Domain ontology construction from biomedical text. international conference on artificial i
生物工程专业英语作业

03
Identify different genetic traits that are inherited in Mendelian inheritance patterns Focus on the impact of genetic traits on human health and issues
Cell Division and Growth: Describe the process of cell division, including mitosis and cytokinesis Explain how cells grow and multiply
Fundamentals of Cell Biology
Identify the different types of cells in the human body and explain their functions Describe the organization of cells into issues and the types of issues found in the human body
Rapid development period
After the mid-20th century, with the development of disciplines such as molecular biology and cell biology, biotechnology entered a period of rapid development.
要点三
Fundamentals of Biochemistry
03
Biotechnology experimental technology
酶工程名词解释

酶工程:由酶学与化学工程技术、基因工程技术、微生物学技术相结合而产生的一门新的技术科学。
它利用酶的催化作用,在一定的生物反应器中,将相应的原料转化成所需的产品。
锁钥学说(酶的专一性):酶与底物分子或底物分子的一部分之间,在结构上有严格的互补关系诱导契合学说:酶分子的构象与底物原来并非恰当吻合,只有当底物分子与酶分子相互碰撞时,可诱导底物的构象发生变化,使其与底物配合,然后才结合形成中间络合物,进而引起底物分子发生相应的化学变化。
酶:由生物体细胞合成的具有选择性催化功能的生物大分子( 包括蛋白质和核酸)单纯酶(simple enzyme):仅由氨基酸残基构成的酶。
结合酶(全酶)(conjugated enzyme):由蛋白部分(酶蛋白apoenzyme)和非蛋白部分(辅助因子cofactor)组成辅酶(coenzyme):与酶蛋白结合疏松,可用透析或超滤的方法除去。
辅基(prosthetic group):与酶结合紧密,不能用透析或超滤的方法除去。
酶的活性中心:酶蛋白上只有少数氨基酸残基参与酶对底物的结合和催化,这些相关氨基酸残基在空间上比较靠近,形成一个与酶显示活性直接有关的区域,称为酶的活性中心。
必需基团:酶活性中心的一些化学基团为酶发挥催化作用所必须,这些基团若经化学修饰使其改变,则酶的活性丧失,称为必需基团。
接触残基(contact residues):和底物直接接触,参与底物的化学转变,是活性中心的重要组成部分。
辅助残基(auxiliary residues):使酶与底物相互结合,辅助接触残基。
结构残基(structural residues):维持蛋白酶形成一种有规则的空间构象非贡献残基(non-contributing residues):不参与酶的催化功能,对酶活性的显示不起作用结合基团:与底物结合的部位,决定酶的专一性;催化基团:促使底物发生化学变化的部位,决定反应的性质。
结构域:蛋白质肽链中一段较独立的具有完整、致密立体结构的区域。
生物技术-南华大学自然生物科技学系

1生物技術生物技術2什麼是生物技術?全世界擁有約一千個公司會員的生技產業組織(The Biotechnology Industry Organization;BIO) ,對於生物科技則有如下的定義:「the use of biological processes to solve problems or make useful products」利用各種與生物相關的步驟或程序,來解決問題生物技術3什麼是生物技術?西元1992年於巴西東南部的Rio de Janeiro城市所舉辦的聯合國生物多樣性會議中(UN Convention on Biological Diversity),對「on Biological Diversity),對Biotechnology」有如下的定義:「Biotechnology means any technological application that uses biological systems, living生物技術4生物技術5什麼是生物技術?生物技術6什麼是生物技術?生物技術7什麼是生物技術?生物技術8什麼是生物技術?生物技術9生物技術10生技產業定義生物技術產業和其他科技產業相比,有下列重要的特色:產品–開發期長、投資龐大、風險大。
惟開發成功後,報酬率高,且產品生命週期長。
–產品與生命健康有關,需要高度的品質與法規管制,且附加價值高。
–技術密集,屬整合性科技,需跨領域的專業人才。
生物技術11我國政府推動生物技術概況生技產業是21 世紀最具成長潛力的科技產業,各國皆將其列為首要發展項目,積極投入大量資源。
年代便將生技產業列為重點科技項目。
我國自1980年代便將生技產業列為重點科技項目–行政院核定「加強生物技術產業推動方案」(1995),作為推動我國生技產業發展之重要指導原則。
–兩兆雙星(Two Trillion & Twin Star, 2002)分為•兩兆產業:「半導體」與「影像顯示」生物技術12生物技術13生物技術14家畜飼養與農業的歷史生物技術的起源可回溯至數萬年前早期農耕社會收集野生植物的種子馴養野生動物保留具有所需飼養最有價值人生物技術15古代篩選的事實-玉米穗生物技術16生物技術17生物技術18採集者--Nikolai VavilovNikolai Vavilov則是二十世紀最具影響力的採集者之一,他對蘇聯以及50個以上的國家進行大規模的旅行收集。
药学导论全部习题详解

第一章1.名词解释:Drug/Medicine(药品):药品系指用于治疗、预防诊断人的疾病,有目的的调解人的生理功能并规定有适应症、功能主治和用法用量的物质Pharmacy/ Pharmaceutical Science (药学) 研究药物的一门学科,揭示药物与人或者药物与药物,或者药物与病原生物体相互作用的科学。
药学也是研究药物的(来源、曾分、性状)(作用机制、用途)(分析鉴定,加工生产,经营使用、以及管理)Pharmacist (药师)受过高等药学教育,活在医疗防御机构、药事机构或制药企业中从事药物制备、调剂。
检定和生产,并经卫生部审核通过的高级药学人才Licensed Pharmacist (执业药师)通过执业药师资格考试的药物工作者Clinical Pharmacist (临床药师)是以系统临床药学专业知识为基础,熟悉药物性能与应用,了解疾病治疗要求和特点,参与药物治疗方案制定、实施与评价的临床专业技术人员。
Drug therapy/Pharmacotherapy (药物治疗)应用药物对疾病进行治疗Rational administration of drug(合理用药)以安全有效经济适当为原则对药品的使用2.问答题:药品有哪些特殊性?既有效又有毒、具有特殊的用途、特殊时效性、特殊消费方式、特殊质量要求的学科。
(专属性、两重性、质量重要性、限时性)药学学科的任务?研制新药、生产供应药品、保证合理用药、科学监管药品、培养药师、药学科学家、企业家药学包含哪些二级学科?药物化学、生药学、药理学、药剂学、药物分析学、微生物与生物制药药学工作的意义?社会地位、经济地位、自然科学地位(p18) 合理用药的内涵(指标)?安全有效经济适当第二章药化复习思考题1. 名词解释:先导化合物:又称原型物是通过各种途径、方法、手段得到某种具有生物活性的化学结构2. 简述药物的分类化学合成药、天然药、生物技术药3. 药物化学的任务是什么?①研究药物的化学结构与理化性质的定性、定量关系籍以讨论其合成、结构改造及其稳定性,以及毒理作用、生物转化关系、生物效应,为临床提供理论依据。
Annotating genomes using ontologies
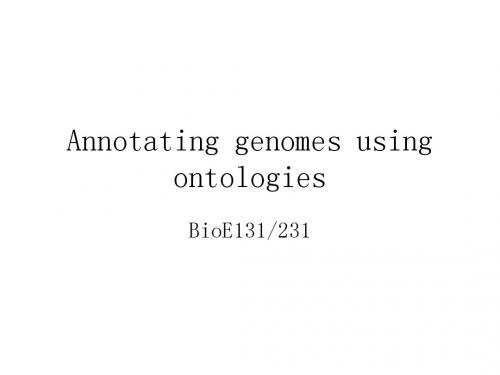
GPCR activity
receptor activity
is_a
Combining with an extracellular or intracellular messenger to initiate a change in cell activity
transmembrane receptor activity
Molecular Function
insulin binding GO:0005009 insulin receptor activity
Molecular Function
GO:0015238 drug transporter activity
Molecular Function
[Term] id: GO:0015238 name: drug transporter activity namespace: molecular_function def: "Enables the directed movement of a drug into, out of, within or between cells. A drug is any naturally occurring or synthetic substance, other than a nutrient, that, when administered or applied to an organism, affects the structure or functioning of the organism; in particular, any such substance used in the diagnosis, prevention, or treatment of disease." [ISBN:0198506732] is_a: GO:0005215 ! transporter activity
ontology介绍

Formal Ontology&informal Ontology
Formal ontology:
着重在子类型和上层类型的区分 用逻辑语言或计算机语言描述 公理化ontology由公理和定义陈述
•
用于数学、物理、工程
基于原型ontology由每个子类的典型成员或原型的 比较区分子类型
其他分类系统的索引
Ontology 环境
合并多个Ontology 诊断、升级Ontology
Chimaera Ontology 环境
Knowledge Systems Laboratory , Stanford University Web-based browser environment 接受15种指定格式的输入 提供潜在的合并候选项 支持分类法决议模式,及发现语义包含关 系功能 提供分析功能套件,包括不完全的测试、 分类学分析、语义检查 提供规则语言,以允许用户添加测试工具
文件:ontology
版本信息:
!version: $Revision: 1.48 $ !date: $Date: 2002/01/15 18:25:40 $ !source: $Source: /share/go/cvs/go/doc/GO.doc.html,v $ ! !Gene Ontology ![domain of file] !editors: Michael Ashburner (FlyBase), Midori Harris (GO), Judith Blake (MGD) !Leonore Reiser (TAIR), Karen Christie (SGD) and colleagues !with software by Suzanna Lewis (FlyBase Berkeley).
OntologyGeneOntologyKEGG
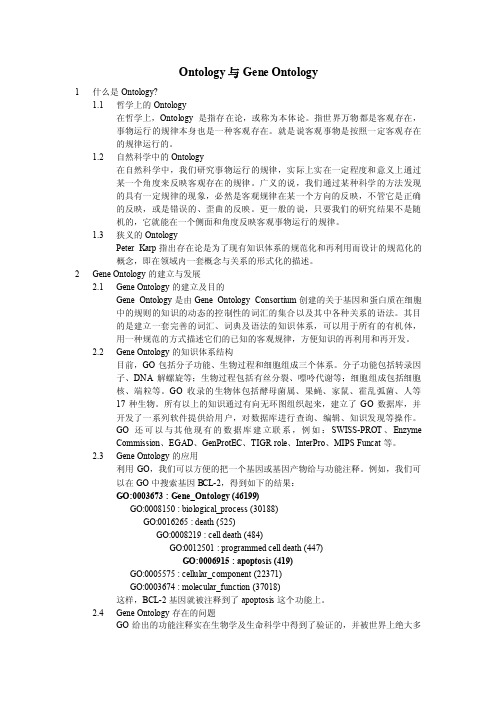
Ontology与Gene Ontology1什么是Ontology?1.1哲学上的Ontology在哲学上,Ontology是指存在论,或称为本体论。
指世界万物都是客观存在,事物运行的规律本身也是一种客观存在。
就是说客观事物是按照一定客观存在的规律运行的。
1.2自然科学中的Ontology在自然科学中,我们研究事物运行的规律,实际上实在一定程度和意义上通过某一个角度来反映客观存在的规律。
广义的说,我们通过某种科学的方法发现的具有一定规律的现象,必然是客观规律在某一个方向的反映,不管它是正确的反映,或是错误的、歪曲的反映。
更一般的说,只要我们的研究结果不是随机的,它就能在一个侧面和角度反映客观事物运行的规律。
1.3狭义的OntologyPeter Karp指出存在论是为了现有知识体系的规范化和再利用而设计的规范化的概念,即在领域内一套概念与关系的形式化的描述。
2Gene Ontology的建立与发展2.1Gene Ontology的建立及目的Gene Ontology是由Gene Ontology Consortium创建的关于基因和蛋白质在细胞中的规则的知识的动态的控制性的词汇的集合以及其中各种关系的语法。
其目的是建立一套完善的词汇、词典及语法的知识体系,可以用于所有的有机体,用一种规范的方式描述它们的已知的客观规律,方便知识的再利用和再开发。
2.2Gene Ontology的知识体系结构目前,GO包括分子功能、生物过程和细胞组成三个体系。
分子功能包括转录因子、DNA解螺旋等;生物过程包括有丝分裂、嘌呤代谢等;细胞组成包括细胞核、端粒等。
GO收录的生物体包括酵母菌属、果蝇、家鼠、霍乱弧菌、人等17种生物。
所有以上的知识通过有向无环图组织起来,建立了GO数据库,并开发了一系列软件提供给用户,对数据库进行查询、编辑、知识发现等操作。
GO还可以与其他现有的数据库建立联系,例如:SWISS-PROT、EnzymeCommission、EGAD、GenProtEC、TIGR role、InterPro、MIPS Funcat等。
植物内生真菌生防作用研究进展
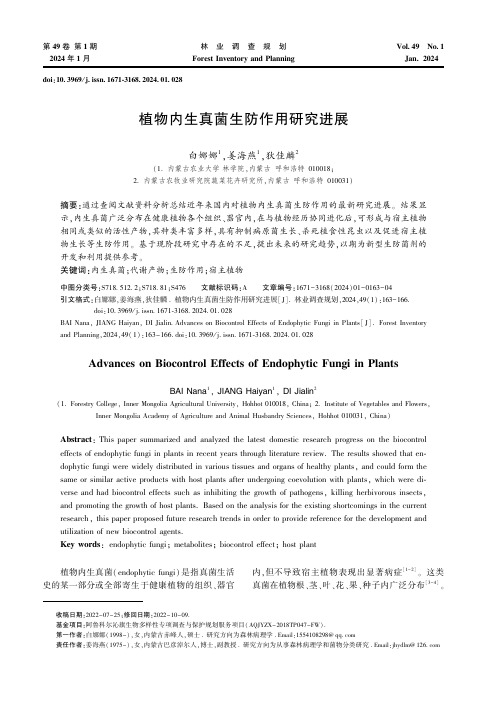
第49卷第1期 林 业 调 查 规 划Vol.49 No.1 doi:10.3969/j.issn.1671⁃3168.2024.01.028植物内生真菌生防作用研究进展白娜娜1,姜海燕1,狄佳麟2(1.内蒙古农业大学林学院,内蒙古呼和浩特010018;2.内蒙古农牧业研究院蔬菜花卉研究所,内蒙古呼和浩特010031)摘要:通过查阅文献资料分析总结近年来国内对植物内生真菌生防作用的最新研究进展。
结果显示,内生真菌广泛分布在健康植物各个组织、器官内,在与植物经历协同进化后,可形成与宿主植物相同或类似的活性产物,其种类丰富多样,具有抑制病原菌生长、杀死植食性昆虫以及促进宿主植物生长等生防作用。
基于现阶段研究中存在的不足,提出未来的研究趋势,以期为新型生防菌剂的开发和利用提供参考。
关键词:内生真菌;代谢产物;生防作用;宿主植物中图分类号:S718.512.2;S718.81;S476 文献标识码:A 文章编号:1671-3168(2024)01-0163-04引文格式:白娜娜,姜海燕,狄佳麟.植物内生真菌生防作用研究进展[J].林业调查规划,2024,49(1):163-166.doi:10.3969/j.issn.1671⁃3168.2024.01.028BAI Nana,JIANG Haiyan,DI Jialin.Advances on Biocontrol Effects of Endophytic Fungi in Plants[J].Forest Inventory and Planning,2024,49(1):163-166.doi:10.3969/j.issn.1671⁃3168.2024.01.028Advances on Biocontrol Effects of Endophytic Fungi in PlantsBAI Nana1,JIANG Haiyan1,DI Jialin2(1.Forestry College,Inner Mongolia Agricultural University,Hohhot010018,China;2.Institute of Vegetables and Flowers,Inner Mongolia Academy of Agriculture and Animal Husbandry Sciences,Hohhot010031,China) Abstract:This paper summarized and analyzed the latest domestic research progress on the biocontrol effects of endophytic fungi in plants in recent years through literature review.The results showed that en⁃dophytic fungi were widely distributed in various tissues and organs of healthy plants,and could form the same or similar active products with host plants after undergoing coevolution with plants,which were di⁃verse and had biocontrol effects such as inhibiting the growth of pathogens,killing herbivorous insects, and promoting the growth of host plants.Based on the analysis for the existing shortcomings in the current research,this paper proposed future research trends in order to provide reference for the development and utilization of new biocontrol agents.Key words:endophytic fungi;metabolites;biocontrol effect;host plant 植物内生真菌(endophytic fungi)是指真菌生活史的某一部分或全部寄生于健康植物的组织、器官内,但不导致宿主植物表现出显著病症[1-2]。
第一课外源基因在大肠杆菌中的表达原理及微量移液器的使用演示文档
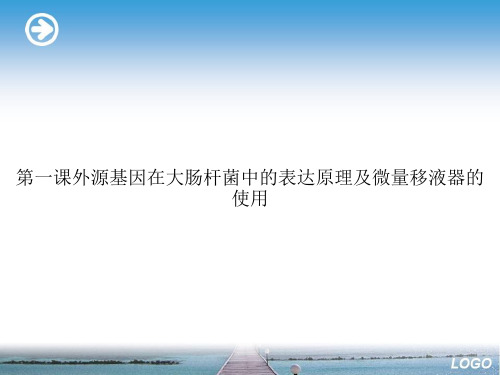
利用大肠杆菌进行外源基因表 达的过程
4. 鉴定目的基因表达产物 ① 抗体鉴定 ② 生物活性鉴定 ③ 序列测定
载体,通过感染或转染进入宿主细胞表达目 的蛋白。 • 优势:能够指导蛋白质的正确折叠,提供复 杂的糖基化等多种翻译后加工功能,因而表 达产物在分子结构、理化特性和生物学功能 方面最接近于天然的哺乳动物蛋白质分子。 • 缺点:表达效率通常不高。
利用大肠杆菌进行外源基因表 达的过程
1. 构建表达载体 • 在大肠杆菌里表达外源基因一般是始于将基因插入
Johns Hopkins University School of Medicine Baltimore, USA
1931 -
质粒的结构:
1. 抗性基因(Antibiotic resistance gene,such as Ampicillin resistance gene, Kanamycine resistance gene)
Werner Arber
Biozentrum der Universität Switzerland 1929 -
Daniel Nathans
Johns Hopkins University School of Medicine Baltimore, USA
1928 - 1999
Hamilton O. Smith
利用大肠杆菌进行外源基因表 达的过程
1. 构建表达载体(质粒) ① 制备感受态大肠杆菌 ② 将质粒DNA转化感受态大肠杆菌 ③ 提取质粒DNA ④ 质粒DNA定量
利用大肠杆菌进行外源基因表 达的过程
1. 构建表达载体 ⑤ 扩增目的基因 • PCR方法:以含目的基因的克隆质粒为模板,
对器官生成的展望英语作文
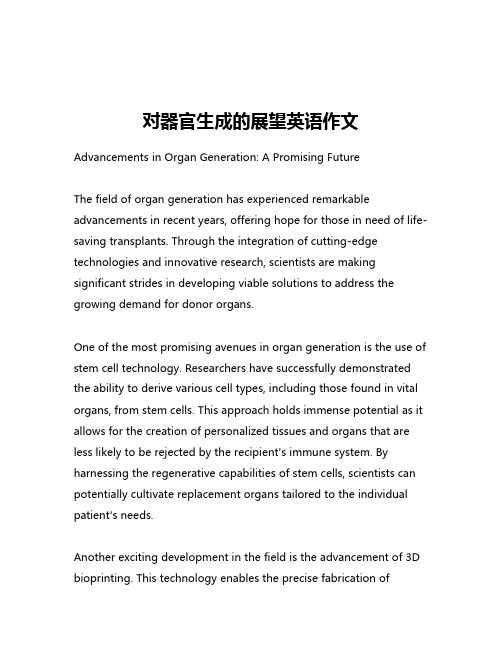
对器官生成的展望英语作文Advancements in Organ Generation: A Promising FutureThe field of organ generation has experienced remarkable advancements in recent years, offering hope for those in need of life-saving transplants. Through the integration of cutting-edge technologies and innovative research, scientists are making significant strides in developing viable solutions to address the growing demand for donor organs.One of the most promising avenues in organ generation is the use of stem cell technology. Researchers have successfully demonstrated the ability to derive various cell types, including those found in vital organs, from stem cells. This approach holds immense potential as it allows for the creation of personalized tissues and organs that are less likely to be rejected by the recipient's immune system. By harnessing the regenerative capabilities of stem cells, scientists can potentially cultivate replacement organs tailored to the individual patient's needs.Another exciting development in the field is the advancement of 3D bioprinting. This technology enables the precise fabrication ofcomplex tissue structures, including blood vessels, nerves, and even entire organs. By utilizing 3D printing techniques, researchers can create customized scaffolds that mimic the natural extracellular matrix, providing a suitable environment for cells to thrive and organize into functional tissues. The integration of 3D bioprinting with stem cell technology further enhances the prospects of generating viable organs for transplantation.Organ decellularization and recellularization is another innovative approach being explored by researchers. This process involves removing the cellular components from a donor organ, leaving behind the extracellular matrix. This scaffold is then seeded with the recipient's own cells, effectively creating a personalized organ that is less likely to be rejected by the body. This technique has shown promising results in animal studies and holds the potential to address the critical shortage of donor organs.In addition to the advancements in organ generation, researchers are also exploring alternative strategies to address the organ shortage. One such approach is the development of xenotransplantation, which involves the use of animal-derived organs for human transplantation. While this concept poses unique challenges, such as the risk of cross-species disease transmission, ongoing research is working to overcome these barriers and establish safe and effective xenotransplantation protocols.Furthermore, the integration of artificial intelligence and machine learning algorithms is revolutionizing the field of organ generation. These technologies are being leveraged to optimize the various stages of organ production, from cell culture and tissue engineering to the prediction of organ function and compatibility. By harnessing the power of AI, researchers can accelerate the development of personalized organ solutions and improve the overall efficiency of the organ generation process.As these innovative technologies continue to evolve, the future of organ generation holds immense promise. The ability to create customized, functional organs has the potential to transform the lives of millions suffering from organ failure. By addressing the critical shortage of donor organs, these advancements can alleviate the burden on healthcare systems, reduce waiting times for transplants, and ultimately save countless lives.However, the realization of this promising future is not without its challenges. Ethical considerations, regulatory hurdles, and the need for continued funding and collaboration among researchers and healthcare professionals must be carefully navigated. Nevertheless, the unwavering commitment and dedication of the scientific community, coupled with the growing public awareness and support, suggest that the future of organ generation is indeed bright.In conclusion, the advancements in organ generation offer a glimmer of hope for those in need of life-saving transplants. From the utilization of stem cell technology to the integration of 3D bioprinting and artificial intelligence, the field is poised to undergo transformative changes that can significantly improve the lives of countless individuals worldwide. As we continue to push the boundaries of scientific understanding and technological innovation, the future of organ generation holds the promise of a world where the shortage of donor organs is a thing of the past.。
人民大2023医学院校研究生英语读与写(第四版)教学课件Unit 12 Text B

other with respect to an ailment than fraternal twins are, then vulnerability to the disease must be rooted at least in part in heredity.
Meaning: Consequently, the RAC guidelines have been loose in stipulating the number and extent of precautions and safeguards for most experiments.
with respect to: in terms of Example: If identical twins are more similar to Nhomakorabeaeach
首都医科大学应用语言学系
Department of Applied Linguistics Capital Medical University
Text B Biotechnology—Ethics and Safety
Outline
Background information Global analysis of the text Detailed study of the text Text structure analysis Reference answers to the exercises
关于生命的科技作文英语
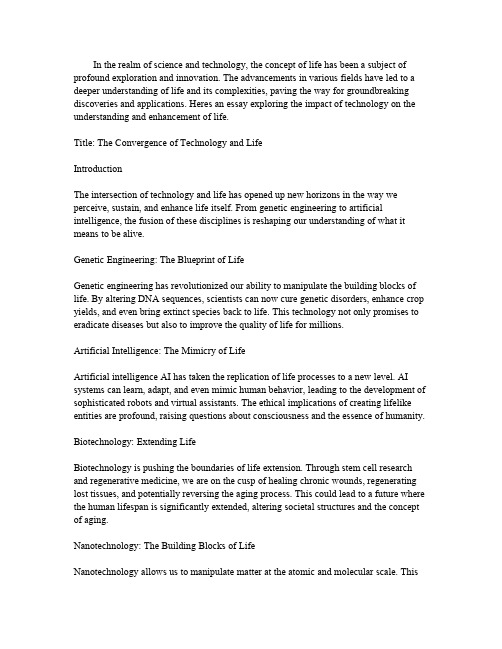
In the realm of science and technology,the concept of life has been a subject of profound exploration and innovation.The advancements in various fields have led to a deeper understanding of life and its complexities,paving the way for groundbreaking discoveries and applications.Heres an essay exploring the impact of technology on the understanding and enhancement of life.Title:The Convergence of Technology and LifeIntroductionThe intersection of technology and life has opened up new horizons in the way we perceive,sustain,and enhance life itself.From genetic engineering to artificial intelligence,the fusion of these disciplines is reshaping our understanding of what it means to be alive.Genetic Engineering:The Blueprint of LifeGenetic engineering has revolutionized our ability to manipulate the building blocks of life.By altering DNA sequences,scientists can now cure genetic disorders,enhance crop yields,and even bring extinct species back to life.This technology not only promises to eradicate diseases but also to improve the quality of life for millions.Artificial Intelligence:The Mimicry of LifeArtificial intelligence AI has taken the replication of life processes to a new level.AI systems can learn,adapt,and even mimic human behavior,leading to the development of sophisticated robots and virtual assistants.The ethical implications of creating lifelike entities are profound,raising questions about consciousness and the essence of humanity. Biotechnology:Extending LifeBiotechnology is pushing the boundaries of life extension.Through stem cell research and regenerative medicine,we are on the cusp of healing chronic wounds,regenerating lost tissues,and potentially reversing the aging process.This could lead to a future where the human lifespan is significantly extended,altering societal structures and the concept of aging.Nanotechnology:The Building Blocks of LifeNanotechnology allows us to manipulate matter at the atomic and molecular scale.Thisprecision opens up possibilities for targeted drug delivery,advanced prosthetics,and even the creation of nanobots capable of repairing damaged cells from within the body.The potential for enhancing life at the cellular level is immense.Synthetic Biology:Designing LifeSynthetic biology takes the concept of life to an entirely new dimension.Scientists are now able to design and construct new biological systems and organisms.This could lead to the creation of organisms tailored to perform specific tasks,such as cleaning up environmental pollutants or producing biofuels.Ethical ConsiderationsWhile the technological advancements offer immense potential,they also bring forth ethical dilemmas.The question of playing God by creating life or altering its course is a contentious issue.Balancing the benefits of these technologies with the potential risks and moral implications is a challenge that society must face.ConclusionThe convergence of technology and life is a testament to human ingenuity and our relentless pursuit of knowledge.As we stand on the precipice of new discoveries,it is crucial to approach these advancements with caution,responsibility,and a deep respect for the sanctity of life.The future holds the promise of a world where technology and life coexist in harmony,enhancing the human experience in ways we are only beginning to imagine.。
生物人工肝反应器三维支架材料选择与优化说明书
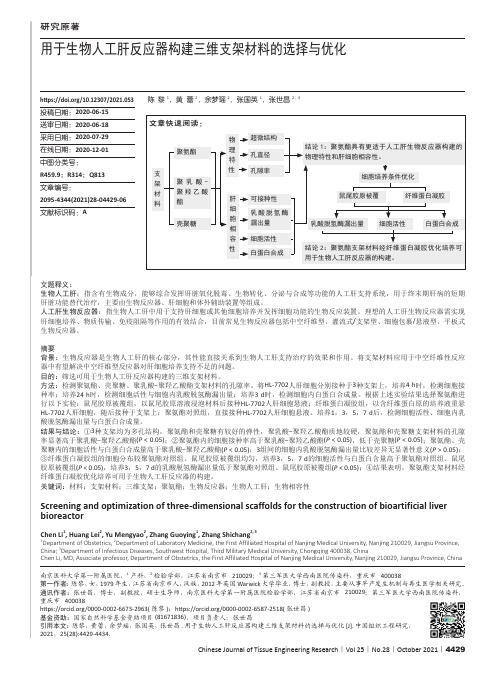
Chinese Journal of Tissue Engineering Research |Vol 25|No.28|October 2021|4429用于生物人工肝反应器构建三维支架材料的选择与优化陈 黎1,黄 蕾2,余梦瑶2,张国英1,张世昌2,3文题释义:生物人工肝:指含有生物成分,能够综合发挥肝脏氧化脱毒、生物转化、分泌与合成等功能的人工肝支持系统,用于终末期肝病的短期肝脏功能替代治疗,主要由生物反应器、肝细胞和体外辅助装置等组成。
人工肝生物反应器:指生物人工肝中用于支持肝细胞或其他细胞培养并发挥细胞功能的生物反应装置。
理想的人工肝生物反应器需实现肝细胞培养、物质传输、免疫阻隔等作用的有效结合,目前常见生物反应器包括中空纤维型、灌流式/支架型、细胞包裹/悬液型、平板式生物反应器。
摘要背景:生物反应器是生物人工肝的核心部分,其性能直接关系到生物人工肝支持治疗的效果和作用。
将支架材料应用于中空纤维性反应器中有望解决中空纤维型反应器对肝细胞培养支持不足的问题。
目的:筛选可用于生物人工肝反应器构建的三维支架材料。
方法:检测聚氨酯、壳聚糖、聚乳酸-聚羟乙酸酯支架材料的孔隙率。
将HL-7702人肝细胞分别接种于3种支架上,培养4 h 时,检测细胞接种率;培养24 h 时,检测细胞活性与细胞内乳酸脱氢酶漏出量;培养3 d 时,检测细胞内白蛋白合成量。
根据上述实验结果选择聚氨酯进行以下实验:鼠尾胶原被覆组,以鼠尾胶原溶液浸泡材料后接种HL-7702人肝细胞悬液;纤维蛋白凝胶组,以含纤维蛋白原的培养液重悬HL-7702人肝细胞,随后接种于支架上;聚氨酯对照组,直接接种HL-7702人肝细胞悬液。
培养1,3,5,7 d 后,检测细胞活性、细胞内乳酸脱氢酶漏出量与白蛋白合成量。
结果与结论:①3种支架均为多孔结构,聚氨酯和壳聚糖有较好的弹性,聚乳酸-聚羟乙酸酯质地较硬,聚氨酯和壳聚糖支架材料的孔隙率显著高于聚乳酸-聚羟乙酸酯(P < 0.05);②聚氨酯内的细胞接种率高于聚乳酸-聚羟乙酸酯(P < 0.05),低于壳聚糖(P < 0.05);聚氨酯、壳聚糖内的细胞活性与白蛋白合成量高于聚乳酸-聚羟乙酸酯(P < 0.05);3组间的细胞内乳酸脱氢酶漏出量比较差异无显著性意义(P > 0.05);③纤维蛋白凝胶组的细胞分布较聚氨酯对照组、鼠尾胶原被覆组均匀,培养3,5,7 d 的细胞活性与白蛋白含量高于聚氨酯对照组、鼠尾胶原被覆组(P < 0.05),培养3,5,7 d 的乳酸脱氢酶漏出量低于聚氨酯对照组、鼠尾胶原被覆组(P < 0.05);④结果表明,聚氨酯支架材料经纤维蛋白凝胶优化培养可用于生物人工肝反应器的构建。
新医科背景下医学细胞生物学形成性评价体系的构建
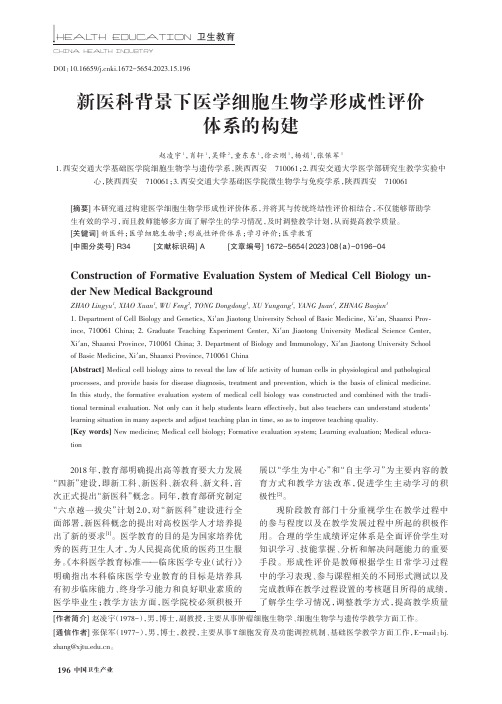
新医科背景下医学细胞生物学形成性评价体系的构建赵凌宇1,肖轩1,吴锋2,童东东1,徐云刚1,杨娟1,张保军31.西安交通大学基础医学院细胞生物学与遗传学系,陕西西安 710061;2.西安交通大学医学部研究生教学实验中心,陕西西安 710061;3.西安交通大学基础医学院微生物学与免疫学系,陕西西安 710061 [摘要] 本研究通过构建医学细胞生物学形成性评价体系,并将其与传统终结性评价相结合,不仅能够帮助学生有效的学习,而且教师能够多方面了解学生的学习情况,及时调整教学计划,从而提高教学质量。
[关键词] 新医科;医学细胞生物学;形成性评价体系;学习评价;医学教育[中图分类号] R34 [文献标识码] A [文章编号] 1672-5654(2023)08(a)-0196-04 Construction of Formative Evaluation System of Medical Cell Biology un⁃der New Medical BackgroundZHAO Lingyu1, XIAO Xuan1, WU Feng2, TONG Dongdong1, XU Yungang1, YANG Juan1, ZHNAG Baojun31. Department of Cell Biology and Genetics, Xi'an Jiaotong University School of Basic Medicine, Xi'an, Shaanxi Prov‐ince, 710061 China;2. Graduate Teaching Experiment Center, Xi'an Jiaotong University Medical Science Center, Xi'an, Shaanxi Province, 710061 China;3. Department of Biology and Immunology, Xi'an Jiaotong University School of Basic Medicine, Xi'an, Shaanxi Province, 710061 China[Abstract]Medical cell biology aims to reveal the law of life activity of human cells in physiological and pathological processes, and provide basis for disease diagnosis, treatment and prevention, which is the basis of clinical medicine. In this study, the formative evaluation system of medical cell biology was constructed and combined with the tradi‐tional terminal evaluation. Not only can it help students learn effectively, but also teachers can understand students' learning situation in many aspects and adjust teaching plan in time, so as to improve teaching quality.[Key words] New medicine; Medical cell biology; Formative evaluation system; Learning evaluation; Medical educa‐tion2018年,教育部明确提出高等教育要大力发展“四新”建设,即新工科、新医科、新农科、新文科,首次正式提出“新医科”概念。
中国科学 生命科学英文版
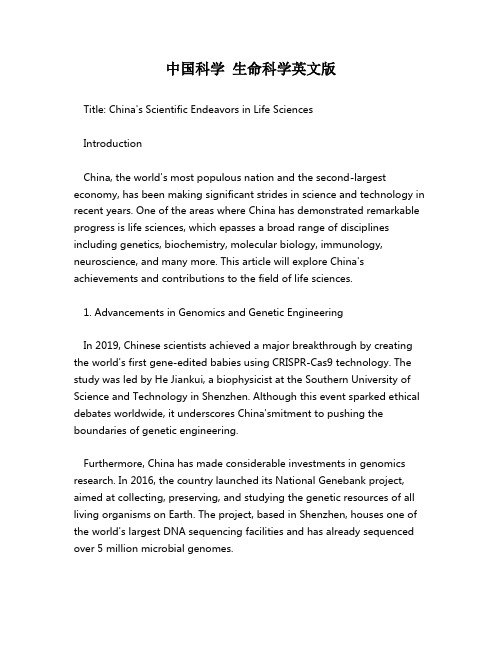
中国科学生命科学英文版Title: China's Scientific Endeavors in Life SciencesIntroductionChina, the world's most populous nation and the second-largest economy, has been making significant strides in science and technology in recent years. One of the areas where China has demonstrated remarkable progress is life sciences, which epasses a broad range of disciplines including genetics, biochemistry, molecular biology, immunology, neuroscience, and many more. This article will explore China's achievements and contributions to the field of life sciences.1. Advancements in Genomics and Genetic EngineeringIn 2019, Chinese scientists achieved a major breakthrough by creating the world's first gene-edited babies using CRISPR-Cas9 technology. The study was led by He Jiankui, a biophysicist at the Southern University of Science and Technology in Shenzhen. Although this event sparked ethical debates worldwide, it underscores China'smitment to pushing the boundaries of genetic engineering.Furthermore, China has made considerable investments in genomics research. In 2016, the country launched its National Genebank project, aimed at collecting, preserving, and studying the genetic resources of all living organisms on Earth. The project, based in Shenzhen, houses one of the world's largest DNA sequencing facilities and has already sequenced over 5 million microbial genomes.2. Biomedical Research and Drug DevelopmentChinese researchers have been actively engaged in biomedical research to develop novel therapeutic strategies for various diseases. For instance, in 2019, Chinese scientists developed a new type of CAR-T cell therapy that showed promising results in treating relapsed or refractory acute lymphoblastic leukemia.Moreover, China has be a global leader in clinical trials. According to a report by IQVIA, a healthcare analytics firm, China surpassed the United States in the number of clinical trials initiated in 2018. With a vast patient pool, streamlined regulatory processes, and growing investment in R&D, China is well-positioned to contribute significantly to the development of new drugs and therapies.3. Synthetic Biology and BioinformaticsSynthetic biology, an interdisciplinary field thatbines principles from biology, engineering, andputer science, is another area where China has shown great promise. Researchers in China are exploring ways to engineer microorganisms to produce biofuels, pharmaceuticals, and other valuablepounds. They are also developingputational tools to design and optimize synthetic biological systems.In addition, China has invested heavily in bioinformatics infrastructure. The China National GeneBank DataBase (CNGBdb) is aprehensive data platform that supports large-scale omics studies and provides open access to genomic data. It plays a critical role in facilitating international collaborations and promoting data sharing in the life sciencesmunity.4. Neuroscience and Brain ResearchNeuroscience is yet another frontier where China is striving to make groundbreaking discoveries. The China Brain Project, launched in 2016, aims to advance our understanding of brain function and develop effective treatments for neurological disorders such as Alzheimer's disease and Parkinson's disease. The initiative involves collaboration among multiple institutions across the country and has received substantial funding from the government.ConclusionChina's rapid advancement in life sciences can be attributed to several factors, including strong government support, substantial financial investments, and a large pool of talented researchers. While there are still challenges to ovee, such as improving research integrity and addressing ethical concerns, China's scientific achievements in life sciences hold great potential for improving human health and transforming the global biotechnology landscape. As China continues to push the frontiers of knowledge in this field, it is poised to play an increasingly influential role in shaping the future of life sciences worldwide.。
- 1、下载文档前请自行甄别文档内容的完整性,平台不提供额外的编辑、内容补充、找答案等附加服务。
- 2、"仅部分预览"的文档,不可在线预览部分如存在完整性等问题,可反馈申请退款(可完整预览的文档不适用该条件!)。
- 3、如文档侵犯您的权益,请联系客服反馈,我们会尽快为您处理(人工客服工作时间:9:00-18:30)。
Domain Ontology Construction from Biomedical Text Saurav Sahay1, Baoli Li1, Ernest V Garcia2, Eugene Agichtein3, Ashwin Ram1 1College of Computing, Georgia Institute of Technology, Ph -404.894.78652Department of Radiology, Emory University,Ph – 404.353.01433Department of Mathematics and Computer Science, Emory University, Ph – 404.727.7962{ssahay, baoli, ashwin}@, eugene@,Ernest.Garcia@Keywords: Ontology Learning, Information Extraction, Natural Language ProcessingSaurav Sahay – Presenting author(submitted to International Conference on Artificial Intelligence (ICAI), 2007)AbstractNLM's Unified Medical Language System (UMLS) is a very large ontology of biomedical and health data. In order to be used effectively for knowledge processing, it needs to be customized to a specific domain. In this paper, we present techniques to automatically discover domain-specific concepts, discover relationships between these concepts, build a context map from these relationships, link these domain concepts with the best-matching concept identifiers in UMLS using our context map and UMLS concept trees, and finally assign categories to the discovered relationships. This specific domain ontology of terms and relationships using evidential information can serve as a basis for applications in analysis, reasoning and discovery of new relationships. We have automatically built an ontology for the Nuclear Cardiology domain as a testbed for further enhancing our techniques.1. IntroductionOne of the broad goals of intelligent information processing is domain modeling and knowledge creation for machine processable knowledge-aware applications. Biomedical informatics research has been actively looking at incorporating domain knowledge in ontologies that can be shared by many applications. Various ontologies and knowledge bases have been developed for several domains. UMLS[1] is one such large consolidated repository of medical terms and their relationships, spread across multiple languages and disciplines by combining more than 100 different source vocabularies. This ontology organizes information from various source vocabularies, each with its attributes, describes relationships between concepts and organizes these concepts under a semantic network of broad category types and links. However, UMLS ontology does not incorporate complex domain relationships between its resources. For example, although UMLS contains details about many diseases, viruses and bacteria, it does not incorporate relations between diseases and the causes of the diseases.[2] We propose an approach of constructing a domain-specific ontology directly from a corpus using statistical NLP techniques. The discovered relationships between concepts are used to build a network of terms and relationships for the domain. We call this network our context map. We link the context map with the UMLS network by the concept matcher process to discover similar concepts based on lexical and conceptual metrics of similarity among two concepts. We create richer semantics in our ontology by explicitly capturing the type of the relationship between concepts. The advantage of this approach is that it directly uses thedomain data to construct smaller and manageable domain specific ontologies that can be used directly for several knowledge processing tasks.Some important related work in this area point to TextToOnto (Maedche and Staab, 2000; Cimiano et al., 2005) and OntoLT (Buitelaar et al., 2004). Knowledge Engineering based approaches in ontology modeling assisted with Protégé or GATE are also quite popular.2. Domain ontology construction frameworkThis section explains our technique for discovering domain-specific terms and relationships for constructing the ontology. We show the flow diagram and the techniques used therein in Table 1.Table 1. Flow Diagram for our Ontology Construction System2.1 Concept ExtractionOur goal is to build domain ontology for the Nuclear Cardiology discipline that deals with clinical trial experiments and does not involve animal subjects. We have built an information processing infrastructure which utilizes Pubmed web services to fetch desired abstracts from the Journal of Nuclear Cardiology and convert them to xml format. Our system retrieves abstracts from Pubmed and invokes the Mmtx system[3] to map all possible phrases in the abstracts to their possible UMLS concept identifiers and Semantic Types. Noun Phrases from titles, abstracts and associated Mesh Terms are extracted using MedPostSKRTagger[4], a Part of Speech tagger bundled with UMLS resources, trained on Pubmed corpus. Approximate matching heuristics such as occurrence of gaps and permutations of words in retrieved phrases are applied to look up the variants, synonyms and abbreviations for matching domain terminology with UMLS concepts. A higher weight is given to terms occurring in Titles compared to terms occurring elsewhere. A stopword list used in SMART [5] system is used to discard non-informative words from our extracted phrases. Term frequencies are used as the keyword weighting scheme for ranking our phrases. A list of ranked retrieved phrases with their matching UMLS concept identifiers and Semantic Types is constructed. To build a clean ontology, we have to assign the best-matching concept identifier to the phrase which may map to several possible concept identifiers.2.2 Context ExtractionThe next step is to build a context map of related terms for our domain. This is done using the MedPostSKR Tagger, which is used to retrieve NounPhrase verbPhrase NounPhrase (Subject verb Object (SVO) triple patterns) patterns from sentences.For each abstract AFor each sentence S in AFind occurrences of domain concept pairs in S Æ PairsFor each concept pair <C1, C2> in PairsApply verbGroup matching classify <C1,C2> into relations Æ R Add R to all Relationsreturn RelationsFigure 1: Relation extraction algorithmThis above pseudo-code in Figure 1 is applied to find SVO patterns that map to one or many concept identifiers in UMLS. Categories of verbGroups are created using regular expression patterns for identifying a rich set of labeled relationships. GraphViz tool [6] is used to visualize the relationships between concepts.2.3 Concept Matcher Process2.3.1 Semantic MatchThis step finds best matching concept from UMLS and assigns them to the domain phrase along with the match value. In order to find the conceptual match between the phrase and the possible concepts, the context map is used to find related phrases. These related phrases can map to a unique UMLS concept or many concepts. We look for related phrases with uniquely mapping concepts and then build a suffix tree for the concept hierarchy compute the conceptual distance [7] between the possible phrase concepts and the unique concept. The shortest distance between two concepts is computed by a spreading activation search on the UMLS hierarchy graph. Two concepts share a common concept in the graph. There may be several common concepts between two concepts. (through various parents of these two concepts) The shortest path common concept links the closest matching concepts. This notion of conceptual similarity is based on the premise that “related terms in the context map are closely related to each other in the UMLS graph”.For each phrase P with multiple matching concepts <C1,C2 … Cn>For each related concept C from the context mapBuild concept graph using PARENT links as a suffix treeFind TS(C,C1), TS(C,C2)… TS(C,Cn)shortestDist = min{ TS(C,C1), TS(C,C2)… TS(C,Cn)}return shortestDistFigure 2: Concept Matcher algorithmFigure 2 above describes the pseudo-code for finding concept identifier for terms with ambiguous concepts.The tree similarity TS between two concepts C 1 and C 2, can be computed according to the following formula [8]:)()(),(2),(212121C Depth C Depth C C Common C C TS +×=where common(C1, C2) denotes the number of common nodes in the paths between the root and the given concepts, and depth (C) is the number of nodes in the path connecting the root and the given concept C.2.3.2 Lexical MatchWe may not find a semantic match between arbitrary concepts in UMLS. ‘It should bepointed out that UMLS defined a parent Concept Unique Identifiers (CUI) only for a minority of CUIs, usually mutuating the parents from the titles of classification sections (e.g."Bronchial-Diseases").[9]’ Other links such as ‘broader’ or ‘narrower’ in theMetathesaurus are not well defined as they reference related terms from different vocabularies and can contain cycles and other ambiguities.In order to be able to assign a unique class label to all our domain terms, we define another Lexical Match metric to assign the best possible categories to our terminology :⎥⎦⎤⎢⎣⎡−+−−∩=),())()(()(,0Cpt Phr Max Phr Cpt Num Cpt Phr Num Cpt Phr Num Max ch LexicalMat where we subtract the unique tokens from the common tokens and normalize by themaximum length token. This metric is suitable for multi word tokens (phrases and concepts) and computes the lexical similarity between words.2.4 Relationship Matcher ProcessA user might be interested in certain types of relationships. It is necessary to assign types to the retrieved verbs in relationship triples. We have created patterns for the 54 UMLSSemantic Network relationship links. We assign these categories to the extracted verbs. In order to increase the accuracy of this technique, we have built a system that uses WordNet resource for finding all word sense synonyms for the verb to match against our patterns list.patterns = List of patterns for Semantic Network relationships synonyms = List of synonyms for extracted domain verbinitialize a HashMap of patternMatches for each s in synonyms {patternMatches = longestStringMatch(s,patterns) }return patternMatchesFigure 3: Relation Matcher algorithm3. Experiments3.1 Concept ExtractionThe abstracts from 618 original scientific articles published in the Journal of NuclearCardiology (JNC) were used for this research and experiments. These abstracts summarizethe knowledge of the entire scientific manuscripts published by JNC from 1995 to 2004. We have used this resource to build the knowledgebase of relevant terms and relations. We extracted 10191 term (phrases) from these articles. These terms are noun phrases that have at least one mapping in the UMLS metathesaurus.We used an expert provided list of 42 relevant domain terms for the Myocardial Perfusion Imaging domain (a sub-domain of nuclear cardiology). Of these, we found exactly matching 31 terms, 2 morphological variants and 8 phrases that contained the terms from the expert list. We missed to report 1 term in our ontology – ‘dyperidamole’ – which is a drug given during pregnancy. We found that this term didn’t exist in the 618 JNC abstracts we were using. Instead, another term, diperidamole (spelling variant) existed in out term list. To do away with noise in our data, we calculated the average frequency (2.9) of the 10191 retrieved terms and set that as our threshold. We pruned the list to 1415 terms – this list included all the 41 relevant domain terms or their variants.Figure 4: Top 500 Word Frequency Distribution from extracted terms3.2 Context ExtractionMany complicated and expensive techniques have been applied extensively in literature to extract relationships and dependencies from data. These techniques generally rely on a deep parse results that gives a rich representation which is used for applications in knowledge extraction and semantics. The problem with biomedical data parsing is that there is no exhaustive tagged training corpus available to build models for efficient parsing on test data. Also, deep parsing is computationally very expensive compared to shallow parsing that uses the finite state automaton techniques. UMLS graciously provides such a shallow parser[4] that is trained on entire Medline corpus and claims to achieve over 97% accuracy.We ran the shallow parser on our JNC abstracts and extracted NounPhrase verb NounPhrase triples for the 1415 extracted terms in our domain. We ignored non-informative verbs (‘be’,‘have’ modal verbs) to extract meaningful relationships. We checked for all possible relations in all the sentences of the corpus. We retrieved 2562 such relations.Some example relations extracted from our technique :Left ventricle DIVIDED regional myocardial uptakesAcipimox REDUCES serum free fatty acidMental stress FOUND significant hemodynamic responsesTl-201 washout rate CALCULATED lv end-diastolic volume indexTl-201/bmipp subtraction polar map SHOWED focal uptake patternCarvedilol IMPROVES left ventricular functionFigure 5. Extracted Relations using a shallow parserThe goal of this stage was to build local contextual maps of terms and their relationships with other terms. We merged all the extracted relations and created visual maps for facilitating interpretation and analysis.Figure 6. Part of context map with ranks and semantic types.This context map shows two relationships {ejection fraction increased left-ventricular end-diastolic volume} and {results showed ejection fraction fluctuations}. The domain terms inthis map are attached to their possible semantic labels and a similarity score between the term and the concepts.3.3 Concept Matcher ProcessThe goal of this step is to assign unique categories to the domain terms. One term in UMLS can map to several Metathesaurus categories depending on it’s lexical match (overmatch, undermatch, match with gaps), acronyms, variant forms, and it’s context information.In order to disambiguate this, we have used a combination of semantic and lexical match to assign best possible categories to the domain terms in the ontology. Paper[4] has described conceptual match experiments. We ran into several problems with conceptual similarity metric as the concept tree grows exponentially in size following the concept node’s parent links. We had to limit our searches to 500 parent nodes and search in this region of graph for the related term’s concept hierarchy matches. We implemented a suffix tree based graph search algorithm to quickly scan for nodes in the suffix tree. We found very few matches with our limited implementation that we report here and we leave further discussions on this technique for future work.We found “i-123 mibg” correctly mapping to “3-Iodobenzylguanidine” using the related node “analysis aspect”. Similarly, ‘nonfatal myocardial infarction’ mapped to ‘myocardial infarction’ using ‘unstable angina’We assigned most categories to the domain terms using Lexical Match Metric.intracoronary sestamibi <C1337333:SESTAMIBI>endothelium-dependent regulation <C0851285:Regulation>left ventricular end-diastolic volume <C0455833:Left ventricular end-diastolic cavity size>17-segment model <C0026336:Study models>body <C0242821:Human body>opposite the difference <C0443199:Differential quality>successful evaluation <C1550157:Processing type - Evaluation>thrombolytic therapy <C0040044:Thrombolytic Therapy>diabetic nephropathy <C1442864:DIANPH gene>cox regression analysis <C0034980:Regression Analysis>Figure 7: Categorizing Domain Terms.3.4 Relationship MatcherFigure 3 describes our Relationship Matcher algorithm. UMLS assigns 54 relationship types in it’s Semantic Network. We have used those label names had-crafted more patterns for those relationships. We check for verbs and their verbal synonym synsets and match against all patterns to assign the largest string match pattern as it’s category. This way, we have found several ‘OTHER’ patterns that do not belong to any of the 54 link patterns. On studying these, we have mostly found these ‘OTHER’ patterns to be noise in our data and safely discarded them. After all these steps, we have eventually found 1150 relationships between our domain concepts.left ventricle DIVIDED <PART_OF> regional myocardial uptakesstudy EVALUATED <MEASURES> impactprognosis population COMPRISED <CONTAINS> 16,020 consecutive patients cardioinhibitory response SHOWED <EXHIBITS> vasodepressor responseeighteen patients UNDERWENT <BRINGS_ABOUT> i-123 mibgconstant supply SUSTAIN <PREVENTS> contractile functionreversible regional wall motion abnormalitiesPREDICT <CAUSES> future caFigure 8: Relation Matcher Results4. ConclusionThis paper introduced an on-the-fly ontology construction methodology from text using existing resources and parsing and extraction. Using this elaborate framework and techniques, we have automatically and efficiently discovered relationships between resources.We are working with experts in Nuclear Cardiology to extensively evaluate our results. We have been encouraged to learn about our promising results. As future work, we are looking at several techniques to refine and enrich our ontology. We are also in the process of converting our ontology to RDFS format. Once we have this machine processable knowledgebase in place, we can easily extend this to an environment where we can carry out knowledge reuse, decision support and reasoning for biomedical applications.References[1] /[2] Sougata Mukherjea and Saurav Sahay. Discovering Biomedical Relations Utilizing the World-Wide Web.Pacific Symposium on Biocomputing 11:164-175(2006)[3] /[4] L. Smith, T. Rindflesch, and W. J. Wilbur. Medpost: a part-of-speech tagger for biomedical text.Bioinformatics, 20(14), 2004.[5] Buckley, C., Salton, G., and Allan, J. 1993. The SMART information retrieval project. In Proceedings of theWorkshop on Human Language Technology (Princeton, New Jersey, March 21 - 24, 1993). Human Language Technology Conference. Association for Computational Linguistics, Morristown, NJ, 392-392.[6] Gansner, E. R. and North, S. C. 2000. An open graph visualization system and its applications to softwareengineering. Softw. Pract. Exper. 30, 11 (Sep. 2000), 1203-1233.[7] Caviedes, J. E. and Cimino, J. J. 2004. Towards the development of a conceptual distance metric for theUMLS. J. of Biomedical Informatics 37, 2 (Apr. 2004), 77-85.[8] I Spasic, S Ananiadou, J McNaught, A Kumar. Text mining and ontologies in biomedicine: Making sense outof Raw Text. Briefings in Bioinformatics, 2005 - Oxford Univ Press[9] DM Pisanelli, A Gangemi, G Steve 1998. An Ontological Analysis of the UMLS Metathesaurus.Proc AMIA Symp, 1998[10] Q. Li, P. Shilane, N. F. Noy, & M. A. Musen. Ontology Acquisition from On-line Knowledge Sources In theProceedings of the AMIA Annual Symposium, Los Angeles, CA.[11] Maedche, A. and Staab, S. 2001. Ontology Learning for the Semantic Web. IEEE Intelligent Systems 16, 2(Mar. 2001), 72-79.[12] Kietz, J., Volz, R., and Maedche, A. 2000. Extracting a domain-specific ontology from a corporate intranet. InProceedings of the 2nd Workshop on Learning Language in Logic and the 4th Conference on Computational Natural Language Learning - Volume 7 (Lisbon, Portugal, September 13 - 14, 2000). Annual Meeting of the ACL. Association for Computational Linguistics, Morristown, NJ, 167-175.。