决策树示例
决策树ID3例题经典案例

决策树ID3例题经典案例
决策树ID3例题是探究决策树原理的经典案例。
它的主要内容是利用决策树对一组状态变量输入,按照规则变化,最终得出一组结论。
决策树ID3例题的经典案例如下:
假设有一组客户,他们的信息如下:
客户年龄收入是否有孩子是否有信用卡
A 35 150 有有
B 25 100 有无
C 60 150 无有
D 35 200 无有
这个案例中,状态变量为年龄、收入、是否有孩子、是否有信用卡,如果想要利用决策树分析得出最终结论,可以根据如下规则进行对比:
(1)根据年龄,将客户分为年轻客户和中老年客户;
(2)根据收入,将客户分为低收入和高收入;
(3)根据是否有孩子,将客户分为家庭客户和非家庭客户;
(4)根据是否有信用卡,将客户分为信用良好客户和信用较差客户。
以上就是用决策树ID3例题的经典案例,通过综合分析多个状态变量,最终得出一组划分客户的结论,以便采取相应的策略。
- 1 -。
《建设工程监理实务与案例分析》—12决策树
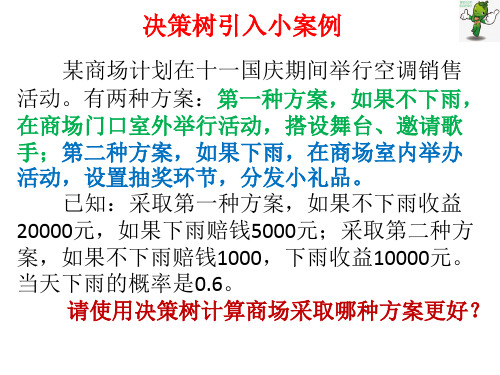
某商场计划在十一国庆期间举行空调销售 活动。有两种方案:第一种方案,如果不下雨, 在商场门口室外举行活动,搭设舞台、邀请歌 手;第二种方案,如果下雨,在商场室内举办 活动,设置抽奖环节,分发小礼品。
已知:采取第一种方案,如果不下雨收益 20000元,如果下雨赔钱5000元;采取第二种方 案,如果不下雨赔钱1000,下雨收益10000元。 当天下雨的概率是0.6。
数据进行分析,得到每种方案的利润和出现概 率见表4-8。如果投标未果,则会损失3万元 (投标准备费)。
决策树示例:
案例:
某具有相应资质的承包商经研究决定Байду номын сангаас与该
工程投标。经造价工程师估价,该工程估算成本 为1500万元,其中材料费占60%。经研究有高、 中、低三个报价方案,其利润率为10%、7%、 4%,根据过去类似工程的投标经验,相应的中 标概率分别为0.3、0.6、0.9。编制投标文件的费 用为5万元。该工程业主在投标文件中明确规定 采用固定总价合同。据统计,在施工过程中材料 费可能平均上涨3%,其发生概率为0.4。
决策树示例: 某工程监理单位拥有的资源有限,只能在
A和B两项大型工程中选A或B进行投标,或均不 参加投标。若投标,根据过去投标经验,对两 项工程各有高低报价两种策略。投高价标,中 标机会为30%;投低价标,中标机会为50%。 这样,该工程监理单位共有A高、A低、不投标、 B高、B低五种方案。
工程监理单位根据过去承担过的类似工程
请使用决策树计算商场采取哪种方案更好?
决策树分析法
决策树分析法是适用于风险型决策分
析的一种简便易行的实用方法,其特点是 用一种树状图表示决策过程,通过事件出 现的概率和损益期望值的计算比较,帮助 决策者对行动方案作出抉择。当工程监理 单位不考虑竞争对手的情况,仅根据自身 实力决定某些工程是否投标及如何报价时, 则是典型的风险型决策问题,适用于决策 树进行分析。
人工智能决策树例题经典案例
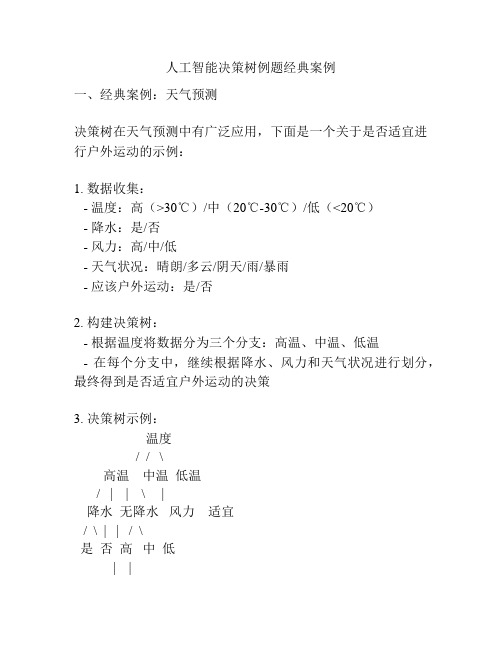
人工智能决策树例题经典案例一、经典案例:天气预测决策树在天气预测中有广泛应用,下面是一个关于是否适宜进行户外运动的示例:1. 数据收集:- 温度:高(>30℃)/中(20℃-30℃)/低(<20℃)- 降水:是/否- 风力:高/中/低- 天气状况:晴朗/多云/阴天/雨/暴雨- 应该户外运动:是/否2. 构建决策树:- 根据温度将数据分为三个分支:高温、中温、低温- 在每个分支中,继续根据降水、风力和天气状况进行划分,最终得到是否适宜户外运动的决策3. 决策树示例:温度/ / \高温中温低温/ | | \ |降水无降水风力适宜/ \ | | / \是否高中低| |不适宜适宜- 如果温度是高温且有降水,则不适宜户外运动- 如果温度是高温且无降水,则根据风力判断,如果风力是高,则不适宜户外运动,如果风力是中或低,则适宜户外运动 - 如果温度是中温,则不论降水和风力如何,都适宜户外运动- 如果温度是低温,则需要考虑风力,如果风力是高,则适宜户外运动,如果风力是中或低,则不适宜户外运动4. 参考内容:决策树的构建和应用:决策树通过对输入特征进行划分,构建了一棵树形结构,用于解决分类或回归问题。
构建决策树主要包括数据预处理、特征选择、划分策略和停止条件等步骤。
特征选择可以使用信息增益、基尼指数等算法,划分策略可以使用二叉划分或多叉划分,停止条件可以是叶子节点纯度达到一定阈值或达到预定的树深度。
决策树的应用包括数据分类、特征选择和预测等任务。
天气预测案例中的决策树:将天气预测问题转化为分类问题,通过构建决策树,可以得到识别是否适宜户外运动的规则。
决策树的决策路径可以用流程图或树状图表示,帮助理解和解释决策过程。
决策树的节点表示特征值,分支表示判断条件,叶子节点表示分类结果。
决策树的生成算法可以基于启发式规则或数学模型,如ID3、C4.5、CART等。
决策树的优缺点:决策树具有可解释性强、易于理解和实现、能处理非线性关系等优点。
决策树在生活中的例子

决策树在生活中的例子决策树是一种常见的机器学习算法,它通过一系列的决策规则来对数据进行分类或预测。
这些决策规则可以用树状图形式表示,每个内部节点表示一个特征或属性,每个叶子节点表示一个类别或是一个预测的结果。
决策树的可解释性和易于理解的特点使得它在生活中有着广泛的应用。
以下是生活中常见的决策树的例子:1.疾病诊断决策树:医生在进行疾病诊断时,通常会根据患者的症状和检测结果,利用决策树来判断患者可能患有的疾病类型。
例如,医生可以通过询问患者是否有发烧、咳嗽等症状来进行初步判断,然后根据进一步的检测结果来确认疾病的类型。
2.贷款申请决策树:银行在进行贷款申请审核时,会根据申请人的个人信息(如年龄、工作年限、征信记录等)以及贷款金额等因素,利用决策树来判断该申请是否应该得到批准。
例如,银行可以根据申请人的年龄和工作年限来判断其信用等级,从而决定是否批准贷款申请。
3.产品推荐决策树:电商网站通常会根据用户的浏览历史、购买记录等信息,利用决策树来推荐相关产品给用户。
例如,当一个用户浏览了几个相似的产品时,网站可以根据用户的浏览记录和购买记录来推测该用户对其他相似产品的兴趣,从而向用户推荐相关的产品。
4.旅行路线决策树:当人们计划旅行时,他们通常会面临选择旅行目的地和路线的问题。
决策树可以帮助人们做出决策。
例如,当人们根据旅行偏好、时间和预算等因素,在一组候选目的地中进行选择时,决策树可以根据这些因素来帮助人们决定最佳的旅行目的地和路线。
5.个人理财决策树:当人们面临个人理财决策时,决策树可以作为辅助工具。
例如,当人们考虑是否购买其中一种投资产品时,决策树可以根据他们的风险偏好、投资目标和市场状况等因素来帮助他们决策。
决策树还可以帮助人们制定个人预算和理财计划。
决策树在生活中的应用不仅限于上述例子,而且还可以扩展到更多的领域,例如教育、市场营销、自然灾害预测等。
通过采用适当的特征选择和数据预处理方法,决策树能够提供准确和可解释的结果,使得人们能够更好地做出决策。
决策树算法最经典应用案例

决策树算法最经典应用案例决策树算法是一种常用的机器学习算法,它可以应用于各种实际问题,帮助人们做出决策。
下面列举了决策树算法的十个经典应用案例。
1. 银行贷款风险评估银行可以使用决策树算法来评估客户的信用风险,根据客户的个人信息、收入情况、信用记录等特征,构建决策树模型,预测客户是否有偿还贷款的能力。
2. 电商推荐系统电商平台可以利用决策树算法根据用户的历史购买记录、浏览行为、个人偏好等信息,构建决策树模型,实现个性化的商品推荐,提高用户购买转化率。
3. 医学诊断医生可以使用决策树算法来辅助诊断疾病。
根据患者的症状、生理指标、病史等特征,构建决策树模型,帮助医生判断患者是否患有某种疾病,从而指导治疗方案。
4. 电影评分预测在线视频平台可以利用决策树算法根据用户的观看历史、评分记录、影片类型等信息,构建决策树模型,预测用户对未观看的电影的评分,从而为用户推荐感兴趣的电影。
5. 股票市场预测投资者可以使用决策树算法来预测股票市场的涨跌。
根据股票的历史交易数据、市场指标、财务数据等特征,构建决策树模型,预测股票的涨跌趋势,指导投资决策。
6. 人脸识别人脸识别系统可以利用决策树算法根据人脸图像的特征,构建决策树模型,识别出不同的人脸。
决策树的每个节点表示一个特征的判断,通过逐层判断,最终确定人脸的身份。
7. 自然语言处理自然语言处理任务中,如情感分析、文本分类等,可以使用决策树算法来构建模型,根据文本的词频、句法结构等特征,判断文本的情感倾向或类别。
8. 网络安全检测网络安全检测系统可以使用决策树算法来识别恶意攻击。
根据网络流量的特征、用户行为等信息,构建决策树模型,判断网络流量是否存在安全风险。
9. 智能交通智能交通系统可以利用决策树算法根据交通流量、车速、天气等信息,构建决策树模型,预测交通拥堵情况,为驾驶员提供最佳出行路线。
10. 疾病预测医疗领域可以利用决策树算法根据患者的基因、病史、生活习惯等特征,构建决策树模型,预测患者是否患有某种遗传性疾病,从而进行早期干预和治疗。
决策树例题经典案例280
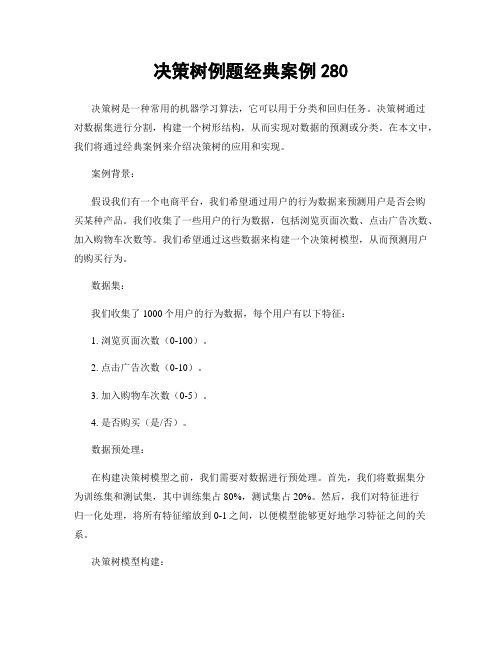
决策树例题经典案例280决策树是一种常用的机器学习算法,它可以用于分类和回归任务。
决策树通过对数据集进行分割,构建一个树形结构,从而实现对数据的预测或分类。
在本文中,我们将通过经典案例来介绍决策树的应用和实现。
案例背景:假设我们有一个电商平台,我们希望通过用户的行为数据来预测用户是否会购买某种产品。
我们收集了一些用户的行为数据,包括浏览页面次数、点击广告次数、加入购物车次数等。
我们希望通过这些数据来构建一个决策树模型,从而预测用户的购买行为。
数据集:我们收集了1000个用户的行为数据,每个用户有以下特征:1. 浏览页面次数(0-100)。
2. 点击广告次数(0-10)。
3. 加入购物车次数(0-5)。
4. 是否购买(是/否)。
数据预处理:在构建决策树模型之前,我们需要对数据进行预处理。
首先,我们将数据集分为训练集和测试集,其中训练集占80%,测试集占20%。
然后,我们对特征进行归一化处理,将所有特征缩放到0-1之间,以便模型能够更好地学习特征之间的关系。
决策树模型构建:我们使用Python中的scikit-learn库来构建决策树模型。
首先,我们选择合适的决策树算法,比如ID3、CART等。
然后,我们通过训练集来训练模型,调整模型的参数,如最大深度、最小样本分割数等,以提高模型的准确性和泛化能力。
模型评估:在模型训练完成后,我们需要对模型进行评估。
我们使用测试集来评估模型的准确性、精确度、召回率等指标,以及绘制ROC曲线、混淆矩阵等来评估模型的性能。
模型预测:最后,我们使用训练好的决策树模型来对新的用户数据进行预测。
当有新的用户数据输入时,模型可以根据用户的行为特征来预测用户是否会购买产品,从而为电商平台提供决策支持。
总结:通过本案例的介绍,我们了解了决策树在电商平台中的应用。
决策树模型可以通过用户的行为数据来预测用户的购买行为,为电商平台提供决策支持。
同时,我们也学习了如何使用Python中的scikit-learn库来构建和评估决策树模型。
python决策树经典案例

python决策树经典案例以Python决策树经典案例为题,列举以下十个案例。
1. 预测鸢尾花品种鸢尾花数据集是一个经典的分类问题,其中包含了三个不同品种的鸢尾花的测量数据。
通过使用决策树算法,我们可以根据花瓣和花萼的长度、宽度等特征,预测鸢尾花的品种。
2. 判断信用卡申请的风险在信用卡申请过程中,银行需要评估申请人的信用风险。
使用决策树算法,我们可以根据申请人的个人信息(如年龄、收入、债务等),预测其信用卡申请是否有风险。
3. 识别垃圾邮件垃圾邮件是每个人都会遇到的问题,而决策树可以帮助我们自动识别垃圾邮件。
通过对邮件的主题、发送者、内容等特征进行分析,决策树可以判断一封邮件是否为垃圾邮件。
4. 预测房价房价预测是房地产市场中的一个重要问题。
通过使用决策树算法,我们可以根据房屋的各种特征(如面积、地理位置、卧室数量等),预测房屋的价格。
5. 识别植物病害农作物病害的及时识别对于农业生产非常重要。
使用决策树算法,可以根据植物叶片的形状、颜色、纹理等特征,判断植物是否受到病害的侵袭。
6. 预测股票涨跌股票市场的波动性很大,而决策树可以用来预测股票的涨跌。
通过分析股票的历史数据和各种市场指标,决策树可以预测股票的未来走势。
7. 判断病人是否患有某种疾病医疗诊断是决策树算法的另一个应用领域。
通过分析病人的症状、体征等信息,决策树可以帮助医生判断病人是否患有某种疾病,并给出相应的治疗建议。
8. 预测客户流失率对于一家公司来说,客户流失是一个重要的问题。
通过使用决策树算法,我们可以根据客户的消费行为、购买记录等信息,预测客户的流失率,并采取相应的措施来留住客户。
9. 判断某人是否适合借贷在金融行业中,决策树可以用来评估某个人是否适合借贷。
通过分析个人的收入、信用记录、职业等信息,决策树可以判断一个人是否有能力偿还借款。
10. 识别手写数字手写数字识别是机器学习领域中的一个经典问题。
通过使用决策树算法,可以根据手写数字的像素点信息,准确地识别出手写数字是哪个数字。
决策树例题经典案例
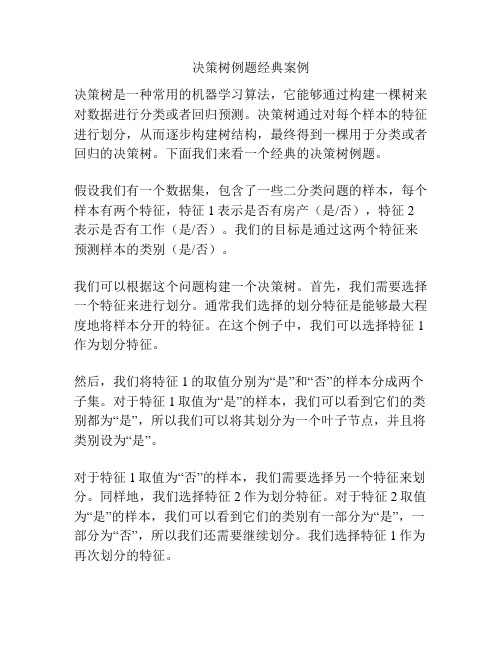
决策树例题经典案例决策树是一种常用的机器学习算法,它能够通过构建一棵树来对数据进行分类或者回归预测。
决策树通过对每个样本的特征进行划分,从而逐步构建树结构,最终得到一棵用于分类或者回归的决策树。
下面我们来看一个经典的决策树例题。
假设我们有一个数据集,包含了一些二分类问题的样本,每个样本有两个特征,特征1表示是否有房产(是/否),特征2表示是否有工作(是/否)。
我们的目标是通过这两个特征来预测样本的类别(是/否)。
我们可以根据这个问题构建一个决策树。
首先,我们需要选择一个特征来进行划分。
通常我们选择的划分特征是能够最大程度地将样本分开的特征。
在这个例子中,我们可以选择特征1作为划分特征。
然后,我们将特征1的取值分别为“是”和“否”的样本分成两个子集。
对于特征1取值为“是”的样本,我们可以看到它们的类别都为“是”,所以我们可以将其划分为一个叶子节点,并且将类别设为“是”。
对于特征1取值为“否”的样本,我们需要选择另一个特征来划分。
同样地,我们选择特征2作为划分特征。
对于特征2取值为“是”的样本,我们可以看到它们的类别有一部分为“是”,一部分为“否”,所以我们还需要继续划分。
我们选择特征1作为再次划分的特征。
对于特征2取值为“否”的样本,我们可以看到它们的类别都为“否”,所以我们可以将其划分为一个叶子节点,并且将类别设为“否”。
对于特征2取值为“是”,特征1取值为“否”的样本,我们可以看到它们的类别全部为“否”,所以我们可以将其划分为一个叶子节点,并且将类别设为“否”。
最后,我们得到的决策树如下:特征1是|--- 类别:是特征1否|--- 特征2是| |--- 类别:是||--- 特征2否|--- 类别:否通过这个决策树,我们可以根据样本的特征值来进行预测。
例如,对于一个样本,特征1为“是”,特征2为“否”,按照决策树的规则,我们可以预测该样本的类别为“否”。
决策树是一种直观、易于解释的机器学习算法,它能够根据数据集的特征进行分类或者预测,对于解决一些简单的二分类问题非常有效。
决策树算法例题

决策树算法例题
一、决策树基本概念与原理
决策树是一种基于树结构的分类与回归模型。
它通过一系列的问题对数据进行划分,最终得到叶子节点对应的分类结果或预测值。
决策树的构建过程通常采用自上而下、递归划分的方法。
二、决策树算法实例解析
以一个简单的决策树为例,假设我们要预测一个人是否喜欢户外运动。
已知特征:性别、年龄、是否喜欢晒太阳。
可以通过以下决策树划分:
1.根据性别划分,男性为喜欢户外运动,女性为不喜欢户外运动。
2.若性别为男性,再根据年龄划分,年龄小于30分为喜欢户外运动,大于30分为不喜欢户外运动。
3.若性别为女性,无论年龄如何,均分为喜欢户外运动。
通过这个决策树,我们可以预测一个人是否喜欢户外运动。
三、决策树算法应用场景及优缺点
1.应用场景:分类问题、回归问题、关联规则挖掘等。
2.优点:易于理解、可解释性强、泛化能力较好。
3.缺点:容易过拟合、对噪声敏感、构建过程耗时较长。
四、实战演练:构建决策树解决实际问题
假设我们要预测房价,已知特征:面积、卧室数量、卫生间数量、距市中心距离。
可以通过构建决策树进行预测:
1.选择特征:根据相关性分析,选择距市中心距离作为最佳划分特征。
2.划分数据集:将数据集划分为训练集和测试集。
3.构建决策树:采用递归划分方法,自上而下构建决策树。
4.模型评估:使用测试集评估决策树模型的预测性能。
通过以上步骤,我们可以运用决策树算法解决实际问题。
决策树例题(详细分析“决策树”共10张)

• 第四步:确定决策方案:在比较方案考虑的是收益值 时,那么取最大期望值;假设考虑的是损失时,那么 取最小期望值。
• 根据计算出的期望值分析,此题采取开工方案较 好。
第7页,共10页。
决策树例题
第1页,共10页。
决策树的画法
• A、先画一个方框作为出发点,又称决策节点; • B、从出发点向右引出假设干条直线,这些直线叫做方案
枝;
• C、在每个方案枝的末端画一个圆圈,这个圆圈称为 概率分叉点,或自然状态点;
• D、从自然状态点引出代表各自然状态的分枝,称 为概率分枝;
• E、如果问题只需要一级决策,那么概率分枝末端画 三角形,表示终点 。
第四步:确定决策方案:在比较方案考虑的是收益值时,那么取最大期望值;
B、从出发点向右引出假设干条直线,这些直线叫做方案枝;
天气好 0.3 根据计算出的期望值分析,此题采取开工方案较好。
假设考虑的是损失时,那么取最小期望值。 A、先画一个方框作为出发点,又称决策节点;
40000
-1000
天气坏
0.7
-10000
第8页,共10页。
【例题9】
方案 A高 A低 B高 B低
效果
优 一般 赔 优 一般 赔 优 一般 赔 优 一般 赔
可能的利润(万元)
5000 1000 -3000 4000 500 -4000 7000 2000 -3000 6000 1000 -1000
概率
0.3 0.5 0.2 0.2 0.6 0.2 0.3 0.5 0.2 0.3 0.6 0.1
决策树例题经典案例

决策树例题经典案例决策树是一种常见的机器学习算法,它通过对数据集进行划分,构建一颗树形结构来进行决策预测。
在实际应用中,决策树被广泛运用于金融、医疗、营销等领域,帮助人们进行决策分析和预测。
下面我们将通过几个经典案例来深入理解决策树的应用。
案例一,贷款申请。
假设银行需要根据客户的个人信息来决定是否批准其贷款申请。
我们可以利用决策树来构建一个贷款申请的决策模型。
首先,我们需要收集客户的个人信息,比如年龄、收入、信用记录等。
然后,我们将这些信息作为特征,构建决策树模型。
通过对客户信息的分析,决策树可以帮助银行进行贷款申请的预测,提高贷款申请的审批效率。
案例二,疾病诊断。
医疗领域也是决策树的重要应用场景之一。
假设医生需要根据患者的症状来进行疾病诊断,我们可以利用决策树来构建一个疾病诊断的模型。
首先,我们收集患者的症状信息,比如发烧、咳嗽、头痛等。
然后,我们将这些症状作为特征,构建决策树模型。
通过对患者症状的分析,决策树可以帮助医生进行疾病的诊断,提高诊断的准确性。
案例三,产品营销。
在营销领域,决策树也被广泛应用于产品推荐和客户分类。
假设一家电商平台需要根据用户的购物行为来进行产品推荐,我们可以利用决策树来构建一个产品推荐的模型。
首先,我们收集用户的购物记录、浏览记录等信息。
然后,我们将这些信息作为特征,构建决策树模型。
通过对用户行为的分析,决策树可以帮助电商平台进行个性化推荐,提高用户的购物体验。
通过以上经典案例的介绍,我们可以看到决策树在不同领域的应用场景。
无论是贷款申请、疾病诊断还是产品营销,决策树都能够帮助我们进行决策分析和预测,提高工作效率和决策准确性。
因此,掌握决策树算法是非常重要的,它不仅可以帮助我们解决实际问题,还可以提升我们在机器学习领域的竞争力。
希望通过本文的介绍,读者能够对决策树有更深入的理解,为实际问题的解决提供更多的思路和方法。
决策树在生活中的例子
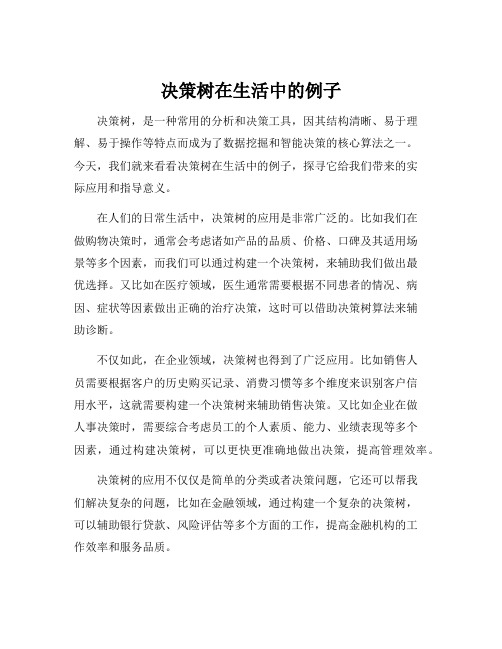
决策树在生活中的例子决策树,是一种常用的分析和决策工具,因其结构清晰、易于理解、易于操作等特点而成为了数据挖掘和智能决策的核心算法之一。
今天,我们就来看看决策树在生活中的例子,探寻它给我们带来的实际应用和指导意义。
在人们的日常生活中,决策树的应用是非常广泛的。
比如我们在做购物决策时,通常会考虑诸如产品的品质、价格、口碑及其适用场景等多个因素,而我们可以通过构建一个决策树,来辅助我们做出最优选择。
又比如在医疗领域,医生通常需要根据不同患者的情况、病因、症状等因素做出正确的治疗决策,这时可以借助决策树算法来辅助诊断。
不仅如此,在企业领域,决策树也得到了广泛应用。
比如销售人员需要根据客户的历史购买记录、消费习惯等多个维度来识别客户信用水平,这就需要构建一个决策树来辅助销售决策。
又比如企业在做人事决策时,需要综合考虑员工的个人素质、能力、业绩表现等多个因素,通过构建决策树,可以更快更准确地做出决策,提高管理效率。
决策树的应用不仅仅是简单的分类或者决策问题,它还可以帮我们解决复杂的问题,比如在金融领域,通过构建一个复杂的决策树,可以辅助银行贷款、风险评估等多个方面的工作,提高金融机构的工作效率和服务品质。
在生活中,我们还可以通过决策树来帮助我们做出更为明智、准确的决策。
例如,我们在选择投资项目时,通过构建决策树,可以考虑各个投资因素的权重,预测投资风险,并选择一个风险最小、收益最大的投资项目。
又比如,在做旅游决策时,我们可以考虑自己的出行时间、预算、兴趣爱好等因素,构建一个旅游决策树,以便在众多选择中,找到一条最为理想的旅游路线。
决策树不仅在实际工作和生活中广泛应用,而且具有可扩展性,易于操作和灵活性等特点,使其成为一种经典的智能决策算法。
决策树的应用范围不断扩大,与此同时,也需要加强对决策树算法的理解和应用,使其在实际场景中发挥更大作用。
相信通过对决策树在生活中的应用的介绍及分析,对于我们应用决策树算法来说会更有指导性和启发性。
决策树算法例题经典

决策树算法例题经典
案例1:购物产品推荐。
假设当前我们需要进行购物产品推荐工作,用户可以选择若干项属性,例如品牌、价格、颜色、是否有折扣等等,在已知一些样本的基础上,构
建一棵决策树,帮助用户快速得到最佳购买推荐。
如果用户选择的品牌为A,则直接推荐产品P3;如果选择品牌为B,
则继续考虑价格,如果价格低于100,则推荐产品P1,否则推荐产品P2。
如果用户选择的品牌为C,则直接推荐产品P4。
当然,这只是一个简单的例子,实际应用场景中可能会有更多的属性
和样本。
因此,在构建决策树时需要考虑选取最优特征,避免过度拟合等
问题。
案例2:疾病预测。
假设有一组医学数据,其中包括患者的年龄、性别、身高、体重、血
压等指标以及是否患有糖尿病的标签信息。
我们希望构建一个决策树来帮
助医生快速判断患者是否可能患有糖尿病。
如果患者年龄大于45岁,则进一步考虑体重,如果体重高于120kg,则判断为高风险群体;否则判断为低风险群体。
如果患者年龄不超过45岁,则直接判断为低风险群体。
当然,这只是一个简单的例子,实际应用场景中可能会有更多的指标
和样本。
因此,在构建决策树时需要考虑选取最优特征,避免过度拟合等
问题。
决策树简单案例
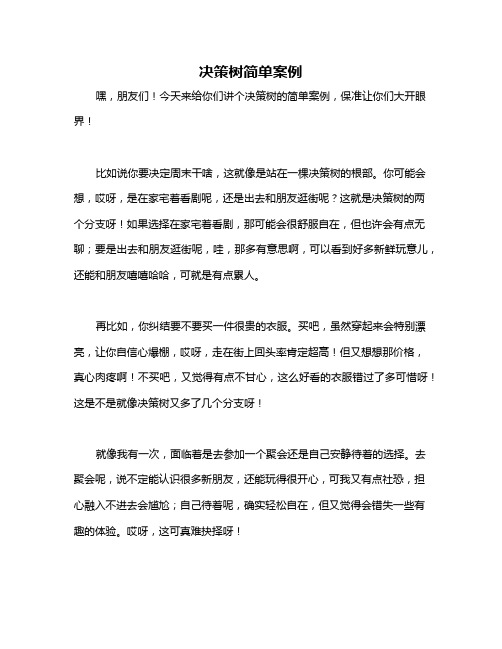
决策树简单案例
嘿,朋友们!今天来给你们讲个决策树的简单案例,保准让你们大开眼界!
比如说你要决定周末干啥,这就像是站在一棵决策树的根部。
你可能会想,哎呀,是在家宅着看剧呢,还是出去和朋友逛街呢?这就是决策树的两个分支呀!如果选择在家宅着看剧,那可能会很舒服自在,但也许会有点无聊;要是出去和朋友逛街呢,哇,那多有意思啊,可以看到好多新鲜玩意儿,还能和朋友嘻嘻哈哈,可就是有点累人。
再比如,你纠结要不要买一件很贵的衣服。
买吧,虽然穿起来会特别漂亮,让你自信心爆棚,哎呀,走在街上回头率肯定超高!但又想想那价格,真心肉疼啊!不买吧,又觉得有点不甘心,这么好看的衣服错过了多可惜呀!这是不是就像决策树又多了几个分支呀!
就像我有一次,面临着是去参加一个聚会还是自己安静待着的选择。
去聚会呢,说不定能认识很多新朋友,还能玩得很开心,可我又有点社恐,担心融入不进去会尴尬;自己待着呢,确实轻松自在,但又觉得会错失一些有趣的体验。
哎呀,这可真难抉择呀!
决策树就是这么神奇,它能让我们把各种可能的情况和后果都清楚地摆在眼前,就像帮我们打开了一个思维的地图。
所以啊,朋友们,决策树真的太有用啦!它能让我们在面对各种选择时更加理智,更清楚地看到每种选择的利弊。
下次当你犹豫不决的时候,不妨也试着画一棵决策树,说不定就能找到最好的答案呢!总之,决策树就是我们做决策的好帮手,能让我们少走弯路,何乐而不为呢!。
管理学决策树例题经典案例

管理学决策树例题经典案例
嘿,朋友们!今天咱来聊聊管理学决策树的例题经典案例。
就说开个餐厅吧,你瞧,这可不是个简单事儿!
比如说,你得考虑选址吧。
是选在繁华的商业街呢,还是在居民区里呢?这就像走在岔路口,得做出选择!要是选了商业街,人流量大,可租金也高得吓人啊,那成本压力不就山大了嘛!但要是在居民区,虽然租金相对低些,可客流量能有那么大吗?哎呀呀,这可真让人头疼呢!
然后呢,菜单怎么设计?是各种菜品都来点,还是主打特色菜呢?这就好比在决策树上又多了几条分支呀。
要是啥都有,备菜成本高,还可能啥都不精;可只搞特色,会不会太局限了呢?
再说说定价,定高了,顾客觉得贵不来了;定低了,自己又觉得亏了。
这不是左右为难嘛!
这不就跟我们人生的选择一样嘛!你看,就像你大学毕业,是去大城市闯荡呢,还是回到家乡安稳过日子呢?每一个选择都可能带来完全不同的结果。
还有招聘员工,找经验丰富的吧,工资要求高;找新手吧,又得花时间精力去培养。
哇,这一个个问题就像决策树上的节点,每个都得慎重考虑呀!
咱回过头看,开餐厅的每一个决定不都影响着餐厅的未来嘛!所以说,管理学决策树真的太重要啦!它能帮我们理清思路,做出更明智的选择。
就好像有了一张地图,告诉我们该往哪走,怎么才能走到成功的彼岸!我觉得,不管是干啥,都得学会用这决策树的思维,毕竟,选择不对,努力白费呀,大家说是不是呢!。
人工智能决策树例题经典案例
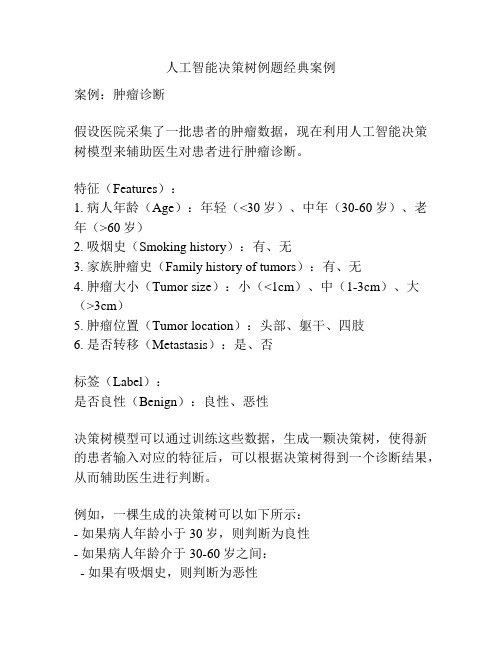
人工智能决策树例题经典案例案例:肿瘤诊断假设医院采集了一批患者的肿瘤数据,现在利用人工智能决策树模型来辅助医生对患者进行肿瘤诊断。
特征(Features):1. 病人年龄(Age):年轻(<30岁)、中年(30-60岁)、老年(>60岁)2. 吸烟史(Smoking history):有、无3. 家族肿瘤史(Family history of tumors):有、无4. 肿瘤大小(Tumor size):小(<1cm)、中(1-3cm)、大(>3cm)5. 肿瘤位置(Tumor location):头部、躯干、四肢6. 是否转移(Metastasis):是、否标签(Label):是否良性(Benign):良性、恶性决策树模型可以通过训练这些数据,生成一颗决策树,使得新的患者输入对应的特征后,可以根据决策树得到一个诊断结果,从而辅助医生进行判断。
例如,一棵生成的决策树可以如下所示:- 如果病人年龄小于30岁,则判断为良性- 如果病人年龄介于30-60岁之间:- 如果有吸烟史,则判断为恶性- 如果没有吸烟史:- 如果有家族肿瘤史,则判断为恶性- 如果没有家族肿瘤史:- 如果肿瘤大小为中或大,则判断为恶性- 如果肿瘤大小为小,且肿瘤位置为头部,则判断为恶性- 如果肿瘤大小为小,且肿瘤位置不在头部,则判断为良性- 如果病人年龄大于60岁,则判断为恶性利用这个决策树模型,当新的患者输入其特征时,比如一个40岁的病人,没有吸烟史,没有家族肿瘤史,肿瘤大小为小,位置在头部,没有转移,根据决策树的判断,该病人的肿瘤很可能是良性的。
通过人工智能决策树模型,可以减轻医生的负担,提供更加准确和快速的诊断结果,帮助医生做出适当的治疗决策。
决策树过拟合例子
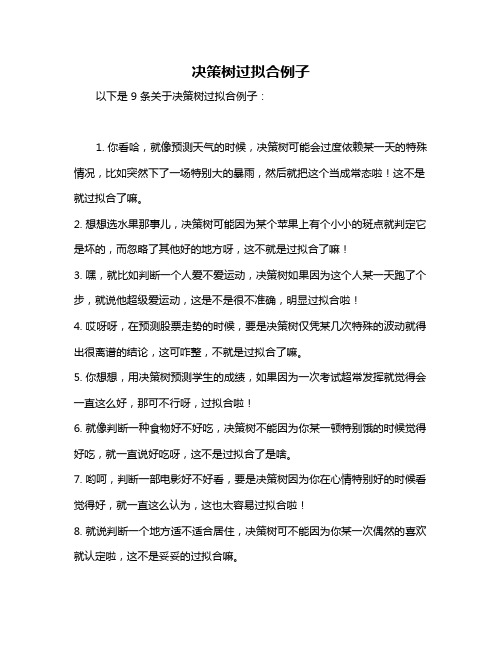
决策树过拟合例子
以下是 9 条关于决策树过拟合例子:
1. 你看哈,就像预测天气的时候,决策树可能会过度依赖某一天的特殊情况,比如突然下了一场特别大的暴雨,然后就把这个当成常态啦!这不是就过拟合了嘛。
2. 想想选水果那事儿,决策树可能因为某个苹果上有个小小的斑点就判定它是坏的,而忽略了其他好的地方呀,这不就是过拟合了嘛!
3. 嘿,就比如判断一个人爱不爱运动,决策树如果因为这个人某一天跑了个步,就说他超级爱运动,这是不是很不准确,明显过拟合啦!
4. 哎呀呀,在预测股票走势的时候,要是决策树仅凭某几次特殊的波动就得出很离谱的结论,这可咋整,不就是过拟合了嘛。
5. 你想想,用决策树预测学生的成绩,如果因为一次考试超常发挥就觉得会一直这么好,那可不行呀,过拟合啦!
6. 就像判断一种食物好不好吃,决策树不能因为你某一顿特别饿的时候觉得好吃,就一直说好吃呀,这不是过拟合了是啥。
7. 哟呵,判断一部电影好不好看,要是决策树因为你在心情特别好的时候看觉得好,就一直这么认为,这也太容易过拟合啦!
8. 就说判断一个地方适不适合居住,决策树可不能因为你某一次偶然的喜欢就认定啦,这不是妥妥的过拟合嘛。
9. 最后呀,我觉得决策树过拟合真的是要很小心呢,不然得出的结果可就太不靠谱啦!。
决策树的例子
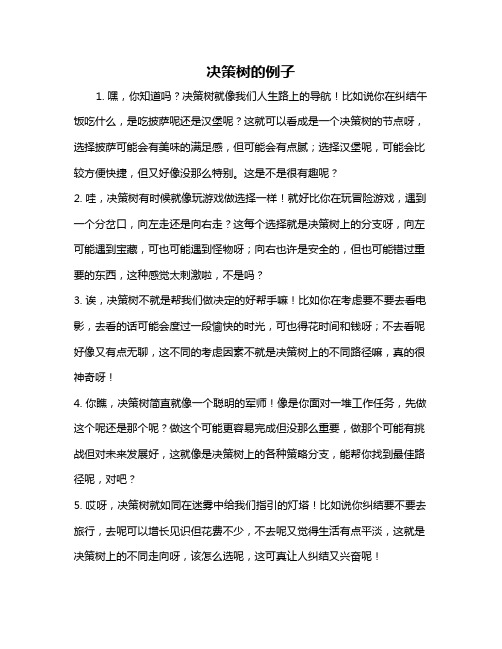
决策树的例子1. 嘿,你知道吗?决策树就像我们人生路上的导航!比如说你在纠结午饭吃什么,是吃披萨呢还是汉堡呢?这就可以看成是一个决策树的节点呀,选择披萨可能会有美味的满足感,但可能会有点腻;选择汉堡呢,可能会比较方便快捷,但又好像没那么特别。
这是不是很有趣呢?2. 哇,决策树有时候就像玩游戏做选择一样!就好比你在玩冒险游戏,遇到一个分岔口,向左走还是向右走?这每个选择就是决策树上的分支呀,向左可能遇到宝藏,可也可能遇到怪物呀;向右也许是安全的,但也可能错过重要的东西,这种感觉太刺激啦,不是吗?3. 诶,决策树不就是帮我们做决定的好帮手嘛!比如你在考虑要不要去看电影,去看的话可能会度过一段愉快的时光,可也得花时间和钱呀;不去看呢好像又有点无聊,这不同的考虑因素不就是决策树上的不同路径嘛,真的很神奇呀!4. 你瞧,决策树简直就像一个聪明的军师!像是你面对一堆工作任务,先做这个呢还是那个呢?做这个可能更容易完成但没那么重要,做那个可能有挑战但对未来发展好,这就像是决策树上的各种策略分支,能帮你找到最佳路径呢,对吧?5. 哎呀,决策树就如同在迷雾中给我们指引的灯塔!比如说你纠结要不要去旅行,去呢可以增长见识但花费不少,不去呢又觉得生活有点平淡,这就是决策树上的不同走向呀,该怎么选呢,这可真让人纠结又兴奋呢!6. 嘿呀,决策树就像一个神秘的魔法图!像是在选择职业的时候,这个职业工资高但压力大,那个职业轻松但发展有限,这不就是决策树上的不同节点嘛,我们得好好思考该怎么走呢,是不是很有意思呀!7. 哇塞,决策树不就像走迷宫一样嘛!比如你在考虑要不要换个发型,换个新的可能很时尚但也可能不适合自己,不换呢又觉得没变化,这每一步的考虑都是决策树上的一个过程呀,让人又期待又紧张呢!8. 诶哟,决策树就像帮我们解决难题的好朋友!像在决定要不要投资的时候,投这个可能收益高但风险大,投那个可能稳妥但回报少,这就是决策树上的各种可能性呀,真的得好好斟酌呀!我觉得决策树真的太有用啦,能让我们更清晰地看到不同选择的后果,从而做出更好的决定!。
决策树例题经典案例python
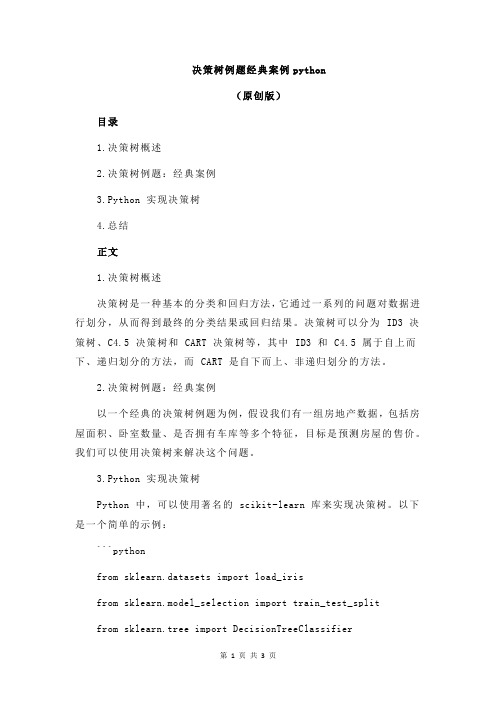
决策树例题经典案例python(原创版)目录1.决策树概述2.决策树例题:经典案例3.Python 实现决策树4.总结正文1.决策树概述决策树是一种基本的分类和回归方法,它通过一系列的问题对数据进行划分,从而得到最终的分类结果或回归结果。
决策树可以分为 ID3 决策树、C4.5 决策树和 CART 决策树等,其中 ID3 和 C4.5 属于自上而下、递归划分的方法,而 CART 是自下而上、非递归划分的方法。
2.决策树例题:经典案例以一个经典的决策树例题为例,假设我们有一组房地产数据,包括房屋面积、卧室数量、是否拥有车库等多个特征,目标是预测房屋的售价。
我们可以使用决策树来解决这个问题。
3.Python 实现决策树Python 中,可以使用著名的 scikit-learn 库来实现决策树。
以下是一个简单的示例:```pythonfrom sklearn.datasets import load_irisfrom sklearn.model_selection import train_test_splitfrom sklearn.tree import DecisionTreeClassifierfrom sklearn.metrics import accuracy_score# 加载数据集iris = load_iris()X = iris.datay = iris.target# 划分训练集和测试集X_train, X_test, y_train, y_test = train_test_split(X, y, test_size=0.3, random_state=42)# 创建决策树模型clf = DecisionTreeClassifier()# 训练模型clf.fit(X_train, y_train)# 预测y_pred = clf.predict(X_test)# 评估模型print("Accuracy:", accuracy_score(y_test, y_pred))```在这个例子中,我们使用了 scikit-learn 内置的 Iris 数据集,并将数据集划分为训练集和测试集。
决策树例题
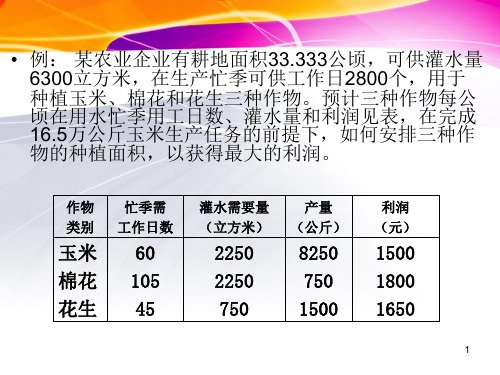
• 建设小工厂投资280万元,如销路好,3年后扩建,扩 建需要投资400万元,可使用7年,每年赢利190万元。 不扩建则每年赢利80万元。如销路不好则每年赢利60 万元。
• 试用决策树法选出合理的决策方案。 经过市场调查, 市场销路好的概率为0.7,销路不好的概率为0.3。
25
益损值 方案
甲 乙 丙
状态 需求量较 需求量一 高般
600 400 800 350 350 220
需求量 较低
-150 -350 50
需求量很低
-350 -700 -100
丁
400 250
90
-50
益损值 方案
状态 需求 量较 高
需求量 一般
需求量 需求量 最大后 较低 很低 悔值
甲
200 0
13
➢最后比较决策点1的情况: • 由于点③(719万元)与点②(680万元)
相比,点③的期望利润值较大,因此取 点③而舍点②。这样,相比之下,建设 大工厂的方案不是最优方案,合理的策 略应采用前3年建小工厂,如销路好,后 7年进行扩建的方案。
14
决策树法的一般程序是: (1)画出决策树图形 决策树指的是某个决策问题未来发展情 况的可能性和可能结果所做的估计,在图纸上的描绘决策树 (2)计算效益期望值 两个行动方案的效益期望值计算过程: 行动方案A1(建大厂)的效益期望值: 13.5×0.8×10+25.5×0.2×10-25=134万元 行动方案A2(建小厂)的效益期望值: 15×0.8×10+15×0.2×10-10=140万元 (3)将效益期望值填入决策树图 首先在每个结果点后面填上 相应的效益期望值;其次在每个方案节点上填上相应的期望值, 最后将期望值的角色分支删减掉。只留下期望值最大的决策分 支,并将此数值填入决策点上面,至此决策方案也就相应选出
- 1、下载文档前请自行甄别文档内容的完整性,平台不提供额外的编辑、内容补充、找答案等附加服务。
- 2、"仅部分预览"的文档,不可在线预览部分如存在完整性等问题,可反馈申请退款(可完整预览的文档不适用该条件!)。
- 3、如文档侵犯您的权益,请联系客服反馈,我们会尽快为您处理(人工客服工作时间:9:00-18:30)。
决策树示例%**************************************************************%* mex interface to Andy Liaw et al.'s C code (used in R package randomForest)%* Added by Abhishek Jaiantilal ( abhishek.jaiantilal@ )%* License: GPLv2%* Version: 0.02%% Calls Regression Random Forest% A wrapper matlab file that calls the mex file% This does training given the data and labels% Documentation copied from R-packages pdf% /web/packages/randomForest/randomForest.pdf% Tutorial on getting this working in tutorial_ClassRF.m%%**************************************************************% function model = classRF_train(X,Y,ntree,mtry, extra_options)%%___Options% requires 2 arguments and the rest 3 are optional% X: data matrix% Y: target values% ntree (optional): number of trees (default is 500). also if set to 0% will default to 500% mtry (default is floor(sqrt(size(X,2))) D=number of features in X). also if set to 0% will default to 500%%% Note: TRUE = 1 and FALSE = 0 below% extra_options represent a structure containing various misc. options to% control the RF% extra_options.replace = 0 or 1 (default is 1) sampling with or without% replacement% extra_options.strata = (not Implemented)% extra_options.sampsize = Size(s) of sample to draw. For classification,% if sampsize is a vector of the length the number of strata, then sampling is stratified by strata,% and the elements of sampsize indicate the numbers to be drawn from the strata. I don't yet know how this works.% extra_options.nodesize = Minimum size of terminal nodes. Setting this number larger causessmaller trees% to be grown (and thus take less time). Note that the default values are different% for classification (1) and regression (5).% extra_options.importance = Should importance of predictors be assessed?% extra_options.localImp = Should casewise importance measure be computed? (Setting this to TRUE will% override importance.)% extra_options.proximity = Should proximity measure among the rows be calculated?% extra_options.oob_prox = Should proximity be calculated only on 'out-of-bag' data?% extra_options.do_trace = If set to TRUE, give a more verbose output as randomForest is run. If set to% some integer, then running output is printed for every% do_trace trees.% extra_options.keep_inbag = Should an n by ntree matrix be returned that keeps track of which samples are% 'in-bag' in which trees (but not how many times, if sampling with replacement)% extra_options.corr_bias = which happens only for regression. perform bias correction for regression? Note: Experimental. Use at your own% risk.% extra_options.nPerm = Number of times the OOB data are permuted per tree for assessing variable% importance. Number larger than 1 gives slightly more stable estimate, but not% very effective. Currently only implemented for regression.%%%___Returns model which has% importance = a matrix with nclass + 2 (for classification) or two (for regression) columns.% For classification, the first nclass columns are the class-specific measures% computed as mean decrease in accuracy. The nclass + 1st column is the% mean decrease in accuracy over all classes. The last column is the mean decrease% in Gini index. For Regression, the first column is the mean decrease in% accuracy and the second the mean decrease in MSE. If importance=FALSE,% the last measure is still returned as a vector.% importanceSD = The ?standard errors? of the permutation-based importance measure. For classification,% a p by nclass + 1 matrix corresponding to the first nclass + 1% columns of the importance matrix. For regression, a length p vector.% localImp = a p by n matrix containing the casewise importance measures, the [i,j] element% of which is the importance of i-th variable on the j-th case. NULL if% localImp=FALSE.% ntree = number of trees grown.% mtry = number of predictors sampled for spliting at each node.% votes (classification only) a matrix with one row for each input data point and one% column for each class, giving the fraction or number of ?votes? from the random% forest.% oob_times number of times cases are 'out-of-bag' (and thus used in computing OOB error% estimate)% proximity if proximity=TRUE when randomForest is called, a matrix of proximity% measures among the input (based on the frequency that pairs of data points are% in the same terminal nodes).% errtr = first column is OOB Err rate, second is for class 1 and so on% mse =(regression only) vector of mean square errors: sum of squared residuals divided% by n.% rsq (regression only) 'pseudo R-squared': 1 - mse / Var(y).function model=regRF_train(X,Y,ntree,mtry, extra_options)%function model = regRF_predict(X,Y,ntree,mtry)%requires 2 arguments and the rest 2 are optional%X: data matrix%Y: target values%ntree (optional): number of trees (default is 500)%mtry (default is max(floor(D/3),1) D=number of features in X)DEBUG_ON=0;DEFAULTS_ON=0;TRUE=1;FALSE=0;if exist('extra_options','var')if isfield(extra_options,'DEBUG_ON'); DEBUG_ON = extra_options.DEBUG_ON; endif isfield(extra_options,'replace'); replace = extra_options.replace; endif isfield(extra_options,'classwt'); classwt = extra_options.classwt; endif isfield(extra_options,'cutoff'); cutoff = extra_options.cutoff; endif isfield(extra_options,'strata'); strata = extra_options.strata; endif isfield(extra_options,'sampsize'); sampsize = extra_options.sampsize; endif isfield(extra_options,'nodesize'); nodesize = extra_options.nodesize; endif isfield(extra_options,'importance'); importance = extra_options.importance; endif isfield(extra_options,'localImp'); localImp = extra_options.localImp; endif isfield(extra_options,'nPerm'); nPerm = extra_options.nPerm; endif isfield(extra_options,'proximity'); proximity = extra_options.proximity; endif isfield(extra_options,'oob_prox'); oob_prox = extra_options.oob_prox; end%if isfield(extra_options,'norm_votes'); norm_votes = extra_options.norm_votes; endif isfield(extra_options,'do_trace'); do_trace = extra_options.do_trace; endif isfield(extra_options,'corr_bias'); corr_bias = extra_options.corr_bias; endif isfield(extra_options,'keep_inbag'); keep_inbag = extra_options.keep_inbag; endend%set defaults if not already setif ~exist('DEBUG_ON','var') DEBUG_ON=FALSE; endif ~exist('replace','var'); replace = TRUE; end%if ~exist('classwt','var'); classwt = []; end %will handle these three later%if ~exist('cutoff','var'); cutoff = 1; end%if ~exist('strata','var'); strata = 1; endif ~exist('sampsize','var');if (replace)sampsize = size(X,1);elsesampsize = ceil(0.632*size(X,1));end;endif ~exist('nodesize','var'); nodesize = 5; end %classification=1, regression=5if ~exist('importance','var'); importance = FALSE; endif ~exist('localImp','var'); localImp = FALSE; endif ~exist('nPerm','var'); nPerm = 1; end%if ~exist('proximity','var'); proximity = 1; end %will handle these two later%if ~exist('oob_prox','var'); oob_prox = 1; end%if ~exist('norm_votes','var'); norm_votes = TRUE; endif ~exist('do_trace','var'); do_trace = FALSE; endif ~exist('corr_bias','var'); corr_bias = FALSE; endif ~exist('keep_inbag','var'); keep_inbag = FALSE; endif ~exist('ntree','var') | ntree<=0ntree=500;DEFAULTS_ON=1;endif ~exist('mtry','var') | mtry<0 | mtry> size(X,2)mtry = max(floor(size(X,2)/3),1);DEFAULTS_ON=1;endaddclass=0;[N D] = size(X);if length(unique(Y))<=5, warning('Do you want regression? there are just 5 or less unique values'); endif N==0, error('Data (X) has 0 rows'); endif mtry<1 || mtry>D , warning('Invalid mtry. reset to within valid range'); DEFAULTS_ON=1; endmtry = max(1, min(D,round(mtry)));if DEFAULTS_ONfprintf('\tSetting to defaults %d trees and mtry=%d\n',ntree,mtry);endif length(Y)~=N || length(Y)==0error('length of Y not the same as X or Y is null');endif ~isempty(find(isnan(X))); error('NaNs in X'); endif ~isempty(find(isnan(Y))); error('NaNs in Y'); end%now handle categories. Problem is that categories in R are more%enhanced. In this i ask the user to specify the column/features to%consider as categories, 1 if all the values are real values else%specify the number of categories hereif exist ('extra_options','var') && isfield(extra_options,'categories')ncat = extra_options.categories;elsencat = ones(1,D);endmaxcat = max(ncat);if maxcat>32error('Can not handle categorical predictors with more than 32 categories');end%classRF - line 88 in randomForest.default.Rnclass = length(unique(Y));addclass = FALSE;if ~exist('proximity','var')proximity = addclass;oob_prox = proximity;endif ~exist('oob_prox','var')oob_prox = proximity;end%i handle the below in the mex file% if proximity% prox = zeros(N,N);% proxts = 1;% else% prox = 1;% proxts = 1;% end%i handle the below in the mex fileif localImpimportance = TRUE;% impmat = zeors(D,N);else% impmat = 1;endif importanceif (nPerm<1)nPerm = int32(1);elsenPerm = int32(nPerm);end%regRF% impout = zeros(D,2);% impSD = zeros(D,1);else% impout = zeros(D,1);% impSD = 1;end%i handle the below in the mex file%somewhere near line 157 in randomForest.default.R if addclass% nsample = 2*n;else% nsample = n;endStratify = (length(sampsize)>1);if (~Stratify && sampsize>N)error('Sampsize too large')endif Stratifyerror('Sampsize should be of length one') end%i handle the below in the mex file% nrnodes = 2*floor(sampsize/max(1,nodesize-4))+1;% xtest = 1;% ytest = 1;% ntest = 1;% labelts = FALSE;% nt = ntree;Options = int32([importance,localImp,nPerm]);if DEBUG_ON%print the parameters that i am sending infprintf('size(x) %d\n',size(X));fprintf('size(y) %d\n',size(Y));fprintf('nclass %d\n',nclass);fprintf('size(ncat) %d\n',size(ncat));fprintf('maxcat %d\n',maxcat);fprintf('size(sampsize) %d\n',size(sampsize));fprintf('sampsize[0] %d\n',sampsize(1));fprintf('Stratify %d\n',Stratify);fprintf('Proximity %d\n',proximity);fprintf('oob_prox %d\n',oob_prox);fprintf('ntree %d\n',ntree);fprintf('mtry %d\n',mtry);fprintf('nodesize %f\n',nodesize);fprintf('replace %f\n',replace);end[ldau,rdau,nodestatus,nrnodes,upper,avnode,...mbest,ndtree,ypred,mse,impout,impmat,...impSD,prox,coef,oob_times,inbag]...= mexRF_train (X',Y,ntree,mtry,sampsize,nodesize,...int32(Options),int32(ncat),int32(maxcat),int32(do_trace), int32(proximity), int32(oob_prox), ...int32(corr_bias), keep_inbag, replace );%done in R file so doing it too.ypred(oob_times==0)=NaN;model.lDau=ldau;model.rDau=rdau;model.nodestatus=nodestatus;model.nrnodes=nrnodes;model.upper=upper;model.avnode=avnode;model.mbest=mbest;model.ndtree=ndtree;model.ntree = ntree;model.Y_hat = ypred;model.mse = mse;model.importance = impout;model.importanceSD = impSD;model.localImp = impmat;model.proximity = prox;model.coef = coef;model.oob_times = oob_times;model.inbag = inbag;model.nPerm = nPerm;model.biasCorr = corr_bias;model.rsq = 1 - mse / (var(Y) * (N-1) / N);clear mexRF_train。