商务与经济统计方法-全球数据集各章习题中文答案
商务与经济统计习题答案(第8版,中文版)SBE9
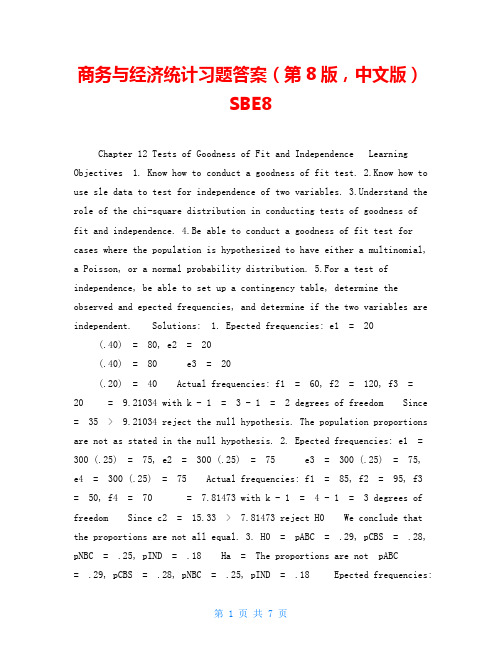
商务与经济统计习题答案(第8版,中文版)SBE8Chapter 12 Tests of Goodness of Fit and Independence Learning Objectives 1. Know how to conduct a goodness of fit test. 2.Know how to use sle data to test for independence of two variables. 3.Understand the role of the chi-square distribution in conducting tests of goodness offit and independence. 4.Be able to conduct a goodness of fit test for cases where the population is hypothesized to have either a multinomial, a Poisson, or a normal probability distribution. 5.For a test of independence, be able to set up a contingency table, determine the observed and epected frequencies, and determine if the two variables are independent. Solutions: 1. Epected frequencies: e1 = 20 (.40) = 80, e2 = 20(.40) = 80 e3 = 20(.20) = 40 Actual frequencies: f1 = 60, f2 = 120, f3 =20 = 9.21034 with k - 1 = 3 - 1 = 2 degrees of freedom Since = 35 > 9.21034 reject the null hypothesis. The population proportions are not as stated in the null hypothesis. 2. Epected frequencies: e1 = 300 (.25) = 75, e2 = 300 (.25) = 75 e3 = 300 (.25) = 75, e4 = 300 (.25) = 75 Actual frequencies: f1 = 85, f2 = 95, f3 = 50, f4 = 70 = 7.81473 with k - 1 = 4 - 1 = 3 degrees of freedom Since c2 = 15.33 > 7.81473 reject H0 We conclude that the proportions are not all equal. 3. H0 = pABC = .29, pCBS = .28, pNBC = .25, pIND = .18 Ha = The proportions are not pABC= .29, pCBS = .28, pNBC = .25, pIND = .18 Epected frequencies:300 (.29) = 87, 300 (.28) = 84 300 (.25) = 75, 300 (.18) =54 e1 = 87, e2 = 84, e3 = 75, e4 = 54 Actual frequencies: f1 = 95, f2 = 70, f3 = 89, f4 = 46 = 7.81 (3 degrees of freedom) Do not reject H0; there is no signifant change in the vieg audience proportions. 4. Observed Epected Hypothesized Frequency Frequency Category Proportion (fi) (ei) (fi - ei)2 / ei Brown 0.30 177 151.8 4.18 Yellow 0.20 135 101.2 11.29 Red 0.20 79 101.2 4.87 Orange0.10 41 50.6 1.82 Green 0.10 36 50.6 4.21 Blue 0.10 38 50.6 3.14 Totals: 506 29.51 = 11.07 (5 degrees of freedom) Since 29.51 >11.07, we conclude that the percentage figures reported by the company have changed. 5. Observed Epected Hypothesized Frequency Frequency Category Proportion (fi) (ei) (fi - ei)2 / ei Full Serve 1/3 264 249.330.86 Discount 1/3 255 249.33 0.13 Both 1/3 229 249.33 1.66 Totals: 748 2.65 = 4.61 (2 degrees of freedom) Since 2.65 9.24, we conclude that there is a difference in the proportion of ads with guilt ealsamong the si types of magazines. 7. Epected frequencies: ei = (1 / 3) (135) = 45 With 2 degrees of freedom, = 5.99 Do not reject H0; there is no justifation for concluding a difference in preference eists. 8. H0: p1 = .03, p2 = .28, p3 = .45, p4 = .24 df = 3 = 11.34 Reject H0 if c2 > 11.34 Rating Observed Epected (fi - ei)2 / eiEcellent 24 .03(400) = 12 12.00 Good 124 .28(400) = 112 1.29 Fair172 .45(400) = 180 .36 Poor 80 .24(400) = 96 2.67 400 400 c2 =16.31 Reject H0; conclude that the ratings differ. A comparison of observed and epected frequencies show telephone serve is slightly better with more ecellent and good ratings. 9. H0 = The column variable is independent of the row variable Ha = The column variable is not independent of the row variable Epected Frequencies: A B C P 28.539.9 45.6 Q 21.5 30.1 34.4 = 7.37776 with (2 - 1) (3 - 1)= 2degrees of freedom Since c2 = 7.86 > 7.37776 Reject H0 Conclude that the column variable is not independent of the row variable.10. H0 = The column variable is independent of the row variable Ha = The column variable is not independent of the row variable Epected Frequencies: A B C P 17.5000 30.6250 21.8750 Q 28.7500 50.3125 35.9375R 13.7500 24.0625 17.1875 = 9.48773 with (3 - 1) (3 - 1)= 4 degrees of freedom Since c2 = 19.78 > 9.48773 Reject H0Conclude that the column variable is not independent of f the row variable. 11. H0 : Type of tket purchased is independent of the type of flight Ha: Type of tket purchased is not independent of the type of flight. Epected Frequencies: e11 = 35.59 e12 = 15.41 e21 = 150.73e22 = 65.27 e31 = 455.68 e32 = 197.32 Observed Epected Frequency Frequency Tket Flight (fi) (ei) (fi - ei)2 / ei First Domest 29 35.59 1.22 First International 22 15.41 2.82 Business Domest 95 150.73 20.61 Business International 121 65.27 47.59 Full Fare Domest 518455.68 8.52 Full Fare International 135 197.32 19.68 Totals: 920 100.43 = 5.99 with (3 - 1)(2 - 1) = 2 degrees of freedom Since 100.43 > 5.99, we conclude that the type of tket purchased is not independent of the type of flight. 12.a.Observed Frequency (fij)Domest European Asian Total Same 125 55 68 248 Different 140 105 107 352 Total 265 160 175 600 Epected Frequency (eij) Domest EuropeanAsian Total Same 109.53 66.13 72.33 248 Different 155.47 93.87 102.67352 Total 265 160 175 600 Chi Square (fij - eij)2 / eij Domest European Asian Total Same 2.18 1.87 0.26 4.32 Different 1.54 1.32 0.183.04 c2 = 7.36 Degrees of freedom = 2 = 5.99 RejectH0; conclude brand loyalty is not independent of manufacturer. b.Brand Loyalty Domest 125/265 = .472 (47.2) ¬ Highest European 55/160= .344 (34.4) Asian 68/175 = .389 (38.9) 13. Industry Major OilChemal Electral Computer Business 30 22.5 17.5 30 Enneering 30 22.5 17.530 Note: Values shown above are the epected frequencies. =11.3449 (3 degrees of freedom: 1 3 = 3) c2 = 12.39 Reject H0; conclude that major and industry not independent.14. Epected Frequencies: e11 = 31.0 e12 = 31.0 e21 = 29.5 e22 = 29.5 e31 = 13.0 e32 = 13.0e41 = 5.5 e42 = 5.5 e51 = 7.0 e52 = 7.0 e61 = 14.0 e62 = 14.0 Observed Epected Frequency Frequency Most Diffult Gender (fi) (ei)(fi - ei)2 / ei Spouse Men 37 31.0 1.16 Spouse Women 25 31.0 1.16 Parents Men 28 29.5 0.08 Parents Women 31 29.5 0.08 Children Men 7 13.0 2.77 Children Women 19 13.0 2.77 Siblings Men 8 5.5 1.14Siblings Women 3 5.5 1.14 In-Laws Men 4 7.0 1.29 In-Laws Women 107.0 1.29 Other Relatives Men 16 14.0 0.29 Other Relatives Women 1214.0 0.29 Totals: 2013.43 = 11.0705 with (6 - 1) (2 - 1) = 5 degrees of freedom Since 13.43 > 11.0705.we conclude that gender is not independent of the most diffult person to buy for. 15. Epected Frequencies: e11 = 17.16e12 = 12.84 e21 = 14.88 e22 = 11.12 e31 = 28.03 e32 = 20.97 e41 =22.31 e42 = 16.69 e51 = 17.16 e52 = 12.84 e61 = 15.45 e62 = 11.55 Observed Epected Frequency Frequency Magazine eal (fi) (ei) (fi -ei)2 / ei News Guilt 20 17.16 0.47 News Fear 10 12.84 0.63 GeneralGuilt 15 14.88 0.00 General Fear 11 11.12 0.00 Family Guilt 30 28.030.14 Family Fear 19 20.97 0.18 Business Guilt 22 22.31 0.00 BusinessFear 17 16.69 0.01 Female Guilt 16 17.16 0.08 Female Fear 14 12.840.11 Afran-Ameran Guilt 12 15.45 0.77 Afran-Ameran Fear 15 11.55 1.03 Totals: 201 3.41 = 15.09 with (6 - 1) (2 - 1) = 5 degrees offreedom Since 3.41 13.28, we conclude that the ratings are not independent. 20. First estimate m from the sle data. Sle size =120.Therefore, we use Poisson probabilities with m = 1.3 to computeepected frequencies. Observed Frequency Poisson Probability Epected Frequency Difference (fi - ei) 0 39 .2725 32.700 6.300 1 30 .354342.516 -12.516 2 30 .2303 27.636 2.364 3 18 .0998 11.976 6.024 4 or more 3 .0430 5.160 - 2.160 = 7.81473 with 5 - 1 - 1 = 3degrees of freedom Since c2 = 9.0348 > 7.81473 Reject H0 Conclude that the data do not follow a Poisson probability distribution.21. With n = 30 we will use si classes with 16 2/3 of the probability associated with each class. = 22.80 s = 6.2665 The z valuesthat create 6 intervals, each with probability .1667 are -.98, -.43,0, .43, .98 z Cut off value of -.98 22.8 - .98 (6.2665) = 16.66 -.43 22.8 - .43 (6.2665) = 20.11 0 22.8 + 0 (6.2665) = 22.80 .43 22.8+ .43 (6.2665) = 25.49 .98 22.8 + .98 (6.2665) = 28.94 Interval Observed Frequency Epected Frequency Difference less than 16.66 3 5 -2 16.66 - 20.11 7 5 2 20.11 - 22.80 5 5 0 22.80 - 25.49 7 5 2 25.49-28.94 3 5 -2 28.94 and up 5 5 0 = 9.34840 with 6 - 2 - 1 = 3 degrees of freedom Since c2 = 3.20 £ 9.34840 Do not reject H0 The claim that the data comes from a normal distribution cannot be rejected. e Poisson probabilities with m = 1. c2 = 4.30 = 5.99147 (2 degrees of freedom) Do not reject H0; the assumption of a Poisson distribution cannot be rejected. 23. Observed Poisson Probabilities Epected 0 15 .1353 13.53 1 31 .2707 27.07 2 20 .270727.07 3 15 .1804 18.04 4 13 .0902 9.02 5 or more 6 .0527 5.27 c2 = 4.98 = 7.77944 (4 degrees of freedom) Do not reject H0; the assumption of a Poisson distribution cannot be rejected. 24. = 24.5 s = 3 n = 30 Use 6 classes Interval Observed Frequency Epected Frequency less than 21.56 5 5 21.56 - 23.21 4 5 23.21 - 24.50 3 5 24.50- 25.79 7 5 25.79 - 27.44 7 5 27.41 up 4 5 c2 = 2.8 = 6.25139 (3 degrees of freedom: 6 - 2 - 1 = 3) Do not reject H0; theassumption of a normal distribution cannot be rejected. 25. = 71 s = 17 n = 25 Use 5 classes Interval Observed Frequency Epected Frequency less than 56.7 7 5 56.7 - 66.5 7 5 66.5 - 74.6 1 5 74.6 - 84.5 1 5 84.5 up 9 5 c2 = 11.2 = 9.21034 (2 degrees of freedom) Reject H0; conclude the distribution is not a normal distribution. 26. Observed 60 45 59 36 Epected 50 50 50 50 c2 = 8.04 = 7.81473 (3 degrees of freedom) Reject H0; conclude that the order potentials are not the same in each sales territory. 27. Observed 48 323 79 16 63 Epected 37.03 306.82 126.96 21.16 37.03 Since 41.69 > 13.2767,reject H0. Mutual fund investors' attitudes toward corporate bonds differ from their attitudes toward corporate stock. 28. Observed 20 20 40 60 Epected 35 35 35 35 Since 31.43 > 7.81473, reject H0. The park manager should not plan on the same number attending eachday.Plan on a larger staff for Sundays and holidays. 29. Observed 13 16 28 17 16 Epected 18 18 18 18 18 c2 = 7.44 = 9.48773 Do not reject H0; the assumption that the number of riders is uniformly distributed cannot be rejected.30. Observed Epected Hypothesized Frequency Frequency Category Proportion (fi) (ei) (fi - ei)2 / ei Very Satisfied 0.28 105 140 8.75 Somewhat Satisfied 0.46 235 230 0.11 Neither 0.12 55 60 0.42 Somewhat Dissatisfied 0.10 90 50 32.00 Very Dissatisfied 0.04 15 20 1.25 Totals: 500 42.53 = 9.49 (4 degrees of freedom) Since 42.53 > 9.49, we conclude that the job satisfaction for computer programmers is different than the job satisfaction for IS managers. 31. Epected Frequencies: Quality Shift Good Defective 1st 368.44 31.56 2nd 276.33 23.67 3rd 184.22 15.78 c2 = 8.11 =5.99147 (2 degrees of freedom) Reject H0; conclude that shift and quality are not independent. 32. Epected Frequencies: e11 = 1046.19e12 = 632.81 e21 = 28.66 e22 = 17.34 e31 = 258.59 e32 = 156.41 e41= 516.55 e42 = 312.45 Observed Epected Frequency Frequency Employment Reon (fi) (ei) (fi - ei)2 / ei Full-Time Eastern 1105 1046.19 3.31 Full-time Western 574 632.81 5.46 Part-Time Eastern 31 28.660.19 Part-Time Western 15 17.34 0.32 Self-Employed Eastern 229258.59 3.39 Self-Employed Western 186 156.41 5.60 Not EmployedEastern 485 516.55 1.93 Not Employed Western 344 312.45 3.19 Totals: 2969 23.37 = 7.81 with (4 - 1) (2 - 1) = 3 degrees of freedom Since 23.37 > 7.81, we conclude that employment status is notindependent of reon. 33. Epected frequencies: Loan roval Decision Loan Offes roved Rejected Miller 24.86 15.14 McMahon 18.64 11.36 Games 31.07 18.93 Runk 12.43 7.57 c2 = 2.21 = 7.81473 (3 degrees of freedom) Do not reject H0; the loan decision does not ear to be dependent on the offer. 34.a.Observed Frequency (fij) Never Married Married Divorced Total Men 234 106 10 350 Women 216 168 16 400 Total 450 274 26 750 Epected Frequency (eij) Never Married Married Divorced Total Men 210 127.87 12.13 350 Women 240 146.13 13.87 400 Total 450 27426 750 Chi Square (fij - eij)2 / eij Never Married MarriedDivorced Total Men 2.74 3.74 .38 6.86 Women 2.40 3.27 .33 6.00 c2 = 12.86 Degrees of freedom = 2 = 9.21 Reject H0; conclude martial status is not independent of gender. b.Martial Status Never Married Married Divorced Men 66.9 30.3 2.9 Women 54.0 42.0 4.0 Men100 - 66.9 = 33.1 have been married Women 100 - 54.0 = 46.0 have been married 35. Epected Frequencies: = 9.48773 (4 degrees of freedom) Since 9.76 7.81473.Do not reject H0; conclude that the assumption of a binomial distribution cannot be rejected.。
商务统计第四版课后习题答案09

Chapter 9Hypothesis TestingLearning Objectives1. Learn how to formulate and test hypotheses about a population mean and/or a population proportion.2. Understand the types of errors possible when conducting a hypothesis test.3. Be able to determine the probability of making various errors in hypothesis tests.4. Know how to compute and interpret p-values.5. Be able to use critical values to draw hypothesis testing conclusions.6. Be able to determine the size of a simple random sample necessary to keep the probability ofhypothesis testing errors within acceptable limits.7. Know the definition of the following terms:null hypothesis two-tailed testhypothesis p-valuealternativeType I error level of significanceType II error critical valuetestone-tailChapter 9Solutions:1. a. H0: µ≤ 600 Manager’s claim.H a: µ > 600b. We are not able to conclude that the manager’s claim is wrong.c. The manager’s claim can be rejected. We can conclude that µ > 600.2. a. H0: µ≤ 14H a: µ > 14 Research hypothesisb. There is no statistical evidence that the new bonus plan increases sales volume.c. The research hypothesis that µ > 14 is supported. We can conclude that the new bonus planincreases the mean sales volume.3. a. H0: µ = 32 Specified filling weightH a: µ≠ 32 Overfilling or underfilling existsb. There is no evidence that the production line is not operating properly. Allow the productionprocess to continue.µ≠ 32 and that overfilling or underfilling exists. Shut down and adjust the production c. Concludeline.4. a. H0: µ≥ 220H a: µ < 220 Research hypothesis to see if mean cost is less than $220.b. We are unable to conclude that the new method reduces costs.µ < 220. Consider implementing the new method based on the conclusion that it lowers c. Concludethe mean cost per hour.5. a. The Type I error is rejecting H0 when it is true. This error occurs if the researcher concludes thatyoung men in Germany spend more than 56.2 minutes per day watching prime-time TV when thenational average for Germans is not greater than 56.2 minutes.b. The Type II error is accepting H0 when it is false. This error occurs if the researcher concludes thatthe national average for German young men is ≤ 56.2 minutes when in fact it is greater than 56.2minutes.6. a. H0: µ≤ 1 The label claim or assumption.H a: µ > 1µ > 1 when it is not. This is the error of rejecting the product’s claim when the claim is b. Claimingtrue.Hypothesis Testing c. Concluding µ ≤ 1 when it is not. In this case, we miss the fact that the product is not meeting itslabel specification.7. a. H 0: µ ≤ 8000H a : µ > 8000 Research hypothesis to see if the plan increases average sales.b. Claiming µ > 8000 when the plan does not increase sales. A mistake could be implementing theplan when it does not help.c. Concludingµ ≤ 8000 when the plan really would increase sales. This could lead to not implementing a plan that would increase sales.8. a. H 0: µ ≥ 220H a : µ < 220b. Claimingµ < 220 when the new method does not lower costs. A mistake could be implementing the method when it does not help.c. Concludingµ ≥ 220 when the method really would lower costs. This could lead to not implementing a method that would lower costs.9. a. 2.12x z ===−b. Area = .4830p -value = .5000 - .4830 = .0170c. p -value ≤ .05, reject H 0d. Reject H 0 if z ≤ -1.645-2.12 ≤ -1.645, reject H 010. a. 1.48x z ===b. Area = .4306p -value = .5000 - .4306 = .0694c. p -value > .01, do not reject H 0d. Reject H 0 if z ≥ 2.331.48 <2.33, do not reject H 0Chapter 9 11. a. 2.00x z ===−b. Area = .4772p -value = 2(.5000 - .4772) = .0456c. p -value ≤ .05, reject H 0d. Reject H 0 if z ≤ -1.96 or z ≥ 1.96-2.00 ≤ -1.96, reject H 012. a. 1.25x z ===−p -value = .5000 - .3944 = .1056p -value > .01, do not reject H 0b. 2.50x z ===−p -value = .5000 - .4938 = .0062p -value ≤ .01, reject H 0c. 3.75x z ===−p -value ≈ 0p -value ≤ .01, reject H 0d. .83x z ===Area to left of z = .83p -value = .5000 + .2967 = .7967p -value > .01, do not reject H 013. Reject H 0 if z ≥ 1.645a. 2.42x z ===2.42 ≥ 1.645, reject H 0Hypothesis Testing b. .97x z ===.97 < 1.645, do not reject H 0c. 1.74x z ===1.74 ≥ 1.645, reject H 014. a. .87x z ===p -value = 2(.5000 - .3078) = .3844p -value > .01, do not reject H 0b. 2.68x z ===p -value = 2(.5000 - .4963) = .0074p -value ≤ .01, reject H 0c. 1.73x z ===−p -value = 2(.5000 - .4582) = .0836p -value > .01, do not reject H 015. a. H 0: µ ≥ 1056H a : µ < 1056b. 1.83x z ===−p -value = .5000 - .4664 = .0336c. p -value ≤ .05, reject H 0. Conclude the mean refund of “last minute” filers is less than $1056.d. Reject H 0 if z ≤ -1.645-1.83 ≤ -1.645, reject H 016. a. H 0: µ ≤ 895H a : µ > 895Chapter 9 b. 1.19x z ===Area = .3830p -value = .5000 - .3830 = .1170c. Do not reject H 0. We cannot conclude the rental rates have increased.d. Recommend withholding judgment and collecting more data on apartment rental rates beforedrawing a final conclusion.17. a. H 0: µ = 39.2H a : µ ≠ 39.2b. 1.54x z ===−p -value = 2(.5000 - .4382) = .1236c. p -value > .05, do not reject H 0. We cannot conclude that the mean length of a work week haschanged.d. Reject H 0 if z ≤ -1.96 or z ≥ 1.96z = -1.54; cannot reject H 018. a. H 0: µ = 4.1H a : µ ≠ 4.1b. 2.21x z ===−p -value = 2(.5000 - .4864) = .0272c. p -value = .0272 < .05Reject H 0 and conclude that the return for Mid-Cap Growth Funds differs significantly form that forU.S. Diversified funds.19. H 0: µ ≤ 14.32H a : µ > 14.322.15x z ===Area = .4842p -value = .5000 - .4842 = .0158Hypothesis Testing p -value ≤ .05, reject H 0. Conclude that there has been an increase in the mean hourly wage ofproduction workers.20. a. H 0: µ ≥ 181,900H a : µ < 181,900b. 2.93x z ===−c. p -value = .5000 - .4983 = .0017d. p -value ≤ .01; reject H 0. Conclude mean selling price in South is less than the national mean sellingprice.21. a. H 0: µ ≤ 15H a : µ > 15b. 2.96x z ===c. p -value = .5000 - .4985 = .0015d. p -value ≤ .01; reject H 0; the premium rate should be charged.22. a. H 0: µ = 8H a : µ ≠ 8b. 1.37x z ===p -value = 2(.5000 - .4147) = .1706c. Do not reject H 0. Cannot conclude that the population mean waiting time differs from 8 minutes.d. .025(x z σ±8.4 ± 1.96(3.28.4 ± .57 (7.83 to 8.97)Yes;µ = 8 is in the interval. Do not reject H 0.23. a. 2.31x t ===Chapter 9b. Degrees of freedom = n – 1 = 24Using t table, p -value is between .01 and .025.Actual p -value = .0147c. p -value ≤ .05, reject H 0.d. With df = 24, t .05 = 1.711Reject H 0 if t ≥ 1.7112.31 > 1.711, reject H 0.24. a. 1.54x t ===−b. Degrees of freedom = n – 1 = 47Area in lower tail is between .05 and .10Using t table, p -value (two-tail) is between .10 and .20Actual p -value = .1304c. p -value > .05, do not reject H 0.d. With df = 47, t .025 = 2.012Reject H 0 if t ≤ -2.012 or t ≥ 2.012t = -1.54; do not reject H 025. a. 1.15x t ===−Degrees of freedom = n – 1 = 35Using t table, p -value is between .10 and .20Actual p -value = .1282p -value > .01, do not reject H 0b. 2.61x t ===−Using t table, p -value is between .005 and .01Actual p -value = .0066Hypothesis Testingp -value ≤ .01, reject H 0c. 1.20x t ===Using t table, area in upper tail is between .10 and .20p -value (lower tail) is between .80 and .90Actual p -value = .8809p -value > .01, do not reject H 026. a. 2.10x t ===Degrees of freedom = n – 1 = 64Using t table, area in tail is between .01 and .025p -value (two tail) is between .02 and .05Actual p -value = .0394p -value ≤ .05, reject H 0b. 2.57x t ===−Using t table, area in tail is between .005 and .01p -value (two tail) is between .01 and .02Actual p -value = .0127p -value ≤ .05, reject H 0c. 1.54x t ===Using t table, area in tail is between .05 and .10p -value (two tail) is between .10 and .20Actual p -value = .1295p -value > .05, do not reject H 027. a. H 0: µ ≥ 238Chapter 9H a : µ < 238b. .88x t ===−Degrees of freedom = n – 1 = 99Using t table, p -value is between .10 and .20Actual p -value = .1918c. p -value > .05; do not reject H 0. Cannot conclude mean weekly benefit in Virginia is less than thenational mean.d. df = 99 t .05 = -1.66Reject H 0 if t ≤ -1.66-.88 > -1.66; do not reject H 028. a. H 0: µ ≤ 3530H a : µ > 3530b. 2.49x t ===Degrees of freedom = n – 1 = 91Using t table, p -value is between .005 and .01Actual p -value = .0074c. p -value ≤ .01; reject H 0. The mean attendance per game has increased. Anticipate a new all-timehigh season attendance during the 2002 season.29. a. H 0: µ = 5600H a : µ ≠ 5600b. 2.26x t ===Degrees of freedom = n – 1 = 24Using t table, area in tail is between .01 and .025Actual p -value = .0332c. p -value ≤ .05; reject H 0. The mean diamond price in New York City differs.d. df = 24 t .025 = 2.064Reject H 0 if t < -2.064 or t > 2.0642.26 > 2.064; reject H 030. a. H 0: µ = 600H a : µ ≠ 600b. 1.17x t ===df = n - 1 = 39Using t table, area in tail is between .10 and .20p -value is between .20 and .40Actual p -value = .2501c. With α= .10 or less, we cannot reject H 0. We are unable to conclude there has been a change in themean CNN viewing audience.d. The sample mean of 612 thousand viewers is encouraging but not conclusive for the sample of 40days. Recommend additional viewer audience data. A larger sample should help clarify the situationfor CNN.31. H 0: µ ≤ 47.50H a : µ > 47.502.33x t ===Degrees of freedom = n - 1 = 63Using t table, p -value is between .01 and .025Actual p -value = .0114Reject H 0; Atlanta customers are paying a higher mean water bill.32. a. H 0: µ = 10,192H a : µ ≠ 10,192b. 2.23x t ===−Degrees of freedom = n – 1 = 49Using t table, area in tail is between .01 and .025p -value is between .02 and .05Actual p -value = .0302c. p -value ≤ .05; reject H 0. The population mean price at this dealership differs from the national meanprice $10,192.33. a. H 0: µ ≤ 280H a : µ > 280b. 286.9 - 280 = 6.9 yardsc. 2.07x t ===Degrees of freedom = n – 1 = 8Using t table, p -value is between .025 and .05Actual p -value = .0361c. p -value ≤ .05; reject H 0. The population mean distance for the new driver is greater than the USGAapproved driver.34. a. H 0: µ = 2H a : µ ≠ 2b. 22 2.210i x x nΣ===c..516s ==d. 1.22x t ===Degrees of freedom = n - 1 = 9 Using t table, area in tail is between .10 and .20p -value is between .20 and .40Actual p -value = .2518e. p -value > .05; do not reject H 0. No reason to change from the 2 hours for cost estimating purposes.35. a. 1.25p z ===−b. Area in tail = (.5000 - .3944) = .1056p -value = 2(.1056) = .2112c. p -value > .05; do not reject H 0d. z .025 = 1.96Reject H 0 if z ≤ -1.96 or z ≥ 1.96z = 1.25; do not reject H −036. a. 2.80p z ===−p -value = .5000 - .4974 = .0026p -value ≤ .05; reject H 0b. 1.20z ==−p -value = .5000 - .3849 = .1151p -value > .05; do not reject H 0c. 2.00z ==−p -value = .5000 - .4772 = .0228p -value ≤ .05; reject H 0d. .80z ==p -value = .5000 + .2881 = .7881p -value > .05; do not reject H 037. a. H 0: p ≤ .40H a : p > .40b. 189.45 425p==1.88pz===Area = .4699p-value = .5000 - .4699 = .0301c. p-value ≤ .05; reject H0. Conclude more than 40% receive more than 10 e-mail messages per day.38. a. H0: p= .64H a: p≠ .64b.52.52100p==2.50pz===−Area = .4938p-value = 2(.5000 - .4938) = .0124c. p-value ≤ .05; reject H0. Proportion differs from the reported .64.d. Yes.Sincep= .52, it indicates that fewer than 64% of the shoppers believe the supermarket brand is as good as the name brand.39. a. H0: p= .70H a: p≠ .70b. Wisconsin252.72350p==.82pz===Area = .2939p-value = 2(.5000 - .2939) = .4122Cannot reject H0.California 189.63 300p==2.65z==−Area = .4960p-value = 2(.5000 - .4960) = .0080RejectH0. California has a different (lower) percentage of adults who do not exercise regularly. 40. a.414.27021532p== (27%)b. H0: p≤ .22H a: p > .224.75pz===p-value ≈ 0RejectH0; There has been a significant increase in the intent to watch the TV programs.c. These studies help companies and advertising firms evaluate the impact and benefit of commercials.41. a. H0: p≥ .75H a: p < .75b. 1.20pz===−Area = .3849p-value = .5000 - .3849 = .1151c. p-value > .05; do not reject H0. The executive's claim cannot be rejected.42. H0: p≤ .2493.31300p==2.84pz===Area = .4977p-value = .5000 - .4977 = .0023p-value ≤ .05; reject H0. In 2003, an estimated 31% of people who moved selected to be convenient to work as their primary reason. This is an increase compared to 1990.43. a. H0: p= .48H a: p≠ .48b.360.45800p==1.70pz===−Area = .4554p-value = 2(.5000 - .4554) = .0892c. p-value > .05; do not reject H0. There is no reason to conclude the proportion has changed.44. a. H0: p≤ .51H a: p > .51b.232.58400p==2.80pz===p-value = .5000 - .4974 = .0026c. Sincep-value = .0026 ≤ .01, we reject H0 and conclude that people working the night shift get drowsy while driving more often than the average for the entire population.45. a. H0: p = .44b. 205.41 500p==1.35pz===−Area = .4115p-value = 2(.5000 - .4115) = .1770p-value > .05; do not reject H0. No change.c.245.49500p==2.25z==Area = .4878p-value = 2(.5000 - .4878) = .0244p-value ≤ .05; reject H0. There has been a change: an increase in repeat customers.46. a. H0: µ = 16H a: µ≠ 16b. 2.19xz===Area = .4857p-value = 2(.5000 - .4857) = .0286p-value ≤ .05; reject H0. Readjust production line.c. 1.23xz===−Area = .3907p-value = 2(.5000 - .3907) = .2186p-value > .05; do not reject H0. Continue the production line.d. RejectH0 if z≤ -1.96 or z≥ 1.96For x = 16.32, z = 2.19; reject H 0Forx = 15.82, z = -1.23; do not reject H 0Yes, same conclusion.47. a. H 0: µ = 900H a : µ ≠ 900b..025x z ± 935±935 ± 25 (910 to 960)c. Reject H 0 because µ = 900 is not in the interval.d. 2.75x z ===p -value = 2(.5000 - .4970) = .006048. a. H 0: µ ≤ 45,250H a : µ > 45,250b. 2.71x z ===p -value = .5000 - .4966 = .0034p -value ≤ .01; reject H 0. Conclude New York City has higher mean salary.49. a. H 0: µ ≤ 37,000H a : µ > 37,000b. 1.47x t ===Degrees of freedom = n – 1 = 47Using t table, p -value is between .05 and .10Actual p -value = .0747c. p -value > .05, do not reject H 0. Cannot conclude mean greater than $37,000. A larger sample isdesirable.50. H 0: µ = 6000H a : µ ≠ 6000.93x t ===−Degrees of freedom = n – 1 = 31Using t table, area in tail is between .10 and .20p -value is between .20 and .40Actual p -value = .3581Do not reject H 0. There is no evidence to conclude that the mean number of freshman applicationshas changed.51. a. H 0: µ ≥ 6883H a : µ < 6883b. 1.89x t ===−Using the t table with df = 39, the p -value is between .05 and .025.Actual p -value = .033c. We should conclude that Medicare spending per enrollee in Indianapolis is less than the nationalaverage.d. Using the critical value approach we would:Reject H 0 if t = -1.685.05t ≤−Since t = -1.89-1.685, we reject H ≤0.52. H 0: µ ≤ 125,000H a : µ > 125,0002.26x t ===Degrees of freedom = 32 – 1 = 31Using t table, p -value is between .01 and .025Actual p -value = .0154p -value ≤ .05; reject H 0. Conclude that the mean cost is greater than $125,000 per lot.53. a. H 0: µ = 3H a : µ ≠ 3b. 2.8i x x n Σ==c..70s ==d. .90x t ===−Degrees of freedom = 10 - 1 = 9 Using t table, area in tail is between .10 and .20p -value is between .20 and .40Actual p -value = .3902e. p -value > .05; do not reject H 0. There is insufficient evidence to conclude a difference compared toprior year.54. a. H 0: p ≤ .50H a : p > .50b. 64.64100p ==c. 2.80p z ===Area in tail = .4974p -value = .5000 - .4974 = .0026p -value ≤ .01; reject H 0. College graduates have a greater stop-smoking success rate.55. a. H 0: p = .6667H a : p ≠ .6667b. 355.6502546p ==c. .82p z ===−Hypothesis Testingp -value = 2(.5000 - .2939) = .4122p -value > .05; do not reject H 0; Cannot conclude that the population proportion differs from 2/3.56. a.H 0: p ≤ .50H a : p > .50b. 67.6381105p == (64%)c. 2.83p z ===p -value = .5000 - .4977 = .0023p -value ≤ .01; reject H 0. Conclude that the four 10-hour day schedules is preferred by more than 50% of the office workers.57. a. 503.553910p ==b. H 0: p ≤ .50H a : p > .50c. 3.19p z ===p -value .00≈You can tell the manager that the observed level of significance is very close to zero and that thismeans the results are highly significant. Any reasonable person would reject the null hypotheses andconclude that the proportion of adults who are optimistic about the national outlook is greater than.5058. H 0: p ≥ .90H a : p < .9049.844858p ==1.40p z ===−p -value = .5000 - .4192 = .0808Chapter 9p -value > .05; do not reject H 0. Claim of at least 90% cannot be rejected.59. a. H 0: p ≥ .47H a : p < .47b. 44.352125p ==c. 2.64p z ===−p -value = .5000 - .4959 = .0041d. p -value ≤ .01; reject H 0. The proportion of foods containing pesticides has declined.。
大学课件商务与经济统计习题答案(第8版,中文版)
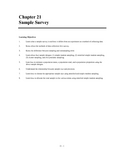
Chapter 21Sample SurveyLearning Objectives1. Learn what a sample survey is and how it differs from an experiment as a method of collecting data.2. Know about the methods of data collection for a survey.3. Know the difference between sampling and nonsampling error.4. Learn about four sample designs: (1) simple random sampling, (2) stratified simple random sampling,(3) cluster sampling, and (4) systematic sampling.5. Lean how to estimate a population mean, a population total, and a population proportion using theabove sample designs.6. Understand the relationship between sample size and precision.7. Learn how to choose the appropriate sample size using stratified and simple random sampling.8. Learn how to allocate the total sample to the various strata using stratified simple random sampling.Chapter 21Solutions:1. a. x = 215 is an estimate of the population mean.b. 2.7386xs=c. 215 ± 2(2.7386) or 209.5228 to 220.47722. a. Estimate of population total = N x = 400(75) = 30,000b. Estimate of Standard Error =xNs400320xNs=c. 30,000 ± 2(320) or 29,360 to 30,6403. a. p= .30 is an estimate of the population proportionb. .0437ps=c. .30 ± 2(.0437) or .2126 to .38744. B = 15222(70)490072.983067.1389(15)(70)4450n===+A sample size of 73 will provide an approximate 95% confidence interval of width 30.5. a. x= 149,670 and s = 73,42010,040.83xs==approximate 95% confidence interval149,670 ± 2(10,040.83)or$129,588.34 to $169,751.66b. X= N x= 771(149,670) = 115,395,570xs= Nxs= 771(10,040.83) = 7,741,479.93Sample Surveyapproximate 95% confidence interval115,395,770 ± 2(7,741,479.93)or$99,912,810.14 to $130,878,729.86c.p= 18/50 = 0.36 and .0663p s =approximate 95% confidence interval0.36 ± 2(0.0663)or0.2274 to 0.4926This is a rather large interval; sample sizes must be rather large to obtain tight confidence intervals on a population proportion.6. B = 5000/2 = 2500 Use the value of s for the previous year in the formula to determine the necessary sample size.222(31.3)979.69336.00512.9157(2.5)(31.3)4724n ===+A sample size of 337 will provide an approximate 95% confidence interval of width no larger than$5000.7. a. Stratum 1: = 138 Stratum 2: 2x = 103Stratum 3: 3x = 210b. Stratum 1 1x = 1381 6.3640x s =138 ± 2(6.3640)or125.272 to 150.728Stratum 22x = 103Chapter 2124.2817xs==103 ± 2(4.2817)or94.4366 to 111.5634 Stratum 33x= 21038.6603xs==210 ± 2(8.6603)or192.6794 to 227.3206c.200250100138103210550550550stx⎛⎫⎛⎫⎛⎫=++⎪ ⎪ ⎪⎝⎭⎝⎭⎝⎭= 50.1818 + 46.8182 + 38.1818= 135.1818stxs=3.4092=approximate 95% confidence interval135.1818 ± 2(3.4092)or128.3634 to 142.00028. a. Stratum 1:11N x = 200(138) = 27,600Stratum 2:22N x = 250(103) = 25,750Stratum 3:33N x = 100(210) = 21,000b.stN x = 27,600 + 25,750 + 21,000 = 74,350Note: the sum of the estimate for each stratum total equalsstN xc.stN x= 550(3.4092) = 1875.06 (see 7c)Sample Surveyapproximate 95% confidence interval74,350 ± 2(1875.06)or70,599.88 to 78,100.129. a. Stratum 11p = .501.1088p s ==50 ± 2(.1088)or.2824 to .7176Stratum 22p = .782.0722p s.78 ± 2(.0722)or.6356 to .9244Stratum 33p = .213.0720p s =.21 ± 2(.0720)or .066 to .354b. 200250100(.50)(.78)(.21).5745550550550st p =++=c.st p s =.0530==d.approximate 95% confidence intervalChapter 21.5745 ± 2(.0530)or.4685 to .680510. a.[]222222300(150)600(75)500(100)(140,000)92.8359196,000,00015,125,000(20)(1400)300(150)600(75)500(100)2n ++===+⎛⎫⎡⎤+++⎪⎣⎦⎝⎭Rounding up we choose a total sample of 93.1300(150)9330140,000n ⎛⎫== ⎪⎝⎭2600(75)9330140,000n ⎛⎫== ⎪⎝⎭3500(100)9333140,000n ⎛⎫== ⎪⎝⎭b. With B = 10, the first term in the denominator in the formula for n changes.2222(140,000)(140,000)305.653049,000,00015,125,000(10)(1400)15,125,0004n ===+⎛⎫+ ⎪⎝⎭Rounding up, we see that a sample size of 306 is needed to provide this level of precision.1300(150)30698140,000n ⎛⎫== ⎪⎝⎭2600(75)30698140,000n ⎛⎫== ⎪⎝⎭3500(100)306109140,000n ⎛⎫== ⎪⎝⎭Due to rounding, the total of the allocations to each strata only add to 305. Note that even thoughthe sample size is larger, the proportion allocated to each stratum has not changed.222(140,000)(140,000)274.606056,250,00015,125,000(15,000)15,125,0004n ===++Rounding up, we see that a sample size of 275 will provide the desired level of precision.The allocations to the strata are in the same proportion as for parts a and b.Sample Survey1300(150)27598140,000n ⎛⎫== ⎪⎝⎭2600(75)27588140,000n ⎛⎫== ⎪⎝⎭3500(100)27598140,000n ⎛⎫== ⎪⎝⎭Again, due to rounding, the stratum allocations do not add to the total sample size. Another item could be sampled from, say, stratum 3 if desired.11. a. 1x = 29.53332x = 64.7753x = 45.2125 4x = 53.0300 b. Indianapolis29.5332± 29.533 ± 10.9086(.9177)or19.5222 to 39.5438Louisville64.7752± 64.775 ± 17.7248(.9068)or48.7022 to 80.8478St. Louis45.21252± 45.2125 ± (13.7238) (.9487)or32.1927 to 58.2323Memphis53.03002±53.0300 ± 18.7719(.9258)or35.6510 to 70.4090Chapter 21c.381455803705.426923362338233823310st p ⎛⎫⎛⎫⎛⎫⎛⎫⎛⎫⎛⎫⎛⎫⎛⎫=+++= ⎪⎪ ⎪⎪ ⎪⎪ ⎪⎪⎝⎭⎝⎭⎝⎭⎝⎭⎝⎭⎝⎭⎝⎭⎝⎭d.11111115(1)66()38(32)33.777815p p N N n n ⎛⎫⎛⎫⎪⎪-⎝⎭⎝⎭-==-22222253(1)88()45(37)55.747817p p N N n n ⎛⎫⎛⎫⎪⎪-⎝⎭⎝⎭-==-33333335(1)88()80(72)192.857117p p N N n n ⎛⎫⎛⎫⎪⎪-⎝⎭⎝⎭-==-44444455(1)1010()70(60)116.666719p p N N n n ⎛⎫⎛⎫⎪⎪-⎝⎭⎝⎭-==-.0857st p s ==approximate 95% confidence interval.4269 ± 2(.0857)or.2555 to .598312. a. St. Louis total = 11N x = 80 (45.2125) = 3617In dollars: $3,617,000b. Indianapolis total = 11N x = 38 (29.5333) = 1122.2654 In dollars: $1,122,265c.3845807029.533364.77545.212553.030048.7821233233233233st x ⎛⎫⎛⎫⎛⎫⎛⎫=+++= ⎪ ⎪ ⎪ ⎪⎝⎭⎝⎭⎝⎭⎝⎭2211111(13.3603)()38(32)36,175.5176s N N n n -==2222222(25.0666)()45(37)130,772.18s N N n n -==Sample Survey2233333(19.4084)()80(72)271,213.918s N N n n -==2244444(29.6810)()70(60)370,003.9410s N N n n -==st x s =3.8583==approximate 95% confidence interval2st st x x s ±48.7821 ± 2(3.8583)or41.0655 to 56.4987In dollars: $41,066 to $56,499d.approximate 95% confidence interval2st st x Nx Ns ±233(48.7821) ± 2(233)(3.8583)11,366.229 ± 1797.9678or9,568.2612 to 13,164.197In dollars: $9,568,261 to $13,164,19713.[]222222250(80)38(150)35(45)(11,275)27.33943,404,0251,245,875(30)(123)50(80)38(150)35(45)4n ++===+⎛⎫⎡⎤+++ ⎪⎣⎦⎝⎭Rounding up we see that a sample size of 28 is necessary to obtain the desired precision.150(80)281011,275n ⎛⎫== ⎪⎝⎭238(150)281411,275n ⎛⎫== ⎪⎝⎭335(45)28411,275n ⎛⎫== ⎪⎝⎭Chapter 21b. [][]2222222250(100)38(100)35(100)123(100)333,404,025123(100)(30)(123)50(100)38(100)35(100)4n ++===+⎛⎫⎡⎤+++ ⎪⎣⎦⎝⎭150(100)331312,300n ⎛⎫== ⎪⎝⎭238(100)331012,300n ⎛⎫== ⎪⎝⎭335(100)33912,300n ⎛⎫== ⎪⎝⎭This is the same as proportional allocation . Note that for each stratumh h N n n N ⎛⎫= ⎪⎝⎭14. a. 7501550i c i x x M ∑===∑ c X M x = = 300(15) = 450015.3050i c i a p M ∑===∑ b. 2()i c i x x M ∑- = [ 95 - 15 (7) ]2 + [ 325 - 15 (18) ]2 + [ 190 - 15 (15) ]2 + [ 140 - 15 (10)]2 = (-10)2 + (55)2 + (-35)2 + (-10)2 = 44501.4708c x s == c x X s Ms == 300(1.4708) = 441.242()i c i a p M ∑- = [ 1 - .3 (7) ]2 + [ 6 - .3 (18) ]2 + [ 6 - .3 (15) ]2 + [2 - .3 (10) ]2 = (-1.1)2 + (.6)2 + (1.5)2 + (-1)2 = 4.82.0484c p s c. approximate 95% confidence Interval for Population Mean: 15 ± 2(1.4708) or12.0584 to 17.9416d. approximate 95% confidenceInterval for Population Total:4500 ± 2(441.24)or3617.52 to 5382.48e. approximate 95% confidenceInterval for Population Proportion:.30 ± 2(.0484)or.2032 to .396815. a.10,400 80130c x ==c X M x = = 600(80) = 48,00013.10130c p ==b. 2()i c i x x M ∑- = [ 3500 - 80 (35) ]2 + [ 965 - 80 (15) ]2 + [ 960 - 80 (12) ]2+ [ 2070 - 80 (23) ]2 + [ 1100 - 80 (20) ]2 + [ 1805 - 80 (25) ]2= (700)2 + (-235)2 + (0)2 + (230)2 + (-500)2 + (-195)2= 886,1507.6861c x s =approximate 95% confidenceInterval for Population Mean:80 ± 2(7.6861)or64.6278 to 95.3722c. X s = 600(7.6861) = 4611.66approximate 95% confidenceInterval for Population Total:48,000 ± 2(4611.66)or38,776.68 to 57,223.322()i c i a p M ∑- = [ 3 - .1 (35) ]2 + [ 0 - .1 (15) ]2 + [ 1 - .1 (12) ]2 + [4 - .1 (23) ]2+ [ 3 - .1 (20) ]2 + [ 2 - .1 (25) ]2= (-.5)2 + (-1.5)2 + (-.2)2 + (1.7)2 + (1)2 + (-.5)2 = 6.68.0211c p sapproximate 95% confidenceInterval for Population Proportion:.10 ± 2(.0211)or.0578 to .142216. a.2000 4050c x ==Estimate of mean age of mechanical engineers: 40 yearsb. 35.7050c p ==Estimate of proportion attending local university: .70c. 2()i c i x x M ∑- = [ 520 - 40 (12) ]2 + · · · + [ 462 - 40 (13) ]2= (40)2 + (-7)2 + (-10)2 + (-11)2 + (30)2 + (9)2 + (22)2 + (8)2 + (-23)2+ (-58)2= 72922.0683c x s ==approximate 95% confidenceInterval for Mean age:40 ± 2(2.0683)or35.8634 to 44.1366d. 2()i c i a p M ∑- = [ 8 - .7 (12) ]2 + · · · + [ 12 - .7 (13) ]2= (-.4)2 + (-.7)2 + (-.4)2 + (.3)2 + (-1.2)2 + (-.1)2 + (-1.4)2 + (.3)2+ (.7)2 + (2.9)2= 13.3.0883c p s =approximate 95% confidence Interval for Proportion Attending Local University:.70 ± 2(.0883)or.5234 to .876617. a.17(37)35(32)57(44)11,24036.9737173557304c x ++⋅⋅⋅+===++⋅⋅⋅+Estimate of mean age: 36.9737 yearsb. Proportion of College Graduates: 128 / 304 = .4211Proportion of Males: 112 / 304 = .3684c. 2()i c i x x M ∑- = [ 17 (37) - (36.9737) (17) ]2 + · · · + [ 57 (44) - (36.9737) (44) ]2= (.4471)2 + (-174.0795)2 + (-25.3162)2 + (-460.2642)2 + (173.1309)2+ (180.3156)2 + (-94.7376)2 + (400.4991)2= 474,650.682.2394c x s ==approximate 95% confidenceInterval for Mean Age of Agents:36.9737 ± 2(2.2394)or32.4949 to 41.4525d. 2()i c i a p M ∑- = [ 3 - .4211 (17) ]2 + · · · + [ 25 - .4211 (57) ]2= (-4.1587)2 + (-.7385)2 + (-2.9486)2 + (10.2074)2 + (-.1073)2 + (-3.0532)2+ (-.2128)2 + (.9973)2= 141.0989.0386c p s =approximate 95% confidenceInterval for Proportion of Agents that are College Graduates:.4211 ± 2(.0386)or.3439 to .4983e. 2()i c i a p M ∑- = [ 4 - .3684 (17) ]2 + · · · + [ 26 - .3684 (57) ]2= (-2.2628)2 + (-.8940)2 + (-2.5784)2 + (3.6856)2 + (-3.8412)2 + (1.5792)2+ (-.6832)2 + (5.0012)2= 68.8787.0270c p s =approximate 95% confidenceInterval for Proportion of Agents that are Male:.3684 ± 2(.0270)or.3144 to .422418. a. p = 0.190.0206p s ==Approximate 95% Confidence Interval:0.19 ± 2(0.0206)or0.1488 to 0.2312b. p = 0.310.0243p s ==Approximate 95% Confidence Interval:0.31 ± 2(0.0243)or0.2615 to 0.3585c. p = 0.170.0197p s ==Approximate 95% Confidence Interval:0.17 ± 2(0.0197)or0.1306 to 0.2094d. The largest standard error is when p = .50.At p = .50, we get0.0262p s =Multiplying by 2, we get a bound of B = 2(.0262) = 0.0525For a sample of 363, then, they know that in the worst case (p= 0.50), the bound will beapproximately 5%.e. If the poll was conducted by calling people at home during the day the sample results would only berepresentative of adults not working outside the home. It is likely that the Louis Harris organization took precautions against this and other possible sources of bias.19. a. Assume (N - n) / N≈ 1p= .550.0222s==pb. p= .31s==0.0206pc. The estimate of the standard error in part (a) is larger because p is closer to .50.d. Approximate 95% Confidence interval:.55 ± 2(.0222)or.5056 to .5944e. Approximate 95% Confidence interval:.31 ± 2(.0206).2688 to .351220. a. 204.9390s==xApproximate 95% Confidence Interval for Mean Annual Salary:23,200 ± 2(204.9390)or$22,790 to $23,610b. N x = 3000 (23,200) = 69,600,000s = 3000 (204.9390) = 614,817xApproximate 95% Confidence Interval for Population Total Salary:69,600,000 ± 2(614,817)or$68,370,366 to $70,829,634c. p= .73.0304psApproximate 95% Confidence Interval for Proportion that are Generally Satisfied:.73 ± 2(.0304)or.6692 to .7908d. If management administered the questionnaire and anonymity was not guaranteed we would expect adefinite upward bias in the percent reporting they were “generally satisfied” with their job. Aprocedure for guaranteeing anonymity should reduce the bias.21. a. p= 1/3.0840ps=Approximate 95% Confidence Interval:.3333 ± 2(.0840)or.1653 to .5013b. 2X = 760 (19 / 45) = 320.8889c. p= 19 / 45 = .4222.0722psApproximate 95% Confidence Interval:.4222 ± 2(.0722)or.2778 to .5666d.38010760192607.3717 140030140045140025stp⎛⎫⎛⎫⎛⎫⎛⎫⎛⎫⎛⎫=++=⎪⎪ ⎪⎪ ⎪⎪⎝⎭⎝⎭⎝⎭⎝⎭⎝⎭⎝⎭(1)(1/3)(2/3)()380(350)129h h h h h h p p N N n n ⎡⎤-∑-=⎢⎥-⎣⎦(19/45)(26/45)(7/25)(18/25)760(715)260(235)4424++= 1019.1571 + 3012.7901 + 513.2400 = 4545.1892.0482st p s ==Approximate 95% Confidence Interval:.3717 ± 2(.0482)or.2753 to .468122. a. X = 380 (9 / 30) + 760 (12 / 45) + 260 (11 / 25) = 431.0667Estimate approximately 431 deaths due to beating.b. 38097601226011.3079140030140045140025st p ⎛⎫⎛⎫⎛⎫⎛⎫⎛⎫⎛⎫=++= ⎪⎪ ⎪⎪ ⎪⎪⎝⎭⎝⎭⎝⎭⎝⎭⎝⎭⎝⎭[](1)()1h h h h h h p p N N n n -∑--= (380) (380 - 30) (9 / 30) (21 / 30) / 29 + (760) (760 - 45) (12 / 45) (33 / 45) / 44 + (260) (260 - 25)(11 / 25) (14 / 25) / 24= 4005.5079.0452st p s ==Approximate 95% Confidence Interval:.3079 ± 2(.0452)or.2175 to .3983c. 380217603426015.7116140030140045140025st p ⎛⎫⎛⎫⎛⎫⎛⎫⎛⎫⎛⎫=++= ⎪⎪ ⎪⎪ ⎪⎪⎝⎭⎝⎭⎝⎭⎝⎭⎝⎭⎝⎭[](1)()1h h h h h h p p N N n n -∑--= (380) (380 - 30) (21 / 30) (9 / 30) / 29 + (760) (760 - 45) (34 / 45) (11 / 45) / 44 +(260) (260 - 25) (15 / 25) (10 / 25) / 24= 3855.0417.0443st p s =Approximate 95% Confidence Interval:.7116 ± 2(.0443)or.6230 to .8002d. X = 1400 (.7116) = 996.24Estimate of total number of black victims ≈ 99623. a. []222222223000(80)600(150)250(220)100(700)50(3000)(20)(4000)3000(80)600(150)250(220)100(700)50(3000)4n ++++=⎛⎫+++++ ⎪⎝⎭366,025,000,000170.73651,600,000,000543,800,000==+Rounding up, we need a sample size of 171 for the desired precision.13000(80)17168605,000n ⎛⎫== ⎪⎝⎭2600(150)17125605,000n ⎛⎫== ⎪⎝⎭3250(220)17116605,000n ⎛⎫== ⎪⎝⎭4100(700)17120605,000n ⎛⎫== ⎪⎝⎭550(3000)17142605,000n ⎛⎫== ⎪⎝⎭24. a.14(61)7(74)96(78)23(69)71(73)29(84)18,06675.27514796237129240c x +++++===+++++Estimate of mean age is approximately 75 years old.b.122308102284.3514796237129240cp+++++===+++++2()i c ia p M∑-= [12 - .35 (14) ]2 + [ 2 - .35 (7) ]2 + [30 - .35 (96) ] 2+ [ 8 - .35 (23) ]2 + [ 10 - .35 (71) ]2 + [ 22 - .35 (29) ]2= (7.1)2 + (-.45)2 + (-3.6)2 + (-.05)2 + (-14.85)2 + (11.85)2= 424.52.0760cps=Approximate 95% Confidence Interval:.35 ± 2(.0760)or.198 to .502X= 4800 (.35) = 1680Estimate of total number of Disabled Persons is 1680.。
商务统计学第七版课后题答案第四章

商务统计学第七版课后题答案第四章一、单选题(每题2分,15小题共30分)1、下列标志中属于品质标志的是() [单选题] *A、学生年龄B、学生性别(正确答案)C、学生体重D、学生成绩2、某城市对占全市储蓄额4/5的几个大储蓄所进行调查,以了解全市储蓄的一般情况,这是() [单选题] *A、重点调查(正确答案)B、抽样调查C、典型调查D、普查3、时间数列的构成要素是() [单选题] *A、变量和次数B、时间和指标数值(正确答案)C、时间和次数D、主词和宾词4、某企业某月产品销售额为20万元,月末库存商品为30万元,这两个总量指标是() [单选题] *A、时期指标B、时点指标C、前者是时期指标,后者是时点指标(正确答案)D、前者是时点指标,后者是时期指标5、某企业报告期产品产量比基期增长25%,单位成本下降20%,则总成本()[单选题] *A、增加5%B、增加25%C、减少20%D、没有变化(正确答案)6、一个统计总体() [单选题] *A、只能有一个标志B、只能有一个指标C、可以有多个标志D、可以有多个指标(正确答案)7、下列是时期指标的是() [单选题] *A、出勤率B、合格率C、平均工资D、销售额(正确答案)8、抽样推断的主要目的是() [单选题] *A、广泛运用数学方法B、计算和控制抽样误差C、修正普查资料D、用样本指标来推算总体指标(正确答案)9、已知变量x与y之间存在着负相关关系,那么下列回归方程中肯定错误的是() [单选题] *A、yc =-10-0.8xB、yc =100-0.8xC、yc =-150+0.9x(正确答案)D、yc =25-0.7x10、下列属于比较相对数的是() [单选题] *A、中国人口和美国人口对比(正确答案)B、人口密度C、中国GDP增长速度D、职工人数11、某工人月工资5000元,则“工资”是() [单选题] *A、数量标志(正确答案)B、品质标志C、质量指标D、数量指标12、有意识地选取若干有代表性的单位进行调查时() [单选题] *A、重点调查B、抽样调查C、典型调查(正确答案)D、普查13、组数与组距的关系是() [单选题] *A、组数越多,组距越小(正确答案)B、组数越多,组距越大C、组数与组距无关D、组数越少,组距越小14、下列属于总量指标的是() [单选题] *A、出勤率B、合格率C、人均产粮D、工人人数(正确答案)15、标志变异指标中易受极端值影响的指标有() [单选题] *A、全距(正确答案)B、标准差C、平均差D、平均差系数二、多选题(每题2分,10小题共20分)1、下列是相对指标的是() *A、结构相对数(正确答案)B、比例相对数(正确答案)C、比较相对数(正确答案)D、强度相对数(正确答案)E、动态相对数(正确答案)2、专门调查包括() *A、统计报表B、重点调查(正确答案)C、典型调查(正确答案)D、普查(正确答案)E、抽样调查(正确答案)3、下列是连续型变量的是() *A、存款余额(正确答案)B、产值(正确答案)C、销售额(正确答案)D、学生人数E、企业数4、抽样推断中的抽样误差() *A、是不可避免要产生的(正确答案)B、可以事先计算(正确答案)C、可以通过不同的抽样方式消除D、误差范围可事先控制(正确答案)E、只能事后进行计算5、下列现象存在负相关关系的有() *A、商品的消费量(y)与居民收入(x)之间的关系B、商品的消费量(y)与物价(x)之间的关系(正确答案)C、商品销售额(y)与广告费支出(x)之间的关系D、收入水平(y)与受教育程度(x)之间的关系E、产品单位成本(y)与产量(x)之间的关系(正确答案)6、下列属于时期指标的有() *A、销售额(正确答案)B、人口出生数(正确答案)C、库存额D、职工人数E、工业总产值(正确答案)7、一个完整的指标具有() *A、指标名称(正确答案)B、指标所属时间(正确答案)C、一定的地点(正确答案)D、指标的数值(正确答案)E、计量单位(正确答案)8、相对指标有() *A、强度相对指标(正确答案)B、计划完成程度相对指标(正确答案)C、比较相对指标(正确答案)D、结构相对指标(正确答案)E、中位数9、标志变异指标有() *A、全距(正确答案)B、平均差(正确答案)C标准差(正确答案)D、标准差系数(正确答案)E、众数10、下列属于相关关系的有() *A、农作物收获量与施肥量之间的关系(正确答案)B、家庭收入与支出之间的关系(正确答案)C、圆面积与半径之间的关系D、身高与体重之间的关系(正确答案)E、产品单位成本与产量之间的关系(正确答案)三、判断题(每小题2分,15小题共30分)1、数量指标的表现形式是绝对数,质量指标的表现形式是相对数和平均数。
安德森《商务与经济统计》(第10版)(上册)课后习题详解(离散型概率分布)
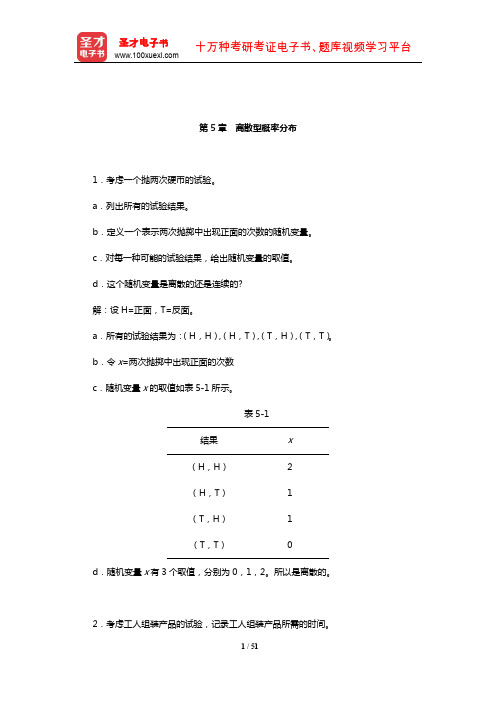
a.所有的试验结果为:(H,H),(H,T),(T,H),(T,T)。
b.令 x=两次抛掷中出现正面的次数
c.随机变量 x 的取值如表 5-1 所示。
表 5-1
结果
x
(H,H)
2
(H,T)
1
(T,H取值,分别为 0,1,2。所以是离散的。
2.考虑工人组装产品的试验,记录工人组装产品所需的时间。
表 5-4
3 / 51
圣才电子书 十万种考研考证电子书、题库视频学习平台
解:a.回答正确的问题数的取值为:0,1,2,….,20。所以随机变量是离散的。 b.到达收费站的汽车数的取值为:0,1,2,….。所以随机变量是离散的。 c.出现错误的报告数的取值为:0,1,2,….,50。所以随机变量是离散的。
表 5-2 试(Y,Y,Y)(Y,Y,N)(Y,N,Y)(Y,N,N)(N,Y,Y)(N,Y,N)(N,N,Y)(N,N,N) 验 结 果
2 / 51
圣才电子书 十万种考研考证电子书、题库视频学习平台
N3
2
2
1
2
1
1
0
4.已知佛罗里达州的 12 个租借机构的房屋抵押率。令随机变量表示这些租借机构中 提供 30 年固定利率为 8.5%或更少的机构的个数。试问,这个随机变量可以取什么值?
4 / 51
圣才电子书 十万种考研考证电子书、题库视频学习平台
d.当 x>30 时,f(35)=0.40,即 x>30 的概率是 0.40。
8.以下是坦帕总医院(Tampa General Hospital)20 天内手术室的使用情况:有 3 天只使用 1 间,有 5 天使用 2 间,有 8 天使用 3 间,有 4 天医院的 4 问手术室都被使用。
商务统计学(第四版)课后习题答案第六章
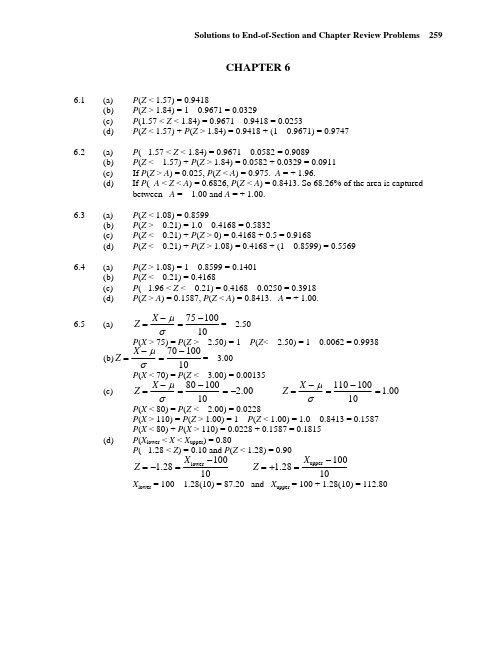
(b) P(X > 50) = P(Z > 1.384) = 0.0832
(c) P(30 < X < 40) = P(–0.616 < Z < 0.384) = 0.3806
(d) P(X < A) = 0.99
A − 36.16
Z = 2.3263 =
10
A = $59.42
Note: The above answers are obtained using PHStat. They may be slightly different when
6.5 (a)
Z
=
X– σ
µ
=
75 –100
= – 2.50
10
P(X > 75) = P(Z > – 2.50) = 1 – P(Z< – 2.50) = 1 – 0.0062 = 0.9938
(b) Z
=
X– σ
µ
=
70 – 100
=
10
– 3.00
P(X < 70) = P(Z < – 3.00) = 0.00135
(a) P(21.9 < X < 22.00) = P(– 25.5 < Z < – 0.5) = 0.3085
(b) P(21.9 < X < 22.01) = P(– 25.5 < Z < 2) = 0.9772
(c) P(X > A) = 0.02
Z = 2.05
A = 22.0102
6.14 With 39 observations, the smallest of the standard normal quantile values covers an area under the normal curve of 0.025. The corresponding Z-value is – 1.96. The largest of the standard normal quantile values covers an area under the normal curve of 0.975 and its corresponding Z-value is +1.96.
商务统计试题7参考答案及评分标准
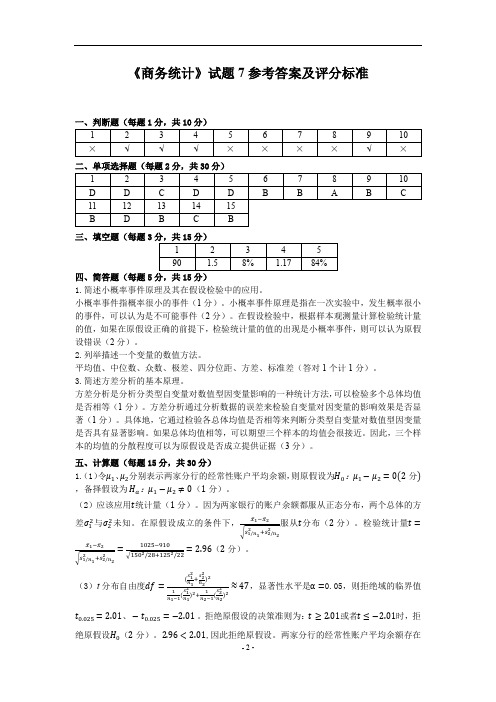
《商务统计》试题7参考答案及评分标准一、判断题(每题1分,共10分)三、填空题(每题3四、简答题(每题5分,共15分)1.简述小概率事件原理及其在假设检验中的应用。
小概率事件指概率很小的事件(1分)。
小概率事件原理是指在一次实验中,发生概率很小的事件,可以认为是不可能事件(2分)。
在假设检验中,根据样本观测量计算检验统计量的值,如果在原假设正确的前提下,检验统计量的值的出现是小概率事件,则可以认为原假设错误(2分)。
2.列举描述一个变量的数值方法。
平均值、中位数、众数、极差、四分位距、方差、标准差(答对1个计1分)。
3.简述方差分析的基本原理。
方差分析是分析分类型自变量对数值型因变量影响的一种统计方法,可以检验多个总体均值是否相等(1分)。
方差分析通过分析数据的误差来检验自变量对因变量的影响效果是否显著(1分)。
具体地,它通过检验各总体均值是否相等来判断分类型自变量对数值型因变量是否具有显著影响。
如果总体均值相等,可以期望三个样本的均值会很接近。
因此,三个样本的均值的分散程度可以为原假设是否成立提供证据(3分)。
五、计算题(每题15分,共30分)1.(1)令μ1、μ2分别表示两家分行的经常性账户平均余额,则原假设为H0:μ1−μ2=0(2分),备择假设为H a:μ1−μ2≠0(1分)。
(2)应该应用t统计量(1分)。
因为两家银行的账户余额都服从正态分布,两个总体的方差σ12与σ22未知。
在原假设成立的条件下,12√s1/n1+s2/n2t分布(2分)。
检验统计量t=12√s1/n1+s2/n2=√1502/28+1252/22=2.96(2分)。
(3)t分布自由度df=(s12n1+s22n2)21n1−1(s12n1)2+1n2−1(s22n2)2≈47,显著性水平是α=0.05,则拒绝域的临界值t0.025=2.01、−t0.025=−2.01 。
拒绝原假设的决策准则为:t≥2.01或者t≤−2.01时,拒绝原假设H0(2分)。
商务与经济统计习题答案(第8版,中文版)
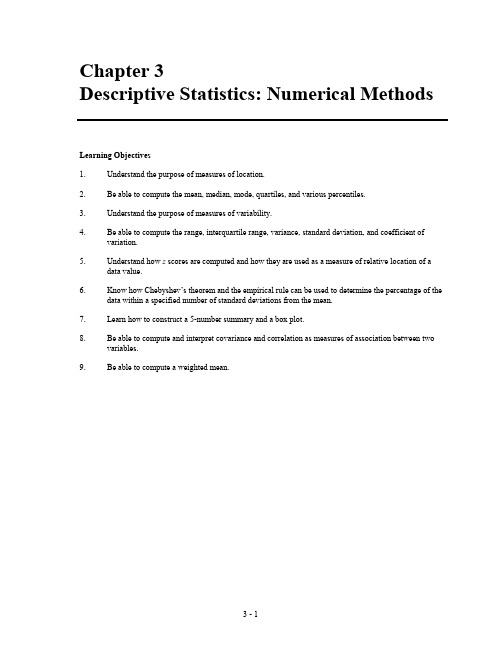
Mode: 15.3
Country
17.2 17.4 18.3 18.5 18.6 18.6 18.7 19.0 19.2 19.4 19.4 20.6 21.1
Median
Mode:18.6, 19.4
The median and modal mileages are also better in the country than in the city.
9.Be able to compute a weighted mean.
Solutions:
1.
10, 12, 16, 17, 20
Median = 16 (middle value)
2.
10, 12, 16, 17, 20, 21
Median =
3.15, 20, 25, 25, 27, 28, 30, 32
For the samples we see that the mean mileage is betterin the country than in the city.
City
13.2 14.4 15.2 15.3 15.3 15.3 15.9 16 16.1 16.2 16.2 16.7 16.8
b.
Q1(3rd position) = 45
Q3(7th position) = 55
11.
Median = 25
Do not report a mode since five values appear twice.
ForQ1,
ForQ3,
ing the mean we get =15.58, = 18.92
3.Understand the purpose of measures of variability.
商务统计学(第四版)课后习题答案第八章
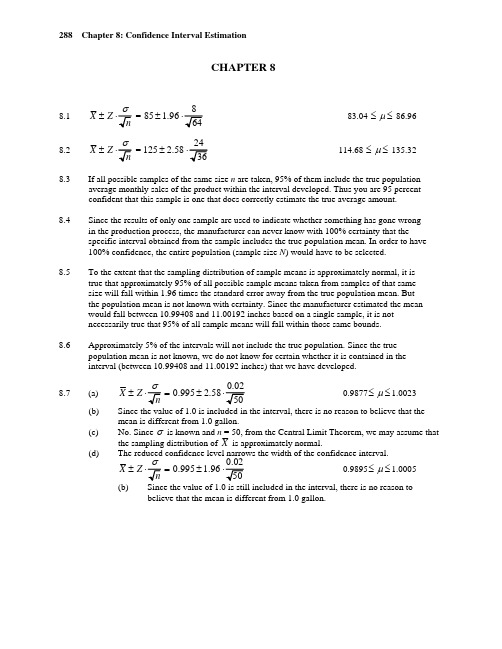
CHAPTER 88.1 X ±Z ⋅σn= 85±1.96⋅864 83.04 ≤µ≤ 86.968.2 X ±Z ⋅σn= 125±2.58⋅2436114.68 ≤µ≤ 135.328.3 If all possible samples of the same size n are taken, 95% of them include the true population average monthly sales of the product within the interval developed. Thus you are 95 percent confident that this sample is one that does correctly estimate the true average amount. 8.4Since the results of only one sample are used to indicate whether something has gone wrong in the production process, the manufacturer can never know with 100% certainty that the specific interval obtained from the sample includes the true population mean. In order to have 100% confidence, the entire population (sample size N ) would have to be selected.8.5To the extent that the sampling distribution of sample means is approximately normal, it is true that approximately 95% of all possible sample means taken from samples of that same size will fall within 1.96 times the standard error away from the true population mean. But the population mean is not known with certainty. Since the manufacturer estimated the mean would fall between 10.99408 and 11.00192 inches based on a single sample, it is not necessarily true that 95% of all sample means will fall within those same bounds. 8.6 Approximately 5% of the intervals will not include the true population. Since the true population mean is not known, we do not know for certain whether it is contained in the interval (between 10.99408 and 11.00192 inches) that we have developed.8.7 (a) X ±Z ⋅σn=0.995±2.58⋅0.02500.9877≤µ≤1.0023(b) Since the value of 1.0 is included in the interval, there is no reason to believe that the mean is different from 1.0 gallon.(c) No. Since σ is known and n = 50, from the Central Limit Theorem, we may assume that the sampling distribution of X is approximately normal.(d) The reduced confidence level narrows the width of the confidence interval.X ±Z ⋅σn=0.995±1.96⋅0.02500.9895≤µ≤1.0005(b)Since the value of 1.0 is still included in the interval, there is no reason to believe that the mean is different from 1.0 gallon.8.8 (a)350 1.96X Z ±=± 325.5≤µ≤374.50(b) No. The manufacturer cannot support a claim that the bulbs last an average 400 hours. Based on the data from the sample, a mean of 400 hours would represent a distance of 4 standard deviations above the sample mean of 350 hours.(c)No. Since σ is known and n = 64, from the Central Limit Theorem, we may assume that the sampling distribution of X is approximately normal.(d) The confidence interval is narrower based on a process standard deviation of 80hours rather than the original assumption of 100 hours.(a)X ±Z ⋅σn=350±1.96⋅8064330.4≤µ≤369.6(b)Based on the smaller standard deviation, a mean of 400 hours wouldrepresent a distance of 5 standard deviations above the sample mean of 350 hours. No, the manufacturer cannot support a claim that the bulbs have a mean life of 400 hours.8.9 (a) X ±Z ⋅σn=1.99±1.96⋅0.051001.9802≤µ≤1.9998 (b) No. Since σ is known and n = 100, from the central limit theorem, we may assume thatthe sampling distribution of X is approximately normal.(c) An individual value of 2.02 is only 0.60 standard deviation above the sample mean of 1.99. The confidence interval represents bounds on the estimate of the average of a sample of 100, not an individual value.(d)A shift of 0.02 units in the sample average shifts the confidence interval by the same distance without affecting the width of the resulting interval.(a)X ±Z ⋅σn=1.97±1.96⋅0.051001.9602≤µ≤1.97988.10 (a) t 9 = 2.2622 (b) t 9 = 3.2498 (c) t 31 = 2.0395 (d) t 64 = 1.9977 (e) t 15 = 1.75318.11 75 2.0301X t ±=± 66.8796 ≤µ≤ 83.1204 8.12 50 2.9467X t ±=± 38.9499 ≤µ≤ 61.05018.13 Set 1: 4.5±2.3646⋅3.74178 1.3719 ≤µ≤ 7.6281 Set 2: 4.5±2.3646⋅2.449582.4522 ≤µ≤ 6.5478The data sets have different confidence interval widths because they have different values forthe standard deviation.8.14 Original data:5.8571±2.4469⋅6.46607 – 0.1229 ≤µ≤ 11.8371 Altered data: 4.00±2.4469⋅2.160272.0022 ≤µ≤ 5.9978The presence of an outlier in the original data increases the value of the sample mean and greatly inflates the sample standard deviation.8.15 (a) 1.67 2.0930X t ±=± $1.52 ≤µ≤ $1.82(b)The store owner can be 95% confident that the population mean retail value ofgreeting cards that the store has in its inventory is somewhere in between $1.52 and $1.82. The store owner could multiply the ends of the confidence interval by the number of cards to estimate the total value of her inventory.8.16 (a) 32 2.0096X t ±=± 29.44 ≤µ≤ 34.56 (b) The quality improvement team can be 95% confident that the population meanturnaround time is somewhere in between 29.44 hours and 34.56 hours.(c)The project was a success because the initial turnaround time of 68 hours does not fall inside the 95% confidence interval.8.17 (a) 195.3 2.1098X t ±=±≤µ≤ 205.9419 (b)No, a grade of 200 is in the interval.(c) It is not unusual. A tread-wear index of 210 for a particular tire is only 0.69 standard deviation above the sample mean of 195.3.8.18 (a) 23 2.0739X t ±=±≤µ≤ $24.99 (b) You can be 95% confident that the mean bounced check fee for the population issomewhere between $21.01 and $24.99.8.19 (a)7.1538 2.0595X t ±=±≤µ≤ $8.39 (b)You can be 95% confident that the population mean monthly service fee, if acustomer’s account falls below the minimum required balance, is somewhere between $5.92 and $8.39.8.20 (a)43.04 2.0096X t ±=±≤µ≤ 54.96 (b) The population distribution needs to be normally distribution.(c)Normal Probability Plot-3-2-10123Z ValueDa y sBox-and-whisker Plot050100150Both the normal probability plot and the box-and-whisker plot suggest that the distribution is skewed to the right.(d)Even though the population distribution is not normally distributed, with a sample of 50, the t distribution can still be used due to the Central Limit Theorem.8.21 (a)43.8889 2.0555X t ±=± 33.89 ≤µ≤ 53.89 (b) The population distribution needs to be normally distributed.(c)Box-and-whisker Plot1030507090Normal Probability Plot0102030405060708090100-2-1.5-1-0.50.511.52Z ValueT i m eBoth the normal probability plot and the box-and-whisker show that the population distribution is not normally distributed and is skewed to the right.8.22 (a)182.4 2.0930X t ±=± $161.68 ≤µ≤ $203.12(b) 45 2.0930X t ±=± $40.31 ≤µ≤ $49.69(c)The population distribution needs to be normally distributed.8.22 (d) cont.Normal Probability Plot050100150200250300-2-1.5-1-0.50.511.52Z ValueH o t e lBox-and-whisker Plot110160210260310Both the normal probability plot and the box-and-whisker show that the population distribution for hotel cost is not normally distributed and is skewed to the right.8.22 (d) cont.Normal Probability Plot010********607080-2-1.5-1-0.500.511.52Z ValueC a r sBox-and-whisker Plot3040506070Both the normal probability plot and the box-and-whisker show that the population distribution for rental car cost is not normally distributed and is skewed to the right.8.23 (a)0.00023 1.9842X t ±=−± –0.000566 ≤µ≤ 0.000106(b)The population distribution needs to be normally distributed. However, with a sampleof 100, the t distribution can still be used as a result of the Central Limit Theorem even if the population distribution is not normal.(c)Both the normal probability plot and the box-and-whisker plot suggest that the distribution is skewed to the right.(d)We are 95% confident that the mean difference between the actual length of the steel part and the specified length of the steel part is between -0.000566 and 0.000106 inches, which is narrower than the plus or minus 0.005 inches requirement. The steel mill is doing a good job at meeting the requirement. This is consistent with the finding in Problem 2.23.Normal Probability Plot-0.004-0.003-0.002-0.00100.0010.0020.0030.0040.0050.006Z ValueE r r orBox-and-whisker Plot-0.006-0.004-0.00200.0020.0040.0068.2450200X p n === 0.25 0.25p Z ±=±0.19 π≤≤ 0.318.2525400X p n === 0.0625 0.0625p Z ±=± 0.0313 0.09378.26 (a)135500X p n === 0.27 0.27p Z ±=±0.22π≤≤ 0.32(b)The manager in charge of promotional programs concerning residential customers can infer that the proportion of households that would purchase an additionaltelephone line if it were made available at a substantially reduced installation cost is between 0.22 and 0.32 with a 99% level of confidence.8.27 (a) 330500X p n === 0.66 0.66p Z ±=± 0.62 π≤≤ 0.70(b) You can be 95% confident that the population proportion of highly educated womenwho left careers for family reasons that want to return to work is between 0.62 and 0.70.8.28 (a) p = 0.77 0.77p Z ±=±0.74 π≤≤ 0.80(b) p = 0.77 0.77p Z ±=± 0.75 π≤≤ 0.79(c) The 95% confidence interval is wider. The loss in precision reflected as a widerconfidence interval is the price you have to pay to achieve a higher level of confidence.8.29 (a) p = 0.27 0.27p Z ±=±0.25 π≤≤ 0.29 (b)You can be 95% confident that the population proportion of older consumers that don’t think they have enough time to be good money managers is somewhere between 0.25 and 0.29.8.30 (a)0.46Xp n== 0.46p Z ±=±0.41630.5037π<<(b) 0.10Xp n== 0.10p Z ±=±0.07370.1263π<<8.31 (a) p = 0.2697 0.2697p Z ±=±0.24 π≤≤ 0.30(b) p = 0.2697 0.2697p Z ±=±0.23π≤≤ 0.31 (c)The 99% confidence interval is wider. The loss in precision reflected as a widerconfidence interval is the price one has to pay to achieve a higher level of confidence.8.32 (a) 4500.451000X p n ===0.45p Z ±=±0.41920.4808π<<(b) You are 95% confidence that the proportion of all working women in North America who believe that companies should hold positions for those on maternity leave for more than six months is between 0.4192 and 0.4808.298 Chapter 8: Confidence Interval Estimation8.33 (a)1260.21600X p n === 0.21p Z ±=±0.18π≤≤ 0.24(b)1260.21600X p n === 0.21p Z ±=±0.17π≤≤ 0.25 (c) You are 95% confidence that the population proportion of employers who have amandatory mail order program in place or are adopting one by the end of 2004 is between 0.18 and 0.24.You are 99% confidence that the population proportion of employers who have amandatory mail order program in place or are adopting one by the end of 2004 is between 0.17 and 0.25.(d)When the level of confidence is increased, the confidence interval becomes wider. The loss in precision reflected as a wider confidence interval is the price youhave to pay to achieve a higher level of confidence.8.34 n =Z 2σ2e 2=1.962⋅15252= 34.57 Use n = 358.35 n =Z 2σ2e 2=2.582⋅1002202= 166.41 Use n = 1678.36 2222(1–) 2.58(0.5)(0.5)(0.04)Z n e ππ== = 1,040.06Use n = 1,0418.37 2222(1–) 1.96(0.4)(0.6)(0.02)Z n e ππ== = 2,304.96Use n = 2,3058.38 (a) n =Z 2σ2e 2=1.962⋅4002502= 245.86 Use n = 246 (b) 2222221.9640025Z n eσ⋅== = 983.41 Use n = 9848.39 n =Z 2σ2e 2=1.962⋅(0.02)2(0.004)2= 96.04 Use n = 978.40 n =Z 2σ2e 2=1.962⋅(100)2(20)2= 96.04 Use n = 978.41 n =Z 2σ2e 2=1.962⋅(0.05)2(0.01)2= 96.04 Use n = 97Solutions to End-of-Section and Chapter Review Problems 2998.42 (a) n =Z 2σ2e 2=2.582⋅25252= 166.41 Use n = 167 (b) n =Z 2σ2e 2=1.962⋅25252= 96.04 Use n = 978.43 (a) n =Z 2σ2e 2=1.6452⋅45252= 219.19 Use n = 220 (b) n =Z 2σ2e 2=2.582⋅45252= 539.17 Use n = 5408.44 n =Z 2σ2e 2=1.962⋅20252= 61.47 Use n = 628.45 (a) 2222(1–) 1.96(0.1577)(10.1577)(0.06)Z n e ππ−== = 141.74 Use n = 142 (b) 2222(1–) 1.96(0.1577)(10.1577)(0.04)Z n e ππ−== = 318.91 Use n = 319 (c) 2222(1–) 1.96(0.1577)(10.1577)(0.02)Z n eππ−== = 1275.66 Use n = 12768.46 (a) 2222(1) 1.96(0.45)(0.55)(0.02)Z n e ππ−== = 2376.8956 Use n = 2377 (b) 2222(1) 1.96(0.29)(0.71)(0.02)Z n eππ−== = 1977.3851 Use n = 1978(c) The sample sizes differ because the estimated population proportions are different.(d)Since purchasing groceries at wholesale clubs and purchasing groceries at convenience stores are not necessary mutually exclusive events, it is appropriate to use one sample and ask the respondents both questions. However, drawing two separate samples is also appropriate for the setup.8.47 (a) 2222(1) 1.96(0.35)(10.35)546.2068(0.04)Z n eππ−−=== Use n = 547 (b) ()22222.5758(0.35)(10.35)(1)943.4009(0.04)Z n e ππ−−=== Use n = 944(c)2222(1) 1.96(0.35)(10.35)2184.8270(0.02)Z n e ππ−−=== Use n = 2185 (d) ()22222.5758(0.35)(10.35)(1)3773.6034(0.02)Z n e ππ−−=== Use n = 3774(e)Holding everything else constant, the higher the confidence level desired or the lowerthe acceptable sampling error, the larger the sample size needed.300 Chapter 8: Confidence Interval Estimation8.48 (a) 3030.9294326X p n ===0.9294 1.96p Z ±=±0.90170.9572π≤≤(b)You are 95% confident that the population proportion of business men and women whohave their presentations disturbed by cell phones is between 0.9017 and 0.9572.(c) 2222(1) 1.96(0.9294)(10.9294)157.5372(0.04)Z n e ππ−−=== Use n = 158(d)2222(1) 2.5758(0.9294)(10.9294)272.0960(0.04)Z n e ππ−−=== Use n = 2738.49 (a) 0.52 1.96p Z ±=±0.50530.5347π≤≤(b)You are 95% confident that the proportion of families that held stocks in 2001 isbetween 0.5053 and 0.5347.(c) 2222(1) 1.96(0.52)(10.52)9588.2695(0.01)Z n e ππ−−=== Use n = 95898.50The only way to have 100% confidence is to obtain the parameter of interest, rather than a sample statistic. From another perspective, the range of the normal and t distribution is infinite, so a Z or t value that contains 100% of the area cannot be obtained.8.51 The t distribution is used for obtaining a confidence interval for the mean when σ isunknown. 8.52 If the confidence level is increased, a greater area under the normal or t distribution needs to be included. This leads to an increased value of Z or t , and thus a wider interval.8.53When estimating the rate of noncompliance, it is commonplace to use a one-sided confidence interval instead of a two-sided confidence interval since only the upper bound on the rate of noncompliance is of interest.8.54 In some applications such as auditing, interest is primarily on the total amount of a variable rather than the mean amount.8.55 Difference estimation involves determining the difference between two amounts, rather than a single amount.Solutions to End-of-Section and Chapter Review Problems 3018.56 (a) The population from which this sample was drawn was the collection of all the people who visited the magazine's web site.(b) The sample is not a random sample from this population. The sample consisted of only those who visited the magazine's web site and chose to fill out the survey. (c)This is not a statistically valid study. There was selection bias since only those who visited the magazine's web site and chose to answer the survey were represented. There was possibly nonresponse bias as well. Visitors to the web site who chose to fill out the survey might not answer all questions and there was no way for the magazine to get back to them to follow-up on the nonresponses if this was an anonymous survey.(d)To avoid the above potential pitfalls, the magazine could have drawn a random sample from t he list of all subscribers to the magazine and offer them the option of filling out the survey over the Internet or on the survey form that is mailed to the subscribers. The magazine should also keep track of the subscribers who are invited to fill out the survey and follow up on the nonresponses after a specified period of time with mail or telephone to encourage them to participate in the survey.The sample size needed is 2222(1) 1.96(0.6195)(10.6195)2264(0.02)Z n e ππ⋅⋅−⋅⋅−===8.57 (a) 0.44 1.96p Z ±=± 0.39650.4835π<<(b)Since the range that covers 0.5 and above is not contained in the 95% confidenceinterval, we can conclude that less than half of all applications contain inaccuracies with past employers.(c)0.44 1.96p Z ±=± 0.37120.5088π<<(d) Since the 95% confidence interval contains 0.5, it is incorrect to conclude that lessthan half of all applications contain inaccuracies with past employers.(e)The smaller sample size in (c) results in a wider confidence interval and, hence, results in a loss of precision in the interval estimate.8.58 (a) 0.58 1.96p Z ±=±0.51160.6484π<<(b) 0.50 1.96p Z ±=±0.43070.5693π<<(c) 0.22 1.96p Z ±=±0.16260.2774π<<(d)0.19 1.96p Z ±=±0.13560.2444π<<(e) 2222(1) 1.96(0.5)(0.5)2400.92401(0.02)Z n eππ⋅⋅−⋅⋅===≅302 Chapter 8: Confidence Interval Estimation8.59 Cheat at golf:0.8204 1.96p Z ±=± 0.78290.8580π<<Hate others who cheat at golf:0.8204 1.96p Z ±=± 0.78290.8580π<<Believe business and golf behavior parallel:0.7207 1.96p Z ±=± 0.67680.7646π<<Would let a client win to get business:0.1995 1.96p Z ±=± 0.16040.2386π<<Would call in sick to play golf:0.0998 1.96p Z ±=±0.07040.1291π<<From these confidence intervals, we can conclude with 95% level of confidence that more thanhalf of the CEOs will cheat at golf, hate others who cheat at golf and believe business and golf behavior parallel and less than half of the CEOs will let a client win to get business or call in sick to play golf.8.60 (a) 15.3 2.0227X t ±=± 14.085 ≤µ≤ 16.515(b)0.675 1.96p Z ±=± 0.530 π≤≤ 0.820 (c) n =Z 2⋅σ2e 2=1.962⋅5222= 24.01 Use n = 25(d) 2222(1–) 1.96(0.5)(0.5)(0.035)Z n eππ⋅⋅⋅⋅== = 784 Use n = 784(e)If a single sample were to be selected for both purposes, the larger of the two samplesizes (n = 784) should be used.8.61 (a) 1759 2.6490X t ±=± 1,638.69 ≤µ≤ 1,879.31(b)0.60 1.96p Z ±=±π≤≤ 0.715Solutions to End-of-Section and Chapter Review Problems 3038.62 (a) 9.7 2.0639X t ±=± 8.049 ≤µ≤ 11.351(b)0.48 1.96p Z ±=±π≤≤ 0.676 (c) n =Z ⋅e 2=1.962⋅4.521.52= 34.57 Use n = 35 (d) 2222(1–) 1.645(0.5)(0.5)(0.075)Z n e ππ⋅⋅⋅⋅== = 120.268 Use n = 121 (e)If a single sample were to be selected for both purposes, the larger of the two samplesizes (n = 121) should be used.8.63 (a) n =Z 2⋅σ2e 2=2.582⋅18252= 86.27Use n = 87Note : If the Z -value used is carried out to 2.5758, the value of n is 85.986 and only 86 women would need to be sampled.(b)2222(1–) 1.645(0.5)(0.5)(0.045)Z n e ππ⋅⋅⋅⋅== = 334.07 Use n = 335(c)If a single sample were to be selected for both purposes, the larger of the two samplesizes (n = 335) should be used.8.64 (a) $28.52 1.9949X t ±=± $25.80 ≤µ≤ $31.24(b)0.40 1.645p Z ±=±π≤≤ 0.4963 (c) n =Z ⋅σe 2=1.962⋅10222= 96.04 Use n = 97 (d) 2222(1–) 1.645(0.5)(0.5)(0.04)Z n e ππ⋅⋅⋅⋅== = 422.82 Use n = 423 (e) If a single sample were to be selected for both purposes, the larger of the two samplesizes (n = 423) should be used.8.65 (a) $21.34 1.9949X t ±=± $19.14 ≤µ≤ $23.54(b) 0.3714 1.645p Z ±=±0.2764 π≤≤ 0.4664(c)n =Z 2⋅σ2e 2=1.962⋅1021.52= 170.74 Use n = 171 (d)2222(1–) 1.645(0.5)(0.5)(0.045)Z n e ππ⋅⋅⋅⋅== = 334.08 Use n = 335304 Chapter 8: Confidence Interval Estimation8.65 (e) If a single sample were to be selected for both purposes, the larger of the two sample cont. sizes (n = 335) should be used.8.66 (a)$38.54 2.0010X t ±=±≤µ≤ $40.42(b) 0.30 1.645p Z ±=±π≤≤ 0.3973 (c) n =Z 2⋅σ2e 2=1.962⋅821.52= 109.27 Use n = 110 (d) 2222(1–) 1.645(0.5)(0.5)(0.04)Z n e ππ⋅⋅⋅⋅== = 422.82 Use n = 423 (e)If a single sample were to be selected for both purposes, the larger of the two samplesizes (n = 423) should be used.8.67 (a) 2222(1) 1.96(0.5)(0.5)(0.05)Z n eππ⋅⋅−⋅⋅== = 384.16 Use n = 385If we assume that the population proportion is only 0.50, then a sample of 385 wouldbe required. If the population proportion is 0.90, the sample size required is cut to 103.(b) 0.84 1.96p Z ±=± 0.7384≤ π ≤ 0.9416 (c) The representative can be 95% confidence that the actual proportion of bags that will dothe job is between 74.5% and 93.5%. He/she can accordingly perform a cost-benefit analysis to decide if he/she wants to sell the Ice Melt product.8.68 (a) 8.4209 2.0106X t ±=± 8.418.43µ≤≤(b) With 95% confidence, the population mean width of troughs is somewhere between 8.41and 8.43 inches. Hence, the company's requirement of troughs being between 8.31 and 8.61 is being met with a 95% level of confidence.8.69 (a) 5.5014 2.6800X t ±=± 5.46 5.54µ≤≤(b) Since 5.5 grams is within the 99% confidence interval, the company can claim that themean weight of tea in a bag is 5.5 grams with a 99% level of confidence.Solutions to End-of-Section and Chapter Review Problems 3058.70 (a)0.2641 1.9741X t ±=±0.24250.2856µ≤≤(b)0.218 1.9772X t ±=±0.19750.2385µ≤≤(c)Normal Probability Plot00.10.20.30.40.50.60.70.80.9-3-2-1123Z ValueV e r m o n tNormal Probability Plot00.20.40.60.811.2-3-2-1123Z ValueB o s t o nThe amount of granule loss for both brands are skewed to the right.(d)Since the two confidence intervals do not overlap, we can conclude that the mean granule loss of Boston shingles is higher than that of Vermont Shingles.306 Chapter 8: Confidence Interval Estimation8.71 (a)3124.2147 1.9665X t ±=±3120.663127.77µ≤≤(b)3704.0424 1.9672X t ±=± 3698.98<3709.10µ<(c)Normal Probability Plot3000305031003150320032503300-4-3-2-11234Z ValueB o s t o nNormal Probability Plot35503600365037003750380038503900-4-3-2-11234Z ValueV e r m o n tThe weight for Boston shingles is slightly skewed to the right while the weight for Vermont shingles appears to be slightly skewed to the left.(d)Since the two confidence intervals do not overlap, the mean weight of Vermont shingles is greater than the mean weight of Boston shingles.Solutions to End-of-Section and Chapter Review Problems 3078.72(a)NY, Food: 20.1 2.0096X t ±=± 19.5120.69µ≤≤ LI, 20.54 2.0096X t ±=± 19.7321.35µ≤≤ NY, 17.12 2.0096X t ±=± 16.3517.89µ≤≤ LI, 17.64 2.0096X t ±=± 16.6518.63µ≤≤ NY, 18.4 2.0096X t ±=± 17.7419.06µ≤≤ LI, 19.04 2.0096X t ±=±18.3719.71µ≤≤NY, 39.74 2.0096X t ±=± 37.0042.48µ≤≤ LI, 33.7400 2.0096X t ±=± 31.5535.93µ≤≤(b)With 95% confidence you can conclude the mean price per person in New York City isgreater than the mean in Long Island. With 95% confidence you can conclude that there are no differences in the ratings for food, décor and service between the two cities.。
安德森《商务与经济统计》(第10版)(上册)课后习题详解(描述统计Ⅰ:表格法和图形法)

6 / 90
圣才电子书 十万种考研考证电子书、题库视频学习平台
构建下列项目汇总数据:
a.相对频数分布和百分数频数分布。
b.构建条形图。
c.构建饼形图。
d.根据这些数据,最常见的 3 个姓氏是哪些?
解:a.制作频数分布表,如表 2-7 所示。
表 2-7 频数分布表
8 / 90
圣才电子书 十万种考研考证电子书、题库视频学习平台
前 50 名的单个节目的名单(The New York Times Almanac,2006)。表 2-8 是制作这些 收视率前 50 名的电视节目的电视网的数据。
pA =60/120=0.5, pB =24/120=0.2, pC =36/120=0.3
制作频数分布和相对频数分布,如表2-1所示。
表2-1 频数、相对频数分布表
组别
频数
相对频数
A
60
0.5
B
24
0.2
C
36
0.3
合计
120
1
2.表 2-2 给出了一部分相对频数分布。
表 2-2 部分相对频数分布表
b.若样本容量为 200,则组别 D 的频数为:200×0.20=40。
c.若样本容量为 200,则组别 A、B、C 的频数分别为:200×0.22=44,200×0.18=36,
200×0.40=80。制作频数分布表,如表 2-3 第 2 列所示。
d.组别 A、B、C、D 的百分数频数分别为:100×0.22=22,100×0.18=18,100×0.40=40,
表 2-4 50 名电视观众偏爱数据
a.这些数据是品质型的还是数值型的? b.给出这些数据的频数分布和百分数频数分布。 c.画出这些数据的条形图和饼形图。
商务与经济统计课后答案

商务与经济统计课后答案商务与经济统计课后答案【篇一:商务经济统计学复习题】.简答题1.简要谈谈你对统计与统计学的初步认识。
2.谈谈你对统计的三种含义的理解,并举出现实经济生活中你所了解到的运用统计学的一个例子3.试就统计数据的四种类型给出统计整理与显示的方法(统计图要求划出示意图)。
4.概述数据的离散程度的常用的测度方法(异众比率标准差离散系数)。
5.什么是个体指数? 什么是总指数?它们的作用分别是什么?6.试简要说明总量指标、平均指标和相对指标的在统计学中的作用。
7.只能用统计条形图和饼图来展示的是哪种类型的数据?画出这两种图形的示意图。
8.自己用一个实例画出统计条形图和饼图的示意图,它们通常可以用来展示哪种类型的数据?9.某高校毕业生就业指导中心想对2007届本校大学本科毕业生的毕业去向做一网上调查,请你为此设计一份半开放式(即:既含有封闭式问题又含有开放式问题)调查问卷。
(要求涉及学生的性别、专业、意向中的毕业去向:如出国、考研、自主创业、自主择业,以及意向中的就业领域、工薪待遇、单位性质、工作地区等等信息)。
二.填空题1.将下列指标按要求分类(只填写标号即可)(1)我国高等院校2006届本科毕业生就业率;(2)某贺岁片在国内上演第一周的票房收入;(3)2006年第3季度一汽大众销售的某品牌小汽车台数占其全部小汽车销售量的比率;,(5)进藏铁路开通后第一周,每天乘火车前往西藏的旅客的累计人数;(6)第3季度某商场的月平均销售额。
哪些是时点时标;哪些是时期指标;哪些是平均指标;哪些是相对指标。
2.统计调查方式除了重点调查,典型调查之外,另三种主要方式是 3.加权调和平均公式为4.异众比率公式是其含义是5.一组数据中非众数组所占的比率叫做,它可测度分类数据的趋势;离散系数测度的是总体的平均离散程度,它的计算公式是v?=。
6.将下列指标分类:(1)2005年我国人均占有粮食产量(2)我国第五次人口普查总人口数(3)股价指数(4)销售量指数(5)单位产品成本(6)某商店全年销售额(7)某企业在岗职工人数和下岗职工人数的比例 (8)我国高等院校“十五”期间年平均招生人数哪些是时期指标哪些是时点指标;哪些是一般平均数, 哪些是序时平均数;哪些是相对指标7.个体指数是反映项目或变量变动的相对数;反映多种项目或变量变动的相对数是。
商务与经济统计习题答案(第8版,中文版)SBE8-SM20
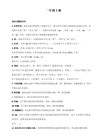
Chapter 20Statistical Methods for Quality ControlLearning Objectives1. Learn about the importance of quality control and how statistical methods can assist in the qualitycontrol process.2. Learn about acceptance sampling procedures.3. Know the difference between consumer’s risk and producer’s risk.4. Be able to use the binomial probability distribution to develop acceptance sampling plans.5. Know what is meant by multiple sampling plans.6. Be able to construct quality control charts and understand how they are used for statistical processcontrol.7. Know the definitions of the following terms:producer's risk assignable causesconsumer's risk common causesacceptance sampling control chartsacceptable criterion upper control limitoperating characteristic curve lower control limitChapter 20Solutions:1. a. For n = 4 UCL = μ + 3(σ / n ) = 12.5 + 3(.8 / 4) = 13.7LCL = μ - 3(σ / n ) = 12.5 - 3(.8 / 4) = 11.3b. For n = 8 UCL = μ + 3(.8 /8) = 13.35LCL = μ - 3(.8 /8) = 11.65For n = 16UCL = μ + 3(.8 /16) = 13.10 LCL = μ - 3(.8 /16) = 11.90c. UCL and LCL become closer together as n increases. If the process is in control, the larger samplesshould have less variance and should fall closer to 12.5.2. a. μ==6775255542.(). b. UCL = μ + 3(σ / n ) = 5.42 + 3(.5 / 5) = 6.09 LCL = μ - 3(σ / n ) = 5.42 - 3(.5 / 5) = 4.753. a.p ==1352510000540().b. σp p p n=-==().(.).1005400946010000226c. UCL = p + 3σp = 0.0540 + 3(0.0226) = 0.1218LCL = p - 3σp = 0.0540 -3(0.0226) = -0.0138Use LCL = 0 4. R Chart: UCL = RD 4= 1.6(1.864) = 2.98 LCL = RD 3= 1.6(0.136) = 0.22x Chart:UCL = 2x A R += 28.5 + 0.373(1.6) = 29.10LCL = x A R -2= 28.5 - 0.373(1.6) = 27.905. a. UCL = μ + 3(σ / n ) = 128.5 + 3(.4 / 6) = 128.99LCL = μ - 3(σ / n ) = 128.5 - 3(.4 / 6) = 128.01Statistical Methods for Quality Controlb.x x n i ===∑/..7724612873 in controlc. x x n i ===∑/..7743612905 out of control6. Process Mean = 2012199022001...+=UCL = μ + 3(σ / n ) = 20.01 + 3(σ / 5) = 20.12Solve for σ: σ=-=(..).201220015300827.Sample NumberObservationsx i R i 1 31 42 28 33.67 14 2 26 18 35 26.33 17 3 25 30 34 29.67 9 4 17 25 21 21.00 8 5 38 29 35 34.00 9 6 41 42 36 39.67 6 7 21 17 29 22.33 12 8 32 26 28 28.67 6 9 41 34 33 36.00 8 10 29 17 30 25.33 13 11 26 31 40 32.33 14 12 23 19 25 22.33 6 13 17 24 32 24.33 15 14 43 35 17 31.67 26 15 18 25 29 24.00 11 16 30 42 31 34.33 12 17 28 36 32 32.00 8 18 40 29 31 33.33 11 19 18 29 28 25.00 11 2022342627.3312R = 11.4 and x =2917.R Chart: UCL = RD 4= 11.4(2.575) = 29.35 LCL = RD 3= 11.4(0) = 0x Chart: UCL = x A R +2= 29.17 + 1.023(11.4) = 40.8 LCL = x A R -2= 29.17 - 1.023(11.4) = 17.5Chapter 20R Chart:x Chart:8. a. p ==1412015000470().b. σp p p n=-==().(.).1004700953015000173UCL = p + 3σp = 0.0470 + 3(0.0173) = 0.0989LCL = p - 3σp = 0.0470 -3(0.0173) = -0.0049Statistical Methods for Quality ControlUse LCL = 0c. p ==12150008.Process should be considered in control.d. p = .047, n = 150 UCL = np + 3np p ()1-= 150(0.047) + 315000470953(.)(.) = 14.826LCL = np - 3np p ()1-= 150(0.047) - 315000470953(.)(.) = -0.726Thus, the process is out of control if more than 14 defective packages are found in a sample of 150.e. Process should be considered to be in control since 12 defective packages were found.f. The np chart may be preferred because a decision can be made by simply counting the number ofdefective packages. 9. a. Total defectives: 165p ==1652020000413().b. σp p p n=-==().(.).1004130958720000141UCL = p + 3σp = 0.0413 + 3(0.0141) = 0.0836LCL = p - 3σp = 0.0413 + 3(0.0141) = -0.0010Use LCL = 0c.p ==20200010. Out of control d. p = .0413, n = 200 UCL = np + 3np p ()1-= 200(0.0413) + 32000041309587(.)(.) = 16.702LCL = np - 3np p ()1-= 200(0.0413) - 32000041309587(.)(.) = 0.1821e. The process is out of control since 20 defective pistons were found.10. f x n x n x p p x n x ()!!()!()=---1When p = .02, the probability of accepting the lot isf ()!)!(.)(.).0250!(250002100206035025=--=Chapter 20When p = .06, the probability of accepting the lot isf()!)!(.)(.).250!(250006100602129025=--=11. a. Using binomial probabilities with n = 20 and p0 = .02.P (Accept lot) = f (0) = .6676Producer’s risk: α = 1 - .6676 = .3324b. P (Accept lot) = f (0) = .2901Producer’s risk: α = 1 - .2901 = .709912. At p0 = .02, the n = 20 and c = 1 plan providesP (Accept lot) = f (0) + f (1) = .6676 + .2725 = .9401Producer’s risk: α = 1 - .9401 = .0599At p0 = .06, the n = 20 and c = 1 plan providesP (Accept lot) = f (0) + f (1) = .2901 + .3703 = .6604Producer’s risk: α = 1 - .6604 = .3396For a given sample size, the producer’s risk decreases as the acce ptance number c is increased.13. a. Using binomial probabilities with n = 20 and p0 = .03.P(Accept lot) = f (0) + f (1)= .5438 + .3364 = .8802Producer’s risk: α = 1 - .8802 = .1198b. With n = 20 and p1 = .15.P(Accept lot) = f (0) + f (1)= .0388 + .1368 = .1756Consumer’s risk: β = .1756c. The consumer’s risk is acceptable; however, the producer’s risk associated with the n = 20, c = 1 plan isa little larger than desired.Statistical Methods for Quality Control14.c P (Accept) p 0 = .05 Producer’s Risk α P (accept) p 1 = .30 Consumer’s Risk β (n = 10)0 .5987 .4013 .0282 .0282 1 .9138 .0862 .1493 .1493 2 .9884 .0116 .3828 .3828 (n = 15)0 .4633 .5367 .0047 .0047 1 .8291 .1709 .0352 .0352 2 .9639 .0361 .1268 .1268 3 .9946 .0054 .2968 .2968 (n = 20)0 .3585 .6415 .0008 .0008 1 .7359 .2641 .0076 .0076 2 .9246 .0754 .0354 .03543.9842.0158.1070.1070The plan with n = 15, c = 2 is close with α = .0361 and β = .1268. However, the plan with n = 20, c = 3 is necessary to meet both requirements.15. a. P (Accept) shown for p values below:c p = .01 p = .05 p = .08 p = .10 p = .15 0 .8179 .3585 .1887 .1216 .0388 1 .9831 .7359 .5169 .3918 .1756 2 .9990 .9246 .7880 .6770 .4049The operating characteristic curves would show the P (Accept) versus p for each value of c . b. P (Accept)c At p 0 = .01 Producer’s Risk At p 1 = .08 Consumer’s Risk 0 .8179 .1821 .1887 .1887 1 .9831 .0169 .5169 .5169 2 .9990 .0010 .7880 .788016. a. μ===∑x 20190820954. b. UCL = μ + 3(σ / n ) = 95.4 + 3(.50 / 5) = 96.07 LCL = μ - 3(σ / n ) = 95.4 - 3(.50 / 5) = 94.73 c. No; all were in control17. a. For n = 10Chapter 20UCL = μ + 3(σ / n ) = 350 + 3(15 / 10) = 364.23LCL = μ - 3(σ / n ) = 350 - 3(15 / 10) = 335.77For n = 20 UCL = 350 + 3(15 / 20) = 360.06LCL = 350 - 3(15 / 20) = 339.94For n = 30 UCL = 350 + 3(15 / 30) = 358.22LCL = 350 - 3(15 / 30) = 343.78b. Both control limits come closer to the process mean as the sample size is increased.c. The process will be declared out of control and adjusted when the process is in control.d. The process will be judged in control and allowed to continue when the process is out of control.e. All have z = 3 where area = .4986 P (Type I) = 1 - 2 (.4986) = .002818. R Chart: UCL = RD 4= 2(2.115) = 4.23LCL = RD 3= 2(0) = 0x Chart:UCL = x A R +2= 5.42 + 0.577(2) = 6.57LCL = x A R -2= 5.42 - 0.577(2) = 4.27Estimate of Standard Deviation:..σ===R d 22232608619. R = 0.665 x = 95.398x Chart:UCL = x A R +2= 95.398 + 0.577(0.665) = 95.782 LCL = x A R -2= 95.398 - 0.577(0.665) = 95.014R Chart: UCL = RD 4= 0.665(2.115) = 1.406LCL = RD 3= 0.665(0) = 0Statistical Methods for Quality ControlThe R chart indicated the process variability is in control. All sample ranges are within the control limits. However, the process mean is out of control. Sample 11 (x = 95.80) and Sample 17 (x =94.82) fall outside the control limits.20. R = .053 x = 3.082x Chart:UCL = x A R +2= 3.082 + 0.577(0.053) = 3.112 LCL = x A R -2= 3.082 - 0.577(0.053) = 3.051R Chart: UCL = RD 4= 0.053(2.115) = 0.1121LCL = RD 3= 0.053(0) = 0All data points are within the control limits for both charts.21.a.LC L U CL.02.04.06.08Warning: Process should be checked. All points are within control limits; however, all points are also greater than the process proportion defective.Chapter 20b.Warning: Process should be checked. All points are within control limits yet the trend in points show a movement or shift toward UCL out-of-control point.22. a. p = .04σp p p n =-==().(.).100409620000139 UCL = p + 3σp = 0.04 + 3(0.0139) = 0.0817LCL = p - 3σp = 0.04 - 3(0.0139) = -0.0017Use LCL = 0b.Statistical Methods for Quality ControlFor month 1 p= 10/200 = 0.05. Other monthly values are .075, .03, .065, .04, and .085. Only the last month with p = 0.085 is an out-of-control situation.23. a. Use binomial probabilities with n = 10.At p0 = .05,P(Accept lot) = f (0) + f (1) + f (2)= .5987 + .3151 + .0746 = .9884Producer’s Risk: α = 1 - .9884 = .0116At p1 = .20,P(Accept lot) = f (0) + f (1) + f (2)= .1074 + .2684 + .3020 = .6778Consumer’s risk: β = .6778b. The consumer’s risk is unacceptably high. Too many bad lots would be accepted.c. Reducing c would help, but increasing the sample size appears to be the best solution.24. a. P (Accept) are shown below: (Using n = 15)p = .01 p = .02 p = .03 p = .04 p = .05f (0) .8601 .7386 .6333 .5421 .4633f (1) .1303 .2261 .2938 .3388 .3658.9904 .9647 .9271 .8809 .8291α = 1 - P (Accept) .0096 .0353 .0729 .1191 .1709Using p0 = .03 since α is close to .075. Thus, .03 is the fraction defective where the producer willtolerate a .075 probability of rejecting a good lot (only .03 defective).b. p = .25f (0) .0134Chapter 20f (1) .0668 β = .080225. a. P (Accept) when n = 25 and c = 0. Use the binomial probability function withf x n x n x p p x n x ()!!()!()=---1orf p p p ()!!()()0250!251102525=-=-If f (0) p = .01 .7778 p = .03 .4670 p = .10 .0718 p = .20 .0038b.c. 1 - f (0) = 1 - .778 = .22226. a. μ = np = 250(.02) = 5 σ=-==np p ()(.)(.).1250002098221 P (Accept) = P (x ≤ 10.5)z =-=1055221249...P (Accept) = .5000 + .4936 = .9936Statistical Methods for Quality Control Producer’s Risk: α = 1 - .9936 = .0064b. μ = np = 250 (.08) = 20σ=-==np p()(.)(.).1250008092429P (Accept) = P (x≤ 10.5)z=-=-1055429221 ...P (Accept) = 1 - .4864 = .0136Consumer’s Risk: β = .0136c. The advantag e is the excellent control over the producer’s and the consumer’s risk. The disadvantage isthe cost of taking a large sample.。
商务数据分析习题+答案
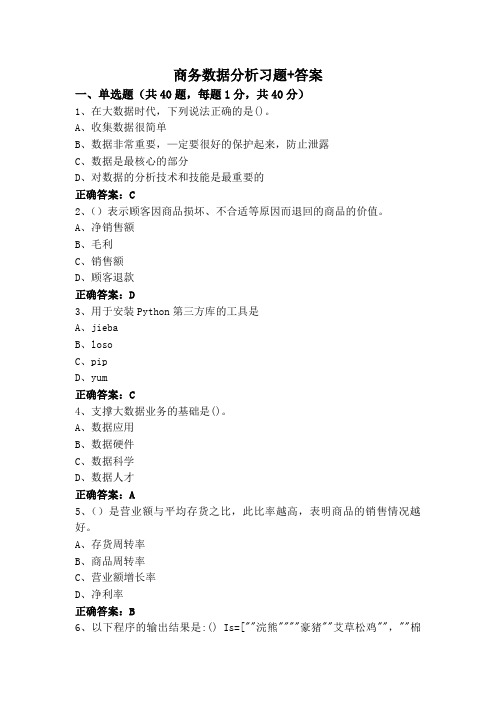
商务数据分析习题+答案一、单选题(共40题,每题1分,共40分)1、在大数据时代,下列说法正确的是()。
A、收集数据很简单B、数据非常重要,—定要很好的保护起来,防止泄露C、数据是最核心的部分D、对数据的分析技术和技能是最重要的正确答案:C2、()表示顾客因商品损坏、不合适等原因而退回的商品的价值。
A、净销售额B、毛利C、销售额D、顾客退款正确答案:D3、用于安装Python第三方库的工具是A、jiebaB、losoC、pipD、yum正确答案:C4、支撑大数据业务的基础是()。
A、数据应用B、数据硬件C、数据科学D、数据人才正确答案:A5、()是营业额与平均存货之比,此比率越高,表明商品的销售情况越好。
A、存货周转率B、商品周转率C、营业额增长率D、净利率正确答案:B6、以下程序的输出结果是:() Is=[""浣熊""""豪猪""艾草松鸡"",""棉尾兔"",""叉角羚""]x=""豪猪"" print(ls.index(x0)A、1B、OC、-4D、-3正确答案:A7、数据分析有很多方法,其中()也叫立体分析法,通常用来分析某两个变量之间的关系,如产品销量和地区的关系。
A、频数分析法B、分组分析法C、交叉分析法D、平均分析法正确答案:C8、货龄其根本是销售者和生产者辨析该产品的()的一个定量指标。
A、生产期B、保质期C、销售期D、制造期正确答案:C9、涉及库存预警原因不包括()业A、指标B、对象C、时间D、地点正确答案:D10、100(1-α)%是( )。
A、置信水平B、置信上限C、置信下限D、置信区间正确答案:A11、在Excel中工作表中单击B1,按住鼠标左键,拖至D4,这个操作选取的区域是A、B1:D6B、B1:C4C、D1:D4D、B1:D4正确答案:D12、若A1单元格中的字符串是“华为”A2单元格的字符串是Mate30”,想要在A3单元格中显示“华为Mate30proSG手机”,则应在A3单元格中键入的公式为0。
商务与经济统计精要版答案

商务与经济统计精要版答案【篇一:经管类书单推荐】与管理学院 2016.10.17管理类推荐读物孙耀君,《西方管理学名著提要》,江西人民出版社1)管理学邢以群,《管理学》,浙江大学出版社周三多,《管理学》,复旦大学出版社2)管理信息系统kenneth udon/ jane udon ,《管理信息系统—网络化企业的组织与技术》(第六版,影印版),高等教育出版社薛华成,《管理信息系统》(第三版),清华大学出版社小威廉d.佩勒尔特 e.杰罗姆.麦卡锡,《市场营销学基础》:全球管理(英文版.第12版)--国际通用mba教材》,机械工业出版社郭毅等,《市场营销学原理》,电子工业出版社malhotra,n.k.著,《市场营销研究应用导向(第3版)》,电子工业出版社4)战略管理项保华,《战略管理——艺术与实务》,华夏出版社斯蒂文斯(英),《战略性思维》,机械工业出版社arthur a. thompson, jr. and a. j. strickland Ⅲ.crafting implementing strategy. 6th ed. richard d. irwin, inc., 1995中文版《战略管理学:概念与案例(英文版.第十版)-- 国际通用mba教材》,机械工业出版社david besanko, david dranove, mark shanley. the economics of strategy. john wiley sons, inc., 1996alan j. rowe; et al.. strategic management: a methodological approach. 4th ed. addison-wesley publishing company, inc., 19945)组织行为学卢盛忠等,《组织行为学:理论与实践》,浙江教育出版社英文版《human resource management: gaining a competitive advantage》,清华大学出版社约翰.m.伊万切维奇,《人力资源管理(英文版.原书第8版)-- 国际通用mba教材》,机械工业出版社luis r. gomez-mejia,david b.balkin,robert l. cardy,《管理人力资源 managing human resources 》(英文版第三版),北京大学出版社、培生教育出版集团7)财务管理8)管理统计david r.anderson dennis j.sweeney thomasa.william,《商务与经济统计(第七版)-- 经济教材译丛》,机械工业出版社david r.anderson;dennis j.sweeney;thomas a.william,《商务与经济统计(英文版.第8版)--21世纪经典原版经济管理教材文库》,机械工业出版9)会计学张启銮等,《会计学――工商管理硕士(mba)系列教材》,大连理工大学出版社cktde p.stickney roman l.weil,《财务会计(英文版第9版) --国际通用mba教材》,机械工业出版社ronald w.hilton,《管理会计(英文版.第三版)-- 国际通用mba教材》,机械工业出版10)西方经济学[美]平狄克等:《微观经济学》,中国人民大学出版社梁小民,《宏观经济学》,中国社会科学出版社梁小民,《西方经济学导论》,北京大学出版社宋承先,《现代西方经济学》(宏观经济学),复旦大学出版社[美] h.克雷格.彼得森等:《管理经济学》,中国人民大学出版社[美]s.charles maurice christopher r.thomas, managerial economics, 机械工业出社,2000年影印版11)运筹学胡运权,《运筹学教程(第二版)》,清华大学出版社蒋绍忠,《运筹学讲义》,浙江大学管理学院12)经济法高程德,《经济法》(第九版),上海人民出版社13)电子商务daniel amor,《电子商务:变革与演进--经济教材译丛》,机械工业出版社14)组织理论[美]达夫特著,李维安译.《组织理论与设计精要》,机械工业出版社[英]bernard burnes.《变革时代的管理》,云南大学出版社15)质量管理刘广第,《质量管理学(第二版)》,清华大学出版社16)生产管理陈荣秋、马士华,生产与运作管理,高等教育出版社,1999潘家轺等,现代生产管理学,北京:清华大学出版社,1994黄卫伟,生产与作业管理,北京:人民大学出版社,199717)项目管理毕星、翟丽,《项目管理》,复旦大学出版社,2000[美]杰克.吉多等著, 《成功的项目管理》,机械工业出版社,1999白思俊主编, 《现代项目管理》(上,中,下),机械工业出版社,20022.认知心理学,robert j.sternberg,中国轻工业出版社3.人格理论,jess feist,gregory j.feist,人民卫生出版社4.社会心理学,elliott aronson等,中国轻工业出版社5.集合起来——群体理论与团队技巧,david w. johnson等,中国轻工业出版社8.招贤纳士自有道,lance a. berger等,清华大学出版社11. 内向者优势,martin olsen laney,华东师范大学出版社13. 超级冷静,铃木丈织,上海人民出版社31. 刘墉的书:我不是教你诈、你不可不知的人性、人生的真相补充书籍:经济类专业推荐读物 1、《摩根财团——美国一代银行王朝和现代金融业的崛起》第二版获得1990年美国国家图书奖推荐理由:摩根家族的发迹史和19-20世纪美国金融市场和银行业发展演变的全景图,前任财政部副部长、现任亚洲开发银行副行长金立群先生的翻译代表了中文相关专业译著的最高水平。
商务与经济统计作业(仅供参考)
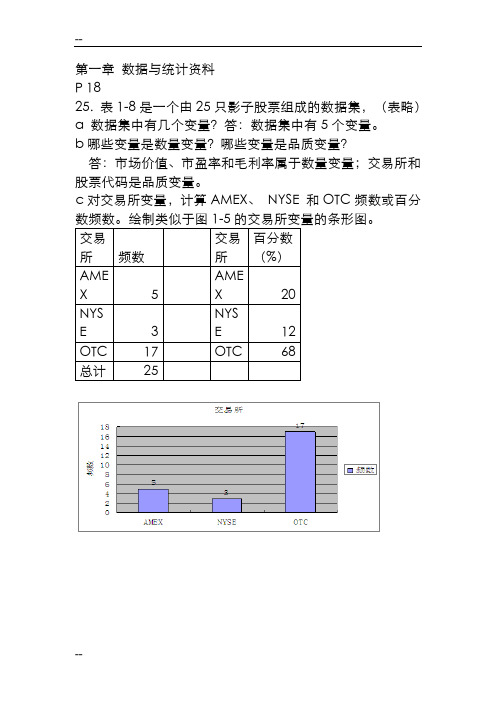
第一章数据与统计资料P 1825. 表1-8是一个由25只影子股票组成的数据集,(表略)a 数据集中有几个变量?答:数据集中有5个变量。
b哪些变量是数量变量?哪些变量是品质变量?答:市场价值、市盈率和毛利率属于数量变量;交易所和股票代码是品质变量。
c对交易所变量,计算AMEX、NYSE 和OTC频数或百分数频数。
绘制类似于图1-5的交易所变量的条形图。
e 平均市盈率是多少?答:利用EXCEL的求平均值功能得出平均市盈率是20.2第二章表格法和图形法P 235按字母顺序,美国最常见的6个姓氏为:布朗、戴维斯、约翰逊、琼斯、史密斯和威廉姆斯。
假设根据一个由50个人组成的样本,得到如下的姓氏数据(图略)a相对频数分布和百分数频数分布。
mstotal 50 b构建条形图c 构建饼形图d根据这些数据,最常见的3个姓氏是哪些?答:最常见的3个姓氏分别是史密斯、约翰逊和威廉姆斯。
P5051 表2-17 给出了50家《财富》500强公司的所有者权益、市场价值和利润数据。
(图略)a.构建所有者权益和利润变量的交叉分组表。
对利润数据以0-200,200-400,…,1000-1200分组,对所有者权益数据以0-1200,1200-2400,…,4800-6000分组。
b. 计算(a)中交叉分组表的行百分数。
P 5153. 参考表2-17中的数据集a. 绘出显示利润和所有者权益变量之间关系的散点图。
b. 评价这两个变量之间的关系。
答:二者呈正相关的关系,即所有者权益增加,利润也增加。
但因为所有点并不在一条直线上,所以这种关系不是完全的。
案例2-1 Pelican 商店1. 主要变量的百分数频数分布2. 条形图或饼形图,以显示因促销活动而使顾客购买的百分数。
3. 顾客类型(常规性或奖励性)与销售额的交叉分组表,并评价其相似性与差异性。
动取得了显著成效。
使用折扣赠券购买的奖励性顾客占全体顾客总数的70%,分布于各个销售额区域,尤其在销售额100内的范围里做出了突出贡献,尽管未使用折扣赠券的常规性顾客也主要集中在该销售额区域,但比重明显低于奖励性顾客,且在200以上的销售额区域则无常规性顾客,奖励性顾客的消费金额也扩大到300。
商务统计学第七版第十四章课后题答案
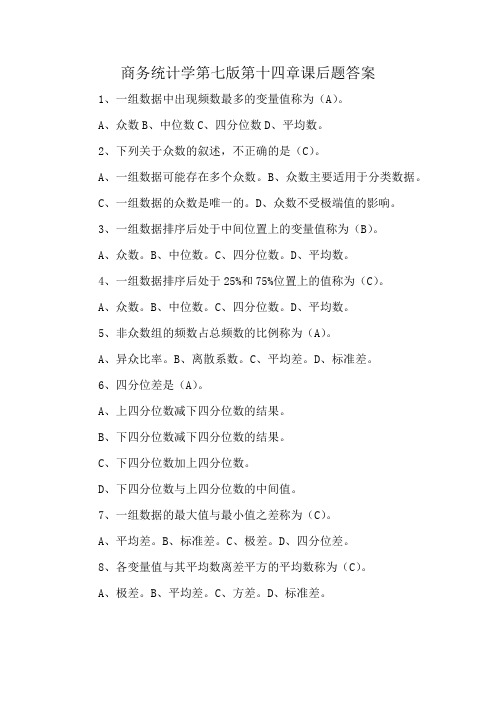
商务统计学第七版第十四章课后题答案
1、一组数据中出现频数最多的变量值称为(A)。
A、众数
B、中位数
C、四分位数
D、平均数。
2、下列关于众数的叙述,不正确的是(C)。
A、一组数据可能存在多个众数。
B、众数主要适用于分类数据。
C、一组数据的众数是唯一的。
D、众数不受极端值的影响。
3、一组数据排序后处于中间位置上的变量值称为(B)。
A、众数。
B、中位数。
C、四分位数。
D、平均数。
4、一组数据排序后处于25%和75%位置上的值称为(C)。
A、众数。
B、中位数。
C、四分位数。
D、平均数。
5、非众数组的频数占总频数的比例称为(A)。
A、异众比率。
B、离散系数。
C、平均差。
D、标准差。
6、四分位差是(A)。
A、上四分位数减下四分位数的结果。
B、下四分位数减下四分位数的结果。
C、下四分位数加上四分位数。
D、下四分位数与上四分位数的中间值。
7、一组数据的最大值与最小值之差称为(C)。
A、平均差。
B、标准差。
C、极差。
D、四分位差。
8、各变量值与其平均数离差平方的平均数称为(C)。
A、极差。
B、平均差。
C、方差。
D、标准差。