Super-resolution Restoration of Remote-sensing Images
超分辨率成像技术的实现及应用
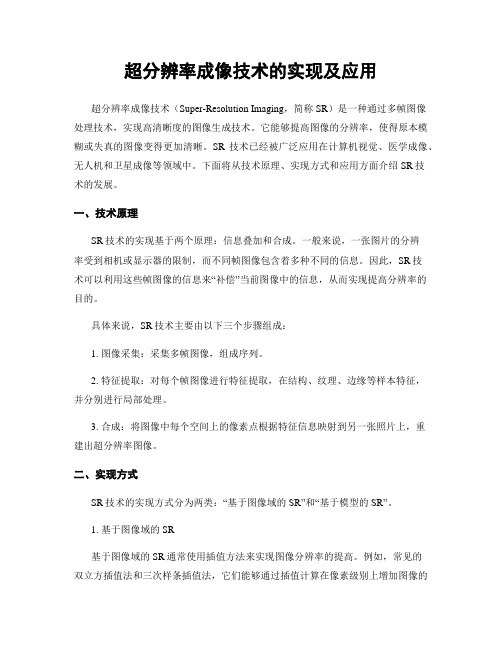
超分辨率成像技术的实现及应用超分辨率成像技术(Super-Resolution Imaging,简称SR)是一种通过多帧图像处理技术,实现高清晰度的图像生成技术。
它能够提高图像的分辨率,使得原本模糊或失真的图像变得更加清晰。
SR技术已经被广泛应用在计算机视觉、医学成像、无人机和卫星成像等领域中。
下面将从技术原理、实现方式和应用方面介绍SR技术的发展。
一、技术原理SR技术的实现基于两个原理:信息叠加和合成。
一般来说,一张图片的分辨率受到相机或显示器的限制,而不同帧图像包含着多种不同的信息。
因此,SR技术可以利用这些帧图像的信息来“补偿”当前图像中的信息,从而实现提高分辨率的目的。
具体来说,SR技术主要由以下三个步骤组成:1. 图像采集:采集多帧图像,组成序列。
2. 特征提取:对每个帧图像进行特征提取,在结构、纹理、边缘等样本特征,并分别进行局部处理。
3. 合成:将图像中每个空间上的像素点根据特征信息映射到另一张照片上,重建出超分辨率图像。
二、实现方式SR技术的实现方式分为两类:“基于图像域的SR”和“基于模型的SR”。
1. 基于图像域的SR基于图像域的SR通常使用插值方法来实现图像分辨率的提高。
例如,常见的双立方插值法和三次样条插值法,它们能够通过插值计算在像素级别上增加图像的分辨率。
此外,更复杂和更高级的方法还包括基于低分辨率图像的遥感SR (Remote Sensing SR)、基于低分辨率图像的视频SR(Video SR),基于组合的SR算法等。
2. 基于模型的SR基于模型的SR主要是使用一些概率模型或神经网络模型,对图像重建进行优化。
模型被训练成为一个图像重建器,使其能够识别模糊和失真的部分,并对其进行恢复。
此类方法的复杂性较高,但是它们能够从低分辨率信息和环境的约束条件中推断出高分辨率图像的可能性。
三、应用方面SR技术的应用十分广泛,其中最常见的包括计算机视觉、医学成像、卫星成像和无人机。
大学生创新创业训练计划项目申请书大创项目申报表

大学生创新创业训练计划项目申请书大创项目申报表附件1国家级大学生创新创业训练计划项目申报表项目名称:LED水下成像探测系统的图像恢复技术研究项目类型:√□创新训练项目□创业训练项目□创业实践项目所属一级学科名称:电子与通信技术项目负责人:联系电话:指导教师:联系电话:申报日期:2014年6月25日二○一四年六月项目名称LED水下成像探测系统的图像恢复技术研究项目所属一级学科510电子与通信技术项目类型(√)创新训练项目()创业训练项目()创业实践项目项目实施时间起始时间:2014年9月完成时间:2015年12月申请人或申请团队姓名年级学号所在院系/专业联系电话E-mail 主持人成员指导教师第一指导教师姓名单位计信学院年龄29 专业技术职务讲师主要成果第一作者发表SCI论文7篇,EI 1 篇,中文核心1篇:列出其中4篇如下[1] Y uzhang Chen, Wei Li*, Min Xia, Kecheng Yang,Super-resolution reconstruction for underwater imaging, OpticaApplicata, 41(4), pp841~853, 2011 SCI收录[2] Yuzhang Chen*, Kecheng Yang, Edge RegularizedRestoration for Laser Bubble Detection, Journal of RussianLaser Research, 33(5), pp475-482, 2012 SCI收录[3]Yuzhang Chen*,Kecheng Yang, MAP-regularized robustreconstruction for underwater imaging detection, OPTIK,124 (2013) 4514– 4518, 2013 SCI收录[4]Yuzhang Chen*, Kecheng Yang, Spatial adaptiveregularized MAP reconstruction for LD-based night vision,OPTIK, 10.1016/j.ijleo.2014.01.033, 2014 SCI收录一、申请理由随着海洋信息处理的理论与技术的研究备受人们关注,水下成像技术被广泛应用于水下目标检测、海洋地理工程勘察及海洋军事等领域,同时水下成像技术的应用也不断深化。
ALOS_PRISM遥感影像超分辨率重建
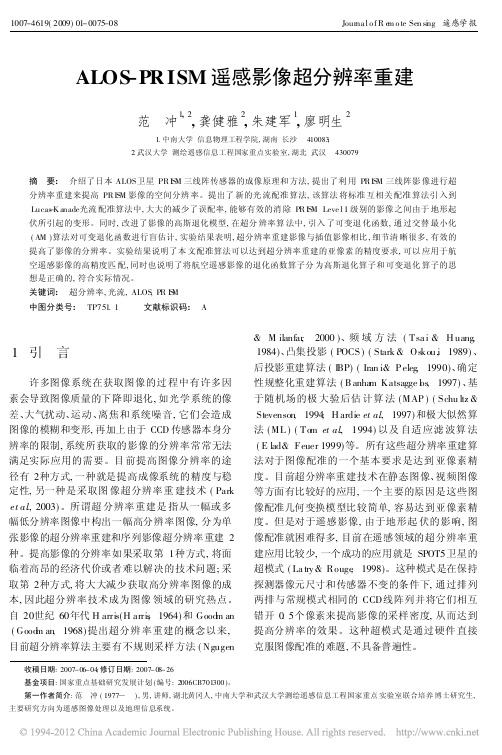
E
2
Cg i (hj ) - C gj (h i ) ( 16)
3
PR IS M 遥感影像亚像素配准算法
式中, Cg i ( hj ) = DH j g i。 21 3 AM 迭代算法 为 了 获 取 更 优 的 解, Sroubek ( Sroubek & F lu sser , 2003 ; 2006 ; 2007) 改进了超分辨率重建数 T 学模型式 ( 6 ), 在其中加入了 Q ( h ) = h Lh 约束项 , 于是式 ( 6 )变为: E ( f, h ) =
21 1 图像规则化项目 Q (f ) 对于超分辨率重建 , 规 整化项目 Q ( f ) 常常 采 用 T ikhonov 规整化, 即 Q ( f) = ¨f Q
2 T
= f Lf
( 7)
式中, L 是高通滤波算子。 T ikhonov 规整化的基本 想法是限制解为一个平滑解 , 实际的超分辨率重建 中 , 解的平滑常常是不符合实 际情况的, 因为 实际 图像总有许多棱边和点构成的细节 , 损失这些细节 意味 损 失 信 息。为 了 克 服 这 些 问 题 , 必 须 拓 广 T ikhonov 规整化的概念, 强调解必须在物理上合理 并且连续的依赖。这种概念不再强调数据的 平滑 性限制 , 而是引入其他符合物理事实的限制。比较 典型的限制就是总变分最小化, 采用总变分最小化 的规整化形式类似于式 ( 7) : Q ( f) = ¨f Q = f Lf
K
( 8)
式中 , L 是高通滤波算子。 21 2 点退化函数规则化项目 R ( h ) H arikum ar等 人在 多 通道 图像 恢 复算 法 中 提 出 : 根据子空间 理论 , 如果 各通 道的 质 , 则 hk的解满足如下方程: g i @ hj - gj @ h i = 0 , 1[ i< j [ K 有式 ( 9) 的点扩散函数写成一个公式 : N h= 0 ( 10 ) 式中, N 是一个矩阵 , 其形式由式 ( 11 )、 式 ( 12 ) 和式 ( 13 )给出: SK- 1 S (C gK - C gK - 1 ) C g t+ 1 C g Cg t+ 2 St S
超分辨率成像技术的原理与应用
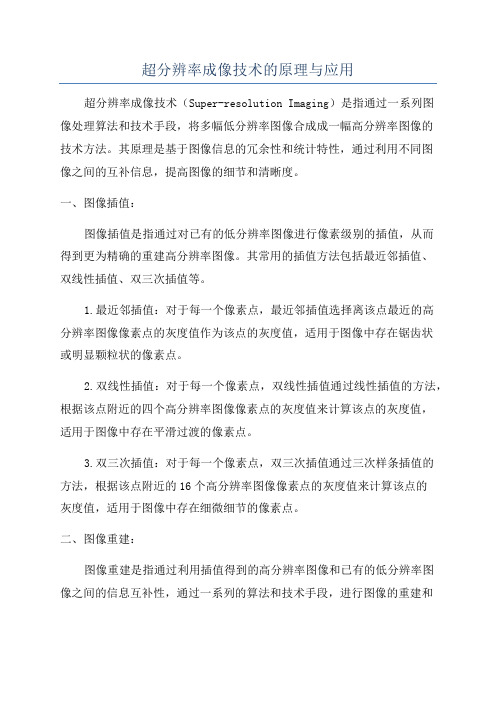
超分辨率成像技术的原理与应用超分辨率成像技术(Super-resolution Imaging)是指通过一系列图像处理算法和技术手段,将多幅低分辨率图像合成成一幅高分辨率图像的技术方法。
其原理是基于图像信息的冗余性和统计特性,通过利用不同图像之间的互补信息,提高图像的细节和清晰度。
一、图像插值:图像插值是指通过对已有的低分辨率图像进行像素级别的插值,从而得到更为精确的重建高分辨率图像。
其常用的插值方法包括最近邻插值、双线性插值、双三次插值等。
1.最近邻插值:对于每一个像素点,最近邻插值选择离该点最近的高分辨率图像像素点的灰度值作为该点的灰度值,适用于图像中存在锯齿状或明显颗粒状的像素点。
2.双线性插值:对于每一个像素点,双线性插值通过线性插值的方法,根据该点附近的四个高分辨率图像像素点的灰度值来计算该点的灰度值,适用于图像中存在平滑过渡的像素点。
3.双三次插值:对于每一个像素点,双三次插值通过三次样条插值的方法,根据该点附近的16个高分辨率图像像素点的灰度值来计算该点的灰度值,适用于图像中存在细微细节的像素点。
二、图像重建:图像重建是指通过利用插值得到的高分辨率图像和已有的低分辨率图像之间的信息互补性,通过一系列的算法和技术手段,进行图像的重建和增强。
常用的重建方法有基于边缘的重建、基于模型的重建和基于深度学习的重建等。
1.基于边缘的重建:该方法主要通过提取低分辨率图像和高分辨率图像之间的边缘信息,通过边缘的插值和重建,提高图像的边缘分辨率和清晰度。
2.基于模型的重建:该方法利用图像之间的相关性和统计特性,通过构建图像模型,通过模型的推断和优化过程,从而得到重建的高分辨率图像。
3.基于深度学习的重建:深度学习方法是目前超分辨率成像技术中应用最为广泛和有效的方法之一、通过搭建深度卷积神经网络(CNN)模型,利用大量的低分辨率和高分辨率图像对进行训练,从而得到提高图像分辨率的能力。
超分辨率成像技术的应用非常广泛。
图像增强的方法有哪些

图像增强的方法有哪些
图像增强的方法包括以下几种:
1. 直方图均衡化(Histogram equalization):通过调整图像的像素分布,增强图像的对比度。
2. 自适应直方图均衡化(Adaptive histogram equalization):与直方图均衡化类似,但是对图像的小区域进行局部均衡化,可以更好地保留细节信息。
3. 均值滤波(Mean filter):用图像中像素的平均值替代该像素的值,平滑图像的同时增强细节。
4. 中值滤波(Median filter):用图像中像素的中值替代该像素的值,能够有效去除椒盐噪声,保留图像边缘。
5. 高斯滤波(Gaussian filter):使用高斯函数对图像进行平滑,可以模糊图像的同时去除噪声。
6. 锐化增强(Sharpening):利用锐化算子对图像进行卷积,突出图像的边缘和细节。
7. 退化与恢复(Degradation and restoration):通过建立图像模糊模型和噪
声模型,对退化图像进行恢复。
8. 增强滤波(Enhancement filter):通过设计特定的增强滤波器,对图像进行增强,如Sobel滤波器、Prewitt滤波器等。
9. 超分辨率(Super-resolution):通过使用多帧图像或者其他方法,提高低分辨率图像的细节和清晰度。
以上仅是图像增强的一些常见方法,随着图像处理技术的不断发展,还有很多其他方法可以用于图像增强。
90个必考词根词缀大串讲-讲义版
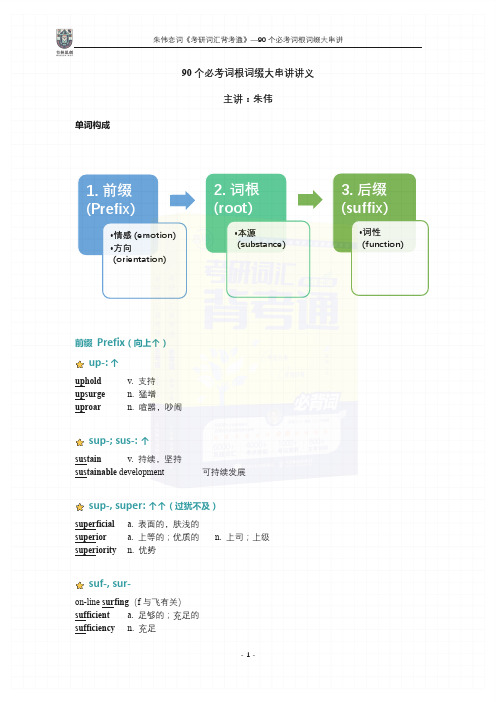
90个必考词根词缀大串讲讲义 主讲:朱伟 单词构成 前缀 Prefix (向上↑)"# up-: ↑uphold v. 支持upsurge n. 猛增uproar n. 喧嚣,吵闹"# sup-; sus-: ↑sustain v. 持续,坚持sustainable development 可持续发展"# sup-, super: ↑↑(过犹不及)superficial a. 表面的,肤浅的superior a. 上等的;优质的 n. 上司;上级superiority n. 优势"# suf-, sur-on-line surfing (f 与飞有关)sufficient a. 足够的;充足的sufficiency n. 充足1. 前缀(Prefix)•情感(emotion)•方向(orientation)2. 词根(root)•本源(substance)3. 后缀(suffix)•词性(function)suffice v. 足够;足以#over-"1. 上; 重叠overlap v. 重叠overlook v. 忽视2. 过(Bite off more than one can chew)over-sensitive a. 过于敏感的over-insistence n. 过于坚持,偏执overwhelm v. 淹没overawe v. 威慑overcome v. 克服(你是弱者)#hyper-: 过"hypersensitive a. 非常敏感的hypercritical a. 吹毛求疵的;过于苛刻的"#mal-: ↓malice n. 恶意,怨恨malicious a. 恶意的maltreat v. 虐待#mis: ↓"mistreat v. 虐待;不公平对待mislead v. 误导misunderstanding n. 误解misjudgment n. 误判#cata =向下,分离"catalogue n. 目录cataclysm n. 灾难;剧变(政治或社会方面的大变动)catastrophe n. 灾难catastrophic a. 灾难性的,大灾难的#a-的用法"#un-"unscrupulous a. 无耻的"#un- + n. → v.unveil v. 揭幕,表明(un. + veil,veil n. 面纱)unleash v.释放,解放(un. + leash,leash n. 拴狗链v. 束缚)"#in-1. 在……里面(当后面跟着的部分并非是独立单词时)inanity n. 空虚2. 向下(当后面跟着的部分是独立单词时)invalidity n. 无效invalid a. 无用的,无效的inconvenient a. 不方便的"#in-, ill-, im-, imm-, irr-immune a. 有免疫力的immunity n. 免疫力immunology n. 免疫学immunologist n. 免疫学家#dipl, dupl, dub"diplomatic immunity 外交豁免权#imm(以imm开头的单词)"immortality n. 永垂不朽immortal a. 不死的;不朽的#im-:否定(=imm =ill =irr)/ 进入,向内"impartial a. 不偏不倚的,公正的impart v. 传授impact n. 影响;撞击v. 对……产生影响;撞击impair v. 损害imprudent a. 轻率的impudent a. 无礼的immobile a. 固定的;稳定的illegal a. 不合法的(多指行为表现、行为方式)illegitimate a. 不合法的(多指法律资格、法律效力)"#ir- = notirreplaceable a. 不可取代的;至关重要的irrational a. 不合理的#em-: 在……里面"embed v. (使)嵌入embellish v. 美化,装饰embarrass v. 使窘迫,使尴尬embarrassing a. 令人尴尬的embarrassment n. 尴尬#ab-: off 剥离,抽离,分开"aberrant a. 不正常的;畸变的abolish v. 废除;废止(法律、规定或惯例等)abstract n. 摘要 a. 抽象的v. 抽取;使分离abound v. 充满;到处存在#di-, de-, dis-: away ↓"display n. 展览v. 陈列;展示deforest v. 滥砍滥伐deforestation n. 滥砍滥伐dilemma n. (难以抉择、进退两难的)窘境,困境#dis-: away 分开/ not 否定"dispense v. 分发distribute v. 分发;配送,分销前缀Prefix(向下↓)#of-: ↓(= op-, ob-)"offend v.得罪,冒犯offensive a. 无礼的,冒犯的offense n.进攻;冒犯#op-: ↓"oppose v. 反对opponent n. 反对者oppress v. 压迫oppressed a. 受压制的,受压迫的the oppressed 被压迫者"#ob-obstacle(分析:stack 捆)n. 障碍,阻碍oblige(ob=对立, 对面)v. 迫使,责成oblige sb. to do sth. 迫使某人做某事"#anti-: ↓anti-human offense 反人类罪anti-cancerantibodyanticipate(= expect)v. 期望#counter-: ↓对,假"counterpart n. 对应的人(或事物)counterbalance n. 平衡力v. 对……起平衡作用counterfeit currency 伪造的(犯罪性质)#e- = ex- = out"evoke(vok=voice =VOA à呼唤, 唤醒)evocative a. 被唤起的, 被引起的extinguisher n.灭火器extinguish v. 熄灭;消灭extinct volcano 死火山extinction n. 灭绝,消亡#pri- = pre- = pro-"primitive n. 原始人a. 远古的prophet n. 预言家proceed v. 推进precede v. 处在……之前,先于precedence n. 优先priority n. 优先权precedent n. 先例"#re- : a/b1. =againreconsider v. 重新考虑restore v. 恢复restoration n. 恢复,还原renaissance n. 复兴,再生;文艺复兴recommend : commend v. 夸奖recommendation n. 提议;推荐reassure v. 确保,保证2. =back撤,拆retreat v. 撤退recall v. 召回n. 召回【搭】recall crisis 召回危机refuge n. 避难(所)=shelter n.(交警的)安全岛refugee n. 难民,避难者repent of后悔,悔恨resent: 送出去的东西想要回来v. 怨恨, 仇恨, 愤怒retract:tract=收缩revoke: re=back(收回, 否认)renouncerevolve=旋转(自转)revolutionary a. 革命的revolutionize = change sth. vastly v. 使彻底变革resolve v. 解决,解析resolution n. 决心,解析度,分辨率resolute a. 有决心的,果断的reverse v. 逆转;撤销,推翻#with- = back"withdraw v. 撤销,取款withhold v. 扣留;拒绝给予#centri=center中"ethnocentric a. 种族优越的;民族中心主义的white-centered a. 白人种族优越的self-centered a. 自私的eccentric a. 古怪的,异乎寻常的"#ethno=ethnic=人种的,种族的ethnology n. 人种学ethnography n. 人种志ethnic a. 种族的ethnicity n. 种族特点= the characteristic of a race or a tribe #med=mid=mod"meddle v. 干涉meddle in / with干涉,干预med=mid=mod=inter=middlemediate+ in/between调停,斡旋meditate v. 沉思modulate v. 调适,调配moderate a. 得体的modest a. 谦虚的,谦逊的。
图像超分辨率重建和插值算法研究
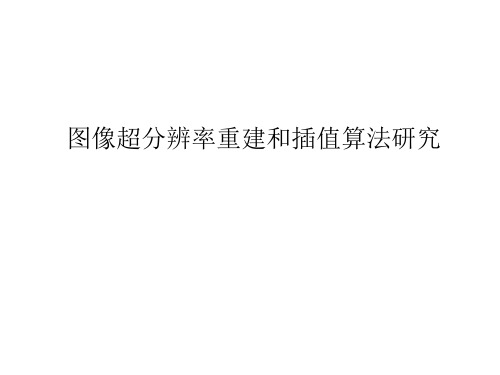
• 超分辨率重建的背景和意义
图像的高分辨率是指着图像含有的像素密度高, 能提供丰富的细节信息,对客观场景的描述更准 确细致。
高分辨率图像在信息时代的需求非常广泛,诸 如卫星遥感图像、视频安全监控、军事侦查航拍 领域、医学数字影像和视频标准转换等领域都具 有十分重要的应用。
(HMT)模型SRR方法的改进,对SRR插值方法的改进, 以及对SRR重构方法的改进。超分辨率的概念最早出现在 光学领域,是指复原衍射极限以外数据的过程。第一次超 分辨率概念的提出是在1955年Toraldo di Francia关于光学 成像的雷达文献中。
图像SRR的研究要始于上世纪80年代,Tsai和Huang[4]首 先提出了基于序列或多帧图像的SRR问题,他们分析并证 明了:彼此间互相有平移的图像序列中获取分辨率增强的 静态图像的可能性,而且给出了在频域里解决问题的方法。
随机性正则化方法主要有:最大似然估计(ML)、最大 后验概率估计(MAP)等。MAP方法的优点是结合空域 先验信息的能力强,结合有效的最优化方法进行求解利于 扩展和改进,因此得到广泛应用。MAP方法可以加入不同 的图像先验模型,在解中加入先验约束,能够保证解的唯 一存在性。虽然空域方法的缺点是计算量大,代价高。为 减少计算复杂度,又产生了针对空间模糊不变和平移运动 情形的快速SRR算法。 美国加州大学Milanfar等提出了的大量实用超分辨率图像 复原算法,Chan等从总变差正则方面,Nagy等从数学方 法、多帧图像的去卷积和彩色图像的超分辨率增强方面, 对超分辨率图像恢复进行了研究。此外,Rajan和Wood等 分别从物理学和成像透镜散射的角度提出了新的超分辨率 图像恢复方法;韩国Pohang理工大学对各向异性扩散用 于超分辨率。图像超分辨率重建技术的研究如火如荼,带 动了视频超分辨率重建的研究,但是仍然还有许多实际问 题需要有待解决。
ps生成式ai术语
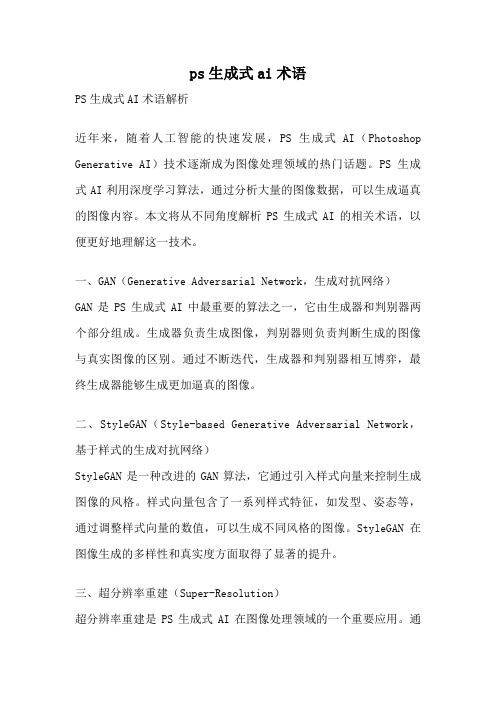
ps生成式ai术语PS生成式AI术语解析近年来,随着人工智能的快速发展,PS生成式AI(Photoshop Generative AI)技术逐渐成为图像处理领域的热门话题。
PS生成式AI利用深度学习算法,通过分析大量的图像数据,可以生成逼真的图像内容。
本文将从不同角度解析PS生成式AI的相关术语,以便更好地理解这一技术。
一、GAN(Generative Adversarial Network,生成对抗网络)GAN是PS生成式AI中最重要的算法之一,它由生成器和判别器两个部分组成。
生成器负责生成图像,判别器则负责判断生成的图像与真实图像的区别。
通过不断迭代,生成器和判别器相互博弈,最终生成器能够生成更加逼真的图像。
二、StyleGAN(Style-based Generative Adversarial Network,基于样式的生成对抗网络)StyleGAN是一种改进的GAN算法,它通过引入样式向量来控制生成图像的风格。
样式向量包含了一系列样式特征,如发型、姿态等,通过调整样式向量的数值,可以生成不同风格的图像。
StyleGAN在图像生成的多样性和真实度方面取得了显著的提升。
三、超分辨率重建(Super-Resolution)超分辨率重建是PS生成式AI在图像处理领域的一个重要应用。
通过PS生成式AI技术,可以将低分辨率的图像转化为高分辨率的图像,并且保持图像的细节和清晰度。
这对于图像放大、图像增强等应用具有重要意义。
四、图像修复(Image Inpainting)图像修复是PS生成式AI的另一个应用领域,它可以根据图像的上下文信息,自动填补图像中的缺失部分。
通过学习大量图像数据,PS生成式AI可以准确地预测缺失区域的内容,使图像看起来完整无缺。
五、图像生成(Image Generation)PS生成式AI最核心的功能之一就是图像生成。
通过学习大量真实图像的特征,PS生成式AI可以生成与之相似的新图像。
2023届上海市普陀区高三下学期二模英语试题
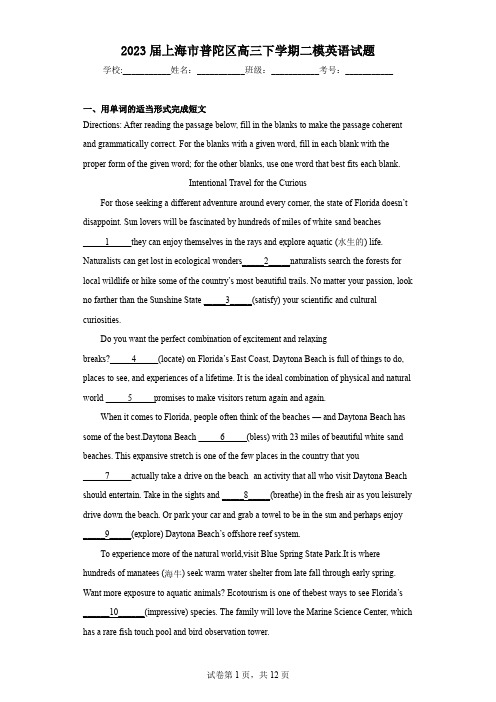
2023届上海市普陀区高三下学期二模英语试题学校:___________姓名:___________班级:___________考号:___________一、用单词的适当形式完成短文Directions: After reading the passage below, fill in the blanks to make the passage coherent and grammatically correct. For the blanks with a given word, fill in each blank with the proper form of the given word; for the other blanks, use one word that best fits each blank.Intentional Travel for the CuriousFor those seeking a different adventure around every corner, the state of Florida doesn’t disappoint. Sun lovers will be fascinated by hundreds of miles of white-sand beaches_____1_____they can enjoy themselves in the rays and explore aquatic (水生的) life. Naturalists can get lost in ecological wonders_____2_____naturalists search the forests for local wildlife or hike some of the country’s most beautiful trails. No matter your passion, look no farther than the Sunshine State _____3_____(satisfy) your scientific and cultural curiosities.Do you want the perfect combination of excitement and relaxingbreaks?_____4_____(locate) on Florida’s East Coast, Daytona Beach is full of things to do, places to see, and experiences of a lifetime. It is the ideal combination of physical and natural world _____5_____promises to make visitors return again and again.When it comes to Florida, people often think of the beaches — and Daytona Beach has some of the best.Daytona Beach _____6_____(bless) with 23 miles of beautiful white-sand beaches. This expansive stretch is one of the few places in the country that you_____7_____actually take a drive on the beach- an activity that all who visit Daytona Beach should entertain. Take in the sights and _____8_____(breathe) in the fresh air as you leisurely drive down the beach. Or park your car and grab a towel to be in the sun and perhaps enjoy _____9_____(explore) Daytona Beach’s offshore reef system.To experience more of the natural world,visit Blue Spring State Park.It is where hundreds of manatees (海牛) seek warm-water shelter from late fall through early spring. Want more exposure to aquatic animals? Ecotourism is one of thebest ways to see Florida’s ______10______(impressive) species. The family will love the Marine Science Center, which has a rare fish touch pool and bird observation tower.二、选用适当的单词或短语补全短文Directions: Fill in each blank with a proper word chosen from the box. Each word can beA New Exoplanet in the SystemIn 2014, data from NASA’s Kepler Space Telescope allowed astronomers to announce the detection of three planets orbiting Kepler-138. This wasstar, a transit.Benneke and his colleague Diana Dragomir, from the University of New Mexico, came up with the idea of re-observing the planetary _____12_____with the Hubble and Spitzer space telescopes between 2014 and 2016 to catch more transits of Kepler-138d, the third planet in the system, in order to study its atmosphere.While earlier NASA Kepler space telescope observations only showed transits of three small planets around Kepler-138, Piaulet and her team were _____13_____to find that the Hubble and Spitzer observations suggested the presence of a fourth planet in the system, Kepler-138e.This newly found planet is small and farther from its star than the three others, taking 38 days to complete an orbit.The planet is in the _____14_____zone of its star, a temperate region where a planet receives just the right amount of heat from its cool star to be neither too hot nor too cold to allow the presence of liquid water. Observing the exoplanet’s transit would have allowed astronomers to _____15_____its size.With Kepler-138e, the masses of the_____16_____known planets were measured again via the transit timing-variation method, which consists of _____17_____small variations in the precise moments of the planets’ transits in front of their star caused by the gravitational pull of other nearby planets.The researchers had another surprise: they found that the two water worlds Kepler-138c and d are twin planets, with ____18____the same size and mass, while they were previously thought to be greatly different. The closer-in planet,Kepler-138b, on the other hand, is____19____ to be a small Mars-mass planet, one of the smallest exoplanets known to date.“As our instruments and techniques become _____20_____ enough to find and study planets that are farther from their stars, we might start finding a lot more water worlds like Kepler-138 c and d,” Benneke concluded.三、完形填空“Challenge-based learning is often focused on the challenges that have global impact.analyze the challenge from multiple points of view, and from different scientific perspectives,” says Vilma Sukacke, a researcher at Kaunas University of Technology (KTU), Lithuania.Such a learning approach is very fitting to sustainability education, which, according to various scholars,_____23_____a contextual, problem-oriented, reflective, interdisciplinary (跨学科), collaborative, participatory and empowered learning environment. In other words, educators have to _____24_____from more traditional teacher-centred education to becoming instructional designers of student-centred education.Aiming to evaluate the _____25_____of such approaches, a group of researchers from Lithuanian, Danish, German, Portuguese and Spanish universities conducted a systematic literature review,_____26_____the three active learning methods, i.e.. project, problem and challenge-based learning according to the ADDIE (analysis, design, development, implementation, and evaluation) framework._____27_____the educators agree that problem-based learning (PBL), project-based learning (PJBL) and, more recently, challenge-based learning (CBL) are efficient in teaching the students to _____28_____technology in real-life situations and improving their transversal (横向) skills, such as teamwork, communication and conflict resolution, the application of these methods in the classroom may be challenging for both sides.“In my practice, I have noticed that sometimes students are _____29_____innovative learning methods and are considering them as a sort of a game. As these classes often take place in a play full environment, full of different pencils, colorful notes and building blocks, it’s difficult for the students to take them _____30_____. Therefore, it’s very importantto_____31_____the methods and the goals to the learners very clearly,” says Professor Saule Petroniene from KTU Faculty of Social Sciences, Arts and Humanities, a co-author of thestudy.According to her, to successfully apply unconventional teaching methods is a challenge for a beginning teacher. However, this effort _____32_____, especially when students continue their activities outside school and focus on solving _____33_____social problems.Rescarchers believe that the _____34_____of CBL, PJBL, and PBL requires a paradigm (范式) shift, where organization, staff and students change their view to education and learning. In this process, both teachers and students need to apply new skills and_____35_____roles that they might not have needed before.21.A.allow B.introduce C.accept D.expect 22.A.carrying on B.focusing on C.applying for D.inquiring about 23.A.cares about B.tells of C.turns to D.calls for 24.A.shift B.differ C.suffer D.learn 25.A.outcome B.efficiency C.impact D.value 26.A.exploring B.applying C.modifying D.explaining 27.A.Whether B.As long as C.Although D.Because 28.A.develop B.include C.associate D.integrate 29.A.approving B.questioning C.preventing D.adopting 30.A.affectionately B.calmly C.seriously D.coolly 31.A.transfer B.change C.communicate D.express 32.A.pays off B.goes away C.gives off D.breaks down 33.A.subjective B.real C.imaginary D.virtual 34.A.combination B.organization C.realization D.cooperation 35.A.take on B.put away C.send for D.deal with四、阅读理解Mona Lisa, also called Portrait of Lisa Gherardini, wife of Francesco del Giocondo, Italian La Gioconda, or French La Joconde, oil painting on a wood panel by Leonardo da Vinci, is probably the world’s most famous painting. It was painted sometime between 1503 and 1519, when Leonardo was living in Florence, and it now hangs in the Louvre Museum, Paris, attracting people throughout the world to see it in the 21st century. The sitter’s mysterious smile and her unproven identity have made the painting a source of ongoinginvestigation and fascination.But at least a dozen excellent copies of the Mona Lisa exist, many of them by Leonardo’s students. One such copy at the Prado Museum in Madrid was thought to have been painted years after the original. However, during the restoration of the painting in the early 2010s, which included using advanced reflexology to examine the work beneath the surface, conservators discovered that the painting had changes that mirrored those of the original. The findings suggested that the artist—likely one of the master’s assistants—painted the copy as Leonardo worked on the Mona Lisa in his studio. Thus, “the Prado version” became the only known copy completed during Leonardo’s lifetime. Conservators cleaned the entire painting and removed its black background, revealing a detailed landscape resembling Leonardo’s version and vibrant colors, possibly arousing those of the original before the paint applied by early restorers darkened over time.Other copies of the Mona Lisa include so-called Isleworth Mona Lisa, which some critics claim is da Vinci’s first edition. The claim was a controversial one, with several leading Leonardo scholars flatly denying it. Numerous interpretations, often referred to as Monna Vanna, also exist and were likely completed by Leonardo’s students with occasional input from their master.In June, a copy of the Mona Lisa is said to have been sold for a record 2.9 million euros (22 million yuan) at Christie’s in Paris. The copy is believed to have been made by a follower of Leonardo da Vinci in the early 17th century and later was purchased by Harkin in 1953 from an art dealer in Nice, France, hence it got the name Heggin’s Mona Lisa. High imitation is also worth money! As the art investment market heats up. various art reproductions continue to emerge. Collectors have not shut out these fine copies, some of which have not only made it into the house, but also sold for high prices. Is it really worth it to pay a high price for an art reproduction? This issue is getting more and more attention from collectors. 36.What can we learn about Mona Lisa in Para. 1?A.This painting has been sold out.B.Her smile and identity arouse people’s curiosity.C.The painting is said to be hanging in Florence now.D.The painting was created by Leonardo da Vinci in France.37.What does “the Prado version“refer to in the passage?A.All the restored copies.B.The works of early restorers.C.A copy from the Prado Museum.D.An excellent copy of da Vinci. 38.Why don’t collectors turn away these wonderful reproductions?A.Because of the entertainment.B.Because of the love for painters. C.Because of the short supply.D.Because of the high returns. 39.Which of the following is the best title of the passage?A.Mona Lisa B.Other Mona LisasC.Mona Lisa’s Smile D.Mona Lisa’s ValueIf you really want to see all that Yellowstone Park has to offer, it would take you a lifetime! However, most of us do not have a lifetime to spend in Yellowstone, but in 2-3 full days, you will be able to hit most of the highlights.There is no such thing as racing around the park. Yellowstone has 154 miles of a main highway, known as the Grand Loop Road. The general park speed limit is 45 miles per hour but in normal traffic, you will be lucky to average 30 miles per hour. Figure at least an hour of driving time for every thirty miles of distance. If you plan to get off the bus and visit the park, it will take at least 1.2 to 2 hours at almost every stop. In addition, there will be any mumber of unscheduled stops, such as waiting for wild animals to cross the roadA suggested starting point for your tour of Yellowstone National Park is to enter through the South Gate, which is approximately sixty-two miles north of Jackson, Wyoming. Everyone comes to Yellowstone to see Old Faithful.Although,not the largest geyser (间歇泉) to erupt in the Park,it is the most reliable regarding the time of eruption. The time of the eruption,the length of the eruption and the height of the eruption vary from year to year. As you enter the Park, ask the Park Information Office if he has information on the expected times for the eruption of Old Faithful.As you drive north on the Grand Loop Road, keep an eye out for wildlife. At Grant Village Junction, turn left (west) and head toward Old Faithful Village. Once at Old Faithful Village, take some time to walk around the boardwalks and visit some of the many other geysers in the Upper Geyser Basin. Include the 1.4-mile walk to Morning Glory Pool, one of the most colorful thermal (热能) features in all of Yellowstone.And do not forget to visit theOld Faithful Inn,which is the single most impressive human structure in Yellowstone.Continue on north and visit the Lower Geyser Basin. The area is scattered and features regularly erupting geysers, hot springs, and a fascinating mud pool. After leaving the Lower Geyser Basin, continue north to Norris Junction and stay to the right to travel to Norris Geyser Basin. One of Yellowstone’s most popular geyser basins, Norris is home to one of the Park’s most popular geysers and Steamboat. In addition, there are several miles of boardwalks from which you can explore dozens of multi-colored thermal features.As for more, go on a Sightseeing Tour of Yellowstone to see it all.Yellowstone Scenic Tours - Private Day TripsYellowstone YearRound AdventuresExperience Montana With Front Seat Guided Bus Tour40.What can you learn from the first paragraph?A.Some people live in parks all their lives.B.Hardly anyone can see the whole park. C.The park can provide you with everything.D.It’s enough to spend a few days in the park.41.What does the underlined phrase “unscheduled stops” refer to?A.The walking speed of wild animals being affected.B.Travelers’ stopping and going in the park.C.The vehicles’ being parked in the proper area.D.The traffic’s stopping to make way for wild animals.42.Which of the following travel maps is true according to Paragraph 4-5?A.B.C.D.The world is wasting the opportunity to ”build back better“ from the Covid-19 pandemic, and faces disastrous temperature rises of at least 2.7°C if countries fail to strengthen their climate commitments, according to a report from the UN.Tuesday’s publication warns that countries’ current commitments would reduce carbon by only about 7.5% by 2030,far less than the 45% cut, which scientists say is needed to limit global temperature rises to 1.5°℃, the aim of the Cop26summit that opens in Glasgow this Sunday.António Guterres, the UN secretary-general, described the findings as a “thundering wake-up call“ to world leaders. while experts called for action against fossil fuel companies.Although more than 100 countries have promised to reach net zero emissions around mid-century, this would not be enough to avoid climate disasters, according to the UN emissions report, which examines the shortfall between countries’ intentions and actions needed on the climate. Many of the net zero commitments were found to be unclear, and unless accompanied by strict cuts in emissions this decade would allow global heating of a potentially disastrous extent.Guterres said: “The heat is on, and as the contents of the report show, the leadership we need is off. Far off. Countries are wasting a massive opportunity to invest Covid-19 finance and recovery resources in sustainable, cost-saving, planet-saving ways. As world leaders prepare for Cop26, this report is another thundering wake-up call. How many do we need?”Inger Andersen, the director of the UN Environment Programme (UNEP) said: “Climate change is no longer a future problem. It is a now problem. To stand a chance of limiting global warming to 1.5℃, we have 8 years to almost halve greenhouse gas emissions: 8 years to make the plans, put in place the policies, carry them out and deliver the cuts.The clock is ticking loudly.”Emissions fell by about 5.4% last year during Covid lockdowns, the report found, but only about one-fifth of the economic recovery spending goes towards reducing carbonemissions. This failure to ”build back better“ despite promises by governments around the world cast doubt on the world’s willingness to make the economic shift necessary to settle the climate crisis, the UN said.In the run-up to Cop26, countries were supposed to submit national plans to cut emissions - called nationally determined contributions (NDCs) - for the next decade, a requirement under the 2015 Paris climate agreement. But the UNEP report found only half of countries had submitted new NDCs, and some governments had presented weak plans. 43.Why were the findings described as a “thundering wake-up call”in Para. 3? A.Because the world has failed to deliver on its current promises.B.Because the serious problems were brought about by global fossil fuels.C.Because a global temperature rise of at least 2.7°C would be a disaster.D.Because the opportunities presented by covid-19 have been wasted.44.According to the author, in what circumstances is global warming avoidable?A.New plans will be made to protect the environment.B.Measures will be taken to reduce emissions in the coming ten years.C.Transitions will be made in response to the global climate crisis.D.Global sustainable environmental resources will be greatly developed.45.What is the author’s purpose in saying ”How many do we need?“ in Para. 5?A.To show the number of alarm clocks required.B.To inquire the number of the countries attending the meeting.C.To explain the reason for the world’s wasting chances.D.To stress the need to save energy and reduce emissions.46.What can be inferred from the last paragraph?A.Few countries have submitted plans to reduce the emissions.B.Most of the countries work under the Paris Climate Agreement.C.Plans to cut emissions of many countries are far from satisfactory.D.Much progress in reducing emissions has been made these years.五、六选四Huizhou Architecture Comes to LifeHuizhou has a long history. When successful Huizhou businessmen got old,they oftenwent back to their hometown and built houses to spend their remainingyears._____47_____Now, walking along the bluestone streets there,visitors can appreciate the distinctive Huizhou-style houses,featuring white walls,dark tiles (瓦片) and layered horse-head roofs, and feel like they are lost in a traditional Chinese ink painting.Huizhou architecture, with its long tradition and great diversity, occupies an important place in Chinese architectural landscape, says Zhang Wangnan, director of the China Huizhou Culture Museum in Huangshan. Huizhou houses tend to be built on the natural places, since Huizhou is a mountainous area with few flat areas of land, according to Zhang. Moreover, daylight is valued in Huizhou houses, reflected in the building of open interior courtyards, allowing sunshine to enter the rooms. “Huizhou businessmen also believed that water symbolizes wealth._____48_____It stands for keeping windfall profits,” says Zhang.Huizhou is famous for its stone, wood and brick carvings, which are widely used to decorate local houses. “____49____In this way,they integrate their aesthetic (审美) values and emotions into the buildings,making them more beautiftul and educating their children through the meanings contained in the patterns,” says Zhang._____50_____ “If you look at the outside of a Huizhou house, it seems simple, enclosed by walls, but the insides are open and broad, with complicated decorations. It is just like Huizhou people, who are often reserved toward strangers but friendly when you get to know them.” says Zhang. He also says that Ming houses differ from those of the QingDynasty in many aspects, for example, having fewer horse-head roofs and less complicated carvings. A.When it rains, water falling on the roof soon flows to the courtyard.B.Many of their houses are well-preserved today, especially in Xidi and Hongcun.C.To talk about Huizhou culture, we must first understand Huizhou.D.People carved beautiful patterns and historical stories on the walls, windows and wooden posts of their houses.E.Features of Huizhou houses display the characteristics of local people.F.Huizhou people have established many schools in the field of culture.六、概要写作51.Directions: Read the following passage. Summarize the main idea and the main point(s) of the passage in no more than 60 words. Use your own words as far as possible.How to Stay Healthy in AutumnIn recent days, the weather in most regions is no longer hot, and people can relax and enjoy the cool autumn. However, there is a big gap in temperature in the morning and evening, and the air is also drier. All of these factors can lead to dry mouth and nose, sore throat, dry cough, dry hair loss and other symptoms. People are more likely to get sick during the seasonal change from summer through autumn. So, here are some tips to keep you healthy and comfortable into the winter months.Do eat watery food. According to traditional Chinese medicine, autumn corresponds to the lungs of the human body.Pay attention to wet lungs in dry weather.The most convenient and easy way to protect lungs in autumn is to drink more water. In addition, you can adopt the diet therapy of traditional Chinese medicine, eating some watery food, like pear and duck meat.Do have easily digested food. People’s spleen (牌) and stomach functions are weakened because of the raw and cold food they had during the long, hot summer. So in autumn, you should give your spleen and stomach a rest. For example,you can choose some nutritious food that is easy to digest to eat, such as fish and red bean.Be sure to take vitamin supplements. Vitamins play an important role in the body’s immune system. The number and vitality of immune cells are related to vitamins when the body resists foreign invasion. It is recommended that you eat plenty of fresh vegetables and fruits rich in vitamin C, such as kiwi and oranges.Sleep is an important means for people to restore their physical strength, ensure their health and enhance their immunity. In autumn, it is suggested that you go to bed at 9 pm, or try to fall asleep before 11 o’clock. If you sleep at this time, it is great for your body and you can get a good quality of sleep.___________________________________________________________________________ ___________________________________________________________________________ ___________________________________________________________________________ ___________________________________________________________________________ ___________________________________________________________________________ ___________________________________________________________________________ _________________________七、汉译英(整句)52.不要乱扔垃圾,你会被罚款的。
超分辨率技术的发展
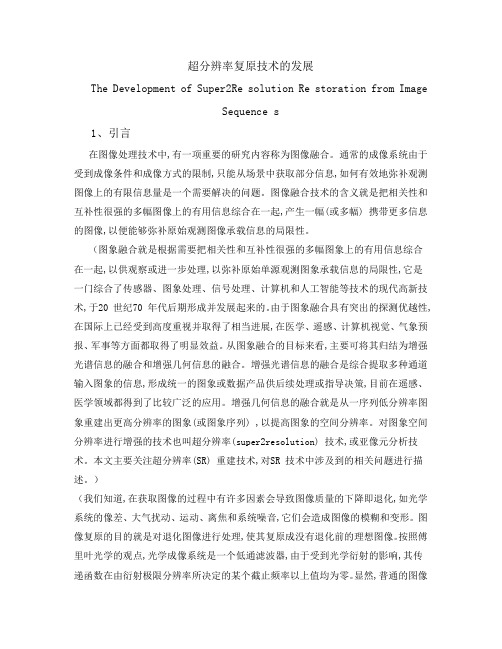
超分辨率复原技术的发展The Development of Super2Re solution Re storation from ImageSequence s1、引言在图像处理技术中,有一项重要的研究内容称为图像融合。
通常的成像系统由于受到成像条件和成像方式的限制,只能从场景中获取部分信息,如何有效地弥补观测图像上的有限信息量是一个需要解决的问题。
图像融合技术的含义就是把相关性和互补性很强的多幅图像上的有用信息综合在一起,产生一幅(或多幅) 携带更多信息的图像,以便能够弥补原始观测图像承载信息的局限性。
(图象融合就是根据需要把相关性和互补性很强的多幅图象上的有用信息综合在一起,以供观察或进一步处理,以弥补原始单源观测图象承载信息的局限性,它是一门综合了传感器、图象处理、信号处理、计算机和人工智能等技术的现代高新技术,于20 世纪70 年代后期形成并发展起来的。
由于图象融合具有突出的探测优越性,在国际上已经受到高度重视并取得了相当进展,在医学、遥感、计算机视觉、气象预报、军事等方面都取得了明显效益。
从图象融合的目标来看,主要可将其归结为增强光谱信息的融合和增强几何信息的融合。
增强光谱信息的融合是综合提取多种通道输入图象的信息,形成统一的图象或数据产品供后续处理或指导决策,目前在遥感、医学领域都得到了比较广泛的应用。
增强几何信息的融合就是从一序列低分辨率图象重建出更高分辨率的图象(或图象序列) ,以提高图象的空间分辨率。
对图象空间分辨率进行增强的技术也叫超分辨率(super2resolution) 技术,或亚像元分析技术。
本文主要关注超分辨率(SR) 重建技术,对SR 技术中涉及到的相关问题进行描述。
)(我们知道,在获取图像的过程中有许多因素会导致图像质量的下降即退化,如光学系统的像差、大气扰动、运动、离焦和系统噪音,它们会造成图像的模糊和变形。
图像复原的目的就是对退化图像进行处理,使其复原成没有退化前的理想图像。
反卷积层英语缩写
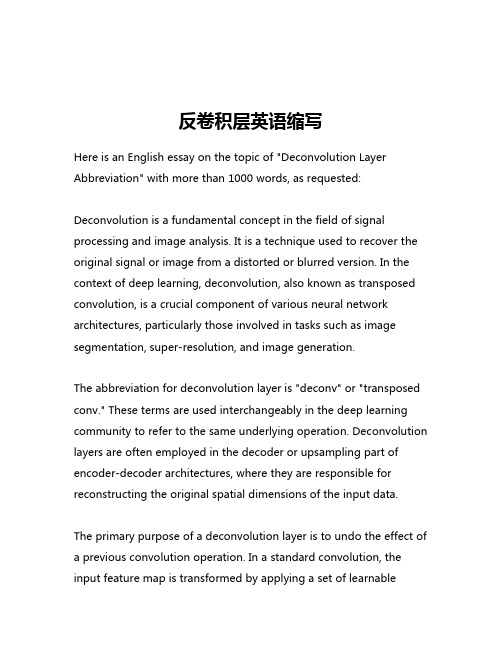
反卷积层英语缩写Here is an English essay on the topic of "Deconvolution Layer Abbreviation" with more than 1000 words, as requested:Deconvolution is a fundamental concept in the field of signal processing and image analysis. It is a technique used to recover the original signal or image from a distorted or blurred version. In the context of deep learning, deconvolution, also known as transposed convolution, is a crucial component of various neural network architectures, particularly those involved in tasks such as image segmentation, super-resolution, and image generation.The abbreviation for deconvolution layer is "deconv" or "transposed conv." These terms are used interchangeably in the deep learning community to refer to the same underlying operation. Deconvolution layers are often employed in the decoder or upsampling part of encoder-decoder architectures, where they are responsible for reconstructing the original spatial dimensions of the input data.The primary purpose of a deconvolution layer is to undo the effect of a previous convolution operation. In a standard convolution, the input feature map is transformed by applying a set of learnablefilters, resulting in a smaller output feature map. This reduction in spatial size is often necessary for tasks that require extracting high-level features from the input data. However, in certain applications, such as image segmentation or image generation, it is necessary to restore the original spatial dimensions of the feature maps. This is where deconvolution layers come into play.Deconvolution layers work by essentially reversing the convolution operation. They take a smaller input feature map and expand it to a larger output feature map, effectively "deconvolving" the input. This is achieved by applying a set of learnable transposed convolution filters, which are essentially the transpose of the original convolution filters. The transposed convolution operation can be thought of as a convolution operation with the input and output feature maps swapped, and the filter kernel flipped both horizontally and vertically.One of the key advantages of deconvolution layers is their ability to effectively upsample the feature maps, thereby increasing the spatial resolution of the output. This makes them particularly useful in tasks where high-resolution output is desired, such as image super-resolution or semantic segmentation. By stacking multiple deconvolution layers, the network can gradually increase the spatial dimensions of the feature maps, effectively reconstructing the original input.However, the use of deconvolution layers is not without its challenges. One common issue is the presence of checkerboard artifacts in the output, which can be caused by the transposed convolution operation. These artifacts can be mitigated by using alternative upsampling techniques, such as bilinear interpolation or learnable upsampling layers.Another challenge is the potential for the deconvolution layers to introduce a shift in the output feature maps relative to the input. This shift can be problematic in certain applications, such as pixel-wise prediction tasks, where accurate spatial alignment is crucial. To address this issue, researchers have proposed various techniques, such as the use of padding or the incorporation of additional spatial transformation layers.Despite these challenges, deconvolution layers have proven to be invaluable in a wide range of deep learning applications. They have been successfully used in various network architectures, including fully convolutional networks (FCNs), encoder-decoder models, and generative adversarial networks (GANs). In these architectures, deconvolution layers play a crucial role in the upsampling and reconstruction of the output, enabling the networks to generate high-quality, spatially-aware predictions.In conclusion, deconvolution layers, or transposed convolution layers,are an essential component in deep learning, particularly for tasks that require the restoration of spatial dimensions or the generation of high-resolution outputs. While they come with their own set of challenges, such as the potential for checkerboard artifacts and spatial shifts, ongoing research and advancements in the field continue to refine and improve the use of deconvolution layers in various deep learning applications.。
超分辨率算法综述
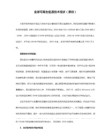
超分辨率复原技术的发展The Development of Super2Re solution Re storation from ImageSequence s1、引言在图像处理技术中,有一项重要的研究内容称为图像融合。
通常的成像系统由于受到成像条件和成像方式的限制,只能从场景中获取部分信息,如何有效地弥补观测图像上的有限信息量是一个需要解决的问题。
图像融合技术的含义就是把相关性和互补性很强的多幅图像上的有用信息综合在一起,产生一幅(或多幅)携带更多信息的图像,以便能够弥补原始观测图像承载信息的局限性。
(图象融合就是根据需要把相关性和互补性很强的多幅图象上的有用信息综合在一起,以供观察或进一步处理,以弥补原始单源观测图象承载信息的局限性,它是一门综合了传感器、图象处理、信号处理、计算机和人工智能等技术的现代高新技术,于20 世纪70 年代后期形成并发展起来的。
由于图象融合具有突出的探测优越性,在国际上已经受到高度重视并取得了相当进展,在医学、遥感、计算机视觉、气象预报、军事等方面都取得了明显效益。
从图象融合的目标来看,主要可将其归结为增强光谱信息的融合和增强几何信息的融合。
增强光谱信息的融合是综合提取多种通道输入图象的信息,形成统一的图象或数据产品供后续处理或指导决策,目前在遥感、医学领域都得到了比较广泛的应用。
增强几何信息的融合就是从一序列低分辨率图象重建出更高分辨率的图象(或图象序列) ,以提高图象的空间分辨率。
对图象空间分辨率进行增强的技术也叫超分辨率(super2resolution) 技术,或亚像元分析技术。
本文主要关注超分辨率(SR) 重建技术,对SR 技术中涉及到的相关问题进行描述。
)(我们知道,在获取图像的过程中有许多因素会导致图像质量的下降即退化,如光学系统的像差、大气扰动、运动、离焦和系统噪音,它们会造成图像的模糊和变形。
图像复原的目的就是对退化图像进行处理,使其复原成没有退化前的理想图像。
基于单像素成像的遥感图像分辨率增强模型
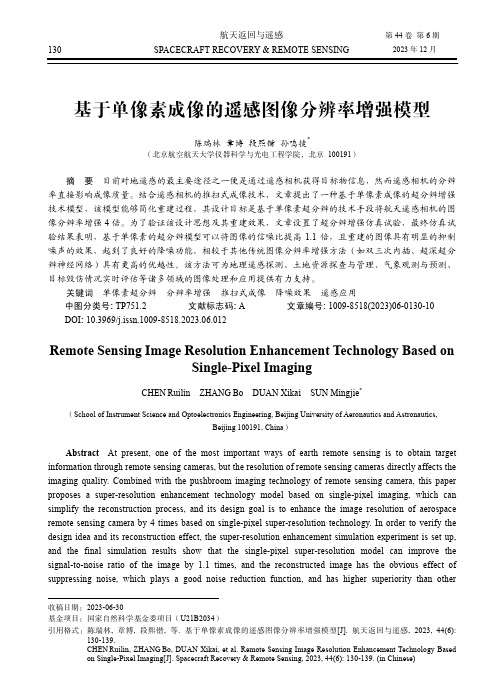
航天返回与遥感第44卷第6期130 SPACECRAFT RECOVERY & REMOTE SENSING2023年12月基于单像素成像的遥感图像分辨率增强模型陈瑞林章博段熙锴孙鸣捷*(北京航空航天大学仪器科学与光电工程学院,北京100191)摘要目前对地遥感的最主要途径之一便是通过遥感相机获得目标物信息,然而遥感相机的分辨率直接影响成像质量。
结合遥感相机的推扫式成像技术,文章提出了一种基于单像素成像的超分辨增强技术模型,该模型能够简化重建过程,其设计目标是基于单像素超分辨的技术手段将航天遥感相机的图像分辨率增强4倍。
为了验证该设计思想及其重建效果,文章设置了超分辨增强仿真试验,最终仿真试验结果表明,基于单像素的超分辨模型可以将图像的信噪比提高1.1倍,且重建的图像具有明显的抑制噪声的效果,起到了良好的降噪功能,相较于其他传统图像分辨率增强方法(如双三次内插、超深超分辨神经网络)具有更高的优越性。
该方法可为地理遥感探测、土地资源探查与管理、气象观测与预测、目标毁伤情况实时评估等诸多领域的图像处理和应用提供有力支持。
关键词单像素超分辨分辨率增强推扫式成像降噪效果遥感应用中图分类号: TP751.2文献标志码: A 文章编号: 1009-8518(2023)06-0130-10 DOI: 10.3969/j.issn.1009-8518.2023.06.012Remote Sensing Image Resolution Enhancement Technology Based onSingle-Pixel ImagingCHEN Ruilin ZHANG Bo DUAN Xikai SUN Mingjie*(School of Instrument Science and Optoelectronics Engineering, Beijing University of Aeronautics and Astronautics,Beijing 100191, China)Abstract At present, one of the most important ways of earth remote sensing is to obtain target information through remote sensing cameras, but the resolution of remote sensing cameras directly affects the imaging quality. Combined with the pushbroom imaging technology of remote sensing camera, this paper proposes a super-resolution enhancement technology model based on single-pixel imaging, which can simplify the reconstruction process, and its design goal is to enhance the image resolution of aerospace remote sensing camera by 4 times based on single-pixel super-resolution technology. In order to verify the design idea and its reconstruction effect, the super-resolution enhancement simulation experiment is set up, and the final simulation results show that the single-pixel super-resolution model can improve the signal-to-noise ratio of the image by 1.1 times, and the reconstructed image has the obvious effect of suppressing noise, which plays a good noise reduction function, and has higher superiority than other收稿日期:2023-06-30基金项目:国家自然科学基金委项目(U21B2034)引用格式:陈瑞林, 章博, 段熙锴, 等. 基于单像素成像的遥感图像分辨率增强模型[J]. 航天返回与遥感, 2023, 44(6): 130-139.CHEN Ruilin, ZHANG Bo, DUAN Xikai, et al. Remote Sensing Image Resolution Enhancement Technology Based on Single-Pixel Imaging[J]. Spacecraft Recovery & Remote Sensing, 2023, 44(6): 130-139. (in Chinese)第6期陈瑞林等: 基于单像素成像的遥感图像分辨率增强模型 131traditional image resolution enhancement methods (such as bicubic interpolation and ultra-deep super-resolution neural network). This method can provide strong support for image processing and application in many fields, such as geographic remote sensing detection, land resources exploration and management, meteorological observation and prediction, and real-time assessment of target damage.Keywords single-pixel super-resolution; resolution enhancement; push-broom imaging; noise reduction effect; remote sensing application0 引言对地遥感成像的主要途径之一就是航天遥感相机,由于其具有覆盖范围广、成像速度快、风险低等优势,在国土资源管理、气象预报、地理测绘等领域发挥着举足轻重的作用。
图像超分辨率重建技术研究综述
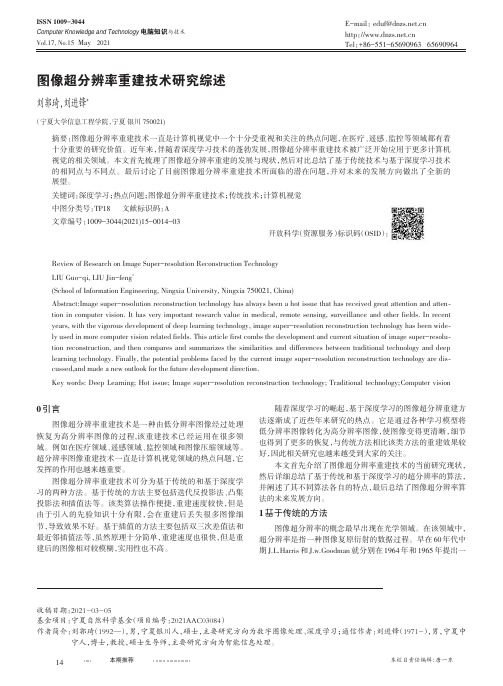
本栏目责任编辑:唐一东本期推荐图像超分辨率重建技术研究综述刘郭琦,刘进锋*(宁夏大学信息工程学院,宁夏银川750021)摘要:图像超分辨率重建技术一直是计算机视觉中一个十分受重视和关注的热点问题,在医疗、遥感、监控等领域都有着十分重要的研究价值。
近年来,伴随着深度学习技术的蓬勃发展,图像超分辨率重建技术被广泛开始应用于更多计算机视觉的相关领域。
本文首先梳理了图像超分辨率重建的发展与现状,然后对比总结了基于传统技术与基于深度学习技术的相同点与不同点。
最后讨论了目前图像超分辨率重建技术所面临的潜在问题,并对未来的发展方向做出了全新的展望。
关键词:深度学习;热点问题;图像超分辨率重建技术;传统技术;计算机视觉中图分类号:TP18文献标识码:A文章编号:1009-3044(2021)15-0014-03开放科学(资源服务)标识码(OSID ):Review of Research on Image Super-resolution Reconstruction Technology LIU Guo-qi,LIU Jin-feng *(School of Information Engineering,Ningxia University,Ningxia 750021,China)Abstract:Image super-resolution reconstruction technology has always been a hot issue that has received great attention and atten⁃tion in computer vision.It has very important research value in medical,remote sensing,surveillance and other fields.In recent years,with the vigorous development of deep learning technology,image super-resolution reconstruction technology has been wide⁃ly used in more computer vision related fields.This article first combs the development and current situation of image super-resolu⁃tion reconstruction,and then compares and summarizes the similarities and differences between traditional technology and deep learning technology.Finally,the potential problems faced by the current image super-resolution reconstruction technology are dis⁃cussed,and made a new outlook for the future development direction.Key words:Deep Learning;Hot issue;Image super-resolution reconstruction technology;Traditional technology;Computer vision0引言图像超分辨率重建技术是一种由低分辨率图像经过处理恢复为高分辨率图像的过程,该重建技术已经运用在很多领域。
通信常用缩略语大词典
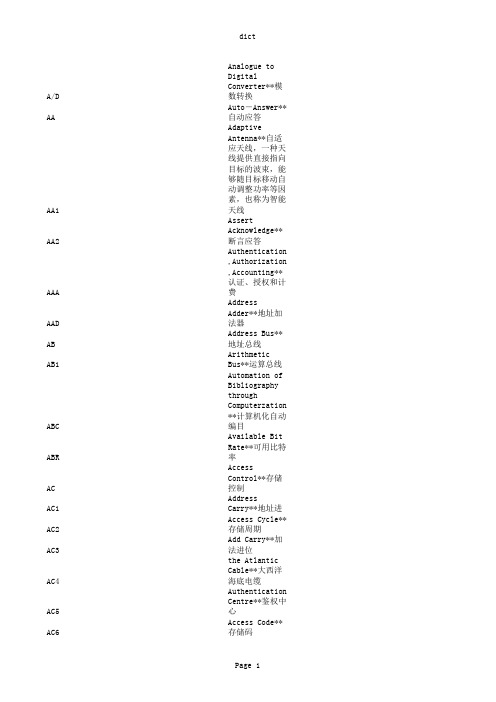
A/D Analogue to Digital Converter**模数转换AA Auto-Answer**自动应答AA1Adaptive Antenna**自适应天线,一种天线提供直接指向目标的波束,能AA2Assert Acknowledge**断言应答AAA Authentication,Authorization,Accounting**认证、授权和计费AAD Address Adder**地址加法器AB Address Bus**地址总线AB1Arithmetic Bus**运算总线ABC Automation of Bibliography through Computerzation**计算机化自动编ABR Available Bit Rate**可用比特率AC Access Control**存储控制AC1Address Carry**地址进位AC2Access Cycle**存储周期AC3Add Carry**加法进位AC4the Atlantic Cable**大西洋海底电缆AC5Authentication Centre**鉴权中心AC6Access Code**存储码ACCH Associated Control Channel**随路控制信道ACD Automatic Call Distribution**自动呼叫分配A-CDMA Asynchronous Code Division Multiple Access**异步码分多址ACI Adjacent-Channel Interference**邻频干扰ACIR Adjacent Channel Interference Ratio**邻信道干扰比ACK1ACKnowledgement**确认ACL Asynchronous Connectionless Link**异步无连接ACLR Adjacent Channel Leakage Ratio**邻信道泄漏比ACLR1Adjacent Channel Leakage Power Ratio**邻信道泄漏功率比ACLR2Adjacent Channel Leakage Ratio**邻信道泄漏比ACLR3Adjacent Channel Leakage Power Ratio**邻信道泄漏功率比acoustic amplitude logger acoustic amplitude logger**声波幅度测井仪acoustic amplitude logger1acoustic amplitude logger**声波幅度测井仪ACP Adjacent Channel Power**邻道功率ACP1Adjacent Channel Power**邻道功率acquisition time acquisition time**取数据时间acquisition time1acquisition time**取数据时间ACR Average Cell Rate**平均信元率ACR1Allowed Cell Rate**允许的信元率ACR2Average Cell Rate**平均信元率ACU Antenna Combining Unit**天线组合单元ACU1Acknowledgement Signal Unit**证实信号单元 表示正确地或ACU2Antenna Control Unit**天线控制器ADC Analog-to-Digital Converter**摸数转换器ADC;DAMPS North America digital cellular system**北美数字蜂窝系统ADFE Automatic Decree Feedback Equalizer**自适应判决反馈均衡器 一种利ADM Add Drop Multiplexer**分插复用器,一种新型的网络单元,将同步复用ADM1Add-Drop Multiplexer**上/下路复用器ADPCM Adaptive Differential Pulse Code Modulation**自适应差分脉冲编码调ADR Analogue-Digital Recorder**模拟-数字记录器ADR1Address Digit Receiver**数字地址接收器ADR2Advisory Route**建议路由ADR3Analogue Data Recognition**模拟数据识别ADRC Automatic Call Distribution Remote Capture**自动呼叫分配(分布)ADSL asymmetric digital subscriber line**不对称数字用户线ADSL2Asymetric Digital Subscriber Loop**非对称数字用户环路技术ADSS All Dielectric self-support**全介质自承式光缆 主要应用在强电场合AEB Analog Expansion Bus**模拟扩展总线AEIR Application Entity Installer and Remover**应用实体安装和拆除AFC Automatic Frequency Control**自动频率控制AFT Automatic Frequency Tune**自动频率调谐AG Audio Gateway**语音网关AGC Automatic Gain Control**自动增益控制AGCH Access Grant Channel**允许接续信道AIS Alarm Indication Signal**告警指示信号AIUR Air Interface User Rate**空中接口用户速率AJ Anti-Jamming**抗干扰AKA Authentication and Key Agreement**鉴权和密钥同意alarm summery panel alarm summery panel**报警汇总画面ALC Auto Level Control**自动电平控制ALS Automatic Laser Shutdown**自动激光关闭 在再生段光缆断开的情况下,ALT Automatic Link Transfer**自动链路转移AM/CM Administration Module/Communication Module**管理/通信模块AM1Amplitude Modulation**振幅调制AM-ADDR Active Member Address**活动的成员地址AMI Aliernate Mark Inversion Code**交替传号反转码AMI1Alternate Mark Inversion**信号交替反转码 一种数字传输中常用的编码AMPS Aduanced Mobile Phone System**高极移动电话系统AMPS 2Advanced Mobile Phone Institute**先进移动电话业务AMR Automatic Meter Reading**自动抄表AMR1Adaptive Multi Rate**自适应多速率AMRC Adaptive Multirate Codec**自适应多码率编译码器AN1Access Node**接入节点ANSI1American National Standard Institute**美国国家标准局AN-SMF AN system management function**接入网系统管理功能AN-SMF1Access Network System Management Function**接入网系统管理功能ANT1Antenna**天线AON Active Optical Network**有源光网络,属于一点对多点的光通信网络,AON1All Optical Net**全光网 就是网中直到端用户节点之间的信号通道仍然AON2All Optical Network**全光纤网络AOWC All Optical Wave Converter**全光波长转换器 是指不经过电域处理,直AP1Access Point**接入点APD1Avalanche Diode**雪崩光电二极管 利用雪崩倍增效应使光电流得到倍增APD2Avalanche Photo Detector**雪崩光电探测器API Application Programming Interface**应用编程接口APOC Advanced Paging Operator Code**先进寻呼操作码APON ATM Passive Optical Network**无源光网络,一种结合ATM多业务多比特APON1ATM-Based PON**ATM无源光网络APP A Posteriori Probability**后验概率APR1Automatic Programming and Recording**自动程序设计与记录APR2Automatic Position Reporting**自动位置报告APS Automatic Protection Switching**自动保护倒换APSK Absolute Phase Shift Keying**绝对相移键控AR Alternative Routing**迂回路由选择AR1Agent Request**代理请求AR2Access Rate**接入率ARA Average Response Amplitude**平均响应幅度AR-ADDR Access Request Address**访问请求地址ARB All Routes Busy**路由全忙ARC Automatic Remote Control**自动遥控ARC1Alternative Route Cancel**消除迂回路由ARDT Automatic Remote Data Terminal**自动远端数据终端ARF Access to the Resources Function**资源访问功能ARFCN Absolute Radio Frequency Channel Number**完全的无线频率信道号码ARFCN1Absolute RF Channel Number**绝对频道号 以整数表示的绝对射频信道号ARI Access Rights Identity**存取权识别ARIB Association of Radio Industry Businesses**无线行业企业协会ARL Acceptable Reliability Level**合格的可靠性(标准)ARM1Asynchronous Response Mode**异步应答方式ARP Address Resolution Protocol**地址解析协议ARPA Advanced Research Projects Agency**(美)高级研究计划署ARPANET Advanced Research Projects Agency Network**(美)高级研究计划署网络ARPANETq Advanced Research Projects Agency NETwork**(美国国防部)高级研究ARPS Advanced Real-Time Processing System**高级实时处理系统ARQ Automatic Repeat Request**自动重复请求ARS Address Resolution Server**地址解析服务器ART1Alarm Reporting Telephone**报警电话ARTq Average Restoration Time**平均恢复时间AS1Anti-Spoofing**反电子欺骗 通过对P码进行加密处理,防止非授权用户干ASCI Advanced Speed Call**GSM phaseII+ 标准指定的新业务,其中包括优先ASE Application Service Element**应用服务单元 在单个应用联系上对一指ASEAT Autoregressive Spectral Estimation Acquisition Technique**自回归ASIC Application Specific Integrated Circuit**专用集成电路ASK Amplitude Shift Keying**振幅键控(幅移键控)ASK1Amplitude Shift Keying,Amplitude Shift modulation**幅移键控ASN.1Abstract Syntax Notation One**抽象语法定义,在采用开放系统OSI通信ASON Automatic Switched Optical Network**自动交换光网络ASP1Application Service Provider**应用服务提供商ASR1Automatic Send-Receiver**自动收发机ASR2Automatic Send and Receive**自动收发ASR3Automatic Speech Recognition**自动语音识别ASR4Answer Seizure Ratio**应答占用率ASR5Access Service Request**访问业务请求ASRTE Alternative Select Route**迂回选择路由ASTN Automatic Switched Transport Network**自动交换传送网ATI Automatic Transmitter Identification**自动发射机识别ATM Alternative Test Method**(光纤、光缆)替代测试法(ATM)ATM 2Asynchronous Transfer Mode**异步传输模式ATM1asynachronous transfer model**同步传输模式ATME automatic transmission measuring and signalling testing equipmen atmosphere Personal Digital Cellular**个人数字蜂窝系统ATPC Automatic Transfer Power Control**自动发信功率控制 技术的要点是微ATR Asynchronous Transfer Region**异步转移(传递)区ATR1Answer To Reset**回答到复位ATR2Answering Time Recorder**应答时间记录器ATR3Automatic Traffic Recorder**自动话务量记录器ATU Adapter Unit**适配单元ATU-C ADSL transceiver unit,central office end**ADSL局端发送接收单元 位ATU-T ADSL transceiver unit,remote terminal end**ADSL远端发送接收单元 ATU-T1ADSL transceiver unit,remote terminal end**ADSL远端发送接收单元ATX AteXternal**是由Intel 公司首创以提升微机主板整体性能的AU Access Unit**访问单元AU1Administrative Unit**管理单元,提供高阶通道层和复用段层之间适配功AUC Authentication Center**鉴权中心AUG Administrative Unit Group**管理单元组AUL Average Useful Life**平均使用寿命AU-n Administrative Unit-n**管理单元auroral Packet Randem Access Channel**分组随机接入信道AVL Average Voice Level**平均话音电平AVL1Automatic Vehicle Location system**自动车辆定位系统 车队的某一车AWF All Wave Fiber**全波光纤 消除了光纤1383nm的水峰,这样就在1350-1 AWG Array Waveguide Grating**阵列波导光栅AWG1array wave guide**阵列式波导光栅AWGN Additive White Gaussian Noise**加性高斯白噪声BA Booster(power) Amplifier**光功率放大器 可补偿光复用器的损耗,提高BA1Booster Amplifier**光功率放大器,可补偿光复用器的损耗,提高入纤功BAIC Barring of All Incoming Calls supplementary service**限制所有呼入BAOC Barring of All Outgoing Calls supplementary service**限制所有呼出BB Baseband**基带BBER Background Block Error Ratio**背景误块比 对于一个确定的测试时间而BBS Bulletion Board System**电子公告栏BC Billing Center**计费中心BCC Base Transceiver Station (BTS) Colour Code**基站代码BCCH Broadcast Control Channel**广播控制信道BCD Binary Coded Decimal**二进制编码的十进制BCH Bose,Chaudhuri&Hocquenghem Type of code**博斯-乔赫里-霍克文黑姆BCH1Broadcast Channel**广播信道BCJR Bahl-Cocke-Jelinek-Raviv**算法BCP BTS Control Processor**BTS控制处理器BDPSK Binary Differential Phase Shift Keying**二相差分相移键控BER BIT ERROR RATIO**误比特率 二值信号的差错比值。
光学成像中的超分辨率技术研究

光学成像中的超分辨率技术研究超分辨率(Super-resolution)技术是一项光学成像中的重要研究领域,其目标是通过利用图像处理方法,从低分辨率图像中恢复出高分辨率的细节信息。
超分辨率技术在许多领域中都有广泛的应用,例如医学成像、军事监控、航天遥感等。
在本文中,将介绍超分辨率技术的原理和常见方法,并讨论其在光学成像中的应用。
超分辨率技术的原理是基于信号处理和图像处理的理论,旨在通过从多个低分辨率图像中提取高频细节信息来增加图像的分辨率。
常见的超分辨率技术包括插值方法、子像素运动估计和重建方法、基于学习的方法等。
插值方法是最简单也是最常用的超分辨率技术之一、它通过对低分辨率图像进行插值操作,使其像素数量增加,从而增加图像的分辨率。
常见的插值方法有最邻近插值、双线性插值和双三次插值等。
这些方法的缺点是在增加分辨率的同时会引入模糊和伪像等问题。
子像素运动估计和重建方法是一种通过对多张低分辨率图像进行像素级别的运动估计和像素插值操作来恢复高分辨率图像的方法。
运动估计过程中,算法会根据图像的模糊程度和几何变换等因素来估计像素的位移信息。
重建过程中,算法会根据估计的位移信息对低分辨率图像进行插值操作,从而恢复出高分辨率图像。
这种方法在处理连续运动物体的图像时效果较好,但在存在遮挡物或非刚性运动的情况下效果较差。
基于学习的方法是一种利用机器学习算法从大量训练样本中学习低分辨率图像与高分辨率图像之间的映射关系,并使用学习到的映射关系对新的低分辨率图像进行超分辨率的方法。
常见的学习方法包括基于插值的方法、基于统计的方法和基于正则化的方法等。
这些方法的优点是能够利用大量的训练样本,提高图像的重建质量和细节恢复能力。
超分辨率技术在光学成像中具有重要的应用意义。
例如,在医学成像中,超分辨率技术可以提高病理切片的图像质量,帮助医生更准确地诊断和治疗疾病。
在军事监控中,超分辨率技术可以提高图像的清晰度和细节恢复能力,帮助军事人员更准确地判断和分析目标情况。
Image Super-Resolution as Sparse Representation of Raw Image Patches
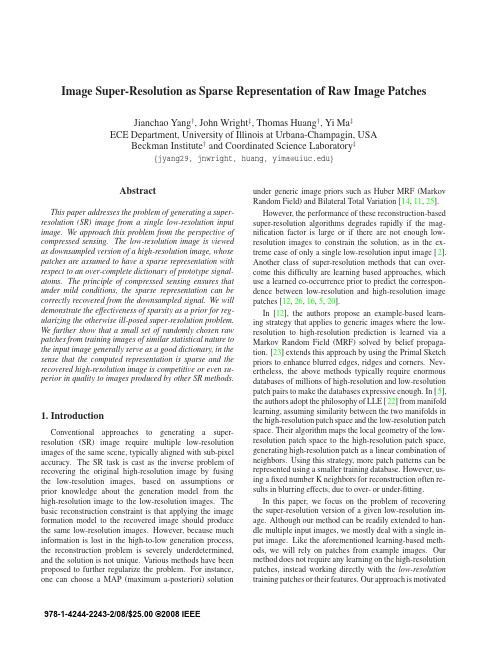
Image Super-Resolution as Sparse Representation of Raw Image Patches Jianchao Yang†,John Wright‡,Thomas Huang†,Yi Ma‡ECE Department,University of Illinois at Urbana-Champagin,USABeckman Institute†and Coordinated Science Laboratory‡{jyang29,jnwright,huang,yima@}AbstractThis paper addresses the problem of generating a super-resolution(SR)image from a single low-resolution input image.We approach this problem from the perspective of compressed sensing.The low-resolution image is viewed as downsampled version of a high-resolution image,whose patches are assumed to have a sparse representation with respect to an over-complete dictionary of prototype signal-atoms.The principle of compressed sensing ensures that under mild conditions,the sparse representation can be correctly recovered from the downsampled signal.We will demonstrate the effectiveness of sparsity as a prior for reg-ularizing the otherwise ill-posed super-resolution problem. We further show that a small set of randomly chosen raw patches from training images of similar statistical nature to the input image generally serve as a good dictionary,in the sense that the computed representation is sparse and the recovered high-resolution image is competitive or even su-perior in quality to images produced by other SR methods.1.IntroductionConventional approaches to generating a super-resolution(SR)image require multiple low-resolution images of the same scene,typically aligned with sub-pixel accuracy.The SR task is cast as the inverse problem of recovering the original high-resolution image by fusing the low-resolution images,based on assumptions or prior knowledge about the generation model from the high-resolution image to the low-resolution images.The basic reconstruction constraint is that applying the image formation model to the recovered image should produce the same low-resolution images.However,because much information is lost in the high-to-low generation process, the reconstruction problem is severely underdetermined, and the solution is not unique.Various methods have been proposed to further regularize the problem.For instance, one can choose a MAP(maximum a-posteriori)solutionunder generic image priors such as Huber MRF(Markov Random Field)and Bilateral Total Variation[14,11,25].However,the performance of these reconstruction-based super-resolution algorithms degrades rapidly if the mag-nification factor is large or if there are not enough low-resolution images to constrain the solution,as in the ex-treme case of only a single low-resolution input image[2].Another class of super-resolution methods that can over-come this difficulty are learning based approaches,which use a learned co-occurrence prior to predict the correspon-dence between low-resolution and high-resolution image patches[12,26,16,5,20].In[12],the authors propose an example-based learn-ing strategy that applies to generic images where the low-resolution to high-resolution prediction is learned via a Markov Random Field(MRF)solved by belief propaga-tion.[23]extends this approach by using the Primal Sketch priors to enhance blurred edges,ridges and corners.Nev-ertheless,the above methods typically require enormous databases of millions of high-resolution and low-resolution patch pairs to make the databases expressive enough.In[5], the authors adopt the philosophy of LLE[22]from manifold learning,assuming similarity between the two manifolds in the high-resolution patch space and the low-resolution patch space.Their algorithm maps the local geometry of the low-resolution patch space to the high-resolution patch space, generating high-resolution patch as a linear combination of ing this strategy,more patch patterns can be represented using a smaller training database.However,us-ing afixed number K neighbors for reconstruction often re-sults in blurring effects,due to over-or under-fitting.In this paper,we focus on the problem of recovering the super-resolution version of a given low-resolution im-age.Although our method can be readily extended to han-dle multiple input images,we mostly deal with a single in-put image.Like the aforementioned learning-based meth-ods,we will rely on patches from example images.Our method does not require any learning on the high-resolution patches,instead working directly with the low-resolution training patches or their features.Our approach is motivated 1978-1-4244-2243-2/08/$25.00 ©2008 IEEEFigure1.Reconstruction of a raccoon face with magnification fac-tor2.Left:result by our method.Right:the original image.Thereis little noticeable difference.by recent results in sparse signal representation,which en-sure that linear relationships among high-resolution signals can be precisely recovered from their low-dimensional pro-jections[3,9].To be more precise,let D∈R n×K be an overcompletedictionary of K prototype signal-atoms,and suppose a sig-nal x∈R n can be represented as a sparse linear combi-nation of these atoms.That is,the signal vector x can bewritten as x=Dα0whereα0∈R K is a vector with very few( K)nonzero entries.In practice,we might observeonly a small set of measurements y of x:y.=L x=L Dα0,(1)where L∈R k×n with k<n.In the super-resolution context,x is a high-resolution image(patch),while y is its low-resolution version(or features extracted from it).If the dictionary D is overcomplete,the equation x=Dαis underdetermined for the unknown coefficientsα.The equa-tion y=L Dαis even more dramatically underdetermined. Nevertheless,under mild conditions,the sparsest solution α0to this equation is unique.Furthermore,if D satisfies an appropriate near-isometry condition,then for a wide va-riety of matrices L,any sufficiently sparse linear represen-tation of a high-resolution image x in terms of the D can be recovered(almost)perfectly from the low-resolution image [9,21].Figure1shows an example that demonstrates the capabilities of our method derived from this principle.Even for this complicated texture,sparse representation recovers a visually appealing reconstruction of the original signal.Recently sparse representation has been applied to many other related inverse problems in image processing,such as compression,denoising[10],and restoration[17],often improving on the state-of-the-art.For example in[10],the authors use the K-SVD algorithm[1]to learn an overcom-plete dictionary from natural image patches and success-fully apply it to the image denoising problem.In our set-ting,we do not directly compute the sparse representation of the high-resolution patch.Instead,we will work with two coupled dictionaries,D for high-resolution patches,and D =L D for low-resolution patches.The sparse repre-sentation of a low-resolution patch in terms of D will be directly used to recover the corresponding high-resolution patch from D .We obtain a locally consistent solution by allowing patches to overlap and demanding that the recon-structed high-resolution patches agree on the overlapped ar-eas.Finally,we apply global optimization to eliminate the reconstruction errors in the recovered high-resolution im-age from local sparse representation,suppressing noise and ensuring consistency with the low-resolution input.Compared to the aforementioned learning-based meth-ods,our algorithm requires a much smaller database.The online recovery of the sparse representation uses the low-resolution dictionary only–the high-resolution dictionary is used only to calculate thefinal high-resolution image. The computation,mainly based on linear programming,is reasonably efficient and scalable.In addition,the computed sparse representation adaptively selects the most relevant patches in the dictionary to best represent each patch of the given low-resolution image.This leads to superior perfor-mance,both qualitatively and quantitatively,compared to methods[5]that use afixed number of nearest neighbors, generating sharper edges and clearer textures.The remainder of this paper is organized as follows.Sec-tion2details our formulation and solution to the image super-resolution problem based on sparse representation.In Section3,we discuss how to prepare a dictionary from sam-ple images and what features to use.Various experimental results in Section4demonstrate the efficacy of sparsity as a prior for image super-resolution.2.Super-resolution from SparsityThe single-image super-resolution problem asks:given a low-resolution image Y,recover a higher-resolution image X of the same scene.The fundamental constraint is that the recovered X should be consistent with the input,Y:Reconstruction constraint.The observed low-resolution image Y is a blurred and downsampled version of the solu-tion X:Y=DH X(2) Here,H represents a blurringfilter,and D the downsam-pling operator.Super-resolution remains extremely ill-posed,since for a given low-resolution input Y,infinitely many high-resolution images X satisfy the above reconstruction con-straint.We regularize the problem via the following prior on small patches x of X:Sparse representation prior.The patches x of the high-resolution image X can be represented as a sparse linear combination in a dictionary D of high-resolution patches sampled from training images:1x≈D αfor someα∈R K with α 0 K.(3) 1Similar mechanisms–sparse coding with an overcomplete dictionary –are also believed to be employed by the human visual system[19].To address the super-resolution problem using the sparse representation prior,we divide the problem into two steps. First,using the sparse prior(3),wefind the sparse repre-sentation for each local patch,respecting spatial compati-bility between neighbors.Next,using the result from this local sparse representation,we further regularize and refine the entire image using the reconstruction constraint(2).In this strategy,a local model from the sparse prior is used to recover lost high-frequency for local details.The global model from the reconstruction constraint is then applied to remove possible artifacts from thefirst step and make the image more consistent and natural.2.1.Local Model from Sparse RepresentationAs in the patch-based methods mentioned previously, we try to infer the high-resolution patch for each low-resolution patch from the input.For this local model,we have two dictionaries D and D :D is composed of high-resolution patches and D is composed of corresponding low-resolution patches.We subtract the mean pixel value for each patch,so that the dictionary represents image tex-tures rather than absolute intensities.For each input low-resolution patch y,wefind a sparse representation with respect to D .The corresponding high-resolution patches D will be combined according to these coefficients to generate the output high-resolution patch x. The problem offinding the sparsest representation of y can be formulated as:min α 0s.t. F D α−F y 22≤ ,(4) where F is a(linear)feature extraction operator.The main role of F in(4)is to provide a perceptually meaningful con-straint2on how closely the coefficientsαmust approximate y.We will discuss the choice of F in Section3.Although the optimization problem(4)is NP-hard in general,recent results[7,8]indicate that as long as the desired coefficientsαare sufficiently sparse,they can be efficiently recovered by instead minimizing the 1-norm,as follows:min α 1s.t. F D α−F y 22≤ .(5) Lagrange multipliers offer an equivalent formulationminλ α 1+12 F D α−F y 22,(6) where the parameterλbalances sparsity of the solution and fidelity of the approximation to y.Notice that this is es-sentially a linear regression regularized with 1-norm on the coefficients,known in statistical literature as the Lasso[24].2Traditionally,one would seek the sparsestαs.t. D α−y 2≤ . For super-resolution,it is more appropriate to replace this2-norm with a quadratic norm · F T F that penalizes visually salient high-frequency errors.Solving(6)individually for each patch does not guar-antee compatibility between adjacent patches.We enforce compatibility between adjacent patches using a one-passalgorithm similar to that of[13].3The patches are pro-cessed in raster-scan order in the image,from left to right and top to bottom.We modify(5)so that the super-resolution reconstruction D αof patch y is constrained to closely agree with the previously computed adjacent high-resolution patches.The resulting optimization problem is min α 1s.t. F D α−F y 22≤ 1P D α−w 22≤ 2,(7)where the matrix P extracts the region of overlap be-tween current target patch and previously reconstructed high-resolution image,and w contains the values of the pre-viously reconstructed high-resolution image on the overlap. The constrained optimization(7)can be similarly reformu-lated as:minλ α 1+12 ˜Dα−˜y 22,(8) where˜D=F DβP Dand˜y=F yβw.The parameterβcontrols the tradeoff between matching the low-resolution input andfinding a high-resolution patch that is compatible with its neighbors.In all our experiments,we simply set β=1.Given the optimal solutionα∗to(8),the high-resolution patch can be reconstructed as x=D α∗.2.2.Enforcing Global Reconstruction ConstraintNotice that(5)and(7)do not demand exact equality between the low-resolution patch y and its reconstruction D α.Because of this,and also because of noise,the high-resolution image X0produced by the sparse repre-sentation approach of the previous section may not satisfy the reconstruction constraint(2)exactly.We eliminate this discrepency by projecting X0onto the solution space of DH X=Y,computingX∗=arg minXX−X0 s.t.DH X=Y.(9) The solution to this optimization problem can be efficiently computed using the back-projection method,originally de-veloped in computer tomography and applied to super-resolution in[15,4].The update equation for this iterative method isX t+1=X t+((Y−DH X t)↑s)∗p,(10) where X t is the estimate of the high-resolution image af-ter the t-th iteration,p is a“backprojection”filter,and↑s denotes upsampling by a factor of s.3There are different ways to enforce compatibility.In[5],the values in the overlapped regions are simply averaged,which will result in blurring effects.The one-pass algorithm[13]is shown to work almost as well as the use of a full MRF model[12].Algorithm1(Super-resolution via Sparse Representation). 1:Input:training dictionaries D and D ,a low-resolution image Y.2:for each3×3patch y of Y,taken starting from the upper-left corner with1pixel overlap in each direction,•Solve the optimization problem with˜D and˜y de-fined in(8):minλ α 1+12 ˜Dα−˜y 22.•Generate the high-resolution patch x=D α∗.Put the patch x into a high-resolution image X0. 3:end4:Using back-projection,find the closest image to X0 which satisfies the reconstruction constraint:X∗=arg minXX−X0 s.t.DH X=Y. 5:Output:super-resolution image X∗.We take result X∗from backprojection as ourfinal es-timate of the high-resolution image.This image is as close as possible to the initial super-resolution X0given by spar-sity,while satisfying the reconstruction constraint.The en-tire super-resolution process is summarized as Algorithm1.2.3.Global Optimization InterpretationThe simple SR algorithm outlined above can be viewed as a special case of a general sparse representation frame-work for inverse problems in image processing.Related ideas have been profitably applied in image compression, denoising[10],and restoration[17].These connections provide context for understanding our work,and also sug-gest means of further improving the performance,at the cost of increased computational complexity.Given sufficient computational resources,one could in principle solve for the coefficients associated with all patches simultaneously.Moreover,the entire high-resolution image X itself can be treated as a variable. Rather than demanding that X be perfectly reproduced by the sparse coefficientsα,we can penalize the difference be-tween X and the high-resolution image given by these co-efficients,allowing solutions that are not perfectly sparse, but better satisfy the reconstruction constraints.This leads to a large optimization problem:X∗=arg minX,{αij}DH X−Y 22+ηi,jαij 0+γi,j D αij−P ij X 22+τρ(X).(11)Here,αij denotes the representation coefficients for the (i,j)th patch of X,and P ij is a projection matrix that se-lects the(i,j)th patch from X.ρ(X)is a penalty function that encodes prior knowledge about the high-resolution im-age.This function may depend on the image category,or may take the form of a generic regularization term(e.g., Huber MRF,Total Variation,Bilateral Total Variation).Algorithm1can be interpreted as a computationally effi-cient approximation to(11).The sparse representation step recovers the coefficientsαby approximately minimizing the sum of the second and third terms of(11).The sparsity term αij 0is relaxed to αij 1,while the high-resolution fidelity term D αij−P ij X 2is approximated by its low-resolution version F D αij−F y ij 2.Notice,that if the sparse coefficientsαarefixed,the third term of(11)essentially penalizes the difference be-tween the super-resolution image X and the reconstruc-tion given by the coefficients:i,jD αij−P ij X 22≈ X0−X 22.Hence,for smallγ,the back-projection step of Algorithm1approximately minimizes the sum of thefirst and third terms of(11).Algorithm1does not,however,incorporate any prior be-sides sparsity of the representation coefficients–the term ρ(X)is absent in our approximation.In Section4we will see that sparsity in a relevant dictionary is a strong enough prior that we can already achieve good super-resolution per-formance.Nevertheless,in settings where further assump-tions on the high-resolution signal are available,these pri-ors can be incorperated into the global reconstruction step of our algorithm.3.Dictionary Preparation3.1.Random Raw Patches from Training ImagesLearning an over-complete dictionary capable of opti-mally representing broad classes of image patches is a dif-ficult problem.Rather than trying to learn such a dictionary [19,1]or using a generic set of basis vectors[21](e.g., Fourier,Haar,curvelets etc.),we generate dictionaries by simply randomly sampling raw patches from training im-ages of similar statistical nature.We will demonstrate that so simply prepared dictionaries are already capable of gen-erating high-quality reconstructions,4when used together with the sparse representation prior.Figure2shows several training images and the patches sampled from them.For our experiments,we prepared two dictionaries:one sampled fromflowers(Figure2top), which will be applied to generic images with relative sim-ple textures,and one sampled from animal images(Figure 2bottom),withfine furry or fractal textures.For each high-resolution training image X,we generate the correspond-ing low-resolution image Y by blurring and downsampling. For each category of images,we sample only about100,000 patches from about30training images to form each dic-tionary,which is considerably smaller than that needed by 4The competitiveness of such random patches has also been noticed empirically in the context of content-based image classification[18].Figure2.Left:three out of the30training images we use in our experiments.Right:the training patches extracted from them.Figure4.Theflower and girl image magnified by a factor of3.Left to right:input,bicubic interpolation,neighbor embedding[5],our method,and the original.(Also see Figure8for the same girl image magnified by a factor of4).Figure5.Results on an image of the Parthenon with magnification factor3.Top row:low-resolution input,bicubic interpolation,back projection.Bottom row:neighbor embedding[5],soft edge prior[6],and our method.proposed method based on a learned soft edge prior[6].Theresult from back projection has many jagged effects alongthe edges.Neighbor embedding generates sharp edges inplaces,but blurs the texture on the temple’s facade.Thesoft edge prior method gives a decent reconstruction,butintroduces undesired smoothing that is not present in ourresult.Additional results on generic images using this dic-tionary are shown in Figure7left and center.Notice that inboth cases,the algorithm significantly improves the imageresolution by sharpening edges and textures.We now conduct more challenging experiments on moreintricate textures found in animal images,using the ani-mal dictionary with merely100,000training patches(sec-ond row of Figure2).As already shown in Figure1,ourmethod performs quite well in magnifying the image of araccoon face by a factor of2.When complex textures suchas this one are down-sampled further,the SR task will be-come more difficult than on images with simpler textures,such asflowers or faces.In Figure6,we apply our methodto the same raccoon face image with magnification factor3.Since there are no explicit edges in most part of the image,methods proposed in[12],[23],and[6]would have tremen-dous difficulty pared to neighbor embedding[5],our method gives clearer fur and sharper whiskers.Figure7shows an additional image of a cat face reconstructed usingthis dictionary.We compare several SR methods quantita-tively in terms of their RMS errors for some of the imagesshown above.The results are shown in Table1.Finally,we test our algorithm on the girl image again,butwith a more challenging magnification factor4.The resultsare shown in Figure8.Here,back-projection again yieldsjagged edges.Freeman et.al’s method[12]introduces manyartifacts and fails to capture the facial texture,despite rely-ing on a much larger pared to the soft edgeFigure6.A raccoon face magnified by a factor of3.The input image,bicubic interpolation,neighbor embedding,and our method.Figure7.More results on a few more generic(left and center)and animal(right)images.Top:input images.Bottom:super-resolution images by our method,with magnification factor3.Figure8.The girl image magnified by a factor of4.From left to right:low-resolution input,back projection,learning-based method in [12],soft edge prior[6],and our method.Images Bicubic NE[5]Our methodFlower 3.5052 4.1972 3.2276Girl 5.9033 6.6588 5.6175Parthenon12.743113.556212.2491Raccoon9.73999.84909.1874Table1.The RMS errors of different methods for super-resolution with magnification factor3,respect to the original images.prior method[6],our method generates shaper edges and is more faithful to the original facial texture.5.DiscussionThe experimental results of the previous section demon-strate the effectiveness of sparsity as a prior for patch-based super-resolution.However,one of the most important questions for future investigation is to determine,in terms of the within-category variation,the number of raw sam-ple patches required to generate a dictionary satisfying the sparse representation prior.Tighter connections to the the-ory of compressed sensing may also yield conditions on the appropriate patch size or feature dimension. AcknowledgementThis work was partially supported by the following grants:NSF EHS-0509151,NSF CCF-0514955,ONR YIP N00014-05-1-0633,NSF IIS07-03756,and ern-ment V ACE Program.References[1]M.Aharon,M.Elad,and A.Bruckstein.K-SVD:An algo-rithm for designing overcomplete dictionaries for sparse rep-resentation.IEEE Transactions on Signal Processing,V ol.54,No.11,Novermber2006.2,4[2]S.Baker and T.Kanade.Limits on super-resolution and howto break them.IEEE TPAMI,24(9):1167-1183,2002.1 [3] pressive sensing.Proc.InternationalCongress of Mathematicians,2006.2[4] D.Capel.Image mosaicing and super-resolution.Ph.D.Thesis,Department of Eng.Science,University of Oxford, 2001.3[5]H.Chang,D.-Y.Yeung,and Y.Xiong.Super-resolutionthrough neighbor embedding.CVPR,2004.1,2,3,5,6, 8[6]S.Dai,M.Han,W.Xu,Y.Wu,and Y.Gong.Soft edgesmoothness prior for alpha channel super resolution Proc.ICCV,2007.5,6,7,8[7] D.L.Donoho.For most large underdetermined systems oflinear equations,the minimal 1-norm solution is also the sparsest m.on Pure and Applied Math,V ol.59,No.6,2006.3[8] D.L.Donoho.For most large underdetermined systemsof linear equations,the minimal 1-norm near-solution ap-proximates the sparsest near-solution.Preprint,accessed at /˜donoho/.2004.3 [9] pressed sensing.Preprint,accessed at/˜donoho/.2005.2 [10]M.Elad and M.Aharon.Image denoising via sparse andredundant representations over learned dictionaries.IEEE TIP,V ol.15,No.12,2006.2,4[11]S.Farsiu,M.D.Robinson,M.Elad,and anfar.Fastand robust multiframe super-resolution.IEEE TIP,2004.1 [12]W.T.Freeman,E.C.Pasztor,and O.T.Carmichael.Learn-ing low-level vision.IJCV,2000.1,3,5,6,7[13]W.T.Freeman,T.R.Jones,and E.C.Pasztor.Example-based super-resolution.IEEE Computer Graphics and Ap-plications,V ol.22,Issue2,2002.3[14]R.C.Hardie,K.J.Barnard,and E.A.Armstrong.Joint MAPregistration and high-resolution image estimation using a se-quence of undersampled images.IEEE TIP,1997.1 [15]M.Irani and S.Peleg.Motion analysis for image enhance-ment:resolution,occlusion and transparency.JVCI,1993.3[16] C.Liu,H.Y.Shum,and W.T.Freeman.Face hallucina-tion:theory and practice.IJCV,V ol.75,No.1,pp.115-134, October,2007.1[17]J.Mairal,G.Sapiro,and M.Elad.Learning multi-scale sparse representations for image and video restoration.SIAM Multiscale Modeling and Simulation,2008.2,4 [18] E.Nowak,F.Jurie,and B.Triggs.Sampling strategies forbag-of-features image classification.Proc.ECCV,2006.4 [19] B.Olshausen and D.Field.Sparse coding wih an overcom-plete basis set:A strategy employed by V1?Vision Re-search,37:3311-3325,1997.2,4[20]L.C.Pickup,S.J.Roberts,and A.Zisserman.A sampledtexture prior for image super-resolution.Proc.NIPS,2003.1[21]H.Rauhut,K.Schnass,and pressedsensing and redundant dictionaries.Preprint,accessed at http://homepage.univie.ac.at/holger.rauhut/.2007.2,4[22]S.T.Roweis and L.K.Saul.Nonlinear dimensionality re-duction by locally linear embedding.Science,290(5500): 2323-2326,2000.1[23]J.Sun,N.-N.Zheng,H.Tao,and H.Shum.Image halluci-nation with primal sketch priors.Proc.CVPR,2003.1,5, 6[24]R.Tibshirani.Regression shrinkge and selection via theLasso.J.Royal Statist.Soc B.,V ol.58,No.1,pages267-288,1996.3[25]M.E.Tipping and C.M.Bishop.Bayesian image super-resolution.Proc.NIPS,2003.1[26]Q.Wang,X.Tang,and H.Shum.Patch based blind imagesuper resolution.Proc.ICCV,2005.1。
一种有效的图像复原方法
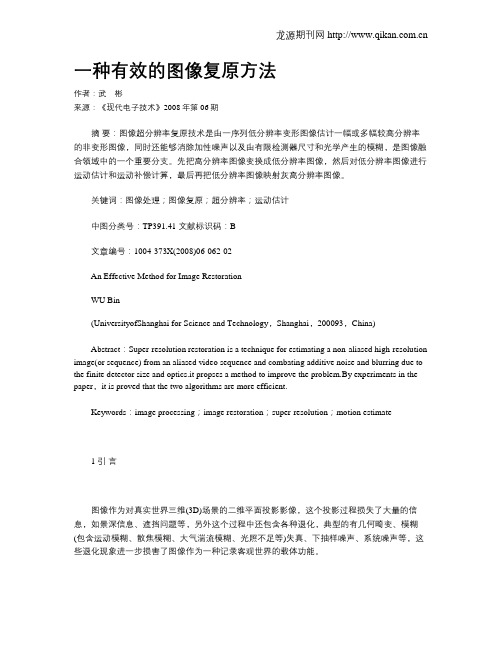
一种有效的图像复原方法作者:武彬来源:《现代电子技术》2008年第06期摘要:图像超分辨率复原技术是由一序列低分辨率变形图像估计一幅或多幅较高分辨率的非变形图像,同时还能够消除加性噪声以及由有限检测器尺寸和光学产生的模糊,是图像融合领域中的一个重要分支。
先把高分辨率图像变换成低分辨率图像,然后对低分辨率图像进行运动估计和运动补偿计算,最后再把低分辨率图像映射灰高分辨率图像。
关键词:图像处理;图像复原;超分辨率;运动估计中图分类号:TP391.41 文献标识码:B文章编号:1004-373X(2008)06-062-02An Effective Method for Image RestorationWU Bin(UniversityofShanghai for Science and Technology,Shanghai,200093,China)Abstract:Super-resolution restoration is a technique for estimating a non-aliased high-resolution image(or sequence) from an aliased video sequence and combating additive noise and blurring due to the finite detector size and optics.it propses a method to improve the problem.By experiments in the paper,it is proved that the two algorithms are more efficient.Keywords:image processing;image restoration;super-resolution;motion estimate1 引言图像作为对真实世界三维(3D)场景的二维平面投影影像,这个投影过程损失了大量的信息,如景深信息、遮挡问题等,另外这个过程中还包含各种退化,典型的有几何畸变、模糊(包含运动模糊、散焦模糊、大气湍流模糊、光照不足等)失真、下抽样噪声、系统噪声等,这些退化现象进一步损害了图像作为一种记录客观世界的载体功能。
基于深度卷积残差学习的图像超分辨
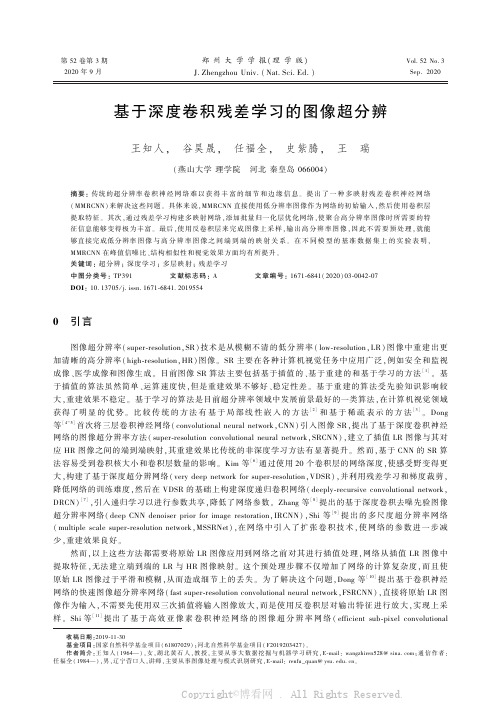
㊀第52卷第3期郑州大学学报(理学版)Vol.52No.3㊀2020年9月J.Zhengzhou Univ.(Nat.Sci.Ed.)Sep.2020收稿日期:2019-11-30基金项目:国家自然科学基金项目(61807029);河北自然科学基金项目(F2019203427)㊂作者简介:王知人(1964 ),女,湖北黄石人,教授,主要从事大数据挖掘与机器学习研究,E-mail:wangzhiren528@;通信作者:任福全(1984 ),男,辽宁营口人,讲师,主要从事图像处理与模式识别研究,E-mail:renfu_quan@㊂基于深度卷积残差学习的图像超分辨王知人,㊀谷昊晟,㊀任福全,㊀史紫腾,㊀王㊀瑞(燕山大学理学院㊀河北秦皇岛066004)摘要:传统的超分辨率卷积神经网络难以获得丰富的细节和边缘信息㊂提出了一种多映射残差卷积神经网络(MMRCNN)来解决这些问题㊂具体来说,MMRCNN 直接使用低分辨率图像作为网络的初始输入,然后使用卷积层提取特征㊂其次,通过残差学习构建多映射网络,添加批量归一化层优化网络,使聚合高分辨率图像时所需要的特征信息能够变得极为丰富㊂最后,使用反卷积层来完成图像上采样,输出高分辨率图像,因此不需要预处理,就能够直接完成低分辨率图像与高分辨率图像之间端到端的映射关系㊂在不同模型的基准数据集上的实验表明,MMRCNN 在峰值信噪比㊁结构相似性和视觉效果方面均有所提升㊂关键词:超分辨;深度学习;多层映射;残差学习中图分类号:TP391㊀㊀㊀㊀㊀文献标志码:A㊀㊀㊀㊀㊀文章编号:1671-6841(2020)03-0042-07DOI :10.13705/j.issn.1671-6841.20195540㊀引言图像超分辨率(super-resolution,SR)技术是从模糊不清的低分辨率(low-resolution,LR)图像中重建出更加清晰的高分辨率(high-resolution,HR)图像㊂SR 主要在各种计算机视觉任务中应用广泛,例如安全和监视成像㊁医学成像和图像生成㊂目前图像SR 算法主要包括基于插值的㊁基于重建的和基于学习的方法[1]㊂基于插值的算法虽然简单㊁运算速度快,但是重建效果不够好㊁稳定性差㊂基于重建的算法受先验知识影响较大,重建效果不稳定㊂基于学习的算法是目前超分辨率领域中发展前景最好的一类算法,在计算机视觉领域获得了明显的优势㊂比较传统的方法有基于局部线性嵌入的方法[2]和基于稀疏表示的方法[3]㊂Dong 等[4-5]首次将三层卷积神经网络(convolutional neural network,CNN)引入图像SR,提出了基于深度卷积神经网络的图像超分辨率方法(super-resolution convolutional neural network,SRCNN),建立了插值LR 图像与其对应HR 图像之间的端到端映射,其重建效果比传统的非深度学习方法有显著提升㊂然而,基于CNN 的SR 算法容易受到卷积核大小和卷积层数量的影响㊂Kim 等[6]通过使用20个卷积层的网络深度,使感受野变得更大,构建了基于深度超分辨网络(very deep network for super-resolution,VDSR),并利用残差学习和梯度裁剪,降低网络的训练难度,然后在VDSR 的基础上构建深度递归卷积网络(deeply-recursive convolutional network,DRCN)[7],引入递归学习以进行参数共享,降低了网络参数㊂Zhang 等[8]提出的基于深度卷积去噪先验图像超分辨率网络(deep CNN denoiser prior for image restoration,IRCNN),Shi 等[9]提出的多尺度超分辨率网络(multiple scale super-resolution network,MSSRNet),在网络中引入了扩张卷积技术,使网络的参数进一步减少,重建效果良好㊂然而,以上这些方法都需要将原始LR 图像应用到网络之前对其进行插值处理,网络从插值LR 图像中提取特征,无法建立端到端的LR 与HR 图像映射㊂这个预处理步骤不仅增加了网络的计算复杂度,而且使原始LR 图像过于平滑和模糊,从而造成细节上的丢失㊂为了解决这个问题,Dong 等[10]提出基于卷积神经网络的快速图像超分辨率网络(fast super-resolution convolutional neural network,FSRCNN),直接将原始LR 图像作为输入,不需要先使用双三次插值将输入图像放大,而是使用反卷积层对输出特征进行放大,实现上采样㊂Shi 等[11]提出了基于高效亚像素卷积神经网络的图像超分辨率网络(efficient sub-pixel convolutional㊀第3期王知人,等:基于深度卷积残差学习的图像超分辨neural network,ESPCN),网络直接对LR 图像进行特征映射,并且引入了一个有效的亚像素卷积层,它的作用和反卷积层一样用来实现上采样㊂以上方法都是直接在低分辨率空间中进行映射,并通过引入反卷积层或亚像素卷积层对网络最后输出的低分辨率特征进行放大㊂这些操作使网络计算的复杂度大大减小,增加网络训练的效率㊂另一方面,这些网络都是以链式方式堆叠构建模块的㊂它们忽略了充分利用来自多层卷积层的信息,只考虑了卷积层最后输出的特征㊂每一个卷积层都会产生不同的独特特征㊂深层网络生成的分层特征对提高图像SR 重建效果具有很大作用㊂Ledig 等[12]首次将生成对抗网络用于图像超分辨率重建(super resolution generative networks,SRGAN),其中的生成网络部分利用残差网络(residual networks,ResNets)实现了信息层与层之间的传递㊂Tong 等[13]构建了基于密集网络的图像超分辨率网络(super-resolution dense network,SR-DenseNet),通过密集网络加强了特征传播,实现了多层特征的融合,进一步提升了重建效果㊂本文提出了一种多映射残差卷积神经网络(multi-mapping residual convolutional neural network,MMRC-NN),它使用深度网络作为基本框架,使用跳跃连接来显著增加映射阶段的输出特征数量,降低深度网络训练的难度㊂此外,本文引入批量归一化(batch normalization,BN),以提高MMRCNN 的模型训练性能㊂为了建立从原始LR 到HR 图像的端到端映射,避免额外的计算开销和重建伪像,本文选择在重建期间进行上采样而不是预处理㊂本文的创新点主要有3方面:1)提出了一个从原始LR 到HR 图像的端到端映射㊂直接对LR 图像进行训练,不进行预处理㊂计算成本较低,避免了重建伪像㊂2)利用残差学习建立多映射网络㊂不仅可以解决网络退化问题,而且可以使用多个分支映射来获得更丰富的鲁棒特征,克服了单一级联网络输出导致的特征集不足的弊端㊂3)使用批量归一化来优化网络㊂最后,本文的MMRCNN 在量化指标和视觉质量方面都能实现较好的效果㊂1㊀MMRCNN1.1㊀网络结构如图1所示,MMRCNN 可以分解为3个部分:特征提取㊁非线性映射和超分辨率重建㊂前两部分是卷积层(convolutional layer),用Conv 来表示,最后一部分是反卷积层(deconvolutional layer),用Deconv 来表示㊂下面为MMRCNN 模型的网络结构介绍㊂图1㊀MMRCNN 网络架构Figure 1㊀The architecture of MMRCNN network1.1.1㊀特征提取㊀第1层为Conv +PReLU 层,使用64个大小为5ˑ5ˑc 的滤波器来生成64个特征图,然后使用参数修正线性单元(parametric rectified linear unit,PReLU)进行非线性处理㊂这里c 表示图像通道的数量,灰度图像时c =1,彩色图像时c =3㊂1.1.2㊀非线性映射㊀第2~16层为Conv +BN +PReLU 层,使用64个尺寸为3ˑ3ˑ64的滤波器,并且在卷积与PReLU 之间添加批量归一化操作[14]㊂此外,将非线性映射中每3个卷积层分为1个单元,即将15个卷积层分为5个单元,这样可以使映射之后得到的特征与原有的特征存在一定的差异性㊂最后,对卷积层添加5条跳跃连接来建立多映射网络模型,保证每一个单元的输出可以直接与重建模块相连接,充分地提取图像的34郑州大学学报(理学版)第52卷内部信息㊂1.1.3㊀重建㊀最后一层为Deconv 层,反卷积层可以看作是卷积的逆过程㊂本文使用反卷积操作对图像进行上采样,用c 个大小为9ˑ9ˑ64的滤波器来重建输出㊂1.2㊀残差学习残差网络和跳跃连接由He 等[15]提出,这种网络结构使得深层网络的训练更加容易,从而可以提升深度网络的性能㊂图2为残差块的基本结构㊂图2㊀残差块的基本结构Figure 2㊀The basic structure of the residual block如图2所示,残差网络在卷积层上使用跳跃连接来建立层与层之间的连接㊂残差块函数定义为y =F (x ,{W i })+x ,(1)其中,x 和y 分别代表残差结构的输入和输出,函数F (x ,{W i })代表残差映射㊂深层网络会产生退化问题,这种情况是由冗余的网络层产生的㊂假设网络中的冗余层学习到的映射为恒等映射,即y =x ,那么深层网络就相当于变成了浅层网络,模型的训练效果会更好㊂残差网络的残差结构使原始要学习的y ,被表示成y =F (x )+x ,因此对恒等映射y =x 的学习,就转换为对残差函数F (x )=y -x 的学习㊂当F (x )=0时,就相当于拟合恒等映射函数y =x ,相比于用非线性层的堆叠来拟合恒等映射,网络层学习F (x )=0更为容易㊂利用这种残差学习策略,可以使深层卷积神经网络的训练变得更为容易,提升模型重建效果㊂本文对映射中的卷积层添加5条跳跃连接,建立多映射模型,充分提取每部分的特征信息,提高超分辨的效果㊂1.3㊀批量归一化本文在网络的每一层的非线性映射之前使用批量归一化[14]和缩放移位步骤,以减轻每个卷积层输入数据分布的变化,即减轻内部协变量偏移㊂批量归一化每次激活时仅添加两个参数,并且可以使用反向传播来更新参数㊂批量归一化具有训练速度快㊁性能好㊁初始化灵敏度低等优点㊂通过实验发现,在残差学习基础上使用批量归一化可以实现更好的超分辨率性能㊂2㊀实验2.1㊀实验设置数据集:本文参考了不同的基于学习的SR 方法的训练集㊂SRCNN 采用Yang 等[3]的91幅图像(91images)以及ImageNet 数据集两种训练集来训练㊂FSRCNN 使用General-100与91images 联合作为训练集㊂VDSR 使用291幅图像作为训练集,其中包括91images 及BSD200,并使用旋转以及缩放处理,来增加训练集㊂本文采用的训练集同样为291幅图像,包括91images㊁General-100和BSD100[16],并使用与VDSR 相同的方式进行数据增强㊂首先,将原有图像进行旋转90ʎ㊁180ʎ和270ʎ处理,这样数据量会增加为原先的4倍,然后再将旋转后的图像进行0.6㊁0.7㊁0.8和0.9倍的缩放处理㊂经过多角度的转换后,本文的训练数据变为原来的20倍,即291ˑ4ˑ5=5820张训练样本㊂测试阶段选取了图像集5(set5)[17]和图像集14(set14)[18]两个通用的数据集㊂实验细节:训练时,首先对训练图像进行随机剪裁,获得一系列33ˑ33像素的HR 子图像{X i },然后对子图像{X i }进行双三次下采样,缩到原图的1/3大小,得到一系列低分辨率图像,然后再次使用双三次上采样,放大3倍,得到与HR 图像样本{X i }相对应的LR 图像样本{Y i }㊂本文按照步长为14的大小从原始训练图像中提取样本,经过实验,更小的步幅没有带来明显的效果提升㊂从实验效果来看,训练集足以训练本文的深度网络㊂网络的最大迭代次数设置为1ˑ105,每种算法的放大倍数都选取为3倍㊂本文在训练过程中仅仅针对Y 通道进行训练㊂本文实验环境为Matlab2016a 和Caffe 深度学习框架㊂4454㊀第3期王知人,等:基于深度卷积残差学习的图像超分辨评价标准:本文选择峰值信噪比(peak signal-to-noise ratio,PSNR)和结构相似性(structural similarity, SSIM)[19]来作为评价图像重建质量的重要指标㊂两者数值越高,模型效果越好㊂2.2㊀实验结果与评价2.2.1㊀客观量化评价㊀本文在set5和set14这两个测试集上详细对比了Bicubic(BI)㊁ScSR[3]㊁KSVD[20]㊁ANR[1]㊁NE+NNLS[2]㊁NE+LLE[21]的PSNR值,具体数据如表1和表2所示㊂在整体数据集上对比了Bicu-bic㊁SRCNN-Ex[5]㊁FSRCNN[10]㊁VDSR[6]㊁MSSRNet[9]㊁ZSSR[22]的PSNR值和SSIM值,其中除Bicubic外,其他5种方法都是基于深度学习的图像超分辨方法,具体数据如表3所示㊂表1~3中最好的结果用粗体表示㊂表1㊀set5测试集上,不同图像超分辨率方法的PSNR对比Table1㊀The PSNR of different image super-resolution methods on the set5test set set5BI ScSR KSVD ANR NE+NNLS NE+LLE MMRCNN baby33.9134.2935.0835.0634.7735.0435.47 bird32.5734.1134.5734.4934.2634.5636.77 butterfly24.0425.5825.9425.8525.6225.7529.99 head32.8833.1733.5633.6333.4533.6233.99 woman28.5629.9430.3730.3329.8830.2232.41 average30.3931.4231.9031.8731.6031.8433.73表2㊀set14测试集上,不同图像超分辨率方法的PSNR对比Table2㊀The PSNR of different image super-resolution methods on the set14test set set14BI ScSR KSVD ANR NE+NNLS NE+LLE MMRCNN baboon23.2123.5023.5223.5623.4923.5523.80 barbara26.2526.3926.7626.6926.6626.7426.02 bridge24.4024.8025.0225.0124.8624.9825.46 coastguard26.5527.0027.1527.0827.0127.0727.51 comic23.1223.9023.9624.0423.8323.9825.09 face32.8233.1033.5333.6233.4533.5633.99 flowers27.2328.2528.4228.4928.2128.3030.03 foreman31.1632.0433.1933.2132.8733.2134.89 lenna31.6832.6433.0133.0832.8233.0133.96 man27.0127.7627.9027.9227.2027.8728.81 monarch29.4330.7131.1031.0930.7630.9534.66 pepper32.3833.3234.0733.8233.5633.8035.30 ppt323.7124.9825.2425.0324.8124.9327.85 zebra26.6327.9528.5028.4328.1228.3329.74 average27.5428.3128.6728.6528.4028.5929.79表3㊀set5和set14上,不同图像超分辨率方法的平均PSNR对比Table3㊀The average PSNR of different image super-resolution methods on the set5and set14 Datasets BI SRCNN-Ex FSRCNN VDSR MSSRNet ZSSR MMRCNNset530.39032.75033.16033.66033.38033.42033.7300.8680.9090.9140.9210.9180.9180.922set1427.54029.28029.43029.77029.57029.78029.7900.7740.8210.8240.8310.8280.8300.832㊀㊀由表1~3的实验数据可以看到:在set5数据集上,MMRCNN算法的重建质量要优于其他几种传统算法㊂在set14数据集上,除barbara图像略低于其他几种传统算法外,另外几种情况下的重建质量也要优于其他几种传统算法㊂在整体数据对比上,MMRCNN的重建效果比其他几种基于深度学习的算法较好㊂2.2.2㊀视觉效果㊀图3~8所示为部分测试图像的重建效果图,本文选择了6个图像来进行观察㊂这里只郑州大学学报(理学版)第52卷显示了BI㊁SRCNN-EX [5]㊁FSRCNN [10]和MMRCNN 的超分辨率重建图像㊂从视觉效果来看,MMRCNN 算法具有较好的重建效果,细节更完善,更接近原始图像㊂例如,由图3~6的局部放大图可以看到,MMRCNN 算法重建的图像更加清晰㊁噪声更少㊁细节更加完整㊂由图7~8的局部放大图可以看到,MMRCNN 算法的重建图像纹理更加清晰㊁边缘更加完整㊁锐度更强㊁与原图效果更逼近㊂图3㊀关于图像baby 的重建效果比较Figure 3㊀Comparison of reconstruction effect of imagebaby 图4㊀关于图像butterfly 的重建效果比较Figure 4㊀Comparison of reconstruction effect of imagebutterfly 图5㊀关于图像baboon 的重建效果比较Figure 5㊀Comparison of reconstruction effect of imagebaboon 图6㊀图像pepper 的重建效果比较Figure 6㊀Comparison of reconstruction effect of imagepepper 图7㊀图像zebra 的重建效果比较Figure 7㊀Comparison of reconstruction effect of image zebra64㊀第3期王知人,等:基于深度卷积残差学习的图像超分辨图8㊀关于图像ppt3的重建效果比较Figure 8㊀Comparison of reconstruction effect of image ppt33㊀结论本文提出了一种用于图像超分辨率的多映射残差卷积神经网络,有效地解决了传统方法中单一映射输出不能完全提取图像特征集的问题㊂该算法通过跳跃连接大大提高重建模块的训练特征集,提高超分辨率重建图像的质量㊂同时该算法集成了批量归一化,加快了训练过程,提高了超分辨率性能㊂实验结果表明,本文算法的重建效果在客观图像量化评价和视觉效果上均有所改善和提高,能够恢复更多的图像细节,具有更好的视觉效果㊂在未来,将继续研究合适的CNN 模型,进一步提高模型的重建性能㊂参考文献:[1]㊀TIMOFTE R,DE V,GOOL L V.Anchored neighborhood regression for fast example-based super-resolution[C]ʊ2013IEEEInternational Conference on Computer Vision.Australia,2013:1920-1927.[2]㊀CHANG H,YEUNG D Y,XIONG Y M.Super-resolution through neighbor embedding [C]ʊProceedings of the 2004IEEE Computer Society Conference on Computer Vision and Pattern Recognition.Washington,2004:272-282.[3]㊀YANG J C,WRIGHT J,HUANG T S,et al.Image super-resolution via sparse representation[J].IEEE transactions on image processing,2010,19(11):2861-2873.[4]㊀DONG C,LOY C C,HE K M,et al.Learning a deep convolutional network for image super-resolution [M ].Switzerland:Springer International Publishing,2014:184-199.[5]㊀DONG C,LOY C C,HE K M,et al.Image super-resolution using deep convolutional networks[J].IEEE transactions on pat-tern analysis and machine intelligence,2016,38(2):295-307.[6]㊀KIM J,LEE J K,LEE K M.Accurate image super-resolution using very deep convolutional networks[C]ʊ2016IEEE Confer-ence on Computer Vision and Pattern s Vegas,2016:1646-1654.[7]㊀KIM J,LEE J K,LEE K M.Deeply-recursive convolutional network for image super-resolution[C]ʊ2016IEEE Conference on Computer Vision and Pattern s Vegas,2016:1637-1645.[8]㊀ZHANG K,ZUO W M,GU S H,et al.Learning deep CNN denoiser prior for image restoration[C]ʊ2017IEEE Conference on Computer Vision and Pattern Recognition.Honolulu,2017:2808-2817.[9]㊀SHI W Z,JIANG F,ZHAO D B.Single image super-resolution with dilated convolution based multi-scale information learning inception module[C]ʊ2017IEEE International Conference on Image Processing.Beijing,2017:977-981.[10]DONG C,LOY C C,TANG X O.Accelerating the super-resolution convolutional neural network [M ].Amsterdam:Springer International Publishing,2016:391-407.[11]SHI W Z,CABALLERO J,HUSZAR F,et al.Real-time single image and video super-resolution using an efficient sub-pixel convolutional neural network [C]ʊ2016IEEE Conference on Computer Vision and Pattern s Vegas,2016:1874-1883.[12]LEDIG C,THEIS L,HUSZAR F,et al.Photo-realistic single image super-resolution using a generative adversarial network [C]ʊ2017IEEE Conference on Computer Vision and Pattern Recognition.Honolulu,2017:105-114.[13]TONG T,LI G,LIU X J,et al.Image super-resolution using dense skip connections[C]ʊ2017IEEE International Conference on Computer Vision.Venice,2017:4809-4817.[14]IOFFE S,SZEGEDY C.Batch normalization:accelerating deep network training by reducing internal covariate shift[C]ʊPro-7484郑州大学学报(理学版)第52卷ceedings of the32nd International Conference on Machine Learning.Lille,2015:448-456.[15]HE K M,ZHANG X Y,REN S Q,et al.Deep residual learning for image recognition[C]ʊ2016IEEE Conference on Comput-er Vision and Pattern s Vegas,2016:770-778.[16]MARTIN D,FOWLKES C,TAL D,et al.A database of human segmented natural images and its application to evaluating seg-mentation algorithms and measuring ecological statistics[C]ʊProceedings Eighth IEEE International Conference on Computer Vision.Vancouver,2001:416-423.[17]BEVILACQUA M,ROUMY A,GUILLEMOT C,et al.Single-image super-resolution via linear mapping of interpolated self-ex-amples[J].IEEE transactions on image processing,2014,23(12):5334-5347.[18]ZHANG K B,GAO X B,TAO D C,et al.Single image super-resolution with non-local means and steering kernel regression[J].IEEE transactions on image processing,2012,21(11):4544-4556.[19]YE Y X,SHAN J,BRUZZONE L,et al.Robust registration of multimodal remote sensing images based on structural similarity[J].IEEE transactions on geoscience and remote sensing,2017,55(5):2941-2958.[20]ZEYDE R,ELAD M,PROTTER M.On single image scale-up using sparse-representations[M].Berlin:Springe,2012:711-730.[21]BEVILACQUA M,ROUMY A,GUILLEMOT C,et al.Low-complexity single image super-resolution based on nonnegativeneighbor embedding[C]ʊProcedings of the British Machine Vision Conference2012.Surrey,2012:1-10.[22]SHOCHER A,COHEN N,IRANI M.Zero-shot super-resolution using deep internal learning[C]ʊ2018IEEE/CVF Conferenceon Computer Vision and Pattern Recognition.Salt Lake City,2018:3118-3126.Residual Learning of Deep CNN for Image Super-resolutionWANG Zhiren,GU Haosheng,REN Fuquan,SHI Ziteng,WANG Rui(College of Sciences,Yanshan University,Qinhuangdao066004,China) Abstract:Abundant details and edge information were difficult to obtain by the traditional super-resolu-tion convolutional neural networks.A multi-mapping residual convolutional neural network(MMRCNN) was proposed to solve this problem.Specifically,the low-resolution image was directly used as the initial input of MMRCNN,and then features were extracted by using the convolutional layer.Then,the multi-mapping network was constructed through residual learning,and the batch normalization layer was added to optimize the network,so that the feature information required for the aggregation of high-resolution ima-ges became extremely rich.Finally,the deconvolution layer was used to complete image up-sampling and output high-resolution images,so the end-to-end mapping between low-resolution images and high-resolu-tion images was directly completed without preprocessing.Experiments on benchmark datasets with differ-ent models showed that the MMRCNN presented improved in terms of peak signal-to-noise ratio,structur-al similarity and visual effect.Key words:super-resolution;deep learning;multi-mapping;residual learning(责任编辑:方惠敏)。
超分辨率图像重建算法综述

第39卷第8期自动化学报Vol.39,No.8 2013年8月ACTA AUTOMATICA SINICA August,2013超分辨率图像重建方法综述苏衡1,2周杰1张志浩1摘要由于广泛的实用价值与理论价值,超分辨率图像重建(Super-resolution image reconstruction,SRIR或SR)技术成为计算机视觉与图像处理领域的一个研究热点,引起了研究者的广泛关注.本文将超分辨率图像重建问题按照不同的输入输出情况进行系统分类,将超分辨率问题分为基于重建的超分辨率、视频超分辨率、单帧图像超分辨率三大类.对于其中每一大类问题,分别全面综述了该问题的发展历史、常用算法的分类及当前的最新研究成果等各种相关问题,并对不同算法的特点进行了比较分析.本文随后讨论了各不同类别超分辨率算法的互相融合和图像视频质量评价的方法,最后给出了对这一领域未来发展的思考与展望.关键词超分辨率图像重建,计算机视觉,图像处理,方法综述引用格式苏衡,周杰,张志浩.超分辨率图像重建方法综述.自动化学报,2013,39(8):1202−1213DOI10.3724/SP.J.1004.2013.01202Survey of Super-resolution Image Reconstruction MethodsSU Heng1,2ZHOU Jie1ZHANG Zhi-Hao1Abstract Because of its extensive practical and theoretical values,the super-resolution image reconstruction(SRIR or SR)technique has become a hot topic in the areas of computer vision and image processing,attracting many researchers attentions.This paper categorizes the SR problems according to their input and output conditions into three main cat-egories:reconstruction-based SR,video SR and single image SR.For each category,the development history,common algorithm classes and state-of-the-art research achievements are reviewed comprehensively.We also analyze the charac-teristics of different algorithms.Afterwards,we discuss the combination of different super-resolution categories and the evaluation of image and video qualities.Thoughts and foresights of thisfield are given at the end of this paper.Key words Super-resolution image reconstruction,computer vision,image processing,surveyCitation Su Heng,Zhou Jie,Zhang Zhi-Hao.Survey of super-resolution image reconstruction methods.Acta Auto-matica Sinica,2013,39(8):1202−1213超分辨率图像重建(Super resolution image re-construction,SRIR或SR)是指用信号处理和图像处理的方法,通过软件算法的方式将已有的低分辨率(Low-resolution,LR)图像转换成高分辨率(High-resolution,HR)图像的技术.它在视频监控(Video surveillance)、图像打印(Image printing)、刑侦分析(Criminal investigation analysis)、医学图像处理(Medical image processing)、卫星成像(Satellite imaging)等领域有较广泛的应用.收稿日期2011-08-31录用日期2013-01-29Manuscript received August31,2011;accepted January29, 2013国家自然科学基金重大国际(地区)合作研究项目(61020106004),国家自然科学基金(61005023,61021063),国家杰出青年科学基金项目(61225008),教育部博士点基金(20120002110033)资助Supported by Key International(Regional)Joint Research Pro-gram of National Natural Science Foundation of China(6102010 6004),National Natural Science Foundation of China(61005023, 61021063),National Science Fund for Distinguished Young Scholars(61225008),and Ph.D.Programs Foundation of Min-istry of Education of China(20120002110033)1.清华大学自动化系北京1000842.北京葫芦软件技术开发有限公司北京1000841.Department of Automation,Tsinghua University,Beijing 1000842.Beijing Hulu Inc.,Beijing100084超分辨率问题的解决涉及到许多图像处理(Im-age processing)、计算机视觉(Computer vision)、优化理论(Optimization problem)等领域中的基本问题[1],例如图像配准(Image registration)、图像分割(Image segmentation)、图像压缩(Image com-pression)、图像特征提取(Image feature extrac-tion)、图像质量评价(Image quality estimation)、机器学习(Machine learning)、最优化算法(Opti-mization algorithm)等,超分辨率是这些基本问题的一个具体应用领域,同时也对它们的研究进展起到了推动的作用.因此超分辨率问题本身的研究具有重要的理论意义.目前超分辨率问题已经成为相关研究领域的热点之一.在上世纪80∼90年代,就有人开始研究超分辨率图像重建的方法,1984年Tsai的论文[2]是最早提出这个问题的文献之一.在这之后有很多相关的研究对超分辨率的问题进行更加深入的讨论.有关超分辨率问题的研究成果,在计算机视觉、图像处理与信号处理领域的顶级会议和期刊都有大量收录. 1998年,Borman等[3]发表了一篇超分辨率图像重建的综述文章.2001年,Kluwer出版了一本详细介8期苏衡等:超分辨率图像重建方法综述1203绍超分辨率相关领域前沿问题的书籍[4].2003年,IEEE Signal Processing Magazine 刊出了一期超分辨率图像重建的专刊[5].这些较早期的综述文章主要介绍传统的基于重建的超分辨率算法的研究情况.近年来相关的超分辨率的综述文章,包括介绍单帧超分辨率问题的文献[6]与介绍基于重建的超分辨率问题的文献[7],总结了近年提出的各类算法,并对研究的未来进行了展望.与这些综述文章不同,本文将超分辨率问题按不同的输入输出情况进行系统分类,综述近年来超分辨率图像重建算法与理论研究的进展,全面介绍基于重建的超分辨率,视频超分辨率与单帧图像超分辨率等各类超分辨率问题的研究情况,并对不同的超分辨率方法进行比较分析,以供相关领域的研究者参考.本文的章节安排如下:第1节对超分辨率问题进行系统分类;第2∼4节分别对不同类型的超分辨率算法进行详细综述;在第5节,介绍了与超分辨率相关的其他研究,如理论研究与图像质量评价等;最后在第6节中给出结论与展望.1超分辨率问题的分类概括而言,按算法的输入输出的不同类型组合,超分辨率问题可以分为几类子问题,见图1.输入为低分辨率图像序列(视频),输出为单帧高分辨率图像的超分辨率问题,称为基于重建的超分辨率问题(Reconstruction-based super-resolution);输入与输出均为图像序列(视频)的超分辨率问题,称为视频超分辨率问题(Video super-resolution);输入与输出均为单帧图像的超分辨率问题,称为单帧图像超分辨率问题(Single image super-resolution,SISR)[6].根据是否依赖训练样本,超分辨率问题又可以分为[8−9]增强边缘的超分辨率问题(Edge-focused super-resolution)(无训练样本)与基于学习的超分辨率问题(Learning-based super-resolution)(有训练样本)两种.对于输入为单帧低分辨率图像,输出为图像序列(视频)的问题,由于其缺失的信息太多,研究的实际意义不大,几乎没有相关的研究,不在本文的讨论范围之内.以下章节分别综述各种不同类型的超分辨率算法,为了叙述的方便,如果没有特殊说明,用H 代表目标高分辨率图像,用L 代表输入的低分辨率图像,如果输入或输出为图像序列,则用下标k =1,···,n 来区分不同的图像帧,n 为总帧数.2基于重建的超分辨率方法基于重建的超分辨率问题是较早提出的一类传统超分辨率问题,在综述前人提出的基于重建的超分辨率算法之前,首先简单介绍算法常用的数学模型.如图2所示,假设输入的低分辨率图像共有n 帧,则基于重建的超分辨率问题的成像模型通常可以表示为[10]:L k =DB (2)k M k B (1)k H +N k ,k =1,···,n(1)模型认为,每一帧观察到的低分辨率图像L k 是由未知的原始高分辨率图像H 经过一系列的图像变换过程得到的,依次为:由环境因素引起的大气模糊算子B (1)k ,运动变换算子M k ,由相机成像系统引起的成像模糊算子B (2)k (包括运动模糊因素与CCD 模糊因素等),以及最后的降采样算子D .N k 代表在成像过程中引入的加性噪声.已知输入L k ,则基于重建的超分辨率的目标就是寻找真实高分辨率图像H的最优估计ˆH.图1超分辨率问题的分类Fig.1The categories of super-resolution problems1204自动化学报39卷图2基于重建的超分辨率问题的常用成像模型Fig.2The common imaging model of the reconstruction-based super-resolution problem2.1频域方法频域处理的方法是最早提出的基于重建的超分辨率方法之一,由于图像的卷积、平移、旋转等运算在频域可以方便地转化为易于处理的算术运算形式,频域方法是处理超分辨率问题的一个直接想法.目前公认最早的超分辨率方法是一个采用频域处理算法的典型例子[2].在假设的低分辨率图像L k 的生成模型条件下,文献[2]分别对低分辨率图像和目标原始图像进行离散傅里叶变换(Discrete Fourier transform,DFT)和连续傅里叶变换(Continuous Fourier transform,CFT),并根据傅里叶变换的性质,在频域中建立起二者之间的线性关系:L k =Φk H (2)其中花体字母分别代表对应正体变量的傅里叶变换向量化之后的结果.首先通过计算得到生成模型的参数,即可得到Φk ,求解H 即转化为求解H ,进一步转化为求解式(2)的反问题.总体来说,超分辨率的频域方法经历了两个阶段.在超分辨率重建问题提出的初期(20世纪80∼90年代),频域方法是超分辨率的重要方法.文献[11]用离散余弦变换(Discrete cosine transform,DCT)进行处理.文献[12]则提出了一个泛化的基于频域变换的算法框架.由于频域计算本身的特性,这种基于傅里叶变换的频域方法在理论推导和计算上都有一定的优势.随后,研究者很快意识到,这种方法的缺点也是比较明显的:它难于处理图像噪声问题,只能处理空间不变的噪声模型,难于在处理过程中添加先验信息.另外由于频域与空域之间复杂的变换关系,传统的频域方法只能处理输入低分辨率图像之间只存在全局整体运动(Global motion)的情况,而难以处理具有局部运动(Local motion),即场景中存在相对运动物体的情况,具有比较大的局限性.因此在之后的很长一段时间里,频域方法的发展几乎处于停滞的状态.但近期一些关键性方法的提出,使得频域方法又重新展现了活力.文献[13]提出应用可以刻画图像局部性质的小波变换(Wavelet transform)进行超分辨率重建,使频域方法可以较好地处理图像局部的不同情况.在2009年,文献[14]系统地研究了基于小波变换的超分辨率的理论,并且提出了一种新的鲁棒算法,它先对所有输入低分辨率图像同时进行对准,再通过一个迭代的去噪过程得到目标高分辨率图像.需要注意的是,在文献[14]中的运动模型仍然为全局运动的投影变换模型,并未处理局部运动情况,但小波变换的局部性质有效提高了重建图像的局部质量.2.2非均匀图像插值方法非均匀图像插值方法(Non-uniform interpola-tion)是最简单直观的超分辨率重建方法.由于其方法比较简单,适应性不强,相关的研究比较少,但这种方法的思想与其他类型的方法有所不同,因此将其单独进行介绍.非均匀图像插值将基于重建的超分辨率问题看作是一个图像插值(Image interpola-tion)问题(如图3):将输入的视频帧配准到目标图像平面后,这些输入图像信息转化为对目标图像特定位置的内容约束:H (p i )=c i ,i =1,···,m (3)则图像重建的过程本质上就是对目标图像平面格点上未知图像信息的拟合(Fitting)或插值(Inter-polation)过程,由于这种图像插值的已知图像信息不是均匀地分布在图像平面上的,因此称为非均匀(Non-uniform)的图像插值.早期的非均匀图像插值方法主要致力于算法的具体处理方法.例如,基于德劳内三角剖分(Delaunay triangulation)的非均匀图像插值[15],将图像平面分割为局部的小三角形进行计算,由于Delaunay 三角剖分具有最大化最小角等优良性质,因此可以得到稳定的重建结果.由于此类方法存在着适应性较差的问题,最近的相关研究已经不多,主要目标为提高输出结果的视觉质量和算法的鲁棒性上.发表于2008年的文献[16]在进行三角剖分后采用B 样条(B-spline)基进行线性滤波,还提出引入预滤波环节去除频率混叠现象.文献[17]引入了加权中值滤波以提高算法的鲁棒性.另外,非均匀图像插值方法的运算速度一般较快,具有较好的实用价值.最近发表了一些相关的应用研究文献,如Lin 等[18]将非均匀图像插值方法应用于车牌图像增强识别等等.8期苏衡等:超分辨率图像重建方法综述1205图3非均匀图像插值方法示意图Fig.3Non-uniform interpolation method非均匀图像插值方法的特点是非常简单直观,将超分辨率问题与图像插值问题联系在一起,但它的适应性相对较差,难于处理输入图像中的模糊现象、图像引入噪声等问题,不易加入图像的先验信息,因此相关的研究相对较少.2.3凸集投影方法凸集投影(Projection onto convex sets,POCS)方法是一种采用迭代的超分辨率方法.文献[19]是首先提出POCS 方法的文献之一.经典的POCS 方法[20]要求将对目标HR 图像的各种限制分别定义为在HR 图像空间中的闭凸集C i (i =1,···,m ),这些凸集的交集C s = mi =1C i 也是一个凸集,如果C s 不为空集,则其中的每一个元素都同时满足所有的限制条件,也就是原超分辨率问题的一个可行解.这样的可行解可以通过从HR 图像空间中任意的一点ˆH0开始,轮流不断向各个凸集进行投影(Projection)而得到,即POCS 方法的每一步迭代过程可以表示为ˆHn +1=P m P m −1···P 1ˆH n (4)其中,P i 表示将HR 图像空间中的任何一点投影到凸集C i 上的投影算子.通常在每个LR 图像的每个像素(x,y )上定义一个最基本的凸集约束C k (x,y )(k =1,···,n ),这些约束组成了由观测LR 图像序列决定的一致性凸集约束.在POCS 方法中可以加入先验信息对结果的影响,例如对目标图像峰值像素的约束[19]等.这里的先验信息同样要表现为在HR 图像空间中的凸集的形式,定义了这些先验信息决定的凸集以及对应的投影运算,就可以代入式(4)进行处理.文献[21]在设计凸集的形式时考虑到了相机曝光时间,空间模糊效果以及噪声的影响,其改进版本[22]则引入了置信掩模(Confidence map)的概念以避免运动匹配的误差.文献[23]介绍了用POCS 去除图像边缘部分的振铃效应(Ringing artifacts)的方法.POCS 方法本质上是一种有效的求取复杂优化问题可行解的方法,它的优势在于思想比较简单,方法形式也比较灵活,先验知识的加入也比较方便,在这一方法提出的初期引起了很多研究者的关注,将其应用于超分辨率问题.但POCS 方法的计算复杂度高,收敛速度比较慢,另外它的目标解一般不唯一,而是一个可行解的集合.因此早期的学者对这一算法的相关理论进行了较为深入的研究,如文献[24]提出了改进的椭圆约束方法,将最终交集椭圆的中心作为最终的求解目标而使目标解具有唯一性.进入21世纪,此类方法的相关研究目标主要是对生成图像实验效果的改进,例如文献[25]对POCS 方法进行改进以去除图像边缘部分的颜色失真现象.2.4最大后验概率方法最大后验概率(Maximum a posterior,MAP)方法是一种基于概率的算法框架,是目前实际应用和科学研究中运用最多的一类方法,很多具体的超分辨率算法都可以归入这一概率化的框架.最大后验概率方法的基本思想来源于条件概率,将已知LR 图像序列作为观测结果,对未知的HR 图像进行估计.最大后验概率方法的算法思想可以表示为ˆH=arg max(p (H |L 1,L 2,···,L n ))=arg max(ln p (L 1,L 2,···,L n |H )+ln p (H ))(5)这里ˆH表示对目标高分辨率图像H 的估计,也就是算法最终的输出结果.其中的先验概率项ln p (H )表示高分辨率图像H 出现的先验概率,代表了对高分辨率图像的一种评价标准以避免病态问题的出现.在MAP 及相关的超分辨率方法中,体现加入的HR 图像的先验知识的这一项ln p (H )又称为正则项(Regularization term),对控制最终结果的图像质量起到了比较关键的作用.MAP 这类方法比较灵活,尤其是在MAP 方法的正则项部分,可以自由加入对具体问题的具体约束.MAP 方法的研究可以大体分为以下几个互相重叠的发展阶段.在提出了基本的MAP 方法框架之后,如何设计一个有效的正则项首先成为领域的研究热点.较为常用的正则项包括二范数形式的Tikhonov 正则项[26]、一范数形式的的全变差(Total1206自动化学报39卷variation,TV)正则项[27]以及双边全变差(Bilat-eral TV,BTV)正则项[10],还有更复杂的Student-t 正则项[28]等.在提出了多种的正则项形式之后,正则项的相关理论研究逐渐增多.这种先验信息中通常表达了对目标图像平滑程度的要求,使得结果图像具有比较强的空间连续性,但也造成了图像边缘等细节信息的损失.文献[10,29]分别引入了具有保边缘趋向的先验概率估计,文献[10]同时说明了在先验概率和正则项中,采用一范数代替通常使用的二范数具有更强的鲁棒性,可以适应数据、模型的变化而得到较好的实验结果.从本质上说,这是因为这些正则项通常具有长尾(Heavy tail)特性,即正则项函数对图像的高频部分具有较高的响应.在超分辨率重建中,由于具有长尾特性的正则项在优化过程中鼓励了图像边缘处的高频信息,因此这种正则项形式更倾向于恢复图像的锐利边缘[28],但同时也更有可能引入高频的图像噪声[30].之后,除了正则项本身的形式设计之外,一些其他的相关问题也开始进入了研究者的视野.文献[31]在说明正则项系数选取的重要性的同时,提出了一种使用L曲线(L-curve)确定最优正则项系数的方法.2010年,文献[32]对文献[31]进行了改进,提出用更有效的U曲线(U-curve)求取正则项系数.文献[33]将式(5)中的两项进行解耦,在迭代过程中轮流进行优化,提高了计算速度.在未知图像生成模型的点扩散函数(Point spread function,PSF)的情况下进行超分辨率重建的算法又称为盲超分辨率(Blind super-resolution). Wang等[34]提出了一种基于分块的MAP盲超分辨率方法,利用Gibbs采样和模拟退火算法同时求取PSF参数和对应目标高分辨率图像的最大后验概率解.最大后验概率方法具有较好的灵活性和鲁棒性[5].另外,在噪声V k的概率分布满足一定条件的前提下,原概率推断问题具有唯一解,这时可以选择高效的梯度下降算法而不必担心收敛于局部极值.综上所述,最大后验概率方法是一种比较有效的超分辨率重建方法.2.5其他相关问题绝大部分的基于重建的超分辨率算法依赖于输入视频帧间的运动匹配(Motion registration)的结果,一个有效的重建算法需要运动匹配具有亚像素(Sub-pixel)的匹配精度,而在复杂运动的情况下,目前的运动匹配技术很难达到这样的要求.因此,如何处理运动匹配不准对超分辨率算法造成的影响是一个非常重要的问题.最直观的想法是增加运动匹配的精确程度[35−36].联合估计方法(Joint estimation)[37−38]在迭代过程中同时对运动估计结果进行更新以提高估计效果,但这种方法的收敛性难以保证.置信掩模方法(Confidence map)[22]移除或减小了错误的运动匹配对输出结果的贡献.文献[39]提出了一个超分辨率算法融合的框架,首先对高分辨率图像平面进行自适应分块,将运动匹配精度、图像纹理情况等信息作为区块特征,应用机器学习的方法为不同的区域选择不同的传统超分辨率算法,提高了算法的鲁棒性.文献[30]则提出利用输入视频帧之间更加鲁棒的稀疏的特征点匹配结果进行超分辨率重建.近年来提出的另外一类方法,包括有向核回归(Steering kernel regression)[40−41]与概率运动估计[42−43]等算法不显式地估计运动场,而度量图像局部的时空相似程度进行模糊的运动估计,有效地提高了算法的鲁棒性,在帧间运动较为复杂剧烈的情况下也可以得到较好的重建结果.由于输入的LR图像数据通常是经过压缩的,研究视频压缩与超分辨率的相互影响,如何利用视频压缩的数据流简化超分辨率的计算,如何在超分辨率结果中去除视频压缩噪声,也是超分辨率图像重建的一个重要的研究方向[44].在视频压缩编码流中可以利用的信息主要包括两个方面[4]:运动矢量信息和误差量化信息.文献[45]通过压缩数据中运动的矢量方向估计运动模糊的程度,完成超分辨率图像拼接(Mosaic)的任务.文献[46]将这些量化信息作为POCS中的凸集约束,文献[44]采用概率统计模型对误差进行建模,在基于概率的超分辨率框架中减小压缩噪声的影响,文献[47]引入考虑了压缩噪声的正则化处理对结果进行优化.以上这些方法分别有各自的特点,不同的特点决定了它们不同的适用场合[5,48].例如,MAP方法在存在充分的先验信息时特别有效,而迭代的方法对图像匹配的精度有比较高的要求,以防止匹配误差随迭代逐步放大.在表1中,我们总结了各类基于重建的超分辨率算法总体的不同特点.从工程实际中算法实现复杂度的角度来看,非均匀图像插值方法只需要进行运动配准和简单的插值计算,运算效率较高,效果一般也比频域的方法好,如果运算资源相对丰富,则可以选择合适的最大后验概率方法得到更高的重建图像质量.3视频超分辨率方法与基于重建的超分辨率不同,视频超分辨率方法8期苏衡等:超分辨率图像重建方法综述1207表1各类基于重建的超分辨率算法比较表1Table 1Comparison ofdifferent reconstruction-based super-resolution algorithms算法名称频域方法非均匀图像插值POCS方法MAP方法解的唯一性唯一唯一不唯一唯一处理复杂运动难于处理可以处理可以处理可以处理图像先验知识可以加入难于加入可以加入可以加入算法灵活性较差差高高计算复杂度小中等大较大1本表中列出的是各类算法的典型特点,少量特殊算法可能有特例的情况.的输出为整个高分辨率视频,后者的应用范围相对更加广泛.基于重建的超分辨率可以被看成是视频超分辨率的一种特例,而两者的解决方法之间也有着千丝万缕的联系.然而,前者虽然也可以用来实现对视频的超分辨率化,但它们之间存在很大不同.前者的目标是生成高质量的单帧图像,使得图像中原本模糊的部分更加清晰,而后者则主要为了增强输出视频的视觉体验;与之相对应,它们的应用领域也有所不同,前者可以应用于视频监控、人脸识别等领域,而后者通常作为视频增强的算法出现.基于这些考虑,我们将它们分为两类进行介绍.一个直观的想法是,用基于重建的超分辨率算法分别依次重建每个高分辨率视频帧,将得到的结果连接成为视频,就可以进行视频的超分辨率化.事实上,有些上面提到的基于重建的超分辨率方法(如文献[40])并没有对这两种超分辨率问题加以严格的区分,而是也提到了视频超分辨率的情况.文献[41]还提到了时空超分辨率的概念:除了在空间上对图像分辨率加以增强,还在时间上增加帧率使输出视频更加流畅.2011年发表的文献[49]利用马尔科夫随机场(Markov random field,MRF)模型,用著名的图切割(Graph-cut)算法进行时空超分辨率化.专门设计的视频超分辨率方法可以实现从基于重建的超分辨率到视频超分辨率的性能提升.视频超分辨率方法主要包括两大类[50]:增量视频超分辨率(Incremental video super-resolution)[51]与同时视频超分辨率(Simultaneous video super-resolution),参见图4.增量视频超分辨率方法依次对视频帧中的每一帧进行超分辨率化,可以在上面提到的基于重建的超分辨率方法上引入简单的节省计算复杂度的考虑,提高运算速度.例如,Su 等[52]提出采用增量运动估计方法提高算法效率.同时视频超分辨率将整个输入低分辨率视频作为整体输入,通过一个完整的优化过程一次得到所有输出视频帧.文献[53]提出了基于条件随机场(Conditionalrandom field,CRF)的同时视频超分辨率方法.另一种同时视频超分辨率算法[54]不显式地估计视频帧间的运动,而是将运动信息转化为先验概率进行处理.(a)增量视频超分辨率(a)Incremental video super-resolution(b)同时视频超分辨率(b)Simultaneous video super-resolution图4视频超分辨率方法的分类Fig.4Categories of video super-resolution methods表2总结了两种视频超分辨率算法的优缺点.增量视频超分辨率方法的特点是一般速度比较快,而且支持增量输入数据(输入数据按时间顺序逐步输入),比较适合实时或是半实时的处理,但效果一般比同时超分辨率稍差.同时超分辨率方法可以较好地考虑视频帧之间的一致性因素,生成质量更高的输出视频,但计算速度一般比较慢,另外这类方法需要同时将所有的低分辨率视频帧作为整体输入,因此一般无法满足实时性的要求.因此在工程实践中,如果运算资源允许,通常采用增量视频超分辨率。