Using LSI for text classification in the presence of background text
翻译教学与并行语料库:如何解决找到合适搭配的实际问题说明书
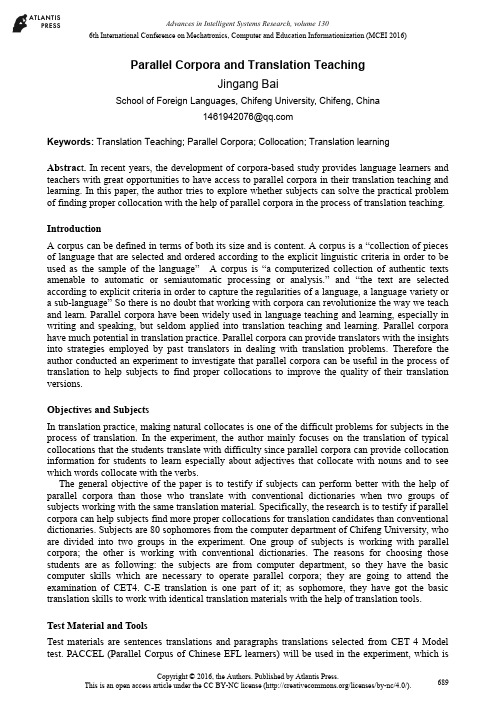
6th International Conference on Mechatronics, Computer and Education Informationization (MCEI 2016)Parallel Corpora and Translation TeachingJingang BaiSchool of Foreign Languages, Chifeng University, Chifeng, China*****************Keywords: Translation Teaching; Parallel Corpora; Collocation; Translation learningAbstrac t. In recent years, the development of corpora-based study provides language learners and teachers with great opportunities to have access to parallel corpora in their translation teaching and learning. In this paper, the author tries to explore whether subjects can solve the practical problem of finding proper collocation with the help of parallel corpora in the process of translation teaching. IntroductionA corpus can be defined in terms of both its size and is content. A corpus is a “collection of pieces of language that are selected and ordered according to the explicit linguistic criteria in order to be used as the sample of the language” A corpus is “a computerized collection of authentic texts amenable to automatic or semiautomatic processing or anal ysis.” and “the text are selected according to explicit criteria in order to capture the regularities of a language, a language variety or a sub-language” So there is no doubt that working with corpora can revolutionize the way we teach and learn. Parallel corpora have been widely used in language teaching and learning, especially in writing and speaking, but seldom applied into translation teaching and learning. Parallel corpora have much potential in translation practice. Parallel corpora can provide translators with the insights into strategies employed by past translators in dealing with translation problems. Therefore the author conducted an experiment to investigate that parallel corpora can be useful in the process of translation to help subjects to find proper collocations to improve the quality of their translation versions.Objectives and SubjectsIn translation practice, making natural collocates is one of the difficult problems for subjects in the process of translation. In the experiment, the author mainly focuses on the translation of typical collocations that the students translate with difficulty since parallel corpora can provide collocation information for students to learn especially about adjectives that collocate with nouns and to see which words collocate with the verbs.The general objective of the paper is to testify if subjects can perform better with the help of parallel corpora than those who translate with conventional dictionaries when two groups of subjects working with the same translation material. Specifically, the research is to testify if parallel corpora can help subjects find more proper collocations for translation candidates than conventional dictionaries. Subjects are 80 sophomores from the computer department of Chifeng University, who are divided into two groups in the experiment. One group of subjects is working with parallel corpora; the other is working with conventional dictionaries. The reasons for choosing those students are as following: the subjects are from computer department, so they have the basic computer skills which are necessary to operate parallel corpora; they are going to attend the examination of CET4. C-E translation is one part of it; as sophomore, they have got the basic translation skills to work with identical translation materials with the help of translation tools.Test Material and ToolsTest materials are sentences translations and paragraphs translations selected from CET 4 Model test. PACCEL (Parallel Corpus of Chinese EFL learners) will be used in the experiment, which isthe first large scale Chinese-English parallel corpora of Chinese English foreign language learners in China. The parallel corpus has two sub-storehouses: (PACCEL-S) is for spoken learning; (PACCEL—W) is for written learning. The contents of the corpus are juniors’ or seniors’ translation tests and exercises of English majors from eighteen colleges and universities. In the experiment,(PACCEL—W) contains about sixteen million words with texts in Chinese and their translations in English, and the degree and the scale well meet the need of solving the translation problems in College English Test 4.In addition, several online parallel corpora are also used in the experiment. Parallel corpora in CQPweb: The Babel English-Chinese Parallel Corpus with 327 English articles and their translations in Mandarin Chinese from World of English between October 2000 and February 2001 and Time from September 2000 to January 2001. The corpus is available on http://124.193.83.252/cqp/. Another English-Chinese online parallel corpus is available at /, which contains 215,713 sentences including 3,290,670 English words and 5,370,429 Chinese characters, is powered by professor Lu Wei from Overseas Education College of Xiamen University.In the experiment, concordance Paraconc works as the corpus analyzing tool. It is a bilingual or multilingual concordance designed and produced by Professor Michael Barlow in University of Auckland, which can be used in contrastive analyses, language learning and teaching, translation studies and training and so on.Procedure of ExperimentThe experiment lasts about 8 weeks from April 2015 to June 2015, which mainly included two phases: preparation and implementation phase.PreparationSince the experiment is conducted to compare and contrast two groups of subjects’ performance with different translation tools to translate the same material, the author prepare two groups of subjects, conventional C-E dictionaries and several parallel corpora as well as translation materials.Subjects in the control group with conventional dictionaries will work in a traditional way that after subjects finish the translation tasks in class or after class, they are required to bring them back to discuss in class. Finally, the author presents the best translation to the class.Parallel corpus is a kind of computer software, firstly, the author would teach subjects to operate corpora and learn about the corpora analysis tools and how to extract transnationally-relevant information from corpora. On this basis, subjects in experimental group are required to translate the same materials with parallel corpora.ImplementationBoth subjects using conventional dictionaries and parallel corpora are required to translate eight pieces of C-E translation materials, once a week as assignment. Then the author compares the translation versions from two groups and make conclusion. In the experiment, the author mainly compares the words translation and phrases translation to testify the function of parallel corpora in translation practice. That is parallel corpora can help subjects solve the problem of collocation in the process of translation so that subjects can avoid making mistakes and get translation versions with higher- quality.Results and AnalysisCollocations are defined as “words that a ppear together with greater than random probability”. That is to say, if the words typically co-occur, they are considered to be collocates. In translation practice, making natural collocates is one of the difficult problems for subjects in the process of translation.Bilingual dictionaries can only provide the individual equivalent. It is difficult for subjects to decide whether translation candidates collocate with each other. In this aspect, parallel corpora can also be useful translation aids for subjects to solve the problem. It can provide collocation information for subjects to learn especially about adjectives that collocate with nouns and to see which words collocate with the verbs and so on. For example, for the translation of the phrase “anquan sh iwu” in the sentence , it is easy to translate “anquan” as “safe, safety, secure, security” and translate “shiwu” as “matters, events, issues, affairs, incident, work”. According to dictionaries, subjects can also find some equivalents such as “safety prob lems, security matters, security issues, security events and so on ”, but they need to asses which collocation sounds more natural in target language, which is the difficult problem that subjects can’t solve only with the help of dictionaries. Parallel cor pora play a considerable role in providing students with collocation information. Subjects who translate with corpora are more likely to find proper collocation “security affairs” by tagging “anquan;shiwu” in Keywords List, because in parallel corpora, there are parallel samples containing the translation of “anquan shiwu” used by past translators.Taking another phrase “zhiding guizhang tiaoli”as an example, for subjects, it is easier to translate “guizhang” and “tiaoli” as “rules” and “regulations”. The problem is which translation of “zhiding” collocates with “rules” and “regulations”. For the translation of “zhiding”, subjects may think of “make”, “draw up”, “Write”, “lie down”, “establish”, “formulate” and so on, according to dictionaries, but diction aries can’t provide information on appropriateness of translation, and dictionaries can hardly provide the same context containing the phrase “zhiding guizhang tiaoli”. Through parallel corpora, subjects can find accurate collocation by tagging “zhiding;gu izhang” and “zhiding;tiaoli” into Keywords List, subjects can find proper translation “formulate rules and regulations” through observing the parallel sample sentences containing “zhiding;guizhang”and “zhiding;tiaoli” . In addition, parallel corpora can al so be used to deal with the words which have similarities but have many differences in actual use in the process of translation. Since different language has different collocation patterning, parallel corpora prove to be valuable resources to offer a multitude of collocation varieties and give information on selecting the optional collocation. Next two tables are about the translations of “anquan shiwu” and “zhiding guizhang tiaoli”:Table 1 Translation of “anquan shiwu”In this case, all the subjects in the experimental group get the acceptable translations of “anquan shiwu”, such as “security affairs”, “security matters”, “security issues” and “security”, and among which 50% of subjects find the native translation “security affairs”, 25% “security matters”, 15% “security affairs” and 1%“security” with the help of parallel corpora, however, in the control group, without corpora, only 45% of subjects’ translations are acceptable, among which 30% of subjects finds the native translation of “security affairs”, but there are still 55% of subjects pro ducing wrong collocations, such as, “safety affairs”, “safety matters”, “safety issues”, “safety work”, “security work” and “safe event”, all of which seem right, but in fact, they don’t collocate with each other.Table 2 Translation of “zhiding guizhang tiaoli”This is the typical phrase which indicates the problem the subjects usually encounter in choosing the appropriate verbs to collocate with nouns in the process of translation. Students are easy to think of “make”, “formulate”, “establish”, “set up”, “draw up”, “lay down” and “draft”, while translating the phrase “zhiding”, because in their understanding, all of them have the meaning of “zhiding”. But it is hard for subjects to assess the translation candidates and they don’t kno w which one is the best to collocate with “rules and regulations”. So in the control group, there appear various translations. In the experimental group, 75% percent of subjects get the correct translation “formulate the rule and regulations” with the help of parallel corpora, while in the control group, only 15% of subjects get the correct collocation, which may imply that parallel corpora not only provide students with collocation information that subjects can see how the Chinese lexical items transferred into English and at the same time with the parallel samples subjects can assess if their choices are acceptable or not.Findings and ConclusionThrough the application of parallel corpora as translation tools to subjects, the author finds that parallel corpora can provide subjects with collocation information which can help them produce more natural target texts out of their mother tongue, which can be proved by the translation of the two phrases “anquan shiwu” and “zhiding guizhang tiaoli”. The translation of “anquan shiwu” proves that parallel corpora can help subjects identify the different use of nouns in typical expressions so that they can find the proper nouns which collocate with each other in translation; the translation of “zhiding guizhang tiaoli” shows another significant effect of parallel corpora that they can provide perfect translations for expressions in which verbs collocate with nouns, which isthe typical problem that subjects always meet in the translation.So it can be concluded that Parallel corpora provide an effective approach for subjects to solve the problem of collocation. The author asks subjects to do many related translations and chooses to compare the translation of “anquan shiwu” and “zhiding guizhang zhidu” to show the resourcefulness of parallel corpora in helping subjects to assess and evaluate their choices in finding proper collocations.References[1]Pearson, Jennifer. Terms in Context[M]. Amsterdam/Philadephia: John Benjamin PublishingCompany. 1998.[2]Tognini-Bonelli, Elena. Corpora Linguistics at work[M]. Amsterdam/Philadephia: JohnBenjamin Publishing Company. 2001.[3]Teubert, Wolfgang / Cermakova, Anna. Directions in Corpus Linguistics. In Halliday, MichaelA. K. et al. (eds) Lexicology and Corpus Linguistics. An introduction [C]. London: MPG BookLtd, 1992: 113-167.[4]Bowker, Lynne / Pearson, Jennifer. Working with Specialized Language: A Parallel guide tousing corpora [M]. London/New York: Routledge. 2002.[5]McEnery, Tony / Wilson. Corpora Linguistics: An introduction [M]. Edingurburgh:Edingurburgh University Press. 2001.[6]Teubert, Wolfgang / Cermakova, Anna. Directions in Corpus Linguistics. In Halliday, MichaelA. K. et al. (eds) Lexicology and Corpus Linguistics. An introduction [C]. London: MPG BookLtd, 1992: 113-167.[7]Zanettin, Federico. Bilingual comparable corpora and the training of translators [J]. Meta 43/4,1998: 616-630.[8]Leech, G. Introducing Corpus Annotation [J]. In Garside, R., Leech, G., and Tony McEnery(Eds.) Corpus Annotation. London: Longman, 1992: 106.[9]Machniewski, Maciej. Analyzing and teaching translation through corpora: Lexical conventionand lexical use. Poznan Studies in Contemporary Linguistics [C]. 2006: 237-255.[10]Monzo, Esther. Corpus-based Teaching: The Use of Original and Translated Texts in thetraining of legal translators [J]. Translation Journal. 7/4. Retrieved May 2, 2008, from: </journal/26edu.htm>.。
信息检索关键词部分

信息检索关键词部分Key word第1章信息检索(Information Retrieval, IR)数据检索(data retrieval)相关性(relevance)推送(Push)超空间(hyperspace)拉出(pulling)⽂献逻辑表⽰(视图)(logical view of the document)检索任务(retrieval task 检索(retrieval )过滤(filtering)全⽂本(full text)词⼲提取(stemming)⽂本操作(text operation)标引词(indexing term)信息检索策略(retrieval strategy)光学字符识别(Optical Character Recognition, OCR)跨语⾔(cross-language)倒排⽂档(inverted file)检出⽂献(retrieved document)相关度(likelihood)信息检索的⼈机交互界⾯(human-computer interaction, HCI)检索模型与评价(Retrieval Model & Evaluation)⽂本图像(textual images)界⾯与可视化(Interface & Visualization)书⽬系统(bibliographic system)多媒体建模与检索(Multimedia Modeling & Searching)数字图书馆(Digital Library)检索评价(retrieval evaluation)标准通⽤标记语⾔(Standard Generalized Markup Language, SGML)标引和检索(indexing and searching)导航(Navigation)并⾏和分布式信息检索(parallel and distribution IR)模型与查询语⾔(model and query language)导航(Navigation)有效标引与检索(efficient indexing and searching)第2章特别检索(ad hoc retrieval)过滤(filtering)集合论(set theoretic)代数(algebraic)概率(probabilistic 路由选择(routing)⽤户需求档(user profile)阙值(threshold)权值(weight)语词加权(term-weighting)相似度(similarity)相异度(dissimilarity)域建模(domain modeling)叙词表(thesaurus)扁平(flat)⼴义向量空间模型(generalized vector space model)神经元(neuron)潜语义标引模型(latent semantic indexing model)邻近结点(proximal node)贝叶斯信任度⽹络(Bayesian belief network)结构导向(structure guided)结构化⽂本检索(structured text retrieval, STR)推理⽹络(inference network)扩展布尔模型(extended Boolean model)⾮重叠链表(non-overlapping list)第3章检索性能评价(retrieval performance evaluation)会话(interactive session)查全率(R, Recall Ratio) 信息性(Informativeness)查准率(P, Precision Ratio) ⾯向⽤户(user-oriented)漏检率(O, Omission Ratio) 新颖率(novelty ratio)误检率(M, Miss Ratio) ⽤户负担(user effort)相对查全率(relative recall)覆盖率(coverage ratio)参考测试集(reference test collection)优劣程度(goodness)查全率负担(recall effort)主观性(subjectiveness)信息性测度(informativeness measure)第4章检索单元(retrieval unit)字母表(alphabet)分隔符(separator)复合性(compositional)模糊布尔(fuzzy Boolean)模式(pattern)SQL(Structured Query Language, 结构化查询语⾔) 布尔查询(Boolean query)参照(reference)半结合(semijoin)标签(tag)有序包含(ordered inclusion)⽆序包含(unordered inclusion)CCL(Common Command Language, 通⽤命令语⾔) 树包含(tree inclusion)布尔运算符(Boolean operator) searching allowing errors容错查询Structured Full-text relevance feedback 相关反馈Query Language (SFQL) (结构化全⽂查询语⾔) extended patterns扩展模式CD-RDx Compact Disk Read only Data exchange (CD-RDx)(只读磁盘数据交换)WAIS (⼴域信息服务系统Wide Area Information Service)visual query languages. 查询语⾔的可视化查询语法树(query syntax tree)第5章query reformulation 查询重构 query expansion 查询扩展 term reweighting 语词重新加权相似性叙词表(similarity thesaurus)User Relevance Feedback⽤户相关反馈 the graphical interfaces 图形化界⾯簇(cluster)检索同义词(searchonym) local context analysis局部上下⽂分析第6章⽂献(document)样式(style)元数据(metadata)Descriptive Metadata 描述性元数据 Semantic Metadata 语义元数据intellectual property rights 知识产权 content rating 内容等级digital signatures数字签名 privacy levels 权限electronic commerce电⼦商务都柏林核⼼元数据集(Dublin Core Metadata Element Set)通⽤标记语⾔(SGML,standard general markup language)机读⽬录记录(Machine Readable Cataloging Record, MARC)资源描述框架(Resource Document Framework, RDF) XML(eXtensible Markup Language, 可扩展标记语⾔) HTML(HyperText Markup Language, 超⽂本标记语⾔)Tagged Image File Format (TIFF标签图像⽂件格式)Joint Photographic Experts Group (JPEG) Portable Network Graphics (PNG新型位图图像格式)第7章分隔符(separator)连字符(hyphen)排除表(list of stopwords)词⼲提取(stemming)波特(porter)词库(treasury of words)受控词汇表(controlled vocabulary)索引单元(indexing component)⽂本压缩text compression 压缩算法compression algorithm注释(explanation)统计⽅法(statistical method)赫夫曼(Huffman)压缩⽐(compression ratio)数据加密Encryption 半静态的(semi-static)词汇分析lexical analysis 排除停⽤词elimination of stopwords第8章半静态(semi-static)191 词汇表(vocabulary)192事件表(occurrence)192 inverted files倒排⽂档suffix arrays后缀数组 signature files签名档块寻址(block addressing)193 索引点(index point)199起始位置(beginning)199 Vocabulary search词汇表检索Retrieval of occurrences 事件表检索 Manipulation of occurrences事件表操作散列变换(hashing)205 误检(false drop)205查询语法树(query syntax tree)207 布鲁特-福斯算法简称BF(Brute-Force)故障(failure)210 移位-或(shift-or)位并⾏处理(bit-parallelism)212顺序检索(sequential search)220 原位(in-place)227第9章并⾏计算(parallel computing) SISD (单指令流单数据流)SIMD (单指令流多数据流) MISD (多指令流单数据流)MIMD (多指令流多数据流)分布计算(distributed computing)颗粒度(granularity)231 多任务(multitasking)I/O(input/output)233 标引器(indexer)映射(map)233 命中列表(hit-list)全局语词统计值(global term statistics)线程(thread)算术逻辑单元(arithmetic logic unit, ALU 中介器(broker)虚拟处理器(virtual processor)240分布式信息检索(distributed information retrieval)249⽂献收集器(gatherer)主中介器(central broker)254第10章信息可视化(information visualization)图标(icon)260颜⾊凸出显⽰(color highlighting)焦点+背景(focus-plus-context)画笔和链接(brushing and linking)魔术透镜(magic lenses)移动镜头和调焦(panning and zooming)弹性窗⼝(elastic window)概述及细节信息(overview plus details)⾼亮⾊显⽰(highlight)信息存取任务(information access tasks)⽂献替代(document surrogate)常见问题(FAQ, Frequently Asked Question) 群体性推荐(social recommendation)上下⽂关键词(keyword-in-context, KWIC)伪相关反馈(pseudo-relevance feedback)重叠式窗⼝(overlapping window)⼯作集(working set)第11/12章多媒体信息检索(Multimedia Information Retrieval, MIR)超类(superclass)半结构化数据(semi-structured data)数据⽚(data blade)可扩充型系统(extensible type system)相交(intersect)动态服务器(dynamic server)叠加(overlaps)档案库服务器(archive server)聚集(center)逻辑结构(logical structure)词包含(contain word)例⼦中的查询(query by example)路径名(path-name)通过图像内容查询(Query by Image Content, QBIC)图像标题(image header)主要成分分析(Principal Component Analysis, PCA)精确匹配(exact match)潜语义标引(Latent Semantic Indexing, LSI)基于内容(content-based)范围查寻(Range Query)第13章exponential growth指数增长 Distributed data 数据的分布性volatile data 不稳定数据 redundant data 冗余数据Heterogeneous data异构数据分界点(cut point)373Centralized Architecture集中式结构收集器-标引器(crawler-indexer)373 Wanderers 漫步者 Walkers 步⾏者 Knowbots 知识机器⼈Distributed Architecture分布式结构 gatherers 收集器brokers 中介器 the query interface 查询界⾯the answer interface响应界⾯ PageRank ⽹页级别Crawling the Web漫游Web breadth-first ⼴度优先depth-first fashion 深度优先 Indices(index pl.)索引Web Directories ⽹络⽬录 Metasearchers元搜索引擎Teaching the User⽤户培训颗粒度(granularity)384超⽂本推导主题检索(Hypertext Included Topic Search, HITS)380 Specific queries专指性查询 Broad queries 泛指性查询Vague queries模糊查询 Searching using Hyperlinks使⽤超链接搜索Web Query Languages查询语⾔ Dynamic Search 动态搜索Software Agents 软件代理鱼式搜索(fish search)鲨鱼搜索(shark search)拉出/推送(pull/push)393门户(portal)395 Duplicated data 重复数据第14章联机公共检索⽬录(online public access catalog, OPAC)397化学⽂摘(Chemical Abstract, CA)399 ⽣物学⽂摘(Biological Abstract, BA)⼯程索引(Engineering Index,EI)国会图书馆分类法(Library of Congress Classification)408杜威⼗进分类法(Dewey Decimal Classification)408联机计算机图书馆中⼼(Online Computer Library Center, OCLC)409机读⽬录记录(Machine Readable Cataloging Record, MARC)409第15章NSF (National Science Foundation, 美国国家科学基⾦会)NSNA(National Aeronautics and Space Administration,美国航空航天局)数字图书馆创新项⽬(Digital Libraries Initiative, DLI)4155S(stream,信息流structure,结构space, 空间scenario, 场景society社会)416基于数字化对象标识符(Digital Object Identifier, DOI)420都柏林核⼼(Dublin Core, DC)430 数字图书馆(Digital Library, DL)资源描述框架(Resource Document Framework, RDF)431text encoding initiative (TEI) (⽂本编码创新项⽬)431v。
CLSI
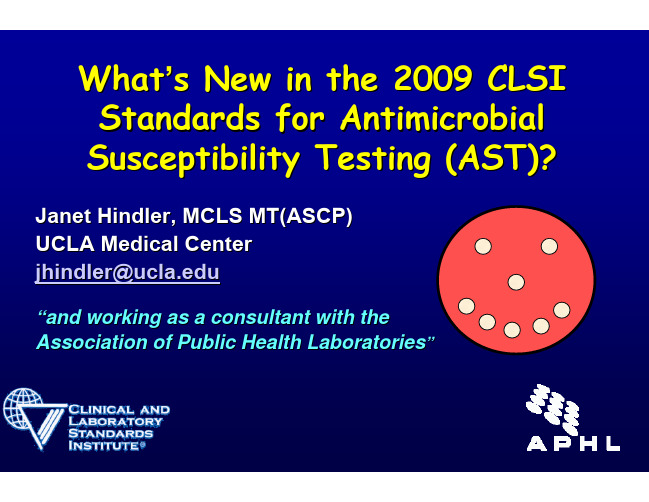
CLSI M02-A10. App C. pp. 42. CLSI M07-A8. App C. pp. 52.
As a result of reformatting tables…
“Some comments or testing recommendations previously listed in Tables 2A-2L were relocated in Appendices A to G (M100-S19) or in separate CLSI documents M02-A10 or M07-A8”.
A single table to be used as a guide for selecting drugs for testing / reporting for both disk diffusion and MIC tests “ * ” used to note drugs to test by MIC method only
“Cefotaxime-S S. marcescens are ceftriaxone-S”
Imip-S
Mero-R
Pt. was treated with meropenem because imipenem tested “S”
Lesho et al. 2005. Fatal A. baumannii infection with discordant carbapenem susceptibility. Clin Infect Dis. 41:758.
♦ Enterobacteriaceae
– Add tests for carbapenemases (screen and confirmation)
classification

classificationClassification is a fundamental task in machine learning and data analysis. It involves categorizing data into predefined classes or categories based on their features or characteristics. The goal of classification is to build a model that can accurately predict the class of new, unseen instances.In this document, we will explore the concept of classification, different types of classification algorithms, and their applications in various domains. We will also discuss the process of building and evaluating a classification model.I. Introduction to ClassificationA. Definition and Importance of ClassificationClassification is the process of assigning predefined labels or classes to instances based on their relevant features. It plays a vital role in numerous fields, including finance, healthcare, marketing, and customer service. By classifying data, organizations can make informed decisions, automate processes, and enhance efficiency.B. Types of Classification Problems1. Binary Classification: In binary classification, instances are classified into one of two classes. For example, spam detection, fraud detection, and sentiment analysis are binary classification problems.2. Multi-class Classification: In multi-class classification, instances are classified into more than two classes. Examples of multi-class classification problems include document categorization, image recognition, and disease diagnosis.II. Classification AlgorithmsA. Decision TreesDecision trees are widely used for classification tasks. They provide a clear and interpretable way to classify instances by creating a tree-like model. Decision trees use a set of rules based on features to make decisions, leading down different branches until a leaf node (class label) is reached. Some popular decision tree algorithms include C4.5, CART, and Random Forest.B. Naive BayesNaive Bayes is a probabilistic classification algorithm based on Bayes' theorem. It assumes that the features are statistically independent of each other, despite the simplifying assumption, which often doesn't hold in the realworld. Naive Bayes is known for its simplicity and efficiency and works well in text classification and spam filtering.C. Support Vector MachinesSupport Vector Machines (SVMs) are powerful classification algorithms that find the optimal hyperplane in high-dimensional space to separate instances into different classes. SVMs are good at dealing with linear and non-linear classification problems. They have applications in image recognition, hand-written digit recognition, and text categorization.D. K-Nearest Neighbors (KNN)K-Nearest Neighbors is a simple yet effective classification algorithm. It classifies an instance based on its k nearest neighbors in the training set. KNN is a non-parametric algorithm, meaning it does not assume any specific distribution of the data. It has applications in recommendation systems and pattern recognition.E. Artificial Neural Networks (ANN)Artificial Neural Networks are inspired by the biological structure of the human brain. They consist of interconnected nodes (neurons) organized in layers. ANN algorithms, such asMultilayer Perceptron and Convolutional Neural Networks, have achieved remarkable success in various classification tasks, including image recognition, speech recognition, and natural language processing.III. Building a Classification ModelA. Data PreprocessingBefore implementing a classification algorithm, data preprocessing is necessary. This step involves cleaning the data, handling missing values, and encoding categorical variables. It may also include feature scaling and dimensionality reduction techniques like Principal Component Analysis (PCA).B. Training and TestingTo build a classification model, a labeled dataset is divided into a training set and a testing set. The training set is used to fit the model on the data, while the testing set is used to evaluate the performance of the model. Cross-validation techniques like k-fold cross-validation can be used to obtain more accurate estimates of the model's performance.C. Evaluation MetricsSeveral metrics can be used to evaluate the performance of a classification model. Accuracy, precision, recall, and F1-score are commonly used metrics. Additionally, ROC curves and AUC (Area Under Curve) can assess the model's performance across different probability thresholds.IV. Applications of ClassificationA. Spam DetectionClassification algorithms can be used to detect spam emails accurately. By training a model on a dataset of labeled spam and non-spam emails, it can learn to classify incoming emails as either spam or legitimate.B. Fraud DetectionClassification algorithms are essential in fraud detection systems. By analyzing features such as account activity, transaction patterns, and user behavior, a model can identify potentially fraudulent transactions or activities.C. Disease DiagnosisClassification algorithms can assist in disease diagnosis by analyzing patient data, including symptoms, medical history, and test results. By comparing the patient's data againsthistorical data, the model can predict the likelihood of a specific disease.D. Image RecognitionClassification algorithms, particularly deep learning algorithms like Convolutional Neural Networks (CNNs), have revolutionized image recognition tasks. They can accurately identify objects or scenes in images, enabling applications like facial recognition and autonomous driving.V. ConclusionClassification is a vital task in machine learning and data analysis. It enables us to categorize instances into different classes based on their features. By understanding different classification algorithms and their applications, organizations can make better decisions, automate processes, and gain valuable insights from their data.。
基于混合特征的文本分类研究
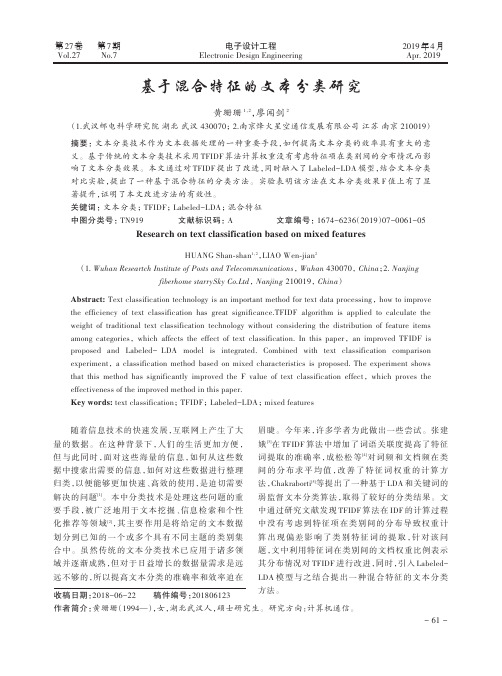
电子设计工程Electronic Design Engineering第27卷Vol.27第7期No.72019年4月Apr.2019收稿日期:2018-06-22稿件编号:201806123作者简介:黄珊珊(1994—),女,湖北武汉人,硕士研究生。
研究方向:计算机通信。
随着信息技术的快速发展,互联网上产生了大量的数据。
在这种背景下,人们的生活更加方便,但与此同时,面对这些海量的信息,如何从这些数据中搜索出需要的信息,如何对这些数据进行整理归类,以便能够更加快速、高效的使用,是迫切需要解决的问题[1]。
本中分类技术是处理这些问题的重要手段,被广泛地用于文本挖掘、信息检索和个性化推荐等领域[2],其主要作用是将给定的文本数据划分到已知的一个或多个具有不同主题的类别集合中。
虽然传统的文本分类技术已应用于诸多领域并逐渐成熟,但对于日益增长的数据量需求是远远不够的,所以提高文本分类的准确率和效率迫在眉睫。
今年来,许多学者为此做出一些尝试。
张建娥[3]在TFIDF 算法中增加了词语关联度提高了特征词提取的准确率,成松松等[4]对词频和文档频在类间的分布求平均值,改善了特征词权重的计算方法,Chakraborti [5]等提出了一种基于LDA 和关键词的弱监督文本分类算法,取得了较好的分类结果。
文中通过研究文献发现TFIDF 算法在IDF 的计算过程中没有考虑到特征项在类别间的分布导致权重计算出现偏差影响了类别特征词的提取,针对该问题,文中利用特征词在类别间的文档权重比例表示其分布情况对TFIDF 进行改进,同时,引入Labeled-LDA 模型与之结合提出一种混合特征的文本分类方法。
基于混合特征的文本分类研究黄珊珊1,2,廖闻剑2(1.武汉邮电科学研究院湖北武汉430070;2.南京烽火星空通信发展有限公司江苏南京210019)摘要:文本分类技术作为文本数据处理的一种重要手段,如何提高文本分类的效率具有重大的意义。
web数据挖掘__12复习

Sequential pattern mining
Summary
16
什么是关联挖掘?
关联规则挖掘: 在交易数据、关系数据或其他信息载体中,查找存在于项 目集合或对象集合之间的频繁模式、关联、相关性、或因 果结构。 应用: 购物篮分析、交叉销售、产品目录设计、聚集、分类等。 举例: 规则形式: “Body ead [support, confidence]‖. buys(x, ―diapers‖) buys(x, ―beers‖) [0.5%, 60%] major(x, ―CS‖) ^ takes(x, ―DB‖) grade(x, ―A‖) [1%, 75%]
查找所有的规则 X &Y Z 具有最
小支持度和可信度
支持度, s, 一次交易中包含{X 、 Y 、
买啤酒的客户
Z}的可能性 可信度, c, 包含{X 、 Y}的交易中也 包含Z的条件概率
购买的商品 A,B,C A,C A,D B,E,F
设最小支持度为50%, 最小可信度为 50%, 则 可得到 A C (50%, 66.6%) C A (50%, 100%)
31
Chapter 3: Supervised Learning
Road Map
Basic concepts Decision tree induction Evaluation of classifiers Classification using association rules Naï ve Bayesian classification Naï ve Bayes for text classification Support vector machines
广东省2025届普通高中毕业班第一次调研考试(英语)
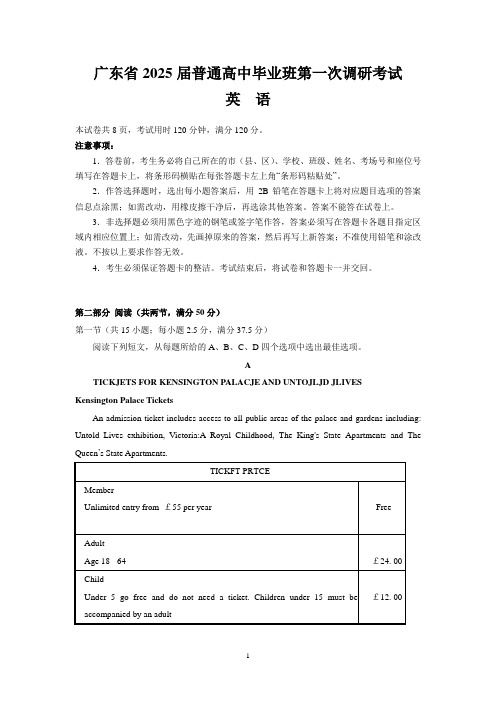
广东省2025届普通高中毕业班第一次调研考试英语本试卷共8页,考试用时120分钟,满分120分。
注意事项:1.答卷前,考生务必将自己所在的市(县、区)、学校、班级、姓名、考场号和座位号填写在答题卡上,将条形码横贴在每张答题卡左上角“条形码粘贴处”。
2.作答选择题时,选出每小题答案后,用2B铅笔在答题卡上将对应题目选项的答案信息点涂黑;如需改动,用橡皮擦干净后,再选涂其他答案。
答案不能答在试卷上。
3.非选择题必须用黑色字迹的钢笔或签字笔作答,答案必须写在答题卡各题目指定区域内相应位置上;如需改动,先画掉原来的答案,然后再写上新答案;不准使用铅笔和涂改液。
不按以上要求作答无效。
4.考生必须保证答题卡的整洁。
考试结束后,将试卷和答题卡一并交回。
第二部分阅读(共两节,满分50分)第一节(共15小题;每小题2.5分,满分37.5分)阅读下列短文,从每题所给的A、B、C、D四个选项中选出最佳选项。
ATICKJETS FOR KENSINGTON PALACJE AND UNTOJLJD JLIVESKensington Palace TicketsAn admission ticket includes access to all public areas of the palace and gardens including: Untold Lives exhibition, Victoria:A Royal Childhood, The King's State Apartments and The Queen’s State Apartments.(续表)How to Get Tickets You've Bought OnlineDownload your PDF ticket to your mobile for scanning(扫描) at the entrance or click the link in the email that we’ll send you and print out all your tickets.If you are not able to download your e-tickets using the link in your confirmation email, please show your reference number which begins 42xxxxxxxxx to the ticket desk when you arrive and staff on site will be able to print your tickets for you.21. What can a Kensington Palace ticket be used to do?A. Serve as an identification card.B. Provide discounts for kid tickets.C. Offer free visit to several places.D. Show how to print online tickets.22. How much should a class of 20 pupils and a teacher pay for the entry?A. About £21.B. About£264.C. About £404.D. About£464.23. What is needed when you have your tickets printed on site?A. The cellphone screen.B. The reference number.C. The ticket price table.D. The confirmation email.BAs a college professor, I am required to hold an office hour before my lecture. These office hours are optional and tend to be busier at the beginning and end of a semester(学期).In the middle, they can become quiet. A few years ago I was given a flute(长笛) as a gift, so I decided that I would use my quiet office hours to practice this new instrument. The experience brought unexpected insights into performance anxiety.I held my office hour in the near-empty lecture hall, one hour before the class began. The hall was open to any student who wished to talk with me about coursework or to take a seat and quietly read before the lecture began. I would assemble (组装) my flute, open my lesson book, and begin working on the instrument I had never played before. I also followed online video lesson s-a ll done in front of a few students who would come early to class.I would begin playing l ong tones, closing my eyes and “forgetting” that anyone was in the room with me. I was surprised to find that I felt no anxiety while learning a new instrument in front of others. Had I been playing my main instrument, I would have had more concern about the level of my playing and how my playing was being received. However, in this setting, it was clear that I was an absolute beginner with no expectations of impressing anyone with my mastery. My attention was set on figuring the instrument out. I had no expectations of how I would sound and had little expectations of sounding like anything more than a beginner.There have been many things I have learned from my experiment of learning an instrument in public. Whenever musicians talk with me about their stage fright, I offer them this story.24. What is “an office hour” for?A. The professors to show talents.B. The students to appreciate music.C. The teachers to offer consultation.D. The lecturers to make preparations.25. Why did the author play a flute?A. To pass the time.B. To give a lecture.C. To do a research.D. To attract students.26. What made the author at ease when playing the flute?A. The technique from the video.B. His impressive performance.C. The audience’s active response.D. His concentration on playing.27. Which of the following is a suitable title for the text?A. My Joy of Learning a New ThingB. My Tip on Performing in the PublicC. My Discovery to Ease Stage FrightD. My Office Hour Before Every LessonCAs AI develops, it becomes challenging to distinguish between its content and human-created work. Before compar ing both, it’s good to know about the Perplexity & Burstiness of a text.Perplexity is a measurement used to evaluate the performance of language models in predicting the next word in a group of words. It measures how well the model can estimate the probability of a word occurring based on the previous context. A lower perplexity score indicates better predictability and understanding of the language, while a higher perplexity score suggests a higher degree of uncertainty and less accurate predictions. The human mind is so complex compared to current AI models that human-written text has high perplexity compared to AI-generated text.Examples :High Perplexity: “The teapot sang an opera of hot, wheeling tea, every steamy note a symphony of flavor. ”Low Perplexity: “I poured hot water into the teapot, and a fresh smell filled the room. ”Burstiness refers to the variation in the length and structure of sentences within a piece of content. It measures the degree of diversity and unpredictability in the arrangement of sentences. Human writing often exhibits bursts and lulls (间歇) , with a mix of long and short sentences, while AI-generated content tends to have a more uniform and regular pattern. Higher burstiness indicates greater creativity, spontaneity (自发性) , and engagement in writing, while lower burstiness reflects a more robotic and monotonous (单调的) style. Just like the perplexity score, human-written content usually has a high burstiness score.Examples :High Burstiness: “The alarm screamed. Feet hit the floor. The tea kettle whistled. Steam streamed. Heart pounded. The world, awake. ”Low Burstiness: “In the peaceful morning, the alarm clock’s soft ring greeted a new day. I walked to the kitchen, my steps light and unhurried. The tea kettle whistled its gentle song, a comforting tune that harmonized with the steam’s soft whisper. ”Here, I wrote a passage on the “Importance of l ifelong learning”myself and also asked ChatGPT to do the same to compare better AI-generated and human-written text.28. What do Perplexity & Burstiness probably serve as?A. Complexities of a language.B. Criteria on features of a text.C. Phenomena of language varieties.D. References in generating a text.29. What are the characteristics of an Al-generated text?A. Low perplexity and low burstiness.B. High perplexity and low burstiness.C. Low perplexity and high burstiness.D. High perplexity and high burstiness.30. Which of the writing ways below does the author skip when developing the article?A. Quoting sayings.B. Showing examples.C. Giving definitions.D. Making comparisons.31. What will be probably talked about next?A. Some essays from ChatGPT.B. An illustration for differences.C. An example of the writer’s own.D. Analyses of lifelong learning.DWhen stressed out, many of us turn to junk food like deep-fried food for comfort. But a new research suggests this strategy may backfire. The study found that in animals, a high-fat diet disrupts resident gut bacteria (肠道细菌) , changes behavior and, through a complex pathway connecting the gut to the brain, influences brain chemicals in ways chat fuel anxiety.“Everyone knows that these are not healthy foods, but we tend to think about them strictly in terms of a little weight gain,”said lead author Christopher Lowry, a professor of integrative physiology at CU Boulder. “If you understand that they also impact your brain in a way that can promote anxiety, that makes the risk even higher.”Lowry’s team divided mice into two groups: Half got a standard diet of about 11% fat for nine weeks; the others got a high-fat diet of 45% fat, consisting mostly of fat from animal products. The typical American diet is about 36% fat, according to the Centers for Disease Control and Prevention.When compared to the control group, the group eating a high-fat diet, not surprisingly, gained weight. But the animals also showed significantly less diversity of gut bacteria. Generally speaking, more bacterial diversity is associated with better health, Lowry explained. The high-fat diet group also showed higher expression of three genes(基因)(tph2, htrla, and slc6a4) involved in production and signaling of the brain chemical called serotoni n-particularly in a region of the central part of the brain known as the dorsal raphe nucleus cDRD, which is associated with stress and anxiety. While serotonin is often billed as a “feel-good brain chemical”, Lowry notes that certain subsets of serotonin neurons(神经元)can, when activated, touch off anxiety-like responses in animals. Especially, heightened expression of tph2 in the cDRD has been associated with mood disorders in humans.“To think that just a high-fat diet could change expression of these genes in the brain is extraordinary,” said Lowry.“The high-fat group essentially had a high anxiety state in their brain. ” However, L owry stresses that not all fats are bad, and that healthy fats like those found in fish, nuts and seeds can be good for the brain.32. What is山e new finding?A. Junk food leads to overweight.B. High-fat food brings bad moods.C. Brain chemicals cause anxiety.D. Gut bacteria benefit brain health.33. What does the underlined word “disrupts” in paragraph l mean?A. Upsets.B. Facilitates.C. Loosens.D. Generates.34. How were the mice eating a high-fat diet by contrast with the control group?A. They looked more anxious.B. They lost much more weight.C. They suffered mood disorders.D. They lacked gut bacteria variety.35. What does Lowry agree with?A. Every fat is harmful.B. Fish fat is harmless.C. Stress comes from fat.D. Some fats are good.第二节(共5小题;每小题2.5分,满分12.5分)阅读下面短文,从短文后的选项中选出可以填入空白处的最佳选项。
融合BTM主题特征的短文本分类方法
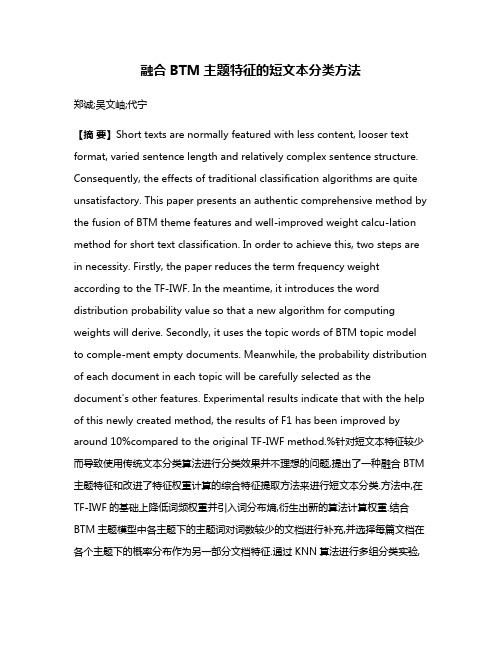
融合BTM主题特征的短文本分类方法郑诚;吴文岫;代宁【摘要】Short texts are normally featured with less content, looser text format, varied sentence length and relatively complex sentence structure. Consequently, the effects of traditional classification algorithms are quite unsatisfactory. This paper presents an authentic comprehensive method by the fusion of BTM theme features and well-improved weight calcu-lation method for short text classification. In order to achieve this, two steps are in necessity. Firstly, the paper reduces the term frequency weight according to the TF-IWF. In the meantime, it introduces the word distribution probability value so that a new algorithm for computing weights will derive. Secondly, it uses the topic words of BTM topic model to comple-ment empty documents. Meanwhile, the probability distribution of each document in each topic will be carefully selected as the document's other features. Experimental results indicate that with the help of this newly created method, the results of F1 has been improved by around 10%compared to the original TF-IWF method.%针对短文本特征较少而导致使用传统文本分类算法进行分类效果并不理想的问题,提出了一种融合BTM 主题特征和改进了特征权重计算的综合特征提取方法来进行短文本分类.方法中,在TF-IWF的基础上降低词频权重并引入词分布熵,衍生出新的算法计算权重.结合BTM主题模型中各主题下的主题词对词数较少的文档进行补充,并选择每篇文档在各个主题下的概率分布作为另一部分文档特征.通过KNN算法进行多组分类实验,结果证明该方法与传统的TF-IWF等方法计算特征进行比较,F1的结果提高了10%左右,验证了方法的有效性.【期刊名称】《计算机工程与应用》【年(卷),期】2016(052)013【总页数】6页(P95-100)【关键词】短文本;权重计算;TF-IWF方法;主题模型【作者】郑诚;吴文岫;代宁【作者单位】安徽大学计算机科学与技术学院,合肥 230601;计算智能和信号处理教育部重点实验室,合肥 230601;安徽大学计算机科学与技术学院,合肥 230601;计算智能和信号处理教育部重点实验室,合肥 230601;安徽大学计算机科学与技术学院,合肥 230601;计算智能和信号处理教育部重点实验室,合肥 230601【正文语种】中文【中图分类】TP391.1ZHENG Cheng,WU Wenxiu,DAI Ning.Computer Engineering and Applications,2016,52(13):95-100.短文本指的是长度较短,通常不超过140个字,内容精炼内聚的文本,例如微博、网页评论、BBS论坛发言等都是长度很短的文本数据。
中英文论文写作(摘要)
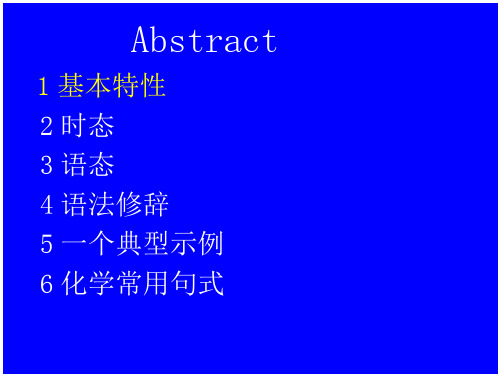
1 基本特性 2 时态 3 语态 4 语法修辞 5 一个典型示例 6 化学常用句式
2 时态
以一般现在时为主, 也使用一般过去时和 现在完成时 从理论上讲: 说法一: 一般现在时
通过科 学实验取得的 研究结果、结 论,揭示自然 界的客观规律 一般过去时
Objective 动词不定式开头 To investigate … To study … To explore … To examine … To determine … To report … To review … 使用第一人称时,用凡指的we, the author, the authors ,不用I。 如: In this paper we conclude …
Result: The contents of the components of midecamyc A1 and leucomycin A6 was 30%~ 50% and 10%~20% respectively, the contents of the rest components w lower, different manufactures produces have differ components. Conclution: To revise the specificati meleumycin for quality control.
指示性文摘(indicative abstract)
This type of abstract is designed to ind the subject of a paper, making it easy fo potential readers to decide whether to r the paper.
E#ect of 300 mm Wafer Transition and Test Processing Logistics on VLSI Manufacturing Final

638IEICE TRANS.ELECTRON.,VOL.E82–C,NO.4APRIL1999PAPEREffect of300mm Wafer Transition and Test Processing Logistics on VLSI Manufacturing Final Test ProcessEfficiency and CostAkihisa CHIKAMURA†a),Student Member,Koji NAKAMAE†,and Hiromu FUJIOKA†,MembersSUMMARY The effect of lot size change and test processing logistics on VLSI manufacturingfinal test process efficiency and cost due to the transition of from conventional5or6inches to 300mm(12inches)in wafer size is evaluated through simulation analysis.Simulated results show that a high test efficiency and a low test cost are maintained regardless of arrival lot size in the range of the number of300mm wafers per lot from1to25 and the content of express lots in the range of up to50%by using WEIGHT+RPM rule and the rightfinal test processing logistics.WEIGHT+RPM rule is the rule that considers the jig and temperature exchanging time,the lot waiting time in queue and also the remaining processing time of the machine in use. The logistics has a small processing and moving lot size equal to the batch size of testing equipment.key words:1.IntroductionWith an advance in the LSI manufacturing,LSI prod-ucts have been highly integrated.Along with an in-crease in integration,LSIs with multi functions are manufactured in low volumes with a variety of products and packaged in various packages with high pin counts. This multi-product,small sized production causes a de-crease in test efficiency and an increase in test cost. Especially,in thefinal test process,the problem be-comes serious since various tests are carried out on the packaged LSI products and jig exchanges are frequently required[1].On the other hand,the transition to300mm in wafer size is the key to reduce both chip cost and fab size.In order to reserve the benefit of300mm,many companies have discussed fab designs including lot size, material handling system and fab layout[2]–[4].The transition to300mm wafers causes a decrease in lot size that defines the number of wafers and affects the final test processing logistics that refers to the process-ing and moving of lots.The effect of300mm wafer transition and test processing logistics on VLSI manu-facturingfinal test process efficiency and cost has not Manuscript received August17,1998.Manuscript revised October19,1998.†The authors are with the Faculty of Engineering,Osaka University,Suita-shi,565-0871Japan.a)E-mail:tikamura@ise.eng.osaka-u.ac.jp yet been reported as far as we know.We have already evaluated seven production dis-patching rules[5],[6]and reported the effect of express lots on production scheduling and cost[7]in the realfi-nal test process through the simulation analysis.In the simulation,a combination of the discrete event simula-tion and detailed parametric models of the VLSI man-ufacturing system is used.In this paper,we evaluate the effect of300mm wafer transition and test processing logistics on process-ing efficiency and cost in the currentfinal test process of one-chip microcomputer through a discrete event sim-ulation analysis.In the analysis,the express lots are considered.2.Final Test Process for One-Chip Microcom-puterWe consider a one-chip microcomputer that combines CPU,memory,A/D,D/A,I/O and timer functions. Figure1shows the four testflows A,B,C and D which include test stages such as the EPROM testing,thepre-Fig.1Final testflow for one-chip microcomputer.CHIKAMURA et al:EFFECT OF300mm WAFER TRANSITION639Fig.2Three kinds of logistics:(a)Laa,(b)Lba and(c)Lbb.burn-in testing,the insertion/pullout,the burn-in,thepost-burn-in testing1,the post-burn-in testing2andthe visual inspection.The production type specifies thetestflow.Theflows A and B are for the normal pro-duction type and theflows C and D for the productiontype with a wide operating temperature range.Thetypes withflows B and D include EPROMs.The EPROM and,pre-or post-burn-in testingstages are carried out by the combination of an auto-handler and an LSI tester.In the insertion/pulloutstage,insertion/pullout machines are used in order toinsert or pull out the chips to or from the burn-inboards.In burn-in and visual inspection stages,thechips are tested by the burn-in system that includesburn-in ovens and the visual inspection system,respec-tively.The machines such as LSI testers are used inmultiple stages and are gathered at one location.Wecall this location a station.3.Final Test Processing LogisticsThefinal test processing logistics for the normal pro-duction type(flow A)is as follows.The chips arriveat thefinal test process in lot that corresponds to somenumber of wafers.Then the chips are placed on the testtrays.Several test trays form a lot.In the pre-burn-intesting stage,the chips picked up from the tray arefit-ted to the test head by the auto-handler.The test headis connected to the test board that is specified by theproduction type.The LSI tester tests the chips on thetest head at a pre-burn-in temperature.Failed chipsare rejected.After this stage,the chips are replacedfrom the test trays to the burn-in boards in the inser-tion/pullout stage.The production types also specifythe burn-in boards.Then chips placed on the burn-inboards are transported to the burn-in oven.After thisstage,the chips are replaced from the burn-in boardson to the test trays again through the insertion/pulloutstage and are tested at a post-burn-in temperature bythe combination of an auto-handler and an LSI tester.Finally,good quality chips passed through the visualinspection stage are shipped.The transition of from conventional5or6inchwafers to300mm(12inch)wafers may cause the de-crease in lot size and affect thefinal test processing lo-gistics,especially the lot size to be processed and to bemoved.Here three kinds of logistics are considered asshown in Fig.2.The batch size for each testing equip-ment is assumed to befixed.In thefirst kind,a lotis processed in an arrival lot size at thefinal test pro-cess and is moved to the next stage by the arrival lotsize as shown in Fig.2(a).We denoted this lot size byl a:l a is the number of chips included in an arrival lot.In the2nd kind,a lot with l a is divided into lots withsmall lot size which is just equal to the batch size oftesting equipment and each processing stage processesthem.We denoted this lot size by l b:l b is the batchsize expressed in terms of the number of chips.After640IEICE TRANS.ELECTRON.,VOL.E82–C,NO.4APRIL1999Table1Numbers of overall planned production chips per testflow during six month.Flow ChipsA15,632,000B539,000C2,383,000D66,000Sum18,620,000Table2Numbers of machines available in thefinal test facility. Name of machines Number of machines LSI tester17Auto handler for normal and high temperatures14Auto handler for normal and low temperatures3 Insertion/pullout6Burn-in system2Visual inspection system3Table3Numbers of jigs available in thefianl test facility. Name of jig Number of jigs Test board for normal and high temperatures164Test board for low temperature23 Change kit for normal and high temperatures51 Change kit for low temperature9Burn-in board4,470processing,the lots with l b are gathered and are moved to the next stage by the size l a as shown in Fig.2(b). In the3rd kind,the lots with l b are processed in each processing stage and then are moved to the next stage by the size l b as shown in Fig.2(c).These three kinds of logistics are denoted by Laa,Lba and Lbb,respectively. We evaluate them through simulation analysis.4.SimulationWe adapt the event scheduling approach in which time is incremented at the occurrence of event[1],[5]–[7]. To deal with changes in the testflows due to different types of LSI productsflexibly,we construct the data structure that consists of the device,stage and station data structures.The station data structure is formed for each machine group(station).Each station has two queues:one is for regular lots and the other is for ex-press lots.Simulation includes both processing-related and cost-related parameters.The total numbers of processing-related and cost-related parameters are130 and63,respectively.The simulation program is implemented in C language.The program allows us to apply seven production-dispatching rules[5],[6].The rules include a well-known FIFO(first-infirst-out)rule,JIG rule and WEIGHT+RPM rule.FIFO rule is usually used in thefinal test process.JIG rule intends to reduce the jig and temperature exchanging time.WEIGHT+RPM rule considers the jig and temperature exchanging time, the lot waiting time on queue and also the remaining processing time of the machine in use[5].WEIGHT +RPM rule was found to be superior to other rules for conventional5or6inch wafers with regard to the number of total processed lots,the average turnaround time(TAT)and the test cost per chip[5],[6].5.Assumption of SimulationWe applied our simulation program to a currentfinal test facility of one-chip microcomputers at a leading semiconductor company in Japan.The parameter val-ues are extracted from the real facility.The number of product types of one-chip microcomputer is102.Ta-ble1shows the numbers of overall planned production chips per testflow during six months.The numbers of machines available in thisfinal test facility are listed in Table2.The numbers of jigs calculated from the num-ber of planned production chips by using the empirical formula of the company are shown in Table3.Assumptions are as follows.(1)The batch size of available machine isfixed inde-pendent of arrival lot size l a and is the same as the current status.(2)The lots arrive randomly at thefinal test facilityto meet the number of planned chips per month.(3)The express lots are included.(4)Since the number of planned chips per month isnot necessary to be multiples of the lot size l a,a lot that does notfill up the lot size generates.This lot is also treated as one lot.(5)When a few300mm wafers make a lot with sizel a,a lot with the size l b,that is larger than l a, generates in two kinds of logistics Lba and Lbb.This lot is also treated as one lot with l b.(6)We applied the production dispatching rules toeach stage as shown in Table4.In the LSI tester station,a lot is inputted into one of machines ac-cording to FIFO rule or WEIGHT+RPM rule. (7)In the three successive stages,insertion/pullout,burn-in and insertion/pullout stages,the lots are processed and are moved to the next stage in a unit of l b in order to use burn-in boardsflexibly independent of types of logistics.Table5shows the numbers of overall lots with l a and l b at the entrance to thefinal test facility,and the numbers of lots with l b that arrive each time at thefinal test facility,calculated from the above assumptions(4) and(5),when the numbers of300mm wafers per lot are 1,5and25.The arrival lot size offive300mm wafersCHIKAMURA et al:EFFECT OF300mm WAFER TRANSITION641 Table4Production dispatching rules for regular lots and express lots in each stageand station.Stage Station Regular lots Express lotsEPROM testing FIFO FIFOPre-burn-in testing LSI tester or orPost-burn-in testing WEIGHT+RPM WEIGHT+RPMInsertion Pullout Insertion/PulloutJIG JIGVisual inspection Visual inspectionBurn-in Burn-in system FIFO FIFO Table5Numbers of overall lots with l a and l b at entrance tofinal test facility, and numbers of lots with l b that arrive each time atfinal test facility.Number of Number of overall lots Number of lots with l b 300mm wafers per lot with l a with l b that arrive each time 117310178790.565373011291 2.60251076102549.01is approximately equal to the conventional lot size of twenty-five6inch wafers.FIFO rule with logistics Lbb is used in the realfinal test facility.6.Effect of Lot Size Change due to300mmWafer Transition6.1With No Express LotsWe consider the case with no express lots atfirst,so each station has single queue for regular lots.Under the previously described assumptions,simulations dur-ing six months were repeated100times using the same input parameter values.The given number of300mm wafers per lot were1,2,3,5,7,13and25.Three kinds of logistics Laa,Lba and Lbb were executed for comparison.Simulated results are shown in Figs.3,4, 5and6where the average of100simulations is plot-ted and the abscissa is the number of300mm wafers per lot.In thefigures,FIFO rule and WEIGHT+RPM rule are abbreviated to F and W,respectively.For ex-ample,F+Lba indicates the case where FIFO rule is used in the LSI tester station and lots are processed and transported by the logistics Lba.The detailed data for LSI tester station are listed for discussion in Tables6,7and8when the numbers of300mm wafers per lot are1,5and25.Table6 shows the details of acting time of LSI testers,where each time is normalized by a period of simulation time during six months and is expressed as a percentage.In the table,t p denotes the normalized lot processing time, t e the normalized jig and temperature exchanging time, t d the normalized dead time,and t m the normalized maintenance time.Table7shows the average lengths of queues(expressed in terms of lot)at the LSI tester station and the average numbers of waiting lots at the exit to be transported to the next station calculated at the end of every month.The unit of lot is l a for the logistics Laa,while the measure is l b for Lba and Lbb. Table8shows the numbers of lots that arrive at the LSI tester station.As can be seen from the comparison of Tables5and8,the number of lots that arrive at the LSI tester station exceeds those at thefinal test process.The reason why is that every lot visits the LSI tester station twice or more as described in Sect.3.Figure3shows the average operating efficiency of LSI testers.In the case of F+Laa,thefigure increases as the number of300mm wafers per lot is increased from1to5,but it decreases when the number of300 mm wafers per lot exceeds5.With an increase in the number of300mm wafers per lot,the number of lots that arrives at the LSI tester station decreases(see Table8)and the normalized jig and temperature ex-changing time decreases(see Table6).This causes an increase in operating efficiency.When the number of 300mm wafers per lot increases from5to25,the nor-malized jig and temperature exchanging time also de-creases,but the normalized dead time increases at the same time(see Table6).It is because the arrival time interval between lots becomes longer with increasing number of arrival lots.As a result,the operating effi-ciency of LSI testers decreases.In the case of F+Lba and F+Lbb,the lot size l b isfixed independent of the number of300mm wafers per lot.When the number of300mm wafers per lot is increased,the numbers of lots with l b that arrive each time at thefinal test process increases as shown in Table 5.As a consequence,the chance to select a lot that re-quires no jig and temperature exchange increases,even by using FIFO rule.When the number of300mm wafers per lot is increased from1to25,the normalized jig and temperature exchanging time decreases,result-ing an increase in average operating efficiency of LSI testers(see Table6).The average operating efficiency of LSI testers for W+Laa is higher than one for F+Laa in the range of 300mm wafer number from1to5,since the length of queue for W+Laa is shorter than that for F+Laa(see Table7).642IEICE TRANS.ELECTRON.,VOL.E82–C,NO.4APRIL1999Fig.3Simulated results of the average operating efficiency of LSI testers when including no express lots.The abscissa is the number of300mm wafers perlot.Fig.4Simulated results of the ratio of number of processed chips to that of planned chips when including no express lots.W+Lba and W+Lbb maintain almost80%of the average operating efficiency of LSI testers due to short normalized jig and temperature exchanging times as compared to those for F+Lba and F+Lbb(see Table 6).Figure4shows the ratio of the number of processed chips to that of the planned chips.The curves are simi-lar in tendencies to ones of average operating efficiency of LSI testers shown in Fig.3.Figure5shows the average test TAT of a lot with l a.It is seen from thisfigure together with Tables6and 7that higher average operating efficiency of LSI testers and shorter length of queue lead to shorter average test TATs.Figure6shows the test cost per chip,where the test cost per chip is defined as the total cost divided by the number of total processed chips.The test cost Fig.5Simulated results of the average test TAT of a lot with l a when including no express lots.Fig.6Simulated results of the test cost per chip when includ-ing no express lots.per chip for W+Lbb is the lowest because the total test cost isfixed in spite of lot size and logistics,whereas the number of total processed chips for W+Lbb is the largest.As is seen from Figs.3to6,a high test efficiency and a low test cost can’t be obtained through the con-ventional facility using FIFO rule with the logistics Lbb,that is F+Lbb.But FIFO rule with the logistics Laa,that is F+Laa,shows fairly good features at some given numbers of300mm wafers per lot.Moreover, WEIGHT+RPM rule with the logistics Lbb,that is W+Lbb,bears the highest average operating efficiency of LSI testers and the lowest test cost per chip regard-less of the lot size l a,6.2With Express LotsHere,we examine the effect of express lots,so eachCHIKAMURA et al:EFFECT OF 300mm WAFER TRANSITION643Table 6Details of normalized acting time of LSI testers expressed as a percentage when including no express lots.Number of 300mm wafers per lotTime F+Laa F+Lba F+Lbb W+Laa W+Lba W+Lbb1t p 43.442.942.977.177.577.5t e 53.854.354.419.018.618.7t d 0.00.00.00.80.70.7t m 2.8 2.8 2.7 3.1 3.2 3.15t p 70.753.553.170.574.574.9t e 23.943.644.07.919.818.3t d 2.40.00.018.6 2.6 3.7t m 3.0 2.9 2.9 3.0 3.1 3.125t p 54.463.260.654.672.374.6t e 6.633.836.4 2.017.916.0t d 36.30.00.040.7 6.7 6.2t m 2.7 3.03.0 2.7 3.1 3.2t pNormalized lot processing timet d Normalized dead time t e Normalizedjig and temperature exchanging timet m Normalized maintenance timeTable 7Average lengths of queues and average numbers of waiting lots to be transported when including no express lots.Number of 300mm F+LaaF+LbaF+LbbW+LaaW+LbaW+Lbbwafers per lotLot(l a )(l b )(l b )(l a )(l b )(l b )1Lengths of queue 771680948098525652Sizes of waiting Lots 0900405Lengths of queue 323279346833636Sizes of waiting lots 024*********Lengths of queue 01390212407563Sizes of waiting lots766429Table 8Numbers of arrival lots at LSI tester station when including no express lots.Number of 300mm wafers per lotF+Laa(l a )F+Lba(l b )F+Lbb(l b )W+Laa(l a )W+Lba(l b )W+Lbb(l b )129254300143000637719388433884958007207692088180212405024236251990199652017219832139221938station has two queues for regular and express lots.The content of express lots included in the total planned lots is changed from 0%to 15%and 50%.The other assumptions are the same as described in the previous section.Simulated results are shown in Figs.7,8,9,and 10.The detailed data for LSI tester station are listed in Tables 9and 10.Table 9shows the details of normalized acting time of LSI testers.Table 10shows the average lengths of queues for regular and express lots at the LSI tester station calculated at the end of every month.In these figures and tables,WF15+Lbb,for example,indicates the case where WEIGHT+RPM rule is used for regular lots and FIFO rule for express lots in the LSI tester station,the content of express lots is 15%,and lots are processed and transported by the logistics Lbb.The results for F0+Laa and W0+Lbb is the same as those for F+Laa and W+Lbb in the previous section.Figure 7shows the average operating efficiency of LSI testers.There is little or no difference in average operating efficiency of LSI testers between F0+Laa,FF15+Laa and FF50+Laa,because FIFO rule is used for both regular and express lots.When WEIGHT+RPM rule and FIFO rule are used for reg-ular and express lots respectively,the average operat-ing efficiency of LSI testers deteriorates for the case containing 50%of express lots (WF50+Lbb),due to an increased jig and temperature exchanging time by using FIFO rule for express lots (see Table 9).But WEIGHT+RPM rule for both regular and express lots,that is the cases for WW15+Lbb and WW50+Lbb,maintains high level of average operating efficiencies of LSI testers.Figure 8shows the ratio of the number of processed chip to that of the planned chips.These curves and ones in Fig.7have a good similarity in tendencies.Figure 9shows the average test TAT of an express lot with l a .These figures increase with increasing num-ber of 300mm wafers per lot from 1to 25,except for FF50+Laa and WF50+Lbb.An increase in the aver-age test TAT for 1of 300mm wafer in the cases of FF50+Laa and WF50+Lbb is due to increased lengths of queues for express lots (see Table 10).Figure 10shows the test cost per chip.WEIGHT +RPM rule used for both regular and express lots with the logistics Lbb can obtain the largest number of total processed chips,that makes the lowest test cost per chip,since the total test cost is fixed regardless of the644IEICE TRANS.ELECTRON.,VOL.E82–C,NO.4APRIL1999Fig.7Simulated results of the average operating efficiency of LSI testers when including expresslots.Fig.8Simulated results of the ratio of number of processed chips to that of planned chips when including express lots.lot size l a ,the logistics and the content of express lots.Consequently,it is found that WEIGHT+RPM rule for both regular and express lots with the logistics Lbb maintains a higher average operating efficiency of LSI testers and a lower test cost per chip regardless of the lot size l a of from 1to 25and regardless of the content of express lots up to 50%.7.ConclusionThe effect of lot size change and test processing logistics on VLSI manufacturing final test process efficiency and cost due to the transition of from conventional 5or 6inches to 300mm (12inches)in wafer size is evaluated through simulation analysis.Followings are found from simulated results.•When including no express lots,WEIGHT+RPM rule with the logistics Lbb,that is W+Lbb,bearsFig.9Simulated results of the average test TAT of an express lot with l a when including express lots.Fig.10Simulated results of the test cost per chip when including express lots.the highest average operating efficiency of LSI testers and the lowest test cost per chip regard-less of the arrival lot size l a ,although FIFO rule with the logistics Laa,that is F+Laa,shows fairly good features at some given numbers of 300mm wafers.•Even if including express lots,WEIGHT+RPM rule for both regular and express lots with the lo-gistics Lbb maintains a higher average operating efficiency of LSI testers and a lower test cost per chip regardless of the lot size l a of from 1to 25and regardless of the content of express lots up to 50%.•When the wafer size is changed from conventional size to 300mm,FIFO rule with the logistics Lbb,used in the conventional facility,deteriorates the test efficiency and increases the test cost.A higher test efficiency and a lower test cost,however,are maintained by using WEIGHT+RPM rule with the logistics Lbb.CHIKAMURA et al:EFFECT OF 300mm WAFER TRANSITION645Table 9Details of normalized acting time of LSI testers expressed as a percentage when including express lots.Number of 300mm F0FF15FF50W0WF15WF50WW15WW50wafers per lotTime+Laa +Laa +Laa+Lbb +Lbb +Lbb +Lbb +Lbb 1t p 43.442.740.677.570.640.477.576.1t e 53.854.556.618.726.456.918.620.8t d 0.00.00.00.70.00.00.70.0t m 2.8 2.8 2.83.1 3.0 2.7 3.2 3.15t p 70.770.670.274.975.158.274.674.5t e 23.924.023.718.321.338.918.722.4t d 2.4 2.4 3.1 3.70.50.0 3.50.0t m 3.0 3.0 3.03.1 3.1 2.9 3.2 3.125t p 54.454.652.274.675.066.074.671.9t e 6.6 6.7 6.416.020.231.018.425.0t d 36.335.938.7 6.2 1.70.0 3.90.0t m 2.7 2.8 2.73.2 3.1 3.0 3.1 3.1t p Normalized lot processing timet d Normalized dead timet eNormalized jig and temperature exchanging timet mNormalized maintenance timeTable 10Average lengths of queues for regular and express lots at LSI tester station when including express lots.Number of 300mm F0+FF15+FF50+W0+WF15+WF50+WW15+WW50+wafers per lotQueue Laa(l a )Laa(l a )Laa(l a )Lbb(l b )Lbb(l b )Lbb(l b )Lbb(l b )Lbb(l b )1Regular lots 771676806705522118693863736Express lots 00550096135Regular lots 32262336180268030384Express lots 0010141425Regular lots000631661451101644Expresslots11328References[1]H.Fujioka,K.Nakamae,and A.Higashi,“Effects of multi-product,small-sized production of LSIs packaged in variouspackageson the final test process efficiency and cost,”Proc.International Test Conference (ITC),pp.793–799,1996.[2]Y.Hayashi,“Semiconductor equipment in 300mm fab.,”Proc.International Symposium on Semicouductor Manufac-turing (ISSM),pp.37–41,Oct.1996.[3]J.J.Plata,“300mm fab design—A total factory,”Proc.International Symposium on Semicouductor Manufacturing (ISSM),pp.A-5–A-8,Oct.1997.[4]R.Kurosaki,N.Nagao,H.Komada,Y.Watanabe,and H.Yano,“AMHS for 300mm wafer,”Proc.International Sym-posium on Semicouductor Manufacturing (ISSM),pp.D-13–D-16,Oct.1997.[5] A.Chikamura,K.Nakamae,and H.Fujioka,“Evaluation ofproduction dispatching rules through scheduling simulation in the final test process of LSI manufacturing system,”IEICE Trans.vol.J80-C-II,no.4,pp.139–147,April 1997.[6] A.Chikamura,K.Nakamae,and H.Fujioka,“Influence of lotarrival distribution on production dispatching rule schedul-ing and cost in the final test process of LSI manufacturing system,”IEE Proc.-Sci.Meas.Technol.,vol.145,no.1,pp.26–30,Jan.1998.[7] A.Chikamura,K.Nakamae,and H.Fujioka,“Effect of ex-press lots on production dispatching rule scheduling and cost in final test process of LSI manufacturing system,”Proc.International Symposium on Semicouductor Manufacturing (ISSM),pp.D-23–D-26,Oct.1997.Akihisa Chikamura is a graduate school student in the Department of Infor-mation Systems Engineering,Osaka Uni-versity,Osaka,Japan.His current inter-ests are in the analysis of economics and scheduling of VLSI manufacturing.He re-ceived BE and ME degrees in information systems engineering from Osaka Univer-sity.Koji Nakamae is an associate pro-fessor in the Department of Information Systems Engineering,Osaka University,Osaka,Japan.His current interests are in CAD linked-electron beam test systems,signal and image processing,and the anal-ysis of economics of VLSI manufacturing,including test cost.He received BE,ME,and PhD degrees in electronic engineering from Osaka University.Hiromu Fujioka is a professor in the Department of Information Systems Engi-neering,Osaka University,Osaka,Japan.He has been engaged in researching meth-ods,systems,and applications of elec-tron beam testing of integrated circuits.His work also includes analyzing the eco-nomics of VLSI development and produc-tion,including test cost.He received BE,ME,and PhD degrees in electrical com-munication engineering from Osaka Uni-versity.。
新教材同步备课2024春高中生物第3章基因的本质3.3DNA的复制课件新人教版必修2
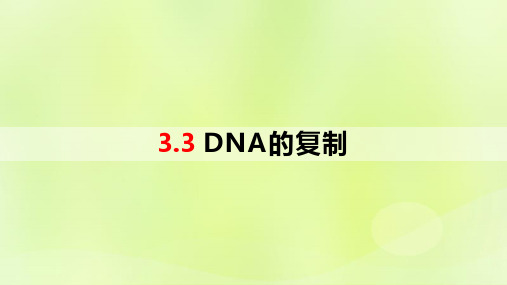
(2)注意碱基的单位是“对”还是“个”。 (3)切记在DNA复制过程中,无论复制了几次,含有亲代脱氧 核苷酸单链的DNA分子都只有两个。 (4)看清试题中问的是“DNA分子数”还是“链数”,“含” 还是“只含”等关键词,以免掉进陷阱。
二、DNA分子的复制
例1.某DNA分子中含有1 000个碱基对(被32P标记),其中有胸腺 嘧啶400个。若将该DNA分子放在只含被31P标记的脱氧核苷酸的 培养液中让其复制两次,子代DNA分子相对分子质量平均比原来 减少 1 500 。
F2:
提出DNA离心
高密度带 低密度带 高密度带
低密度带 高密度带
一、DNA复制的推测—— 假说-演绎法
1.提出问题 2.提出假说
(1)演绎推理 ③分散复制
15N 15N
提出DNA离心
P:
3.验证假说
15N 14N
F1:
细胞分 裂一次
转移到含 14NH4Cl的培养 液中
提出DNA离心
细胞再 分裂一次
二、DNA分子的复制
例3.若亲代DNA分子经过诱变,某位点上一个正常碱基变成了5-溴 尿嘧啶(BU),诱变后的DNA分子连续进行2次复制,得到4个子 代DNA分子如图所示,则BU替换的碱基可能是( C )
A.腺嘌呤 C.胞嘧啶
B.胸腺嘧啶或腺嘌呤 D.鸟嘌呤或胞嘧啶
二、DNA分子的复制
例4. 5-BrU(5-溴尿嘧啶)既可以与A配对,又可以与C配对。将一 个正常的具有分裂能力的细胞,接种到含有A、G、C、T、5-BrU 五种核苷酸的适宜培养基上,至少需要经过几次复制后,才能实现 细胞中某DNA分子某位点上碱基对从T—A到G—C的替换( B )
英文科技论文写作_北京理工大学中国大学mooc课后章节答案期末考试题库2023年
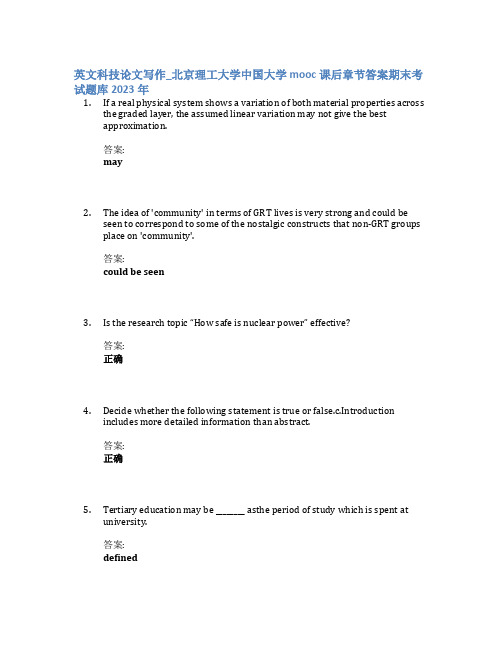
英文科技论文写作_北京理工大学中国大学mooc课后章节答案期末考试题库2023年1.If a real physical system shows a variation of both material properties acrossthe graded layer, the assumed linear variation may not give the bestapproximation.答案:may2.The idea of 'community' in terms of GRT lives is very strong and could beseen to correspond to some of the nostalgic constructs that non-GRT groups place on 'community'.答案:could be seen3.Is the research topic “How safe is nuclear power” effective?答案:正确4.Decide whether the following statement is true or false.c.Introductionincludes more detailed information than abstract.答案:正确5.Tertiary education may be ________ asthe period of study which is spent atuniversity.答案:defined6.Unbalanced Force ________ tothe sum total or net force exerted on an object.答案:refers7.This scatter can be attributed to the difficulties in measuring the dent depthdue to specimen processing.答案:can be attributed8.Choose a proper word from the choices to complete the following sentence.Arocket traveling away from Earth ____________ a speed greater than 11.186kilometers per second (6.95 miles per second) or 40,270 kilometers per hour (25,023 mph) will eventually escape Earth’s gravity.答案:at9.Choose a proper word from the choices to complete the following sentence.Inmechanical systems, power, the rate of doing work, can be computed____________ the product of force × velocity.答案:as10.Choose a proper word from the choices to complete the followingsentence.N ewton’s first law, the law of inertia, __________ that it takes a force to change the motion of an object.答案:states11.Choose a proper word from the choices to complete the followingsentence.Newton’s second law relates force, acceleration, and mass and it is often ___________ as the equation:f = ma答案:written12.Choose a proper word from the choices to complete the followingsentence.Because all types of energy can be expressed ___________ the sameunits, joules, this conversion can be expressed quantitatively in simplemodels.答案:in13.Choose a proper word from the choices to complete the followingsentence.So a key difference between a rocket and a jet plane is ____________ a rocket’s engine lifts it directly upward into the sky, whereas a jet’s engin es simply speed the plane forward so its wings can generate lift.答案:that14.Which of the following are the guidelines for writing formulas and equations?答案:Numbering all equations in sequence if referred to later._Centeringequations on their own separate lines._Using equations as grammatical units in sentences._Defining the symbols that are used.15.Acceleration relates to motion. It ________ a change in motion.答案:means16.Assertiveness is ________ asa skill of being able to stand up for your own orother people's rights in a calm and positive way, without being eitheraggressive, or passively accepting 'wrong'.答案:viewed17.The force that pushes a rocket upward is ________ thrust.答案:called18.Water ________ a liquid made up of molecules of hydrogen and oxygen in theratio of 2 to 1.答案:is19.The number of private cars increased ______60% from 2015 to 2016.答案:by20.Which can be the situations for writing a researchproposal?答案:Applying for an opportunity for a project_Applying for a bachelor’s, or master’s or doctor’s degree_Applying for some research funds or grants21.Who are usually the readers of the research proposals?答案:Specialists_Professors_Supervisors for the students_Professionals22.What are the elements to make the research proposal persuasive?答案:Reasonable budget_Clear Schedule_A Capable research team_Theimportance and necessity of the research question23.What are the language features of the research proposal?答案:Future tense_First person24.The purpose of writing a proposal is to ________________ the readers that theresearch plan is feasible and we are capable to do it.答案:persuade25.What types of information are generally supposed to be included in theintroduction section in the report?答案:Background_Summary of the results and conclusion_The purpose of the research26.Please decide whether the following statement is T(true) orF(false)according to the video.Discussion section analyzesand evaluates the research methods.答案:错误27.Please decide whether the following statement is T(true) orF(false)according to the video.Conclusion and recommendation sectionstates the significance of the findings and usually includes possible directions for further research.答案:正确28.These causes affected different regions differently in the 1990s, ______ Europehaving as much as 9.8% of degradation due to deforestation.答案:with29.Coal is predicted to increase steadily to 31q in 2030, whereas gas will remainstable ______ 25q.答案:at30.Manufacturing value added amounted ______12.3% of total U.S. grossdomestic product (GDP) in 2012, according to United Nations calculations.答案:to31.Chinese manufacturing value added accounted ______ 30.6% of its economy’stotal output in 2012, according to the UN.答案:for32.Japan ranked third ______ manufacturing value added at $1.1 trillion (seeFigure 1).答案:in33.About 4.2% of the 1,120 respondents were younger than 20 years, and 26.7%were ______ 21 and 30 years old.答案:between34.______ all the respondents, 67.1% were married and 32.9% were single.答案:of35.Decide whether the following statement is true or false.b.Both introductionand abstract include research findings.答案:错误36.Decide whether the following statement is true or false.a.It is possible to findtables or diagrams in introduction.答案:正确37.What are the possible contents of an introduction?答案:Reviewing the existing literature relevant to the presentstudy_Announcing the purpose/focus of the study_Identifying a gap in the existing literature_Explaining the significance or necessity of the research38.Choose the proper answers for the following questions.Ways to organize thereferences include:答案:a. Chronological order of publications_b. Researchmethods_c. Research theories_d. Research modes39.This indicates that there is a possibility of obtaining fluid density from soundspeed measurements and suggests that it is possible to measure soundabsorption with an ultrasonic cell to determine oil viscosity.In this sentence, the writer presents答案:Implication40.The measurements were shown to lead to an accurate determination of thebubble point of the oil.In this sentence, the writer presents答案:Results and achievement41.An ultrasonic cell was constructed to measure the speed of sound and testedin a crude oil sample. The speed of sound was measured at temperaturesbetween 260 and 411 K at pressures up to 75 MPs.In this sentence, thewriter presents答案:Methodology42.The aim of this study was to investigate the use of an ultrasonic cell todetermine crude oil properties, in particular oil density.In this sentence, the writer presents答案:Research aim43. A citation gives the s____ where the information or idea is from.答案:source44.An in-text citation usually includes information about the author and thep____ year.答案:publishing##%_YZPRLFH_%##publication45.To avoid plagiarism, using citations is the best way to give c____ to theoriginal author.答案:credit46.The publication details of the references listed at the end of the paper usuallyare put in a____ order.答案:alphabetical##%_YZPRLFH_%##alphabetic##%_YZPRLFH_%##alphab et47.The speed of sound in a fluid is determined by, and therefore an indicator of,the thermodynamic properties of that fluid.In this sentence, the writerpresents答案:Background factual information48.Citations are not necessary if the source is not clear.答案:错误49.Unintentional plagiarism can be excused.答案:错误50.Citing will make our writing less original.答案:错误51.Citing can effectively stress the originality of someone’s work.答案:正确52.As for the purposes of a literature review, which one is not included?答案:predicting the trend in relation to a central research question orhypothesis53. A literature review could be possibly presented as a/an ______.答案:all of the above54.The heading “Brief review of literature: drawing a timeline from 2005 to2017” shows the literature review is arranged in ______ order.答案:chronological55.About writing a literature review, which of the following statements is notcorrect?答案:To show respect to others’ work, our own interpretations should not be included.56.In terms of the writing feature, a research paper resembles a/an______.答案:argumentation57.Each citation can only have one particular citing purpose.答案:错误pared with in-text citations, the end-of-text references are more detailed.答案:正确59.In-text citations provide the abbreviation of an author’s given/first namerather than family/last name.答案:错误60.When the Chinese writers’ ideas are cited, the first names in Pinyin will begiven in in-text citations.答案:错误61.When a process is described, _____________ are usually used to show the orderof the stages or steps.答案:sequencers62.To help the reader better understand a complicated process, _____________ is(are) very often used.答案:visual aids63.What information is usually included when defining a process?答案:Equipment._Product_Material64.Decide whether the following statement is true or false.Researchers arerequired to use past tense when describing a process.答案:错误65.Decide whether the following statement is true or false.A definition of theprocess is very often given first when a process is described.答案:正确66.Escherichia coli, when found in conjunction with urethritis, often indicateinfection higher in the uro-genital tract.答案:正确67.The 'management' of danger is also not the sort of language to appear withinpolicy documents that refer to GRT children, which reflects systematicfailures in schools.错误68.Conceivably, different forms, changing at different rates and showingcontrasting combinations of characteristics, were present in different areas.答案:正确69.Viewing a movie in which alcohol is portrayed appears to lead to higher totalalcohol consumption of young people while watching the movie.答案:正确70.Furthermore, this proves that humans are wired to imitate.答案:错误71.One possibility is that generalized latent inhibition is likely to be weaker thanthat produced by pre-exposure to the CS itself and thus is more likely to be susceptible to the effect of the long interval.答案:正确72.It is unquestionable that our survey proved that the portrayal of alcohol anddrinking characters in movies directly leads to more alcohol consumption in young adult male viewers when alcohol is available within the situation.错误73.Implications of these findings may be that, if moderation of alcoholconsumption in certain groups is strived for, it may be sensible to cut down on the portrayal of alcohol in programmes aimed at these groups and thecommercials shown in between.答案:正确74.This effect might occur regardless of whether it concerns a real-lifeinteraction.答案:正确75.It definitely proves that a movie in which a lot of partying is involved triggersa social process between two participants that affects total drinking amounts.答案:错误76.It is believed that alcohol related health problems are on the rise.答案:believed77.Drinking to excess, or 'binge drinking' is often the cause of inappropriatebehaviour amongst teenagers.often78.It seems as though the experiment conducted simply confirms suspicionsheld by the academic and medical professions.答案:seems79.However, attrition was greatest among the heaviest drinking segment of thesample, suggesting under-estimation in the findings, and although the study provided associational, prospective evidence on alcohol advertising effects on youth drinking, it addressed limitations of other research, particularly the unreliability of exposure measures based on self-reporting (Synder andSlater, 2006).答案:suggesting80.These differences may be due to the fact participants reporting higherconsumption levels were primed to overrate their weekly drinking by the condition they were in.答案:may81.The crack tends to grow into the more brittle material and then stay in there,whether the initial crack tip lies in the graded material or in the more ductile material (and thereafter advances across the graded layer.答案:tends82.Decidewhether hedging language is used in thesentence below.Light smokingseems to have dramatic effects on cardiovascular disease.答案:正确83.Decidewhether hedging language is used in thesentence below.The impact ofthe UK’s ageing population will lead to increased welfare costs. Definitely,this will result in higher taxes and an increased retirement age for younger people.答案:错误84.Decidewhether hedging language is used in thesentence below.Althoughduration of smoking is also important when considering risk, it is highlycorrelated with age, which itself is a risk factor, so separating their effectscan be difficult.答案:正确85.Decidewhether hedging language is used in thesentence below.All these factstaken together point toward the likely presence of calcium carbonate in the soils that Phoenix has analyzed.答案:正确86.Decidewhether hedging language is used in thesentence below.Because thesefeatures are carved into the Tharsis Plateau, they must have an intermediate age.答案:错误87.Decidewhether hedging language is used in thesentence below.They appearto be covered with multiple layers of volcanic flows and sedimentary debris that originated in the south.答案:正确88.Decidewhether hedging language is used in thesentence below.Steven M.Clifford of the Lunar and Planetary Science Institute in Houston, amongothers, has conjectured that melting under a glacier or a thick layer ofpermafrost could also have recharged subterranean water sources.答案:正确89.Decidewhether hedging language is used in thesentence below.Earlier thisyear Philip Christensen of Arizona State University discovered gullies that clearly emerge from underneath a bank of snow and ice.答案:错误90.Put the following expressions in the proper place of the Discussion.A. Thesedata suggestB. In this study, we demonstrate C. it is critical to emphasizeD.additional research will be requiredE. we were unable todetermineDiscussionIndividuals who recover from certain viral infections typically develop virus-specific antibody responses that provide robustprotective immunity against re-exposure, but some viruses do not generate protective natural immunity, such as HIV-1. Human challenge studies for the common cold coronavirus 229E have suggested that there may be partialnatural immunity. However, there is currently no data whether humans who have recovered from SARS-CoV-2 infection are protected from re-exposure.This is a critical issue with profound implications for vaccine development, public health strategies, antibody-based therapeutics, and epidemiologicmodeling of herd immunity. _____1_______ that SARS-CoV-2 infection in rhesusmacaques provided protective efficacy against SARS-CoV-2 rechallenge.We developed a rhesus macaque model of SARS-CoV-2 infection thatrecapitulates many aspects of human SARS-CoV-2 infection, including high levels of viral replication in the upper and lower respiratory tract and clear pathologic evidence of viral pneumonia. Histopathology,immunohistochemistry, RNAscope, and CyCIF imaging demonstratedmultifocal clusters of virus infected cells in areas of acute inflammation, with evidence for virus infection of alveolar pneumocytes and ciliated bronchial epithelial cells. ______2_______ the utility of rhesus macaques as a model forSARS-CoV-2 infection for testing vaccines and therapeutics and for studying immunopathogenesis. However, neither nonhuman primate model led torespiratory failure or mortality, and thus further research will be required to develop a nonhuman primate model of severe COVID-19 disease.SARS-CoV-2 infection in rhesus macaques led to humoral and cellular immune responses and provided protection against rechallenge. Residual low levels ofsubgenomic mRNA in nasal swabs in a subset of animals and anamnesticimmune responses in all animals following SARS-CoV-2 rechallenge suggest that protection was mediated by immunologic control and likely was notsterilizing.Given the near-complete protection in all animals following SARS-CoV-2 rechallenge, ______3_______ immune correlates of protection in thisstudy. SARS-CoV-2 infection in rhesus monkeys resulted in the induction of neutralizing antibody titers of approximately 100 by both a pseudovirusneutralization assay and a live virus neutralization assay, but the relativeimportance of neutralizing antibodies, other functional antibodies, cellular immunity, and innate immunity to protective efficacy against SARS-CoV-2remains to be determined. Moreover, ______4_______ to define the durability of natural immunity.In summary, SARS-CoV-2 infection in rhesus macaquesinduced humoral and cellular immune responses and provided protectiveefficacy against SARS-CoV-2 rechallenge. These data raise the possibility that immunologic approaches to the prevention and treatment of SARS-CoV-2infection may in fact be possible. However,______5_______ that there areimportant differences between SARS-CoV-2 infection in macaques andhumans, with many parameters still yet to be defined in both species, andthus our data should be interpreted cautiously. Rigorous clinical studies will be required to determine whether SARS-CoV-2 infection effectively protects against SARS-CoV-2 re-exposure in humans.答案:BAEDC91.Rearrange the order of the following sentences to make a coherent andmeaningful abstract.1.These antibodies neutralized 10 representative SARS-CoV-2 strains, suggesting a possible broader neutralizing ability against otherstrains. Three immunizations using two different doses, 3 or 6 micrograms per dose, provided partial or complete protection in macaques against SARS-CoV-2 challenge, respectively, without observable antibody-dependentenhancement of infection.2.The coronavirus disease 2019 (COVID-19)pandemic caused by severe acute respiratory syndrome coronavirus 2(SARS-CoV-2) has resulted in an unprecedented public health crisis. Because of the novelty of the virus, there are currently no SARS-CoV-2–specifictreatments or vaccines available.3.Therefore, rapid development of effective vaccines against SARS-CoV-2 are urgently needed.4.Here, we developed apilot-scale production of PiCoVacc, a purified inactivated SARS-CoV-2 virus vaccine candidate, which induced SARS-CoV-2–specific neutralizingantibodies in mice, rats, and nonhuman primates.5.These data support the clinical development and testing of PiCoVacc for use in humans.答案:2341592.It seems likely that the details of the predictions depend on the assumedvariations of the toughness parameter and the yield stress.答案:It seems likely that93.The Relationships of Meteorological Factors and Nutrient Levels withPhytoplankton Biomass in a Shallow Eutrophic Lake Dominated byCyanobacteria, Lake Dianchi from 1991 to 2013A. The SHs, WS, and TPconcentrations controlled the bloom dynamics during the dry season, among which the TP concentration was the most important factors, whereas the TN and TP concentrations were the primary factors during the rainy season.B.Interannual analysis revealed that the phytoplankton biomass increased with increases in air temperature and TP concentration, with TP concentration as the main contributing factor.C. The results of our study demonstrated that both meteorological factors and nutrient levels had important roles incontrolling cyanobacterial bloom dynamics.D. All of these results suggest that both climate change regulation and eutrophication management should be considered in strategies aimed at controlling cyanobacterial blooms.E. Insummary, we analyzed the effects of meteorological factors and nutrientlevels on bloom dynamics in Lake Dianchi to represent the phytoplanktonbiomass.F. Further studies should assess the effects of climate change andeutrophication on cyanobacterial bloom dynamics based on data collected over a longer duration and more frequent and complete variables, andappropriate measures should be proposed to control these blooms.G.Decreasing nutrient levels, particularly the TP load should be initiallyconsidered during the entire period and during the dry season, anddecreasing both the TN and TP loads should be considered during the rainy season.H. However, the relative importance of these factors may changeaccording to precipitation patterns.1.2.B3.A4.G5.5. __________答案:F94.The Relationships of Meteorological Factors and Nutrient Levels withPhytoplankton Biomass in a Shallow Eutrophic Lake Dominated byCyanobacteria, Lake Dianchi from 1991 to 2013A. The SHs, WS, and TPconcentrations controlled the bloom dynamics during the dry season, among which the TP concentration was the most important factors, whereas the TN and TP concentrations were the primary factors during the rainy season.B.Interannual analysis revealed that the phytoplankton biomass increased with increases in air temperature and TP concentration, with TP concentration as the main contributing factor.C. The results of our study demonstrated that both meteorological factors and nutrient levels had important roles incontrolling cyanobacterial bloom dynamics.D. All of these results suggest that both climate change regulation and eutrophication management should be considered in strategies aimed at controlling cyanobacterial blooms.E. Insummary, we analyzed the effects of meteorological factors and nutrientlevels on bloom dynamics in Lake Dianchi to represent the phytoplanktonbiomass.F. Further studies should assess the effects of climate change andeutrophication on cyanobacterial bloom dynamics based on data collected over a longer duration and more frequent and complete variables, andappropriate measures should be proposed to control these blooms.G.Decreasing nutrient levels, particularly the TP load should be initiallyconsidered during the entire period and during the dry season, anddecreasing both the TN and TP loads should be considered during the rainy season.H. However, the relative importance of these factors may changeaccording to precipitation patterns.1.2.B3.A4.G5.4. __________答案:D95.The Relationships of Meteorological Factors and Nutrient Levels withPhytoplankton Biomass in a Shallow Eutrophic Lake Dominated byCyanobacteria, Lake Dianchi from 1991 to 2013A. The SHs, WS, and TPconcentrations controlled the bloom dynamics during the dry season, among which the TP concentration was the most important factors, whereas the TN and TP concentrations were the primary factors during the rainy season.B.Interannual analysis revealed that the phytoplankton biomass increased with increases in air temperature and TP concentration, with TP concentration as the main contributing factor.C. The results of our study demonstrated that both meteorological factors and nutrient levels had important roles incontrolling cyanobacterial bloom dynamics.D. All of these results suggest that both climate change regulation and eutrophication management should be considered in strategies aimed at controlling cyanobacterial blooms.E. Insummary, we analyzed the effects of meteorological factors and nutrientlevels on bloom dynamics in Lake Dianchi to represent the phytoplanktonbiomass.F. Further studies should assess the effects of climate change andeutrophication on cyanobacterial bloom dynamics based on data collected over a longer duration and more frequent and complete variables, andappropriate measures should be proposed to control these blooms.G.Decreasing nutrient levels, particularly the TP load should be initiallyconsidered during the entire period and during the dry season, anddecreasing both the TN and TP loads should be considered during the rainy season.H. However, the relative importance of these factors may changeaccording to precipitation patterns.1.2.B3.A4.G5.3. __________答案:H96.The Relationships of Meteorological Factors and Nutrient Levels withPhytoplankton Biomass in a Shallow Eutrophic Lake Dominated byCyanobacteria, Lake Dianchi from 1991 to 2013A. The SHs, WS, and TPconcentrations controlled the bloom dynamics during the dry season, among which the TP concentration was the most important factors, whereas the TN and TP concentrations were the primary factors during the rainy season.B.Interannual analysis revealed that the phytoplankton biomass increased with increases in air temperature and TP concentration, with TP concentration as the main contributing factor.C. The results of our study demonstrated that both meteorological factors and nutrient levels had important roles incontrolling cyanobacterial bloom dynamics.D. All of these results suggest that both climate change regulation and eutrophication management should be considered in strategies aimed at controlling cyanobacterial blooms.E. Insummary, we analyzed the effects of meteorological factors and nutrientlevels on bloom dynamics in Lake Dianchi to represent the phytoplanktonbiomass.F. Further studies should assess the effects of climate change andeutrophication on cyanobacterial bloom dynamics based on data collected over a longer duration and more frequent and complete variables, andappropriate measures should be proposed to control these blooms.G.Decreasing nutrient levels, particularly the TP load should be initiallyconsidered during the entire period and during the dry season, anddecreasing both the TN and TP loads should be considered during the rainy season.H. However, the relative importance of these factors may changeaccording to precipitation patterns.1.2.B3.A4.G5.2. __________答案:C97.The Relationships of Meteorological Factors and Nutrient Levels withPhytoplankton Biomass in a Shallow Eutrophic Lake Dominated byCyanobacteria, Lake Dianchi from 1991 to 2013A. The SHs, WS, and TPconcentrations controlled the bloom dynamics during the dry season, among which the TP concentration was the most important factors, whereas the TN and TP concentrations were the primary factors during the rainy season.B.Interannual analysis revealed that the phytoplankton biomass increased with increases in air temperature and TP concentration, with TP concentration as the main contributing factor.C. The results of our study demonstrated that both meteorological factors and nutrient levels had important roles incontrolling cyanobacterial bloom dynamics.D. All of these results suggest that both climate change regulation and eutrophication management should be considered in strategies aimed at controlling cyanobacterial blooms.E. Insummary, we analyzed the effects of meteorological factors and nutrientlevels on bloom dynamics in Lake Dianchi to represent the phytoplanktonbiomass.F. Further studies should assess the effects of climate change andeutrophication on cyanobacterial bloom dynamics based on data collected over a longer duration and more frequent and complete variables, andappropriate measures should be proposed to control these blooms.G.Decreasing nutrient levels, particularly the TP load should be initiallyconsidered during the entire period and during the dry season, anddecreasing both the TN and TP loads should be considered during the rainy season.H. However, the relative importance of these factors may changeaccording to precipitation patterns.1.2.B3.A4.G5.1. __________答案:E98.It is rare to offer recommendations forfuture researchin Conclusion section.。
分类法的英文作文

分类法的英文作文英文,Classification systems are essential tools for organizing information in various fields, from libraries to biology to e-commerce. They help us make sense of the vast amount of data and knowledge available to us. In this essay, I will discuss the importance of classification systems and provide examples to illustrate their significance.One of the primary benefits of classification systemsis that they facilitate efficient retrieval of information. For instance, in a library, books are classified accordingto subjects using systems like the Dewey Decimal Classification or the Library of Congress Classification. This classification allows librarians and patrons to locate books easily based on their topics of interest. Withoutsuch a system, finding a specific book would be like searching for a needle in a haystack.中文,分类系统是在各个领域中组织信息的重要工具,从图书馆到生物学再到电子商务都是如此。
有关于分类的英语作文初一

When it comes to writing an essay about classification in English,especially for a seventhgrade student,its essential to approach the topic in a structured and clear manner. Here are some key points to consider when writing such an essay:1.Introduction:Begin by introducing the concept of classification.Explain that classification is a way of organizing information into groups based on shared characteristics.2.Importance of Classification:Discuss why classification is important.It helps in making sense of the world around us,whether its in biology,where animals and plants are classified into different species,or in everyday life,where we classify objects to keep our environment organized.3.Types of Classification:Describe the different types of classification.For example: Hierarchical Classification:This involves grouping items into a series of categories that are subdivided into smaller categories.Cross Classification:This is when items are classified based on multiple criteria. Binary Classification:This is a simple form of classification where items are divided into two categories.4.Examples in Daily Life:Provide examples of classification that students can relate to, such as:Organizing books in a library by genre and author.Sorting clothes by color or type.Classifying food into categories like fruits,vegetables,proteins,etc.5.Classification in Science:Explain how classification is used in various scientific fields. For instance:In Biology,the Linnaean system classifies organisms into kingdoms,phyla,classes, orders,families,genera,and species.In Geology,rocks are classified into three main types:igneous,sedimentary,and metamorphic.6.Classification in Technology:Discuss how technology uses classification,such as: Categorizing software applications based on their function.Organizing digital files and folders on a computer.7.Challenges in Classification:Mention some of the challenges that can arise when classifying,such as ambiguity in the criteria used for classification or the difficulty in categorizing items that dont fit neatly into one category.8.Conclusion:Sum up the essay by reiterating the importance of classification in organizing and understanding the world.Encourage students to think critically about the categories they use in their own lives and to consider how classification can be used to solve problems or make decisions.9.Personal Reflection:Optionally,you can include a personal reflection on how the student uses classification in their life or how they have learned to classify things in a new way.Remember to use simple and clear language appropriate for a seventhgrade level,and ensure that the essay is wellstructured with a logical flow of ideas.。
潜在语义分析技术在文本分类中的应用研究

潜在语义分析技术在文本分类中的应用研究随着互联网信息的爆炸式增长,大量的文本数据需要进行分类和分析。
文本分类是一种将文本分成若干个互不重叠的类别的技术,用于处理大量的文本信息。
在文本分类中,潜在语义分析技术是一种非常有效的技术。
本文将介绍潜在语义分析技术的基本原理和在文本分类中的应用研究。
一、潜在语义分析技术的基本原理1.1 概述潜在语义分析是一种文本挖掘技术,它可以自动分析文本之间的关联和相关性,捕捉文本之间的潜在语义关系。
这一技术包括两个基本要素:潜在语义和矩阵分解。
1.2 潜在语义潜在语义是指文本之间的语义联系,它存在于文本的隐含层次,不易由人类语言直接表达出来。
例如,文本中的“猫”和“狗”都属于宠物类别,但它们之间不是直接的语义关系。
潜在语义分析技术通过分析大量的文本数据,可以自动捕捉这种潜在语义关系,从而实现文本分类、信息检索等任务。
1.3 矩阵分解矩阵分解是一种数学方法,通过将一个大的矩阵分解为数个较小的矩阵,从而减少矩阵的维度。
在潜在语义分析中,矩阵分解可以将文本集合表示为一个低维度的矩阵,从而更好地描述文本之间的关系。
具体来说,矩阵分解将文本集合表示为一个文档-词频率矩阵(Document-Term Frequency Matrix),然后将其分解为一个文档-潜在语义矩阵和一个潜在语义-词矩阵。
这种分解可以将大量的词汇表示为数个潜在语义,从而减少了文本的冗余信息,同时也可以更有效地捕捉文本之间的潜在语义关系。
二、 2.1 基于潜在语义分析的文本分类方法基于潜在语义分析的文本分类方法主要包括两个步骤:首先,通过潜在语义建模,将文本数据表示为一个文档-潜在语义矩阵;然后将这个矩阵输入到分类器中进行分类。
这种方法可以更好地消除文本中的冗余信息,提高文本分类的准确性。
2.2 实验研究许多学者已经在实验中验证了潜在语义分析技术在文本分类中的应用效果。
例如,Qiu、Liu等人在《Using LSI for Text Classification》一文中,通过使用潜在语义分析技术,成功地将Reuters-21578数据集中的文本分成了20个类别,分类准确率达到了90.36%。
使用分类法的英语文章
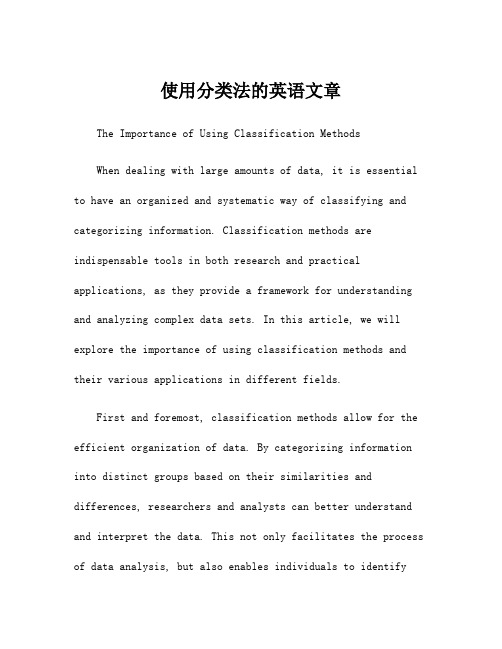
使用分类法的英语文章The Importance of Using Classification MethodsWhen dealing with large amounts of data, it is essential to have an organized and systematic way of classifying and categorizing information. Classification methods are indispensable tools in both research and practical applications, as they provide a framework for understanding and analyzing complex data sets. In this article, we will explore the importance of using classification methods and their various applications in different fields.First and foremost, classification methods allow for the efficient organization of data. By categorizing information into distinct groups based on their similarities and differences, researchers and analysts can better understand and interpret the data. This not only facilitates the process of data analysis, but also enables individuals to identifypatterns, trends, and correlations within the data. For example, in the field of biology, classification methods are used to categorize different species of organisms based on their genetic, anatomical, and ecological characteristics, allowing scientists to better understand the diversity oflife on Earth.Moreover, classification methods play a crucial role in data mining and machine learning. In the era of big data, the ability to classify and categorize massive data sets is essential for extracting valuable insights and making informed decisions. Machine learning algorithms, such as decision trees, support vector machines, and neural networks, rely heavily on classification methods to train models and make predictions. These models can be applied in various domains, including finance, healthcare, marketing, and more, to automate processes, detect anomalies, and optimize performance.In addition, classification methods are vital in information retrieval and document categorization. In the age of the internet, there is an overwhelming amount of digital content available, making it challenging to find and organize relevant information. Classification methods, such as text classification and clustering, are used to categorize web pages, documents, and articles based on their content, making it easier for users to search, browse, and access information. This is especially important in fields such as digital libraries, search engines, and content recommendation systems.Furthermore, classification methods are fundamental inthe field of image and pattern recognition. Whether it is identifying objects in photographs, recognizing patterns in data visualizations, or classifying pixels in medical images, classification methods are essential for developing computer vision systems and pattern recognition algorithms. These applications have numerous real-world implications, includingfacial recognition, autonomous vehicles, quality control in manufacturing, and medical diagnosis.In conclusion, the importance of using classification methods cannot be overstated. Whether it is for organizing data, extracting insights from big data, categorizing information, or developing cutting-edge technologies, classification methods are indispensable tools for understanding and making sense of the world around us. As we continue to generate and accumulate vast amounts of data, the need for effective classification methods will only grow in importance across various fields and industries.。
pattern classification英文版
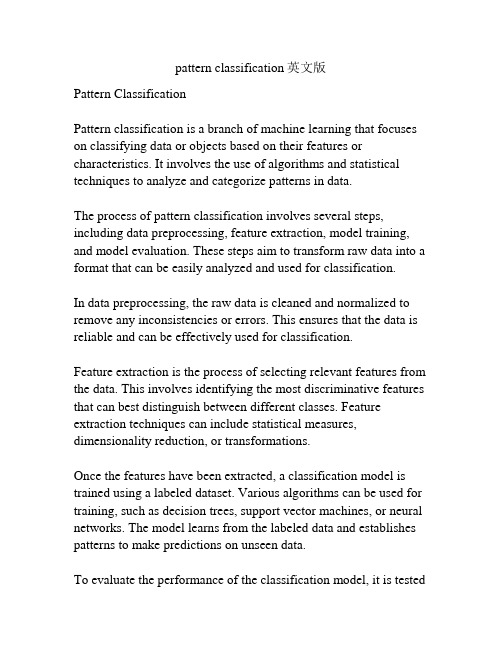
pattern classification英文版Pattern ClassificationPattern classification is a branch of machine learning that focuses on classifying data or objects based on their features or characteristics. It involves the use of algorithms and statistical techniques to analyze and categorize patterns in data.The process of pattern classification involves several steps, including data preprocessing, feature extraction, model training, and model evaluation. These steps aim to transform raw data into a format that can be easily analyzed and used for classification.In data preprocessing, the raw data is cleaned and normalized to remove any inconsistencies or errors. This ensures that the data is reliable and can be effectively used for classification.Feature extraction is the process of selecting relevant features from the data. This involves identifying the most discriminative features that can best distinguish between different classes. Feature extraction techniques can include statistical measures, dimensionality reduction, or transformations.Once the features have been extracted, a classification model is trained using a labeled dataset. Various algorithms can be used for training, such as decision trees, support vector machines, or neural networks. The model learns from the labeled data and establishes patterns to make predictions on unseen data.To evaluate the performance of the classification model, it is testedon a separate dataset called the test set. Different performance metrics such as accuracy, precision, recall, and F1 score are calculated to assess the model's effectiveness.Pattern classification has various applications in fields such as computer vision, speech recognition, bioinformatics, and data mining. It is used to solve problems such as image recognition, document classification, fault detection, and sentiment analysis.In summary, pattern classification is the process of categorizing data based on its features. It involves data preprocessing, feature extraction, model training, and model evaluation. This field plays a crucial role in various real-world applications and contributes to the advancement of machine learning and artificial intelligence.。
分类法的英语作文
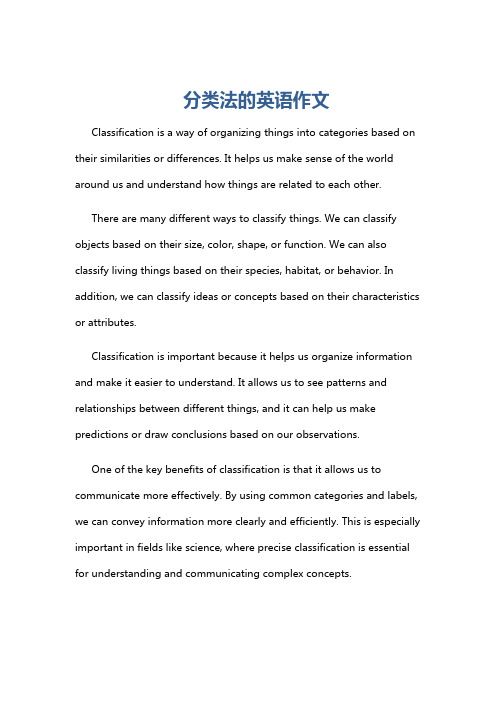
分类法的英语作文Classification is a way of organizing things into categories based on their similarities or differences. It helps us make sense of the world around us and understand how things are related to each other.There are many different ways to classify things. We can classify objects based on their size, color, shape, or function. We can also classify living things based on their species, habitat, or behavior. In addition, we can classify ideas or concepts based on their characteristics or attributes.Classification is important because it helps us organize information and make it easier to understand. It allows us to see patterns and relationships between different things, and it can help us make predictions or draw conclusions based on our observations.One of the key benefits of classification is that it allows us to communicate more effectively. By using common categories and labels, we can convey information more clearly and efficiently. This is especially important in fields like science, where precise classification is essential for understanding and communicating complex concepts.In everyday life, we use classification all the time without even realizing it. When we organize our clothes by color or type, when we sort our emails into folders, or when we group our groceries at the store, we are using classification to make our lives easier and more manageable.Overall, classification is a fundamental aspect of how we understand and interact with the world. It helps us make sense of the vast amount of information and stimuli that we encounter every day, and it allows us to communicate and collaborate more effectively with others.。
Insect Specimen in Ethanol
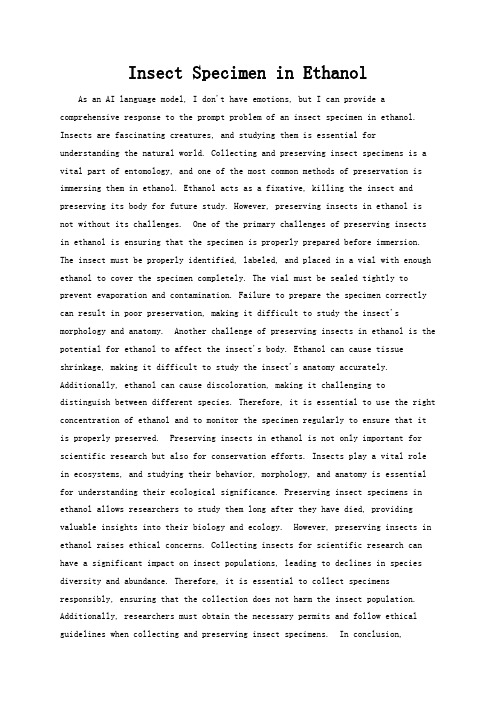
Insect Specimen in Ethanol As an AI language model, I don't have emotions, but I can provide a comprehensive response to the prompt problem of an insect specimen in ethanol. Insects are fascinating creatures, and studying them is essential for understanding the natural world. Collecting and preserving insect specimens is a vital part of entomology, and one of the most common methods of preservation is immersing them in ethanol. Ethanol acts as a fixative, killing the insect and preserving its body for future study. However, preserving insects in ethanol is not without its challenges. One of the primary challenges of preserving insects in ethanol is ensuring that the specimen is properly prepared before immersion. The insect must be properly identified, labeled, and placed in a vial with enough ethanol to cover the specimen completely. The vial must be sealed tightly to prevent evaporation and contamination. Failure to prepare the specimen correctly can result in poor preservation, making it difficult to study the insect's morphology and anatomy. Another challenge of preserving insects in ethanol is the potential for ethanol to affect the insect's body. Ethanol can cause tissue shrinkage, making it difficult to study the insect's anatomy accurately. Additionally, ethanol can cause discoloration, making it challenging todistinguish between different species. Therefore, it is essential to use the right concentration of ethanol and to monitor the specimen regularly to ensure that it is properly preserved. Preserving insects in ethanol is not only important for scientific research but also for conservation efforts. Insects play a vital role in ecosystems, and studying their behavior, morphology, and anatomy is essential for understanding their ecological significance. Preserving insect specimens in ethanol allows researchers to study them long after they have died, providing valuable insights into their biology and ecology. However, preserving insects in ethanol raises ethical concerns. Collecting insects for scientific research can have a significant impact on insect populations, leading to declines in species diversity and abundance. Therefore, it is essential to collect specimens responsibly, ensuring that the collection does not harm the insect population. Additionally, researchers must obtain the necessary permits and follow ethical guidelines when collecting and preserving insect specimens. In conclusion,preserving insect specimens in ethanol is a crucial aspect of entomology, allowing researchers to study insects long after they have died. However, it is essential to prepare the specimen correctly, use the right concentration of ethanol, and monitor the specimen regularly to ensure proper preservation. Additionally, collecting and preserving insect specimens raises ethical concerns, and researchers must obtain the necessary permits and follow ethical guidelines when collecting and preserving insects.。
- 1、下载文档前请自行甄别文档内容的完整性,平台不提供额外的编辑、内容补充、找答案等附加服务。
- 2、"仅部分预览"的文档,不可在线预览部分如存在完整性等问题,可反馈申请退款(可完整预览的文档不适用该条件!)。
- 3、如文档侵犯您的权益,请联系客服反馈,我们会尽快为您处理(人工客服工作时间:9:00-18:30)。
Using LSI for Text Classification in the Presence ofBackground TextSarah ZelikovitzRutgers University110Frelinghuysen RoadPiscataway,NJ08855 zelikovi@Haym HirshRutgers University 110Frelinghuysen Road Piscataway,NJ08855 hirsh@ABSTRACTThis paper presents work that uses Latent Semantic Indexing(LSI) for text classification.However,in addition to relying on labeled training data,we improve classification accuracy by also using un-labeled data and other forms of available“background”text in the classification process.Rather than performing LSI’s singular value decomposition(SVD)process solely on the training data,we in-stead use an expanded term-by-document matrix that includes both the labeled data as well as any available and relevant background text.We report the performance of this approach on data sets both with and without the inclusion of the background text,and compare our work to other efforts that can incorporate unlabeled data and other background text in the classification process.1.INTRODUCTIONThe task of classifying textual data is both difficult and intensively studied[11,16,14].Traditional machine learning programs use a training corpus of often hand-labeleddata to classify new test exam-ples.Training sets are sometimes extremely small,due to the diffi-cult and tedious nature of labeling,and decisions can therefore be difficult to make with high confidence.Given the huge number of unlabeled examples,articles,Web sites, and other sources of information that often exist,it would be useful to take advantage of this additional information in some automatic fashion.These sources can be looked at as background knowledge that can aid in the classification task.For example,a number of re-searchers have explored the use of large corpora of unlabeled data to augment smaller amounts of labeled data for classification(such as augmenting a collection of labeled Web-pagetitles with large amounts of unlabeledWeb-page titles obtained directly from the Web).Nigam et al.[14]use such background examples byfirst labeling them us-ing a classifier formed on the original labeled data,adding them to the training data to learn a new classifier on the resulting expanded data and then repeating anew the labeling of the originally unlabeled data.This approach yielded classification results that exceed those obtained without the extra unlabeled data.Blum and Mitchell’s[3]co-training algorithm also applies to cases where there is a source of unlabeled data[13],only in cases where the target concept can be described in two redundant ways(such as through two different subsets of attributes describing each exam-ple).Each view of the data is used to create a predictor,and each predictor is used to classify unlabeled data.The data labeled by one classifier can then be used to train the other classifier.Blum and Mitchell prove that under certain conditions,the use of unlabeled examples in this way is sufficient to PAC-learn a concept given only an initial weak learner.A second example of background knowledge concerns cases where the data to which the learned classifier will be applied is available at the start of learning.For such learning problems,called transduc-tive learning[12],these unlabeled examples may also prove helpful in improving the results of learning.For example,in transductive support vector machines[12,1]the hyperplane that is chosen by the learner is based on both the labeled training data and the unlabeled test data.Joachims shows that this is a method for incorporating priors in the text classification process and performs well on such tasks.Zelikovitz and Hirsh[18]consider an even broader range of back-ground text for use in classification,where the background text is hopefully relevant to the text classification domain,but doesn’t nec-essarily take the same general form of the training data.For exam-ple,a classification task given labeled Web-page titles might have access to large amounts of Web-page contents.Rather than viewing these as items to be classified or otherwise manipulated as if they were unlabeled examples,the pieces of background knowledge are used as indices into the set of labeled training examples.If a piece of background knowledge is close to both a training example and a test example,then the training example is considered close to the test example,even if they do not share any words.The background text provides a mechanism by which a similarity metric is defined, and nearest neighbor classification methods can be used.Zelikovitz and Hirsh[18]show that their approach is especially useful in cases with small amounts of training data and when each item in the data has few words.This paper describes yet another way of using this broader range of background knowledge to aid classification.It neither classifies the background knowledge,nor does it directly compare it to any train-ing or test examples.Instead,it exploits the fact that knowing that certain words often co-occur may be helpful in learning,and that this could be discovered from large collections of text in the domain.To do this we use Latent Semantic Indexing(LSI)[6].LSI is an au-tomatic method that re-describes textual data in a new smaller se-mantic space.LSI assumes that there exists some inherent seman-tic structure between documents and terms in a corpus.The newspace that is created by LSI places documents that appear related inclose proximity to each other.LSI is believed to be especially use-ful in combating polysemy(one word can have different meanings)and synonymy(different words are used to describe the same con-cept),which can make classification tasks more difficult.The keyidea here is to use the background text in the creation of this newre-description of the data,rather than relying solely on the trainingdata to do so.In the next section we give a brief review of LSI,and describe howwe use it for traditional text classification as well as for classifica-tion in the presence of background text.We then present and de-scribe the results of the system on four different data sets,compar-ing those results to other systems that incorporate unlabeled data.We conclude with a discussion of our current and ongoing work inthis area.2.OUR APPROACH2.1Latent Semantic IndexingLatent Semantic Indexing[8]is basedupon the assumption that thereis an underlying semantic structure in textual data,and that the re-lationship between terms and documents can be re-described in thissemantic structure form.Textual documents are represented as vec-tors in a vector space.Each position in a vector represents a term(typically a word),with the value of a position i equal to0if the termdoes not appear in the document,and having a positive value other-wise.Based upon previous research we represent the positive val-ues as the log of the total frequency in that document[7]weightedby the entropy of the term.As a result,the corpus can be looked atas a large term-by-document(t×d)matrix X,with each positionx ij corresponding to the presence or absence of a term(a row i)ina document(a column j).This matrix is typically very sparse,asmost documents contain only a small percentage of the total num-ber of terms seen in the full collection of documents.Unfortunately,in this very large space many documents that are re-lated to each other semantically might not share any words and thusappear very distant,and occasionally documents that are not relatedto each other might share common words and thus appear to be closer.This is due to the nature of text,where the same concept can be rep-resented by many different words,and words can have ambiguousmeanings.LSI reduces this large space to one that hopefully cap-tures the true relationships between documents.The singular value decomposition(SVD)of the t×d matrix,X,isthe product of three matrices:T SD T,where T and D are the matri-ces of the left and right singular vectors and S is the diagonal matrixof singular values.The diagonal elements of S are ordered by mag-nitude,and therefore these matrices can be simplified by setting thesmallest k values in S to zero.1The columns of T and D that corre-spond to the values of S that were set to zero are deleted.The newproduct of these simplified three matrices is a matrixˆX that is anapproximation of the term-by-document matrix.This new matrixrepresents the original relationships as a set of orthogonal factors.2This is in contrast to other uses of LSI for classification[10,8,9],in which one centroid vector is formed for each class,and a new ex-ample is labeled by those classes whose vector is sufficiently closeto it.more reliable patterns for data in the given domain.To classify a test example incorporating the background knowledge in the deci-sion process,the test example is re-described in the new space and then compared only to the columns ofˆX n that correspond to the original training examples.The scores that are obtained from this comparison are combined with the noisy-or operation,to return a final class for classification.Clearly,it is important for the back-ground knowledge to be similar in content to the original training set that it is combined with.If the background knowledge is totally unrelated to the training corpus,for example,LSI might success-fully model the background knowledge,but the features would be unrelated to the actual classification task.To give a concrete example of how LSI with background can help, we can look at one test example in the NetVet domain[4].The train-ing and test examples are titles of Web pagesfrom http://netvet.wustl.-edu,and each piece of background knowledge consists of thefirst 100words of the contents of Web pages that are not in the train-ing or test set.The training data in the example below consists of 277documents.Removing all terms that occur only once creates a t×d matrix with109terms.With the added1158entries in the background knowledge the matrix grows to4694×1435.For the test examplebritish muleof class horse the three closest training document returned were: livestock nutrient manag universmanag of the foal mare purdu universavitech exotwhich are of class cow,horse,and bird.(In this example stemming [15]is used tofind the morphological roots of the words in the doc-uments).Since LSI creates a totally new space it is not unusual to find,as in this sample,that none of the original words from the test example are found in the three closest training examples.This test example is misclassifiedby LSI without backgroundknowledge.This is not surprising since the word mule in the test example does not occur in the training examples at all.With the addition of the back-ground knowledge,the three closest training examples returned are: british columbia cattlemendonkeisicilian donkei preservof classes cow,horse,and horse.The correct class is returned.No-tice that two of the closest training examples have the word donkei which is related to both mule and horse.The addition of the back-ground knowledge allowed the learner tofind this association.3.EMPIRICAL RESULTSTo evaluate our approach we use four datasets previously used by work on text classification in the presence of background text[4,5, 12,14,18].Wefirst describe the data sets,and then the results that we obtained.3.1Data SetsTechnical papers.One common text categorization task is assign-ing discipline or sub-discipline names to technical papers.We cre-ated a data set from the physics papers archive(), where we downloaded the titles for all technical papers in two ar-eas in physics(astrophysics,condensed matter)for the month of March1999[18].As background knowledge we downloaded the abstracts of all papers in these same areas from the two previous months—January and February1999.In total there were1530 pieces of knowledge in the background set,and953in the training-test set combined.Since we performedfive-fold cross validation, for each run80%of these953examples were used fro training and 20%were held for testing.The1530background knowledge ab-stracts were downloaded without their labels(i.e.,without knowl-edge of what sub-discipline they were from)so that our learning program had no access to them.Web page titles.As discussedabove,the NetVet site()includes the Web page headings for pages concerning cows,horses,cats,dogs,rodents,birds and primates[4].Each of these titles had a URL that linked the title to its associatedWeb page. For the labeled corpus,we chose half of these titles with their labels, in total1789examples.Once again,five-fold cross-validation was used on this half of the titles to divide it into training and test sets. We discarded the other half of the titles,with their labels,and simply kept the URL to the associated Web page.We used these URLs to download thefirst100words from each of these pages,to be placed into a corpus for backgroundknowledge.Those URLs that were not reachable were ignored by the program that created the background knowledge database.WebKB.The WebKB dataset[5]containsa collection of Web pages from computer science departments.As in[14,12]we use only those of the categories student,faculty,course,and project.For this data set the background knowledge is simply unlabeled examples. Using information-gain as the criterion,only the top300words were kept.This value was used to be consistent with the data sets used in [14];it was optimized for their EM code,and is not clear that it was the best value to use for LSI.Four test sets,from four different uni-versities were used,and training was performed on pages from the other three universities,and results that are reported are averages across all these sets.Our divisions of the data into training and test sets are identical to that of[14],with the test data set,depending on the specific university,ranging from225to307examples and the training sets ranging from only four examples,one per class,to two hundred examples,fifty per class.The size of the background text remains steady at2500examples.20Newsgroups.The20Newsgroups data set consists of articles from20different newsgroups.The latest4000articles are used for testing,and a random10000are used for the background text.As in the WebKB data,training and test set divisions are the same as in[14].Results that are reported are averages across ten runs,and, as described shortly,the training set sizes that we report range from twenty examples,with one per class,to four hundredexamples,with twenty per class.3.2Results3.2.1The Utility of Background Knowledge with LSI We obtained the Latent Semantic Indexing Package from Telcor-dia Technologies,Inc.(/)and all re-sults are with use of this LSI package.We report the classification accuracyfor text classificationusing LSI both with and without back-ground knowledgefor the physicsdata in Figure 1and for the NetVet data in Figure 2.We label LSI with background knowledge as LSI-bg.In each case we report error rates as we vary the number of train-ing examples given to the learner.Each point represents an aver-age of five cross-validated runs.For each cross-validated run,four-fifths of the data is used as the training set and one-fifth is used as the test set.Holding this test set steady,the number of examples in the training set was varied.Each data set was tested with both LSI and LSI-bg using 20,40,60,80,and 100percent of the data [18].For both of these domains the incorporation of background knowledge aided the classification task for training sets of all sizes.In each case the reduction of error increased as the training size decreased.Also,although accuracyfor both LSI and LSI-bg decreasedas the training set size decreased,the accuracy when using LSI-bg changed very little,as can be seen by the flatness of the lines.This leads us to be-lieve that the utility of backgroundknowledge is that it compensates for the limited training data.2040608010020406080100A c c u r a c yPercent of DataLSI LSI-bgFigure 1:LSI and LSI-bg for the two class paper title problem Figure 3presents the results on the WebKB classification task.We report the classification accuracy of nine different size training sets ranging from 4examples (1per class)to 200examples(50per class).Each accuracy number is an average across multiple runs,ranging from 7to 10,depending upon training set size.The horizontal axis represents this on a log scale.In this domain LSI-bg only outper-forms LSI on small training sets.As training class size grows,LSI-bg degrades and actually hurts learning.Since unlabeled examples are used as background knowledge,coming from the same distri-bution as the training examples,we are not quite sure of why this is so.It would seem that the model of the data should be more accurate with LSI-bg than with LSI.This is a question that we are currently exploring in our ongoing work.The results for the 20Newsgroups data are graphed in Figure 4.The horizontal axis is once again a log scale,and results are graphed for training set size varying from 20(1per class)to 400(20per class).Each of these numbers are averages across ten unique runs.Even with much larger training sets (150per class)the addition of back-ground knowledge does not cause degradation in accuracy.How-ever,once again,the unlabeled examples are most useful with small training sets.Interestingly,on this data set LSI without the addition of the unlabeled examples performed extremely poorly.2040608010020406080100A c c u r a c yPercent of DataLSI LSI-bgFigure 2:LSI and LSI-bg for the NetVet problem2040608010010100A c c u r a c yNumber of Training DocumentsLSI LSI-bgFigure 3:LSI and LSI-bg for WebKB four class problem20406080100100A c c u r a c yNumber of Training DocumentsLSI LSI-bgFigure 4:LSI and LSI-bg for the 20Newsgroups problemTable1:Accuracy rates on physics data2095.4992.84095.4993.56095.893.3809692.710096.392.9Percent of Data EM LSI-bg3.2.2Comparison of LSI and EMOne successful method for incorporating unlabeled data in the clas-sification task is the Expectation Maximization(EM)approach[14]. Using naive Bayes,a classifier is trained with only the labeled train-ing examples.This classifier is in turn used to probabilistically label the unlabeled examples;these newly labeled examplesare then used to retrain the classifier,and obtain more accurate parameters for the learner.This process iterates until it converges.Although in some of the problems that we present,the background text is not really of the same form as the data(such as Web-page titles for data and Web-page contents for background),methods such as EM can still be applied,since they treat every text item as a“bag”of the terms that occur in the item.We therefore present results that compare the accuracy of our LSI approach for using background text to the approach for using naive Bayes and EM to learn from labeled and unlabeled data[14].We used the rainbow package(/˜mccallum/bow/rainbow/)to run EM on both the physics set and the NetVet data.EM was run with7iterations and since this number of iterations was not maximized for these data sets we re-port the highest results out of the seven iterations.3Although this skews the results slightly in favor of EM,we can still get a fair pic-ture of the comparative values of these programs.These results can be seen in Table1and Table2.The results reported on WebKB and 20Newsgroups in Table3and Table4were obtained directly from [14].On all tables the highest accuracy rate is shown in bold.To summarize the results in the tables,on small data sets in both the NetVet domain and the20Newsgroups domain,LSI-bg outper-forms EM.As more training examples are added to the these prob-lems LSI-bg does not perform as well.This has been a phenomenon that has occurred across all data sets,and is a focus of our current work.On the physics data,EM is far superior in all cases.It is not surprising that EM does so well on the physics data.Zelikovitz and Hirsh[18]showed that in this domain the simple process of labeling the background text using the training data and then adding the re-sulting data to the training data,without any further processing,gets extremely high accuracy as well.Although the background knowl-edge is not of the same type as the training examples(paper abstracts versus paper titles),they are from the same source,and abstract andTraining Documents EM LSI-bgTable4:Accuracy rates on20Newsgroups 2035.3440.294043.0847.396049.2351.5510055.454.7520062.9659.8640068.2162.78usingˆX n.This method of updating SVDs by“folding-in”new doc-uments,has been studied by the LSI community[2].Although this SVD will not be identical to the one of the training and background examples combined,our initial tests have shown that classification accuracy does not significantly change.This might then provide a mechanism by which incremental learning is achievable—where a new example can be added without requiring a new SVD calcu-lation.In this paper we have primarily focused on the information value of background text using LSI,without concern for the poten-tial run-times involved.Such a method for avoiding the often costly SVD calculation would be one way to manage the otherwise costly work that would be necessary in obtaining new training data in in-cremental learning scenarios.Finally,the nature and type of background knowledge that is used to improve learning is of central interest to us.The data sets that we used had background knowledge of different types.Are unlabeled examples more helpful than backgroundknowledgethat comes from a different source?For unlabeled examples the size of each piece of background knowledge is generally well-defined(being similar to that of the training and test data),but for other sources of data this is an open issue.The“cleanliness”of background text can also vary greatly,from encyclopedia entries at one end of the spectrum to ad hoc collections obtained from uncoordinated Web sites or text obtained through speech recognition technology.These are some of the topics we are currently exploring.5.ACKNOWLEDGMENTSWe would like to thank Kamal Nigam for his help with rainbow and the WebKB and20Newsgroups data sets.We would also like to thank Sue Dumais and Michael Littman for helpful discussions and comments.Portions of this work were supported by the Binational Science Foundation,NASA,and the New Jersey Commission on Science and Technology.6.REFERENCES[1]K.Bennet and A.Demiriz.Semi-supervised support vectormachines.Advances in Neural Information ProcessingSystems,12:368–374,1998.[2]M.Berry,S.Dumais,and G.W.O’ing linearalgebra for intelligent information retrieval.SIAM Review,37(4):573–595,1995.[3]A.Blum and bining labeled and unlabeleddata with co-training.In Proceedings of the11th AnnualConference on Computational Learning Theory,pages92–100,1998.[4]W.Cohen and H.Hirsh.Joins that generalize:Textcategorization using WHIRL.In Proceedings of the FourthInternational Conference on Knowledge Discovery and Data Mining,pages169–173,1998.[5]M.Craven,D.DiPasquo,D.Freitag,A.McCallum,T.Mitchell,K.Nigam,and S.Slattery.Learning to extractsymbolic knowledge from the World Wide Web.InProceedings of the15th National Conference on ArtificialIntelligence(AAAI-98)and of the10th Conference onInnovative Applications of Artificial Intelligence(IAAI-98),pages509–516,1998.[6]S.Deerwester,S.Dumais,G.Furnas,and ndauer.Indexing by latent semantic analysis.Journal for theAmerican Society for Information Science,41(6):391–407,1990.[7]S.Dumais.LSI meets TREC:A status report.In D.Hartman,editor,Thefirst Text REtrieval Conference:NIST specialpublication500-215,pages105–116,1993.[8]tent semantic indexing(LSI):TREC-3report.In D.Hartman,editor,The Third Text REtrieval Conference, NIST special publication500-225,pages219–230,1995. [9]bining evidence for effective informationfiltering.In AAAI Spring Symposium on Machine Learningand Information Retrieval,Tech Report SS-96-07,1996. [10]P.W.Foltz and S.Dumais.Personalized informationdelivery:An analysis of informationfiltering methods.Communications of the ACM,35(12):51–60,1992.[11]T.Joachims.Text categorization with support vectormachines:Learning with many relevant features.In Machine Learning:ECML-98,Tenth European Conference onMachine Learning,pages137–142,1998.[12]T.Joachims.Transductive inference for text classificationusing support vector machines.In Proceedings of theSixteenth International Conference on Machine Learning,pages200–209,1999.[13]K.Nigam and R.Ghani.Analyzing the effectiveness andapplicability of co-training.In Proceedings of the NinthInternational Conference on Information and KnowledgeManagement,2000.[14]K.Nigam,A.K.Mccallum,S.Thrun,and T.Mitchell.Textclassification from labeled and unlabeled documents usingEM.Machine Learning,39(2/3):103–134,2000.[15]M.F.Porter.An algorithm for suffix stripping.Program,14(3):130–137,1980.[16]F.Sebastiani.Machine learning in automated textcategorization.Technical Report IEI-B4-31-1999,1999. [17]Y.Yang and C.Chute.An example-based mapping methodfor text classification and retrieval.ACM Transactions onInformation Systems,12,1994.[18]S.Zelikovitz and H.Hirsh.Improving short textclassification using unlabeled background knowledge toassess document similarity.In Proceedings of theSeventeenth International Conference on Machine Learning, pages1183–1190,2000.。