DeepDiscovery_3.0_Performance Test Report_2012_1H
discovery studio libdock打分 -回复
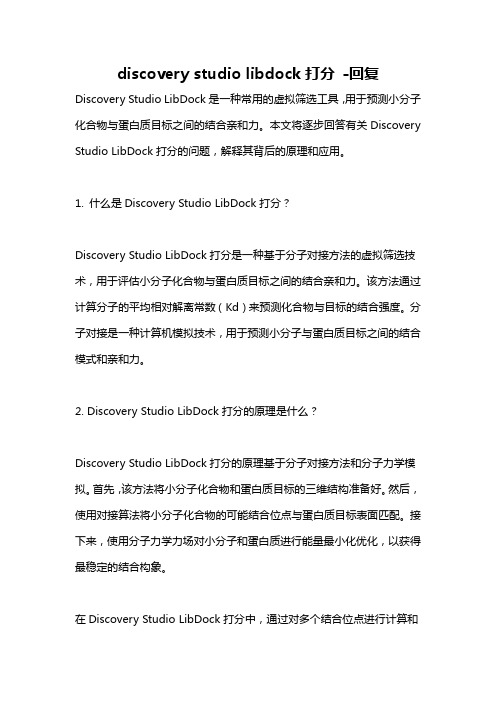
discovery studio libdock打分-回复Discovery Studio LibDock是一种常用的虚拟筛选工具,用于预测小分子化合物与蛋白质目标之间的结合亲和力。
本文将逐步回答有关Discovery Studio LibDock打分的问题,解释其背后的原理和应用。
1. 什么是Discovery Studio LibDock打分?Discovery Studio LibDock打分是一种基于分子对接方法的虚拟筛选技术,用于评估小分子化合物与蛋白质目标之间的结合亲和力。
该方法通过计算分子的平均相对解离常数(Kd)来预测化合物与目标的结合强度。
分子对接是一种计算机模拟技术,用于预测小分子与蛋白质目标之间的结合模式和亲和力。
2. Discovery Studio LibDock打分的原理是什么?Discovery Studio LibDock打分的原理基于分子对接方法和分子力学模拟。
首先,该方法将小分子化合物和蛋白质目标的三维结构准备好。
然后,使用对接算法将小分子化合物的可能结合位点与蛋白质目标表面匹配。
接下来,使用分子力学力场对小分子和蛋白质进行能量最小化优化,以获得最稳定的结合构象。
在Discovery Studio LibDock打分中,通过对多个结合位点进行计算和评分,可以获得多个分子构象的平均Kd值。
较低的Kd值表示更强的结合亲和力。
3. Discovery Studio LibDock打分的具体步骤是什么?Discovery Studio LibDock打分的具体步骤如下:第一步:准备小分子和蛋白质的三维结构。
对于小分子,可以使用软件如ChemDraw进行建模并生成三维结构。
对于蛋白质,可以使用蛋白质晶体结构或生物信息学工具进行三维结构的获取。
第二步:确定小分子和蛋白质的结合位点。
可以使用蛋白质结合位点预测工具如SiteMap来确定可能的结合位点。
第三步:运行Discovery Studio LibDock。
深度学习中的超参数调优技巧
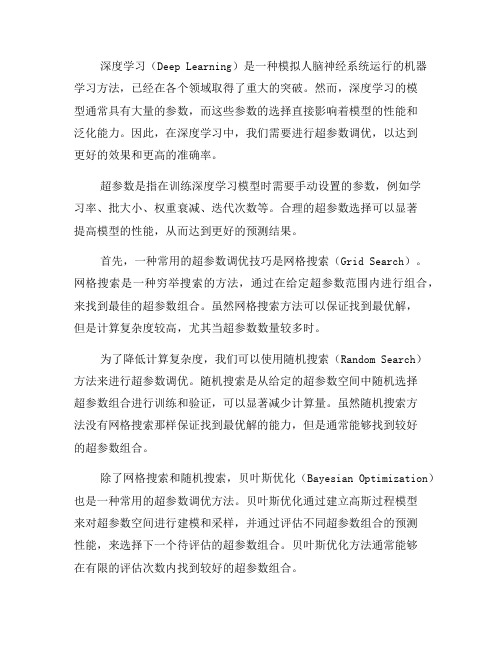
深度学习(Deep Learning)是一种模拟人脑神经系统运行的机器学习方法,已经在各个领域取得了重大的突破。
然而,深度学习的模型通常具有大量的参数,而这些参数的选择直接影响着模型的性能和泛化能力。
因此,在深度学习中,我们需要进行超参数调优,以达到更好的效果和更高的准确率。
超参数是指在训练深度学习模型时需要手动设置的参数,例如学习率、批大小、权重衰减、迭代次数等。
合理的超参数选择可以显著提高模型的性能,从而达到更好的预测结果。
首先,一种常用的超参数调优技巧是网格搜索(Grid Search)。
网格搜索是一种穷举搜索的方法,通过在给定超参数范围内进行组合,来找到最佳的超参数组合。
虽然网格搜索方法可以保证找到最优解,但是计算复杂度较高,尤其当超参数数量较多时。
为了降低计算复杂度,我们可以使用随机搜索(Random Search)方法来进行超参数调优。
随机搜索是从给定的超参数空间中随机选择超参数组合进行训练和验证,可以显著减少计算量。
虽然随机搜索方法没有网格搜索那样保证找到最优解的能力,但是通常能够找到较好的超参数组合。
除了网格搜索和随机搜索,贝叶斯优化(Bayesian Optimization)也是一种常用的超参数调优方法。
贝叶斯优化通过建立高斯过程模型来对超参数空间进行建模和采样,并通过评估不同超参数组合的预测性能,来选择下一个待评估的超参数组合。
贝叶斯优化方法通常能够在有限的评估次数内找到较好的超参数组合。
除了上述提及的超参数调优技巧,还有一些其他的方法可以进一步优化深度学习模型的性能。
例如,使用自适应学习率算法,如Adagrad、Adam等,这些算法可以根据模型表现来自动调整学习率。
此外,还可以引入正则化方法来缓解过拟合问题,例如L1正则化和L2正则化等。
此外,还可以通过集成学习(Ensemble Learning)来提高深度学习模型的性能。
集成学习是通过训练多个独立的模型并将它们的预测结果进行组合,来提高模型的准确性和泛化能力。
低频活动漂浮潜水船声探测系统(LFATS)说明书

LOW-FREQUENCY ACTIVE TOWED SONAR (LFATS)LFATS is a full-feature, long-range,low-frequency variable depth sonarDeveloped for active sonar operation against modern dieselelectric submarines, LFATS has demonstrated consistent detection performance in shallow and deep water. LFATS also provides a passive mode and includes a full set of passive tools and features.COMPACT SIZELFATS is a small, lightweight, air-transportable, ruggedized system designed specifically for easy installation on small vessels. CONFIGURABLELFATS can operate in a stand-alone configuration or be easily integrated into the ship’s combat system.TACTICAL BISTATIC AND MULTISTATIC CAPABILITYA robust infrastructure permits interoperability with the HELRAS helicopter dipping sonar and all key sonobuoys.HIGHLY MANEUVERABLEOwn-ship noise reduction processing algorithms, coupled with compact twin line receivers, enable short-scope towing for efficient maneuvering, fast deployment and unencumbered operation in shallow water.COMPACT WINCH AND HANDLING SYSTEMAn ultrastable structure assures safe, reliable operation in heavy seas and permits manual or console-controlled deployment, retrieval and depth-keeping. FULL 360° COVERAGEA dual parallel array configuration and advanced signal processing achieve instantaneous, unambiguous left/right target discrimination.SPACE-SAVING TRANSMITTERTOW-BODY CONFIGURATIONInnovative technology achievesomnidirectional, large aperture acousticperformance in a compact, sleek tow-body assembly.REVERBERATION SUPRESSIONThe unique transmitter design enablesforward, aft, port and starboarddirectional transmission. This capabilitydiverts energy concentration away fromshorelines and landmasses, minimizingreverb and optimizing target detection.SONAR PERFORMANCE PREDICTIONA key ingredient to mission planning,LFATS computes and displays systemdetection capability based on modeled ormeasured environmental data.Key Features>Wide-area search>Target detection, localization andclassification>T racking and attack>Embedded trainingSonar Processing>Active processing: State-of-the-art signal processing offers acomprehensive range of single- andmulti-pulse, FM and CW processingfor detection and tracking. Targetdetection, localization andclassification>P assive processing: LFATS featuresfull 100-to-2,000 Hz continuouswideband coverage. Broadband,DEMON and narrowband analyzers,torpedo alert and extendedtracking functions constitute asuite of passive tools to track andanalyze targets.>Playback mode: Playback isseamlessly integrated intopassive and active operation,enabling postanalysis of pre-recorded mission data and is a keycomponent to operator training.>Built-in test: Power-up, continuousbackground and operator-initiatedtest modes combine to boostsystem availability and accelerateoperational readiness.UNIQUE EXTENSION/RETRACTIONMECHANISM TRANSFORMS COMPACTTOW-BODY CONFIGURATION TO ALARGE-APERTURE MULTIDIRECTIONALTRANSMITTERDISPLAYS AND OPERATOR INTERFACES>State-of-the-art workstation-based operator machineinterface: Trackball, point-and-click control, pull-down menu function and parameter selection allows easy access to key information. >Displays: A strategic balance of multifunction displays,built on a modern OpenGL framework, offer flexible search, classification and geographic formats. Ground-stabilized, high-resolution color monitors capture details in the real-time processed sonar data. > B uilt-in operator aids: To simplify operation, LFATS provides recommended mode/parameter settings, automated range-of-day estimation and data history recall. >COTS hardware: LFATS incorporates a modular, expandable open architecture to accommodate future technology.L3Harrissellsht_LFATS© 2022 L3Harris Technologies, Inc. | 09/2022NON-EXPORT CONTROLLED - These item(s)/data have been reviewed in accordance with the InternationalTraffic in Arms Regulations (ITAR), 22 CFR part 120.33, and the Export Administration Regulations (EAR), 15 CFR 734(3)(b)(3), and may be released without export restrictions.L3Harris Technologies is an agile global aerospace and defense technology innovator, delivering end-to-endsolutions that meet customers’ mission-critical needs. The company provides advanced defense and commercial technologies across air, land, sea, space and cyber domains.t 818 367 0111 | f 818 364 2491 *******************WINCH AND HANDLINGSYSTEMSHIP ELECTRONICSTOWED SUBSYSTEMSONAR OPERATORCONSOLETRANSMIT POWERAMPLIFIER 1025 W. NASA Boulevard Melbourne, FL 32919SPECIFICATIONSOperating Modes Active, passive, test, playback, multi-staticSource Level 219 dB Omnidirectional, 222 dB Sector Steered Projector Elements 16 in 4 stavesTransmission Omnidirectional or by sector Operating Depth 15-to-300 m Survival Speed 30 knotsSize Winch & Handling Subsystem:180 in. x 138 in. x 84 in.(4.5 m x 3.5 m x 2.2 m)Sonar Operator Console:60 in. x 26 in. x 68 in.(1.52 m x 0.66 m x 1.73 m)Transmit Power Amplifier:42 in. x 28 in. x 68 in.(1.07 m x 0.71 m x 1.73 m)Weight Winch & Handling: 3,954 kg (8,717 lb.)Towed Subsystem: 678 kg (1,495 lb.)Ship Electronics: 928 kg (2,045 lb.)Platforms Frigates, corvettes, small patrol boats Receive ArrayConfiguration: Twin-lineNumber of channels: 48 per lineLength: 26.5 m (86.9 ft.)Array directivity: >18 dB @ 1,380 HzLFATS PROCESSINGActiveActive Band 1,200-to-1,00 HzProcessing CW, FM, wavetrain, multi-pulse matched filtering Pulse Lengths Range-dependent, .039 to 10 sec. max.FM Bandwidth 50, 100 and 300 HzTracking 20 auto and operator-initiated Displays PPI, bearing range, Doppler range, FM A-scan, geographic overlayRange Scale5, 10, 20, 40, and 80 kyd PassivePassive Band Continuous 100-to-2,000 HzProcessing Broadband, narrowband, ALI, DEMON and tracking Displays BTR, BFI, NALI, DEMON and LOFAR Tracking 20 auto and operator-initiatedCommonOwn-ship noise reduction, doppler nullification, directional audio。
JBL MRX525 音频系统说明书

JBL Incorporated, 8500 Balboa Boulevard, P .O.Box 2200, Northridge, California 91329 U.S.A.J B L M R X 525 R E V HPACKAGE WIRING DIAGRAM03-12T echnical ManualJBL MRX525SPECIFICATIONSACOUSTIC & ELECTRICAL SPECIFICATIONS:•Nominal Impedance: 4 Ohms •Power Capacity:800W / 1600W / 3200W, 2 hrs.(Cont/ Prog/Peak):700W / 1400W / 2800W, 100 hrs • Frequency Range:40 Hz - 20 kHz (-10 dB)•Frequency Response:57 Hz – 20 kHz (±3 dB) •Maximum SPL:129 dB SPL continuous (135 dB SPL peak)•System Sensitivity:100 dB SPL (1 watt @ 1 meter)•Crossover Frequency:1.8 kHzSYSTEM COMPONENTS:•Cabinet:Enclosure (Not for Sale)•Grille:362524-001•Low Frequency 265H-1, 380 mm (15 in) Differential Transducer:Drive woofer •DC Resistance: 5.0 ohm ±10%•High Frequency 2408H, 37.5 mm (1.5 in) annularTransducer:polymer diaphragm, neodymium compression driver•DC Resistance:4.8 ohm +/- 0.2 ohmSYSTEM COMPONENTS:(CONT’D) • Crossover Network:364969-001AURAL SWEEP TEST SPECIFICATIONS:A.System Aural Sweep T est:7.0V Input, 50 Hz to 20 kHzB.L.F .Aural Sweep Test:7.0V Input, 10 Hz to 300 HzC.H.F .Aural Sweep Test:0.75V Input, 200 Hz to 1.5 kHz PHYSICAL SPECIFICATIONS:•Enclosure Dimensions:1240 x 535 x 460 mm D (H x W x D)(48.75 x 21.0 x 18.0 in D)•Net Weight (ea):84.0 lb (38.2 kg)WARRANTY INFORMATION:•Refer to Warranty Statement packed with each product.Warranty 59660Manual362053-001Carton362293-001Fillers (2)362294-002Pkg, Microfoam (8) 49790PPop Sticker 362896-001NETWORK SCHEMATIC - (364969-001)Driver, High Freq 2408H(361549-001X) Driver Repl D8R2408Driver,Low Freq (2)265H(362048-001X)Cone Repl C8R265 265H-1 (2)(363837-003X)Cone Repl C8R265-1Network Assembly 364969-001YEL YEL/BLKGRN GRN/BLKWH/BLK WH NOTE:INDIVIDUAL PARTS FOR THE ABOVE CROSSOVER ARE NOT AVAILABLE.A COMPLETE REPLACEMENT ASSEMBL Y SHOULD BE ORDERED.JBL MRX525804-11110-1610-32 x 1, FLT, PH,BLK OXIDE, LCSNetwork, Input364969-001Screw (6)882-41110-106 x 5/8, PAN, PH,BLK ZINC, LCSNameplate 362051-001Grille Assembly w/ Screen362524-001 Screw (26)882-51110-12Foot (4)353445-001Screw (4)884-41110-1210 x 3/4, PAN, PH,PB, BLK ZINC, LCSDriver, Low Freq (2)265H(362048-001X)Cone ReplC8R265265H-1(363837-003X)Cone ReplC8R265-1Network, Input362292-001Screw (2)882-41110-106 x 5/8, PAN, PH,BLK ZINC, LCS。
rdn原理 -回复
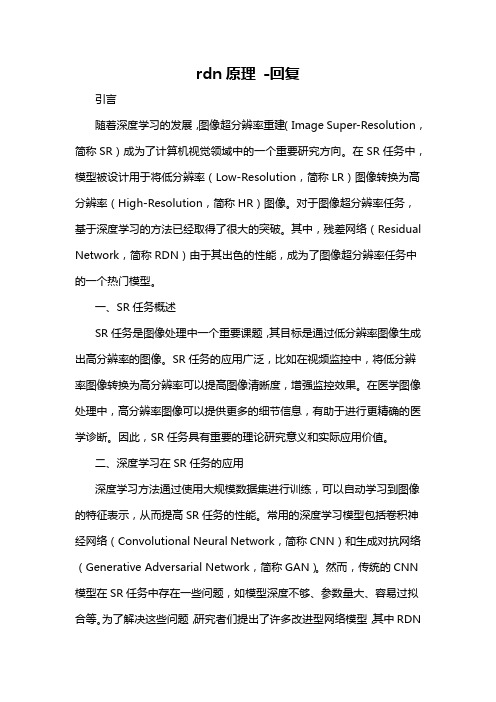
rdn原理-回复引言随着深度学习的发展,图像超分辨率重建(Image Super-Resolution,简称SR)成为了计算机视觉领域中的一个重要研究方向。
在SR任务中,模型被设计用于将低分辨率(Low-Resolution,简称LR)图像转换为高分辨率(High-Resolution,简称HR)图像。
对于图像超分辨率任务,基于深度学习的方法已经取得了很大的突破。
其中,残差网络(Residual Network,简称RDN)由于其出色的性能,成为了图像超分辨率任务中的一个热门模型。
一、SR任务概述SR任务是图像处理中一个重要课题,其目标是通过低分辨率图像生成出高分辨率的图像。
SR任务的应用广泛,比如在视频监控中,将低分辨率图像转换为高分辨率可以提高图像清晰度,增强监控效果。
在医学图像处理中,高分辨率图像可以提供更多的细节信息,有助于进行更精确的医学诊断。
因此,SR任务具有重要的理论研究意义和实际应用价值。
二、深度学习在SR任务的应用深度学习方法通过使用大规模数据集进行训练,可以自动学习到图像的特征表示,从而提高SR任务的性能。
常用的深度学习模型包括卷积神经网络(Convolutional Neural Network,简称CNN)和生成对抗网络(Generative Adversarial Network,简称GAN)。
然而,传统的CNN 模型在SR任务中存在一些问题,如模型深度不够、参数量大、容易过拟合等。
为了解决这些问题,研究者们提出了许多改进型网络模型,其中RDN是一种具有代表性的模型。
三、RDN模型介绍RDN模型是由何佳林等人于2018年提出的一种深度残差网络。
RDN 模型通过引入密集连接(Dense Connection)和残差学习(Residual Learning)的思想,提高了SR任务的性能。
具体而言,RDN模型使用了残差单元(Residual Unit)进行特征提取,将低分辨率图像通过多个残差单元进行特征增强。
深度学习模型的调优与测试方法
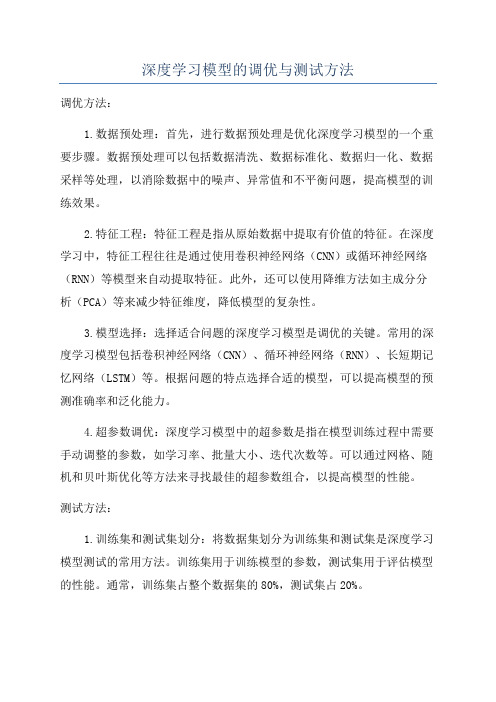
深度学习模型的调优与测试方法调优方法:1.数据预处理:首先,进行数据预处理是优化深度学习模型的一个重要步骤。
数据预处理可以包括数据清洗、数据标准化、数据归一化、数据采样等处理,以消除数据中的噪声、异常值和不平衡问题,提高模型的训练效果。
2.特征工程:特征工程是指从原始数据中提取有价值的特征。
在深度学习中,特征工程往往是通过使用卷积神经网络(CNN)或循环神经网络(RNN)等模型来自动提取特征。
此外,还可以使用降维方法如主成分分析(PCA)等来减少特征维度,降低模型的复杂性。
3.模型选择:选择适合问题的深度学习模型是调优的关键。
常用的深度学习模型包括卷积神经网络(CNN)、循环神经网络(RNN)、长短期记忆网络(LSTM)等。
根据问题的特点选择合适的模型,可以提高模型的预测准确率和泛化能力。
4.超参数调优:深度学习模型中的超参数是指在模型训练过程中需要手动调整的参数,如学习率、批量大小、迭代次数等。
可以通过网格、随机和贝叶斯优化等方法来寻找最佳的超参数组合,以提高模型的性能。
测试方法:1.训练集和测试集划分:将数据集划分为训练集和测试集是深度学习模型测试的常用方法。
训练集用于训练模型的参数,测试集用于评估模型的性能。
通常,训练集占整个数据集的80%,测试集占20%。
2.交叉验证:交叉验证是检验模型稳定性和泛化性能的一种方法。
常用的交叉验证方法包括k折交叉验证和留一交叉验证。
k折交叉验证将数据集分成k个子集,模型在每个子集上训练和测试k次,最终取平均性能作为模型的评估指标。
3.混淆矩阵和评估指标:使用混淆矩阵可以直观地评估模型的性能。
混淆矩阵包括真正例(TP)、假正例(FP)、真反例(TN)和假反例(FN)。
根据混淆矩阵可以计算准确率、召回率、精确率和F1值等评估指标,以衡量模型的分类效果。
4.网格和交叉验证:在测试过程中,可以使用网格和交叉验证来找到最佳的模型参数。
网格将所有可能的参数组合进行排列组合,然后在交叉验证中评估每个参数组合的性能,最终选择性能最好的参数组合。
nsight system 常用方法
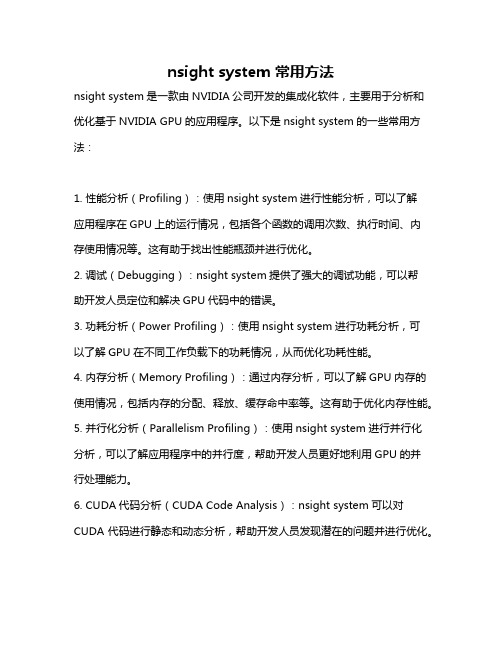
nsight system 常用方法nsight system是一款由NVIDIA公司开发的集成化软件,主要用于分析和优化基于NVIDIA GPU的应用程序。
以下是nsight system的一些常用方法:1. 性能分析(Profiling):使用nsight system进行性能分析,可以了解应用程序在GPU上的运行情况,包括各个函数的调用次数、执行时间、内存使用情况等。
这有助于找出性能瓶颈并进行优化。
2. 调试(Debugging):nsight system提供了强大的调试功能,可以帮助开发人员定位和解决GPU代码中的错误。
3. 功耗分析(Power Profiling):使用nsight system进行功耗分析,可以了解GPU在不同工作负载下的功耗情况,从而优化功耗性能。
4. 内存分析(Memory Profiling):通过内存分析,可以了解GPU内存的使用情况,包括内存的分配、释放、缓存命中率等。
这有助于优化内存性能。
5. 并行化分析(Parallelism Profiling):使用nsight system进行并行化分析,可以了解应用程序中的并行度,帮助开发人员更好地利用GPU的并行处理能力。
6. CUDA代码分析(CUDA Code Analysis):nsight system可以对CUDA代码进行静态和动态分析,帮助开发人员发现潜在的问题并进行优化。
7. OpenACC代码分析(OpenACC Code Analysis):nsight system也支持OpenACC标准,可以对OpenACC代码进行分析和优化。
以上是nsight system的一些常用方法,它们可以帮助开发人员更好地理解和优化基于NVIDIA GPU的应用程序。
IBM TRIRIGA 产品文档说明书
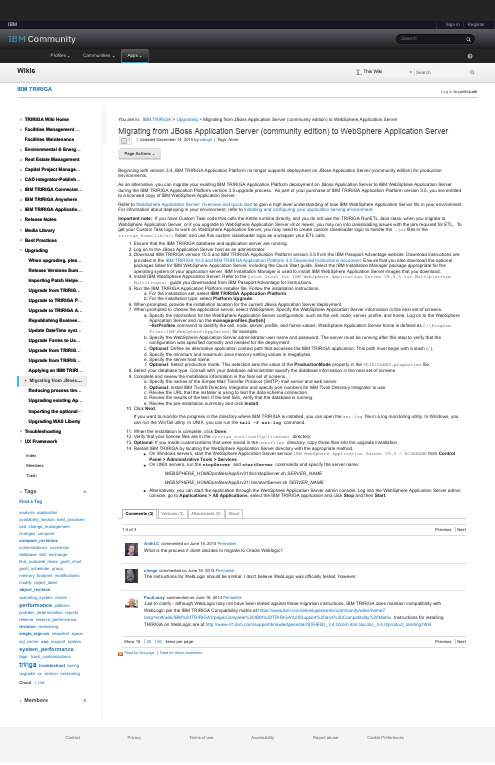
Sign in | Register IBMSearchIBM Profiles Communities Apps1-3 of 3Previous Next Show 102550 items per page Previous Next TRIRIGA Wiki HomeFacilities Management …Facilities MaintenanceEnvironmental & Energ …Real Estate ManagementCapital Project Manage …CAD Integrator-Publish …IBM TRIRIGA Connector …IBM TRIRIGA AnywhereIBM TRIRIGA Applicatio …Release NotesMedia LibraryBest PracticesUpgradingWhen upgrading, plea …Release Versions Sum …Importing Patch Helpe …Upgrade from TRIRIG …Upgrade to TRIRIGA P …Upgrade to TRIRIGA A …Republishing Busines …Update DateTime syst …Upgrade Forms to Us …Upgrade from TRIRIG …Upgrade from TRIRIG …Applying an IBM TRIRI …▪Migrating from JBoss …Reducing process tim …Upgrading existing Ap …Importing the optional …Upgrading WAS LibertyTroubleshootingUX FrameworkContact Privacy Terms of use Accessibility Report abuse Cookie Preferences You are in: IBM TRIRIGA > Upgrading > Migrating from JBoss Application Server (community edition) to WebSphere Application Server Feed for this page |Feed for these commentsSubmit WikisSearch This Wiki IBM TRIRIGA Log in to participate IndexMembersTrash TagsFind a Taganalysis applicationavailability_section best_practicescad change_managementchanges comparecompare_revisionscustomizations customizedatabase db2 exchangefind_available_times gantt_chartgantt_scheduler groupmemory_footprint modificationsmodify object_labelobject_revisionoperating_system oracle performance platformproblem_determination reportsreserve reserve_performancerevision revisioningsingle_sign-on snapshot spacesql_server sso support system system_performancetags: track_customizations tririga troubleshoot tuningupgrade ux version versioningCloud ListMembersMigrating from JBoss Application Server (community edition) to WebSphere Application Server |Updated December 14, 2015 by clange |Tags: None Page Actions 1Beginning with version 3.4, IBM TRIRIGA Application Platform no longer supports deployment on JBoss Application Server (community edition) for production environments.As an alternative, you can migrate your existing IBM TRIRIGA Application Platform deployment on JBoss Application Server to IBM WebSphere Application Server during the IBM TRIRIGA Application Platform version 3.5 upgrade process. As part of your purchase of IBM TRIRIGA Application Platform version 3.5, you are entitled to a licensed copy of IBM WebSphere Application Server.Refer to WebSphere Application Server: Overview and quick start to gain a high level understanding of how IBM WebSphere Application Server fits in your environment.For information about deploying in your environment, refer to Installing and configuring your application serving environment .Important note: If you have Custom Task code that calls the Kettle runtime directly, and you do not use the TRIRIGA RunETL Java class, when you migrate to WebSphere Application Server, or if you upgrade to WebSphere Application Server v8 or newer, you may run into classloading issues with the jars required for ETL, To get your Custom Task logic to work on WebSphere Application Server, you may need to create custom classloader logic to handle the .jar files in the tririga_home /lib/etl folder, and use this custom classloader logic as a wrapper your ETL calls.1.Ensure that the IBM TRIRIGA database and application server are running.2.Log on to the JBoss Application Server host as an administrator.3.Download IBM TRIRIGA version 10.5 and IBM TRIRIGA Application Platform version 3.5 from the IBM Passport Advantage website. Download instructions are provided in the IBM TRIRIGA 10.5 and IBM TRIRIGA Application Platform 3.5 Download Instructions document . Ensure that you also download the optional packages listed for IBM WebSphere Application Server, including the Quick Start guide. Select the IBM Installation Manager package appropriate for the operating system of your application server. IBM Installation Manager is used to install IBM WebSphere Application Server images that you download.4.Install IBM WebSphere Application Server. Refer to the Quick Start for IBM WebSphere Application Server V8.5.5 for Multiplatform Multilingual guide you downloaded from IBM Passport Advantage for instructions.5.Run the IBM TRIRIGA Application Platform installer file. Follow the installation instructions.a.For the installation set, select IBM TRIRIGA Application Platform .b.For the installation type, select Platform Upgrade .6.When prompted, provide the installation location for the current JBoss Application Server deployment.7.When prompted to choose the application server, select WebSphere. Specify the WebSphere Application Server information in the next set of screens.a.Specify the information for the WebSphere Application Server configuration, such as the cell, node, server, profile, and home. Log on to the WebSphere Application Server and run the manageprofiles.[bat|sh]–listProfiles command to identify the cell, node, server, profile, and home values. WebSphere Application Server home is defined as C:\Program Files\IBM\WebSphere\AppServer , for example.b.Specify the WebSphere Application Server administrator user name and password. The server must be running after this step to verify that the configuration was specified correctly and needed for the deployment.c.Optional : Define an alternative application context path that accesses the IBM TRIRIGA application. This path must begin with a slash (/).d.Specify the minimum and maximum Java memory setting values in megabytes.e.Specify the server host name.f.Optional : Select production mode. This selection sets the value of the ProductionMode property in the TRIRIGAWEB.properties file.8.Select your database type. Consult with your database administrator specify the database information in the next set of plete and review the installation information in the final set of screens.a.Specify the names of the Simple Mail Transfer Protocol (SMTP) mail server and web server.b.Optional : Install IBM Tivoli® Directory Integrator and specify port numbers for IBM Tivoli Directory Integrator to use.c.Review the URL that the installer is using to test the data schema connection.d.Review the results of the test. If the test fails, verify that the database is running.e.Review the pre-installation summary and click Install .10.Click Next .If you want to monitor the progress in the directory where IBM TRIRIGA is installed, you can open the ant.log file in a log monitoring utility. In Windows, you can run the WinTail utility. In UNIX, you can run the tail –f ant.log command.11.When the installation is complete, click Done .12.Verify that your license files are in the tririga_root \config\licenses directory.13.Optional : If you made customizations that were saved in the userfiles directory, copy those files into the upgrade installation.14.Restart IBM TRIRIGA by locating the WebSphere Application Server directory with the appropriate method.On Windows servers, start the WebSphere Application Server service IBM WebSphere Application Server V8.5 - NODENAME from Control Panel > Administrative Tools > Services .On UNIX servers, run the stopServer and startServer commands and specify the server name:WEBSPHERE_HOME /profiles/AppSrv01/bin/stopServer.sh SERVER_NAMEWEBSPHERE_HOME /profiles/AppSrv01/ bin/startServer.sh SERVER_NAMEAlternatively, you can start the application through the WebSphere Application Server admin console. Log into the WebSphere Application Server admin console, go to Applications > All Applications , select the IBM TRIRIGA application and click Stop and then Start .AnthLC commented on June 15, 2014 Permalink What is the process if client decides to migrate to Oracle Weblogic?clange commented on June 16, 2014 Permalink The instructions for WebLogic should be similar. I don't believe WebLogic was officially tested, however.PaulLacey commented on June 18, 2014 PermalinkJust to clarify - although WebLogic may not have been tested against these migration instructions, IBM TRIRIGA does maintain compatibility with WebLogic per the IBM TRIRIGA Compatibility matrix at https:///developerworks/community/wikis/home?lang=en#/wiki/IBM%20TRIRIGA1/page/Complete%20IBM%20TRIRIGA%20Support%20and%20Compatibility%20Matrix. Instructions for installing TRIRIGA on WebLogic are at /support/knowledgecenter/SSHEB3_3.4.0/com.ibm.tap.doc_3.4.0/product_ments (3)Versions (3)Attachments (0)About。
dperf使用方法

dperf使用方法一、安装dperf要使用dperf,首先需要将其安装到您的系统中。
dperf是Go语言编写的,您可以从dperf的GitHub仓库中获取和安装它。
以下是安装dperf的步骤:1. 确保已安装Go语言的运行时环境。
可以通过在终端中运行`go version`命令来检查。
2. 打开终端并执行以下命令以获取dperf的源代码:``````3. 安装成功之后,在终端中执行以下命令以编译和安装dperf:``````4. 安装完成后,您可以在终端中执行`dperf`命令来验证dperf是否已成功安装。
如果您看到dperf的帮助信息,则说明安装成功。
二、配置dperf在运行dperf之前,您需要对其进行一些配置。
以下是配置dperf的主要步骤:1.创建一个配置文件在您的工作目录中创建一个名为`dperf.conf`的文本文件。
这个文件将用于配置dperf的行为。
2. 配置dperf服务器在`dperf.conf`文件中,您需要配置dperf服务器的参数。
以下是一些重要的参数:- `host`:dperf服务器的主机名或IP地址。
- `port`:dperf服务器监听的端口。
- `report_interval`:性能报告的时间间隔。
- `report_file`:性能报告的输出文件。
例如,以下是一个配置示例:```[server]host = localhostport = 8080report_interval = 10sreport_file = /path/to/report.txt```3. 配置dperf客户端在`dperf.conf`文件中,您还需要配置dperf客户端的参数。
以下是一些重要的参数:- `server_host`:dperf服务器的主机名或IP地址。
- `server_port`:dperf服务器监听的端口。
- `duration`:性能测试的持续时间。
- `concurrent_users`:并发用户数。
Micro Focus z Server消息和诊断说明书
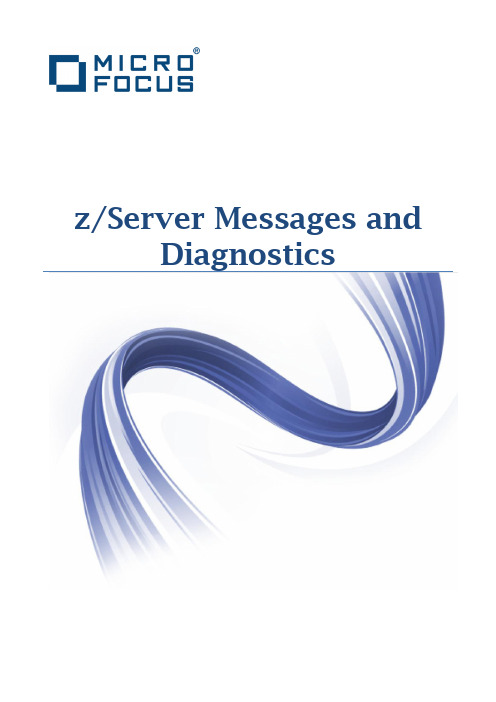
z/Server Messages and Diagnosticsz/Server Messages and DiagnosticsMicro FocusThe Lawn22-30 Old Bath RoadNewbury, Berkshire RG14 1QNUKCopyright © 2011-2014 Micro Focus. All rights reserved.MICRO FOCUS and the Micro Focus logo are trademarks or registered trademarks of Micro Focus or its subsidiaries or affiliatedcompanies in the United States, United Kingdom and other countries. All other marks are the property of their respective owners.z/Server Messages and Diagnostics Contents1 Introduction (27)1.1 Message format (27)1.2 Error message example (28)1.3 Warning message example (29)1.4 Information message example (29)1.5 Messages and GTF (29)2 Messages (30)2.1 API - Application Programming Interface (30)2.1.1 API0001I (30)2.1.2 API0002I (31)2.1.3 API0007E (31)2.1.4 API0008I (31)2.1.5 API0009E (31)2.1.6 API0010I (31)2.1.7 API0011I (32)2.1.8 API0015I (32)2.1.9 API0054S (32)2.1.10 API0065I (32)2.1.11 API0069E (32)2.1.12 API0072W (33)2.1.13 API0099I (33)2.1.14 API0106W (33)2.1.15 API0107E (33)2.1.16 API0108W (33)2.1.17 API0109I (34)2.1.18 API0110I (34)2.1.19 API0111I (34)2.2 BND - IEWBIND (Binder) Interface (35)2.2.1 BND0001E (35)2.2.2 BND0002I (35)2.2.3 BND0003I (35)2.2.4 BND0004E (35)z/Server Messages and Diagnostics2.2.5 BND0005E (35)2.2.6 BND0006I (36)2.3 CSI - Catalog Search Interface (37)2.3.1 CSI0001E (37)2.3.2 CSI0002I (37)2.3.3 CSI0003E (37)2.3.4 CSI0004I (37)2.3.5 CSI0005I (37)2.3.6 CSI0006I (38)2.3.7 CSI0007I (38)2.3.8 CSI0008E (38)2.3.9 CSI0009I (38)2.3.10 CSI0010E (39)2.3.11 CSI0011I (39)2.3.12 CSI0012E (39)2.3.13 CSI0013E (39)2.3.14 CSI0014W (39)2.3.15 CSI0015I (39)2.3.16 CSI0016E (40)2.3.17 CSI0017I (40)2.3.18 CSI0018I (40)2.3.19 CSI0019I (40)2.3.20 CSI0020E (40)2.3.21 CSI0021E (40)2.3.22 CSI0022E (41)2.3.23 CSI0023E (41)2.3.24 CSI0024E (41)2.3.25 CSI0025E (41)2.3.26 CSI0026I (41)2.3.27 CSI0027I (42)2.3.28 CSI0028I (42)2.3.29 CSI0029E (42)2.3.30 CSI0030W (42)2.3.31 CSI0031I (42)z/Server Messages and Diagnostics2.3.32 CSI0032I (43)2.3.33 CSI0033E (43)2.3.34 CSI0034I (43)2.3.35 CSI0035E (43)2.3.36 CSI0036E (43)2.3.37 CSI0037E (43)2.3.38 CSI0038E (44)2.3.39 CSI0039E (44)2.3.40 CSI0040I (44)2.3.41 CSI0041I (44)2.3.42 CSI0042E (44)2.3.43 CSI0043I (44)2.3.44 CSI0044E (45)2.3.45 CSI0045E (45)2.3.46 CSI0046E (45)2.3.47 CSI0047E (45)2.3.48 CSI0048E (45)2.3.49 CSI0049I (45)2.3.50 CSI0050E (46)2.3.51 CSI0051E (46)2.3.52 CSI0052E (46)2.3.53 CSI0053E (46)2.3.54 CSI0054I (46)2.3.55 CSI0055E (46)2.3.56 CSI0056E (47)2.3.57 CSI0057W (47)2.3.58 CSI0058E (47)2.3.59 CSI0059E (47)2.3.60 CSI0060E (47)2.3.61 CSI0061I (47)2.3.62 CSI0062I (48)2.3.63 CSI0063E (48)2.4 DSC - Holder Task Configuration (49)2.4.1 DSC0001E (49)z/Server Messages and Diagnostics2.4.2 DSC0002E (49)2.4.3 DSC0003E (49)2.4.4 DSC0004E (49)2.4.5 DSC0005E (49)2.4.6 DSC0008E (49)2.4.7 DSC0009E (50)2.4.8 DSC0020E (50)2.4.9 DSC0022E (50)2.4.10 DSC0023 (50)2.4.11 DSC0024 (50)2.4.12 DSC0025 (50)2.4.13 DSC0026W (50)2.4.14 DSC0027E (50)2.4.15 DSC0099I (51)2.4.16 DSC0100E (51)2.4.17 DSC0101I (51)2.4.18 DSC0102I (51)2.4.19 DSC0200E (51)2.5 EMC - Extended MCS Interface (52)2.5.1 EMC0001E (52)2.5.2 EMC0002I (52)2.5.3 EMC0003E (52)2.5.4 EMC0004E (53)2.5.5 EMC0005I (53)2.5.6 EMC0006E (53)2.5.7 EMC0007E (53)2.5.8 EMC0008I (53)2.5.9 EMC0009I (54)2.5.10 EMC0010W (54)2.5.11 EMC0011E (54)2.5.12 EMC0012E (54)2.5.13 EMC0013I (54)2.5.14 EMC0014E (54)2.5.15 EMC0015I (55)z/Server Messages and Diagnostics2.5.16 EMC0016I (55)2.5.17 EMC0017I (55)2.5.18 EMC0018I (55)2.5.19 EMC0019I (55)2.5.20 EMC0020I (55)2.5.21 EMC0021I (56)2.5.22 EMC0022E (56)2.5.23 EMC0023E (56)2.6 HLD - Holder Task Interface (57)2.6.1 HLD0001I (57)2.6.2 HLD0002I (57)2.6.3 HLD0003I (57)2.6.4 HLD0004I (57)2.6.5 HLD0005I (58)2.6.6 HLD0006I (58)2.6.7 HLD0007I (58)2.6.8 HLD0008E (58)2.6.9 HLD0009I (59)2.6.10 HLD0010E (59)2.6.11 HLD0011I (59)2.6.12 HLD0012I (59)2.6.13 HLD0013E (59)2.6.14 HLD0014E (59)2.6.15 HLD0015E (60)2.6.16 HLD0016W (60)2.6.17 HLD0017E (60)2.6.18 HLD0018I (60)2.6.19 HLD0019I (60)2.6.20 HLD0020I (61)2.6.21 HLD0021I (61)2.6.22 HLD0022I (61)2.6.23 HLD0024I (61)2.6.24 HLD0025I (61)2.6.25 HLD0026I (62)z/Server Messages and Diagnostics2.6.26 HLD0027I (62)2.6.27 HLD0028I (62)2.6.28 HLD0029I (62)2.6.29 HLD0030I (63)2.6.30 HLD0031I (64)2.6.31 HLD0032I (64)2.6.32 HLD0033I (64)2.6.33 HLD0034I (64)2.6.34 HLD0035I (64)2.6.35 HLD0036E (64)2.6.36 HLD0038E (65)2.6.37 HLD0039I (65)2.6.38 HLD0040E (65)2.6.39 HLD0041I (65)2.6.40 HLD0042I (65)2.6.41 HLD0043I (66)2.6.42 HLD0044I (66)2.6.43 HLD0045E (66)2.6.44 HLD0046E (66)2.6.45 HLD0047I (66)2.6.46 HLD0048I (66)2.6.47 HLD0049I (67)2.6.48 HLD0050I (67)2.6.49 HLD0051I (67)2.6.50 HLD0052I (67)2.6.51 HLD0053E (67)2.6.52 HLD0054I (67)2.6.53 HLD0055E (68)2.6.54 HLD0056E (68)2.6.55 HLD0057W (68)2.6.56 HLD0058E (68)2.6.57 HLD0059E (68)2.6.58 HLD0060I (69)2.6.59 HLD0061E (69)z/Server Messages and Diagnostics2.6.60 HLD0062I (69)2.6.61 HLD0063I (69)2.6.62 HLD0064I (69)2.6.63 HLD0065I (69)2.6.64 HLD0066I (70)2.6.65 HLD0067E (70)2.6.66 HLD0066I (70)2.6.67 HLD0066I (70)2.6.68 HLD2001W (70)2.7 IPC - Command Task (71)2.7.1 IPC0001W (71)2.7.2 IPC0002W (71)2.7.3 IPC0003W (71)2.7.4 IPC0004W (71)2.7.5 IPC0005I (71)2.7.6 IPC0006W (71)2.7.7 IPC0007W (72)2.7.8 IPC0040I (72)2.7.9 IPC0042I (72)2.7.10 IPC0043I (72)2.7.11 IPC0044I (72)2.7.12 IPC0052I (72)2.7.13 IPC0061I (73)2.7.14 IPC0062I (73)2.7.15 IPC0063I (73)2.7.16 IPC0064I (73)2.7.17 IPC0065E (73)2.7.18 IPC0066I (73)2.7.19 IPC0067I (74)2.7.20 IPC0068I (74)2.7.21 IPC0069E (74)2.7.22 IPC0070E (74)2.7.23 IPC0071I (74)2.7.24 IPC0072E (74)z/Server Messages and Diagnostics2.7.25 IPC0073I (75)2.7.26 IPC0074I (75)2.7.27 IPC0075I (75)2.7.28 IPC0076I (75)2.7.29 IPC0077E (75)2.7.30 IPC0078E (75)2.7.31 IPC0079I (76)2.7.32 IPC0080I (76)2.7.33 IPC0081E (76)2.7.34 IPC0082I (76)2.7.35 IPC0083E (76)2.7.36 IPC0084I (76)2.7.37 IPC0085E (77)2.7.38 IPC0086W (77)2.7.39 IPC0087E (77)2.7.40 IPC0088E (77)2.7.41 IPC0090I (78)2.7.42 IPC0091I (78)2.7.43 IPC0092E (78)2.7.44 IPC0093E (78)2.7.45 IPC0094I (78)2.7.46 IPC0095I (78)2.7.47 IPC0096I (79)2.7.48 IPC0097E (79)2.7.49 IPC0098I (79)2.7.50 IPC0099I (79)2.7.51 IPC0100E (79)2.7.52 IPC0101I (79)2.8 JES - Job Entry Subsystem Interface (80)2.8.1 JES0001I (80)2.8.2 JES0002I (80)2.8.3 JES0003E (80)2.8.4 JES0004E (81)2.8.5 JES0005E (81)2.8.7 JES0007E (81)2.8.8 JES0008I (82)2.8.9 JES0009I (82)2.8.10 JES0010I (82)2.9 LIC - License Interface (83)2.9.1 LIC0001E (83)2.9.2 LIC0002W (83)2.9.3 LIC0003I (83)2.9.4 LIC0004W (83)2.9.5 LIC0005E (83)2.9.6 LIC0006E (84)2.9.7 LIC0007W (84)2.9.8 LIC0008E (84)2.9.9 LIC0009I (84)2.9.10 LIC0010E (84)2.9.11 LIC0011I (84)2.10 MAL - Email Interface (86)2.10.1 MAL0001E (86)2.10.2 MAL0002E (86)2.10.3 MAL0003E (86)2.10.4 MAL0004E (86)2.10.5 MAL0006I (86)2.10.6 MAL0007I (86)2.10.7 MAL0008I (87)2.10.8 MAL0009I (87)2.10.9 MAL0010I (87)2.10.10 MAL0011E (87)2.10.11 MAL0012E (87)2.10.12 MAL0013E (88)2.10.13 MAL0014I (88)2.11 REC - Recovery Exception Handler (89)2.11.1 REC0001E (89)2.11.2 REC0002E (89)2.11.4 REC0004I (90)2.11.5 REC0005I (90)2.11.6 REC0006I (90)2.11.7 REC0007I (90)2.11.8 REC0008I (90)2.11.9 REC0009E (90)2.11.10 REC0010W (91)2.11.11 REC0011E (91)2.11.12 REC0012E (91)2.11.13 REC0013E (91)2.11.14 REC0014W (91)2.11.15 REC0015I (92)2.11.16 REC0016I (92)2.11.17 REC0017I (92)2.11.18 REC0018I (92)2.11.19 REC0019I (92)2.12 REX - REXX Interface (93)2.12.1 REX0001E (93)2.12.2 REX0002I (93)2.12.3 REX0003I (93)2.12.4 REX0004I (93)2.12.5 REX0005E (94)2.12.6 REX0006E (94)2.12.7 REX0007I (94)2.12.8 REX0008E (94)2.12.9 REX0009E (94)2.12.10 REX0010E (94)2.12.11 REX0011E (95)2.12.12 REX0012I (95)2.12.13 REX0013W (95)2.12.14 REX0014I (95)2.12.15 REX0015E (95)2.12.16 REX0016W (96)2.12.18 REX0018E (96)2.12.19 REX0019S (96)2.12.20 REX0020I (96)2.12.21 REX0021I (96)2.12.22 REX0022E (97)2.12.23 REX0023S (97)2.12.24 REX0024I (97)2.12.25 REX0025E (97)2.12.26 REX0026I (97)2.12.27 REX0027I (98)2.12.28 REX0028I (98)2.12.29 REX0029E (98)2.12.30 REX0030E (98)2.12.31 REX0031I (98)2.12.32 REX0032E (98)2.12.33 REX0033I (99)2.12.34 REX0034I (99)2.12.35 REX0035E (99)2.12.36 REX0036I (99)2.12.37 REX0037I (99)2.12.38 REX0038I (100)2.12.39 REX0039I (100)2.12.40 REX0040I (100)2.12.41 REX0041I (100)2.12.42 REX0042E (100)2.12.43 REX0043I (100)2.12.44 REX0044I (101)2.12.45 REX0045I (101)2.12.46 REX0046I (101)2.12.47 REX0047I (101)2.12.48 REX0048E (101)2.12.49 REX0049E (101)2.12.50 REX0050E (102)2.12.52 REX0052E (102)2.12.53 REX0053I (102)2.12.54 REX0054E (102)2.12.55 REX0055I (102)2.12.56 REX0056I (103)2.12.57 REX0057E (103)2.12.58 REX0058I (103)2.12.59 REX0059I (103)2.12.60 REX0060I (103)2.12.61 REX0062E (103)2.12.62 REX0063I (104)2.12.63 REX0064I (104)2.12.64 REX0065E (104)2.12.65 REX0066W (104)2.12.66 REX0067I (105)2.12.67 REX0068I (105)2.12.68 REX0069E (105)2.12.69 REX0070I (105)2.12.70 REX0071E (105)2.12.71 REX0072E (105)2.13 SJB - Started Job (107)2.13.1 SJB0001E (107)2.13.2 SJB0002E (107)2.13.3 SJB0003E (107)2.13.4 SJB0004I (107)2.13.5 SJB0005E (108)2.13.6 SJB0006I (108)2.14 SLR - TSO Scheduler Interface (109)2.14.1 SLR0001I (109)2.14.2 SLR0002I (109)2.14.3 SLR0003I (109)2.14.4 SLR0004I (109)2.14.5 SLR0005E (109)2.14.7 SLR0007I (110)2.14.8 SLR0008E (110)2.14.9 SLR0009E (110)2.14.10 SLR0010E (110)2.14.11 SLR0011E (110)2.14.12 SLR0012E (110)2.14.13 SLR0013E (110)2.14.14 SLR0014E (111)2.14.15 SLR0015E (111)2.14.16 SLR0016E (111)2.14.17 SLR0017E (111)2.14.18 SLR0018I (111)2.14.19 SLR0019I (111)2.14.20 SLR0020S (112)2.14.21 SLR0021S (112)2.14.22 SLR0021S (112)2.14.23 SLR0023I (112)2.14.24 SLR0024E (112)2.14.25 SLR0025E (112)2.14.26 SLR0026I (113)2.14.27 SLR0027W (113)2.14.28 SLR0028E (113)2.14.29 SLR0029W (113)2.14.30 SLR0030I (113)2.14.31 SLR0031S (113)2.14.32 SLR0032E (113)2.14.33 SLR0033E (114)2.14.34 SLR0034E (114)2.14.35 SLR0035I (114)2.14.36 SLR0036I (114)2.14.37 SLR0037I (114)2.14.38 SLR0038W (115)2.14.39 SLR0039I (115)2.14.41 SLR0041I (115)2.14.42 SLR0042I (116)2.14.43 SLR0043I (116)2.14.44 SLR0044I (116)2.14.45 SLR0045E (116)2.14.46 SLR0046I (116)2.14.47 SLR0047I (117)2.14.48 SLR0048I (117)2.14.49 SLR0049I (117)2.14.50 SLR0050E (117)2.14.51 SLR0051E (117)2.14.52 SLR0052E (118)2.14.53 SLR0053W (118)2.14.54 SLR0054I (118)2.14.55 SLR0055E (118)2.14.56 SLR0056E (118)2.14.57 SLR0057I (118)2.14.58 SLR0058I (119)2.14.59 SLR0059E (119)2.14.60 SLR0060E (119)2.14.61 SLR0061E (119)2.14.62 SLR0062E (119)2.14.63 SLR0063E (119)2.14.64 SLR0064I (120)2.14.65 SLR0065E (120)2.14.66 SLR0066E (120)2.14.67 SLR0067E (120)2.14.68 SLR0068I (120)2.14.69 SLR0069E (120)2.14.70 SLR0070I (120)2.14.71 SLR0071E (121)2.14.72 SLR0072E (121)2.14.73 SLR0073E (121)2.14.75 SLR0075I (121)2.14.76 SLR0076I (122)2.14.77 SLR0077I (122)2.14.78 SLR0078E (122)2.14.79 SLR0079E (122)2.14.80 SLR0080E (122)2.14.81 SLR0081I (123)2.14.82 SLR0082E (123)2.14.83 SLR0083I (123)2.14.84 SLR0084E (123)2.14.85 SLR0085E (123)2.14.86 SLR0086I (124)2.14.87 SLR0087E (124)2.14.88 SLR0088E (124)2.14.89 SLR0089E (124)2.14.90 SLR0090E (124)2.14.91 SLR0091E (125)2.14.92 SLR0092E (125)2.14.93 SLR0093E (125)2.14.94 SLR0094E (125)2.14.95 SLR0095E (125)2.14.96 SLR0096E (126)2.14.97 SLR0097E (126)2.14.98 SLR0098E (126)2.14.99 SLR0099E (126)2.14.100 SLR0100E (126)2.14.101 SLR0101I (127)2.14.102 SLR0102W (127)2.14.103 SLR0103E (127)2.14.104 SLR0104E (127)2.14.105 SLR0105E (127)2.14.106 SLR0106E (127)2.14.107 SLR0107I (128)2.14.109 SLR0109I (128)2.14.110 SLR0110I (128)2.14.111 SLR0111I (128)2.14.112 SLR0112E (129)2.14.113 SLR0113I (129)2.14.114 SLR0114E (129)2.14.115 SLR0115I (129)2.14.116 SLR0116I (129)2.14.117 SLR0117E (129)2.14.118 SLR0118E (130)2.14.119 SLR0119E (130)2.14.120 SLR0120E (130)2.14.121 SLR0121E (130)2.14.122 SLR0122E (130)2.14.123 SLR0123E (131)2.14.124 SLR0124E (131)2.14.125 SLR0125E (131)2.14.126 SLR0126I (131)2.14.127 SLR0127E (131)2.14.128 SLR0128E (131)2.14.129 SLR0129E (132)2.14.130 SLR0130E (132)2.14.131 SLR0131E (132)2.14.132 SLR0132E (132)2.14.133 SLR0133E (132)2.14.134 SLR0134E (133)2.14.135 SLR0135S (133)2.14.136 SLR0136I (133)2.14.137 SLR0137E (133)2.14.138 SLR0139E (133)2.14.139 SLR0140E (134)2.14.140 SLR0141E (134)2.14.141 SLR0142E (134)2.15 SPR - Standard Pool Routine (135)2.15.1 SPR0001I (135)2.15.2 SPR0002E (135)2.15.3 SPR0003E (135)2.15.4 SPR0004I (135)2.15.5 SPR0005I (136)2.15.6 SPR0006I (136)2.15.7 SPR0007E (136)2.15.8 SPR0008W (136)2.15.9 SPR0009E (136)2.15.10 SPR0010S (136)2.15.11 SPR0011I (137)2.15.12 SPR0012I (137)2.15.13 SPR0013I (137)2.15.14 SPR0014I (137)2.15.15 SPR0015I (137)2.15.16 SPR0016I (138)2.16 SRV - Server Interface (139)2.16.1 SRV0001I (139)2.16.2 SRV0002I (139)2.16.3 SRV0003E (139)2.16.4 SRV0004W (139)2.16.5 SRV0005I (139)2.16.6 SRV0006I (140)2.16.7 SRV0007I (140)2.16.8 SRV0008I (140)2.16.9 SRV0009I (140)2.17 SSI - Subsystem Interface Routine (141)2.17.1 SSI0001I (141)2.17.2 SSI0002I (141)2.17.3 SSI0003I (141)2.17.4 SSI0004I (141)2.17.5 SSI0005I (141)2.17.7 SSI0007I (142)2.17.8 SSI0008I (142)2.17.9 SSI0009I (142)2.18 STA - Started Task Interface (143)2.18.1 STA0001E (143)2.18.2 STA0002E (143)2.18.3 STA0003I (143)2.18.4 STA0004E (143)2.18.5 STA0005E (143)2.18.6 STA0006I (143)2.18.7 STA0007E (144)2.19 TAU – Eclipse Client Interface (145)2.19.1 TAU0001I (145)2.19.2 TAU0002I (145)2.19.3 TAU0003I (145)2.19.4 TAU0004I (145)2.19.5 TAU0005I (145)2.19.6 TAU0006I (146)2.19.7 TAU0007E (146)2.19.8 TAU0008I (146)2.19.9 TAU0009E (146)2.19.10 TAU0010I (146)2.19.11 TAU0011I (147)2.19.12 TAU0012E (147)2.19.13 TAU0013I (147)2.19.14 TAU0014I (147)2.19.15 TAU0015I (147)2.19.16 TAU0016I (147)2.19.17 TAU0017I (147)2.19.18 TAU0018I (148)2.19.19 TAU0019W (148)2.19.20 TAU0020E (148)2.19.21 TAU0021I (148)2.19.23 TAU0023I (148)2.19.24 TAU0024I (148)2.19.25 TAU0025I (149)2.19.26 TAU0026W (149)2.19.27 TAU0027E (149)2.19.28 TAU0028W (149)2.19.29 TAU0029I (149)2.19.30 TAU0030E (150)2.19.31 TAU0031E (150)2.19.32 TAU0032E (150)2.19.33 TAU0033E (150)2.19.34 TAU0034E (150)2.19.35 TAU0035E (151)2.19.36 TAU0036I (151)2.19.37 TAU0037I (151)2.19.38 TAU0038I (151)2.19.39 TAU0039I (151)2.19.40 TAU0040E (151)2.19.41 TAU0041I (152)2.19.42 TAU0042I (152)2.19.43 TAU0043E (152)2.19.44 TAU0044E (152)2.19.45 TAU0045I (152)2.19.46 TAU0046I (152)2.19.47 TAU0047S (153)2.19.48 TAU0048W (153)2.19.49 TAU0049W (153)2.19.50 TAU0050E (153)2.19.51 TAU0051W (153)2.19.52 TAU0052I (153)2.19.53 TAU0053I (154)2.19.54 TAU0054S (154)2.19.55 TAU0055I (154)2.19.57 TAU0057E (154)2.19.58 TAU0058E (154)2.19.59 TAU0059I (155)2.19.60 TAU0060I (155)2.19.61 TAU0061I (155)2.19.62 TAU0062E (155)2.19.63 TAU0063S (155)2.19.64 TAU0064W (156)2.19.65 TAU0065I (156)2.19.66 TAU0066I (156)2.19.67 TAU0067I (156)2.19.68 TAU0068I (156)2.19.69 TAU0069E (156)2.19.70 TAU0070I (157)2.19.71 TAU0071I (157)2.19.72 TAU0072W (157)2.19.73 TAU0073S (157)2.19.74 TAU0074I (157)2.19.75 TAU0075W (157)2.19.76 TAU0076I (158)2.19.77 TAU0077I (158)2.19.78 TAU0078E (158)2.19.79 TAU0079E (158)2.19.80 TAU0080E (159)2.19.81 TAU0081E (159)2.19.82 TAU0082E (159)2.19.83 TAU0083W (159)2.19.84 TAU0084E (159)2.19.85 TAU0085E (159)2.19.86 TAU0086I (160)2.19.87 TAU0087I (160)2.19.88 TAU0088S (160)2.19.89 TAU0089E (160)2.19.91 TAU0091E (161)2.19.92 TAU0092I (161)2.19.93 TAU0093I (161)2.19.94 TAU0099I (161)2.19.95 TAU0100E (161)2.19.96 TAU0101I (162)2.19.97 TAU0102I (162)2.19.98 TAU0103W (162)2.19.99 TAU0104I (162)2.19.100 TAU0105E (162)2.19.101 TAU0106W (163)2.19.102 TAU0107I (163)2.19.103 TAU0108E (163)2.19.104 TAU0109W (163)2.19.105 TAU0110I (163)2.19.106 TAU0111I (164)2.19.107 TAU0112I (164)2.19.108 TAU0113E (164)2.19.109 TAU0114I (164)2.19.110 TAU0115E (164)2.19.111 TAU0116I (165)2.19.112 TAU0117E (165)2.19.113 TAU0118I (165)2.19.114 TAU0119E (165)2.19.115 TAU0120E (165)2.19.116 TAU0121E (165)2.19.117 TAU0122W (166)2.19.118 TAU0123S (166)2.19.119 TAU0124E (166)2.19.120 TAU0125I (166)2.19.121 TAU0126I (166)2.19.122 TAU0127E (167)2.19.123 TAU0128E (167)2.19.125 TAU0130E (167)2.19.126 TAU0131I (167)2.19.127 TAU0132E (168)2.19.128 TAU0133E (168)2.19.129 TAU0134I (168)2.19.130 TAU0135S (168)2.19.131 TAU0136S (168)2.19.132 TAU0137I (169)2.19.133 TAU0138E (169)2.19.134 TAU0139E (169)2.19.135 TAU0140E (170)2.19.136 TAU0141I (170)2.19.137 TAU0142I (170)2.19.138 TAU0143S (170)2.19.139 TAU0144W (170)2.19.140 TAU0145I (171)2.19.141 TAU0146I (171)2.19.142 TAU0147I (171)2.19.143 TAU0148E (171)2.19.144 TAU0149E (171)2.19.145 TAU0150I (172)2.19.146 TAU0151I (172)2.19.147 TAU0152I (172)2.19.148 TAU0153I (172)2.19.149 TAU0154S (172)2.19.150 TAU0155W (172)2.19.151 TAU0156E (173)2.19.152 TAU0157I (173)2.20 UNI - Unicode code conversion services (174)2.20.1 UNI0001I (174)2.20.2 UNI0002I (174)2.20.3 UNI0003I (174)2.20.4 UNI0004W (174)2.20.6 UNI0006W (175)2.20.7 UNI0007E (175)2.20.8 UNI0008W (175)2.20.9 UNI0009W (176)2.20.10 UNI0010W (176)2.20.11 UNI0011W (176)2.20.12 UNI0012E (176)2.20.13 UNI0013I (176)2.21 XML - XML System Parser Interface (177)2.21.1 XML0001E (177)2.21.2 XML0002I (177)2.21.3 XML0003E (177)2.21.4 XML0004E (177)2.21.5 XML0005E (178)2.21.6 XML0006I (178)2.21.7 XML0007E (178)2.21.8 XML0008E (178)2.21.9 XML0009I (178)2.21.10 XML0010I (178)2.21.11 XML0011I (179)2.21.12 XML0012I (179)2.21.13 XML0013E (179)2.21.14 XML0014E (179)2.21.15 XML0015E (179)2.21.16 XML0016E (179)2.21.17 XML0017I (179)2.21.18 XML0018I (180)2.21.19 XML0019E (180)2.21.20 XML0020E (180)3 Abend Codes (181)3.1 ABEND Code U2222 (181)3.1.1 Reason Code 1 (181)3.2 Language Environment Abends (181)4 Troubleshooting (182)4.1 Holder (182)4.1.1 MAINTASK (182)4.1.2 SYSTSPRINT (182)4.1.3 DSPPRT (182)4.2 Scheduler (182)4.2.1 MAINTASK (183)4.2.2 CMDTASK (183)4.2.3 SRVTASK (183)4.2.4 T000000x (183)4.2.5 SYSTSPRT (184)4.2.6 ZCOTSPRT (184)4.3 User Server (184)4.3.1 MAINTASK (184)4.3.2 CMDTASK (184)4.3.3 SRVTASK (185)4.3.4 T0000001 (185)4.3.5 SYSTSPRT (185)4.3.6 ZCOTSPRT (185)4.3.7 SYSPRINT (185)4.3.8 ISPLOG (185)4.4 Trace Level (185)4.5 Required Information for Customer Support (186)1 IntroductionThis document provides a description of messages issued by z/Server including possible corrective actions. Every message has an 8-character identification and an associated message text.Troubleshooting often requires knowledge of configuration settings, such as the name of the holder task, the scheduler task, and the user server task(s). Please see the z/Server Installation Guide for details about these settings. Most messages only appear in the JESx job log in different DD names and not in the z/OS hardcopy log. True hardcopy log messages are marked.1.1 Message formatThe message identification has the form: pfxnnnns, where:pfx The z/Server component prefix (facility ID)The following facility IDs exist:nnnn The message number for the component, for example, 0051. The message numbers are unique within a component.s The message severity:I InformationW WarningE ErrorS Severe errorInformational messages are normally used for tracing or debugging purposes. Errors apply to the associated service and are typically caused by incorrect arguments. Severe errors may require z/Server to be restarted.The message description provides information to identify the cause of the problem. Many messages contain placeholders ([mmm], where mmm is a consecutive number; for example, [002] is the second placeholder). These placeholders are replaced with specific values in the actual message.The message description may contain messages, return and reason codes, etc. passed from invoked services (for example, the XML system parser or the security product). In these cases, the IBM manual to be consulted for details of the problem are given.With very few exceptions, the first placeholder ([001]) of each message is the timestamp when the message was issued (hh:mm:ss.ttt):hh hourmm minutess secondsttt millisecondsThe explanation for any message describes if the message is only issued at a certain IPTRACE level. This documented trace level always implies that the message is issued at this trace level and up (since a higher trace level includes all lower trace levels).1.2 Error message exampleError messages have severity E or S. There are three general classes of error:∙Errors caused by incorrect user input, such as incorrect password (SLR0071E) or filter did not find any data sets (CSI0051E). Such errors can be rectified by correcting the input and retrying the action. Some of these error messages can only occur due to incorrect input to the set of z/Server APIs, typically from an eclipse client.∙Errors that indicate problems in the configuration file, such as EMC0011E (port is not available). Such errors may be corrected by changing the configuration (a task for the system administrator), although they can also indicate some other error that needs to be investigated (also a task for the system administrator).Errors that indicate an internal z/Server error, such as API0007E (error during call to EZASOKET). If the message information provided does not help to identify the problem, please contact the support hotline.As an example, the CSI0051E message is issued by the CSI facility (CSI prefix) and has a severity of E (=error).The message is shown as follows in this book:CSI0051E [001] No data sets for filter [002] foundThis message has two placeholders: [001] and [002]. The placeholders are replaced with the timestamp and the associated filter. An actual CSI0051E error message would look like this:CSI0051E 07:48:53.935 No data sets for filter SYS2 foundThe two placeholders have been replaced with the time (07:48) and the filter (SYS2) that could not be resolved. In this case, using a filter that covers available data sets would prevent the error message.1.3 Warning message exampleWarning messages have severity W. Such messages are normally written to the associated JESx DD name, see below. Warnings normally indicate that some standard action has been taken. If this action or default is not required or wanted, change the appropriate setting.TAU0019W 09:14:05.443 Environment-Variable REATTACH was not specified, using defaults !TAU0019W 09:14:05.651 Environment-Variable CCSID was not specified, using defaults !TAU0019W 09:14:05.652 Environment-Variable TSOE_JOB_CLASS was not specified, using defaults !TAU0019W 09:14:05.652 Environment-Variable TSOE_SCHED_TAB was not specified, using defaults !LIC0007W 09:14:05.659 This server may only be used through a licensed client.1.4 Information message exampleInformation messages have severity I. Such messages are normally written to the associated JESx DD name, see below.SLR0111I 09:14:05.998 TSO/E-Scheduler Init/Term-Routine called with function STARTSLR0001I 09:14:06.170 TSO-Scheduler initializationHLD0030I 09:14:06.188 User administration ended with RC 00000000 (hex)1.5 Messages and GTFFor debugging purposes, it is recommended to start GTF and trace user records x'3E8'. In such a case, there will be significantly less messages written to the JESx spool. Most messages will be written to GTF only, regardless of the IPTRACE level being set. It may get difficult to even follow what happens withinz/Server using just the remaining messages to hardcopy log and JESx job log.2 Messages2.1 API - Application Programming InterfaceAll API messages are issued b y TAURIP03. It is used as an interface for all TCPIP communication. Standard return codes are described in the table below. This interface is called using z/Server API calls, both internally and externally.2.1.1 API0001IAPI0001I hh:mm:ss.ttt Before call IP03 starting call in [002] environmentFunction-Code : [003]Task-Area : [004] [005]Explanation:[002] is either server or client.[003] is described in SC31-8788 z/OS Communications Server IP Sockets Application Programming Interface Guide and Reference.[004] is the address of the task area.[005] is global (if [002] is server), otherwise local (if [002] is client)User response: None.Issued by: TAURIP032.1.2 API0002IAPI0002I hh:mm:ss.ttt After Call IP03 ending call. Rc is : [002] Explanation: This is an informational message due to IPTRACE level 4 being set.User response: None.Issued by: TAURIP032.1.3 API0007EAPI0007E hh:mm:ss.ttt Error during call to EZASOKETFunction : [002]Reason-Code : [003]Explanation: This message is issued when IPTRACE level 4 is set. See SC31-8788 z/OS Communications Server IP Sockets Application Programming Interface Guide and Reference for details about the function used and the returned error.System administrator response: Determine why the error occurred. If necessary, contact customer support.Issued by: TAURIP032.1.4 API0008IAPI0008I hh:mm:ss.ttt Timeout for SELECT is [002] seconds and [003] microseconds.Explanation: This is an informational message due to IPTRACE level 4 being set.User response: None.Issued by: TAURIP032.1.5 API0009EAPI0009E hh:mm:ss.ttt For function PeekMsg the sixth Parameter must be 'P'. Explanation: This message is issued in response to an API call.User response: Correct the calling program.Issued by: TAURIP032.1.6 API0010IAPI0010I hh:mm:ss.ttt Using static z/Server environment for client processing at address [002]Explanation: This is an informational message due to IPTRACE level 4 being set.User response: None.Issued by: TAURIP03。
discovery studio libdock打分 -回复
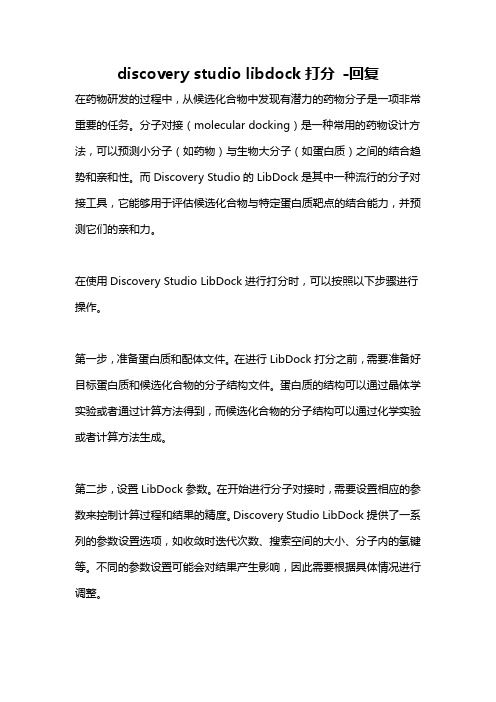
discovery studio libdock打分-回复在药物研发的过程中,从候选化合物中发现有潜力的药物分子是一项非常重要的任务。
分子对接(molecular docking)是一种常用的药物设计方法,可以预测小分子(如药物)与生物大分子(如蛋白质)之间的结合趋势和亲和性。
而Discovery Studio的LibDock是其中一种流行的分子对接工具,它能够用于评估候选化合物与特定蛋白质靶点的结合能力,并预测它们的亲和力。
在使用Discovery Studio LibDock进行打分时,可以按照以下步骤进行操作。
第一步,准备蛋白质和配体文件。
在进行LibDock打分之前,需要准备好目标蛋白质和候选化合物的分子结构文件。
蛋白质的结构可以通过晶体学实验或者通过计算方法得到,而候选化合物的分子结构可以通过化学实验或者计算方法生成。
第二步,设置LibDock参数。
在开始进行分子对接时,需要设置相应的参数来控制计算过程和结果的精度。
Discovery Studio LibDock提供了一系列的参数设置选项,如收敛时迭代次数、搜索空间的大小、分子内的氢键等。
不同的参数设置可能会对结果产生影响,因此需要根据具体情况进行调整。
第三步,运行LibDock分子对接。
当参数设置好之后,可以运行LibDock 分子对接来评估候选化合物与蛋白质之间的亲和性。
LibDock会将候选化合物在蛋白质上进行位置和取向的搜索,并给出每个候选化合物的打分。
较高的分数表示较好的结合亲和性。
第四步,分析和解释结果。
分析和解释LibDock分子对接的结果是非常关键的一步。
可以通过分子对接得分、结合位点的残基相互作用和空间构型等来评估候选化合物与蛋白质之间的结合趋势和亲和力。
根据这些信息,可以选择潜在的药物分子进行进一步的验证和优化。
总结起来,Discovery Studio LibDock是一种常用的分子对接工具,能够用于评估候选化合物与特定蛋白质靶点的结合能力,并预测它们的亲和力。
深研生物 cellsep pro 参数 -回复

深研生物cellsep pro 参数-回复深研生物是一家专注于生物科技研发的公司,其CellSep Pro是一款先进的细胞分离系统。
本文将详细介绍CellSep Pro的参数和特点,并逐步回答有关该系统的问题。
第一部分:CellSep Pro简介CellSep Pro是深研生物推出的一款高效、精确的细胞分离系统。
它基于先进的显微镜成像技术和智能分析算法,能够实现对复杂样本中不同类型细胞的快速分离和准确鉴定。
该系统广泛应用于生命科学研究、临床诊断和药物开发等领域。
第二部分:CellSep Pro的参数1. 分辨率:CellSep Pro的高分辨率显微镜可提供清晰、高质量的图像,使用户能够准确地分辨不同类型的细胞。
2. 自动化程度:该系统具有高度自动化的特点,能够快速而准确地完成细胞分离和分析过程。
3. 处理容量:CellSep Pro可同时处理多个样本,提高实验效率。
4. 分选效率:该系统采用先进的分选算法,能够高效地分离目标细胞,并确保其纯度和活力。
5. 多样本类型适应性:CellSep Pro适用于各种复杂样本,包括血液、组织和体液等。
第三部分:CellSep Pro的特点1. 高度精确的细胞分离:CellSep Pro采用先进的显微镜成像技术和智能分析算法,能够准确地识别和分离目标细胞。
2. 高通量分离:该系统具有高度自动化的特点,可同时处理多个样本,大大提高实验效率。
3. 可编程性:CellSep Pro具有多种可调节的参数,如分选速度和灵敏度,用户可以根据实际需要进行个性化设置。
4. 可视化操作界面:该系统配备了直观、易操作的界面,使用户能够直观地监控和控制分离过程。
5. 数据分析和存储功能:CellSep Pro可实时分析和存储分离过程中的关键数据,方便用户进行后续数据处理和分析。
6. 灵活的样本处理:该系统支持多种样本处理方法,如细胞悬液、细胞板、载物片等,满足不同实验需求。
第四部分:CellSep Pro的应用案例1. 癌症研究:CellSep Pro可快速分离和鉴定癌细胞,为癌症研究提供有力的工具。
deep-did法 -回复
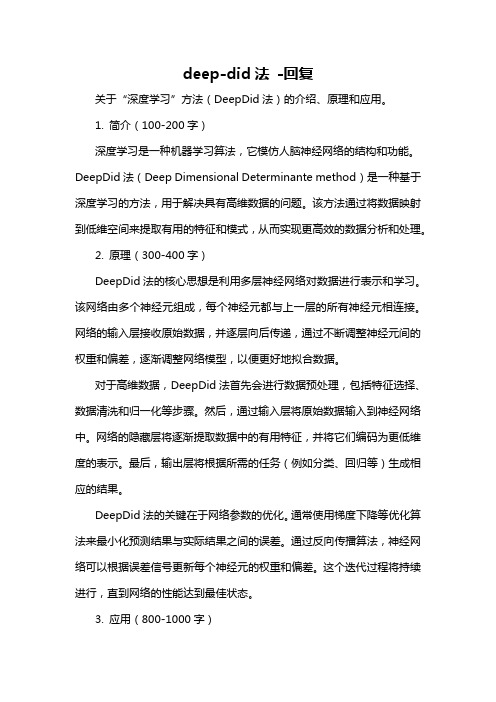
deep-did法-回复关于“深度学习”方法(DeepDid法)的介绍、原理和应用。
1. 简介(100-200字)深度学习是一种机器学习算法,它模仿人脑神经网络的结构和功能。
DeepDid法(Deep Dimensional Determinante method)是一种基于深度学习的方法,用于解决具有高维数据的问题。
该方法通过将数据映射到低维空间来提取有用的特征和模式,从而实现更高效的数据分析和处理。
2. 原理(300-400字)DeepDid法的核心思想是利用多层神经网络对数据进行表示和学习。
该网络由多个神经元组成,每个神经元都与上一层的所有神经元相连接。
网络的输入层接收原始数据,并逐层向后传递,通过不断调整神经元间的权重和偏差,逐渐调整网络模型,以便更好地拟合数据。
对于高维数据,DeepDid法首先会进行数据预处理,包括特征选择、数据清洗和归一化等步骤。
然后,通过输入层将原始数据输入到神经网络中。
网络的隐藏层将逐渐提取数据中的有用特征,并将它们编码为更低维度的表示。
最后,输出层将根据所需的任务(例如分类、回归等)生成相应的结果。
DeepDid法的关键在于网络参数的优化。
通常使用梯度下降等优化算法来最小化预测结果与实际结果之间的误差。
通过反向传播算法,神经网络可以根据误差信号更新每个神经元的权重和偏差。
这个迭代过程将持续进行,直到网络的性能达到最佳状态。
3. 应用(800-1000字)DeepDid法在各个领域都有广泛的应用。
下面我们将介绍几个例子,以展示其在不同问题上的强大能力。
3.1 图像处理在图像处理领域,DeepDid法已经取得了显著的成果。
通过训练神经网络,可以实现图像分类、目标检测、人脸识别等任务。
例如,通过使用适当的数据集来训练神经网络,可以对图像进行分类,比如判断一个图像是猫还是狗。
此外,DeepDid法还可以生成逼真的图像,如风景、肖像等,并用于图像增强和修复。
3.2 语音识别DeepDid法在语音识别领域也有成功的应用。
dperf使用方法(一)
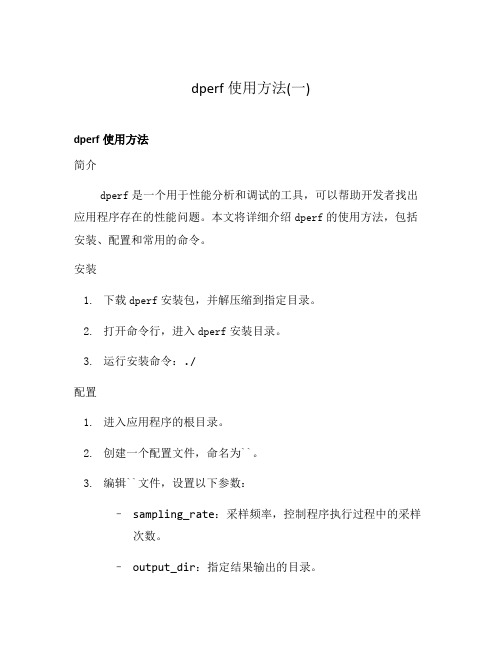
dperf使用方法(一)dperf使用方法简介dperf是一个用于性能分析和调试的工具,可以帮助开发者找出应用程序存在的性能问题。
本文将详细介绍dperf的使用方法,包括安装、配置和常用的命令。
安装1.下载dperf安装包,并解压缩到指定目录。
2.打开命令行,进入dperf安装目录。
3.运行安装命令:./配置1.进入应用程序的根目录。
2.创建一个配置文件,命名为``。
3.编辑``文件,设置以下参数:–sampling_rate:采样频率,控制程序执行过程中的采样次数。
–output_dir:指定结果输出的目录。
–log_file:指定日志文件路径。
–include_path:指定需要检查的代码文件路径。
–exclude_path:指定不需要检查的代码文件路径。
命令下面是一些常用的dperf命令:1.dperf start:开始性能分析。
2.dperf stop:停止性能分析。
3.dperf report:生成性能报告。
4.dperf config:修改配置文件。
5.dperf clear:清除之前的性能数据。
示例以下是一个使用dperf进行性能分析的示例:1.在终端中进入应用程序的根目录。
2.运行命令dperf start开始性能分析。
3.进行一段时间的应用程序操作。
4.运行命令dperf stop停止性能分析。
5.运行命令dperf report生成性能报告。
6.打开报告文件,查看性能分析结果。
结论通过dperf工具,开发者可以方便地进行性能分析和调试,找出应用程序存在的性能问题。
掌握dperf的使用方法,有助于提高应用程序的性能和效率。
以上是dperf的使用方法的详细介绍。
希望本文能够帮助读者更好地使用dperf进行性能分析和调试。
deepsort 拓展卡尔曼滤波 -回复

deepsort 拓展卡尔曼滤波-回复deepsort是一种优秀的多目标跟踪算法,它能够在摄像头视频中同时跟踪多个目标。
在deepsort算法中,卡尔曼滤波(或者拓展卡尔曼滤波)是一个重要的组成部分,它用于预测和修正多目标的位置和速度信息。
那么,在本文中,我们将详细介绍deepsort算法中的拓展卡尔曼滤波,并分步回答以下问题:1. 什么是拓展卡尔曼滤波(EKF)?2. 在deepsort中为什么需要使用拓展卡尔曼滤波?3. 拓展卡尔曼滤波在deepsort中是如何工作的?4. 拓展卡尔曼滤波的优点和局限性是什么?让我们逐步回答这些问题。
1. 什么是拓展卡尔曼滤波(EKF)?拓展卡尔曼滤波是一种卡尔曼滤波器的变种,用于估计非线性系统的状态。
在标准卡尔曼滤波器中,系统和观测模型都被假设为线性的,而拓展卡尔曼滤波则允许非线性模型的应用。
EKF通过以高斯分布来近似非线性函数生成的状态分布,从理论上可以达到与线性卡尔曼滤波器相同的性能。
2. 在deepsort中为什么需要使用拓展卡尔曼滤波?在deepsort中,我们需要对多目标的位置和速度进行估计。
然而,视频中的目标通常经历非线性的运动轨迹,因此传统的线性卡尔曼滤波器无法很好地适应这种情况。
这时,拓展卡尔曼滤波器就派上了用场,它可以对非线性的目标运动进行建模和估计。
3. 拓展卡尔曼滤波在deepsort中是如何工作的?在deepsort中,拓展卡尔曼滤波器用于预测和修正多目标的位置和速度。
首先,我们需要建立一个非线性的状态转移模型和观测模型,用于描述目标的运动特征。
这些模型的选择需要根据具体的问题和场景进行调整。
假设我们的目标状态为x,系统的状态更新可以通过以下步骤进行:- 预测步骤:根据上一时刻的目标状态和模型,利用非线性状态转移方程进行状态预测。
这一步骤主要是为了预测目标的位置和速度。
- 修正步骤:根据当前观测到的目标位置信息和模型,通过拓展卡尔曼滤波的修正步骤,更新目标的状态分布。
一场举重比赛作文英语

I remember vividly the day I competed in a weightlifting competition it was a pivotal moment in my life, a day that tested my strength, both physical and mental. The atmosphere was electric, filled with the clanging of weights, the cheers of the crowd, and the palpable tension of athletes poised to push their limits.I had been training for months, waking up before the sun to hit the gym, lifting weights that seemed to grow heavier with each passing week. My coach, a stern man with a voice that echoed through the gym, had pushed me to my limits, always demanding more. But it was my own determination that drove me to keep going, to lift just one more rep, to push through the pain that seared through my muscles.The day of the competition, I woke up with a mix of excitement and nervousness. My hands were sweaty, my heart pounded in my chest as I walked into the venue. The gym was transformed into a battleground, with banners hanging from the ceiling, spotlights illuminating the stage, and the smell of chalk and determination in the air.As I stepped onto the stage, the crowd roared. The judges, with their stern faces and clipboards, scrutinized every movement. I took a deep breath, feeling the weight of expectation on my shoulders. My first lift was a personal record, a testament to the countless hours I had spent in the gym. The barbell felt heavy, but my resolve was stronger. With a grunt, I lifted it above my head, the weight suspended in the air for what felt like an eternity before I lowered it back to the cradle.The crowd erupted in applause, but my focus was on the next lift. The weight was increased, and the pressure mounted. I could feel my muscles trembling, my body screaming for rest, but I knew I couldnt give in. I visualized the lift, the perfect form, the triumphant moment when I would raise the bar above my head.As I lifted the bar, my world narrowed to the weight in my hands. I felt a surge of power, a rush of adrenaline that fueled my muscles. But just as I was about to complete the lift, I faltered. The weight was too much, my strength waning. I tried to push through, but the bar slipped from my grip, crashing to the floor with a deafening clang.The disappointment was crushing. I had come so far, trained so hard, only to fall short in the moment of truth. But as I walked off the stage, the crowds applause was a reminder that I had given it my all. My coach, with a look of pride in his eyes, patted me on the back. He told me that it wasnt about winning or losing, but about the courage to compete, the strength to push beyond my limits.That day, I learned more than just about weightlifting. I learned about resilience, about the power of determination, and the importance of striving for excellence, even in the face of defeat. The competition was more than just a test of my physical strength it was a journey of selfdiscovery, a testament to the indomitable spirit that resides within each of us.Looking back, I realize that the weightlifting competition was a metaphorfor life itself. We all face challenges, obstacles that seem insurmountable. But its not about the weight we lift or the records we break its about the courage to face those challenges headon, to push ourselves to be better, to never give up, no matter the odds.So, as I continue my journey, whether its in the gym or in life, I carry with me the lessons of that competition. I remember the feeling of lifting that bar, the rush of adrenaline, the taste of victory, and the sting of defeat. And I know that no matter what challenges I face, I have the strength to lift them, to rise above them, and to emerge stronger on the other side.。
基于深度学习的恶意PE文件检测系统

基于深度学习的恶意PE文件检测系统随着互联网的快速发展,网络安全问题已经成为人们关注的焦点之一。
恶意PE文件作为一种常见的网络攻击工具,对用户敏感数据的泄露和系统稳定性造成了严重威胁。
因此,研发一种高效准确的恶意PE文件检测系统变得尤为重要。
本文将介绍一种基于深度学习的恶意PE文件检测系统,并分析其优势和应用前景。
一、引言恶意PE文件是指恶意程序被嵌入到可执行文件中,以实现对系统的攻击和入侵。
常见的恶意PE文件包括病毒、木马、僵尸网络等。
传统的恶意PE文件检测方法主要依赖于特征工程和规则定义,但由于恶意PE文件的复杂性和变异性,这些方法往往难以适应多变的网络攻击。
基于深度学习的恶意PE文件检测系统因其能够从海量数据中学习特征和规律,逐渐成为研究热点。
二、基于深度学习的恶意PE文件检测系统基于深度学习的恶意PE文件检测系统的核心技术是卷积神经网络(CNN)和递归神经网络(RNN)。
CNN可以有效提取PE文件的结构和特征信息,帮助系统分辨正常文件和恶意文件。
RNN则可以捕捉PE文件中的序列信息,包括指令序列、API调用序列等,实现对文件行为的分析和判断。
该系统的工作流程如下:1. 数据准备:从恶意PE文件和正常PE文件中提取样本数据,并进行预处理,包括文件解析、特征提取等。
2. 数据标记:对样本数据进行标记,将恶意PE文件标记为1,正常PE文件标记为0。
3. 模型训练:使用标记好的样本数据训练深度学习模型,包括CNN和RNN模型。
4. 模型测试:使用测试数据对训练好的模型进行测试和评估,计算准确率、召回率等指标。
5. 恶意PE文件检测:将待检测的PE文件输入训练好的模型,判断其是否为恶意文件。
具体实现过程中,可以使用开源深度学习框架如TensorFlow或PyTorch来构建模型,并使用大规模的PE文件数据集来训练和评估模型。
同时,可以结合GPU加速技术来提高系统的检测性能和效率。
三、优势和应用前景相比传统的恶意PE文件检测方法,基于深度学习的恶意PE文件检测系统具有以下优势:1. 自动学习特征:深度学习模型能够从大量数据中自动学习特征,不需要依赖人工定义的规则和特征工程。
深度发现检测器 (Deep Discovery Inspector) 数据表说明书
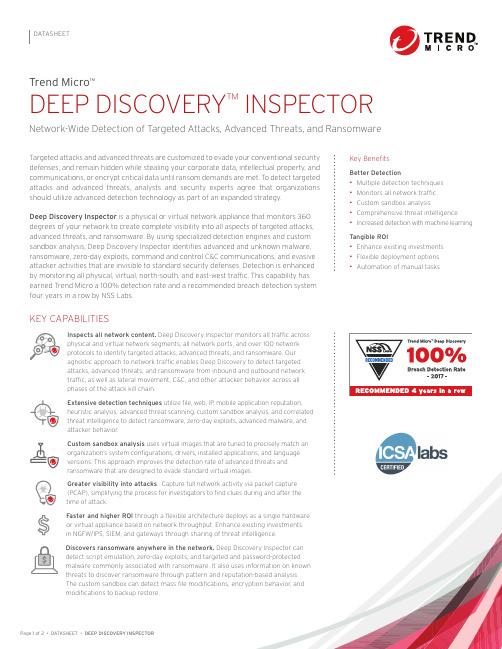
A KEY PART OF TREND MICRO CONNECTED THREAT DEFENSE
To adequately protect against the current threat landscape, you need a multi-layered protection platform that delivers the full lifecycle of threat defense. Trend Micro Connected Threat Defense is a layered approach to security that gives your organization a better way to quickly prevent, detect, and respond to new threats that are targeting you, while improving visibility and control across your network. •• Prevent: Assess potential vulnerabilities and proactively protect endpoints, servers,
Custom sandbox analysis uses virtual images that are tuned to precisely match an organization’s system configurations, drivers, installed applications, and language versions. This approach improves the detection rate of advanced threats and ransomware that are designed to evade standard virtual images.
deep-did法
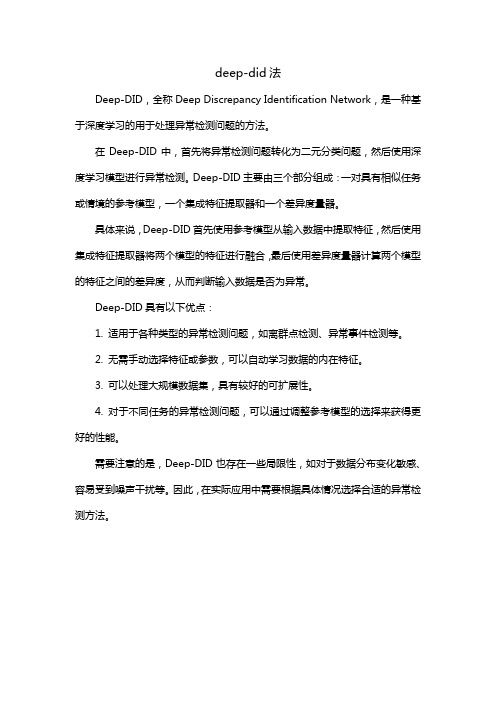
deep-did法
Deep-DID,全称Deep Discrepancy Identification Network,是一种基于深度学习的用于处理异常检测问题的方法。
在Deep-DID中,首先将异常检测问题转化为二元分类问题,然后使用深度学习模型进行异常检测。
Deep-DID主要由三个部分组成:一对具有相似任务或情境的参考模型,一个集成特征提取器和一个差异度量器。
具体来说,Deep-DID首先使用参考模型从输入数据中提取特征,然后使用集成特征提取器将两个模型的特征进行融合,最后使用差异度量器计算两个模型的特征之间的差异度,从而判断输入数据是否为异常。
Deep-DID具有以下优点:
1. 适用于各种类型的异常检测问题,如离群点检测、异常事件检测等。
2. 无需手动选择特征或参数,可以自动学习数据的内在特征。
3. 可以处理大规模数据集,具有较好的可扩展性。
4. 对于不同任务的异常检测问题,可以通过调整参考模型的选择来获得更好的性能。
需要注意的是,Deep-DID也存在一些局限性,如对于数据分布变化敏感、容易受到噪声干扰等。
因此,在实际应用中需要根据具体情况选择合适的异常检测方法。
- 1、下载文档前请自行甄别文档内容的完整性,平台不提供额外的编辑、内容补充、找答案等附加服务。
- 2、"仅部分预览"的文档,不可在线预览部分如存在完整性等问题,可反馈申请退款(可完整预览的文档不适用该条件!)。
- 3、如文档侵犯您的权益,请联系客服反馈,我们会尽快为您处理(人工客服工作时间:9:00-18:30)。
Deep Discovery3.0Performance Test ReportPerformance Tuning PlanContents1.OBJECTIVES (1)1.1Introduction (1)1.2Objectives (1)2.TEST RESULT (2)2.1Overall Throughput while enable and disable DTAS (2)2.1.1Result from R710 (2)2.1.2Result from R410 (2)2.2Internal TMSP Performance (3)2.2.1Execution Time (3)2.2.2System resource usage (3)2.3Web Console Response Time (4)3.TESTING METHODOLOGY (5)3.1Test Environment and Configuration (5)3.1.1DUT (5)3.1.2Product Configuration (5)3.2Testing Methodology (6)3.3Test Kits (6)3.3.1Tools (6)3.3.2Test profile/sample definition (7)3.4Performance metrics (7)3.4.1System Resource Related Usage (8)3.4.2Product Related Metrics (8)4.ISSUES (9)1.Objectives1.1IntroductionDeep Discovery 3.0 provides the enhancements for user experience on admin console and some new features, such as refining the design of Fstream_serv daemon, applying postgresql database to extend the time of log storage, generating threat correlation report and integrating with the Sandcastle. High performance is always a key index of Deep Discovery. But the new added and enhanced features may degrade or upgrade the performance. So we will test on Deep Discovery 3.0 to figure out its real performance.1.2ObjectivesBelow are the performance objectives of Deep Discovery 3.0.1.Overall system maximum throughput under following situation.a)Enable DTASb)Disable DTAS2.Internal TMSP performance involving correlation, summary and report3.For query operations on the admin consolea)All the widgets on Dashboard should be shown out no longer than 15 seconds.b)Query out the first page in log query shall be within 30 secondsc)All other UI pages should be opened within 5 secondsPerformance Tuning Plan2.Test ResultBelow is the result summary based on our test data.2.1Overall Throughput while enable and disable DTAS2.1.1Result from R7102.1.2Result from R410Note:After investigating the real customer data, the rate of threat file generation is about 0.2/secunder 500Mbps. In our test, we generated the threat file in the rate of 1/sec, 5/sec and 10/secrespectively and the backend traffic is secure.Conclusions:Based on the result above, we can see that:1.For the Maximum throughput of each scenario, there’s not drop if enable DTAS at all.2.The range of the reliable throughput on R710 would be 1Gbps~1.28Gbps, on R410 would be400Mbps~500MbpsThreat Discovery Appliance 2.6 SP12.2Internal TMSP Performance2.2.1Execution Time2.2.2System resource usageConclusions:1.Correlation spends the most of the time, but the total time usage of three daily tasks is not muchmore.2.While the tasks was running, they don’t consume much system resource3.Consider that internal TMSP tasks only start up at midnight, they won’t impact the overallperformance of Deep Discovery.Performance Tuning Plan2.3Web Console Response TimeConclusions:1.All of the widget can be shown out within 15 second based on mass of data in database.2.The time to query out the first page of 30 days raw logs spent a little bit more time but still within ourexpected.Threat Discovery Appliance 2.6 SP13.Testing MethodologyIn this section, we will describe the test scenario that will be covered, and major testing methodology that will be used during the test, including test technology, tools, strategy, samples and metrics.3.1Test Environment and ConfigurationBelow are the general test environment that will be used for our performance testing.3.1.1DUT1. HW Platform:Dell R410Four Intel(R) Xeon(R) CPU E5504 @ 2.00GHz8GB memoryHard disk: 320G2 * Gbps NICs (2 data ports)Dell R710Eight Intel® Xeon® CPU,E5530@2.4GHz8GB memory,Hard disk: 250G8 * Gbps NICs (4 data ports)2. NetworkLAN, 1000Mbps network environmentSwitch: 2 * Cisco 35603.1.2Product ConfigurationBelow is the product configuration.Testing build: 1266ComponentsPerformance Tuning Plan3.2Testing MethodologyFollowing chart is the test topology.Avalanche, acts as a client and a server, can simulate to send out the HTTP traffic. All of the traffic could be mirrored to Deep Discovery for scan. Monitor PC was used to monitor the Deep Discovery resource usage and collect the statistics data after testing.3.3Test KitsBelow are the test tools and test samples that will be used for performance test.3.3.1ToolsTable 3-1 Test ToolsThreat Discovery Appliance 2.6 SP13.3.2Test profile/sample definition∙For Overall Throughput while enable/disable DTAS•Protocol: HTTP 1.1 (persistence) / HTTP 1.0 (non-persistence)•Concurrent Connection: 10k•Sample set:∙For Internal TMSP Performance•Raw data baseline–CAV log: 1000000 records/day * 2 * 30–DAE log: 1000000 records/day * 2 * 30–TMUFE log: 300000 records/day * 2 * 30∙For Web Console Response Time•Data baseline:–CAV log : 9,000,000 (300,000 * 30 days)–DAE log : 9,000,000 (300,000 * 30 days)–TMUFE log : 3,000,000 (100,000 * 30 days)–Aggregated log : 127953 (30 days)–Aggr logs for Geomap (total): 28455•Note:These raw data was exported from a real customer.3.4Performance metricsBesides the performance index, we need to collect more data during test to do performance analysis and check if the result is normal.Performance Tuning Plan3.4.1System Resource Related Usage♦System Level⏹Average CPU usage⏹Memory usage⏹Packet loss⏹Overall Bandwidth3.4.2Product Related Metrics♦Filescan daemon⏹Average CPU usage⏹Average Memory usage♦Fstream_serv daemon⏹Average CPU usage⏹Average Memory usage♦cav log filter (cavd)⏹Average CPU usage⏹Average Memory usage♦tmsyslogd⏹Average CPU usage⏹Average Memory usage⏹I/O estimation (database, /var/log)♦Web console⏹Response TimeThreat Discovery Appliance 2.6 SP14.IssuesN/AProprietary and Secret March 29, 2011 Page 9。