所有 其 他顶 点 的边数 , dge( ) = l k (,, )有 l k( , ) =d ge( )一 即 ere i s' V n c i s n ere l k( , ) 结果 ,n s , ) i s . n l k ( 度量 了不 同社 团之 间边 的权重 。 I i f 是社 团 内顶点 的数 目, 度量 了一
异质性数据的联邦学习关键技术研究
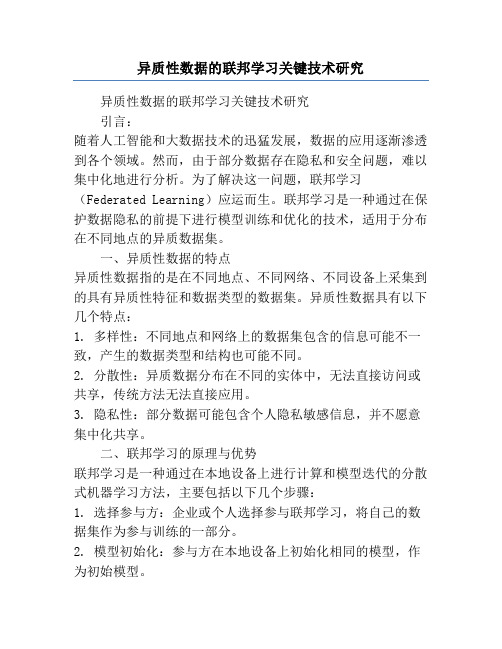
异质性数据的联邦学习关键技术研究异质性数据的联邦学习关键技术研究引言:随着人工智能和大数据技术的迅猛发展,数据的应用逐渐渗透到各个领域。
然而,由于部分数据存在隐私和安全问题,难以集中化地进行分析。
为了解决这一问题,联邦学习(Federated Learning)应运而生。
联邦学习是一种通过在保护数据隐私的前提下进行模型训练和优化的技术,适用于分布在不同地点的异质数据集。
一、异质性数据的特点异质性数据指的是在不同地点、不同网络、不同设备上采集到的具有异质性特征和数据类型的数据集。
异质性数据具有以下几个特点:1. 多样性:不同地点和网络上的数据集包含的信息可能不一致,产生的数据类型和结构也可能不同。
2. 分散性:异质数据分布在不同的实体中,无法直接访问或共享,传统方法无法直接应用。
3. 隐私性:部分数据可能包含个人隐私敏感信息,并不愿意集中化共享。
二、联邦学习的原理与优势联邦学习是一种通过在本地设备上进行计算和模型迭代的分散式机器学习方法,主要包括以下几个步骤:1. 选择参与方:企业或个人选择参与联邦学习,将自己的数据集作为参与训练的一部分。
2. 模型初始化:参与方在本地设备上初始化相同的模型,作为初始模型。
3. 本地训练和优化:各个参与方使用自己的数据进行模型训练和优化,得到更新的模型参数。
4. 参数聚合:参与方将自己的模型参数上传到中央服务器,通过聚合算法将各方的模型参数进行整合。
5. 更新模型:中央服务器将聚合后的模型参数下发给各个参与方,参与方更新本地模型参数。
6. 重复迭代:重复进行本地训练、参数聚合和更新模型的过程,直到模型性能达到预期。
联邦学习的优势有:1. 保护数据隐私:联邦学习通过在本地设备上进行计算和优化,不需要直接共享数据,保护了数据隐私。
2. 分布式计算:联邦学习利用参与方本地设备的计算资源,分布式进行计算和优化,加快了模型训练的速度。
3. 解决数据分散性问题:由于数据分布在不同实体中,联邦学习通过模型参数聚合的方式解决了数据分散性问题,提高了数据的利用率。
集成学习的不二法门bagging、boosting和三大法宝结合策略平均法,投票法和学习法。。。

集成学习的不⼆法门bagging、boosting和三⼤法宝结合策略平均法,投票法和学习法。
单个学习器要么容易⽋拟合要么容易过拟合,为了获得泛化性能优良的学习器,可以训练多个个体学习器,通过⼀定的结合策略,最终形成⼀个强学习器。
这种集成多个个体学习器的⽅法称为集成学习(ensemble learning)。
集成学习通过组合多种模型来改善机器学习的结果,与单⼀的模型相⽐,这种⽅法允许产⽣更好的预测性能。
集成学习属于元算法,即结合数个“好⽽不同”的机器学习技术,形成⼀个预测模型,以此来降⽅差(bagging),减偏差(boosting),提升预测准确性(stacking)。
1. 集成学习之个体学习器个体学习器(⼜称为“基学习器”)的选择有两种⽅式:集成中只包含同种类型的个体学习器,称为同质集成。
集成中包含不同类型的个体学习器,为异质集成。
⽬前同质集成的应⽤最⼴泛,⽽基学习器使⽤最多的模型是CART决策树和神经⽹络。
按照个体学习器之间是否存在依赖关系可以分为两类:个体学习器之间存在强依赖关系,⼀系列个体学习器基本必须串⾏⽣成,代表是boosting系列算法。
个体学习器之间不存在强依赖关系,⼀系列个体学习器可以并⾏⽣成,代表是bagging系列算法。
1.1 boosting算法原理boosting的算法原理如下所⽰:Boosting算法的⼯作机制是:(1)先从初始训练集训练出⼀个基学习器;(2)再根据基学习器的表现对样本权重进⾏调整,增加基学习器误分类样本的权重(⼜称重采样);(3)基于调整后的样本分布来训练下⼀个基学习器;(4)如此重复进⾏,直⾄基学习器数⽬达到事先指定的个数,将这个基学习器通过集合策略进⾏整合,得到最终的强学习器。
Boosting系列算法⾥最著名算法主要有AdaBoost算法和提升树(boosting tree)系列算法。
提升树系列算法⾥⾯应⽤最⼴泛的是梯度提升树(Gradient Boosting Tree)<GDBT>。
分布式认知
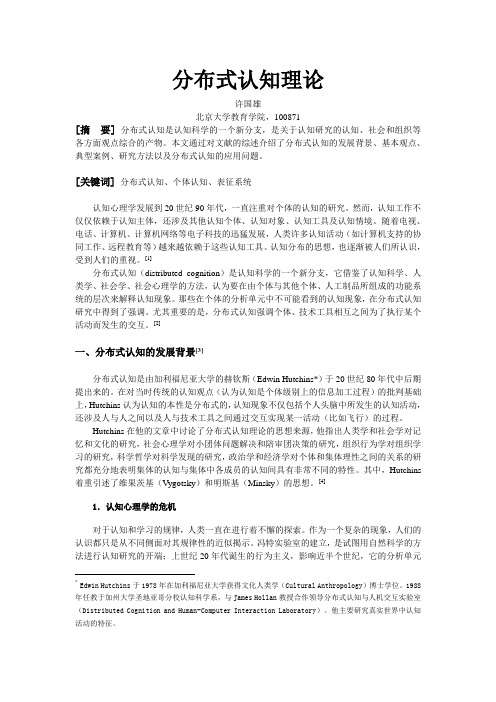
分布式认知理论许国雄北京大学教育学院,100871[摘要] 分布式认知是认知科学的一个新分支,是关于认知研究的认知、社会和组织等各方面观点综合的产物。
本文通过对文献的综述介绍了分布式认知的发展背景、基本观点、典型案例、研究方法以及分布式认知的应用问题。
[关键词] 分布式认知、个体认知、表征系统认知心理学发展到20世纪90年代,一直注重对个体的认知的研究。
然而,认知工作不仅仅依赖于认知主体,还涉及其他认知个体、认知对象、认知工具及认知情境。
随着电视、电话、计算机、计算机网络等电子科技的迅猛发展,人类许多认知活动(如计算机支持的协同工作、远程教育等)越来越依赖于这些认知工具。
认知分布的思想,也逐渐被人们所认识,受到人们的重视。
[1]分布式认知(distributed cognition)是认知科学的一个新分支,它借鉴了认知科学、人类学、社会学、社会心理学的方法,认为要在由个体与其他个体、人工制品所组成的功能系统的层次来解释认知现象。
那些在个体的分析单元中不可能看到的认知现象,在分布式认知研究中得到了强调。
尤其重要的是,分布式认知强调个体、技术工具相互之间为了执行某个活动而发生的交互。
[2]一、分布式认知的发展背景[3]分布式认知是由加利福尼亚大学的赫钦斯(Edwin Hutchins*)于20世纪80年代中后期提出来的。
在对当时传统的认知观点(认为认知是个体级别上的信息加工过程)的批判基础上,Hutchins认为认知的本性是分布式的,认知现象不仅包括个人头脑中所发生的认知活动,还涉及人与人之间以及人与技术工具之间通过交互实现某一活动(比如飞行)的过程。
Hutchins在他的文章中讨论了分布式认知理论的思想来源,他指出人类学和社会学对记忆和文化的研究,社会心理学对小团体问题解决和陪审团决策的研究,组织行为学对组织学习的研究,科学哲学对科学发现的研究,政治学和经济学对个体和集体理性之间的关系的研究都充分地表明集体的认知与集体中各成员的认知间具有非常不同的特性。
为家乡作贡献的英语作文
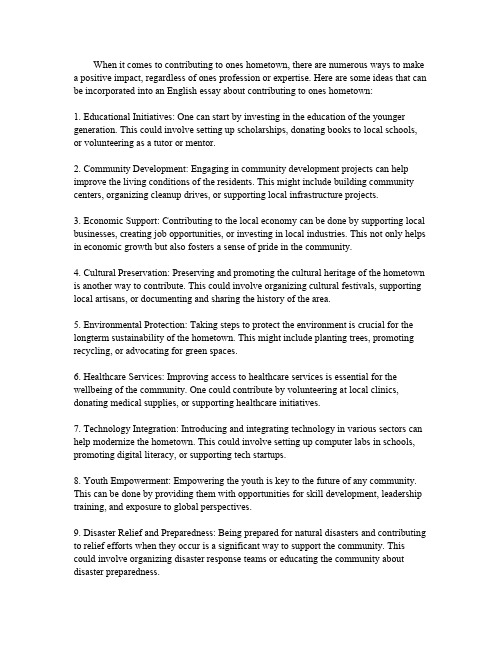
When it comes to contributing to ones hometown,there are numerous ways to make a positive impact,regardless of ones profession or expertise.Here are some ideas that can be incorporated into an English essay about contributing to ones hometown:cational Initiatives:One can start by investing in the education of the younger generation.This could involve setting up scholarships,donating books to local schools, or volunteering as a tutor or mentor.munity Development:Engaging in community development projects can help improve the living conditions of the residents.This might include building community centers,organizing cleanup drives,or supporting local infrastructure projects.3.Economic Support:Contributing to the local economy can be done by supporting local businesses,creating job opportunities,or investing in local industries.This not only helps in economic growth but also fosters a sense of pride in the community.4.Cultural Preservation:Preserving and promoting the cultural heritage of the hometown is another way to contribute.This could involve organizing cultural festivals,supporting local artisans,or documenting and sharing the history of the area.5.Environmental Protection:Taking steps to protect the environment is crucial for the longterm sustainability of the hometown.This might include planting trees,promoting recycling,or advocating for green spaces.6.Healthcare Services:Improving access to healthcare services is essential for the wellbeing of the community.One could contribute by volunteering at local clinics, donating medical supplies,or supporting healthcare initiatives.7.Technology Integration:Introducing and integrating technology in various sectors can help modernize the hometown.This could involve setting up computer labs in schools, promoting digital literacy,or supporting tech startups.8.Youth Empowerment:Empowering the youth is key to the future of any community. This can be done by providing them with opportunities for skill development,leadership training,and exposure to global perspectives.9.Disaster Relief and Preparedness:Being prepared for natural disasters and contributing to relief efforts when they occur is a significant way to support the community.This could involve organizing disaster response teams or educating the community about disaster preparedness.10.Advocacy and Policy Making:Participating in local governance and advocating for policies that benefit the community can lead to systemic changes.This might involve running for local office,joining community boards,or lobbying for beneficial legislation. In conclusion,contributing to ones hometown is a multifaceted endeavor that requires a combination of passion,resources,and a commitment to the communitys wellbeing.By engaging in these activities,individuals can play a vital role in shaping the future of their hometown and ensuring its prosperity for generations to come.。
基础研究 英文
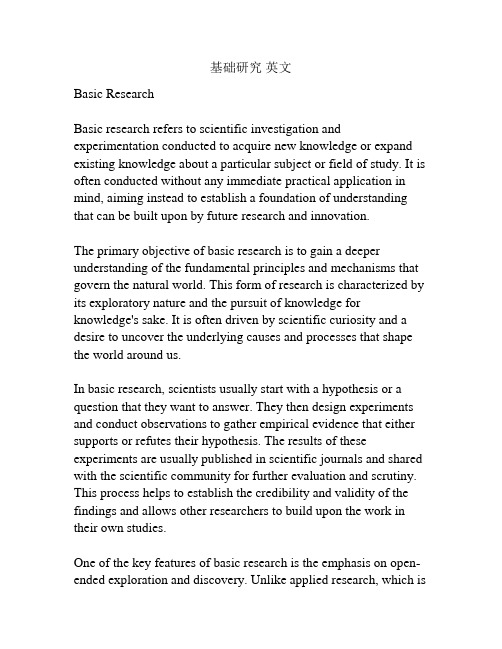
基础研究英文Basic ResearchBasic research refers to scientific investigation and experimentation conducted to acquire new knowledge or expand existing knowledge about a particular subject or field of study. It is often conducted without any immediate practical application in mind, aiming instead to establish a foundation of understanding that can be built upon by future research and innovation.The primary objective of basic research is to gain a deeper understanding of the fundamental principles and mechanisms that govern the natural world. This form of research is characterized by its exploratory nature and the pursuit of knowledge for knowledge's sake. It is often driven by scientific curiosity and a desire to uncover the underlying causes and processes that shape the world around us.In basic research, scientists usually start with a hypothesis or a question that they want to answer. They then design experiments and conduct observations to gather empirical evidence that either supports or refutes their hypothesis. The results of these experiments are usually published in scientific journals and shared with the scientific community for further evaluation and scrutiny. This process helps to establish the credibility and validity of the findings and allows other researchers to build upon the work in their own studies.One of the key features of basic research is the emphasis on open-ended exploration and discovery. Unlike applied research, which isfocused on solving specific problems or developing practical applications, basic research aims to increase our understanding of the underlying principles and mechanisms that govern various phenomena. This knowledge can then be used as a foundational framework for applied research and innovation in various fields such as medicine, engineering, and technology.Basic research has made significant contributions to scientific progress and has led to numerous breakthroughs and discoveries. For example, the discovery of penicillin, one of the most important antibiotics, was a result of basic research conducted by Alexander Fleming. Similarly, the development of the internet and many other technological advancements can be traced back to basic research into computing and communication.In addition to its contribution to knowledge and innovation, basic research also plays a crucial role in education and training. It provides opportunities for aspiring scientists and researchers to learn and practice scientific methods, develop critical thinking skills, and gain hands-on experience conducting experiments and analyzing data. Moreover, the dissemination of basic research findings through academic journals and conferences helps to foster intellectual growth and collaboration within the scientific community.In conclusion, basic research serves as the foundation for scientific progress and innovation. It aims to expand our understanding of the natural world and uncover the underlying principles and mechanisms that govern various phenomena. While it may nothave immediate practical applications, its contributions to knowledge, education, and technology are invaluable.。
大学俱乐部的趋势英语作文
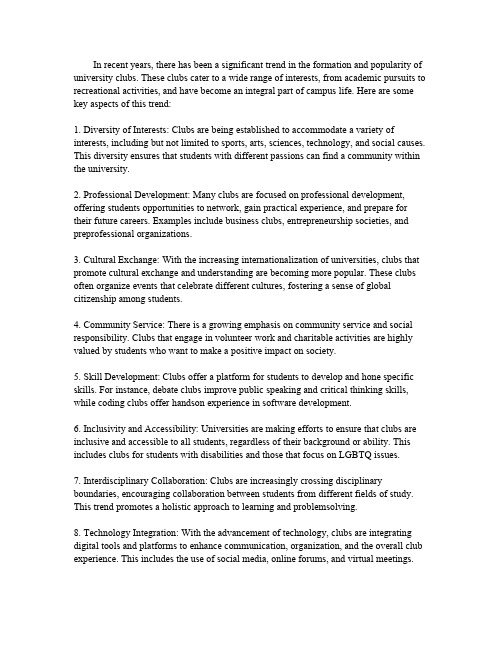
In recent years,there has been a significant trend in the formation and popularity of university clubs.These clubs cater to a wide range of interests,from academic pursuits to recreational activities,and have become an integral part of campus life.Here are some key aspects of this trend:1.Diversity of Interests:Clubs are being established to accommodate a variety of interests,including but not limited to sports,arts,sciences,technology,and social causes. This diversity ensures that students with different passions can find a community within the university.2.Professional Development:Many clubs are focused on professional development, offering students opportunities to network,gain practical experience,and prepare for their future careers.Examples include business clubs,entrepreneurship societies,and preprofessional organizations.3.Cultural Exchange:With the increasing internationalization of universities,clubs that promote cultural exchange and understanding are becoming more popular.These clubs often organize events that celebrate different cultures,fostering a sense of global citizenship among students.munity Service:There is a growing emphasis on community service and social responsibility.Clubs that engage in volunteer work and charitable activities are highly valued by students who want to make a positive impact on society.5.Skill Development:Clubs offer a platform for students to develop and hone specific skills.For instance,debate clubs improve public speaking and critical thinking skills, while coding clubs offer handson experience in software development.6.Inclusivity and Accessibility:Universities are making efforts to ensure that clubs are inclusive and accessible to all students,regardless of their background or ability.This includes clubs for students with disabilities and those that focus on LGBTQ issues.7.Interdisciplinary Collaboration:Clubs are increasingly crossing disciplinary boundaries,encouraging collaboration between students from different fields of study. This trend promotes a holistic approach to learning and problemsolving.8.Technology Integration:With the advancement of technology,clubs are integrating digital tools and platforms to enhance communication,organization,and the overall club experience.This includes the use of social media,online forums,and virtual meetings.9.Sustainability Focus:There is a rising trend of clubs focusing on environmental sustainability,promoting ecofriendly practices and raising awareness about climate change and conservation efforts.10.Mental Health Awareness:Recognizing the importance of mental health,some clubs are dedicated to providing support and resources for students dealing with stress,anxiety, and other mental health issues.11.Extracurricular Opportunities:Clubs offer a way for students to balance their academic life with extracurricular activities,which can be crucial for personal development and overall wellbeing.12.CareerRelated Clubs:Many students join clubs that are directly related to their field of study or career aspirations,providing them with a deeper understanding and connection to their chosen industry.These trends reflect the evolving needs and interests of university students,as well as the broader societal shifts towards inclusivity,technology integration,and a focus on personal and professional development.As universities continue to adapt to these trends, the role of clubs in fostering a vibrant and supportive campus community becomes ever more significant.。
prompt distribution learning解读
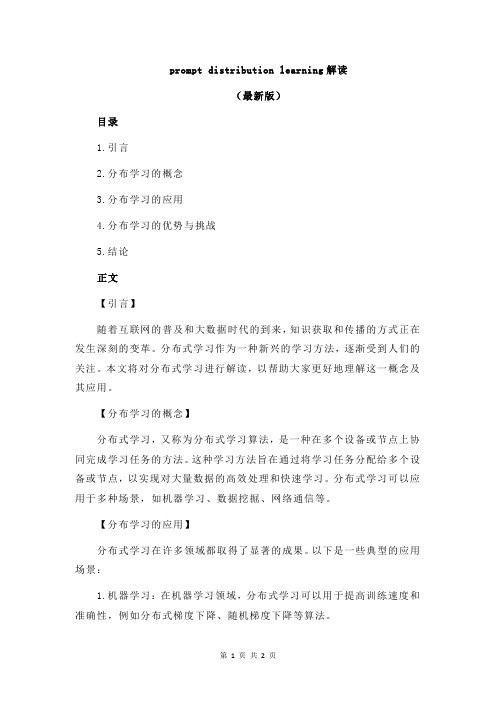
prompt distribution learning解读(最新版)目录1.引言2.分布学习的概念3.分布学习的应用4.分布学习的优势与挑战5.结论正文【引言】随着互联网的普及和大数据时代的到来,知识获取和传播的方式正在发生深刻的变革。
分布式学习作为一种新兴的学习方法,逐渐受到人们的关注。
本文将对分布式学习进行解读,以帮助大家更好地理解这一概念及其应用。
【分布学习的概念】分布式学习,又称为分布式学习算法,是一种在多个设备或节点上协同完成学习任务的方法。
这种学习方法旨在通过将学习任务分配给多个设备或节点,以实现对大量数据的高效处理和快速学习。
分布式学习可以应用于多种场景,如机器学习、数据挖掘、网络通信等。
【分布学习的应用】分布式学习在许多领域都取得了显著的成果。
以下是一些典型的应用场景:1.机器学习:在机器学习领域,分布式学习可以用于提高训练速度和准确性,例如分布式梯度下降、随机梯度下降等算法。
2.数据挖掘:在数据挖掘领域,分布式学习可以用于对海量数据进行高效挖掘,例如分布式 K-means 聚类算法。
3.网络通信:在网络通信领域,分布式学习可以用于实现负载均衡、路由优化等任务,例如分布式路由选择协议。
【分布学习的优势与挑战】分布式学习具有以下优势:1.提高学习速度:通过将学习任务分配给多个设备或节点,可以显著减少单个设备的计算负担,从而提高学习速度。
2.扩展学习能力:分布式学习可以充分利用网络中的多个设备或节点的计算能力,从而提高整体的学习能力。
3.增强鲁棒性:分布式学习可以通过多个设备或节点的协同工作,提高系统的鲁棒性,降低单点故障的风险。
然而,分布式学习也面临一些挑战,如:1.通信复杂性:在分布式学习中,设备或节点之间需要进行大量的通信,可能导致网络拥塞和通信延迟。
2.协同困难:由于设备或节点之间可能存在异构性,协同学习时可能面临算法兼容性、数据同步等问题。
3.安全性问题:分布式学习涉及多个设备或节点,可能面临数据泄露、隐私保护等安全性问题。
利用压缩多语言BERT知识增强的低资源神经机器翻译
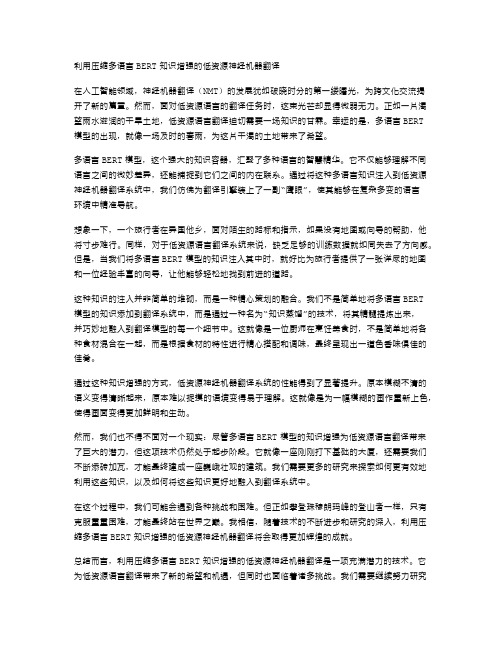
利用压缩多语言BERT知识增强的低资源神经机器翻译在人工智能领域,神经机器翻译(NMT)的发展犹如破晓时分的第一缕曙光,为跨文化交流揭开了新的篇章。
然而,面对低资源语言的翻译任务时,这束光芒却显得微弱无力。
正如一片渴望雨水滋润的干旱土地,低资源语言翻译迫切需要一场知识的甘霖。
幸运的是,多语言BERT模型的出现,就像一场及时的春雨,为这片干渴的土地带来了希望。
多语言BERT模型,这个强大的知识容器,汇聚了多种语言的智慧精华。
它不仅能够理解不同语言之间的微妙差异,还能捕捉到它们之间的内在联系。
通过将这种多语言知识注入到低资源神经机器翻译系统中,我们仿佛为翻译引擎装上了一副“鹰眼”,使其能够在复杂多变的语言环境中精准导航。
想象一下,一个旅行者在异国他乡,面对陌生的路标和指示,如果没有地图或向导的帮助,他将寸步难行。
同样,对于低资源语言翻译系统来说,缺乏足够的训练数据就如同失去了方向感。
但是,当我们将多语言BERT模型的知识注入其中时,就好比为旅行者提供了一张详尽的地图和一位经验丰富的向导,让他能够轻松地找到前进的道路。
这种知识的注入并非简单的堆砌,而是一种精心策划的融合。
我们不是简单地将多语言BERT模型的知识添加到翻译系统中,而是通过一种名为“知识蒸馏”的技术,将其精髓提炼出来,并巧妙地融入到翻译模型的每一个细节中。
这就像是一位厨师在烹饪美食时,不是简单地将各种食材混合在一起,而是根据食材的特性进行精心搭配和调味,最终呈现出一道色香味俱佳的佳肴。
通过这种知识增强的方式,低资源神经机器翻译系统的性能得到了显著提升。
原本模糊不清的语义变得清晰起来,原本难以捉摸的语境变得易于理解。
这就像是为一幅模糊的画作重新上色,使得画面变得更加鲜明和生动。
然而,我们也不得不面对一个现实:尽管多语言BERT模型的知识增强为低资源语言翻译带来了巨大的潜力,但这项技术仍然处于起步阶段。
它就像一座刚刚打下基础的大厦,还需要我们不断添砖加瓦,才能最终建成一座巍峨壮观的建筑。
- 1、下载文档前请自行甄别文档内容的完整性,平台不提供额外的编辑、内容补充、找答案等附加服务。
- 2、"仅部分预览"的文档,不可在线预览部分如存在完整性等问题,可反馈申请退款(可完整预览的文档不适用该条件!)。
- 3、如文档侵犯您的权益,请联系客服反馈,我们会尽快为您处理(人工客服工作时间:9:00-18:30)。
Practical,Distributed Network CoordinatesRuss Cox,Frank Dabek,Frans Kaashoek,Jinyang Li,Robert MorrisMIT Laboratory for Computer Science{rsc,fdabek,kaashoek,jinyang,rtm}@ ABSTRACT–Vivaldi is a distributed algorithm that assignssynthetic coordinates to Internet hosts,so that the Euclidean dis-tance between two hosts’coordinates predicts the network latencybetween them.Each node in Vivaldi computes its coordinates by sim-ulating its position in a network of physical springs.Vivaldi is bothdistributed and efficient:nofixed infrastructure need be deployedand a new host can compute useful coordinates after collecting la-tency information from only a few other hosts.Vivaldi can rely onpiggy-backing latency information on application traffic instead ofgenerating extra traffic by sending its own probe packets.This paper evaluates Vivaldi through simulations of750hosts,with a matrix of inter-host latencies derived from measurements be-tween750real Internet hosts.Vivaldifinds synthetic coordinates thatpredict the measured latencies with a median relative error of14percent.The simulations show that a new host joining an existingVivaldi system requires fewer than10probes to achieve this accu-racy.Vivaldi is currently used by the Chord distributed hash tableto perform proximity routing,replica selection,and retransmissiontimer estimation.1IntroductionSynthetic coordinate systems are an approach to predictinginter-host Internet latencies.Nodes compute synthetic coordi-nates such that the Euclidean distance between the syntheticcoordinates of different nodes predict latency in the Internet.Thus,if a node x learns about the coordinates of a node y withwhich it hasn’t communicated before,x doesn’t have to per-form an explicit measurement to determine the latency to y;instead,the Euclidean distance between x and y in the spaceis an accurate predictor of the latency.The ability to predict latency without prior communicationallows systems to use proximity for better performance withless measurement overhead.A coordinate system could beused to select which of a number of replicated servers to fetcha data item from;such a system is particularly helpful whenthe number of potential servers is large or the amount of datais small.In either case it would not be practical tofirst probeall the servers tofind the closest,since the cost of the probeswould outweigh the benefit of an intelligent choice.Contentdistribution andfile-sharing systems such as KaZaA[10],Bit-Torrent[2],and CoDeeN[23]are examples of systems thatoffer a large number of replica servers.CFS[5]and DNS[12]is the latency between x and y.The output is a set of coordi-nates.Finding the best coordinates is equivalent to minimiz-ing the error(E)between predicted distances and the supplied distances.We use a simple squared error function:E= x y(M xy−dist(x,y))2where dist(x,y)is the standard Euclidean distance between coordinates of x and y.Vivaldi uses an algorithm based on a simulation of a net-work of physical springs to minimize E.This algorithm was inspired by work on model reconstruction[9];it mirrors a similar recent approach using forcefields[20].Conceptually, Vivaldi places a spring between each pair of nodes for which it knows the network latency,with the rest length set to that latency.The length of each spring is the distance between the current coordinates of the two nodes.The potential energy of a spring is proportional to the displacement from its rest length squared:this displacement is identical to the prediction error of the coordinates.Therefore minimizing the potential energy of the spring system corresponds to minimizing the prediction error E.Simulating spring relaxation requires much less computa-tion than more general optimization optimization algorithms such as the simplex algorithm(used by GNP)and produces similarly accurate results.The spring-based algorithm outper-forms simplex mainly because it takes advantage of gradient information to move the solution toward a minimal error so-lution;simplex does not depend on such gradient information and explores the the solution space in a less directed manner.Vivaldi simulates the physical spring system by running the system through a series of small time steps.At each time step,the force on each node is calculated and the node moves in the direction of that force.The node moves a distance pro-portional to the applied force and the size of the time step. Each time a node moves it decreases the energy of the system; however,the energy of the system stored in the springs will typically never reach zero since network latencies don’t actu-ally reflect an Euclidean space.Neither the spring relaxation nor the simplex algorithm is guaranteed tofind the global minimal solution;both can converge to a local minimum. 2.2Distributed calculationIn the distributed version of Vivaldi,each node simulates a piece of the overall spring system.A node maintains an es-timate of its own current coordinates,starting at the origin. Whenever two nodes communicate,the two nodes measure the latency between them and exchange their current syn-thetic coordinates.In RPC-based systems,this measurement can be accomplished by timing the RPC;in a stream oriented system,the receiver might echo a timestamp.An application might choose to make several measurements and report the minimum(or median)to Vivaldi,however,in the current de-ployment of Vivaldi on the Chord distributed lookup system //called for each new measurement.//s_c is the other host’s coordinates.//s_l is the one-way latency to that host.//δstarts at 1.0.update(s_c,s_l){//unit vector towards other hostVector dir=s_c-my_c;dir=dir/length(dir);//Distance from spring’s rest positiond=dist(s_c,my_c)-s_l;//displacement from rest positionVector x=dir*d;//reduceδat each sampleδ-=0.025;//but stop at0.05δ=max(0.05,δ);x=x*δ;//apply the forcemy_c=my_c+x;}Figure1:Pseudo-code for the Vivaldi update routine.update() moves the node’s coordinates(myUnless otherwise noted,we perform the simulations as fol-lows.Each simulation involves750nodes.Each node starts with its synthetic location at the origin.Nodes take latency samples from randomly chosen other nodes.Synthetic co-ordinates have5dimensions;more dimensions provide bet-ter accuracy,but the improvement is small after two dimen-sions.This result is supported by principle component analy-sis on the matrix of latencies(omitted here but available sepa-rately[4]),and by similar observations by Ng and Zhang[14].3.1Latency dataThe Vivaldi simulations are driven by a matrix of inter-host Internet latencies;Vivaldi uses a subset of the latencies for its samples,and the full matrix is needed to evaluate the quality of Vivaldi’s resulting predictions.Since deriving realistic la-tencies from Internet topology models is difficult,we chose to use measured latencies.We built a tool based on the King method[8]to collect a full matrix of latencies among750Internet DNS servers. To determine the distance between DNS server A and server B wefirst measure the round trip time to server A and then ask server A to recursively resolve a domain served by B. The difference in times between the two operations yields an estimate of the round trip time between A and B.We use half the round trip time as the latency.We harvested the addresses of recursive DNS servers by extracting the NS records for IP addresses of hosts participat-ing in a Gnutella network.If a domain is served by multiple, geographically diverse name servers,queries targeted at do-main D(and intended for name server B)could be forwarded to a different name server,C,which also serves D.Our tool cannot control where queries are forwarded.To avoid this er-ror,the list of target domains and name servers wasfiltered to include only those domains where all authoritative name servers are on the same subnet(i.e.the IP addresses of the name servers are identical except for the low octet).An asynchronous tool was used to determine the N(N−1) pair-wise latencies using the King method.Thefinal latency for a given pair was taken to be the minimum of10tri-als,in order tofilter out queuing delays and misses in DNS server caches.Collecting all pairwise latencies required sev-eral hours.Figure2shows the cumulative distribution of la-tencies produced from this data set.Because they are name servers,the machines included in the King trace are likely to be well connected to the Internet.The servers are geographi-cally diverse,however,and include machines in North Amer-ica,Europe,and Asia.For comparison,Figure2also shows pair-wise latencies obtained by direct measurements of192 PlanetLab hosts[1].The King data has higher median la-tency(100msec)than the PlanetLab data set(75msec);this is likely due to the fact that most PlanetLab hosts are located at North American universities with fast Internet2connections.We used the N(N−1)latencies produced by the King tool as the input to a packet-level peer-to-peer simulator[7].0100200300400500Latency (milliseconds)0.00.20.40.60.81.0CumulativefractionofnodepairsFigure2:The cumulative distribution of pairwise round-trip laten-cies intedSamplesError(msec)Figure3:A comparison of Vivaldi’s convergence time using dif-ferent,fixed values ofδ.Curves plot the error after the given num-ber of samples;the error is calculated as the median of all round-trip pair-wise differences between predicted and actual latency.The lines marked with numbers indicate performance with the givenfixedδ; the bold line marked“decreasing”shows the actual algorithm’s per-formance.The simulator delays each RPC packet by the time specified in the King data.Each node runs an instance of Vivaldi which sends RPCs to other nodes,measures the RPCs’RTTs,and uses those RTTs to update its synthetic coordinates.3.2Setting the timestepTheδvariable(the timestep used during the spring simula-tion)in Figure1affects how fast Vivaldi converges.Figure3 compares Vivaldi’s performance with a range offixedδval-ues against the actual algorithm’s slowly decreasingδ.Each curve in Figure3shows results from a simulation with a dif-ferentfixedδ.Each simulation begins with all750nodes starting Vivaldi at the same time.The x-axis reflects how much time has passed;the units are the number of samples each node has taken.The y-axis shows median error over all pair-wise predictions.Figure3shows that smallδvalues(such as0.001)result in long convergence times.Intermediate values(as large as# Samples20406080E r r o r (m s e c )Initial nodes Final nodeFigure 4:This figure compares Vivaldi’s convergence time whenall nodes join at once with the convergence time when a few nodes join an existing converged system.The solid line shows a node join-ing an already stable system:the node converges after collecting fewer than 10samples.0.5)result in much faster convergence at the cost of some accuracy.As δincreases,final accuracy decreases,since large δvalues allow nodes to vibrate more around their “correct”positions.Very large δvalues (such as 1.0)cause the system to oscillate and fail to converge.The bold line marked “decreasing”in Figure 3shows the performance with a decreasing δ.The initial value is large,so the error decreases quickly.δeventually becomes small,so the error converges to a low value and does not ing the decreasing δ(with a minimum of 0.05),the median error drops below 20ms after 70samples.When δis fixed at 0.05from the start of the simulation,the error does not drop below 20ms until sample 157.Vivaldi has no user-tunable parameters other than δ.This simplicity makes the algorithm more robust and easier to de-ploy.3.3Time to ConvergenceFigure 3also shows that when all nodes join at the same time with incorrect initial coordinates,the system converges slowly (the median error is still dropping after 500timesteps).Many applications of Vivaldi are likely to involve new nodes joining a larger existing system;thus new nodes are likely to join a system whose Vivaldi coordinates have already con-verged.Figure 4shows what happens when 10new nodes join a converged Vivaldi system of 740nodes.The solid line shows the error for one of the 10nodes.The dashed line shows how long 740nodes take to converge when they all start at once.New nodes arrive at good coordinates with less than ten samples.The algorithm’s large initial δallows the new node to converge rapidly in this case.The spike in the solid line around sample three is caused by the fact that the initial δis probably too large and allows a brief initial period of oscilla-tion.3.4Vivaldi AccuracyTo understand the accuracy of Vivaldi we measured the er-ror in the prediction of each pairwise latency using a relative error metric [14]:abs (measured −predicted )0.00.51.01.52.0Relative error0.00.20.40.60.81.0C u m u l a t i v e f r a c t i o n o f n o d e p a i r sGNP (20 landmarks)Vivaldi (random comm.)Vivaldi (32 neighbors)Vivaldi (64 neighbors)Figure 5:Vivaldi and GNP accuracy.The solid line shows Vivaldi’saccuracy when nodes’communication is unrestricted.The dashed line shows Vivaldi’s accuracy when nodes are restricted to commu-nicating with 32neighbors.Several GNP experiments were run,each with a different set of 32random landmarks.The dotted line denotes the median result,the error bars show the best and worst prediction errors.cations such as KaZaA.We developed Vivaldi to assist prox-imity routing and server selection in the Chord [21]peer-to-peer lookup system.We will describe that application in detail here.Chord uses coordinates to efficiently build routing tables based on proximity so that lookups are likely to proceed to nearby nodes.A node receives a list of candidate nodes and selects the one that is closest in coordinate space as its routing table entry;coordinates allow the node to make this decision without probing each candidate.Chord utilizes coordinates when performing an iterative lookup.When a node n 1initiates a lookup and routes it through some node n 2,n 2chooses a next hop that is close to n 1based on Vivaldi coordinates.In an iterative lookup n 1sends an RPC to each intermediate node in the route,so prox-imity to n 1is more important than proximity to n 2.A new version of DHash [5],a distributed hash table built on top of Chord,uses Vivaldi to perform server selection.DHash uses erasure coding to divide blocks into a number of fragments.Only a subset of these fragments are necessary to reconstruct the original block.A node fetches a block by asking the block’s successor for a list of the nodes holding the fragments,along with the coordinates of those nodes.The fetching node then fetches fragments from the nodes with the closest coordinates.If the node had to first measure the la-tency to each of these nodes,the extra round-trip time would probably cancel out the benefit of choosing the closest frag-ments.Chord (and DHash)also use Vivaldi in their RPC system.Chord sends RPCs over UDP,so Chord must handle retrans-missions.Chord often contacts other nodes just once,so it cannot profitably measure the round-trip time in order to set the RPC retransmission timer.Instead,Chord uses a small multiple of the latency predicted by Vivaldi as the initial RPCretransmission timer.Chord and DHash required a few modifications to use Vi-valdi.Whenever one node sends an RPC request or reply to another,it includes its own coordinates.The RPC system times each RPC and tells Vivaldi the measured latency and the coordinates of the other node.This allows Vivaldi to col-lect information without much added overhead,since the co-ordinates increase the size of each RPC message by just 12bytes.In addition,whenever nodes exchange routing infor-mation about other nodes,they send along the coordinates of those other nodes as well as their IP addresses.Thus Chord always knows the coordinates of any node it is about to con-tact,even if it has never talked to that node before.After we modified DHash to perform proximity routing and replica selection using Vivaldi the time required to fetch a block decreased by a factor of two when the system was run on the PlanetLab test bed.5Related workVivaldi was inspired by GNP [14],which demonstrated that coordinates can effectively describe the latencies between hosts on the Internet.Vivaldi’s contribution is a distributed al-gorithm that computes coordinates without landmark nodes.IDMaps [6]is a proposed infrastructure to help hosts pre-dict Internet latency to each other.The IDMaps infrastruc-ture consists of a few hundred or thousand tracer nodes.Ev-ery tracer measures the Internet latency to every other tracer.The tracers also measure the latency to every CIDR address prefix,and jointly determine which tracer is closest to each prefix.Then the latency between host h 1and host h 2can be estimated as the latency from the prefix of h 1to that prefix’s tracer,plus the latency from the prefix of h 2to that prefix’s tracer,plus the latency between the two tracers.One advan-tage of IDMaps over Vivaldi is that IDMaps reasons about IP address prefixes,so it can make predictions about hosts that are not even aware of the IDMaps system.Vivaldi,on the other hand,requires no separate infrastructure.Waldvogel and Rinaldi [22,19]describe a spring relax-ation technique to assign IDs to landmark nodes as part of the Mithos proximity-aware overlay network.A number of as-pects of their algorithm require a centralized implementation.Lighthouse [15]is an extension of GNP that is intended to be more scalable.Lighthouse,like GNP,has a special set of landmark nodes.Unlike GNP,a node that joins Lighthouse does not have to query those global landmarks.Instead,the new node can query any existing set of nodes to find its coor-dinates relative to that set,and then optionally transform those coordinates into coordinates relative to the global landmarks.Priyantha et al.[16]describe a distributed node localiza-tion system for wireless sensor networks that uses spring re-laxation.The sensors use ultrasound propagation times to measure inter-sensor distances and cooperate to derive co-ordinates consistent with those distances.Much of the algo-rithm is devoted to solving a problem that doesn’t affect Vi-valdi:the fact that two non-adjacent sensors cannot measure the distance between themselves makes it hard for the system to avoid letting the coordinate space double back on itself.Rao et.al.[17]compute virtual coordinates for use in geo-graphic forwarding in a wireless ad-hoc network.Their algo-rithm does not attempt to predict latencies;instead,the pur-pose is to make sure that directional routing works.A number of peer-to-peer networks incorporate proximity routing[18,3,11].We hope that many of these systems will benefit from using synthetic coordinates.6Conclusions and DiscussionVivaldi is a simple,distributed algorithm forfinding syn-thetic coordinates that accurately predict Internet latencies. Vivaldi is fully distributed;for example,it does not require a pre-selected subset of nodes to be designated as landmarks. Vivaldi is simple;it has only one tunable parameter.These properties make it easy to deploy Vivaldi in distributed sys-tems:we have used Vivaldi to improve the performance of the Chord peer-to-peer lookup system.Additional work remains to further understand and im-prove Vivaldi’s performance.For example,we are exploring how nodes’communication patterns affect prediction accu-racy.We are also interested in determining to what extent Internet latencies can be embedded in a N-dimensional Eu-clidean space and the reasons such an embedding is possible. Finally,we plan to modify additional distributed applications to take advantage of Vivaldi and quantify how much Vivaldi improves performance.References[1].[2]BitTorrent./BitTorrent/protocol.html.[3]Miguel Castro,Peter Druschel,Y.C.Hu,and Antony Row-stron.Exploiting network proximity in peer-to-peer overlay networks.Technical Report MSR-TR-2002-82,Microsoft Re-search,June2002.[4]Russ Cox and Frank Dabek.Learning Euclidean coordinatesfor Internet /˜rsc/6867.pdf,De-cember2002.[5]Frank Dabek,M.Frans Kaashoek,David Karger,Robert Mor-ris,and Ion Stoica.Wide-area cooperative storage with CFS.In Proc.18th ACM Symposium on Operating Systems Prin-ciples(SOSP’01),October2001.http://www.pdos.lcs./chord.[6]P.Francis,S.Jamin,C.Jin,Y.Jin,D.Raz,Y.Shavitt,andL.Zhang.IDMaps:A global Internet host distance estima-tion service.IEEE/ACM Transactions on Networking,October 2001.[7]Thomer Gil,Jinyang Li,Frans Kaashoek,and Robert Mor-ris.Peer-to-peer simulator,2003.Source available at: .[8]Krishna P.Gummadi,Stefan Saroiu,and Steven D.Gribble.King:Estimating latency between arbitrary Internet end hosts.In Proc.of SIGCOMM IMW2002,November2002.[9]H.Hoppe.Surface reconstruction from unorganized points.PhD thesis,Department of Computer Science and Engineer-ing,University of Washington,1994.[10]KaZaA media dekstop./.[11]John Kubiatowicz,David Bindel,Yan Chen,Steven Czer-winski,Patrick Eaton,Dennis Geels,Ramakrishna Gummadi, Sean Rhea,Hakim Weatherspoon,Westley Weimer,Chris Wells,and Ben Zhao.OceanStore:An architecture for global-scale persistent storage.In Proceeedings of the Ninth interna-tional Conference on Architectural Support for Programming Languages and Operating Systems(ASPLOS2000),pages 190–201,Boston,MA,November2000.[12]P.Mockapetris and K.J.Dunlap.Development of the Do-main Name System.In Proc.ACM SIGCOMM,pages123–133,Stanford,CA,1988.[13]Eugene Ng.Gnp software,2003.Source available at:/~eugeneng/research/gnp/ software.html.[14]T.S.Eugene Ng and Hui Zhang.Predicting Internet networkdistance with coordinates-based approaches.In Proceedings of IEEE Infocom2002,2002.[15]Marcelo Pias,Jon Crowcroft,Steve Wilbur,Tim Harris,andSalem Bhatti.Lighthouses for scalable distributed location.In IPTPS,2003.[16]N.Priyantha,H.Balakrishnan,E.Demaine,and S.Teller.Anchor-free distributed localization in sensor networks.Tech-nical Report TR-892,MIT LCS,April2003.[17]Ananth Rao,Sylvia Ratnasamy,Christos Papadimitriou,ScottShenker,and Ion Stoica.Geographic routing without location information.In ACM MobiCom Conference,September2003.[18]Sylvia Ratnasamy,Mark Handley,Richard Karp,and ScottShenker.Topologically-aware overlay construction and server selection.In Proceedings of IEEE Infocom2002,2002. [19]Roberto Rinaldi and Marcel Waldvogel.Routing and data lo-cation in overlay peer-to-peer networks.Research Report RZ–3433,IBM,July2002.[20]Yuval Shavitt and Tomer Tankel.Big-bang simulation for em-bedding network distances in Euclidean space.In Proc.of IEEE Infocom,April2003.[21]Ion Stoica,Robert Morris,David Liben-Nowell,David Karger,M.Frans Kaashoek,Frank Dabek,and Hari Balakrishnan.Chord:A scalable peer-to-peer lookup protocol for internet applications.IEEE/ACM Transactions on Networking,pages 149–160,2002.[22]Marcel Waldvogel and Roberto Rinaldi.Efficient topology-aware overlay network.In Hotnets-I,2002.[23]Limin Wang,Vivek Pai,and Larry Peterson.The Effectivenessof Request Redirecion on CDN Robustness.In Proceedings of the Fifth Symposium on Operating Systems Design and Imple-mentation,Boston,MA USA,December2002.。