Data Mining Techniques for Microarray Datasets
Affymetrix生物芯片简介
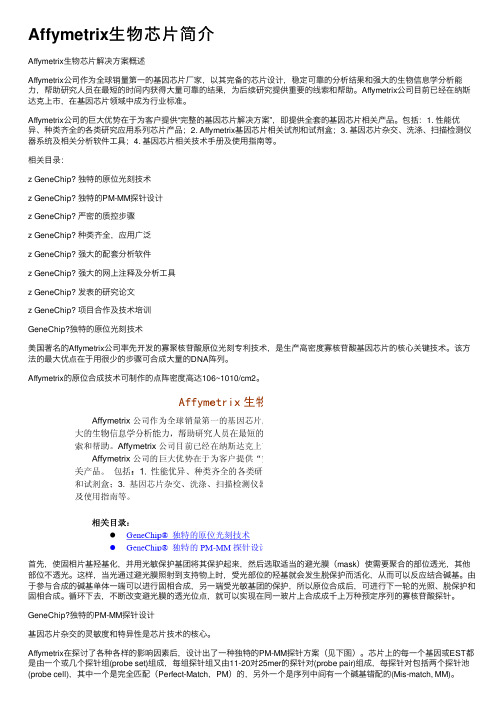
Affymetrix⽣物芯⽚简介Affymetrix⽣物芯⽚解决⽅案概述Affymetrix公司作为全球销量第⼀的基因芯⽚⼚家,以其完备的芯⽚设计,稳定可靠的分析结果和强⼤的⽣物信息学分析能⼒,帮助研究⼈员在最短的时间内获得⼤量可靠的结果,为后续研究提供重要的线索和帮助。
Affymetrix公司⽬前已经在纳斯达克上市,在基因芯⽚领域中成为⾏业标准。
Affymetrix公司的巨⼤优势在于为客户提供“完整的基因芯⽚解决⽅案”,即提供全套的基因芯⽚相关产品。
包括:1. 性能优异、种类齐全的各类研究应⽤系列芯⽚产品;2. Affymetrix基因芯⽚相关试剂和试剂盒;3. 基因芯⽚杂交、洗涤、扫描检测仪器系统及相关分析软件⼯具;4. 基因芯⽚相关技术⼿册及使⽤指南等。
相关⽬录:z GeneChip? 独特的原位光刻技术z GeneChip? 独特的PM-MM探针设计z GeneChip? 严密的质控步骤z GeneChip? 种类齐全,应⽤⼴泛z GeneChip? 强⼤的配套分析软件z GeneChip? 强⼤的⽹上注释及分析⼯具z GeneChip? 发表的研究论⽂z GeneChip? 项⽬合作及技术培训GeneChip?独特的原位光刻技术美国著名的Affymetrix公司率先开发的寡聚核苷酸原位光刻专利技术,是⽣产⾼密度寡核苷酸基因芯⽚的核⼼关键技术。
该⽅法的最⼤优点在于⽤很少的步骤可合成⼤量的DNA阵列。
Affymetrix的原位合成技术可制作的点阵密度⾼达106~1010/cm2。
⾸先,使固相⽚基羟基化,并⽤光敏保护基团将其保护起来,然后选取适当的避光膜(mask)使需要聚合的部位透光,其他部位不透光。
这样,当光通过避光膜照射到⽀持物上时,受光部位的羟基就会发⽣脱保护⽽活化,从⽽可以反应结合碱基。
由于参与合成的碱基单体⼀端可以进⾏固相合成,另⼀端受光敏基团的保护,所以原位合成后,可进⾏下⼀轮的光照、脱保护和固相合成。
非小细胞肺癌微转移的研究进展
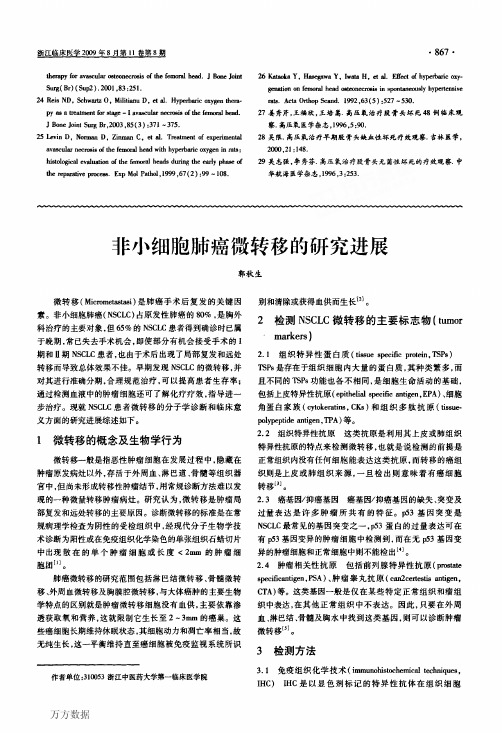
therapyforavascularosteonecrosisofthefemoralhead.JBoneJointSurg(Br)(Sup2).2001。
83:251.24ReisND。
SchwartzO,MilitianuD,eta1.Hyperbaricoxygenthem-PY鹅atreatmentforstage—Iavascularnocrosi¥ofthefemoralhead.JBoneJointSurgBr,2003,85(3):371—375.25LevinD,NormanD。
ZinmanC,eta1.Treatmentofexperimentalavaseularnecrosisofthefemoralheadwithhyperbaricoxygeninrats:histologicalevaluationofthefemoralheadsduringtheearlyphaseofthereparativeprocess.ExpMolPathol,1999,67(2):99—108.・867・26gataokaY,m删aY,1wataH,eta1.Effectofhyperbaricoxy-genationonfemoralheadoatecnecresisinspontaneouslyhypertensivem协.ActaOrthopScand.1992,63(5):527—530.27姜秀芹,王福欣,王培嵩.高压氧治疗股骨头坏死48倒临床现察.高压氧医学杂志,1996,5:90.28吴限.高压氧治疗早期股骨头缺血性坏死疗效观察.吉林医学,2000.21:148.29吴志强,李秀芬.高压氧治疗股骨头无菌性坏死的疗效观察.中华航海医学杂志,1996,3:253.非小细胞肺癌微转移的研究进展郭秋生微转移(Micrometastasi)是肺癌手术后复发的关键因素。
非小细胞肺癌(NSCLC)占原发性肺癌的80%,是胸外科治疗的主要对象,但65%的NSCLC患者得到确诊时已属于晚期,常已失去手术机会,即使部分有机会接受手术的I期和Ⅱ期NSCLC患者,也由于术后出现了局部复发和远处转移而导致总体效果不佳。
Data Mining是什么意思
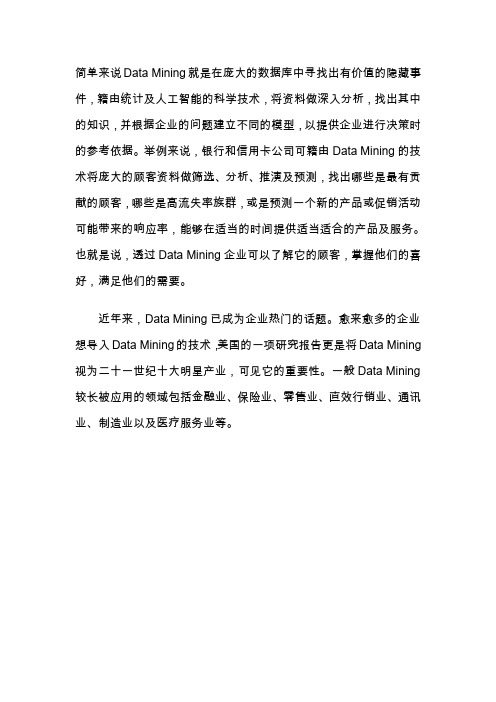
简单来说Data Mining就是在庞大的数据库中寻找出有价值的隐藏事件,籍由统计及人工智能的科学技术,将资料做深入分析,找出其中的知识,并根据企业的问题建立不同的模型,以提供企业进行决策时的参考依据。
举例来说,银行和信用卡公司可籍由Data Mining的技术将庞大的顾客资料做筛选、分析、推演及预测,找出哪些是最有贡献的顾客,哪些是高流失率族群,或是预测一个新的产品或促销活动可能带来的响应率,能够在适当的时间提供适当适合的产品及服务。
也就是说,透过Data Mining企业可以了解它的顾客,掌握他们的喜好,满足他们的需要。
近年来,Data Mining已成为企业热门的话题。
愈来愈多的企业想导入Data Mining的技术,美国的一项研究报告更是将Data Mining 视为二十一世纪十大明星产业,可见它的重要性。
一般Data Mining 较长被应用的领域包括金融业、保险业、零售业、直效行销业、通讯业、制造业以及医疗服务业等。
Xplore Integrated 图像和数据管理产品说明书
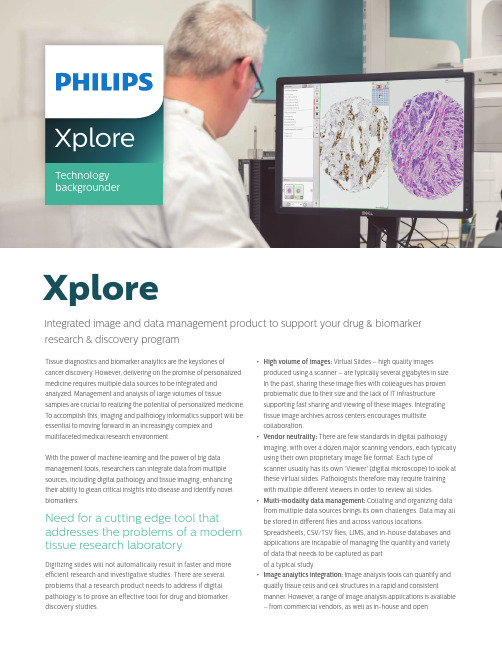
XploreIntegrated image and data management product to support your drug & biomarker r esearch & discovery programTissue diagnostics and biomarker analytics are the keystones of cancer discovery. However, delivering on the promise of personalized medicine requires multiple data sources to be integrated and analyzed. Management and analysis of large volumes of tissue samples are crucial to realizing the potential of personalized medicine. To accomplish this, imaging and pathology informatics support will be essential to moving forward in an increasingly complex and multifaceted medical research environment.With the power of machine learning and the power of big data management tools, researchers can integrate data from multiple sources, including digital pathology and tissue imaging, enhancing their ability to glean critical insights into disease and identify novel biomarkers.Need for a cutting edge tool that addresses the problems of a modern tissue research laboratoryDigitizing slides will not automatically result in faster and more efficient research and investigative studies. There are several problems that a research product needs to address if digital pathology is to prove an effective tool for drug and biomarker discovery studies.• High volume of images: Virtual Slides – high quality images produced using a scanner – are typically several gigabytes in size. In the past, sharing these image files with colleagues has proven problematic due to their size and the lack of IT infrastructure supporting fast sharing and viewing of these images. Integrating tissue image archives across centers encourages multisite collaboration.• Vendor neutrality: There are few standards in digital pathology imaging, with over a dozen major scanning vendors, each typically using their own proprietary image file format. Each type of scanner usually has its own ‘Viewer’ (digital microscope) to look at these virtual slides. Pathologists therefore may require training with multiple different viewers in order to review all slides.• Multi-modality data management: Collating and organizing data from multiple data sources brings its own challenges. Data may all be stored in different files and across various locations.Spreadsheets, CSV/TSV files, LIMS, and in-house databases and applications are incapable of managing the quantity and variety of data that needs to be captured as part of a typical study.• Image analytics integration: Image analysis tools can quantify and qualify tissue cells and cell structures in a rapid and consistent manner. However, a range of image analysis applications is available– from commercial vendors, as well as in-house and openXploreTechnology backgroundersolutions. Managing slides that are used in multiple applications and the data produced by their algorithms can be difficult, due to limited interoperability.• TMA management: Tissue microarrays (TMAs) provide the means for high-throughput analysis of multiple tissues and cells. The issues involved in collating, organizing and associating data with whole sections of tissue is multiplied with a TMA, as a single TMA slide may contain 200 cores, each potentially representing a different patient. A TMA study of a single 20x10 block, with five stains, three scoring criteria per stain and two reviewing pathologists will result in six thousand ‘scores’. Mapping these scores to different patients, comparing scores and results, and identifying trends across different cohorts will be time-consuming and prone to human error. Data mining tools, detailed in the next section, are required to solve the challenges of conducting large biomarker investigative studies.• Cross study data management: Given the range and volume of datamentioned in the points above, and the high quantity of slides thatmay be part of a typical study, the organizing slides in different ways,and maintaining a link with the aforementioned data, will provechallenging. Slides may need to be included as part of severalstudies. Studies may also be organized in many different ways.• Lab ecosystem: There are many different platforms and applicationsin a lab today and there is a need to ensure flexibility andinteroperability through and image management system that canprovide some context. Labs also have differing views on deploymentmethods for applications.Key design principles that allow a solution to these problemsTo address the problems listed above, Xplore was designed in conjunction with key opinion leaders from across the pathologyspectrum, to impact and accelerate tissue-centric discovery in drug and biomarker research. There are four key design principals that the solution adheres to.An Open solution, allowing institutions to use slides from multiple scanner vendors in a single Viewer, but also launch multiple image analysis vendors.A Flexible solution, providing institutions with tools to manage research the way you want, allowing you to design, organize, manage, search, and interrogate studies in a variety of ways.An Integrated solution, allowing institutions to store image, analytic data with slides both within and across studies, with search and datamining tools to help retrieve important data quickly.A Connected solution, bringing together researchers across an organization, across multiple sites and across geographies, amplifying the expertise of all those involved anywhere.Let us explore in detail how some of the issues highlighted earlier in the biomarker research process can be addressed through an effective tissue research product.View virtual slides in a single digital viewerOur Solution – the Xplore ViewerXplore supports all major scanning vendors in a single web-based viewer, thereby reducing training needs across multiple platforms. Both bright field and fluorescent slides are supported, in addition to z-stacking and multi-regions.Ve n dor Exte n sion Philips .isyntax / .tiffHamamatsu .ndpi / .ndpisLeica / Ariol .scnPerkin Elmer .qptiffAperio .svsVentana .bif / .tif / .svsZeiss .cziOlympus .vsiOmnyx .rtsSakura .svs3DHistec h .mrsxHuron .tif Mikroscan.svsTrestle .tifVendor Neutrality - Support for all major scanning vendorsXplore offers a range of tools that you would expect in a modern digital pathology viewer, including the following features,extremely important in a research setting, Annotation & Measuring tools, Split screen (Sync up to four whole slide images or TMA Cores), Fluorescent multi-channel image adjustments, Counting tools to assist validating image analysis algorithms, Screenshots and Z-Stack (switch between different planes).Manage and organize images and data in ascalable user centric mannerOur Solution – Flexible Folder, Study & Slide Management The Philips Xplore product can ingest thousands of images in seconds, provides a database for easily organizing Whole Slide Images (WSI’s), documents, image analysis and slide associated metadata within aflexible hierarchical structure. Users are assigned as owners of a particular study with permissions to share slides and data within and across teams of researchers.Xplore’s configurable study-based folder hierarchy makes a very flexible product for research. Xplore has no fixed study hierarchy,Xplore - Key design principlesallowing multi-disciplinary research.Slides can be included as part of multiple studies, without duplicating/multiplying the large file sizes on disk. Slides can also be added to a hierarchy of the researcher’s choosing, for example stain, body site, sample ID or case ID or principal investigator. Slides can therefore be better organized to meet the context/purpose of the study.Maintain metadata and third party dataOur Solution – Datasets, import, barcodes and documentsDatasets can be created with custom fields and metadata. Image analysis data can be uploaded into Xplore and easily searched or interrogated for biomarker evaluation, case selection and correlations.1D, 2D, Datamatrix support, allows metadata on the slide to be automatically added to the Xplore database on slide acquisition. Xplore also has an import facility, to associate additional information not included in the barcode with slides and studies using CSV or TSV files. Data can be uploaded against the slide name or by TMA core position/ID, or by information collected on the barcode, for example Sample ID.The document management system allows supporting research material, journals, publications and presentations to be stored alongside studies.Manage high volumes of slides, identify trends and outliers and create cohortsOur Solution – Precision SearchXplore’s Search engine allows user to identify cohorts, based on searching a range of data across folders and slides, or studies, slides, cores and scoring data in the case of TMA’s.Search across system generated fields, barcode metadata, clinical/image analysis data uploaded via CSV, and manual scoring data, to perform a deep and detailed analysis of any study managed in Xplore, and get a better understanding of how biomarkers are expressing themselves on different TMA cores, whole slides and therefore patients.Use the results of Search to create additional research studies; create charts from Search results to more easily spot trends and outliers; save for future use; or export data for use in other 3rd partyapplications.Conduct large biomarker investigative and evaluation studiesOur Solution – Comprehensive TMA moduleXplore’s TMA (Tissue Microarray) module is designed to speed up research studies that require manual scores of TMA cores, thereby helping evaluate new tissue biomarkers in TMAs quickly and ing scoring templates, map templates, and an automated TMA de-arrayer, TMA cores can be segmented, identified, and sent out for scoring. Patient information (managed through datasets), scoring criteria and the TMA Core tissue are available through a single interface. The TMA Core tissue is locked to the question(s) being asked upon it, so the user is unable to answer scoring questions on a TMA Core that is not visible on screen.Virtual TMAThe Virtual TMA module in Xplore allows selected cores from multiple recipient blocks to be combined in a single, score-able Virtual TMA Study.Given there are discrepancies or anomalies in the data that has been captured through image analysis or manual scoring,individual TMA Cores from one or more TMA Slides or TMA StudiesCross study data management - Example Folder & Study structures in XploreThe Xplore Search interface can build complex queries across multi-modality dataTMA Management - TMA Scoring interface in Xplorecan be segmented into a new, composite, ‘Virtual TMA’ study. This study can then be sent out again for scoring, but only providing the researcher with the TMA Cores that need to be scored, rather than the several hundred that may be on a single slide. This greatly increases the efficiency of getting selected TMA Cores re-scored.Easily identify trends and spot outliersOur solution – ChartsThe Charts tools in Xplore will help researchers and pathologists more easily quantify data, and help spot trends and outliers in Xplore. The results of a study or advanced search can be opened in a variety of charts. Tools for the researcher allow him or her to directly open the relevant data point to obtain a full breakdown of the data that has been captured against a whole slide or TMA core.By providing a charts and graphing facility within Xplore, the link with the virtual slide and therefore tissue is maintained. The process of matching a data point in a spreadsheet or other application with the original tissue can be extremely difficult, but Xplore allows the researcher to click a data point and open the tissue in the viewer within seconds.Integrate with multiple imageanalysis vendorsOur solution – Image Analysis agnosticXplore’s open product structure offers interoperability with offerings from third party image analysis vendors, such as Visiopharm OncoTopix. Xplore’s advanced search engine provides tools for cohort and training set selection, allowing researchers to launch slides and associated annotations in third party applications.Image analysis results can then be imported back into Xplore, providing a central repository for virtual slides, clinical, genomic and molecular data and image analysis results. As before, any data captured alongside the slide can be searched upon, allowing both image analysis and manual scoring data to be queried alongside patient information.Embed in the lab eco systemOur solution Flexible platform that integrates and brings together expertise Xplore embeds easily with a lab ecosystem through the use of current lab credential systems with a single sign on capability. Xplore supports both cloud and on premise deployments.Connect your team to a shared archiveOur solution – Web-based technology and advancedsystem architectureXplore enables knowledge sharing and expertise across multiple research studies and centres by providing tools for sharing studies with colleagues.Entire studies, or parts of a study, can be shared with one or more individuals. Different access levels can be provided, to allow for review of study results, or collaborative studies, in which multiple pathologists can score and annotate slides.What Next?Digitizing slides without providing tools to manage the images and associated data will not automatically lead to efficiencies in pathological research studies. Xplore sits at the center of a research pathology workflow. Xplore will continue to develop with the vision of becoming the centerpiece for pathology informatics and data integration across the spectrum of tissue-centricdiscovery.© 2020 Koninklijke Philips N.V.All rights are reserved. Reproduction or transmission in whole or in part, in any form or by any means, electronic, mechanical or otherwise, is prohibited without the prior written consent of the copyright owner.4522 207 41601 - January 2020Visit us on: /computationalpathology or /digitalpathologyScatter Chart in Xplore with clickable data-points linking to the Viewer to view the tissue in more detail。
farmer
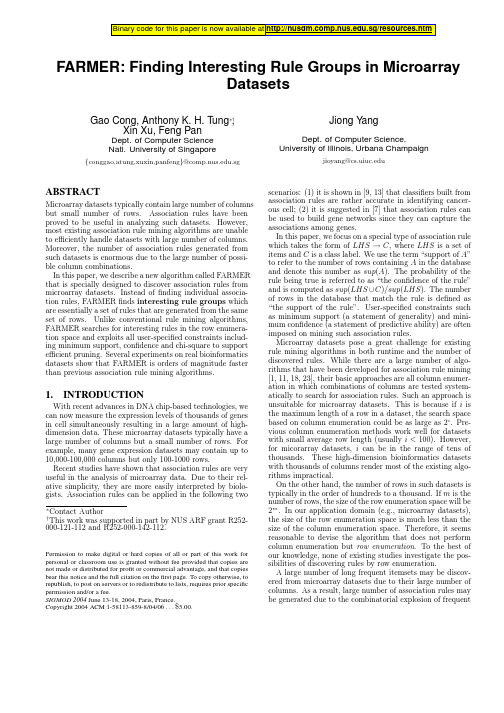
Permission to make digital or hard copies of all or part of this work for personal or classroom use is granted without fee provided that copies are not made or distributed for profit or commercial advantage, and that copies bear this notice and the full citation on the first page. To copy otherwise, to republish, to post on servers or to redistribute to lists, requires prior specific permission and/or a fee. SIGMOD 2004 June 13-18, 2004, Paris, France. Copyright 2004 ACM 1-58113-859-8/04/06 . . . $5.00.
Dept. of Computer Science, University of Illinois, Urbana Champaign
jioyang@du
ABSTRACT
Microarray datasets typically contain large number of columns but small number of rows. Association rules have been proved to be useful in analyzing such datasets. However, most existing association rule mining algorithms are unable to efficiently handle datasets with large number of columns. Moreover, the number of association rules generated from such datasets is enormous due to the large number of possible column combinations. In this paper, we describe a new algorithm called FARMER that is specially designed to discover association rules from microarray datasets. Instead of finding individual association rules, FARMER finds interesting rule groups which are essentially a set of rules that are generated from the same set of rows. Unlike conventional rule mining algorithms, FARMER searches for interesting rules in the row enumeration space and exploits all user-specified constraints including minimum support, confidence and chi-square to support efficient pruning. Several experiments on real bioinformatics datasets show that FARMER is orders of magnitude faster than previous association rule mining algorithms.
计算机算法在生物信息学中运用对策探究
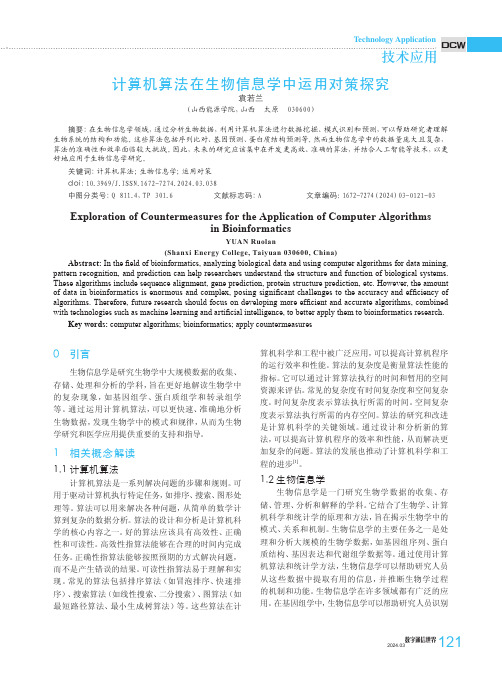
DCWTechnology Application技术应用121数字通信世界2024.030 引言生物信息学是研究生物学中大规模数据的收集、存储、处理和分析的学科,旨在更好地解读生物学中的复杂现象,如基因组学、蛋白质组学和转录组学等。
通过运用计算机算法,可以更快速、准确地分析生物数据,发现生物学中的模式和规律,从而为生物学研究和医学应用提供重要的支持和指导。
1 相关概念解读1.1 计算机算法计算机算法是一系列解决问题的步骤和规则。
可用于驱动计算机执行特定任务,如排序、搜索、图形处理等。
算法可以用来解决各种问题,从简单的数学计算到复杂的数据分析。
算法的设计和分析是计算机科学的核心内容之一。
好的算法应该具有高效性、正确性和可读性。
高效性指算法能够在合理的时间内完成任务。
正确性指算法能够按照预期的方式解决问题,而不是产生错误的结果。
可读性指算法易于理解和实现。
常见的算法包括排序算法(如冒泡排序、快速排序)、搜索算法(如线性搜索、二分搜索)、图算法(如最短路径算法、最小生成树算法)等。
这些算法在计算机科学和工程中被广泛应用,可以提高计算机程序的运行效率和性能。
算法的复杂度是衡量算法性能的指标。
它可以通过计算算法执行的时间和暂用的空间资源来评估。
常见的复杂度有时间复杂度和空间复杂度。
时间复杂度表示算法执行所需的时间。
空间复杂度表示算法执行所需的内存空间。
算法的研究和改进是计算机科学的关键领域。
通过设计和分析新的算法,可以提高计算机程序的效率和性能,从而解决更加复杂的问题。
算法的发展也推动了计算机科学和工程的进步[1]。
1.2 生物信息学生物信息学是一门研究生物学数据的收集、存储、管理、分析和解释的学科。
它结合了生物学、计算机科学和统计学的原理和方法,旨在揭示生物学中的模式、关系和机制。
生物信息学的主要任务之一是处理和分析大规模的生物学数据,如基因组序列、蛋白质结构、基因表达和代谢组学数据等。
通过使用计算机算法和统计学方法,生物信息学可以帮助研究人员从这些数据中提取有用的信息,并推断生物学过程的机制和功能。
基于深度学习的教育数据挖掘中学生学习成绩的...(IJEME-V10-N6-4)

I.J. Education and Management Engineering, 2020, 6, 27-33Published Online December 2020 in MECS (/)DOI: 10.5815/ijeme.2020.06.04Predicting Students' Academic Performance in Educational Data Mining Based on Deep Learning Using TensorFlowMussa S. Abubakari *, Fatchul ArifinDepartment of Electronics & Informatics Engineering Education, Postgraduate Program, Universitas Negeri Yogyakarta, Yogyakarta 55281, IndonesiaE-mail: abu.mussaside@*, fatchul@uny.ac.idGilbert G. HungiloDepartment of Informatics Engineering, Graduate Program, University Atma Jaya Yogyakarta, Yogyakarta 55281, IndonesiaE-mail: gutabagaonline@Received:07 May 2020; Accepted: 26 July 2020; Published: 08 December 2020Abstract: The study was aimed to create a predictive model for predicting students’ academic performance based on a neural network algorithm. This is because recently, educational data mining has become very helpful in decision making inan educational context and hence improving students’ academic outcomes. This study implemented a Neural Network algorithm as a data mining technique to extract knowledge patterns from student’s dataset consisting of 480 instances (students) with 16 attributes for each student. The classification metric used is accuracy as the model quality measurement. The accuracy result was below 60% when the Adam model optimizer was used. Although, after applying the Stochastic Gradient Descent optimizer and dropout technique, the accuracy increased to more than 75%. The final stable accuracy obtained was 76.8% which is a satisfactory result. This indicates that the suggested NN model can be reliable for prediction, especially in social science studies.Index Terms: Classification, Data Mining Techniques, Educational Data Mining, Neural Network Algorithm, Predictive Model.1.IntroductionCurrently, data mining has become an interesting topic for many researchers in various fields such as medicine, engineering, and even educational field. Especially in educational context, through mining of students’ information, it has become easier to make decisions concerning students in their academic performance [1, 2]. The prediction of students’ performance is a vital matter in educational context as predicting future performance of students after being admitted into a college, can determine who would attain poor marks and who would perform well. These results can help make efficient decisions during admission and hence improve the academic services quality [3–5].Analysis of educational data using data-mining techniques helps extract unique information of students from educational database and use that hidden information to solve various academic problems of students by understanding learners, improve teaching-learning methods and process [6, 7]. Moreover, these data mining techniques help educational stakeholders to make quality decisions to enhance students’ outcomes.Various methods like Decision tree and Naïve Bayesian were used by many researchers for predicting learners’ academic performance and make decisions to help those who need help immediately [7]. Other researchers used ensemble methods such as Random Forest (RF), AdaBoosting, and Bagging as classification methods [7, 8]. Different data mining methods can solve different educational problems such as classification and clustering. The famous known data mining method in prediction models is classification. Various deep learning algorithms like Neural Networks, are used under28 Predicting Students' Academic Performance in Educational Data Mining Based on Deep Learning Using TensorFlow classification matter [9].In the current study, neural network (NN) classification algorithm is implemented to create a predictive model in predicting academic performance of students in a particular academic institution by using students’ characteristics and their distinctive demographic data. A predictive model based on NN approach can be useful in decision making on academic success of students and therefore enhancing academic management and improving quality education.2. Related WorksVarious studies have been conducted concerning data mining in educational context for uncovering knowledge patterns from students’ information for improving academic performance of students. This current study will base its theoretical background on the previous research done on the educational data mining contexts as explained below.The study was conducted on engineering students based on different mining techniques for making academic decisions. Techniques involving classification rules and association rules for discovering knowledge patterns, were used to predict the engineering student’s performance. The study experiment also clustered the students based on k-means clustering algorithm [10]. In another study, students’ performance was evaluated based on association rule algorithm. The research was done by assessing the performance of students based on different features. The experiment was implemented based on real time dataset found in the school premises using Weka [11].Baradwaj and Pal explained in their study on student’s assessment by using a number of data mining methods. Their study facilitated teachers to identify students who need special attention to reduce the fail percentage and help to take valid measure for next semesters [3]. Also, another study was done to develop a classification model to predict student performance using Deep Learning which learns multiple levels of representation automatically. They used unsupervised learning algorithm to pre-train hidden layers of features layer-wisely based on a sparse auto-encoder from unlabeled data, and then supervised training was used for the parameters fine-tuning. The resulted model was trained on a relatively huge real-world students’ dataset, and the experimental findings indicate the effectiveness of the proposed method to be implemented into academic pre-warning mechanism [12].Other researchers developed models to predict students' university performance based on students' personal attributes, university performance and pre-university characteristics. The studies included the data of 10,330 students Bulgaria with every student having 20 attributes. Algorithms such as the K-nearest neighbour (KNN), decision tree, Naive Bayes, and rule learner's algorithms were applied to classify the students into 5 classes: Excellent, Very Good, Good, Bad or Average. Overall accuracy was below 69%. However, decision tree classifier showed best performance having the highest overall accuracy, followed by the rule learner [13, 14].Recently, the study was conducted to predict user’s intention to utilize peer-to-peer (P2P) mobile application for transactions. Logistic regression (LR) analysis technique together with neural network were used to predict the technology adoption. The results indicated that NN model has higher accuracy than LR model [15]. Another study proposed a student performance model with behavioral characteristics. These characteristics are associated with the student interactivity with an e-learning platform. Data mining techniques such as Naïve Bayesian and Decision Tree classifiers were used to evaluate the impact of such features on student’s academic performance. The results of that study revealed that there is a strong relationship between learner behaviors and its academic achievement [16].In this study, a predictive model is created based on neural network (NN) classification algorithm in predicting academic performance of students by using students’ behavioral characteristics and their distinctive demographic data as variables. A predictive model using NN data mining approach can help in making decisions and conclusions on academic success of students hence enhancing academic management and improve education quality.3. Methodology3.1 Data CollectionThe student data implemented in this project were obtained from educational dataset collected by [16] from learning management system (LMS) in The University of Jordan, Amman, Jordan during the study conducted in 2015. The dataset is available in the kaggle website (https:///aljarah/xAPI-Edu-Data). The dataset comprised of 480 (instances) of student records and their 16 respective attributes. These attributes were grouped into three classes, namely (i) Behavioral attributes include parents answering survey, school satisfaction, opening resources, and raised hand on class, (ii) Academic background attributes including grade Level, educational stage, and section, and (iii) Demographic features including nationality and gender. The dataset also includes 175 females and 305 males. The students have different nationalities including from Kuwait (179), USA (6), Jordan (172), Iraq (22), Lebanon (17), Tunis (12), Saudi Arabia (11), Egypt (9), from Iran, Syria, and Libya were 7 each, Morocco (4), 28 students from Palestine, and one from Venezuela.Another attribute is school attendance having two groups based on days of class absence: 191 students exceeded 7 days and 289 students were absent under 7 days. Moreover, the dataset includes also a new kind of attribute namely parent participation having two sub attributes: Parent School Satisfaction and Parent Answering Survey. 270 parents participated in a survey answering and 210 did not, 292 parents were satisfied from the school and 188 were not. The students arePredicting Students' Academic Performance in Educational Data Mining Based on Deep Learning Using TensorFlow 29 grouped into three classes based on their total grades, namely High-Level, Middle-Level, and Low-Level [8]. Appendix A summarizes the students’ attributes and their description.3.2 Methods and Data PreparationFor this study, authors used Anaconda software environment for python machine learning language together with keras machine learning library and specifically TensorFlow utility which is powerful to create and evaluate the proposed NN classification model [17–19]. Keras is a python library widely used in deep-learning that run on top of TensorFlow and Theano, providing an intuitive best API for Python in NNs [20, 21]. Since the dataset used in this study contains variables (attributes) with different categories, there was a need to transform them into a form the computer and NN model can understand. The dataset explained above consists of three main categories of variables. First are nominal variables with two categories such as gender (male or female), semester (first or second), and others. Second, are variables with numerical values such as visited resources, raised hand, and others. And third, are nominal variables with more than three categories such as grade levels (G-01 to G-12), topic (English, Math, Chemistry, and so on), and other variables as it can be seen in Appendix A.Nominal variables with two categories were transformed using label encoder mechanism. While, those with three or more categories were transformed using one-hot encoding (dummies method). Furthermore, continuous numerical variables were transformed by normalizing them using min-max scaler mechanism for normal distribution.4. Experiment Process and ResultsAfter data transformation as explained above, the inputs increased from 16 inputs to 39 inputs and the output (classification outputs) of 3 outputs making a total of 42 columns in the NN model. After that, the dataset was split into train data and test data with data for testing consisting of less than 26% of all dataset and the remaining percentage for training.The following step was to create a predictive model based on Artificial Neural Network (ANN) classification technique to evaluate the attributes which influence directly or indirectly student's academic success. ANN technique is an implementation of artificial neural network that involves training data inputs for the best accuracy achievement. A cross validation with 10-fold was used to divide the dataset for training and testing process. Then the process was followed by fitting the model by 200 iteration (epochs) with 10 batch-size of inputs and then followed by the results evaluation for generating knowledge representation. The evaluation measure used is accuracy for classification quality. Accuracy is the proportion or ratio of the total number of correct predictions to incorrectly predicted.Fig. 1. The NN Model Structure.Figure 1 above shows the NN model structure created by a python code as can be seen in the last code line in Appendix B. The NN predictive model used in this study consists of three layers: (1) input layer with 39 neurons, (2) hidden layer30 Predicting Students' Academic Performance in Educational Data Mining Based on Deep Learning Using TensorFlowwith 19 neurons and (3) an output layer with 3 outputs. The input layer receives input data from 16 attributes and the output layer send output of three grade categories, namely Low (L). Middle (M), and High (H). There is a hidden layer between the input layer and output layer. Appendix B illustrate the python code used to create, fit, and validate the NN model.In this study, we used accuracy as the metric for prediction quality of the developed NN model. Also, only NN algorithm was used for classification of the student dataset.The result of the experiment has two versions due to the implementation of two different model (function) optimizers namely, Adam and Stochastic gradient descent (SGD) as well as due to the introduction of dropout technique to the NN model development to drop (20% of neurons were dropped in this study) loosely connected neuron. The result indicates that when we applied Adam optimization technique the accuracy was below 60%. While, when we applied the SGD optimizer the accuracy improved to more than 76%.Moreover, the dropout technique helped to improve the accuracy value to more than 76.5%. The dropout technique is used to remove the loosely connected neurons as the NN technique performs better with fully connected neurons. The final stable result was 76.8% accuracy.5. Conclusion and Future WorkEducation is a vital element in any community for their social-economic development. Data mining techniques or business intelligence allows extracting knowledge patterns from students’ raw data offering interesting chances for the educational context. Particularly, various studies have implemented machine learning techniques like Decision Tree and Random Forest to enhance the management of college resources and hence improving education quality.In this study, the authors have presented a predictive model using NN technique to learn the patterns from students’ data and predict their academic performance. By applying data mining techniques on students’ database, academic stakeholders can find the important factors which have direct or indirect impacts on the student’s academic success. The knowledge patterns and results discovered in this study after applying NN classification method indicate that different attributes of students have impacts on their learning process as it can be seen in the classification accuracy results. The final classification accuracy obtained in this study is 76.9% which is more than satisfactory percentage for our predictive model developed using NN algorithm.Like other studies, this study is with some limitations too. One of which is the dataset can only be applied to the similar context as this study. Also, the results presented here involves the accuracy as the only predictive measure of model quality. Moreover, only one algorithm, NN algorithm was used for classification purpose.For future studies, authors intend to use the localized student data from a particular university in Yogyakarta, especially from Yogyakarta State University. Also, in the future we expect to apply other data mining methods such as RF, DT, and others in the localized dataset. Moreover, future experiments will add more measurement classification qualities such as Precision, sensitivity, and Recall.AcknowledgementsMuch appreciation to my close friends who inspired me to do this work.References[1]S. K. Mohamad and Z. Tasir, “Educational Data Mining: A Review,” Procedia - Soc. Behav. Sci., vol. 97, pp. 320–324, 2013.[2]M. Chalaris, S. Gritzalis, M. Maragoudakis, C. Sgouropoulou, and A. Tsolakidis, “Improving Quality of Educational ProcessesProviding New Knowledge Using Data Mining Techniques,” Procedia - Soc. Behav. Sci., vol. 147, pp. 390–397, 2014.[3] B. Brijesh Kumar and P. Saurabh, “Mining Educational Data to Analyze Students‟ Performance,” Int. J. Adv. Comput. Sci.Appl., vol. 2, no. No. 6, pp. 59–63, 2011.[4]W. F. W. Yaacob, S. A. M. Nasir, W. F. W. Yaacob, and N. M. Sobri, “Supervised data mining approach for predicting studentperformance,” Indones. J. Electr. Eng. Comput. Sci., vol. 16, no. 3, pp. 1584–1592, 2019.[5]H. Aldowah, H. Al-Samarraie, and W. M. Fauzy, “Educational data mining and learning analytics for 21st century highereducation: A review and synthesis,” Telemat. Informatics, vol. 37, pp. 13–49, 2019.[6]S. Hussain, N. A. Dahan, F. M. Ba-Alwib, and N. Ribata, “Educational data mining and analysis of students’ academicperformance using WEKA,” Indones. J. Electr. Eng. Comput. Sci., vol. 9, no. 2, pp. 447–459, 2018.[7]S. S. M. Ajibade, N. B. Ahmad, and S. M. Shamsuddin, “A data mining approach to predict academic performance of studentsusing ensemble techniques,” in Advances in Intelligent Systems and Computing, 2020, vol. 940, no. March, pp. 749–760.[8] E. A. Amrieh, T. Hamtini, and I. Aljarah, “Mining Educational Data to Predict Student’s academic Performance using EnsembleMethods,” Int. J. Database Theory Appl., vol. 9, no. 8, pp. 119–136, 2016.[9] A. M. Shahiri, W. Husain, and N. A. Rashid, “A Review on Predicting Student’s Performance Using Data Mining Techniques,”in Procedia Computer Science, 2015, vol. 72, pp. 414–422.[10]R. Singh, “An Empirical Study of Applications of Data Mining Techniques for Predicting Student Performance in HigherEducation,” Int. J. Comput. Sci. Mob. Comput., vol. 2, no. February, pp. 53–57, 2013.Predicting Students' Academic Performance in Educational Data Mining Based on Deep Learning Using TensorFlow 31 [11]S. Borkar and K. Rajeswari, “Predicting students academic performance using education data mining,” Int. J. Comput. Sci. Mob.Comput., vol. 2, no. 7, pp. 273–279, 2013.[12]B. Guo, R. Zhang, G. Xu, C. Shi, and L. Yang, “Predicting Students Performance in Educational Data Mining,” in Proceedings -2015 International Symposium on Educational Technology, ISET 2015, 2016, pp. 125–128.[13]D. Kabakchieva, “Predicting student performance by using data mining methods for classification,” Cybern. Inf. Technol., vol.13, no. 1, pp. 61–72, 2013.[14]D. Kabakchieva, K. Stefanova, and V. Kisimov, “Analyzing university data for determining student profiles and predictingperformance,” in EDM 2011 - Proceedings of the 4th International Conference on Educational Data Mining, 2011, pp. 347–348.[15]J. Lara-Rubio, A. F. Villarejo-Ramos, and F. Liébana-Cabanillas, “Explanatory and predictive model of the adoption of P2Ppayment systems,” Behav. Inf. Technol., vol. 0, no. 0, pp. 1–14, 2020.[16]E. A. Amrieh, T. Hamtini, and I. Aljarah, “Preprocessing and analyzing educational data set using X-API for improvingstudent’s performance,” in 2015 IEEE Jordan Conference on Applied Electrical Engineering and Computing Technologies, AEECT 2015, 2015.[17]P. S. Janardhanan, “Project repositories for machine learning with TensorFlow,” Procedia Comput. Sci., vol. 171, pp. 188–196,2020.[18]L. Hao, S. Liang, J. Ye, and Z. Xu, “TensorD: A tensor decomposition library in TensorFlow,” Neurocomputing, vol. 318, pp.196–200, 2018.[19]R. Orus Perez, “Using TensorFlow-based Neural Network to estimate GNSS single frequency ionospheric delay (IONONet),”Adv. Sp. Res., vol. 63, no. 5, pp. 1607–1618, 2019.[20]V.-H. Nhu et al., “Effectiveness assessment of Keras based deep learning with different robust optimization algorithms forshallow landslide susceptibility mapping at tropical area,” CATENA, vol. 188, p. 104458, 2020.[21]K. Akyol, “Comparing of deep neural networks and extreme learning machines based on growing and pruning approach,”Expert Syst. Appl., vol. 140, p. 112875, 2020.Authors’ ProfilesMussa S. Abubakari was born in Kondoa, Tanzania in 1990. He received the B.Sc. degree inTelecommunications Engineering from the University of Dodoma, Tanzania in 2016. Currently he is thepostgraduate candidate taking master degree in Electronics & Informatics Engineering Education atUniversitas Negeri Yogyakarta, Indonesia. His research interests include technology enhanced learning,human computer interaction, technology acceptance, Internet of Things, mobile technologies, intelligentsystems, and signal processing.Dr. Fatchul Arifin was born on 08 Mei 1972. He received a B.Sc. in Electric Engineering at UniversitasDiponegoro and PH.D. degree in Electric Engineering from Institut Teknologi Surabaya, in 1996 and 2014,respectively. Currently he is the lecturer at both undergraduate faculty of engineering and postgraduateprogram at Universitas Negeri Yogyakarta. His research interests include but not limited to intelligentcontrol systems, machine learning, expert systems, and neural-fuzzy system.Gilbert G. Hungilo is a master degree graduate from department of Informatics Engineering at theUniversity Atma Jaya Yogyakarta, Indonesia. He received Bachelor of Science in Computer Science fromthe University of Dar es salaam, Tanzania. His research interests include technology adoption, big dataanalytics, and machine learning.How to cite this paper: Mussa S. Abubakaria, Fatchul Arifin, Gilbert G. Hungilo. "Predicting Students' Academic Performance in Educational Data Mining Based on Deep Learning Using TensorFlow ", International Journal of Education and Management Engineering (IJEME), Vol.10, No.6, pp.27-33, 2020. DOI: 10.5815/ijeme.2020.06.0432 Predicting Students' Academic Performance in Educational Data Mining Based on Deep Learning Using TensorFlowAppendix A. Students’ Attributes [16]SN Attribute Description Variable Type1 Gender Gender of Student: Female or Male. Nominal(binary)2 Nationality Student's Origin: Kuwait, Iraq, Libya Lebanon, Egypt, USA,Morocco, Jordan, Iran, Tunis, Syria, Palestine, Saudi Arabia,Venezuela.Nominal(dummy)3 Birth Place Student's Birth Place: Kuwait, Iraq, Libya Lebanon, Egypt,USA, Morocco, Jordan, Iran, Tunis, Syria, Palestine, SaudiArabia, Venezuela.Nominal(dummy)4 Stage ID Student Educational Level: High School, Middle School,Lower level.Nominal(dummy)5 Grade ID Student Grade: G-01 up to G-12. Nominal(dummy)6 Section ID Classroom student belongs: A, B, C. Nominal(dummy)7 Topic Course Studied: Arabic, Biology, Chemistry, English,Geology, French, Spanish, IT, Math, Science, History, Quran.Nominal(dummy)8 Semester School year semester: First, Second. Nominal(binary)9 Relation Responsible Parent: Mom, Father. Nominal(binary)10 Raised hand Frequency of raising hand in classroom: 0-100. Numeric11 VisitedresourcesFrequency of visiting course online content: 0-100. Numeric12 AnnouncementsViewFrequency of checking the new online announcement: 0-100. Numeric13 Discussion Frequency of participating in online discussion forums: 0-100. Numeric14 Parent SurveyAnsweringWhether Parents answered or not the survey: Yes, No. Nominal(binary)15 Parent SchoolSatisfactionWhether a parent is satisfied or not: Yes, No. Nominal(binary)16 Student AbsenceDays The number of absence days a student was absent: Above orUnder 7 days.Nominal(binary)17 Class The grade class: High-Level (H): from 90-100; Middle-Level(M): from 70 to 89; Low-Level (L): from 0 to 69.Nominal(dummy)Predicting Students' Academic Performance in Educational Data Mining Based on Deep Learning Using TensorFlow 33 Appendix B. A Piece of Python Code Used to Create and Validate an NN Model。
Int J Oncol Vol35 n2 page 393
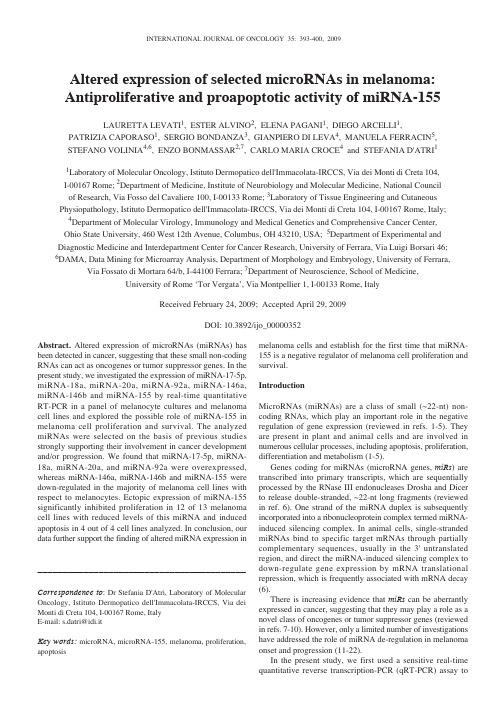
Abstract.Altered expression of microRNAs (miRNAs) has been detected in cancer, suggesting that these small non-coding RNAs can act as oncogenes or tumor suppressor genes. In the present study, we investigated the expression of miRNA-17-5p, miRNA-18a, miRNA-20a, miRNA-92a, miRNA-146a, miRNA-146b and miRNA-155 by real-time quantitative RT-PCR in a panel of melanocyte cultures and melanoma cell lines and explored the possible role of miRNA-155 in melanoma cell proliferation and survival. The analyzed miRNAs were selected on the basis of previous studies strongly supporting their involvement in cancer development and/or progression. We found that miRNA-17-5p, miRNA-18a, miRNA-20a, and miRNA-92a were overexpressed, whereas miRNA-146a, miRNA-146b and miRNA-155 were down-regulated in the majority of melanoma cell lines with respect to melanocytes. Ectopic expression of miRNA-155 significantly inhibited proliferation in 12 of 13 melanoma cell lines with reduced levels of this miRNA and induced apoptosis in 4 out of 4 cell lines analyzed. In conclusion, our data further support the finding of altered miRNA expression in melanoma cells and establish for the first time that miRNA-155 is a negative regulator of melanoma cell proliferation and survival.IntroductionMicroRNAs (miRNAs) are a class of small (~22-nt) non-coding RNAs, which play an important role in the negative regulation of gene expression (reviewed in refs. 1-5). They are present in plant and animal cells and are involved in numerous cellular processes, including apoptosis, proliferation, differentiation and metabolism (1-5).Genes coding for miRNAs (microRNA genes, miRs) are transcribed into primary transcripts, which are sequentially processed by the RNase III endonucleases Drosha and Dicer to release double-stranded, ~22-nt long fragments (reviewed in ref. 6). One strand of the miRNA duplex is subsequently incorporated into a ribonucleoprotein complex termed miRNA-induced silencing complex. In animal cells, single-stranded miRNAs bind to specific target mRNAs through partially complementary sequences, usually in the 3' untranslated region, and direct the miRNA-induced silencing complex to down-regulate gene expression by mRNA translational repression, which is frequently associated with mRNA decay (6).There is increasing evidence that miRs can be aberrantlyexpressed in cancer, suggesting that they may play a role as a novel class of oncogenes or tumor suppressor genes (reviewedin refs. 7-10). However, only a limited number of investigations have addressed the role of miRNA de-regulation in melanoma onset and progression (11-22).In the present study, we first used a sensitive real-time quantitative reverse transcription-PCR (qRT-PCR) assay toAltered expression of selected microRNAs in melanoma:Antiproliferative and proapoptotic activity of miRNA-155LAURETTA LEVATI1, ESTER ALVINO2, ELENA PAGANI1, DIEGO ARCELLI1, PATRIZIA CAPORASO1, SERGIO BONDANZA3, GIANPIERO DI LEVA4, MANUELA FERRACIN5, STEFANO VOLINIA4,6, ENZO BONMASSAR2,7, CARLO MARIA CROCE4and STEFANIA D'ATRI11Laboratory of Molecular Oncology, Istituto Dermopatico dell'Immacolata-IRCCS, Via dei Monti di Creta 104, I-00167 Rome;2Department of Medicine, Institute of Neurobiology and Molecular Medicine, National Council of Research, Via Fosso del Cavaliere 100, I-00133 Rome; 3Laboratory of Tissue Engineering and Cutaneous Physiopathology, Istituto Dermopatico dell'Immacolata-IRCCS, Via dei Monti di Creta 104, I-00167 Rome, Italy;4Department of Molecular Virology, Immunology and Medical Genetics and Comprehensive Cancer Center, Ohio State University, 460 West 12th Avenue, Columbus, OH 43210, USA; 5Department of Experimental and Diagnostic Medicine and Interdepartment Center for Cancer Research, University of Ferrara, Via Luigi Borsari 46;6DAMA, Data Mining for Microarray Analysis, Department of Morphology and Embryology, University of Ferrara, Via Fossato di Mortara 64/b, I-44100 Ferrara; 7Department of Neuroscience, School of Medicine,University of Rome ‘Tor Vergata’, Via Montpellier 1, I-00133 Rome, ItalyReceived February 24, 2009; Accepted April 29, 2009DOI: 10.3892/ijo_00000352_________________________________________Correspondence to:Dr Stefania D'Atri, Laboratory of MolecularOncology, Istituto Dermopatico dell'Immacolata-IRCCS, Via deiMonti di Creta 104, I-00167 Rome, ItalyE-mail: s.datri@idi.itKey words:microRNA, microRNA-155, melanoma, proliferation,apoptosisevaluate, in a panel of melanoma cell lines and normal melanocytes, the expression levels of seven miRNAs, namely miRNA-17-5p, miRNA-18a, miRNA-20a, miRNA-92a,miRNA-146a, miRNA-146b and miRNA-155. Actually,previous studies indicated that alterations in the expression of these miRNAs may have a role in cancer development and/or progression (7-10). Thereafter we focused our attention on miRNA-155, since it resulted markedly down-regulated in the majority of melanoma cell lines. In order to establish the possible biological significance of the low miRNA-155expression in melanoma, we investigated the effects of the ectopic expression of the miRNA on in vitro melanoma cell proliferation and apoptosis. Materials and methodsCell lines and normal melanocytes . Seventeen human melanoma cell lines were used in this study and cultured as previously described (23). GR-Mel and PNP-Mel were derived from primary melanomas, whereas the other cell lines were originated from metastatic lesions.Human melanocytes were isolated from normal skin biopsies of 10 different donors and cultured, as previously described (24).All biological material was obtained with the patient's informed consent and the study was conducted according to the Declaration of Helsinki Principles.Low molecular weight (LMW) RNA isolation and qRT-PCR analysis of miRNA expression . LMW RNA was isolated from melanocytes and melanoma cell lines using the mir Vana™miRNA isolation kit (Ambion, Austin, TX) according to the manufacturer's protocol. LMW RNA was quantified using the NanoDrop ND-1000 spectrophotometer (Thermo Fisher Scientific Inc, Waltham, MA).To evaluate the expression of U6 small nuclear RNA (snRNA) and mature miRNAs, the TaqMan®MicroRNA Reverse Transcription kit, the TaqMan Universal PCR Master Mix No AmpErase ®UNG and the TaqMan MicroRNA Assay for U6 snRNA and the selected miRNAs, all purchased from Applied Biosystems (Foster City, CA), were used. All experimental procedures were performed according to the manufacturer's protocols. One or 10 ng of LMW RNA were reverse transcribed in a final volume of 15 μl and qRT-PCR was done on an ABI PRISM 7000 Sequence Detection System (Applied Biosystems) in a final volume of 20 μl. All qRT-PCR reactions were run in duplicate. The expression of the miRNAs under investigation relative to miRNA-16 was determined using the formula 2-ΔC T , where ΔC T = (C TmiRNA - C TmiRNA-16) and C T (i.e. threshold cycle) indicates the fractional cycle number at which the amount of amplified target reaches a fixed threshold (25).Transfection . Pre-miR hsa-miR-155 miRNA Precursor (pre-miRNA-155) and Pre-miR miRNA Precursor Negative Control #1 (dsRNA-CTRL) were obtained from Ambion.To evaluate the effect of miRNA-155 on cell growth,melanoma cells were seeded into 24-well plates (Falcon,Becton and Dickinson Labware, Franklin Lakes, NJ) and allowed to adhere at 37˚C for 18 h. The cells were thentransfected with pre-miRNA-155 or dsRNA-CTRL. Trans-fection was performed using Oligofectamine or Lipofectamine 2000 (Invitrogen Corporation, Carlsbad, CA) in serum-free medium, according to the manufacturer's protocol. Additional controls consisted in melanoma cells left untreated or exposed to the transfection reagent only (mock-transfected cells). Three replica wells were used for each group. After 72 h of culture,the cells were subjected to a second transfection. Seventy-two hours later, the cells were harvested by trypsinization and cell growth was evaluated in terms of viable cell count.Transfection efficiency was evaluated using a fluorescein-labeled double-stranded RNA oligomer designated BLOCK-iT™fluorescent oligonucleotide (Invitrogen).Evaluation of apoptosis . Melanoma cells were plated in duplicate in 24-well plates and transfected with 100 nM pre-miRNA-155 or dsRNA-CTRL, as described above. Forty-eight hours after a single transfection procedure, apoptotic death was evaluated using the Cell Death Detection ELISA PLUS kit (Roche Diagnostics GmbH, Mannheim, Germany), according to the manufacturer's protocols. This kit allows the quantitative determination of mono- and oligonucleosomes that accumulate in the cytoplasm of cells undergoing apoptosis before plasma membrane breakdown. Signals were determined in a Microplate Reader 3550-UV (Bio-Rad, Hercules, CA). Data were expressed in terms of ‘Enrichment Factor’, calculated as the ratio between the adsorbance values of pre-miRNA-155-transfected cells and those of dsRNA-CTRL-transfected cells.Statistical analysis of qRT-PCR data . We performed two different statistical analyses to assess the significance of the differences in miRNA expression within a class of samples and between classes of samples.The statistical differences within a class of samples were determined using a customized script employing Bioconductor packages () and based on the R language (). In particular, we used ‘Permtest’ and ‘BootPR’ R-packages to perform t-test in conjunction with bootstrap analysis in order to determine which gene between U6 snRNA and miRNA-16 had the lowest variability among melanocytes. Fifty-thousand permutations were applied to the test in order to define the confidence limits and the corresponding significance thresholds. The statistical significance of the differential expression of U6 snRNA or miRNA-16 among the melanocytes was assessed by computing a P-value for each 2-CT value. No specific parametric form was assumed for the distribution of the test statistics. To determine the P-value, we used a permutation procedure in which the expression value of U6 snRNA or miRNA-16 was permuted 500,000 times, and for each permutation, two-sample t-statistics were computed for each value. The permutation P-value for a particular value is the proportion of the permutations (out of 500,000) in which the permuted test statistic exceeds the observed test statistic in absolute values.The same statistical analysis was applied to assess variability of miRNA-16 expression within the group of melanoma cell lines.The statistical significance of the differences in miRNA expression between melanoma cell lines and melanocytes was assessed by Student's t-test analysis performed on 2-ΔCT values.ResultsSelection of the internal control gene for the evaluation of miRNA expression by qRT-PCR. Previous studies showed that melanin inhibits RT-PCR (26). Therefore, to reduce melanin contamination and to enrich the miRNA content, we used column purified LMW RNA for qRT-PCR. U6 snRNA and miRNA-16 have been previously used as internal control genes to determine the relative expression of a large number of miRNAs in cell lines by qRT-PCR performed on total RNA (11,27). Therefore, preliminary experiments were carried out to select, between the two genes, the best internal control to be adopted for the present study.The first set of experiments showed that the 2-CT value of miRNA-16 was significantly higher (P<0.01 according to Student's t-test) than that of U6 snRNA in melanocytes isolated from normal skin biopsies of 10 different donors (data not shown). Moreover, t-test in conjunction with bootstrap analysis showed that variability of U6 snRNA expression among the different melanocyte samples was higher than that of miRNA-16 (i.e. P<0.01 or P<0.05 in 8 out of 10 samples in the case of U6 snRNA vs. no statistically significant variation in the case of miRNA-16, data not shown).In a subsequent set of experiments, the expression level of miRNA-16, in terms of 2-CT value, was determined in parallel in the 10 melanocyte samples and in 17 melanoma cell lines. The results of t-test in conjunction with bootstrap analysis confirmed that no significant differences existed in the expression of miRNA-16 within the group of melanocytes (Fig. 1). The same statistical analysis applied to melanoma cell lines identified three outliers (Fig. 1), indicating that de-regulation of miRNA-16 can occur in some melanomas. These 3 cell lines were then excluded from further analysis. Student's t-test analysis performed on miRNA-16 2-CT values relative to the remaining 14 melanoma cell lines and to the melanocytes showed no significant difference (Fig. 1). On these bases we selected miRNA-16 as the internal control gene for qRT-PCR assays.Expression of mature miRNAs in normal cultured melanocytes and melanoma cell lines. All qRT-PCR assays were performed using 1.33 μl of cDNA reverse transcribed from 1 ng of LMW RNA. However, the levels of miRNA-155 were found to be extremely low in melanoma cells. Therefore, the expression of this miRNA was evaluated using 1.33 μl of cDNA reverse transcribed from 10 ng of LMW RNA.As illustrated in Table I, all melanocyte samples expressed the miRNAs under investigation, being miRNA-146a the most expressed and miRNA-18a the less expressed miRNA in all samples.To identify miRNAs dysregulated in melanoma cell lines with respect to melanocytes, we performed Student's t-test analysis between the two groups (i.e. all melanoma cell lines vs. all melanocytes) and between each melanoma cell line and the group of melanocytes. When melanoma cell lines and melanocytes were compared as two groups, miRNA-17-5p, miRNA-18a, and miRNA-20a, which are encoded by the miR-17-92cluster, resulted overexpressed in the melanoma group (Table I). miRNA-92a, which is generated from the transcription of two different miRs(i.e. miR-92a-1in the miR-17-92cluster and miR-92a-2, in the miR-106a-363cluster) (reviewed in ref. 28) was also up-regulated in the melanoma group (Table I). In contrast, the expression of miRNA-146a, miRNA-146b and miRNA-155, was significantly reduced in the melanoma group with respect to that of melanocytes (Table I).When each melanoma cell line was compared with the melanocyte group, the four miRNAs encoded by the miR-17-92 cluster were up-regulated simultaneously in 9 cell lines. In contrast, miRNA-146a and miRNA-146b, were found to be concomitantly down-regulated in 10 melanomas and miRNA-155 resulted to be significantly reduced in 13 cell lines (Table I).Transfection of pre-miRNA-155 into melanoma cell lines inhibits proliferation. To investigate the biological significance of miRNA-155 down-regulation in melanoma cells, we decidedFigure 1. Expression levels of miRNA-16 in normal melanocytes and melanoma cell lines. Equal amounts (1.33 μl) of cDNA reverse transcribed from 1 ng of LMW RNA were used to determined by qRT-PCR the expression of miRNA-16 in normal skin melanocytes obtained from 10 different donors and in 17 melanoma cell lines. Data are expressed in terms of 2-CT x109. Each value represents the mean of six independent experiments, in which qRT-PCRs were run in duplicate. Bars, standard error of the mean. Among the melanoma group, three cell lines were found to be outliers (**P<0.01, according to t-test in conjunction with bootstrap analysis). The mean expression value for the melanocyte group was 155±8.13 and the mean expression value for the melanoma cell line group, not including CG-Mel, CR-Mel and MR-Mel, was 155±8.25.to assess the effect of ectopic expression of the miRNA on cell proliferation. To this end, all the melanoma cell lines were left untreated, mock-transfected or subjected to two sequential transfection procedures with 100 nM of a double-stranded RNA mimicking the endogenous precursor of miRNA-155(pre-miRNA-155) or with a double-stranded control RNA (dsRNA-CTRL). Cell growth was evaluated in terms of viable cell count 72 h after the second transfection.In preliminary experiments, the cell lines were transfected with either Oligofectamine or Lipofectamine 2000 and the BLOCK-iT™fluorescent oligonucleotide (100 nM) and assayed for transfection efficiency after 24 h. The cell lines were also subjected to two sequential transfections with the transfection reagents alone and assayed for proliferation 72 h after the second transfection. Based on the results of this set of experiments (data not shown), in 13 out of 14 cell lines we were able to select for the transfection reagent providing an acceptable transfection efficiency and minimal effects on proliferation (Table II and Fig. 2). In the remaining cell line (i.e. CT-Mel), we used Lipofectamine 2000 despite its marked inhibitory effect on cell growth because transfection efficiency with Oligofectamine was only 10%.The effects of pre-miRNA-155 transfection on melanoma cell growth are illustrated in Fig. 2. In 12 melanoma cell lines,growth inhibition induced by transfection of pre-miRNA-155was significantly higher than that observed in dsRNA-CTRL-transfected cells, with percentages of cell growth inhibition ranging between 30 and 98%. In the remaining cell lines (i.e. GR-Mel and PNM-Mel), no increase of cell growth inhibition was induced by pre-miRNA-155 transfection with respect to dsRNA-CTRL. Notably, GR-Mel, was the only cell line showing miRNA-155 levels comparable to those of melanocytes.To further assess the inhibitory activity of miRNA-155 on melanoma cell growth, a concentration-response curve was set up with CH-Mel, DR-Mel, GL-Mel and SK-Mel-28 cell lines.The results illustrated in Fig. 3 show that pre-miRNA-155,but not dsRNA-CTRL induced a concentration-dependent inhibition of cell growth.Table I. Expression of miRNAs in human normal melanocytes and melanoma cell lines.–––––––––––––––––––––––––––––––––––––––––––––––––––––––––––––––––––––––––––––––––––––––––––––––––––––Melanoma cell lines showing Melanoma cell lines showingmiRNA Relative expression level (2-ΔCT )aup-regulation of the miRNAdown-regulation of the miRNA–––––––––––––––––––––––––––––––––––––––––––––––––––––––––––––––––––––––––––––––––––––––––––––––––––––Melanocytes Melanoma P b No.c MC:NM Ratio d No.c MC:NM Ratio d–––––––––––––––––––––––––––––––––––––––––––––––––––––––––––––––––––––––––––––––––––––––––––––––––––––17-5p 0.045±0.0020.132±0.020<0.0110 2.3-5.50-18a 0.016±0.0010.049±0.008<0.0110 2.1-6.40-20a 0.247±0.0100.449±0.054<0.0111 1.5-3.420.7-0.692a 0.227±0.0180.367±0.041<0.0110 1.3-3.110.5146a 2.057±0.142 1.189±0.169<0.010-100.8-0.2146b 1.770±0.1440.861±0.140<0.010-100.6-0.11550.069±0.0110.005±0.004<0.010-130.2-0.0004–––––––––––––––––––––––––––––––––––––––––––––––––––––––––––––––––––––––––––––––––––––––––––––––––––––a The expression of miRNAs was determined by qRT-PCRs in melanocytes obtained from 10 different donors and in 14 melanoma cell lines.Values represent the mean ±standard error of the mean of the melanocyte or the melanoma group. For each melanocyte sample and melanoma cell line at least three independent experiments, in which qRT-PCRs were run in duplicate, were performed. b P, probability according to Student's t-analysis comparing miRNA expression values of melanocytes with those of melanoma cell lines.c Number of melanoma cell lines in which miRNA expression value was significantly higher or lower (P<0.05 according to Student's t-test analysis) than the mean expression value of the melanocyte group. d Range of the ratio between the mean expression value of each melanoma cell line (MC) and the mean expression value of the melanocyte group (NM).–––––––––––––––––––––––––––––––––––––––––––––––––––––––––––––––––––––––––––––––––––––––––––––––––––––Figure 2. Ectopic expression of miRNA-155 inhibits melanoma cell growth.Melanoma cells were left untreated, mock-transfected or subjected to two sequential transfections with 100 nM pre-miRNA-155 or dsRNA-CTRL, as described under Materials and methods. Seventy-two hours after the second transfection, the cells were harvested by trypsinization and cell growth was evaluated in terms of viable cell count. Data are expressed in terms of percentage of growth inhibition of target cells transfected with pre-miRNA-155, or dsRNA-CTRL or mock-transfected with respect to the untreated cells. Each value represents the mean of at least three independent experiments performed with triplicate samples, with bars indicating standard error of the mean. **P<0.01, according to Student's t-test, comparing the percentages of cell growth inhibition of pre-miRNA-155-transfected cells with those of dsRNA-CTRL-transfected cells. Percentages were subjected to angular transformation in order to obtain normally distributed data.Thereafter, conventional standard error calculation and Student's t-test statistics were performed on converted data. However, the data are expressed in non-transformed percentages, following conversion of transformed data into the original values.Transfection of pre-miRNA-155 into melanoma cell lines induces apoptosis. To investigate whether the growth inhibitory effect of pre-miRNA-155 could be due, at least in part, to the triggering of apoptosis, experiments were performed on 4 different cell lines, that were subjected to a single transfection with 100 nM pre-miRNA-155 or dsRNA-CTRL and assayed for apoptosis 48 h later. Ectopic expression of miRNA-155 was able to induce apoptosis in all the 4 cell lines tested (Fig. 4). Moreover, apoptosis was particularly pronounced in the two cell lines in which transfection with pre-miRNA-155 was followed by strong cell growth suppression (i.e. CH-Mel and DR-Mel).DiscussionAberrant expression of the miR-17-92cluster or single components of the cluster, as well as of miR-146a, miR-146b and miR-155can have a role in tumorigenesis (7,8,10,28). To investigate whether these miRs could be deregulated in melanoma, we comparatively analyzed the expression levels of the corresponding mature miRNAs in a panel of human melanoma cell lines and cultured normal melanocytes. Notably, the qRT-PCR assays were performed on RNA preparations enriched for miRNAs, with conceivably reduced content of melanin. Moreover, the internal control gene (i.e. miRNA-16) was selected following an experimental survey of two candidate small RNA molecules largely used as reference gene transcripts. We found that miRNA-17-5p, miRNA-18a, miRNA-20a, and miRNA-92a were up-regulated, whereas miRNA-146a, miRNA-146b and miRNA-155 were down-regulated in the majority of the melanoma cell lines analyzed.We focused our attention on the biological role of miRNA-155, that was found, for the first time, to be a candidate gene able to control melanoma cell growth and survival. Indeed, we observed that enforced expression of miRNA-155 was able to inhibit proliferation and induce apoptosis in melanoma cells expressing low levels of this miRNA.In humans, miR-155resides within the BIC gene on chromosome 21 (7,8). High levels of miRNA-155 have been found in B-CLL, B-cell lymphomas, papillary tyroid carcinoma, breast cancer (7-10), pancreatic ductal adeno-carcinoma (29) and other tumors (30). Moreover, enforced expression of miRNA-155 is sufficient to trigger murine B lymphoma (7). On the other hand, miRNA-155 was reported to be expressed in healthy pancreas and essentially absent in endocrine pancreatic tumors (10). Moreover, the levels of this miRNA were found to be reduced in ovarian cancer (31). These findings suggest that miR-155can act either as oncogene or as tumor suppressor gene, depending on the cell background in which miRNA-155 is performing its specific target gene controlling function.Our results demonstrate that miRNA-155, which appears to be the most altered miRNA among those analyzed, is a negative regulator of melanoma cell growth. Actually, ectopic expression of miRNA-155 significantly inhibited proliferation in 12 out of 13 melanoma cell lines endowed with low miRNA-155 levels. In contrast, enforced expression of this miRNA did not affect the growth of GR-Mel cells, which display miRNA-155 levels comparable to those of melano-cytes. It must be noted that the transfection efficiency of GR-Mel cells was ~70-80%, thus eliminating the possibility that ineffective pre-miRNA-155 uptake might underlie the lack of response. Moreover, a concentration-dependent inhibitionTable II. Transfection efficiency and cell growth inhibition relative to the transfection reagent selected for melanoma cell lines.–––––––––––––––––––––––––––––––––––––––––––––––––––––––––––––––––––––––––––––––––––––––––––––––––––––Transfection efficiency a Cell growth inhibition b Transfection reagent Cell line Range (%)Range (%)–––––––––––––––––––––––––––––––––––––––––––––––––––––––––––––––––––––––––––––––––––––––––––––––––––––Oligofectamine CH-Mel80-9010-20CN-Mel80-905-10DR-Mel80-9010-20GL-Mel60-70<5WM-266-470-80<5 Lipofectamine 2000CL-Mel80-9010-20CT-Mel90-10060-70GR-Mel70-805-20M1470-8015-25PNM-Mel90-10015-20PNP-Mel80-905-15SK-Mel-2870-805-20SN-Mel80-9015-25397-Mel80-9015-25–––––––––––––––––––––––––––––––––––––––––––––––––––––––––––––––––––––––––––––––––––––––––––––––––––––a Melanoma cells were seeded into 24-well plates, allowed to adhere at 37˚C for 18 h and then transfected with 100 nM BLOCK-iT™fluorescent oligonucleotide using Oligofectamine or Lipofectamine 2000. Transfection efficiency was evaluated 24 h after transfection using a fluorescence microscope (Axiovert 135, Zeiss, Oberkochen, Germany).b Melanoma cells were subjected to two sequential transfection procedures with the transfection reagent alone, as described in Materials and methods. Cell proliferation was evaluated 72 h after the second transfection.–––––––––––––––––––––––––––––––––––––––––––––––––––––––––––––––––––––––––––––––––––––––––––––––––––––of cell growth was observed upon transfection of pre-miRNA-155 but not of dsRNA-CTRL, thus further supporting the specificity of the inhibitory effects of miRNA-155. Impairment of melanoma cell proliferation appears to be dependent, at least in part, on miRNA-155-mediated induction of apoptosis.Indeed, in 4 out of 4 cell lines tested ectopic expression of miRNA-155 caused significantly higher levels of apoptosis than ectopic expression of dsRNA-CTRL.Since miRNAs can regulate a large number of target genes,several algorithms have been developed to predict in silico the targets of selected miRNAs. We adopted the TargetScan (/) and PicTar (/) algorithms to identify putative miRNA-155 targets and found that 160 targets are predicted by both algorithms.Down-regulation of one or more of these target genes might be involved in miRNA-155-induced impairment of melanoma cell proliferation and survival. For instance, the MAP3K14genes code for nuclear factor-inducing kinase (NIK), which plays a central role in the activation of the non-canonical NF-κB pathway in response to a subset of NF-κB-inducing stimuli (reviewed in ref. 32). It has been shown that NIK expression and/or activity is significantly higher in melanoma cells than in normal melanocytes and that overexpression of a kinase-deficient mutant of NIK strongly reduces basal NF-κBFigure 4. Ectopic expression of miRNA-155 induces apoptosis in melanoma cells. CH-Mel, DR-Mel, SK-Mel-28 and 397-Mel cells were subjected to a single transfection procedure with 100 nM pre-miRNA-155 or dsRNA-CTRL,as described in Materials and methods. Forty-eight hours after transfection,the cytoplasmic amount of mono- and oligonucleosomes originated from apoptotic DNA degradation was quantified using an ELISA assay. Data areexpressed in terms of Enrichment Factor (EF), calculated as the ratio between the adsorbance value of pre-miRNA-155-transfected cells and that of dsRNA-CTRL-transfected cells, to which the arbitrary value of 1.0 was assigned. Each value represents the mean of at least four independent experiments. Bars, standard error of the mean. **P<0.01 and *P<0.05,according to Student's t-test, comparing the adsorbance values of pre-miRNA-155-transfected cells with those of dsRNA-CTRL-transfected cells.Figure 3. Ectopic expression of miRNA-155 induces a concentration-dependent inhibition of melanoma cell growth. CH-Mel (a), DR-Mel (b), GL-Mel (c) and SK-Mel-28 (d) cells were left untreated or subjected to two sequential transfections with the indicated concentrations of pre-miRNA-155 or dsRNA-CTRL, as describe under Materials and methods. Seventy-two hours after the second transfection, the cells were harvested by trypsinization and cell growth was evaluated in terms of viable cell count. Data are expressed in terms of percentage of growth inhibition of target cells transfected with pre-miRNA-155 or dsRNA-CTRL with respect to untreated cells. Each value represents the mean of at least three independent experiments performed with triplicate samples,with bars indicating standard error of the mean. Percentages were subjected to angular transformation in order to obtain normally distributed data. Thereafter,conventional standard error calculation was performed on converted data. However, the data are expressed in non-transformed percentages, following conversion of transformed data into the original values.。
A_review_of_feature_selection_techniques_in_bioinformatics

A review of feature selection techniques in bioinformaticsAbstractFeature selection techniques have become an apparent need in many bioinformatics applications. In addition to the large pool of techniques that have already been developed in the machine learning and data mining fields, specific applications in bioinformatics have led to a wealth of newly proposed techniques.In this article, we make the interested reader aware of the possibilities of feature selection, providing a basic taxonomy of feature selection techniques, and discussing their use, variety and potential in a number of both common as well as upcoming bioinformatics applications.1 INTRODUCTIONDuring the last decade, the motivation for applying feature selection (FS) techniques in bioinformatics has shifted from being an illustrative example to becoming a real prerequisite for model building. In particular, the high dimensional nature of many modelling tasks in bioinformatics, going from sequence analysis over microarray analysis to spectral analyses and literature mining has given rise to a wealth of feature selection techniques being presented in the field.In this review, we focus on the application of feature selection techniques. In contrast to other dimensionality reduction techniques like those based on projection (e.g. principal component analysis) or compression (e.g. using information theory), feature selection techniques do not alter the original representation of the variables, but merely select a subset of them. Thus, they preserve the original semantics of the variables, hence, offering the advantage of interpretability by a domain expert.While feature selection can be applied to both supervised and unsupervised learning, we focus here on the problem of supervised learning (classification), where the class labels are known beforehand. The interesting topic of feature selection for unsupervised learning (clustering) is a more complex issue, and research into this field is recently getting more attention in several communities (Liu and Yu, 2005; Varshavsky et al., 2006).The main aim of this review is to make practitioners aware of the benefits, and in some cases even the necessity of applying feature selection techniques. Therefore, we provide an overview of the different feature selection techniques for classification: we illustrate them by reviewing the most important application fields in the bioinformatics domain, highlighting the efforts done by the bioinformatics community in developing novel and adapted procedures. Finally, we also point the interested reader to some useful data mining and bioinformatics software packages that can be used for feature selection.Previous SectionNext Section2 FEATURE SELECTION TECHNIQUESAs many pattern recognition techniques were originally not designed to cope with large amounts of irrelevant features, combining them with FS techniques has become a necessity in many applications (Guyon and Elisseeff, 2003; Liu and Motoda, 1998; Liu and Yu, 2005). The objectives of feature selection are manifold, the most important ones being: (a) to avoid overfitting andimprove model performance, i.e. prediction performance in the case of supervised classification and better cluster detection in the case of clustering, (b) to provide faster and more cost-effective models and (c) to gain a deeper insight into the underlying processes that generated the data. However, the advantages of feature selection techniques come at a certain price, as the search for a subset of relevant features introduces an additional layer of complexity in the modelling task. Instead of just optimizing the parameters of the model for the full feature subset, we now need to find the optimal model parameters for the optimal feature subset, as there is no guarantee that the optimal parameters for the full feature set are equally optimal for the optimal feature subset (Daelemans et al., 2003). As a result, the search in the model hypothesis space is augmented by another dimension: the one of finding the optimal subset of relevant features. Feature selection techniques differ from each other in the way they incorporate this search in the added space of feature subsets in the model selection.In the context of classification, feature selection techniques can be organized into three categories, depending on how they combine the feature selection search with the construction of the classification model: filter methods, wrapper methods and embedded methods. Table 1 provides a common taxonomy of feature selection methods, showing for each technique the most prominent advantages and disadvantages, as well as some examples of the most influential techniques.Table 1.A taxonomy of feature selection techniques. For each feature selection type, we highlight a set of characteristics which can guide the choice for a technique suited to the goals and resources of practitioners in the fieldFilter techniques assess the relevance of features by looking only at the intrinsic properties of the data. In most cases a feature relevance score is calculated, and low-scoring features are removed. Afterwards, this subset of features is presented as input to the classification algorithm. Advantages of filter techniques are that they easily scale to very high-dimensional datasets, they are computationally simple and fast, and they are independent of the classification algorithm. As a result, feature selection needs to be performed only once, and then different classifiers can be evaluated.A common disadvantage of filter methods is that they ignore the interaction with the classifier (the search in the feature subset space is separated from the search in the hypothesis space), and that most proposed techniques are univariate. This means that each feature is considered separately, thereby ignoring feature dependencies, which may lead to worse classification performance when compared to other types of feature selection techniques. In order to overcome the problem of ignoring feature dependencies, a number of multivariate filter techniques were introduced, aiming at the incorporation of feature dependencies to some degree.Whereas filter techniques treat the problem of finding a good feature subset independently of the model selection step, wrapper methods embed the model hypothesis search within the feature subset search. In this setup, a search procedure in the space of possible feature subsets is defined, and various subsets of features are generated and evaluated. The evaluation of a specific subset of features is obtained by training and testing a specific classification model, rendering this approach tailored to a specific classification algorithm. To search the space of all feature subsets, a search algorithm is then ‘wrapped’ around the classification model. However, as the space of feature subsets grows exponentially with the number of features, heuristic search methods are used to guide the search for an optimal subset. These search methods can be divided in two classes: deterministic and randomized search algorithms. Advantages of wrapper approaches include the interaction between feature subset search and model selection, and the ability to take into account feature dependencies. A common drawback of these techniques is that they have a higher risk of overfitting than filter techniques and are very computationally intensive, especially if building the classifier has a high computational cost.In a third class of feature selection techniques, termed embedded techniques, the search for an optimal subset of features is built into the classifier construction, and can be seen as a search in the combined space of feature subsets and hypotheses. Just like wrapper approaches, embedded approaches are thus specific to a given learning algorithm. Embedded methods have the advantage that they include the interaction with the classification model, while at the same time being far less computationally intensive than wrapper methods.Previous SectionNext Section3 APPLICATIONS IN BIOINFORMATICS3.1 Feature selection for sequence analysisSequence analysis has a long-standing tradition in bioinformatics. In the context of feature selection, two types of problems can be distinguished: content and signal analysis. Content analysis focuses on the broad characteristics of a sequence, such as tendency to code for proteins or fulfillment of a certain biological function. Signal analysis on the other hand focuses on the identification of important motifs in the sequence, such as gene structural elements or regulatory elements.Apart from the basic features that just represent the nucleotide or amino acid at each position in a sequence, many other features, such as higher order combinations of these building blocks (e.g.k-mer patterns) can be derived, their number growing exponentially with the pattern length k. As many of them will be irrelevant or redundant, feature selection techniques are then applied to focus on the subset of relevant variables.3.1.1 Content analysisThe prediction of subsequences that code for proteins (coding potential prediction) has been a focus of interest since the early days of bioinformatics. Because many features can be extracted from a sequence, and most dependencies occur between adjacent positions, many variations of Markov models were developed. To deal with the high amount of possible features, and the often limited amount of samples, (Salzberg et al., 1998) introduced the interpolated Markov model (IMM), which used interpolation between different orders of the Markov model to deal with small sample sizes, and a filter method (χ2) to select only relevant features. In further work, (Delcher et al., 1999) extended the IMM framework to also deal with non-adjacent feature dependencies, resulting in the interpolated context model (ICM), which crosses a Bayesian decision tree with a filter method (χ2) to assess feature relevance. Recently, the avenue of FS techniques for coding potential prediction was further pursued by (Saeys et al., 2007), who combined different measures of coding potential prediction, and then used the Markov blanket multivariate filter approach (MBF) to retain only the relevant ones.A second class of techniques focuses on the prediction of protein function from sequence. The early work of Chuzhanova et al. (1998), who combined a genetic algorithm in combination with the Gamma test to score feature subsets for classification of large subunits of rRNA, inspired researchers to use FS techniques to focus on important subsets of amino acids that relate to the protein's; functional class (Al-Shahib et al., 2005). An interesting technique is described in Zavaljevsky et al. (2002), using selective kernel scaling for support vector machines (SVM) as a way to asses feature weights, and subsequently remove features with low weights.The use of FS techniques in the domain of sequence analysis is also emerging in a number of more recent applications, such as the recognition of promoter regions (Conilione and Wang, 2005), and the prediction of microRNA targets (Kim et al., 2006).3.1.2 Signal analysisMany sequence analysis methodologies involve the recognition of short, more or less conserved signals in the sequence, representing mainly binding sites for various proteins or protein complexes. A common approach to find regulatory motifs, is to relate motifs to gene expressionlevels using a regression approach. Feature selection can then be used to search for the motifs that maximize the fit to the regression model (Keles et al., 2002; Tadesse et al.,2004). In Sinha (2003), a classification approach is chosen to find discriminative motifs. The method is inspired by Ben-Dor et al. (2000) who use the threshold number of misclassification (TNoM, see further in the section on microarray analysis) to score genes for relevance to tissue classification. From the TNoM score, a P-value is calculated that represents the significance of each motif. Motifs are then sorted according to their P-value.Another line of research is performed in the context of the gene prediction setting, where structural elements such as the translation initiation site (TIS) and splice sites are modelled as specific classification problems. The problem of feature selection for structural element recognition was pioneered in Degroeve et al. (2002) for the problem of splice site prediction, combining a sequential backward method together with an embedded SVM evaluation criterion to assess feature relevance. In Saeys et al. (2004), an estimation of distribution algorithm (EDA, a generalization of genetic algorithms) was used to gain more insight in the relevant features for splice site prediction. Similarly, the prediction of TIS is a suitable problem to apply feature selection techniques. In Liu et al. (2004), the authors demonstrate the advantages of using feature selection for this problem, using the feature-class entropy as a filter measure to remove irrelevant features.In future research, FS techniques can be expected to be useful for a number of challenging prediction tasks, such as identifying relevant features related to alternative splice sites and alternative TIS.3.2 Feature selection for microarray analysisDuring the last decade, the advent of microarray datasets stimulated a new line of research in bioinformatics. Microarray data pose a great challenge for computational techniques, because of their large dimensionality (up to several tens of thousands of genes) and their small sample sizes (Somorjai et al., 2003). Furthermore, additional experimental complications like noise and variability render the analysis of microarray data an exciting domain.In order to deal with these particular characteristics of microarray data, the obvious need for dimension reduction techniques was realized (Alon et al., 1999; Ben-Dor et al., 2000; Golub et al., 1999; Ross et al., 2000), and soon their application became a de facto standard in the field. Whereas in 2001, the field of microarray analysis was still claimed to be in its infancy (Efron et al., 2001), a considerable and valuable effort has since been done to contribute new and adapt known FS methodologies (Jafari and Azuaje, 2006). A general overview of the most influential techniques, organized according to the general FS taxonomy of Section 2, is shown in Table 2.Table 2.Key references for each type of feature selection technique in the microarray domain3.2.1 The univariate filter paradigm: simple yet efficientBecause of the high dimensionality of most microarray analyses, fast and efficient FS techniques such as univariate filter methods have attracted most attention. The prevalence of these univariate techniques has dominated the field, and up to now comparative evaluations of different classification and FS techniques over DNA microarray datasets only focused on the univariate case (Dudoit et al., 2002; Lee et al., 2005; Li et al., 2004; Statnikov et al., 2005). This domination of the univariate approach can be explained by a number of reasons:the output provided by univariate feature rankings is intuitive and easy to understand;the gene ranking output could fulfill the objectives and expectations that bio-domain experts have when wanting to subsequently validate the result by laboratory techniques or in order to explore literature searches. The experts could not feel the need for selection techniques that take into account gene interactions;the possible unawareness of subgroups of gene expression domain experts about the existence of data analysis techniques to select genes in a multivariate way;the extra computation time needed by multivariate gene selection techniques.Some of the simplest heuristics for the identification of differentially expressed genes include setting a threshold on the observed fold-change differences in gene expression between the states under study, and the detection of the threshold point in each gene that minimizes the number of training sample misclassification (threshold number of misclassification, TNoM (Ben-Dor etal.,2000)). However, a wide range of new or adapted univariate feature ranking techniques has since then been developed. These techniques can be divided into two classes: parametric and model-free methods (see Table 2).Parametric methods assume a given distribution from which the samples (observations) have been generated. The two sample t-test and ANOVA are among the most widely used techniques in microarray studies, although the usage of their basic form, possibly without justification of their main assumptions, is not advisable (Jafari and Azuaje, 2006). Modifications of the standard t-test to better deal with the small sample size and inherent noise of gene expression datasets include a number of t- or t-test like statistics (differing primarily in the way the variance is estimated) and a number of Bayesian frameworks (Baldi and Long, 2001; Fox and Dimmic, 2006). Although Gaussian assumptions have dominated the field, other types of parametrical approaches can also be found in the literature, such as regression modelling approaches (Thomas et al., 2001) and Gamma distribution models (Newton et al.,2001).Due to the uncertainty about the true underlying distribution of many gene expression scenarios, and the difficulties to validate distributional assumptions because of small sample sizes,non-parametric or model-free methods have been widely proposed as an attractive alternative to make less stringent distributional assumptions (Troyanskaya et al., 2002). Many model-free metrics, frequently borrowed from the statistics field, have demonstrated their usefulness in many gene expression studies, including the Wilcoxon rank-sum test (Thomas et al., 2001), the between-within classes sum of squares (BSS/WSS) (Dudoit et al., 2002) and the rank products method (Breitling et al., 2004).A specific class of model-free methods estimates the reference distribution of the statistic using random permutations of the data, allowing the computation of a model-free version of the associated parametric tests. These techniques have emerged as a solid alternative to deal with the specificities of DNA microarray data, and do not depend on strong parametric assumptions (Efron et al., 2001; Pan, 2003; Park et al., 2001; Tusher et al., 2001). Their permutation principle partly alleviates the problem of small sample sizes in microarray studies, enhancing the robustness against outliers.We also mention promising types of non-parametric metrics which, instead of trying to identify differentially expressed genes at the whole population level (e.g. comparison of sample means), are able to capture genes which are significantly disregulated in only a subset of samples (Lyons-Weiler et al., 2004; Pavlidis and Poirazi, 2006). These types of methods offer a more patient specific approach for the identification of markers, and can select genes exhibiting complex patterns that are missed by metrics that work under the classical comparison of two prelabelled phenotypic groups. In addition, we also point out the importance of procedures for controlling the different types of errors that arise in this complex multiple testing scenario of thousands of genes (Dudoit et al., 2003; Ploner et al., 2006; Pounds and Cheng, 2004; Storey, 2002), with a special focus on contributions for controlling the false discovery rate (FDR).3.2.2 Towards more advanced models: the multivariate paradigm for filter, wrapperand embedded techniquesUnivariate selection methods have certain restrictions and may lead to less accurate classifiers by, e.g. not taking into account gene–gene interactions. Thus, researchers have proposed techniques that try to capture these correlations between genes.The application of multivariate filter methods ranges from simple bivariate interactions (Bø and Jonassen, 2002) towards more advanced solutions exploring higher order interactions, such as correlation-based feature selection (CFS) (Wang et al., 2005; Yeoh et al., 2002) and several variants of the Markov blanket filter method (Gevaert et al., 2006; Mamitsuka, 2006; Xing et al., 2001). The Minimum Redundancy-Maximum Relevance (MRMR) (Ding and Peng, 2003) and Uncorrelated Shrunken Centroid (USC) (Yeung and Bumgarner, 2003) algorithms are two other solid multivariate filter procedures, highlighting the advantage of using multivariate methods over univariate procedures in the gene expression domain.Feature selection using wrapper or embedded methods offers an alternative way to perform a multivariate gene subset selection, incorporating the classifier's; bias into the search and thus offering an opportunity to construct more accurate classifiers. In the context of microarray analysis, most wrapper methods use population-based, randomized search heuristics (Blanco et al., 2004; Jirapech-Umpai and Aitken, 2005; Li et al., 2001; Ooi and Tan, 2003), although also a few examples use sequential search techniques (Inza et al., 2004; Xiong et al., 2001). An interesting hybrid filter-wrapper approach is introduced in (Ruiz et al., 2006), crossing a univariatelypre-ordered gene ranking with an incrementally augmenting wrapper method.Another characteristic of any wrapper procedure concerns the scoring function used to evaluate each gene subset found. As the 0–1 accuracy measure allows for comparison with previous works, the vast majority of papers uses this measure. However, recent proposals advocate the use of methods for the approximation of the area under the ROC curve (Ma and Huang, 2005), or the optimization of the LASSO (Least Absolute Shrinkage and Selection Operator) model (Ghosh and Chinnaiyan, 2005). ROC curves certainly provide an interesting evaluation measure, especially suited to the demand for screening different types of errors in many biomedical scenarios.The embedded capacity of several classifiers to discard input features and thus propose a subset of discriminative genes, has been exploited by several authors. Examples include the use of random forests (a classifier that combines many single decision trees) in an embedded way to calculate the importance of each gene (Díaz-Uriarte and Alvarez de Andrés, 2006; Jiang et al., 2004). Another line of embedded FS techniques uses the weights of each feature in linear classifiers, such as SVMs (Guyon et al., 2002) and logistic regression (Ma and Huang, 2005). These weights are used to reflect the relevance of each gene in a multivariate way, and thus allow for the removal of genes with very small weights.Partially due to the higher computational complexity of wrapper and to a lesser degree embedded approaches, these techniques have not received as much interest as filter proposals. However, an advisable practice is to pre-reduce the search space using a univariate filter method, and only then apply wrapper or embedded methods, hence fitting the computation time to the available resources.3.3 Mass spectra analysisMass spectrometry technology (MS) is emerging as a new and attractive framework for disease diagnosis and protein-based biomarker profiling (Petricoin and Liotta, 2003). A mass spectrum sample is characterized by thousands of different mass/charge (m / z) ratios on the x-axis, each with their corresponding signal intensity value on the y-axis. A typical MALDI-TOF low-resolution proteomic profile can contain up to 15 500 data points in the spectrum between 500 and 20 000 m / z, and the number of points even grows using higher resolution instruments.For data mining and bioinformatics purposes, it can initially be assumed that each m / z ratio represents a distinct variable whose value is the intensity. As Somorjai et al. (2003) explain, the data analysis step is severely constrained by both high-dimensional input spaces and their inherent sparseness, just as it is the case with gene expression datasets. Although the amount of publications on mass spectrometry based data mining is not comparable to the level of maturity reached in the microarray analysis domain, an interesting collection of methods has been presented in the last 4–5 years (see Hilario et al., 2006; Shin and Markey, 2006 for recent reviews) since the pioneering work of Petricoin et al.(2002).Starting from the raw data, and after an initial step to reduce noise and normalize the spectra from different samples (Coombes et al., 2007), the following crucial step is to extract the variables that will constitute the initial pool of candidate discriminative features. Some studies employ the simplest approach of considering every measured value as a predictive feature, thus applying FS techniques over initial huge pools of about 15 000 variables (Li et al., 2004; Petricoin et al., 2002), up to around 100 000 variables (Ball et al.,2002). On the other hand, a great deal of the current studies performs aggressive feature extraction procedures using elaborated peak detection and alignment techniques (see Coombes et al., 2007; Hilario et al., 2006; Shin and Markey, 2006 for a detailed description of these techniques). These procedures tend to seed the dimensionality from which supervised FS techniques will start their work in less than 500 variables (Bhanot et al., 2006; Ressom et al., 2007; Tibshirani et al., 2004). A feature extraction step is thus advisable to set the computational costs of many FS techniques to a feasible size in these MS scenarios. Table 3 presents an overview of FS techniques used in the domain of mass spectrometry. Similar to the domain of microarray analysis, univariate filter techniques seem to be the most common techniques used, although the use of embedded techniques is certainly emerging as an alternative. Although the t-test maintains a high level of popularity (Liu et al., 2002; Wu et al., 2003), other parametric measures such as F-test (Bhanot et al., 2006), and a notable variety of non-parametric scores (Tibshirani et al., 2004; Yu et al., 2005) have also been used in several MS studies. Multivariate filter techniques on the other hand, are still somewhat underrepresented (Liu et al., 2002; Prados et al., 2004).Table 3.Key references for each type of feature selection technique in the domain of mass pectrometryWrapper approaches have demonstrated their usefulness in MS studies by a group of influential works. Different types of population-based randomized heuristics are used as search engines in the major part of these papers: genetic algorithms (Li et al., 2004; Petricoin et al., 2002), particle swarm optimization (Ressom et al., 2005) and ant colony procedures (Ressom et al., 2007). It is worth noting that while the first two references start the search procedure in ∼ 15 000 dimensions by considering each m / z ratio as an initial predictive feature, aggressive peak detection and alignment processes reduce the initial dimension to about 300 variables in the last two references (Ressom et al., 2005; Ressom et al., 2007).An increasing number of papers uses the embedded capacity of several classifiers to discard input features. Variations of the popular method originally proposed for gene expression domains by Guyon et al. (2002), using the weights of the variables in the SVM-formulation to discard features with small weights, have been broadly and successfully applied in the MS domain (Jong et al., 2004; Prados et al., 2004; Zhang et al., 2006). Based on a similar framework, the weights of the input masses in a neural network classifier have been used to rank the features'importance in Ball et al. (2002). The embedded capacity of random forests (Wu et al., 2003) and other types of decision tree-based algorithms (Geurts et al., 2005) constitutes an alternative embedded FS strategy.Previous SectionNext Section4 DEALING WITH SMALL SAMPLE DOMAINSSmall sample sizes, and their inherent risk of imprecision and overfitting, pose a great challenge for many modelling problems in bioinformatics (Braga-Neto and Dougherty, 2004; Molinaro et al., 2005; Sima and Dougherty, 2006). In the context of feature selection, two initiatives have emerged in response to this novel experimental situation: the use of adequate evaluation criteria, and the use of stable and robust feature selection models.4.1 Adequate evaluation criteria。
statement of research interest

Statement of Research InterestsXinghua LuMy research interests concentrate on applying statistical data mining and machine learning techniques to system biology. I am especially interested in developing and applying statistical learning algorithms to identify patterns from large amounts of high dimensional data that reflect the states of the signal transduction system. As a pharmacologist, I am always intrigued by cellular signal transduction pathways and complexity of the system. Before my transition to the computational biology field two years ago, my research as a pharmacologist had mainly concentrated on individual pathways or protein molecules. It often occurred to me that the biomedical research of the last few decades had accumulated a wealth of knowledge at the molecular level, and it is time for one to take a step back and view the cellular signal transduction system as a full-fledged forest with most of the leaves painted colorfully. Advance in biological techniques, such as DNA microarray and high through-put screening, has produced large amounts of data regarding many aspects of cell. These data offer biologists opportunities to study the cellular system, but also pose challenges for conventional biologists. The transition from an experimental to computational biologist was quite natural for me because of my long-lasting interest and experience in scientific computing. Winning the National Library of Medicine training grant award provided me a great opportunity to extend my research ability in this direction. My study and research benefited greatly from the exceptionally excellent artificial intelligence and statistics community in Pittsburgh area.My current research in computational biology falls in two major areas, which are described below: The first is to develop a latent variable generative model, variational Bayesian cooperative vector quatizer (VBCVQ) model, to analyze the DNA microarray data and model the gene transcription regulation pathways. I have finished mathematical derivation and implementation of the model. In addition to its potential biological application, the model can be used in a wide range of applications, e.g. image processing, image compression and content-based image retrieval. The model closely simulates the gene expression regulation system. It can overcome some drawbacks of the commonly used existing techniques and address questions other models fail to address. Generally, the model has following advantages: (1) Data dimension reduction. (2) Identification of the key components of gene expression regulation pathways. (3) Capability of inferring the state of key components when given new microarray data. Such information can be useful for further exploring the mechanism of disease, drug effect or toxicity and the construction of diagnosis tools. Full Bayesian learning of the model allows us to address questions like ``what is the most efficient way to encode the information controlling gene transcription?'' or ``what are the key signal transduction components that control gene expression in a given kind of cell?'' Currently, I am testing the model with image encoding and mixed image separation. Once this stage finished, I will apply the model in microarray analysis.The second area I am working on is to identify and predict the function of a protein motif using data mining approaches. The Gene Ontology is a set of annotations that describe the biological system in a hierarchical fashion. The current Gene Ontology database can also serve as aknowledge base to facilitate biological discovery because it contains a large amount of information regarding the molecular function, biological process and cellular location of proteins. To make effective use of such a knowledge base, a biologist would like to query the knowledge base in the following fashion: ``what is the protein motif that encodes a given molecular function?'' or ``what is the potential function of a conserved motif we identified?'' However, the current Gene Ontology database can not answer such queries due to the way of information being stored and the potential ambiguity caused by a conventional database query, even though the information is actually available. Working with collaborators at the University of Pittsburgh and Carnegie Mellon University, I have developed a general method to address the issue using data mining approaches. We have extracted a set of features that help to disambiguate the association of protein motifs and the Gene Ontology terms. Then, we trained a statistical classifier to determine whether a Gene Ontology term should be assigned to a protein motif, using probability to reflect the confidence or uncertainty. The method performs well when tested on known protein motifs from PROSITE. I will further extend the work in two directions: (1) To develop a system based on the method and make it available to the scientific community for data mining. (2) To study the evolution of protein sequence motifs by further exploiting the knowledge in Gene Ontology with hierarchical aspect models. These studies will help identify the key residues among the motifs, and allow us to address the questions like ``what amino acid plays the key role in proteins that act as kinase orreductase/oxidase?''Overall, my training in both experimental and computational biology enables me to combine the knowledge of both fields without any communication gap. I foresee that my research will follow both directions of computational method development and biological discovery. As a computational biologist, I will extensively collaborate with both experimental biologists and computer scientists to solve interesting biological problems. My short term goal is to further extend my current research as described above. In the long run, I will continue to learn, identify, develop and apply computational methods in the fields of drug discovery, drug toxicity prediction and developing diagnostic tools based on biological data.。
芯片数据分析
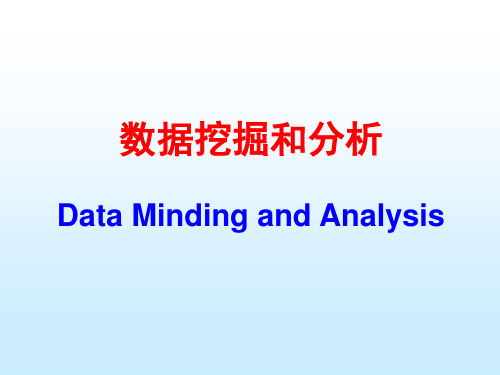
单因素多组数据统计分析
目的:只考虑一种影响因素,筛选两组以上样品 之间的差异基因。 要求:一个影响因素下的多组数据,每组数据3 个以上生物学重复。 Cy3通道信号和Cy5通道信号在常规实验设计中 不能独立分开作为两组单通道信号值进行数据分 析。
多因素数据统计分析
目的:根据一个以上不同的条件综合评判,筛选 多个条件对于两组样品造成的差异基因。
1. 图像分析
芯片分析的第一步是将芯片扫描得到的杂交信号 转化为原始的代表信号强度的数据 激光扫描仪(Scaner)得到的Cy3/Cy5图像文件 通过划格(Griding),确定杂交点范围,过滤背 景噪音,提取得到基因表达的荧光信号强度值, 最后以列表形式输出
目前可用于这一步分析工作的软件有Quantarray、 Genpix、ChipReader和ScanAlyze等
要求:多个影响因素下的2组数据,每组数据3个 以上生物学重复。
Cy3通道信号和Cy5通道信号在常规实验设计中 不能独立分开作为两组单通道信号值进行数据分 析。
SAM 分析
目的:SAM(Significant Analysis of Microarray) 分析方法是在多组实验中寻找具有差异表达的基 因。 要求:每组3个以上生物学重复。 Cy3通道信号和Cy5通道信号在常规实验设计中 不能独立分开作为两组单通道信号值进行数据分 析。
由于样本差异、荧光标记效率和检出率的不平衡, 需对Cy3和Cy5的原始提取信号进行均衡和修正 才能进一步分析实验数据。 Normalization正是基于此种目的。
Normalization的方法有很多种,包括中值法、总 体信号强度法以及指定使用芯片上的某些点来对 数据做标准化
Data Mining Techniques
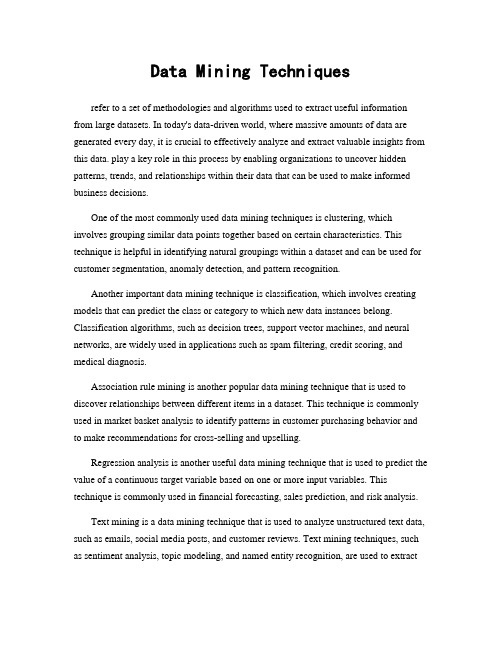
Data Mining Techniquesrefer to a set of methodologies and algorithms used to extract useful information from large datasets. In today's data-driven world, where massive amounts of data are generated every day, it is crucial to effectively analyze and extract valuable insights from this data. play a key role in this process by enabling organizations to uncover hidden patterns, trends, and relationships within their data that can be used to make informed business decisions.One of the most commonly used data mining techniques is clustering, which involves grouping similar data points together based on certain characteristics. This technique is helpful in identifying natural groupings within a dataset and can be used for customer segmentation, anomaly detection, and pattern recognition.Another important data mining technique is classification, which involves creating models that can predict the class or category to which new data instances belong. Classification algorithms, such as decision trees, support vector machines, and neural networks, are widely used in applications such as spam filtering, credit scoring, and medical diagnosis.Association rule mining is another popular data mining technique that is used to discover relationships between different items in a dataset. This technique is commonly used in market basket analysis to identify patterns in customer purchasing behavior and to make recommendations for cross-selling and upselling.Regression analysis is another useful data mining technique that is used to predict the value of a continuous target variable based on one or more input variables. This technique is commonly used in financial forecasting, sales prediction, and risk analysis.Text mining is a data mining technique that is used to analyze unstructured text data, such as emails, social media posts, and customer reviews. Text mining techniques, such as sentiment analysis, topic modeling, and named entity recognition, are used to extractuseful information from text data to understand customer sentiments, identify key topics, and extract important entities.Other data mining techniques include anomaly detection, feature selection, and dimensionality reduction, which are used to identify outliers in data, select the most relevant features for analysis, and reduce the complexity of high-dimensional data, respectively.In conclusion, data mining techniques are powerful tools that can help organizations gain valuable insights from their data and make informed business decisions. By using a combination of clustering, classification, association rule mining, regression analysis, text mining, and other techniques, organizations can unlock the full potential of their data and drive business growth.。
EBI简介
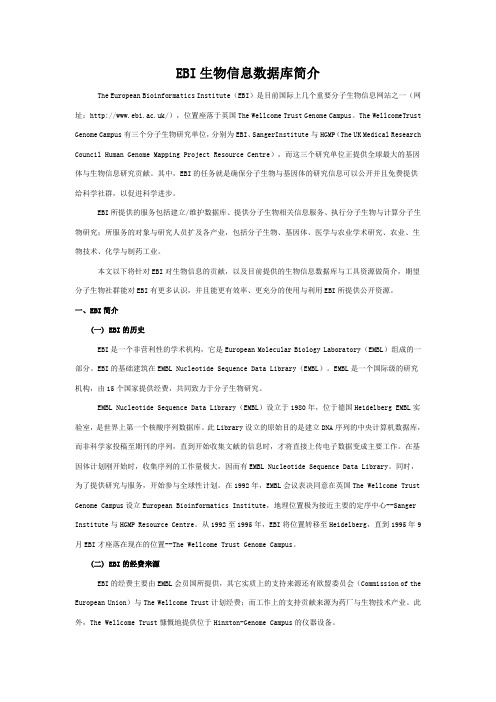
EBI生物信息数据库简介The European Bioinformatics Institute(EBI)是目前国际上几个重要分子生物信息网站之一(网址:/),位置座落于英国The Wellcome Trust Genome Campus。
The WellcomeTrust Genome Campus有三个分子生物研究单位,分别为EBI、SangerInstitute与HGMP(The UK Medical Research Council Human Genome Mapping Project Resource Centre),而这三个研究单位正提供全球最大的基因体与生物信息研究贡献。
其中,EBI的任务就是确保分子生物与基因体的研究信息可以公开并且免费提供给科学社群,以促进科学进步。
EBI所提供的服务包括建立/维护数据库、提供分子生物相关信息服务、执行分子生物与计算分子生物研究;所服务的对象与研究人员扩及各产业,包括分子生物、基因体、医学与农业学术研究、农业、生物技术、化学与制药工业。
本文以下将针对EBI对生物信息的贡献,以及目前提供的生物信息数据库与工具资源做简介,期望分子生物社群能对EBI有更多认识,并且能更有效率、更充分的使用与利用EBI所提供公开资源。
一、EBI简介(一) EBI的历史EBI是一个非营利性的学术机构,它是European Molecular Biology Laboratory(EMBL)组成的一部分。
EBI的基础建筑在EMBL Nucleotide Sequence Data Library(EMBL)。
EMBL是一个国际级的研究机构,由15个国家提供经费,共同致力于分子生物研究。
EMBL Nucleotide Sequence Data Library(EMBL)设立于1980年,位于德国Heidelberg EMBL实验室,是世界上第一个核酸序列数据库。
此Library设立的原始目的是建立DNA序列的中央计算机数据库,而非科学家投稿至期刊的序列,直到开始收集文献的信息时,才将直接上传电子数据变成主要工作。
数据挖掘第一章

CS512 Coverage (Chapters 11, 12, 13 + More Advanced Topics)
Cluster Analysis: Advanced Methods (Chapter 11) Outlier Analysis (Chapter 12) Mining data streams, time-series, and sequence data Mining graph data Mining social and information networks Mining object, spatial, multimedia, text and Web data Mining complex data objects Spatial and spatiotemporal data mining Multimedia data mining Text and Web mining Additional (often current) themes if time permits
Database Systems:
Text information systems
Bioinformatics
Yahoo!-DAIS seminar (CS591DAIS—Fall and Spring. 1 credit unit)
2
CS412 Coverage (Chapters 1-10, 3rd Ed.)
Summary
7
Why Data Mining?
Tfrom terabytes to petabytes
Research on the big data feature mining technology based on the cloud computing

2019 No.3Research on the big data feature mining technologybased on the cloud computingWANG YunSichuan Vocational and Technical College, Suining, Sichuan, 629000Abstract: The cloud computing platform has the functions of efficiently allocating the dynamic resources, generating the dynamic computing and storage according to the user requests, and providing the good platform for the big data feature analysis and mining. The big data feature mining in the cloud computing environment is an effective method for the elficient application of the massive data in the information age. In the process of t he big data mining, the method of the big data feature mining based on the gradient sampling has the poor logicality. It only mines the big data features from a single-level perspective, which reduces the precision of t he big data feature mining.Keywords: Cloud computing; big data features; mining technology; model methodWith the development of the times, people need more and more valuable data. Therefore, a new technology is needed to process a large amount of the data and extract the information we need. The data mining technology is a wide-ranging subject, which integrates the statistical methods and surpasses the traditional statistical analysis. The data mining is the process of extracting the useful data we need from the massive data by using the technical means. Experiments show that this method has the high data mining performances, and can provide an effective means for the big data feature mining in all sectors of the social production.1. Feature mining method for the big data feature miningmodel1-1. The big data feature mining model in the cloud computing environmentThis paper uses the big data feature mining model in the cloud computing environment to realize the big data feature mining. The model mainly includes the big data storage system layer, the big data mining processing layer and the user layer. The following is the detailed study.1-2. The big data storage system layerThe interaction of the multi-source data information and the integration of the network technology in the cloud computing depends on the three different models in the cloud computing environment: I/O, USB and the disk layer, and the architecture of the big data storage system layer in the computing environment. It can be seen that the big data storage system in the cloud computing environment includes the multi-source information resource service layer, the core technology layer, the multi-source information resource platform service layer and the multi-source information resource basic layer.1-3. The big data feature mining and processing layerIn order to solve the problem of the low classification accuracy and the long time-consuming in the process of the big data feature mining, a new and efficient method of the big data feature classification mining based on the cloud computing is proposed in this paper. The first step is to decompose the big data training set by the map, and then generate the big data training set. The second step is to acquire the frequent item-sets. The third step is to implement the merging according to reduce, and the association rules can be acquired through the frequent item-sets, and then pruning to acquire the classification rules. Based on the classification rules, a classifier of the big data features is constructed to realize the effective classification and the mining of the big data features.1 -4. Client layerThe user input module in the client layer provides a platform for the users to express their requests. The module analyses the data information input by the users and matches the reasonable data mining methods. This method is used to mine the data features of the pre-processed data. Users of the result-based displaying module can obtain the corresponding results of the big data feature mining, and realize the big data feature mining in the cloud computing environment.2. Parallel distributed big data mining2-1. Platform system architectureHadoop provides a platform for the programmers to easily develop and run the massive data applications. Its distributed file system HDFS is a file system that can reliably store the big data sets on a large cluster. It has the characteristics of reliability and the strong fault tolerance. Map Reduce provides a programming mode for the efficient parallel programming. Based on this, we developed a parallel data mining platform, PD Miner, which stores the large-scale data on HDFS, and implements various parallel data preprocessing and data mining algorithms through Map Reduce.2-2. Workflow subsystemThe workflow subsystem provides a friendly and unified user interface (UI), which enables the users to easily establish the data mining tasks. In the process of creating the mining tasks, the ETL data preprocessing algorithm, the classification algorithm, the clustering algorithm, and the association rule algorithm can be selected. The right drop-down box can select the specific algorithm of the service unit. The workflow subsystem provides the services for the users through the graphical UI interface, and flexibly establishes the self-customized mining tasks that conform to the business application workflow. Through the workflow interface, the multiple workflow tasks can be established, not only within each mining task, but also among different data mining tasks.2-3. User interface subsystemThe user interface subsystem consists of two modules: the user input module and the result display module. The user interface subsystem is responsible for the interaction with the users, reading and writing the parameter settings, accepting the user operation52International English Education Researchrequests, and displaying the results according to the interface. For example, the parameter setting interface of the parallel Naive Bayesian algorithm in the parallel classification algorithm can easily set the parameters of the algorithm. These parameters include the training data, the test data, the output results and the storage path of the model files, and also include the setting of the number of Map and Reduce tasks. The result display part realizes the visual understanding of the results, such as generating the histograms and the pie charts and so on.2- 4. Parallel ETL algorithm subsystemThe data preprocessing algorithm plays a very important role in the data mining, and its output is usually the input of the data mining algorithm. Due to the dramatic increase of the data volume, the serial data preprocessing process needs a lot of time to complete the operation process. In order to improve the efficiency of the preprocessing algorithm, 19 preprocessing algorithms are designed and developed in the parallel ETL algorithm subsystem, including the parallel sampling (Sampling), the parallel data preview (PD Preview), the parallel data add label (PD Add Label), the parallel discretization (Discreet), the parallel addition of sample (ID), and the parallel attribute exchange (Attribute Exchange).3. Analysis of the big data feature mining technology basedon the cloud computingThe emergence of the cloud computing provides a new direction for the development of the data mining technology. The data mining technology based on the cloud computing can develop the new patterns. As far as the specific implementation is concerned, the development of the several key technologies is crucial.3- 1. Cloud computing technologyThe distributed computing is the key technology of the cloud computing platform. It is one of the effective means to deal with the massive data mining tasks and improve the data mining efficiency. The distributed computing includes the distributed storage and the parallel computing. The distributed storage effectively solves the storage problem of the massive data, and realizes the key functions of the data storage, such as the high fault tolerance, the high security and the high performance. At present, the distributed file system theory proposed by Google is the basis of the popular distributed file system in the industry. Google File System (GFS) is developed to solve the storage, search and analysis of its massive data. The distributed parallel computing framework is the key to efficiently accomplish the data mining and the computing tasks. At present, some popular distributed parallel computing frameworks encapsulate some technical details of the distributed computing, so that users only need to consider the logical relationship between the tasks without paying too much attention to these technical details, which not only greatly improves the efficiency of the research and development, but also effectively reduces the costs of the system maintenance. The typical distributed parallel computing frameworks such as Map Reduce parallel computing framework proposed by Google and the Pregel iterative processing computing framework and so on.3-2. Data aggregation scheduling technologyThe data aggregation and scheduling technology needs toachieve the aggregation and scheduling of different types of thedata accessing cloud computing platform. The data aggregationand scheduling needs to support different formats of the source data, but also provides a variety of the data synchronization methods. To solve the problem of the protocol of different data isthe task of the data aggregation and scheduling technology. The technical solutions need to consider the support of the data formats generated by different systems on the network, such as the on-line transaction processing system (OLTP) data, the on-line analysis processing system (OLAP) data, various log data, and the crawlerdata and so on. Only in this way can the data mining and analysisbe realized.3-3. Service scheduling and service management technologyIn order to enable different business systems to use this computing platform, the platform must provide the service scheduling and the service management functions. The service scheduling is based on the priority of the services and the matchingof the services and the resources, to solve the parallel exclusionand isolation of the services, to ensure that the cloud services of thedata mining platform are safe and reliable, and to schedule and control according to the service management. The service management realizes the functions of the unified service registration and the service exposure. It not only supports the exposure of the local service capabilities, but also supports the access of the third-party data mining capabilities, and extends the service capabilities of the data mining platform.3- 4. Parallelization technology of the mining algorithmsThe parallelization of the mining algorithms is one of the key technologies for effectively utilizing the basic capabilities providedby the cloud computing platform, which involves whether the algorithms can be parallel or not, and the selection of the parallel strategies. The data mining algorithms mainly include the decisiontree algorithm, the association rule algorithm and the K-means algorithm. The parallelization of the algorithm is the key technology of the data mining using the cloud computing platform.4. Data mining technology based on the cloud computing4- 1. Data mining research method based on the cloud computingOne is the data association mining. The relevant data miningcan centralize the divergent network data information when analyzing the details and extracting the values of the massive data information. The relevant data mining is usually divided into three steps. First, determine the scope of the data to be mined and collectthe data objects to be processed, so that the attributes of the relevance research can be clearly defined. Secondly, large amountsof the data are pre-processed to ensure the authenticity and integrity of the mining data, and the results of the pre-processingwill be stored in the mining database. Thirdly, implement the data mining of the shaping training. The entity threshold is analyzed bythe permutation and combination.The second is the data fuzziness learning method. Its principleis to assume that there are a certain number of the information samples under the cloud computing platform, then describe any information sample, calculate the standard deviation of all the information samples, and finally realize the data mining value532019 No.3information operation and the high compression. Faced with the massive data mining, the key of applying the data fuzziness learning method is to screen and determine the fuzzy membership function, and finally realize the actual operation of the fuzzification of the value information of the massive data mining based on the cloud computing. But here we need to pay attention to the need to activate the conditions in order to achieve the network data node information collection.The third is the data mining Apriori algorithm. The Apriori algorithm is an algorithm for mining the association rules. It is a basic algorithm designed by Agrawal, et al. It is based on the idea of the two-stage mining and is implemented by scanning the transaction databases many times. Unlike other algorithms, the Apriori algorithm can effectively avoid the problem that the convergence of the data mining algorithm is poor due to the redundancy and complexity of the massive data. On the premise of saving the investment cost as much as possible, using the computer simulation will greatly improve the speed of mining the massive data.4-2. Data mining architecture based on the cloud computingThe data mining based on the cloud computing relies on the massive storage capacity of the cloud computing and the parallel processing ability of the massive data information, so as to solve the problem that the traditional data mining faces in dealing with the massive data information. Figure 1shows the architecture of the data mining based on the cloud computing. The data mining architecture based on the cloud computing is mainly divided into three layers. The first layer is the cloud computing service layer, which provides the storage and parallel processing services for the massive data information. The second layer is the data mining processing layer, which includes the data preprocessing and the data mining algorithm parallelization. Through the data information preprocessing, it can effectively improve the quality of the data mined, and make the entire mining process easier and more effective. The third layer is the user-oriented layer, which mainly receives the data mining requests from the users and passes the requests to the second and the first layers, and displays the final data mining results to the users in the display module.5. ConclusionThe cloud computing technology itself has been in a period of the rapid development, so it will also lead to some deficiencies in the data mining architecture based on the cloud computing. One is the demand for the personalized and diversified services brought about by the cloud computing. The other is that the number of the data mined and processed may continue to increase. In addition, the dynamic data, the noise data and the high-dimensional data also hinder the data mining and processing. The third is how to choose the appropriate algorithm, which is directly related to the final mining results. The fourth is the data mining process. There may be many uncertainties, and how to deal with these uncertainties and minimize the negative impact caused by these uncertainties is also a problem to be considered in the data mining based on the cloud computing.References[1] Kong Jie; Liu Yang. Data Mining Technology Analysis [J], Computer Knowledge and Technology, 2017, (11): 105-106.[2] Wang Xiaoxue; Zhang Jiazhen; Guo He; Wang Hao. Application of the Big Data in the Mining of the Learning Behavior Patterns of College Students [J], Intelligent Computer and Applications, 2017, (12): 122-123.[3] Deng Yijun. Discussion on the Data Mining and the Knowledge Classification in University Libraries [J], Popular Science & Technology, 2018, (09): 142-143.[4] Wang Mao. Application of the Data Mining Technology in the Computer Forensic Analysis System [J], Automation & Instrumentation, 2018, (12): 100-101.[5] Li Guanli. NCRE Achievement Prediction and Analysis Based on the Rapid Miner Data Mining Technology [J], Journal of Nanjing Radio & TV University, 2018, (12): 154-155.54。
- 1、下载文档前请自行甄别文档内容的完整性,平台不提供额外的编辑、内容补充、找答案等附加服务。
- 2、"仅部分预览"的文档,不可在线预览部分如存在完整性等问题,可反馈申请退款(可完整预览的文档不适用该条件!)。
- 3、如文档侵犯您的权益,请联系客服反馈,我们会尽快为您处理(人工客服工作时间:9:00-18:30)。
Data Mining Techniques for Microarray Datasets Lei Liu Jiong Yang Anthony K. H. Tung U. of Illinois, Urbana-Champaign Case Western Reserve U. National U.of Singapore leiliu@ jiong@ atung@.sgDevelopment in microarray technology has result in revolutionary changes in biological research. Using microarrays, the expression level for thousands of genes can be monitored simultaneously, providing biologists with new ways to gain insight into the complex interaction in living organisms. To do so however, biologists must first overcome the challenge involved in analyzing the large and complex datasets that are generated from microarray experiments. Data mining research, which focuses on scalable and effective knowledge discovery from databases, can provide timely solutions for the biologists in these aspects. In this proposed seminar, we aim to provide platform in which various aspects of microarray data analysis will be introduced. In the first part of the seminar, we will discuss in layman term how microarray datasets are generated and used in biological research. We will use example from the real projects that we participate in to illustrate the potential of different technologies. In the second part of the tutorial, we will discuss existing data mining tools and methods used for analyzing the microarray data sets and their biological implications. Finally, we will present a set of open problems and future research directions for microarray data analysis.Our goal is to make this tutorial a practical guide for microarray data analysis while at the same time to highlight some of the interesting research issues that arise in mining microarray datasets. P articipants without any exposure to bioinformatics will be able to learn the basic concepts and techniques of microarray analysis. For those who are familiar with the technologies, we will offer a wide range of analysis tools that can be applied to microarray gene expression analysis. For people that would like to start research in this area, we would present a set of open problems and potential research directions. Previous exposure to some basic knowledge of molecular biology will be helpful, but not required for the tutorial. But we will assume audience’s familiarity with basic data mining concepts.BiographyAs the founding director of the bioinformatics unit, Dr. Lei Liu joined the W. M. Keck Center for Comparative and Functional Genomics in 1999. Prior to coming to the University of Illinois, he worked as a postdoctoral fellow for two years at the Department of Computer Science and Engineering at the University of Connecticut, where he also received a Ph.D. in cell biology. His expertise is in the areas of comparative genomics, biological databases, and data mining. He has been working in the microarray analysis and data mining for more than three years and co-authored several papers in that area. He has been organizing and participating in many workshops on microarray analysis at the University of Illinois. He has participated recently in the international workshop on “Statistical Methods in Microarray Analysis” in Singapore and presented a talk on multiple platform comparison. He collaborates with computer scientists and statisticians on developing new algorithm for microarray data mining.Dr. Jiong Yang currently is a Schroeder Assistant P rofessor of the EECS department at Case Western Reserve University. He received his master and P h.D. degree in Computer Science from UCLA at 1996 and 1999, respectively. He has been working on mining biological data in the past several years. Recently, he has authored and co-authored several publications in various database, data mining, and bioinformatics conferences and journals on the topic of mining microarray data sets. He has worked on frequent pattern discovery, clustering, and classification algorithms on various microarray data sets. He is an instructor of the course “data mining on bioinformatics” which was offered at UIUC. He recently has organized a workshop on Data Mining in Bioinformatics and he is a guest editor fro the TKDE special issue on Mining Biological Data.Dr. Anthony K. H. Tung is currently an Assistant rofessor in the Department of Computer Science, National University of Singapore (NUS). He received both his B.Sc. and M.Sc. in computer sciences from the National University of Singapore in 1997 and 1998 respectively. In 2001, he received the Ph.D. in computer sciences from Simon Fraser University (SFU). His research interests involve various aspects of databases and data mining (KDD) including buffer management, frequent pattern discovery, spatial clustering, outlier detection and classification analysis. Recent interest also includes data mining for microarray data and 3D protein structures, spatial indexing and sequences searches.Proceedings of the 21st International Conference on Data Engineering (ICDE 2005) 1084-4627/05 $20.00 © 2005IEEE。