NetScaler_introduction_April_2010
川大林峰计网第一次作业
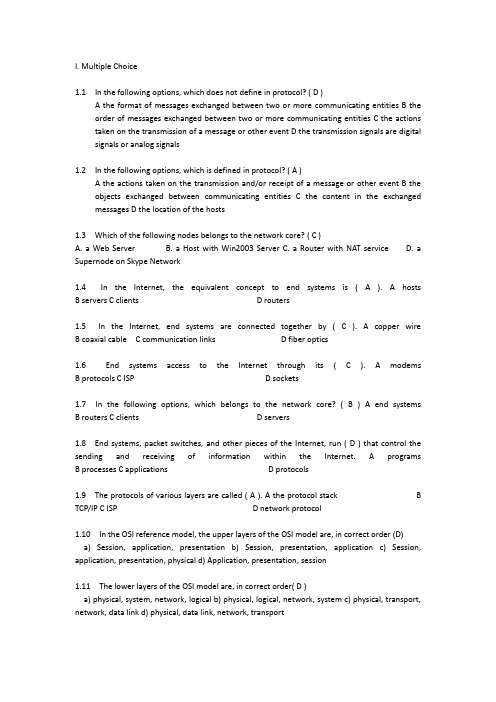
I. Multiple Choice1.1In the following options, which does not define in protocol? ( D )A the format of messages exchanged between two or more communicating entitiesB theorder of messages exchanged between two or more communicating entities C the actions taken on the transmission of a message or other event D the transmission signals are digital signals or analog signals1.2In the following options, which is defined in protocol? ( A )A the actions taken on the transmission and/or receipt of a message or other eventB theobjects exchanged between communicating entities C the content in the exchanged messages D the location of the hosts1.3 Which of the following nodes belongs to the network core? ( C )A. a Web ServerB. a Host with Win2003 ServerC. a Router with NAT serviceD. a Supernode on Skype Network1.4 In the Internet, the equivalent concept to end systems is ( A ). A hostsB serversC clientsD routers1.5 In the Internet, end systems are connected together by ( C ). A copper wireB coaxial cableC communication linksD fiber optics1.6 End systems access to the Internet through its ( C ). A modemsB protocolsC ISPD sockets1.7 In the following options, which belongs to the network core? ( B ) A end systemsB routersC clientsD servers1.8 End systems, packet switches, and other pieces of the Internet, run ( D ) that control the sending and receiving of information within the Internet. A programs B processes C applications D protocols1.9 The protocols of various layers are called ( A ). A the protocol stack B TCP/IP C ISP D network protocol1.10 In the OSI reference model, the upper layers of the OSI model are, in correct order (D)a) Session, application, presentation b) Session, presentation, application c) Session, application, presentation, physical d) Application, presentation, session1.11 The lower layers of the OSI model are, in correct order( D )a) physical, system, network, logical b) physical, logical, network, system c) physical, transport, network, data link d) physical, data link, network, transport1.12 Which of the following protocol layers is not explicitly part of the Internet Protocol Stack? ___B______ A. application layer B. session layer C. data link layer D. transport layer1.13 The 5-PDU is called__D_ A. message B. segment C. datagram D. frame1.14 Transport-layer packets are called: BA. messageB. segmentC. datagramD. frame1.15 The units of data exchanged by a link-layer protocol are called ( A ). A FramesB SegmentsC DatagramsD bit streams1.16 There are two fundamental approaches to building a network core, ( B ) and packet switching. A electrical current switching B circuit switching C data switching D message switching1.17 There are two classes of packet-switched networks: ( B ) networks and virtualcircuit networks. A datagramB circuit-switchedC televisionD telephone1.18 ( A ) means that the switch must receive the entire packet before it can begin to transmit the first bit of the packet onto the outbound link.A Store-and-forward transmissionB FDMC End-to-end connectionD TDM1.19 In ( C ) networks, the resources needed along a path to provide for communication between the end system are reserved for the duration of the communication session. A packet-switched B data-switched C circuit-switched D message-switched1.20 In ( A ) networks, the resources are not reserved; a session’s messages use the resources on demand, and as a consequence, may have to wait for access to communication link. A packet-switched B data-switched C circuit-switched D message-switched1.21 Which of the following option belongs to the circuit-switched networks? ( D ) A FDMB TDMC VC networksD both A and B1.22 In a circuit-switched network, if each link has n circuits, for each link used by the end-to-end connection, the connection gets ( A ) of the link’s bandwidth for the duration of the connection. A a fraction 1/n B all C 1/2D n times1.23 For ( C ), the transmission rate of a circuit is equal to the frame rate multiplied by the number of bits in a slot. A CDMA B packet-switched network C TDM D FDM1.24 The network that forwards packets according to host destination addresses is called ( D ) network. A circuit-switched B packet-switched C virtual-circuit D datagram1.25 The time required to propagate from the beginning of the link to the next router is ( C ). A queuing delay B processing delay C propagation delay D transmission delay1.26 Processing delay does not include the time to ( B ). A examine the packet’s header B wait to transmit the packet onto the link C determine where to direct the packet D check bit-error in the packet1.27 In the following four descriptions, which one is correct? ( C )A The traffic intensity must be greater than 1.B The fraction of lost packets increases as the traffic intensity decreases.C If the traffic intensity is close to zero, the average queuing delay will be close to zero.D If the traffic intensity is close to one, the average queuing delay will be close to one.1.28 Suppose, a is the average rate at which packets arrive at the queue, R is the transmission rate, and all packets consist of L bits, then the traffic intensity is ( B ), A LR/a B La/R C Ra/L D LR/a1.29 and it should no greater than ( B ). A 2 B 1 C 0D -11.30 Suppose there is exactly one packet switch between a sending host and a receiving host. The transmission rates between the sending host and the switch and between the switch and the receiving host are R1 and R2, respectively. Assuming that the switch uses store-and-forward packet switching, what is the total end-to-end delay to send a packet of length L? (Ignore queuing delay, propagation delay, and processing delay.) ( A ) A L/R1+L/R2 B L/R1 C L/R2 D none of the above1.31 We are sending a 30 Mbit MP3 file from a source host to a destination host. Suppose there is only one link between source and destination and the link has a transmission rate of 10 Mbps. Assume that the propagation speed is 2 * 108 meters/sec, and the distance between source and destination is 10,000 km. Also suppose that message switching is used, with the message consisting of the entire MP3 file. How many bits will the source have transmitted when the first bit arrives at the destination? CA. 1 bitB. 30,000,000 bitsC. 500,000 bitsD. none of the above1.32 Access networks can be loosely classified into three categories: residential access, company access and ( B ) access. A cabled B wireless C campus D city area1.33 The following technologies may be used for residential access, except DA. HFCB. DSLC. Dial-up modemD. FDDI1.34 Which kind of media is not a guided media? ( D ) A twisted-pair copper wireB a coaxial cableC fiber opticsD digital satellite channelII. True or False1.35 There is no network congestion in a circuit switching network.False1.36 Consider an application that transmits data at a steady rate, and once this application starts, it will stay on for a relatively long period of time. According to the characteristic, a packet-switched network would be more appropriate for this application than a circuit-switched network.FalseIII. Please Answer Following Questions Briefly1.37 How many layers are there in the Internet protocol stack? What are they? What are the principal responsibilities of each of these layers?5层。
基于BP神经网络的变压器故障诊断研究毕业设计
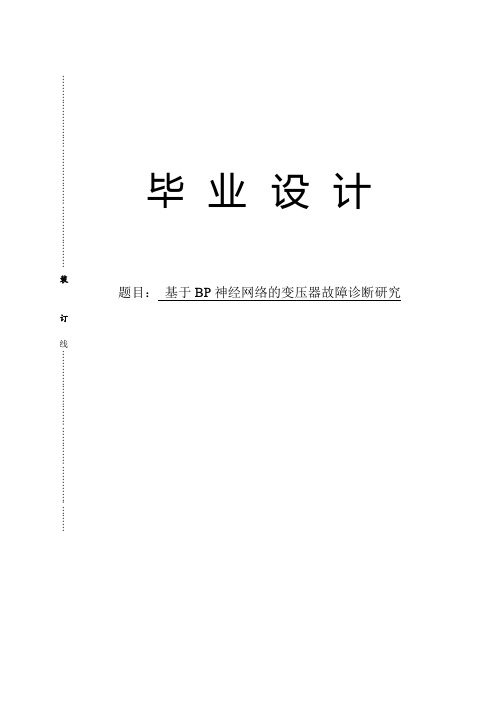
……………………. ………………. …………………毕业设计装题目:基于BP神经网络的变压器故障诊断研究订线……………….……. …………. …………. ………毕业设计(论文)原创性声明和使用授权说明原创性声明本人郑重承诺:所呈交的毕业设计(论文),是我个人在指导教师的指导下进行的研究工作及取得的成果。
尽我所知,除文中特别加以标注和致谢的地方外,不包含其他人或组织已经发表或公布过的研究成果,也不包含我为获得及其它教育机构的学位或学历而使用过的材料。
对本研究提供过帮助和做出过贡献的个人或集体,均已在文中作了明确的说明并表示了谢意。
作者签名:日期:指导教师签名:日期:使用授权说明本人完全了解大学关于收集、保存、使用毕业设计(论文)的规定,即:按照学校要求提交毕业设计(论文)的印刷本和电子版本;学校有权保存毕业设计(论文)的印刷本和电子版,并提供目录检索与阅览服务;学校可以采用影印、缩印、数字化或其它复制手段保存论文;在不以赢利为目的前提下,学校可以公布论文的部分或全部内容。
作者签名:日期:学位论文原创性声明本人郑重声明:所呈交的论文是本人在导师的指导下独立进行研究所取得的研究成果。
除了文中特别加以标注引用的内容外,本论文不包含任何其他个人或集体已经发表或撰写的成果作品。
对本文的研究做出重要贡献的个人和集体,均已在文中以明确方式标明。
本人完全意识到本声明的法律后果由本人承担。
作者签名:日期:年月日学位论文版权使用授权书本学位论文作者完全了解学校有关保留、使用学位论文的规定,同意学校保留并向国家有关部门或机构送交论文的复印件和电子版,允许论文被查阅和借阅。
本人授权大学可以将本学位论文的全部或部分内容编入有关数据库进行检索,可以采用影印、缩印或扫描等复制手段保存和汇编本学位论文。
涉密论文按学校规定处理。
作者签名:日期:年月日导师签名:日期:年月日注意事项1.设计(论文)的内容包括:1)封面(按教务处制定的标准封面格式制作)2)原创性声明3)中文摘要(300字左右)、关键词4)外文摘要、关键词5)目次页(附件不统一编入)6)论文主体部分:引言(或绪论)、正文、结论7)参考文献8)致谢9)附录(对论文支持必要时)2.论文字数要求:理工类设计(论文)正文字数不少于1万字(不包括图纸、程序清单等),文科类论文正文字数不少于1.2万字。
Network coding for distributed storage systems
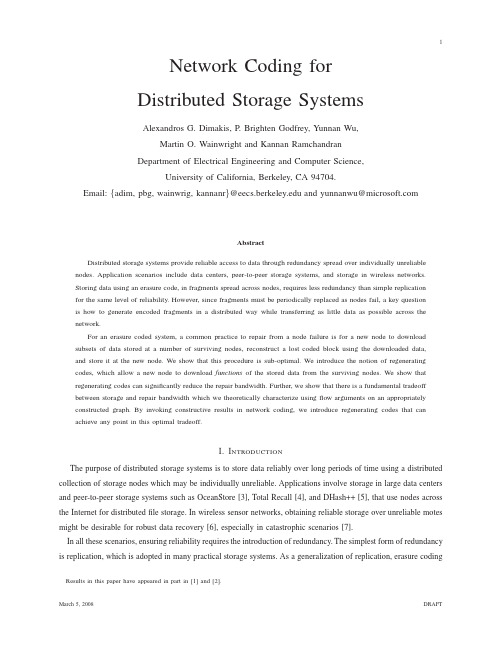
March 5, 2008
DRAFT
3
to replication; see, e.g., [9]. However, a complication arises: In distributed storage systems, redundancy must be continually refreshed as nodes fail or leave the system, which involves large data transfers across the network. This problem is best illustrated in the simple example of Fig. 1: a data object is divided in two fragments y 1 , y 2 (say, each of size 1Mb) and these encoded into four fragments x1 , . . . x4 of same size, with the property that any two out of the four can be used to recover the original y 1 , y 2 . Now assume that storage node x4 fails and a new node x5 , the newcomer, needs to communicate with existing nodes and create a new encoded packet, such that any two out of x1 , x2 , x3 , x5 suffice to recover. Clearly, if the newcomer can download any two encoded fragments (say from x1 , x2 ), reconstruction of the whole data object is possible and then a new encoded fragment can be generated (for example by making a new linear combination that is independent from the existing ones). This, however, requires the communication of 2Mb in the network to generate an erasure encoded fragment of size 1Mb at x5 . In general, if an object of size M is divided in k initial fragments, the repair bandwidth with this strategy is M bits to generate a fragment of size M/k . In contrast, if replication is used instead, a new replica may simply be copied from any other existing node, incurring no bandwidth overhead. It was commonly believed that this k -factor overhead in repair bandwidth is an unavoidable overhead that comes with the benefits of coding (see, for example, [10]). Indeed, all known coding constructions require access to the original data object to generate encoded fragments. In this paper we show that, surprisingly, there exist erasure codes that can be repaired without communicating the whole data object. In particular, for the (4, 2) example, we show that the newcomer can download 1.5Mb to repair a failure and that this is the information theoretic minimum (see Fig. 2 for an example). More generally, we identify a tradeoff between storage and repair bandwidth and show that codes exist that achieve every point on this optimal tradeoff curve. We call codes that lie on this optimal tradeoff curve regenerating codes. Note that the tradeoff region computed corrects an error in the threshold ac computed in [1] and generalizes the result to every feasible (α, γ ) pair. The two extremal points on the tradeoff curve are of special interest and we refer to them as minimum-storage regenerating (MSR) codes and minimum-bandwidth regenerating (MBR) codes. The former correspond to Maximum Distance Separable (MDS) codes that can also be efficiently repaired. At the other end of the tradeoff are the MBR codes, which have minimum repair bandwidth. We show that if each storage node is allowed to store slightly more than M/k bits, the repair bandwidth can be significantly reduced. The remainder of this paper is organized as follows. In Section II we discuss relevant background and related work from network coding theory and distributed storage systems. In Section III we introduce the notion of the information flow graph, which represents how information is communicated and stored in the network as nodes join and leave the system. In Section III-B we characterize the minimum storage and repair bandwidth and show that there is a tradeoff between these two quantities that can be expressed in terms of a maximum flow on this graph. We further show that for any finite information flow graph, there exists a regenerating code that can achieve any point on the minimum storage/ bandwidth feasible region we computed. Finally, in Section IV we evaluate the performance of the proposed regenerating codes using traces of failures in real systems and compare to alternative
T-REC-G.8265-201010-P!!PDF-E
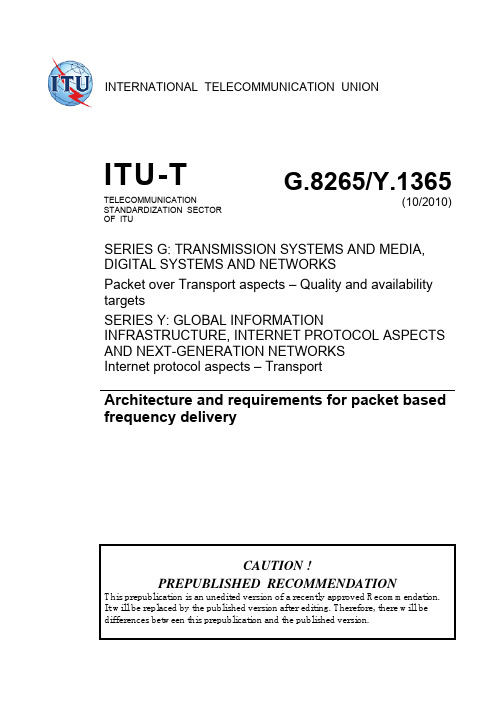
INTERNATIONAL TELECOMMUNICATION UNIONITU-T G.8265/Y.1365 TELECOMMUNICATION(10/2010) STANDARDIZATION SECTOROF ITUSERIES G: TRANSMISSION SYSTEMS AND MEDIA, DIGITAL SYSTEMS AND NETWORKSPacket over Transport aspects – Quality and availability targetsSERIES Y: GLOBAL INFORMATION INFRASTRUCTURE, INTERNET PROTOCOL ASPECTS AND NEXT-GENERATION NETWORKSInternet protocol aspects – TransportArchitecture and requirements for packet basedfrequency deliveryCAUTION !PREPUBLISHED RECOMMENDATIONThis prepublication is an unedited version of a recently approved Recommendation.It will be replaced by the published version after editing. Therefore, there will be differences between this prepublication and the published version.FOREWORDThe International Telecommunication Union (ITU) is the United Nations specialized agency in the field of telecommunications, information and communication technologies (ICTs). The ITU Telecommunication Standardization Sector (ITU-T) is a permanent organ of ITU. ITU-T is responsible for studying technical, operating and tariff questions and issuing Recommendations on them with a view to standardizing telecommunications on a worldwide basis.The World Telecommunication Standardization Assembly (WTSA), which meets every four years, establishes the topics for study by the ITU-T study groups which, in turn, produce Recommendations on these topics.The approval of ITU-T Recommendations is covered by the procedure laid down in WTSA Resolution 1.In some areas of information technology which fall within ITU-T's purview, the necessary standards are prepared on a collaborative basis with ISO and IEC.NOTEIn this Recommendation, the expression "Administration" is used for conciseness to indicate both a telecommunication administration and a recognized operating agency.Compliance with this Recommendation is voluntary. However, the Recommendation may contain certain mandatory provisions (to ensure, e.g., interoperability or applicability) and compliance with the Recommendation is achieved when all of these mandatory provisions are met. The words "shall" or some other obligatory language such as "must" and the negative equivalents are used to express requirements. The use of such words does not suggest that compliance with the Recommendation is required of any party.INTELLECTUAL PROPERTY RIGHTSITU draws attention to the possibility that the practice or implementation of this Recommendation may involve the use of a claimed Intellectual Property Right. ITU takes no position concerning the evidence, validity or applicability of claimed Intellectual Property Rights, whether asserted by ITU members or others outside of the Recommendation development process.As of the date of approval of this Recommendation, ITU [had/had not] received notice of intellectual property, protected by patents, which may be required to implement this Recommendation. However, implementers are cautioned that this may not represent the latest information and are therefore strongly urged to consult the TSB patent database at http://www.itu.int/ITU-T/ipr/.ITU 2010All rights reserved. No part of this publication may be reproduced, by any means whatsoever, without the prior written permission of ITU.Recommendation ITU-T G.8265/Y.1365Architecture and requirements for packet based frequency deliverySummaryThis Recommendation describes the architecture and requirements for packet based frequency distribution in telecom networks. Examples of packet based frequency distribution include NTP and IEEE1588-2008 and are briefly described. Details necessary to utilize IEEE™-1588-2008 in a manner consistent with the architecture are defined in other Recommendations.Recommendation ITU-T G.8265/Y.1365Architecture and requirements for packet based frequency delivery1 ScopeThis recommendation describes the general architecture of frequency distribution using packet based methods. This version of the recommendation focuses on the delivery of frequency using methods such as NTP or the IEEE Std 1588™-2008 Precision Time Protocol (PTP). The requirements and architecture form a base for the specification of other functionality needed to achieve packet based frequency distribution in a carrier environment. The architecture described covers the case where protocol interaction is at the end points of the network only, between a packet master clock and a packet slave clock. Details of requirements for other architectures involving devices that participate between the packet master and packet slave clocks are for further study.2 ReferencesThe following ITU-T Recommendations and other references contain provisions, which, through reference in this text, constitute provisions of this Recommendation. At the time of publication, the editions indicated were valid. All Recommendations and other references are subject to revision; users of this Recommendation are therefore encouraged to investigate the possibility of applying the most recent edition of the Recommendations and other references listed below. A list of the currently valid ITU-T Recommendations is regularly published.The reference to a document within this Recommendation does not give it, as a stand-alone document, the status of a Recommendation.[1] Recommendation ITU-T G.8260 (2010), Definitions and terminology for synchronizationin packet networks[2] IEEE™1588-2008, Standard for a Precision Clock Synchronization Protocol forNetworked Measurement and Control Systems[3]Recommendation ITU-T G.8264, Amendment 1 (2010), Distribution of timing informationthrough packet networks.Network Time Protocol Version 4 Protocol And Algorithms Specification, June [4] RFC5905,20103 Definitions3.1 Terms defined elsewhere:This Recommendation uses the following terms defined elsewhere:3.1.1 Packet slave clock [G.8260]3.1.2 Packet master clock [G.8260]3.1.3 Packet timing signal [G8260]4 Abbreviations and acronymsThis Recommendation uses the following abbreviations and acronyms:CDMA Code Division Multiple AccessDSL Digital Subscriber LineEEC Ethernet Equipment ClockMasterGM GrandGNSS Global Navigation Satellite SystemLTE Long Term EvolutionMINPOLL Minimum Poll intervalNTP Network Time ProtocolPON Passive Optical NetworkPDV Packet Delay VariationPRC Primary Reference ClockPTP Precision Time ProtocolLevelQL QualityRTP Real Time ProtocolSDH Synchronous Digital HierarchySEC SDH equipment ClockSSM Synchronization Status MessageTDM Time Domain MultiplexingVLAN Virtual Local Area NetworkInteroperability for Microwave AccessWIMAX WorldwideLSP Label Switched Path5 ConventionsWithin this Recommendation, the term PTP refers to the PTP version 2 protocol defined in IEEE Std 1588™-2008. NTP refers to Network Time Protocol as defined in RFC5905.6 General introduction to packet based frequency distributionThe modern telecom network has relied on accurate distribution of frequency in order to optimize transmission and TDM cross-connection. In contrast, packet networks and packet services are highly buffered by their nature and, as a result, do not require accurate timing for their operation. The migration towards converged packet networks on the surface leads to the belief that frequency distribution will not be required as packet network technology becomes more prevalent in the network.While this may be true for certain services (Internet is one example), the underlying transport mechanism that deliver these timing agnostic services may require stringent timing requirements that must be provided in the new converged network paradigm. For example, in some cases, support of circuit emulation services over a packet based infrastructure requires the presence of a stable frequency reference to enable the service. Likewise, in wireless access technologies (e.g. GSM, LTE, WIMAX, CDMA, etc.) the air interface requirements have stringent synchronization requirements that need to be met, even thought the end-user service (e.g. mobile internet) may seemingly not require timing.In order to enable timing distribution in packet based networks, the ITU-T has developed specifications Synchronous Ethernet [G.8261, G.8262, G.8264] for the physical layer frequency distribution that is similar to what was provided by SDH. This recommendation describes the use of packet based mechanisms that are intended to be used to transport frequency over a packet network in the absence of physical layer timing.6.1 Requirements for packet timingPacket based mechanisms for frequency distribution must meet the following requirements:1.Mechanisms must be specified to allow interoperability between master and slave devices(clocks)2.Mechanisms must permit consistent operation over managed wide area telecom networks.3.Packet based mechanisms must allow interoperation with existing SDH and SynchronousEthernet based frequency synchronization networks.4.Packet based mechanisms must allow the synchronization network to be designed andconfigured in a fixed arrangement5.Protection schemes used by packet based systems must be based on standard telecomoperational practice and allow slave clocks the ability to take timing from multiplegeographically separate master clocks.6.Source [clock] selection should be consistent with existing practices for physical layersynchronization and permit source selection based on received QL level and priority.7.Packet based mechanisms must permit the operation of existing, standards-based securitytechniques to help ensure the integrity of the synchronization.7 Architecture of packet based frequency distributionIn contrast to physical layer synchronization, where the significant edges of a data signal define the timing content of the signal, packet-based methods rely on the transmission of dedicated ”event packets”. These “event packets” form the significant instants of a packet timing signal. The timing of these significant instances is precisely measured relative to a master time source, and this timing information is encoded in the form of a time-stamp which is a machine readable representation of a specific instance of time1. The time-stamp is generated at a packet master function and is carried over a packet network to a packet slave clock. As time is the integral of frequency, the time-stamps can therefore be used to derive frequency.7.1 Packet based frequency distribution1 Note, in some cases, frequency may be derived from the arrival rate of incoming packets where the packets do not contain a time-stamp, but rather, are generated at precise intervals. As this Recommendation deals with the use of time-based protocols, methods to derive frequency from the arrival rate of packets are outside the scope of this recommendation.The three main components are the packet master clock, the packet slave and the packet network. A packet timing signal generated by the packet master clock is transported over the packet network so that the packet slave clock can generate a clock frequency traceable to the input timing signal available at the packet master clock. The packet master clock is presented with a timing signal traceable to a PRC. The clock produced at the packet slave clock represents the clock traceable to PRC plus some degradation (δ) due to the packet network. The general architectural topology is shown in Figure 1. The synchronization flow is from the Master to Slave. In cases where the reference to the master is provided over a synchronization distribution network, additionaldegradation of the frequency signal may be present at the input to the master and therefore also present at the output of the slave.Figure 1/G.8265: General packet network timing architecture7.2. T iming protection7.2.1 Packet master protectionIn traditional synchronization networks, timing availability is enhanced by the use of timing protection where by the timing to a slave clock (e.g. SEC, or EEC) may be provided over one or more alternative network paths. In the case of the packet based timing architecture, the slave clocks may have visibility to two or more master clocks as show in Figure 2.In contrast to physical layer timing, where the selection of the clock is performed at the slave clock, selection of a secondary master clock may involve some communication and negotiation between the master and the slave and the secondary master and slave.PacketPacketPacket PacketReference 1: Note, the reference may be from a PRC directly, GNSS or via a synchronization networkPacket Timing Signals Slave Clock Slave ClockSlave clockPacket Network Master clock1F iF out +δ2F out + δ3F out +δ1Figure 2/G.8265: Packet network timing (frequency) protection(Note: for clarity, the network reference signals to masters are not shown)7.2.2 Packet Master / Slave Selection FunctionsFunctions required in order to support packet reference selection are described in the following clauses.7.2.2.1 Temporary Master Exclusion – Lock-out functionTo protect the downstream architecture it must be possible in the slaves to exclude temporarily a master from a list of candidate masters (lock-out functionality).7.2.2.2 Slave Wait to Restore Time functionTo protect the downstream architecture a Wait to Restore Time must be implemented in the slave. If a master fails or is unreachable, a slave will switch to a backup master. However, upon the recovery of the primary master, the slave will not switch back to the primary master until the wait to restore time expires.7.2.2.3 Slave non-reversion functionTo protect the downstream architecture a slave non-reversion function may be implemented to protect against slaves “flipping” between masters.In the slave this will ensure that if a master fails or is not anymore reachable, a slave will switch to a backup master but will not switch back to the primary master if the non-revertive mode is implemented and activated.7.2.2.4 Forced Traceability of Master functionIt must be possible to force the QL traceability value at the input of the packet master clock.Network implementations and scenarios making use of this functionality will need to be defined by the operator on a case by case basis and are dependent on the operator’s architecture.The function illustration is presented in Figure 3.Figure 3/G.8265: Example of use case where forcing the QL value at the input of the PTPv2 master is needed7.2.2.5 Packet Slave Clock QL Hold off functionIn the case where sufficient holdover performance exists within the Packet slave clock it must be possible to delay the transition of the QL value at the output of the slaves. This will allow the operator to limit downstream switching of the architecture under certain network implementations when traceability to the packet master is lost.Note: the QL hold off is highly dependent on the quality of the clock implemented in the slave and is for further study.These network implementations and scenarios will need to be defined by the operator on a case by case basis.The function illustration is presented in Figure 4.Figure 4/G.8265: Example of use case where the QL hold off at the output of the Packet slaveclock7.2.2.6 Slave Output Squelch functionNetwork PacketMasterClock ExternalinputReference(no QL)Packet Slave Clock QL value associated tothe external inputreference by the masterIn case the Packet slave provides an external output synchronization interface (e.g. 2 MHz) asquelch function must be implemented in order to protect the downstream architecture and certain end applications.This function is used under certain upstream packet timing signal failure conditions between the packet master and the packet slave.These network implementations and scenarios will need to be defined by the operator on a case by case basis. For example one application will be the case of a Packet slave external to the endequipment, such as base station, which may implement better holdover conditions compared to the Packet slave: in this case, it is recommended to squelch the signal at the output of the Packet slave in packet timing failure conditions so that the end equipment would switch into holdover rather than synchronizing the end equipment with the holdover of the Packet slave.Architectural implementations using this function are for further study. The function is illustrated in Figure 5.Figure 5/G.8265: Squelching at output of Packet Slave7.3 Packet network partitioningPacket networks may be partitioned into a number of different administrative domains. The transport of timing across the packet network must consider the partitioning of networks into different administration domains as illustrated in Figure 6. This could mean, for example, that packet master clocks may be located in different administrative domains. Operation in this configuration may be limited due to the protocol capabilities and is for further study .Figure 6/G.8265: Packet timing flow over partitioned network .Passing packet based timing between administrative domains is not currently specified in this version of the recommendation and is for further study. Issues surrounding the demarcation of the packet timing flow and the transferred performance between operators exist.Due to the operation of packet based networks and their impact on packet based timing recovery, especially under stress conditions, derived performance is difficult to characterise. Concerning the end to end recovery of timing from the packet timing flow, situations can exist where it is difficult to determine the location of performance problems especially if the packet timing is passing through multiple administrative domains.Network Packet MasterCloc Packet Slave ClocNetworkOperatorNetwork Operator Operator Clock Clock Timing FlowWhen multiple administrative domains are involved, other methods that are based on physical layer synchronization (for example, Synchronous Ethernet over OTN) may be applicable for frequency distribution. The details are outside the scope of this recommendation. Further information can be found in G.8264, Clause 11.7.4 Mixed technologiesPacket services may be carried over a packet switching network where the core and access are carried over different technologies which may impact packet delay variation performance and the ability of the slave clock to derive frequency. For example, within the core, packets containing time-stamps may traverse routers, switches or bridges interconnected by Ethernet links, while in the access portion interconnect may be xDSL or PON.A connection through a network may consist of a concatenation of different technologies. The PDV performance may be different based on these technologies. The aggregate PDV may therefore differ when mixes of different technologies are deployed. A slave clock may need to accommodate the impact of these different technologies.Details of the PDV contributions of individual transport technologies and the performance of slave clocks are for further study.8 Packet based protocols for frequency distribution8.1 Packet based protocolsAs noted in Clause 6, frequency transfer over packet networks is not inherent at the packet layer. In cases where frequency transfer is required, methods such as circuit emulation may be employed, which utilize either differential or adaptive clock recovery methods. (See RecommendationG.8261)Protocols for distribution of time exist such as NTP and IEEE1588™-2008 (PTP). Although the protocols are primarily intended for the distribution of time, it is also possible to derive frequency.A general description on the protocols as well as clarifications on the need to define further details when using these protocols for the purpose of frequency distribution is provided below. Note that the performance achievable may also depend on factors outside of the protocol definitions.8.2 PTP/IEEE™1588 general descriptionIEEE1588™-2008 describes the “Precision Time Protocol”, commonly referred to as PTP. The PTP protocol enables the accurate time-transfer between two entities (clocks) by the transmission of messages containing accurate timestamps representing an estimate of the time at which the packet is sent. The repeated transmission of messages also allows the derivation of frequency.The PTP protocol supports unicast and multi-cast operation. Additionally, the protocol provides the support for two clock modes, a one-step mode and a two-step mode, which involves the transmission of an additional Follow-up message. Additional messages are also defined for other purposes, such as Signaling and Management.While the first version IEEE1588™ was developed for industrial automation, the second version (IEEE1588™-2008) was extended to be applicable to other applications such as telecom. The protocol may be tailored to specific applications by the creation of “profiles” which specify which subset of functionality may be required, together with any related configuration settings, to satisfy a specific application. ITU-T is concerned with application to Telecom environments.IEEE1588™-2008 defines several types of clocks; ordinary, boundary and transparent clocks. While the standard defines clocks, these are only high level constructs. The performance achievable by the PTP protocol is based on factors that are outside the scope of the IEEE1588™-2008 standard.ITU-T Recommendation G.8265.1 contains a PTP profile applicable for telecom applications using ordinary clocks in a unicast environment. Profiles developed by the ITU-T are intended to meet all the high-level requirements specified in this Recommendation.8.3 NTP - general descriptionNTPv4 is defined in RFC 5905, which obsoletes both RFC 1305 (NTP v3), and RFC 4330 (SNTP). RFC5905 defines both a protocol and an algorithm to distribute time synchronization, however the NTP on-wire protocol can also be used to distribute a frequency reference. In this case, however, one must develop a specific algorithm to recover frequency and only the packet format and protocol aspects need to be considered. The specific implementation in the client for the purpose of frequency synchronization clock recovery can be considered similar to an implementation using other packet protocols.According to RFC5905, an SNTP client is not required to implement the NTP algorithms specified in RFC 5905. In particular RFC5905 notes that Primary servers and clients complying with a subset of NTP, called the Simple Network Time Protocol (SNTP), do not need to implement the mitigation algorithms described in the relevant sections of RFC5905. The SNTP client can operate with any subset of the NTP on-wire protocol, the simplest approach using only the transmit timestamp of the server packet and ignoring all other fields.Among the aspects to consider is that in some applications the required packet rate may need to be higher (lower MINPOLL value) than the limit currently suggested for the time synchronization algorithm specified in the RFC 5905. On this aspect the following is stated in RFC 5905 section 7.3 “Packet Header Variables”, with respect to the MINPOLL parameter: “These are in 8-bit signed integer format in log2 (log base 2) seconds.” and “Suggested default limits for minimum and maximum poll intervals are 6 and 10, respectively”.Note: the detailed way of using NTP for the specific application (e.g. including the method to support SSM according to the requirements of clause 6)., is for further study.More details on the use of timing packets (such as NTP) for the purpose of frequency transfer are provided in Appendix XII (Basic Principles of Timing over Packet Networks) in G.8261.9 Security aspectsUnlike traditional timing streams where frequency is carried over the physical layer, packet based timing streams may be observed at different points in the network. There may be cases where timing packets flow across multiple network domains which may introduce specific securityrequirements. There may also be aspects of security that may be related to both the network (e.g. authentication and/or authorization) and to the PTP protocol itself.It is important to permit the operation with existing, standards-based security techniques to help ensure the integrity of the synchronization. Examples may include encryption and/or authentication techniques, or network techniques for separating traffic, such as VLANs or LSPs. Specifically;-slaves should be prevented from connecting to rogue masters(this could be either by an authentication process or by using network separation toprevent rogue masters from accessing slaves)-masters should be prevented from providing service to unauthorised slavesIt may not be possible to implement some of these requirements without actually degrading the overall level of timing or system performance.Security aspects are for further study.Appendix IBibliography[B1] RFC1305 Network Time Protocol (Version 3) Specification, Implementation and Analysis, March 2009[B2] RFC 4330, Simple Network Time Protocol (SNTP) Version 4 for IPv4, IPv6 and OSI, January 2006[B3] RFC5905, Network Time Protocol Version 4: Protocol and Algorithms Specification, June 2010______________。
Ucinet_Guide
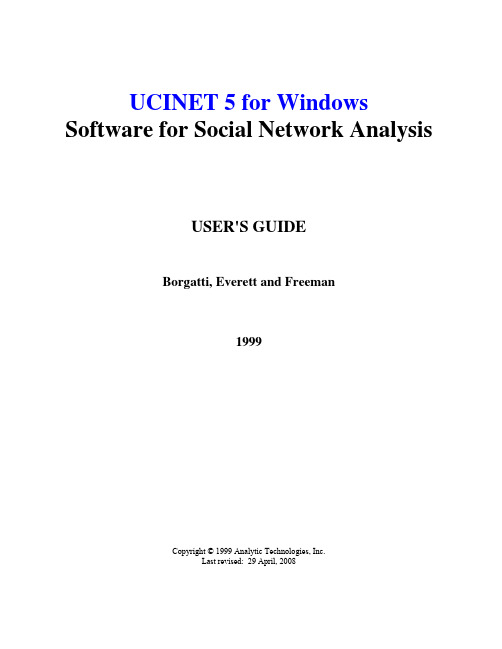
UCINET 5 for Windows Software for Social Network AnalysisUSER'S GUIDEBorgatti, Everett and Freeman1999Copyright © 1999 Analytic Technologies, Inc.Last revised: 29 April, 2008UCINET 5© 1999 Analytic Technologies. All rights reserved.Analytic Technologies11 Ohlin LaneHarvard, MA 01451 USAVoice: (978) 456-7372Fax: (978) 456-7373Table of ContentsPreface 0 Conventions 0.1 Notational0.2 AcknowledgementsProgramming Considerations 0.3Content 0.40.5OrientationMatrixStarted 1 GettingHardware 1.11.2 Installation1.3ProgramQuittingthe1.4SupportTechnical1.5ProgramTheCitingLicense and Limited Warranty 1.62EnvironmentUCINETTheHelp 2.1 MenusandForms 2.2Running An Analysis 2.32.4FileTheLogDatasets 2.5Organization 2.6 Program2.7FileSubmenuSubmenu 2.8Data2.9TransformSubmenuSubmenu 2.10ToolsSubmenu2.11Networks2.12SubmenuOptionsData 3 ImportingFiletype 3.1 RAWFiletype 3.2 Excel3.3DLFiletype3.4FormatFullMatrixMatrices 3.5Rectangular3.6Labels3.7MatricesMultiple3.8FileDataExternalAbsent 3.9DiagonalLowerhalf and Upperhalf Matrices 3.103.11FormatBlockmatrix3.12FormatsListLinkedNodelists 3.12.1EdgelistFormats 3.12.23.12.3EdgearrayFormatEditor 3.15 SpreadsheetUCINETProcessing 4 DataSubgraphs and Submatrices 4.14.2 MergingDatasetsPermutations and Sorts 4.3 Transposing and Reshaping 4.4 Recodes 4.5 Transformations 4.6 LinearSymmetrizing 4.7 Geodesic Distances and Reachability 4.84.9 AggregationNormalizing and Standardizing 4.10Changes 4.11 ModeWhere is it now? 5~ 0 ~Preface0.1 Notational ConventionsUCINET is menu-driven Windows program. This means you choose what you want to do by selecting items from a menu. Menus may be nested, so that choosing an item from a menu may call up a submenu with additional choices, which in turn may call up submenus of their own. Consequently, to get to certain choices, you may have to select through a number of menus along the way. To represent the options you must take to a given choice, we use angle brackets. For example, to run the hierarchical clustering procedure, you must first start UCINET, then click on the top toolbar and point to Tools, from the drop down menu that appears highlight Cluster and from the submenu that appears click on Hierarchical. We will represent this series of choices asTools>Cluster>Hierarchical0.2 AcknowledgementsDozens of people have contributed to UCINET 5 for Windows by making suggestions, contributing technical expertise, finding bugs, and providing moral and financial support. We especially thank Charles Kadushin, David Krackhardt, Ron Rice and Lee Sailer for their early and continued support. We also thank those who have contributed technical expertise for specific algorithms, including Pip Pattison (semigroups), Kim Romney (correspondence analysis) and Stan Wasserman (p1).We are also grateful to Brian Kneller (University of Greenwich, London) for doing much of the initial programming on the Windows interface. The non-metric MDS program is adapted from UCINET 3.0 (MacEvoy and Freeman, 1985), which in turn was drawn from the University of Edinburgh's MINISSA program. Many of the procedures make use of routines found in Numerical Recipes in Pascal by Press, Flannery, Teukolsky and Vetterling, and EISPACK (Smith et. al. 1976, Springer-Verlag).0.3 Programming ConsiderationsTo paraphrase an old song (and reverse the meaning), UCINET 5.0 is built for speed, not for comfort. Oftentimes during the programming of UCINET, we had to choose between using a fast algorithm that used a lot of memory (and therefore reduced the maximum size of network it could handle), and a slow algorithm that saved memory and could handle much larger datasets. In previous versions we tried to strike a balance between the two. In this version, we usually chose speed. One reason for this is that it is precisely when working with large datasets that speed is essential: what good is an algorithm that can handle thousands of nodes if it takes days to execute? The other reason is that advances in hardware and operating system software continually extend the amount of memory programs can access, so it seems a waste of programming time to work out ways to economize on memory.One consequence of menu systems is the need to organize program capabilities into categories and subcategories in a way that is logical and comprehensible. Of course, this has proved to be impossible. With only a little effort one can discover several competing schemes for classifying all the functions that UCINET 5.0 offers. None of the schemes is perfect: each does an elegant job of classifying certain items but seems forced and arbitrary with others. The scheme we have settled on is no exception. The basic idea is that under "network" we put techniques whose reason for being is fundamentally network-theoretic: techniques whose interpretation is forced when applied tonon-network data. An example of such a technique is a centrality measure. In contrast, under "tools" we put techniques that are frequently used by network analysts, but are also commonly used in contexts having nothing to do with networks. Multidimensional scaling and cluster analysis are examples of such procedures. Inevitably, of course, there are techniques that are either difficult to classify or for some reason are convenient to misclassify. 0.4 ContentUCINET 5.0 is basically a re-engineered version of UCINET IV, and so users familiar with UCINET IV should easily adapt to the new environment. We have extended UCINET's capabilities and re-organised the routines into what we believe to be more sensible categories.Perhaps the most fundamental design consideration we have faced is choosing what capabilities the program should have. Since Freeman's first version was released, UCINET has incorporated a diverse collection of network techniques. The techniques are diverse both in the sense of what they do (detect cohesive subgroups, measure centrality, etc), and where they come from (having been developed by different individuals from different mathematical, methodological, and substantive points of view). In UCINET 5.0 we continue that tradition, seeing ourselves more as editors and publishers of diverse works than as authors with a single pervading perspective. One problem with this approach is that different techniques implicitly assume different views of what their data are. For example, graph-theoretic techniques describe their data in terms of abstract collections of points and lines, algebraic techniques view their data as sets and relations, and statistical techniques understand their data to be variables, vectors, or matrices. Sometimes the mathematical traditions intersect and the same operation is found in the repertoire of each tradition; cognates, if you will. For example, a graph automorphism, which is a 1-1 mapping of a graph to itself, corresponds in the world of 1-mode matrices to a re-ordering of the rows and corresponding columns of a matrix. Likewise, the converse of a graph, in which the direction of all directed arcs is reversed, translates to the transpose of a matrix, which is an exchange of rows and columns. Unfortunately, there are also false cognates among the lexicons. For example, a relation may be expressed as a matrix, but in discrete algebra, the "inverse" of a relation is the transpose of that matrix, not the matrix which, when multiplied by the original, yields the identity.0.5 Matrix OrientationIn UCINET 5.0, all data are described as matrices. While the prompts and outputs of some procedures may reflect the language most commonly associated with that technique (e.g. "networks" for centrality measures, "relations" for ego-algebras), it is extremely important for the user to maintain a "matrix-centered" view of the data. All UCINET data are ultimately stored and described as collections of matrices. Understanding how graphs, networks, relations, hypergraphs, and all the other entities of network analysis are represented as matrices is essential to efficient, trouble-free usage of the system.~ 1 ~Getting Started1.1 HardwareUCINET 5.0 requires a computer running Windows 95 (from 1997 or more recent), Windows 98, NT or other compatible operating system. The program requires 5 mb of hard disk storage space and 16mb of RAM.1.2 InstallationThe UCINET program must be installed before it can be used. If you have a CD then place the disk in the drive and the program should start the installation automatically. If this does not happen then from the start button select the run option change to your CD drive and click on "setup.exe". If you have an electronic version then from run select the folder containing UCINET and select the file "setup.exe". The installation wizard will guide you through the installation procedure.1.3 Quitting the ProgramTo leave the program, choose F ile>Exit from the toolbar, click on the door icon, or press Ctl+x (the Control key and the x key together). If the program is in the middle of executing an analysis and you want to interrupt it, you can try pressing esc. This works only for iterative procedures such as MDS or TABU SEARCH. Otherwise press Control Alt Delete (simultaneously) and this brings up the operating system window click on the button marked task manager, you can now select UCINET and end the task. Some procedures offer a “Calculating …” dialogue box with a STOP button that will stop execution at the next convenient point.1.4 Technical SupportRegistered owners of UCINET 5.0 (professional version) are entitled to technical support. Please e-mail, write or call the authors during standard business hours with any questions, suggestions and bug reports. They can be reached at the following addresses:Steve BorgattiAnalytic Technologies11 Ohlin LaneHarvard, MA 01451 USATel: +1 978 456 7372Fax: +1 978 456 7373Email: support@Martin EverettSchool of Computing and Mathematical SciencesUniversity of Greenwich30 Park RowGreenwichLondon SE10 9LSTel: +44(0)20 8331 8716Fax: +44(0)20 8331 8665email: M.G.Everett@Please communicate bugs as soon as possible: if at all possible, we will immediately replace the software with a corrected copy at our expense.1.5 Citing The ProgramIf you use UCINET 5.0 to perform any analyses which result in a publication or presentation, you must cite it (this is required by your licensing agreement). The citation should treat the program as though it were a book. For example:Borgatti, S.P., M.G. Everett, and L.C. Freeman. 1999. UCINET 5.0 Version 1.00. Natick: AnalyticTechnologies.In other words, the title of the "book" is the name of the program, and the publisher is Analytic Technologies. Since the program will change over time, it is important to include the version number in the title in order to allow others to replicate your work.1.6 License and Limited WarrantyThis software is protected by both United States copyright law and international treaty provisions. You must treat this software like a book, except that (i) you may copy it to the hard disk of a single computer or to the hard disk of any computer(s) that you personally have exclusive use of, and (ii) you can make archival copies of the software for the sole purpose of backing it up to protect your investment from loss.By "treat this software like a book" we mean that the software may be used by any number of people, and may be freely moved from one computer to another, as long as there is no possibility of it being used simultaneously on two different computers. Just as a given copy of a book cannot be read by two different people in different places at the same time, neither can the software be legally used by different people on different computers at the same time. Analytic Technologies warrants the physical CD and physical documentation associated with the UCINET 5.0 product to be free of defects in materials and workmanship for a period of sixty days from the date of purchase or licensing. If Analytic Technologies receives notification within the warranty period of defects in materials or workmanship, and such notification is determined by Analytic Technologies to be correct, we will replace the defective materials at no charge. However, do not return any product until you have called us and obtained authorization.The entire and exclusive liability and remedy for breach of this Limited Warranty shall be limited to replacement of CD(s) or documentation and shall NOT include or extend to any claim for or right to recover any other damages, including but not limited to, loss of profit or prestige or data or use of the software, or special, incidental or consequential damages or other similar claims, even if Analytic Technologies has been specifically advised of the possibility of such damages. In no event will Analytic Technologies's liability for any damages to you or any other person ever exceed the price of the license to use the software, regardless of any form of the claim.Analytic Technologies specifically disclaims all other warranties, express or implied, including but not limited to, any implied warranty of merchantability or fitness for a particular purpose. Specifically, Analytic Technologies makes no representation or warranty that the software is fit for any particular purpose and any implied warranty of merchantability is limited to the sixty-day duration of the Limited Warranty covering the physical CD(s) and physical documentation only (and not the software) and is otherwise expressly and specifically disclaimed.This limited warranty gives you specific legal rights; you may have others which vary from state to state. Some states do not allow the exclusion of incidental or consequential damages, or the limitation on how long an implied warranty lasts, so some of the above may not apply to you.This License and Limited Warranty shall be construed, interpreted and governed by the laws of Massachusetts, and any action hereunder shall be brought only in Massachusetts. If any provision is found void, invalid or unenforceable it will not affect the validity of the balance of this License and Limited Warranty which shall remain valid and enforceable according to its terms. If any remedy hereunder is determined to have failed of its essential purpose, all limitations of liability and exclusion of damages set forth herein shall remain in full force and effect. This License and Limited Warranty may only be modified in writing signed by you and a specifically authorized representative of Analytic Technologies. All use, duplication or disclosure by the U.S. Government of the computer software and documentation in this package shall be subject to the restricted rights under DFARS 52.227-7013 applicable to commercial computer software. All rights not specifically granted in this statement are reserved by Analytic Technologies.~ 2 ~The UCINET Environment In this chapter we give an overview of running the program, covering such topics as menus and forms, the nature of UCINET 5.0 datasets, running analyses, and dealing with output.2.1 Menus and HelpThe program is menu-driven, which makes it very easy to use, even if you haven't used it in a while. After you start up the program, you will find yourself in the main window. The main window provides the following choices: File, Data, Transform, Tools, Network, Options, and Help together with three buttons. Each of these choices is itself a submenu with additional choices. The leftmost button directly calls the spreadsheet editor, as already stated the middle button exits from the program and the button on the right allows the user to change the default folder. There are two ways to choose an item from a menu. The simplest method is to use the mouse to highlight the desired choice, then give a left click to activate a routine. If a highlighted choice has a submenu, that submenu will automatically appear. Another method is to press the highlighted letter of the desired choice, which is usually the first letter. For example, if the Network menu is highlighted then to get to Roles and Positions you can press L, you can now select another option if your choice is at the bottom level of the menu then the routine is activated. After selecting Roles and Positions typing S will select Structural and then P will select and run the Profile routine.Sometimes, however, there is a way to circumvent the menus. Some menu items, such as displaying datasets found in Data>Display, have been assigned "hot-keys" which can be used to invoke that item directly without going through the menus. Provided no menus are highlighted then pressing Ctrl+D will immediately invoke the Data>Display routine.All the hot keys are Control or Alt letter combinations. They are as follows:FolderDefaultCtrl+F File>ChangeFile>ExitAlt+XCtrl+S Data>SpreasheetCtrl+D Data>DisplayCtrl+B Data>DescribeCtrl+X Data>ExtractCtrl+M Data>Random>Matrix2.2 FormsSuppose you choose a procedure from the menu. With few exceptions, the next thing you are likely to see is a parameter form. A form is a collection of one or more fill-in-the-blank questions. For example, suppose you click on networks>Subgroups>Cliques from the File menu. The form you see will consist of the following questions:Inputdataset: Camp92.##hsize: 3MinimumAnalyze pattern of overlaps?: YESType Tree DiagramDiagram(Output) Clique indicator matrix: CliqueSets(Output) Co-membership matrix: CliqueOverlap(Output) Partition indicator matrix: CliquePartThe left-hand items (in bold type) are the question. The right-hand side contains the default answers. Often you will leave the default values as they are, expect for the input dataset, which is where you enter the name of the dataset that you want to analyze. You can either type the name yourself or double-click on that area and a dialog box will appear allowing you to choose the file you want from a list.Once all the form is completed to your satisfaction, you activate the routine by clicking on the OK button (not shown above).The next time you choose Clique from the menu, you will find that the default input dataset is whatever dataset you used the last time you ran Clique, unless the option Smartdefaultnames is on. In that case, the program puts in the default name that it thinks you might want to use, based on what you have done earlier. If this is the file you want to edit, just click on OK. If not, just start typing the name of the new file or click on the button to the right of the box. The moment you hit a key, the old filename will vanish: there is no need to backspace to the beginning of the name. If the filename is almost right but needs editing, use the mouse to place the cursor in the correct position so that you can fix it.Whenever you are being prompted for a filename, then the button to the right of the box with three dots on it will activate the standard Windows procedure for selecting a file.Incidentally, whenever you are called upon to enter a list of actors, you should refer to the actors by number (i.e. row or column in the data matrix) rather than by name or other label. Separate the numbers by spaces or commas. UCINET gives help in this process by allowing you to click on labels and automatically entering in the correct actor or matrix numbers. The button labeled with an L immediately next to the file selection button will give a list of labels you can now select from this list using the mouse. To select more than one entry you should press the Control key at the same time as you click on your choice. In addition, you can indicate groups of numbers using the following conventions:to1033-105first30lastThe first two lines both specify the set of id numbers 3, 4, 5, 6, 7, 8, 9, 10. The last line only works when it is clear to the program what the total number of actors is. All of these conventions can be mixed, as follows:List of actors to DROP: first 4, 18 12,5 19 to 25, last 22.3 Running An AnalysisAs the diagram below indicates, the typical UCINET 5.0 procedure takes two kinds of input, and produces two kinds of output.-----------input parameters ---> | | ---> output text| PROCEDURE |input datasets ---> | | ---> output datasets-----------One kind of input consists of UCINET 5.0 datasets. Most procedures take a single dataset as input, but that dataset may contain multiple matrices, often representing different social relations measured on the same actors. Other procedures (such as the Join and QAP Regression procedures) take multiple datasets as input, each of which may contain several matrices.Another kind of input consists of a set of parameters which either describe the data or alter the way the program runs. For example, as you saw earlier, the parameters for the Clique procedure are: the name of the input dataset, the minimum size of clique to report, and the names of various output files. Most parameters have default values which the user will have an opportunity to either accept or override.Parameters are specified in forms that appear immediately after a procedure is selected from the menu. The first time a procedure is invoked in a given session, the fields for all parameters will contain "factory-set" default values (wherever possible). If you change the values of any parameters, these changes will remain in effect for subsequent runs, until you change them again or exit UCINET. The only exception to this rule occurs when some default settings depend on the values of other default settings, in which case changing certain parameter values will cause the program to change other defaults, even if you have previously set them.One set of parameters that always have default values is the names of a procedure's output files. Output files are UCINET 5.0 datasets that can be read by all of the other UCINET procedures and therefore used as input for further analyses. For example, one of the outputs of the CLIQUE program is an actor-by-actor matrix whose ijth cell gives the number of cliques that actors i and j are both members of. This matrix is suitable for input to the MDS program to obtain a spatial representation of the pattern of overlaps. By default, the name of this dataset is CliqueOverlap.In addition to output datasets, most UCINET procedures also produce a textual report as output. This report is always saved to an text file called a Log File (usually stored in the \Windows\System directory), and is also automatically displayed on the screen.Let us run through an example of a clique analysis. The first step is to clique on Network>Subgroups>Cliques from the toolbar. This will pop up a form that contains the following:Input dataset:Minimum size: 3Analyze pattern of overlaps?: YESDiagram Type Tree Diagram(Output) Clique indicator matrix: CliquesSets(Output) Co-membership matrix: CliquesOver(Output) Partition indicator matrix: CliquesPartThe first line asks for the name of the UCINET 5.0 dataset containing the data. If the dataset is called TARO and is located in the \uci3 folder of the c: drive, you would fill in the blank with c:\uci3\TARO. (Of course, naming the drive and folder is only necessary if the data are located in a different drive/folder than the current default). You could also select this file by clicking on the button to the right of the question and selecting the file using the mouse from the window that opens up.The second line asks for the minimum size of clique to report. A default answer of 3 is already filled in. To override the default, just type over it.The third line asks whether to compute and analyze an actor-by-actor matrix that counts up, for each pair of actors, the number of cliques they belong to in common. This matrix is then submitted to hierarchical clustering. The default answer here is YES, but to save processing time you may wish to override the default when working with large datasets.The fourth line asks a question about what type of clustering diagram you would like to view. The default is a tree diagram; the only other alternative is a dendrogram which is a variation on the same theme.The fifth through seventh lines ask for the names of datasets to contain the key outputs from the analysis. These datasets can then be used as input to other analyses. Default names are supplied for all three.When you are through entering or modifying these parameters, click on OK to begin the analysis. Since TARO is a standard UCINET dataset then you will be able to run this analysis. The result will be a tree diagram appearing on the screen. If you now clique on the OK button then the following Log File will be displayed.CLIQUES------------------------------------------------------------------------------Minimum Set Size: 3Input dataset: C:\Uci3\TARO10 cliques found.1: 2 3 172: 1 2 173: 17 18 224: 4 5 65: 4 6 76: 5 20 217: 8 9 108: 11 20 219: 12 13 1410: 12 14 15Group Co-Membership Matrix1 1 1 1 1 1 1 1 1 12 2 21 2 3 4 5 6 7 8 9 0 1 2 3 4 5 6 7 8 9 0 1 2- - - - - - - - - - - - - - - - - - - - - -1 1 1 0 0 0 0 0 0 0 0 0 0 0 0 0 0 1 0 0 0 0 02 1 2 1 0 0 0 0 0 0 0 0 0 0 0 0 0 2 0 0 0 0 03 0 1 1 0 0 0 0 0 0 0 0 0 0 0 0 0 1 0 0 0 0 04 0 0 0 2 1 2 1 0 0 0 0 0 0 0 0 0 0 0 0 0 0 05 0 0 0 1 2 1 0 0 0 0 0 0 0 0 0 0 0 0 0 1 1 06 0 0 0 2 1 2 1 0 0 0 0 0 0 0 0 0 0 0 0 0 0 07 0 0 0 1 0 1 1 0 0 0 0 0 0 0 0 0 0 0 0 0 0 08 0 0 0 0 0 0 0 1 1 1 0 0 0 0 0 0 0 0 0 0 0 09 0 0 0 0 0 0 0 1 1 1 0 0 0 0 0 0 0 0 0 0 0 010 0 0 0 0 0 0 0 1 1 1 0 0 0 0 0 0 0 0 0 0 0 011 0 0 0 0 0 0 0 0 0 0 1 0 0 0 0 0 0 0 0 1 1 012 0 0 0 0 0 0 0 0 0 0 0 2 1 2 1 0 0 0 0 0 0 013 0 0 0 0 0 0 0 0 0 0 0 1 1 1 0 0 0 0 0 0 0 014 0 0 0 0 0 0 0 0 0 0 0 2 1 2 1 0 0 0 0 0 0 015 0 0 0 0 0 0 0 0 0 0 0 1 0 1 1 0 0 0 0 0 0 016 0 0 0 0 0 0 0 0 0 0 0 0 0 0 0 0 0 0 0 0 0 017 1 2 1 0 0 0 0 0 0 0 0 0 0 0 0 0 3 1 0 0 0 118 0 0 0 0 0 0 0 0 0 0 0 0 0 0 0 0 1 1 0 0 0 119 0 0 0 0 0 0 0 0 0 0 0 0 0 0 0 0 0 0 0 0 0 020 0 0 0 0 1 0 0 0 0 0 1 0 0 0 0 0 0 0 0 2 2 021 0 0 0 0 1 0 0 0 0 0 1 0 0 0 0 0 0 0 0 2 2 022 0 0 0 0 0 0 0 0 0 0 0 0 0 0 0 0 1 1 0 0 0 1SINGLE-LINK HIERARCHICAL CLUSTERING1 1 1 1 1 1 1 12 2 1 1 2Level 8 9 0 3 2 4 5 6 9 5 6 4 7 1 1 0 1 3 2 7 8 2----- - - - - - - - - - - - - - - - - - - - - - -2 . . . . XXX . . . . XXX . . XXX . . XXX . .1 XXXXX XXXXXXX . . XXXXXXXXXXXXX XXXXXXXXXXX0 XXXXXXXXXXXXXXXXXXXXXXXXXXXXXXXXXXXXXXXXXXXGroup indicator matrix saved as dataset CliquesSetsClique co-membership matrix saved as dataset CliquesOverClique co-membership partition-by-actor indicator matrix saved as dataset CliquesPartDate and time: 15 Jan 99 13:38:43Running time: 00:00:01The output from the CLIQUE program is typical of most of the analytical procedures. It begins with a report of the parameter settings used to make the run. This makes it easy to interpret and reproduce the output at a later time. The next bit of output is a report on the number of cliques found, followed by a listing of each clique (one to a line). The first number on each line identifies the clique. The remaining numbers identify the actors that belong in that clique. If the data had contained actor labels (names), you would see names instead.After the listing of cliques is an actor-by-actor clique co-membership matrix that gives the number of cliques each pair of actors has in common. This is the basis for the next table, which gives the results of a single-link hierarchical clustering of the co-membership matrix. If you would prefer a different clustering algorithm, you are free to submit the co-membership matrix, which is automatically saved , to the clustering program of your choice. The final bit of output is a set of statements indicating what output files were created by the procedure. The main output file is a clique-by-actor binary matrix that indicates which actors belong to which clique. This matrix can be analyzed further, or used to extract a subgraph from the larger network using the Data>Extract procedure. For example, if you want to pull out just the network of relationships among members of, say, the fourth clique, just tell the Extract program what the name of the original dataset is, what the name of the clique-by-actor indicator matrix is (the default is CliquesSets) and which clique you want (#4 specified as ROW 4). That's all there is to it.2.4 The Log FileTextual output such as shown above is normally written to a text file in the \windows\system directory whose default name is Log File Number x, where x is an integer. When you run an analysis, the program writes output to the Log File and then displays the contents to the screen the number of the file is displayed in a box in the tool bar. The file can edited, saved, printed in the normal way using the buttons and options on the toolbar. When you run another procedure a new Log File with a number one higher than the last is created. You may leave Log Files open on the screen or you may close them. You can re-open a previously closed Log Files by clicking on File>Edit Previous Log File, this opens up a window which displays the number of the last Log File and gives a list of the。
NetScaler 配置手册

目录1项目背景 (2)2 实施准备 (2)2.1 NetScaler IP准备 (2)2.2 IP地址类型: (2)3 设备的初始化进入 (2)4 配置网络路由 (6)5 license的导入 (7)6 LDAP认证服务器配置 (9)7虚拟IP配置 (13)8 配置证书请求文件 (14)9证书的生成及上传 (17)10 虚拟服务器AG的建立和配置 (22)10.1 为虚拟服务器配置profile (22)10.2 为虚拟服务器配置polices (24)10.3开始配置 (24)11 WI配置 (27)11.1 WI安装根证书安装 (28)11.2 新建一个Wi站点 (32)11.3 站点的配置 (36)12 Sslvpn配置 (39)12.1 配置vpn用户可访问的应用资源 (39)12.2 为该资源建立一个标签 (40)12.3 为VPN配置profiles (41)12.4 建立polices并与profiles建立关联 (43)12.5配置DNS (43)12.6 测试VPN访问 (48)13 邮件负载配置 (49)13.1 配置两个服务器用于负载 (49)13.2添加虚拟服务器 (51)1项目背景2 实施准备2.1 NetScaler IP准备2.2 IP地址类型:NSIP:NetScaler设备管理IP,每台设备单独拥有一个。
MIP:NetScaler与认证服务器通信,提供ADNS服务,通过MEP协议与远程站点通信IP。
VIP:虚拟AG服务器IP。
3 设备的初始化进入设备初始IP:192.168.100.1/255.255.0.0。
初始用户名密码:nsroot/nsroot。
在登陆进去后按照安装配置向导进行设备基本的配置:启用SSL功能和Access Gateway功能能启用SSL4 配置网络路由如果在初始化配置中未配置默认路由,此处需要在Network --Routes部分添加路由.5 license的导入进入System界面中点击license选项,会看见有哪些授权了哪些没授权点击下面的Manage license.选择已经购买的license—Add找到license文件后点击Select。
计算机网络第五版(英文版)
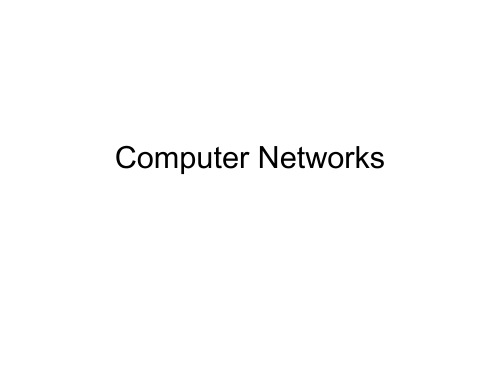
• Co-operator: EPFL • 正申请上海市科委项目
This is a Bilingual Course
• Why we give this bilingual course?
– To meet the needs of excellent engineer training program and internationalization
– Final exam (40%), middle exam (20%), experiments (20%), reports/problems (10%), and others (10%) – After study Chapter 4, there will be mid-term exam
– Deployment Models
• • • • Private Cloud Community Cloud Public Cloud Hybrid Cloud
Source: Wikipedia
1.2 Uses of Computer Networks (5)
• Client-server module
Source: David Lazer et al., “Computational Social Science”, SCIENCE, 323, 721-724 (2009)
Exploring Study (1)
1. Use complex network theory and dynamics of human behavior (DHB) to analyze social networks and optimize social networking service (SNS). 2. Research on opinion evolution and interference model on Internet, specially on social networking service (SNS).
Microeconometrics using stata
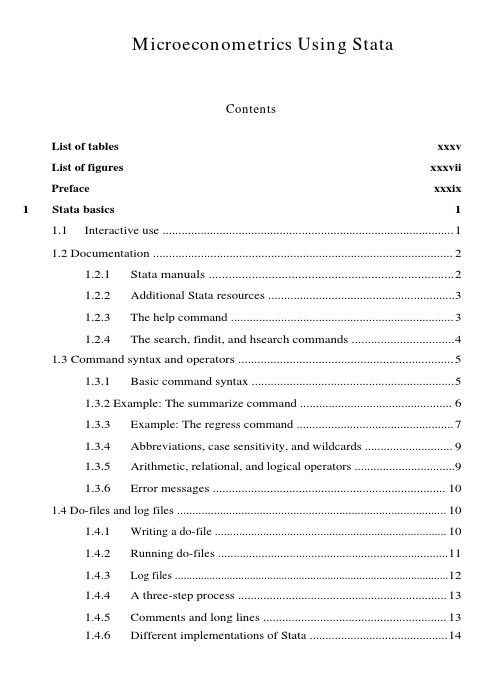
Microeconometrics Using StataContentsList of tables xxxv List of figures xxxvii Preface xxxix 1Stata basics1............................................................................................1.1Interactive use 1..............................................................................................1.2 Documentation 2..........................................................................1.2.1Stata manuals 2...........................................................1.2.2Additional Stata resources 3.......................................................................1.2.3The help command 3................................1.2.4The search, findit, and hsearch commands 41.3 Command syntax and operators 5...................................................................................................................................1.3.1Basic command syntax 5................................................1.3.2 Example: The summarize command 61.3.3Example: The regress command 7..............................................................................1.3.4Abbreviations, case sensitivity, and wildcards 9................................1.3.5Arithmetic, relational, and logical operators 9.........................................................................1.3.6Error messages 10........................................................................................1.4 Do-files and log files 10.............................................................................1.4.1Writing a do-file 101.4.2Running do-files 11.........................................................................................................................................................................1.4.3Log files 12..................................................................1.4.4 A three-step process 131.4.5Comments and long lines 13......................................................................................................1.4.6Different implementations of Stata 141.5Scalars and matrices (15)1.5.1Scalars (15)1.5.2Matrices (15)1.6 Using results from Stata commands (16)1.6.1Using results from the r-class command summarize (16)1.6.2Using results from the e-class command regress (17)1.7 Global and local macros (19)1.7.1Global macros (19)1.7.2Local macros (20)1.7.3Scalar or macro? (21)1.8 Looping commands (22)1.8.1The foreach loop (23)1.8.2The forvalues loop (23)1.8.3The while loop (24)1.8.4The continue command (24)1.9 Some useful commands (24)1.10 Template do-file (25)1.11 User-written commands (25)1.12 Stata resources (26)1.13 Exercises (26)2 Data management and graphics292.1Introduction (29)2.2 Types of data (29)2.2.1Text or ASCII data (30)2.2.2Internal numeric data (30)2.2.3String data (31)2.2.4Formats for displaying numeric data (31)2.3Inputting data (32)2.3.1General principles (32)2.3.2Inputting data already in Stata format (33)2.3.3Inputting data from the keyboard (34)2.3.4Inputting nontext data (34)2.3.5Inputting text data from a spreadsheet (35)2.3.6Inputting text data in free format (36)2.3.7Inputting text data in fixed format (36)2.3.8Dictionary files (37)2.3.9Common pitfalls (37)2.4 Data management (38)2.4.1PSID example (38)2.4.2Naming and labeling variables (41)2.4.3Viewing data (42)2.4.4Using original documentation (43)2.4.5Missing values (43)2.4.6Imputing missing data (45)2.4.7Transforming data (generate, replace, egen, recode) (45)The generate and replace commands (46)The egen command (46)The recode command (47)The by prefix (47)Indicator variables (47)Set of indicator variables (48)Interactions (49)Demeaning (50)2.4.8Saving data (51)2.4.9Selecting the sample (51)2.5 Manipulating datasets (53)2.5.1Ordering observations and variables (53)2.5.2Preserving and restoring a dataset (53)2.5.3Wide and long forms for a dataset (54)2.5.4Merging datasets (54)2.5.5Appending datasets (56)2.6 Graphical display of data (57)2.6.1Stata graph commands (57)Example graph commands (57)Saving and exporting graphs (58)Learning how to use graph commands (59)2.6.2Box-and-whisker plot (60)2.6.3Histogram (61)2.6.4Kernel density plot (62)2.6.5Twoway scatterplots and fitted lines (64)2.6.6Lowess, kernel, local linear, and nearest-neighbor regression652.6.7Multiple scatterplots (67)2.7 Stata resources (68)2.8Exercises (68)3Linear regression basics713.1Introduction (71)3.2 Data and data summary (71)3.2.1Data description (71)3.2.2Variable description (72)3.2.3Summary statistics (73)3.2.4More-detailed summary statistics (74)3.2.5Tables for data (75)3.2.6Statistical tests (78)3.2.7Data plots (78)3.3Regression in levels and logs (79)3.3.1Basic regression theory (79)3.3.2OLS regression and matrix algebra (80)3.3.3Properties of the OLS estimator (81)3.3.4Heteroskedasticity-robust standard errors (82)3.3.5Cluster–robust standard errors (82)3.3.6Regression in logs (83)3.4Basic regression analysis (84)3.4.1Correlations (84)3.4.2The regress command (85)3.4.3Hypothesis tests (86)3.4.4Tables of output from several regressions (87)3.4.5Even better tables of regression output (88)3.5Specification analysis (90)3.5.1Specification tests and model diagnostics (90)3.5.2Residual diagnostic plots (91)3.5.3Influential observations (92)3.5.4Specification tests (93)Test of omitted variables (93)Test of the Box–Cox model (94)Test of the functional form of the conditional mean (95)Heteroskedasticity test (96)Omnibus test (97)3.5.5Tests have power in more than one direction (98)3.6Prediction (100)3.6.1In-sample prediction (100)3.6.2Marginal effects (102)3.6.3Prediction in logs: The retransformation problem (103)3.6.4Prediction exercise (104)3.7 Sampling weights (105)3.7.1Weights (106)3.7.2Weighted mean (106)3.7.3Weighted regression (107)3.7.4Weighted prediction and MEs (109)3.8 OLS using Mata (109)3.9Stata resources (111)3.10 Exercises (111)4Simulation1134.1Introduction (113)4.2 Pseudorandom-number generators: Introduction (114)4.2.1Uniform random-number generation (114)4.2.2Draws from normal (116)4.2.3Draws from t, chi-squared, F, gamma, and beta (117)4.2.4 Draws from binomial, Poisson, and negative binomial . . . (118)Independent (but not identically distributed) draws frombinomial (118)Independent (but not identically distributed) draws fromPoisson (119)Histograms and density plots (120)4.3 Distribution of the sample mean (121)4.3.1Stata program (122)4.3.2The simulate command (123)4.3.3Central limit theorem simulation (123)4.3.4The postfile command (124)4.3.5Alternative central limit theorem simulation (125)4.4 Pseudorandom-number generators: Further details (125)4.4.1Inverse-probability transformation (126)4.4.2Direct transformation (127)4.4.3Other methods (127)4.4.4Draws from truncated normal (128)4.4.5Draws from multivariate normal (129)Direct draws from multivariate normal (129)Transformation using Cholesky decomposition (130)4.4.6Draws using Markov chain Monte Carlo method (130)4.5 Computing integrals (132)4.5.1Quadrature (133)4.5.2Monte Carlo integration (133)4.5.3Monte Carlo integration using different S (134)4.6Simulation for regression: Introduction (135)4.6.1Simulation example: OLS with X2 errors (135)4.6.2Interpreting simulation output (138)Unbiasedness of estimator (138)Standard errors (138)t statistic (138)Test size (139)Number of simulations (140)4.6.3Variations (140)Different sample size and number of simulations (140)Test power (140)Different error distributions (141)4.6.4Estimator inconsistency (141)4.6.5Simulation with endogenous regressors (142)4.7Stata resources (144)4.8Exercises (144)5GLS regression1475.1Introduction (147)5.2 GLS and FGLS regression (147)5.2.1GLS for heteroskedastic errors (147)5.2.2GLS and FGLS (148)5.2.3Weighted least squares and robust standard errors (149)5.2.4Leading examples (149)5.3 Modeling heteroskedastic data (150)5.3.1Simulated dataset (150)5.3.2OLS estimation (151)5.3.3Detecting heteroskedasticity (152)5.3.4FGLS estimation (154)5.3.5WLS estimation (156)5.4System of linear regressions (156)5.4.1SUR model (156)5.4.2The sureg command (157)5.4.3Application to two categories of expenditures (158)5.4.4Robust standard errors (160)5.4.5Testing cross-equation constraints (161)5.4.6Imposing cross-equation constraints (162)5.5Survey data: Weighting, clustering, and stratification (163)5.5.1Survey design (164)5.5.2Survey mean estimation (167)5.5.3Survey linear regression (167)5.6Stata resources (169)5.7Exercises (169)6Linear instrumental-variables regression1716.1Introduction (171)6.2 IV estimation (171)6.2.1Basic IV theory (171)6.2.2Model setup (173)6.2.3IV estimators: IV, 2SLS, and GMM (174)6.2.4Instrument validity and relevance (175)6.2.5Robust standard-error estimates (176)6.3 IV example (177)6.3.1The ivregress command (177)6.3.2Medical expenditures with one endogenous regressor . . . (178)6.3.3Available instruments (179)6.3.4IV estimation of an exactly identified model (180)6.3.5IV estimation of an overidentified model (181)6.3.6Testing for regressor endogeneity (182)6.3.7Tests of overidentifying restrictions (185)6.3.8IV estimation with a binary endogenous regressor (186)6.4 Weak instruments (188)6.4.1Finite-sample properties of IV estimators (188)6.4.2Weak instruments (189)Diagnostics for weak instruments (189)Formal tests for weak instruments (190)6.4.3The estat firststage command (191)6.4.4Just-identified model (191)6.4.5Overidentified model (193)6.4.6More than one endogenous regressor (195)6.4.7Sensitivity to choice of instruments (195)6.5 Better inference with weak instruments (197)6.5.1Conditional tests and confidence intervals (197)6.5.2LIML estimator (199)6.5.3Jackknife IV estimator (199)6.5.4 Comparison of 2SLS, LIML, JIVE, and GMM (200)6.6 3SLS systems estimation (201)6.7Stata resources (203)6.8Exercises (203)7Quantile regression2057.1Introduction (205)7.2 QR (205)7.2.1Conditional quantiles (206)7.2.2Computation of QR estimates and standard errors (207)7.2.3The qreg, bsqreg, and sqreg commands (207)7.3 QR for medical expenditures data (208)7.3.1Data summary (208)7.3.2QR estimates (209)7.3.3Interpretation of conditional quantile coefficients (210)7.3.4Retransformation (211)7.3.5Comparison of estimates at different quantiles (212)7.3.6Heteroskedasticity test (213)7.3.7Hypothesis tests (214)7.3.8Graphical display of coefficients over quantiles (215)7.4 QR for generated heteroskedastic data (216)7.4.1Simulated dataset (216)7.4.2QR estimates (219)7.5 QR for count data (220)7.5.1Quantile count regression (221)7.5.2The qcount command (222)7.5.3Summary of doctor visits data (222)7.5.4Results from QCR (224)7.6Stata resources (226)7.7Exercises (226)8Linear panel-data models: Basics2298.1Introduction (229)8.2 Panel-data methods overview (229)8.2.1Some basic considerations (230)8.2.2Some basic panel models (231)Individual-effects model (231)Fixed-effects model (231)Random-effects model (232)Pooled model or population-averaged model (232)Two-way-effects model (232)Mixed linear models (233)8.2.3Cluster-robust inference (233)8.2.4The xtreg command (233)8.2.5Stata linear panel-data commands (234)8.3 Panel-data summary (234)8.3.1Data description and summary statistics (234)8.3.2Panel-data organization (236)8.3.3Panel-data description (237)8.3.4Within and between variation (238)8.3.5Time-series plots for each individual (241)8.3.6Overall scatterplot (242)8.3.7Within scatterplot (243)8.3.8Pooled OLS regression with cluster—robust standard errors ..2448.3.9Time-series autocorrelations for panel data (245)8.3.10 Error correlation in the RE model (247)8.4 Pooled or population-averaged estimators (248)8.4.1Pooled OLS estimator (248)8.4.2Pooled FGLS estimator or population-averaged estimator (248)8.4.3The xtreg, pa command (249)8.4.4Application of the xtreg, pa command (250)8.5 Within estimator (251)8.5.1Within estimator (251)8.5.2The xtreg, fe command (251)8.5.3Application of the xtreg, fe command (252)8.5.4Least-squares dummy-variables regression (253)8.6 Between estimator (254)8.6.1Between estimator (254)8.6.2Application of the xtreg, be command (255)8.7 RE estimator (255)8.7.1RE estimator (255)8.7.2The xtreg, re command (256)8.7.3Application of the xtreg, re command (256)8.8 Comparison of estimators (257)8.8.1Estimates of variance components (257)8.8.2Within and between R-squared (258)8.8.3Estimator comparison (258)8.8.4Fixed effects versus random effects (259)8.8.5Hausman test for fixed effects (260)The hausman command (260)Robust Hausman test (261)8.8.6Prediction (262)8.9 First-difference estimator (263)8.9.1First-difference estimator (263)8.9.2Strict and weak exogeneity (264)8.10 Long panels (265)8.10.1 Long-panel dataset (265)8.10.2 Pooled OLS and PFGLS (266)8.10.3 The xtpcse and xtgls commands (267)8.10.4 Application of the xtgls, xtpcse, and xtscc commands . . . (268)8.10.5 Separate regressions (270)8.10.6 FE and RE models (271)8.10.7 Unit roots and cointegration (272)8.11 Panel-data management (274)8.11.1 Wide-form data (274)8.11.2 Convert wide form to long form (274)8.11.3 Convert long form to wide form (275)8.11.4 An alternative wide-form data (276)8.12 Stata resources (278)8.13 Exercises (278)9Linear panel-data models: Extensions2819.1Introduction (281)9.2 Panel IV estimation (281)9.2.1Panel IV (281)9.2.2The xtivreg command (282)9.2.3Application of the xtivreg command (282)9.2.4Panel IV extensions (284)9.3 Hausman-Taylor estimator (284)9.3.1Hausman-Taylor estimator (284)9.3.2The xthtaylor command (285)9.3.3Application of the xthtaylor command (285)9.4 Arellano-Bond estimator (287)9.4.1Dynamic model (287)9.4.2IV estimation in the FD model (288)9.4.3 The xtabond command (289)9.4.4Arellano-Bond estimator: Pure time series (290)9.4.5Arellano-Bond estimator: Additional regressors (292)9.4.6Specification tests (294)9.4.7 The xtdpdsys command (295)9.4.8 The xtdpd command (297)9.5 Mixed linear models (298)9.5.1Mixed linear model (298)9.5.2 The xtmixed command (299)9.5.3Random-intercept model (300)9.5.4Cluster-robust standard errors (301)9.5.5Random-slopes model (302)9.5.6Random-coefficients model (303)9.5.7Two-way random-effects model (304)9.6 Clustered data (306)9.6.1Clustered dataset (306)9.6.2Clustered data using nonpanel commands (306)9.6.3Clustered data using panel commands (307)9.6.4Hierarchical linear models (310)9.7Stata resources (311)9.8Exercises (311)10 Nonlinear regression methods31310.1 Introduction (313)10.2 Nonlinear example: Doctor visits (314)10.2.1 Data description (314)10.2.2 Poisson model description (315)10.3 Nonlinear regression methods (316)10.3.1 MLE (316)10.3.2 The poisson command (317)10.3.3 Postestimation commands (318)10.3.4 NLS (319)10.3.5 The nl command (319)10.3.6 GLM (321)10.3.7 The glm command (321)10.3.8 Other estimators (322)10.4 Different estimates of the VCE (323)10.4.1 General framework (323)10.4.2 The vce() option (324)10.4.3 Application of the vce() option (324)10.4.4 Default estimate of the VCE (326)10.4.5 Robust estimate of the VCE (326)10.4.6 Cluster–robust estimate of the VCE (327)10.4.7 Heteroskedasticity- and autocorrelation-consistent estimateof the VCE (328)10.4.8 Bootstrap standard errors (328)10.4.9 Statistical inference (329)10.5 Prediction (329)10.5.1 The predict and predictnl commands (329)10.5.2 Application of predict and predictnl (330)10.5.3 Out-of-sample prediction (331)10.5.4 Prediction at a specified value of one of the regressors (321)10.5.5 Prediction at a specified value of all the regressors (332)10.5.6 Prediction of other quantities (333)10.6 Marginal effects (333)10.6.1 Calculus and finite-difference methods (334)10.6.2 MEs estimates AME, MEM, and MER (334)10.6.3 Elasticities and semielasticities (335)10.6.4 Simple interpretations of coefficients in single-index models (336)10.6.5 The mfx command (337)10.6.6 MEM: Marginal effect at mean (337)Comparison of calculus and finite-difference methods . . . (338)10.6.7 MER: Marginal effect at representative value (338)10.6.8 AME: Average marginal effect (339)10.6.9 Elasticities and semielasticities (340)10.6.10 AME computed manually (342)10.6.11 Polynomial regressors (343)10.6.12 Interacted regressors (344)10.6.13 Complex interactions and nonlinearities (344)10.7 Model diagnostics (345)10.7.1 Goodness-of-fit measures (345)10.7.2 Information criteria for model comparison (346)10.7.3 Residuals (347)10.7.4 Model-specification tests (348)10.8 Stata resources (349)10.9 Exercises (349)11 Nonlinear optimization methods35111.1 Introduction (351)11.2 Newton–Raphson method (351)11.2.1 NR method (351)11.2.2 NR method for Poisson (352)11.2.3 Poisson NR example using Mata (353)Core Mata code for Poisson NR iterations (353)Complete Stata and Mata code for Poisson NR iterations (353)11.3 Gradient methods (355)11.3.1 Maximization options (355)11.3.2 Gradient methods (356)11.3.3 Messages during iterations (357)11.3.4 Stopping criteria (357)11.3.5 Multiple maximums (357)11.3.6 Numerical derivatives (358)11.4 The ml command: if method (359)11.4.1 The ml command (360)11.4.2 The If method (360)11.4.3 Poisson example: Single-index model (361)11.4.4 Negative binomial example: Two-index model (362)11.4.5 NLS example: Nonlikelihood model (363)11.5 Checking the program (364)11.5.1 Program debugging using ml check and ml trace (365)11.5.2 Getting the program to run (366)11.5.3 Checking the data (366)11.5.4 Multicollinearity and near coilinearity (367)11.5.5 Multiple optimums (368)11.5.6 Checking parameter estimation (369)11.5.7 Checking standard-error estimation (370)11.6 The ml command: d0, dl, and d2 methods (371)11.6.1 Evaluator functions (371)11.6.2 The d0 method (373)11.6.3 The dl method (374)11.6.4 The dl method with the robust estimate of the VCE (374)11.6.5 The d2 method (375)11.7 The Mata optimize() function (376)11.7.1 Type d and v evaluators (376)11.7.2 Optimize functions (377)11.7.3 Poisson example (377)Evaluator program for Poisson MLE (377)The optimize() function for Poisson MLE (378)11.8 Generalized method of moments (379)11.8.1 Definition (380)11.8.2 Nonlinear IV example (380)11.8.3 GMM using the Mata optimize() function (381)11.9 Stata resources (383)11.10 Exercises (383)12 Testing methods38512.1 Introduction (385)12.2 Critical values and p-values (385)12.2.1 Standard normal compared with Student's t (386)12.2.2 Chi-squared compared with F (386)12.2.3 Plotting densities (386)12.2.4 Computing p-values and critical values (388)12.2.5 Which distributions does Stata use? (389)12.3 Wald tests and confidence intervals (389)12.3.1 Wald test of linear hypotheses (389)12.3.2 The test command (391)Test single coefficient (392)Test several hypotheses (392)Test of overall significance (393)Test calculated from retrieved coefficients and VCE (393)12.3.3 One-sided Wald tests (394)12.3.4 Wald test of nonlinear hypotheses (delta method) (395)12.3.5 The testnl command (395)12.3.6 Wald confidence intervals (396)12.3.7 The lincom command (396)12.3.8 The nlcom command (delta method) (397)12.3.9 Asymmetric confidence intervals (398)12.4 Likelihood-ratio tests (399)12.4.1 Likelihood-ratio tests (399)12.4.2 The lrtest command (401)12.4.3 Direct computation of LR tests (401)12.5 Lagrange multiplier test (or score test) (402)12.5.1 LM tests (402)12.5.2 The estat command (403)12.5.3 LM test by auxiliary regression (403)12.6 Test size and power (405)12.6.1 Simulation DGP: OLS with chi-squared errors (405)12.6.2 Test size (406)12.6.3 Test power (407)12.6.4 Asymptotic test power (410)12.7 Specification tests (411)12.7.1 Moment-based tests (411)12.7.2 Information matrix test (411)12.7.3 Chi-squared goodness-of-fit test (412)12.7.4 Overidentifying restrictions test (412)12.7.5 Hausman test (412)12.7.6 Other tests (413)12.8 Stata resources (413)12.9 Exercises (413)13 Bootstrap methods41513.1 Introduction (415)13.2 Bootstrap methods (415)13.2.1 Bootstrap estimate of standard error (415)13.2.2 Bootstrap methods (416)13.2.3 Asymptotic refinement (416)13.2.4 Use the bootstrap with caution (416)13.3 Bootstrap pairs using the vce(bootstrap) option (417)13.3.1 Bootstrap-pairs method to estimate VCE (417)13.3.2 The vce(bootstrap) option (418)13.3.3 Bootstrap standard-errors example (418)13.3.4 How many bootstraps? (419)13.3.5 Clustered bootstraps (420)13.3.6 Bootstrap confidence intervals (421)13.3.7 The postestimation estat bootstrap command (422)13.3.8 Bootstrap confidence-intervals example (423)13.3.9 Bootstrap estimate of bias (423)13.4 Bootstrap pairs using the bootstrap command (424)13.4.1 The bootstrap command (424)13.4.2 Bootstrap parameter estimate from a Stata estimationcommand (425)13.4.3 Bootstrap standard error from a Stata estimation command (426)13.4.4 Bootstrap standard error from a user-written estimationcommand (426)13.4.5 Bootstrap two-step estimator (427)13.4.6 Bootstrap Hausman test (429)13.4.7 Bootstrap standard error of the coefficient of variation . . (430)13.5 Bootstraps with asymptotic refinement (431)13.5.1 Percentile-t method (431)13.5.2 Percentile-t Wald test (432)13.5.3 Percentile-t Wald confidence interval (433)13.6 Bootstrap pairs using bsample and simulate (434)13.6.1 The bsample command (434)13.6.2 The bsample command with simulate (434)13.6.3 Bootstrap Monte Carlo exercise (436)13.7 Alternative resampling schemes (436)13.7.1 Bootstrap pairs (437)13.7.2 Parametric bootstrap (437)13.7.3 Residual bootstrap (439)13.7.4 Wild bootstrap (440)13.7.5 Subsampling (441)13.8 The jackknife (441)13.8.1 Jackknife method (441)13.8.2 The vice(jackknife) option and the jackknife command . . (442)13.9 Stata resources (442)13.10 Exercises (442)14 Binary outcome models44514.1 Introduction (445)14.2 Some parametric models (445)14.2.1 Basic model (445)14.2.2 Logit, probit, linear probability, and clog-log models . . . (446)14.3 Estimation (446)14.3.1 Latent-variable interpretation and identification (447)14.3.2 ML estimation (447)14.3.3 The logit and probit commands (448)14.3.4 Robust estimate of the VCE (448)14.3.5 OLS estimation of LPM (448)14.4 Example (449)14.4.1 Data description (449)14.4.2 Logit regression (450)14.4.3 Comparison of binary models and parameter estimates . (451)14.5 Hypothesis and specification tests (452)14.5.1 Wald tests (453)14.5.2 Likelihood-ratio tests (453)14.5.3 Additional model-specification tests (454)Lagrange multiplier test of generalized logit (454)Heteroskedastic probit regression (455)14.5.4 Model comparison (456)14.6 Goodness of fit and prediction (457)14.6.1 Pseudo-R2 measure (457)14.6.2 Comparing predicted probabilities with sample frequencies (457)14.6.3 Comparing predicted outcomes with actual outcomes . . . (459)14.6.4 The predict command for fitted probabilities (460)14.6.5 The prvalue command for fitted probabilities (461)14.7 Marginal effects (462)14.7.1 Marginal effect at a representative value (MER) (462)14.7.2 Marginal effect at the mean (MEM) (463)14.7.3 Average marginal effect (AME) (464)14.7.4 The prchange command (464)14.8 Endogenous regressors (465)14.8.1 Example (465)14.8.2 Model assumptions (466)14.8.3 Structural-model approach (467)The ivprobit command (467)Maximum likelihood estimates (468)Two-step sequential estimates (469)14.8.4 IVs approach (471)14.9 Grouped data (472)14.9.1 Estimation with aggregate data (473)14.9.2 Grouped-data application (473)14.10 Stata resources (475)14.11 Exercises (475)15 Multinomial models47715.1 Introduction (477)15.2 Multinomial models overview (477)15.2.1 Probabilities and MEs (477)15.2.2 Maximum likelihood estimation (478)15.2.3 Case-specific and alternative-specific regressors (479)15.2.4 Additive random-utility model (479)15.2.5 Stata multinomial model commands (480)15.3 Multinomial example: Choice of fishing mode (480)15.3.1 Data description (480)15.3.2 Case-specific regressors (483)15.3.3 Alternative-specific regressors (483)15.4 Multinomial logit model (484)15.4.1 The mlogit command (484)15.4.2 Application of the mlogit command (485)15.4.3 Coefficient interpretation (486)15.4.4 Predicted probabilities (487)15.4.5 MEs (488)15.5 Conditional logit model (489)15.5.1 Creating long-form data from wide-form data (489)15.5.2 The asclogit command (491)15.5.3 The clogit command (491)15.5.4 Application of the asclogit command (492)15.5.5 Relationship to multinomial logit model (493)15.5.6 Coefficient interpretation (493)15.5.7 Predicted probabilities (494)15.5.8 MEs (494)15.6 Nested logit model (496)15.6.1 Relaxing the independence of irrelevant alternatives as-sumption (497)15.6.2 NL model (497)15.6.3 The nlogit command (498)15.6.4 Model estimates (499)15.6.5 Predicted probabilities (501)15.6.6 MEs (501)15.6.7 Comparison of logit models (502)15.7 Multinomial probit model (503)15.7.1 MNP (503)15.7.2 The mprobit command (503)15.7.3 Maximum simulated likelihood (504)15.7.4 The asmprobit command (505)15.7.5 Application of the asmprobit command (505)15.7.6 Predicted probabilities and MEs (507)15.8 Random-parameters logit (508)15.8.1 Random-parameters logit (508)15.8.2 The mixlogit command (508)15.8.3 Data preparation for mixlogit (509)15.8.4 Application of the mixlogit command (509)15.9 Ordered outcome models (510)15.9.1 Data summary (511)15.9.2 Ordered outcomes (512)15.9.3 Application of the ologit command (512)15.9.4 Predicted probabilities (513)15.9.5 MEs (513)15.9.6 Other ordered models (514)15.10 Multivariate outcomes (514)15.10.1 Bivariate probit (515)15.10.2 Nonlinear SUR (517)15.11 Stata resources (518)15.12 Exercises (518)16 Tobit and selection models52116.1 Introduction (521)16.2 Tobit model (521)16.2.1 Regression with censored data (521)16.2.2 Tobit model setup (522)16.2.3 Unknown censoring point (523)。
pytorch net函数
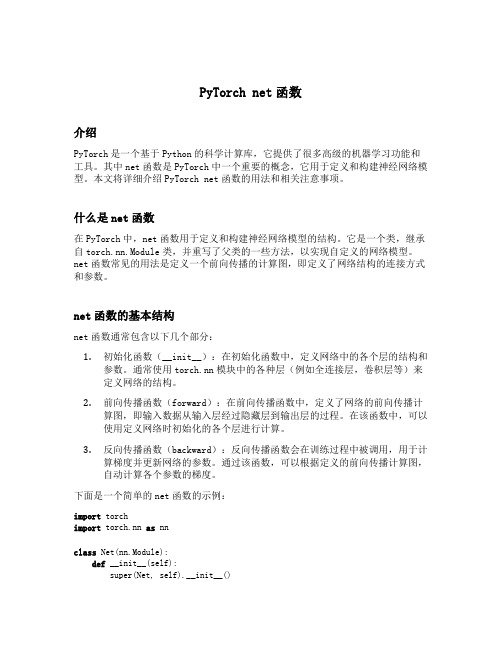
PyTorch net函数介绍PyTorch是一个基于Python的科学计算库,它提供了很多高级的机器学习功能和工具。
其中net函数是PyTorch中一个重要的概念,它用于定义和构建神经网络模型。
本文将详细介绍PyTorch net函数的用法和相关注意事项。
什么是net函数在PyTorch中,net函数用于定义和构建神经网络模型的结构。
它是一个类,继承自torch.nn.Module类,并重写了父类的一些方法,以实现自定义的网络模型。
net函数常见的用法是定义一个前向传播的计算图,即定义了网络结构的连接方式和参数。
net函数的基本结构net函数通常包含以下几个部分:1.初始化函数(__init__):在初始化函数中,定义网络中的各个层的结构和参数。
通常使用torch.nn模块中的各种层(例如全连接层,卷积层等)来定义网络的结构。
2.前向传播函数(forward):在前向传播函数中,定义了网络的前向传播计算图,即输入数据从输入层经过隐藏层到输出层的过程。
在该函数中,可以使用定义网络时初始化的各个层进行计算。
3.反向传播函数(backward):反向传播函数会在训练过程中被调用,用于计算梯度并更新网络的参数。
通过该函数,可以根据定义的前向传播计算图,自动计算各个参数的梯度。
下面是一个简单的net函数的示例:import torchimport torch.nn as nnclass Net(nn.Module):def __init__(self):super(Net, self).__init__()self.fc1 = nn.Linear(10, 20)self.fc2 = nn.Linear(20, 2)def forward(self, x):x = self.fc1(x)x = self.fc2(x)return x在该示例中,Net类继承自nn.Module类,并重写了__init__和forward方法。
PoC手册 - 3 增强功能 - Lab 01 NetScaler基本安装及配置
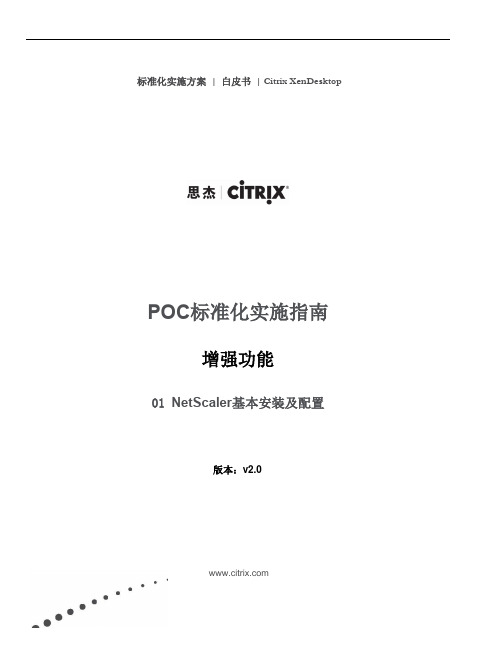
标准化实施方案| 白皮书| Citrix XenDesktopPOC标准化实施指南增强功能01 NetScaler基本安装及配置版本:v2.0第1章基本过程 (3)第2章安装配置环境一览表 (3)第3章安装NetScaler VPX及初始化 (4)3.1 安装NetScaler VPX (4)3.2 基本配置 (5)第4章创建证书 (9)4.1 安装配置Windows CA服务 (9)4.2 创建Certificate Request文件(.csr) (14)4.3 创建Certificate文件(.cer) (17)第5章配置NetScaler (22)5.1 创建Access Gateway 的Virtual Server (22)5.2 创建Load Balancing 的Virtual Server (27)第6章为NetScaler创建一个专用WI站点 (30)第7章测试并验证Web Site (33)第8章变更默认的PNAgent Service site (39)产品版本 (40)修正历史 (41)第1章基本过程本章节介绍了通过NetScaler实现ica proxy的基本过程。
其包括了:∙NetScaler的安装∙Windows CA的安装∙配置NetScaler本章节开始前,请确认“PoC手册- 1 基础环境”的基础构架安装的环境均已完成。
并且确认有有效的测试NetScaler License文件供此测试使用。
另:如在PoC Runbook hands on lab培训中,请参考《PoC手册- 附录- Windows 路由器- NAT》创建一台Windows路由器以模拟内外网环境。
第2章安装配置环境一览表通过NetScaler实现ica proxy有多种实现方式,本文档只作为PoC Runbook使用,考虑到简化部署:∙不使用AG做身份认证∙NetScaler对外只有一个IP地址即Access Gateway的Virtual Server IP 当用户请求Web站点时,Access Gateway Virtual Server将把请求转给Load Balancing Virtual Server。
QCSG 110017.35-2012南方电网一体化电网运行智能系统技术规范 第3部分:数据 第5篇:电网公共信息模型规范
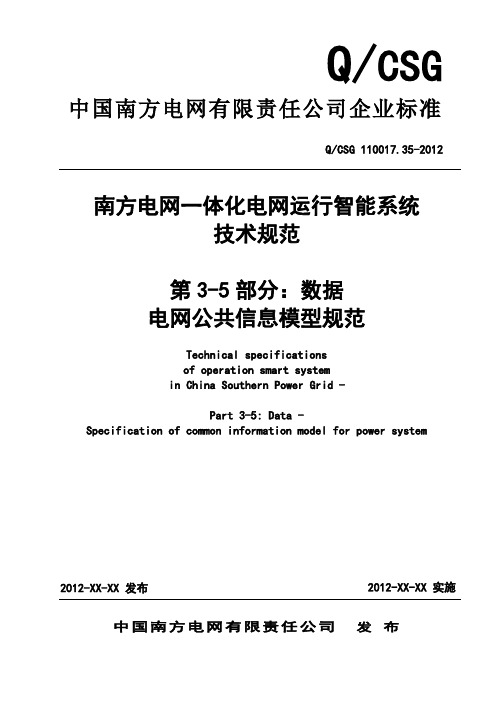
Q/CSG 中国南方电网有限责任公司企业标准中国南方电网有限责任公司 发 布Q/CSG 110017.35-2012目次前言 (III)1范围 (1)2规范性引用文件 (1)3术语和定义 (1)4总则 (1)5建模表达方法 (1)5.1建模描述方法 (1)5.2CIM包 (1)5.3CIM类和关系 (2)6CIM包 (2)6.1核心包(Core) (2)6.1.1Core包描述 (3)6.1.2各类的描述 (4)6.1.3间隔类(Bay) (4)6.1.4命名类(Naming) (4)6.1.5电力系统资源类型类(PSRType) (4)6.1.6子控制区类(SubControlArea) (5)6.1.7变电站类(Substation) (6)6.2域包(Domain) (6)6.2.1Domain包描述 (6)6.2.2各类的描述 (6)6.3发电包(Generation) (6)6.3.1Generation包描述 (6)6.3.2Production包的描述 (7)6.3.3Production各类的描述 (7)6.3.4GenerationDynamics包的描述 (14)6.3.5GenerationDynamics各类的描述 (14)6.4负荷模型包(LoadModel) (14)6.4.1负荷模型包的描述 (14)6.4.2LoadModel各类的描述 (14)6.5量测包(Meas) (14)6.5.1量测包的描述 (14)6.5.2Meas包各类的描述 (15)6.6停运包(Outage) (25)6.6.1停运包的描述 (25)6.6.2Outage包各类的描述 (25)6.7保护包(Protection) (25)6.7.1保护包的描述 (25)6.7.2Protection包各类的描述 (25)6.8拓扑包(Topology) (26)6.8.1Topology包描述 (26)6.8.2各类的描述 (26)6.9电线包(Wires) (26)6.9.1Wires包描述 (26)6.9.2各类的描述 (26)6.10SCADA包(SCADA) (28)IQ/CSG 110017.35-20126.10.1SCADA包描述 (28)6.10.2各类的描述 (29)6.11计量包(Metering) (34)6.11.1计量包描述 (34)6.11.2各类的描述 (35)6.12通用包(Common) (39)6.12.1通用包的描述 (39)6.12.2各类的描述 (39)6.13辅助设备包(AuxiliaryEquipment) (40)6.13.1辅助设备包的描述 (40)6.13.2各类的描述 (40)6.14暂态模型包(TransientModel) (41)6.14.1暂态模型包的描述 (41)6.14.2各类的描述 (41)附录A (规范性附录)MeasurementType扩展 (58)附录B (规范性附录)RDF文件命名空间 (58)IIQ/CSG 110017.35-2012前言为落实公司二次一体化的工作要求,提高电网一体化运行水平,解决二次系统种类繁杂、运行信息割裂、缺乏统一的建设和运行标准等问题,经研究国内外电网运行技术支持系统建设思路和实践案例,提出建设一体化电网运行智能系统的总体解决方案。
(Linyuan)Link prediction in complex networks-A survey
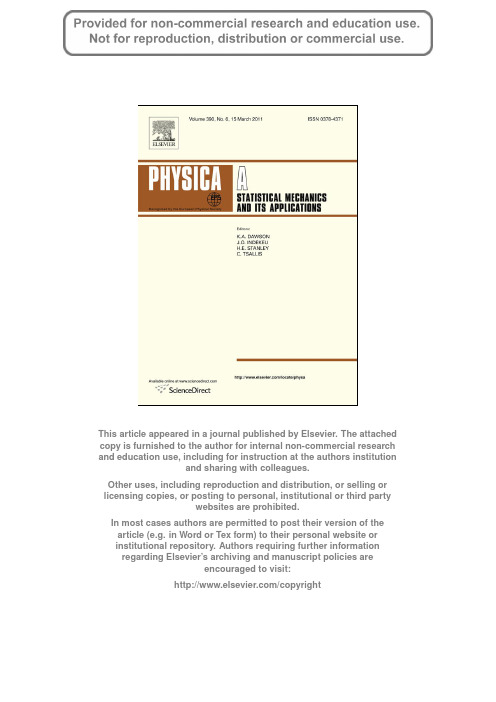
Author's personal copy
Physica A 390 (2011) 1150–1170
Contents lists available at ScienceDirect
Physica A
journal homepage: /locate/physa
Article history: Received 5 October 2010 Received in revised form 10 November 2010 Available online 2 December 2010 Keywords: Link prediction Complex networks Node similarity Maximum likelihood methods Probabilistic models
article
info
abstract
Link prediction in complex networks has attracted increasing attention from both physical and computer science communities. The algorithms can be used to extract missing information, identify spurious interactions, evaluate network evolving mechanisms, and so on. This article summaries recent progress about link prediction algorithms, emphasizing on the contributions from physical perspectives and approaches, such as the random-walkbased methods and the maximum likelihood methods. We also introduce three typical applications: reconstruction of networks, evaluation of network evolving mechanism and classification of partially labeled networks. Finally, we introduce some applications and outline future challenges of link prediction algorithms. © 2010 Elsevier B.V. All rights reserved.
ACM-GIS%202006-A%20Peer-to-Peer%20Spatial%20Cloaking%20Algorithm%20for%20Anonymous%20Location-based%
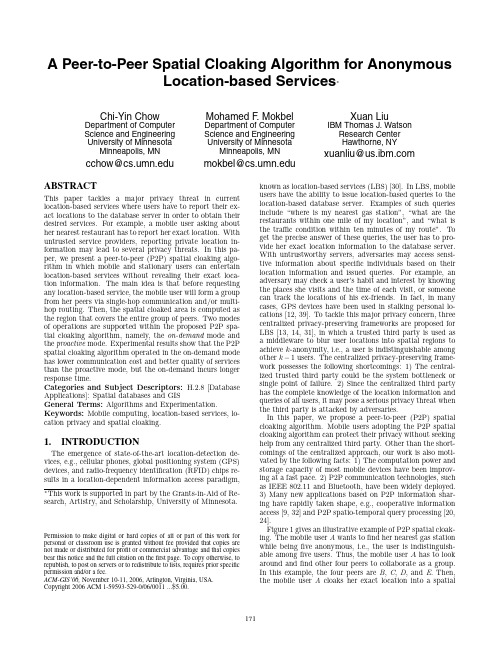
A Peer-to-Peer Spatial Cloaking Algorithm for AnonymousLocation-based Services∗Chi-Yin Chow Department of Computer Science and Engineering University of Minnesota Minneapolis,MN cchow@ Mohamed F.MokbelDepartment of ComputerScience and EngineeringUniversity of MinnesotaMinneapolis,MNmokbel@Xuan LiuIBM Thomas J.WatsonResearch CenterHawthorne,NYxuanliu@ABSTRACTThis paper tackles a major privacy threat in current location-based services where users have to report their ex-act locations to the database server in order to obtain their desired services.For example,a mobile user asking about her nearest restaurant has to report her exact location.With untrusted service providers,reporting private location in-formation may lead to several privacy threats.In this pa-per,we present a peer-to-peer(P2P)spatial cloaking algo-rithm in which mobile and stationary users can entertain location-based services without revealing their exact loca-tion information.The main idea is that before requesting any location-based service,the mobile user will form a group from her peers via single-hop communication and/or multi-hop routing.Then,the spatial cloaked area is computed as the region that covers the entire group of peers.Two modes of operations are supported within the proposed P2P spa-tial cloaking algorithm,namely,the on-demand mode and the proactive mode.Experimental results show that the P2P spatial cloaking algorithm operated in the on-demand mode has lower communication cost and better quality of services than the proactive mode,but the on-demand incurs longer response time.Categories and Subject Descriptors:H.2.8[Database Applications]:Spatial databases and GISGeneral Terms:Algorithms and Experimentation. Keywords:Mobile computing,location-based services,lo-cation privacy and spatial cloaking.1.INTRODUCTIONThe emergence of state-of-the-art location-detection de-vices,e.g.,cellular phones,global positioning system(GPS) devices,and radio-frequency identification(RFID)chips re-sults in a location-dependent information access paradigm,∗This work is supported in part by the Grants-in-Aid of Re-search,Artistry,and Scholarship,University of Minnesota. Permission to make digital or hard copies of all or part of this work for personal or classroom use is granted without fee provided that copies are not made or distributed for profit or commercial advantage and that copies bear this notice and the full citation on thefirst page.To copy otherwise,to republish,to post on servers or to redistribute to lists,requires prior specific permission and/or a fee.ACM-GIS’06,November10-11,2006,Arlington,Virginia,USA. Copyright2006ACM1-59593-529-0/06/0011...$5.00.known as location-based services(LBS)[30].In LBS,mobile users have the ability to issue location-based queries to the location-based database server.Examples of such queries include“where is my nearest gas station”,“what are the restaurants within one mile of my location”,and“what is the traffic condition within ten minutes of my route”.To get the precise answer of these queries,the user has to pro-vide her exact location information to the database server. With untrustworthy servers,adversaries may access sensi-tive information about specific individuals based on their location information and issued queries.For example,an adversary may check a user’s habit and interest by knowing the places she visits and the time of each visit,or someone can track the locations of his ex-friends.In fact,in many cases,GPS devices have been used in stalking personal lo-cations[12,39].To tackle this major privacy concern,three centralized privacy-preserving frameworks are proposed for LBS[13,14,31],in which a trusted third party is used as a middleware to blur user locations into spatial regions to achieve k-anonymity,i.e.,a user is indistinguishable among other k−1users.The centralized privacy-preserving frame-work possesses the following shortcomings:1)The central-ized trusted third party could be the system bottleneck or single point of failure.2)Since the centralized third party has the complete knowledge of the location information and queries of all users,it may pose a serious privacy threat when the third party is attacked by adversaries.In this paper,we propose a peer-to-peer(P2P)spatial cloaking algorithm.Mobile users adopting the P2P spatial cloaking algorithm can protect their privacy without seeking help from any centralized third party.Other than the short-comings of the centralized approach,our work is also moti-vated by the following facts:1)The computation power and storage capacity of most mobile devices have been improv-ing at a fast pace.2)P2P communication technologies,such as IEEE802.11and Bluetooth,have been widely deployed.3)Many new applications based on P2P information shar-ing have rapidly taken shape,e.g.,cooperative information access[9,32]and P2P spatio-temporal query processing[20, 24].Figure1gives an illustrative example of P2P spatial cloak-ing.The mobile user A wants tofind her nearest gas station while beingfive anonymous,i.e.,the user is indistinguish-able amongfive users.Thus,the mobile user A has to look around andfind other four peers to collaborate as a group. In this example,the four peers are B,C,D,and E.Then, the mobile user A cloaks her exact location into a spatialA B CDEBase Stationregion that covers the entire group of mobile users A ,B ,C ,D ,and E .The mobile user A randomly selects one of the mobile users within the group as an agent .In the ex-ample given in Figure 1,the mobile user D is selected as an agent.Then,the mobile user A sends her query (i.e.,what is the nearest gas station)along with her cloaked spa-tial region to the agent.The agent forwards the query to the location-based database server through a base station.Since the location-based database server processes the query based on the cloaked spatial region,it can only give a list of candidate answers that includes the actual answers and some false positives.After the agent receives the candidate answers,it forwards the candidate answers to the mobile user A .Finally,the mobile user A gets the actual answer by filtering out all the false positives.The proposed P2P spatial cloaking algorithm can operate in two modes:on-demand and proactive .In the on-demand mode,mobile clients execute the cloaking algorithm when they need to access information from the location-based database server.On the other side,in the proactive mode,mobile clients periodically look around to find the desired number of peers.Thus,they can cloak their exact locations into spatial regions whenever they want to retrieve informa-tion from the location-based database server.In general,the contributions of this paper can be summarized as follows:1.We introduce a distributed system architecture for pro-viding anonymous location-based services (LBS)for mobile users.2.We propose the first P2P spatial cloaking algorithm for mobile users to entertain high quality location-based services without compromising their privacy.3.We provide experimental evidence that our proposed algorithm is efficient in terms of the response time,is scalable to large numbers of mobile clients,and is effective as it provides high-quality services for mobile clients without the need of exact location information.The rest of this paper is organized as follows.Section 2highlights the related work.The system model of the P2P spatial cloaking algorithm is presented in Section 3.The P2P spatial cloaking algorithm is described in Section 4.Section 5discusses the integration of the P2P spatial cloak-ing algorithm with privacy-aware location-based database servers.Section 6depicts the experimental evaluation of the P2P spatial cloaking algorithm.Finally,Section 7con-cludes this paper.2.RELATED WORKThe k -anonymity model [37,38]has been widely used in maintaining privacy in databases [5,26,27,28].The main idea is to have each tuple in the table as k -anonymous,i.e.,indistinguishable among other k −1tuples.Although we aim for the similar k -anonymity model for the P2P spatial cloaking algorithm,none of these techniques can be applied to protect user privacy for LBS,mainly for the following four reasons:1)These techniques preserve the privacy of the stored data.In our model,we aim not to store the data at all.Instead,we store perturbed versions of the data.Thus,data privacy is managed before storing the data.2)These approaches protect the data not the queries.In anonymous LBS,we aim to protect the user who issues the query to the location-based database server.For example,a mobile user who wants to ask about her nearest gas station needs to pro-tect her location while the location information of the gas station is not protected.3)These approaches guarantee the k -anonymity for a snapshot of the database.In LBS,the user location is continuously changing.Such dynamic be-havior calls for continuous maintenance of the k -anonymity model.(4)These approaches assume a unified k -anonymity requirement for all the stored records.In our P2P spatial cloaking algorithm,k -anonymity is a user-specified privacy requirement which may have a different value for each user.Motivated by the privacy threats of location-detection de-vices [1,4,6,40],several research efforts are dedicated to protect the locations of mobile users (e.g.,false dummies [23],landmark objects [18],and location perturbation [10,13,14]).The most closed approaches to ours are two centralized spatial cloaking algorithms,namely,the spatio-temporal cloaking [14]and the CliqueCloak algorithm [13],and one decentralized privacy-preserving algorithm [23].The spatio-temporal cloaking algorithm [14]assumes that all users have the same k -anonymity requirements.Furthermore,it lacks the scalability because it deals with each single request of each user individually.The CliqueCloak algorithm [13]as-sumes a different k -anonymity requirement for each user.However,since it has large computation overhead,it is lim-ited to a small k -anonymity requirement,i.e.,k is from 5to 10.A decentralized privacy-preserving algorithm is proposed for LBS [23].The main idea is that the mobile client sends a set of false locations,called dummies ,along with its true location to the location-based database server.However,the disadvantages of using dummies are threefold.First,the user has to generate realistic dummies to pre-vent the adversary from guessing its true location.Second,the location-based database server wastes a lot of resources to process the dummies.Finally,the adversary may esti-mate the user location by using cellular positioning tech-niques [34],e.g.,the time-of-arrival (TOA),the time differ-ence of arrival (TDOA)and the direction of arrival (DOA).Although several existing distributed group formation al-gorithms can be used to find peers in a mobile environment,they are not designed for privacy preserving in LBS.Some algorithms are limited to only finding the neighboring peers,e.g.,lowest-ID [11],largest-connectivity (degree)[33]and mobility-based clustering algorithms [2,25].When a mo-bile user with a strict privacy requirement,i.e.,the value of k −1is larger than the number of neighboring peers,it has to enlist other peers for help via multi-hop routing.Other algorithms do not have this limitation,but they are designed for grouping stable mobile clients together to facil-Location-based Database ServerDatabase ServerDatabase ServerFigure 2:The system architectureitate efficient data replica allocation,e.g.,dynamic connec-tivity based group algorithm [16]and mobility-based clus-tering algorithm,called DRAM [19].Our work is different from these approaches in that we propose a P2P spatial cloaking algorithm that is dedicated for mobile users to dis-cover other k −1peers via single-hop communication and/or via multi-hop routing,in order to preserve user privacy in LBS.3.SYSTEM MODELFigure 2depicts the system architecture for the pro-posed P2P spatial cloaking algorithm which contains two main components:mobile clients and location-based data-base server .Each mobile client has its own privacy profile that specifies its desired level of privacy.A privacy profile includes two parameters,k and A min ,k indicates that the user wants to be k -anonymous,i.e.,indistinguishable among k users,while A min specifies the minimum resolution of the cloaked spatial region.The larger the value of k and A min ,the more strict privacy requirements a user needs.Mobile users have the ability to change their privacy profile at any time.Our employed privacy profile matches the privacy re-quirements of mobiles users as depicted by several social science studies (e.g.,see [4,15,17,22,29]).In this architecture,each mobile user is equipped with two wireless network interface cards;one of them is dedicated to communicate with the location-based database server through the base station,while the other one is devoted to the communication with other peers.A similar multi-interface technique has been used to implement IP multi-homing for stream control transmission protocol (SCTP),in which a machine is installed with multiple network in-terface cards,and each assigned a different IP address [36].Similarly,in mobile P2P cooperation environment,mobile users have a network connection to access information from the server,e.g.,through a wireless modem or a base station,and the mobile users also have the ability to communicate with other peers via a wireless LAN,e.g.,IEEE 802.11or Bluetooth [9,24,32].Furthermore,each mobile client is equipped with a positioning device, e.g.,GPS or sensor-based local positioning systems,to determine its current lo-cation information.4.P2P SPATIAL CLOAKINGIn this section,we present the data structure and the P2P spatial cloaking algorithm.Then,we describe two operation modes of the algorithm:on-demand and proactive .4.1Data StructureThe entire system area is divided into grid.The mobile client communicates with each other to discover other k −1peers,in order to achieve the k -anonymity requirement.TheAlgorithm 1P2P Spatial Cloaking:Request Originator m 1:Function P2PCloaking-Originator (h ,k )2://Phase 1:Peer searching phase 3:The hop distance h is set to h4:The set of discovered peers T is set to {∅},and the number ofdiscovered peers k =|T |=05:while k <k −1do6:Broadcast a FORM GROUP request with the parameter h (Al-gorithm 2gives the response of each peer p that receives this request)7:T is the set of peers that respond back to m by executingAlgorithm 28:k =|T |;9:if k <k −1then 10:if T =T then 11:Suspend the request 12:end if 13:h ←h +1;14:T ←T ;15:end if 16:end while17://Phase 2:Location adjustment phase 18:for all T i ∈T do19:|mT i .p |←the greatest possible distance between m and T i .pby considering the timestamp of T i .p ’s reply and maximum speed20:end for21://Phase 3:Spatial cloaking phase22:Form a group with k −1peers having the smallest |mp |23:h ←the largest hop distance h p of the selected k −1peers 24:Determine a grid area A that covers the entire group 25:if A <A min then26:Extend the area of A till it covers A min 27:end if28:Randomly select a mobile client of the group as an agent 29:Forward the query and A to the agentmobile client can thus blur its exact location into a cloaked spatial region that is the minimum grid area covering the k −1peers and itself,and satisfies A min as well.The grid area is represented by the ID of the left-bottom and right-top cells,i.e.,(l,b )and (r,t ).In addition,each mobile client maintains a parameter h that is the required hop distance of the last peer searching.The initial value of h is equal to one.4.2AlgorithmFigure 3gives a running example for the P2P spatial cloaking algorithm.There are 15mobile clients,m 1to m 15,represented as solid circles.m 8is the request originator,other black circles represent the mobile clients received the request from m 8.The dotted circles represent the commu-nication range of the mobile client,and the arrow represents the movement direction.Algorithms 1and 2give the pseudo code for the request originator (denoted as m )and the re-quest receivers (denoted as p ),respectively.In general,the algorithm consists of the following three phases:Phase 1:Peer searching phase .The request origina-tor m wants to retrieve information from the location-based database server.m first sets h to h ,a set of discovered peers T to {∅}and the number of discovered peers k to zero,i.e.,|T |.(Lines 3to 4in Algorithm 1).Then,m broadcasts a FORM GROUP request along with a message sequence ID and the hop distance h to its neighboring peers (Line 6in Algorithm 1).m listens to the network and waits for the reply from its neighboring peers.Algorithm 2describes how a peer p responds to the FORM GROUP request along with a hop distance h and aFigure3:P2P spatial cloaking algorithm.Algorithm2P2P Spatial Cloaking:Request Receiver p1:Function P2PCloaking-Receiver(h)2://Let r be the request forwarder3:if the request is duplicate then4:Reply r with an ACK message5:return;6:end if7:h p←1;8:if h=1then9:Send the tuple T=<p,(x p,y p),v maxp ,t p,h p>to r10:else11:h←h−1;12:Broadcast a FORM GROUP request with the parameter h 13:T p is the set of peers that respond back to p14:for all T i∈T p do15:T i.h p←T i.h p+1;16:end for17:T p←T p∪{<p,(x p,y p),v maxp ,t p,h p>};18:Send T p back to r19:end ifmessage sequence ID from another peer(denoted as r)that is either the request originator or the forwarder of the re-quest.First,p checks if it is a duplicate request based on the message sequence ID.If it is a duplicate request,it sim-ply replies r with an ACK message without processing the request.Otherwise,p processes the request based on the value of h:Case1:h= 1.p turns in a tuple that contains its ID,current location,maximum movement speed,a timestamp and a hop distance(it is set to one),i.e.,< p,(x p,y p),v max p,t p,h p>,to r(Line9in Algorithm2). Case2:h> 1.p decrements h and broadcasts the FORM GROUP request with the updated h and the origi-nal message sequence ID to its neighboring peers.p keeps listening to the network,until it collects the replies from all its neighboring peers.After that,p increments the h p of each collected tuple,and then it appends its own tuple to the collected tuples T p.Finally,it sends T p back to r (Lines11to18in Algorithm2).After m collects the tuples T from its neighboring peers, if m cannotfind other k−1peers with a hop distance of h,it increments h and re-broadcasts the FORM GROUP request along with a new message sequence ID and h.m repeatedly increments h till itfinds other k−1peers(Lines6to14in Algorithm1).However,if mfinds the same set of peers in two consecutive broadcasts,i.e.,with hop distances h and h+1,there are not enough connected peers for m.Thus, m has to relax its privacy profile,i.e.,use a smaller value of k,or to be suspended for a period of time(Line11in Algorithm1).Figures3(a)and3(b)depict single-hop and multi-hop peer searching in our running example,respectively.In Fig-ure3(a),the request originator,m8,(e.g.,k=5)canfind k−1peers via single-hop communication,so m8sets h=1. Since h=1,its neighboring peers,m5,m6,m7,m9,m10, and m11,will not further broadcast the FORM GROUP re-quest.On the other hand,in Figure3(b),m8does not connect to k−1peers directly,so it has to set h>1.Thus, its neighboring peers,m7,m10,and m11,will broadcast the FORM GROUP request along with a decremented hop dis-tance,i.e.,h=h−1,and the original message sequence ID to their neighboring peers.Phase2:Location adjustment phase.Since the peer keeps moving,we have to capture the movement between the time when the peer sends its tuple and the current time. For each received tuple from a peer p,the request originator, m,determines the greatest possible distance between them by an equation,|mp |=|mp|+(t c−t p)×v max p,where |mp|is the Euclidean distance between m and p at time t p,i.e.,|mp|=(x m−x p)2+(y m−y p)2,t c is the currenttime,t p is the timestamp of the tuple and v maxpis the maximum speed of p(Lines18to20in Algorithm1).In this paper,a conservative approach is used to determine the distance,because we assume that the peer will move with the maximum speed in any direction.If p gives its movement direction,m has the ability to determine a more precise distance between them.Figure3(c)illustrates that,for each discovered peer,the circle represents the largest region where the peer can lo-cate at time t c.The greatest possible distance between the request originator m8and its discovered peer,m5,m6,m7, m9,m10,or m11is represented by a dotted line.For exam-ple,the distance of the line m8m 11is the greatest possible distance between m8and m11at time t c,i.e.,|m8m 11|. Phase3:Spatial cloaking phase.In this phase,the request originator,m,forms a virtual group with the k−1 nearest peers,based on the greatest possible distance be-tween them(Line22in Algorithm1).To adapt to the dynamic network topology and k-anonymity requirement, m sets h to the largest value of h p of the selected k−1 peers(Line15in Algorithm1).Then,m determines the minimum grid area A covering the entire group(Line24in Algorithm1).If the area of A is less than A min,m extends A,until it satisfies A min(Lines25to27in Algorithm1). Figure3(c)gives the k−1nearest peers,m6,m7,m10,and m11to the request originator,m8.For example,the privacy profile of m8is(k=5,A min=20cells),and the required cloaked spatial region of m8is represented by a bold rectan-gle,as depicted in Figure3(d).To issue the query to the location-based database server anonymously,m randomly selects a mobile client in the group as an agent(Line28in Algorithm1).Then,m sendsthe query along with the cloaked spatial region,i.e.,A,to the agent(Line29in Algorithm1).The agent forwards thequery to the location-based database server.After the serverprocesses the query with respect to the cloaked spatial re-gion,it sends a list of candidate answers back to the agent.The agent forwards the candidate answer to m,and then mfilters out the false positives from the candidate answers. 4.3Modes of OperationsThe P2P spatial cloaking algorithm can operate in twomodes,on-demand and proactive.The on-demand mode:The mobile client only executesthe algorithm when it needs to retrieve information from the location-based database server.The algorithm operatedin the on-demand mode generally incurs less communica-tion overhead than the proactive mode,because the mobileclient only executes the algorithm when necessary.However,it suffers from a longer response time than the algorithm op-erated in the proactive mode.The proactive mode:The mobile client adopting theproactive mode periodically executes the algorithm in back-ground.The mobile client can cloak its location into a spa-tial region immediately,once it wants to communicate withthe location-based database server.The proactive mode pro-vides a better response time than the on-demand mode,but it generally incurs higher communication overhead and giveslower quality of service than the on-demand mode.5.ANONYMOUS LOCATION-BASEDSERVICESHaving the spatial cloaked region as an output form Algo-rithm1,the mobile user m sends her request to the location-based server through an agent p that is randomly selected.Existing location-based database servers can support onlyexact point locations rather than cloaked regions.In or-der to be able to work with a spatial region,location-basedservers need to be equipped with a privacy-aware queryprocessor(e.g.,see[29,31]).The main idea of the privacy-aware query processor is to return a list of candidate answerrather than the exact query answer.Then,the mobile user m willfilter the candidate list to eliminate its false positives andfind its exact answer.The tighter the spatial cloaked re-gion,the lower is the size of the candidate answer,and hencethe better is the performance of the privacy-aware query processor.However,tight cloaked regions may represent re-laxed privacy constrained.Thus,a trade-offbetween the user privacy and the quality of service can be achieved[31]. Figure4(a)depicts such scenario by showing the data stored at the server side.There are32target objects,i.e., gas stations,T1to T32represented as black circles,the shaded area represents the spatial cloaked area of the mo-bile client who issued the query.For clarification,the actual mobile client location is plotted in Figure4(a)as a black square inside the cloaked area.However,such information is neither stored at the server side nor revealed to the server. The privacy-aware query processor determines a range that includes all target objects that are possibly contributing to the answer given that the actual location of the mobile client could be anywhere within the shaded area.The range is rep-resented as a bold rectangle,as depicted in Figure4(b).The server sends a list of candidate answers,i.e.,T8,T12,T13, T16,T17,T21,and T22,back to the agent.The agent next for-(a)Server Side(b)Client SideFigure4:Anonymous location-based services wards the candidate answers to the requesting mobile client either through single-hop communication or through multi-hop routing.Finally,the mobile client can get the actualanswer,i.e.,T13,byfiltering out the false positives from thecandidate answers.The algorithmic details of the privacy-aware query proces-sor is beyond the scope of this paper.Interested readers are referred to[31]for more details.6.EXPERIMENTAL RESULTSIn this section,we evaluate and compare the scalabilityand efficiency of the P2P spatial cloaking algorithm in boththe on-demand and proactive modes with respect to the av-erage response time per query,the average number of mes-sages per query,and the size of the returned candidate an-swers from the location-based database server.The queryresponse time in the on-demand mode is defined as the timeelapsed between a mobile client starting to search k−1peersand receiving the candidate answers from the agent.On theother hand,the query response time in the proactive mode is defined as the time elapsed between a mobile client startingto forward its query along with the cloaked spatial regionto the agent and receiving the candidate answers from theagent.The simulation model is implemented in C++usingCSIM[35].In all the experiments in this section,we consider an in-dividual random walk model that is based on“random way-point”model[7,8].At the beginning,the mobile clientsare randomly distributed in a spatial space of1,000×1,000square meters,in which a uniform grid structure of100×100cells is constructed.Each mobile client randomly chooses itsown destination in the space with a randomly determined speed s from a uniform distribution U(v min,v max).When the mobile client reaches the destination,it comes to a stand-still for one second to determine its next destination.Afterthat,the mobile client moves towards its new destinationwith another speed.All the mobile clients repeat this move-ment behavior during the simulation.The time interval be-tween two consecutive queries generated by a mobile client follows an exponential distribution with a mean of ten sec-onds.All the experiments consider one half-duplex wirelesschannel for a mobile client to communicate with its peers with a total bandwidth of2Mbps and a transmission range of250meters.When a mobile client wants to communicate with other peers or the location-based database server,it has to wait if the requested channel is busy.In the simulated mobile environment,there is a centralized location-based database server,and one wireless communication channel between the location-based database server and the mobile。
RTransferEntropy包:时间序列之间的信息流量测量说明书

Package‘RTransferEntropy’February1,2023Type PackageTitle Measuring Information Flow Between Time Series with Shannon andRenyi Transfer EntropyVersion0.2.21Description Measuring informationflow between time series with Shannon and Rényi transfer en-tropy.See also Dimpfland Peter(2013)<doi:10.1515/snde-2012-0044>and Dimpfland Pe-ter(2014)<doi:10.1016/j.intfin.2014.03.004>for theory and applications tofinancial time se-ries.Additional references can be found in the theory part of the vignette.License GPL-3URL https:///BZPaper/RTransferEntropyBugReports https:///BZPaper/RTransferEntropy/issuesEncoding UTF-8Depends R(>=3.1.2)Imports future(>=1.19.0),future.apply,RcppLazyData trueRoxygenNote7.2.0LinkingTo RcppSuggests data.table,ggplot2,gridExtra,knitr,quantmod,rmarkdown,testthat,vars,xts,zooVignetteBuilder knitrNeedsCompilation yesAuthor David Zimmermann[aut,cre],Simon Behrendt[aut],Thomas Dimpfl[aut],Franziska Peter[aut]Maintainer David Zimmermann<******************************>Repository CRANDate/Publication2023-02-0117:30:05UTC1R topics documented:calc_ete (2)calc_te (4)coef.transfer_entropy (6)is.transfer_entropy (7)print.transfer_entropy (7)set_quiet (9)stocks (10)summary.transfer_entropy (10)transfer_entropy (11)Index14 calc_ete Calculates the Effective Transfer Entropy for two time seriesDescriptionCalculates the Effective Transfer Entropy for two time seriesUsagecalc_ete(x,y,lx=1,ly=1,q=0.1,entropy="Shannon",shuffles=100,type="quantiles",quantiles=c(5,95),bins=NULL,limits=NULL,burn=50,seed=NULL,na.rm=TRUE)Argumentsx a vector of numeric values,ordered by time.Also allowed are xts,zoo,or ts objects.y a vector of numeric values,ordered by time.Also allowed are xts,zoo,or ts objects.lx Markov order of x,i.e.the number of lagged values affecting the current value of x.Default is lx=1.ly Markov order of y,i.e.the number of lagged values affecting the current value of y.Default is ly=1.q a weighting parameter used to estimate Renyi transfer entropy,parameter is be-tween0and1.For q=1,Renyi transfer entropy converges to Shannon transferentropy.Default is q=0.1.entropy specifies the transfer entropy measure that is estimated,either’Shannon’or ’Renyi’.Thefirst character can be used to specify the type of transfer entropyas well.Default is entropy= Shannon .shuffles the number of shuffles used to calculate the effective transfer entropy.Default is shuffles=100.type specifies the type of discretization applied to the observed time series:’quantiles’,’bins’or’limits’.Default is type= quantiles .quantiles specifies the quantiles of the empirical distribution of the respective time series used for discretization.Default is quantiles=c(5,95).bins specifies the number of bins with equal width used for discretization.Default is bins=NULL.limits specifies the limits on values used for discretization.Default is limits=NULL.burn the number of observations that are dropped from the beginning of the boot-strapped Markov chain.Default is burn=50.seed a seed that seeds the PRNG(will internally just call set.seed),default is seed= NULL.na.rm if missing values should be removed(will remove the values at the same point in the other series as well).Default is TRUE.Valuea single numerical value for the effective transfer entropySee Alsocalc_te and transfer_entropyExamples#construct two time-seriesset.seed(1234567890)n<-1000x<-rep(0,n+1)y<-rep(0,n+1)for(i in seq(n)){x[i+1]<-0.2*x[i]+rnorm(1,0,2)y[i+1]<-x[i]+rnorm(1,0,2)}x<-x[-1]y<-y[-1]#calculate the X->Y transfer entropy valuecalc_ete(x,y)#calculate the Y->X transfer entropy valuecalc_ete(y,x)#Compare the results#even with the same seed,transfer_entropy might return slightly different#results from calc_etecalc_ete(x,y,seed=123)calc_ete(y,x,seed=123)transfer_entropy(x,y,nboot=0,seed=123)calc_te Calculates the Transfer Entropy for two time seriesDescriptionCalculates the Transfer Entropy for two time seriesUsagecalc_te(x,y,lx=1,ly=1,q=0.1,entropy="Shannon",shuffles=100,type="quantiles",quantiles=c(5,95),bins=NULL,limits=NULL,burn=50,seed=NULL,na.rm=TRUE)Argumentsx a vector of numeric values,ordered by time.Also allowed are xts,zoo,or ts objects.y a vector of numeric values,ordered by time.Also allowed are xts,zoo,or ts objects.lx Markov order of x,i.e.the number of lagged values affecting the current value of x.Default is lx=1.ly Markov order of y,i.e.the number of lagged values affecting the current value of y.Default is ly=1.q a weighting parameter used to estimate Renyi transfer entropy,parameter is be-tween0and1.For q=1,Renyi transfer entropy converges to Shannon transferentropy.Default is q=0.1.entropy specifies the transfer entropy measure that is estimated,either’Shannon’or ’Renyi’.Thefirst character can be used to specify the type of transfer entropyas well.Default is entropy= Shannon .shuffles the number of shuffles used to calculate the effective transfer entropy.Default is shuffles=100.type specifies the type of discretization applied to the observed time series:’quantiles’,’bins’or’limits’.Default is type= quantiles .quantiles specifies the quantiles of the empirical distribution of the respective time series used for discretization.Default is quantiles=c(5,95).bins specifies the number of bins with equal width used for discretization.Default is bins=NULL.limits specifies the limits on values used for discretization.Default is limits=NULL.burn the number of observations that are dropped from the beginning of the boot-strapped Markov chain.Default is burn=50.seed a seed that seeds the PRNG(will internally just call set.seed),default is seed= NULL.na.rm if missing values should be removed(will remove the values at the same point in the other series as well).Default is TRUE.Valuea single numerical value for the transfer entropySee Alsocalc_ete and transfer_entropyExamples#construct two time-seriesset.seed(1234567890)n<-1000x<-rep(0,n+1)y<-rep(0,n+1)for(i in seq(n)){x[i+1]<-0.2*x[i]+rnorm(1,0,2)y[i+1]<-x[i]+rnorm(1,0,2)}x<-x[-1]y<-y[-1]#calculate the X->Y transfer entropy valuecalc_te(x,y)#calculate the Y->X transfer entropy valuecalc_te(y,x)#Compare the resultscalc_te(x,y,seed=123)calc_te(y,x,seed=123)transfer_entropy(x,y,nboot=0,seed=123)coef.transfer_entropy Extract the Coefficient Matrix from a transfer_entropyDescriptionExtract the Coefficient Matrix from a transfer_entropyUsage##S3method for class transfer_entropycoef(object,...)Argumentsobject a transfer_entropy...additional arguments,currently not in useValuea Matrix containing the coefficientsExamplesset.seed(1234567890)n<-500x<-rep(0,n+1)y<-rep(0,n+1)for(i in seq(n)){x[i+1]<-0.2*x[i]+rnorm(1,0,2)y[i+1]<-x[i]+rnorm(1,0,2)}x<-x[-1]y<-y[-1]te_result<-transfer_entropy(x,y,nboot=100)coef(te_result)is.transfer_entropy Checks if an object is a transfer_entropyDescriptionChecks if an object is a transfer_entropyUsageis.transfer_entropy(x)Argumentsx an objectValuea boolean value if x is a transfer_entropyExamples#see?transfer_entropyprint.transfer_entropyPrints a transfer-entropy resultDescriptionPrints a transfer-entropy resultUsage##S3method for class transfer_entropyprint(x,digits=4,boot=TRUE,probs=c(0,0.25,0.5,0.75,1),tex=FALSE,ref=NA,file=NA,table=TRUE,...)Argumentsx a transfer_entropydigits the number of digits to display,defaults to4boot if the bootstrapped results should be printed,defaults to TRUEprobs numeric vector of quantiles for the bootstrapstex if the data should be outputted as a TeX-stringref the reference string of the LaTeX table(label)applies only if table=TRUE and tex=TRUE,defaults to FALSEfile afile where the results are printed totable if the table environment should be printed as well(only applies if tex=TRUE), defaults to TRUE...additional arguments,currently not in useValueinvisible the textExamples#construct two time-seriesset.seed(1234567890)n<-500x<-rep(0,n+1)y<-rep(0,n+1)for(i in seq(n)){x[i+1]<-0.2*x[i]+rnorm(1,0,2)y[i+1]<-x[i]+rnorm(1,0,2)}x<-x[-1]y<-y[-1]set_quiet9#Calculate Shannon s Transfer Entropyte_result<-transfer_entropy(x,y,nboot=100)print(te_result)#change the number of digitsprint(te_result,digits=10)#disable boot-printprint(te_result,boot=FALSE)#specify the quantiles of the bootstrapsprint(te_result,probs=c(0,0.1,0.4,0.5,0.6,0.9,1))#get LaTeX output:print(te_result,tex=TRUE)#set the reference label for LaTeX tableprint(te_result,tex=TRUE,ref="tab:te_result")##Not run:#file outputprint(te_result,file="te_result_file.txt")print(te_result,tex=TRUE,file="te_result_file.tex")##End(Not run)set_quiet Set the quiet-parameter for all RTransferEntropy CallsDescriptionSet the quiet-parameter for all RTransferEntropy CallsUsageset_quiet(quiet)Argumentsquiet if FALSE,the functions will give feedback on the progressValuenothingExamples#see?transfer_entropy10summary.transfer_entropy stocks Daily stock data for10stocks from2000-2017DescriptionA dataset containing the daily stock returns for10stocks and the S&P500market returns for thetime-period2000-01-04until2017-12-29UsagestocksFormatA data frame(or data.table if loaded)with46940rows and4variables:date date of the observationticker ticker of the stockret Return of the stocksp500Return of the S&P500stock market indexSourceyahoofinance using getSymbolssummary.transfer_entropyPrints a summary of a transfer-entropy resultDescriptionPrints a summary of a transfer-entropy resultUsage##S3method for class transfer_entropysummary(object,digits=4,probs=c(0,0.25,0.5,0.75,1),...)Argumentsobject a transfer_entropydigits the number of digits to display,defaults to4probs numeric vector of quantiles for the bootstraps...additional arguments,passed to printCoefmatValueinvisible the objectExamples#construct two time-seriesset.seed(1234567890)n<-500x<-rep(0,n+1)y<-rep(0,n+1)for(i in seq(n)){x[i+1]<-0.2*x[i]+rnorm(1,0,2)y[i+1]<-x[i]+rnorm(1,0,2)}x<-x[-1]y<-y[-1]#Calculate Shannon s Transfer Entropyte_result<-transfer_entropy(x,y,nboot=100)summary(te_result)transfer_entropy Function to estimate Shannon and Renyi transfer entropy between twotime series x and y.DescriptionFunction to estimate Shannon and Renyi transfer entropy between two time series x and y. Usagetransfer_entropy(x,y,lx=1,ly=1,q=0.1,entropy="Shannon",shuffles=100,type="quantiles",quantiles=c(5,95),bins=NULL,limits=NULL,nboot=300,burn=50,quiet=NULL,seed=NULL,na.rm=TRUE)Argumentsx a vector of numeric values,ordered by time.Also allowed are xts,zoo,or ts objects.y a vector of numeric values,ordered by time.Also allowed are xts,zoo,or ts objects.lx Markov order of x,i.e.the number of lagged values affecting the current value of x.Default is lx=1.ly Markov order of y,i.e.the number of lagged values affecting the current value of y.Default is ly=1.q a weighting parameter used to estimate Renyi transfer entropy,parameter is be-tween0and1.For q=1,Renyi transfer entropy converges to Shannon transferentropy.Default is q=0.1.entropy specifies the transfer entropy measure that is estimated,either’Shannon’or ’Renyi’.Thefirst character can be used to specify the type of transfer entropyas well.Default is entropy= Shannon .shuffles the number of shuffles used to calculate the effective transfer entropy.Default is shuffles=100.type specifies the type of discretization applied to the observed time series:’quantiles’,’bins’or’limits’.Default is type= quantiles .quantiles specifies the quantiles of the empirical distribution of the respective time series used for discretization.Default is quantiles=c(5,95).bins specifies the number of bins with equal width used for discretization.Default is bins=NULL.limits specifies the limits on values used for discretization.Default is limits=NULL.nboot the number of bootstrap replications for each direction of the estimated transfer entropy.Default is nboot=300.burn the number of observations that are dropped from the beginning of the boot-strapped Markov chain.Default is burn=50.quiet if FALSE(default),the function gives feedback.seed a seed that seeds the PRNG(will internally just call set.seed),default is seed= NULL.na.rm if missing values should be removed(will remove the values at the same point in the other series as well).Default is TRUE.Valuean object of class transfer_entropy,containing the transfer entropy estimates in both directions, the effective transfer entropy estimates in both directions,standard errors and p-values based on bootstrap replications of the Markov chains under the null hypothesis of statistical independence, an indication of statistical significance,and quantiles of the bootstrap samples(if nboot>0).See Alsocoef,print.transfer_entropyExamples#construct two time-seriesset.seed(1234567890)n<-500x<-rep(0,n+1)y<-rep(0,n+1)for(i in seq(n)){x[i+1]<-0.2*x[i]+rnorm(1,0,2)y[i+1]<-x[i]+rnorm(1,0,2)}x<-x[-1]y<-y[-1]#Calculate Shannon s Transfer Entropyte_result<-transfer_entropy(x,y,nboot=100)te_resultsummary(te_result)#Parallel Processing using the future-packagelibrary(future)plan(multisession)te_result2<-transfer_entropy(x,y,nboot=100)te_result2#revert back to sequential executionplan(sequential)te_result2<-transfer_entropy(x,y,nboot=100)te_result2#General set of quietset_quiet(TRUE)a<-transfer_entropy(x,y,nboot=0)set_quiet(FALSE)a<-transfer_entropy(x,y,nboot=0)#close multisession,see also?planplan(sequential)Index∗datasetsstocks,10calc_ete,2,5calc_te,3,4coef,13coef.transfer_entropy,6getSymbols,10is.transfer_entropy,7print.transfer_entropy,7,13 printCoefmat,10set_quiet,9stocks,10summary.transfer_entropy,10transfer_entropy,3,5,11ts,2,4,12xts,2,4,12zoo,2,4,1214。
IPv6
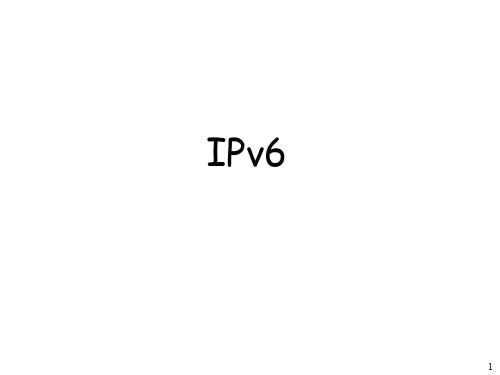
Flow Label
• Real-time applications
– What kind of performance are they sensitive to? – Special handling by intermediate nodes: How? Identify flows
• Source, destination addresses, flow label • QoS for different applications
9
Hop-by-hop Options Header
• Immediately after the main IPv6 header • Router Alert option
– Processing at every node on the delivery path – Install state at every node
• Fragmentation header
– No length field because of a fixed length of eight octets
13
Fragmentation Header (2)
– Fragment offset: the order of fragments within the original – m flag: if the received fragment is the last (0) or not (1); Inform the receiving host if reassembly can start – Identification: Unique value for each large packet for reassembly
Microsoft TechNet Deutschland 技术指南说明书

http://aka.ms/VirtLabs
http://aka.ms/kostenlostesten
IT Camps
Exklusive Workshops zu den Themen Hybrid IT, Datacenter Modernization, Enterprise Mobility,
IT Pro Career Center
Kostenloses Angebot mit dem IT-Professionals Ihre Kenntnisse gezielt erweitern und verfeinern können, um bestens für die Aufgaben und Herausforderungen in Cloud-Umgebungen vorbereitet zu sein.
Die TechNet Library bietet detaillierte technische Informationen zu allen wichtigen Microsoft-Technologien.
http://aka.ms/library
Foren
Holen Sie sich in den TechNet Foren Antworten und Lösungen direkt von der Community oder helfen Sie anderen.
http://aka.ms/newsfeed
http://aka.ms/technet_flash
http://aka.ms/TechNetBlogs
http://aka.ms/technet_de
http://aka.ms/itprofb
网络安全日志数据集 介绍

无法下载的数据集
恶意软件数据集
该数据集由West Virginia University的Yanfang Ye 提供。 包括二个部分,其中第一个用于恶意软件检测,包含50000个实例,其中一半是恶 意软件中提取的特征,另外一半是良性文件中提取的特征,通过该数据集,可以在 数据挖掘和大数据建模技术的基础上,通过Win API调用提取特征集进行恶意软件检 测
基于主机的网络流量统计特征
Honeynet数据集
数据集是由HoneyNet组织收集的黑客攻击数据集,能较好地反映黑客攻击模式, 数据集包括从2000年4月到2011年2月,累计11个月的Snort报警数据,每月大概603000多条Snort报警记录,其网络由8个IP地址通过ISDN连接到ISP
(15)su_attempted. 若出现”su root” 命令则为1,否则为0,连续,0或1。
(16)num_root. root用户访问次数,连续,[0, 7468]。 (17)num_file_creations. 文件创建操作的次数,连续,[0, 100]。
(18)num_shells. 使用shell命令的次数,连续,[0, 5]。 (19)num_access_files. 访问控制文件的次数,连续,[0, 9]。例如对 /etc/passwd 或 .rhosts 文件的访问。
Aug 4 23:32:00 lisa snort[17482]: SCAN-SYN FIN: 202.61.204.176:109 -> 216.80.71.99:109
Aug 4 23:32:00 lisa snort[17482]: SCAN-SYN FIN: 202.61.204.176:109 -> 216.80.71.101:109
- 1、下载文档前请自行甄别文档内容的完整性,平台不提供额外的编辑、内容补充、找答案等附加服务。
- 2、"仅部分预览"的文档,不可在线预览部分如存在完整性等问题,可反馈申请退款(可完整预览的文档不适用该条件!)。
- 3、如文档侵犯您的权益,请联系客服反馈,我们会尽快为您处理(人工客服工作时间:9:00-18:30)。
NetScaler Speeds User Application Delivery
SharePoint
0.22 2.04 1.1 5.22
SAP
With NetScaler
Siebel CRM 1.3 4 6.41
Without NetScaler
Oracle Forms
0 2 4 6
10.1
8 10 12
Transforming Infrastructure into a shared service Cloud-scale performance – unmatched entity scaling Accelerating Web 2.0 and video in the cloud Supporting easy migration and management of app infrastructure Bringing enterprise SLAs to the cloud
Source: Energy Star Report
NetScaler: Simplify Web Application Delivery
Eliminate application downtime Increase performance by 5x Block 100% of web attacks Improve web server utilization by 60%
• SSL processing
• Access Gateway SSL VPN • Application firewall
• Intelligent service health monitoring P2P
App Expert Admin
NetScaler Maximizes Application Availability
Site A
B2C
B2B
Site B
P2P
AppExpert Rate Controls
• Make sure the right users get
appropriate capacity
Partners
• Make sure wrong users get nothing • Ensure no single user/app overwhelms
Team Blogs
Millions of Clients
Team Calendars
WIKIS
• NetScaler support for streaming
• Proactively "broadcasts" new content • Minimal back-end server connections • Service over 2 Million persistent client connections
NetScaler Way
● ● ● ●
75% lower Power Consumption Less Data Center Space Lower Cooling Completely Integrated
~1800 Watts
450 Watts
Savings $Thousands/Every Year
Enterprise Datacenter
Cloud • • • • • 1. 2. 3. 4. 5. Utility cost model Partial control Self-service Fully elastic Unknown security
• • • • •
Fixed cost Full control IT dependent Fixed capacity Known security
Response Time in Seconds
Tested, Certified and Recommended by Leading ISVs
Reduced Load on Servers
CUSTOMERS
SSL
PARTNERS
EMPLOYEES
• SSL Offload • TCP Multiplexing and Buffering • Static and Dynamic Caching • Hardware Compression
Successful Web Application Delivery with NetScaler
B2C
Availability
B2B • World-class L4-L7 load balancing
Performance
Offload
Security
• Caching • Compression
• Cost savings
• Improves server utilization by 5-10x
Minimized Server Needs
Reduce Servers by 60%
Before NetScaler
After NetScaler
Fewer Servers. More Apps.
have become critical to the business
Users Apps
Workforce and IT Trends
USERS
APPS APPS APPS
• • • • •
Globalization Flex Working Branch Expansion Mobility E-Commerce
Mashups More Client Types Microsoft SharePoint 2007
Servers: Still Multiplying
Projected Server and Electricity Use
Servers Electricity Use
18.0
16.0
120
100 14.0 Servers Installed (millions) 12.0 10.0 60 8.0 6.0 4.0 20 2.0 0.0 2000 2001 2002 2003 2004 2005 Year 2006 2007 2008 2009 2010 0 40 80 Annual Electricity Use (billions/kWh)
a shared infrastructure
Lines of Business
• Built into core NetScaler policy engine
Customers
• Usable within multiple NetScaler
modules
Spiders, botnets, scrapers, etc.
Customer
Server Offload Attained 50% 50% 66% 95% 87%
Other Benefits
• Improved response time by 110% • 40% savings on mgmt. costs • Capacity to support 100X traffic spikes • Significant decreases in application latency and mgmt. costs • 10X improvement in application performance • 60% reduction in application latency • Estimated $390K savings in capital investment
Cost Savings of NetScaler vs. Discrete Point Products
• • • • • • • • Load Balancer/Content Switch (1 Gbps): Web Application Firewall: SSL VPN (100 concurrent users): Global Traffic Management: Caching Link Load Balancer Centralized Management Performance Monitoring License: $10K to $15K $24K to $45K $20K to $30K $28K to $40K $10K to $20K $16K $17K $10K
Next Generation Web Apps: Rich, Complex, Demanding
Content Sharing More Protocols
More Blogs Team Connections
More Wikis Chatty
More Calendar Team Applications
Path Traversal
Web App Users Internet
• • • • • •
Green Datacenters Security/Compliance Business Continuity Web and Enterprise 2.0 SaaS, XML, SOA Cloud Computing
Datacenters Are Evolving
Cloud Agility and Scale
Total Per Single Unit
NetScaler MPX 7500 Platinum Edition
~$160K
$45,000 70% Less
Going Green with NetScaler
One Way
Point Products
Load Balancer/L7 Switch Caching Appliance Application Firewall SSL VPN Global Server Load Balancing Performance Monitoring