基于人工免疫模型的在线手写签名识别方法
如何使用AI技术进行手写识别
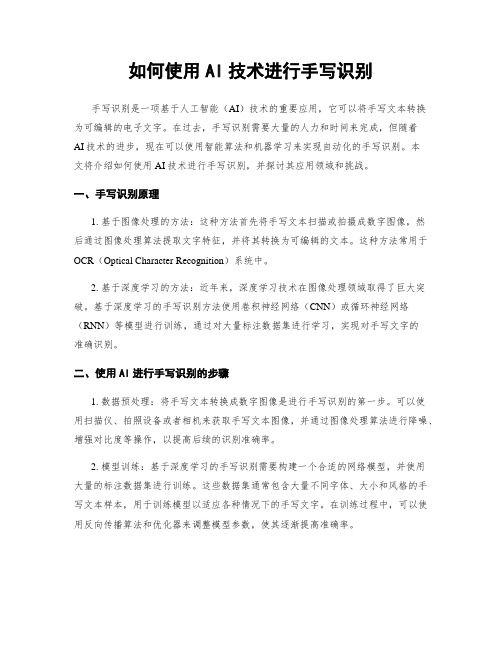
如何使用AI技术进行手写识别手写识别是一项基于人工智能(AI)技术的重要应用,它可以将手写文本转换为可编辑的电子文字。
在过去,手写识别需要大量的人力和时间来完成,但随着AI技术的进步,现在可以使用智能算法和机器学习来实现自动化的手写识别。
本文将介绍如何使用AI技术进行手写识别,并探讨其应用领域和挑战。
一、手写识别原理1. 基于图像处理的方法:这种方法首先将手写文本扫描或拍摄成数字图像,然后通过图像处理算法提取文字特征,并将其转换为可编辑的文本。
这种方法常用于OCR(Optical Character Recognition)系统中。
2. 基于深度学习的方法:近年来,深度学习技术在图像处理领域取得了巨大突破。
基于深度学习的手写识别方法使用卷积神经网络(CNN)或循环神经网络(RNN)等模型进行训练,通过对大量标注数据集进行学习,实现对手写文字的准确识别。
二、使用AI进行手写识别的步骤1. 数据预处理:将手写文本转换成数字图像是进行手写识别的第一步。
可以使用扫描仪、拍照设备或者相机来获取手写文本图像,并通过图像处理算法进行降噪、增强对比度等操作,以提高后续的识别准确率。
2. 模型训练:基于深度学习的手写识别需要构建一个合适的网络模型,并使用大量的标注数据集进行训练。
这些数据集通常包含大量不同字体、大小和风格的手写文本样本,用于训练模型以适应各种情况下的手写文字。
在训练过程中,可以使用反向传播算法和优化器来调整模型参数,使其逐渐提高准确率。
3. 测试与验证:在模型训练完成后,需要利用测试数据集对模型进行验证和评估。
测试数据集应该包含与训练数据集不同的样本,以更好地了解模型的泛化能力和准确性。
通过不断迭代优化模型参数并评估性能,可以达到最佳识别效果。
4. 实际应用:完成模型验证后,可以将其应用于实际场景中。
无论是数字签名验证、自动表单填充还是电子化文档管理,手写识别技术都能够极大地提高工作效率和准确性。
基于人工智能笔迹鉴定相似度标准
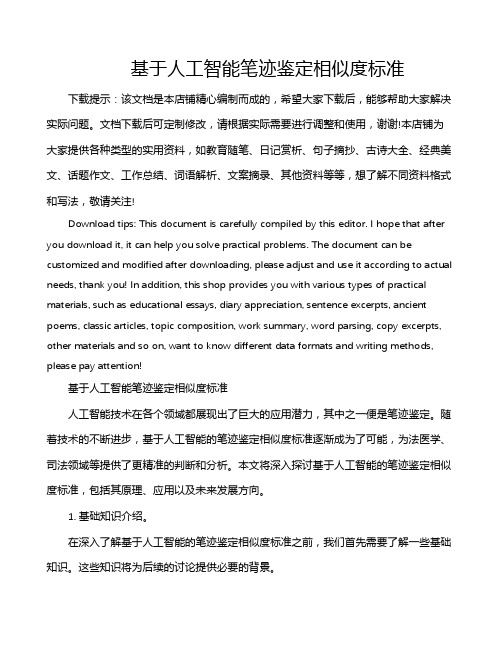
基于人工智能笔迹鉴定相似度标准下载提示:该文档是本店铺精心编制而成的,希望大家下载后,能够帮助大家解决实际问题。
文档下载后可定制修改,请根据实际需要进行调整和使用,谢谢!本店铺为大家提供各种类型的实用资料,如教育随笔、日记赏析、句子摘抄、古诗大全、经典美文、话题作文、工作总结、词语解析、文案摘录、其他资料等等,想了解不同资料格式和写法,敬请关注!Download tips: This document is carefully compiled by this editor. I hope that after you download it, it can help you solve practical problems. The document can be customized and modified after downloading, please adjust and use it according to actual needs, thank you! In addition, this shop provides you with various types of practical materials, such as educational essays, diary appreciation, sentence excerpts, ancient poems, classic articles, topic composition, work summary, word parsing, copy excerpts, other materials and so on, want to know different data formats and writing methods, please pay attention!基于人工智能笔迹鉴定相似度标准人工智能技术在各个领域都展现出了巨大的应用潜力,其中之一便是笔迹鉴定。
基于ANN和HMM的联机手写体汉字识别系统

基于ANN和HMM的联机手写体汉字识别系统
张冬霞
【期刊名称】《微计算机信息》
【年(卷),期】2005(000)024
【摘要】为了解决联机手写体汉字笔划顺序、笔划数目及笔划形状变化问题,提出了一种新的联机手写体汉字识别方法:人工神经网络(ANN)和隐马尔可夫模型(HMM)相结合的汉字识别方法,首先通过BP神经网络进行笔划识别,再通过笔划类型和笔划间位置关系的隐马尔可夫模型进行整字识别.实验证明,该联机手写体汉字识别系统具有较高地识别准确率.
【总页数】3页(P144-146)
【作者】张冬霞
【作者单位】100083,北京科技大学计算机应用技术系
【正文语种】中文
【中图分类】TP391.43
【相关文献】
1.基于部件HMM级联的联机手写体汉字识别方法 [J], 赵巍;刘家锋;唐降龙
2.基于ANN/HMM混合模型汉语大词表连续语音识别系统 [J], 蒋瑞;李海峰;马琳
3.基于ANN和HMM的联机手写体汉字识别系统 [J], 张冬霞
4.基于HMM的联机汉字识别系统及其改进的训练方法 [J], 刘家锋;黄健华;唐降龙
5.基于HMM和ANN汉语普通话口语测评系统的实现 [J], 马亮;程陈;任海军;王文青;周辉
因版权原因,仅展示原文概要,查看原文内容请购买。
手写文字在线识别的方法
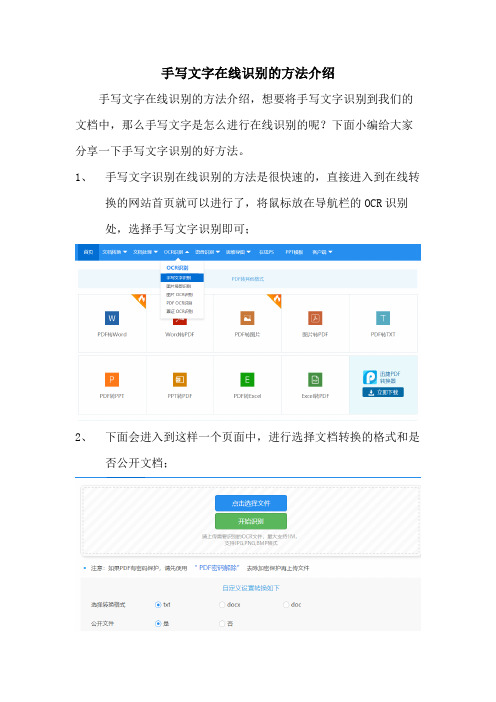
手写文字在线识别的方法介绍
手写文字在线识别的方法介绍,想要将手写文字识别到我们的文档中,那么手写文字是怎么进行在线识别的呢?下面小编给大家分享一下手写文字识别的好方法。
1、手写文字识别在线识别的方法是很快速的,直接进入到在线转
换的网站首页就可以进行了,将鼠标放在导航栏的OCR识别处,选择手写文字识别即可;
2、下面会进入到这样一个页面中,进行选择文档转换的格式和是
否公开文档;
3、选择自定义设置之后就可以进行文件的打开,点击选择手写文
字,如果嫌麻烦的话可以直接将图片拖拽到界面中,进行打开;
4、图片选择完成之后就可以进行手写文字识别的识别环节了,点
击开始识别即可;
5、识别是需要一定的过程的,我们需要耐心的等待一段时间,等
图片是识别完成即可;
6、识别完成之后就可以进行文件的直接打开或是下载了。
以上就是小编所介绍的手写文字在线识别的简单方法了,希望可以帮助到大家。
基于人工免疫模型的在线手写签名识别方法

何 、 理和 时序等 多 种 线 索来 捕 捉 用户 的签 名 习 惯 物 和区分 用户签 名 的 差异 , 有 较 高 的 正确 性 和 安 全 具
性 , 为手写 签 名 识 别 领 域 的主 流 技 术 . 有 对 在 成 已 线手写 签名识 别 的研 究 主 要 包 括 两 大方 面 : 征选 特 择和样 本训 练 . 方 面 , 效 的 签 名 特 征 表 示 是 手 一 有
Ke r o lneh n — itn sg a ur e o niin;f a u es lc in;s mp e tani y wo ds n i a d wrte i n t e r c g to e t r ee to a l r i ng;a tfca mmu riiili ne
c mmo y u e sgn t r fa u e , a u i z s he efla nig nd efa p a in o a tfca i o nl s d i a u e e t r s nd tl e t s l—e r n a s l— da t to f riiil mmu i ne t e r t o t i ne h o y o b an w mo l wih des t hi e d si gus bi t whe t tani s mp e a e i td. gh r itn iha l y i n he r i ng a ls r l mie Ex rme ss o t a hi p o c ro msweli a l r i i g a d r s ls i a ifc o y v rfc to pe i nt h w h tt sa pr a h pe f r l n s mpe t an n n e u t n s ts a t r e iia i n r t nd i n iia in r t . a e a de tfc to a e
人工免疫系统在手写希腊字符识别中的应用

人工免疫系统在手写希腊字符识别中的应用基于自然免疫系统的人工免疫系统是人工神经网络和进化计算之后的新型计算智能,以其强大的信息处理和问题解决能力被广泛应用到众多领域。
本文提出了基于人工免疫系统的手写希腊字符识别策略并进行了实验仿真。
利用3*3子网格算法对24*24字符图像样本提取64个特征参数,通过人工免疫算法训练图像样本,建立24个抗体库用于识别手写希腊字符。
另外,利用BP神经网络进行了比照实验。
实验结果说明,人工免疫系统模型在手写希腊字符识别中较BP神经网络具有更大的优势。
希腊字母广泛应用于数学、物理、生物和天文学中,手写希腊字母的识别对于手写公式识别是非常重要的。
然而,目前手写识别的相关研究主要集中在数字、英文字符、中文字符、阿拉伯字符的手写识别中,手写希腊字母还没有很好的识别算法。
因此,它的研究具有重要的理论和实践价值。
人工免疫系统是基于脊椎动物免疫系统处理过程的计算系统,通过改变系统结构和函数增强其适应性。
免疫算法应用了免疫系统的学习和记忆特征,因此广泛用于解决数学、工程、信息技术等领域的计算问题。
本文将人工免疫系统用于识别手写希腊字符识别并取得了较好的效果。
预处理是字符识别重要过程,有助于特征提取、提高识别正确率和识别效率。
本文对24个手写希腊字母进行了初步识别。
1680个原始字符图像由70个学生完成,即每个字符类别为70个样本。
预处理被应用于图像样本以消除与字符分类不相关因素的影响。
首先,利用3*3 KNN 平滑滤波器进行去噪,使图像的特征信息更加突出。
其次,对手写图像样本进行归一化。
归一化方式包括位置、大小和灰度,使用线性拉伸与压缩算法,将字符图像归一化为24*24。
归一化后,利用Pavlidis 算法尽可能地将字符比划的宽度降低到一个像素。
最后,使用值算法将图像进行二值化。
目前存在很多图像特征提取方式,提取方式的选择对于识别效果是至关重要的。
本文采用了网格特征提取用于手写希腊字符识别。
基于神经网络的在线手写签名识别技术
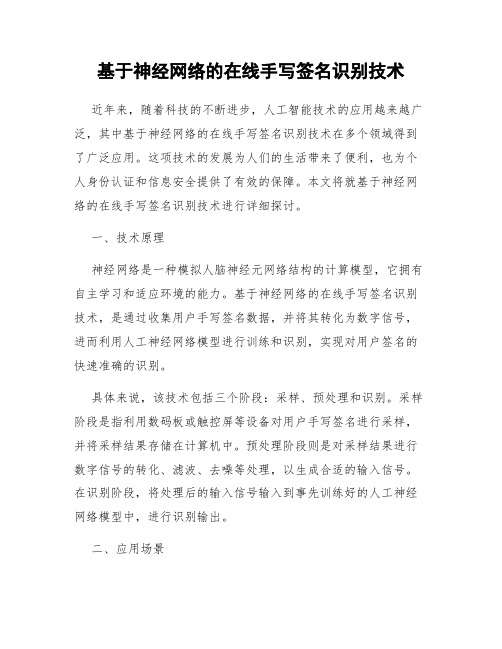
基于神经网络的在线手写签名识别技术近年来,随着科技的不断进步,人工智能技术的应用越来越广泛,其中基于神经网络的在线手写签名识别技术在多个领域得到了广泛应用。
这项技术的发展为人们的生活带来了便利,也为个人身份认证和信息安全提供了有效的保障。
本文将就基于神经网络的在线手写签名识别技术进行详细探讨。
一、技术原理神经网络是一种模拟人脑神经元网络结构的计算模型,它拥有自主学习和适应环境的能力。
基于神经网络的在线手写签名识别技术,是通过收集用户手写签名数据,并将其转化为数字信号,进而利用人工神经网络模型进行训练和识别,实现对用户签名的快速准确的识别。
具体来说,该技术包括三个阶段:采样、预处理和识别。
采样阶段是指利用数码板或触控屏等设备对用户手写签名进行采样,并将采样结果存储在计算机中。
预处理阶段则是对采样结果进行数字信号的转化、滤波、去噪等处理,以生成合适的输入信号。
在识别阶段,将处理后的输入信号输入到事先训练好的人工神经网络模型中,进行识别输出。
二、应用场景基于神经网络的在线手写签名识别技术已经得到了广泛应用,尤其在口头承诺、交易确认、信息认证等需要身份验证的场景中,该技术发挥了重要作用。
其中,电子合同中的在线签名便是一个重要应用场景。
以国内电子合同平台“合同锁”为例,其在线手写签名识别技术可以实现对用户签名的快速识别,并在多个企业间验证签名的合法性,从而大大提高了电子合同的安全性和可靠性。
此外,基于神经网络的在线手写签名识别技术也被广泛应用于人脸识别、银行卡支付、智能门禁等领域,并在追踪罪犯和预防欺诈等领域中发挥了重要作用。
三、技术优势相比传统的手写签名识别技术,基于神经网络的在线手写签名识别技术具有以下明显优势:(1)数据量大:与传统手写识别技术相比,基于神经网络的识别技术可以接受更多的训练数据,从而提高了模型的识别能力和准确度。
(2)误差率低:由于神经网络具有学习和适应环境的特点,其可以在多方面进行优化,从而降低了识别误差率,提高了识别的精度和准确度。
基于人工智能技术的手写体识别与分析研究

基于人工智能技术的手写体识别与分析研究手写体识别与分析是人工智能技术领域的一个重要应用,它使得计算机能够自动识别和理解手写的字符和文字。
本文将探讨基于人工智能技术的手写体识别与分析的研究现状、方法和应用。
一、研究现状手写体识别与分析是一门跨学科的研究领域,涉及图像处理、机器学习、模式识别等多个学科。
近年来,随着深度学习算法的发展,基于人工智能技术的手写体识别与分析取得了显著的进展。
目前,许多研究团队致力于提高手写体识别的准确性和稳定性。
他们采用了大规模数据集进行训练,使用深度神经网络模型,如卷积神经网络(CNN)和循环神经网络(RNN),来提取字符的特征信息。
同时,通过引入注意力机制和迁移学习等方法,进一步提高了手写体识别的性能。
此外,研究者们还在手写体识别与分析的应用领域做了大量的工作。
例如,在金融行业,手写体识别可以应用在支票和汇票的自动处理中;在教育领域,手写体识别可以帮助学生进行作文评分和错别字检测;在医疗保健领域,手写体识别可以用于医生的处方识别和病历自动归档等。
二、方法基于人工智能技术的手写体识别与分析主要包括以下几个步骤:图像获取、图像预处理、特征提取、分类与识别。
1. 图像获取:首先需要获取手写字符或文字的图像。
可以通过扫描仪、摄像头或者数码相机等设备获得高质量的手写图像。
2. 图像预处理:手写图像往往存在噪点、光照变化等问题,需要进行预处理以提高识别的准确性。
预处理包括图像去噪、图像增强等步骤。
3. 特征提取:手写字符和文字的特征信息对于识别任务非常重要。
可以使用传统的特征提取方法,如HOG、SIFT等,也可以使用深度学习方法自动学习特征。
4. 分类与识别:经过特征提取后,通过训练分类器对手写图像进行识别。
常用的分类器包括支持向量机(SVM)、随机森林以及深度神经网络等。
三、应用领域基于人工智能技术的手写体识别与分析在各个领域都有广泛的应用。
1. 金融行业:手写体识别可以应用在银行支票和汇票的自动处理中,提高处理效率和准确性。
基于人工神经网络的无分割手写Devnagari签名实时识别(IJIGSP-V5-N4-4)
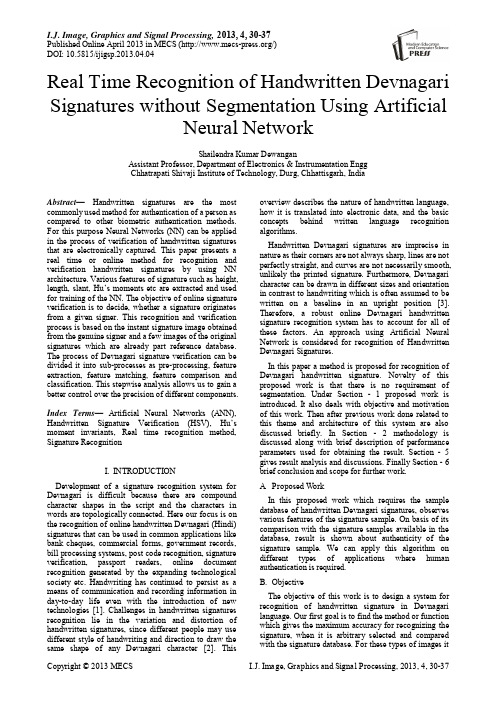
I.J. Image, Graphics and Signal Processing, 2013, 4, 30-37Published Online April 2013 in MECS (/)DOI: 10.5815/ijigsp.2013.04.04Real Time Recognition of Handwritten Devnagari Signatures without Segmentation Using ArtificialNeural NetworkShailendra Kumar DewanganAssistant Professor, Department of Electronics & Instrumentation EnggChhatrapati Shivaji Institute of Technology, Durg, Chhattisgarh, IndiaAbstract—Handwritten signatures are the most commonly used method for authentication of a person as compared to other biometric authentication methods. For this purpose Neural Networks (NN) can be applied in the process of verification of handwritten signatures that are electronically captured. This paper presents a real time or online method for recognition and verification handwritten signatures by using NN architecture. Various features of signature such as height, length, slant, Hu’s moments etc are extracted and used for training of the NN. The objective of online signature verification is to decide, whether a signature originates from a given signer. This recognition and verification process is based on the instant signature image obtained from the genuine signer and a few images of the original signatures which are already part reference database. The process of Devnagari signature verification can be divided it into sub-processes as pre-processing, feature extraction, feature matching, feature comparison and classification. This stepwise analysis allows us to gain a better control over the precision of different components. Index Terms—Artificial Neural Networks (ANN), Handwritten Signature Verification (HSV), Hu’s moment invariants, Real time recognition method, Signature RecognitionI.INTRODUCTIONDevelopment of a signature recognition system for Devnagari is difficult because there are compound character shapes in the script and the characters in words are topologically connected. Here our focus is on the recognition of online handwritten Devnagari (Hindi) signatures that can be used in common applications like bank cheques, commercial forms, government records, bill processing systems, post code recognition, signature verification, passport readers, online document recognition generated by the expanding technological society etc. Handwriting has continued to persist as a means of communication and recording information in day-to-day life even with the introduction of new technologies [1]. Challenges in handwritten signatures recognition lie in the variation and distortion of handwritten signatures, since different people may use different style of handwriting and direction to draw the same shape of any Devnagari character [2]. This overview describes the nature of handwritten language, how it is translated into electronic data, and the basic concepts behind written language recognition algorithms.Handwritten Devnagari signatures are imprecise in nature as their corners are not always sharp, lines are not perfectly straight, and curves are not necessarily smooth, unlikely the printed signature. Furthermore, Devnagari character can be drawn in different sizes and orientation in contrast to handwriting which is often assumed to be written on a baseline in an upright position [3]. Therefore, a robust online Devnagari handwritten signature recognition system has to account for all of these factors. An approach using Artificial Neural Network is considered for recognition of Handwritten Devnagari Signatures.In this paper a method is proposed for recognition of Devnagari handwritten signature. Novelty of this proposed work is that there is no requirement of segmentation. Under Section - 1 proposed work is introduced. It also deals with objective and motivation of this work. Then after previous work done related to this theme and architecture of this system are also discussed briefly. In Section - 2 methodology is discussed along with brief description of performance parameters used for obtaining the result. Section - 5 gives result analysis and discussions. Finally Section - 6 brief conclusion and scope for further work.A.Proposed WorkIn this proposed work which requires the sample database of handwritten Devnagari signatures, observes various features of the signature sample. On basis of its comparison with the signature samples available in the database, result is shown about authenticity of the signature sample. We can apply this algorithm on different types of applications where human authentication is required.B.ObjectiveThe objective of this work is to design a system for recognition of handwritten signature in Devnagari language. Our first goal is to find the method or function which gives the maximum accuracy for recognizing the signature, when it is arbitrary selected and compared with the signature database. For these types of images itis too much difficult to choose such a function which can be the best for the process of feature extraction and comparison for standardization.C.MotivationThe motivation behind the project is the growing need for a full proof signature verification scheme which can guarantee maximum possible security against fake signatures. The prospect of minimizing the memory required for storing the extracted feature points has also been a driving force in the commencement of this project.D.Previous WorkDevnagari script recognition is very challenging due to the complex structural properties of the script. Two different methods for extracting features from handwritten Devnagari characters can be used are the Curvelet Transform and the Character Geometry [1]. Topographical features of strokes visible with respect to views from different directions which can be successfully used for recognition of face, handwritten signature and printed characters [2]. Three feature extraction techniques as Hu’s Moment Invariants, Zernike moments and Krawtchouk moment can be used for recognition of signatures. These methods provide the stastical data which can be tested for various parameters like the length of feature vector, redundancy and accuracy [3]. A Neural Network based on-line signature verification system can be designed by using a set of feature vectors that can represent the characters of the signatures. By forming and verification of feather sets false acceptance (FA) and false rejection (FR) rate can be reduced [4].A writer independent offline handwritten signature verification system based on grey level feature extraction and Real Adaboost algorithm can be designed by using both global and local features of the script [5]. Object recognition can also be successfully done by using methods based on image moments. Various types of moments (geometric moments, complex moments) and moment-based invariants with respect to various image degradations and distortions (rotation, scaling, affine transform, image blurring, etc.) can be used as shape descriptors for classification [6]. Also efficient numerical algorithms can be used for moment computation and demonstrate practical examples of using moment invariants in real applications [7].E.Architecture of Recognition SystemThe entire process of recognition of handwritten signature can be categorized six sections. The architecture of the signature recognition system is shown in Fig. 1.(a) The first section is to obtain sample signature image from the signer. This sample image is later on compared with the database which is generated by collecting genuine signatures, collected from various human subjects.(b) In the second section the loaded sample signature image is cropped, because further testing process is done on that cropped section only. Cropping refers to the process of removing unwanted areas from aphotographic or illustrated image.Fig. 1 : Architecture of Signature Recognition System (c) In the third section the cropped image is converted into binary image. A binary image is a digital image that has only two possible values for each pixel. Typically the two colors used for a binary image are black and white though any two colors can be used.(d) The fourth section all the specified features of sample signature are extracted. Feature extraction involves simplifying the amount of resources required to describe a large set of data accurately. When performing analysis of complex data one of the major problems stems from the number of variables involved.(e) The fifth section all the features of sample signature image are compared with the signature database.(f) On basis of this comparison it is decided that whether the sample signature belongs to the signature database or it is an authorized signature.F.Designing ProcessThe entire designing process can be subdivided in two steps which are described as followings.a)System Design Process :In order to make a project which can be applicable for real world situation, we are required to do reallybrainstorming. First thing of discussion are that how many signature image signals are taken as an input signal for generalizing the features of that sample signature for our study and also it is important to decide that in which format the images should be taken? Here we have collected 10 samples of signatures of each person.b)Program Design :In our program we have made software using MATLAB programming for analysis of Devnagari handwritten signature using moment invariant analysis. The first step of the process was the acquisition of the features of the particular signature sample, then after according to the available features a threshold value is obtained and it is compared with the database of sample signatures. After comparison the signature sample is identified as genuine or unauthorized one.II.METHODOLOGYThis section describes the methodology behind the system development. It discusses the pre-processing performed, the signature database, and the NN features. Signature Recognition Systems need to preprocess the data [4] [5]. It includes a series of operations to get the results. The major steps are as follows -A.Data Acquisition :For recognition purpose at the first stage the signatures are required to be processed by the system. Therefore they should be in the digital image format. We need to obtain the instant sample signature image from the signer for the verification purpose. For this purpose devices such as digital tablets can be used shown in Fig. 2. Data acquisition process is a process where the real time inputs of signature from the digitizing tablet and the special pen are read into the CPU for processing and to store the signature in to the database. The digitizing tablet is sending the real time inputs to the CPU for further processing and storage. B.Signature Pre-processing :The signature samples are required to be normalized and resized up to proper dimensions. Then after the background noise is removed and thinning of the signature sample is performed. This yields a signature template which can be used for extracting the features. Therefore minimal signature pre-processing is required.C.Feature Extraction :The features extracted from signatures or handwriting play a vital role in the success of any feature based HSV system. If a poorly constructed feature set is used with little insight into the writer’s natural style, then no amount of modeling or analysis is going to result in a successful system. Further, it is necessary to have multiple, meaningful features in the input vector to guarantee useful learning by the NN. In this paper we have considered the most important feature under our consideration for the process of signature verification, is Hu’s Moment Invariants.Fig. 2 : Digital TabletD.Hu’s Moment Invari ants :In image processing, computer vision and related fields image moments are very useful for image analysis. Hu derived these expressions from algebraic invariants applied to the moment generating function under a rotation transformation [6]. They consist of groups of nonlinear centralized moment expressions. The result is a set of absolute orthogonal (i.e. rotation) moment invariants, which can be used for scale, position, and rotation invariant pattern identification. Simple properties of images which are found via image moments include area, total intensity, centroid and information about its orientation. They are computed from normalized centralized moments up to order three I n and n th Hu invariant moment. Here mean values of xand y are represented by equation 2.1 and 2.2,(2.1)(2.2) The central moments can be represented by followingequation 2.3 and 2.4,=(2.3)(2.4)If f(x,y) is a digital image, then the previous equationbecomes,(2.5)The central moments of order up to 3 are:(2.6)(2.7)(2.8)(2.9)(2.10)+2(2.11)+2(2.12)(2.13)(2.14)Central moments are translational invariant. Information about image orientation can be derived by first using the second order central moments to construct a covariance matrix. Rotational invariants moment equations are given as below :(2.15)(2.16)(2.17)It is possible to calculate moments which are invariant under translation, changes in scale, and also rotation. Most frequently used are the Hu set of invariant moments are :M 1 = η20+ η02, (2.18) M 2 = (η20 – η02)2 + 4η211, (2.19) M 3 = (η30 – 3η12)2 + (3η21 – η03)2 , (2.20) M 4 = (η30 + η12)2 + (η21 + η03)2 , (2.21)M 5 = (η30 – 3η12) (η12 + η30) [(η12 + η30)2 – 3(η21 + η03)2] + (3η21 – η03) (η21 + η03) [3(η30 + η12)2 – (η21 + η03)2] , (2.22) M 6 = (η20 – η02) [(η30 + η12)2 – (η21 + η03)2] + 4η11(η30 + η12) (η21 + η03) , (2.23) M 7 = (3η21 – η03) (η30 + η12) [(η12 + η30)2 – 3(η21 + η03)2] + (3η12 – η30) (η21 + η03) [3(η30 + η12)2 – (η21 + η03)2] , (2.24) The first one, M 1, is analogous to the moment of inertia around the image's centroid, where the pixels' intensities are analogous to physical density. The last one, M 7, is skew invariant, which enables it to distinguish mirror images of otherwise identical images. A general theory on deriving complete and independent sets of rotation invariant moments was proposed by J. Flusser [7] and T. Suk [8]. They showed that the traditional Hu's invariant set is neither independent nor complete. M 2 and M 3 are not very useful for pattern recognition, as they are dependent. On the original Hu's set of moment invariants there is a missing third order independent moment invariant which given by following equation:M 8 = η11 [(η30 – η12)2 – (η03 + η21)2] – (η20 – η02) (η30 + η12) (η03 + η21) (2.25) E. Performance Parameters :For ensuring good performance for signature recognition system few parameters are required to be set. Such performance parameters for our Devnagari handwritten signature recognition system are discussed below :a) False Accept Rate or False Match Rate (FAR or FMR) :The false acceptance rate is given by the number of fake signatures accepted by the system with respect to the total number of comparisons made. It is the probability that the system incorrectly matches the input pattern to a non-matching template in the database. It measures the percent of invalid inputs which are incorrectly accepted.b) False Reject Rate or False Non-Match Rate (FRR or FNMR) :The false rejection rate is the total number of genuine signatures rejected by the system with respect to the total number of comparisons made. Both FAR and FRR depend on the threshold variance parameter taken to decide the genuineness of an image. If we choose a high threshold variance then the FRR is reduced, but at the same time the FAR also increases. If we choose a low threshold variance then the FAR is reduced, but at the same time the FRR also increases. The FRR is the measurement of the probability that a biometric system will fail to identify an individual who is properly enrolled. It measures the percent of valid inputs which are incorrectly rejected .c)Receiver Operating Characteristic or Relative Operating Characteristic (ROC) :The ROC plot is a visual characterization of the trade-off between the FAR and the FRR. In general, the matching algorithm performs a decision based on a threshold which determines how close to a template the input needs to be for it to be considered a match. If the threshold is reduced, there will be less false non-matches but more false accepts. Correspondingly, a higher threshold will reduce the FAR but increase the FRR. This more linear graph illuminates the differences for higher performances.d) 2.5.4 Equal Error Rate or Crossover Error Rate (EER or CER) :The rates at which both accept and reject errors are equal. The value of the EER can be easily obtained from the ROC curve. The EER is a quick way to compare the accuracy of devices with different ROC curves. In general, the device with the lowest EER is most accurate.III.EXPERIMENTAL S ET - U PWe have been concerned here with evaluating moment invariants as a feature space in a pattern recognition problem, predicting how they will behave given the classes and noise conditions of the problem. For this purpose, we accumulated enough background to help understand the nature of moment invariants and derived basic performance results in terms of the classes and noise.A.Training and TestingA total of 500 genuine signatures were collected from a population of 50 human subjects which included 22 women and 28 men. Out of which seven of them are left handed writers. Our main task is to recognizing the signature with good feature recognition techniques which provide good results on the signature recognition dataset. We train the system using a training set of signature obtained from a person. Designing of a classifier is a separate area of research. The decision thresholds required for the classification are calculated by considering the variation of features among the training set. Separate set of thresholds (user Specific) is calculated for each person enrolled, some system also use common threshold form all users.For training and testing general fuzzy minimum maximum (FMM) neural network is used in our work. Fuzzy networks are useful for clustering (unsupervised) and classification (supervised) problems. Application of FMM is an attempt to improve classification performance of the recognition system. By using FMM training the total time required to train the database was achieved less than 173.77 µs and the time taken to recognize a signature sample was less than 125.67 ms. B.Reason for using Hu’s Moment InvariantsThis work is based on analysis of values of Hu’s seven moment invariants. They consist of groups of nonlinear centralized moment expressions. The result is a set of absolute orthogonal (i.e. rotation) moment invariants, which can be used for scale, position, and rotation invariant pattern identification. These can be efficiently used in a simple pattern recognition application for successfully identifying various patterns [9]. The first one, M1, is analogous to the moment of inertia around the image's centroid, where the pixels' intensities are analogous to physical density. The last one, M7, is skew invariant, which enables it to distinguish mirror images of otherwise identical images. We have done analysis of fluctuation in moment invariant values of the same image for different positions. We took the original test image, scaled by one half of the test image and mirrored test image. For analyzing rotation effect we have taken rotated test image by 450, 900 and 1800. These images are shown in Fig. 3 under (a) Original Test Image, (b) Scaled by one half, (c) Rotated by 450, (d)Rotated by 900, (e) Rotated by 1800,(f) Mirrored Image.Fig. 3 : Different images for analysisThe moment values were observed for these six differently scaled and rotated images of the same signature image. The experimental values for these six images are shown in Table 3.1.Table 3.1 : Closeness of Moment Invariants values for Different ImagesWe found the closeness of the values of the moments, which is independent of translation, scale change, mirroring and rotation (as shown in Table 3.1). There is only difference in sign of M 7, which is for the mirrored image. This property can be used to detect whether the image is a mirrored image. This was the reason behind using Hu’s moment invariants for this work. C. Results and DiscussionsAfter process of training the signature database we started testing each sample signature. On basis ofrecognition and rejection the experimental results regarding FRR, FAR and AER are shown in Table 3.2. These results show that with the increase of similarity false reject rate (FRR) the false acceptance rate (FAR) is decreased. The results of our examination show that in this method, thebest value for the percentage of signature similarity is nearly 31%. In this point we obtain the minimum error rate (MinErrRate = min (FAR, FRR)). If we consider Average Error Rate (AER) are as following:AER = (FAR + FRR) / 2 (3.1)Table 3.2 : Variation of FAR and FRR with the threshold VarianceAverage Error Rate (AER) will be the smallest amount in SS ∈ [25, 35]. On the other hand, we have the best performance of the system in (25% ≤ SS ≤ 35%). In this interval, we have the minimum value forAverage Error Rate (AER) (shown in Fig. 4).Fig. 4 : Performance of HDSR systemThe minimum value of Average Error Rate (AER) for our work is achieved to be 33.0 %. Where the value of the FAR and the FRR meet one another, the point iscalled Equal Error Rate (EER) as it is shown in Fig. 5.Fig. 5 : Signature Similarity PercentageAs a matter of fact, getting the best performance, we should consider 25% ≤ SS ≤ 35%. The fundamental result of this study is obtaining the average of minimum errors not in the maximum surface similarity. In other words, if the correctness of a signature is its high similarity to the original one, the correct signatures will be rejected because of minor differences and this trend will decrease the efficiency of the system. The method is tested using genuine and forgery signature producedby 50 different persons. The highest accuracy rate achieved by our Devnagari handwritten signature recognition system was 96.12 %.The proposed algorithm can be used as an effective signature verification system. The algorithm proposed was successfully made rotation invariant by the rotation of the image. The Equal Error Rate (EER) was found to be 31%. It is the point where the value of the FAR and the FRR meet one another (shown in Fig. 5). The Equal Error Rate (EER) can further be improved by using better techniques for rotation, blurring and thinning. Using these algorithm random and simple forgeries can be easily detected. A great number of skilled forgeries can also be removed. It uses a compact and memory efficient storage of feature points which reduces memory overhead and results in faster comparisons of the data to be verified.The overall experimental results are categorized in Table 3.3. However it is not sufficient to verify the validity of a signature only by comparing the physical image of it.Table 3.3 : Overall Experimental ResultsIV. C ONCLUSIONRecognition of devnagari handwritten signature is a complex problem, which is not easily solvable. This work has presented a review of moment-based invariant functions, their history, basic principles, and methods how to construct them. We demonstrated that invariant functional can be used in image analysis as features for description and recognition of objects in degraded images. Invariant-based approach is a significant step towards robust and reliable object recognition methods. It has a deep practical impact because many pattern recognition problems would not be solvable otherwise. In practice, image acquisition is always degraded by unrecoverable errors and the knowledge of invariants with respect to these errors is a crucial point. This observation should influence future research directions and should be also incorporated in the education. To decrease the fluctuation of moment invariants, the image spatial resolution must be higher than the threshold of scaling and rotation. However, the resolution cannot be too high, because the computation will remarkablyincrease as the resolution increases. Therefore, the choice of resolution must balance computation and resolution on the real application. This approach can be used in multilingual character recognition as well. The computation increases quickly as resolution increases. From the experimental studies, we find that the choice of image spatial resolution is very important to keep invariant features. To decrease the fluctuation of moment invariants, the image spatial resolution must be higher than the threshold of scaling and rotation. However, the resolution cannot be too high, because the computation will remarkably increase as the resolution increases. Therefore, the choice of resolution must balance computation and resolution on the real application.Scope of Future Work : The concepts of Neural Networks as well as Hu’s moment invariants hold a lot of promise in building systems with high accuracy. It can be extended for the recognition of words, sentence and documents. Another research interest will be on the character images degraded or blurred by various reasons. This approach can be used in multilingual character recognition as well.REFERENCES[1] Brijmohan Singh, Ankush Mittal and DebashisGhosh, “An Evaluation of Different Feature Extractors and Classifiers for Offline Handwritten Devnagari Character Recognition”, Journal of Pattern Recognition Research, pp. 269-277, 2011. [2] Soumen Bag and Gaurav Harit, “TopographicFeature Extraction For Bengali And Hindi Character Images”, International Journal of Signal & Image Processing, Vol. 2, No. 2, pp. 181-196, June 2011. [3] Bhupendra M. Chaudhari, Abhay B. Nehete,Kantilal P. Rane and Ulhas B. Shinde, “Efficient Feature Extraction Technique for Signature Recognition ”, International Journal of Advanced Engineering & Application (IJAEA), pp. 64-70, January 2011. [4] Nan Xu, Li Cheng, Yan Guo, Xiaogang Wu andJiali Zhao, “A Method for Online Signature Verification Based on Neural Network ”, IEEE Trans. of 3rd International Conference on Communication Software and Networks (ICCSN), Wuhan, China, pp. 357-360, May 2011. [5] Juan Hu and Youbin Chen, “Writer IndependentOffline Handwritten Signature Verification based on Real Adaboost ”, IEEE Trans. of 2nd International Conference on Artificial Intelligence, Management Science and Electronic Commerce (AIMSEC), pp. 6095-6098, August 2011. [6] M. K. Hu, "Visual Pattern Recognition by MomentInvariants", IRE Trans. of Information Theory, Vol. 8, pp. 179-187, 1962.[7] J. Flusser, "On the Independence of RotationMoment Invariants", Journal of Pattern Recognition, Vol. 33, pp. 1405-1410, 2000. [8] J. Flusser and T. Suk, "Rotation Moment Invariantsfor Recognition of Symmetric Objects", IEEE Trans. of Image Processing, Vol. 15, pp. 3784-3790, 2006. [9] R. C. Gonzalez and R. E. Woods, “Digital ImageProcessing”, 3rd Edition, Prentice Hall of India, New Delhi, India, 2009.AUTHOR’S PROFILE:Shailendra Kumar Dewangan received B.E. in Electronics & Telecommunication in year 2005 and M.E. in Communication Engineering from Shri Shankaracharya College of Engineering & Technology (SSCET),Bhilai, Chhattisgarh, India. He is currently working as an Assistant Professor in the Department of Electronics & Instrumentation Engineering at Chhatrapati Shivaji Institute of Technology (CSIT), Durg, Chhattisgarh, India. His areas of interest include Digital Signal Processing, information security, digital watermarking, advancements in communication technology, etc. He has published multiple articles in various International and National Journals & Conferences. Besides he has lifetime membership of Indian Society of Technical Education (ISTE) and Associate membership of Institute of Electronics & Telecommunication Engineers (IETE).。
基于人工神经网络的手写字符识别技术研究
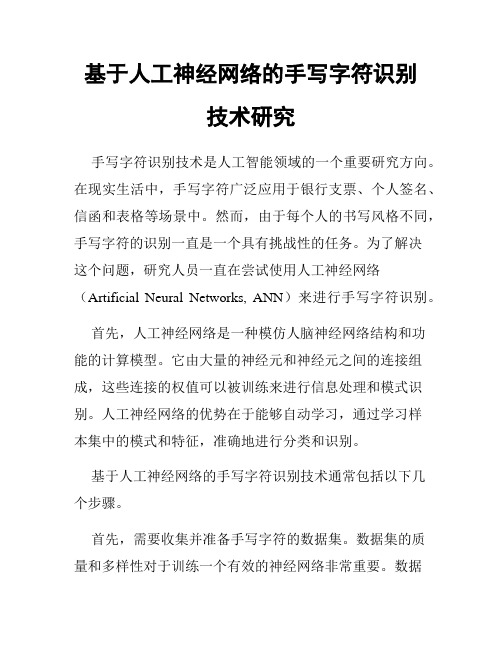
基于人工神经网络的手写字符识别技术研究手写字符识别技术是人工智能领域的一个重要研究方向。
在现实生活中,手写字符广泛应用于银行支票、个人签名、信函和表格等场景中。
然而,由于每个人的书写风格不同,手写字符的识别一直是一个具有挑战性的任务。
为了解决这个问题,研究人员一直在尝试使用人工神经网络(Artificial Neural Networks, ANN)来进行手写字符识别。
首先,人工神经网络是一种模仿人脑神经网络结构和功能的计算模型。
它由大量的神经元和神经元之间的连接组成,这些连接的权值可以被训练来进行信息处理和模式识别。
人工神经网络的优势在于能够自动学习,通过学习样本集中的模式和特征,准确地进行分类和识别。
基于人工神经网络的手写字符识别技术通常包括以下几个步骤。
首先,需要收集并准备手写字符的数据集。
数据集的质量和多样性对于训练一个有效的神经网络非常重要。
数据集可以包含多种不同的手写字符样本,包括不同字体、大小和笔画变化的字符。
此外,还需要为每个字符样本标注标签,以便进行训练和评估。
接下来,需要对数据集进行预处理。
预处理的目标是将手写字符的图像转换为神经网络可以理解和处理的数字表示。
常见的预处理步骤包括灰度化、二值化和图像大小归一化。
灰度化将彩色图像转换为灰度图像,简化了后续的处理过程。
二值化将灰度图像转换为黑白图像,将字符与背景分离。
图像大小归一化可以将所有字符样本调整为相同的大小,减少样本间的尺度差异。
然后,需要设计一个合适的神经网络结构。
神经网络的结构包括输入层、隐藏层和输出层。
输入层接受预处理后的手写字符图像作为输入,隐藏层用于处理图像的特征和模式,输出层用于对字符进行分类。
常用的神经网络模型包括多层感知机(Multi-Layer Perceptron, MLP)和卷积神经网络(Convolutional Neural Network, CNN)。
选择合适的神经网络模型和参数是手写字符识别技术中的关键一步。
教你如何使用AI技术进行手写识别

教你如何使用AI技术进行手写识别如何使用AI技术进行手写识别手写识别是一项基于人工智能技术的重要应用,它可以将手写文字转化为电子文本,提供了便捷高效的数据处理方式。
本文将为您介绍如何使用AI技术来实现手写识别,并探讨其在各个领域中的应用。
一、背景介绍手写识别是一项复杂而富有挑战性的任务。
传统的方法依赖于特征提取和机器学习算法,但面对不同的书写风格和字体,其准确率较低。
然而,随着深度学习与人工智能的发展,利用神经网络模型进行手写识别成为了新的解决方案。
二、数据预处理在进行手写识别之前,首先需要对原始手写图像进行预处理。
这包括图像去噪、二值化、缩放等步骤。
去噪过程可以通过滤波算法(如中值滤波)来消除图像中的噪声干扰;二值化可以将灰度图像转化为黑白图像,方便后续处理;缩放则是将图像统一调整到合适大小,保证数据输入模型的一致性。
三、建立神经网络模型利用神经网络技术进行手写识别是目前最为先进的方法之一。
常用的网络结构包括卷积神经网络(CNN)和循环神经网络(RNN)。
其中CNN适合处理图像识别问题,而RNN则更适合处理序列数据,如文本。
可以根据实际需求选择适合的网络结构。
四、训练模型在建立好神经网络模型后,需要使用大量的手写样本对其进行训练。
训练数据集应尽可能覆盖不同字体和书写风格,并标注对应的标签。
通过反向传播算法和梯度下降优化算法,不断调整神经网络中各个参数,使其能够对手写文字进行准确分类。
五、模型评估与优化在完成训练后,需要对模型进行评估以验证其性能表现。
可以使用测试数据集来计算准确率、精确率、召回率等指标。
如果发现模型存在欠拟合或过拟合等问题,可以通过调整学习率、增加样本数量等方法进行优化。
六、应用场景手写识别技术在多个领域有着广泛的应用。
例如,在邮政领域中,手写识别技术可以实现地址识别和邮件分拣;在银行领域中,可以用于支票自动处理和签名验证;在教育领域中,可以实现批改试卷和纠正学生书写等。
随着人工智能技术的不断进步,手写识别将有更广阔的应用前景。
基于深度学习的手写体识别技术
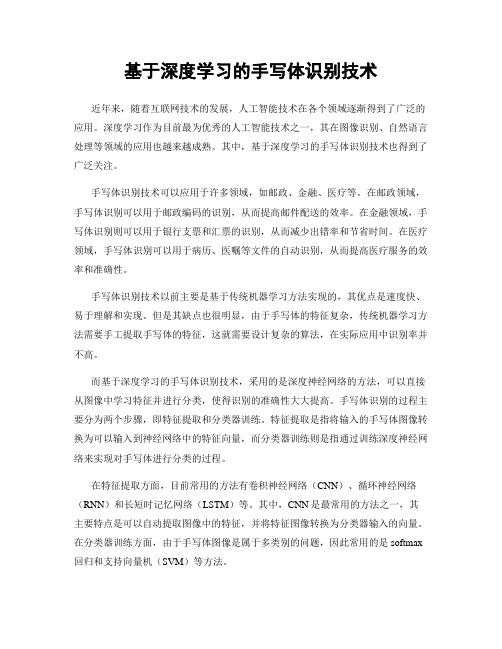
基于深度学习的手写体识别技术近年来,随着互联网技术的发展,人工智能技术在各个领域逐渐得到了广泛的应用。
深度学习作为目前最为优秀的人工智能技术之一,其在图像识别、自然语言处理等领域的应用也越来越成熟。
其中,基于深度学习的手写体识别技术也得到了广泛关注。
手写体识别技术可以应用于许多领域,如邮政、金融、医疗等。
在邮政领域,手写体识别可以用于邮政编码的识别,从而提高邮件配送的效率。
在金融领域,手写体识别则可以用于银行支票和汇票的识别,从而减少出错率和节省时间。
在医疗领域,手写体识别可以用于病历、医嘱等文件的自动识别,从而提高医疗服务的效率和准确性。
手写体识别技术以前主要是基于传统机器学习方法实现的,其优点是速度快、易于理解和实现。
但是其缺点也很明显,由于手写体的特征复杂,传统机器学习方法需要手工提取手写体的特征,这就需要设计复杂的算法,在实际应用中识别率并不高。
而基于深度学习的手写体识别技术,采用的是深度神经网络的方法,可以直接从图像中学习特征并进行分类,使得识别的准确性大大提高。
手写体识别的过程主要分为两个步骤,即特征提取和分类器训练。
特征提取是指将输入的手写体图像转换为可以输入到神经网络中的特征向量,而分类器训练则是指通过训练深度神经网络来实现对手写体进行分类的过程。
在特征提取方面,目前常用的方法有卷积神经网络(CNN)、循环神经网络(RNN)和长短时记忆网络(LSTM)等。
其中,CNN是最常用的方法之一,其主要特点是可以自动提取图像中的特征,并将特征图像转换为分类器输入的向量。
在分类器训练方面,由于手写体图像是属于多类别的问题,因此常用的是softmax 回归和支持向量机(SVM)等方法。
目前,基于深度学习的手写体识别在各个领域都有了广泛的应用。
以邮件配送为例,深度学习技术可以通过对邮件上的地址进行文字识别,从而实现邮递员派送邮件的自动化。
在医疗领域,手写体识别技术可以通过对医生手写的医嘱、病历等文件进行识别,从而降低医疗服务的人工成本。
如何使用AI技术进行手写识别与识别

如何使用AI技术进行手写识别与识别一、手写识别的概述手写识别是指通过人工智能技术对手写文字进行自动识别和转换成可编辑文本的过程。
随着科技的不断进步,人工智能技术在手写识别领域取得了显著突破,并广泛应用于各个领域,如自动化办公、数字化档案管理和智能手机等。
二、AI技术在手写识别中的应用1. 基于图像处理的手写识别图像处理是手写识别中常用且基础的技术。
该方法通过扫描或拍摄手写文字图片,然后使用图像处理算法提取字符特征,再通过训练模型进行分类和预测来实现手写识别任务。
其中,常见的图像处理算法包括边缘检测、二值化和形态学变换等。
2. 基于机器学习的手写识别机器学习在手写识别中起到关键作用。
它利用大量标注好的手写字符数据进行训练,并提取数据中的特征,建立模型来预测新输入样本所属类别。
支持向量机(SVM)、神经网络和决策树等机器学习算法广泛应用于手写识别领域,并取得了很好的效果。
3. 基于深度学习的手写识别深度学习是当今手写识别领域最热门的技术。
通过建立深层神经网络模型,它可以自动地从原始数据中进行特征提取和表征学习。
在手写识别中,卷积神经网络(CNN)是一种常用的深度学习技术。
它能够有效地处理图像和序列数据,大幅提升手写字符的准确性。
三、使用AI技术进行手写识别的步骤1. 数据准备在进行手写识别之前,首先需要收集大量标注好的手写字符数据集。
这个数据集应具有多样性和代表性,包括不同人群、不同书写风格和各种书写质量等。
同时,还需要对数据集进行预处理、归一化以及分割等操作,以便更好地输入到训练模型中。
2. 模型训练根据所选择的机器学习或深度学习算法,利用准备好的数据集开始进行模型训练。
在训练过程中,应选择合适的损失函数和优化算法,并根据模型在验证集上的表现进行调参,以提升模型的性能指标。
可以使用开源工具如TensorFlow或PyTorch来实现模型训练。
3. 模型评估与优化在完成模型训练后,需要对训练好的模型进行评估。
基于深度学习的手写字符识别算法实现
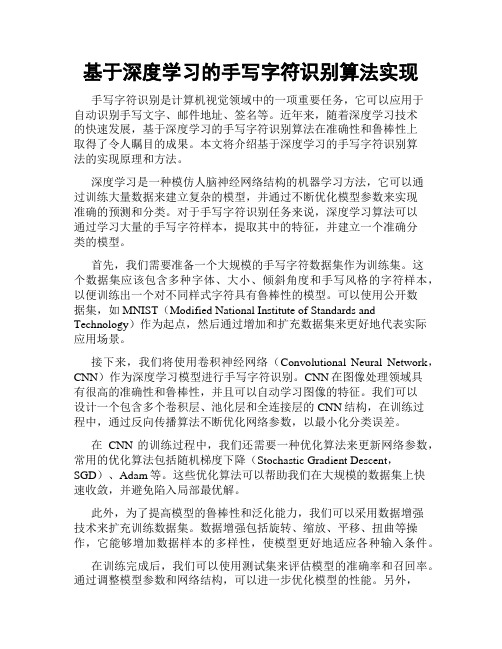
基于深度学习的手写字符识别算法实现手写字符识别是计算机视觉领域中的一项重要任务,它可以应用于自动识别手写文字、邮件地址、签名等。
近年来,随着深度学习技术的快速发展,基于深度学习的手写字符识别算法在准确性和鲁棒性上取得了令人瞩目的成果。
本文将介绍基于深度学习的手写字符识别算法的实现原理和方法。
深度学习是一种模仿人脑神经网络结构的机器学习方法,它可以通过训练大量数据来建立复杂的模型,并通过不断优化模型参数来实现准确的预测和分类。
对于手写字符识别任务来说,深度学习算法可以通过学习大量的手写字符样本,提取其中的特征,并建立一个准确分类的模型。
首先,我们需要准备一个大规模的手写字符数据集作为训练集。
这个数据集应该包含多种字体、大小、倾斜角度和手写风格的字符样本,以便训练出一个对不同样式字符具有鲁棒性的模型。
可以使用公开数据集,如MNIST(Modified National Institute of Standards and Technology)作为起点,然后通过增加和扩充数据集来更好地代表实际应用场景。
接下来,我们将使用卷积神经网络(Convolutional Neural Network,CNN)作为深度学习模型进行手写字符识别。
CNN在图像处理领域具有很高的准确性和鲁棒性,并且可以自动学习图像的特征。
我们可以设计一个包含多个卷积层、池化层和全连接层的CNN结构,在训练过程中,通过反向传播算法不断优化网络参数,以最小化分类误差。
在CNN的训练过程中,我们还需要一种优化算法来更新网络参数,常用的优化算法包括随机梯度下降(Stochastic Gradient Descent,SGD)、Adam等。
这些优化算法可以帮助我们在大规模的数据集上快速收敛,并避免陷入局部最优解。
此外,为了提高模型的鲁棒性和泛化能力,我们可以采用数据增强技术来扩充训练数据集。
数据增强包括旋转、缩放、平移、扭曲等操作,它能够增加数据样本的多样性,使模型更好地适应各种输入条件。
如何应用AI技术进行手写体识别
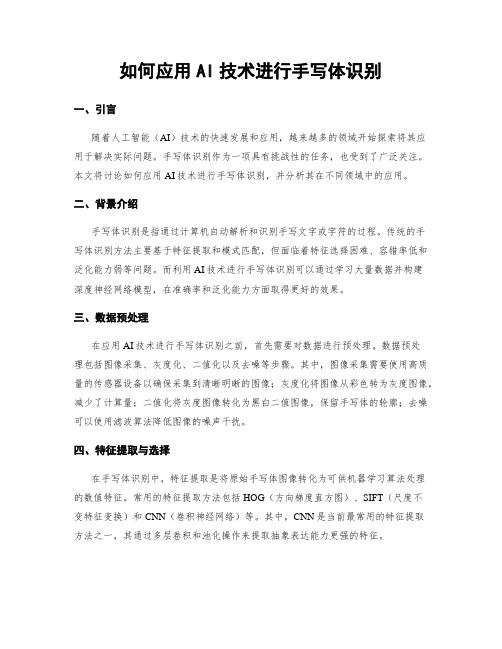
如何应用AI技术进行手写体识别一、引言随着人工智能(AI)技术的快速发展和应用,越来越多的领域开始探索将其应用于解决实际问题。
手写体识别作为一项具有挑战性的任务,也受到了广泛关注。
本文将讨论如何应用AI技术进行手写体识别,并分析其在不同领域中的应用。
二、背景介绍手写体识别是指通过计算机自动解析和识别手写文字或字符的过程。
传统的手写体识别方法主要基于特征提取和模式匹配,但面临着特征选择困难、容错率低和泛化能力弱等问题。
而利用AI技术进行手写体识别可以通过学习大量数据并构建深度神经网络模型,在准确率和泛化能力方面取得更好的效果。
三、数据预处理在应用AI技术进行手写体识别之前,首先需要对数据进行预处理。
数据预处理包括图像采集、灰度化、二值化以及去噪等步骤。
其中,图像采集需要使用高质量的传感器设备以确保采集到清晰明晰的图像;灰度化将图像从彩色转为灰度图像,减少了计算量;二值化将灰度图像转化为黑白二值图像,保留手写体的轮廓;去噪可以使用滤波算法降低图像的噪声干扰。
四、特征提取与选择在手写体识别中,特征提取是将原始手写体图像转化为可供机器学习算法处理的数值特征。
常用的特征提取方法包括HOG(方向梯度直方图)、SIFT(尺度不变特征变换)和CNN(卷积神经网络)等。
其中,CNN是当前最常用的特征提取方法之一,其通过多层卷积和池化操作来提取抽象表达能力更强的特征。
在特征选择阶段,可以使用PCA(主成分分析)等方法对提取到的特征进行降维处理,以剔除冗余信息并减少计算复杂性。
合理选择并优化特征提取和选择方法对于建立高效精确的手写体识别模型非常关键。
五、模型构建与训练利用AI技术进行手写体识别通常需要构建深度学习模型,并通过大量数据进行训练。
深度学习模型可以利用卷积神经网络(CNN)、循环神经网络(RNN)或者注意力机制等多种结构。
在构建模型时,需要根据具体任务的需求进行选择和优化。
模型训练阶段包括确定损失函数、选择合适的优化算法以及设置超参数等过程。
如何利用AI技术进行手写字符识别
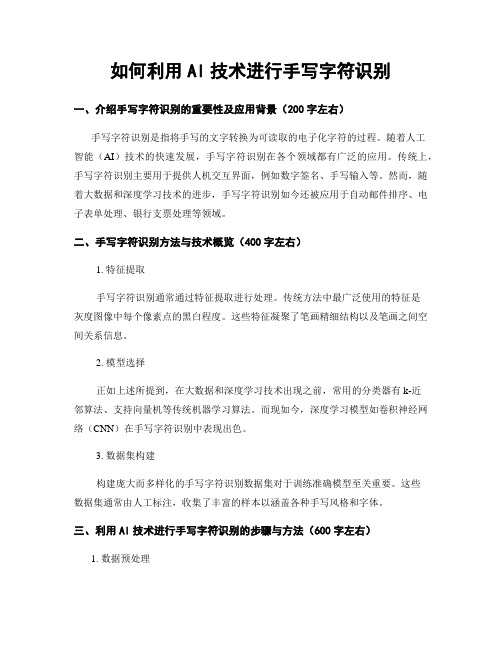
如何利用AI技术进行手写字符识别一、介绍手写字符识别的重要性及应用背景(200字左右)手写字符识别是指将手写的文字转换为可读取的电子化字符的过程。
随着人工智能(AI)技术的快速发展,手写字符识别在各个领域都有广泛的应用。
传统上,手写字符识别主要用于提供人机交互界面,例如数字签名、手写输入等。
然而,随着大数据和深度学习技术的进步,手写字符识别如今还被应用于自动邮件排序、电子表单处理、银行支票处理等领域。
二、手写字符识别方法与技术概览(400字左右)1. 特征提取手写字符识别通常通过特征提取进行处理。
传统方法中最广泛使用的特征是灰度图像中每个像素点的黑白程度。
这些特征凝聚了笔画精细结构以及笔画之间空间关系信息。
2. 模型选择正如上述所提到,在大数据和深度学习技术出现之前,常用的分类器有k-近邻算法、支持向量机等传统机器学习算法。
而现如今,深度学习模型如卷积神经网络(CNN)在手写字符识别中表现出色。
3. 数据集构建构建庞大而多样化的手写字符识别数据集对于训练准确模型至关重要。
这些数据集通常由人工标注,收集了丰富的样本以涵盖各种手写风格和字体。
三、利用AI技术进行手写字符识别的步骤与方法(600字左右)1. 数据预处理AI技术进行手写字符识别之前需要对原始图像进行预处理,以便提高模型的准确性和稳定性。
其中一些常见的预处理包括:- 去噪:通过滤波算法去除图像中的噪声,使得图像更加清晰;- 归一化:将图像尺寸统一到固定大小,以便后续处理;- 二值化:将彩色图像转换为黑白图像,方便特征提取。
2. 特征提取与选择特征提取是训练模型前的重要步骤。
对于传统机器学习方法,常用的特征是基于每个像素点的灰度信息。
然而,在深度学习中,可以使用卷积神经网络(CNN)直接从原始图像中学习特征。
CNN具有自动提取图像中局部和全局特征的能力,能够更好地捕捉手写字体的信息。
3. 模型训练与优化通过将预处理后的数据输入到选定的模型中,进行模型训练。
基于人工智能技术的手写字符识别研究
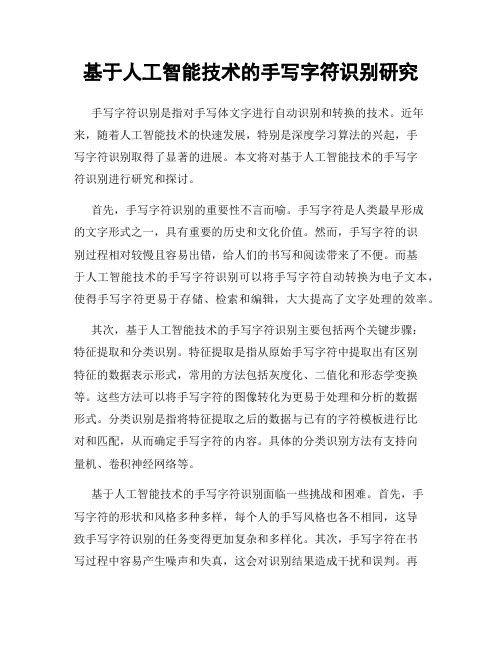
基于人工智能技术的手写字符识别研究手写字符识别是指对手写体文字进行自动识别和转换的技术。
近年来,随着人工智能技术的快速发展,特别是深度学习算法的兴起,手写字符识别取得了显著的进展。
本文将对基于人工智能技术的手写字符识别进行研究和探讨。
首先,手写字符识别的重要性不言而喻。
手写字符是人类最早形成的文字形式之一,具有重要的历史和文化价值。
然而,手写字符的识别过程相对较慢且容易出错,给人们的书写和阅读带来了不便。
而基于人工智能技术的手写字符识别可以将手写字符自动转换为电子文本,使得手写字符更易于存储、检索和编辑,大大提高了文字处理的效率。
其次,基于人工智能技术的手写字符识别主要包括两个关键步骤:特征提取和分类识别。
特征提取是指从原始手写字符中提取出有区别特征的数据表示形式,常用的方法包括灰度化、二值化和形态学变换等。
这些方法可以将手写字符的图像转化为更易于处理和分析的数据形式。
分类识别是指将特征提取之后的数据与已有的字符模板进行比对和匹配,从而确定手写字符的内容。
具体的分类识别方法有支持向量机、卷积神经网络等。
基于人工智能技术的手写字符识别面临一些挑战和困难。
首先,手写字符的形状和风格多种多样,每个人的手写风格也各不相同,这导致手写字符识别的任务变得更加复杂和多样化。
其次,手写字符在书写过程中容易产生噪声和失真,这会对识别结果造成干扰和误判。
再者,手写字符的多变性和多样性使得训练样本的获取和标注变得困难,需要大量的数据集和专业人员的标注工作。
为了解决这些问题,研究者们提出了一系列的解决方案和创新方法。
一方面,他们通过深度学习算法,如卷积神经网络和循环神经网络,提高了手写字符识别的准确率和鲁棒性。
这些算法可以通过学习大量的手写字符样本,自动提取出关键特征,从而实现更精确的分类和识别。
另一方面,他们通过数据增强技术和强化学习方法,扩充了手写字符数据集的规模,并提高了模型的泛化能力。
这些方法可以有效地解决数据不足和样本负载不均衡的问题。
如何利用深度学习技术进行手写体识别
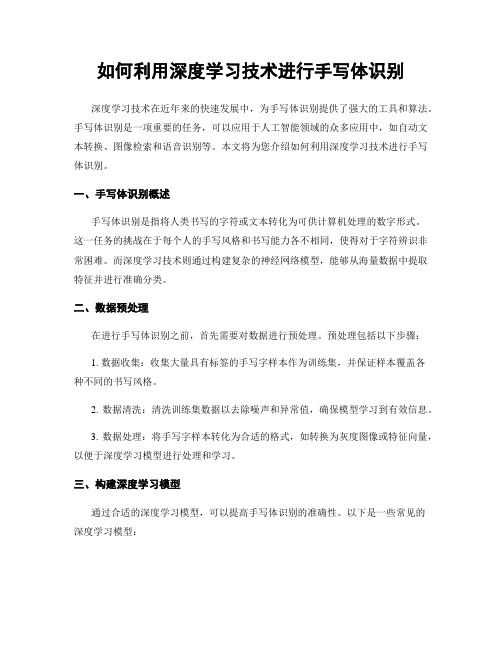
如何利用深度学习技术进行手写体识别深度学习技术在近年来的快速发展中,为手写体识别提供了强大的工具和算法。
手写体识别是一项重要的任务,可以应用于人工智能领域的众多应用中,如自动文本转换、图像检索和语音识别等。
本文将为您介绍如何利用深度学习技术进行手写体识别。
一、手写体识别概述手写体识别是指将人类书写的字符或文本转化为可供计算机处理的数字形式。
这一任务的挑战在于每个人的手写风格和书写能力各不相同,使得对于字符辨识非常困难。
而深度学习技术则通过构建复杂的神经网络模型,能够从海量数据中提取特征并进行准确分类。
二、数据预处理在进行手写体识别之前,首先需要对数据进行预处理。
预处理包括以下步骤:1. 数据收集:收集大量具有标签的手写字样本作为训练集,并保证样本覆盖各种不同的书写风格。
2. 数据清洗:清洗训练集数据以去除噪声和异常值,确保模型学习到有效信息。
3. 数据处理:将手写字样本转化为合适的格式,如转换为灰度图像或特征向量,以便于深度学习模型进行处理和学习。
三、构建深度学习模型通过合适的深度学习模型,可以提高手写体识别的准确性。
以下是一些常见的深度学习模型:1. 卷积神经网络(CNN):由于图像具有空间结构的特点,CNN在图像分类任务中表现出色。
对于手写体识别,可以使用卷积层和池化层来提取特征,并通过全连接层将提取到的特征映射到类别上。
2. 循环神经网络(RNN):RNN主要用于序列数据的处理,在手写体识别中可用于连续字符之间的预测。
通过将时间步骤作为输入,并在隐藏状态中保存先前时刻的信息,RNN可以捕捉到字符间的上下文关系。
3. 长短时记忆网络(LSTM):LSTM是一种RNN变体, 它能够更好地捕捉到长期依赖关系。
在手写体识别中, LSTM可以帮助模型理解笔画之间存在复杂关联性, 提供更高水平的准确性。
四、模型训练与优化在构建好深度学习模型之后,需要进行数据集的划分,并进行模型的训练和优化。
1. 数据集划分:将手写体数据集划分为训练集、验证集和测试集。
基于人工免疫模型的在线手写签名认证方法
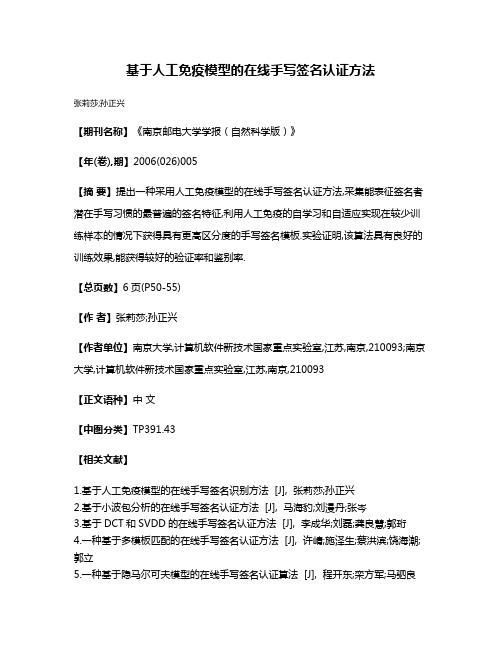
基于人工免疫模型的在线手写签名认证方法
张莉莎;孙正兴
【期刊名称】《南京邮电大学学报(自然科学版)》
【年(卷),期】2006(026)005
【摘要】提出一种采用人工免疫模型的在线手写签名认证方法,采集能表征签名者潜在手写习惯的最普遍的签名特征,利用人工免疫的自学习和自适应实现在较少训练样本的情况下获得具有更高区分度的手写签名模板.实验证明,该算法具有良好的训练效果,能获得较好的验证率和鉴别率.
【总页数】6页(P50-55)
【作者】张莉莎;孙正兴
【作者单位】南京大学,计算机软件新技术国家重点实验室,江苏,南京,210093;南京大学,计算机软件新技术国家重点实验室,江苏,南京,210093
【正文语种】中文
【中图分类】TP391.43
【相关文献】
1.基于人工免疫模型的在线手写签名识别方法 [J], 张莉莎;孙正兴
2.基于小波包分析的在线手写签名认证方法 [J], 马海豹;刘漫丹;张岑
3.基于DCT和SVDD的在线手写签名认证方法 [J], 李成华;刘磊;龚良慧;郭珩
4.一种基于多模板匹配的在线手写签名认证方法 [J], 许崝;施泽生;蔡洪滨;饶海潮;郭立
5.一种基于隐马尔可夫模型的在线手写签名认证算法 [J], 程开东;栾方军;马驷良
因版权原因,仅展示原文概要,查看原文内容请购买。
- 1、下载文档前请自行甄别文档内容的完整性,平台不提供额外的编辑、内容补充、找答案等附加服务。
- 2、"仅部分预览"的文档,不可在线预览部分如存在完整性等问题,可反馈申请退款(可完整预览的文档不适用该条件!)。
- 3、如文档侵犯您的权益,请联系客服反馈,我们会尽快为您处理(人工客服工作时间:9:00-18:30)。
第19卷第3期2007年3月计算机辅助设计与图形学学报JOURNAL OF COMPU TER 2AIDED DESIGN &COMPU TER GRAPHICSVol 119,No 13Mar 1,2007收稿日期:2006-06-08;修回日期:2006-11-101基金项目:国家自然科学基金(69903006,60373065);教育部“新世纪优秀人才资助计划”(NCET 20420460)1张莉莎,女,1979年生,博士研究生,主要研究方向为生物计算技术、智能人机交互1孙正兴,男,1964年生,博士,教授,博士生导师,主要研究方向为智能人机交互、多媒体计算、计算机视觉1基于人工免疫模型的在线手写签名识别方法张莉莎 孙正兴(南京大学计算机软件新技术国家重点实验室 南京 210093)(szx @nju 1edu 1cn )摘要 采集能表征签名者潜在手写习惯的签名特征,利用人工免疫模型的自学习和自适应实现在较少训练样本的条件下获得具有更高区分度的手写签名模板1实验结果表明,文中方法识别具有良好的训练效果,能获得较好的验证率和鉴别率1关键词 在线手写签名识别;特征选择;样本训练;人工免疫模型;抗原;抗体中图法分类号 TP39113Online Signature R ecognition Using Artif icial Immune ModelZhang Lisha Sun Zhengxing(State Key L aboratory f or Novel Sof t w are Technology ,N anji ng U niversity ,N anji ng 210093)Abstract This paper presents an approach for online signature recognition which extracts the most com 2monly used signature features ,and utilizes the self 2learning and self 2adaptation of artificial immune theory to obtain new models with higher distinguishability when the training samples are limited 1Experiments show that this approach performs well in sample training and results in satisfactory verification rate and identifica 2tion rate 1K ey w ords online hand 2written signature recognition ;feature selection ;sample training ;artificial immune model ;antigen ;antibody 手写签名识别以其自然流畅的书写方式[1]成为身份验证中的一种常用技术1相比离线签名识别[1],在线签名识别[2]直接利用用户输入笔迹的几何、物理和时序等多种线索来捕捉用户的签名习惯和区分用户签名的差异,具有较高的正确性和安全性,成为手写签名识别领域的主流技术1已有对在线手写签名识别的研究主要包括两大方面:特征选择和样本训练1一方面,有效的签名特征表示是手写签名识别的基础1Seiichiro 等[3]采用数据手套搜集签名者手部18个主要关节的动作信号进行分析和特征选择;J ulian 等[4]对手写签名中的100种全局特征进行了实验分析,验证了如签名持续时间、提笔次数、签名速率的变化等动态信息具有较强的区分用户的能力;Christian 等提出采用多功能数字化笔[5]记录手写过程中笔的三维压力和倾斜角度,通过粗糙集分析和特征筛选得到50维特征向量表示用户的签名模板,以再现良好的书写风格1国内一些研究人员在研究手写签名识别时也对特征选择和抽取问题做了相应的分析[627],这些研究为在线签名识别的有效特征表示提供了有力依据1但如何能既发挥多种签名特征的效用,又保证特征表示的实用性仍是在线签名识别需要进一步探索的重要课题1另一方面,手写签名样本的训练直接关系到签名模板的代表性和合理性1已有在线手写签名识别研究的样本训练主要采用统计方法和机器学习方法:动态时间牵引算法已在在线签名识别中得到广泛的应用[8];Stephan 等[9]提出的将先验形状知识和结构模板相结合的ASSM (active sketch shape model )可变形状模板统计识别方法,在手绘草图识别和手写符号鉴别上有良好效果;机器学习方法中以支撑向量机(support vector machine,SVM)和隐马尔可夫模型(hidden Markov model,HMM)应用较为广泛[10212]1然而,这些方法都存在训练样本需求量较大的问题1从方法的原理来看,SVM是为区分2类问题而设计的,其扩展到多类问题的能力有限; HMM方法基于状态转换概率的思想,更适合于描述时序性强的问题;从实验结果来看,ASSM可变形状模板描述模型的能力很强,弹性调整算法的识别率较高,但实验中错误率仍较高[9]1研究人员为了达到较高的识别率,往往要求采集较大数量的训练样本,由于手写签名识别是面向数量庞大的多用户的,在实际应用中要求众多签名者主动、耐心地提供大量训练样本是不现实的1因而,较少训练样本条件下获得具有较高代表性和区分度的样本训练方法是在线手写签名识别需要进一步研究的问题1人工免疫系统对病毒的识别和学习机制已成功地应用于模式识别领域[13216],其对细胞的选择、克隆、变异等操作又可以自动生成大量相似的细胞1本文提出一种基于人工免疫模型的在线手写签名识别方法,该方法采用普通输入方式(鼠标或手写笔)所能获取的签名特征,利用免疫的自学习和自适应等计算能力来解决在线手写签名识别中的2个问题:将免疫系统自动生成与抗体相近的细胞的能力应用到手写签名识别中,令其自主生成与签名者所提供的初始签名样本相似的训练样本,从而达到对初始训练样本需求量较小的目的;利用克隆选择原理中对抗原识别度越高的免疫细胞越有可能被选择参与繁殖过程和自动生成新的细胞的能力,使免疫算法更具针对性和完备性,以解决庞大用户群引起签名模板区分度小的问题1实验结果表明,本文算法虽仍未达到比其他算法更优的验证率和鉴别率,但对样本训练有良好的改进效果,可以在少量训练样本的情况下达到与多样本情况相仿的识别效果11 基于人工免疫模型的手写签名识别机理根据手写签名识别存在的问题,本文提出了一种如图1所示手写签名识别系统的框架1除了传统手写签名识别系统中的样本数据获取、预处理、特征抽取和特征匹配,该框架包括另外2个重要的模块: 1)离线训练模块1通过人工免疫算法对签名样本的特征表示进行克隆、变异等操作,生成更多与原始样本相似、与签名特征匹配的样本作为用户签名模板,在小样本训练条件下生成符合用户签名特征的模板;2)在线自适应模块1在结果评价时添加新的有代表性的签名模板,同时淘汰过时的、与签名者当前签名习惯不一致的模板,使模板库与签名者细微的习惯变化保持同步1本文的研究重点是对签名进行特征选择,并进行基于人工免疫模型的样本训练1在此训练方式下,特征匹配部分也以人工免疫模型为基础,从而形成基于人工免疫模型的签名识别方式1图1 基于人工免疫模型的手写签名识别系统框架图111 手写签名特征选择手写签名中能描述用户签名习惯的特征很多,在实际应用中首先应考虑采用普遍性高、一般设备就能获取的、可以良好表达签名习惯的特征,包括笔画曲率、笔尖压力、速率等,而上述对手指关节信息感应的数据手套[3]则属于比较复杂和昂贵的信息获取设备;其次,基于特征集选择的冗余度和多元预测[17]问题,我们所选择的特征及特征组合应具有较小的相关性和良好的用户区分性,即特征集所表达信息的冗余度尽可能小,但能够区分不同签名者的签名,达到较高的鉴别率和验证率1本文在B样条曲线拟合、误差计算及采用动态规划算法对签名笔画进行分段的基础上,首先抽取样本的原始几何特征和动态特征,如x坐标值、y坐标值、压力、速率、笔画持续时间、签名持续时间,再与模板进行差值计算,获得10个间接特征,最后对这16个特征(如表1所示)进行用户区分性计算1实验结果表明,所采用的特征具有较好的用户区分性1112 基于人工免疫模型的样本训练同一用户的签名样本之间的差别比较小,对签名求得的均值样本在一定程度上代表了用户的签名习惯1然而能采集的样本毕竟是有限的,实际应用中也不可能对单个用户搜集大量签名,模型训练的目的是为了自动产生更多与样本数据相似、反映签名者手写特点的模板1基于人工免疫原理的样本训213计算机辅助设计与图形学学报2007年练中,本文采用克隆选择方法,其基本思想是只有那些能识别抗原的细胞才会被繁殖,描述了当B细胞识别非自体抗原模式时免疫应答的形成过程以及该免疫应答的基本特征,其维持抗体类型多样性的性质是模式识别的基础1算法中一些相关的基本概念在手写签名的训练和识别过程的定义如表2所示1表1 签名特征的符号和描述特征类型特征符号特征描述直接特征几何特征x特征点的x轴坐标值y特征点的y轴坐标值动态特征p特征点的笔尖压力值v特征点的笔尖速率值st r dr各笔画的持续时间sig2dr签名样本的整体持续时间间接特征几何特征x2mean各笔画x坐标轴上的平均值与模板对应笔画的该平均值的差值x2dev各笔画x坐标轴上的标准差值与模板对应笔画的该标准差值的差值y2mean各笔画y坐标轴上的平均值与模板对应笔画的该平均值的差值y2dev各笔画y坐标轴上的标准差值与模板对应笔画的该标准差值的差值动态特征p2mean各笔画笔尖压力的平均值与模板对应笔画的该平均值的差值p2dev各笔画笔尖压力的标准差值与模板对应笔画的该标准差值的差值v2mean各笔画笔尖速率的平均值与模板对应笔画的该平均值的差值v2dev各笔画笔尖速率的标准差值与模板对应笔画的该标准差值的差值st r2duration各笔画持续时间与模板对应笔画的该时间值的差值sig2duration签名样本的整体持续时间与模板的整体持续时间的差值表2 克隆选择算法中的基本概念模型概念训练识别抗原分子用户提呈的手写签名训练样本用户提呈的手写签名待测样本抗体分子经历克隆、变异后与原始样本较相似的个体训练后得到的所有签名模板记忆细胞能够匹配抗原的抗体分子多次匹配待测样本的签名模板亲和力 原始训练样本与新个体的匹配程度待测样本与签名模板的匹配程度克隆操作复制亲和力符合要求的抗体变异操作对抗体的特征位随机进行突变2 人工免疫算法基于人工免疫模型的手写签名样本训练是为不同的签名建立相应类别的细胞群体,所有签名样本都表示成包含几何特征和动态特征的免疫细胞个体,通过离线的个体训练和在线的个体识别检验用户签名的合法性1211 个体定义和笔画调整设签名个体的表示形式为IN D ij={S k}(1≤i≤N sig,1≤j≤N sample,1≤k≤N s)1其中,N sig为签名者个数,N sample为每个签名者所提供的签名样本数,N s为签名所含笔画数,IN D ij为第i个签名者的第j个样本,该样本包含N s个笔画1签名中第k个笔画表示为S k=(G k,D k),G k为该笔画的几何特征,D k为该笔画的动态特征,分别定义如下:G k=(x k,y k,x2mean k,x2dev k,y2mean k,y2dev k), D k=(p k,v k,st roke2dr k,sig2dr k,p2mean k,p2dev k,v2mean k,v2dev k,st roke2duration k)1 采用B样条曲线拟合签名个体IN D ij,一个包括N s个笔画的个体被拟合成N s条B样条曲线,每一笔画对应一条B样条曲线1但存在以下问题:1)不同签名中汉字所包含的笔画个数不一样;2)汉字中有些笔画转折较大,如 、 等,而且人们在书写汉字时常常出现多个笔画(指汉字书写规则中定义的规范笔画)的连笔,其衔接处有时连贯,有时突兀,都是因人而异的;3)即使同一用户书写同一签名的多个样本,依然会存在细微的差异,这种差异可能导致笔画分割时产生不同的结果1因此,直接使用固定维数的特征向量表示不同类别手写签名的几何性质是很困难的1本文采用基于阈值的动态规划方法(threshold based dynamic programming,TBDP)调整特征向量维度,在获得一个合适的笔画组合序列时会受到笔画长度阈值和B样条曲线拟合误差的约束,但由于2个相似的签名样本在同样的笔画分割3133期张莉莎等:基于人工免疫模型的在线手写签名识别方法算法下得到的笔画数较为接近、笔画形状也相似,因此可以预定义一个阈值,当满足认证样本中某个笔画的长度认证样本笔画的总长度- 签名模板中对应笔画的长度签名模板笔画的总长度<阈值,说明当前笔画组合序列是合适的;反之,则是不可取的1从而使得任意2个个体的特征维度一致,再进行亲和力计算1这样可以事先排除一些不可能的笔画组合序列,提高个体匹配时的效率1212 样本训练本文的样本训练方法采用克隆选择算法,以用户提呈的训练样本作为初始训练群体,通过迭代地选择、克隆、变异,生成更多与原始群体相似、具有用户签名特征的抗体集合,并将符合亲和力要求的抗体作为记忆细胞,如图2所示1定义细胞群体M i(1≤i ≤N sig )为第i 个用户的签名样本集合,M m i 为M i 的模板细胞,即M i 中与所有其他免疫细胞的亲和力最大的记忆细胞;M =∑N sigMi为全体签名者的记忆细胞集合1图4 鉴别的识别流程示意图2 基于人工免疫模型的样本训练流程示意算法分为8个步骤,循环执行Step3~Step8,直至满足收敛条件为止1Step11生成初始记忆细胞集合(M ),它由用户的手写签名训练样本组成,M =M 1+…+M N sig ,计算每个子集M i 的模板细胞M m i 1Step21随机生成r 个新的未标记细胞群体P r 1Step31生成候选方案的一个集合P ,它由记忆细胞集合M 和新的细胞群体P r 组成,即P =M +P r 1Step41选择h 个具有高亲和力的个体(h 视用户数N sig和每个用户样本数N sample 而定)1Step51克隆这h 个最好的个体,组成一个临时的克隆群体C 1与抗原亲和力越高,个体在克隆时的规模也就越大1Step61把克隆群体提交到高频变异,根据亲和力的大小决定变异1产生一个成熟的抗体群体C 31Step71对C 3进行再选择:a 1将标记个体i 与M m i 进行亲和力计算,亲和力大于阈值t 1的保留;b 1将未标记个体与M m i (1≤i ≤N sig )计算亲和力,若存在亲和力大于阈值t 1,则将其标记为亲和力最大的模板细胞的标记1选中的个体加入对应的记忆细胞集合M i 1Step81生成r ′个新的个体取代P 中t 个低亲和力的个体,保持多样性1213 签名识别签名识别分为2种:1)验证1鉴定某个手写签名是否所宣称签名者的真实签名1提呈一个宣称为某签名者S ig i 的签名样本,计算这个样本和记忆细胞集合M 中标记为签名者S ig i 的所有记忆细胞的亲和力1如果存在一定比例p 的记忆细胞亲和力高于阈值t ,那么提呈样本就是该签名者的真实签名;否则,为假冒签名1图3 验证的识别流程示意2)鉴别1识别某个手写签名属于哪一位签名者,其算法流程如图4所示1提呈一个未知的签名样本,计算这个样本和记忆细胞集合M 中所有记忆细胞的亲和力1判别方法有2种:a 1选择具有最高亲和力且亲和力高于阈值t 的记忆细胞的标记作413计算机辅助设计与图形学学报2007年为该签名样本所属的签名者标记;b 1选择具有最高亲和力的记忆细胞所在的集合M i 的模板细胞M m i ,计算其与签名样本的亲和力,若高于阈值t ,那么该样本属于该签名者13 实验设计和结果分析311 样本收集我们收集了15个用户的签名,采集的实验样本分为2部分:1)真实签名1每个用户在一个连续的时间内提供50个手写签名,并均分为5组;2)仿冒签名1每个用户仿效另外特定5人(平均分配)的签名习惯(主要是签名的几何形状特征),在熟练后各假冒10个签名,即每个用户提交10×5=50个假冒的签名,每个特定的签名有10×5=50个相应的假冒签名,并均分为5组1312 人工免疫训练实验图5 训练模板与未训练模板的识别结果比较31211 免疫算法的训练能力为了验证经过免疫算法训练的模板库比未经过训练的模板库有更好的识别效果,我们建立3个签名模板库:将原始样本中的第1组样本,第1,2组样本分别作为模板库M a 和M b ,原始样本的第1组样本经过人工免疫算法训练,生产新的模板库M c ,然后用模板库M a ,M b 和M c 分别识别某类别签名的同一组真实签名样本,观察识别结果1部分实验结果如图5所示,纵坐标表示测试样本与模板中所有记忆细胞的亲和力1从图5中可以看出:1)模板M b 对应的亲和力明显高于模板M a ;每一类签名中,经过训练的模板M c 对应的亲和力几乎都高于未经训练的模板M a ,在某些样本上甚至高于模板M b 的亲和力1第4类签名中,经过训练的模板M c 对应的亲和力明显高于未经训练的2组模板12)未经训练的样本亲和力波动幅度较大,而训练模板的折线段相对平稳1由此可知,人工免疫训练具有以下优势:1)使一些与原始样本亲和力较低的样本与模板整体的亲和力得到提高;2)减少了训练所需的样本数,使得在只提供少量签名样本的情况下仍能达到与大量签名样本相仿、甚至更为理想的识别效果131212 算法稳定性和多样性为了测试算法在训练签名样本方面的稳定性和多样性,进行如下2个子实验1实验11对相同的训练样本进行多次训练,比较所得签名模板对测试样本的识别效果1图6所示为对某用户的2组训练样本分别进行3次训练得到3组不同的模板,再对另一组测试样本的识别结果的比较1实验表明,相同的样本经过人工免疫算法的训练,保留了样本所包含的签名者内在习惯特征,生成的模板库基本相似,但又存在一定的差异性,使其更好地适应用户签名固有的、细微的变化1图6 训练次数对识别结果的影响比较实验21对同一签名者的不同训练样本进行训练,比较所得签名模板对测试样本的识别效果1本文对所有签名者的各4组签名样本分别训练得到相应的模板,再用第1组签名样本和另一类别的签名样本分别测试1图7所示为某类签名者的实验结果,4条折线段都比较接近1实验表明,经过5133期张莉莎等:基于人工免疫模型的在线手写签名识别方法人工免疫算法的训练,即使2组原始样本之间存在差异,但训练所得的记忆细胞仍比较相似,对识别结果的影响很小1图7 训练样本与测试样本类别关系识别结果比较实验1,2说明,采用人工免疫算法对样本进行训练,在可以较好地保留原有样本的特征的同时;对原有个体的克隆、变异和一定条件下随机生成新个体等固有机制,能获得与原有个体十分相近的新个体,弥补了少量样本未能充分反映签名者书写习惯的缺陷,从而在只提供了少量训练样本的情况下也能获得反映签名者潜在书写习惯的、更加丰富和完善的签名模板1313 实验结果分析1)验证实验选取10个用户的签名进行验证1对于每个用户类别,我们选择真实签名中第1组样本进行训练获得签名模板,再验证其余40个真实签名和其中40个仿冒签名1实验结果如表3所示,显示了真实签名的识别率和错误拒绝率、仿冒签名的判别率和错误接受率1由表3可以看出,真实签名的识别率几乎都在70%,仿冒签名的判别率几乎都在85%,且两者大致是反相关的1识别率越高的签名类别,其判别率普遍较低;反之,亦然1这种现象并非由于当前采用的特征对真实和仿冒签名本身不可分,主要原因在于:采用人工免疫算法训练样本后,接受或拒绝某个签名的阈值设置得不够理想,不足以达到最优区分真实签名和仿冒签名的目的1表3 10个类别签名的验证结果%类别识别率错误拒绝率判别率错误接受率class 110010008510015100class 29715021508715012150class 39010010100971502150class 47215027150951005100class 510010008215017150class 675100251007215027150class 760100401001001000class 890100101008715012150class 970100301001001000class 109010010100100100 2)鉴别实验采用14个用户的签名进行鉴别1选用真实签名中第1组样本进行训练,用第2,3组共20个真实签名与所有类别的模板比较,进行鉴别,实验结果如表4所示1由表4可以看出,14个签名者中,几乎所有签名者的鉴别率都超过80%(包括80%),2Π3的签名者的鉴别率超过90%(包括90%)1表3 14个类别签名的鉴别结果签名者鉴别样本数鉴别率Π%签名者鉴别样本数鉴别率Π%sig 101890100sig 171995100sig 111680100sig 1820100100sig 121890100sig 191995100sig 131785100sig 11020100100sig 1420100100sig 1111890100sig 1520100100sig 1121785100sig 161785100sig 11319951004 结 论针对目前手写签名识别中模板区分度不高和训练样本数量较大的问题,本文采用签名中一些常用的、易采集的几何特征和表征签名者潜在手写习惯的动态特征,在对其进行特征相关性和用户区分性分析的基础上,构造基于人工免疫模型的样本训练方法,对多个签名者有限的签名样本进行训练,使其613计算机辅助设计与图形学学报2007年在训练样本较少的情况下获得具有良好区分度的手写签名模板,再对不同签名者的样本进行验证和鉴别1实验结果表明,采用人工免疫算法进行样本训练具有良好的自学习和自适应能力,能在样本有限的情况下模拟出代表用户签名习惯的新样本,所组成的签名模板与原始样本既相似又有差别;在保留用户签名习惯特征的基础上又获得了因原始样本数量有限而未能显示的特征信息,从而使得其识别效果优于未经训练的原始模板库,并达到较好的识别率1这也说明,人工免疫算法在在线手写签名识别中具有良好的应用前景1参 考 文 献[1]Plamondon Rejean,Srihari Sargur N1On2line and off2line hand2writing recognition:a comprehensive survey[J]1IEEE Trans2actions on Pattern Analysis and Machine Intelligence,2000,22(1):63-84[2]Y an Wan,Zheng Jianbin,Zhou Li,et al1Overview and fore2cast of personal identification based on on2Line handwritten sig2nature verification[J]1Journal of Application of ElectronicTechnique,2004,30(9):1-3(in Chinese)(颜 琬,郑建彬,周 莉,等1基于在线手写签名的身份认证技术研究和展望[J]1电子技术应用,2004,30(9):1-3) [3]Hangai Seiichiro,Higuchi Takeshi1Writer Identification usingfinger2bend in writing signature[C]ΠΠProceedings of BiometricAuthentication Workshop,Prague,2004:229-237[4]J ulian Fierrez2Aguilar,Loris Nanni,Jaime Lopez2Penalba,etal1An on2line signature verification system based on fusion of lo2cal and global information[C]ΠΠProceedings of Audio2andVideo2based Biometric Person Authentication,New Y ork,LNCS3546,2005:523-532[5]Christan H,J uergen K,G eorg S1A novel digitizing pen for theanalysis of pen pressure and inclination in handwriting biometrics[C]ΠΠProceedings of Biometric Authentication Workshop,Prague,2004:283-294[6]Zuo Wenming,Li Shaofa,Zeng Xiangui1Analysis of texturefeatures of offline handwritten signatures[J]1Journal of Micro2electronics and Computer,2005,22(2):154-157(in Chinese)(左文明,黎绍发,曾宪贵1脱机手写签名纹理特征分析[J]1微电子学与计算机,2005,22(2):154-157)[7]Zhao Guimin,Xia Limin,Chen Aibin1Rapid verification forhandwriting signature[J]1Journal of Computer Engineer,2003,29(7):56-58(in Chinese)(赵桂敏,夏利民,陈爱斌1手写签名的快速认证[J]1计算机工程,2003,29(7):56-58)[8]Jain A K,Griess Friederike D,Connell Scott D1On2line signa2ture verification[J]1Pattern Recognition,2002,35(12):2963-2972[9]Stephan Al2Zubi,Arslan Broemme,K laus Toennies1Using anactive shape structural model for biometric sketch recognition[C]ΠΠProceedings of Deutsche Arbeitsgemeinschaft für Muster2erkennung(DA GM),Magdeburg,2003:187-195[10]J ustino Edson J R,Bortolozzi Flávio,Sabourin Robert1A com2parison of SVM and HMM classifiers in the off2line signatureverification[J]1Pattern Recognition Letters,2005,26(9):1377-1385[11]K ashi R S,Hu J,Nelson W L,et al1On2line handwritten sig2nature verification using hidden Markov model features[C]ΠΠProceedings of International Conference of Document Analysisand Recognition,Ulm,1997:253-257[12]Van B Ly,G arcia2Salicetti S,Dorizzi B1Fusion of HMM’slikelihood and Viterbi path for on2line signature verification[C]ΠΠProceedings of Biometric Authentication Workshop,Prague,2004:318-331[13]De Castro,von Zuben L N F1An evolutionary immune networkfor data clustering[C]ΠΠProceedings of the6th Brazilian Sympo2sium on Neural Networks,Rio de Janiero,2000:84-89 [14]Tarakanov A O,Skormin V A1Pattern recognition by immuno2computing[C]ΠΠProceedings of the Special Sessions on ArtificialImmune Systems in Congress on Evolutionary Computation,Honolulu,2002:938-943[15]White Jennifer A,G arrett Simon M1Improved pattern recogni2tion with artificial Clonal selection?[C]ΠΠProceedings of the2nd International Conference on Artificial Immune Systems(I2CARIS),Edinburgh,2003:181-193[16]Xiao Renbin1Mechanism path synthesis based on immune com2puting[J]1Journal of Computer2Aided Design&ComputerGraphics,2004,16(6):812-818(in Chinese)(肖人彬1基于免疫计算的机构轨迹综合[J]1计算机辅助设计与图形学学报,2004,16(6):812-818)[17]Dougherty E R1Feature2selection overfitting with small2sampleclassifier design[J]1IEEE Intelligent Systems Magazine,2005,20(6):64-667133期张莉莎等:基于人工免疫模型的在线手写签名识别方法。