Distributional clustering of english words
语言学重要概念梳理(中英文对照版)完整版

第一节语言的本质一、语言的普遍特征(Design Features)1.任意性 Arbitratriness:shu 和Tree都能表示“树”这一概念;同样的声音,各国不同的表达方式2.双层结构Duality:语言由声音结构和意义结构组成(the structure ofsounds and meaning)3.多产性productive: 语言可以理解并创造无限数量的新句子,是由双层结构造成的结果(Understand and create unlimited number withsentences)4.移位性 Displacemennt:可以表达许多不在场的东西,如过去的经历、将来可能发生的事情,或者表达根本不存在的东西等5.文化传播性 Cultural Transmission:语言需要后天在特定文化环境中掌握二、语言的功能(Functions of Language)1.传达信息功能 Informative:最主要功能The main function2.人际功能 Interpersonal:人类在社会中建立并维持各自地位的功能establish and maintain their identity3.行事功能 performative:现实应用——判刑、咒语、为船命名等Judge,naming,and curses4.表情功能 Emotive Function:表达强烈情感的语言,如感叹词/句exclamatory expressions5.寒暄功能 Phatic Communion:应酬话phatic language,比如“吃了没?”“天儿真好啊!”等等6.元语言功能 Metalingual Function:用语言来谈论、改变语言本身,如book可以指现实中的书也可以用“book这个词来表达作为语言单位的“书”三、语言学的分支1. 核心语言学 Core linguistic1)语音学 Phonetics:关注语音的产生、传播和接受过程,着重考察人类语言中的单音。
推理正确率分布曲线 英文
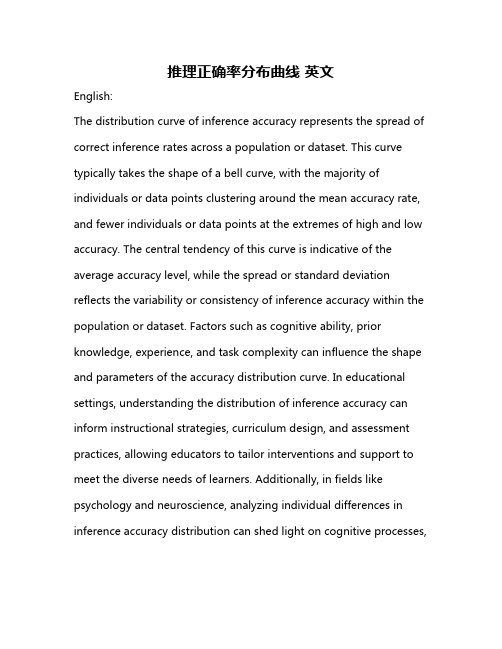
推理正确率分布曲线英文English:The distribution curve of inference accuracy represents the spread of correct inference rates across a population or dataset. This curve typically takes the shape of a bell curve, with the majority of individuals or data points clustering around the mean accuracy rate, and fewer individuals or data points at the extremes of high and low accuracy. The central tendency of this curve is indicative of the average accuracy level, while the spread or standard deviation reflects the variability or consistency of inference accuracy within the population or dataset. Factors such as cognitive ability, prior knowledge, experience, and task complexity can influence the shape and parameters of the accuracy distribution curve. In educational settings, understanding the distribution of inference accuracy can inform instructional strategies, curriculum design, and assessment practices, allowing educators to tailor interventions and support to meet the diverse needs of learners. Additionally, in fields like psychology and neuroscience, analyzing individual differences in inference accuracy distribution can shed light on cognitive processes,learning mechanisms, and neural substrates underlying reasoning and decision-making.Translated content:推理正确率分布曲线代表了在人群或数据集中正确推理率的分布情况。
现代语言学前五章课后习题答案

Chapter 1 Introduction1.Explain the following definition of linguistics: Linguistics is the scientific study oflanguage. 请解释以下语言学的定义:语言学是对语言的科学研究。
Linguistics investigates not any particular languagebut languages in general.Linguistic study is scientific because it is baxxxxsed on the systematic investigation of authentic language data.No serious linguistic conclusion is reached until after the linguist has done the following three things: observing the way language is actually usedformulating some hypothesesand testing these hypotheses against linguistic facts to prove their validity.语言学研究的不是任何特定的语言,而是一般的语言。
语言研究是科学的,因为它是建立在对真实语言数据的系统研究的基础上的。
只有在语言学家做了以下三件事之后,才能得出严肃的语言学结论:观察语言的实际使用方式,提出一些假设,并用语言事实检验这些假设的正确性。
1.What are the major branches of linguistics? What does each of them study?语言学的主要分支是什么?他们每个人都研究什么?Phonetics-How speech sounds are produced and classified语音学——语音是如何产生和分类的Phonology-How sounds form systems and function to convey meaning音系学——声音如何形成系统和功能来传达意义Morphology-How morphemes are combined to form words形态学——词素如何组合成单词Sytax-How morphemes and words are combined to form sentences句法学-词素和单词如何组合成句子Semantics-The study of meaning ( in abstraction)语义学——意义的研究(抽象)Pragmatics-The study of meaning in context of use语用学——在使用语境中对意义的研究Sociolinguistics-The study of language with reference to society社会语言学——研究与社会有关的语言Psycholinguistics-The study of language with reference to the workings of the mind心理语言学:研究与大脑活动有关的语言Applied Linguistics-The application of linguistic principles and theories to language teaching and learning应用语言学——语言学原理和理论在语言教学中的应用1.What makes modern linguistics different from traditional grammar?现代语言学与传统语法有何不同?Modern linguistics is descxxxxriptive;its investigations are baxxxxsed on authenticand mainly spoken language data.现代语言学是描述性的,它的研究是基于真实的,主要是口语数据。
英语是世界语言
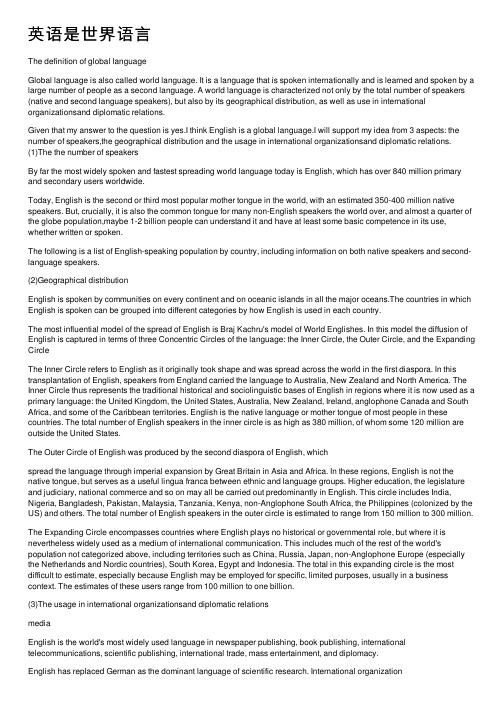
英语是世界语⾔The definition of global languageGlobal language is also called world language. It is a language that is spoken internationally and is learned and spoken by a large number of people as a second language. A world language is characterized not only by the total number of speakers (native and second language speakers), but also by its geographical distribution, as well as use in international organizationsand diplomatic relations.Given that my answer to the question is yes.I think English is a global language.I will support my idea from 3 aspects: the number of speakers,the geographical distribution and the usage in international organizationsand diplomatic relations. (1)The the number of speakersBy far the most widely spoken and fastest spreading world language today is English, which has over 840 million primary and secondary users worldwide.Today, English is the second or third most popular mother tongue in the world, with an estimated 350-400 million native speakers. But, crucially, it is also the common tongue for many non-English speakers the world over, and almost a quarter of the globe population,maybe 1-2 billion people can understand it and have at least some basic competence in its use, whether written or spoken.The following is a list of English-speaking population by country, including information on both native speakers and second-language speakers.(2)Geographical distributionEnglish is spoken by communities on every continent and on oceanic islands in all the major oceans.The countries in which English is spoken can be grouped into different categories by how English is used in each country.The most influential model of the spread of English is Braj Kachru's model of World Englishes. In this model the diffusion of English is captured in terms of three Concentric Circles of the language: the Inner Circle, the Outer Circle, and the Expanding CircleThe Inner Circle refers to English as it originally took shape and was spread across the world in the first diaspora. In this transplantation of English, speakers from England carried the language to Australia, New Zealand and North America. The Inner Circle thus represents the traditional historical and sociolinguistic bases of English in regions where it is now used as a primary language: the United Kingdom, the United States, Australia, New Zealand, Ireland, anglophone Canada and South Africa, and some of the Caribbean territories. English is the native language or mother tongue of most people in these countries. The total number of English speakers in the inner circle is as high as 380 million, of whom some 120 million are outside the United States.The Outer Circle of English was produced by the second diaspora of English, whichspread the language through imperial expansion by Great Britain in Asia and Africa. In these regions, English is not the native tongue, but serves as a useful lingua franca between ethnic and language groups. Higher education, the legislature and judiciary, national commerce and so on may all be carried out predominantly in English. This circle includes India, Nigeria, Bangladesh, Pakistan, Malaysia, Tanzania, Kenya, non-Anglophone South Africa, the Philippines (colonized by the US) and others. The total number of English speakers in the outer circle is estimated to range from 150 million to 300 million. The Expanding Circle encompasses countries where English plays no historical or governmental role, but where it is nevertheless widely used as a medium of international communication. This includes much of the rest of the world's population not categorized above, including territories such as China, Russia, Japan, non-Anglophone Europe (especially the Netherlands and Nordic countries), South Korea, Egypt and Indonesia. The total in this expanding circle is the most difficult to estimate, especially because English may be employed for specific, limited purposes, usually in a business context. The estimates of these users range from 100 million to one billion.(3)The usage in international organizationsand diplomatic relationsmediaEnglish is the world's most widely used language in newspaper publishing, book publishing, international telecommunications, scientific publishing, international trade, mass entertainment, and diplomacy.English has replaced German as the dominant language of scientific research. International organizationBy the time of the foundation of the United Nations at the end of World War II, English had become pre-eminent and is now the main worldwide language of diplomacy and international relations.It is one of six official languages of the United Nations.Many other worldwide international organisations, including the International Olympic Committee, specify English as a working language or official language of the organisation.Many regional international organisations such as the European Free Trade Association, Association of Southeast Asian Nations (ASEAN), and Asia-Pacific Economic Cooperation (APEC) set English as their organisation's sole working language even though most members are not countries with a majority of native English speakers. While the European Union (EU) allows member states to designate any of the national languages as an official language of the Union, in practice English is the main working language of EU organisations.ScienceA working knowledge of English has become a requirement in a number of occupations and professions such as medicineand computing. English has become so important in scientific publishing that more than 80 percent of all scientific journal articles indexed by Chemical Abstracts in 1998 were written in English, as were 90 percent of all articles in natural science publications by 1996 and 82 percent ofarticles in humanities publications by 1995.。
语言学中英术语对照

语言学中英术语对照GlossaryandIndex Aabbreviation缩写词,略语Abercrombieablative夺格,离格abstractness抽象性accent重音(符)accuracy正确性accusative宾格achievementtest成绩测试acousticphonetics声学语音学acquisition习得acronym缩略语actionprocess动作过程actor动作者addition添加addressform称呼形式addressee受话人addresser发话人adjective形容词adjunct修饰成分;附加语adverb副词affix词缀affixhopping词缀跳跃affixation词缀附加法affricate塞擦音agreement一致关系airstream气流alliteration头韵allomorph词/语素变体allophone音位变体allophonicvariation音位变体allophony音位变体现象alveolarridge齿龈alveolar齿龈音ambiguity歧义ambiguous歧义的Americandescriptivelinguistics美国描写语言学AmericanEnglish美式英语AmericanIndianlanguages美国印第安族诸语言Americanstructuralism美国结构主义analogicalcreation类推造字anapest抑抑扬格anaphor前指替代anaphoricreference前指照应Anderson Animalcommunicationsystem动物交际系统animate有生命的annotation注解antecedent先行词;前在词anthropological人类学的anthropologicallinguistics人类语言学anticipatorycoarticulation逆化协同发音antonomasia换称;代类名antonym反义词antonymy反义(关系)appellative称谓性appliedlinguistics应用语言学appliedsociolinguistics应用社会语言学appropriacy适宜性appropriateness适宜性;得体性approximant无摩擦延续音Apteaptitudetest素质测试Arabic阿拉伯语arbitrariness任意性argument中项;中词;主目article冠词articulation发音articulator发音器官articulatoryphonetics发音语音学artificialspeech人工言语aspect体aspirated吐气;送气assimilation同化associative联想associativemeaning联想意义assonance准压韵;半谐音Atkinson,A.M.attributive属性;修饰语;定语auditoryphonetics听觉语音学Austin,JohnLangshawauthenticinput真实投入authorialstyle权威风格authoringprogram编程autonomy自主性auxiliary助词盪血里赵uJSi|enSui|!q互库@赵uoijisoddo|ejaje|iq昙leseu|eiqe|iq昙国赵leiqenqX王弘马lusunoiAeqaq葩耳¥马ssaDOjd|ejnolAeqaq jaAe M^q 切皋吧晋JuouodiuoDasequoijeujJo^DeqaSejsSui|qqeqaq」“人」ei|ixne binarydivision二分法binaryfeature二分特征binarytaxonomy二分分类学binding制约bindingtheory制约论BlackEnglish黑人英语blade舌叶;舌面前部blankverse无韵诗blending混成法borrowing借用;借词boundmorpheme粘着语素boundingtheory管辖论bracketing括号法brevitymaxim简洁准则bridging架接BritishEnglish英式英语broadtranscription宽式音标broadening词义扩大Browncorpus布朗语料库calculability可计算性calque仿造;仿造词语cancellability可删除cardinalnumeral基数cardinalvowel基本元音case格casegrammar格语法casetheory格理论category范畴categoricalcomponent范畴成分causative使役的;使投动词CD-I,compactdisk-interactive交互式激光视盘center中心词centraldeterminer中心限定词chainrelation链状关系chainsystem链状系统Chinese汉语choice选择choicesystem选择系统circumstance环境因子class词类classshift词性变换clause小句;从句click吸气音;咂音clipping截断法closedclass封闭类closedsyllable闭音节cluster音丛coarticulation协同发音coda结尾音节;符尾code语码;信码cognitivepsycholinguistics认知心理语言学cognitivepsychology认知心理学cognitivesystem认知系统coherence相关;关联cohension衔接co-hyponym同下义词colligation类连结collocativemeaning搭配意义colorword色彩词colorwordsystem色彩词系统command指令commoncore共核commonnoun普通名词communication交际communicativecompetence交际能力communicativedynamism,CD交际性动力communicativelanguageteaching,CLT交际语言教学法communicativeSentencePattern,CSP交际性句子模式communicativesyllabus交际教学大纲communicativetest交际性测试communicative-grammaticalapproach交际-语法教学法compactdisk激光盘COMPACTDISD-READONLYMEMORY,CD-ROM激光视盘comparativedegree比较级competence能力complement补语complementaryantonym互补反义词complementaryantonymy互补反义关系complementarydistribution互补分布complexpredicate复合谓语component成分componentialanalysis成分分析compositeproposition复合命题compositionality复合性compound复合词;复合句comprehension理解computation计算computationallinguistics计算语言学computationalsystem计算系统computer计算机;电脑computer-assistedlearning,CAL计算机辅助学习computercorpus计算机语料库computerhardware计算机硬件computerliteracy计算机操作能力computernetworks计算机网络computersystem计算机系统computer-assistedinstruction,CAI计算机辅助教学computer-assistedlearning,CALL计算机辅助语言学习conative意动的concept概念conceptualmeaning概念意concord一致(关系)concordance共现关系concretenoun具体名词concurrent同时发生的conjugation词形变化conjunct连接副词conjunction连接词conjunctionbuttressing连接词支撑connotation内涵consequent跟随成分consonance辅音韵consonant辅音constantopposition不变对立constative表述的constituentcommand成分指令constituentproposition成分命题constituentstructureanalysis成分结构分析constituent成分construct编制constructvalidity编制效度construction构建constructivism构建主义contact接触contentanalysis内容分析contentvalidity内容效度contentword实义词contextdependent语境依赖的contextofsituation情景语境context语境contextualanalyses语境分析contextualmeaning语境意义contrastiveanalysis对比分析controltheory控制理论controlledlanguage有控制的语言convention常规;规约conventionalmeaning常规意义;规约意义conventionality常规性;规约性conversationalimplicature会话含义conversationalmaxim会话准则converseantonymy相反反义现象conversion变换cooperativeprinciple,CP合作原则coordinateconstruction并列结构coordination并列Cordercoreferential互参的coronal舌面前音corpus(pl.corpora)语料;素材corpusdata语料库语料corpuslinguistics语料库语言学context上下文countable可数(名词) counterfactualproposition反事实命题couplet对句;对联creativity创造性;原创性Creole克里澳尔语;混和语cross-cultural跨文化cross-culturalcommunication跨文化交际cross-linguistic跨语言的culture文化culturally-specific文化特异的curriculum教学大纲customizing定制的Ddactyl扬抑抑格Danilanguage达尼语dataretrieval,DR资料检索database数据库dative(case)与格dativemovement与格移动declarative陈述句decoding解码deductive演绎的deepstructure深层结构defeasibility消除可行性definite有定的degeneratedata无用的语料deixis指称delicacy精密阶denotation外延;指称dental齿音dentalization齿音化derivation衍生derivationalaffix.衍生词汇derivationalmorphology派生形态学description描写descriptiveadequacy描写充分性descriptivelinguistics描写语言学designfeature结构特征determiner限定词developinggrammar发展语法deviant变体deviation偏离;变异devoicing清音化diachroniclinguistics历时语言学diachronic历时的diacritic附加符号;变音符diagnostictest诊断性测试dialect方言dialectology方言学dialogue对话dictionary词典digitizedsound数字化语音dimetre二音步诗行diphthong二合元音;双元音directobject直接宾语directspeech,DS直接言语directthought,DT直接思想directionality方向性discourse语篇;话语discourseanalysis语篇分析;话语分析discourseinterpretation语篇理解discrete分离的;离散的discrete-pointgrammar离散语法discretepointtest分立性测试disjunction分离关系displacement移位dissimilation异化(作用)distinctivefeature区别性特征distinguisher辩义成分distribution分布do-insertionruledo添加规则domain范围;领域dorsal舌背音;舌中音dorsum舌背(音)doublecomparative双重比较download下载drama戏剧drill-and-practicesoftware操练软件D-structureD结构dual双数dualisticview二分观点duality二重性economy经济性;简洁性earlyModernEnglish早期现代英语ejective爆发音electronicmail电子邮件ElizabethanEnglish伊利莎白时期英语ellipsis省略(法)ellipticalsentencestructure省略句子结构embeddedelement嵌入成分emic位学的emotive感情的emphasis强调empiricaldata经验主义的语料empirical经验主义的empiricalvalidity经验效度empiricism经验主义emptycategory,EC空范畴enablingskills使成技能化encoding编码endrhyme末端韵endocentricconstruction内向结构entailment蕴涵entrycondition入列条件epenthesis插音;增音equipollentopposition均等对立equivalence相等equivalencereliability相等信度erroranalysis错误分析EST科技英语ethnicityidentity民族认同ethnographyofcommunication交际民族学etic非位的;素的evaluation评估eventprocess事件过程example-basedmachinetranslation基于例句的机器翻译exchangeerror交换错误exchangesequence交际序列exchangestructure交际结构exhaustive穷尽的;彻底的existent存在物existential存在句existentialprocess存在过程existentialquantifier存在数量词exocentric外向的exocentricconstruction外向结构experiential经验的experientialfunction经验功能experimentalpsycholinguistics实验心理语言学explanatoryadequacy解释充分性explicitgrammarinstruction,EGI明显的语法教学法expressionminimization表达最底程度expressive表达的extendedstandardtheory,EST扩展标准理论extensive引申的;扩展的extent-conditionformat程度条件格式externalevaluation外部评估externalqualifier外部修饰语extrinsicsourcesoferror外在的错误来源eyemovement眼部移动。
分布规律 英语

分布规律英语The Distribution PatternThe natural world is a complex and intricate tapestry, woven with the threads of countless living organisms, each occupying its own unique niche and exhibiting distinct patterns of distribution. These distribution patterns, shaped by a myriad of environmental factors and evolutionary adaptations, hold the key to understanding the delicate balance and interconnectedness of ecosystems.At the heart of this distribution lies the concept of habitat, the physical and biotic environment that provides the resources and conditions necessary for the survival and reproduction of a species. Habitat is not merely a static location but a dynamic system, constantly in flux, responding to changes in climate, resource availability, and the interactions between organisms. The distribution of a species is inextricably linked to its ability to thrive within the confines of its habitat, and the factors that govern this distribution can be as diverse as the species themselves.One of the primary determinants of distribution is the physical environment, which encompasses factors such as temperature,precipitation, soil composition, and topography. Each species has evolved a range of tolerance for these environmental conditions, and their distribution is often constrained by the boundaries of their ecological niche. For instance, tropical rainforests are home to a vast array of plant and animal life, adapted to the warm, humid climate and nutrient-rich soil, while the harsh, arid conditions of deserts favor the proliferation of drought-resistant species.Beyond the physical environment, biotic factors, such as the presence of predators, competitors, and symbiotic relationships, also play a crucial role in shaping distribution patterns. Predator-prey dynamics can limit the range of a species, as prey species may be confined to areas where they can effectively evade their predators. Similarly, interspecific competition for limited resources can lead to the exclusion of certain species from particular habitats, forcing them to seek out alternative niches.The concept of dispersal, the movement of individuals or propagules (such as seeds or spores) from one location to another, is another key factor in determining distribution patterns. Dispersal mechanisms, which can range from wind-borne seeds to animal-assisted transport, allow species to colonize new areas and expand their ranges. The success of a species in establishing itself in a new habitat depends on its ability to adapt to the local conditions and outcompete resident species.Temporal factors, such as seasonal changes and long-term climate shifts, can also significantly influence distribution patterns. Migratory species, for instance, may exhibit distinct seasonal distributions, moving between breeding and wintering grounds in response to environmental cues. Similarly, the gradual warming of the planet due to climate change has led to the poleward expansion of many species, as they seek out more favorable environmental conditions.Human activities, both intentional and unintentional, have also had a profound impact on distribution patterns. The introduction of invasive species, often through human-mediated transport, can disrupt the delicate balance of ecosystems, leading to the displacement of native species and the creation of novel distribution patterns. Habitat fragmentation and destruction, driven by urbanization, agriculture, and resource extraction, can also restrict the movement and distribution of species, threatening their long-term survival.Understanding the complex distribution patterns of species is not merely an academic exercise; it holds profound implications for the conservation and management of ecosystems. By studying the factors that govern the distribution of organisms, scientists and policymakers can develop strategies to protect endangered species, restore degraded habitats, and maintain the overall health andresilience of the natural world. This knowledge is particularly crucial in an era of rapid environmental change, where the ability to predict and adapt to shifting distribution patterns may mean the difference between the survival and extinction of countless species.In conclusion, the distribution patterns of living organisms are a testament to the intricate web of relationships that define the natural world. From the physical constraints of the environment to the biotic interactions that shape the landscape, the factors governing distribution are as diverse as the species themselves. By unraveling the mysteries of these patterns, we gain a deeper understanding of the delicate balance that sustains life on our planet, and the vital role we must play in preserving it for generations to come.。
Learning new compositions from given ones
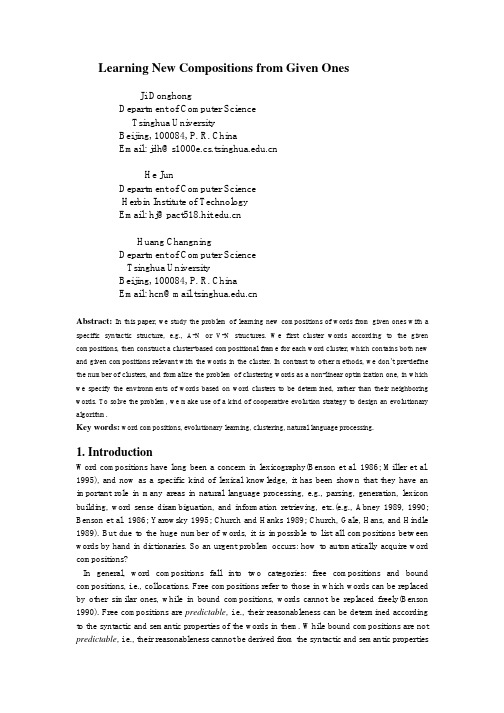
Learning New Compositions from Given OnesJi DonghongDepartment of Computer ScienceTsinghua UniversityBeijing, 100084, P. R. ChinaEmail: jdh@He JunDepartment of Computer ScienceHerbin Institute of TechnologyEmail: hj@Huang ChangningDepartment of Computer ScienceTsinghua UniversityBeijing, 100084, P. R. ChinaEmail: hcn@Abstract:In this paper, we study the problem of learning new compositions of words from given ones with a specific syntactic structure, e.g., A-N or V-N structures. We first cluster words according to the given compositions, then construct a cluster-based compositional frame for each word cluster, which contains both new and given compositions relevant with the words in the cluster. In contrast to other methods, we don’t pre-define the number of clusters, and formalize the problem of clustering words as a non-linear optimization one, in which we specify the environments of words based on word clusters to be determined, rather than their neighboring words. To solve the problem, we make use of a kind of cooperative evolution strategy to design an evolutionary algorithm.Key words: word compositions, evolutionary learning, clustering, natural language processing.1. IntroductionWord compositions have long been a concern in lexicography(Benson et al. 1986; Miller et al. 1995), and now as a specific kind of lexical knowledge, it has been shown that they have an important role in many areas in natural language processing, e.g., parsing, generation, lexicon building, word sense disambiguation, and information retrieving, etc.(e.g., Abney 1989, 1990; Benson et al. 1986; Yarowsky 1995; Church and Hanks 1989; Church, Gale, Hans, and Hindle 1989). But due to the huge number of words, it is impossible to list all compositions between words by hand in dictionaries. So an urgent problem occurs: how to automatically acquire word compositions?In general, word compositions fall into two categories: free compositions and bound compositions, i.e., collocations. Free compositions refer to those in which words can be replaced by other similar ones, while in bound compositions, words cannot be replaced freely(Benson 1990). Free compositions are predictable, i.e., their reasonableness can be determined according to the syntactic and semantic properties of the words in them. While bound compositions are not predictable, i.e., their reasonableness cannot be derived from the syntactic and semantic propertiesof the words in them(Smadja 1993).N ow with the availability of large-scale corpus, automatic acquisition of word compositions, especially word collocations from them have been extensively studied(e.g., Choueka et al. 1988; Church and Hanks 1989; Smadja 1993). The key of their methods is to make use of some statistical means, e.g., frequencies or mutual information, to quantify the compositional strength between words. These methods are more appropriate for retrieving bound compositions, while less appropriate for retrieving free ones. This is because in free compositions, words are related with each other in a more loose way, which may result in the invalidity of mutual information and other statistical means in distinguishing reasonable compositions from unreasonable ones.In this paper, we start from a different point to explore the problem of automatic acquisition of free compositions. Although we cannot list all free compositions, we can select some typical ones as those specified in some dictionaries(e.g., Benson 1986; Zhang et al. 1994). According to the properties held by free compositions, we can reasonably suppose that selected compositions can provide strong clues for others. Furthermore we suppose that words can be classified into clusters, with the members in each cluster similar in their compositional ability, which can be characterized as the set of the words able to combined with them to form meaningful phrases. Thus any given composition, although specifying the relation between two words literally, suggests the relation between two clusters. So for each word(or cluster), there exist some word clusters, the word (or the words in the cluster) can and only can combine with the words in the clusters to form meaningful phrases. We call the set of these clusters compositional frame of the word (or the cluster).A seemingly plausible method to determine compositional frames is to make use of pre-defined semantic classes in some thesauri(e.g., Miller et al. 1993; Mei et al. 1996). The rationale behind the method is to take such an assumption that if one word can be combined with another one to form a meaningful phrase, the words similar to them in meaning can also be combined with each other. But it has been shown that the similarity between words in meaning doesn’t correspond to the similarity in compositional ability(Zhu 1982). So adopting semantic classes to construct compositional frames will result in considerable redundancy.An alternative to semantic class is word cluster based on distributional environment (Brown et al., 1992), which in general refers to the surrounding words distributed around certain word (e.g., Hatzivassiloglou et al., 1993; Pereira et al., 1993), or the classes of them(Bensch et al., 1995), or more complex statistical means (Dagan et al., 1993). According to the properties of the clusters in compositional frames, the clusters should be based on the environment, which, however, is narrowed in the given compositions. Because the given compositions are listed by hand, it is impossible to make use of statistical means to form the environment, the remaining choices are surrounding words or classes of them.Pereira et al.(1993) put forward a method to cluster nouns in V-N compositions, taking the verbs which can combine with a noun as its environment. Although its goal is to deal with the problem of data sparseness, it suffers from the problem itself. A strategy to alleviate the effects of the problem is to cluster nouns and verbs simultaneously. But as a result, the problem of word clustering becomes a bootstrapping one, or a non-linear one: the environment is also to be determined. Bensch et al. (1995) proposed a definite method to deal with the generalized version of the non-linear problem, but it suffers from the problem of local optimization.In this paper, we focus on A-N compositions in Chinese, and explore the problem of learningnew compositions from given ones. In order to copy with the problem of sparseness, we take adjective clusters as nouns’ environment, and take noun clusters as adjectives’ environment. In order to avoid local optimal solutions, we propose a cooperative evolutionary strategy. The method uses no specific knowledge of A-N structure, and can be applied to other structures.The remainder of the paper is organized as follows: in section 2, we give a formal description of the problem. In section 3, we discuss a kind of cooperative evolution strategy to deal with the problem. In section 4, we explore the problem of parameter estimation. In section 5, we present our experiments and the results as well as their evaluation. In section 6, we give some conclusions and discuss future work.2. Problem SettingGiven an adjective set and a noun set, suppose for each noun, some adjectives are listed as its compositional instances1. Our goal is to learn new reasonable compositions from the instances. To do so, we cluster nouns and adjectives simultaneously and build a compositional frame for each noun.Suppose A is the set of adjectives, N is the set of nouns, for any a symbol 206 \f "Symbol" \s 10.5∈}A, let f(a)symbol 205 \f "Symbol" \s 10.5⊆}N be the instance set of a, i.e., the set of nouns in N which can be combined with a, and for any n symbol 206 \f "Symbol" \s 10.5∈}N, let g(n)symbol 205 \f "Symbol" \s 10.5⊆}A be the instance set of n, i.e., the set of adjectives in A which can be combined with n. We first give some formal definitions in the following:Definition 1 partitionSuppose U is a non-empty finite set, we call <U1, U2, ..., U k> a partition of U, if:i) for any U i, and U j, i symbol 185 \f "Symbol" \s 10.5≠}j, U i symbol 199 \f "Symbol" \s10.5∩}U j•symbol 102 \f "Symbol" \s 10.5φ,ii) U=U ii k1≤≤UWe call U i a cluster of U.Suppose U•< A1, A2, ..., A p > is a partition of A, V•<N1, N2, ..., N q> is a partition of N, f and g are defined as above, for any N i, let g(N i)•{A j:_symbol 36 \f "Symbol" \s 10.5∃n symbol 206 \f "Symbol" \s 10.5∈}N i, A j symbol 199 \f "Symbol" \s 10.5∩}g(n)symbol 185 \f "Symbol" \s 10.5≠}symbol 102 \f "Symbol" \s 10.5φ}, and for anyn, let δ<>U V n,()•symbol 124 \f "Symbol" \s 10.5|{a:_symbol 36 \f "Symbol" \s 10.5∃A j, A j symbol 206 \f "Symbol" \s 10.5∈}g(N k), a symbol 206 \f "Symbol" \s 10.5∈}A j}symbol 45 \f "Symbol" \s 10.5−g(n)symbol 124 \f "Symbol" \s 10.5|, where n symbol 206 \f "Symbol" \s 10.5∈}N k. Intuitively,δ<>U V n,()is the number of the new instances relevant with n. We define the general learning amount as the following:Definition 2 learning amountδ<>U V,1 The compositional instances of the adjectives can be inferred from those of the nouns.δ<>U V ,•δ<>∈∑n N n ,()Based on the partitions of both nouns and adjectives, we can define the distance between nouns and that between adjectives.Definition 3 distance between wordsfor any a symbol 206 \f "Symbol" \s 10.5∈}A , let f a ()={N i :_1symbol 163 \f "Symbol" \s 10.5≤}i symbol 163 \f "Symbol" \s 10.5≤}q , N i symbol 199 \f "Symbol" \s 10.5∩}f(a)symbol 185 \f "Symbol" \s 10.5≠}symbol 102 \f "Symbol" \s 10.5φ}, for any n symbol 206 \f "Symbol" \s 10.5∈}N , let g n U ()={A i :_1symbol 163 \f "Symbol" \s 10.5≤}i symbol 163 \f "Symbol" \s 10.5≤}p , A i symbol 199 \f "Symbol" \s 10.5∩}g(n)symbol 185 \f "Symbol" \s 10.5≠}symbol 102 \f "Symbol" \s 10.5φ}, for any two nouns n 1 and n 2, any two adjectives a 1 and a 2, we define the distances between them respectively as the following: i) dis n n U (,)12=1-g n g n g n g n U U U U ()()()()1212∩∪ii) dis a a V (,)12=1-f a f a f a f a V V V V ()()()()1212∩∪According to the distances between words, we can define the distances between word sets .Definition 4 distance between word setsGiven any two adjective sets X 1, X 2symbol 205 \f "Symbol" \s 10.5⊆}A , any two noun sets Y 1, Y 2symbol 205 \f "Symbol" \s 10.5⊆}N , their distances are:i) dis X X V (,)12=a X a X V dis a a 112212∈∈,max{(,)}ii) dis Y Y U (,)12 =n Y n Y dis n n 112212∈∈,max {(,)}Intuitively, the distance between word sets refer to the biggest distance between words respectively in the two sets.We formalize the problem of clustering nouns and adjectives simultaneously as an optimization problem with some constraints. (1)To determine a partition U •<A 1, A 2, ..., A p > of A , and a partition V •<N 1, N 2, ..., N q > of N , where p , q symbol 62 \f "Symbol" \s 10.5>0, which satisfies i) and ii), and minimize δ<>U V ,.i) for any a 1, a 2symbol 206 \f "Symbol" \s 10.5∈}A i , 1symbol 163 \f "Symbol" \s 10.5≤}i symbol 163 \f "Symbol" \s 10.5≤}p , dis a a (,)12<t 1; for A i and A j , 1symbol 163 \f "Symbol" \s 10.5≤}i symbol 185 \f "Symbol" \s 10.5≠}j symbol 163 \f "Symbol" \s 10.5≤}p , dis A A V i j (,)symbol 179 \f "Symbol" \s 10.5≥} t 1;ii) for any n 1, n 2symbol 206 \f "Symbol" \s 10.5∈}N i , 0symbol 163 \f"Symbol" \s 10.5≤}i symbol 163 \f "Symbol" \s 10.5≤}q , dis n n U (,)12<t 1; for N i and N j , 1symbol 163 \f "Symbol" \s 10.5≤}i symbol 185 \f "Symbol" \s 10.5≠}j symbol 163 \f "Symbol" \s 10.5≤}q , dis N N i j (,)symbol 179 \f "Symbol" \s 10.5≥} t 2;where 0symbol 163 \f "Symbol" \s 10.5≤}t 1, t 2symbol 163 \f "Symbol" \s 10.5≤}1.Intuitively, the conditions i) and ii) make the distances between words within clusters smaller, and those between different clusters bigger, and to minimize δ<>U V ,means to minimize the distances between the words within clusters. In fact, (U , V ) can be seen as an abstraction model over given compositions, and t 1, t 2 can be seen as its abstraction degree . Consider the two special case: one is t 1=t 2 =0, i.e., the abstract degree is the lowest, when the result is that one noun forms a cluster and on adjective forms a cluster, which means that no new compositions are learned. The other is t 1=t 2 =1, the abstract degree is the highest, when a possible result is that all nouns form a cluster and all adjectives form a cluster, which means that all possible compositions, reasonable or unreasonable, are learned. So we need estimate appropriate values for the two parameters, in order to make an appropriate abstraction over given compositions, i.e., make the compositional frames contain as many reasonable compositions as possible, and as few unreasonable ones as possible.3. Cooperative EvolutionSince the beginning of evolutionary algorithms, they have been applied in many areas in AI(Davis et al., 1991; Holland 1994). Recently, as a new and powerful learning strategy,cooperative evolution has gained much attention in solving complex non-linear problem. In this section, we discuss how to deal with the problem (1) based on the strategy.According to the interaction between adjective clusters and noun clusters, we adopt such a cooperative strategy: after establishing the preliminary solutions, for any preliminary solution, we optimize N ’s partition based on A ’s partition, then we optimize A ’s partition based on N ’s partition, and so on, until the given conditions are satisfied.3.1 Preliminary SolutionsWhen determining the preliminary population, we also cluster nouns and adjectives respectively.However, we see the environment of a noun as the set of all adjectives which occur with it in given compositions, and that of an adjective as the set of all the nouns which occur with it in given compositions. Compared with (1), the problem is a linear clustering one.Suppose a 1, a 2symbol 206 \f "Symbol" \s 10.5∈}A , f is defined as above, we define the linear distance between them as (2):(2) dis (a 1, a 2)•1-f a f a f a f a ()()()()1212∩∪Similarly, we can define the linear distance between nouns dis (n 1, n 2) based on g . In contrast, we call the distances in definition 3 non-linear distances .According to the linear distances between adjectives, we can determine a preliminary partition of N : randomly select an adjective and put it into an empty set X , then scan the otheradjectives in A , for any adjective in A -X, if its distances from the adjectives in X are all smaller than t 1, then put it into X , finally X forms a preliminary cluster. Similarly, we can build another preliminary cluster in (A -X ). So on, we can get a set of preliminary clusters, which is just a partition of A . According to the different order in which we scan the adjectives, we can get different preliminary partitions of A .Similarly, we can determine the preliminary partitions of N based on the linear distances between nouns. A partition of A and a partition of N forms a preliminary solution of (1), and all possible preliminary solutions forms the population of preliminary solutions, which we also call the population of 0th generation solutions.3.2 Evolution OperationIn general, evolution operation consists of recombination, mutation and selection. Recombination makes two solutions in a generation combine with each other to form a solution belonging to nextgeneration. Suppose <U i 1(); V i 1()> and <U i 2(); V i 2()> are two ith generation solutions, whereU i 1() and U i 2() are two partitions of A , V i 1() and V i 2() are two partitions of N , then <U i 1()•V i 2()>and <U i 2(); V i 1()> forms two possible (i +1)th generation solutions.Mutation makes a solution in a generation improve its fitness , and evolve into a new onebelonging to next generation. Suppose <U i (); V i ()> is a ith generation solution, whereU i ()•<A 1, A 2, ..., A p >•V i ()•<N 1, N 2, ..., N q > are partitions of A and N respectively, the mutation is aimed at optimizing Vi () into V i ()£«1 based on U i (), and makes V i ()£«1 satisfy the condition ii)in (1), or optimizing U i () into Ui ()£«1 based on V i (), and makes U i ()£«1 satisfy the condition i)in (1), then moving words across clusters to minimize δ<>U V ,.We design three steps for mutation operation: splitting , merging and moving , the former two are intended for the partitions to satisfy the conditions in (1), and the third intended to minimizeδ<>U V ,. In the following, we take the evolution of V i ()£«1 as an example to demonstrate the three steps.symbol 183 \f "Symbol" \s 10.5•} Splitting Procedure . For any N k , 1symbol 163 \f "Symbol"\s 10.5≤}k symbol 163 \f "Symbol" \s 10.5≤}q , if there exist n 1, n 2symbol 206 \f "Symbol" \s 10.5∈}N k , such that dis n n U i ()(,)12symbol 179 \f "Symbol" \s 10.5≥} t 2, then splitting N k into two subsets X and Y . The procedure is given as the following:i) Put n 1 into X , n 2 into Y ,ii) Select the noun in (N k -(X symbol 200 \f "Symbol" \s 10.5∪}Y )) whose distance from n 1is the smallest, and put it into X ,iii) Select the noun in (N k -(X symbol 200 \f "Symbol" \s 10.5∪}Y )) whose distance from n 2is the smallest, and put it into Y,iv) Repeat ii) and iii), until X symbol 200 \f "Symbol" \s 10.5∪}Y = N k .For X (or Y ), if there exist n 1, n 2symbol 206 \f "Symbol" \s 10.5∈}X (or Y ), dis n n U i ()(,)12symbol 179 \f "Symbol" \s 10.5≥} t 2, then we can make use of the above procedure to split it into moresmaller sets. Obviously, we can split any N k in V i () into several subsets which satisfy thecondition ii) in (1) by repeating the procedure.symbol 183 \f "Symbol" \s 10.5•} Merging procedure . If there exist N j and N k , where1symbol 163 \f "Symbol" \s 10.5≤}j,k symbol 163 \f "Symbol" \s 10.5≤}q , such that dis N N U j k i ()(,)symbol 60 \f "Symbol" \s 10.5<t 2, then merging them into a new cluster.It is easy to prove that U i () and V i () will meet the condition i) and ii) in (1) respectively, after splitting and merging procedure.symbol 183 \f "Symbol" \s 10.5•} Moving procedure . We call moving n from N j to N k a word move , where 1symbol 163 \f "Symbol" \s 10.5≤}j symbol 185 \f "Symbol" \s 10.5≠}k symbol 163 \f "Symbol" \s 10.5≤}q , denoted as (n , N j , N k ), if the condition (ii) remains satisfied. The procedure is as the following:i) Select a word move (n , N j , N k ) which minimizes δ<>U V ,,ii) Move n from N j to N k ,iii) Repeat i) and ii)•until there are no word moves which reduce δ<>U V ,.After the three steps, U i ()and V i () evolve into U i ()£«1and V i ()£«1 respectively.Selection operation selects the solutions among those in the population of certain generation according to their fitness. We define the fitness of a solution as its learning amount.We use J i to denote the set of ith generation solutions, H (i , i+1), as in (3), specifies the similarity between ith generation solutions and (i +1)th generation solutions.(3) H (i , i+1)=Min U V J Min U V J U V i i i U V i i i i i i i {:_(,)}{:_(,)}()()()(),()(),()()δδ<>+++<>++∈∈11111Let t 3 be a threshold for H (i , i+1), the following is the general evolutionary algorithm:Procedure Clustering (A , N , f , g );begini) Build preliminary solution population I 0,ii) Determine 0th generation solution set J 0 according to their fitness,iii) Determine I i+1 based on J i :a) Recombination: if (U i 1(),V i 1()), (U i 2(),V i 2())symbol 206 \f "Symbol" \s10.5∈}J i , then (U i 1(),V i 2()), (U i 2(),V i 1())symbol 206 \f "Symbol" \s10.5∈}I i+1,b) Mutation: if (U i (),V i ())symbol 206 \f "Symbol" \s 10.5∈}J i , then(U i (),V i ()£«1), (U i ()£«1,V i ())symbol 206 \f "Symbol" \s 10.5∈}I i+1,iv) Determine J i+1 from I i+1 according to their fitness,v) If H (i ,i+1)>t 3, then exit, otherwise goto iii),end •After determining the clusters of adjectives and nouns, we can construct the compositional frame for each noun cluster or each noun. In fact, for each noun cluster N i , g (N i )={A j :_symbol 36 \f "Symbol" \s 10.5∃n symbol 206 \f "Symbol" \s 10.5∈}N i , A j symbol 199 \f "Symbol" \s 10.5∩}g (n )symbol 185 \f "Symbol" \s 10.5≠}symbol 102 \f"Symbol" \s 10.5φ} is just its compositional frame, and for any noun in N i , g (N i ) is also its compositional frame. Similarly, for each adjective (or adjective cluster), we can also determine its compositional frame.4. Parameter EstimationThe parameters t 1 and t 2 in (1) are the thresholds for the distances between the clusters of A and N respectively. If they are too big, the established frame will contain more unreasonable compositions, on the other hand, if they are too small, many reasonable compositions may not be included in the frame. Thus, we should determine appropriate values for t 1 and t 2, which makes the fame contain as many reasonable compositions as possible, meanwhile as few unreasonable ones as possible.Suppose F i is the compositional frame of N i , let F =<F 1, F 2, ..., F q >, for any F i , let A F i •{a :_symbol 36 \f "Symbol" \s 10.5∃X symbol 206 \f "Symbol" \s 10.5∈}F i , a symbol 206 \f "Symbol" \s 10.5∈}X }. Intuitively, A F i is the set of the adjectives learned as the compositionalinstances of the noun in N i . For any n symbol 206 \f "Symbol" \s 10.5∈}N i , we use A n to denote the set of all the adjectives which in fact can modify n to form a meaningful phrase, we now define deficiency rate and redundancy rate of F . For convenience, we use symbol 100 \f "Symbol" \s 10.5δF to represent symbol 100 \f "Symbol" \s 10.5δ(, ).Definition 5 Deficiency rate symbol 97 \f "Symbol" \s 10.5αFsymbol 97 \f "Symbol" \s 10.5αF = n N n F i q nn N i iA A A∈≤≤∈∑∑∑−1Intuitively, symbol 97 \f "Symbol" \s 10.5αF refers to the ratio between the reasonable compositions which are not learned and all the reasonable ones.Definition 6 Redundancy rate symbol 98 \f "Symbol" \s 10.5βFsymbol 98 \f "Symbol" \s 10.5βF •n N F ni q Fi i A A ∈≤≤∑∑−1δIntuitively, symbol 98 \f "Symbol" \s 10.5βF refers to the ratio between unreasonable compositions which are learned and all the learned ones.So the problem of estimating t 1 and t 2 can be formalized as (5):(5) to find t 1 and t 2, which makes symbol 97 \f "Symbol" \s 10.5αF =0, and symbol 98 \f "Symbol" \s 10.5βF =0.But, (5) may exists no solutions, because its constraints are two strong, on one hand, the sparseness of instances may cause symbol 97 \f "Symbol" \s 10.5αF not to get 0 value, even if t 1and t 2 close to 1, on the other hand, the difference between words may cause symbol 98 \f "Symbol" \s 10.5βF not to get 0 value, even if t 1 and t 2 close to 0. So we need to weaken (5).In fact, both symbol 97 \f "Symbol" \s 10.5αF and symbol 98 \f "Symbol" \s 10.5βF can be seen as the functions of t 1 and t 2, denoted as symbol 97 \f "Symbol" \s 10.5αF (t 1, t 2) and symbol 98 \f "Symbol" \s 10.5βF (t 1,t 2) respectively. Given some values for t 1 and t 2, we can compute symbol 97\f "Symbol" \s 10.5αF and symbol 98 \f "Symbol" \s 10.5βF . Although there may exist no values (t 1*, t 2*) for (t 1, t 2), such that symbol 97 \f "Symbol" \s 10.5αF (t 1*, t 2*)=symbol 98 \f "Symbol" \s 10.5βF (t 1*, t 2*)=0, but with t 1 and t 2 increasing, symbol 97 \f "Symbol" \s 10.5αF tends to decrease, while symbol 98 \f "Symbol" \s 10.5βF tends to increase. So we can weaken (5) as (6).(6) to find t 1 and t 2, which maximizes (7). (7) ααF t t T t t F t t T t t t t T t t t t T t t (,)(,)(,)(,)(,)(,)**(,)(,)******12112122121211212212∈∈∑∑−whereT 1(t 1*, t 2*)={(t 1, t 2):_0symbol 163 \f "Symbol" \s 10.5≤}t 1symbol 163\f "Symbol" \s 10.5≤}t 1*, 0symbol 163 \f "Symbol" \s 10.5≤}t 2symbol 163 \f "Symbol" \s 10.5≤}t 2*}, T 2(t 1*, t 2*)={(t 1, t 2):_t 1*<t 1symbol 163 \f "Symbol" \s 10.5≤}1, t 2*<t 2symbol 163 \f "Symbol" \s 10.5≤}1}Intuitively, if we see the area ([0, 1]; [0, 1]) as a sample space for t 1 and t 2, T 1(t 1*, t 2*) and T 2(t 1*, t 2*) are its sub-areas. So the former part of (7) is the mean deficiency rate of the points in T 1(t 1*, t 2*), and the latter part of (7) is the mean deficiency rate of the points in T 2(t 1*, t 2*). To maximize (7) means to maximize its former part, while to minimize its latter part. So ourweakening (5) into (6) lies in finding a point (t 1*, t 2*), such that the mean deficiency rate of thesample points in T 2(t 1*, t 2*) tends to be very low, rather than finding a point(t 1*, t 2*), such that its deficiency rate is 0.5 Experiment Results and EvaluationWe randomly select 30 nouns and 43 adjectives, and retrieve 164 compositions(see Appendix I)between them from Xiandai Hanyu Cihai (Zhang et al. 1994), a word composition dictionary of Chinese. After checking by hand, we get 342 reasonable compositions(see Appendix I), among which 177 ones are neglected in the dictionary. So the sufficiency rate 2(denoted as symbol 103 \f "Symbol" \s 10.5γ) of these given compositions is 47.9%.We select 0.95 as the value of t 3, and let t 1=0.0, 0.1, 0.2, …, 1.0, t 2=0.0, 0.1, 0.2, …, 1.0respectively, we get 121 groups of values for symbol 97 \f "Symbol" \s 10.5αF and symbol 98 \f "Symbol" \s 10.5βF . Fig.1 and Fig.2 demonstrate the distribution of symbol 97 \f "Symbol" \s 10.5αF and symbol 98 \f "Symbol" \s 10.5βF respectively.2 Sufficiency rate refers to the ratio between given reasonable compositions and all reasonable ones.19deficiencyratet2t1 Fig. 1 The distribution of symbol 97 \f "Symbol" \s 10.5αF.19redundancerate(%)t2(1/10)t1(1/10)Fig. 2 The distribution of symbol 98 \f "Symbol" \s 10.5βF.For any given t1, and t2,we found (7) get its biggest value when t1=0.4 and t2=0.4, so we select 0.4 as the appropriate value for both t1 and t2. The result is listed in Appendix II. From Fig.1 and Fig.2, we can see that when t1=0.4 and t2=0.4, both symbol 97 \f "Symbol" \s 10.5αF and symbol 98 \f "Symbol" \s 10.5βF get smaller values. With the two parameters increasing, symbol 97 \f "Symbol" \s 10.5αF decreases slowly, while symbol 98 \f "Symbol" \s 10.5βF increases severely, which demonstrates the fact that the learning of new compositions from the given ones has reached the limit at the point: the other reasonable compositions will be learned at a cost of severely raising the redundancy rate.From Fig.1, we can see that symbol 97 \f "Symbol" \s 10.5αF generally increases as t1 and t2 increase, this is because that to increase the thresholds of the distances between clusters means to raise the abstract degree of the model, then more reasonable compositions will be learned. On the other hand, we can see from Fig.2 that when t1symbol 179 \f "Symbol" \s 10.5≥}0.4, t2symbol 179 \f "Symbol" \s 10.5≥}0.4, symbol 98 \f "Symbol" \s 10.5βF roughly increases as t1 and t2 increase3,3 On some points, it may be not the case.。
英语语言学概论笔记

《英语语言学概论》课程教学大纲一、课程说明:《语言学概论》课程是英语专业本科阶段的一门必修课。
《语言学概论》研究始于20世纪初,其目的是揭示人类深层结构,对语言和语言交际作出客观、科学描述。
现已形成了语音学、音系学、形态学、句法学、语义学、语用学等一系分支学科。
语言学研究社会学等人文学科的结合逐步形成了社会语言学这样的交叉学科。
对于主修语言学的学生来说,了解语言学的知识和语言理论是完全必要和有益的。
本课程的对象是英语专业高年级学生,在本科阶段第6学期和第7学期开设。
其中第一、二、三、四、五、七、八、十一章为必修,其余章节为选修。
二、教学目的及要求:本课程的具体要求是:比较全面,系统地了解《语言学概论》这一领域的研究成果,以及一些最主要、最有影响的语言理论和原则,从而加深对人类语言这一人类社会普遍现象的理性认识,并具备一定的运用语言学理论解释语言现象、解决具体语言问题的能力。
本课程是一门知识性比较强的课程。
在教学过程中,应重点讲授主要理论、原则、和研究方法,使学生着重掌握基本概念和基本理论,在理解消化的基础上记忆。
本课程的对象是英语专业学生,在讲解过程中原则上采用英语范例,但不排除一些有助于学习者理解的、针对性强的汉语例子。
应鼓励学生结合自己的语言实践提供更多的例子来解释相关理论,以达到理论和实践相结合的目的。
三、教学重点与难点:本课程的教学重点是语言学的基本知识和基本理论,语音学、词汇学、句法学、语义学和语用学这些语言学的核心内容。
本课程的教学难点是音韵学理论、句法结构和各个语言学流派的理论观点及其局限性。
四、与其它课程的关系:本课程是一门主干性课程。
与其相关的课程,如语法学、词汇学和语体学等都是语言学的分支,属于选修课程。
五、学时与学分:学时:72学时学分:4学分六、教学内容:第一章绪论本章主要教学内容:1.语言学习的意义。
2.语言的定义。
3.语言的定义特征。
4.语言的起源。
5.语言的功能。
6.语言学的定义。
A divisive information-theoretic feature clustering algorithm for text classification
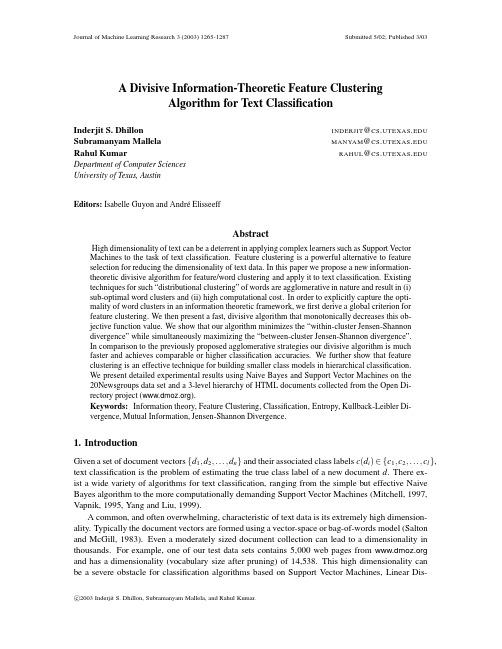
1. Introduction
Given a set of document vectors {d1 , d2 , . . . , dn } and their associated class labels c(di ) ∈ {c1 , c2 , . . . , cl }, text classification is the problem of estimating the true class label of a new document d . There exist a wide variety of algorithms for text classification, ranging from the simple but effective Naive Bayes algorithm to the more computationally demanding Support Vector Machines (Mitchell, 1997, Vapnik, 1995, Yang and Liu, 1999). A common, and often overwhelming, characteristic of text data is its extremely high dimensionality. Typically the document vectors are formed using a vector-space or bag-of-words model (Salton and McGill, 1983). Even a moderately sized document collection can lead to a dimensionality in thousands. For example, one of our test data sets contains 5,000 web pages from and has a dimensionality (vocabulary size after pruning) of 14,538. This high dimensionality can be a severe obstacle for classification algorithms based on Support Vector Machines, Linear Disc 2003 Inderjit S. Dhillon, Subramanyam Mallela, and Rahul Kumar.
English_syntax_2nd_week
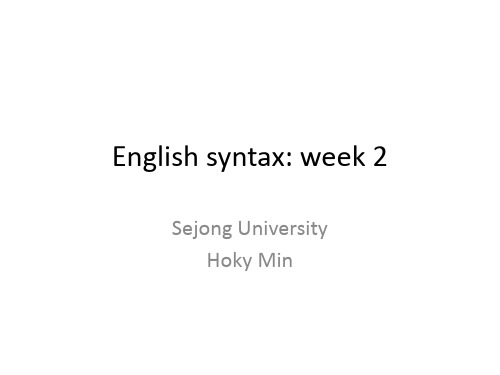
Sejong University Hoky Min
0. Words and why they matter to syntax
• Parts of speech: syntactic category (e.g., verbs, adjectives, adverbs, and prepositions) • Parts of speech tell us how a word is going to function in the sentence.
– The man loved peanut butter cookies. – The king loved peanut butter cookies. – * The in loved peanut butter cookies. – * The sing loved peanut butter cookies.
Nouns
• Derivational suffixes: -ment (basement), -ity (sincerity), -t(ion) (devotion), -ation (expectation), -ist (specialist), -ant(attendant), -ery(shrubbery), -ee(employee), ship(hardship), -aire (billionaire), acy(advocracy)… • Inflectional suffixes: -s(cats), -es(glasses), en(oxen),-ren(children), -i(cacti),-a(addenda).
Verbs
• Derivational suffixes
英语语言学布拉格学派纯英文全解
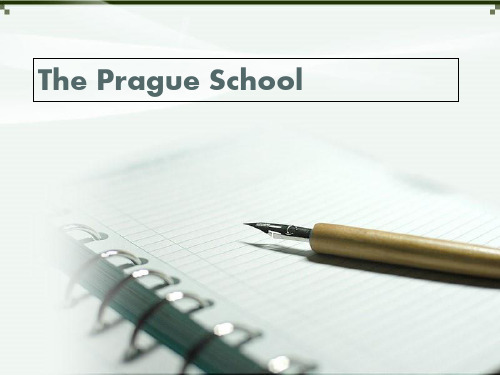
1929 ---- the First International Congress of Slavists in Prague. Came up with Outline
1930 ---- International phonetic system representative conferencein Prague (detail on language, especially on phonetic structure).
multilateral opposition 多边对立 It is a more loosely established relationship: /a/ and /i/ for
instence are alike only to the extent that both are vowels, a quality shared by ant other pairs of vowels.
Second, there was an emphasis on the systemic character of language.
Third, language was looked on as functional in another sense, that is, as a tool performing a number of essential functions or tasks for the community using it.
other, what phonetic features they possess, how they can be classified, etc.
语言学概论知识汇总(英文)
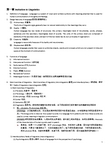
第一章Invitation to Linguistics1.Definition of language:Language is a system of vocal (and written) symbols with meaning attached that is used forhuman communication of thoughts and feelings.2.Design features of language(语言的普遍特征):①.Arbitrariness 任意性:The forms of linguistic signs generally bear no natural relationship to the meanings they carry②.Duality 二重性:Human language has two levels of structures: the primary meaningful level of morphemes, words, phrases, sentences and the secondary meaningless level of sounds. The units of the primary level are composed of elements of the secondary level, and each of the two levels has its own principles of organization.③.Creativity 创造性:Language is resourceful because of its duality and recursiveness.④.Displacement移位性:Human languages enable their users to symbolize objects, events and concepts which are not present in time and space at the moment of communication.3.Functions of language1)Informative function2)Interpersonal function人际功能3)Performative (行为) function4)Emotive function5)Phatic (寒暄) function6)Recreational function7)Metalingual function(元语言功能)指用语言去说明或解释语言的功能4.Main branches of linguistics:Main branches of linguistics (microlinguistics微观) and interdisciplinary(跨领域、跨学科)fields of linguistics (macrolinguistics宏观)1) Main branches of linguistics:(1) Phonetics发音学,语音学;(2) Phonology;(音位学、语音体系)(3) Morphology 词法/ Lexicology词汇学;(4) Syntax句法;(5) Semantics语义学(6) Pragmatics语用学:研究特定情境中的特定话语,在不同的语言交际环境中如何理解和运用语言支。
distribution tutorial翻译
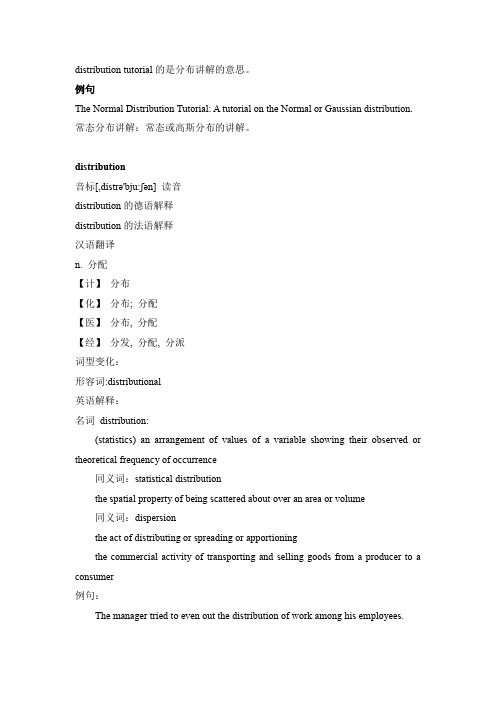
distribution tutorial的是分布讲解的意思。
例句The Normal Distribution Tutorial: A tutorial on the Normal or Gaussian distribution.常态分布讲解:常态或高斯分布的讲解。
distribution音标[,distrә'bju:ʃәn] 读音distribution的德语解释distribution的法语解释汉语翻译n. 分配【计】分布【化】分布; 分配【医】分布, 分配【经】分发, 分配, 分派词型变化:形容词:distributional英语解释:名词distribution:(statistics) an arrangement of values of a variable showing their observed or theoretical frequency of occurrence同义词:statistical distributionthe spatial property of being scattered about over an area or volume同义词:dispersionthe act of distributing or spreading or apportioningthe commercial activity of transporting and selling goods from a producer to a consumer例句:The manager tried to even out the distribution of work among his employees.经理把工作尽量平均分配给雇员。
The distribution and spread, around a targeted region, of spent shrapnel, bomb fragments, or shot from a shotgun.炮弹的散布范围榴* 片、炮弹碎片或短枪射击在靶区的分布和散布面They could not agree about the distribution of the profits.他们对于利润的分配意见不一致。
中英文语义向量模型

中英文语义向量模型Language is a fundamental aspect of human communication and cognition, allowing us to express our thoughts, ideas, and experiences. As the world becomes increasingly interconnected, the need to understand and navigate different languages has become more crucial than ever. One area of language research that has gained significant attention in recent years is the study of semantic vector models, which aim to capture the meaning and relationships between words in a language.Semantic vector models are a class of computational models that represent words or phrases as high-dimensional vectors, where the relative positions of these vectors in the vector space reflect the semantic similarities and differences between the corresponding words or phrases. These models are based on the distributional hypothesis, which states that words with similar meanings tend to appear in similar contexts. By analyzing the patterns of word co-occurrence in large text corpora, semantic vector models can learn the underlying semantic relationships between words and represent them in a compact and efficient manner.One of the most well-known and widely used semantic vector models is the Word2Vec model, developed by researchers at Google in 2013. Word2Vec takes a large corpus of text as input and learns a vector representation for each word, such that words with similar meanings are positioned closer together in the vector space. This allows for the exploration of semantic relationships, such as analogies (e.g., "king" is to "queen" as "man" is to "woman") and clustering of semantically related words.While the Word2Vec model has been successfully applied to a wide range of natural language processing tasks, it was primarily developed and trained on English language data. As the world becomes more multilingual, there is a growing need to extend these semantic vector models to other languages, including Chinese, which is one of the most widely spoken languages in the world.Developing semantic vector models for the Chinese language presents several unique challenges. Unlike English, which is an alphabetic language, Chinese is a logographic language, where each character represents a distinct word or concept. This means that the underlying semantic relationships in Chinese may be more complex and nuanced than in English, requiring different approaches to capture the linguistic nuances.One approach to developing semantic vector models for Chinese is to leverage the rich linguistic information inherent in Chinese characters. Each Chinese character can be decomposed into smaller components, known as radicals, which often carry semantic or phonetic information. By incorporating these character-level features into the vector representation, researchers have been able to improve the performance of Chinese semantic vector models on a variety of tasks, such as word similarity, analogy, and text classification.Another challenge in developing Chinese semantic vector models is the lack of large, high-quality text corpora that are readily available for training. Unlike English, which has a wealth of online resources and digital text, the availability of Chinese language data can be more limited, particularly for specialized domains or regional variations. To address this, researchers have explored techniques such as cross-lingual transfer learning, where pre-trained English models are adapted and fine-tuned to the Chinese language, leveraging the similarities and differences between the two languages.Despite these challenges, the development of Chinese semantic vector models has seen significant progress in recent years. Researchers have proposed various approaches, such as incorporating character-level information, leveraging multilingualcorpora, and exploring transfer learning techniques, to create more accurate and robust Chinese semantic vector models.One notable example is the Chinese Glove (C-GloVe) model, developed by researchers at Tsinghua University. C-GloVe builds upon the successful GloVe (Global Vectors for Word Representation) model, which was originally developed for English, and adapts it to the Chinese language. By incorporating character-level information and leveraging a large-scale Chinese text corpus, C-GloVe has been shown to outperform other Chinese semantic vector models on a range of language tasks, including word similarity, analogy, and text classification.Another example is the Tencent AI Lab Embedding (TenSENT) model, developed by researchers at Tencent, one of the largest technology companies in China. TenSENT is a multilingual semantic vector model that covers a wide range of languages, including Chinese, English, and several other languages. By leveraging a large multilingual corpus and advanced training techniques, TenSENT has demonstrated impressive performance on cross-lingual tasks, such as machine translation and cross-lingual information retrieval.The development of Chinese semantic vector models has not only contributed to the advancement of natural language processing for the Chinese language but has also had broader implications for thefield of computational linguistics. By exploring the unique challenges and opportunities presented by the Chinese language, researchers have gained valuable insights into the nature of language and the underlying principles of semantic representation.Furthermore, the availability of accurate and robust Chinese semantic vector models has opened up new possibilities for cross-cultural collaboration and understanding. As the world becomes increasingly interconnected, the ability to effectively communicate and collaborate across language barriers is crucial. Semantic vector models can serve as a bridge, allowing researchers, businesses, and individuals to better understand and navigate the linguistic and cultural differences between Chinese and other languages.In conclusion, the development of Chinese semantic vector models is an important and ongoing area of research that has significant implications for the field of natural language processing and beyond. By leveraging the unique characteristics of the Chinese language and exploring novel approaches to semantic representation, researchers have made significant strides in creating more accurate and robust models that can enhance our understanding and use of language in a globalized world.。
Summarizing Scientific Articles Experiments with Relevance and Rhetorical Status
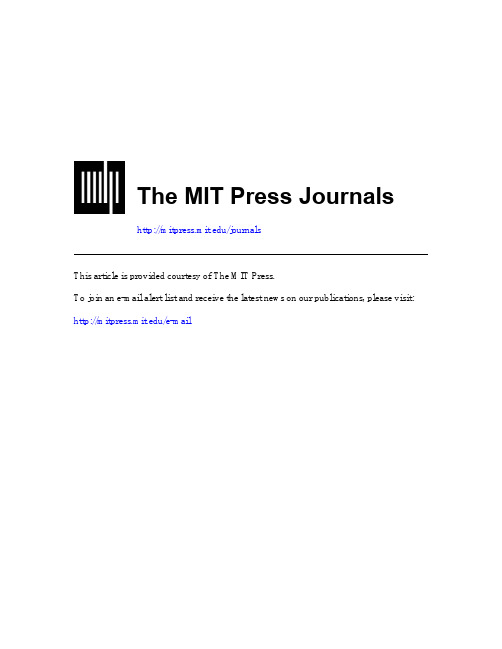
The MIT Press Journals/journalsThis article is provided courtesy of The MIT Press.To join an e-mail alert list and receive the latest news on our publications, please visit: /e-mailSummarizing Scientific Articles:Experiments with Relevance andRhetorical StatusSimone Teufel∗Marc Moens†Cambridge University Rhetorical Systems and University ofEdinburghIn this article we propose a strategy for the summarization of scientific articles that concentrates on the rhetorical status of statements in an article:Material for summaries is selected in such a way that summaries can highlight the new contribution of the source article and situate it with respect to earlier work.We provide a gold standard for summaries of this kind consisting of a substantial corpus of conference articles in computational linguistics annotated with human judgments of the rhetorical status and relevance of each sentence in the articles.We present several experiments measuring our judges’agreement on these annotations.We also present an algorithm that,on the basis of the annotated training material,selects content from unseen articles and classifies it into afixed set of seven rhetorical categories.The output of this extraction and classification system can be viewed as a single-document summary in its own right;alternatively,it provides starting material for the generation of task-oriented and user-tailored summaries designed to give users an overview of a scientificfield.1.IntroductionSummarization systems are often two-phased,consisting of a content selection step followed by a regeneration step.In thefirst step,text fragments(sentences or clauses) are assigned a score that reflects how important or contentful they are.The highest-ranking material can then be extracted and displayed verbatim as“extracts”(Luhn 1958;Edmundson1969;Paice1990;Kupiec,Pedersen,and Chen1995).Extracts are often useful in an information retrieval environment since they give users an idea as to what the source document is about(Tombros and Sanderson1998;Mani et al.1999), but they are texts of relatively low quality.Because of this,it is generally accepted that some kind of postprocessing should be performed to improve thefinal result,by shortening,fusing,or otherwise revising the material(Grefenstette1998;Mani,Gates, and Bloedorn1999;Jing and McKeown2000;Barzilay et al.2000;Knight and Marcu 2000).The extent to which it is possible to do postprocessing is limited,however,by the fact that contentful material is extracted without information about the general discourse context in which the material occurred in the source text.For instance,a sentence describing the solution to a scientific problem might give the main contri-∗Simone Teufel,Computer Laboratory,Cambridge University,JJ Thomson Avenue,Cambridge,CB3OFD,England.E-mail:Simone.Teufel@†Marc Moens,Rhetorical Systems and University of Edinburgh,2Buccleuch Place,Edinburgh,EH89LS, Scotland.E-mail:marc@c 2002Association for Computational LinguisticsComputational Linguistics Volume28,Number4 bution of the paper,but it might also refer to a previous approach that the authors criticize.Depending on its rhetorical context,the same sentence should be treated very differently in a summary.We propose in this article a method for sentence and con-tent selection from source texts that adds context in the form of information about the rhetorical role the extracted material plays in the source text.This added contextual information can then be used to make the end product more informative and more valuable than sentence extracts.Our application domain is the summarization of scientific articles.Summariza-tion of such texts requires a different approach from,for example,that used in the summarization of news articles.For example,Barzilay,McKeown,and Elhadad(1999) introduce the concept of information fusion,which is based on the identification of re-current descriptions of the same events in news articles.This approach works well because in the news domain,newsworthy events are frequently repeated over a short period of time.In scientific writing,however,similar“events”are rare:The main focus is on new scientific ideas,whose main characteristic is their uniqueness and difference from previous ideas.Other approaches to the summarization of news articles make use of the typical journalistic writing style,for example,the fact that the most newsworthy information comesfirst;as a result,thefirst few sentences of a news article are good candidates for a summary(Brandow,Mitze,and Rau1995;Lin and Hovy1997).The structure of scientific articles does not reflect relevance this explicitly.Instead,the introduction often starts with general statements about the importance of the topic and its history in thefield;the actual contribution of the paper itself is often given much later.The length of scientific articles presents another problem.Let us assume that our overall summarization strategy isfirst to select relevant sentences or concepts,and then to synthesize summaries using this material.For a typical10-to20-sentence news wire story,a compression to20%or30%of the source provides a reasonable input set for the second step.The extracted sentences are still thematically connected, and concepts in the sentences are not taken completely out of context.In scientific ar-ticles,however,the compression rates have to be much higher:Shortening a20-page journal article to a half-page summary requires a compression to2.5%of the original. Here,the problematic fact that sentence selection is context insensitive does make a qualitative difference.If only one sentence per two pages is selected,all information about how the extracted sentences and their concepts relate to each other is lost;with-out additional information,it is difficult to use the selected sentences as input to the second stage.We present an approach to summarizing scientific articles that is based on the idea of restoring the discourse context of extracted material by adding the rhetorical status to each sentence in a document.The innovation of our approach is that it defines principles for content selection specifically for scientific articles and that it combines sentence extraction with robust discourse analysis.The output of our system is a list of extracted sentences along with their rhetorical status(e.g.sentence11describes the scientific goal of the paper,and sentence9criticizes previous work),as illustrated in Figure1.(The example paper we use throughout the article is F.Pereira,N.Tishby,and L.Lee’s“Distributional Clustering of English Words”[ACL-1993,cmp lg/9408011];it was chosen because it is the paper most often cited within our collection.)Such lists serve two purposes:in themselves,they already provide a better characterization of scientific articles than sentence extracts do,and in the longer run,they will serve as better input material for further processing.An extrinsic evaluation(Teufel2001)shows that the output of our system is al-ready a useful document surrogate in its own right.But postprocessing could turn 410Teufel and Moens Summarizing Scientific Articles A IM10Our research addresses some of the same questions and uses similar raw data,but we investigate how to factor word association tendencies into associationsof words to certain hidden senses classes and associations between the classesthemselves.11While it may be worthwhile to base such a model on preexisting sense classes(Resnik,1992),in the work described here we look at how to derive the classesdirectly from distributional data.162We have demonstrated that a general divisive clustering procedure for probabilitydistributions can be used to group words according to their participation inparticular grammatical relations with other words.B ASIS19The corpus used in ourfirst experiment was derived from newswire text auto-matically parsed by Hindle’s parser Fidditch(Hindle,1993).113The analogy with statistical mechanics suggests a deterministic annealing pro-cedure for clustering(Rose et al.,1990),in which the number of clusters isdetermined through a sequence of phase transitions by continuously increasingthe parameter EQN following an annealing schedule.C ONTRAST9His notion of similarity seems to agree with our intuitions in many cases,but it isnot clear how it can be used directly to construct word classes and correspondingmodels of association.14Class construction is then combinatorially very demanding and depends onfrequency counts for joint events involving particular words,a potentially un-reliable source of information as we noted above.Figure1Extract of system output for example paper.0This paper’s topic is to automatically classify words according to their contexts of use.4The problem is that for large enough corpora the number of possible joint events is muchlarger than the number of event occurrences in the corpus,so many events are seen rarelyor never,making their frequency counts unreliable estimates of their probabilities.162This paper’s specific goal is to group words according to their participation in particulargrammatical relations with other words,22more specifically to classify nouns accordingto their distribution as direct objects of verbs.Figure2Nonexpert summary,general purpose.the rhetorical extracts into something even more valuable:The added rhetorical con-text allows for the creation of a new kind of summary.Consider,for instance,the user-oriented and task-tailored summaries shown in Figures2and3.Their composi-tion was guided byfixed building plans for different tasks and different user models, whereby the building blocks are defined as sentences of a specific rhetorical status. In our example,most textual material is extracted verbatim(additional material is underlined in Figures2and3;the original sentences are given in Figure5).Thefirst example is a short abstract generated for a nonexpert user and for general information; itsfirst two sentences give background information about the problem tackled.The second abstract is aimed at an expert;therefore,no background is given,and instead differences between this approach and similar ones are described.The actual construction of these summaries is a complex process involving tasks such as sentence planning,lexical choice and syntactic realization,tasks that are outside the scope of this article.The important point is that it is the knowledge about the rhetorical status of the sentences that enables the tailoring of the summaries according to users’expertise and task.The rhetorical status allows for other kinds of applications too:Several articles can be summarized together,contrasts or complementarity among411Computational Linguistics Volume28,Number4 44This paper’s goal is to organise a set of linguistic objects such as words according tothe contexts in which they occur,for instance grammatical constructions or n-grams.22More specifically:the goal is to classify nouns according to their distribution as directobjects of verbs.5Unlike Hindle(1990),9this approach constructs word classes andcorresponding models of association directly.14In comparison to Brown et al.(1992),the method is combinatorially less demanding and does not depend on frequency countsfor joint events involving particular words,a potentially unreliable source of information.Figure3Expert summary,contrastive links.articles can be expressed,and summaries can be displayed together with citation links to help users navigate several related papers.The rest of this article is structured as follows:section2describes the theoretical and empirical aspects of document structure we model in this article.These aspects include rhetorical status and relatedness:•Rhetorical status in terms of problem solving:What is the goal andcontribution of the paper?This type of information is often marked bymetadiscourse and by conventional patterns of presentation(cf.section2.1).•Rhetorical status in terms of intellectual attribution:What information is claimed to be new,and which statements describe other work?This typeof information can be recognized by following the“agent structure”oftext,that is,by looking at all grammatical subjects occurring in sequence(cf.section2.2).•Relatedness among articles:What articles is this work similar to,and in what respect?This type of information can be found by examiningfixedindicator phrases like in contrast to...,section headers,and citations(cf.section2.3).These aspects of rhetorical status are encoded in an annotation scheme that we present in section2.4.Annotation of relevance is covered in section2.5.In section3,we report on the construction of a gold standard for rhetorical status and relevance and on the measurement of agreement among human annotators.We then describe in section4our system that simulates the human annotation.Section5 presents an overview of the intrinsic evaluation we performed,and section6closes with a summary of the contribution of this work,its limitations,and suggestions for future work.2.Rhetorical Status,Citations,and RelevanceIt is important for our task tofind the right definition of rhetorical status to describe the content in scientific articles.The definition should both capture generalizations about the nature of scientific texts and also provide the right kind of information to enable the construction of better summaries for a practical application.Another requirement is that the analysis should be applicable to research articles from different presentational traditions and subject matters.412Teufel and Moens Summarizing Scientific Articles For the development of our scheme,we used the chronologicallyfirst80articles in our corpus of conference articles in computational linguistics(articles presented at COLING,ANLP,and(E)ACL conferences or workshops).Because of the inter-disciplinarity of thefield,the papers in this collection cover a challenging range of subject matters,such as logic programming,statistical language modeling,theoreti-cal semantics,computational dialectology,and computational psycholinguistics.Also, the research methodology and tradition of presentation is very different among these fields;(computer scientists write very different papers than theoretical linguists).We thus expect our analysis to be equally applicable in a wider range of disciplines and subdisciplines other than those named.2.1Rhetorical StatusOur model relies on the following dimensions of document structure in scientific articles.Problem structure.Research is often described as a problem-solving activity(Jordan 1984;Trawinski1989;Zappen1983).Three information types can be expected to occur in any research article:problems(research goals),solutions(methods),and results.In many disciplines,particularly the experimental sciences,this problem-solution struc-ture has been crystallized in afixed presentation of the scientific material as introduc-tion,method,result and discussion(van Dijk1980).But many texts in computational linguistics do not adhere to this presentation,and our analysis therefore has to be based on the underlying logical(rhetorical)organization,using textual representation only as an indication.Intellectual attribution.Scientific texts should make clear what the new contribution is,as opposed to previous work(specific other researchers’approaches)and back-ground material(generally accepted statements).We noticed that intellectual attribu-tion has a segmental character.Statements in a segment without any explicit attribution are often interpreted as belonging to the most recent explicit attribution statement (e.g.,Other researchers claim that).Our rhetorical scheme assumes that readers have no difficulty in understanding intellectual attribution,an assumption that we verified experimentally.Scientific argumentation.In contrast to the view of science as a disinterested“fact factory,”researchers like Swales(1990)have long claimed that there is a strong social aspect to science,because the success of a researcher is correlated with her ability to convince thefield of the quality of her work and the validity of her arguments.Au-thors construct an argument that Myers(1992)calls the“rhetorical act of the paper”: The statement that their work is a valid contribution to science.Swales breaks down this“rhetorical act”into single,nonhierarchical argumentative moves(i.e.,rhetorically coherent pieces of text,which perform the same communicative function).His Con-structing a Research Space(CARS)model shows how patterns of these moves can be used to describe the rhetorical structure of introduction sections of physics articles. Importantly,Swales’s moves describe the rhetorical status of a text segment with re-spect to the overall message of the document,and not with respect to adjacent text segments.Attitude toward other people’s work.We are interested in how authors include refer-ence to other work into their argument.In theflow of the argument,each piece of other work is mentioned for a specific reason:it is portrayed as a rival approach,as a prior approach with a fault,or as an approach contributing parts of the authors’own solution.In well-written papers,this relation is often expressed in an explicit way.The next section looks at the stylistic means available to the author to express the connection between previous approaches and their own work.413Computational Linguistics Volume28,Number4 2.2Metadiscourse and AgentivityExplicit metadiscourse is an integral aspect of scientific argumentation and a way of expressing attitude toward previous work.Examples for metadiscourse are phrases like we argue that and in contrast to common belief,we.Metadiscourse is ubiquitous in scientific writing:Hyland(1998)found a metadiscourse phrase on average after every 15words in running text.A large proportion of scientific metadiscourse is conventionalized,particularly in the experimental sciences,and particularly in the methodology or result section(e.g., we present original work...,or An ANOV A analysis revealed a marginal interaction/a main ef-fect of...).Swales(1990)lists many suchfixed phrases as co-occurring with the moves of his CARS model(pages144,154–158,160–161).They are useful indicators of overall importance(Pollock and Zamora1975);they can also be relatively easily recognized with information extraction techniques(e.g.,regular expressions).Paice(1990)intro-duces grammars for pattern matching of indicator phrases,e.g.,the aim/purpose of this paper/article/study and we conclude/propose.Apart from this conventionalized metadiscourse,we noticed that our corpus con-tains a large number of metadiscourse statements that are less formalized:statements about aspects of the problem-solving process or the relation to other work.Figure4, for instance,shows that there are many ways to say that one’s research is based on somebody else’s(“research continuation”).The sentences do not look similar on the surface:The syntactic subject can be the authors,the originators of the method,or even the method itself.Also,the verbs are very different(base,be related,use,follow). Some sentences use metaphors of change and creation.The wide range of linguistic expression we observed presents a challenge for recognition and correct classification using standard information extraction patterns.With respect to agents occurring in scientific metadiscourse,we make two sug-gestions:(1)that scientific argumentation follows prototypical patterns and employs recurrent types of agents and actions and(2)that it is possible to recognize many of these automatically.Agents playfixed roles in the argumentation,and there are so •We employ Suzuki’s algorithm to learn case frame patterns as dendroid distributions.(9605013)•Our method combines similarity-based estimates with Katz’s back-off scheme,which is widely used for languagemodeling in speech recognition.(9405001)•Thus,we base our model on the work of Clark and Wilkes-Gibbs(1986),and Heeman and Hirst(1992)...(9405013)•The starting point for this work was Scha and Polanyi’s discourse grammar(Scha and Polanyi,1988;Pruest et al.,1994).(9502018)•We use the framework for the allocation and transfer of control of Whittaker and Stenton(1988).(9504007)•Following Laur(1993),we consider simple prepositions(like“in”)as well as prepositional phrases(like“in front of”).(9503007)•Our lexicon is based on afinite-state transducer lexicon(Karttunen et al.,1992).(9503004)•Instead of...we will adopt a simpler,monostratal representation that is more closely related to those found in dependency grammars(e.g.,Hudson(1984)).(9408014)Figure4Statements expressing research continuation,with source article number.414Teufel and Moens Summarizing Scientific Articles few of these roles that they can be enumerated:agents appear as rivals,as contrib-utors of part of the solution(they),as the entire research community in thefield,or as the authors of the paper themselves(we).Note the similarity of agent roles to the three kinds of intellectual attribution mentioned above.We also propose prototypical actions frequently occurring in scientific discourse:thefield might agree,a particular researcher can suggest something,and a certain solution could either fail or be success-ful.In section4we will describe the three features used in our implementation that recognize metadiscourse.Another important construct that expresses relations to other researchers’work is formal citations,to which we will now turn.2.3Citations and RelatednessCitation indexes are constructs that contain pointers between cited texts and citing texts(Garfield1979),traditionally in printed form.When done on-line(as in CiteSeer [Lawrence,Giles,and Bollacker1999],or as in Nanba and Okumura’s[1999]work), citations are presented in context for users to browse.Browsing each citation is time-consuming,but useful:just knowing that an article cites another is often not enough. One needs to read the context of the citation to understand the relation between the articles.Citations may vary in many dimensions;for example,they can be central or perfunctory,positive or negative(i.e.,critical);apart from scientific reasons,there is also a host of social reasons for citing(“politeness,tradition,piety”[Ziman1969]).We concentrate on two citation contexts that are particularly important for the information needs of researchers:•Contexts in which an article is cited negatively or contrastively.•Contexts in which an article is cited positively or in which the authors state that their own work originates from the cited work.A distinction among these contexts would enable us to build more informative citation indexes.We suggest that such a rhetorical distinction can be made manually and automatically for each citation;we use a large corpus of scientific papers along with humans’judgments of this distinction to train a system to make such distinctions.2.4The Rhetorical Annotation SchemeOur rhetorical annotation scheme(cf.Table1)encodes the aspects of scientific argu-mentation,metadiscourse,and relatedness to other work described before.The cat-egories are assigned to full sentences,but a similar scheme could be developed for clauses or phrases.The annotation scheme is nonoverlapping and nonhierarchical,and each sentence must be assigned to exactly one category.As adjacent sentences of the same status can be considered to form zones of the same rhetorical status,we call the units rhetorical zones.The shortest of these zones are one sentence long.The rhetorical status of a sentence is determined on the basis of the global context of the paper.For instance,whereas the O THER category describes all neutral descrip-tions of other researchers’work,the categories B ASIS and C ONTRAST are applicable to sentences expressing a research continuation relationship or a contrast to other work. Generally accepted knowledge is classified as B ACKGROUND,whereas the author’s own work is separated into the specific research goal(A IM)and all other statements about the author’s own work(O WN).415Computational Linguistics Volume28,Number4Table1Annotation scheme for rhetorical status.A IM Specific research goal of the current paperT EXTUAL Statements about section structureO WN(Neutral)description of own work presented in current paper:Method-ology,results,discussionB ACKGROUND Generally accepted scientific backgroundC ONTRAST Statements of comparison with or contrast to other work;weaknesses ofother workB ASIS Statements of agreement with other work or continuation of other workO THER(Neutral)description of other researchers’workThe annotation scheme expresses important discourse and argumentation aspects of scientific articles,but with its seven categories it is not designed to model the full complexity of scientific texts.The category O WN,for instance,could be further sub-divided into method(solution),results,and further work,which is not done in the work reported here.There is a conflict between explanatory power and the simplicity necessary for reliable human and automatic classification,and we decided to restrict ourselves to the rhetorical distinctions that are most salient and potentially most useful for several information access applications.The user-tailored summaries and more in-formative citation indexes we mentioned before are just two such applications;another one is the indexing and previewing of the internal structure of the article.To make such indexing and previewing possible,our scheme contains the additional category T EXTUAL,which captures previews of section structure(section2describes our data...). Such previews would make it possible to label sections with the author’s indication of their contents.Our rhetorical analysis,as noted above,is nonhierarchical,in contrast to Rhetorical Structure Theory(RST)(Mann and Thompson1987;Marcu1999),and it concerns text pieces at a lower level of granularity.Although we do agree with RST that the structure of text is hierarchical in many cases,it is our belief that the relevance and function of certain text pieces can be determined without analyzing the full hierarchical structure of the text.Another difference between our analysis and that of RST is that our analysis aims at capturing the rhetorical status of a piece of text in respect to the overall message,and not in relation to adjacent pieces of text.2.5RelevanceAs our immediate goal is to select important content from a text,we also need a second set of gold standards that are defined by relevance(as opposed to rhetorical status). Relevance is a difficult issue because it is situational to a unique occasion(Saracevic 1975;Sparck Jones1990;Mizzaro1997):Humans perceive relevance differently from each other and differently in different situations.Paice and Jones(1993)report that they abandoned an informal sentence selection experiment in which they used agriculture articles and experts in thefield as participants,as the participants were too strongly influenced by their personal research interest.As a result of subjectivity,a number of human sentence extraction experiments over the years have resulted in low agreementfigures.Rath,Resnick,and Savage (1961)report that six participants agreed on only8%of20sentences they were asked to select out of short Scientific American texts and thatfive agreed on32%of the sentences.They found that after six weeks,subjects selected on average only55% of the sentences they themselves selected previously.Edmundson and Wyllys(1961)416Teufel and Moens Summarizing Scientific Articles find similarly low human agreement for research articles.More recent experiments reporting more positive results all used news text(Jing et al.1998;Zechner1995). As discussed above,the compression rates on news texts are far lower:there are fewer sentences from which to choose,making it easier to agree on which ones to select.Sentence selection from scientific texts also requires more background knowl-edge,thus importing an even higher level of subjectivity into sentence selection experiments.Recently,researchers have been looking for more objective definitions of relevance. Kupiec,Pedersen,and Chen(1995)define relevance by abstract similarity:A sentence in a document is considered relevant if it shows a high level of similarity to a sentence in the abstract.This definition of relevance has the advantage that it isfixed(i.e.,the researchers have no influence over it).It relies,however,on two assumptions:that the writing style is such that there is a high degree of overlap between sentences in the abstract and in the main text and that the abstract is indeed the target output that is most adequate for thefinal task.In our case,neither assumption holds.First,the experiments in Teufel and Moens (1997)showed that in our corpus only45%of the abstract sentences appear elsewhere in the body of the document(either as a close variant or in identical form),whereas Kupiec,Pedersen,and Chen report afigure of79%.We believe that the reason for the difference is that in our case the abstracts were produced by the document authors and by professional abstractors in Kupiec,Pedersen,and Chen’s case.Author summaries tend to be less systematic(Rowley1982)and more“deep generated,”whereas sum-maries by professional abstractors follow an internalized building plan(Liddy1991) and are often created through sentence extraction(Lancaster1998).Second,and more importantly,the abstracts and improved citation indexes we intend to generate are not modeled on traditional summaries,which do not pro-vide the type of information needed for the applications we have in r-mation about related work plays an important role in our strategy for summarization and citation indexing,but such information is rarely found in abstracts.We empir-ically found that the rhetorical status of information occurring in author abstracts is very limited and consists mostly of information about the goal of the paper and specifics of the solution.Details of the analysis we conducted on this topic are given in section3.2.2.We thus decided to augment our corpus with an independent set of human judg-ments of relevance.We wanted to replace the vague definition of relevance often used in sentence extraction experiments with a more operational definition based on rhetorical status.For instance,a sentence is considered relevant only if it describes the research goal or states a difference with a rival approach.More details of the instructions we used to make the relevance decisions are given in section3.Thus,we have two parallel human annotations in our corpus:rhetorical annotation and relevance selection.In both tasks,each sentence in the articles is classified:Each sentence receives one rhetorical category and also the label irrelevant or relevant.This strategy can create redundant material(e.g.,when the same fact is expressed in a sentence in the introduction,a sentence in the conclusion,and one in the middle of the document).But this redundancy also helps mitigate one of the main problems with sentence-based gold standards,namely,the fact that there is no one single best extract for a document.In our annotation,all qualifying sentences in the document are identified and classified into the same group,which makes later comparisons with system performance fairer.Also,later steps cannot onlyfind redundancy in the intermediate result and remove it,but also use the redundancy as an indication of importance.417。
互补分布举例说明英语语言学
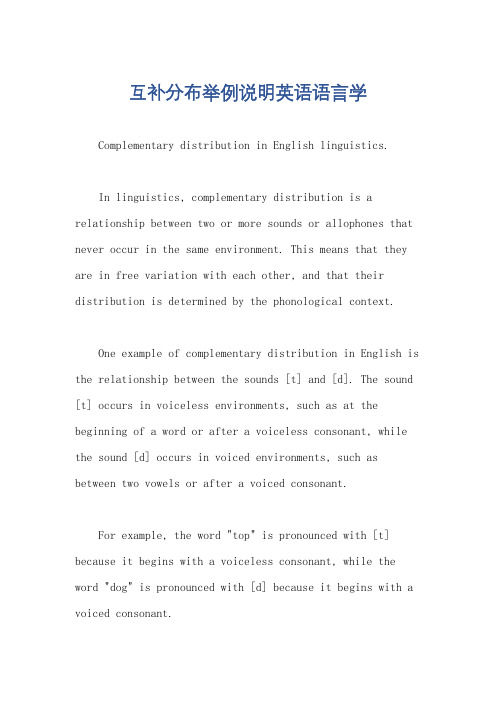
互补分布举例说明英语语言学Complementary distribution in English linguistics.In linguistics, complementary distribution is a relationship between two or more sounds or allophones that never occur in the same environment. This means that they are in free variation with each other, and that their distribution is determined by the phonological context.One example of complementary distribution in English is the relationship between the sounds [t] and [d]. The sound [t] occurs in voiceless environments, such as at the beginning of a word or after a voiceless consonant, while the sound [d] occurs in voiced environments, such as between two vowels or after a voiced consonant.For example, the word "top" is pronounced with [t] because it begins with a voiceless consonant, while the word "dog" is pronounced with [d] because it begins with a voiced consonant.Another example of complementary distribution in English is the relationship between the sounds [p] and [b]. The sound [p] occurs in voiceless environments, such as at the beginning of a word or after a voiceless consonant, while the sound [b] occurs in voiced environments, such as between two vowels or after a voiced consonant.For example, the word "pot" is pronounced with [p] because it begins with a voiceless consonant, while the word "dog" is pronounced with [b] because it begins with a voiced consonant.Complementary distribution is important in phonology because it helps to determine the phonemic inventory of a language. Phonemes are the smallest units of sound that can distinguish one word from another. In English, the sounds [t] and [d] are allophones of the same phoneme /t/, because they are in complementary distribution. This means that they do not contrast with each other, and they do notaffect the meaning of words.However, the sounds [p] and [b] are allophones of different phonemes /p/ and /b/, because they are not in complementary distribution. They contrast with each other, and they can affect the meaning of words. For example, the words "pot" and "dog" are different words because they contain different phonemes.Complementary distribution is a complex topic, but it is an important one for understanding the phonology of English. By understanding how sounds are distributed in a language, we can better understand the way that language works.Additional examples of complementary distribution in English.The sounds [f] and [v] are in complementary distribution. The sound [f] occurs in voiceless environments, such as at the beginning of a word or after a voiceless consonant, while the sound [v] occurs in voiced environments, such as between two vowels or after a voiced consonant.The sounds [s] and [z] are in complementary distribution. The sound [s] occurs in voiceless environments, such as at the beginning of a word or after a voiceless consonant, while the sound [z] occurs in voiced environments, such as between two vowels or after a voiced consonant.The sounds [ʃ] and [ʒ] are in complementary distribution. The sound [ʃ] occurs in voiceless environments, such as at the beginning of a word or after a voiceless consonant, while the sound [ʒ] occurs in voiced environments, such as between two vowels or after a voiced consonant.The sounds [tʃ] and [dʒ] are in complementary distribution. The sound [tʃ] occurs in voiceless environments, such as at the beginning of a word or after a voiceless consonant, while the sound [dʒ] occurs in voiced environments, such as between two vowels or after a voiced consonant.These are just a few examples of complementary distribution in English. There are many other sounds that are in complementary distribution, and these relationships are essential for understanding the phonology of the language.。
语言学选择题

语言学1.__A__ is the study of speech sounds in language or a language with reference to their distribution and patterning and to tacit rules governing pronunciation.A. PhonologyB. LexicographyC. LexicologyD. Morphology2. __C__ is defined as the scientific study of language, studying language in general.A. PsycholinguisticsB. NeurolinguisticsC. LinguisticsD. Phonetics3. Which of the linguistic items listed below is best described as the smallest unit of meaning? BA. the wordB. the morphemeC. the phonemeD. the clause4. A prefix is an affix which appears __B__.A. after the stemB. before the stemC. in the middle of the stemD. below the stem5. Which of the following is true? __C__A. Phonetics is the study of pronunciation.B. Phonetics is the scientific study of the movement of sound waves.C. Phonetics is the scientific study of the sounds of language.D. Phonetics is the scientific study of the organs of speech.6. “What’s in a name? That which we call a roseBy any other name would smell as sweetSo Romeo would, were he not Romeo called,” (Romeo and Juliet, Act 2, Scene 2, 43~5)To what characteristic of language dose Shakespeare refer? __D__A. CreativityB. ProductivityC. DualityD. Arbitrariness7. Language, as a system, consists of two sets of structures or two levels, which is known as __A__, one of a design features of human language.A. DualityB. DisplacementC. ProductivityD. Arbitrariness8. The different members of a phoneme, sounds which are phonetically different but do not make one word different from another in meaning, are __D__.A. phonemesB. phonesC. soundsD. allophones9. What is complementary distribution? __A__A. Different places of occurrence of allophones within a word.B. When a phone can only occur at the end of a word.C. When an allophone occurs at the beginning of a word.D. Contrastive distribution of allophones10. __D__ deals with the analysis and creation of words, idioms and collocations.A. MorphemeB. V ocabularyC. RootD. Lexicon1. Cold and hot are called __B__ antonyms.A. complementaryB. gradableC. reversalD. converse2. “I regret that I can’t help you.” This is an example of __C__.A. representativesB. directivesC. expressivesD. commissives3. What is the duality of the language? __D__A. Letters and soundsB. Sounds and symbolsC. Symbols and meaningD. sounds and meaning4. “I bought some roses” __A__ “I bought some flowers”.A. entailsB. presupposesC. is inconsistent withD. is synonymous with5. Of the following linguists, __C__ should be grouped into Prague School.A. BloomfieldB. SaussureC. JakobsonD. Firth6. Which of the following distinctive features can be used to separate [k] and [g]? (C)A. alveolarB. stopC. voicedD. fricatives7. __A__ A Dictionary of the English Language established a uniform standard for the spelling and word use.A. Samuel Johnson’sB. Bishop Lowth’sC. Firth’sD. Samuel John’s8. What is phonology? __B__A. The study of how speech sounds are made, transmitted and receivedB. The study of the function, behavior and organization of speech sounds as linguisticitems.C. The study of the International Phonetic Alphabet.D. The study of all possible speech sounds.9. The morpheme “cast” in the common word “telecast” is a (n) __D__.A. bound morphemeB. bound formC. inflectional morphemeD. free morpheme10. A phoneme is __D__.A. a set of different realization of a phoneB. a set of contrastive allophones in free variationC. a set of phones in complementary distributionD. a set of phonetically similar noncontrastive phones1. ‘present’ and ‘absent’ are a pair of ________ opposites. (C)A. gradableB. relationalC. complementaryD. converse2. What is defined as “the study of sentence structure”? __D__A. MorphologyB. SemanticsC. PhonologyD. Syntax3. According to Krashen, __D__ refers to the gradual and subconscious development ofability in the first language by using it naturally in daily communicative situations.A. learningB. competenceC. performanceD. acquisition4. There are different types of affixes or morphemes. The affix “ed” in the word “learned” is known as a(n) __C__.A. derivational morphemeB. free morphemeC. inflectional morphemeD. free form5. __C__ studies the total stock of morphemes of a language, especially those items which have clear semantic references.A. PhonologyB. LexicologyC. MorphologyD. Lexicography6. As a type of linguistic system in L2 learning, __A__ is a product of L2 training, mothertongue interference, overgeneralization of the target language rules, and learning and communicative strategies of the learner.A. interlanguageB. interferenceC. language transferD. linguistic relativity7. __A__ means the lack of a logical connection between the form of something and its expression in sounds.A. ArbitrarinessB. AbstractnessC. AmbiguityD. Fuzziness8. The term __B__ linguistics may be defined as a way of referring to the approach whichstudies language change over various periods of time and at various historical stages.A. synchronicB. diachronicC. comparativeD. historical comparative9. When a speech sound changes and becomes more like another sound that follows orprecedes it, it is said to be __D__.A. nasalizedB. voicedC. aspiratedD. assimilated10. F. de Saussure is a (n) __C__ linguist.A. AmericanB. BritishC. SwissD. Russian1. N. Chomsky is a (n) __A__ linguist.A. AmericanB. CanadaC. SwissD. French2. The Sapir-Whorf Hypothesis has two thrusts: __B__ and ____.A. Linguistic description, Linguistic determinismB. Linguistic determinism, Linguistic relativityC. Linguistic relativity, Linguistic descriptionD. Linguistic determinism, Linguistic performance3. The way of forming a new word by joining together the initial part of the first word and the final part of the second word, or by only joining the initial parts of the two words is referred as _________. (C)A. borrowingB. clippingC. blendingD. acronym4. By __B__, we refer to word forms which differ from each other only by one sound, e.g. “pin” and “bin”.A. complementally distributionB. minimal pairC. Adjacency pairD. code—switching5. When two sounds never occur in the same environment they said to be in __A__.A. complementary distributionB. free variationC. co-occurrenceD. minimal pair6. __D__ century is considered to be the beginning of Modern English.A. 18thB. 17thC. 19thD. 16th7. Conventionally a __B__ is put in slashes.A. allophoneB. phonemeC. phoneD. morpheme8. Sapir-whorf hypothesis holds that all higher levels of thinking are dependent on language. This is known as the notion of ____. (B)A. linguistic relativismB. linguistic determinismC. linguistic nativismD. linguistic behaviorism9. According to Searle, those illocutionary acts whose point is to commit the speaker to somefuture course of action are called __C__.A. expressivesB. directivesC. commisivesD. declaratives10. There are ________ morphemes in the word ‘usefulness’. (B)A. twoB. threeC. fourD. five1. Linguistics is the scientific study of __C__.A. a particular languageB. the English languageC. human language in generalD. the system of a particular language2. __A__ is the language that a learner constructs at a given stage of SLA.A. InterlanguageB. IdeologyC. DialectD. Interference3. Phonological rules that govern the combination of sounds in a particular language are called __ B__ rule.A. DeletionB. SequentialC. suprasegmentalD. Assimilation4. “There is no direct link between a linguistic form and what it refers to”. This is the __B__ view concerning the study of meaning.A. naming theoryB. conceptualistC. contextualistD. behaviorist5. English consonants can be classified into stops, fricatives, nasals, etc. , in terms of __A__.A. manner of articulationB. openness of mouthC. place of articulationD. voicing6. According to Chomsky, __A__ is the ideal user’s internalized knowledge of his language.A. competenceB. paroleC. performanceD. langue7. __A__ is not a suprasegmental feature.A. AspirationB. IntonationC. StressD. Tone8. __A__ is a phenomenon that L2 learners subconsciously use their L1language in their learning process.A. Language transferB. BlendingC. InterferenceD. Cooperative9. __C__ are affixes added to an existing form to create a new word, e.g. in-,-er.A. inflectional morphemeB. free morphemeC. derivational morphemeD. root10. Writing is the secondary language form based on __B__.A. soundB. speechC. gestureD. sign1. __C__ covers the study of language use in relation to context, and in particular the study of linguistic communication.A. SemanticsB. SociolinguisticsC. PragmaticsD. Linguistics2. Morphemes that represent “tense”, “number”, “gender”, “case” and so on are called __A__ morphemes.A. inflectionalB. freeC. boundD. derivational3. Which of the following is not a compound word? __C__A. clearwayB. rainbowC. scarcityD. without4. The fact that ability to speak a language is transmitted from generation to generation by process of learning, and not genetically is referred to as __A__.A. culture transmissionB. performanceC. competenceD. acquisition5. __C__ is the language of Angles, Saxons and Jutes who invaded Britain after AD 450.A. Old NorseB. CelticC. Old EnglishD. Middle English6. A group of two or more consonants together in a syllable is called a (n) __C__.A. arresting clusterB. releasing clusterC. consonant clusterD. syllable7. The semantic features of the word “woman” can be expressed as __C__.A. +ANIMATE, -- HUMAN, +ADULT, +MALEB. + ANIMATE, + HUMAN, -- ADULT, + MALEC. + ANIMATE, + HUMAN, + ADULT, -- MALED. + ANIMATE, -- HUMAN, -- ADULT, -- MALE8. Two words that are “opposite” in meani ng are called_________. (C)A. synonymsB. homonymsC. antonymsD. homophones9. __A__ is the study of the relationship between brain and language, including research into how the structure of the brain influences language learning.A. NeurolinguisticsB. PsyhcholingisticsC. Applied LinguisticsD. Sociolinguistics10. Modern synchronic linguistics traditionally dates from the __B__ of Swiss scholar Ferdinand de Saussure.A. Syntactic structureB. Cours de Linguitique GeneralC. De Lingua LatinaD. Language and Mind1.According to the strong version of the __A__ hypothesis, language determines speakers’perceptions and patterns their way of life.A. Sapir WhorfB. inputC. GrimD.Innateness2. Which of the following is true? __D__A. In the history of any language the writing system always came into being before thespoken form.B. A compound is the combination of only two words.C. The division of English into old English, Middle English, and Modern English isnonconventional and not arbitrary.D. If a child is deprived of linguistic environment, he or she is unlikely to learn alanguage successfully later on.3. Which of the following statements is not true? __D__A. Language is a system of arbitrary vocal symbols used for human communication.B. Language is human specificC. Language is relatively stable and systematic while parole is subject to personal and situational constraintsD.The first language was invented by Adam, the first man.4. “Can I borrow your bike?” ________ “You have a bike”.(D)A. is synonymous withB. is inconsistent withC. entailsD. presupposes5. “Your money or your life?” is an example of __C__.A. representativeB. expressiveC. directivesmissives6. Which of the following distinctive features can be used to separate [p] and [b]? _D_A. stopB. fricativesC. bilabialD.voiced7. There are ________ morphemes in the word “speechlessness”.(B)A. twoB. threeC. fourD. five8. ‘boy’ and ‘girl’ are a pair of ________ opposites.(C)A. gradableB. relationalC. complementaryD. converse9. Of all the speech organ, the ________ is /are the most flexible. (C)A. mouthB. lipsC. tongueD. vocal cords10. According to Chomsky, the child is born with a built – in set of rules, which have the specific function of enabling her to construct the grammar of her mother tongue. This view is to be seen as __C__.A. Input hypothesisB. X-theoryC. Language acquisition deviceD. Universal grammar1. “Old” and “Young” are a pair of __D__ opposites.A. complementaryB. relationalC. converseD. gradable2. Systemic-Functional Grammar, one of the most influential linguistic theories in the 20th century, is put forward by __B__.A. ChomskyB. HallidayC. FirthD. Malinowski3. V owels that are produced between the positions for a front and back vowel are called__D__ vowel.A. backB. frontC. unroundedD. central4. From Halliday’s viewpoint, language is a form of realization of __D__ rather than a form of realization of______.A. knowing, doingB. thinking, knowingC. doing, thinkingD. doing, knowing5. The right description of componential analysis for the word ‘man’ i s_____. (C)A. +human, +adult, - maleB. +human, - adult, - maleC. +human, +adult, + maleD. +human, - adult, + male6.__C__studies the physical properties of speech sound, as transmitted between mouth and ear.A. Articulatory phoneticsB. Physiological phoneticsC Acoustic phonetics D. Auditory phonetics7. Creativity refers to __B__.A. the unconscious knowledge that language users have in their mindsB. the capacity of language users to produce and understand an indefinitely large numberof sentencesC. a property claimed to be characteristic of all languagesD. animals’ capacity to learn more than one human language8. The major development in linguistics before 20th century was ___ grammar. (D)A. speculativeB. structuralC. transformational-generativeD. traditional9. “Competence” refers to __B__.A. knowledge of meaning of words and sentencesB. a speaker’s unconscious knowledge about his/her languageC. the actual use of a speaker’s unconscious knowledge about his/her languageD. the laws that pertain to all languages throughout the world10. __A__ refers to unintentionally deviation from the adult grammar of a native speaker.A. An errorB. A mistakeC. A slip of the tongueD. Fossilization1. __C__ is a multiword construction that is a semantic unit whose meaning cannot be deduced from the meanings of its constituents.A. semantic componentB. collocationC. idiomD. reference2. The distinction between langue and parole is similar to that between __B__.A. prescriptive and descriptiveB. competence and performanceC. speech and writingD. synchronic and diachronic3. Nouns, verbs, and adjectives can be classified as __A__.A. open class wordsB. grammatical wordsC. closed class wordsD. function words4. What is the meaning relationship between the two words “furniture/bed”? __B__A. polysemyB. hyponymyC. homonymyD. antonymy5. All of the following are meaningful except for ________. (D)A. morphemeB. allomorphC. lexemeD. phoneme6. Which of the following distinctive features can be used to separate [t] and [d]? (C)A. alveolarB. stopC. voicedD. fricatives7. In structural grammar, distributional analysis is used to define __D__, which are taken as the basic building blocks.A. morphemesB. wordsC. syllableD. phonemes8. “Speech Act T heory” was proposed by __D__ in 1962.A. SaussureB. ChomskyC. Jane AustinD. John Austin9. The major new development in linguistics in 20th century was __D__ grammar.A. speculativeB. traditionalC. structuralD. transformational-generative10. Two words that are differentiated by one phoneme, such as “lead”and “lid”, are called _______. (B)A. codeB. minimal pairC. distinctive featureD. argument1. The most recognizable difference between American English and British English are in __D__ and vocabulary.A. structureB. grammarC. usageD. pronunciation2. The study of how we do things with utterance is the study of __C__, the nature of which is determined by context.A. contextB. pragmaticsC. speech actD. semantics3. A(n) __A__ is a mild, indirect or less offensive word or expression that replaces a taboo word or serves to avoid more direct wording that might be harsh, unpleasantly direct, or offensive, e.g. “pass away” for “die”.A. euphemismsB. deleteC. coinageD. taboo4. In many societies of the world, we find a large number of people who speak more than one language. As a characteristic of societies, __B__ inevitably results from the coming into contact of people with different cultures and different languages.A. transferB. bilingualismC. diglossiaD. inter-language5. Pragmatics differs from traditional semantics in that it studies meaning not in isolation, but in __D__.A. relationshipB. dependenceC. sentenceD. context6. ________ studies the linguistic meaning of words, phrases, and sentences. (A)A. SemanticsB. MorphologyC. pragmaticsD. syntax7. _____ is different from the others according to places of articulation? (D)A. [p]B. [b]C. [m]D. [n]8. __A__ is defined as any regionally or socially definable human group identified by shared linguistic system.A. Speech communityB. A raceC. A societyD. A country9. __A__ invasions established three major groups in England: Saxons, Angles and Jutes.A. GermanicB. NormanC. FrenchD. Roman10. There are ________ morphemes in the word ‘friendships’. (B)A. twoB. threeC. fourD. five1. __C__ is one whose distribution is functionally equivalent to that of one or more of its constituents, i.e. a word or group of words, which serves as a definable “center” or “head”.A. Exocentric constructionB. CoordinationC. Endocentric constructionD. Collocation2. Of the following linguists, __A__ should not be grouped into American school.A. FirthB. SapirC. BloomfieldD. Boas3. When people learn a foreign language for external goals such as passing exams, financial rewards or furthering a career, we say they learn a foreign language with a (n) __D__.A. intrinsic motivationB. resultative motivationC. integrative motivationD. instrumental motivation4. What is the sense relation in the sentence “M y unmarried sister is married to a bachelor.”__B__A. PresupposeB. ContradictionC. EntailmentD. Inconsistent5. ---TRUTH.---Do not say what you believe to be false.---Do not say that for which you lack adequate evidence.Those can be defined as the features of __B__ of Gricean maxims.A. maxim of quantityB. maxim of qualityC. maxim of relationD. maxim of manner6. __C__ caused by the differing rates of vibration of the vocal cords refers to the use of pitch in language to distinguish words.A. IntonationB. StressC. ToneD.Aspiration7. “Autumn” and “fall” are used respectively in Britain and America, but refer to the same thing. The words are __B__ synonyms.A. collocationalB. dialectalC. completeD. stylistic8. Which of the following country are those loanwords “garage, champion, beauty, parliament” borrowed from __D__.A. LatinB. DutchC. GermanD.French9. In the sentence “The angry man went furiously through the rooms.” The first division into immediate constitute should be between __B__.A. angry and manB. man and wentC. furiously and throughD. The and angry10. __C__ refers to the effect of the utterance.A. Illocutionary actB. Locutionary actC. Perlocutionary actD. Speech act1. The consonant sound /p/ is described as __A__.A. voiceless bilabial stopB. voiceless alveolar stopC. voiced bilabial stopD. voiced alveolar stop2. A new word created by cutting the final part or cutting the initial part is referred to as__C__.A. acronymB. borrowingC. clippingD. blending3. According to the author our brain is divided into two hemispheres. Language functions are mainly located in __C__.A. right hemispheresB. front hemispheresC. left hemispheresD. back hemispheres4. “A language pattern which occurs in all known language” is called __C__.A. a phonemic representationB. a phonetic representationC. a language universalD. language change5. In the sentence-------“The child found the puppy”, __C__ is not a constituent.A. The childB. found the puppyC. found theD. the puppy6. __D__ is the abstract syntactic representation of a sentence, namely, the underlying level of structural organization which specifies all the factors governing the way the sentence should be interpreted.A. surface structureB. syntactic ambiguityC. syntactic componentD. deep structure7. __C__ is the learner’s process of adapting to the culture and value system of the target language community.A. AcquisitionB. AssimilationC. AcculturationD. Articulation8. What is the relationship between the two words “flower / rose”? __C__A. HomonymyB. AntonymyC. hyponymyD. Polysemy9. The function of the sentence “How are you?” __D__A. directiveB. informativeC. performativeD. phatic10. Homonyms __C__.A. are words that share the same phonetic features and the same semantic featuresB. are words that share the same semantic features but have different sets ofphonetic featuresC. are words that share the same phonetic features but have different sets of semantic featuresD. are two words that all but one of semantic features in common1. The distinction between language and parole is proposed by __B__.A. HallidayB. SaussureC. ChomskyD. Firth2. In the following dialogue, the maxim of __C__ is not observed.A. What time is it?B. It’s terribly cold in here.A. qualityB. quantityC. relevanceD. manner3. __B__ are linguistic units larger than sentences.A. MovesB. DiscoursesC. TopicsD. Tendencies4. Which of the following two-term sets shows the feature of complementarity? _A_A. single/marriedB. big/smallC. hot / coldD. old /young5. Usually __A__ refers to the use of linguistic research in language teaching, but linguistics is used in other areas, as well.A. applied linguisticsB. theoretical linguisticsC. contextual linguisticsD. general linguistics6. Two words that are differentiated by one phoneme, such as “cat” and “rat”, are known as a __D__.A. distinctive featureB. argumentC. codeD. minimal pair7. __D__ is often regarded as the founder of the study of sociolinguistics.A. SaussureB. HallidayC. ChomskyD. Labov8. __C__ is the academic discipline concerned with the study of the processes by which people learn languages in addition to their native tongue.A. IPAB. IC AnalysisC. SLAD. TG9. The __C__ is the primary lexical unit of a word, which carries the most significant aspects of semantic content and cannot be reduced into smaller constituents.A. bound morphemeB. affixC. rootD. prefix10. In terms of S earle’s classification system of illocutionary acts, the sentence “Ten bucks say that T he Yankee will win the game.” used to bet belongs to __A__.A. representativeB. commissiveC. directiveD. declaration1. Three factors involved in describing vowels are __B__.A. place of articulation / part of the tongue raised / voicingB. tongue height / part of the tongue raised / lip roundingC. articulators / extreme vowel positions / tongue positionD. teeth position / alveolar ridge position / voicing2. In __C__ the structure of words is studied.A. phoneticsB. phonologyC. morphologyD. syntax3. Which one is not a source of error? __D__A the native language B. the target languageC. learner’s style of thinkingD. none4. “Love” and “hate” are __C__.A. binary antonymsB. complementary pairsC. gradable antonymsD. relational opposites5. __A__ refers to sentences not only describe or report information, but also help speakers accomplish things.A. Speech actB. DiscourseC. ContextD. Communication6. The feature that distinguishes “hotdog” and “hot dog” is __B__.A. toneB. stressC. intonationD. aspiration7. __A__ deals with how language is acquired, understood and produced.A. PsycholinguisticsB. SociolinguisticsC. NeurolinguistcsD. Anthropological linguistics8. The study of language at some point of time is generally termed as __D__ linguistics.A. appliedB. diachronicC. comparativeD. synchronic9. Of the following linguists, __A__ should be grouped into London school.A. FirthB. BloomfieldC. BoasD. Trubetzkoy10. London speech that was illustrated by Shakespeare’s writing was generally termed __C__.A. Old EnglishB. Middle EnglishC. Early Modern EnglishD. Late Modern1. The basic essentials of the first language are acquired in the short period from about age two to puberty, which is called the __D__ period for the first language acquisition.A. initialB. one-word stageC. pubertyD. critical2. The study of the linguistic meaning of words, phrases, and sentences is called __A__.A. semanticsB. pragmaticsC. syntaxD. language change3. In making conversation, the general principle that all participants are expected to observe is called the __D__ principle proposed by J. Grice.A. comprehensiveB. generativeC. discourseD. cooperative4. __C__ is concerned with the inherent meaning of the linguistic form.A. referenceB. lexical meaningC. senseD. word。
牛津小学英语上海版
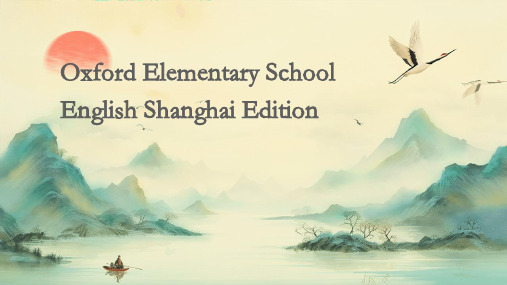
01
Course Introduction
Course objectives
Develop students' English …
The course aims to provide students with the opportunity to improve their listening and speaking abilities through a variety of authentic and engaging activities.
Supplementary materials
The textbook comes with a variety of supplementary materials, including worksheets, quizzes, and audio recordings, to provide extra practice and reinforcement for students outside of class.
Textbook features
Authentic and engaging content
The textbook includes a variety of interesting and relevant topics that are designed to capture students' interest and encourage them to participate actively in class.
Foster students' independent learning and creativity
The course encourages students to think creatively and independently, to solve problems, and to express their ideas and opinions confidently in English.
- 1、下载文档前请自行甄别文档内容的完整性,平台不提供额外的编辑、内容补充、找答案等附加服务。
- 2、"仅部分预览"的文档,不可在线预览部分如存在完整性等问题,可反馈申请退款(可完整预览的文档不适用该条件!)。
- 3、如文档侵犯您的权益,请联系客服反馈,我们会尽快为您处理(人工客服工作时间:9:00-18:30)。
Our research addresses some of the same questions and uses similar raw data, but we investigate how to factor word association tendencies into associations of words to certain hidden senses classes and associations between the classes themselves. While it may be worthwhile to base such a model on preexisting sense classes (Resnik, 1992), in the work described here we look at how to derive the classes directly from distributional data. More speci cally, we model senses as probabilistic concepts or clusters c with corresponding cluster membership probabilities p(cjw) for each word w. Most other class-based modeling techniques for natural language rely instead on \hard" Boolean classes (Brown et al., 1990). Class construction is then combinatorially very demanding and depends on frequency counts for joint events involving particular words, a potentially unreliable source of information as we noted above. Our approach avoids both problems.
direct object for a verb from the likelihoods of that direct object for similar verbs. This requires a reasonable de nition of verb similarity and a similarity estimation method. In Hindle's proposal, words are similar if we have strong statistical evidence that they tend to participate in the same events. His notion of similarity seems to agree with our in clear how it can be used directly to construct word classes and corresponding models of association.
of verbs; the converse problem is formally similar. More
Hindle (1990) proposed dealing with the sparseness problem by estimating the likelihood of unseen events from that of \similar" events that have been seen. For instance, one may estimate the likelihood of a particular
INTRODUCTION
Methods for automatically classifying words according to their contexts of use have both scienti c and practical interest. The scienti c questions arise in connection to distributional views of linguistic (particularly lexical) structure and also in relation to the question of lexical acquisition both from psychological and computational learning perspectives. From the practical point of view, word classi cation addresses questions of data sparseness and generalization in statistical language models, particularly models for deciding among alternative analyses proposed by a grammar.
parser Fidditch (Hindle, 1993). More recently, we have
constructed similar tables with the help of a statisti-
cal part-of-speech tagger (Church, 1988) and of tools
cmp-lg/9408011 22 Aug 1994
Abstract
We describe and experimentally evaluate a method for automatically clustering words according to their distribution in particular syntactic contexts. Deterministic annealing is used to nd lowest distortion sets of clusters. As the annealing parameter increases, existing clusters become unstable and subdivide, yielding a hierarchical \soft" clustering of the data. Clusters are used as the basis for class models of word coocurrence, and the models evaluated with respect to held-out test data.
for regular expression pattern matching on tagged cor-
pora (Yarowsky, 1992). We have not yet compared the
accuracy and coverage of the two methods, or what sys-
tematic biases they might introduce, although we took
care to lter out certain systematic errors, for instance
the misparsing of the subject of a complement clause
Jerusalem 91904, Israel
Lillian Lee
Dept. of Computer Science Cornell University Ithaca, NY
pereira@ tishby@cs.huji.ac.il
llee@
Problem Setting
In what follows, we will consider two major word classes, V and N, for the verbs and nouns in our experiments, and a single relation between them, in our experiments relation between a transitive main verb and the head noun of its direct object. Our raw knowledge about the relation consists of the frequencies fvn of occurrence of particular pairs (v; n) in the required con guration in a training corpus. Some form of text analysis is required to collect such a collection of pairs. The corpus used in our rst experiment was derived from newswire text automatically parsed by Hindle's