Application of the canonical quantization of systems with curved phase space to the EMDA th
The Canonical Metric for Vector Quantization
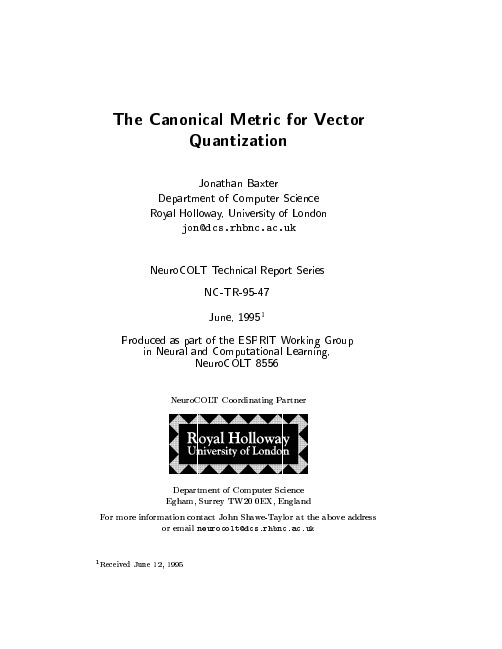
Abstract
1 The Problem
As any real digital communication channel has only nite capacity, transmitting continuous data (e.g speech signals or images) requires rstly that it be transformed into a discrete representation. Typically, given a probability space (X; P; X ), one chooses a quantization of X , fx1; : : : ; xkg X and instead of transmitting x 2 X , the index of the \nearest" quantization point qd (x) = minx d(xi; x) is transmitted, where d is some function (not necessarily a metric) measuring the distance between points in X . d is called a distortion measure. The quantization points are chosen so that the expected distance between x and its quantization is minimal, i.e ~ = fx1; : : : ; xk g are chosen to x minimize the reconstruction error
The Solution 2
Dark Count Statistics in Geiger-Mode Avalanche
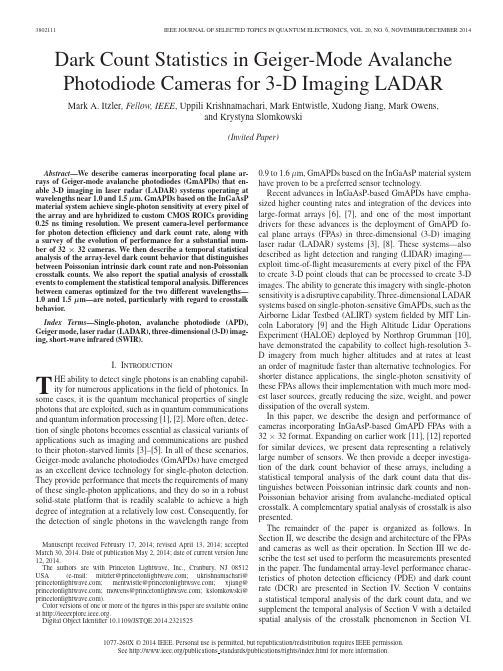
Dark Count Statistics in Geiger-Mode Avalanche Photodiode Cameras for3-D Imaging LADAR Mark A.Itzler,Fellow,IEEE,Uppili Krishnamachari,Mark Entwistle,Xudong Jiang,Mark Owens,and Krystyna Slomkowski(Invited Paper)Abstract—We describe cameras incorporating focal plane ar-rays of Geiger-mode avalanche photodiodes(GmAPDs)that en-able3-D imaging in laser radar(LADAR)systems operating at wavelengths near1.0and1.5μm.GmAPDs based on the InGaAsP material system achieve single-photon sensitivity at every pixel of the array and are hybridized to custom CMOS ROICs providing 0.25ns timing resolution.We present camera-level performance for photon detection efficiency and dark count rate,along with a survey of the evolution of performance for a substantial num-ber of32×32cameras.We then describe a temporal statistical analysis of the array-level dark count behavior that distinguishes between Poissonian intrinsic dark count rate and non-Poissonian crosstalk counts.We also report the spatial analysis of crosstalk events to complement the statistical temporal analysis.Differences between cameras optimized for the two different wavelengths—1.0and1.5μm—are noted,particularly with regard to crosstalk behavior.Index Terms—Single-photon,avalanche photodiode(APD), Geiger mode,laser radar(LADAR),three-dimensional(3-D)imag-ing,short-wave infrared(SWIR).I.I NTRODUCTIONT HE ability to detect single photons is an enabling capabil-ity for numerous applications in thefield of photonics.In some cases,it is the quantum mechanical properties of single photons that are exploited,such as in quantum communications and quantum information processing[1],[2].More often,detec-tion of single photons becomes essential as classical variants of applications such as imaging and communications are pushed to their photon-starved limits[3]–[5].In all of these scenarios, Geiger-mode avalanche photodiodes(GmAPDs)have emerged as an excellent device technology for single-photon detection. They provide performance that meets the requirements of many of these single-photon applications,and they do so in a robust solid-state platform that is readily scalable to achieve a high degree of integration at a relatively low cost.Consequently,for the detection of single photons in the wavelength range fromManuscript received February17,2014;revised April13,2014;accepted March30,2014.Date of publication May2,2014;date of current version June 12,2014.The authors are with Princeton Lightwave,Inc.,Cranbury,NJ08512 USA(e-mail:mitzler@;ukrishnamachari@ ;mentwistle@;xjiang@ ;mowens@;kslomkowski@ ).Color versions of one or more of thefigures in this paper are available online at .Digital Object Identifier10.1109/JSTQE.2014.23215250.9to1.6μm,GmAPDs based on the InGaAsP material system have proven to be a preferred sensor technology.Recent advances in InGaAsP-based GmAPDs have empha-sized higher counting rates and integration of the devices into large-format arrays[6],[7],and one of the most important drivers for these advances is the deployment of GmAPD fo-cal plane arrays(FPAs)in three-dimensional(3-D)imaging laser radar(LADAR)systems[3],[8].These systems—also described as light detection and ranging(LIDAR)imaging—exploit time-of-flight measurements at every pixel of the FPA to create3-D point clouds that can be processed to create3-D images.The ability to generate this imagery with single-photon sensitivity is a disruptive capability.Three-dimensional LADAR systems based on single-photon-sensitive GmAPDs,such as the Airborne Lidar Testbed(ALIRT)systemfielded by MIT Lin-coln Laboratory[9]and the High Altitude Lidar Operations Experiment(HALOE)deployed by Northrop Grumman[10], have demonstrated the capability to collect high-resolution3-D imagery from much higher altitudes and at rates at least an order of magnitude faster than alternative technologies.For shorter distance applications,the single-photon sensitivity of these FPAs allows their implementation with much more mod-est laser sources,greatly reducing the size,weight,and power dissipation of the overall system.In this paper,we describe the design and performance of cameras incorporating InGaAsP-based GmAPD FPAs with a 32×32format.Expanding on earlier work[11],[12]reported for similar devices,we present data representing a relatively large number of sensors.We then provide a deeper investiga-tion of the dark count behavior of these arrays,including a statistical temporal analysis of the dark count data that dis-tinguishes between Poissonian intrinsic dark counts and non-Poissonian behavior arising from avalanche-mediated optical crosstalk.A complementary spatial analysis of crosstalk is also presented.The remainder of the paper is organized as follows.In Section II,we describe the design and architecture of the FPAs and cameras as well as their operation.In Section III we de-scribe the test set used to perform the measurements presented in the paper.The fundamental array-level performance charac-teristics of photon detection efficiency(PDE)and dark count rate(DCR)are presented in Section IV.Section V contains a statistical temporal analysis of the dark count data,and we supplement the temporal analysis of Section V with a detailed spatial analysis of the crosstalk phenomenon in Section VI.1077-260X©2014IEEE.Personal use is permitted,but republication/redistribution requires IEEE permission.See /publications standards/publications/rights/index.html for more information.Fig.1.Schematic illustration of the construction of the GmAPD FPA.See text for a description of the FPA components.A discussion of our results andfinal conclusions is provided in Section VII.II.S ENSOR A RCHITECTURE AND O PERATIONA.FPA DesignThe core functionality of the GmAPD FPA is determined by three semiconductor arrayed devices:the InGaAsP-based GmAPD photodiode array(PDA);a0.18μm CMOS readout integrated circuit(ROIC);and a GaP microlens array(MLA). The PDA pixels are connected to their corresponding ROIC pix-els by indium-bumpflip-chip hybridization.The MLA is then aligned and attached to the substrate side of the rear-illuminated PDA to maintain a high opticalfill factor ofß75%for optical coupling to the34μm diameter active region in each PDA pixel. The ROIC I/O channels are wirebonded to a ceramic interposer which facilitates routing of electrical signals to appropriate pins of a pin grid array in the hermetic housing assembly.An inte-grated thermoelectric cooler maintains a temperature differen-tial of55°C relative to the ambient temperature of the housing; for a typical25°C ambient,the FPA operates at about−30°C.A schematic illustration of the FPA construction is provided in Fig.1.The GmAPD devices in each pixel of the PDA are based on a buried p-n junction fabricated using a zinc dopant diffu-sion process that provides highly uniform and reliable pixels in large scale arrays.PDAs optimized for operation using source lasers with an output wavelength near1μm employ an InGaAsP absorber region that results in spectral response over the wave-length range from900to1150nm.PDAs intended for use with longer-wavelength sources near1.5μm make use of an InGaAs absorber region that results in wider spectral response from900 to1620nm.In previous publications,we have described the de-sign of these GmAPD devices[6],[13]and their incorporation into array formats[11],[14].B.Camera-Level IntegrationThe GmAPD FPA has been integrated into a modular camera head with three principal electronic boards.The FPA board sup-ports the FPA sensor itself,and it has circuitry that controls FPA power and temperature regulation.The FPGA board contains an altera FPGA and a microcontroller that provide extensive on-board functionality throughfirmware programming.In addition to facilitating operation of the ROIC,the FPGA also collects and formats raw data from the ROIC for transfer off of the cam-era head.This data transfer is executed by the interface board using an industry-standard CameraLink protocol,and this board also manages external power regulation and external clock and trigger inputs.The camera head requires only a single15W dc source between12and36V,from which all required biases and power levels are generated internally.Through the CameraLink interface,the camera head com-municates with the system computer that controls all camera functions through comprehensive graphical user interface(GUI) software.Beyond camera control,this software also provides for real-time data storage to solid-state drives(SSDs)in RAID0 configuration,accommodating data rates in excess of4Gb/s at the maximum camera frame rate of182000Hz.C.Sequence of Framed OperationThe framed operation of the GmAPD cameras described in this paper proceeds according to the following sequence.Each data frame is initiated by a trigger signal that can be provided by the internal camera clock;by an external system clock;or by any other synchronizing signal,such as from a“flash detector”that generates a trigger correlated to an out-going laser pulse.Before arming the pixels of the FPA,the camera provides for a variable delay time ranging from0to256μs to allow for the transit time of the ns-scale laser pulse to be reflected from distant objects. During this delay time,the camera remains in its disarmed state, with the reverse bias voltage across the GmAPD detectors set to a disarm value V d below the breakdown voltage V b,where V b–V d is generally on the order of1to4V.(All voltages in this discussion entail reverse biasing of the GmAPD but are expressed as positive values for convenience.)Following the delay time,the pixels are armed by applying an additional5V reverse bias using appropriate transistors in the CMOS ROIC. This brings the reverse voltage of the GmAPDs above V b by an excess bias V e and corresponds to charging up the capacitance of each GmAPD to V e=V b–V d+5V.This charging period of12ns is the only time during which the GmAPD pixels are connected to an external supply with the net voltage exceeding V b.Once the pixels are charged to the target V e value,the external 5V supply is disconnected,and any small leakage of charge from the GmAPD capacitance that would cause a reduction in voltage is compensated in the pixel-level ROIC circuitry,thereby maintaining the target excess bias value.Once the pixel arming sequence is complete,linear-feedback shift register pseudorandom counters in all the pixels are enabled to begin timing during the“range gate.”The counters have13-bit resolution,and a full range gate consists of the counters proceed-ing through8000counter values,ending with afinal“terminal count”value.During the counting sequence,an avalanche at any GmAPD pixel will freeze the corresponding pixel counter in the ROIC,indicating the arrival time of a photon or a dark count,and the pixel is then disarmed by actively quenching thebias voltage of that pixel below V b.A pixel records only onetime-stamp value per frame.At the end of a frame,if a pixelreturns the terminal count,then it did not detect an avalancheevent for that frame.Once the terminal count is reached,all theremaining armed pixels are disarmed,and all counter values areread out along shift registers in each row during a readout periodof3.5μs.By adjusting the ROIC clock frequency,we can vary the timeduration associated with each counter increment from0.25to1.25ns.Given8000of these“time-bins”in each range gate,the total range gate duration can be varied from2to10μs.Thesequential combination of a2μs range gate followed by a3.5μsread-out time provides the maximum frame rate of182kHz.Because the GmAPD in each pixel is disconnected from theROIC external5V bias during the range gate,an avalancheduring the range gate has a chargeflow that is limited to thecharge applied in raising the bias of the device from the dis-armed voltage V d to the excess bias V e.This total bias charge Qis roughly equivalent to(C d+C p)•(5V)where C dß100fF is the APD capacitance and C p is any parasitic capacitance.If weassume C p∼C d,then Qß6×106e−.However,during the 12ns arming period while the pixels are connected to the exter-nal ROIC supply that charges up the APDs to V e,the triggeringof an avalanche can result in continuous chargeflow for theremainder of the arming period.Assuming a5V bias across anestimated5kΩseries impedance in the arming circuit,currentflow for an average duration of6ns(i.e.,half of the12ns arm-ing period)results inß108e−,or roughly10times more thana pixel avalanche during the range gate.This discharging of apixel during the arm cycle causes this pixel to be in the disarmedstate when the counters start,and it registers a count during theveryfirst time bin of the range gate.These counts are oftenreferred to as“earlyfire”events.Moreover,their comparativelylarge currentflow during the arming period can lead to crosstalkevents that generate additional earlyfires.This distinction willbe mentioned later in our discussion of the statistics of crosstalkand the impact of segregating earlyfire counts at the beginningof the range gate.D.FPA Performance AttributesThe GmAPD FPA exhibits a number of critical performanceattributes.The probability of successfully detecting a singlephoton when it arrives at one of the FPA pixels is referred toas the PDE of that pixel.In all of the PDE data presented inthis paper,we provide camera-level PDE values which includeall optical losses associated with elements in the optical path,including theß75%fill factor of the MLA,in which the lenseshave an acceptance angle of6°from normal incidence.There is also afinite probability of false counts being triggeredin the absence of photon arrivals,giving rise to an effective DCR.The measured dark counts actually include counts originatingfrom several mechanisms.The generation of dark carriers bythermal excitation or trap-assisted tunneling dictates the intrinsicDCR,and the earlyfire phenomenon described in the previoussub-section can be classified as a distinct type of dark count.During each avalanche event,the chargeflow described in Section II-C gives rise to photon emission,likely due to intra-band relaxation of hot carriers crossing the diode p-n junction [15],and the photons emitted by this hot-carrier luminescence effect can initiate correlated avalanches at other array pixels. These“crosstalk”counts can be initiated by avalanches associ-ated with photon detections or dark counts,and they therefore are present in any array-level raw data collected for PDE or DCR.One of the new results reported below is the unambigu-ous extraction of the crosstalk contribution to DCR based on a statistical analysis of the temporal attributes of array-level DCR data.Another important attribute of GmAPD FPAs used for time-of-flight measurements is the uncertainty in the recorded photon arrival times,commonly referred to as the timing jitter.We distinguish between the uncertainty among timestamps for all the pixels of a single frame—the“intra-frame”jitter—and the frame-to-frame uncertainty in a given pixel—the“inter-frame”jitter.We have reported typical values for these two types of jitter of175and380ps,respectively[12],although more recent results suggest that both types of jitter are inherently<200ps and that previous measurements of larger inter-frame jitter were actually dominated by system-level jitter from the pulsed laser source[16].Beyond this summary of previous results,we will not address timing jitter performance further in this paper.Afinal performance attribute of frequent importance for GmAPDs is the afterpulsing effect[17]caused by the trap-ping of charges resulting from one avalanche and the occur-rence of correlated dark counts a short time later due to the subsequent detrapping of these charges.Afterpulsing can be mitigated by imposing a sufficiently long“hold-off”time fol-lowing an avalanche event to allow detrapping of substantially all of the trapped charges prior to re-arming a GmAPD.Under typical GmAPD operating conditions(e.g.,3V excess bias and an FPA temperature of248K),afterpulsing effects can be sig-nificant for hold-off times of less thanß1μs,but hold-off times longer than this are adequate to reduce afterpulsing to incon-sequential levels,as found by some of the present authors for discrete GmAPDs[17]as well as by Frechette et al.for GmAPD arrays operated with asynchronous ROICs[18].For the FPAs described in this paper,the GmAPDs are always disarmed dur-ing the3.5μs readout time of our framed ROIC operation,and this readout period acts as a hold-off time that mitigates any afterpulsing effects.III.C HARACTERIZATION T EST S ET-U PGmAPD camera characterization is carried out using the set-up illustrated schematically in Fig.2.The camera can be pow-ered by a15W supply with an input voltage at any value in the range of12to36V.The camera provides a trigger out-put(trig out)to drive an Avtech pulse generator that is used to pulse a laser diode.This trigger can be generated internally by the camera or injected from an external trigger source(Ext Trig).Gain-switched operation of the laser diode generates out-put pulses with a width ofß150ps,and output wavelengths of either1064or1550nm are used depending on which typeFig.2.Schematic layout of experimental apparatus for characterization of GmAPD cameras.PC:personal computer;GUI:graphical user interface;SSD: solid-state drive.of camera is under test.The pulsed diode output is transmitted through two attenuators before being spread by a collimator to a beam diameter ofß5mm with a uniformity of about5%. The collimator attenuates the optical density by approximately 40dB,and a mean photon number of0.1per100μm pitch pixel per pulse is achieved with20dB attenuation from At-ten1and0–10dB attenuation from Atten2.To obtain single pixel measurements,the set-up is modified by replacing the col-limator with a lensedfiber that has a5mm working distance andß10μm spot size.Both the collimator and the lensedfiber can be aligned with sub-micrometer precision using a remotely controlled X/Y/Z translation stage.The camera is connected to a personal computer(PC)using the industry-standard CameraLink protocol,and the frame grab-ber in the PC supports CameraLink operation in base,medium, and full configurations.Full configuration is required to estab-lish the necessary data transfer rate to support the full frame rate of182000Hz.The GUI on the PC allows comprehensive set-up and control of the camera,and this software supports real-time data storage at the maximum camera frame rate to SSDs in RAID0configuration.IV.F UNDAMENTAL DCR V ERSUS PDE T RADEOFFThe most fundamental tradeoff in the operation of GmAPDs is that between DCR and PDE.Higher PDE can be obtained by operating the detector at a larger excess bias,but only at the expense of consequently higher DCR.Both parameters are proportional to the avalanche probability P a,which increases with larger excess bias,and so tofirst order PDE and DCR will increase by the same proportional amount as the excess bias is raised.To the extent that the DCR includes electric field-mediated mechanisms—primarily trap-assisted tunneling effects[19]—the DCR will exhibit a larger rate of increase with excess bias than that of the PDE.The PDE performance of a32×32format GmAPD camera is conveniently summarized by plotting a spatial map ofPDE Fig.3.PDE data for sensor No.261at an operating point with average PDE= 30.5%,average DCR=2.2kHz,and T=248K.(a)Camera-level performance map illustrates PDE for each pixel,and(b)a histogram summarizes distribution of PDE performance of all1024pixels from(a).values obtained for all1024pixels,as shown in Fig.3(a)for an FPA sensor designated as No.261.Pixel-level PDE values are obtained from10000frames of data.During each frame, the array is illuminated by a short laser pulse ofß150ps du-ration that is collimated and calibrated to provide a mean pho-ton number ofμ=0.1photon per pixel area,as described in Section III.PDE for each pixel is determined by the number of counts observed and scaling forμ.(For other measurements in which larger values ofμare used,correcting for the Poisson probability of multiple photons per pixel per pulse is important to arrive at accurate PDE values.)The measured dark counts are subtracted from the illuminated measurements to obtain PDE values,but these PDE values do include crosstalk counts,which are quantified in the next section.The data presented in thefig-ure are obtained at an excess bias corresponding to a mean DCR of2.2kHz(see below)and an FPA temperature of248K.The corresponding distribution of PDE values is described by theFig.4.DCR data(in kHz)for sensor No.261at an operating point with average PDE=30.5%,average DCR=2.2kHz,and T=248K.(a)Camera-level performance map illustrates DCR for each pixel,and(b)a histogram summarizes distribution of DCR performance of all1024pixels from(a). histogram in Fig.3(b),for which the mean PDE is30.5%and the standard deviationσ(PDE)is4.0%.Under the operating conditions used to obtain the PDE per-formance map in Fig.3,we demonstrate the DCR performance for the same camera in the absence of illumination with the map of DCR values of all pixels of the FPA in Fig.4(a).These data are obtained from10000frames of data with the camera oper-ating at an average PDE of30.5%and at an FPA temperature of248K.Each frame had a duration of2μs,and the DCR was computed by dividing the total number of counts observed during the10000frames by the cumulative time of GmAPD operation(i.e.,10000×2μs=20000μs).(In the following section,we discuss the probability of missed counts due to the FPA capturing only one count per pixel per frame.)The distri-bution of DCR values is described by the histogram in Fig.4(b), for which the mean value is2.2kHz and the standard deviation σ(DCR)is0.4kHz.Fig.5.DCR data for18cameras corresponding to PDE=31±1%and operating temperature248±5K.Solid circles(•)indicate the average DCR over the32×32array and error bars indicate the standard deviation(±σ)of the DCR distribution.Spatial variations in pixel-level PDE and DCR across the FPA are generally dominated by systematic variations in the break-down voltage V b of the GmAPDs in each pixel.Because the same disarm voltage V d and5V CMOS bias voltage swing are applied to all pixels in the array,any variation in V b will result in a corresponding variation in excess bias V e(cf.discussion in Section II).A lower V e will lead to lower values for both PDE and DCR,and inspection of the performance maps in Figs.3 and4indicates that there is some systematic reduction in both of these parameters near the edges of the arrays,particularly the lower edge.This is the result of slight differences in fabrica-tion processes for pixels along the edges of the GmAPD PDA, and these variations can be reduced with modest design im-provements to compensate for dopant diffusion loading effects in future iterations of device processing.We also observe sys-tematic,fairly monotonic gradients in performance over longer length scales encompassing full arrays,and these originate from gradients in the properties of the epitaxial wafers used to fabri-cate the GmAPD PDAs.For the metal-organic chemical vapor deposition processes used to grow these wafers,variations in key structural attributes such as epitaxial layer thickness and doping concentration are fairly radial,as evidenced by wafer-level characterization techniques such as Fourier transform in-frared spectroscopy and photoluminescence,and these longer-scale performance variations can be correlated to the underlying wafer properties[20].To demonstrate the maturation of the GmAPD FPA perfor-mance,in Fig.5we summarize the DCR characteristics of18 cameras plotted in chronological order of fabrication and rep-resenting several fabrication lots of GmAPD PDAs.The solid circles indicate the average DCR of each array,and the error bars indicate the standard deviation(±σ)of the DCR distribu-tion for each array.The average DCR has tended to decrease appreciably,and the average ratio of the standard deviation to the mean isσ/DCRß0.3.In addition to inherent improvements in the DCR versus PDE tradeoff at the GmAPD device level,improved optical coupling of the MLA to the PDA allows a target PDE value(e.g.,30%)to be achieved at a lower excess bias with a consequently lower DCR.V.S TATISTICAL T EMPORAL A NALYSIS OF DCRAND C ROSSTALK E XTRACTIONIn the previous section,we presented a basic description of the DCR behavior of the GmAPD FPAs based on the number of frames for which each pixel exhibits a dark count.In this section, we show that we can extract a great deal more information about the measured dark counts by considering their timing information.In principle,dark counts possess the following attributes of a Poisson process[21]:(i)they are memoryless—i.e.,counts from non-overlapping time intervals are mutually independent;and(ii)for sufficiently small time intervals,the probability of a count is proportional to the duration of the time interval,and the probability of more than one count in this interval is negligible.Assuming that dark count occurrences are Poissonian in nature,we expect that the“inter-arrival”times between successive counts will obey an exponential distribution [21]given byf(t)=λe−λt/C(1) where t is the inter-arrival time,λis the average DCR,and the normalization constant C is the total number of counts and guarantees thatÞf(t)dt=1where the integration is performed over all t from0toÝ.This normalization can also be generalized to any sub-section of the measured data for T1≤t≤T2by ensuring thatT2T1f(t)dt=e T1−e T2(2)where C is now the number of counts in this section.Given a group of pixels that exhibit Poisson statistics,their collective behavior—such as the inter-arrival times for dark counts among all of the pixels—will also obey Poisson statistics [21].Noting this fact,we analyze dark count inter-arrival times from the entire array.We also consider all pixels of the array to be an ensemble of identical devices,and this assumption is rea-sonable as long as the standard deviation of the pixel-level DCR distribution is fairly small compared to the mean;for instance, as illustrated in Fig.4for sensor261,σ(DCR)/DCRß0.18.It is important to note that the accuracy of the DCR values obtained in the previous section—based on counting the number of frames for which each pixel exhibits a dark count—depends on the fact that,for each pixel,the probability of a dark count within a single2μs frame is sufficiently low.Each pixel can detect only a single avalanche event per frame,and if there were a significant probability of multiple dark counts per frame, all but thefirst dark count would be missed.However,for the average DCR of2.2kHz in Fig.4,the average time between counts is455μs,and the Poisson probability of more than one dark count[21]during a2μs range gate is<0.001%.Even for the camera with the highest average DCR of16kHz in Fig.5, the probability of a missed dark count on any frame isonly Fig.6.Statistical analysis of the inter-arrival times between consecutive dark counts occurring anywhere on a32×32GmAPD array with an InGaAsP absorber forß1μm sources.Data are from sensor No.261and are normalized as described in the text.The straight-line exponentialfit is from Eq.(1).The inset shows details of data between0to10ns,particularly the peak atß1ns.0.05%.The probability of more than one dark count per2μs range gate exceeds1%only for DCR>75kHz.A.Temporal Analysis of1.0μm FPAsWe proceed with a statistical analysis of the DCR data from 10000frames as follows.From each frame,we order the dark counts collected from all of the pixels according to their time stamp values,and the elapsed time between each pair of succes-sive dark counts provides us with a set of inter-arrival times for that frame.The distribution of all inter-arrival times obtained from all frames is then plotted on a semi-log scale as in Fig.6. The timing resolution of the plot is0.25ns—i.e.,each plotted point corresponds to a0.25ns interval—as dictated by the time bin resolution of the time stamp counters in the array.Except for very short inter-arrival times,the distribution exhibits the expo-nential behavior expected of a Poisson process.An exponential fit according to(1)was obtained for the inter-arrival time data between25and450ns,with appropriate normalization provided by(2).Ideally,thisfit should yield identical values for the pre-factor and exponent,and the values found—2.64×10−3and 2.77×10−3,respectively—agree to within5%.Taking their average as a best estimate forλ,and noting that this is the DCR per nanosecond,we rescale to dark counts per second to obtain an array-level DCR ofß2.7×106Hz.Considering that this rate is for all1024pixels of the array,the DCR per pixel ofλ/1024 is2.6kHz.Based on the assumption of Poissonian behavior for this analysis,this value is the intrinsic pixel-level DCR of the FPA.We note that the value of2.2kHz obtained from Fig.4 agrees to withinß15%.We now consider the deviation of the distribution in Fig.6 from exponential behavior at very short inter-arrival times;this behavior is shown more clearly in the inset of thefigure.All dis-cernible deviation from the Poisson exponential behavior occurs for inter-arrival times<2ns,with a clear peak seen atß1ns.If。
MRS文献

European Journal of Radiology 67(2008)218–229ReviewThe principles of quantification applied toin vivo proton MR spectroscopyGunther Helms ∗MR-Research in Neurology and Psychiatry,Faculty of Medicine,University of G¨o ttingen,D-37075G¨o ttingen,GermanyReceived 27February 2008;accepted 28February 2008AbstractFollowing the identification of metabolite signals in the in vivo MR spectrum,quantification is the procedure to estimate numerical values oftheir concentrations.The two essential steps are discussed in detail:analysis by fitting a model of prior knowledge,that is,the decomposition of the spectrum into the signals of singular metabolites;then,normalization of these signals to yield concentration estimates.Special attention is given to using the in vivo water signal as internal reference.©2008Elsevier Ireland Ltd.All rights reserved.Keywords:MRS;Brain;Quantification;QAContents1.Introduction ............................................................................................................2192.Spectral analysis/decomposition..........................................................................................2192.1.Principles........................................................................................................2192.2.Statistical and systematic fitting errors ..............................................................................2212.3.Examples of analysis software......................................................................................2212.3.1.LCModel ................................................................................................2212.3.2.jMRUI...................................................................................................2213.Signal normalization ....................................................................................................2233.1.Principles........................................................................................................2233.2.Internal referencing and metabolite ratios............................................................................2233.3.External referencing...............................................................................................2233.4.Global transmitter reference........................................................................................2233.5.Local flip angle...................................................................................................2243.6.Coil impedance effects ............................................................................................2243.7.External phantom and local reference ...............................................................................2253.8.Receive only-coils ................................................................................................2253.9.Internal water reference............................................................................................2253.10.Partial volume correction.........................................................................................2264.Calibration .............................................................................................................2275.Discussion..............................................................................................................2286.Experimental ...........................................................................................................2287.Recommendations.......................................................................................................228Acknowledgements .....................................................................................................229References .............................................................................................................229∗Tel.:+495513913132;fax:+495513913243.E-mail address:ghelms@gwdg.de .0720-048X/$–see front matter ©2008Elsevier Ireland Ltd.All rights reserved.doi:10.1016/j.ejrad.2008.02.034G.Helms/European Journal of Radiology67(2008)218–2292191.IntroductionIn vivo MRS is a quantitative technique.This statement is often mentioned in the introduction to clinical MRS studies. However,the quantification of signal produced by the MR imag-ing system is a complex and rather technical issue.Inconsistent terminology and scores of different approaches make the prob-lem appear even more complicated,especially for beginners. This article is intended to give a structured introduction to the principles of quantification.The associated problems and pos-sible systematic errors(“bias”)are explained to encourage a critical appraisal of published results.Quantification is essential for clinical research,less so for adding diagnostic information for which visual inspection often may suffice.Subsequent to the identification of metabolites,its foremost rationale is to provide numbers for comparison of spec-tra from different subjects and brain regions;and–ideally–different scanners and sequences.These numbers are then used for evaluation;e.g.statistical comparison of cohorts or correla-tion with clinical parameters.The problem is that the interaction of the radio-frequency(RF)hardware and the dielectric load of the subject’s body may lead to rather large signal variations(up to30%)that may blur systematic relationships to cohorts or clinical parameters.One of the purposes of quantification is to reduce such hardware related variation in the numbers.Thus, quantification is closely related to quality assurance(QA).In summary,quantification is a procedure of data processing. The post-processing scheme may require additional data acqui-sitions or extraction of adjustment parameters from the scanner. The natural order of steps in the procedure is1.acquisition and pre-processing of raw data,reconstruction ofthe spectrum(e.g.averaging and FFT),2.analysis:estimation of the relative signal for each identifiedmetabolite(here,proton numbers and linewidth should be taken into account),3.normalization of RF-induced signal variations,4.calibration of signals by performing the quantificationscheme on a standard of known concentration.In turn,these steps yield the metabolite signals1.for visual inspection of the displayed spectrum on the ppmscale,2.in arbitrary units,from which metabolite ratios can be cal-culated,3.in institutional units(for your individual MR scanner andquantification scheme;these numbers are proportional to the concentration),4.in absolute units of concentration(commonly inmM=mmol/l);estimated by comparison to a standard of known concentration.The term quantification(or sometimes“quantitation”)is occasionally used to denote singular steps of this process.In this review,it will refer to the whole procedure,and further differ-entiation is made for the sake of clarity.In practice,some these steps may be performed together.Already at this stage it should be made clear that the numbers obtained by“absolute quantifica-tion”are by no means“absolute”but depend on the accuracy and precision of steps1–4.Measurement and reconstruction(step1) must be performed in a consistent way lest additional errors have to be accounted for in individual experiments.Only in theory it should be possible to correct all possible sources of variation;in clinical practice it is generally is too time consum-ing.Yet the more sources of variation are cancelled(starting with the biggest effects)the smaller effects one will be able to detect.Emphasis will be put on the analysis(the models and the automated tools available),the signal normalization(and basic quality assurance issues),and the use of the localized water signal as internal reference.2.Spectral analysis/decomposition2.1.PrinciplesThe in vivo spectrum becomes more complicated with decreasing echo time(TE):next to the singlet resonances and weakly coupled multiplets,signals from strongly coupled metabolites and baseline humps from motion-restricted macro-molecules appear.Contrary to long-TE spectra short-TE spectra should not be evaluated step-by-step and line-by-line.For exam-ple,the left line of the lactate doublet is superposed onto the macromolecular signal at1.4ppm.The total signal at this fre-quency is not of interest but rather the separate contributions of lactate and macromolecules/lipids.Differences between the two whole resonance patterns can be used to separate the metabolites;e.g.the doublet of lactate versus the broad linewidth.In visual inspection,one intuitively uses such‘prior knowledge’about the expected metabolites to discern partly overlying metabolites in a qualitative way.This approach is also used to simplify the problem to automaticallyfind the metabolite resonances to order to evaluate the whole spectrum“in one go”.Comparing the resonance pattern of MR spectra in vivo at highfield and short TE with those of tissue extracts and sin-gle metabolites in vitro at matchedfield strengths hasfirmly established our‘prior’knowledge about which metabolites con-tribute to the in vivo MR spectra[1].Next to TE,thefield strength exerts the second biggest influence on the appearance of in vivo MR spectra.Overlap and degeneration of binomial multiplets due to strong coupling increase at the lowerfield strengths of clinical MR systems(commonly3,2,or1.5T). These effects can be either measured on solutions of single metabolites[2]or simulated fromfirst quantum-mechanical principles,once the chemical shifts and coupling constants(J in Hz)of a certain metabolite have been determined at suffi-ciently highfield[3].Motion-restricted‘macromolecules’are subject to rapid relaxation that blurs the coupling pattern(if the linewidth1/πT∗2>J)and hampers the identification of specific compounds.These usually appear as broad‘humps’that form the unresolved baseline of short-TE spectra(Fig.1).These vanish at longer TE(>135ms).The baseline underlying the metabo-220G.Helms /European Journal of Radiology 67(2008)218–229Fig.1.Including lipids/macromolecules into the basis set.Without inclusion of lipids/macromolecules in the basis set (A)the broad “humps”at 1.3and 0.9ppm are fitted by the baseline.Inclusion of lipids/macromolecules (B)resulted in a better fit and a lower baseline between 2.2and 0.6ppm.The SNR improved from 26to 30.The signals at 2.0ppm partly replaced the co-resonating tNAA.The 6%reduction in tNAA was larger than the fitting error (3%).This may illustrate that the fitting error does not account for the bias in the model.LCModel (exp.details:6.1-0;12.5ml VOI in parietal GM,3T,STEAM,TE/TM/TR/avg =20/10/6000/64).lite signals is constituted from all rapidly relaxing signals that have not decayed to zero at the chosen TE (macromolecules and lipids),the “feet”of the residual water signal,plus possible arte-facts (e.g.echo signals from moving spins that were not fully suppressed by gradient selection).The ‘prior knowledge’about which metabolites to detect and how the baseline will look like is used to construct a math-ematical model to describe the spectrum.Selecting the input signals reduces the complexity of the analysis problem.In con-trast to integrating or fitting singlet lines the whole spectrum is evaluated together (“in one go”)by fitting a superposition of metabolite signals and baseline signals.Thus,the in vivo spec-trum is decomposed into the constituents of the model.Without specifying the resonances this is often too complicated to be per-formed successfully,in the sense that an unaccountable number of ‘best’combinations exist.G.Helms/European Journal of Radiology67(2008)218–229221Prior knowledge may be implemented in the metabolite basis set adapting experimental data(like in LCModel[2]),theoretical patterns simulated fromfirst principles(QUEST[4]),or purely phenomenological functions like a superposition of Gaussians of different width to model strongly coupled signals and baseline humps alike(AMARES[5]).The least squaresfit may be per-formed in either time domain[6]or frequency domain or both [7].For an in-depth discussion of technical details,the reader is referred to a special issue of NMR in Biomedicine(NMR Biomed14[4];2001)dedicated to“quantitation”(in the sense of spectrum analysis)by mathematical methods.2.2.Statistical and systematicfitting errorsModelfitting yields the contribution of each input signal. Usually Cr´a mer–Rao lower bounds(CLRB)are provided as an estimate for thefitting error or the statistical uncertainty of the concentration estimate.These are calculated from the residual error and the Fisher matrix of the partial derivatives of the con-centrations.In the same way,correlations between the input data can be estimated.Overlapping input signals(e.g.from glutamate (Glu)and glutamine(Gln))are inversely correlated.In this case, the sum has a smaller error than the single metabolites.The uncertainties are fairly well proportional to the noise level(both must be given in the same units).The models are always an approximate,but never a com-plete description of the in vivo MR spectrum.Every model thus involves some kind of systematic error or“bias”,in the sense of deviation from the unknown“true”concentration.Contrary to the statistical uncertainty,the bias cannot be assessed within the same model.In particular,the CRLB does not account for the bias.Changes in the model(e.g.,by leaving out a minor metabo-lite)may result in systematic differences that soon become significant(by a paired t-test).These are caused by the pro-cess of minimizing the squared residual difference whenfitting the same data by two different models.Spurious artefacts or“nuisance signals”that are not included in the model will results in errors that are neither statistical nor systematic.It is also useful to know,that for every non-linear function(as used in MRS)there is a critical signal-to-noise (SNR)threshold for convergence onto meaningful values.2.3.Examples of analysis softwareA number of models and algorithms have been published dur-ing the past15years.A few are available to the public and shared by a considerable number of users.These program packages are generally combined with some automated or interactive pre-processing features,such as correction of frequency offset,zero andfirst order,as well as eddy-current induced phase errors.We shall in brief describe the most common programs for analysis of in vivo1H MRS data.2.3.1.LCModelThe Linear Combination Model(LCModel)[2]comes as stand-alone commercial software(/ lcmodel).It comprises automated pre-processing to achieve a high degree of user-independence.An advanced regularization ensures convergence for the vast majority of in vivo spectra.It was thefirst program designed tofit a basis set(or library)of experimental single metabolite spectra to incorporate maximum information and uniqueness.This means that partly overlap-ping spectra(again such as,Glu and Gln)are discerned by their unique features,but show some residual correlation as mentioned above.Proton numbers are accounted for,even“frac-tional proton numbers”in“pseudo-singlets”(e.g.,the main resonance of mIns).Thus,the ratios provided by LCModel refer to the concentrations rather than proton numbers.The basis set of experimental spectra comprises the prior information on neurochemistry(metabolites)as well as technique(TE,field strength,localization technique).The non-analytic line shape is constrained to unit area and capable tofit even distorted lines (due to motion or residual eddy currents).The number of knots of the baseline spline increases with the noise level.Thus,the LCModel is a mixture of experimental and phenomenological features.Although the basis spectra are provided in time domain, the evaluation is performed across a specified ppm interval.LCModel comes with a graphical user interface for routine application.Optionally the water signal may be used as quan-tification reference.Recently,lipids and macromolecular signals have been included to allow evaluation of tumour and muscle spectra.An example is shown in Fig.1.LCModel comprises basic signal normalization(see below) according to the global transmitter reference[8]to achieve a consistent scaling of the basis spectra.An in-house acquired basis set can thus be used to estimate absolute concentrations. Imported basis sets are available for a wide range of scanners and measurement protocols,but require a calibration to match the individual sensitivity(signal level)of the MR system[9]. Owing to LCModel’sflexibility,the basis set may contain also simulated spectra or an experimentally determined baseline to account for macromolecular signals.Such advanced applica-tions require good theoretical understanding and some practical experience.Care must be taken to maintain consistent scaling when adding new metabolite spectra to an existing basis.This is easiest done by cross-evaluation,that is evaluating a reference peak(e.g.,formate)in spectrum to be included by the singlet of the original basis and correcting to the known value.Caveat:The fact that LCModel converges does not ensure reliability of the estimates;least in absolute units(see Sections 3and4).Systematic difference in SNR may translate into bias via the baseline spline(see Fig.2).The same may be due an inconsistent choice of the boundaries of the ppm interval,partic-ularly next to the water resonance.In particular,with decreasing SNR(lower than4)one may observe more often systematically low or high concentrations.This is likely due to the errors in the feet of the non-analytical line shape,as narrow lines lead to underestimation and broad lines to overestimation.The metabo-lite ratios are still valid,as all model spectra are convoluted by the same lineshape.2.3.2.jMRUIThe java-based MR user interface for the processing of in vivo MR-spectra(jMRUI)is provided without charge222G.Helms /European Journal of Radiology 67(2008)218–229Fig.2.Systematic baseline differences between low and high SNR.Single spectrum from an 1.7ml VOI in white matter of the splenium (A)and the averaged spectra of seven healthy subjects (B).Note how the straight baseline leads to a severe underestimation of all metabolites except mIns.Differences were most prominent for Glu +Gln:3.6mM (43%)in a single subject vs.6.7mM (7%)in the averaged spectrum.(http://www.mrui.uab.es/mrui/mrui Overview.shtml ).It comes with a wide range of pre-processing features and interac-tive graphical software applications,including linear prediction and a powerful water removal by Hankel–Laclosz single value decomposition (HLSVD).In contrast to LCModel,it is designed to support user interaction.Several models for analy-sis/evaluation have been implemented in jMRUI,in particular AMARES [5]and QUEST [4].These focus on time-domain analysis,including line shape conversion,time-domain filter-ing and eddy-current deconvolution.Note that in the context of jMRUI ‘quantitation’refers to spectrum analysis.The pre-processing steps may exert a systematic influence on the results of model fitting.jMRUI can handle large data sets as from time-resolved MRS,two-dimensional MRS,and spatially resolved MRS,so-called MR spectroscopic imaging (MRSI)or chemical-shift imaging (CSI).G.Helms/European Journal of Radiology67(2008)218–2292233.Signal normalization3.1.PrinciplesThe signal is provided in arbitrary units of signed integer numbers,similar to MRI,and then converted tofloating complex numbers.In addition to scaling along the scanner’s receiver line, the proportionality between signal strength and number of spins per volume is strongly influenced by interaction of the RF hard-ware and its dielectric and conductive load,the human body.It is the correction of this interaction that forms the non-trivial part of signal normalization.Signal normalization is mainly applied to single-volume MRS,since spatially resolved MRSI poses addi-tional technical problems that are not part of this review.For sake of simplicity we assume homogeneous conditions across the whole volume-of-interest(VOI).Normalization consists of multiplications and divisions that render the signal,S,proportional to the concentration(of spins), C.Regardless whether in time domain(amplitude)or frequency domain(area),the signal is proportional to the size V of the VOI and the receiver gain R.S∼CVR or(1a) S/V/R∼C(1b) Logarithmic(decibel)units of the receiver gain must be con-verted to obtain a linear scaling factor,R.If R can be manually changed,it is advisable to check whether the characteristic of S(R)follows the assumed dependence.If a consistent(often the highest possible)gain used by default for single voxel MRS, one does not have to account for R.Correction of V for partial volume effects is discussed below.The proportionality constant will vary under the influence of the specific sample“loading”the RF coil.The properties of a loaded transmit–receive(T/R)coil are traditionally assessed by measuring the amplitude(or width)of a specific RF pulse,e.g., a180◦rectangular pulse.This strategy may also be pursued in vivo.The signal theory for T/R coils is given in concise form in [10]without use of complex numbers.Here,we develop it by presenting a chronology of strategies of increasing complexity that have been used for in vivo quantification.3.2.Internal referencing and metabolite ratiosBy assuming a concentration C int for the signal(S int)of ref-erence substance acquired in the same VOI,one has not to care about the influence of RF or scanner parameters:SS intC int=C(2)When using the total creatine(tCr)signal,internal referencing is equivalent to converting creatine ratios to absolute units.In early quantification work,the resonance of tCr has been assigned to 10mM determined by biochemical methods[11].However,it turned out that the MRS estimates of tCr are about25%lower and show some spatial dependence.In addition,tCr may increase in the presence of gliosis.3.3.External referencingThe most straightforward way is to acquire a reference sig-nal from an external phantom during the subject examination, with C ext being the concentration of the phantom substance [12,13].The reference signal S ext accounts for any changes in the proportionality constant.It may be normalized like the in vivo signal:S(VR)C extS ext/(V ext R ext)=C(3)If,however,the phantom is placed in the fringefield of the RF receive coil,the associated reduction in S ext will result in an overestimation of C.Care has to be taken to mount the external phantom reproducibly into the RF coil if this bias cannot be corrected otherwise.3.4.Global transmitter referenceAlready in high-field MR spectrometers it has been noticed that by coil load the sample influences both the transmit pulse and the signal:a high load requires a longer RF pulse for a 90◦excitation,which then yields reciprocally less signal from the same number of spins.This is the principle-of-reciprocity (PoR)for transmit/receive(T/R)coils in its most rudimentary form.It has been applied to account for the coil load effect, that is,large heads giving smaller signals than small heads [8].On MRI systems,RF pulses are applied with constant duration and shape.A high load thus requires a higher volt-age U tra(or transmitter gain),as determined during pre-scan calibration.S/V/R∼Ctraor(4a) S U tra/V/R∼C(4b)Of course,U tra must always refer to a pulse of specific shape, duration andflip angle,as used forflip angle calibration.On Siemens scanners,the amplitude of a non-selective rectangu-lar pulse(rect)is used.The logarithmic transmitter gain of GE scanners is independent of the RF pulse,but has to be converted from decibel to linear units[9].Normalization by the PoR requires QA at regular intervals,as the proportionality constant in Eqs.((4a)and(4b))may change in time.This may happen gradually while the performance of the RF power amplifier wears down,or suddenly after parts of the RF hardware have been replaced.For this purpose,the MRS protocol is run on a stable QA phantom of high concentration and the concentration estimate C QA(t i)obtained at time point, t i,is used to refer any concentration C back to time point zero byC→C C QA(t0)C QA(t i)(5)An example of serial QA monitoring is given in Fig.3.224G.Helms /European Journal of Radiology 67(2008)218–229Fig.3.QA measurement of temporal variation.Weekly QA performed on stable phantom of 100mM lactate and 100mM acetate from January 1996to June 1996.The standard single-volume protocol and quantification procedure (LCModel and global reference)were applied.(A)The mean estimated concentration is shown without additional calibration.The A indicates the state after installation,B a gradual breakdown of the system;the sudden jumps were due to replacement of the pre-amplifier (C and D)or head-coil (E),and retuning of the system (F).Results were used to correct proportionality to obtain longitudinally consistency.(B)The percentage deviation from the preceding measurement in Shewhart’s R-diagram indicates the weeks when quantification may not be reliable (data courtesy of Dr.M.Dezortov´a ,IKEM,Prague,Czech Republic).3.5.Local flip angleDanielsen and Hendriksen [10]noted that the PoR is a local relationship,so they used the amplitude of the water suppression pulse,U tra (x ),that had been locally adjusted on the VOI signal.S (x )U tra (x )/V/R ∼C(6)The local transmitter amplitude may also be found be fitting the flip angle dependence of the local signal [14].The example in Fig.4illustrates the consistency of Eq.(6)at the centre (high signal,low voltage)and outside (low signal,high voltage)the volume headcoil.Fig.4.Local verification of the principle of reciprocity.Flip angle dependence of the STEAM signal measured at two positions along the axis of a GE birdcage head-coil by varying the transmitter gain (TG).TG was converted from logarith-mic decibel to linear units (linearized TG,corresponding to U tra ).At coil centre (×)and 5cm outside the coil (+)the received signal,S (x ),was proportional to the transmitted RF,here given by 1/lin TG(x )at the signal maximum or 90◦flip angle.Like in large phantoms,there are considerable flip angle devi-ations across the human head as demonstrated at 3T in Fig.5a [15].The local flip angle,α(x ),may be related to the nominal value,αnom ,by α(x )=f (x )αnom(7)The spatially dependent factor is reciprocal to U tra (x ):f (x )∼1/U tra (x ).The flip angle will also alter the local signal.If a local transmitter reference is used,S (x )needs to be corrected for excitation effects.For the ideal 90◦–90◦–90◦STEAM local-ization and 90◦–180◦–180◦PRESS localization in a T/R coil,the signals areS (x )STEAM ∼M tr (x )∼C2f (x )sin 3(f (x )90◦)(8a)S (x )PRESS ∼M tr (x )∼C f (x )sin 5(f (x )90◦)(8b)The dependence of S (x )was simulated for a parabolic RF profile.A constant plateau is observed as the effects of transmission and reception cancel out for higher flip angles in the centre of the head where the VOI is placed.This is the reason why the global flip angle method works even in the presence of flip angle inhomogeneities.Note that the signal drops rapidly for smaller flip angles,i.e.close to the skull.3.6.Coil impedance effectsOlder quantification studies were performed on MR systems where the coil impedance Z was matched to 50 [8,10].Since the early 1990s,most volume head coils are of the high Q design and approximately tuned and matched by the RF load of the head and the stray capacitance of the shoulders.The residual variation of the impedance Z will affect the signal by S (x )U tra (x )/V/R ∼CZ(9)G.Helms/European Journal of Radiology67(2008)218–229225Fig.5.Flip angle inhomogeneities across the human brain.(Panel A)T1-w sagittal view showing variation in the RFfield.Flip angles are higher in the centre of the brain.The contours correspond to80–120◦localflip angle for a nominal value of90◦.(Panel B)The spatial signal dependence of STEAM and PRESS was simulated for a parabolicflip angle distribution with a maximum of115%relative to the global transmitter reference.This resulted in a constant signal obtained from the central regions of the brain,and a rapid decline at the edges.Reflection losses due to coil mismatch are symmetric in trans-mission and reception and are thus accounted for by U tra.These are likely to occur with exceptionally large or small persons (infants)or with phantoms of insufficient load.3.7.External phantom and local referenceWhen the impedance is not individually matched to50 , the associated change in proportionality must be monitored by a reference signal.In aqueous phantoms,the water signal can be used as internal reference.For in vivo applications,one may resort to an extra measurement in an external phantom[14].An additionalflip angle calibration in the phantom will account for local differences in the RFfield,especially if the phantom is placed in the fringe RFfield:SU tra(x)/(VR)S ext U tra(x ext)/(V ext R ext)C ext=C(10)This is the most comprehensive signal normalization.The com-bination of external reference and localflip angle method corrects for all effects in T/R coils.The reference signal accounts for changes in the proportionality,while the localflip angle cor-rects for RF inhomogeneity.Note also that systematic errors in S,U tra and V cancel out by division.Calibration of each individual VOI may be sped up by rapid RF mapping in three dimensions.3.8.Receive only-coilsThe SNR of the MRS signal can be increased by using sur-face coils or phased arrays of surface coils.The inhomogeneous receive characteristic cannot be mapped directly.The normaliza-tions discussed above(except Section3.2)cannot be performed directly on the received signal,as the coils are not used for trans-mission.Instead,the localized water signal may be acquired with both the receive coil and the body coil to scale the low SNR metabolite signal to obey the receive characteristics of the T/R body coil[16,17]:S rec met S bodywaterS rec water=S bodymet(11)For use with phased array coils it is essential that the metabolite and water signals are combined using consistent weights,since the low SNR of the water suppressed acquisition is most likely influenced by noise.3.9.Internal water referenceThe tissue water appears to be the internal reference of choice, due to its high concentration and well established values for water content of tissues(βper volume[18]):SS waterβ55mol/litre=C(12)It should be kept in mind that in vivo water exhibits a wide range of relaxation times,with the main component relaxing consider-able faster than the main metabolites.T2-times range from much shorter(myelin-associated water in white mater T2of15ms)to much longer(CSF,2400ms in bulk down to700ms in sulci with large surface-to-volume ratio).This implies an influence of TE on the concentration estimates.In addition,relaxation time and water content are subject to change in pathologies.Since the water signal is increasing in most pathologies(by content and relaxation),water referencing tends to give lower concentration estimates in pathologies.Ideally,the water signal should be determined by a multi-componentfit of the T2-decay curve[12].An easy but time-consuming way is to increase TE in consecutive fully relaxed single scans.A reliable way to determine the water sig-nal is tofit a2nd order polynomial through thefirst50ms of the magnitude signal(Fig.6).Thus,determining the amplitude cancels out initial receiver instabilities and avoids linefitting at an ill defined phase.If care is taken to avoid partial saturation by RF leakage from the water suppression pulses,this is consistent with multi-echo measurements using a CPMG MRI sequence [18](Fig.7).。
Quantization

QuantizationRobert M.Gray,Fellow,IEEE,and David L.Neuhoff,Fellow,IEEE(Invited Paper)Abstract—The history of the theory and practice of quan-tization dates to1948,although similar ideas had appearedin the literature as long ago as1898.The fundamental roleof quantization in modulation and analog-to-digital conversionwasfirst recognized during the early development of pulse-code modulation systems,especially in the1948paper of Oliver,Pierce,and Shannon.Also in1948,Bennett published thefirsthigh-resolution analysis of quantization and an exact analysis ofquantization noise for Gaussian processes,and Shannon pub-lished the beginnings of rate distortion theory,which wouldprovide a theory for quantization as analog-to-digital conversionand as data compression.Beginning with these three papers offifty years ago,we trace the history of quantization from itsorigins through this decade,and we survey the fundamentals ofthe theory and many of the popular and promising techniquesfor quantization.Index Terms—High resolution theory,rate distortion theory,source coding,quantization.I.I NTRODUCTIONT HE dictionary(Random House)definition of quantizationis the division of a quantity into a discrete numberof small parts,often assumed to be integral multiples ofa common quantity.The oldest example of quantization isrounding off,which wasfirst analyzed by Sheppard[468]for the application of estimating densities by histograms.Anyreal number,with a resulting quantization error so thatis ordinarily a collection of consecutive integersbeginning with,together with a set of reproductionvalues or points or levelsFig. 2.A uniform quantizer.If the distortion is measured by squarederror,into a binaryrepresentation or channel codeword of the quantizer index possible levels and all of thebinary representations or binary codewords have equal length (a temporary assumption),the binary vectors willneed (or the next largerinteger,,unless explicitly specified otherwise.In summary,the goal of quantization is to encode the data from a source,characterized by its probability density function,into as few bits as possible (i.e.,with low rate)in such a way that a reproduction may be recovered from the bits with as high quality as possible (i.e.,with small average distortion).Clearly,there is a tradeoff between the two primary performance measures:average distortion (or simply distortion ,as we will often abbreviate)and rate.This tradeoff may be quantified as the operational distortion-ratefunction or less.Thatis,or less,which is the inverseofor less.We will also be interested in thebest possible performance among all quantizers.Both as a preview and as an occasional benchmark for comparison,we informally define the class of all quantizers as the class of quantizers that can 1)operate on scalars or vectors instead of only on scalars (vector quantizers),2)have fixed or variable rate in the sense that the binary codeword describing the quantizer output can have length depending on the input,and 3)be memoryless or have memory,for example,using different sets of reproduction levels,depending on the past.In addition,we restrict attention to quantizers that do not change with time.That is,when confronted with the same input and the same past history,a quantizer will produce the same output regardless of the time.We occasionally use the term lossy source code or simply code as alternatives to quantizer .The rate is now defined as the average number of bits per source symbol required to describe the corresponding reproduction symbol.We informally generalize the operational distortion-ratefunctionor less.ThusGRAY AND NEUHOFF:QUANTIZATION2327for special nonasymptotic cases,such as Clavier,Panter, and Grieg’s1947analysis of the spectra of the quantization error for uniformly quantized sinusoidal signals[99],[100], and Bennett’s1948derivation of the power spectral density of a uniformly quantized Gaussian random process[43]. The most important nonasymptotic results,however,are the basic optimality conditions and iterative-descent algorithms for quantizer design,such asfirst developed by Steinhaus(1956) [480]and Lloyd(1957)[330],and later popularized by Max (1960)[349].Our goal in the next section is to introduce in historical context many of the key ideas of quantization that originated in classical works and evolved over the past50years,and in the remaining sections to survey selectively and in more detail a variety of results which illustrate both the historical development and the state of thefield.Section III will present basic background material that will be needed in the remainder of the paper,including the general definition of a quantizer and the basic forms of optimality criteria and descent algorithms. Some such material has already been introduced and more will be introduced in Section II.However,for completeness, Section III will be largely self-contained.Section IV reviews the development of quantization theories and compares the approaches.Finally,Section V describes a number of specific quantization techniques.In any review of a large subject such as quantization there is no space to discuss or even mention all work on the subject. Though we have made an effort to select the most important work,no doubt we have missed some important work due to bias,misunderstanding,or ignorance.For this we apologize, both to the reader and to the researchers whose work we may have neglected.II.H ISTORYThe history of quantization often takes on several parallel paths,which causes some problems in our clustering of topics. We follow roughly a chronological order within each and order the paths as best we can.Specifically,we willfirst track the design and analysis of practical quantization techniques in three paths:fixed-rate scalar quantization,which leads directly from the discussion of Section I,predictive and transform coding,which adds linear processing to scalar quantization in order to exploit source redundancy,and variable-rate quantiza-tion,which uses Shannon’s lossless source coding techniques [464]to reduce rate.(Lossless codes were originally called noiseless.)Next we follow early forward-looking work on vector quantization,including the seminal work of Shannon and Zador,in which vector quantization appears more to be a paradigm for analyzing the fundamental limits of quantizer performance than a practical coding technique.A surprising amount of such vector quantization theory was developed out-side the conventional communications and signal processing literature.Subsequently,we review briefly the developments from the mid-1970’s to the mid-1980’s which mainly concern the emergence of vector quantization as a practical technique. Finally,we sketch briefly developments from the mid-1980’s to the present.Except where stated otherwise,we presume squared error as the distortion measure.A.Fixed-Rate Scalar Quantization:PCM and the Origins of Quantization TheoryBoth quantization and source coding with afidelity crite-rion have their origins in pulse-code modulation(PCM),a technique patented in1938by Reeves[432],who25years later wrote a historical perspective on and an appraisal of the future of PCM with Deloraine[120].The predictions were surprisingly accurate as to the eventual ubiquity of digital speech and video.The technique wasfirst successfully imple-mented in hardware by Black,who reported the principles and implementation in1947[51],as did another Bell Labs paper by Goodall[209].PCM was subsequently analyzed in detail and popularized by Oliver,Pierce,and Shannon in1948[394]. PCM was thefirst digital technique for conveying an analog information signal(principally telephone speech)over an analog channel(typically,a wire or the atmosphere).In other words,it is a modulation technique,i.e.,an alternative to AM, FM,and various other types of pulse modulation.It consists of three main components:a sampler(including a prefilter),a quantizer(with afixed-rate binary encoder),and a binary pulse modulator.The sampler converts a continuous-timewaveform into a sequence ofsamples,whereand the high-frequency power removed by the lowpassfilter.The binary pulse modulator typically uses the bits produced by the quantizer to determine the amplitude,frequency,or phase of a sinusoidal carrier waveform.In the evolutionary development of modulation techniques it was found that the performance of pulse-amplitude modulation in the presence of noise could be improved if the samples were quantized to the nearest of a setoflevels had been transmitted in the presence of noise could be done with such reliability that the overall MSE was substantially reduced.Reducing the number of quantizationlevelsat a value giving acceptably small quantizer MSE and to binary encode the levels,so that the receiver had only to make binary decisions,something it can do with great reliability.The resulting system,PCM,had the best resistance to noise of all modulations of the time.As the digital era emerged,it was recognized that the sampling,quantizing,and encoding part of PCM performs an analog-to-digital(A/D)conversion,with uses extending much beyond communication over analog channels.Even in the communicationsfield,it was recognized that the task of analog-to-digital conversion(and source coding)should be factored out of binary modulation as a separate task.Thus2328IEEE TRANSACTIONS ON INFORMATION THEORY,VOL.44,NO.6,OCTOBER1998 PCM is now generally considered to just consist of sampling,quantizing,and encoding;i.e.,it no longer includes the binarypulse modulation.Although quantization in the information theory literatureis generally considered as a form of data compression,itsuse for modulation or A/D conversion was originally viewedas data expansion or,more accurately,bandwidth expansion.For example,a speech waveform occupying roughly4kHzwould have a Nyquist rate of8kHz.Sampling at the Nyquistrate and quantizing at8bits per sample and then modulatingthe resulting binary pulses using amplitude-or frequency-shiftkeying would yield a signal occupying roughly64kHz,a16–fold increase in bandwidth!Mathematically this constitutescompression in the sense that a continuous waveform requiringan infinite number of bits is reduced to afinite number of bits,but for practical purposes PCM is not well interpreted as acompression scheme.In an early contribution to the theory of quantization,Clavier,Panter,and Grieg(1947)[99],[100]applied Rice’scharacteristic function or transform method[434]to provideexact expressions for the quantization error and its momentsresulting from uniform quantization for certain specific inputs,including constants and sinusoids.The complicated sums ofBessel functions resembled the early analyses of anothernonlinear modulation technique,FM,and left little hope forgeneral closed-form solutions for interesting signals.Thefirst general contributions to quantization theory camein1948with the papers of Oliver,Pierce,and Shannon[394]and Bennett[43].As part of their analysis of PCM forcommunications,they developed the oft-quoted result that forlarge rate or resolution,a uniform quantizer with cellwidthlevels andrate,and the source has inputrange(or support)ofwidthdBshowing that for large rate,the SNR of uniform quantizationincreases6dB for each one-bit increase of rate,which is oftenreferred to as the“6-dB-per-bit rule.”Thefor companders,systems that preceded auniform quantizer by a monotonic smooth nonlinearity calleda“compressor,”saywas givenby is auniform quantizer.Bennett showed that in thiscaseis the cellwidth of the uniformquantizer,and the integral is taken over the granular range ofthe input.(Theconstantmaps to the unit intervalcan be interpreted,as Lloydwould explicitly point out in1957[330],as a constant timesa“quantizer point-densityfunctionnumber of quantizer levelsinover a region gives the fraction ofquantizer reproduction levels in the region,it is evidentthat,which when integratedoverrather than the fraction.In the currentsituationis infinite.Rewriting Bennett’s integral in terms of the point-densityfunction yields its more commonform(7)The idea of a quantizer point-density function will generalizeto vectors,while the compander approach will not in the sensethat not all vector quantizers can be represented as companders[192].Bennett also demonstrated that,under assumptions of highresolution and smooth densities,the quantization error behavedmuch like random“noise”:it had small correlation with thesignal and had approximately aflat(“white”)spectrum.Thisled to an“additive-noise”model of quantizer error,since withthese properties theformulaGRAY AND NEUHOFF:QUANTIZATION2329 is uniformly quantized,providing one of the very few exactcomputations of quantization error spectra.In1951Panter and Dite[405]developed a high-resolutionformula for the distortion of afixed-rate scalar quantizer usingapproximations similar to Bennett’s,but without reference toBennett.They then used variational techniques to minimizetheir formula and found the following formula for the opera-tional distortion-rate function offixed-rate scalar quantization:for large valuesof(9)Indeed,substituting this point density into Bennett’s integraland using the factthat yields(8).As an example,if the input density is Gaussian withvariance,thenasor less.(It was not until Shannon’s1959paper[465]thatthe rate is0.72bits/sample larger thanthat achievable by the best quantizers.In1957,Smith[474]re-examined companding and PCM.Among other things,he gave somewhat cleaner derivations of1They also indicated that it had been derived earlier by P.R.Aigrain.Bennett’s integral,the optimal compressor function,and thePanter–Dite formula.Also in1957,Lloyd[330]made an important study ofquantization with three main contributions.First,he foundnecessary and sufficient conditions for afixed-rate quantizer tobe locally optimal;i.e.,conditions that if satisfied implied thatsmall perturbations to the levels or thresholds would increasedistortion.Any optimal quantizer(one with smallest distortion)will necessarily satisfy these conditions,and so they are oftencalled the optimality conditions or the necessary conditions.Simply stated,Lloyd’s optimality conditions are that for afixed-rate quantizer to be optimal,the quantizer partition mustbe optimal for the set of reproduction levels,and the set ofreproduction levels must be optimal for the partition.Lloydderived these conditions straightforwardly fromfirst principles,without recourse to variational concepts such as derivatives.For the case of mean-squared error,thefirst condition impliesa minimum distance or nearest neighbor quantization rule,choosing the closest available reproduction level to the sourcesample being quantized,and the second condition implies thatthe reproduction level corresponding to a given cell is theconditional expectation or centroid of the source value giventhat it lies in the specified cell;i.e.,it is the minimum mean-squared error estimate of the source sample.For some sourcesthere are multiple locally optimal quantizers,not all of whichare globally optimal.Second,based on his optimality conditions,Lloyd devel-oped an iterative descent algorithm for designing quantizers fora given source distribution:begin with an initial collection ofreproduction levels;optimize the partition for these levels byusing a minimum distortion mapping,which gives a partitionof the real line into intervals;then optimize the set of levels forthe partition by replacing the old levels by the centroids of thepartition cells.The alternation is continued until convergenceto a local,if not global,optimum.Lloyd referred to thisdesign algorithm as“Method I.”He also developed a MethodII based on the optimality properties.First choose an initialsmallest reproduction level.This determines the cell thresholdto the right,which in turn implies the next larger reproductionlevel,and so on.This approach alternately produces a leveland a threshold.Once the last level has been chosen,theinitial level can then be rechosen to reduce distortion andthe algorithm continues.Lloyd provided design examplesfor uniform,Gaussian,and Laplacian random variables andshowed that the results were consistent with the high resolutionapproximations.Although Method II would initially gain morepopularity when rediscovered in1960by Max[349],it isMethod I that easily extends to vector quantizers and manytypes of quantizers with structural constraints.Third,motivated by the work of Panter and Dite butapparently unaware of that of Bennett or Smith,Lloyd re-derived Bennett’s integral and the Panter–Dite formula basedon the concept of point-density function.This was a criticallyimportant step for subsequent generalizations of Bennett’sintegral to vector quantizers.He also showed directly thatin situations where the global optimum is the only localoptimum,quantizers that satisfy the optimality conditionshave,asymptotically,the optimal point density given by(9).2330IEEE TRANSACTIONS ON INFORMATION THEORY,VOL.44,NO.6,OCTOBER1998Unfortunately,Lloyd’s work was not published in an archival journal at the time.Instead,it was presented at the1957Institute of Mathematical Statistics(IMS)meeting and appeared in print only as a Bell Laboratories Technical Memorandum.As a result,its results were not widely known in the engineering literature for many years,and many were independently rediscovered.All of the independent rediscoveries,however,used variational derivations,rather than Lloyd’s simple derivations.The latter were essential for later extensions to vector quantizers and to the development of many quantizer optimization procedures.To our knowledge, thefirst mention of Lloyd’s work in the IEEE literature came in 1964with Fleischer’s[170]derivation of a sufficient condition (namely,that the log of the source density be concave)in order that the optimal quantizer be the only locally optimal quantizer, and consequently,that Lloyd’s Method I yields a globally optimal quantizer.(The condition is satisfied for common densities such as Gaussian and Laplacian.)Zador[561]had referred to Lloyd a year earlier in his Ph.D.dissertation,to be discussed later.Later in the same year in another Bell Telephone Laborato-ries Technical Memorandum,Goldstein[207]used variational methods to derive conditions for global optimality of a scalar quantizer in terms of second-order partial derivatives with respect to the quantizer levels and thresholds.He also provided a simple counterintuitive example of a symmetric density for which the optimal quantizer was asymmetric.In1959,Shtein[471]added terms representing overload distortion totheth-power distortion measures, rediscovered Lloyd’s Method II,and numerically investigated the design offixed-rate quantizers for a variety of input densities.Also in1960,Widrow[529]derived an exact formula for the characteristic function of a uniformly quantized signal when the quantizer has an infinite number of levels.His results showed that under the condition that the characteristic function of the input signal be zero when its argument is greaterthanis a deterministic function of the signal.The“bandlimited”property of the characteristic function implies from Fourier transform theory that the probability density function must have infinite support since a signal and its transform cannot both be perfectly bandlimited.We conclude this subsection by mentioning early work that appeared in the mathematical and statistical literature and which,in hindsight,can be viewed as related to scalar quantization.Specifically,in1950–1951Dalenius et al.[118],[119]used variational techniques to consider optimal group-ing of Gaussian data with respect to average squared error. Lukaszewicz and H.Steinhaus[336](1955)developed what we now consider to be the Lloyd optimality conditions using variational techniques in a study of optimum go/no-go gauge sets(as acknowledged by Lloyd).Cox in1957[111]also derived similar conditions.Some additional early work,which can now be seen as relating to vector quantization,will be reviewed later[480],[159],[561].B.Scalar Quantization with MemoryIt was recognized early that common sources such as speech and images had considerable“redundancy”that scalar quantization could not exploit.The term“redundancy”was commonly used in the early days and is still popular in some of the quantization literature.Strictly speaking,it refers to the statistical correlation or dependence between the samples of such sources and is usually referred to as memory in the information theory literature.As our current emphasis is historical,we follow the traditional language.While not dis-rupting the performance of scalar quantizers,such redundancy could be exploited to attain substantially better rate-distortion performance.The early approaches toward this end combined linear processing with scalar quantization,thereby preserving the simplicity of scalar quantization while using intuition-based arguments and insights to improve performance by incorporating memory into the overall code.The two most important approaches of this variety were predictive coding and transform coding.A shared intuition was that a prepro-cessing operation intended to make scalar quantization more efficient should“remove the redundancy”in the data.Indeed, to this day there is a common belief that data compression is equivalent to redundancy removal and that data without redundancy cannot be further compressed.As will be discussed later,this belief is contradicted both by Shannon’s work, which demonstrated strictly improved performance using vec-tor quantizers even for memoryless sources,and by the early work of Fejes Toth(1959)[159].Nevertheless,removing redundancy leads to much improved codes.Predictive quantization appears to originate in the1946 delta modulation patent of Derjavitch,Deloraine,and Van Mierlo[129],but the most commonly cited early references are Cutler’s patent[117]2605361on“Differential quantization of communication signals”and on DeJager’s Philips technical report on delta modulation[128].Cutler stated in his patent that it“is the object of the present invention to improve the efficiency of communication systems by taking advantage of correlation in the signals of these systems”and Derjavitch et al.also cited the reduction of redundancy as the key to the re-duction of quantization noise.In1950,Elias[141]provided an information-theoretic development of the benefits of predictive coding,but the work was not published until1955[142].Other early references include[395],[300],[237],[511],and[572]. In particular,[511]claims Bennett-style asymptotics for high-resolution quantization error,but as will be discussed later, such approximations have yet to be rigorously derived. From the point of view of least squares estimation theory,if one were to optimally predict a data sequence based on its pastGRAY AND NEUHOFF:QUANTIZATION2331Fig.3.Predictive quantizer encoder/decoder.in the sense of minimizing the mean-squared error,then the resulting error or residual or innovations sequence would be uncorrelated and it would have the minimum possible variance. To permit reconstruction in a coded system,however,the prediction must be based on past reconstructed samples and not true samples.This is accomplished by placing a quantizer inside a prediction loop and using the same predictor to decode the signal.A simple predictive quantizer or differential pulse-coded modulator(DPCM)is depicted in Fig.3.If the predictor is simply the last sample and the quantizer has only one bit, the system becomes a delta-modulator.Predictive quantizers are considered to have memory in that the quantization of a sample depends on previous samples,via the feedback loop. Predictive quantizers have been extensively developed,for example there are many adaptive versions,and are widely used in speech and video coding,where a number of standards are based on them.In speech coding they form the basis of ITU-G.721,722,723,and726,and in video coding they form the basis of the interframe coding schemes standardized in the MPEG and H.26X prehensive discussions may be found in books[265],[374],[196],[424],[50],and[458],as well as survey papers[264]and[198].Though decorrelation was an early motivation for predictive quantization,the most common view at present is that the primary role of the predictor is to reduce the variance of the variable to be scalar-quantized.This view stems from the facts that a)it is the prediction errors rather than the source samples that are quantized,b)the overall quantization error precisely equals that of the scalar quantizer operating on the prediction errors,c)the operational distortion-ratefunctionresults in a scalingof,where is the variance of the sourceandthat is multiplied by an orthogonal matrix(an2332IEEE TRANSACTIONS ON INFORMATION THEORY,VOL.44,NO.6,OCTOBER1998Fig.4.Transform code.orthogonal transform)and the resulting transform coefficients are scalar quantized,usually with a different quantizer for each coefficient.The operation is depicted in Fig.4.This style of code was introduced in1956by Kramer and Mathews [299]and analyzed and popularized in1962–1963by Huang and Schultheiss[247],[248].Kramer and Mathews simply assumed that the goal of the transform was to decorrelate the symbols,but Huang and Schultheiss proved that decorrelating does indeed lead to optimal transform code design,at least in the case of Gaussian sources and high resolution.Transform coding has been extensively developed for coding images and video,where the discrete cosine transform(DCT)[7], [429]is most commonly used because of its computational simplicity and its good performance.Indeed,DCT coding is the basic approach dominating current image and video coding standards,including H.261,H.263,JPEG,and MPEG.These codes combine uniform scalar quantization of the transform coefficients with an efficient lossless coding of the quantizer indices,as will be considered in the next section as a variable-rate quantizer.For discussions of transform coding for images see[533],[422],[375],[265],[98],[374],[261],[424],[196], [208],[408],[50],[458],and More recently,transform coding has also been widely used in high-fidelity audio coding[272], [200].Unlike predictive quantizers,the transform coding approach lent itself quite well to the Bennett high-resolution approx-imations,the classical analysis being that of Huang and Schultheiss[247],[248]of the performance of optimized transform codes forfixed-rate scalar quantizers for Gaussian sources,a result which demonstrated that the Karhunen–Lo`e ve decorrelating transform was optimum for this application for the given assumptions.If the transform is the Karhunen–Lo`e ve transform,then the coefficients will be uncorrelated(and hence independent if the input vector is also Gaussian).The seminal work of Huang and Schultheiss showed that high-resolution approximation theory could provide analytical descriptions of optimal performance and design algorithms for optimizing codes of a given structure.In particular,they showed that under the high-resolution assumptions with Gaussian sources, the average distortion of the best transform code with a given rate is less than that of optimal scalar quantization bythefactor,where is the average of thevariances of the components of the source vectorandcovariance matrix.Note that this reduction indistortion becomes larger for sources with more memory(morecorrelation)because the covariance matrices of such sourceshave smaller determinants.Whenor less.Sincewe have weakened the constraint by expanding the allowedset of quantizers,this operational distortion-rate function willordinarily be smaller than thefixed-rate optimum.Huffman’s algorithm[251]provides a systematic methodof designing binary codes with the smallest possible averagelength for a given set of probabilities,such as those of thecells.Codes designed in this way are typically called Huffmancodes.Unfortunately,there is no known expression for theresulting minimum average length in terms of the probabilities.However,Shannon’s lossless source coding theorem impliesthat given a source and a quantizer partition,one can alwaysfind an assignment of binary codewords(indeed,a prefix set)with average length not morethan,where。
报考说明Application explanation

附件一AnnexⅠ报考说明Application explanation一、考试范围Scope1. 考试专业:渗透检测、磁粉检测、射线照相检测、超声检测Methods: PT 、MT、RT、UT2. 考试级别:Ⅲ级Level:III3. 考试科目:Subject考试科目包括基础考试、规范考试、实际操作考试三个科目。
规范考试一般为Nadcap“基础规范”,根据报考人的雇主需要可增加相应的“雇主规范”。
实际操作考试包括现场“检测操作”和“检测工艺”两项。
General/Specific(Nadcap’s specific & employer’s internal specification(s))/Practialtest(including writing a procedure)4. 考试对象:航空航天相关单位具有下列条件的无损检测人员Candidates:Aerospace employeea) 持有DiNDT Ⅲ级有效证书的人员Holders of current DiNDT Level III该类人员需要参加规范考试和实际操作考试(包括编写工艺规程)Who need take specific/practial test(include writing a procedure)b) 持有DiNDTⅡ级或 NAS410 Ⅱ级有效证书的人员(且实践经历符合要求)Holders of current DiNDT or NAS410 Level II(meet the experience requirements)该类人员需要参加基础考试、规范考试和实际操作考试(包括编写工艺规程)General/Specific/Practial test(including writing a procedure)c) 持有NAS410 Ⅲ级有效证书申请更新认证人员Holders of current NAS410 Level III in need of requalification该类人员仅需要参加规范考试和实际操作考试(包括编写工艺规程)Who only need take specific and practical test(include writing a procedure)d) DiNDT Ⅲ级或NAS410 Ⅲ级证书过期的人员Holders of expired DiNDT or NAS410 Level III需要参加基础考试、规范考试和实际操作考试(包括编写工艺规程)Who need take general/specific/practial test(including writing a procedure)e) 参加NANDTB-CN举办的Ⅲ级资格鉴定考试未通过的人员Re-examination of the failed exam二、报名材料:Application Materialsa) 资格鉴定申请表(见附件二),所有信息要填写齐全;(申请表必须加盖单位公章。
Field Theory and Standard Model
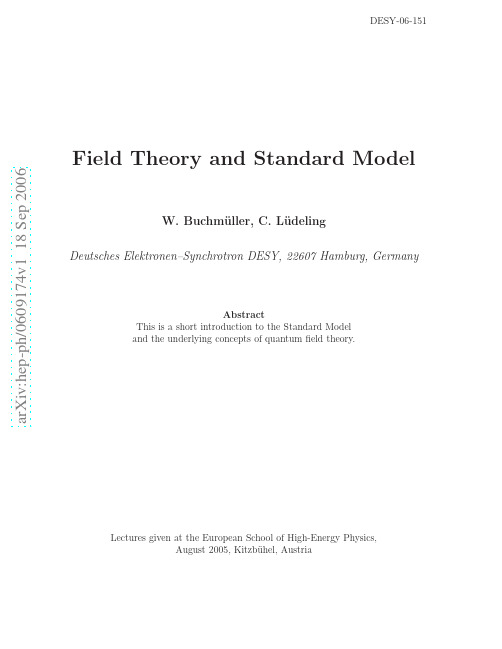
Abstract This is a short introduction to the Standard Model and the underlying concepts of quantum field theory.
Lectures given at the European School of High-Energy Physics, August 2005, Kitzb¨ uhel, Austria
Contents
1 Introduction 1.1 Theoretical Perspective . . . . . . . . . . . . . . . . . . . . . . . . . . . . 1.2 Phenomenological Aspects . . . . . . . . . . . . . . . . . . . . . . . . . . 2 Quantisation of Fields 2.1 Why Fields? . . . . . . . . . . . . . . . . . . . . 2.1.1 Quantisation in Quantum Mechanics . . 2.1.2 Special Relativity Requires Antiparticles 2.2 Multiparticle States and Fies, Creation and Annihilation . . . . 2.2.2 Charge and Momentum . . . . . . . . . 2.2.3 Field Operator . . . . . . . . . . . . . . 2.2.4 Propagator . . . . . . . . . . . . . . . . 2.3 Canonical Quantisation . . . . . . . . . . . . . . 2.4 Fermions . . . . . . . . . . . . . . . . . . . . . . 2.4.1 Canonical Quantisation of Fermions . . . 2.5 Interactions . . . . . . . . . . . . . . . . . . . . 2.5.1 φ4 Theory . . . . . . . . . . . . . . . . . 2.5.2 Fermions . . . . . . . . . . . . . . . . . . 3 Gauge Theories 3.1 Global Symmetries v Gauge Symmetries 3.2 Abelian Gauge Theories . . . . . . . . . 3.3 Non-Abelian Gauge Theories . . . . . . . 3.4 Quantisation . . . . . . . . . . . . . . . . 4 Quantum Corrections 4.1 Anomalous Magnetic Moment . . . 4.2 Divergences . . . . . . . . . . . . . 4.2.1 Dimensional Regularisation 4.2.2 Renormalisation . . . . . . . 4.2.3 Running Coupling in QED . 4.2.4 Running Coupling in QCD . . . . . . . . . . . . . . . . . . . . . . . . . . . . . . . . . . . . . . . . . . . . . . . . . . . . . . . . . . . . . . . . . . . . . . . . . . . . . . . . . . . . . . . . . . . . . . . . . . . . . . . . . . . . . . . . . . . . . . . . . . . . . . . . . . . . . . . . . . . . . . . . . . . . . . . . . . . . . . . . . . . . . . . . . . . . . . . . . . . . . . . . . . . . . . . . . . . . . . . . . . . . . . . . . . . . . . . . . . . . . . . . . . . . . . . . . . . . . . . . . . . . . . . . . . . . . . . . . . . . . . . . . . . . . . . . . . . . . . . . . . . . . . . . . . . . . . . . . . . . . . . . . . . . . . . . . . . . . . . . . . . . . . . . . . . . . . . . . . . . . . . . . . . . . . . . . . . . . . . . . . . . . . . . . . . . . . . . . . . . . . . . . . . . . . . . . . . 3 3 5 6 6 6 8 9 9 10 11 12 12 14 15 17 18 20 22 22 24 27 29 32 32 35 36 38 40 41
Quantum Computing for Computer Scientists

More informationQuantum Computing for Computer ScientistsThe multidisciplinaryfield of quantum computing strives to exploit someof the uncanny aspects of quantum mechanics to expand our computa-tional horizons.Quantum Computing for Computer Scientists takes read-ers on a tour of this fascinating area of cutting-edge research.Writtenin an accessible yet rigorous fashion,this book employs ideas and tech-niques familiar to every student of computer science.The reader is notexpected to have any advanced mathematics or physics background.Af-ter presenting the necessary prerequisites,the material is organized tolook at different aspects of quantum computing from the specific stand-point of computer science.There are chapters on computer architecture,algorithms,programming languages,theoretical computer science,cryp-tography,information theory,and hardware.The text has step-by-stepexamples,more than two hundred exercises with solutions,and program-ming drills that bring the ideas of quantum computing alive for today’scomputer science students and researchers.Noson S.Yanofsky,PhD,is an Associate Professor in the Departmentof Computer and Information Science at Brooklyn College,City Univer-sity of New York and at the PhD Program in Computer Science at TheGraduate Center of CUNY.Mirco A.Mannucci,PhD,is the founder and CEO of HoloMathics,LLC,a research and development company with a focus on innovative mathe-matical modeling.He also serves as Adjunct Professor of Computer Sci-ence at George Mason University and the University of Maryland.QUANTUM COMPUTING FORCOMPUTER SCIENTISTSNoson S.YanofskyBrooklyn College,City University of New YorkandMirco A.MannucciHoloMathics,LLCMore informationMore informationcambridge university pressCambridge,New York,Melbourne,Madrid,Cape Town,Singapore,S˜ao Paulo,DelhiCambridge University Press32Avenue of the Americas,New York,NY10013-2473,USAInformation on this title:/9780521879965C Noson S.Yanofsky and Mirco A.Mannucci2008This publication is in copyright.Subject to statutory exceptionand to the provisions of relevant collective licensing agreements,no reproduction of any part may take place withoutthe written permission of Cambridge University Press.First published2008Printed in the United States of AmericaA catalog record for this publication is available from the British Library.Library of Congress Cataloging in Publication dataYanofsky,Noson S.,1967–Quantum computing for computer scientists/Noson S.Yanofsky andMirco A.Mannucci.p.cm.Includes bibliographical references and index.ISBN978-0-521-87996-5(hardback)1.Quantum computers.I.Mannucci,Mirco A.,1960–II.Title.QA76.889.Y352008004.1–dc222008020507ISBN978-0-521-879965hardbackCambridge University Press has no responsibility forthe persistence or accuracy of URLs for external orthird-party Internet Web sites referred to in this publicationand does not guarantee that any content on suchWeb sites is,or will remain,accurate or appropriate.More informationDedicated toMoishe and Sharon Yanofskyandto the memory ofLuigi and Antonietta MannucciWisdom is one thing:to know the tho u ght by which all things are directed thro u gh allthings.˜Heraclitu s of Ephe s u s(535–475B C E)a s quoted in Dio g ene s Laertiu s’sLives and Opinions of Eminent PhilosophersBook IX,1. More informationMore informationContentsPreface xi1Complex Numbers71.1Basic Definitions81.2The Algebra of Complex Numbers101.3The Geometry of Complex Numbers152Complex Vector Spaces292.1C n as the Primary Example302.2Definitions,Properties,and Examples342.3Basis and Dimension452.4Inner Products and Hilbert Spaces532.5Eigenvalues and Eigenvectors602.6Hermitian and Unitary Matrices622.7Tensor Product of Vector Spaces663The Leap from Classical to Quantum743.1Classical Deterministic Systems743.2Probabilistic Systems793.3Quantum Systems883.4Assembling Systems974Basic Quantum Theory1034.1Quantum States1034.2Observables1154.3Measuring1264.4Dynamics1294.5Assembling Quantum Systems1325Architecture1385.1Bits and Qubits138viiMore informationviii Contents5.2Classical Gates1445.3Reversible Gates1515.4Quantum Gates1586Algorithms1706.1Deutsch’s Algorithm1716.2The Deutsch–Jozsa Algorithm1796.3Simon’s Periodicity Algorithm1876.4Grover’s Search Algorithm1956.5Shor’s Factoring Algorithm2047Programming Languages2207.1Programming in a Quantum World2207.2Quantum Assembly Programming2217.3Toward Higher-Level Quantum Programming2307.4Quantum Computation Before Quantum Computers2378Theoretical Computer Science2398.1Deterministic and Nondeterministic Computations2398.2Probabilistic Computations2468.3Quantum Computations2519Cryptography2629.1Classical Cryptography2629.2Quantum Key Exchange I:The BB84Protocol2689.3Quantum Key Exchange II:The B92Protocol2739.4Quantum Key Exchange III:The EPR Protocol2759.5Quantum Teleportation27710Information Theory28410.1Classical Information and Shannon Entropy28410.2Quantum Information and von Neumann Entropy28810.3Classical and Quantum Data Compression29510.4Error-Correcting Codes30211Hardware30511.1Quantum Hardware:Goals and Challenges30611.2Implementing a Quantum Computer I:Ion Traps31111.3Implementing a Quantum Computer II:Linear Optics31311.4Implementing a Quantum Computer III:NMRand Superconductors31511.5Future of Quantum Ware316Appendix A Historical Bibliography of Quantum Computing319 by Jill CirasellaA.1Reading Scientific Articles319A.2Models of Computation320More informationContents ixA.3Quantum Gates321A.4Quantum Algorithms and Implementations321A.5Quantum Cryptography323A.6Quantum Information323A.7More Milestones?324Appendix B Answers to Selected Exercises325Appendix C Quantum Computing Experiments with MATLAB351C.1Playing with Matlab351C.2Complex Numbers and Matrices351C.3Quantum Computations354Appendix D Keeping Abreast of Quantum News:QuantumComputing on the Web and in the Literature357by Jill CirasellaD.1Keeping Abreast of Popular News357D.2Keeping Abreast of Scientific Literature358D.3The Best Way to Stay Abreast?359Appendix E Selected Topics for Student Presentations360E.1Complex Numbers361E.2Complex Vector Spaces362E.3The Leap from Classical to Quantum363E.4Basic Quantum Theory364E.5Architecture365E.6Algorithms366E.7Programming Languages368E.8Theoretical Computer Science369E.9Cryptography370E.10Information Theory370E.11Hardware371Bibliography373Index381More informationPrefaceQuantum computing is a fascinating newfield at the intersection of computer sci-ence,mathematics,and physics,which strives to harness some of the uncanny as-pects of quantum mechanics to broaden our computational horizons.This bookpresents some of the most exciting and interesting topics in quantum computing.Along the way,there will be some amazing facts about the universe in which we liveand about the very notions of information and computation.The text you hold in your hands has a distinctflavor from most of the other cur-rently available books on quantum computing.First and foremost,we do not assumethat our reader has much of a mathematics or physics background.This book shouldbe readable by anyone who is in or beyond their second year in a computer scienceprogram.We have written this book specifically with computer scientists in mind,and tailored it accordingly:we assume a bare minimum of mathematical sophistica-tion,afirst course in discrete structures,and a healthy level of curiosity.Because thistext was written specifically for computer people,in addition to the many exercisesthroughout the text,we added many programming drills.These are a hands-on,funway of learning the material presented and getting a real feel for the subject.The calculus-phobic reader will be happy to learn that derivatives and integrals are virtually absent from our text.Quite simply,we avoid differentiation,integra-tion,and all higher mathematics by carefully selecting only those topics that arecritical to a basic introduction to quantum computing.Because we are focusing onthe fundamentals of quantum computing,we can restrict ourselves to thefinite-dimensional mathematics that is required.This turns out to be not much more thanmanipulating vectors and matrices with complex entries.Surprisingly enough,thelion’s share of quantum computing can be done without the intricacies of advancedmathematics.Nevertheless,we hasten to stress that this is a technical textbook.We are not writing a popular science book,nor do we substitute hand waving for rigor or math-ematical precision.Most other texts in thefield present a primer on quantum mechanics in all its glory.Many assume some knowledge of classical mechanics.We do not make theseassumptions.We only discuss what is needed for a basic understanding of quantumxiMore informationxii Prefacecomputing as afield of research in its own right,although we cite sources for learningmore about advanced topics.There are some who consider quantum computing to be solely within the do-main of physics.Others think of the subject as purely mathematical.We stress thecomputer science aspect of quantum computing.It is not our intention for this book to be the definitive treatment of quantum computing.There are a few topics that we do not even touch,and there are severalothers that we approach briefly,not exhaustively.As of this writing,the bible ofquantum computing is Nielsen and Chuang’s magnificent Quantum Computing andQuantum Information(2000).Their book contains almost everything known aboutquantum computing at the time of its publication.We would like to think of ourbook as a usefulfirst step that can prepare the reader for that text.FEATURESThis book is almost entirely self-contained.We do not demand that the reader comearmed with a large toolbox of skills.Even the subject of complex numbers,which istaught in high school,is given a fairly comprehensive review.The book contains many solved problems and easy-to-understand descriptions.We do not merely present the theory;rather,we explain it and go through severalexamples.The book also contains many exercises,which we strongly recommendthe serious reader should attempt to solve.There is no substitute for rolling up one’ssleeves and doing some work!We have also incorporated plenty of programming drills throughout our text.These are hands-on exercises that can be carried out on your laptop to gain a betterunderstanding of the concepts presented here(they are also a great way of hav-ing fun).We hasten to point out that we are entirely language-agnostic.The stu-dent should write the programs in the language that feels most comfortable.Weare also paradigm-agnostic.If declarative programming is your favorite method,gofor it.If object-oriented programming is your game,use that.The programmingdrills build on one another.Functions created in one programming drill will be usedand modified in later drills.Furthermore,in Appendix C,we show how to makelittle quantum computing emulators with MATLAB or how to use a ready-madeone.(Our choice of MATLAB was dictated by the fact that it makes very easy-to-build,quick-and-dirty prototypes,thanks to its vast amount of built-in mathematicaltools.)This text appears to be thefirst to handle quantum programming languages in a significant way.Until now,there have been only research papers and a few surveyson the topic.Chapter7describes the basics of this expandingfield:perhaps some ofour readers will be inspired to contribute to quantum programming!This book also contains several appendices that are important for further study:Appendix A takes readers on a tour of major papers in quantum computing.This bibliographical essay was written by Jill Cirasella,Computational SciencesSpecialist at the Brooklyn College Library.In addition to having a master’s de-gree in library and information science,Jill has a master’s degree in logic,forwhich she wrote a thesis on classical and quantum graph algorithms.This dualbackground uniquely qualifies her to suggest and describe further readings.More informationPreface xiii Appendix B contains the answers to some of the exercises in the text.Othersolutions will also be found on the book’s Web page.We strongly urge studentsto do the exercises on their own and then check their answers against ours.Appendix C uses MATLAB,the popular mathematical environment and an es-tablished industry standard,to show how to carry out most of the mathematicaloperations described in this book.MATLAB has scores of routines for manip-ulating complex matrices:we briefly review the most useful ones and show howthe reader can quickly perform a few quantum computing experiments with al-most no effort,using the freely available MATLAB quantum emulator Quack.Appendix D,also by Jill Cirasella,describes how to use online resources to keepup with developments in quantum computing.Quantum computing is a fast-movingfield,and this appendix offers guidelines and tips forfinding relevantarticles and announcements.Appendix E is a list of possible topics for student presentations.We give briefdescriptions of different topics that a student might present before a class of hispeers.We also provide some hints about where to start looking for materials topresent.ORGANIZATIONThe book begins with two chapters of mathematical preliminaries.Chapter1con-tains the basics of complex numbers,and Chapter2deals with complex vectorspaces.Although much of Chapter1is currently taught in high school,we feel thata review is in order.Much of Chapter2will be known by students who have had acourse in linear algebra.We deliberately did not relegate these chapters to an ap-pendix at the end of the book because the mathematics is necessary to understandwhat is really going on.A reader who knows the material can safely skip thefirsttwo chapters.She might want to skim over these chapters and then return to themas a reference,using the index and the table of contents tofind specific topics.Chapter3is a gentle introduction to some of the ideas that will be encountered throughout the rest of the ing simple models and simple matrix multipli-cation,we demonstrate some of the fundamental concepts of quantum mechanics,which are then formally developed in Chapter4.From there,Chapter5presentssome of the basic architecture of quantum computing.Here one willfind the notionsof a qubit(a quantum generalization of a bit)and the quantum analog of logic gates.Once Chapter5is understood,readers can safely proceed to their choice of Chapters6through11.Each chapter takes its title from a typical course offered in acomputer science department.The chapters look at that subfield of quantum com-puting from the perspective of the given course.These chapters are almost totallyindependent of one another.We urge the readers to study the particular chapterthat corresponds to their favorite course.Learn topics that you likefirst.From thereproceed to other chapters.Figure0.1summarizes the dependencies of the chapters.One of the hardest topics tackled in this text is that of considering two quan-tum systems and combining them,or“entangled”quantum systems.This is donemathematically in Section2.7.It is further motivated in Section3.4and formallypresented in Section4.5.The reader might want to look at these sections together.xivPrefaceFigure 0.1.Chapter dependencies.There are many ways this book can be used as a text for a course.We urge instructors to find their own way.May we humbly suggest the following three plans of action:(1)A class that provides some depth might involve the following:Go through Chapters 1,2,3,4,and 5.Armed with that background,study the entirety of Chapter 6(“Algorithms”)in depth.One can spend at least a third of a semester on that chapter.After wrestling a bit with quantum algorithms,the student will get a good feel for the entire enterprise.(2)If breadth is preferred,pick and choose one or two sections from each of the advanced chapters.Such a course might look like this:(1),2,3,4.1,4.4,5,6.1,7.1,9.1,10.1,10.2,and 11.This will permit the student to see the broad outline of quantum computing and then pursue his or her own path.(3)For a more advanced class (a class in which linear algebra and some mathe-matical sophistication is assumed),we recommend that students be told to read Chapters 1,2,and 3on their own.A nice course can then commence with Chapter 4and plow through most of the remainder of the book.If this is being used as a text in a classroom setting,we strongly recommend that the students make presentations.There are selected topics mentioned in Appendix E.There is no substitute for student participation!Although we have tried to include many topics in this text,inevitably some oth-ers had to be left out.Here are a few that we omitted because of space considera-tions:many of the more complicated proofs in Chapter 8,results about oracle computation,the details of the (quantum)Fourier transforms,and the latest hardware implementations.We give references for further study on these,as well as other subjects,throughout the text.More informationMore informationPreface xvANCILLARIESWe are going to maintain a Web page for the text at/∼noson/qctext.html/The Web page will containperiodic updates to the book,links to interesting books and articles on quantum computing,some answers to certain exercises not solved in Appendix B,anderrata.The reader is encouraged to send any and all corrections tonoson@Help us make this textbook better!ACKNOLWEDGMENTSBoth of us had the great privilege of writing our doctoral theses under the gentleguidance of the recently deceased Alex Heller.Professor Heller wrote the follow-ing1about his teacher Samuel“Sammy”Eilenberg and Sammy’s mathematics:As I perceived it,then,Sammy considered that the highest value in mathematicswas to be found,not in specious depth nor in the overcoming of overwhelmingdifficulty,but rather in providing the definitive clarity that would illuminate itsunderlying order.This never-ending struggle to bring out the underlying order of mathematical structures was always Professor Heller’s everlasting goal,and he did his best to passit on to his students.We have gained greatly from his clarity of vision and his viewof mathematics,but we also saw,embodied in a man,the classical and sober ideal ofcontemplative life at its very best.We both remain eternally grateful to him.While at the City University of New York,we also had the privilege of inter-acting with one of the world’s foremost logicians,Professor Rohit Parikh,a manwhose seminal contributions to thefield are only matched by his enduring com-mitment to promote younger researchers’work.Besides opening fascinating vis-tas to us,Professor Parikh encouraged us more than once to follow new directionsof thought.His continued professional and personal guidance are greatly appre-ciated.We both received our Ph.D.’s from the Department of Mathematics in The Graduate Center of the City University of New York.We thank them for providingus with a warm and friendly environment in which to study and learn real mathemat-ics.Thefirst author also thanks the entire Brooklyn College family and,in partic-ular,the Computer and Information Science Department for being supportive andvery helpful in this endeavor.1See page1349of Bass et al.(1998).More informationxvi PrefaceSeveral faculty members of Brooklyn College and The Graduate Center were kind enough to read and comment on parts of this book:Michael Anshel,DavidArnow,Jill Cirasella,Dayton Clark,Eva Cogan,Jim Cox,Scott Dexter,EdgarFeldman,Fred Gardiner,Murray Gross,Chaya Gurwitz,Keith Harrow,JunHu,Yedidyah Langsam,Peter Lesser,Philipp Rothmaler,Chris Steinsvold,AlexSverdlov,Aaron Tenenbaum,Micha Tomkiewicz,Al Vasquez,Gerald Weiss,andPaula Whitlock.Their comments have made this a better text.Thank you all!We were fortunate to have had many students of Brooklyn College and The Graduate Center read and comment on earlier drafts:Shira Abraham,RachelAdler,Ali Assarpour,Aleksander Barkan,Sayeef Bazli,Cheuk Man Chan,WeiChen,Evgenia Dandurova,Phillip Dreizen,C.S.Fahie,Miriam Gutherc,RaveHarpaz,David Herzog,Alex Hoffnung,Matthew P.Johnson,Joel Kammet,SerdarKara,Karen Kletter,Janusz Kusyk,Tiziana Ligorio,Matt Meyer,James Ng,SeverinNgnosse,Eric Pacuit,Jason Schanker,Roman Shenderovsky,Aleksandr Shnayder-man,Rose B.Sigler,Shai Silver,Justin Stallard,Justin Tojeira,John Ma Sang Tsang,Sadia Zahoor,Mark Zelcer,and Xiaowen Zhang.We are indebted to them.Many other people looked over parts or all of the text:Scott Aaronson,Ste-fano Bettelli,Adam Brandenburger,Juan B.Climent,Anita Colvard,Leon Ehren-preis,Michael Greenebaum,Miriam Klein,Eli Kravits,Raphael Magarik,JohnMaiorana,Domenico Napoletani,Vaughan Pratt,Suri Raber,Peter Selinger,EvanSiegel,Thomas Tradler,and Jennifer Whitehead.Their criticism and helpful ideasare deeply appreciated.Thanks to Peter Rohde for creating and making available to everyone his MAT-LAB q-emulator Quack and also for letting us use it in our appendix.We had a gooddeal of fun playing with it,and we hope our readers will too.Besides writing two wonderful appendices,our friendly neighborhood librar-ian,Jill Cirasella,was always just an e-mail away with helpful advice and support.Thanks,Jill!A very special thanks goes to our editor at Cambridge University Press,HeatherBergman,for believing in our project right from the start,for guiding us through thisbook,and for providing endless support in all matters.This book would not existwithout her.Thanks,Heather!We had the good fortune to have a truly stellar editor check much of the text many times.Karen Kletter is a great friend and did a magnificent job.We also ap-preciate that she refrained from killing us every time we handed her altered draftsthat she had previously edited.But,of course,all errors are our own!This book could not have been written without the help of my daughter,Hadas-sah.She added meaning,purpose,and joy.N.S.Y.My dear wife,Rose,and our two wondrous and tireless cats,Ursula and Buster, contributed in no small measure to melting my stress away during the long andpainful hours of writing and editing:to them my gratitude and love.(Ursula is ascientist cat and will read this book.Buster will just shred it with his powerful claws.)M.A.M.。
Econometric and Statistical Computing Using Ox

Econometric and Statistical Computing Using OxFRANCISCO CRIBARI–NETO1and SPYROS G.ZARKOS21Departamento de Estat´ıstica,CCEN,Universidade Federal de Pernambuco,Recife/PE,50740–540,Brazil E-mail:cribari@npd.ufpe.br2National Bank of Greece,86Eolou str.,Athens10232,GreeceE-mail:s.zarkos@primeminister.grAbstract.This paper reviews the matrix programming language Ox from the viewpoint of an econometri-cian/statistician.We focus on scientific programming using Ox and discuss examples of possible interest to econometricians and statisticians,such as random number generation,maximum likelihood estimation,and Monte Carlo simulation.Ox is a remarkable matrix programming language which is well suited to research and teaching in econometrics and statistics.Key words:C programming language,graphics,matrix programming language,maximum likelihood estima-tion,Monte Carlo simulation,OxOne of the cultural barriers that separates computer scientists from regular scientists and engineers is a differing point of view on whether a30%or50%loss of speed is worth worrying about.In many real-time state-of-the art scientific applications,such a loss is catastrophic.The practical scientist is trying to solve tomorrow’s problem with yesterday’s computer;the computer scientist,we think, often has it the other way.Press et.al.(1992,p.25) 1.IntroductionApplied statisticians,econometricians and economists often need to write programs that implement estimation and testing procedures.With computers powerful and affordable as they are nowadays,they tend to do that in programming environments rather than in low level programming languages.The former(e.g.,GAUSS,MATLAB,R,S-PLUS)make programming accessible to the vast majority of researchers,and,in many cases,can be combined with the latter(e.g.,C,Fortran)to achieve additional gains in speed.The existence of pre-packaged routines in statistical software that is otherwise best suited to perform data analysis(such as in S-PLUS)does not make the need for“statistical comput-ing”any less urgent.Indeed,many newly developed techniques are not rapidly implemented into statistical software.If one wishes to use such techniques,he/she would have to program them.Additionally,several techniques are very computer-intensive,and require efficient pro-gramming environments/languages(e.g.,bootstrap within a Monte Carlo simulation,double bootstrap,etc.).It would be nearly impossible to perform such computer-intensive tasks with traditional statistical software.Finally,programming forces one to think harder about the problem at hand,the estimation and testing methods that he/she will choose to use.Of course,the most convincing argument may be the following quote from the late John Tukey:“In a world in which the price of calculation continues to decrease rapidly,but the price of theorem proving continues to hold steady or increase,elementary economics indicates that we ought to spend a larger fraction of our time on calculation.”1The focus of our paper is on the use of Ox for‘econometric computing’.That is,we discuss features of the Ox language that may be of interest to statisticians and econometricians,and exemplify their use through examples.Readers interested in reviews of Ox,including the language structure,its syntax,and its advantages and disadvantages,are referred to Cribari–Neto(1997),Keng and Orzag(1997),Kusters and Steffen(1996)and Podivinsky(1999).1 2.A Brief Overview of OxOx is a matrix programming language with object-oriented support developed by Jur-gen Doornik,a Dutch graduate student(at the time)at Nuffield College,Oxford.The development of Ox started in April1994.Doornik’s primary goal was to develop a matrix programming language for the simulations he wished to perform for his doctoral dissertation. The veryfirst preliminary version of Ox dates back to November1994.In the summer of 1995,two other econometricians at Nuffield College started using Ox for their research:Neil Shephard and Richard Spady.From that point on,the development of Ox became a serious affair.The current Ox version is numbered3.00.Ox binaries are available for Windows and severalflavors of UNIX(including Linux)and can be downloaded from /Users/Doornik/,which is the main Ox web page.All versions are free for educational purposes and academic research,with the exception of the‘Professional Windows version’.This commercial version comes with a nice interface for graphics known as GiveWin(available for purchase from Timberlake Consultants, ).The free Ox versions can be launched from the command line in a console/terminal win-dow,which explains why they are also known as‘console versions’.Doornik also distributes freely a powerful text editor for Windows:OxEdit(see also the OxEdit web page,which is currently at ).It can be used as a front-end not only to Ox(the console version)but also to other programs and languages,such as C,C++,T E X,L a T E X,etc.The Ox syntax is very similar to that of C,C++and Java.In fact,its similarity to C (at least as far as syntax goes)is one of its major advantages.2One characteristic similarity with C/C++is in the indexing,which starts at zero,and not at one.This means that thefirst element of a matrix,say A,is accessed as A[0][0]instead of as A[1][1].A key difference between Ox and languages such as C,C++and Java is that matrix is a basic type in Ox. Also,when programming in Ox one needs to declare the variables that will be used in the program(as is the case in C/C++),but unlike in C/C++,one does not have to specify the type of the variables that are declared.Ox’s most impressive feature is that it comes with a comprehensive mathematical and statistical function library.A number of useful functions and methods are implemented into the language,which makes it very useful for scientific 1A detailed comparison involving GAUSS,Macsyma,Maple,Mathematica,MATLAB,MuPAD,O-Matrix,Ox, R-Lab,Scilab,and S-PLUS can be found at http://www.scientificweb.de/ncrunch/ncrunch.pdf(“Com-parison of mathematical programs for data analysis”by Stefan Steinhaus).Ox is the winner when it comes to speed.2Other important advantages of Ox are the fact that it is fast,free,can be easily linked to C,Fortran, etc.,and can read and write data in several different formats(ASCII,Gauss,Excel,Stata,Lotus,PcGive, etc.).2programming.Ox comes with a comprehensive set of helpfiles in HTML form.The documentation of the language can be also found in Doornik(2001).A good introduction to Ox is Doornik, Draisma and Ooms(1998).3.A Few Simple IllustrationsOurfirst example is a very simple one,and intends to show the similarity between the Ox and C syntaxes.We wish to develop a program that produces a small table converting temperatures in Fahrenheit to Celsius(from0F to300F in steps of20F).The source of this example is Kerninghan and Ritchie(1988).The C code can be written as follows./****************************************************************PROGRAM:celsius.c**USAGE:To generate a conversion table of temperatures(from*Fahrenheit to Celsius).Based on an example in the*Kernighan&Ritchie’s book.****************************************************************/#include<stdio.h>int main(void){int fahr;printf("\nConversion table(F to C)\n\n");printf("\t%3s%5s\n","F","C");/*Loop over temperatures*/for(fahr=0;fahr<=300;fahr+=20){printf("\t%3d%6.1f\n",fahr, 5.0*(fahr-32)/9.0);}printf("\n");return0;}The output produced by compiled C code using the gcc compiler(Stallman,1999)under the Linux operating system(MacKinnon,1999)is:[cribari@edgeworth c]$gcc-O2-o celsius celsius.c[cribari@edgeworth c]$./celsiusConversion table(F to C)F C0-17.820-6.7340 4.46015.68026.710037.812048.914060.016071.118082.220093.3220104.4240115.6260126.7280137.8300148.9The next step is to write the same program in Ox code.The Ox transcription of the celcius.c program follows:/****************************************************************PROGRAM:celsius.ox**USAGE:To generate a conversion table of temperatures(from*Fahrenheit to Celsius).Based on an example in the*Kernighan&Ritchie’s book.***************************************************************/#include<oxstd.h>main(){decl fahr;print("\nConversion table(F to C)\n\n");print("\t F C\n");//Loop over temperaturesfor(fahr=0;fahr<=300;fahr+=20){print("\t","%3d",fahr);print("","%6.1f", 5.0*(fahr-32)/9.0,"\n");}print("\n");}The Ox output is:[cribari@edgeworth programs]$oxl celsiusOx version 3.00(Linux)(C)J.A.Doornik,1994-2001Conversion table(F to C)F C40-17.820-6.740 4.46015.68026.710037.812048.914060.016071.118082.220093.3220104.4240115.6260126.7280137.8300148.9The two programs above show that the Ox and C syntaxes are indeed very similar.Note that Ox accepts C style comments(/*...*/),and also C++like comments to the end of the line(//).3We also note that,unlike C,Ox accepts nested comments.The similarity between the Ox and C syntaxes is a major advantage of Ox over other matrix languages.Kendrick and Amman(1999)provide an overview of programming languages in economics.In the introduction of their paper,they give the following advice to users who are starting to program:“Begin with one of the high-level or modeling languages.(...)Then work downward in the chain and learn either Fortran,C,C++,or Java.”If a user then starts with Ox and‘works downward’to C or C++the transition will be smoother than if he/she starts the chain with other high level languages.As a second illustration of the use of Ox in econometrics and statistics,we develop a simple program thatfirst simulates a large number of coin tosses,and then counts the frequency (percentage)of tails.The code which is an Ox translation,with a smaller total number of runs,of the C code given in Cribari–Neto(1999),thus illustrates Kolmogorov’s Law of Large Numbers.We begin by writing a loop-based version of the coin tossing experiment./*******************************************************************PROGRAM:coin_loop.ox**USE:Simulates a large number of coin tosses and prints*the percentage of tails.**PURPOSE:The program illustrates the first version of the*law of large numbers which dates back to James*Bernoulli.******************************************************************/#include<oxstd.h>/*maximum number of coin tosses*/3Ox also borrows from Java;the println function,for instance,comes from the Java programming language.5const decl COIN_MAX=1000000;main(){decl j,dExecTime,temp,result,tail,s;//Start the clock(to time the execution of the program).dExecTime=timer();//Choose the random number generator.ranseed("GM");//Main loop:for(j=10;j<=COIN_MAX;j*=10){tail=0;for(s=0;s<j;s++){temp=ranu(1,1);tail=temp>0.5?tail:tail+1;}result=100.0*tail/j;print("Percentage of tails from",j,"tosses:","%8.2f",result,"\n");}print("\nEXECUTION TIME:",timespan(dExecTime),"\n");}The instruction tail=temp>0.5?tail:tail+1;does exactly what it does in C: it sets the variable tail equal to itself if the stated condition is true(temp>0.5)and to tail+1otherwise.We now vectorize the above code for speed.The motivation is obvious:vectorization usually leads to efficiency gains,unless of course one runs into memory problems.It is note-worthy that one of the main differences between a matrix programming language and a low level language,such as C and C++,is that programs should exploit vector and matrix opera-tions when written and executed in a matrix-oriented language,such as Ox.The vectorized code for the example at hand is:/*******************************************************************PROGRAM:coin_vec.ox**USE:Simulates a large number of coin tosses and prints*the percentage of tails.**PURPOSE:The program illustrates the first version of the*law of large numbers which dates back to James*Bernoulli.******************************************************************/6#include<oxstd.h>/*maximum number of coin tosses*/const decl COIN_MAX=1000000;main(){decl j,dExecTime,temp,tail;//Start the clock(to time the execution of the program).dExecTime=timer();//Choose the random number generator.ranseed("GM");//Coin tossing:for(j=10;j<=COIN_MAX;j*=10){temp=ranu(1,j);tail=sumr(temp.<0.5)*(100.0/j);print("Percentage of tails from",j,"tosses:","%8.2f",double(tail),"\n");}print("\nEXECUTION TIME:",timespan(dExecTime),"\n");}The output of the loop-based program is:[cribari@edgeworth programs]$oxl coin_loopOx version 3.00(Linux)(C)J.A.Doornik,1994-2001Percentage of tails from10tosses:40.00Percentage of tails from100tosses:53.00Percentage of tails from1000tosses:49.10Percentage of tails from10000tosses:49.69Percentage of tails from100000tosses:49.83Percentage of tails from1000000tosses:49.99EXECUTION TIME: 2.41whereas the vectorized code generates the following output: [cribari@edgeworth programs]$oxl coin_vecOx version 3.00(Linux)(C)J.A.Doornik,1994-2001Percentage of tails from10tosses:40.00Percentage of tails from100tosses:53.00Percentage of tails from1000tosses:49.10Percentage of tails from10000tosses:49.69Percentage of tails from100000tosses:49.83Percentage of tails from1000000tosses:49.99EXECUTION TIME:0.237Note that the empirical frequency of tails approaches1/2,the population mean,as predicted by the Law of Large Numbers.As far as efficiency goes,we see that vectorization leads to a sizeable improvement.The loop-based program yields an execution time which is over10 times greater than that of its vectorized version,on a DELL Pentium III1GHz computer with512MB RAM running on Linux.4Some languages,like C,operate faster on rows than on columns.The same logic applies to Ox.To illustrate the claim,we modify the vectorized code so that the random draws are stored in a column vector(they were previously stored in a row vector).To that end,one only needs to change two lines of code:for(j=10;j<=COIN_MAX;j*=10){temp=ranu(j,1);//1st changetail=sumc(temp.<0.5)*(100.0/j);//2nd changeprint("Percentage of tails from",j,"tosses:","%8.2f",double(tail),"\n");}This new vectorized code now runs in0.35second.That is,we see a speed penalty of over 50%when we transpose the code so that we work with a large column vector instead of working with a large row vector.4.Econometric ApplicationsMaximum likelihood estimates oftentimes need to be computed using a nonlinear op-timization scheme.In order to illustrate how that can be done using Ox,we consider the maximum likelihood estimation of the number of degrees-of-freedom of a Student t distri-bution.Maximization is performed using a quasi-Newton method(known as the‘BFGS’method)with numerical gradient,i.e.,without specifying the score function.(Note that this estimator is substantially biased in small samples.)It is noteworthy that Ox has routines for other optimization methods as well,such as the Newton-Raphson and the BHHH methods. An advantage of the BFGS method is that it allows users to maximize likelihoods without having to specify a score function.See Press et al.(1992,Chapter10)for details on the BFGS and other nonlinear optimization methods.See also Mittelhammer,Judge and Miller(2000,§8.13),who on page199write that“[t]he BFGS algorithm is generally regarded as the best performing method.”The example below uses a random sample of size50,the true value of the parameter is3,and the initial value of the optimization scheme is2.(We have neglected a constant in the log-likelihood function.)/**************************************************************PROGRAM:t.ox**USAGE:Maximum likelihood estimation of the number of*degrees of freedom of a Student t distribution.*************************************************************/4The operating system was Mandrake Linux8.0running on kernel2.4.3.8#include<oxstd.h>#include<oxprob.h>#import<maximize>const decl N=50;static decl s_vx;fLogLik(const vP,const adFunc,const avScore,const amHess) {decl vone=ones(1,N);decl nu=vP[0];adFunc[0]=double(N*loggamma((nu+1)/2)-(N/2)*log(nu)-N*loggamma(nu/2)-((nu+1)/2)*(vone*log(1+(s_vx.^2)/nu)));if(isnan(adFunc[0])||isdotinf(adFunc[0]))return0;elsereturn1;//1indicates success}main(){decl vp,dfunc,dnu,ir;ranseed("GM");vp= 2.0;dnu= 3.0;s_vx=rant(N,1,3);ir=MaxBFGS(fLogLik,&vp,&dfunc,0,TRUE);print("\nCONVERGENCE:",MaxConvergenceMsg(ir));print("\nMaximized log-likelihood:","%7.3f",dfunc);print("\nTrue value of nu:","%6.3f",dnu);print("\nML estimate of nu:","%6.3f",double(vp));print("\nSample size:","%6d",N);print("\n");}Here is the Ox output:[cribari@edgeworth programs]$oxl tOx version 3.00(Linux)(C)J.A.Doornik,1994-2001CONVERGENCE:Strong convergenceMaximized log-likelihood:-72.813True value of nu: 3.0009ML estimate of nu: 1.566Sample size:50The maximum likelihood estimate ofν,whose true value is3,is ν=1.566.This example shows that nonlinear maximization of functions can be done with ease using Ox.Of course, one can estimate more complex models in a similar fashion.For example,the parameters of a nonlinear regression model can be estimated by setting up a log-likelihood function,and maximizing it with a MaxBFGS call.It is important to note,however,that Ox does not come with routines for performing constrained maximization.The inclusion of such functions in Ox would be a great addition to the language.A number of people have developed add-on packages for Ox.These handle dynamic panel data(DPD),ARFIMA models,conditionally heteroskedastic models,stochastic volatil-ity models,state space forms.There is,moreover,Ox code for quantile regressions,and in particular, 1(i.e.,least absolute deviations)regressions.The code corresponds to the al-gorithm described in Portnoy and Koenker(1997)and is available at Roger Koenker’s web page(/roger/research/rqn/rqn.html).We consider,next,the G@RCH2.0package recently developed by S´e bastien Laurent and Jean–Philippe Peters,which is dedicated to the estimation and forecasting of ARCH,GARCH models.The GARCH add-on package comes in two versions,namely:(i)the‘Full Version’which requires a registered copy of Ox Professional3.00,since it is launched from OxPack and makes use of the GiveWin interface,and(ii)the‘Light Version’which only requires the free (‘console’)version of Ox.It relies on Ox’s object-oriented programming capabilities,being a derived class of Ox’s Modelbase type of class.The package is available for download at http://www.egss.ulg.ac.be/garch.We borrow the example program(GarchEstim.ox)in order to illustrate the use of the GARCH code(as with everything else,in the context of the console,i.e.free,version of Ox).The GARCH object(which is created with the source code provided by this add-on package)allows for the estimation of a large number of uni-variate ARCH-type models(e.g.,ARCH,GARCH,IGARCH,FIGARCH,GJR,EGARCH, APARCH,FIEGARCH,FIAPARCH)under Gaussian,Student–t,skewed Student and gen-eralized error distributions.Forecasts(one-step-ahead density forecasts)of the conditional mean and variance are also available,as well as several misspecification tests and graphics commands.#include<oxstd.h>#import<packages/garch/garch>main(){decl garchobj;garchobj=new Garch();//***DATA***//garchobj.Load("Data/demsel.in7");();garchobj.Select(Y_VAR,{"DEM",0,0});10garchobj.SetSelSample(-1,1,-1,1);//***SPECIFICATIONS***//garchobj.CSTS(1,1);//cst in Mean(1or0),cst in Variance(1or0)garchobj.DISTRI(0);//0for Gauss,1for Student,2for GED,3for Skewed-Student garchobj.ARMA(0,0);//AR order(p),MA order(q).garchobj.ARFIMA(0);//1if Arfima wanted,0otherwisegarchobj.GARCH(1,1);//p order,q ordergarchobj.FIGARCH(0,0,1000);//Arg.1:1if Fractionnal Integration wanted.//Arg.2:0->BBM,1->Chung//Arg.3:if BBM,Truncation ordergarchobj.IGARCH(0);//1if IGARCH wanted,0otherwisegarchobj.EGARCH(0);//1if EGARCH wanted,0otherwisegarchobj.GJR(0);//1if GJR wanted,0otherwisegarchobj.APARCH(0);//1if APARCH wanted,0otherwise//***TESTS&FORECASTS***//garchobj.BOXPIERCE(<5;10;20>);//Lags for the Box-Pierce Q-statistics.garchobj.ARCHLAGS(<2;5;10>);//Lags for Engle’s LM ARCH test.garchobj.NYBLOM(1);//1to compute the Nyblom stability test,0otherwisegarchobj.PEARSON(<40;50;60>);//Cells(<40;50;60>)for the adjusted Pearson//Chi-square Goodness-of-fit test,0if not computed//G@RCH1.12garchobj.FORECAST(0,100);//Arg.1:1to launch the forecasting procedure,//0elsewhere//Arg.2:Number of one-step ahead forecasts//***OUTPUT***//garchobj.MLE(1);//0:both,1:MLE,2:QMLEgarchobj.COVAR(0);//if1,prints variance-covariance matrix of the parameters.garchobj.ITER(0);//Interval of iterations between printed intermediary results//(if no intermediary results wanted,enter’0’) garchobj.TESTSONLY(0);//if1,runs tests for the raw Y series,prior to//any estimation.garchobj.GRAPHS(0);//if1,prints graphics of the estimations//(only when using GiveWin).garchobj.FOREGRAPHS(0);//if1,prints graphics of the forecasts//(only when using GiveWin).//***PARAMETERS***//garchobj.BOUNDS(0);//1if bounded parameters wanted,0otherwisegarchobj.DoEstimation(<>);garchobj.STORE(0,0,0,0,0,"01",0);//Arg.1,2,3,4,5:if1->stored.(Res-SqRes-CondV-MeanFor-VarFor)//Arg.6:Suffix.The name of the saved series will be"Res_ARG6"//(or"MeanFor_ARG6",...).//Arg.7:if0,saves as an Excel spreadsheet(.xls).//If1,saves as a GiveWin dataset(.in7)delete garchobj;}11We have run the above code to obtain the MLE and QMLE results of an ARMA(0,0)model in the mean equation and GARCH(1,1)model in the variance equation,assuming Gaussian distributed errors.Some portmanteau tests,such as the Box–Pierce Q-statistic and the LM ARCH test,the Jarque–Bera normality test etc,were also calculated for the daily observations on the Dow Jones Industrial Average(Jan.1982-Dec.1999,a total of4,551observations). The output follows.Ox version 3.00(Linux)(C)J.A.Doornik,1994-2001Copyright for this package:urent and J.P.Peters,2000,2001.G@RCH package version 2.00,object created on14-08-2001----Database information----Sample:1-4313(4313observations)Frequency:1Variables:4Variable#obs#miss min mean max std.devDEM43130-6.3153-0.0022999 3.90740.75333PREC4313000.4259250.82935SUCC4313000.418550.81568OBSVAR43130 3.3897e-060.567539.853 1.3569 **********************SPECIFICATIONS*********************Mean Equation:ARMA(0,0)model.No regressor in the meanVariance Equation:GARCH(1,1)model.No regressor in the varianceThe distribution is a Gauss distribution.Strong convergence using numerical derivativesLog-likelihood=-4651.57Please wait:Computing the Std Errors...Maximum Likelihood EstimationCoefficient Std.Error t-value t-probCst(M)0.0031860.0100190.31800.7505Cst(V)0.0178730.003216 5.5580.0000GARCH(Beta1)0.8702150.01168674.460.0000ARCH(Alpha1)0.1028470.00964210.670.0000Estimated Parameters Vector:0.003186;0.017873;0.870215;0.102847No.Observations:4313No.Parameters:4*************TESTS**12***********Statistic t-Test P-ValueSkewness-0.20031 5.37237.7733e-08Excess Kurtosis 1.868425.061 1.3133e-138Jarque-Bera656.19656.19 3.2440e-143---------------Information Criterium(minimize)Akaike 2.158856Shibata 2.158855Schwarz 2.164763Hannan-Quinn 2.160942---------------BOX-PIERCE:ValueMean of standardized residuals-0.00065Mean of squared standardized residuals0.99808H0:No serial correlation==>Accept H0when prob.is High[Q<Chisq(lag)] Box-Pierce Q-statistics on residualsQ(5)=17.7914[0.00321948]Q(10)=26.4749[0.00315138]Q(20)=44.9781[0.00111103]Box-Pierce Q-statistics on squared residuals-->P-values adjusted by2degree(s)of freedomQ(5)=8.01956[0.0456093]Q(10)=12.4119[0.133749]Q(20)=34.563[0.0107229]--------------ARCH1-2test:F(2,4306)= 2.7378[0.0648]ARCH1-5test:F(5,4300)= 1.5635[0.1668]ARCH1-10test:F(10,4290)= 1.2342[0.2632]--------------Diagnostic test based on the news impact curve(EGARCH vs.GARCH)Test ProbSign Bias t-Test 1.175980.23960Negative Size Bias t-Test 1.828560.06747Positive Size Bias t-Test0.975420.32935Joint Test for the Three Effects 4.468820.21509---------------Joint Statistic of the Nyblom test of stability: 1.77507Individual Nyblom Statistics:Cst(M)0.43501Cst(V)0.22234GARCH(Beta1)0.10147ARCH(Alpha1)0.10050Rem:Asymptotic1%critical value for individual statistics=0.75.Asymptotic5%critical value for individual statistics=0.47.---------------Adjusted Pearson Chi-square Goodness-of-fit testLags Statistic P-Value(lag-1)P-Value(lag-k-1)4078.06890.0002040.0000405089.05190.0004090.00010060103.25320.0003250.00008913Rem.:k=#estimated parameters---------------Elapsed Time: 4.67seconds(or0.0778333minutes).The stochastic volatility package(SvPack),written by Neil Shephard,is essentially a dy-namic link library for Ox of C code that deals with the implementation of likelihood inference in volatility models.The fact that it is written in C guarantees optimal speed,whereas the linking to Ox definitely improves usability.It requires the Ox state space package(SsfPack), which provides for Kalmanfiltering,smoothing and simulation smoothing algorithms of Gaus-sian multivariate state space forms(see Koopman,Shephard and Doornik,1999;Ooms,1999, and also ),as well as ARMS(Adaptive Rejection Metropolis Sam-pling),an Ox front-end for C code for adaptive rejection sampling algorithms(i.e.,routines for efficient sampling from complicated univariate densities)developed and documented by Michael Pitt(based on C code by Wally Gilks).The Arfima package is a set of Ox functions that create a class(an ARFIMA object) for the estimation and testing of AR(F)IMA models(Beran,1994).The models can be esti-mated via exact maximum likelihood,modified profile likelihood and nonlinear least squares. ArfimaSim is an additional simulation class included in the Arfima package that provides the means for Monte Carlo experiments based on the Arfima class.The Dynamic Panel Data package,DPD,like the Arfima and G@RCH packages,is a nice example of object-oriented Ox programming.They are derived classes written in Ox.DPD, which is entirely written in Ox,implements dynamic panel data models,as well as some static ones,and can handle unbalanced panels.Monte Carlo experimentation is possible with the simulation class DPSSim,included in this Ox add-on package.5.GraphicsOx has a number of commands that help create publication-quality graphics.This is, however,one of the areas where more progress is expected.The graphics capabilities of the console version of Ox are not comparable to those of,say,GAUSS,MATLAB,R or S-PLUS.It is important to note,however,that the professional version of Ox comes with an impressive interface for graphics:GiveWin.It allows users,for example,to modify a graph with a few clicks of the mouse.With GiveWin,it is possible to edit all graphs on the screen, manipulate areas,add Greek letters,add labels,change fonts,etc.Therefore,users who intend to make extensive use of the plotting capabilities of the language to produce publication quality graphics should consider using the professional version of Ox.5An alternative strategy would be to use Ox for programming,save the results to afile, read the resultsfile into R,which is also free,and then produce publication quality plots from there.6It is also possible to use GnuDraw,an Ox package written by Charles Bos (http://www2.tinbergen.nl/~cbos/).GnuDraw allows users to create gnuplot(http:// )graphics from Ox,extending the possibilities offered by existing OxDraw 5The newest,just released,version3.00of Ox has improved graphics capabilities.For instance,it now has built-in functions for producing3D plots.6For details on R,see .14。
quantative imagine analysis
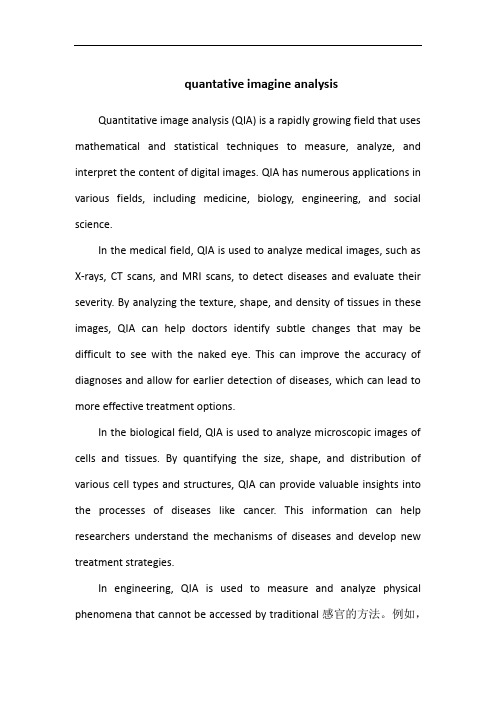
quantative imagine analysisQuantitative image analysis (QIA) is a rapidly growing field that uses mathematical and statistical techniques to measure, analyze, and interpret the content of digital images. QIA has numerous applications in various fields, including medicine, biology, engineering, and social science.In the medical field, QIA is used to analyze medical images, such as X-rays, CT scans, and MRI scans, to detect diseases and evaluate their severity. By analyzing the texture, shape, and density of tissues in these images, QIA can help doctors identify subtle changes that may be difficult to see with the naked eye. This can improve the accuracy of diagnoses and allow for earlier detection of diseases, which can lead to more effective treatment options.In the biological field, QIA is used to analyze microscopic images of cells and tissues. By quantifying the size, shape, and distribution of various cell types and structures, QIA can provide valuable insights into the processes of diseases like cancer. This information can help researchers understand the mechanisms of diseases and develop new treatment strategies.In engineering, QIA is used to measure and analyze physical phenomena that cannot be accessed by traditional感官的方法。
多轴差分吸收光谱法英文
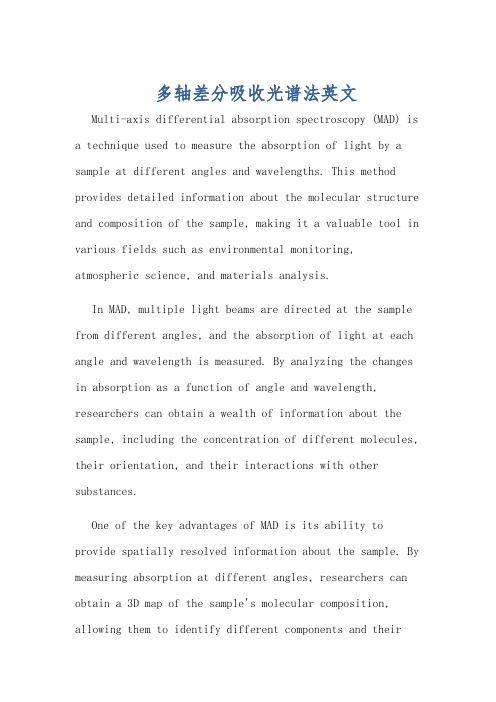
多轴差分吸收光谱法英文Multi-axis differential absorption spectroscopy (MAD) is a technique used to measure the absorption of light by a sample at different angles and wavelengths. This method provides detailed information about the molecular structure and composition of the sample, making it a valuable tool in various fields such as environmental monitoring, atmospheric science, and materials analysis.In MAD, multiple light beams are directed at the sample from different angles, and the absorption of light at each angle and wavelength is measured. By analyzing the changes in absorption as a function of angle and wavelength, researchers can obtain a wealth of information about the sample, including the concentration of different molecules, their orientation, and their interactions with other substances.One of the key advantages of MAD is its ability to provide spatially resolved information about the sample. By measuring absorption at different angles, researchers can obtain a 3D map of the sample's molecular composition, allowing them to identify different components and theirspatial distribution. This makes MAD particularly usefulfor studying complex mixtures or heterogeneous samples.Another important feature of MAD is its high sensitivity. By measuring absorption at multiple angles and wavelengths, researchers can enhance the signal-to-noise ratio anddetect subtle changes in the sample's composition. This makes MAD suitable for studying trace components or low-concentration substances, which may be challenging todetect using traditional spectroscopic techniques.Furthermore, MAD can be used to study dynamic processesin real time. By continuously measuring absorption at multiple angles and wavelengths, researchers can track changes in the sample's composition as a function of time, providing valuable insights into reaction kinetics,diffusion processes, and other dynamic phenomena.In summary, multi-axis differential absorption spectroscopy is a powerful technique for studying the molecular composition and structure of samples. Its ability to provide spatially resolved, sensitive, and real-time information makes it a valuable tool for a wide range ofapplications, from environmental monitoring to materials analysis.多轴差分吸收光谱法(MAD)是一种用于测量样品在不同角度和波长下光吸收的技术。
Motion Estimation
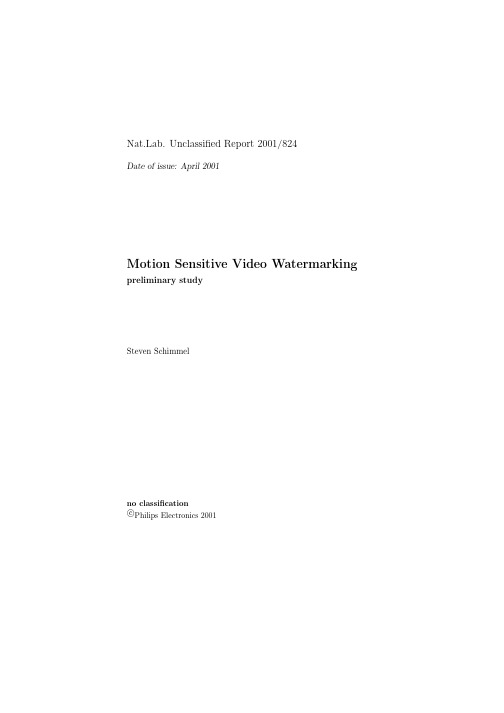
. . . .
. . . .
. . . .
. . . .
. . . .
. . . .
. . . .
. . . .
2 Introduction to Digital Video 2.1 Definitions and terminology . . . . . 2.1.1 Images . . . . . . . . . . . . . 2.1.2 Video sequences . . . . . . . 2.1.3 Video interlacing . . . . . . . 2.1.4 Contrast . . . . . . . . . . . . 2.1.5 Spatial frequency . . . . . . . 2.2 Digital image processing . . . . . . . 2.2.1 Fourier transform . . . . . . . 2.2.2 Convolution . . . . . . . . . . 2.2.3 Digital filters . . . . . . . . . 2.2.4 Correlation . . . . . . . . . . 2.3 MPEG-2 video compression . . . . . 2.3.1 The discrete cosine transform 2.3.2 Quantization . . . . . . . . . 2.3.3 Motion compensation . . . . 2.3.4 Efficient representation . . .
ii
c Philips Electronics N.V. 2001
no classification
Canonical Quantization of Noncommutative Field Theory
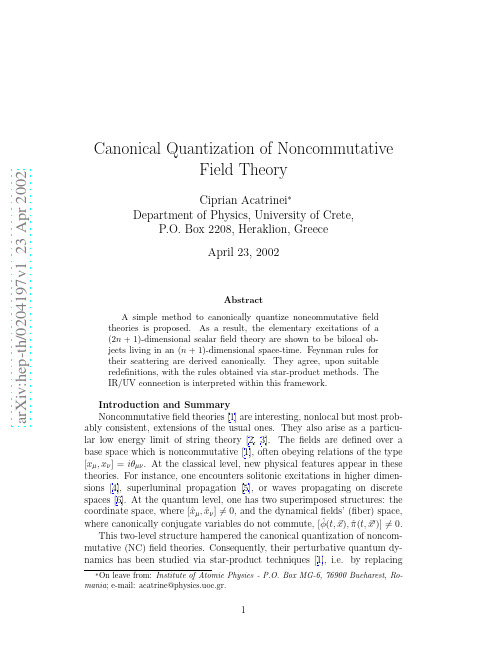
a rXiv:h ep-th/024197v123A pr22Canonical Quantization of Noncommutative Field Theory Ciprian Acatrinei ∗Department of Physics,University of Crete,P.O.Box 2208,Heraklion,Greece April 23,2002Abstract A simple method to canonically quantize noncommutative field theories is proposed.As a result,the elementary excitations of a (2n +1)-dimensional scalar field theory are shown to be bilocal ob-jects living in an (n +1)-dimensional space-time.Feynman rules for their scattering are derived canonically.They agree,upon suitable redefinitions,with the rules obtained via star-product methods.The IR/UV connection is interpreted within this framework.Introduction and Summary Noncommutative field theories [1]are interesting,nonlocal but most prob-ably consistent,extensions of the usual ones.They also arise as a particu-lar low energy limit of string theory [2,3].The fields are defined over a base space which is noncommutative [1],often obeying relations of the type[x µ,x ν]=iθµν.At the classical level,new physical features appear in these theories.For instance,one encounters solitonic excitations in higher dimen-sions [4],superluminal propagation [5],or waves propagating on discrete spaces [6].At the quantum level,one has two superimposed structures:the coordinate space,where [ˆx µ,ˆx ν]=0,and the dynamical fields’(fiber)space,where canonically conjugate variables do not commute,[ˆφ(t, x ),ˆπ(t, x ′)]=0.This two-level structure hampered the canonical quantization of noncom-mutative (NC)field theories.Consequently,their perturbative quantum dy-namics has been studied via star-product techniques [1],i.e.by replacingoperator products with the Groenewold-Moyal one.This leads to deformed theories,living on a commutative space of Weyl symbols.Perturbation the-ory is then defined in the usual way.Loop calculations performed in this set-up pointed to an intriguing mixing between short distance and long dis-tance physics,called the IR/UV connection[7,8,9].The purpose of this paper is to develop simple canonical techniques for the direct quantization of noncommutativefields.We present here the basic idea,describe the nature of the degrees of freedom and their rules of inter-action,as well as some implications.Our motivations are at least two-fold. First,phase space quantization methods[13]are not always the most useful ones,either for particles or forfields.Actually,commutative quantum theo-ries developed mostly through canonical,functional,or propagator methods. Second,canonical quantization offers a clear picture of the degrees of freedom of a theory,picture which is not rigorously established in NC spaces,in spite of many interesting works[10,11].Our elementary operatorial methods will automatically lead to such a picture.We show that the fundamental exci-tations of a(2n+1)−dimensional scalar theory(with commuting time)are bilocal objects living in a lower,(n+1)−,dimensional space-time.We will call them rods,or dipoles,although no charge of any kind enters their de-scription.The information on the remaining n spatial directions is encoded into the length and orientation of the dipoles.Those n parameters are,in turn,proportional to the momentum a noncommutative particle would have in the‘lost’directions.This picture puts on afirmer ground a general belief [10,11]that noncommutative theories are about dipoles,not particles.More-over,it shows that these dipoles live in a lower dimensional space.Rules for their propagation and scattering are obtained canonically.They show that the above dimensional reduction is limited to tree level dynamics:the loop integrations are taken also over the dipole parameters,restoring the(2n+1)-dimensionality of the theory,as far as renormalization is concerned.Upon identification of the rod parameters with the momenta in the conjugate di-rections,our Feynman rules agree with the ones obtained a long time ago through star product technology.The physical interpretation is however dif-ferent,being hopefully more intuitive and adequate for the description of experiments.The interpretation of the IR/UV mixing given in[8]can be adapted to this framework.One also notices that interaction‘vertices’for dipoles have in generalfinite area,and a poligonal boundary.As far as this area is keptfinite,loop amplitudes are effectively regulated by noncommu-tativity.However,once this area shrinks to zero(in planar diagrams,or nonplanar ones with zero external momentum),the NC phase is of no effect, and UV infinities are present.They metamorphose into IR divergences if the cause of the vertex shrinking is an external momentum going to zero.Bilocal objectsLet us consider a(2+1)-dimensional scalarfieldΦ(t,ˆx,ˆy)defined over a commutative time t and a pair of NC coordinates satisfying[ˆx,ˆy]=iθ.(1) The extension to n NC pairs is mutative spatial direc-tions are dropped,for simplicity.The action isS=14!Φ4.Cubic potentialsare actually simpler,but maybe less relevant physically.We(‘doubly’-)quantize thefieldΦby writingΦ= dk x dk y2ω k ˆa k x k y e i(ω k t−k xˆx−k yˆy)+ˆa†k x k y e−i(ω k t−k xˆx−k yˆy) .(3)ˆx andˆy are operators acting on the Hilbert space H,which appeared dueto their noncommutativity.ˆa kx k y andˆa†kx k yact on the usual Fock space Fof a quantumfield theory(FT).We have thus a‘doubly’-quantum FT,with Φacting on a direct product of two Hilbert spaces,namely F⊗H.To prove(3),start with a classicalfield living on a commuting space.Upon usualfield quantization,a and a∗become operators on the Fock space F. To make the underlying space noncommutative,introduce(1)and apply the Weyl quantization procedure[12]to the exponentials e i(k x x+k y y).The resultis(3),which means the following:Φcreates(destroys),viaˆa†kx k y (ˆa kx k y),an excitation represented by a”plane wave”e i(ω k t−k xˆx−k yˆy).We will now describe such an object.We could work withΦas an operator ready to act on both Hilbert spaces F and H.It is however simpler to”saturate”it on H,working with expec-tation values<x′|Φ|x>,which can still act on F.|x>is an eigenstate ofˆx,ˆx|x>=x|x>,ˆy|x>=−iθ∂2δ(x′−x−k yθ).(4)This is a bilocal expression,and we already see that its span along the x axis,(x′−x),is proportional to the momentum along theconjugatey direction,i.e.(x′−x)=θk ing(3,4),one sees that<x′|Φ|x>= dk x2ωk x,k y ˆa k x,k y e i(ω k t−k x x+x′2 .(5) where k y=(x′−x)/θ.Thus,Φannihilates a rod of momentum k x and lengthθk y,and creates a rod of momentum k x and length−θk y.Due to(1), one degree of freedom apparently disappears from(5).Its presence shows up only through the modified dispersion relationω(k x,k y=x′−x k2x+(x′−x)28π2ωkx,e ik x[x3+x42]δ(x4−x3−x2+x1)(7)where k y=(x′−x)/θ,andωk x,k y=(x′−x)/θobeys(6)again.Again,there is no integral along k y.More precisely,if one compares(7)to the(1+1)-dimensional commutative correlator of twofields, 0|φ(X2)φ(X1)|0 ,with X1=(x1+x2)/2and X2=(x3+x4)/2,the only differences are the additional (x′−x)24! dt x,a,b,c<x|Φ|a><a|Φ|b><b|Φ|c><c|Φ|x>.(8)We will have a look at some terms in the Dyson series generated by(8), to illustrate the canonical derivation of the Feynman rules.Let:ˆAˆB: denote normal ordering ofˆAˆB.Once the vacuum correlator(7)is known,the derivation of the diagrammatic rules follows the standard procedure;hence we will not present it in detail.Tofind the basic‘vertex’for four-dipole scattering we evaluate− k3,− k4|: dt x,a,b,c<x|Φ|a><a|Φ|b><b|Φ|c><c|Φ|x>:| k1, k2 (9)| k1, k2 is a Fock space state,meaning two quanta are present,with momenta kand k2.The momenta k i,i=1,2,3,4have each two components: k i=(k i,l i). 1k i is the momentum along x,whereas l i represents the dipole extension along x(corresponding to the momentum along y).Using Eq.(5)and integrating over x,y,z and u,one obtains the conservation laws k1+k2=k3+k4and l1+l2=l3+l4.Thefinal result differs from the four-point scattering vertex of(2+1)commutative particles with momenta k i=(k i,l i)only through the phasee−iθ2π dk loop dl loop integration, together with the dispersion relation(6),brings back into play-as far asdivergences are concerned-the y direction.It is easy to extend the above reasoning to(2n+1)−dimensions:unconstrained dipoles will propagate in a(n+1)-dimensional commutative space-time;their Feynman rules are ob-tained as outlined above.Once the dipole lengths are interpreted as momenta in the conjugate directions,our rules are identical to those obtained long ago via star-product calculus.The calculational aspects have been extensively explored[1,7,8,9]in the last years.Our interpretation is however different, and in this light,we will discuss now the IR/UV connection.IR/UVWe have derived directly from thefield theory the dipolar character of the NC scalarfield excitations.We saw that,in the{|x>}basis,the mo-mentum in the conjugate direction becomes the lenght of the dipole.Thus,aconnection between ultraviolet (large momentum)and infrared physics (large distances)becomes evident.This puts on a more rigorous basis the argument of [8]concerning the IR/UV connection.Moreover,we can provide a geometrical view of the differences between planar and nonplanar loop diagrams,and the role of low momenta in non-planar graphs.Let us go to (4+1)directions,t,ˆx ,ˆy ,ˆz ,ˆu ,and assume[ˆx ,ˆy ]=[ˆz ,ˆw ]=iθ.Consider a {|x,z >}basis.Then we can speak of a commutative space spanned by the axes x and z ,on which dipoles with mo-mentum p =(p x ,p z )and length l =(l x ,l z )=θ(p y ,p w )evolve.Consider the scattering of four such dipoles,Their ‘meeting place’is a poligon with four edges and area A (figure 1a).d d dd d ¨¨¨¨¨¨¨¨%f f f f f w t t t &&b z ¢¢¢¢ e e e e u eeee r r rr j¨¨¨B g g g g ¨¨¨%g g gg y Bgggg %g g g g y t t t t t t &&&&&& ¢¢¢¢¢¢¢¢¢¢A =0A =0A =0A =0Evertex area A in any basis,and is half IR and half UV.NCFT is somehow between usual FT and string theory:when the interaction vertex is a point, UV infinities appear;when it opens up,as in string theory,amplitudes are finite.RemarksWe saw that by dropping n coordinates,intuition is gained:the remaining space admits a notion of distance,although bilocal(and in some sense IR/UV dual)objects probe it.Other bases of H can also be used.For instance,the√basis{|n>},formed by eigenvectors ofˆn=√θ,space is surely NC.For r>>θ√and r<<2000-1060.References[1]M.R.Douglas and N.A.Nekrasov,Rev.Mod.Phys.73(2002)977;R.J.Szabo,hep-th/0109162;J.A.Harvey,hep-th/0102076.These reviews in-clude comprehensive lists of references.[2]A.Connes,M.R.Douglas and A.Schwarz,JHEP9802(1998)003;M.R.Douglas,C.Hull,JHEP9802(1998)008.[3]N.Seiberg and E.Witten,JHEP9909(1999)032.[4]R.Gopakumar,S.Minwalla and A.Strominger,JHEP0005(2000)020.[5]A.Hashimoto and N.Itzhaki,Phys.Rev.D64(2001)046007;Z.Guralnik,R.Jackiw,S.Y.Pi and A.P.Polychronakos,Phys.Lett.B517(2001)450;R.G.Cai,Phys.Lett.B517(2001)457.[6]C.Acatrinei,hep-th/0106006.[7]S.Minwalla,M.Van Raamsdonk and N.Seiberg,JHEP0002(2000)020.[8]A.Matusis,L.Susskind and N.Toumbas,JHEP0012(2000)002.[9]M.Van Raamsdonk and N.Seiberg,JHEP0003(2000)035;I.Ya.Aref’eva,D.M.Belov and A.S.Koshelev,Phys.Lett.B476(2000)431;M.Hayakawa,hep-th/9912167;Y.Kiem and S.Lee,Nucl.Phys.B586 (2000)303;H.Liu and J.Michelson,Phys.Rev.D62(2000)066003;J.Gomis,ndsteiner and E.Lopez,Phys.Rev.D62(2000)105006;L.Griguolo and M.Pietroni,JHEP0105(2001)032;Y.Kinar,G.Lifschytz and J.Sonnenschein,JHEP0108(2001)001;M.Van Raamsdonk,JHEP 0111(2001)006;F.Ruiz Ruiz,hep-th/0202011.[10]D.Bigatti and L.Susskind,Phys.Rev.D62(2000)066004;M.M.Sheikh-Jabbari,Phys.Lett.B455(1999)129.[11]C.S.Chu and P.M.Ho,Nucl.Phys.B550(1999)151;Z.Yin,Phys.Lett.B466(1999)234;S.Iso,H.Kawai and Y.Kitazawa,Nucl.Phys.B576 (2000)375;L.Alvarez-Gaume and J.L.F.Barbon,Int.J.Mod.Phys.A16 (2001)1123;L.Jiang and E.Nicholson,hep-th/0111145.[12]H.Weyl,The Theory of Groups and Quantum Mechanics,Dover1950.[13]C.Zachos,Int.J.Mod.Phys.A17(2002)297,and references therein.。
pwscf说明书
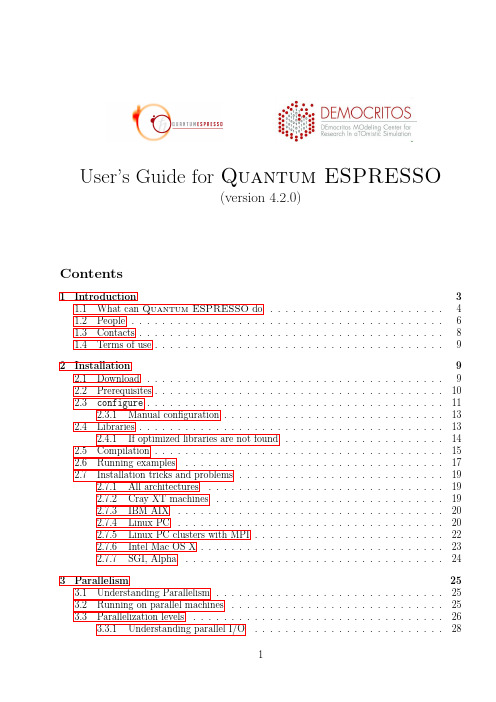
User’s Guide for Quantum ESPRESSO(version4.2.0)Contents1Introduction31.1What can Quantum ESPRESSO do (4)1.2People (6)1.3Contacts (8)1.4Terms of use (9)2Installation92.1Download (9)2.2Prerequisites (10)2.3configure (11)2.3.1Manual configuration (13)2.4Libraries (13)2.4.1If optimized libraries are not found (14)2.5Compilation (15)2.6Running examples (17)2.7Installation tricks and problems (19)2.7.1All architectures (19)2.7.2Cray XT machines (19)2.7.3IBM AIX (20)2.7.4Linux PC (20)2.7.5Linux PC clusters with MPI (22)2.7.6Intel Mac OS X (23)2.7.7SGI,Alpha (24)3Parallelism253.1Understanding Parallelism (25)3.2Running on parallel machines (25)3.3Parallelization levels (26)3.3.1Understanding parallel I/O (28)3.4Tricks and problems (29)4Using Quantum ESPRESSO314.1Input data (31)4.2Datafiles (32)4.3Format of arrays containing charge density,potential,etc (32)5Using PWscf335.1Electronic structure calculations (33)5.2Optimization and dynamics (35)5.3Nudged Elastic Band calculation (35)6Phonon calculations376.1Single-q calculation (37)6.2Calculation of interatomic force constants in real space (37)6.3Calculation of electron-phonon interaction coefficients (38)6.4Distributed Phonon calculations (38)7Post-processing397.1Plotting selected quantities (39)7.2Band structure,Fermi surface (39)7.3Projection over atomic states,DOS (39)7.4Wannier functions (40)7.5Other tools (40)8Using CP408.1Reaching the electronic ground state (42)8.2Relax the system (43)8.3CP dynamics (45)8.4Advanced usage (47)8.4.1Self-interaction Correction (47)8.4.2ensemble-DFT (48)8.4.3Treatment of USPPs (50)9Performances519.1Execution time (51)9.2Memory requirements (52)9.3File space requirements (52)9.4Parallelization issues (52)10Troubleshooting5410.1pw.x problems (54)10.2PostProc (61)10.3ph.x errors (62)11Frequently Asked Questions(F AQ)6311.1General (63)11.2Installation (63)11.3Pseudopotentials (64)11.4Input data (65)11.5Parallel execution (66)11.6Frequent errors during execution (66)11.7Self Consistency (67)11.8Phonons (69)1IntroductionThis guide covers the installation and usage of Quantum ESPRESSO(opEn-Source Package for Research in Electronic Structure,Simulation,and Optimization),version4.2.0.The Quantum ESPRESSO distribution contains the following core packages for the cal-culation of electronic-structure properties within Density-Functional Theory(DFT),using a Plane-Wave(PW)basis set and pseudopotentials(PP):•PWscf(Plane-Wave Self-Consistent Field).•CP(Car-Parrinello).It also includes the following more specialized packages:•PHonon:phonons with Density-Functional Perturbation Theory.•PostProc:various utilities for data postprocessing.•PWcond:ballistic conductance.•GIPAW(Gauge-Independent Projector Augmented Waves):EPR g-tensor and NMR chem-ical shifts.•XSPECTRA:K-edge X-ray adsorption spectra.•vdW:(experimental)dynamic polarizability.•GWW:(experimental)GW calculation using Wannier functions.The following auxiliary codes are included as well:•PWgui:a Graphical User Interface,producing input datafiles for PWscf.•atomic:a program for atomic calculations and generation of pseudopotentials.•QHA:utilities for the calculation of projected density of states(PDOS)and of the free energy in the Quasi-Harmonic Approximation(to be used in conjunction with PHonon).•PlotPhon:phonon dispersion plotting utility(to be used in conjunction with PHonon).A copy of required external libraries are included:•iotk:an Input-Output ToolKit.•PMG:Multigrid solver for Poisson equation.•BLAS and LAPACKFinally,several additional packages that exploit data produced by Quantum ESPRESSO can be installed as plug-ins:•Wannier90:maximally localized Wannier functions(/),writ-ten by A.Mostofi,J.Yates,Y.-S Lee.•WanT:quantum transport properties with Wannier functions.•YAMBO:optical excitations with Many-Body Perturbation Theory.This guide documents PWscf,CP,PHonon,PostProc.The remaining packages have separate documentation.The Quantum ESPRESSO codes work on many different types of Unix machines,in-cluding parallel machines using both OpenMP and MPI(Message Passing Interface).Running Quantum ESPRESSO on Mac OS X and MS-Windows is also possible:see section2.2.Further documentation,beyond what is provided in this guide,can be found in:•the pw forum mailing list(pw forum@).You can subscribe to this list,browse and search its archives(links in /contacts.php).Only subscribed users can post.Please search the archives before posting:your question may have already been answered.•the Doc/directory of the Quantum ESPRESSO distribution,containing a detailed de-scription of input data for most codes infiles INPUT*.txt and INPUT*.html,plus and a few additional pdf documents;people who want to contribute to Quantum ESPRESSO should read the Developer Manual,developer man.pdf.•the Quantum ESPRESSO Wiki:/wiki/index.php/Main Page.This guide does not explain solid state physics and its computational methods.If you want to learn that,you should read a good textbook,such as e.g.the book by Richard Martin: Electronic Structure:Basic Theory and Practical Methods,Cambridge University Press(2004). See also the Reference Paper section in the Wiki.This guide assume that you know the basic Unix concepts(shell,execution path,directories etc.)and utilities.If you don’t,you will have a hard time running Quantum ESPRESSO.All trademarks mentioned in this guide belong to their respective owners.1.1What can Quantum ESPRESSO doPWscf can currently perform the following kinds of calculations:•ground-state energy and one-electron(Kohn-Sham)orbitals;•atomic forces,stresses,and structural optimization;•molecular dynamics on the ground-state Born-Oppenheimer surface,also with variable cell;•Nudged Elastic Band(NEB)and Fourier String Method Dynamics(SMD)for energy barriers and reaction paths;•macroscopic polarization andfinite electricfields via the modern theory of polarization (Berry Phases).All of the above works for both insulators and metals,in any crystal structure,for many exchange-correlation(XC)functionals(including spin polarization,DFT+U,hybrid function-als),for norm-conserving(Hamann-Schluter-Chiang)PPs(NCPPs)in separable form or Ultra-soft(Vanderbilt)PPs(USPPs)or Projector Augmented Waves(PAW)method.Non-collinear magnetism and spin-orbit interactions are also implemented.An implementation offinite elec-tricfields with a sawtooth potential in a supercell is also available.PHonon can perform the following types of calculations:•phonon frequencies and eigenvectors at a generic wave vector,using Density-Functional Perturbation Theory;•effective charges and dielectric tensors;•electron-phonon interaction coefficients for metals;•interatomic force constants in real space;•third-order anharmonic phonon lifetimes;•Infrared and Raman(nonresonant)cross section.PHonon can be used whenever PWscf can be used,with the exceptions of DFT+U and hybrid functionals.PAW is not implemented for higher-order response calculations.Calculations,in the Quasi-Harmonic approximations,of the vibrational free energy can be performed using the QHA package.PostProc can perform the following types of calculations:•Scanning Tunneling Microscopy(STM)images;•plots of Electron Localization Functions(ELF);•Density of States(DOS)and Projected DOS(PDOS);•L¨o wdin charges;•planar and spherical averages;plus interfacing with a number of graphical utilities and with external codes.CP can perform Car-Parrinello molecular dynamics,including variable-cell dynamics.1.2PeopleIn the following,the cited affiliation is either the current one or the one where the last known contribution was done.The maintenance and further development of the Quantum ESPRESSO distribution is promoted by the DEMOCRITOS National Simulation Center of IOM-CNR under the coor-dination of Paolo Giannozzi(Univ.Udine,Italy)and Layla Martin-Samos(Democritos)with the strong support of the CINECA National Supercomputing Center in Bologna under the responsibility of Carlo Cavazzoni.The PWscf package(which included PHonon and PostProc in earlier releases)was origi-nally developed by Stefano Baroni,Stefano de Gironcoli,Andrea Dal Corso(SISSA),Paolo Giannozzi,and many others.We quote in particular:•Matteo Cococcioni(Univ.Minnesota)for DFT+U implementation;•David Vanderbilt’s group at Rutgers for Berry’s phase calculations;•Ralph Gebauer(ICTP,Trieste)and Adriano Mosca Conte(SISSA,Trieste)for noncolinear magnetism;•Andrea Dal Corso for spin-orbit interactions;•Carlo Sbraccia(Princeton)for NEB,Strings method,for improvements to structural optimization and to many other parts;•Paolo Umari(Democritos)forfinite electricfields;•Renata Wentzcovitch and collaborators(Univ.Minnesota)for variable-cell molecular dynamics;•Lorenzo Paulatto(Univ.Paris VI)for PAW implementation,built upon previous work by Guido Fratesi(ano Bicocca)and Riccardo Mazzarello(ETHZ-USI Lugano);•Ismaila Dabo(INRIA,Palaiseau)for electrostatics with free boundary conditions.For PHonon,we mention in particular:•Michele Lazzeri(Univ.Paris VI)for the2n+1code and Raman cross section calculation with2nd-order response;•Andrea Dal Corso for USPP,noncollinear,spin-orbit extensions to PHonon.For PostProc,we mention:•Andrea Benassi(SISSA)for the epsilon utility;•Norbert Nemec(U.Cambridge)for the pw2casino utility;•Dmitry Korotin(Inst.Met.Phys.Ekaterinburg)for the wannier ham utility.The CP package is based on the original code written by Roberto Car and Michele Parrinello. CP was developed by Alfredo Pasquarello(IRRMA,Lausanne),Kari Laasonen(Oulu),Andrea Trave,Roberto Car(Princeton),Nicola Marzari(Univ.Oxford),Paolo Giannozzi,and others. FPMD,later merged with CP,was developed by Carlo Cavazzoni,Gerardo Ballabio(CINECA), Sandro Scandolo(ICTP),Guido Chiarotti(SISSA),Paolo Focher,and others.We quote in particular:•Carlo Sbraccia(Princeton)for NEB;•Manu Sharma(Princeton)and Yudong Wu(Princeton)for maximally localized Wannier functions and dynamics with Wannier functions;•Paolo Umari(Democritos)forfinite electricfields and conjugate gradients;•Paolo Umari and Ismaila Dabo for ensemble-DFT;•Xiaofei Wang(Princeton)for META-GGA;•The Autopilot feature was implemented by Targacept,Inc.Other packages in Quantum ESPRESSO:•PWcond was written by Alexander Smogunov(SISSA)and Andrea Dal Corso.For an introduction,see http://people.sissa.it/~smogunov/PWCOND/pwcond.html•GIPAW()was written by Davide Ceresoli(MIT),Ari Seitsonen (Univ.Zurich),Uwe Gerstmann,Francesco Mauri(Univ.Paris VI).•PWgui was written by Anton Kokalj(IJS Ljubljana)and is based on his GUIB concept (http://www-k3.ijs.si/kokalj/guib/).•atomic was written by Andrea Dal Corso and it is the result of many additions to the original code by Paolo Giannozzi and others.Lorenzo Paulatto wrote the PAW extension.•iotk(http://www.s3.infm.it/iotk)was written by Giovanni Bussi(SISSA).•XSPECTRA was written by Matteo Calandra(Univ.Paris VI)and collaborators.•VdW was contributed by Huy-Viet Nguyen(SISSA).•GWW was written by Paolo Umari and Geoffrey Stenuit(Democritos).•QHA amd PlotPhon were contributed by Eyvaz Isaev(Moscow Steel and Alloy Inst.and Linkoping and Uppsala Univ.).Other relevant contributions to Quantum ESPRESSO:•Andrea Ferretti(MIT)contributed the qexml and sumpdos utility,helped withfile formats and with various problems;•Hannu-Pekka Komsa(CSEA/Lausanne)contributed the HSE functional;•Dispersions interaction in the framework of DFT-D were contributed by Daniel Forrer (Padua Univ.)and Michele Pavone(Naples Univ.Federico II);•Filippo Spiga(ano Bicocca)contributed the mixed MPI-OpenMP paralleliza-tion;•The initial BlueGene porting was done by Costas Bekas and Alessandro Curioni(IBM Zurich);•Gerardo Ballabio wrote thefirst configure for Quantum ESPRESSO•Audrius Alkauskas(IRRMA),Uli Aschauer(Princeton),Simon Binnie(Univ.College London),Guido Fratesi,Axel Kohlmeyer(UPenn),Konstantin Kudin(Princeton),Sergey Lisenkov(Univ.Arkansas),Nicolas Mounet(MIT),William Parker(Ohio State Univ), Guido Roma(CEA),Gabriele Sclauzero(SISSA),Sylvie Stucki(IRRMA),Pascal Thibaudeau (CEA),Vittorio Zecca,Federico Zipoli(Princeton)answered questions on the mailing list, found bugs,helped in porting to new architectures,wrote some code.An alphabetical list of further contributors includes:Dario Alf`e,Alain Allouche,Francesco Antoniella,Francesca Baletto,Mauro Boero,Nicola Bonini,Claudia Bungaro,Paolo Cazzato, Gabriele Cipriani,Jiayu Dai,Cesar Da Silva,Alberto Debernardi,Gernot Deinzer,Yves Ferro, Martin Hilgeman,Yosuke Kanai,Nicolas Lacorne,Stephane Lefranc,Kurt Maeder,Andrea Marini,Pasquale Pavone,Mickael Profeta,Kurt Stokbro,Paul Tangney,Antonio Tilocca,Jaro Tobik,Malgorzata Wierzbowska,Silviu Zilberman,and let us apologize to everybody we have forgotten.This guide was mostly written by Paolo Giannozzi.Gerardo Ballabio and Carlo Cavazzoni wrote the section on CP.1.3ContactsThe web site for Quantum ESPRESSO is /.Releases and patches can be downloaded from this site or following the links contained in it.The main entry point for developers is the QE-forge web site:/.The recommended place where to ask questions about installation and usage of Quantum ESPRESSO,and to report bugs,is the pw forum mailing list:pw forum@.Here you can receive news about Quantum ESPRESSO and obtain help from the developers and from knowledgeable users.You have to be subscribed in order to post to the list.Please browse or search the archive–links are available in the”Contacts”page of the Quantum ESPRESSO web site,/contacts.php–before posting: many questions are asked over and over again.NOTA BENE:only messages that appear to come from the registered user’s e-mail address,in its exact form,will be accepted.Messages”waiting for moderator approval”are automatically deleted with no further processing(sorry,too much spam).In case of trouble,carefully check that your return e-mail is the correct one(i.e.the one you used to subscribe).Since pw forum averages∼10message a day,an alternative low-traffic mailing list,pw users@,is provided for those interested only in Quantum ESPRESSO-related news,such as e.g.announcements of new versions,tutorials,etc..You can subscribe(but not post)to this list from the Quantum ESPRESSO web site.If you need to contact the developers for specific questions about coding,proposals,offersof help,etc.,send a message to the developers’mailing list:user q-e-developers,address.1.4Terms of useQuantum ESPRESSO is free software,released under the GNU General Public License. See /licenses/old-licenses/gpl-2.0.txt,or thefile License in the distribution).We shall greatly appreciate if scientific work done using this code will contain an explicit acknowledgment and the following reference:P.Giannozzi,S.Baroni,N.Bonini,M.Calandra,R.Car,C.Cavazzoni,D.Ceresoli,G.L.Chiarotti,M.Cococcioni,I.Dabo,A.Dal Corso,S.Fabris,G.Fratesi,S.deGironcoli,R.Gebauer,U.Gerstmann,C.Gougoussis,A.Kokalj,zzeri,L.Martin-Samos,N.Marzari,F.Mauri,R.Mazzarello,S.Paolini,A.Pasquarello,L.Paulatto, C.Sbraccia,S.Scandolo,G.Sclauzero, A.P.Seitsonen, A.Smo-gunov,P.Umari,R.M.Wentzcovitch,J.Phys.:Condens.Matter21,395502(2009),/abs/0906.2569Note the form Quantum ESPRESSO for textual citations of the code.Pseudopotentials should be cited as(for instance)[]We used the pseudopotentials C.pbe-rrjkus.UPF and O.pbe-vbc.UPF from.2Installation2.1DownloadPresently,Quantum ESPRESSO is only distributed in source form;some precompiled exe-cutables(binaryfiles)are provided only for PWgui.Stable releases of the Quantum ESPRESSO source package(current version is4.2.0)can be downloaded from this URL:/download.php.Uncompress and unpack the core distribution using the command:tar zxvf espresso-X.Y.Z.tar.gz(a hyphen before”zxvf”is optional)where X.Y.Z stands for the version number.If your version of tar doesn’t recognize the”z”flag:gunzip-c espresso-X.Y.Z.tar.gz|tar xvf-A directory espresso-X.Y.Z/will be created.Given the size of the complete distribution,you may need to download more packages and to unpack them following the same procedure(they will unpack into the same directory).Plug-ins should instead be downloaded into subdirectory plugin/archive but not unpacked or uncompressed:command make will take care of this during installation.Occasionally,patches for the current version,fixing some errors and bugs,may be distributed as a”diff”file.In order to install a patch(for instance):cd espresso-X.Y.Z/patch-p1</path/to/the/diff/file/patch-file.diffIf more than one patch is present,they should be applied in the correct order.Daily snapshots of the development version can be downloaded from the developers’site :follow the link”Quantum ESPRESSO”,then”SCM”.Beware:the develop-ment version is,well,under development:use at your own risk!The bravest may access the development version via anonymous CVS(Concurrent Version System):see the Developer Manual(Doc/developer man.pdf),section”Using CVS”.The Quantum ESPRESSO distribution contains several directories.Some of them are common to all packages:Modules/sourcefiles for modules that are common to all programsinclude/files*.h included by fortran and C sourcefilesclib/external libraries written in Cflib/external libraries written in Fortraniotk/Input/Output Toolkitinstall/installation scripts and utilitiespseudo/pseudopotentialfiles used by examplesupftools/converters to unified pseudopotential format(UPF)examples/sample input and outputfilesDoc/general documentationwhile others are specific to a single package:PW/PWscf:sourcefiles for scf calculations(pw.x)pwtools/PWscf:sourcefiles for miscellaneous analysis programstests/PWscf:automated testsPP/PostProc:sourcefiles for post-processing of pw.x datafilePH/PHonon:sourcefiles for phonon calculations(ph.x)and analysisGamma/PHonon:sourcefiles for Gamma-only phonon calculation(phcg.x)D3/PHonon:sourcefiles for third-order derivative calculations(d3.x)PWCOND/PWcond:sourcefiles for conductance calculations(pwcond.x)vdW/VdW:sourcefiles for molecular polarizability calculation atfinite frequency CPV/CP:sourcefiles for Car-Parrinello code(cp.x)atomic/atomic:sourcefiles for the pseudopotential generation package(ld1.x) atomic doc/Documentation,tests and examples for atomicGUI/PWGui:Graphical User Interface2.2PrerequisitesTo install Quantum ESPRESSO from source,you needfirst of all a minimal Unix envi-ronment:basically,a command shell(e.g.,bash or tcsh)and the utilities make,awk,sed. MS-Windows users need to have Cygwin(a UNIX environment which runs under Windows) installed:see /.Note that the scripts contained in the distribution assume that the local language is set to the standard,i.e.”C”;other settings may break them. Use export LC ALL=C(sh/bash)or setenv LC ALL C(csh/tcsh)to prevent any problem when running scripts(including installation scripts).Second,you need C and Fortran-95compilers.For parallel execution,you will also need MPI libraries and a“parallel”(i.e.MPI-aware)compiler.For massively parallel machines,or for simple multicore parallelization,an OpenMP-aware compiler and libraries are also required.Big machines with specialized hardware(e.g.IBM SP,CRAY,etc)typically have a Fortran-95compiler with MPI and OpenMP libraries bundled with the software.Workstations or“commodity”machines,using PC hardware,may or may not have the needed software.If not,you need either to buy a commercial product(e.g Portland)or to install an open-source compiler like gfortran or g95.Note that several commercial compilers are available free of charge under some license for academic or personal usage(e.g.Intel,Sun).2.3configureTo install the Quantum ESPRESSO source package,run the configure script.This is ac-tually a wrapper to the true configure,located in the install/subdirectory.configure will(try to)detect compilers and libraries available on your machine,and set up things accordingly. Presently it is expected to work on most Linux32-and64-bit PCs(all Intel and AMD CPUs)and PC clusters,SGI Altix,IBM SP machines,NEC SX,Cray XT machines,Mac OS X,MS-Windows PCs.It may work with some assistance also on other architectures(see below).Instructions for the impatient:cd espresso-X.Y.Z/./configuremake allSymlinks to executable programs will be placed in the bin/subdirectory.Note that both Cand Fortran compilers must be in your execution path,as specified in the PATH environment variable.Additional instructions for CRAY XT,NEC SX,Linux PowerPC machines with xlf:./configure ARCH=crayxt4./configure ARCH=necsx./configure ARCH=ppc64-mnconfigure Generates the followingfiles:install/make.sys compilation rules andflags(used by Makefile)install/configure.msg a report of the configuration run(not needed for compilation)install/config.log detailed log of the configuration run(may be needed for debugging) include/fft defs.h defines fortran variable for C pointer(used only by FFTW)include/c defs.h defines C to fortran calling conventionand a few more definitions used by CfilesNOTA BENE:unlike previous versions,configure no longer runs the makedeps.sh shell scriptthat updates dependencies.If you modify the sources,run./install/makedeps.sh or type make depend to updatefiles make.depend in the various subdirectories.You should always be able to compile the Quantum ESPRESSO suite of programs without having to edit any of the generatedfiles.However you may have to tune configure by specifying appropriate environment variables and/or command-line ually the tricky part is toget external libraries recognized and used:see Sec.2.4for details and hints.Environment variables may be set in any of these ways:export VARIABLE=value;./configure#sh,bash,kshsetenv VARIABLE value;./configure#csh,tcsh./configure VARIABLE=value#any shellSome environment variables that are relevant to configure are:ARCH label identifying the machine type(see below)F90,F77,CC names of Fortran95,Fortran77,and C compilersMPIF90name of parallel Fortran95compiler(using MPI)CPP sourcefile preprocessor(defaults to$CC-E)LD linker(defaults to$MPIF90)(C,F,F90,CPP,LD)FLAGS compilation/preprocessor/loaderflagsLIBDIRS extra directories where to search for librariesFor example,the following command line:./configure MPIF90=mpf90FFLAGS="-O2-assume byterecl"\CC=gcc CFLAGS=-O3LDFLAGS=-staticinstructs configure to use mpf90as Fortran95compiler withflags-O2-assume byterecl, gcc as C compiler withflags-O3,and to link withflag-static.Note that the value of FFLAGS must be quoted,because it contains spaces.NOTA BENE:do not pass compiler names with the leading path included.F90=f90xyz is ok,F90=/path/to/f90xyz is not.Do not use environmental variables with configure unless they are needed!try configure with no options as afirst step.If your machine type is unknown to configure,you may use the ARCH variable to suggest an architecture among supported ones.Some large parallel machines using a front-end(e.g. Cray XT)will actually need it,or else configure will correctly recognize the front-end but not the specialized compilation environment of those machines.In some cases,cross-compilation requires to specify the target machine with the--host option.This feature has not been extensively tested,but we had at least one successful report(compilation for NEC SX6on a PC).Currently supported architectures are:ia32Intel32-bit machines(x86)running Linuxia64Intel64-bit(Itanium)running Linuxx8664Intel and AMD64-bit running Linux-see note belowaix IBM AIX machinessolaris PC’s running SUN-Solarissparc Sun SPARC machinescrayxt4Cray XT4/5machinesmacppc Apple PowerPC machines running Mac OS Xmac686Apple Intel machines running Mac OS Xcygwin MS-Windows PCs with Cygwinnecsx NEC SX-6and SX-8machinesppc64Linux PowerPC machines,64bitsppc64-mn as above,with IBM xlf compilerNote:x8664replaces amd64since v.4.1.Cray Unicos machines,SGI machines with MIPS architecture,HP-Compaq Alphas are no longer supported since v.4.2.0.Finally,configure recognizes the following command-line options:--enable-parallel compile for parallel execution if possible(default:yes)--enable-openmp compile for openmp execution if possible(default:no)--enable-shared use shared libraries if available(default:yes)--disable-wrappers disable C to fortran wrapper check(default:enabled)--enable-signals enable signal trapping(default:disabled)and the following optional packages:--with-internal-blas compile with internal BLAS(default:no)--with-internal-lapack compile with internal LAPACK(default:no)--with-scalapack use ScaLAPACK if available(default:yes)If you want to modify the configure script(advanced users only!),see the Developer Manual.2.3.1Manual configurationIf configure stops before the end,and you don’tfind a way tofix it,you have to write working make.sys,include/fft defs.h and include/c defs.hfiles.For the latter twofiles,follow the explanations in include/defs.h.README.If configure has run till the end,you should need only to edit make.sys.A few templates (each for a different machine type)are provided in the install/directory:they have names of the form Make.system,where system is a string identifying the architecture and compiler.The template used by configure is also found there as make.sys.in and contains explanations of the meaning of the various variables.The difficult part will be to locate libraries.Note that you will need to select appropriate preprocessingflags in conjunction with the desired or available libraries(e.g.you need to add-D FFTW)to DFLAGS if you want to link internal FFTW).For a correct choice of preprocessingflags,refer to the documentation in include/defs.h.README.NOTA BENE:If you change any settings(e.g.preprocessing,compilationflags)after a previous(successful or failed)compilation,you must run make clean before recompiling,unless you know exactly which routines are affected by the changed settings and how to force their recompilation.2.4LibrariesQuantum ESPRESSO makes use of the following external libraries:•BLAS(/blas/)and•LAPACK(/lapack/)for linear algebra•FFTW(/)for Fast Fourier TransformsA copy of the needed routines is provided with the distribution.However,when available, optimized vendor-specific libraries should be used:this often yields huge performance gains. BLAS and LAPACK Quantum ESPRESSO can use the following architecture-specific replacements for BLAS and LAPACK:MKL for Intel Linux PCsACML for AMD Linux PCsESSL for IBM machinesSCSL for SGI AltixSUNperf for SunIf none of these is available,we suggest that you use the optimized ATLAS library:see /.Note that ATLAS is not a complete replacement for LAPACK:it contains all of the BLAS,plus the LU code,plus the full storage Cholesky code. Follow the instructions in the ATLAS distributions to produce a full LAPACK replacement.Sergei Lisenkov reported success and good performances with optimized BLAS by Kazushige Goto.They can be freely downloaded,but not redistributed.See the”GotoBLAS2”item at /tacc-projects/.FFT Quantum ESPRESSO has an internal copy of an old FFTW version,and it can use the following vendor-specific FFT libraries:IBM ESSLSGI SCSLSUN sunperfNEC ASLAMD ACMLconfigure willfirst search for vendor-specific FFT libraries;if none is found,it will search for an external FFTW v.3library;if none is found,it will fall back to the internal copy of FFTW.If you have recent versions of MKL installed,you may try the FFTW interface provided with MKL.You will have to compile them(only sources are distributed with the MKL library) and to modifyfile make.sys accordingly(MKL must be linked after the FFTW-MKL interface)MPI libraries MPI libraries are usually needed for parallel execution(unless you are happy with OpenMP multicore parallelization).In well-configured machines,configure shouldfind the appropriate parallel compiler for you,and this shouldfind the appropriate libraries.Since often this doesn’t happen,especially on PC clusters,see Sec.2.7.5.Other libraries Quantum ESPRESSO can use the MASS vector math library from IBM, if available(only on AIX).2.4.1If optimized libraries are not foundThe configure script attempts tofind optimized libraries,but may fail if they have been in-stalled in non-standard places.You should examine thefinal value of BLAS LIBS,LAPACK LIBS, FFT LIBS,MPI LIBS(if needed),MASS LIBS(IBM only),either in the output of configure or in the generated make.sys,to check whether it found all the libraries that you intend to use.If some library was not found,you can specify a list of directories to search in the envi-ronment variable LIBDIRS,and rerun configure;directories in the list must be separated by spaces.For example:./configure LIBDIRS="/opt/intel/mkl70/lib/32/usr/lib/math"If this still fails,you may set some or all of the*LIBS variables manually and retry.For example:./configure BLAS_LIBS="-L/usr/lib/math-lf77blas-latlas_sse"Beware that in this case,configure will blindly accept the specified value,and won’t do any extra search.。
热红外传感史
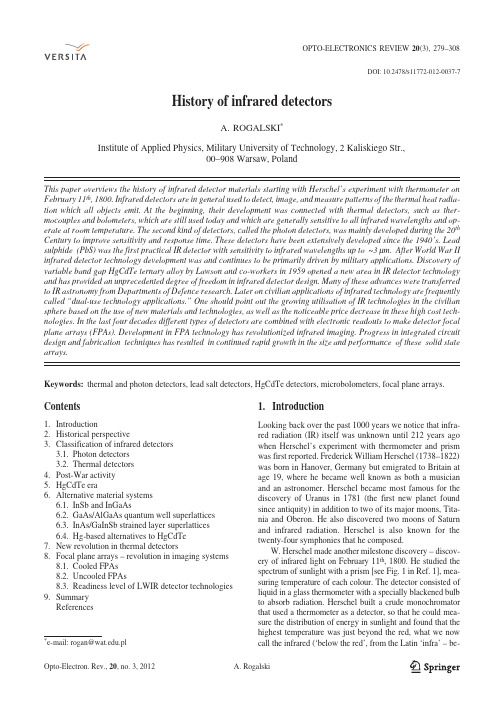
History of infrared detectorsA.ROGALSKI*Institute of Applied Physics, Military University of Technology, 2 Kaliskiego Str.,00–908 Warsaw, PolandThis paper overviews the history of infrared detector materials starting with Herschel’s experiment with thermometer on February11th,1800.Infrared detectors are in general used to detect,image,and measure patterns of the thermal heat radia−tion which all objects emit.At the beginning,their development was connected with thermal detectors,such as ther−mocouples and bolometers,which are still used today and which are generally sensitive to all infrared wavelengths and op−erate at room temperature.The second kind of detectors,called the photon detectors,was mainly developed during the20th Century to improve sensitivity and response time.These detectors have been extensively developed since the1940’s.Lead sulphide(PbS)was the first practical IR detector with sensitivity to infrared wavelengths up to~3μm.After World War II infrared detector technology development was and continues to be primarily driven by military applications.Discovery of variable band gap HgCdTe ternary alloy by Lawson and co−workers in1959opened a new area in IR detector technology and has provided an unprecedented degree of freedom in infrared detector design.Many of these advances were transferred to IR astronomy from Departments of Defence ter on civilian applications of infrared technology are frequently called“dual−use technology applications.”One should point out the growing utilisation of IR technologies in the civilian sphere based on the use of new materials and technologies,as well as the noticeable price decrease in these high cost tech−nologies.In the last four decades different types of detectors are combined with electronic readouts to make detector focal plane arrays(FPAs).Development in FPA technology has revolutionized infrared imaging.Progress in integrated circuit design and fabrication techniques has resulted in continued rapid growth in the size and performance of these solid state arrays.Keywords:thermal and photon detectors, lead salt detectors, HgCdTe detectors, microbolometers, focal plane arrays.Contents1.Introduction2.Historical perspective3.Classification of infrared detectors3.1.Photon detectors3.2.Thermal detectors4.Post−War activity5.HgCdTe era6.Alternative material systems6.1.InSb and InGaAs6.2.GaAs/AlGaAs quantum well superlattices6.3.InAs/GaInSb strained layer superlattices6.4.Hg−based alternatives to HgCdTe7.New revolution in thermal detectors8.Focal plane arrays – revolution in imaging systems8.1.Cooled FPAs8.2.Uncooled FPAs8.3.Readiness level of LWIR detector technologies9.SummaryReferences 1.IntroductionLooking back over the past1000years we notice that infra−red radiation(IR)itself was unknown until212years ago when Herschel’s experiment with thermometer and prism was first reported.Frederick William Herschel(1738–1822) was born in Hanover,Germany but emigrated to Britain at age19,where he became well known as both a musician and an astronomer.Herschel became most famous for the discovery of Uranus in1781(the first new planet found since antiquity)in addition to two of its major moons,Tita−nia and Oberon.He also discovered two moons of Saturn and infrared radiation.Herschel is also known for the twenty−four symphonies that he composed.W.Herschel made another milestone discovery–discov−ery of infrared light on February11th,1800.He studied the spectrum of sunlight with a prism[see Fig.1in Ref.1],mea−suring temperature of each colour.The detector consisted of liquid in a glass thermometer with a specially blackened bulb to absorb radiation.Herschel built a crude monochromator that used a thermometer as a detector,so that he could mea−sure the distribution of energy in sunlight and found that the highest temperature was just beyond the red,what we now call the infrared(‘below the red’,from the Latin‘infra’–be−OPTO−ELECTRONICS REVIEW20(3),279–308DOI: 10.2478/s11772−012−0037−7*e−mail: rogan@.pllow)–see Fig.1(b)[2].In April 1800he reported it to the Royal Society as dark heat (Ref.1,pp.288–290):Here the thermometer No.1rose 7degrees,in 10minu−tes,by an exposure to the full red coloured rays.I drew back the stand,till the centre of the ball of No.1was just at the vanishing of the red colour,so that half its ball was within,and half without,the visible rays of theAnd here the thermometerin 16minutes,degrees,when its centre was inch out of the raysof the sun.as had a rising of 9de−grees,and here the difference is almost too trifling to suppose,that latter situation of the thermometer was much beyond the maximum of the heating power;while,at the same time,the experiment sufficiently indi−cates,that the place inquired after need not be looked for at a greater distance.Making further experiments on what Herschel called the ‘calorific rays’that existed beyond the red part of the spec−trum,he found that they were reflected,refracted,absorbed and transmitted just like visible light [1,3,4].The early history of IR was reviewed about 50years ago in three well−known monographs [5–7].Many historical information can be also found in four papers published by Barr [3,4,8,9]and in more recently published monograph [10].Table 1summarises the historical development of infrared physics and technology [11,12].2.Historical perspectiveFor thirty years following Herschel’s discovery,very little progress was made beyond establishing that the infrared ra−diation obeyed the simplest laws of optics.Slow progress inthe study of infrared was caused by the lack of sensitive and accurate detectors –the experimenters were handicapped by the ordinary thermometer.However,towards the second de−cade of the 19th century,Thomas Johann Seebeck began to examine the junction behaviour of electrically conductive materials.In 1821he discovered that a small electric current will flow in a closed circuit of two dissimilar metallic con−ductors,when their junctions are kept at different tempera−tures [13].During that time,most physicists thought that ra−diant heat and light were different phenomena,and the dis−covery of Seebeck indirectly contributed to a revival of the debate on the nature of heat.Due to small output vol−tage of Seebeck’s junctions,some μV/K,the measurement of very small temperature differences were prevented.In 1829L.Nobili made the first thermocouple and improved electrical thermometer based on the thermoelectric effect discovered by Seebeck in 1826.Four years later,M.Melloni introduced the idea of connecting several bismuth−copper thermocouples in series,generating a higher and,therefore,measurable output voltage.It was at least 40times more sensitive than the best thermometer available and could de−tect the heat from a person at a distance of 30ft [8].The out−put voltage of such a thermopile structure linearly increases with the number of connected thermocouples.An example of thermopile’s prototype invented by Nobili is shown in Fig.2(a).It consists of twelve large bismuth and antimony elements.The elements were placed upright in a brass ring secured to an adjustable support,and were screened by a wooden disk with a 15−mm central aperture.Incomplete version of the Nobili−Melloni thermopile originally fitted with the brass cone−shaped tubes to collect ra−diant heat is shown in Fig.2(b).This instrument was much more sensi−tive than the thermometers previously used and became the most widely used detector of IR radiation for the next half century.The third member of the trio,Langley’s bolometer appea−red in 1880[7].Samuel Pierpont Langley (1834–1906)used two thin ribbons of platinum foil connected so as to form two arms of a Wheatstone bridge (see Fig.3)[15].This instrument enabled him to study solar irradiance far into its infrared region and to measure theintensityof solar radia−tion at various wavelengths [9,16,17].The bolometer’s sen−History of infrared detectorsFig.1.Herschel’s first experiment:A,B –the small stand,1,2,3–the thermometers upon it,C,D –the prism at the window,E –the spec−trum thrown upon the table,so as to bring the last quarter of an inch of the read colour upon the stand (after Ref.1).InsideSir FrederickWilliam Herschel (1738–1822)measures infrared light from the sun– artist’s impression (after Ref. 2).Fig.2.The Nobili−Meloni thermopiles:(a)thermopile’s prototype invented by Nobili (ca.1829),(b)incomplete version of the Nobili−−Melloni thermopile (ca.1831).Museo Galileo –Institute and Museum of the History of Science,Piazza dei Giudici 1,50122Florence, Italy (after Ref. 14).Table 1. Milestones in the development of infrared physics and technology (up−dated after Refs. 11 and 12)Year Event1800Discovery of the existence of thermal radiation in the invisible beyond the red by W. HERSCHEL1821Discovery of the thermoelectric effects using an antimony−copper pair by T.J. SEEBECK1830Thermal element for thermal radiation measurement by L. NOBILI1833Thermopile consisting of 10 in−line Sb−Bi thermal pairs by L. NOBILI and M. MELLONI1834Discovery of the PELTIER effect on a current−fed pair of two different conductors by J.C. PELTIER1835Formulation of the hypothesis that light and electromagnetic radiation are of the same nature by A.M. AMPERE1839Solar absorption spectrum of the atmosphere and the role of water vapour by M. MELLONI1840Discovery of the three atmospheric windows by J. HERSCHEL (son of W. HERSCHEL)1857Harmonization of the three thermoelectric effects (SEEBECK, PELTIER, THOMSON) by W. THOMSON (Lord KELVIN)1859Relationship between absorption and emission by G. KIRCHHOFF1864Theory of electromagnetic radiation by J.C. MAXWELL1873Discovery of photoconductive effect in selenium by W. SMITH1876Discovery of photovoltaic effect in selenium (photopiles) by W.G. ADAMS and A.E. DAY1879Empirical relationship between radiation intensity and temperature of a blackbody by J. STEFAN1880Study of absorption characteristics of the atmosphere through a Pt bolometer resistance by S.P. LANGLEY1883Study of transmission characteristics of IR−transparent materials by M. MELLONI1884Thermodynamic derivation of the STEFAN law by L. BOLTZMANN1887Observation of photoelectric effect in the ultraviolet by H. HERTZ1890J. ELSTER and H. GEITEL constructed a photoemissive detector consisted of an alkali−metal cathode1894, 1900Derivation of the wavelength relation of blackbody radiation by J.W. RAYEIGH and W. WIEN1900Discovery of quantum properties of light by M. PLANCK1903Temperature measurements of stars and planets using IR radiometry and spectrometry by W.W. COBLENTZ1905 A. EINSTEIN established the theory of photoelectricity1911R. ROSLING made the first television image tube on the principle of cathode ray tubes constructed by F. Braun in 18971914Application of bolometers for the remote exploration of people and aircrafts ( a man at 200 m and a plane at 1000 m)1917T.W. CASE developed the first infrared photoconductor from substance composed of thallium and sulphur1923W. SCHOTTKY established the theory of dry rectifiers1925V.K. ZWORYKIN made a television image tube (kinescope) then between 1925 and 1933, the first electronic camera with the aid of converter tube (iconoscope)1928Proposal of the idea of the electro−optical converter (including the multistage one) by G. HOLST, J.H. DE BOER, M.C. TEVES, and C.F. VEENEMANS1929L.R. KOHLER made a converter tube with a photocathode (Ag/O/Cs) sensitive in the near infrared1930IR direction finders based on PbS quantum detectors in the wavelength range 1.5–3.0 μm for military applications (GUDDEN, GÖRLICH and KUTSCHER), increased range in World War II to 30 km for ships and 7 km for tanks (3–5 μm)1934First IR image converter1939Development of the first IR display unit in the United States (Sniperscope, Snooperscope)1941R.S. OHL observed the photovoltaic effect shown by a p−n junction in a silicon1942G. EASTMAN (Kodak) offered the first film sensitive to the infrared1947Pneumatically acting, high−detectivity radiation detector by M.J.E. GOLAY1954First imaging cameras based on thermopiles (exposure time of 20 min per image) and on bolometers (4 min)1955Mass production start of IR seeker heads for IR guided rockets in the US (PbS and PbTe detectors, later InSb detectors for Sidewinder rockets)1957Discovery of HgCdTe ternary alloy as infrared detector material by W.D. LAWSON, S. NELSON, and A.S. YOUNG1961Discovery of extrinsic Ge:Hg and its application (linear array) in the first LWIR FLIR systems1965Mass production start of IR cameras for civil applications in Sweden (single−element sensors with optomechanical scanner: AGA Thermografiesystem 660)1970Discovery of charge−couple device (CCD) by W.S. BOYLE and G.E. SMITH1970Production start of IR sensor arrays (monolithic Si−arrays: R.A. SOREF 1968; IR−CCD: 1970; SCHOTTKY diode arrays: F.D.SHEPHERD and A.C. YANG 1973; IR−CMOS: 1980; SPRITE: T. ELIOTT 1981)1975Lunch of national programmes for making spatially high resolution observation systems in the infrared from multielement detectors integrated in a mini cooler (so−called first generation systems): common module (CM) in the United States, thermal imaging commonmodule (TICM) in Great Britain, syteme modulaire termique (SMT) in France1975First In bump hybrid infrared focal plane array1977Discovery of the broken−gap type−II InAs/GaSb superlattices by G.A. SAI−HALASZ, R. TSU, and L. ESAKI1980Development and production of second generation systems [cameras fitted with hybrid HgCdTe(InSb)/Si(readout) FPAs].First demonstration of two−colour back−to−back SWIR GaInAsP detector by J.C. CAMPBELL, A.G. DENTAI, T.P. LEE,and C.A. BURRUS1985Development and mass production of cameras fitted with Schottky diode FPAs (platinum silicide)1990Development and production of quantum well infrared photoconductor (QWIP) hybrid second generation systems1995Production start of IR cameras with uncooled FPAs (focal plane arrays; microbolometer−based and pyroelectric)2000Development and production of third generation infrared systemssitivity was much greater than that of contemporary thermo−piles which were little improved since their use by Melloni. Langley continued to develop his bolometer for the next20 years(400times more sensitive than his first efforts).His latest bolometer could detect the heat from a cow at a dis−tance of quarter of mile [9].From the above information results that at the beginning the development of the IR detectors was connected with ther−mal detectors.The first photon effect,photoconductive ef−fect,was discovered by Smith in1873when he experimented with selenium as an insulator for submarine cables[18].This discovery provided a fertile field of investigation for several decades,though most of the efforts were of doubtful quality. By1927,over1500articles and100patents were listed on photosensitive selenium[19].It should be mentioned that the literature of the early1900’s shows increasing interest in the application of infrared as solution to numerous problems[7].A special contribution of William Coblenz(1873–1962)to infrared radiometry and spectroscopy is marked by huge bib−liography containing hundreds of scientific publications, talks,and abstracts to his credit[20,21].In1915,W.Cob−lentz at the US National Bureau of Standards develops ther−mopile detectors,which he uses to measure the infrared radi−ation from110stars.However,the low sensitivity of early in−frared instruments prevented the detection of other near−IR sources.Work in infrared astronomy remained at a low level until breakthroughs in the development of new,sensitive infrared detectors were achieved in the late1950’s.The principle of photoemission was first demonstrated in1887when Hertz discovered that negatively charged par−ticles were emitted from a conductor if it was irradiated with ultraviolet[22].Further studies revealed that this effect could be produced with visible radiation using an alkali metal electrode [23].Rectifying properties of semiconductor−metal contact were discovered by Ferdinand Braun in1874[24],when he probed a naturally−occurring lead sulphide(galena)crystal with the point of a thin metal wire and noted that current flowed freely in one direction only.Next,Jagadis Chandra Bose demonstrated the use of galena−metal point contact to detect millimetre electromagnetic waves.In1901he filed a U.S patent for a point−contact semiconductor rectifier for detecting radio signals[25].This type of contact called cat’s whisker detector(sometimes also as crystal detector)played serious role in the initial phase of radio development.How−ever,this contact was not used in a radiation detector for the next several decades.Although crystal rectifiers allowed to fabricate simple radio sets,however,by the mid−1920s the predictable performance of vacuum−tubes replaced them in most radio applications.The period between World Wars I and II is marked by the development of photon detectors and image converters and by emergence of infrared spectroscopy as one of the key analytical techniques available to chemists.The image con−verter,developed on the eve of World War II,was of tre−mendous interest to the military because it enabled man to see in the dark.The first IR photoconductor was developed by Theodore W.Case in1917[26].He discovered that a substance com−posed of thallium and sulphur(Tl2S)exhibited photocon−ductivity.Supported by the US Army between1917and 1918,Case adapted these relatively unreliable detectors for use as sensors in an infrared signalling device[27].The pro−totype signalling system,consisting of a60−inch diameter searchlight as the source of radiation and a thallous sulphide detector at the focus of a24−inch diameter paraboloid mir−ror,sent messages18miles through what was described as ‘smoky atmosphere’in1917.However,instability of resis−tance in the presence of light or polarizing voltage,loss of responsivity due to over−exposure to light,high noise,slug−gish response and lack of reproducibility seemed to be inhe−rent weaknesses.Work was discontinued in1918;commu−nication by the detection of infrared radiation appeared dis−tinctly ter Case found that the addition of oxygen greatly enhanced the response [28].The idea of the electro−optical converter,including the multistage one,was proposed by Holst et al.in1928[29]. The first attempt to make the converter was not successful.A working tube consisted of a photocathode in close proxi−mity to a fluorescent screen was made by the authors in 1934 in Philips firm.In about1930,the appearance of the Cs−O−Ag photo−tube,with stable characteristics,to great extent discouraged further development of photoconductive cells until about 1940.The Cs−O−Ag photocathode(also called S−1)elabo−History of infrared detectorsFig.3.Longley’s bolometer(a)composed of two sets of thin plati−num strips(b),a Wheatstone bridge,a battery,and a galvanometer measuring electrical current (after Ref. 15 and 16).rated by Koller and Campbell[30]had a quantum efficiency two orders of magnitude above anything previously studied, and consequently a new era in photoemissive devices was inaugurated[31].In the same year,the Japanese scientists S. Asao and M.Suzuki reported a method for enhancing the sensitivity of silver in the S−1photocathode[32].Consisted of a layer of caesium on oxidized silver,S−1is sensitive with useful response in the near infrared,out to approxi−mately1.2μm,and the visible and ultraviolet region,down to0.3μm.Probably the most significant IR development in the United States during1930’s was the Radio Corporation of America(RCA)IR image tube.During World War II, near−IR(NIR)cathodes were coupled to visible phosphors to provide a NIR image converter.With the establishment of the National Defence Research Committee,the develop−ment of this tube was accelerated.In1942,the tube went into production as the RCA1P25image converter(see Fig.4).This was one of the tubes used during World War II as a part of the”Snooperscope”and”Sniperscope,”which were used for night observation with infrared sources of illumination.Since then various photocathodes have been developed including bialkali photocathodes for the visible region,multialkali photocathodes with high sensitivity ex−tending to the infrared region and alkali halide photocatho−des intended for ultraviolet detection.The early concepts of image intensification were not basically different from those today.However,the early devices suffered from two major deficiencies:poor photo−cathodes and poor ter development of both cathode and coupling technologies changed the image in−tensifier into much more useful device.The concept of image intensification by cascading stages was suggested independently by number of workers.In Great Britain,the work was directed toward proximity focused tubes,while in the United State and in Germany–to electrostatically focused tubes.A history of night vision imaging devices is given by Biberman and Sendall in monograph Electro−Opti−cal Imaging:System Performance and Modelling,SPIE Press,2000[10].The Biberman’s monograph describes the basic trends of infrared optoelectronics development in the USA,Great Britain,France,and Germany.Seven years later Ponomarenko and Filachev completed this monograph writ−ing the book Infrared Techniques and Electro−Optics in Russia:A History1946−2006,SPIE Press,about achieve−ments of IR techniques and electrooptics in the former USSR and Russia [33].In the early1930’s,interest in improved detectors began in Germany[27,34,35].In1933,Edgar W.Kutzscher at the University of Berlin,discovered that lead sulphide(from natural galena found in Sardinia)was photoconductive and had response to about3μm.B.Gudden at the University of Prague used evaporation techniques to develop sensitive PbS films.Work directed by Kutzscher,initially at the Uni−versity of Berlin and later at the Electroacustic Company in Kiel,dealt primarily with the chemical deposition approach to film formation.This work ultimately lead to the fabrica−tion of the most sensitive German detectors.These works were,of course,done under great secrecy and the results were not generally known until after1945.Lead sulphide photoconductors were brought to the manufacturing stage of development in Germany in about1943.Lead sulphide was the first practical infrared detector deployed in a variety of applications during the war.The most notable was the Kiel IV,an airborne IR system that had excellent range and which was produced at Carl Zeiss in Jena under the direction of Werner K. Weihe [6].In1941,Robert J.Cashman improved the technology of thallous sulphide detectors,which led to successful produc−tion[36,37].Cashman,after success with thallous sulphide detectors,concentrated his efforts on lead sulphide detec−tors,which were first produced in the United States at Northwestern University in1944.After World War II Cash−man found that other semiconductors of the lead salt family (PbSe and PbTe)showed promise as infrared detectors[38]. The early detector cells manufactured by Cashman are shown in Fig. 5.Fig.4.The original1P25image converter tube developed by the RCA(a).This device measures115×38mm overall and has7pins.It opera−tion is indicated by the schematic drawing (b).After1945,the wide−ranging German trajectory of research was essentially the direction continued in the USA, Great Britain and Soviet Union under military sponsorship after the war[27,39].Kutzscher’s facilities were captured by the Russians,thus providing the basis for early Soviet detector development.From1946,detector technology was rapidly disseminated to firms such as Mullard Ltd.in Southampton,UK,as part of war reparations,and some−times was accompanied by the valuable tacit knowledge of technical experts.E.W.Kutzscher,for example,was flown to Britain from Kiel after the war,and subsequently had an important influence on American developments when he joined Lockheed Aircraft Co.in Burbank,California as a research scientist.Although the fabrication methods developed for lead salt photoconductors was usually not completely under−stood,their properties are well established and reproducibi−lity could only be achieved after following well−tried reci−pes.Unlike most other semiconductor IR detectors,lead salt photoconductive materials are used in the form of polycrys−talline films approximately1μm thick and with individual crystallites ranging in size from approximately0.1–1.0μm. They are usually prepared by chemical deposition using empirical recipes,which generally yields better uniformity of response and more stable results than the evaporative methods.In order to obtain high−performance detectors, lead chalcogenide films need to be sensitized by oxidation. The oxidation may be carried out by using additives in the deposition bath,by post−deposition heat treatment in the presence of oxygen,or by chemical oxidation of the film. The effect of the oxidant is to introduce sensitizing centres and additional states into the bandgap and thereby increase the lifetime of the photoexcited holes in the p−type material.3.Classification of infrared detectorsObserving a history of the development of the IR detector technology after World War II,many materials have been investigated.A simple theorem,after Norton[40],can be stated:”All physical phenomena in the range of about0.1–1 eV will be proposed for IR detectors”.Among these effects are:thermoelectric power(thermocouples),change in elec−trical conductivity(bolometers),gas expansion(Golay cell), pyroelectricity(pyroelectric detectors),photon drag,Jose−phson effect(Josephson junctions,SQUIDs),internal emis−sion(PtSi Schottky barriers),fundamental absorption(in−trinsic photodetectors),impurity absorption(extrinsic pho−todetectors),low dimensional solids[superlattice(SL), quantum well(QW)and quantum dot(QD)detectors], different type of phase transitions, etc.Figure6gives approximate dates of significant develop−ment efforts for the materials mentioned.The years during World War II saw the origins of modern IR detector tech−nology.Recent success in applying infrared technology to remote sensing problems has been made possible by the successful development of high−performance infrared de−tectors over the last six decades.Photon IR technology com−bined with semiconductor material science,photolithogra−phy technology developed for integrated circuits,and the impetus of Cold War military preparedness have propelled extraordinary advances in IR capabilities within a short time period during the last century [41].The majority of optical detectors can be classified in two broad categories:photon detectors(also called quantum detectors) and thermal detectors.3.1.Photon detectorsIn photon detectors the radiation is absorbed within the material by interaction with electrons either bound to lattice atoms or to impurity atoms or with free electrons.The observed electrical output signal results from the changed electronic energy distribution.The photon detectors show a selective wavelength dependence of response per unit incident radiation power(see Fig.8).They exhibit both a good signal−to−noise performance and a very fast res−ponse.But to achieve this,the photon IR detectors require cryogenic cooling.This is necessary to prevent the thermalHistory of infrared detectorsFig.5.Cashman’s detector cells:(a)Tl2S cell(ca.1943):a grid of two intermeshing comb−line sets of conducting paths were first pro−vided and next the T2S was evaporated over the grid structure;(b) PbS cell(ca.1945)the PbS layer was evaporated on the wall of the tube on which electrical leads had been drawn with aquadag(afterRef. 38).。
From Data Mining to Knowledge Discovery in Databases
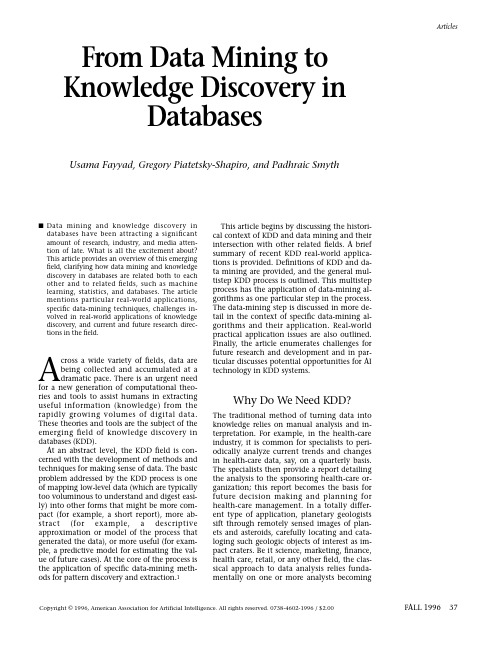
s Data mining and knowledge discovery in databases have been attracting a significant amount of research, industry, and media atten-tion of late. What is all the excitement about?This article provides an overview of this emerging field, clarifying how data mining and knowledge discovery in databases are related both to each other and to related fields, such as machine learning, statistics, and databases. The article mentions particular real-world applications, specific data-mining techniques, challenges in-volved in real-world applications of knowledge discovery, and current and future research direc-tions in the field.A cross a wide variety of fields, data arebeing collected and accumulated at adramatic pace. There is an urgent need for a new generation of computational theo-ries and tools to assist humans in extracting useful information (knowledge) from the rapidly growing volumes of digital data. These theories and tools are the subject of the emerging field of knowledge discovery in databases (KDD).At an abstract level, the KDD field is con-cerned with the development of methods and techniques for making sense of data. The basic problem addressed by the KDD process is one of mapping low-level data (which are typically too voluminous to understand and digest easi-ly) into other forms that might be more com-pact (for example, a short report), more ab-stract (for example, a descriptive approximation or model of the process that generated the data), or more useful (for exam-ple, a predictive model for estimating the val-ue of future cases). At the core of the process is the application of specific data-mining meth-ods for pattern discovery and extraction.1This article begins by discussing the histori-cal context of KDD and data mining and theirintersection with other related fields. A briefsummary of recent KDD real-world applica-tions is provided. Definitions of KDD and da-ta mining are provided, and the general mul-tistep KDD process is outlined. This multistepprocess has the application of data-mining al-gorithms as one particular step in the process.The data-mining step is discussed in more de-tail in the context of specific data-mining al-gorithms and their application. Real-worldpractical application issues are also outlined.Finally, the article enumerates challenges forfuture research and development and in par-ticular discusses potential opportunities for AItechnology in KDD systems.Why Do We Need KDD?The traditional method of turning data intoknowledge relies on manual analysis and in-terpretation. For example, in the health-careindustry, it is common for specialists to peri-odically analyze current trends and changesin health-care data, say, on a quarterly basis.The specialists then provide a report detailingthe analysis to the sponsoring health-care or-ganization; this report becomes the basis forfuture decision making and planning forhealth-care management. In a totally differ-ent type of application, planetary geologistssift through remotely sensed images of plan-ets and asteroids, carefully locating and cata-loging such geologic objects of interest as im-pact craters. Be it science, marketing, finance,health care, retail, or any other field, the clas-sical approach to data analysis relies funda-mentally on one or more analysts becomingArticlesFALL 1996 37From Data Mining to Knowledge Discovery inDatabasesUsama Fayyad, Gregory Piatetsky-Shapiro, and Padhraic Smyth Copyright © 1996, American Association for Artificial Intelligence. All rights reserved. 0738-4602-1996 / $2.00areas is astronomy. Here, a notable success was achieved by SKICAT ,a system used by as-tronomers to perform image analysis,classification, and cataloging of sky objects from sky-survey images (Fayyad, Djorgovski,and Weir 1996). In its first application, the system was used to process the 3 terabytes (1012bytes) of image data resulting from the Second Palomar Observatory Sky Survey,where it is estimated that on the order of 109sky objects are detectable. SKICAT can outper-form humans and traditional computational techniques in classifying faint sky objects. See Fayyad, Haussler, and Stolorz (1996) for a sur-vey of scientific applications.In business, main KDD application areas includes marketing, finance (especially in-vestment), fraud detection, manufacturing,telecommunications, and Internet agents.Marketing:In marketing, the primary ap-plication is database marketing systems,which analyze customer databases to identify different customer groups and forecast their behavior. Business Week (Berry 1994) estimat-ed that over half of all retailers are using or planning to use database marketing, and those who do use it have good results; for ex-ample, American Express reports a 10- to 15-percent increase in credit-card use. Another notable marketing application is market-bas-ket analysis (Agrawal et al. 1996) systems,which find patterns such as, “If customer bought X, he/she is also likely to buy Y and Z.” Such patterns are valuable to retailers.Investment: Numerous companies use da-ta mining for investment, but most do not describe their systems. One exception is LBS Capital Management. Its system uses expert systems, neural nets, and genetic algorithms to manage portfolios totaling $600 million;since its start in 1993, the system has outper-formed the broad stock market (Hall, Mani,and Barr 1996).Fraud detection: HNC Falcon and Nestor PRISM systems are used for monitoring credit-card fraud, watching over millions of ac-counts. The FAIS system (Senator et al. 1995),from the U.S. Treasury Financial Crimes En-forcement Network, is used to identify finan-cial transactions that might indicate money-laundering activity.Manufacturing: The CASSIOPEE trou-bleshooting system, developed as part of a joint venture between General Electric and SNECMA, was applied by three major Euro-pean airlines to diagnose and predict prob-lems for the Boeing 737. To derive families of faults, clustering methods are used. CASSIOPEE received the European first prize for innova-intimately familiar with the data and serving as an interface between the data and the users and products.For these (and many other) applications,this form of manual probing of a data set is slow, expensive, and highly subjective. In fact, as data volumes grow dramatically, this type of manual data analysis is becoming completely impractical in many domains.Databases are increasing in size in two ways:(1) the number N of records or objects in the database and (2) the number d of fields or at-tributes to an object. Databases containing on the order of N = 109objects are becoming in-creasingly common, for example, in the as-tronomical sciences. Similarly, the number of fields d can easily be on the order of 102or even 103, for example, in medical diagnostic applications. Who could be expected to di-gest millions of records, each having tens or hundreds of fields? We believe that this job is certainly not one for humans; hence, analysis work needs to be automated, at least partially.The need to scale up human analysis capa-bilities to handling the large number of bytes that we can collect is both economic and sci-entific. Businesses use data to gain competi-tive advantage, increase efficiency, and pro-vide more valuable services to customers.Data we capture about our environment are the basic evidence we use to build theories and models of the universe we live in. Be-cause computers have enabled humans to gather more data than we can digest, it is on-ly natural to turn to computational tech-niques to help us unearth meaningful pat-terns and structures from the massive volumes of data. Hence, KDD is an attempt to address a problem that the digital informa-tion era made a fact of life for all of us: data overload.Data Mining and Knowledge Discovery in the Real WorldA large degree of the current interest in KDD is the result of the media interest surrounding successful KDD applications, for example, the focus articles within the last two years in Business Week , Newsweek , Byte , PC Week , and other large-circulation periodicals. Unfortu-nately, it is not always easy to separate fact from media hype. Nonetheless, several well-documented examples of successful systems can rightly be referred to as KDD applications and have been deployed in operational use on large-scale real-world problems in science and in business.In science, one of the primary applicationThere is an urgent need for a new generation of computation-al theories and tools toassist humans in extractinguseful information (knowledge)from the rapidly growing volumes ofdigital data.Articles38AI MAGAZINEtive applications (Manago and Auriol 1996).Telecommunications: The telecommuni-cations alarm-sequence analyzer (TASA) wasbuilt in cooperation with a manufacturer oftelecommunications equipment and threetelephone networks (Mannila, Toivonen, andVerkamo 1995). The system uses a novelframework for locating frequently occurringalarm episodes from the alarm stream andpresenting them as rules. Large sets of discov-ered rules can be explored with flexible infor-mation-retrieval tools supporting interactivityand iteration. In this way, TASA offers pruning,grouping, and ordering tools to refine the re-sults of a basic brute-force search for rules.Data cleaning: The MERGE-PURGE systemwas applied to the identification of duplicatewelfare claims (Hernandez and Stolfo 1995).It was used successfully on data from the Wel-fare Department of the State of Washington.In other areas, a well-publicized system isIBM’s ADVANCED SCOUT,a specialized data-min-ing system that helps National Basketball As-sociation (NBA) coaches organize and inter-pret data from NBA games (U.S. News 1995). ADVANCED SCOUT was used by several of the NBA teams in 1996, including the Seattle Su-personics, which reached the NBA finals.Finally, a novel and increasingly importanttype of discovery is one based on the use of in-telligent agents to navigate through an infor-mation-rich environment. Although the ideaof active triggers has long been analyzed in thedatabase field, really successful applications ofthis idea appeared only with the advent of theInternet. These systems ask the user to specifya profile of interest and search for related in-formation among a wide variety of public-do-main and proprietary sources. For example, FIREFLY is a personal music-recommendation agent: It asks a user his/her opinion of several music pieces and then suggests other music that the user might like (<http:// www.ffl/>). CRAYON(/>) allows users to create their own free newspaper (supported by ads); NEWSHOUND(<http://www. /hound/>) from the San Jose Mercury News and FARCAST(</> automatically search information from a wide variety of sources, including newspapers and wire services, and e-mail rele-vant documents directly to the user.These are just a few of the numerous suchsystems that use KDD techniques to automat-ically produce useful information from largemasses of raw data. See Piatetsky-Shapiro etal. (1996) for an overview of issues in devel-oping industrial KDD applications.Data Mining and KDDHistorically, the notion of finding useful pat-terns in data has been given a variety ofnames, including data mining, knowledge ex-traction, information discovery, informationharvesting, data archaeology, and data patternprocessing. The term data mining has mostlybeen used by statisticians, data analysts, andthe management information systems (MIS)communities. It has also gained popularity inthe database field. The phrase knowledge dis-covery in databases was coined at the first KDDworkshop in 1989 (Piatetsky-Shapiro 1991) toemphasize that knowledge is the end productof a data-driven discovery. It has been popular-ized in the AI and machine-learning fields.In our view, KDD refers to the overall pro-cess of discovering useful knowledge from da-ta, and data mining refers to a particular stepin this process. Data mining is the applicationof specific algorithms for extracting patternsfrom data. The distinction between the KDDprocess and the data-mining step (within theprocess) is a central point of this article. Theadditional steps in the KDD process, such asdata preparation, data selection, data cleaning,incorporation of appropriate prior knowledge,and proper interpretation of the results ofmining, are essential to ensure that usefulknowledge is derived from the data. Blind ap-plication of data-mining methods (rightly crit-icized as data dredging in the statistical litera-ture) can be a dangerous activity, easilyleading to the discovery of meaningless andinvalid patterns.The Interdisciplinary Nature of KDDKDD has evolved, and continues to evolve,from the intersection of research fields such asmachine learning, pattern recognition,databases, statistics, AI, knowledge acquisitionfor expert systems, data visualization, andhigh-performance computing. The unifyinggoal is extracting high-level knowledge fromlow-level data in the context of large data sets.The data-mining component of KDD cur-rently relies heavily on known techniquesfrom machine learning, pattern recognition,and statistics to find patterns from data in thedata-mining step of the KDD process. A natu-ral question is, How is KDD different from pat-tern recognition or machine learning (and re-lated fields)? The answer is that these fieldsprovide some of the data-mining methodsthat are used in the data-mining step of theKDD process. KDD focuses on the overall pro-cess of knowledge discovery from data, includ-ing how the data are stored and accessed, howalgorithms can be scaled to massive data setsThe basicproblemaddressed bythe KDDprocess isone ofmappinglow-leveldata intoother formsthat might bemorecompact,moreabstract,or moreuseful.ArticlesFALL 1996 39A driving force behind KDD is the database field (the second D in KDD). Indeed, the problem of effective data manipulation when data cannot fit in the main memory is of fun-damental importance to KDD. Database tech-niques for gaining efficient data access,grouping and ordering operations when ac-cessing data, and optimizing queries consti-tute the basics for scaling algorithms to larger data sets. Most data-mining algorithms from statistics, pattern recognition, and machine learning assume data are in the main memo-ry and pay no attention to how the algorithm breaks down if only limited views of the data are possible.A related field evolving from databases is data warehousing,which refers to the popular business trend of collecting and cleaning transactional data to make them available for online analysis and decision support. Data warehousing helps set the stage for KDD in two important ways: (1) data cleaning and (2)data access.Data cleaning: As organizations are forced to think about a unified logical view of the wide variety of data and databases they pos-sess, they have to address the issues of map-ping data to a single naming convention,uniformly representing and handling missing data, and handling noise and errors when possible.Data access: Uniform and well-defined methods must be created for accessing the da-ta and providing access paths to data that were historically difficult to get to (for exam-ple, stored offline).Once organizations and individuals have solved the problem of how to store and ac-cess their data, the natural next step is the question, What else do we do with all the da-ta? This is where opportunities for KDD natu-rally arise.A popular approach for analysis of data warehouses is called online analytical processing (OLAP), named for a set of principles pro-posed by Codd (1993). OLAP tools focus on providing multidimensional data analysis,which is superior to SQL in computing sum-maries and breakdowns along many dimen-sions. OLAP tools are targeted toward simpli-fying and supporting interactive data analysis,but the goal of KDD tools is to automate as much of the process as possible. Thus, KDD is a step beyond what is currently supported by most standard database systems.Basic DefinitionsKDD is the nontrivial process of identifying valid, novel, potentially useful, and ultimate-and still run efficiently, how results can be in-terpreted and visualized, and how the overall man-machine interaction can usefully be modeled and supported. The KDD process can be viewed as a multidisciplinary activity that encompasses techniques beyond the scope of any one particular discipline such as machine learning. In this context, there are clear opportunities for other fields of AI (be-sides machine learning) to contribute to KDD. KDD places a special emphasis on find-ing understandable patterns that can be inter-preted as useful or interesting knowledge.Thus, for example, neural networks, although a powerful modeling tool, are relatively difficult to understand compared to decision trees. KDD also emphasizes scaling and ro-bustness properties of modeling algorithms for large noisy data sets.Related AI research fields include machine discovery, which targets the discovery of em-pirical laws from observation and experimen-tation (Shrager and Langley 1990) (see Kloes-gen and Zytkow [1996] for a glossary of terms common to KDD and machine discovery),and causal modeling for the inference of causal models from data (Spirtes, Glymour,and Scheines 1993). Statistics in particular has much in common with KDD (see Elder and Pregibon [1996] and Glymour et al.[1996] for a more detailed discussion of this synergy). Knowledge discovery from data is fundamentally a statistical endeavor. Statistics provides a language and framework for quan-tifying the uncertainty that results when one tries to infer general patterns from a particu-lar sample of an overall population. As men-tioned earlier, the term data mining has had negative connotations in statistics since the 1960s when computer-based data analysis techniques were first introduced. The concern arose because if one searches long enough in any data set (even randomly generated data),one can find patterns that appear to be statis-tically significant but, in fact, are not. Clearly,this issue is of fundamental importance to KDD. Substantial progress has been made in recent years in understanding such issues in statistics. Much of this work is of direct rele-vance to KDD. Thus, data mining is a legiti-mate activity as long as one understands how to do it correctly; data mining carried out poorly (without regard to the statistical as-pects of the problem) is to be avoided. KDD can also be viewed as encompassing a broader view of modeling than statistics. KDD aims to provide tools to automate (to the degree pos-sible) the entire process of data analysis and the statistician’s “art” of hypothesis selection.Data mining is a step in the KDD process that consists of ap-plying data analysis and discovery al-gorithms that produce a par-ticular enu-meration ofpatterns (or models)over the data.Articles40AI MAGAZINEly understandable patterns in data (Fayyad, Piatetsky-Shapiro, and Smyth 1996).Here, data are a set of facts (for example, cases in a database), and pattern is an expres-sion in some language describing a subset of the data or a model applicable to the subset. Hence, in our usage here, extracting a pattern also designates fitting a model to data; find-ing structure from data; or, in general, mak-ing any high-level description of a set of data. The term process implies that KDD comprises many steps, which involve data preparation, search for patterns, knowledge evaluation, and refinement, all repeated in multiple itera-tions. By nontrivial, we mean that some search or inference is involved; that is, it is not a straightforward computation of predefined quantities like computing the av-erage value of a set of numbers.The discovered patterns should be valid on new data with some degree of certainty. We also want patterns to be novel (at least to the system and preferably to the user) and poten-tially useful, that is, lead to some benefit to the user or task. Finally, the patterns should be understandable, if not immediately then after some postprocessing.The previous discussion implies that we can define quantitative measures for evaluating extracted patterns. In many cases, it is possi-ble to define measures of certainty (for exam-ple, estimated prediction accuracy on new data) or utility (for example, gain, perhaps indollars saved because of better predictions orspeedup in response time of a system). No-tions such as novelty and understandabilityare much more subjective. In certain contexts,understandability can be estimated by sim-plicity (for example, the number of bits to de-scribe a pattern). An important notion, calledinterestingness(for example, see Silberschatzand Tuzhilin [1995] and Piatetsky-Shapiro andMatheus [1994]), is usually taken as an overallmeasure of pattern value, combining validity,novelty, usefulness, and simplicity. Interest-ingness functions can be defined explicitly orcan be manifested implicitly through an or-dering placed by the KDD system on the dis-covered patterns or models.Given these notions, we can consider apattern to be knowledge if it exceeds some in-terestingness threshold, which is by nomeans an attempt to define knowledge in thephilosophical or even the popular view. As amatter of fact, knowledge in this definition ispurely user oriented and domain specific andis determined by whatever functions andthresholds the user chooses.Data mining is a step in the KDD processthat consists of applying data analysis anddiscovery algorithms that, under acceptablecomputational efficiency limitations, pro-duce a particular enumeration of patterns (ormodels) over the data. Note that the space ofArticlesFALL 1996 41Figure 1. An Overview of the Steps That Compose the KDD Process.methods, the effective number of variables under consideration can be reduced, or in-variant representations for the data can be found.Fifth is matching the goals of the KDD pro-cess (step 1) to a particular data-mining method. For example, summarization, clas-sification, regression, clustering, and so on,are described later as well as in Fayyad, Piatet-sky-Shapiro, and Smyth (1996).Sixth is exploratory analysis and model and hypothesis selection: choosing the data-mining algorithm(s) and selecting method(s)to be used for searching for data patterns.This process includes deciding which models and parameters might be appropriate (for ex-ample, models of categorical data are differ-ent than models of vectors over the reals) and matching a particular data-mining method with the overall criteria of the KDD process (for example, the end user might be more in-terested in understanding the model than its predictive capabilities).Seventh is data mining: searching for pat-terns of interest in a particular representa-tional form or a set of such representations,including classification rules or trees, regres-sion, and clustering. The user can significant-ly aid the data-mining method by correctly performing the preceding steps.Eighth is interpreting mined patterns, pos-sibly returning to any of steps 1 through 7 for further iteration. This step can also involve visualization of the extracted patterns and models or visualization of the data given the extracted models.Ninth is acting on the discovered knowl-edge: using the knowledge directly, incorpo-rating the knowledge into another system for further action, or simply documenting it and reporting it to interested parties. This process also includes checking for and resolving po-tential conflicts with previously believed (or extracted) knowledge.The KDD process can involve significant iteration and can contain loops between any two steps. The basic flow of steps (al-though not the potential multitude of itera-tions and loops) is illustrated in figure 1.Most previous work on KDD has focused on step 7, the data mining. However, the other steps are as important (and probably more so) for the successful application of KDD in practice. Having defined the basic notions and introduced the KDD process, we now focus on the data-mining component,which has, by far, received the most atten-tion in the literature.patterns is often infinite, and the enumera-tion of patterns involves some form of search in this space. Practical computational constraints place severe limits on the sub-space that can be explored by a data-mining algorithm.The KDD process involves using the database along with any required selection,preprocessing, subsampling, and transforma-tions of it; applying data-mining methods (algorithms) to enumerate patterns from it;and evaluating the products of data mining to identify the subset of the enumerated pat-terns deemed knowledge. The data-mining component of the KDD process is concerned with the algorithmic means by which pat-terns are extracted and enumerated from da-ta. The overall KDD process (figure 1) in-cludes the evaluation and possible interpretation of the mined patterns to de-termine which patterns can be considered new knowledge. The KDD process also in-cludes all the additional steps described in the next section.The notion of an overall user-driven pro-cess is not unique to KDD: analogous propos-als have been put forward both in statistics (Hand 1994) and in machine learning (Brod-ley and Smyth 1996).The KDD ProcessThe KDD process is interactive and iterative,involving numerous steps with many deci-sions made by the user. Brachman and Anand (1996) give a practical view of the KDD pro-cess, emphasizing the interactive nature of the process. Here, we broadly outline some of its basic steps:First is developing an understanding of the application domain and the relevant prior knowledge and identifying the goal of the KDD process from the customer’s viewpoint.Second is creating a target data set: select-ing a data set, or focusing on a subset of vari-ables or data samples, on which discovery is to be performed.Third is data cleaning and preprocessing.Basic operations include removing noise if appropriate, collecting the necessary informa-tion to model or account for noise, deciding on strategies for handling missing data fields,and accounting for time-sequence informa-tion and known changes.Fourth is data reduction and projection:finding useful features to represent the data depending on the goal of the task. With di-mensionality reduction or transformationArticles42AI MAGAZINEThe Data-Mining Stepof the KDD ProcessThe data-mining component of the KDD pro-cess often involves repeated iterative applica-tion of particular data-mining methods. This section presents an overview of the primary goals of data mining, a description of the methods used to address these goals, and a brief description of the data-mining algo-rithms that incorporate these methods.The knowledge discovery goals are defined by the intended use of the system. We can distinguish two types of goals: (1) verification and (2) discovery. With verification,the sys-tem is limited to verifying the user’s hypothe-sis. With discovery,the system autonomously finds new patterns. We further subdivide the discovery goal into prediction,where the sys-tem finds patterns for predicting the future behavior of some entities, and description, where the system finds patterns for presenta-tion to a user in a human-understandableform. In this article, we are primarily con-cerned with discovery-oriented data mining.Data mining involves fitting models to, or determining patterns from, observed data. The fitted models play the role of inferred knowledge: Whether the models reflect useful or interesting knowledge is part of the over-all, interactive KDD process where subjective human judgment is typically required. Two primary mathematical formalisms are used in model fitting: (1) statistical and (2) logical. The statistical approach allows for nondeter-ministic effects in the model, whereas a logi-cal model is purely deterministic. We focus primarily on the statistical approach to data mining, which tends to be the most widely used basis for practical data-mining applica-tions given the typical presence of uncertain-ty in real-world data-generating processes.Most data-mining methods are based on tried and tested techniques from machine learning, pattern recognition, and statistics: classification, clustering, regression, and so on. The array of different algorithms under each of these headings can often be bewilder-ing to both the novice and the experienced data analyst. It should be emphasized that of the many data-mining methods advertised in the literature, there are really only a few fun-damental techniques. The actual underlying model representation being used by a particu-lar method typically comes from a composi-tion of a small number of well-known op-tions: polynomials, splines, kernel and basis functions, threshold-Boolean functions, and so on. Thus, algorithms tend to differ primar-ily in the goodness-of-fit criterion used toevaluate model fit or in the search methodused to find a good fit.In our brief overview of data-mining meth-ods, we try in particular to convey the notionthat most (if not all) methods can be viewedas extensions or hybrids of a few basic tech-niques and principles. We first discuss the pri-mary methods of data mining and then showthat the data- mining methods can be viewedas consisting of three primary algorithmiccomponents: (1) model representation, (2)model evaluation, and (3) search. In the dis-cussion of KDD and data-mining methods,we use a simple example to make some of thenotions more concrete. Figure 2 shows a sim-ple two-dimensional artificial data set consist-ing of 23 cases. Each point on the graph rep-resents a person who has been given a loanby a particular bank at some time in the past.The horizontal axis represents the income ofthe person; the vertical axis represents the to-tal personal debt of the person (mortgage, carpayments, and so on). The data have beenclassified into two classes: (1) the x’s repre-sent persons who have defaulted on theirloans and (2) the o’s represent persons whoseloans are in good status with the bank. Thus,this simple artificial data set could represent ahistorical data set that can contain usefulknowledge from the point of view of thebank making the loans. Note that in actualKDD applications, there are typically manymore dimensions (as many as several hun-dreds) and many more data points (manythousands or even millions).ArticlesFALL 1996 43Figure 2. A Simple Data Set with Two Classes Used for Illustrative Purposes.。
蛋白组学操作步骤

MAN0000518
User Manual
Table of Contents
Kit Contents and Storage.................................................................................................................................. iv
SILAC Protein Identification (ID) and Quantitation Kits
For identifying and quantifying phosphoproteins and membrane proteins
Catalog no. SP10001, SM10002, SP10005, SM10006 MS10030, MS10031, MS10032, MS10033
SILAC™ Phosphontents
The kit contents, shipping, and storage for SILAC™ Phosphoprotein and Membrane Protein ID and Quantitation Kits are listed below. For a detailed description of kit contents, see page 4. These kits include appropriate media components, amino acids, and Lysis Buffer. Store all media protected from light. SP10001 SP10005 SM10002 SM10006 Shipping Blue ice Blue ice Dry ice Dry ice Blue ice Blue ice Blue ice Blue ice Blue ice Blue ice Storage 4 C 4 C –20C –20C 4 C 4 C 4 C –20C 4 C 4 C
开启片剂完整性的窗户(中英文对照)
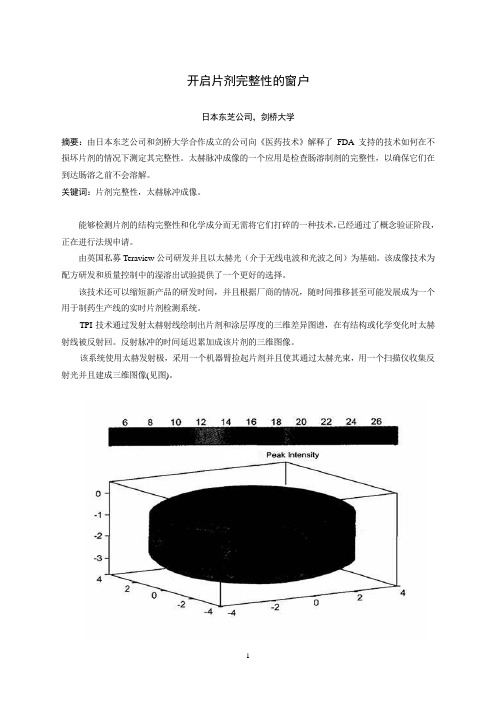
开启片剂完整性的窗户日本东芝公司,剑桥大学摘要:由日本东芝公司和剑桥大学合作成立的公司向《医药技术》解释了FDA支持的技术如何在不损坏片剂的情况下测定其完整性。
太赫脉冲成像的一个应用是检查肠溶制剂的完整性,以确保它们在到达肠溶之前不会溶解。
关键词:片剂完整性,太赫脉冲成像。
能够检测片剂的结构完整性和化学成分而无需将它们打碎的一种技术,已经通过了概念验证阶段,正在进行法规申请。
由英国私募Teraview公司研发并且以太赫光(介于无线电波和光波之间)为基础。
该成像技术为配方研发和质量控制中的湿溶出试验提供了一个更好的选择。
该技术还可以缩短新产品的研发时间,并且根据厂商的情况,随时间推移甚至可能发展成为一个用于制药生产线的实时片剂检测系统。
TPI技术通过发射太赫射线绘制出片剂和涂层厚度的三维差异图谱,在有结构或化学变化时太赫射线被反射回。
反射脉冲的时间延迟累加成该片剂的三维图像。
该系统使用太赫发射极,采用一个机器臂捡起片剂并且使其通过太赫光束,用一个扫描仪收集反射光并且建成三维图像(见图)。
技术研发太赫技术发源于二十世纪九十年代中期13本东芝公司位于英国的东芝欧洲研究中心,该中心与剑桥大学的物理学系有着密切的联系。
日本东芝公司当时正在研究新一代的半导体,研究的副产品是发现了这些半导体实际上是太赫光非常好的发射源和检测器。
二十世纪九十年代后期,日本东芝公司授权研究小组寻求该技术可能的应用,包括成像和化学传感光谱学,并与葛兰素史克和辉瑞以及其它公司建立了关系,以探讨其在制药业的应用。
虽然早期的结果表明该技术有前景,但日本东芝公司却不愿深入研究下去,原因是此应用与日本东芝公司在消费电子行业的任何业务兴趣都没有交叉。
这一决定的结果是研究中心的首席执行官DonArnone和剑桥桥大学物理学系的教授Michael Pepper先生于2001年成立了Teraview公司一作为研究中心的子公司。
TPI imaga 2000是第一个商品化太赫成像系统,该系统经优化用于成品片剂及其核心完整性和性能的无破坏检测。
chatgpt在科研领域的应用英语范文

chatgpt在科研领域的应用英语范文In the realm of scientific research, the application of AI-driven tools like ChatGPT has revolutionized the way data is analyzed, experiments are conducted, and findings are disseminated. The integration of such technology has not only streamlined processes but also fostered a new era of innovation and collaboration.ChatGPT, with its advanced natural language processing capabilities, serves as an invaluable asset for researchers across various disciplines. Its ability to understand and generate human-like text allows for the automation of literature reviews, hypothesis generation, and even the drafting of research papers. This AI model can sift through vast databases of scientific literature within seconds, identifying relevant studies, summarizing findings, and highlighting gaps in the research. Such efficiency in handling information enables scientists to stay abreast of the latest developments without the overwhelming task of manually reviewing each publication.Moreover, ChatGPT's conversational interface provides a user-friendly platform for brainstorming sessions. Researchers can interact with the AI to refine their research questions, explore alternative methodologies, and consider different perspectives on their subject matter. This interactive process not only saves time but also inspires creative approaches to problem-solving.In experimental design, ChatGPT can assist in creating robust methodologies by suggesting variables, conditions, and statistical models that align with the research objectives. It can also simulate potential outcomes, helping researchers to anticipate challenges and plan accordingly. This predictive aspect of ChatGPT ensures that experiments are well-structured and that resources are utilized effectively.The role of ChatGPT extends into the realm of data analysis as well. It can be programmed to perform complex statistical analyses, interpret results, and even generate graphs and charts that succinctly convey the findings. By automating these technical aspects, researchers can focus on the broader implications of their work and engage in more strategic thinking.Collaboration is another area where ChatGPT makes a significant impact. It facilitates seamless communication between researchers, regardless of geographical barriers. The AI can translate discussions, manage project tasks, and ensure that all team members are aligned with the project goals. This level of coordination is particularly beneficial for large-scale, multi-institutional research projects that require synchronized efforts.Furthermore, ChatGPT aids in the dissemination of research findings. It can draft abstracts, prepare manuscripts for publication, and even suggest suitable journals for submission. The AI's understanding of language nuances ensures that the research is presented in a clear and compelling manner, increasing the likelihood of acceptance by peer-reviewed journals.In education and outreach, ChatGPT serves as an educational tool, explaining complex scientific concepts in simpler terms. This makes science more accessible to the public and fosters a greater understanding of research outcomes. It also acts as a mentorfor young researchers, guiding them through the intricacies of scientific inquiry and publication.In conclusion, the application of ChatGPT in scientific research is multifaceted and profoundly beneficial. It enhances efficiency, fosters creativity, and promotes collaboration, ultimately accelerating the pace of scientific discovery. As AI technology continues to evolve, its integration into research practices will undoubtedly deepen, paving the way for more groundbreaking advancements in the field. The future of scientific research, with AI companions like ChatGPT, looks more promising than ever. 。
the intensional qualification of quantification
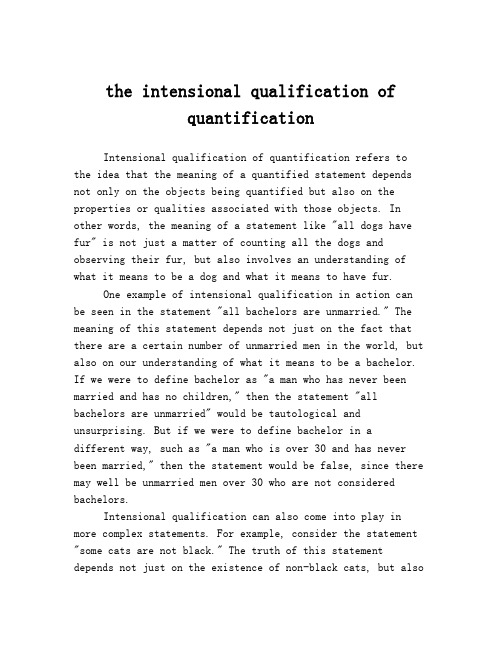
the intensional qualification ofquantificationIntensional qualification of quantification refers to the idea that the meaning of a quantified statement depends not only on the objects being quantified but also on the properties or qualities associated with those objects. In other words, the meaning of a statement like "all dogs have fur" is not just a matter of counting all the dogs and observing their fur, but also involves an understanding of what it means to be a dog and what it means to have fur.One example of intensional qualification in action can be seen in the statement "all bachelors are unmarried." The meaning of this statement depends not just on the fact that there are a certain number of unmarried men in the world, but also on our understanding of what it means to be a bachelor. If we were to define bachelor as "a man who has never been married and has no children," then the statement "all bachelors are unmarried" would be tautological and unsurprising. But if we were to define bachelor in adifferent way, such as "a man who is over 30 and has never been married," then the statement would be false, since there may well be unmarried men over 30 who are not considered bachelors.Intensional qualification can also come into play in more complex statements. For example, consider the statement "some cats are not black." The truth of this statement depends not just on the existence of non-black cats, but alsoon our understanding of what it means to be a cat and what it means to be black. If we were to define cat as "a small,furry animal that meows," and black as "a color associated with darkness," then the statement would be true, since there are certainly cats that are not black. But if we define cat more narrowly as "a member of the Felidae family," and black more broadly as "any color that is not white," then the statement might be false, as all members of the Felidae family are typically black, brown, or orange.Overall, intensional qualification of quantification highlights the importance of context and interpretation in understanding the true meaning of statements involving quantifiers. By considering not just the objects being quantified, but also the qualities or properties associated with those objects, we can arrive at a more nuanced and accurate understanding of the world around us.。
application of confocal

Light and confocal micrographs showing cellular and nuclear morphology in human preimplantation embryos. Nuclei are labeled DAPI (blue). (A) Fragmenting day 2 human embryo, with fragments arrowed. (B) Nuclei from a day 6 blastocyst showing TUNEL-labeled (pink) fragmented nuclei
Nile red标记细胞内脂滴,蓝色为细胞核
利用相应的特异荧光控针标记后 观察单个细胞不同部位或不同组织区域接受 刺激后的整个变化过程
(一)实时定量测定细胞内Ca2+的变化 实时定量测定细胞内Ca2+的变化 (二)测定细胞内PH变化 测定细胞内PH变化 (三) 检测膜电位的变化 (四)检测细胞内活性氧物种的产生 (五)检测药物等跨膜进入组织或细胞过程及其 定位 (六)检测荧光共振能量转移(FRET) 检测荧光共振能量转移(FRET) (七)检测荧光漂白恢复(FRAP) 检测荧光漂白恢复(FRAP)
骨架(微丝,微管和中等纤维) 骨架(微丝,微管和中等纤维) 直标: 一抗+荧光探针( 肌动蛋白actin 直标: 一抗+荧光探针(如:肌动蛋白actin Rhodamine-phalloidin) ) 间标: 二抗+荧光探针( 微管蛋白tublin) 间标: 二抗+荧光探针(如:微管蛋白tublin)
形态学观测
(一)在细胞原位检测核酸 (二)原位检测蛋白质,抗体及其他分 原位检测蛋白质, 子 (三)检测细胞凋亡 (四)细胞器观察及测定 (五)检测细胞融合 (六)观察细胞骨架 (七)检测细胞间缝隙连接通讯 (八)检测细胞内脂肪
- 1、下载文档前请自行甄别文档内容的完整性,平台不提供额外的编辑、内容补充、找答案等附加服务。
- 2、"仅部分预览"的文档,不可在线预览部分如存在完整性等问题,可反馈申请退款(可完整预览的文档不适用该条件!)。
- 3、如文档侵犯您的权益,请联系客服反馈,我们会尽快为您处理(人工客服工作时间:9:00-18:30)。
(9)
ቤተ መጻሕፍቲ ባይዱ(10)
The covariant derivatives of the reper fields and the symplectic metric are zero. By looking at the description of the classical dynamics of the system under consideration, it can
ωAB (Γ) = ha A ωab hb B ,
(0)
a, b = 1, 2, ..., 2N ;
(6)
ab where ω(0) and ωab define a constant symplectic metric which we will assume that it has the form
D ∇C V A ≡ ∂C V A + ∆A CD V ,
(8)
D a where ∆D CA ≡ h a ∂C h A . The commutator of the covariant derivatives is given by the following relations
[∇A , ∇B ] = −ΛC AB ∇C , where
⋆
Theoretical Physics Department, Aristotle University of Thessaloniki 54124 Thessaloniki, Greece
♮
Instituto de F´ ısica y Matem´ aticas, UMSNH Edificio C–3, Ciudad Universitaria, Morelia, Mich. CP 58040 M´ exico
ωab =
(0)
0 IN −IN 0
,
(7)
where IN stands for the unit matrix of dimension N . The covariant derivatives in the phase space are defined as follows ∇C VA ≡ ∂C VA − ∆D CA VD ,
Abstract The canonical quantization of dynamical systems with curved phase space introduced by I.A. Batalin, E.S. Fradkin and T.E. Fradkina is applied to the four– dimensional Einstein–Maxwell Dilaton–Axion theory. The spherically symmetric case with radial fields is considered. The Lagrangian density of the theory in the Einstein frame is written as an expression with first order in time derivatives of the fields. The phase space is curved due to the nontrivial interaction of the dilaton with the axion and the electromagnetic fields.
where ∂A ≡ ∂/∂ ΓA . Next, the reper field with contravariant components hA a (Γ) and its inverse ha A (Γ) are introduced as follows
ab B ω AB (Γ) = hA a ω(0) h b, (0)
The nondegenerate tensor ωAB (Γ) defines on the phase space manifold the covariant components of the symplectic metric. Also it is antisymmetric and satisfies the Jacobi identity. The Poisson bracket for any two functions X (Γ) and Y (Γ) of the phase space variables (1) is defined as follows: {X, Y } = ∂A Xω AB ∂B Y, (5)
3
be verified that the equations of motion written in terms of the Poisson brackets (5) can be derived from the action S= where ω AB (Γ) ≡ ωA (αΓ)αdα. (12) ˙ B − H0 (Γ) dt, ΓA ω AB (Γ)Γ (11)
Application of the canonical quantization of systems with curved phase space to the EMDA theory
J.E. Paschalis⋆,1 and A. Herrera–Aguilar⋆,♮, 2
arXiv:hep-th/0406089v1 10 Jun 2004
2
The Euler–Lagrange equations for the Lagrangian (2) are given by the following relations ˙B = ωAB (Γ)Γ where ωAB (Γ) = ∂ ∂ aB (Γ) − aA (Γ). A ∂Γ ∂ ΓA (4) ∂ H0 (Γ), ∂ ΓA (3)
of certain manifold M. We assume that the dynamical system is unconstrained. The extension of the formalism to systems with constraints is straightforward. The Lagrangian of the system can be presented as an expression with first order in time derivatives (denoted by a dot over the variable) of the phase space variables ΓA [5] ˙ A − H0 (Γ). L = aA (Γ)Γ (2)
2
Outline of the method
Let there be a dynamical system described by the original Hamiltonian H0 = H0 (Γ) given as a function of 2N bosonic phase variables ΓA , A = 1, 2, ..., 2N, (1)
PACS numbers:
1 2
E-mail: paschali@f.auth.gr E-mail: aherrera@auth.gr
1
1
Introduction
The main idea of I.A. Batalin, E.S. Fradkin and T.E. Fradkina [1]–[2] for the canonical quantization of systems with curved phase space consists of performing a dimensionality doubling of the original phase space by introducing a set of new variables, equal in number to the variables of the original phase space, so that each one of them is defined as the conjugate canonical momentum to each original phase variable. Further, the complete set of variables is subjected to special second class constraints in such a way that the formal exclusion of the new canonical momenta reduces the system back to the original phase space. It turns out that the new phase space is flat and its quantization proceeds along the lines of [3]–[4]. In this paper we shall apply this method to the bosonic sector of the truncated four– dimensional effective field theory of the heterotic string at tree level, better known as Einstein–Maxwell Dilaton–Axion (EMDA) theory. Since this truncated effective theory contains just massless bosonic modes, we shall consider a suitable purely bosonic model. The paper is organized as follows: in Sec. 2 we present a brief outline of the generalized canonical quantization method for dynamical systems. We keep the notation and terminology of the authors. In Sec. 3 we consider the action of the four–dimensional EMDA theory, perform the ADM decomposition of the metric and write the Lagrangian density of the matter sector as an expression with first order in time derivatives of the fields. We further consider the spherically symmetric anzats and obtain a Lagrangian density which defines a curved phase space and possesses two irreducible first class constraints. We continue by canonically quantizing the resulting EMDA system along the lines of [3]–[4] in Sec. 4. In order to achieve this aim, a suitable generalization of the method has been performed. We sketch our conclusions in Sec. 5 and, finally, we present some useful mathematical identities and relationships in the Appendix A.