Prescriptions for the scaling variable of the nucleon structure function in nuclei
MRS文献

European Journal of Radiology 67(2008)218–229ReviewThe principles of quantification applied toin vivo proton MR spectroscopyGunther Helms ∗MR-Research in Neurology and Psychiatry,Faculty of Medicine,University of G¨o ttingen,D-37075G¨o ttingen,GermanyReceived 27February 2008;accepted 28February 2008AbstractFollowing the identification of metabolite signals in the in vivo MR spectrum,quantification is the procedure to estimate numerical values oftheir concentrations.The two essential steps are discussed in detail:analysis by fitting a model of prior knowledge,that is,the decomposition of the spectrum into the signals of singular metabolites;then,normalization of these signals to yield concentration estimates.Special attention is given to using the in vivo water signal as internal reference.©2008Elsevier Ireland Ltd.All rights reserved.Keywords:MRS;Brain;Quantification;QAContents1.Introduction ............................................................................................................2192.Spectral analysis/decomposition..........................................................................................2192.1.Principles........................................................................................................2192.2.Statistical and systematic fitting errors ..............................................................................2212.3.Examples of analysis software......................................................................................2212.3.1.LCModel ................................................................................................2212.3.2.jMRUI...................................................................................................2213.Signal normalization ....................................................................................................2233.1.Principles........................................................................................................2233.2.Internal referencing and metabolite ratios............................................................................2233.3.External referencing...............................................................................................2233.4.Global transmitter reference........................................................................................2233.5.Local flip angle...................................................................................................2243.6.Coil impedance effects ............................................................................................2243.7.External phantom and local reference ...............................................................................2253.8.Receive only-coils ................................................................................................2253.9.Internal water reference............................................................................................2253.10.Partial volume correction.........................................................................................2264.Calibration .............................................................................................................2275.Discussion..............................................................................................................2286.Experimental ...........................................................................................................2287.Recommendations.......................................................................................................228Acknowledgements .....................................................................................................229References .............................................................................................................229∗Tel.:+495513913132;fax:+495513913243.E-mail address:ghelms@gwdg.de .0720-048X/$–see front matter ©2008Elsevier Ireland Ltd.All rights reserved.doi:10.1016/j.ejrad.2008.02.034G.Helms/European Journal of Radiology67(2008)218–2292191.IntroductionIn vivo MRS is a quantitative technique.This statement is often mentioned in the introduction to clinical MRS studies. However,the quantification of signal produced by the MR imag-ing system is a complex and rather technical issue.Inconsistent terminology and scores of different approaches make the prob-lem appear even more complicated,especially for beginners. This article is intended to give a structured introduction to the principles of quantification.The associated problems and pos-sible systematic errors(“bias”)are explained to encourage a critical appraisal of published results.Quantification is essential for clinical research,less so for adding diagnostic information for which visual inspection often may suffice.Subsequent to the identification of metabolites,its foremost rationale is to provide numbers for comparison of spec-tra from different subjects and brain regions;and–ideally–different scanners and sequences.These numbers are then used for evaluation;e.g.statistical comparison of cohorts or correla-tion with clinical parameters.The problem is that the interaction of the radio-frequency(RF)hardware and the dielectric load of the subject’s body may lead to rather large signal variations(up to30%)that may blur systematic relationships to cohorts or clinical parameters.One of the purposes of quantification is to reduce such hardware related variation in the numbers.Thus, quantification is closely related to quality assurance(QA).In summary,quantification is a procedure of data processing. The post-processing scheme may require additional data acqui-sitions or extraction of adjustment parameters from the scanner. The natural order of steps in the procedure is1.acquisition and pre-processing of raw data,reconstruction ofthe spectrum(e.g.averaging and FFT),2.analysis:estimation of the relative signal for each identifiedmetabolite(here,proton numbers and linewidth should be taken into account),3.normalization of RF-induced signal variations,4.calibration of signals by performing the quantificationscheme on a standard of known concentration.In turn,these steps yield the metabolite signals1.for visual inspection of the displayed spectrum on the ppmscale,2.in arbitrary units,from which metabolite ratios can be cal-culated,3.in institutional units(for your individual MR scanner andquantification scheme;these numbers are proportional to the concentration),4.in absolute units of concentration(commonly inmM=mmol/l);estimated by comparison to a standard of known concentration.The term quantification(or sometimes“quantitation”)is occasionally used to denote singular steps of this process.In this review,it will refer to the whole procedure,and further differ-entiation is made for the sake of clarity.In practice,some these steps may be performed together.Already at this stage it should be made clear that the numbers obtained by“absolute quantifica-tion”are by no means“absolute”but depend on the accuracy and precision of steps1–4.Measurement and reconstruction(step1) must be performed in a consistent way lest additional errors have to be accounted for in individual experiments.Only in theory it should be possible to correct all possible sources of variation;in clinical practice it is generally is too time consum-ing.Yet the more sources of variation are cancelled(starting with the biggest effects)the smaller effects one will be able to detect.Emphasis will be put on the analysis(the models and the automated tools available),the signal normalization(and basic quality assurance issues),and the use of the localized water signal as internal reference.2.Spectral analysis/decomposition2.1.PrinciplesThe in vivo spectrum becomes more complicated with decreasing echo time(TE):next to the singlet resonances and weakly coupled multiplets,signals from strongly coupled metabolites and baseline humps from motion-restricted macro-molecules appear.Contrary to long-TE spectra short-TE spectra should not be evaluated step-by-step and line-by-line.For exam-ple,the left line of the lactate doublet is superposed onto the macromolecular signal at1.4ppm.The total signal at this fre-quency is not of interest but rather the separate contributions of lactate and macromolecules/lipids.Differences between the two whole resonance patterns can be used to separate the metabolites;e.g.the doublet of lactate versus the broad linewidth.In visual inspection,one intuitively uses such‘prior knowledge’about the expected metabolites to discern partly overlying metabolites in a qualitative way.This approach is also used to simplify the problem to automaticallyfind the metabolite resonances to order to evaluate the whole spectrum“in one go”.Comparing the resonance pattern of MR spectra in vivo at highfield and short TE with those of tissue extracts and sin-gle metabolites in vitro at matchedfield strengths hasfirmly established our‘prior’knowledge about which metabolites con-tribute to the in vivo MR spectra[1].Next to TE,thefield strength exerts the second biggest influence on the appearance of in vivo MR spectra.Overlap and degeneration of binomial multiplets due to strong coupling increase at the lowerfield strengths of clinical MR systems(commonly3,2,or1.5T). These effects can be either measured on solutions of single metabolites[2]or simulated fromfirst quantum-mechanical principles,once the chemical shifts and coupling constants(J in Hz)of a certain metabolite have been determined at suffi-ciently highfield[3].Motion-restricted‘macromolecules’are subject to rapid relaxation that blurs the coupling pattern(if the linewidth1/πT∗2>J)and hampers the identification of specific compounds.These usually appear as broad‘humps’that form the unresolved baseline of short-TE spectra(Fig.1).These vanish at longer TE(>135ms).The baseline underlying the metabo-220G.Helms /European Journal of Radiology 67(2008)218–229Fig.1.Including lipids/macromolecules into the basis set.Without inclusion of lipids/macromolecules in the basis set (A)the broad “humps”at 1.3and 0.9ppm are fitted by the baseline.Inclusion of lipids/macromolecules (B)resulted in a better fit and a lower baseline between 2.2and 0.6ppm.The SNR improved from 26to 30.The signals at 2.0ppm partly replaced the co-resonating tNAA.The 6%reduction in tNAA was larger than the fitting error (3%).This may illustrate that the fitting error does not account for the bias in the model.LCModel (exp.details:6.1-0;12.5ml VOI in parietal GM,3T,STEAM,TE/TM/TR/avg =20/10/6000/64).lite signals is constituted from all rapidly relaxing signals that have not decayed to zero at the chosen TE (macromolecules and lipids),the “feet”of the residual water signal,plus possible arte-facts (e.g.echo signals from moving spins that were not fully suppressed by gradient selection).The ‘prior knowledge’about which metabolites to detect and how the baseline will look like is used to construct a math-ematical model to describe the spectrum.Selecting the input signals reduces the complexity of the analysis problem.In con-trast to integrating or fitting singlet lines the whole spectrum is evaluated together (“in one go”)by fitting a superposition of metabolite signals and baseline signals.Thus,the in vivo spec-trum is decomposed into the constituents of the model.Without specifying the resonances this is often too complicated to be per-formed successfully,in the sense that an unaccountable number of ‘best’combinations exist.G.Helms/European Journal of Radiology67(2008)218–229221Prior knowledge may be implemented in the metabolite basis set adapting experimental data(like in LCModel[2]),theoretical patterns simulated fromfirst principles(QUEST[4]),or purely phenomenological functions like a superposition of Gaussians of different width to model strongly coupled signals and baseline humps alike(AMARES[5]).The least squaresfit may be per-formed in either time domain[6]or frequency domain or both [7].For an in-depth discussion of technical details,the reader is referred to a special issue of NMR in Biomedicine(NMR Biomed14[4];2001)dedicated to“quantitation”(in the sense of spectrum analysis)by mathematical methods.2.2.Statistical and systematicfitting errorsModelfitting yields the contribution of each input signal. Usually Cr´a mer–Rao lower bounds(CLRB)are provided as an estimate for thefitting error or the statistical uncertainty of the concentration estimate.These are calculated from the residual error and the Fisher matrix of the partial derivatives of the con-centrations.In the same way,correlations between the input data can be estimated.Overlapping input signals(e.g.from glutamate (Glu)and glutamine(Gln))are inversely correlated.In this case, the sum has a smaller error than the single metabolites.The uncertainties are fairly well proportional to the noise level(both must be given in the same units).The models are always an approximate,but never a com-plete description of the in vivo MR spectrum.Every model thus involves some kind of systematic error or“bias”,in the sense of deviation from the unknown“true”concentration.Contrary to the statistical uncertainty,the bias cannot be assessed within the same model.In particular,the CRLB does not account for the bias.Changes in the model(e.g.,by leaving out a minor metabo-lite)may result in systematic differences that soon become significant(by a paired t-test).These are caused by the pro-cess of minimizing the squared residual difference whenfitting the same data by two different models.Spurious artefacts or“nuisance signals”that are not included in the model will results in errors that are neither statistical nor systematic.It is also useful to know,that for every non-linear function(as used in MRS)there is a critical signal-to-noise (SNR)threshold for convergence onto meaningful values.2.3.Examples of analysis softwareA number of models and algorithms have been published dur-ing the past15years.A few are available to the public and shared by a considerable number of users.These program packages are generally combined with some automated or interactive pre-processing features,such as correction of frequency offset,zero andfirst order,as well as eddy-current induced phase errors.We shall in brief describe the most common programs for analysis of in vivo1H MRS data.2.3.1.LCModelThe Linear Combination Model(LCModel)[2]comes as stand-alone commercial software(/ lcmodel).It comprises automated pre-processing to achieve a high degree of user-independence.An advanced regularization ensures convergence for the vast majority of in vivo spectra.It was thefirst program designed tofit a basis set(or library)of experimental single metabolite spectra to incorporate maximum information and uniqueness.This means that partly overlap-ping spectra(again such as,Glu and Gln)are discerned by their unique features,but show some residual correlation as mentioned above.Proton numbers are accounted for,even“frac-tional proton numbers”in“pseudo-singlets”(e.g.,the main resonance of mIns).Thus,the ratios provided by LCModel refer to the concentrations rather than proton numbers.The basis set of experimental spectra comprises the prior information on neurochemistry(metabolites)as well as technique(TE,field strength,localization technique).The non-analytic line shape is constrained to unit area and capable tofit even distorted lines (due to motion or residual eddy currents).The number of knots of the baseline spline increases with the noise level.Thus,the LCModel is a mixture of experimental and phenomenological features.Although the basis spectra are provided in time domain, the evaluation is performed across a specified ppm interval.LCModel comes with a graphical user interface for routine application.Optionally the water signal may be used as quan-tification reference.Recently,lipids and macromolecular signals have been included to allow evaluation of tumour and muscle spectra.An example is shown in Fig.1.LCModel comprises basic signal normalization(see below) according to the global transmitter reference[8]to achieve a consistent scaling of the basis spectra.An in-house acquired basis set can thus be used to estimate absolute concentrations. Imported basis sets are available for a wide range of scanners and measurement protocols,but require a calibration to match the individual sensitivity(signal level)of the MR system[9]. Owing to LCModel’sflexibility,the basis set may contain also simulated spectra or an experimentally determined baseline to account for macromolecular signals.Such advanced applica-tions require good theoretical understanding and some practical experience.Care must be taken to maintain consistent scaling when adding new metabolite spectra to an existing basis.This is easiest done by cross-evaluation,that is evaluating a reference peak(e.g.,formate)in spectrum to be included by the singlet of the original basis and correcting to the known value.Caveat:The fact that LCModel converges does not ensure reliability of the estimates;least in absolute units(see Sections 3and4).Systematic difference in SNR may translate into bias via the baseline spline(see Fig.2).The same may be due an inconsistent choice of the boundaries of the ppm interval,partic-ularly next to the water resonance.In particular,with decreasing SNR(lower than4)one may observe more often systematically low or high concentrations.This is likely due to the errors in the feet of the non-analytical line shape,as narrow lines lead to underestimation and broad lines to overestimation.The metabo-lite ratios are still valid,as all model spectra are convoluted by the same lineshape.2.3.2.jMRUIThe java-based MR user interface for the processing of in vivo MR-spectra(jMRUI)is provided without charge222G.Helms /European Journal of Radiology 67(2008)218–229Fig.2.Systematic baseline differences between low and high SNR.Single spectrum from an 1.7ml VOI in white matter of the splenium (A)and the averaged spectra of seven healthy subjects (B).Note how the straight baseline leads to a severe underestimation of all metabolites except mIns.Differences were most prominent for Glu +Gln:3.6mM (43%)in a single subject vs.6.7mM (7%)in the averaged spectrum.(http://www.mrui.uab.es/mrui/mrui Overview.shtml ).It comes with a wide range of pre-processing features and interac-tive graphical software applications,including linear prediction and a powerful water removal by Hankel–Laclosz single value decomposition (HLSVD).In contrast to LCModel,it is designed to support user interaction.Several models for analy-sis/evaluation have been implemented in jMRUI,in particular AMARES [5]and QUEST [4].These focus on time-domain analysis,including line shape conversion,time-domain filter-ing and eddy-current deconvolution.Note that in the context of jMRUI ‘quantitation’refers to spectrum analysis.The pre-processing steps may exert a systematic influence on the results of model fitting.jMRUI can handle large data sets as from time-resolved MRS,two-dimensional MRS,and spatially resolved MRS,so-called MR spectroscopic imaging (MRSI)or chemical-shift imaging (CSI).G.Helms/European Journal of Radiology67(2008)218–2292233.Signal normalization3.1.PrinciplesThe signal is provided in arbitrary units of signed integer numbers,similar to MRI,and then converted tofloating complex numbers.In addition to scaling along the scanner’s receiver line, the proportionality between signal strength and number of spins per volume is strongly influenced by interaction of the RF hard-ware and its dielectric and conductive load,the human body.It is the correction of this interaction that forms the non-trivial part of signal normalization.Signal normalization is mainly applied to single-volume MRS,since spatially resolved MRSI poses addi-tional technical problems that are not part of this review.For sake of simplicity we assume homogeneous conditions across the whole volume-of-interest(VOI).Normalization consists of multiplications and divisions that render the signal,S,proportional to the concentration(of spins), C.Regardless whether in time domain(amplitude)or frequency domain(area),the signal is proportional to the size V of the VOI and the receiver gain R.S∼CVR or(1a) S/V/R∼C(1b) Logarithmic(decibel)units of the receiver gain must be con-verted to obtain a linear scaling factor,R.If R can be manually changed,it is advisable to check whether the characteristic of S(R)follows the assumed dependence.If a consistent(often the highest possible)gain used by default for single voxel MRS, one does not have to account for R.Correction of V for partial volume effects is discussed below.The proportionality constant will vary under the influence of the specific sample“loading”the RF coil.The properties of a loaded transmit–receive(T/R)coil are traditionally assessed by measuring the amplitude(or width)of a specific RF pulse,e.g., a180◦rectangular pulse.This strategy may also be pursued in vivo.The signal theory for T/R coils is given in concise form in [10]without use of complex numbers.Here,we develop it by presenting a chronology of strategies of increasing complexity that have been used for in vivo quantification.3.2.Internal referencing and metabolite ratiosBy assuming a concentration C int for the signal(S int)of ref-erence substance acquired in the same VOI,one has not to care about the influence of RF or scanner parameters:SS intC int=C(2)When using the total creatine(tCr)signal,internal referencing is equivalent to converting creatine ratios to absolute units.In early quantification work,the resonance of tCr has been assigned to 10mM determined by biochemical methods[11].However,it turned out that the MRS estimates of tCr are about25%lower and show some spatial dependence.In addition,tCr may increase in the presence of gliosis.3.3.External referencingThe most straightforward way is to acquire a reference sig-nal from an external phantom during the subject examination, with C ext being the concentration of the phantom substance [12,13].The reference signal S ext accounts for any changes in the proportionality constant.It may be normalized like the in vivo signal:S(VR)C extS ext/(V ext R ext)=C(3)If,however,the phantom is placed in the fringefield of the RF receive coil,the associated reduction in S ext will result in an overestimation of C.Care has to be taken to mount the external phantom reproducibly into the RF coil if this bias cannot be corrected otherwise.3.4.Global transmitter referenceAlready in high-field MR spectrometers it has been noticed that by coil load the sample influences both the transmit pulse and the signal:a high load requires a longer RF pulse for a 90◦excitation,which then yields reciprocally less signal from the same number of spins.This is the principle-of-reciprocity (PoR)for transmit/receive(T/R)coils in its most rudimentary form.It has been applied to account for the coil load effect, that is,large heads giving smaller signals than small heads [8].On MRI systems,RF pulses are applied with constant duration and shape.A high load thus requires a higher volt-age U tra(or transmitter gain),as determined during pre-scan calibration.S/V/R∼Ctraor(4a) S U tra/V/R∼C(4b)Of course,U tra must always refer to a pulse of specific shape, duration andflip angle,as used forflip angle calibration.On Siemens scanners,the amplitude of a non-selective rectangu-lar pulse(rect)is used.The logarithmic transmitter gain of GE scanners is independent of the RF pulse,but has to be converted from decibel to linear units[9].Normalization by the PoR requires QA at regular intervals,as the proportionality constant in Eqs.((4a)and(4b))may change in time.This may happen gradually while the performance of the RF power amplifier wears down,or suddenly after parts of the RF hardware have been replaced.For this purpose,the MRS protocol is run on a stable QA phantom of high concentration and the concentration estimate C QA(t i)obtained at time point, t i,is used to refer any concentration C back to time point zero byC→C C QA(t0)C QA(t i)(5)An example of serial QA monitoring is given in Fig.3.224G.Helms /European Journal of Radiology 67(2008)218–229Fig.3.QA measurement of temporal variation.Weekly QA performed on stable phantom of 100mM lactate and 100mM acetate from January 1996to June 1996.The standard single-volume protocol and quantification procedure (LCModel and global reference)were applied.(A)The mean estimated concentration is shown without additional calibration.The A indicates the state after installation,B a gradual breakdown of the system;the sudden jumps were due to replacement of the pre-amplifier (C and D)or head-coil (E),and retuning of the system (F).Results were used to correct proportionality to obtain longitudinally consistency.(B)The percentage deviation from the preceding measurement in Shewhart’s R-diagram indicates the weeks when quantification may not be reliable (data courtesy of Dr.M.Dezortov´a ,IKEM,Prague,Czech Republic).3.5.Local flip angleDanielsen and Hendriksen [10]noted that the PoR is a local relationship,so they used the amplitude of the water suppression pulse,U tra (x ),that had been locally adjusted on the VOI signal.S (x )U tra (x )/V/R ∼C(6)The local transmitter amplitude may also be found be fitting the flip angle dependence of the local signal [14].The example in Fig.4illustrates the consistency of Eq.(6)at the centre (high signal,low voltage)and outside (low signal,high voltage)the volume headcoil.Fig.4.Local verification of the principle of reciprocity.Flip angle dependence of the STEAM signal measured at two positions along the axis of a GE birdcage head-coil by varying the transmitter gain (TG).TG was converted from logarith-mic decibel to linear units (linearized TG,corresponding to U tra ).At coil centre (×)and 5cm outside the coil (+)the received signal,S (x ),was proportional to the transmitted RF,here given by 1/lin TG(x )at the signal maximum or 90◦flip angle.Like in large phantoms,there are considerable flip angle devi-ations across the human head as demonstrated at 3T in Fig.5a [15].The local flip angle,α(x ),may be related to the nominal value,αnom ,by α(x )=f (x )αnom(7)The spatially dependent factor is reciprocal to U tra (x ):f (x )∼1/U tra (x ).The flip angle will also alter the local signal.If a local transmitter reference is used,S (x )needs to be corrected for excitation effects.For the ideal 90◦–90◦–90◦STEAM local-ization and 90◦–180◦–180◦PRESS localization in a T/R coil,the signals areS (x )STEAM ∼M tr (x )∼C2f (x )sin 3(f (x )90◦)(8a)S (x )PRESS ∼M tr (x )∼C f (x )sin 5(f (x )90◦)(8b)The dependence of S (x )was simulated for a parabolic RF profile.A constant plateau is observed as the effects of transmission and reception cancel out for higher flip angles in the centre of the head where the VOI is placed.This is the reason why the global flip angle method works even in the presence of flip angle inhomogeneities.Note that the signal drops rapidly for smaller flip angles,i.e.close to the skull.3.6.Coil impedance effectsOlder quantification studies were performed on MR systems where the coil impedance Z was matched to 50 [8,10].Since the early 1990s,most volume head coils are of the high Q design and approximately tuned and matched by the RF load of the head and the stray capacitance of the shoulders.The residual variation of the impedance Z will affect the signal by S (x )U tra (x )/V/R ∼CZ(9)G.Helms/European Journal of Radiology67(2008)218–229225Fig.5.Flip angle inhomogeneities across the human brain.(Panel A)T1-w sagittal view showing variation in the RFfield.Flip angles are higher in the centre of the brain.The contours correspond to80–120◦localflip angle for a nominal value of90◦.(Panel B)The spatial signal dependence of STEAM and PRESS was simulated for a parabolicflip angle distribution with a maximum of115%relative to the global transmitter reference.This resulted in a constant signal obtained from the central regions of the brain,and a rapid decline at the edges.Reflection losses due to coil mismatch are symmetric in trans-mission and reception and are thus accounted for by U tra.These are likely to occur with exceptionally large or small persons (infants)or with phantoms of insufficient load.3.7.External phantom and local referenceWhen the impedance is not individually matched to50 , the associated change in proportionality must be monitored by a reference signal.In aqueous phantoms,the water signal can be used as internal reference.For in vivo applications,one may resort to an extra measurement in an external phantom[14].An additionalflip angle calibration in the phantom will account for local differences in the RFfield,especially if the phantom is placed in the fringe RFfield:SU tra(x)/(VR)S ext U tra(x ext)/(V ext R ext)C ext=C(10)This is the most comprehensive signal normalization.The com-bination of external reference and localflip angle method corrects for all effects in T/R coils.The reference signal accounts for changes in the proportionality,while the localflip angle cor-rects for RF inhomogeneity.Note also that systematic errors in S,U tra and V cancel out by division.Calibration of each individual VOI may be sped up by rapid RF mapping in three dimensions.3.8.Receive only-coilsThe SNR of the MRS signal can be increased by using sur-face coils or phased arrays of surface coils.The inhomogeneous receive characteristic cannot be mapped directly.The normaliza-tions discussed above(except Section3.2)cannot be performed directly on the received signal,as the coils are not used for trans-mission.Instead,the localized water signal may be acquired with both the receive coil and the body coil to scale the low SNR metabolite signal to obey the receive characteristics of the T/R body coil[16,17]:S rec met S bodywaterS rec water=S bodymet(11)For use with phased array coils it is essential that the metabolite and water signals are combined using consistent weights,since the low SNR of the water suppressed acquisition is most likely influenced by noise.3.9.Internal water referenceThe tissue water appears to be the internal reference of choice, due to its high concentration and well established values for water content of tissues(βper volume[18]):SS waterβ55mol/litre=C(12)It should be kept in mind that in vivo water exhibits a wide range of relaxation times,with the main component relaxing consider-able faster than the main metabolites.T2-times range from much shorter(myelin-associated water in white mater T2of15ms)to much longer(CSF,2400ms in bulk down to700ms in sulci with large surface-to-volume ratio).This implies an influence of TE on the concentration estimates.In addition,relaxation time and water content are subject to change in pathologies.Since the water signal is increasing in most pathologies(by content and relaxation),water referencing tends to give lower concentration estimates in pathologies.Ideally,the water signal should be determined by a multi-componentfit of the T2-decay curve[12].An easy but time-consuming way is to increase TE in consecutive fully relaxed single scans.A reliable way to determine the water sig-nal is tofit a2nd order polynomial through thefirst50ms of the magnitude signal(Fig.6).Thus,determining the amplitude cancels out initial receiver instabilities and avoids linefitting at an ill defined phase.If care is taken to avoid partial saturation by RF leakage from the water suppression pulses,this is consistent with multi-echo measurements using a CPMG MRI sequence [18](Fig.7).。
AMCA-210-2007

Authority
ANSI/AMCA 210 - ANSI/ASHRAE 51 was approved by the membership of the Air Movement and Control Association on July 28, 2006 and by ASHRAE on March 17, 2008. It was approved by ANSI and became an American National Standard on August 17, 2007.
Air Movement and Control Association International 30 West University Drive Arlington Heights, IL 60004-1893 U.S.A.
or
AMCA International, Incorporated c/o Federation of Environmental Trade Associations 2 Waltham Court, Milley Lane, Hare Hatch Reading, Berkshire RG10 9TH United Kingdom
© 2008 by the Air Movement and Control Association International, Inc. and the American Society of Heating, Refrigerating, and Air Conditioning Engineers
Guidance document on pesticide residue

EUROPEAN COMMISSION12Directorate General Health and Consumer Protection345SANCO/825/00 rev. 8.1 616/11/2010 78Guidance document on pesticide residue 9analytical methods10111213141516[Revision 8 is the version of this guidance document that is currently valid. It is, however, under1718continuous review and will be updated when necessary. The document is aimed at 19manufacturers seeking pesticides authorisations and parties applying for setting or modification 20of an MRL. It gives requirements for methods that would be used in post-registration 21monitoring and control by the competent authorities in Member States in the event that 22authorisations are granted. For authorities involved in post-registration control and monitoring, the document may be considered as being complementary to the documents: Method Validation2324and Quality Control Procedures for Pesticide Residues Analysis in Food and Feed (for the valid revision visit http://ec.europa.eu/food/plant/protection/resources/publications_en.htm) and the2526OECD document “Guidance Document on pesticide residue analytical methods”, 2007.27(ENV/JM/ ENV/JM/MONO(2007)17).1Preamble (4)28292General (5)302.1Good Laboratory Practice (5)312.2Selection of analytes for which methods are required (5)322.3Description of an analytical method and its validation results (5)332.4Hazardous reagents (6)342.5Acceptable analytical techniques considered commonly available (6)352.6Multi-residue methods (7)362.7Single methods and common moiety methods (7)372.8Single methods using derivatisation (7)382.9Method validation (8)392.9.1Calibration (8)2.9.2Recovery and Repeatability (9)40412.9.3Selectivity (11)422.10Confirmation (11)432.10.1Confirmation simultaneous to primary detection (11)442.10.2Confirmation by an independent analytical technique (12)452.11Independent laboratory validation (ILV) (12)2.12Availability of standards (13)46472.13Extraction Efficiency (13)483Analytical methods for residues in plants, plant products, foodstuff (of plant origin),feedingstuff (of plant origin) (Annex IIA Point 4.2.1 of Directive 91/414/EEC; Annex Point IIA,4950Point 4.3 of OECD) (14)513.1Purpose (14)523.2Selection of analytes (14)533.3Commodities and Matrix Groups (14)543.4Limit of quantification (15)553.5Independent laboratory validation (ILV) (15)564Analytical methods for residues in foodstuff (of animal origin) (Annex IIA Point 4.2.1 of 57Directive 91/414/EEC; Annex Point IIA, Point 4.3 of OECD) (16)584.1Purpose (16)594.2Selection of analytes (16)604.3Commodities (16)614.4Limit of quantification (16)4.5Independent laboratory validation (ILV) (16)62635Analytical methods for residues in soil (Annex IIA, Point 4.2.2 of Directive 91/414/EEC;64Annex Point IIA, Point 4.4 of OECD) (17)655.1Purpose (17)665.2Selection of analytes (17)675.3Samples (17)685.4Limit of quantification (17)696Analytical methods for residues in water (Annex IIA, Point 4.2.3 of Directive 91/414/EEC;70Annex Point IIA; Point 4.5 of OECD) (19)716.1Purpose (19)726.2Selection of analytes (19)736.3Samples (19)746.4Limit of quantification (19)756.5Direct injection (20)767Analytical methods for residues in air (Annex IIA, Point 4.2.4 of Directive 91/414/EEC; 77Annex Point IIA; Point 4.7 of OECD) (21)7.1Purpose (21)78797.2Selection of analytes (21)807.3Samples (21)7.4Limit of quantification (21)81827.5Sorbent characteristics (22)837.6Further validation data (22)7.7Confirmatory methods (22)84858Analytical methods for residues in body fluids and tissues (Annex IIA, Point 4.2.5 of86Directive 91/414/EEC; Annex Point IIA Point 4.8 of OECD) (23)8.1Purpose (23)87888.2Selection of analytes (23)898.3Samples (23)908.4Sample set (23)918.5Limit of quantification (23)929Summary - List of methods required (24)10Abbreviations (25)939411References (27)951Preamble96This document provides guidance to applicants, Member States and EFSA on the data 97requirements and assessment for residue analytical methods for post-registration control and 98monitoring purposes. It is not intended for biological agents such as bacteria or viruses. It 99recommends possible interpretations of the provisions of section 3.5.2 of Annex II of 100Regulation (EC) No 1107/2009 [1] and of the provisions of section 4, part A of Annex II and 101section 5, part A of Annex III of Council Directive 91/414/EEC [2]. It also applies to 102applications for setting or modification of an MRL within the scope of Regulation (EC) No 103396/2005 [3]. It has been elaborated in consideration of the ‘Guidance Document on pesticide 104residue analytical methods’ of the OECD [4] and SANCO/10684/2009 “Method validation 105and quality control procedures for pesticide residue analysis in food and feed” [5].106This document has been conceived as an opinion of the Commission Services and elaborated 107in co-operation with the Member States. It does not, however, intend to produce legally 108binding effects and by its nature does not prejudice any measure taken by a Member State nor 109any case law developed with regard to this provision. This document also does not preclude 110the possibility that the European Court of Justice may give one or another provision direct 111effect in Member States.112This guidance document must be amended at the latest if new data requirements as referred to 113in Article 8 (1)(b) and 8 (1)(c) of Regulation (EC) No 1107/2009 will have been established 114in accordance with the regulatory procedure with scrutiny referred to in Article 79 (4).1152General1162.1Good Laboratory Practice117According to Guidance Document 7109/VI/94-Rev. 6.c1 (Applicability of Good Laboratory 118Practice to Data Requirements according to Annexes II, Part A, and III, Part A, of Council 119Directive 91/414/EEC) [6] the development and validation of an analytical method for 120monitoring purposes and post-registration control is not subject to GLP. However, where the 121method is used to generate data for registration purposes, for example residue data, these 122studies must be conducted to GLP.1232.2Selection of analytes for which methods are required124The definition of the residues relevant for monitoring in feed and food as well as in 125environmental matrices and air is not the subject matter of this document. Criteria for the 126selection of analytes in case that no legally binding definition is available are given in the 127respective sections 3 - 8. In addition, sections 5.2, 6.2, 7.2 and 8.2 clarify under which 128circumstances analytical methods for residues may not be necessary.1292.3Description of an analytical method and its validation results130Full descriptions of validated methods shall be provided. The submitted studies must include 131the following points:132•Itemisation of the fortified compounds and the analytes, which are quantified133•Description of the analytical method134•Validation data as described in more detail below135•Description of calibration including calibration data136•Recovery and Repeatability137•Data proving the selectivity of the method138•Confirmatory data, if not presented in a separate study139•References (if needed)140141The following information should be offered in the description of the analytical method:142•An introduction, including the scope of the method143•Outline/summary of method, including validated matrices, limit of quantification (LOQ), 144range of recoveries, fortification levels and number of fortifications per level145•Apparatus and reagents146•instrument parameters used as example if appropriate147•Description of the analytical method, including extraction, clean-up, derivatisation (if148appropriate), chromatographic conditions (if appropriate) and quantification technique149•Hazards or precautions required150•Time required for one sample set151•Schematic diagram of the analytical method152•Stages where an interruption of the method is possible153•Result tables (if results are not presented in separate studies)154•Procedure for the calculation of results from raw data155•Extraction efficiency of solvents used156•Important points and special remarks (e.g. volatility of analyte or its stability with regard 157to pH)158•Information on stability of fortified/incurred samples, extracts and standard solutions (If 159the recoveries in the fortified samples are within the acceptable range of 70-120 %,160stability is sufficiently proven.)161Sometimes it may be necessary for other information to be presented, particularly where 162special methods are considered.1632.4Hazardous reagents164Hazardous reagents (carcinogens category I and II [7]) shall not be used. Among these 165compounds are diazomethane, chromium (VI) salts, chloroform and benzene.1662.5Acceptable analytical techniques considered commonly available167Analytical methods shall use instrumentation regarded as "commonly available":168•GC detectors: FPD, NPD, ECD, FID, MS, MS n (incl. Ion Traps and MS/MS), HRMS169•GC columns: capillary columns170•HPLC detectors: MS, MS/MS, HRMS, FLD, UV, DAD171•HPLC columns: reversed phase, ion-exchange, normal phase172•AAS, ICP-MS, ICP-OES173Other techniques can be powerful tools in residue analysis, therefore the acceptance of 174additional techniques as part of enforcement methods should be discussed at appropriate 175intervals. Whilst it is recognised that analytical methodology is constantly developing, some 176time elapses before new techniques become generally accepted and available.1772.6Multi-residue methods178Multi-residue methods that cover a large number of analytes and that are based on GC-MS 179and/or HPLC-MS/MS are routinely used in enforcement laboratories for the analysis of plant 180matrices. Therefore, validated residue methods submitted for food of plants, plant products 181and foodstuff of plant origin (Section 3) should be multi-residue methods published by an 182international official standardisation body such as the European Committee for 183Standardisation (CEN) (e.g. [8 - 12]) or the AOAC International (e.g. [13]). Single residue 184methods should only be provided if data show and are reported that multi-residue methods 185involving GC as well as HPLC techniques cannot be used.186If validation data for the residue analytical method of an analyte in at least one of the 187commodities of the respective matrix group have been provided by an international official 188standardisation body and if these data have been generated in more than one laboratory with 189the required LOQ and acceptable recovery and RSD data (see Section 2.9.2), no additional 190validation by an independent laboratory is required.1912.7Single methods and common moiety methods192Where a pesticide residue cannot be determined using a multi-residue method, one or where 193appropriate more alternative method(s) must be proposed. The method(s) should be suitable 194for the determination of all compounds included in the residue definition. If this is not 195possible and an excessive number of methods for individual compounds would be needed, a 196common moiety method may be acceptable, provided that it is in compliance with the residue 197definition. However, common moiety methods shall be avoided whenever possible.1982.8Single methods using derivatisation199For the analysis of some compounds by GC, such as those of high polarity or with poor 200chromatographic properties, or for the detection of some compounds in HPLC, derivatisation 201may be required. These derivatives may be prepared prior to chromatographic analysis or as 202part of the chromatographic procedure, either pre- or post-column. Where a derivatisation 203method is used, this must be justified.204If the derivatisation is not part of the chromatographic procedure, the derivative must be 205sufficiently stable and should be formed with high reproducibility and without influence of 206matrix components on yield. The efficiency and precision of the derivatisation step should be 207demonstrated with analyte in sample matrix against pure derivative. The storage stability of 208the derivative should be checked and reported. For details concerning calibration refer to 209Section 2.9.1.210The analytical method is considered to remain specific to the analyte of interest if the 211derivatised species is specific to that analyte. However, where – in case of pre-column 212derivatisation – the derivative formed is a common derivative of two or more active 213substances or their metabolites or is classed as another active substance, the method should be 214considered non-specific and may be deemed unacceptable.2152.9Method validation216Validation data must be submitted for all analytes included in the residue definition for all 217representative sample matrices to be analysed at adequate concentration levels.218Basic validation data are:219•Calibration data220•Concentration of analyte(s) found in blank samples221•Concentration level(s) of fortification experiments222•Concentration and recovery of analyte(s) found in fortified samples223•Number of fortification experiments for each matrix/level combination224•Mean recovery for each matrix/level combination225•Relative standard deviation (RSD) of recovery, separate for each matrix/level combination 226•Limit of quantification (LOQ), corresponding to the lowest validated level227•Representative clearly labelled chromatograms228•Data on matrix effects, e.g. on the response of the analyte in matrix as compared to pure 229standards230.Further data may be required in certain cases, depending on the analytical method used, and 231the residue definition to be covered.2322.9.1Calibration233The calibration of the detection system shall be adequately demonstrated at a minimum of 3 234concentration levels in duplicate or (preferably) 5 concentration levels with single 235determination. Calibration should be generated using standards prepared in blank matrix 236extracts (matrix matched standards) for all sample materials included in the corresponding 237validation study (Sections 3 - 8). Only, if experiments clearly demonstrate that matrix effects 238are not significant (i.e. < 20 %), calibration with standards in solvent may be used. Calibration 239with standards in solvent is also acceptable for methods to detect residues in air (Section 7). 240In case that aqueous samples are analysed by direct injection HPLC-MS/MS calibration shall 241be performed with standards in aqueous solution.242The analytical calibration must extend to at least the range which is suitable for the 243determination of recoveries and for assessment of the level of interferences in control 244samples. For that purpose a concentration range shall be covered from 30 % of the LOQ to 24520 % above the highest level (Section 2.9.2).246All individual calibration data shall be presented together with the equation of the calibration. 247Concentration data should refer to both, the mass fraction in the original sample (e.g. mg/kg) 248and to the concentration in the extract (e.g. µg/L). A calibration plot should be submitted, in 249which the calibration points are clearly visible. A plot showing the response factor1 versus the 250concentration for all calibration points is preferred over a plot of the signal versus the 251concentration.252Linear calibrations are preferred if shown to be acceptable over an appropriate concentration 253range. Other continuous, monotonically increasing functions (e.g. exponential/power, 254logarithmic) may be applied where this can be fully justified based on the detection system 255used.256When quantification is based on the determination of a derivative, the calibration shall be 257conducted using standard solutions of the pure derivative generated by weighing, unless the 258derivatisation step is an integral part of the detection system. If the derivative is not available 259as a reference standard, it should be generated within the analytical set by using the same 260derivatisation procedure as that applied for the samples. Under these circumstances, a full 261justification should be given.2622.9.2Recovery and Repeatability263Recovery and precision data must be reported for the following fortification levels, except for 264body fluids and body tissues (Section 8):265•LOQ 5 samples266•10 times LOQ, or MRL (set or proposed) or other relevant level (≥ 5 x LOQ)2675 samples268Additionally, for unfortified samples residue levels must be reported:269samples•blankmatrix 2270According to the residue definition the LOQ of chiral analytes usually applies to the sum of 271the two enantiomers. In this case it is not necessary to determine the enantiomers separately. 2721 The response factor is calculated by dividing the signal area by the respective analyte concentration.Enantioselective methods would only be required if a single enantiomer is included in the 273residue definition.274In cases of complex residue definitions (e.g. a residue definition which contains more than 275one compound) the validation results shall be reported for the single parts of the full residue 276definition, unless the single elements cannot be analysed separately.277The mean recovery at each fortification level and for each sample matrix should be in the 278range of 70 % - 120 %. In certain justified cases mean recoveries outside of this range will be 279accepted.280For plants, plant products, foodstuff (of plant and animal origin) and in feeding stuff recovery 281may deviate from this rule as specified in Table 1.2282Table 1: Mean recovery and precision criteria for plant matrices and animal matrices [4]283Concentration level Range of mean recovery(%)Precision, RSD(%)> 1 µg/kg ≤ 0.01 mg/kg 60 - 120 30> 0.01 mg/kg ≤ 0.1 mg/kg 70 - 120 20> 0.1 mg/kg ≤ 1.0 mg/kg 70 - 110 15> 1 mg/kg 70 - 110 10284If blank values are unavoidable, recoveries shall be corrected and reported together with the 285uncorrected recoveries.286The precision of a method shall be reported as the relative standard deviation (RSD) of 287recovery at each fortification level. For plants, plant products, foodstuff (of plant and animal 288origin) and feeding stuff the RSD should comply with the values specified in Table 1. In other 289cases the RSD should be ≤ 20 % per level. In certain justified cases, e.g. determination of 290residues in soil lower than 0.01 mg/kg, higher variability may be accepted.291When outliers have been identified using appropriate statistical methods (e.g. Grubbs or 292Dixons test), they may be excluded. Their number must not exceed 1/5 of the results at each 293fortification level. The exclusion should be justified and the statistical significance must be 2942 According to Annex IIA 4.2 of Directive 91/414/EEC the mean recovery should normally be 70 % - 110 % andthe RSD should preferably be ≤ 20 %.clearly indicated. In that case all individual recovery data (including those excluded) shall be 295reported.2962.9.3Selectivity297Representative clearly labelled chromatograms of standard(s) at the lowest calibrated level, 298matrix blanks and samples fortified at the lowest fortification level for each analyte/matrix 299combination must be provided to prove selectivity of the method. Labelling should include 300sample description, chromatographic scale and identification of all relevant components in the 301chromatogram.302When mass spectrometry is used for detection, a mass spectrum (in case of MS/MS: product 303ion spectrum) should be provided to justify the selection of ions used for determination.304Blank values (non-fortified samples) must be determined from the matrices used in 305fortification experiments and should not be higher than 30 % of the LOQ. If this is exceeded, 306detailed justification should be provided.3072.10Confirmation308Confirmatory methods are required to demonstrate the selectivity of the primary method for 309all representative sample matrices (Sections 3 – 8). It has to be confirmed that the primary 310method detects the right analyte (analyte identity) and that the analyte signal of the primary 311method is quantitatively correct and not affected by any other compound.3122.10.1Confirmation simultaneous to primary detection313A confirmation simultaneous to the primary detection using one fragment ion in GC-MS and 314HPLC-MS or one transition in HPLC-MS/MS may be accomplished by one of the following 315approaches:316•In GC-MS, HPLC-MS, by monitoring at least 2 additional fragment ions (preferably317m/z > 100)for low resolution system and at least 1 additional fragment ion for high318resolution/accurate mass system319•In GC-MS n (incl. Ion Traps and MS/MS), HPLC-MS/MS, by monitoring at least 1320additional SRM transition321The following validation data are required for the additional fragment ions (MS and HRMS) 322or the additional SRM transition (MS n and MS/MS): calibration data (Section 2.9.1), recovery 323and precision data according to Section 2.9.2 for samples fortified at the respective LOQ (n = 3245) and for 2 blank samples.325For all mass spectrometric techniques a mass spectrum (in case of single MS) or a product ion 326spectrum (in case of MS n) should be provided to justify the selection of the additional ions. 3272.10.2Confirmation by an independent analytical technique328Confirmation can also be achieved by an independent analytical method. The following are 329considered sufficiently independent confirmatory techniques:330•chromatographic principle different from the original method331• e.g. HPLC instead of GC332•different stationary phase and/or mobile phase with significantly different selectivity333•the following are not considered significantly different:334•in GC: stationary phases of 100 % dimethylsiloxane and of 95 % dimethylsiloxane 335+ 5 % phenylpolysiloxane336•in HPLC: C18- and C8-phases337•alternative detector338• e.g. GC-MS vs. GC-ECD, HPLC-MS vs. HPLC-UV/DAD339•derivatisation, if it was not the first choice method340•high resolution/accurate mass MS341•in mass spectrometry an ionisation technique that leads to primary ions with different m/z 342ratio than the primary method (e.g. ESI negative ions vs. positive ions)343It is preferred that confirmation data are generated with the same samples and extracts used 344for validation of the primary method.345The following validation data are required: calibration data (Section 2.9.1), recovery and 346precision data (Section 2.9.2) for samples fortified at the respective LOQ (n ≥ 3) and of a 347blank sample and proof of selectivity (Section 2.9.3).3482.11Independent laboratory validation (ILV)349A validation of the primary method in an independent laboratory (ILV) must be submitted for 350methods used for the determination of residues in plants, plant products, foodstuff (of plant 351and animal origin) and in feeding stuff. The ILV shall confirm the LOQ of the primary 352method, but at least the lowest action level (MRL).353The extent of independent validation required is given in detail in sections 3 and 4.354In order to ensure independence, the laboratory chosen to conduct the ILV trials must not 355have been involved in the method development and in its subsequent use. In case of multi-356residue methods it would be accepted if the ILV is performed in a laboratory that has already 357experience with the respective method.358The laboratory may be in the applicant’s organisation, but should not be in the same location. 359In the exceptional case that the lab chosen to conduct the ILV is in the same location, 360evidence must be provided that different personnel, as well as different instrumentation and 361stocks of chemicals etc have been used.362Any additions or modifications to the original method must be reported and justified. If the 363chosen laboratory requires communication with the developers of the method to carry out the 364analysis, this should be reported.3652.12Availability of standards366All analytical standard materials used in an analytical method must be commonly available. 367This applies to metabolites, derivatives (if preparation of derivatives is not a part of the 368method description), stable isotope labelled compounds or other internal standards.369If a standard is not commercially available the standard should be made generally available by 370the applicant and contact details be provided.3712.13Extraction Efficiency372The extraction procedures used in residue analytical methods for the determination of residues 373in plants, plant products, foodstuff (of plant and animal origin) and in feeding stuff should be 374verified for all matrix groups for which residues ≥ LOQ are expected, using samples with 375incurred residues from radio-labelled analytes.376Data or suitable samples may be available from pre-registration metabolism studies or 377rotational crop studies or from feeding studies. In cases where such samples are no longer 378available to validate an extraction procedure, it is possible to "bridge" between two solvent 379systems (details in [4]). The same applies if new matrices are to be included.3803Analytical methods for residues in plants, plant products, foodstuff (of 381plant origin), feedingstuff (of plant origin)382(Annex IIA Point 4.2.1 of Directive 91/414/EEC; Annex Point IIA, Point 3834.3 of OECD)3843.1Purpose385•Analysis of plants and plant products, and of foodstuff and feeding stuff of plant origin for 386compliance with MRL [3].3873.2Selection of analytes388The selection of analytes for which methods for food and feed are required depends upon the 389definition of the residue for which a maximum residue level (MRL) is set or is applied for 390according to Regulation (EC) No 396/2005.3913.3Commodities and Matrix Groups392Methods validated according to Section 2.9 and 2.10 must be submitted for representative 393commodities (also called “matrices” by analytical chemists) of all four matrix groups in 394Table 2.395396Table 2: Matrix groups and typical commoditiesMatrix group Examples for commoditiesbarley, rice, rye, wheat, dry legume vegetables dry commodities (high protein/highstarch content)commodities with high water content apples, bananas, cabbage, cherries, lettuce, peaches,peppers, tomatoescommodities with high oil content avocados, linseed, nuts, olives, rape seedcommodities with high acid content grapefruits, grapes, lemons, oranges397Important Note: This list of commodities is not a comprehensive list of commodities/matrices.398Applicants may consult regulatory authorities for advice on the use of other commodities.If samples with high water content are extracted at a controlled pH a particular method or 399validation for commodities with high acid content is not required.400Where a previously validated method has been adopted to a new matrix group, validation data 401must be submitted for representative matrices of this group.402。
锟糂USINESS CONDITIONS AND EXPECTED RETURNS ON STOCKS AND BONDS
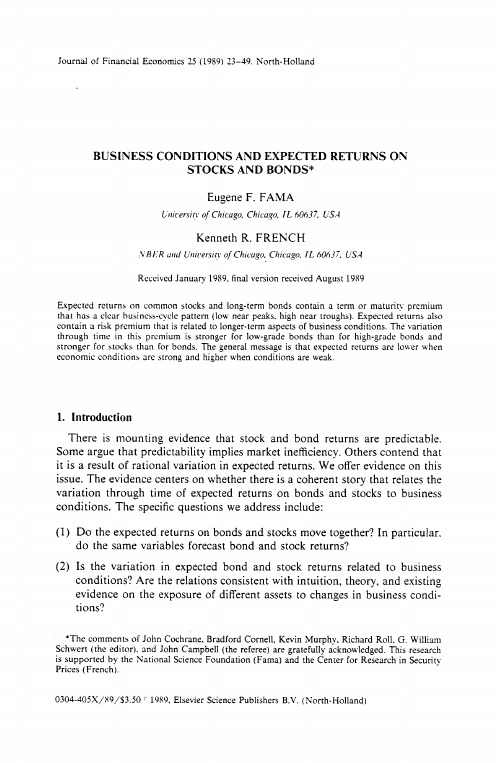
*The comments of John Cochrane. Bradford Cornell, Kevin Murphy. Richard Roll. G. William Schwert (the editor). and John Campbell (the referee) are gratefully acknowledged. This research is supported by the National Science Foundation (Fama) and the Center for Research in Security Prices (French).
(1)
Do the expected returns on bonds and stocks move together? do the same variables forecast bond and stock returns?
In particular.
(2) Is the variation
in expected bond and stock returns related to business conditions? Are the relations consistent with intuition, theory, and existing evidence on the exposure of different assets to changes in business conditions?
1989. final version received August
1989
returns on common stocks and long-term bonds contain a term or maturity premium a clear business-cycle pattern (low near peaks. high near troughs). Expected returns also a risk premium that is related to longer-term aspects of business conditions. The variation time in this premium is stronger for low-grade bonds than for high-grade bonds and for stocks than for bonds. The general message is that expected returns are lower when conditions are strong and higher when conditions are weak.
最新细胞生物学考研历年真题

细胞生物学考研历年真题中科院1993-2006年细胞生物学考研历年真题中国科学院1993年细胞生物学硕士生入学试题(A卷)是非题。
(每题1分,共20分。
答是写“+”,答非写“—”。
)题目:1、溶酶体在动物、植物、原生动物和细菌中均有存在。
()考查点:溶酶体的分布课本内容:溶酶体几乎存在于所有的动物细胞中,植物细胞内也有与溶酶体功能相似的细胞器——圆球体及植物中央液泡。
原生动物细胞也有类似溶酶体的结构。
答案:非,-相关内容:溶酶体的形成,溶酶体酶及其功能。
题目:2、干细胞是一类未分化的,能够无限分裂增殖的细胞。
()考查点:细胞全能性与细胞分化课本内容:具有多潜能性的细胞称干细胞。
在整个发育过程中,细胞分化潜能逐渐受到限制二变窄,既由全能性细胞转化为多能干细胞和单能干细胞。
答案:非,-。
多能干细胞和单能干细胞均已经分化。
相关内容:脱分化、再生、胚胎发育。
题目:3、在核质相互关系中,细胞质决定着细胞的类型,细胞核中的基因组决定着细胞的基因型。
()考查点:核质互作、基因组、基因型的概念。
答案:是,+相关内容:基因表达调控。
题目:4、细胞对外界环境因子反应敏感性与细胞的增殖能力成正比,而与分化程度成反比。
()考查点:细胞敏感性。
答案:非,-。
细胞对外界因子的敏感性岁受分化程度的影响,但无比例关系。
相关内容:细胞增殖、细胞分化。
题目:5、细胞分化过程一般是不稳定的,可逆的。
()考查点:细胞分化的概念。
课本内容:在个体发育中,由一种相同的细胞类型经过细胞分裂后逐渐在形态、结构、功能上形成稳定性差异,产生不同的细胞类群的过程称细胞分化(cell differentiation )。
答案:非,-题目:6、同一有机体的不同组织细胞核仁的大小和数目都有很大的变化,这种变化和细胞中蛋白质合成的旺盛程度有关。
()考查点:核仁的概念、功能。
课本内容:核仁通常表现为单一或多个均匀的球形小体。
核仁的大小、形状和数目随生物种类、细胞类型和细胞的代谢状态而变化。
自动化专业英语IntroductionstoOptimalControlSystems课件

专业英语
12
2. 译成汉语的联合复句
He made notes as he was listening to the lecture. (时间状语从句译成汉语的 并列关系)
他边听课,边做记录。
2024年8月5日2时7分
专业英语
13
3. 译成汉语的简单句 When I make a speech, I get nervous. 我演讲时总是有些紧张。
7. compromise n.妥协,和解
8. tractable adj.易驾驭的
9. payload n.有效载荷[负载]
10. vehicle n.车辆,运载工具
11. residual
adj. 剩余的,残留的
12. propellant adj.推进的
n. 推进物,火药
13. velocity n.速度,速率,迅速
optimal control system within limits imposed by physical constraints是并 列宾语。连词that引导的宾语从句作介 词in的宾语。
2024年8月5日2时7分
专业英语
5
(2) In solving problems of optimal control systems, we may have the goal of finding a rule for determining the present control decision, subject to certain constraints which will minimize some measure of a deviation from ideal behavior.
小学上册B卷英语第二单元暑期作业

小学上册英语第二单元暑期作业英语试题一、综合题(本题有100小题,每小题1分,共100分.每小题不选、错误,均不给分)1.My friend is very ________.2.The __________ can provide critical insights into environmental sustainability.3.The ______ (气候) affects what plants can grow in an area.4.My dad is my strong _______ who teaches me important lessons in life.5.The process of ______ can create sedimentary layers in rocks.6.The invention of the bicycle changed personal _____.7.My ________ (玩具名称) is an astronaut toy.8.Which holiday is celebrated on October 31st?A. ChristmasB. ThanksgivingC. HalloweenD. New YearC9.The stars are _______ (fading) at dawn.10.She has a nice ________.11.What is the opposite of "big"?A. LargeB. SmallC. HugeD. TinyB12.I like to ______ (参与) in cultural celebrations.13.The capital of Georgia is _______.14.What is the name of the famous palace in Versailles, France?A. Buckingham PalaceB. Palace of VersaillesC. Neuschwanstein CastleD. Palace of WestminsterB Palace of Versailles15.My cousin is a professional ____ ( dancer).16.The __________ is the layer of skin that helps to protect against injury.17.The capital of Cambodia is _______.18.My friend, ______ (我的朋友), is a great storyteller.19.The starfish has five _______ (手臂).20.The dog is ___ (barking/howling).21.What is the opposite of 'hot'?A. WarmB. CoolC. ColdD. FreezingC22.The element with the symbol Co is __________.23.How many zeros are in one thousand?A. OneB. TwoC. ThreeD. FourC24.My friends are very . (我的朋友们很。
均数、标准差、标准误(Mean,standarddeviation,standarderror)
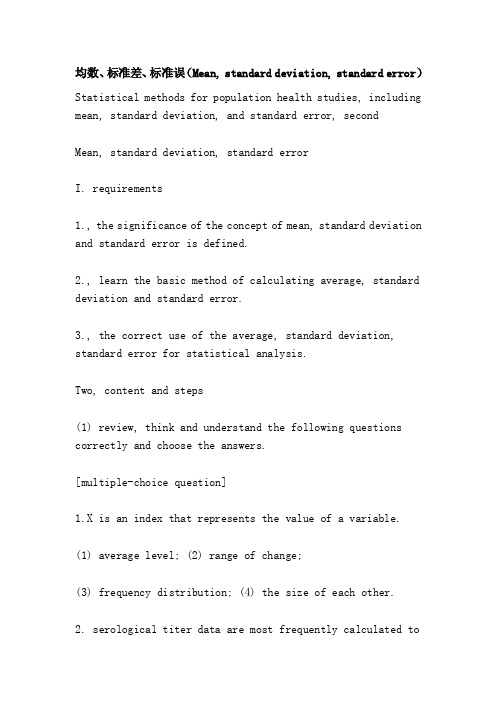
均数、标准差、标准误(Mean, standard deviation, standard error)Statistical methods for population health studies, including mean, standard deviation, and standard error, secondMean, standard deviation, standard errorI. requirements1., the significance of the concept of mean, standard deviation and standard error is defined.2., learn the basic method of calculating average, standard deviation and standard error.3., the correct use of the average, standard deviation, standard error for statistical analysis.Two, content and steps(1) review, think and understand the following questions correctly and choose the answers.[multiple-choice question]1.X is an index that represents the value of a variable. (1) average level; (2) range of change;(3) frequency distribution; (4) the size of each other.2. serological titer data are most frequently calculated toindicate their average level.(1) arithmetic mean; (2) median;(3) geometric mean; (4) total distance.3. simple method to calculate the mean.(1) equal distance between groups; (2) no group spacing is required;(3) the group spacing is equal and unequal; (4) the values of variables are relatively close.4. use frequency distribution performance and formula M=L+i/fx (n/2- Sigma fL) to calculate median.(1) the group distance is equal; (2) the distance between groups is equal;(3) the data distribution is symmetrical; (4) the data is required to be lognormal distribution.5. the original data is divided by a constant that is neither equal to 0 nor equal to 1.(1) X invariant and M variable; (M is median); (2) X change and M invariant;(3) both X and M remain unchanged; (4) X and m are both changed.6. the raw data is subtracted from the same constant that is not equal to 0.(1) X invariant, s change; (2) X change, s invariant;(3) X and s remain unchanged; (4) X and s are both changed.7. according to the standard deviation of the normal distribution, the 95% constant value range can be estimated.(1) X + 1.96s (2) X + 2.58s;(3) X + t0.05 (n′) (4) X + t0.05 (n′) s;In 8., X and s.(1) X will be negative, s will not; (2) s will be negative; X will not;(3) neither will; (4) both will.9. coefficient of variation CV.(1) must be greater than 1; (2) must be less than 1;(3) may be greater than 1; also may be less than 1; (4) must be smaller than S.10. the 95% confidence limit of the population mean can be expressed.(1) + 1.96 sigma; (2) + + 1.96 Sigma X;(3) X + t0.05 (n′) sX (4) X + 1.96s.11. of the two samples from the same population, the smaller sample estimate is more reliable.(1) sX; (2) CV;(3) s; (4) t0.05 (n′) sX.12. in the same normal population, the sample with N content is randomly extracted. In theory, 99% of the samples are in the range.(1) X + 2.58sX; (2) X + 1.96sX;(3) + 1.96 Sigma X; (4) + + 2.58 Sigma X;13. sigma x indicates.(1) the standard error of population mean; (2) the degree of dispersion of population mean:(3) the reliability of the variable value X; (4) the standard deviation of the sample mean.14., the most feasible way to reduce the sampling error is.(1) increasing the number of observations (2) to control individual variability(3) follow the randomization principle (4), select the observation objects strictly15. as the standard deviation of a set of observations is 0.(1) the sample number was 0 (2), and the sampling error was 0(3) the average number is 0 (4) or more16., a set of data is normally distributed, in which less than X+1.96s variables have values.(1) 5% (2) 95%(3) 97.5% (4) 92.5%[a yes, no problem]The values of the mean and median of the data of the 1. symmetrical distributions are consistent. ()2. standard error is the index of individual difference distribution. ()3., the standard deviation is large, then the sampling error is also inevitable. ()4. in sampling studies, when the sample size tends to infinity,the X tends to be equal to mu, and the sX tends to be equal to sigma X. ()5. calculate the mean by the frequency table, and the spacing of each group must be equal. ()(two) exercises1. data of total cholesterol in serum of 101 healthy men aged 30~49 years in a given area, please calculate their mean, standard deviation and standard error.(mg/dl)184219.7151.7181.4178.8157.5185.0117.5168.9172.6170.0130.0176.0201.0183.1139.4185.1206.2175.7166.3131.2207.8237.0168.8199.9135.2171.6204.8163.8129.3176.7150.9150.0152.5208.0222.6169.0171.1191.7166.9188.022.07104.2177.7137.4243.1184.9188.6155.7122.7184.0160.9252.9177.5172.6163.2201.0197.8241.2225.7199.1245.6225.7183.6157.9140.6166.3278.8200.6205.5157.9196.7188.5199.2177.9230.0167.6181.7214.0197.0173.6129.2226.3214.3174.6168.8211.5199.9237.1125.1117.9159.2251.4181.1164.0153.4246.9196.6155.4175.7One hundred and eighty-nine point two2. after 10 people were inoculated with a vaccine, the titer of antibody was determined as follows:1:2, 1:2, 1:4, 1:4, 1:4, 1:4, 1:8, 1:16, 1:32.Antibody titer of the vaccine was determined3. this 94 electric ophthalmia patients, the disease from contact welding time (hours) the following table, mean and median trial suggests that exposure to welding to the average time of onset. What indicators of civilization do you think is appropriate?Total 0-2-4-6-8-10-12-14-16-18-20-22-24- at onset contact welding hoursThe number of cases was 810211922640, 10012944., a spot check 120 samples of berberine content (mg/100g) average 4.38, standard deviation of 0.18, assuming that the data obey normal distribution:(1) what range is the content of berberine in 95% samples of Coptis?What is the overall average of the content of berberine inCoptis chinensis Franch?There is a sample of Coptis chinensis. The content of berberine is 4.80. How do you evaluate it?In the 120 samples, how many samples are there in the sample between 4 and 4.4?5. the 95% normal range and 95% confidence interval of serum total cholesterol in 101 healthy men were calculated by 1.T testI. requirements。
Benefit Guide

Employee Benefits and ServicesA Comprehensive Guide toBENEFIT GUIDE2021 - 2022WELCOME TO YOUR BENEFITSWe are proud to offer a variety of benefitoptions and health care resources to meet your individual needs. We encourage you to review this material with your family to help determine the benefit options that are best for you and your dependents.Keep this guide for future reference – it contains rates, contact information, enrollment instructions, and otherinformation you will need to get the most out of your benefits throughout the year.Benefit Contacts16Frequently Asked Questions 15Additional Benefits14Disability Benefits 13Life Insurance Options 12Vision Benefits 11Dental Benefits 10Accident / Critical Illness 9Telemedicine8Health Savings Account 7Prescription Benefits 6Medical Options Overview 5Medical Plan Comparison 4How to Enroll3Eligibility 2Benefit Highlights 1TABLE OF CONTENTSCOMPANY-PAID BENEFITSCOST-SAVINGS TOOLSWe provide these valuable benefits automatically, at no cost to you.•Basic Life and Accidental Death and Dismemberment (AD&D) Insurance •Long Term Disability Insurance •Employee Assistance ProgramTake advantage of these tools to access quality, convenient care and save money:•Telemedicine – Medical plan members can get medical care over the phone or web through MDLive• – Shop around for the best price on prescription drugsEMPLOYEE-FUNDED BENEFITSIf elected, you pay all or some of the cost for these voluntary options.Health Benefits Financial BenefitsChoose from a variety of plans to protect and improve your health:•Medical and Prescription Benefits •Health Savings Account•Supplemental Health Plans(Accident and Critical Illness Insurance)•Vision Insurance •Dental Insurance2021 - 2022 Benefit Guide |1Consider these financial benefits, which can provide protection for your future:•Voluntary Life and Accidental Death and Dismemberment (AD&D) Insurance •401(k) Retirement SavingsEMPLOYEESYou are eligible for the benefits listed in this guide if you are a full-time employee working 30 or more hours per week.DEPENDENTSIf you elect coverage for yourself, you may also elect certain coverages for your dependents as shown in the table below.IMPORTANT: If your spouse is employed and has health insurance available through their employer, theymay not enroll in your group health plan.Dependents are defined as:•Legal spouse•Your legal dependent children younger than age 26•Yo ur dependent children 26 and older who cannot care for themselves. (Contact HR for more information) BENEFITS ELIGIBILITY BY PLAN2 |2021 - 2022 Benefit GuideNEW HIRESThe effective date of your benefit coverage depends on your date of hire as shown to the right.You must complete your benefit enrollment prior toyour eligibility date.MAKING CHANGES DURING THE YEARThe IRS has rules about when you can make changes to your benefits during the year. Once you’ve submitted yourbenefits elections, you cannot change your medical, dental, vision care, or HSA elections outside the Annual Enrollment period, which typically takes place each spring, unless you experience an IRS-defined life event as listed below.YOU HAVE 31 DAYS TO REQUEST CHANGESIf you experience one of these life events, please contact the Benefits Department as soon as possible because you have only 31 days from the date of the event to make changes. If you do not, you must wait until the next Annual Enrollment period to make changes.2021 - 2022 Benefit Guide |34 |2020 - 2021 Benefit GuideCHOICE PLANHere’s a quick refresher oncommonly used insurance terms:A PREMIUM is the amount you pay for insurance, using pre-tax or post-tax dollars. (Note: Inmost cases, the Company pays a portion of the premium.)A COPAYMENT (COPAY) is a fixed amount you pay for health care services or prescription drugs.A DEDUCTIBLE is the amount you pay before your insurance begins covering certain services such as hospitalization or outpatient surgery.COINSURANCE is the amount you pay, as a percentage of the cost of your allowed services, after you reach the deductible until you reach the plan’s out-of-pocket maximum.An ALLOWABLE CHARGE is the dollar amount typically considered payment in full by an insurance company and an associated network of health care providers.An OUT-OF-POCKETMAXIMUM is the most you pay per Plan Year for health care expenses, including prescription drugs. Once this limit is met, the plan pays 100% for the remainder of the Plan Year.GLOSSARY2021 -2022 Benefit Guide |5Our medical plans are administered by Blue Cross Blue Shield of Texas.A summary of each plan is below. Be sure to compare the plans’key features using the chart on page 4.WHERE TO FILL PRESCRIPTIONSYou have two options when filling prescriptions: retaillocations or mail order program.6 |2021 -2022 Benefit GuideBOTH MEDICAL PLANS INCLUDEPRESCRIPTION DRUG COVERAGE.As you can see in the PRESCRIPTIONS section of themedical comparison chart on page 4, the price you’ll payfor medications depends on the tier and the type of drug.The amount you pay for prescriptions also depends onwhich of our medical plans you enroll in, as explainedbelow.IF YOU ENROLL IN THE PREMIERMEDICAL PLAN , YOU ARE ELIGIBLE TO OPEN A HEALTH SAVINGS ACCOUNT (HSA).An HSA is a savings account where you set aside money –TAX FREE – to pay for healthcare expenses, including medical, prescription drug, dental, and vision expenses.It can do more than help cover your current healthcare needs like deductibles and coinsurance. It can even help you boost your retirement savings!*Your HSA belongs to you, not the Company. Any money left over at the end of the year rolls over. You can use this money to offset your medical and prescription expenses as soon as it is in your account.*Note: Unused HSA funds can only be withdrawn without penalty for non-medical purposes after age 65.Save Two Ways with These Tax Benefits1.Your HSA contributions are tax-free when they are made through payroll deductions. Not only do you save money on qualified health care expenses, but your taxable income is lowered.2.Your HSA is tax-free when you spend it on qualifiedhealth care expenses.2021 -2022 Benefit Guide |72021 Contribution LimitsFunding Your HSADuring Annual Enrollment or as a new hire, you select the amount you want to deposit from each paycheck into your HSA. The total amount you contribute cannot exceed the limits shown in the table above.Accessing Your HSA AccountContact WEX (formerly Discovery Benefits) at 866-451-3399 or online account athttps:///Login.aspxSave Your Receipts!It’s important to save the receipts for every purchase you make with the card. You may need the receipts to verify expenses. To find out more about qualified healthcare expenses, go to .TELEMEDICINE – INCLUDED WITH ALL MEDICAL PLAN OPTIONSOur medical plans include access to Telemedicine Services. You can interact with in-network, U.S. board certified physicians 24 hours a day/365 days a year,without having to make an appointment, via secure video chat or phone without leaving your home or office! Using telemedicine can help get you the doctor visit and prescription you need while also saving you time and money.When should you use Telemedicine?Some of the most common uses include:•Cold & flu symptoms such as cough, fever,earaches, and headaches •Allergies & sinus infections •Fever•Bladder infections, UTIs8 |2021 -2022 Benefit Guide2021 -2022 Benefit Guide |9Wellness Benefit:Get a one-time yearly benefit of $50 for each coveredperson who receives preventive and wellness services during the plan year.•Hospital admission: $1,500•Emergency room visit: $200•Outpatient surgery: $300Examples:Benefit Payment Accidents happen, with Accident insurance through UNUM, ACCIDENT INSURANCEget coverage for yourself as well as your family.those extra expenses or anything else you wish. You can you are paid a lump-sum cash benefit to help take care ofPreventive care: Annual exams, cleanings, and X-rays Basic services: Fillings, scaling and root planning, oral surgery Major Services:Endodontics, periodontics, crowns, bridges, dentures10 |2021 -2022 Benefit GuideChild Orthodontia:Braces are covered 50%for children up to age 19($1,500 lifetime maximum)The dental plans available through MetLife allow you to seek treatment from the dental provider of your choice. It is important that you understand your dentist may not have contracted rates with MetLife, so you must discuss billing with your provider prior to receiving services. This will give you the peace of mind of knowing what your estimated portion of the bill will be. Visit MetLife’s website at /mybenefits or call 800-942-0854 to find a dentist.GET COVERAGE FOR A VARIETY OF SERVICESRoutine eye exam: You payjust $10Contact Lenses: Covered up to $150Contact fitting and evaluation: Won’texceed $60BENEFITS AVAILABLE ONCE PER PLAN YEAR2021 -2022 Benefit Guide |11Frames:Covered up to $175 after $25 materials copayOur vision plan is administered by MetLife. When you enroll in this plan, you will receive access to care from great eye doctors, quality eye wear and the affordability you deserve, all at the lowest out-of-pocket costs. To locate a provider visit or call 800-942-0854.*Spouse rate is based on employee’s age or by contacting a member of HR.*Evidence of insurability can be accessed onBASIC TERM LIFE AND ACCIDENTAL DEATH AND DISMEMBERMENT (AD&D) INSURANCE12 |2021 -2022 Benefit Guidecoverage elected.complete an evidence of insurability for any amount of only during Annual Enrollment and will be required to days of your initial eligibility date, you can apply for coverage If you and your eligible dependents do not enroll within 31insurability, which is a medical questionnaire.for your spouse without providing an evidence of coverage up to $300,000for yourself and up to $50,000your initial eligibility date, you may apply for any amount of If you or your eligible dependents enroll within 31 days of When You Enroll Makes a Difference•Child(ren): $2,000 to $10,000voluntary term life amount •Spouse: up to 100% of the employee’s $500,000)salary in increments of $10,000 (not to exceed •Employee: lesser of up to five times your annual you, your spouse, and your dependent child(ren).Term Life and AD&D coverage is available to purchase for provides enough financial protection you need. Voluntary and AD&D coverage, it’s important to ask yourself if it Although the Company provides you with Basic Term Life DISMEMBERMENT (AD&D) INSURANCELIFE AND ACCIDENTAL DEATH AND EMPLOYEE-PAID VOLUNTARY TERM The Company understands how important it is toprotect your finances in case of an unexpected passing or accidental dismemberment. Therefore, we offer term life insurance and AD&D at no cost to you. The benefit amount is two times your annual salary up to a maximum of $300,000 for both life and AD&D. You receive thiscoverage automatically – there is no need to enroll.COMPANY-PAID LONG TERM DISABILITYThe Company provides eligible employees with Long Term Disability (LTD) coverage at no cost. The benefit amount is equal to 60% of base, pre-disability monthly earnings up to a maximum of $10,000 per month.How the Plan WorksBenefits become payable 90 calendar days after date of disability. You receive this coverage automatically – thereis no need to enroll.EMPLOYEE-PAID SHORT TERM DISABILITYAlthough the Company provides company-paid Long-Term Disability coverage, you may elect Voluntary Short-TermDisability (STD) coverage. Long Term Disability does not offer a benefit until after 90 calendar days of missed work, so if you would like financial protection before those 90 days, STD would be a great option to help bridge the gap during that period.How the Plan WorksIf your absence is determined to be an approveddisability, you will receive 60% of your base pay, up to a maximum of a $2,000 benefit per week. (There is a 7-day waiting period before Short Term Disability goes into effect).PLEASE NOTE: Pregnancy is considered a pre-existing condition if you are pregnant prior to your plan’s effective date.Calculating Your STD Plan CostThe cost of your STD plan depends on how much you earn. The plan pays 60% of your weekly base pay. Below is an example of the bi-weekly cost for an employee earning $500 per week in base salary.2021 -2022 Benefit Guide |13401(K)As an employee, you have an opportunity totake advantage of the company’s 401(k) plan. A401(k)plan is a fantastic way for you to save for retirement. You can set aside funds on a pre-tax orafter-tax (Roth) basis – or both. It’s your choice how much you would like to contribute, up to the IRS limit each year.When Can I Start?You are eligible to begin participating the quarter following 90 days of employment. The chart below shows when you will become eligible for participation. Approximately 30 days prior to your eligibility date, you will receive a reminder either by email or mailed to your home address.ADDITIONAL BENEFITSCompany MatchYou are eligible for the Company match after completing one year of service. The company matches 100% of your tax deferred contributions, up to 4% of your compensation each pay period.VestingYou are 100% vested in the contributions you make to the plan.How do I find out what benefits I am enrolled in today?You may access your current benefits elections by logging into UKG Pro.Can I add someone to my medical, dental, and/or vision coverage during Annual Enrollment?Yes, eligible dependents may be added to the plan, this includes, a legal spouse, or children who are under the age of 26.If I decide to waive benefits as a new hire or during Annual Enrollment, will I be able to add coverage later on?Yes, but you will have to wait until the next Annual Enrollment period. You are not allowed to enroll or make changes to your benefit elections mid-year unless you have a qualifying life event, such as marriage, divorce, birth or adoption of a child, or death of a dependent. For a list of qualifying events, please review page 3 of this guide.Who can open a Health Savings Account (HSA)?HSAs are governed by the Internal Revenue Code (IRC), and you must meet the following eligibility requirements to qualify for a HSA:•You must be enrolled in the Premier Plan.•You are not enrolled in Medicare or Tricare.•You are not claimed as a tax dependent on someone else’s tax return.What are the 2021 HSA Limits?•$3,600 for Individuals•$7,200 for Family•Individuals over the age of 55 can contribute an additional $1,000 per year2021 -2022 Benefit Guide|15For more information please visit https:// or contact us at: Phone: (817) 693-2890;Fax (817) 212-3310; E mail: benefits@.© 2021Wilks Brothers, LLC. All Rights Reserved.1718 | 2019 - 2020 Benefit Guide。
Are overconfident CEOs better innovators
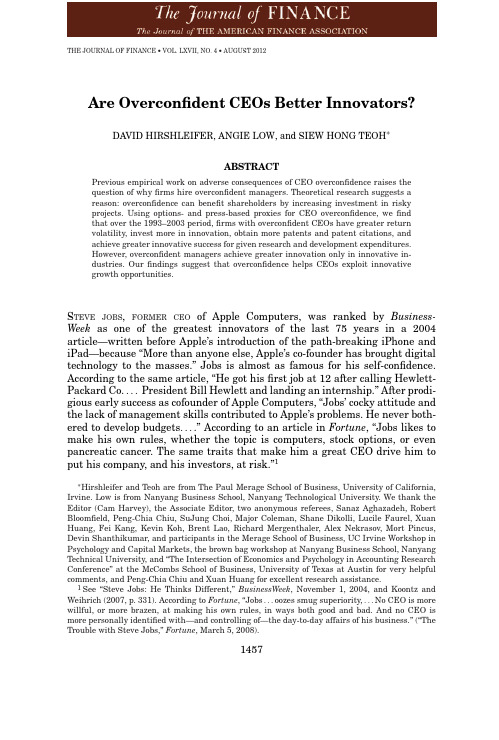
THE JOURNAL OF FINANCE•VOL.LXVII,NO.4•AUGUST2012Are Overconfident CEOs Better Innovators?DAVID HIRSHLEIFER,ANGIE LOW,and SIEW HONG TEOH∗ABSTRACTPrevious empirical work on adverse consequences of CEO overconfidence raises thequestion of whyfirms hire overconfident managers.Theoretical research suggests areason:overconfidence can benefit shareholders by increasing investment in riskying options-and press-based proxies for CEO overconfidence,wefindthat over the1993–2003period,firms with overconfident CEOs have greater returnvolatility,invest more in innovation,obtain more patents and patent citations,andachieve greater innovative success for given research and development expenditures.However,overconfident managers achieve greater innovation only in innovative in-dustries.Ourfindings suggest that overconfidence helps CEOs exploit innovativegrowth opportunities.S TEVE JOBS,FORMER CEO of Apple Computers,was ranked by Business-Week as one of the greatest innovators of the last75years in a2004 article—written before Apple’s introduction of the path-breaking iPhone and iPad—because“More than anyone else,Apple’s co-founder has brought digital technology to the masses.”Jobs is almost as famous for his self-confidence. According to the same article,“He got hisfirst job at12after calling Hewlett-Packard Co....President Bill Hewlett and landing an internship.”After prodi-gious early success as cofounder of Apple Computers,“Jobs’cocky attitude and the lack of management skills contributed to Apple’s problems.He never both-ered to develop budgets....”According to an article in Fortune,“Jobs likes to make his own rules,whether the topic is computers,stock options,or even pancreatic cancer.The same traits that make him a great CEO drive him to put his company,and his investors,at risk.”1∗Hirshleifer and Teoh are from The Paul Merage School of Business,University of California, Irvine.Low is from Nanyang Business School,Nanyang Technological University.We thank the Editor(Cam Harvey),the Associate Editor,two anonymous referees,Sanaz Aghazadeh,Robert Bloomfield,Peng-Chia Chiu,SuJung Choi,Major Coleman,Shane Dikolli,Lucile Faurel,Xuan Huang,Fei Kang,Kevin Koh,Brent Lao,Richard Mergenthaler,Alex Nekrasov,Mort Pincus, Devin Shanthikumar,and participants in the Merage School of Business,UC Irvine Workshop in Psychology and Capital Markets,the brown bag workshop at Nanyang Business School,Nanyang Technical University,and“The Intersection of Economics and Psychology in Accounting Research Conference”at the McCombs School of Business,University of Texas at Austin for very helpful comments,and Peng-Chia Chiu and Xuan Huang for excellent research assistance.1See“Steve Jobs:He Thinks Different,”BusinessWeek,November1,2004,and Koontz and Weihrich(2007,p.331).According to Fortune,“Jobs...oozes smug superiority,...No CEO is more willful,or more brazen,at making his own rules,in ways both good and bad.And no CEO is more personally identified with—and controlling of—the day-to-day affairs of his business.”(“The Trouble with Steve Jobs,”Fortune,March5,2008).14571458The Journal of Finance RIs this combination of visionary innovation and extraordinary overconfidence a coincidence?Here,we examine a different possibility—that for CEOs,the two go hand in hand.A recent literature in corporatefinance examines how managers’psycholog-ical biases or characteristics affectfirm decisions(see,for example,Bertrand and Schoar(2003),Baker,Pan,and Wurgler(2009)).Our focus is on overconfi-dence,the tendency of individuals to think that they are better than they really are in terms of characteristics such as ability,judgment,or prospects for suc-cessful life outcomes(the last item is sometimes called“optimism”).Theoretical research analyzes why overconfidence exists(Benabou and Tirole(2002),Van den Steen(2004)).Psychological and other research indicates that people,in-cluding experts,tend to be overconfident along a variety of dimensions,but that there is substantial and persistent individual variation in the degree of confidence(see,for example,Oskamp(1965),Weinstein(1980),Wagenaar and Keren(1986),Brenner et al.(1996),and Puri and Robinson(2007)).Overconfident individuals tend to overestimate the net discounted expected payoffs from uncertain endeavors,either because of a general tendency to ex-pect good outcomes,or because they overestimate their own efficacy in bringing about success.Furthermore,people tend to be more overconfident about their performance on hard rather than easy tasks(Griffin and Tversky(1992)).Ac-cordingly,we expect relatively overconfident CEOs to be especially enthusiastic about risky,challenging,and talent-and vision-sensitive enterprises. Innovative projects—which apply new business methods,develop new tech-nologies,or offer new products or services—are risky and challenging.We therefore expect managerial overconfidence to be potentially important for such undertakings.Reinforcing this conjecture,the outcomes of innovative projects take a long time to resolve,and overconfidence tends to be more severe in set-tings with ambiguous and deferred feedback(Einhorn(1980)).Adopting inno-vative projects may also be viewed as indicative of superior managerial“vision.”Innovative projects are thus likely to appeal to self-aggrandizing managers. We therefore hypothesize that(even after including standardfirm-level con-trols and industry and yearfixed effects)firms with overconfident managers accept greater risk,invest more heavily in innovative projects,and achieve greater innovation.2The effect of overconfidence on project selection could come from either overestimation of expected cashflows or underestimation of risk. Whether overconfident CEOs will be better innovators after controlling for the level of spending on research and development(R&D)is less clear.On the one hand,overconfident managers who pursue innovation aggressively may undertake projects with low expected payoff.On the other hand,rational managers may,from the viewpoint of shareholders,excessively prefer the“D”2Some studies fail tofind evidence of overconfidence in certain contexts(see,for example, Gigerenzer,Hoffrage,and Kleinbolting(1991)versus Griffin and Tversky(1992)).Although there are exceptions,the preponderance of evidence supports a general tendency toward overconfidence in various manifestations(see,for example,DeBondt and Thaler(1995)and Rabin(1998)).How-ever,it is not crucial for our purposes whether CEOs are,on average,overconfident.Our tests rely upon substantial differences in the degree of confidence across managers.Are Overconfident CEOs Better Innovators?1459 in R&D—fairly reliable projects rather than risky but more promising inno-vative ones.Overconfident managers can potentially achieve higher average innovative productivity by accepting good but risky projects.This benefit of managerial overconfidence is reflected in recent theoretical models(Goel and Thakor(2008),Gervais,Heaton,and Odean(2011)).As a result,we do not hypothesize the direction of the effect of overconfidence on the effectiveness of the CEO in generating innovation for given R&D expenditures.The biggest puzzle raised by existing research on managerial beliefs and corporate policy is thatfirms often employ overconfident managers and give them leeway to follow their beliefs in making major investment andfinanc-ing decisions(Malmendier and Tate(2005a,2005b,2008)and Ben-David, Graham,and Harvey(2010)).This is counterintuitive,as we would normally view unbiased beliefs as preferable.Furthermore,Graham,Harvey,and Puri (2010)provide evidence of a matching of growthfirms with more confident managers(as proxied by height).This puts the most confident managers into thosefirms where overconfidence can radically influence strategy,investment choices,and survival.By measuring ex post success,we suggest a possible so-lution to this overconfident manager puzzle:overconfident managers are better innovators.To test our hypotheses,we use alternative proxies for managerial overconfi-dence based on options exercise behavior or press coverage.The options exercise measure(Malmendier and Tate(2005a))builds on the idea that a manager who chooses to be exposed to thefirm’s idiosyncratic risk is likely to be confident about thefirm’s prospects.Under this approach,a CEO who voluntarily retains stock options after the vesting period in which exercise becomes permissible is viewed as overconfident.3Our second measure of overconfidence is based on the portrayal of the CEO in the news media,as developed by Malmendier and Tate(2005b,2008).This measure employs counts of words relating to over-confidence or its opposite in proximity to the company name and the keyword “CEO.”We measure thefirm’s innovation-related investment by the level of R&D expenditures.Ourfirst measure of innovative output and R&D success is the number of patents applied for during the year from the U.S.Patents and Trademarks Office.Patents differ greatly in their importance,so,following Trajtenberg(1990),our second measure of innovative output is total citation count.This is the total number of citations subsequently received by the patents applied for during the year,where citations are made by other newer patents. Wefind that over the1993–2003period,firms with overconfident CEOs have higher stock return volatility,consistent with their undertaking riskier projects.Overconfident CEOs invest more heavily in R&D and achieve greater innovation as measured by patent and citation counts.Greater innovative out-put is not just a result of greater resource input;overconfident CEOs achieve 3Malmendier and Tate(2005a,2008)develop measures of CEO overconfidence based on options exercise behavior and insider net stock purchases.Billett and Qian(2008),Liu and Taffler(2008), and Campbell et al.(2011)also adopt this measurement approach.1460The Journal of Finance Rgreater innovative success even after controlling for the level of R&D expendi-tures.Patenting may be less relevant for certain industries,either because they are less innovative or because,in these industries,innovation does not result in patents.Wefind that overconfident managers achieve greater total patents and citations than non-overconfident managers only in industries where in-novation is important.We also provide evidence that our results are not due to overconfident CEOs having private information about future profits or to overconfident CEOs just being more risk-tolerant.The greater innovative output for given R&D input achieved by overconfi-dent CEOs does not necessarily translate into higherfirm value.Hall,Jaffe, and Trajtenberg(2005)show that,on average,patent citations are positively correlated withfirm value,but overconfident CEOs could be overpaying to achieve increased citation counts(possibly using resources other than R&D expenditures),reducingfirm value.A possible way to address this issue is to regressfirm value on CEO overconfidence or the innovation that results from it.However,such a test is subject to endogeneity problems.Instead,using an instrument for exogenous growth opportunities,we examine a more limited question:are overconfident CEOs better at translating external growth oppor-tunities intofirm value?Wefind that the answer is yes,and that this relation is especially strong among industries where innovation is important. Throughout,wefind that the effect of overconfidence on innovation is mainly found among innovative industries.Since innovative industries should contain more good risky growth opportunities,our results are consistent with models such as those of Goel and Thakor(2008)and Gervais,Heaton,and Odean (2011)that imply high benefits to overconfidence when such opportunities are present.Recent work identifies other important effects of managerial overconfidence onfirm investments.Malmendier and Tate(2005a)propose that overconfident managers are optimistic about investment opportunities,but overestimate the value of theirfirms’equity and therefore the cost of externalfinancing.This implies thatfirms with overconfident CEOs will have greater investment–cash flow sensitivity.Their evidence is consistent with this prediction.Ben-David, Graham,and Harvey(2010)document thatfirms whose CFOs are overcon-fident in the sense of having miscalibrated beliefs undertake greater capital expenditures.Our paper differs from these contributions in focusing on inno-vative investments,for which we would expect overconfidence to be especially important,and on the effectiveness of this investment as measured by patent and citation counts for a given level of innovative investment.With regard to otherfirm behaviors,Hribar and Yang(2011)find that overconfident managers are more likely to issue optimistically biased fore-casts.Schrand and Zechman(2010)find that overconfidence is associated with a greater likelihood of earnings management andfinancial fraud.Graham, Harvey,and Puri(2010)document a relation between managerial traits,in-cluding confidence,and a variety of corporate policies.Malmendier,Tate,and Yan(2011)find that overconfident managers are less likely to use external finance,and issue less equity.Malmendier and Tate(2008)find that CEOAre Overconfident CEOs Better Innovators?1461 overconfidence is associated with making acquisitions,and with more negative market reactions to acquisition announcement.Most of thesefindings add to the puzzle of whyfirms are willing to hire overconfident managers.4The paper proceeds as follows.We describe the data and variable construction in Section I.In Section II,we examine the relation between overconfident CEOs and stock return volatility,while in Section III,we test the relation between overconfident CEOs and innovative activities.We provide several extensions and consider alternative explanations for ourfindings in Section IV.In Sec-tion V,we test whether overconfidence is associated with increased innovative efficiency andfirm value.We conclude in Section VI.I.Data and Descriptive StatisticsA.The DataWe use several databases to construct our sample.Standard and Poor’s Ex-ecucomp database provides information on CEOs and their compensation,and we use the data on option compensation to construct one of our two measures of CEO overconfidence.The second overconfidence measure relies on keyword searches of the text of press articles in Factiva.All accounting data are from Compustat and stock returns are from CRSP.Patent-related data are from the 2006edition of the NBER patent database.The sample consists offirms in the intersection of Execucomp,Compustat, CRSP,and the patent database.All Execucompfirms that operate in the same four-digit SIC industries as thefirms in the patent database are included;the sample is therefore not limited tofirms with patents.Firm-years with miss-ing data on any of the control variables and dependent variables are deleted. We further require that there be information on at least one of the CEO over-confidence measures.Since these measures are lagged by1year,we require that the CEO be the same one in the prior year to ensure that we observe the characteristics of the CEO in place at the time the innovation is being measured.Financialfirms and utilities are excluded.Thefinal sample con-sists of2,577CEOs from9,807firm-year observations between1993and2003. Of these observations,8,939firm-years have information on the options-based measure,while7,762firm-years have information on the press-based measure of overconfidence.4After developing this paper,we became aware of a recent paper that examines the relation between managerial overconfidence and innovation(Galasso and Simcoe(2010)).Our papers differ in several ways.We examine how overconfidence affects risk-taking as well as innovation,and we show that the effects of managerial overconfidence come solely from innovative industries. We also examine the effects of overconfidence onfirm performance.To ensure the robustness of our conclusions,we use the press-based measure of overconfidence as well as the options-based measure.Finally,our time period and sample size differ substantially.Our time period,1993to 2003,encompasses the millennial high-tech boom,and overlaps little with their1980–1994sample. Our sample is also much larger,as it is drawn from the top1,500firms covered by Execucomp.In particular,our sample consists of1,771firms and9,807firm-year observations,while their sample covers290firms and3,648firm-years.1462The Journal of Finance RTo test our hypothesis that overconfident CEOs undertake riskier projects, as the dependent variable we use the standard deviation of daily stock returns during thefiscal year.We measure innovation using R&D expenditures and patenting activities,which we describe in detail in the next subsection.The measurement of CEO overconfidence and the associated control variables are also discussed below.A detailed summary of variable definitions is provided in the Appendix.A.1.Measuring InnovationWe measure resource input into innovation with R&D scaled by book assets. Firm-years with missing R&D information are assigned a0R&D value.5Our output-oriented measures of innovation are based on patent counts and patent citations.Data for patent counts and patent citations are constructed using the2006 edition of the NBER patent database(Hall,Jaffe,and Trajtenberg(2001)). This covers over3.2million patent grants and23.6million patent citations from1976to2006.Our second measure of innovation is the number of patent applications by afirm during the year.Patents are included in the database only if they are eventually granted. Furthermore,there is,on average,a2-year lag between patent application and patent grant.Since the latest year in the database is2006,patents applied for in2004and2005may not appear in the database.As suggested by Hall,Jaffe, and Trajtenberg(2001),we end our sample period in2003and include year fixed effects in our regressions to address potential time truncation issues. Simple patent counts capture innovation success imperfectly(see,for exam-ple,Griliches,Pakes,and Hall(1987))as patent innovations vary widely in their technological and economic importance.A measure of the importance of a patent is its citation count.Patents continue to receive citations from other patents for many years subsequent to granting.Trajtenberg(1990)concludes that citations are related to the social value created by the innovation;Hall, Jaffe,and Trajtenberg(2005)show that forward citations are related tofirm value as measured by Tobin’s Q.Therefore,our third measure of innovation is the total number of citations ultimately received by the patents applied for during the given year.This measure takes into account both the number of patents and the number of citations per patent.(Results are similar when we exclude self-citations.)Survivorship bias is minimal in the patent database.6However,owing to the finite length of the sample,citations suffer from a time truncation bias.Since5Our results are robust to deletingfirm-years with missing R&D instead.The major robust-ness checks in the paper that are not tabulated in the main text are contained in the Inter-net Appendix,which is available online in the”Supplements and Datasets”section at http:// /supplements.asp.6An ultimately successful patent application is counted and attributed to the applyingfirm at the time of application even if thefirm is later acquired or goes bankrupt.Furthermore,citations are specific to a patent and not afirm.Therefore,a patent that belongs to a bankruptfirm can continue to receive citations in the database for many years after thefirm goes out of existence.Are Overconfident CEOs Better Innovators?1463 citations are received for many years after a patent is created,patents created near the ending year of the sample have less time to accumulate citations.To address this,we follow the recommendations of Hall,Jaffe,and Trajtenberg (2001,2005)and adjust the citation count of each patent in two different ways.For thefirst adjustment,each patent’s citation count is multiplied by the weighting index from Hall,Jaffe,and Trajtenberg(2001,2005),also found in the NBER patent database.7The variable Qcitation count is the sum of the adjusted patent citations across all patents applied for during eachfirm-year. For the second adjustment,each patent’s citation count is scaled by the average citation count of all patents in the same technology class and year.The variable TTcitation count is the sum of the adjusted citation count across all patents applied for by thefirm during the year.8A.2.Options-Based Measure of CEO OverconfidenceThe options-based overconfidence measure is based on the premise that it is typically optimal for risk-averse,undiversified executives to exercise their own-firm stock options early if the option is sufficiently in the money(Hall and Murphy(2002)).Following Malmendier and Tate(2005a,2008),Confident CEO(Options)takes a value1if a CEO postpones the exercise of vested options that are at least67%in the money,and0otherwise.If a CEO is identified as overconfident by this measure,she remains so for the rest of the sample period. This treatment is consistent with the notion that overconfidence is a persistent trait.As we do not have detailed data on a CEO’s options holdings and exercise prices for each option grant,we follow Campbell et al.(2011)in calculating the average moneyness of the CEO’s option portfolio for each year.First,for each CEO-year,we calculate the average realizable value per option by dividing the total realizable value of the options by the number of options held by the CEO.The strike price is calculated as thefiscal year-end stock price minus the average realizable value.The average moneyness of the options is then calculated as the stock price divided by the estimated strike price minus one. As we are only interested in options that the CEO can exercise,we include only the vested options held by the CEO.Malmendier and Tate(2005a,2008)classify a CEO who failed to exercise a 67%in-the-money option and who has5years of remaining duration as overcon-fident.In contrast,our overconfidence measure is based solely on nonexercise when average moneyness is ing this measure with the Execucomp7The weighting index is created using a quasi-structural approach where the shape of the citation-lag distribution is econometrically estimated.8An advantage of TTcitation count is that it takes into account the differing propensity for patents in a different technology class to cite other patents.However,such an adjustment assumes that any average difference in citation rates across technologyfields is an artifact of different citation habits acrossfields rather than an actual difference in the value of the knowledge created. Therefore,we also report results with Qcitation count.1464The Journal of Finance Rsample allows us to include morefirms and to cover a more recent period that includes the millennial high-tech boom.Although this measure is less precise, Malmendier,Tate,and Yan(2011)show that it works well after controlling for past stock return performance.Furthermore,Campbell et al.(2011)show that this measure of overconfidence generates results similar to those in Mal-mendier and Tate(2005a).A.3.Press-Based Measure of CEO OverconfidenceFollowing Malmendier and Tate(2005b,2008)and Hribar and Yang(2011), we also use a press-based measure of CEO overconfidence.9We search Factiva for articles referring to the CEO in The New Y ork Times,BusinessWeek,Fi-nancial Times,The Wall Street Journal,The Economist,Fortune,and Forbes. Specifically,we retrieve all articles using the available unique company code in Factiva and the search keyword“CEO.”For each CEO and year,we record (1)the total number of articles,(2)the number of articles containing the words “confident,”“confidence,”or variants such as overconfidence and overconfident, (3)the number of articles containing the words“optimistic,”“optimism,”or variants such as overoptimistic and overoptimism,(4)the number of arti-cles using“pessimistic,”“pessimism,”or variants such as overpessimistic,and (5)the number of articles using“reliable,”“steady,”“practical,”“conservative,”“frugal,”“cautious,”or“gloomy.”Category5also contains articles in which “confident”and“optimistic”are negated.For each year,we compare the number of articles that use the“Confident”terms,that is,categories2and3,and the number of articles that use the “Cautious”terms,that is,categories4and5.We measure CEO overconfidencefor each CEO i in year t asConf ident C EO(Press)it=⎧⎪⎪⎨⎪⎪⎩1ifts=1a is>ts=1b is0otherwise,(1)where a is is the number of articles using the Confident terms and b is is the number of articles using the Cautious terms.We cumulate articles starting from thefirst year the CEO is in office(for CEOs who assumed office after 1992)or1992,when we begin our article search and also thefirst year of Execucomp data.Following Malmendier and Tate(2008),we also control for the total number of press mentions over the same period(TotalMention).The press may be biased toward positive stories and this would imply a higher number of mentions as “confident”or“optimistic”when there is more attention in the press.In our regression tests,the CEO overconfidence measures are lagged by one period 9Other approaches to measuring executive overconfidence include surveys and psychometric tests(Ben-David,Graham,and Harvey(2010)and Graham,Harvey,and Puri(2010))and the CEO’s prevalence in photographs in the annual report(Schrand and Zechman(2010)).Are Overconfident CEOs Better Innovators?1465 with respect to the dependent variable.Thus,only past articles are used to predict innovation.In one of our robustness checks,we define our press-based confidence measure using only news articles in the past1year;the results are generally similar.A.4.Other Explanatory V ariablesWhen explaining patenting activities,following Hall and Ziedonis(2001), we include controls forfirm size and capital intensity,wherefirm size is the natural logarithm of sales.Capital intensity is proxied by the natural logarithm of the ratio of net property,plant,and equipment in2006dollars to the number of employees.Aghion,Van Reenen,and Zingales(2009)show that innovative activities are affected by institutional holdings,so we include a measure of the percentage of shares held by institutional investors.Both of our measures of overconfidence may be affected by past stock per-formance.High returns increase the moneyness of options held by CEOs.So, in addition to reflecting overconfidence in the exercise decision of the CEO, our overconfidence measure may reflect stock price performance subsequent to the option grant date.High past returns could also be associated with greater press usage of the word“confident.”If good stock performance is also associated with more innovation,our tests may capture a spurious association between measured overconfidence and innovation.We therefore control for the buy and hold stock return over thefiscal year preceding the measurement of the de-pendent variable.In additional tests,we verify the robustness of the results to controlling for stock returns over longer periods.When explaining stock return volatility and R&D expenditures,we include as control variablesfirm size,capital intensity,Tobin’s Q,sales growth,return on assets(ROA),stock return,book leverage,and cash holdings.All the regres-sions include year and industryfixed effects,where the industry is defined at the two-digit SIC level.We also include controls that take into account CEO tenure and incentives: CEO delta and CEO option holdings vega.Delta is defined as the dollar change in a CEO’s stock and option portfolio for a1%change in stock price,and measures the CEO’s incentives to increase stock price.Vega is the dollar change in a CEO’s option holdings for a1%change in stock return volatility,and measures the risk-taking incentives generated by the CEO’s option holdings. We calculate delta and vega values using the1-year approximation method of Core and Guay(2002).The results are robust to controlling for CEO incentives using percentage stock ownership and option holdings instead of delta and vega.All control variables are lagged by one period and winsorized at the1% level in both tails.B.Descriptive StatisticsTable I describes the frequency of overconfident CEOs in our sample.Steve Jobs of Apple Computers turns out to be overconfident in our sample using。
三千多个 植物学名词中英文对照1
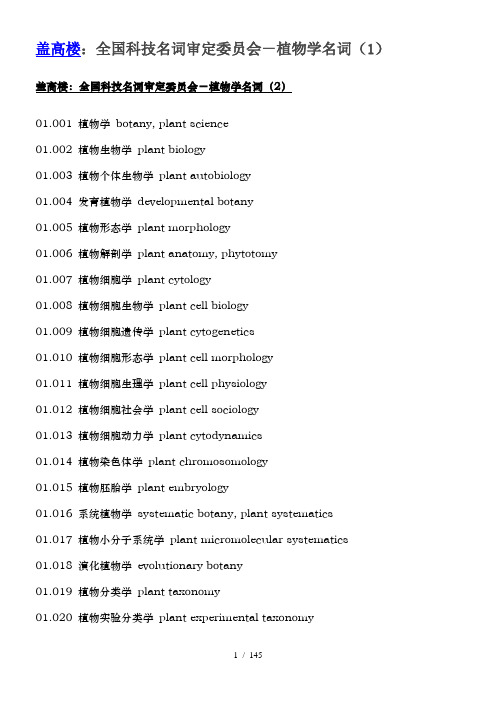
盖高楼:全国科技名词审定委员会-植物学名词(1)盖高楼:全国科技名词审定委员会-植物学名词(2)01.001 植物学botany, plant science01.002 植物生物学plant biology01.003 植物个体生物学plant autobiology01.004 发育植物学developmental botany01.005 植物形态学plant morphology01.006 植物解剖学plant anatomy, phytotomy01.007 植物细胞学plant cytology01.008 植物细胞生物学plant cell biology01.009 植物细胞遗传学plant cytogenetics01.010 植物细胞形态学plant cell morphology01.011 植物细胞生理学plant cell physiology01.012 植物细胞社会学plant cell sociology01.013 植物细胞动力学plant cytodynamics01.014 植物染色体学plant chromosomology01.015 植物胚胎学plant embryology01.016 系统植物学systematic botany, plant systematics01.017 植物小分子系统学plant micromolecular systematics01.018 演化植物学evolutionary botany01.019 植物分类学plant taxonomy01.020 植物实验分类学plant experimental taxonomy01.021 植物化学分类学plant chemotaxonomy01.022 植物化学系统学plant chemosystematics 01.023 植物血清分类学plant serotaxonomy01.024 植物细胞分类学plant cellular taxonomy 01.025 植物数值分类学plant numerical taxonomy 01.026 植物分子分类学plant molecular taxonomy 01.027 植物病毒学plant virology01.028 藻类学phycology01.029 真菌学mycology01.030 地衣学lichenology01.031 苔藓植物学bryology01.032 蕨类植物学pteridology01.033 孢粉学palynology01.034 古植物学paleobotany01.035 植物生理学plant physiology01.036 植物化学phytochemistry01.037 植物生态学plant ecology, phytoecology01.038 植物地理学plant geography, phytogeography 01.039 植物气候学plant climatology01.040 植物病理学plant pathology, phytopathology 01.041 植物病原学plant aetiology01.042 植物毒理学plant toxicology01.043 植物历史学plant history01.044 民族植物学ethnobotany01.045 人文植物学humanistic botany 01.046 植物遗传学plant genetics01.047 植物发育遗传学plant phenogenetics 01.048 分子植物学molecular botany01.049 分类单位taxon 又称“分类群”。
数学专业英语修改

7
第8页/共31页
e.g . A ratio is always an abstract number; i.e., it has no units, a number considered apart from the measured units from which it came.
第27页/共31页
27
课堂作业 1
定义 设函数 y f (x) 在点 x0的某一邻域内有定义, 如
果 lim y x0
lim
x0
f
( x0
x)
f
(x0 )
0,
那么就称函数
y f (x)
在 x0连续.
第28页/共31页
28
课堂作业 2 Theorem 2. If f (x) is bounded on a,b and if it is
3、能用英语书写文章摘要、学术会议 通知、学术交流信件等。同时培养简单 的英语会话能力。 4、为部分优秀学生攻读研究生奠定数 学专业英语的基础,同时让大部分同学 了解数学专业英语与生活英语的区别, 为今后走 上工作岗位,特别是服务于IT业或外资 企业有独当一面的能力。
第3页/共31页
本课程分四部分讲解:
B(a,r), we have h(x)d (x) h(a) . B
第12页/共31页
特点二:科学内容的完整性与表达形式的精 炼性要求
1、长句较多 2、非限定动词使用频率高
科技文章要求叙述准确,推理谨严,因此一句话里包 含三四个甚至五六个分句的,并非少见.译成汉语时, 必须按照汉语习惯破成适当数目的分句,才能条理清楚, 避免洋腔洋调. 这种复杂长句居科技英语难点之首,要 学会运用语法分析方法来加以解剖,以便以短代长,化 难为易.
BClustLonG 软件包说明书

Package‘BClustLonG’October12,2022Type PackageTitle A Dirichlet Process Mixture Model for Clustering LongitudinalGene Expression DataVersion0.1.3Author Jiehuan Sun[aut,cre],Jose D.Herazo-Maya[aut],Naftali Kaminski[aut],Hongyu Zhao[aut],and Joshua L.Warren[aut], Maintainer Jiehuan Sun<*********************>Description Many clustering methods have been proposed,butmost of them cannot work for longitudinal gene expression data.'BClustLonG'is a package that allows us to perform clustering analysis forlongitudinal gene expression data.It adopts a linear-mixed effects frameworkto model the trajectory of genes over time,while clustering is jointlyconducted based on the regression coefficients obtained from all genes.To account for the correlations among genes and alleviate thehigh dimensionality challenges,factor analysis models are adoptedfor the regression coefficients.The Dirichlet process prior distributionis utilized for the means of the regression coefficients to induce clustering.This package allows users to specify which variables to use for clustering(intercepts or slopes or both)and whether a factor analysis model is desired.More de-tails about this method can be found in Jiehuan Sun,et al.(2017)<doi:10.1002/sim.7374>. License GPL-2Encoding UTF-8LazyData trueDepends R(>=3.4.0),MASS(>=7.3-47),lme4(>=1.1-13),mcclust(>=1.0)Imports Rcpp(>=0.12.7)Suggests knitr,latticeVignetteBuilder knitrLinkingTo Rcpp,RcppArmadilloRoxygenNote7.1.0NeedsCompilation yes12BClustLonGRepository CRANDate/Publication2020-05-0704:10:02UTCR topics documented:BClustLonG (2)calSim (3)data (4)Index5 BClustLonG A Dirichlet process mixture model for clustering longitudinal gene ex-pression data.DescriptionA Dirichlet process mixture model for clustering longitudinal gene expression data.UsageBClustLonG(data=NULL,iter=20000,thin=2,savePara=FALSE,infoVar=c("both","int")[1],factor=TRUE,hyperPara=list(v1=0.1,v2=0.1,v=1.5,c=1,a=0,b=10,cd=1,aa1=2, aa2=1,alpha0=-1,alpha1=-1e-04,cutoff=1e-04,h=100) )Argumentsdata Data list with three elements:Y(gene expression data with each column being one gene),ID,and years.(The names of the elements have to be matachedexactly.See the data in the example section more info)iter Number of iterations(excluding the thinning).thin Number of thinnings.savePara Logical variable indicating if all the parameters needed to be saved.Default value is FALSE,in which case only the membership indicators are saved.infoVar Either"both"(using both intercepts and slopes for clustering)or"int"(using only intercepts for clustering)factor Logical variable indicating whether factor analysis model is wanted.hyperPara A list of hyperparameters with default values.calSim3 Valuereturns a list with following objects.e.mat Membership indicators from all iterations.All other parametersonly returned when savePara=TRUE.ReferencesJiehuan Sun,Jose D.Herazo-Maya,Naftali Kaminski,Hongyu Zhao,and Joshua L.Warren."A Dirichlet process mixture model for clustering longitudinal gene expression data."Statistics in Medicine36,No.22(2017):3495-3506.Examplesdata(data)##increase the number of iterations##to ensure convergence of the algorithmres=BClustLonG(data,iter=20,thin=2,savePara=FALSE,infoVar="both",factor=TRUE)##discard the first10burn-ins in the e.mat##and calculate similarity matrix##the number of burn-ins has be chosen s.t.the algorithm is converged.mat=calSim(t(res$e.mat[,11:20]))clust=maxpear(mat)$cl##the clustering results.##Not run:##if only want to include intercepts for clustering##set infoVar="int"res=BClustLonG(data,iter=10,thin=2,savePara=FALSE,infoVar="int",factor=TRUE)##if no factor analysis model is wanted##set factor=FALSEres=BClustLonG(data,iter=10,thin=2,savePara=FALSE,infoVar="int",factor=TRUE)##End(Not run)calSim Function to calculate the similarity matrix based on the cluster mem-bership indicator of each iteration.DescriptionFunction to calculate the similarity matrix based on the cluster membership indicator of each itera-tion.4dataUsagecalSim(mat)Argumentsmat Matrix of cluster membership indicator from all iterationsExamplesn=90##number of subjectsiters=200##number of iterations##matrix of cluster membership indicators##perfect clustering with three clustersmat=matrix(rep(1:3,each=n/3),nrow=n,ncol=iters)sim=calSim(t(mat))data Simulated dataset for testing the algorithmDescriptionSimulated dataset for testing the algorithmUsagedata(data)FormatAn object of class list of length3.Examplesdata(data)##this is the required data input formathead(data.frame(ID=data$ID,years=data$years,data$Y))Index∗datasetsdata,4BClustLonG,2calSim,3data,45。
医学院英语试题及答案
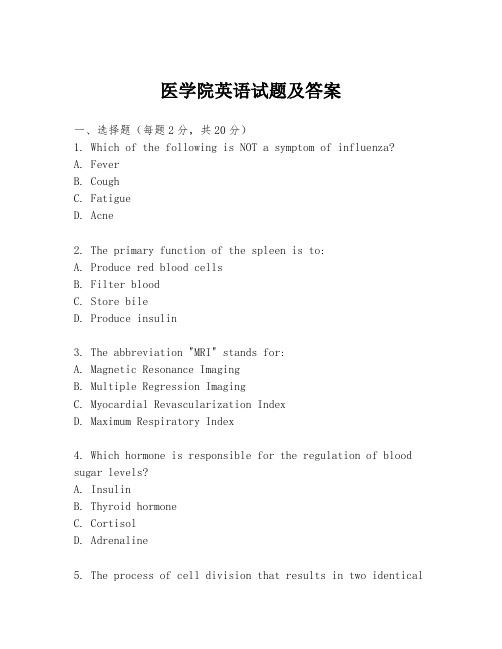
医学院英语试题及答案一、选择题(每题2分,共20分)1. Which of the following is NOT a symptom of influenza?A. FeverB. CoughC. FatigueD. Acne2. The primary function of the spleen is to:A. Produce red blood cellsB. Filter bloodC. Store bileD. Produce insulin3. The abbreviation "MRI" stands for:A. Magnetic Resonance ImagingB. Multiple Regression ImagingC. Myocardial Revascularization IndexD. Maximum Respiratory Index4. Which hormone is responsible for the regulation of blood sugar levels?A. InsulinB. Thyroid hormoneC. CortisolD. Adrenaline5. The process of cell division that results in two identicalcells is called:A. MitosisB. MeiosisC. ApoptosisD. Cytokinesis6. In medical terms, "icterus" refers to:A. JaundiceB. AnemiaC. EdemaD. Hemorrhage7. The "ABCs" of first aid are:A. Airway, Breathing, CirculationB. Ambulance, Bandage, CPRC. Alert, Breathe, CompressionD. Assess, Bleed, Clean8. The study of the structure of the body is called:A. PhysiologyB. AnatomyC. PathologyD. Pharmacology9. Which of the following is a type of cancer?A. MelanomaB. DiabetesC. InfluenzaD. Pneumonia10. The standard unit of measurement for blood pressure is:A. mmHgB. cmH2OC. kPaD. mmol/L二、填空题(每题2分,共20分)1. The largest organ in the human body is the __________.2. The medical term for a broken bone is __________.3. The __________ is the part of the brain responsible for voluntary movement.4. A __________ is a medical professional who specializes in the diagnosis and treatment of diseases of the heart and blood vessels.5. The process by which the body maintains a stable internal environment is called __________.6. The __________ is the largest gland in the human body and is responsible for metabolism.7. The __________ is a type of white blood cell that plays a critical role in the immune response.8. A __________ is a medical condition characterized by a persistently high level of glucose in the blood.9. The __________ is the study of the causes and effects of diseases.10. The __________ is a medical device used to measure blood pressure.三、阅读理解(每题2分,共20分)Read the following passage and answer the questions that follow.The human body is a complex system composed of various organsand systems that work together to maintain life. Thecirculatory system, for example, is responsible for transporting blood, oxygen, and nutrients throughout the body. The respiratory system facilitates the exchange of gases,while the digestive system processes food and absorbs nutrients. Each system plays a crucial role in the overall health and well-being of an individual.1. What is the primary function of the circulatory system?2. Which system is responsible for gas exchange?3. What does the digestive system do?4. How many systems are mentioned in the passage?5. What is the importance of these systems to an individual's health?四、翻译题(每题5分,共20分)1. 请将以下句子翻译成英文:"糖尿病是一种以高血糖为特征的慢性疾病。
New Evidence on Measuring Financial
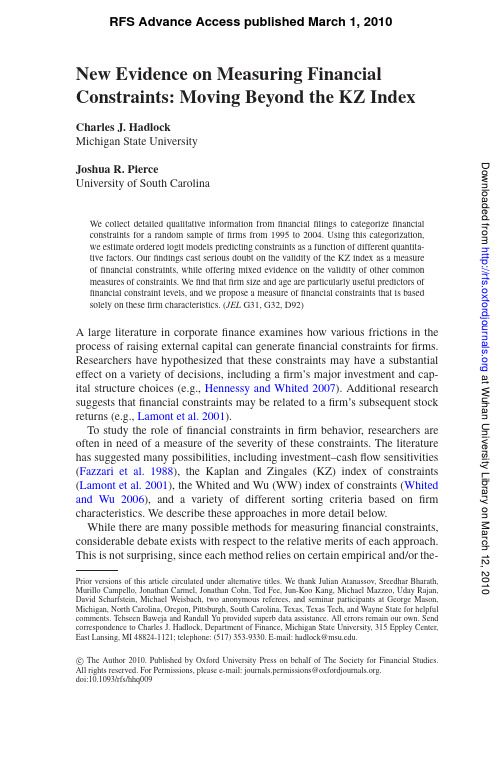
New Evidence on Measuring FinancialConstraints:Moving Beyond the KZ Index Charles J.HadlockMichigan State UniversityJoshua R.PierceUniversity of South CarolinaWe collect detailed qualitative information from financial filings to categorize financial constraints for a random sample of firms from 1995to ing this categorization,we estimate ordered logit models predicting constraints as a function of different quantita-tive factors.Our findings cast serious doubt on the validity of the KZ index as a measure of financial constraints,while offering mixed evidence on the validity of other common measures of constraints.We find that firm size and age are particularly useful predictors of financial constraint levels,and we propose a measure of financial constraints that is based solely on these firm characteristics.(JEL G31,G32,D92)A large literature in corporate finance examines how various frictions in the process of raising external capital can generate financial constraints for firms.Researchers have hypothesized that these constraints may have a substantial effect on a variety of decisions,including a firm’s major investment and cap-ital structure choices (e.g.,Hennessy and Whited 2007).Additional research suggests that financial constraints may be related to a firm’s subsequent stock returns (e.g.,Lamont et al.2001).To study the role of financial constraints in firm behavior,researchers are often in need of a measure of the severity of these constraints.The literature has suggested many possibilities,including investment–cash flow sensitivities (Fazzari et al.1988),the Kaplan and Zingales (KZ)index of constraints (Lamont et al.2001),the Whited and Wu (WW)index of constraints (Whited and Wu 2006),and a variety of different sorting criteria based on firm characteristics.We describe these approaches in more detail below.While there are many possible methods for measuring financial constraints,considerable debate exists with respect to the relative merits of each approach.This is not surprising,since each method relies on certain empirical and/or the-Prior versions of this article circulated under alternative titles.We thank Julian Atanassov,Sreedhar Bharath,Murillo Campello,Jonathan Carmel,Jonathan Cohn,Ted Fee,Jun-Koo Kang,Michael Mazzeo,Uday Rajan,David Scharfstein,Michael Weisbach,two anonymous referees,and seminar participants at George Mason,Michigan,North Carolina,Oregon,Pittsburgh,South Carolina,Texas,Texas Tech,and Wayne State for helpful comments.Tehseen Baweja and Randall Yu provided superb data assistance.All errors remain our own.Send correspondence to Charles J.Hadlock,Department of Finance,Michigan State University,315Eppley Center,East Lansing,MI 48824-1121;telephone:(517)353-9330.E-mail:hadlock@.c The Author 2010.Published by Oxford University Press on behalf of The Society for Financial Studies.All rights reserved.For Permissions,please e-mail:journals.permissions@.doi:10.1093/rfs/hhq009RFS Advance Access published March 1, 2010 at Wuhan University Library on March 12, 2010 Downloaded fromThe Review of Financial Studies/v00n002010oretical assumptions that may or may not be valid.In addition,many of these methods rely on endogenousfinancial choices that may not have a straightfor-ward relation to constraints.For example,while an exogenous increase in cash on hand may help alleviate the constraints that a givenfirm faces,the fact that afirm chooses to hold a high level of cash may be an indication that thefirm is constrained and is holding cash for precautionary reasons.In this article,we studyfinancial constraints by exploiting an approachfirst advocated by Kaplan and Zingales(1997).In particular,we use qualitative in-formation to categorize afirm’sfinancial constraint status by carefully reading statements made by managers in SECfilings for a sample of randomly selected firms from1995to2004.1This direct approach to categorizingfinancial con-straints is not practical for large samples,since it requires extensive hand data collection.However,by studying the relation between constraint categories and variousfirm characteristics,we can make inferences that are useful for thinking about how to measurefinancial constraints in larger samples.We exploit our qualitative data onfinancial constraints for two purposes. First,we critically evaluate methods commonly used in the literature to mea-surefinancial constraints.We pay particular attention to the KZ index,given its relative prominence in the literature and the fact that our data are particularly useful for evaluating this measure.Second,after examining past approaches, we propose a simple new approach for measuring constraints that has substan-tial support in the data and considerable intuitive appeal.We then subject thisnew measure to a variety of robustness checks.To evaluate the KZ index,we estimate ordered logit models in which afirm’s categorized level of constraints is modeled as a function offive Compustat-based variables.This modeling approach parallels the analysis of Lamont et al. (2001),who create the original KZ index by estimating similar models using the original Kaplan and Zingales(1997)sample.The KZ index,which is based on the estimated coefficients from one of the Lamont,Polk,and Saa-Requejo models,loads positively on leverage and Q,and negatively on cashflow,cash levels,and dividends.In the ordered logit models we estimate,only two of thefive components of the KZ index,cashflow and leverage,are consistently significant with a sign that agrees with the KZ index.For two of the otherfive components, Q and dividends,the coefficientsflip signs across estimated models and in many cases are insignificant,particularly for the dividend variable.Finally,in contrast to its negative loading in the KZ index,wefind that cash holdings generally display a positive and significant coefficient in models predicting constraints.This positive relation is consistent with constrainedfirms holding cash for precautionary reasons.1The information we use includes statements regarding the strength of afirm’s liquidity position and thefirm’s ability to raise any needed external funds.Additional details are provided below.2 at Wuhan University Library on March 12, 2010 Downloaded fromNew Evidence on Measuring Financial ConstraintsOur estimates differ substantially from the KZ index coefficients even though we use a parallel modeling approach.Upon further investigation,we find that the differences most likely arise from the fact that the dependent variable in the original modeling underlying the KZ index includes quantita-tive information in addition to qualitative information.This treatment adds a hard-wired element to the estimates underlying the KZ index,since the same information is mechanically built into both the dependent and the independent variables.In our treatment,we are careful to avoid this problem.Once this problem is addressed,ourfindings indicate that many of the estimated coefficients change substantially.Clearly our evidence raises serious questions about the use of the KZ index. To explore this issue further,we calculate the KZ index for the entire Com-pustat universe and compare this to an index constructed using the coefficient estimates from one of our models.Wefind that the correlation between the tra-ditional index and our alternative version of this index is approximately zero. This provides compelling evidence that the KZ index is unlikely to be a useful measure offinancial constraints.Thus,it would appear that researchers should apply extreme caution when using the traditional KZ index or interpreting re-sults based on index sorts.An alternative index offinancial constraints has been proposed by Whited and Wu(2006),who exploit a Euler equation approach from a structural model of investment to create the WW index.This index loads on six different factorscreated from Compustat data.When we use these six factors as explanatory variables in ordered logit models predicting constraints,only three of the six variables have significant coefficients that agree in sign with the WW index. Two of these variables,cashflow and leverage,are essentially the same vari-ables thatfigure prominently in the KZ index.Thus,the only truly new variable from the WW index that offers marginal explanatory power in our models is firm size.As one would expect,smallerfirms are more likely to be constrained.A more traditional approach to identifyingfinancially constrainedfirms is to sort by afirm characteristic that is believed to be associated with constraints. To evaluate this approach,we study the relation between several common sort-ing variables and ourfinancial constraint categories.Wefind that some of these sorting variables are not significantly related to constraint categories.Two vari-ables that do appear to be closely related tofinancial constraints arefirm size and age.An appealing feature of these variables is that they are much less en-dogenous than most other sorting variables.Once we control forfirm size and age,some of the variables that are significantly related to constraints in a uni-variate sense become insignificant.Thus,it appears that some common sorting variables are largely proxies forfirm size and/or age.The only variables that consistently predict afirm’s constraint status in our sample after controlling for size and age are afirm’s leverage and cash flow.However,given the endogenous nature of these variables,particularly the leverage variable,we are hesitant to recommend any measure of constraints3 at Wuhan University Library on March 12, 2010 Downloaded fromThe Review of Financial Studies/v00n002010that is derived from a model that relies on these factors.In addition,as we explain below,typical disclosure practices may lead us to under-detect the presence of constraints infirms with low leverage,thus possibly leading to a spurious coefficient on leverage.Given these concerns,we recommend that researchers rely solely onfirm size and age,two relatively exogenousfirm characteristics,to identify constrainedfirms.To provide further guidance on the role of size and age infinancial con-straints,we examine the relation between these factors and constraints for sub-samples grouped byfirm characteristics and time period.While there is minor variation across groups,the general form of the relation between size,age,and financial constraint categories appears to be robust.Wefind that the role of both size and age in predicting constraints is nonlinear.At certain points,roughly the sample ninety-fifth percentiles($4.5billion in assets,thirty-seven years in age),the relation between constraints and thesefirm characteristics is essen-tiallyflat.Below these cutoffs,we uncover a quadratic relation between size and constraints and a linear relation between age and constraints.We represent this relation in what we call the size–age or SA index.2This index indicates thatfinancial constraints fall sharply as young and smallfirms start to mature and grow.Eventually,these relations appear to level off.Since all measures offinancial constraints have potential shortcomings,we attempt to provide corroboratory evidence regarding our proposed index.In particular,we exploit the cashflow sensitivity of cash approach advanced byAlmeida et al.(2004).When we sortfirms into constrained and unconstrained groups using the SA index,wefind that the constrainedfirms display a sig-nificant sensitivity of cash to cashflow,whereas the unconstrainedfirms do not.This evidence increases our confidence in the SA index as a reasonable measure of constraints.While we cannot prove that our index is the optimal measure of constraints, it has many advantages over other approaches,including its intuitive appeal, its independence from various theoretical assumptions,and the presence of corroborating evidence from an alternative approach.The correlation between the SA index and the KZ index is negligible,casting additional doubt on the usefulness of the KZ index.The correlation between the SA index and the WW index is much higher,but this largely reflects the fact that the WW index includesfirm size as one of its six components.For completeness,we use our data to revisit the Kaplan and Zingales (1997)assertion that investment–cashflow sensitivities are dubious measures2This index is derived from coefficients in one of our ordered logit models presented below.The index is cal-culated as(−0.737*Size)+(0.043*Size2)−(0.040*Age),where Size equals the log of inflation-adjustedbook assets,and Age is the number of years thefirm is listed with a non-missing stock price on Compustat. In calculating this index,Size is winsorized(i.e.,capped)at(the log of)$4.5billion,and Age is winsorized at thirty-seven years.4 at Wuhan University Library on March 12, 2010 Downloaded fromNew Evidence on Measuring Financial Constraintsoffinancial constraints.3Ourfindings here are consistent with what Kaplan and Zingales(1997)report.In particular,using both our direct qualitative categorization of constraints and the SA index,wefind that investment–cash flow sensitivities are not monotonically increasing in afirm’s level offinancial constraints.The rest of the article is organized as follows.In Section1,we detail our sample selection procedure and our assignment offirms intofinancial constraint groups using qualitative information.In Section2,we use our data to critically evaluate past approaches for measuringfinancial constraints.In Section3,we further explore the relation betweenfinancial constraints and the size and age of afirm and propose a simple index based on thesefirm characteristics.In Section4,we revisit the prior evidence on investment–cash flow sensitivities.Section5concludes.1.Sample Construction and Categorization of Financial Constraints1.1Sample selection and data collectionOur goal is to study a large and representative sample of modern public firms.We begin with the set of all Compustatfirms in existence at some point between1995and2004.From this universe,we eliminate allfinancial firms(SIC Codes6000–6999),regulated utilities(SIC Codes4900–4949), andfirms incorporated outside the United States.We then sortfirms byCompustat identifier and select every twenty-fourthfirm for further analysis. This procedure results in a random sample of407firms that should be broadly representative of the overall Compustat universe.After selecting the initial sample,we locate eachfirm’s annual reports and 10-Kfilings from Lexis-Nexis and SEC EDGAR.We restrict the sample to firm years for which we can locate at least one of these electronicfilings.In addition,we impose the requirement that thefirm has nonzero sales and assets in the observation year and sufficient accounting data to calculate all of the components of the KZ index.The resulting sample consists of356uniquefirms and1,848firm years during the1995–2004period.4To collect qualitative information onfinancial constraints,we carefully read annual reports and10-Kfilings following the general procedure outlined by Kaplan and Zingales(1997).In particular,for eachfirm year,we read the annual letter to shareholders and the management discussion and analysis section.In addition,we perform an electronic search of the entire text of the annual report and/or10-K to identify all sections of text that include 3For critiques of the investment–cashflow approach,see Cleary(1999),Kaplan and Zingales(1997),Erickson and Whited(2000),Alti(2003),and Moyen(2004).For a defense,see Fazzari et al.(2000).4While we borrow heavily from Kaplan and Zingales(2000),the sample we study is quite different from theirs. They study a small sample(forty-ninefirms)from the1970s and1980s that satisfies a variety of sampling requirements pertaining to industry,size,growth,dividend policy,and survival.5 at Wuhan University Library on March 12, 2010 Downloaded fromThe Review of Financial Studies/v00n002010the following keywords:financing,finance,investing,invest,capital,liquid, liquidity,note,covenant,amend,waive,violate,and credit.Using these procedures,we extract every statement that pertains to a firm’s ability to raise funds orfinance its current or future operations.5In manyfilings,we identify multiple statements.We assign to each individual statement an integer code from1to5,with higher(lower)numbers being more indicative of the presence(lack)of constraints.These codes are based on the description provided by KZ regarding their categorization scheme. Later,we aggregate these codes to derive a single overall categorization of a firm’sfinancial constraint status in any given year.It is important to note that there are literally hundreds of different types of relevant statements made by samplefirms.Grouping such a large number of statements intofive categories necessarily requires some judgment.Specific examples of how we code different types of statements are reported in the Appendix.Following the spirit of the KZ algorithm,we assign to category1all state-ments that indicate that afirm has excessive or more than sufficient liquidity to fund all of its capital needs.In category2,we place all statements that in-dicate that afirm has adequate or sufficient liquidity to fund its needs.The main difference between category1and category2is the strength of thefirm’s language.In category3,we place all statements that provide some qualifica-tion regarding thefirm’s ability to fund future needs,but that do not indicate any type of current problem.Most of these statements are soft warnings,oftengeneric or boilerplate in character,indicating that under some possible future scenario thefirm could have difficulty raising funds orfinancing desired in-vestments.Category3also includes all statements that are opaque and thus not easy to classify into the other groups.We place all statements that indicate some current liquidity problem into category4,but with no direct indication that these problems have led to a substantive change in thefirm’s investment policy or to overtfinancial stress. This would include difficulties in obtainingfinancing or the postponing of a security issue.Finally,category5includes all cases of clearfinancial prob-lems/constraints including a current and substantive covenant violation,a rev-elation that investment has been affected by liquidity problems,going concern statements,or involuntary losses of usual sources of credit.65We were assisted by two trained accountants in our search and categorization efforts.Allfilings were searched independently by at least two individuals to minimize the probability of missing any relevant disclosure.6Somefirms indicate that a covenant had been waived or amended.Often thesefirms indicate that the violation was technical in nature and not of substantive concern.For example,somefirms indicate that a covenant was routinely waived,and others indicate that an accounting ratio fell below a threshold because of a one-time event such as an asset sale or special charge.Since these cases are quite different from and less serious than current violations,in our baseline coding,we ignore waived/amended covenants.Alternative treatments of these cases are discussed below.6 at Wuhan University Library on March 12, 2010 Downloaded fromNew Evidence on Measuring Financial Constraints1.2Categorization of afirm’s overallfinancial constraint statusWe proceed to assign eachfirm year to a singlefinancial constraint group. Borrowing from the KZ algorithm and terminology,we createfive mutually exclusive groups:notfinancially constrained(NFC),likely notfinancially con-strained(LNFC),potentiallyfinancially constrained(PFC),likelyfinancially constrained(LFC),andfinancially constrained(FC).We place in the NFC groupfirms with at least one statement coded as a1and no statement coded below a2.These arefirms that indicate more than sufficient liquidity and re-veal no evidence to the contrary.In the LNFC category,we place allfirms with statements solely coded as2s.These arefirms that indicate adequate or sufficient liquidity with no statements of excessive liquidity and no statements indicating any weakness.7We place allfirms with mixed information on their constraint status into the PFC category.Specifically,we include all observations in which thefirm re-veals a statement coded as2or better(indicatingfinancial strength),but also reveals a statement coded as3or worse(indicating possiblefinancial weak-ness).We also include in this category cases in which all of thefirm’s state-ments are coded as3.The LFC category includesfirms with at least one statement coded as4,no statement coded as5,and no statement coded better than3.These arefirms that indicate some current liquidity problems,with no offsetting positive statement and no statement that is so severe that they are brought into the lowest(FC)category.Finally,all observations with at least one statement coded as5and no other statement coded better than3are assigned to the FC category.These are firms that clearly indicate the presence of constraints with no strong offsetting positive revelation.We refer to this initial categorization scheme as qualitative scheme1and report a sample breakdown in Column1of table1.For comparison purposes, we report in Column4the correspondingfigures reported by KZ.One peculiar feature of qualitative scheme1is that a large number offirms are placed in the PFC category(32.36%versus7.30%in the KZ sample).This elevated rate primarily reflects the fact that manyfirms in our sample provide boilerplate generic warnings about future uncertainties that could affect afirm’s liquidity position.These statements place manyfirms that otherwise report strongfi-nancial health into the PFC category.In our estimation,many of these generic warning statements are uninformative.In particular,they appear to be included as a blanket protection against future legal liability and often pertain to unfore-seen or unlikely contingencies that could potentially affect almost anyfirm.In light of these observations,we prefer an alternative assignment procedure that ignores all generic or soft nonspecific warnings regarding afirm’s future liquidity position.This procedure,which we refer to as qualitative scheme2,7We also place in this group the few observations with no useful qualitative disclosure that could be used to ascertain afirm’sfinancial constraint status.If we exclude these observations,the ordered logit results we report below in tables3,4,and6are substantively unchanged.7 at Wuhan University Library on March 12, 2010 Downloaded fromThe Review of Financial Studies/v00n002010Table1Frequency of Financial Constraint CategoriesConstraint assignment procedureQualitative Qualitative Qual./quant.KZ samplescheme1scheme2schemeNotfinancially const:NFC10.28%10.98%55.84%54.50% Likely notfinancially const.:LNFC50.49%71.59%31.01%30.90% Potentiallyfinancially const.:PFC32.36%10.55% 6.44%7.30% Likelyfinancially const.:LFC0.32%0.32% 2.49% 4.80% Financially const.:FC 6.55% 6.55% 4.22% 2.60% Correlation with qual.scheme1 1.00Correlation with qual.scheme20.89 1.00Correlation with qual./quant.scheme0.750.87 1.00This table reports the fraction of allfirm-year observations in which an observation is assigned to the indicated financial constraint group.Thefigures in Columns1–3pertain to our random sample of1,848Compustatfirm years representing356firms operating during the1995–2004period for observations with non-missing data on thefive components of the KZ index.Qualitative scheme1uses only qualitative statements made byfirms in their filings subsequent to thefiscal year-end regarding thefirm’s liquidity position and ability to fund investments. The exact algorithm used in coding and categorizing this information is detailed in the text.Qualitative scheme 2is constructed identically to scheme1except that it ignores all soft and generic nonspecific warnings made byfirms regarding possible future scenarios under which thefirm could experience a liquidity problem.The qualitative/quantitative scheme augments scheme2by movingfirms upward one category if thefirm materially increases dividends,repurchases shares,or has a high(top quartile)level of(cash/capital expenditures)on hand. Additional details concerning the assignment procedures are provided in the text and the Appendix.Thefigures in Column4are taken from table2of Kaplan and Zingales(1997)and are based on their sample and algorithm for categorizing constraints.The correlationfigures represent simple correlations over the sample between the two constraint assignment procedures in the indicated cell.is identical to qualitative scheme1outlined earlier except that it ignores this one class of statements.As we report in Column2of table1,this modification moves many(a few)firms from the PFC grouping up into the LNFC(NFC) grouping.It is important to emphasize that the categorization schemes outlined above deliberately differ from the KZ procedure in one key respect.In particular, in our categorization,we choose to ignore quantitative information on both the size of afirm’s cash position and its recent dividend/repurchase behavior. We do so this because it seems inappropriate to incorporate this information into categories that will eventually be used for coding our dependent variables, given that this same information will later be used to construct some of the in-dependent variables.Such treatment would lead to uninformative coefficients that are hardwired and potentially misleading in terms of their ability to de-scribe the underlying relation between quantitative variables and qualitative disclosures of constraints.For completeness,we experiment with modifying our qualitative scheme2 categorization to more closely match the exact KZ treatment by incorporating quantitative information on dividends,repurchases,and cash balances.In par-ticular,we move afirm’s constraint status up one notch in a given year(e.g., from PFC to LNFC)if any of the following criteria are met:(i)thefirm ini-tiates a dividend;(ii)thefirm has a material increase in dividends(change in dividends/assets greater than thefifth percentile of dividend increasers);(iii) 8 at Wuhan University Library on March 12, 2010 Downloaded fromNew Evidence on Measuring Financial ConstraintsTable2Sample Characteristics(1)(2)(3)(4)(5)(6) Statistic Mean Mean Mean Median Median Median Cashflow/K−2.379−0.915−9.3150.2430.327−0.907 Cash/K 3.689 3.579 4.2080.4390.5080.199 Dividends/K0.0770.0640.1390.0000.0000.000 Tobin’s Q 2.672 2.036 5.686 1.535 1.489 1.809 Debt/total capital0.3380.2770.6290.2750.2240.728 Capital exp./K0.4110.4150.3920.2140.2290.133 Prop.,plant,278.370303.457159.48020.66429.594 2.951 equip.(PPE)Book assets782.928872.877356.647124.627167.08914.800 Age13.92314.71610.1659.0009.0007.000 Sales growth0.2720.2470.3940.0570.070−0.049#of qualitative 3.37 3.32 3.62 3.00 3.00 3.00 statementsWhich observations All Less More All Less Moreconstrained constrained constrained constrained Thefigures in each column represent the mean or median of the indicated variable over the indicated set of observations.Thefigures in Columns1and4refer to our random sample of1,848Compustatfirm years repre-senting356firms operating during the1995–2004period.Thefigures in Columns2and5are calculated over the subset of observations in which thefirm was classified as less constrained(NFC/LNFC)using qualitative scheme2to categorize constraints.Thefigures in Columns3and6are calculated over the subset of observa-tions in which thefirm was classified as more constrained(PFC/LFC/FC).All variables are constructed from Compustat information.The PPE and book assets statistics are in millions of inflation adjusted year2004dol-lars.All variables that are normalized by K are divided by beginning-of-period PPE.Cashflow is defined to be operating income plus depreciation(Compustat item18+item14).Cash is defined to be cash plus marketable securities(item1).Dividends are total annual dividend payments(item21+item19).Tobin’s Q is defined as (book assets minus book common equity minus deferred taxes plus market equity)/book assets calculated as [item6−item60−item74+(item25×item24)]/item6.Debt is defined as short-term plus long-term debt(item9+item34).Total capital is defined as debt plus total stockholders’equity(item9+item34+item216). If stockholders’equity is negative,we set debt/total capital equal to1.Capital expenditures are item128.Age is defined to be the number of years preceding the observation year that thefirm has a non-missing stock price on the Compustatfile.Sales growth is defined as(sales in year t minus sales in year t−1)/sales in year t−1. Sales arefirst inflation adjusted before making this growth calculation.The number of statements row refers to the number of qualitative statements from disclosurefilings that were used in assigning thefirm to a constraint grouping using qualitative scheme2,as outlined in the text.thefirm repurchases a material number of shares(repurchases/assets greater than thefifth percentile of repurchasers);or(iv)thefirm’s balance of cash and marketable securities normalized by capital expenditures falls in the top sample quartile.The resulting categorization is referred to in what follows as the qualitative/quantitative categorization scheme.We report in Column3of table1the percentage offirms in each of the constraint categories using this alternative scheme.As thefigures illustrate,the sample frequencies using the qualitative/quantitative scheme more closely resemble thefigures reported by KZ,with the modal category beingfirms in the most unconstrained(NFC) category.In table2,we present summary statistics for the sample as a whole and for subsamples grouped by the level of constraints using our preferred constraint assignment procedure,qualitative scheme2.Several interesting differences be-tween the more constrained and less constrainedfirms emerge.In particular, comparing both the reported means and medians for the subsamples grouped9 at Wuhan University Library on March 12, 2010 Downloaded from。
Engineering Viscoelasticity(工程粘弹性)
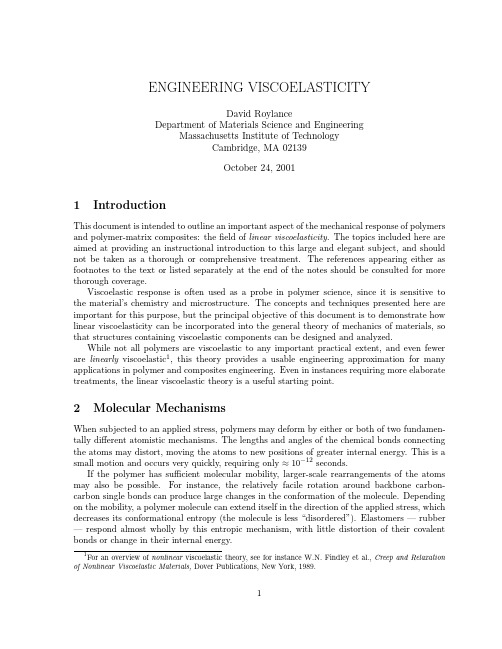
Figure 1: Temperature dependence of rate. Conversely, at temperatures much less than Tg , the rates are so slow as to be negligible. Here the chain uncoiling process is essentially “frozen out,” so the polymer is able to respond only by bond stretching. It now responds in a “glassy” manner, responding instantaneously 2
where E † is an apparent activation energy of the process and R = 8.314J/mol − ◦ K is the Gas Constant. At temperatures much above the “glass transition temperature,” labeled Tg in Fig. 1, the rates are so fast as to be essentially instantaneous, and the polymer acts in a rubbery manner in which it exhibits large, instantaneous, and fully reversible strains in response to an applied stress.
1
Introduction
This document is intended to outline an important aspect of the mechanical response of polymers and polymer-matrix composites: the field of linear viscoelasticity. The topics included here are aimed at providing an instructional introduction to this large and elegant subject, and should not be taken as a thorough or comprehensive treatment. The references appearing either as footnotes to the text or listed separately at the end of the notes should be consulted for more thorough coverage. Viscoelastic response is often used as a probe in polymer science, since it is sensitive to the material’s. The concepts and techniques presented here are important for this purpose, but the principal objective of this document is to demonstrate how linear viscoelasticity can be incorporated into the general theory of mechanics of materials, so that structures containing viscoelastic components can be designed and analyzed. While not all polymers are viscoelastic to any important practical extent, and even fewer are linearly viscoelastic1 , this theory provides a usable engineering approximation for many applications in polymer and composites engineering. Even in instances requiring more elaborate treatments, the linear viscoelastic theory is a useful starting point.
confounding variable
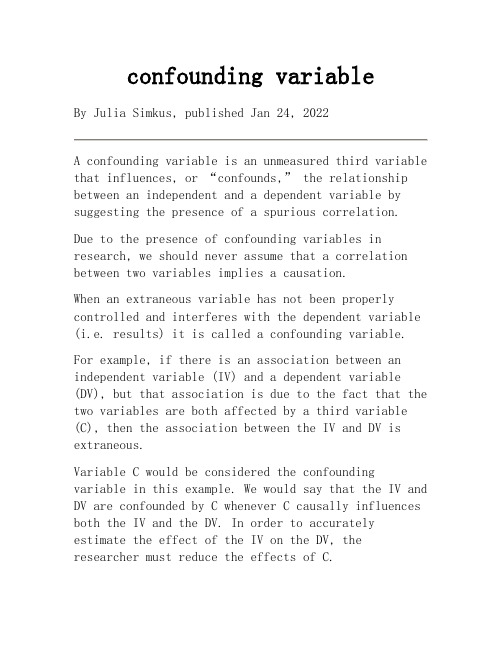
confounding variableBy Julia Simkus, published Jan 24, 2022A confounding variable is an unmeasured third variable that influences, or “confounds,” the relationship between an independent and a dependent variable by suggesting the presence of a spurious correlation.Due to the presence of confounding variables in research, we should never assume that a correlation between two variables implies a causation.When an extraneous variable has not been properly controlled and interferes with the dependent variable (i.e. results) it is called a confounding variable.For example, if there is an association between an independent variable (IV) and a dependent variable (DV), but that association is due to the fact that the two variables are both affected by a third variable (C), then the association between the IV and DV is extraneous.Variable C would be considered the confoundingvariable in this example. We would say that the IV and DV are confounded by C whenever C causally influences both the IV and the DV. In order to accurately estimate the effect of the IV on the DV, the researcher must reduce the effects of C.If you identify a causal relationship between the independent variable and the dependent variable, that relationship might not actually exist because it could be affected by the presence of a confounding variable.Even if the cause and effect relationship does exist, the confounding variable still might overestimate or underestimate the impact of the independent variable on the dependent variable.How to reduce the impact of confounding variablesIt is important to identify all possible confounding variables and consider the impact of them in your research design in order to ensure the internalvalidity of your results.Here are some techniques to reduce the effects of these confounding variables:1.Random allocation: randomization will helpeliminate the impact of confounding variables.You can randomly assign half of your subjects toa treatment group and the other half to a controlgroup.This will ensure that confounders will have thesame effect on both groups, so they cannotcorrelate with your independent variable.2.Control variables: This involves restricting thetreatment group to only include subjects with the same potential for confounding factors.For example, you can restrict your subject poolby age, sex, demographic, level of education, orweight (etc.) to ensure that these variables arethe same among all subjects and thus cannotconfound the cause and effect relationship athand.3.Within-subjects design: In a within-subjectsdesign, all participants take part in everycondition.4.Case-control studies: Case control studies assignconfounders to both groups (the experimentalgroup and the control group) equally.Suppose we wanted to measure the effects of caloric intake (IV) on weight (DV). We would have to try to ensure that confounding variables did not affect the results. These variables could include:•Metabolic rate: If you have a faster metabolism,you tend to burn calories quicker.•Age: Age can have a different effect on weightgain as younger individuals tend to burn calories quicker than older individuals.•Physical Activity: Those who exercise or are more active will burn more calories and could weighless, even if they consume more.•Height: Taller individuals tend to need toconsume more calories in order to gain weight.•Sex: Men and women have different caloric needsto maintain a certain weight.Frequently asked questions about confounding variables1. What is the difference between an extraneous variable and a confounding variable?A confounding variable is a type of extraneous variable. Confounding variables affect both the independent and dependent variables. They influence the dependent variable directly and either correlate with or causally affect the independent variable.An extraneous variable is any variable that you are not investigating that can influence the dependent variable.2. What is Confounding Bias?Confounding bias is bias that is the result of having confounding variables in your study design. If the observed association overestimates the effect of the independent variable on the dependent variable, thisis known as positive confounding bias.If the observed association underestimates the effect of the independent variable on the dependent variable, this is known as negative confounding bias.About the AuthorJulia Simkus is an undergraduate student at Princeton University, majoring in Psychology. She plans to pursue a PhD in Clinical Psychology upon graduation from Princeton in 2023. Julia has co-authored two journal articles, one titled “Substance Use Disorde rsand Behavioral Addictions During the COVID-19 Pandemic and COVID-19-Related Restrictions," which waspublished in Frontiers in Psychiatry in April 2021 and the other titled “Food Addiction: Latest Insights on the Clinical Implications," to be published in Handbook of Substance Misuse and Addictions: From Biology to Public Health in early 2022.How to reference this article:How to reference this article:Simkus, J. (2022, Jan 24). What is a Confounding Variable? Simply Psychology.SourcesGlen, Stephanie. Confounding Variable: SimpleDefinition and Example. Retrieved from StatisticsHowTo: Elementary Statistics for the rest of us!Thomas, L. (2021). Understanding confounding variables. Scribbr. Retrieved fromUniversity of Michigan. (n.d.). Confounding Variables. ICPSR. Retrieved fromHome|About Us|Privacy Policy|Advertise|Contact UsSimply Psychology's content is for informational and educational purposes only. Our website is not intended to be a substitute for professional medical advice,diagnosis, or treatment.© Simply Scholar Ltd - All rights reserved report this ad。
Intraclass correlation
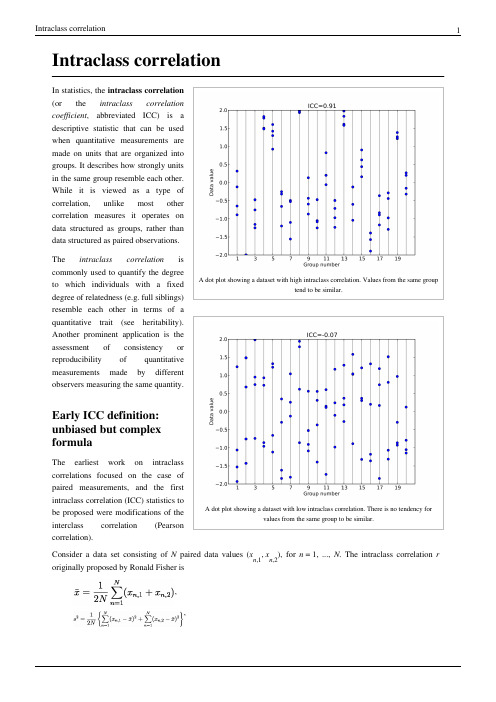
Intraclass correlation
3
Modern ICC definitions: simpler formula but positive bias
Beginning with Ronald Fisher, the intraclass correlation has been regarded within the framework of analysis of variance (ANOVA), and more recently in the framework of random effects models. A number of ICC estimators have been proposed. Most of the estimators can be defined in terms of the random effects model
assumed to have expected value zero and to be
identically distributed, and the variance of εij is denoted σε2.
εij
are
assumed
to
be
uncorrelated with each other. Also, identically distributed. The variance
, ,
Intraclass correlation
2
.
Later versions of this statistic used the proper degrees of freedom 2N −1 in the denominator for calculating s2 and N −1 in the denominator for calculating r, so that s2 becomes unbiased, and r becomes unbiased if s is known. The key difference between this ICC and the interclass (Pearson) correlation is that the data are pooled to estimate the mean and variance. The reason for this is that in the setting where an intraclass correlation is desired, the pairs are considered to be unordered. For example, if we are studying the resemblance of twins, there is usually no meaningful way to order the values for the two individuals within a twin pair. Like the interclass correlation, the intraclass correlation for paired data will be confined to the interval [-1, +1]. The intraclass correlation is also defined for data sets with groups having more than two values. For groups consisting of 3 values, it is defined as
GWAS分析详解
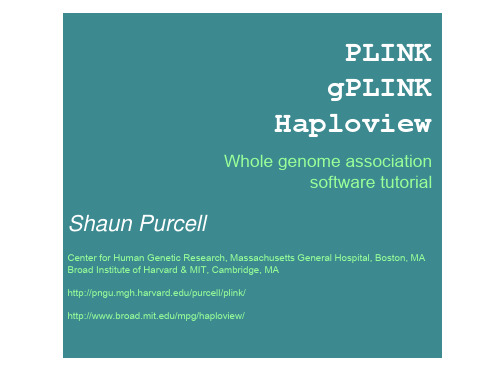
example.fam pop.phe qt.phe
PLINK tutorial, December 2006; Shaun Purcell, shaun@
The Truth…
Chinese Japanese Case Control 34 11 7 38 Case Control
gPLINK / PLINK in “remote mode”
Secure Shell networking
Server, or cluster head node
gPLINK & Haploview: initiating and viewing jobs
WWW
PLINK, WGAS data & computation
Load and filter binary PED file
Basic association analysis
~11 minutes
~5 minutes
PLINK tutorial, December 2006; Shaun Purcell, shaun@
/purcell/plink/ /mpg/haploview/
PLINK tutorial, December 2006; Shaun Purcell, shaun@
PLINK tutorial, December 2006; Shaun Purcell, shaun@
A simulated WGAS dataset Summary statistics and quality control Whole genome SNP-based association Whole genome haplotype-based association Assessment of population stratification
- 1、下载文档前请自行甄别文档内容的完整性,平台不提供额外的编辑、内容补充、找答案等附加服务。
- 2、"仅部分预览"的文档,不可在线预览部分如存在完整性等问题,可反馈申请退款(可完整预览的文档不适用该条件!)。
- 3、如文档侵犯您的权益,请联系客服反馈,我们会尽快为您处理(人工客服工作时间:9:00-18:30)。
Using the Bethe–Salpeter technique Molockhov derived the following expression of the nucleon structure function in nucleus: F2A (x, Q2 ) = xA |VAN (PA , p)|2 id4 p F2 (xN , Q2 ) , 2 (2π )4 xN (p2 − m2 )2 (PA − p)2 − MA −1 xN = mx . (3) p0 − βp3
is the scaling variable of target nucleus, MA is its mass and xN is the where xA = Amx MA in-medium scaling variable of nucleon. The immediate question is as follows: is the inmedium structure function F2m the same as that of free nucleon? Note that in Eq. (1) we used the in-medium scaling variable xN which is different from x. The usual hope was that choosing an appropriate definition of xN one may absorb the in-medium dependence of the function F2m and describe the data using the free-nucleon structure function, i.e. putting F2m (x, Q2 , p, ε) = F2 (xN , Q2 ). The analysis of the available data showed that this is not the case [1]. But as discussed in [2] all the previous calculations are based on seemingly evident but erroneous assumption that the quantity S (p, ε) is the ground-state spectral function of the target nucleus. Actually it is the spectral function of the doorway states for one-nucleon transfer reactions. Indeed, the nucleon hole (which is just the relevant doorway state) is formed in the ground state of target nucleus when the struck nucleon is destroyed by DIS. This state is not the eigenstate of nuclear Hamiltonian thus being fragmented over the actual states of residual nucleus because of the correlations between nucleons. The observed spreading width of the hole states is 20 MeV [3] the fragmentation time thus being 3 · 10−23sec. .7 But the interaction times of DIS is 2q/Q2 = (mx)−1 = 0x · 10−24 sec thus being less than 3 · 10−24 sec for x > 0.3. So the DIS interaction time in the one-nucleon region is an order of magnitude less than that of the fragmentation and therefore the correlation processes do not have time to come into play. As a consequence the quantity S (p, ε) entering (1) is the spectral function of the doorway states. As discussed in [4] it can be unambiguously calculated in a model-independent way in contrast to the ground-state spectral function. So the theory of doorway states provides a natural way for testing the models of nucleon structure functions in nuclei. In [2] we performed the EMC calculations assuming the nucleon structure function in the doorway state λ to be the same as that of free nucleon however dependent upon the in-medium scaling variable in this state: F2m (x, Q , p, ε2 ) = F2 (xN , Q ),
Prescriptions for the scaling variable of the nucleon structure function in nuclei
arXiv:nucl-th/0610102v1 26 Oct 2006
V. I. Ryazanov, B. L. Birbrair and M. G. Ryskin Petersburg Nuclear Physics Institute Gatchina, St. Petersburg 188300, Russia
The vertex VAN (PA , p) describes the wave function of nucleon in nucleus, see Eq. (6). The meaning of other entering quantities is clear from Fig. 1, where Eq. (3) is graphically represented. In a more detailed form F2A (x, Q2 ) = xA × id3 pdp0 (2π )4 (3a)
2 2
mx xN = , m + ελ − βp3
|q | 4m2 x2 β= = 1+ q0 Q2
1/2
, (2)
where ελ < 0 is the nucleon binding energy in the state λ and the axis 3 is chosen along the momentum of virtual photon. The results do not agree with all the available EMC data thus indicating that F2m is different from F2 . In the present work we are testing two different choices of the in-medium scaling variable, the first belonging to Molochkov [5] and the second to Pandharipande and coworkers [6].
Abstract We tested several choices of the in-medium value of the Bjorken scaling variable assuming the nucleon structure function in nucleus to be the same as that of free nucleon. The results unambiguously show that it is different.
1
Introduction
As well known, the deep inelastic scattering (hereafter DIS) of leptons on nucleons begins by the formation of parton with the size (Compton wave length in the rest frame) (mx)−1 = 0.21 fm, x = Q2 /(2mq0 ), x and q0 are the Bjorken scaling variable and the energy of virtual x photon in the rest frame of nucleon. Accordingly three interaction regions are inherent for the DIS on nuclei: I. Correlation region, 0 < x < 0.2. In this region the size of parton exceeds the distance between nucleons r0 = 1.2 fm, and therefore two or even several nucleons take part in the process. For this reason the correlations between nucleons, both short-range and long-range ones, are of importance. II. One-nucleon region, 0.2 < x < 0.8. In this region (mx)−1 < r0 , and therefore the virtual γ -quantum is absorbed by one nucleon only. III. Competition region, 0.8 < x ∼ 1. In this region for a not very large Q2 the competition occurs between DIS, elastic lepton–nucleon scattering and the possible formation of heavier baryons through the reaction ℓN → ℓ′ B , B being ∆33 , N ∗ etc. In the one-nucleon region we are dealing with the in-medium nucleon structure function; F2m (x, Q2 , p, ε) depending upon the momentum p and binding energy ε of nucleon in nucleus in addition to x and Q2 must be averaged over the energy-momentum distribution S (p, ε) of nucleon, i.e. F2A (x, Q2 ) = xA d3 pdεS (p, ε) 1 F2m (x, Q2 , p, ε) , xN (1)