Stroboscopic model and bifurcations in TCPRED
Microbiota Modulate Behaviora

Microbiota Modulate Behavioral and Physiological Abnormalities Associatedwith Neurodevelopmental DisordersElaine Y.Hsiao,1,2,*Sara W.McBride,1Sophia Hsien,1Gil Sharon,1Embriette R.Hyde,3Tyler McCue,3Julian A.Codelli,2 Janet Chow,1Sarah E.Reisman,2Joseph F.Petrosino,3Paul H.Patterson,1,4,*and Sarkis K.Mazmanian1,4,*1Division of Biology and Biological Engineering,California Institute of Technology,Pasadena,CA91125,USA2Division of Chemistry and Chemical Engineering,California Institute of Technology,Pasadena,CA91125,USA3Alkek Center for Metagenomics and Microbiome Research,Baylor College of Medicine,Houston,TX77030,USA4These authors contributed equally to this work*Correspondence:ehsiao@(E.Y.H.),php@(P.H.P.),sarkis@(S.K.M.)/10.1016/j.cell.2013.11.024SUMMARYNeurodevelopmental disorders,including autism spectrum disorder(ASD),are defined by core behav-ioral impairments;however,subsets of individuals display a spectrum of gastrointestinal(GI)abnormal-ities.We demonstrate GI barrier defects and micro-biota alterations in the maternal immune activation (MIA)mouse model that is known to display features of ASD.Oral treatment of MIA offspring with the hu-man commensal Bacteroides fragilis corrects gut permeability,alters microbial composition,and ame-liorates defects in communicative,stereotypic,anxi-ety-like and sensorimotor behaviors.MIA offspring display an altered serum metabolomic profile,and B.fragilis modulates levels of several metabolites. Treating naive mice with a metabolite that is increased by MIA and restored by B.fragilis causes certain behavioral abnormalities,suggesting that gut bacterial effects on the host metabolome impact behavior.Taken together,thesefindings support a gut-microbiome-brain connection in a mouse model of ASD and identify a potential probiotic therapy for GI and particular behavioral symptoms in human neurodevelopmental disorders.INTRODUCTIONNeurodevelopmental disorders are characterized by impaired brain development and behavioral,cognitive,and/or physical abnormalities.Several share behavioral abnormalities in socia-bility,communication,and/or compulsive activity.Most recog-nized in this regard is autism spectrum disorder(ASD),a serious neurodevelopmental condition that is diagnosed based on the presence and severity of stereotypic behavior and deficits in lan-guage and social interaction.The reported incidence of ASD has rapidly increased to1in88births in the United States as of2008(Autism and Developmental Disabilities Monitoring Network Sur-veillance Year2008Principal Investigators and CDC,2012),rep-resenting a significant medical and social problem.However, therapies for treating core symptoms of autism are limited. Much research on ASD has focused on genetic,behavioral, and neurological aspects of disease,though the contributions of environmental risk factors(Hallmayer et al.,2011),immune dysregulation(Onore et al.,2012),and additional peripheral dis-ruptions(Kohane et al.,2012)in the pathogenesis of ASD have gained significant attention.Among several comorbidities in ASD,gastrointestinal(GI) distress is of particular interest,given its reported prevalence (Buie et al.,2010;Coury et al.,2012)and correlation with symp-tom severity(Adams et al.,2011).While the standardized diag-nosis of GI symptoms in ASD is yet to be clearly defined,clinical as well as epidemiological studies have reported abnormalities such as altered GI motility and increased intestinal permeability (Boukthir et al.,2010;D’Eufemia et al.,1996;de Magistris et al.,2010).Moreover,a recent multicenter study of over 14,000ASD individuals reveals a higher prevalence of inflamma-tory bowel disease(IBD)and other GI disorders in ASD patients compared to controls(Kohane et al.,2012).GI abnormalities are also reported in other neurological diseases,including Rett syn-drome(Motil et al.,2012),cerebral palsy(Campanozzi et al., 2007),and major depression(Graff et al.,2009).The causes of these GI problems remain unclear,but one possibility is that they may be linked to gut bacteria.Indeed,dysbiosis of the microbiota is implicated in the patho-genesis of several human disorders,including IBD,obesity,and cardiovascular disease(Blumberg and Powrie,2012),and several studies report altered composition of the intestinal mi-crobiota in ASD(Adams et al.,2011;Finegold et al.,2010;Fine-gold et al.,2012;Kang et al.,2013;Parracho et al.,2005;Williams et al.,2011;Williams et al.,2012).Commensal bacteria affect a variety of complex behaviors,including social,emotional,and anxiety-like behaviors,and contribute to brain development and function in mice(Collins et al.,2012;Cryan and Dinan, 2012)and humans(Tillisch et al.,2013).Long-range interactions between the gut microbiota and brain underlie the ability of microbe-based therapies to treat symptoms of multiplesclerosis Cell155,1451–1463,December19,2013ª2013Elsevier Inc.1451and depression in mice (Bravo et al.,2011;Ochoa-Repa´raz et al.,2010),and the reported efficacy of probiotics in treating emotional symptoms of chronic fatigue syndrome and psycho-logical distress in humans (Messaoudi et al.,2011;Rao et al.,2009).Based on the emerging appreciation of a gut-microbiome-brain connection,we asked whether modeling some of the behavioral features of ASD in a mouse model also causes GI abnormalities.Several mouse models of genetic and/or environ-mental risk factors are used to study ASD.We utilize the maternal immune activation (MIA)model,which is based on large epide-miological studies linking maternal infection to increased autismrisk in the offspring (Atlado´ttir et al.,2010;Gorrindo et al.,2012).A number of studies link increased ASD risk to familial autoim-mune disease (Atlado´ttir et al.,2009;Comi et al.,1999)and elevated levels of inflammatory factors in the maternal blood,placenta,and amniotic fluid (Abdallah et al.,2013;Brown et al.,2013;Croen et al.,2008).Modeling MIA in mice by injecting preg-nant dams with the viral mimic poly(I:C)yields offspring that exhibit the core communicative,social,and stereotyped impair-ments relevant to ASD,as well as a common autism neuro-pathology—a localized deficiency in cerebellar Purkinje cells (Malkova et al.,2012;Shi et al.,2009).Furthermore,pregnant monkeys exposed to poly(I:C)yield offspring with symptoms of ASD (Bauman et al.,2013).Although several environmental and genetic risk factors for ASD have been investigated in pre-clinical models,GI abnormalities have not been reported.We show herein that offspring of MIA mice,which display behavioral abnormalities,have defects in intestinal integrity and alterations in the composition of the commensal microbiota that are analo-gous to features reported in human ASD.To explore the potential contribution of GI complications to these symptoms,weF I T C i n t e n s i t y /m l s e r u m (f o l d c h a n g e )Adult (8-10 weeks)F I T C i n t e n s i t y /m l s e r u mAdolescent (3 weeks)S O C S N O S I L 1T N F I L 1I L m R N A /A C T B (f o l d c h a n g e )*S PF G F -b a s iI F N -I L -1I L -I L -I L -I L -12p 40/p 7I L -1I P -1K M C P -M I M I P -1T N F -V E G 300350400450p g /m g p r o t e i nT J P T J P O C L C L D N C L D N C L D N C L D N C L D N C L D N 1C L D N 1m R N A /A C T B (f o l d c h a n g e )S PABCDFigure 1.MIA Offspring Exhibit GI Barrier Defects and Abnormal Expression of Tight Junction Components and Cytokines(A)Intestinal permeability assay,measuring FITC intensity in serum after oral gavage of FITC-dextran.Dextran sodium sulfate (DSS):n =6,S (saline+vehicle):adult n =16;adolescent n =4,P (poly(I:C)+vehicle):adult n =17;adolescent n =4.Data are normalized to saline controls.(B)Colon expression of tight junction components relative to b -actin.Data for each gene are normalized to saline controls.n =8/group.(C)Colon expression of cytokines and inflamma-tory markers relative to b -actin.Data for each gene are normalized to saline controls.n =6–21/group.(D)Colon protein levels of cytokines and chemo-kines relative to total protein content.n =10/group.For each experiment,data were collected simul-taneously for poly(I:C)+B.fragilis treatment group (See Figure 3).See also Figure S1.examine whether treatment with the gut bacterium Bacteroides fragilis ,demon-strated to correct GI pathology in mouse models of colitis (Mazmanian et al.,2008)and to protect against neuroinflam-mation in mouse models of multiple sclerosis (Ochoa-Repa´raz et al.,2010),impacts ASD-related GI and/or behavioral abnormalities in MIA offspring.Our study reflects a mechanistic investigation of how alterations in the commensal microbiota impact behavioral abnormalities in a mouse model of neurodeve-lopmental disease.Our findings suggest that targeting the microbiome may represent an approach for treating subsets of individuals with behavioral disorders,such as ASD,and comor-bid GI dysfunction.RESULTSOffspring of Immune-Activated Mothers Exhibit GI Symptoms of Human ASDSubsets of ASD children are reported to display GI abnormal-ities,including increased intestinal permeability or ‘‘leaky gut’’(D’Eufemia et al.,1996;de Magistris et al.,2010;Ibrahim et al.,2009).We find that adult MIA offspring,which exhibit a number of behavioral and neuropathological symptoms of ASD (Malkova et al.,2012),also have a significant deficit in intestinal barrier integrity,as reflected by increased translocation of FITC-dextran across the intestinal epithelium,into the circulation (Figure 1A,left).This MIA-associated increase in intestinal permeability is similar to that of mice treated with dextran sodium sulfate (DSS),which induces experimental colitis (Figure 1A,left).Defi-cits in intestinal integrity are detectable in 3-week-old MIA offspring (Figure 1A,right),indicating that the abnormality is established during early life.Consistent with the leaky gut phenotype,colons from adult MIA offspring contain decreased gene expression of TJP1,TJP2,OCLN ,and CLDN8and increased expression of CLDN15(Figure 1B).Deficient expres-sion of TJP1is also observed in small intestines of adult MIA1452Cell 155,1451–1463,December 19,2013ª2013Elsevier Inc.offspring(Figure S1A available online),demonstrating a wide-spread defect in intestinal barrier integrity.Gut permeability is commonly associated with an altered immune response(Turner,2009).Accordingly,colons from adult MIA offspring display increased levels of interleukin-6(IL-6) mRNA and protein(Figures1C and1D)and decreased levels of the cytokines/chemokines IL-12p40/p70and MIP-1a(Fig-ure1D).Small intestines from MIA offspring also exhibit altered cytokine/chemokine profiles(Figure S1C).Changes in intestinal cytokines are not accompanied by overt GI pathology,as as-sessed by histological examination of gross epithelial morphology from hematoxylin-and eosin-stained sections (data not shown).Overall,wefind that adult offspring of im-mune-activated mothers exhibit increased gut permeability and abnormal intestinal cytokine profiles.MIA Offspring Display Dysbiosis of the Gut Microbiota Abnormalities related to the microbiota have been identified in ASD individuals,including disrupted community composition (Adams et al.,2011;Finegold et al.,2010;Finegold et al., 2012;Parracho et al.,2005;Williams et al.,2011;Williams et al.,2012),although it is important to note that a well-defined ASD-associated microbial signature is lacking thus far.To eval-uate whether MIA induces microbiota alterations,we surveyed the fecal bacterial population by16S rRNA gene sequencing of samples isolated from adult MIA or control offspring.Alpha di-versity,i.e.,species richness and evenness,did not differ signif-icantly between control and MIA offspring,as measured by several indices(Figures S2A and S2B).In contrast,unweighted UniFrac analysis,which measures the degree of phylogenetic similarity between microbial communities,reveals a strong ef-fect of MIA on the gut microbiota of adult offspring(Figure2). MIA samples cluster distinctly from controls by principal coordi-nate analysis(PCoA)and differ significantly in composition(Ta-ble S3,with ANOSIM R=0.2829,p=0.0030),indicating robust differences in the membership of gut bacteria between MIA offspring and controls(Figure2A).The effect of MIA on altering the gut microbiota is further evident when sequences from the classes Clostridia and Bacteroidia,which account for approxi-mately90.1%of total reads,are exclusively examined by PCoA(R=0.2331,p=0.0070;Figure2B),but not when Clostri-dia and Bacteroidia sequences are specifically excluded from PCoA of all other bacterial classes(R=0.1051,p=0.0700;Fig-ure2C).This indicates that changes in the diversity of Clostridia and Bacteroidia operational taxonomic units(OTUs)are the pri-mary drivers of gut microbiota differences between MIA offspring and controls.Sixty-seven of the1,474OTUs detected across any of the samples discriminate between treatment groups,including those assigned to the bacterial families Lachnospiraceae, Ruminococcaceae,Erysipelotrichaceae,Alcaligenaceae,Por-phyromonadaceae,Prevotellaceae,Rikenellaceae,and un-classified Bacteroidales(Figure2D and Table S1).Of these67 discriminatory OTUs(relative abundance:13.3%±1.65%con-trol,15.93%±0.62%MIA),19are more abundant in the control samples and48are more abundant in MIA samples.Consistent with the PCoA results(Figures2A–2C),the majority of OTUs that discriminate MIA offspring from controls are assigned to theclasses Bacteroidia(45/67OTUs or67.2%;12.02%±1.62% control,13.48%±0.75%MIA)and Clostridia(14/67OTUs or 20.9%;1.00%±0.25%control,1.58%±0.34%MIA).Interest-ingly,Porphyromonadaceae,Prevotellaceae,unclassified Bac-teriodales(36/45discriminatory Bacteroidial OTUs or80%;4.46%±0.66%control,11.58%±0.86%MIA),and Lachnospir-iceae(8/14discriminatory Clostridial OTUs or57%;0.28%±0.06%control,1.13%±0.26%MIA)were more abundant in MIA offspring.Conversely,Ruminococcaceae(2OTUs),Erysipe-lotrichaceae(2OTUs),and the beta Proteobacteria family Alca-ligenaceae(2OTUs)were more abundant in control offspring (Figure2D and Table S1;0.95%±0.31%control,0.05%±0.01%MIA).This suggests that specific Lachnospiraceae,along with other Bacteroidial species,may play a role in MIA pathogen-esis,while other taxa may be protective.Importantly,there is no significant difference in the overall relative abundance of Clostridia(13.63%± 2.54%versus14.44%± 2.84%;p= 0.8340)and Bacteroidia(76.25%±3.22%versus76.22%±3.46%;p=0.9943)between MIA offspring and controls(Fig-ure2E,left),indicating that alterations in the membership of Clostridial and Bacteroidial OTUs drive major changes in the gut microbiota between experimental groups.Overall,wefind that MIA leads to dysbiosis of the gut microbiota,driven primarily by alterations in specific OTUs of the bacterial classes Clostridia and Bacteroidia.Changes in OTUs classified as Lachnospiraceae and Ruminococcaceae of the order Clostridiales parallel select reports of increased Clos-tridium species in the feces of subjects with ASD(Finegold et al.,2012).Altogether,modeling MIA in mice induces not only behavioral and neuropathological features of ASD(Malkova et al.,2012;Shi et al.,2009)but also microbiome changes as described in subsets of ASD individuals.Bacteroides fragilis Improves Gut Barrier Integrity in MIA OffspringGut microbes play an important role in the development,mainte-nance and repair of the intestinal epithelium(Turner,2009).To determine whether targeting the gut microbiota could impact MIA-associated GI abnormalities,we treated mice with the human commensal B.fragilis at weaning,and tested for GI abnormalities at8weeks of age.B.fragilis has previously been shown to ameliorate experimental colitis(Mazmanian et al., 2008;Round and Mazmanian,2010).Remarkably,B.fragilis treatment corrects intestinal permeability in MIA offspring(Fig-ure3A).In addition,B.fragilis treatment ameliorates MIA-associ-ated changes in expression of CLDNs8and15,but not TJP1, TJP2,or OCLN(Figure3B).Similar changes are observed in pro-tein levels of CLDNs8and15in the colon,with restoration by B.fragilis treatment(Figures3C and3D).No effects of B.fragilis on tight junction expression are observed in the small intestine(Figure S1B),consistent with the fact that Bacteroides species are predominantly found in the colon.Finally,the pres-ence of GI defects prior to probiotic administration(Figure1A, right)suggests that B.fragilis may treat,rather than prevent, this pathology in MIA offspring.B.fragilis treatment also restores MIA-associated increases in colon IL-6mRNA and protein levels(Figures3E and3F).Levels of other cytokines are altered in both colons and small intestines of Cell155,1451–1463,December19,2013ª2013Elsevier Inc.1453MIA offspring (Figures 1D and S1C),but these are not affected by B.fragilis treatment,revealing specificity for IL-6.We further find that recombinant IL-6treatment can modulate colon levels of both CLDN 8and 15in vivo and in in vitro colon organ cultures (data not shown),suggesting that B.fragilis -mediated restora-tion of colonic IL-6levels could underlie its effects on gut permeability.Collectively,these findings demonstrate that B.fragilis treatment of MIA offspring improves defects in GIbarrier integrity and corrects alterations in tight junction and cytokine expression.B.fragilis Treatment Restores Specific Microbiota Changes in MIA OffspringThe finding that B.fragilis ameliorates GI defects in MIA offspring prompted us to examine its effects on the intestinal microbiota.No significant differences are observedfollowingFigure 2.MIA Offspring Exhibit Dysbiosis of the Intestinal Microbiota(A)Unweighted UniFrac-based 3D PCoA plot based on all OTUs from feces of adult saline+vehicle (S)and poly(I:C)+vehicle (P)offspring.(B)Unweighted UniFrac-based 3D PCoA plot based on subsampling of Clostridia and Bacteroidia OTUs (2003reads per sample).(C)Unweighted UniFrac-based 3D PCoA plot based on subsampling of OTUs remaining after subtraction of Clostridia and Bacteroidia OTUs (47reads per sample).(D)Relative abundance of unique OTUs of the gut microbiota (bottom,x axis)for individual biological replicates (right,y axis),where red hues denote increasing relative abundance of a unique OTU.(E)Mean relative abundance of OTUs classified at the class level for the most (left)and least (right)abundant taxa.n =10/group.Data were simultaneously collected and analyzed for poly(I:C)+B.fragilis treatment group (See Figure 4).See also Figure S2and Table S1.1454Cell 155,1451–1463,December 19,2013ª2013Elsevier Inc.B.fragilis treatment of MIA offspring by PCoA (ANOSIM R =0.0060p =0.4470;Table S3),in microbiota richness (PD:p =0.2980,Observed Species:p =0.5440)and evenness (Gini:p =0.6110,Simpson Evenness:p =0.5600;Figures 4A,S2A and S2B),or in relative abundance at the class level (Fig-ure S2C).However,evaluation of key OTUs that discriminate adult MIA offspring from controls reveals that B.fragilis treatment significantly alters levels of 35OTUs (Table S2).Specifically,MIA offspring treated with B.fragilis display significant restoration in the relative abundance of 6out of the 67OTUs found to discriminate MIA from control offspring (Figure 4B and Table S2),which are taxonomically assigned as unclassified Bacteroidia and Clostridia of the family Lachnospiraceae (Figure 4B and Table S2).Notably,these alterations occur in the absence of persistent colonization of B.fragilis ,which remains undetectable in fecal and cecal samples isolated from treated MIA offspring (Figures S2D and S2E).Phylogenetic reconstruction of the OTUs that are altered by MIA and restored by B.fragilis treatment reveals that the Bacteroidia OTUs cluster together into a monophyletic group and the Lachnospiraceae OTUs cluster into two monophyletic groups (Figure 4C).This result suggests that,although treatment of MIA offspring with B.fragilis may not lead to persistent colonization,this probiotic corrects the relative abundance of specific groups of related microbes of the Lachnospiraceae family as well as unclassified Bacteriodales.Altogether,we demonstrate that treatment of MIA offspring with B.fragilis ameliorates particular MIA-associ-ated alterations in the commensalmicrobiota.C LD N 8/T U B B (f o l d c h a n g e )F I T C i n t e n s i t y /m l s e r u m (f o l d c h a n g e )*****CLDN8: -tubulin: CLDN15:-tubulin:I L 6 m R N A /A C T B (f o l d c h a n g e )**CLDN8T J PT J P O C L C L D N C L D N 1m R N A /A C T B (f o l d c h a n g e )n.s.n.s.*****P P+BFS I L -I L -12p 40/p 7I P -1M I M I P -1p g /m g p r o t e i n (f o l dc h a ng e )C LD N 15/T U B B (f o l d c h a n g e )ABCDEFFigure 3.B.fragilis Treatment Corrects GI Deficits in MIA Offspring(A)Intestinal permeability assay,measuring FITC intensity in serum after oral gavage of FITC-dextran.Data are normalized to saline controls.Data for DSS,saline +vehicle (S)and poly(I:C)+vehicle (P)are as in Figure 1.poly(I:C)+B.fragilis (P+BF):n =9/group.(B)Colon expression of tight junction components relative to b -actin.Data for each gene are normalized saline controls.Data for S and P are as in Figure 1.Asterisks directly above bars indicate significance compared to saline control (normalized to 1,as denoted by the black line),whereas asterisks at the top of the graph denote statistical significance between P and P+BF groups.n =8/group.(C)Immunofluorescence staining for claudin 8.Representative images for n =5.(D)Colon protein levels of claudin 8(left)and claudin 15(right).Representative signals are depicted below.Data are normalized to signal intensity in saline controls.n =3/group.(E)Colon expression of IL-6relative to b -actin.Data are normalized to saline controls.Data for S and P are as in Figure 1.P+BF:n =3/group.(F)Colon protein levels of cytokines and chemokines relative to total protein content.Data are normalized to saline controls.Data for S and P are as in Figure 1.n =10/group.See also Figure S1.Cell 155,1451–1463,December 19,2013ª2013Elsevier Inc.1455B.fragilis Treatment Corrects ASD-Related Behavioral AbnormalitiesStudies suggest that GI issues can contribute to the develop-ment,persistence,and/or severity of symptoms seen in ASD and related neurodevelopmental disorders (Buie et al.,2010;Coury et al.,2012).To explore the potential impact of GI dysfunc-tion on core ASD behavioral abnormalities,we tested whetherB.fragilis treatment impacts anxiety-like,sensorimotor,repeti-tive,communicative,and social behavior in MIA offspring.We replicated previous findings that adult MIA offspring display several core behavioral features of ASD (Malkova et al.,2012).Open field exploration involves mapping an animal’s movement in an open arena to measure locomotion and anxiety (Bourin et al.,2007).MIA offspring display decreased entries and time0.2LachnospiraceaeBFigure 4.B.fragilis Treatment Alters the Intestinal Microbiota and Corrects Species-Level Abnormalities in MIA Offspring(A)Unweighted UniFrac-based 3D PCoA plot based on all OTUs.Data for saline (S)and poly(I:C)(P)are as in Figure 2.(B)Relative abundance of key OTUs of the family Lachnospiraceae (top)and order Bacteroidales (bottom)that are significantly altered by MIA and restored by B.fragilis treatment.(C)Phylogenetic tree based on nearest-neighbor analysis of 16S rRNA gene sequences for key OTUs presented in (B).Red clades indicate OTUs of the family Lachnospiraceae and green clades indicate OTUs of the order Bacteriodales.Purple taxa indicate OTUs that are significantly elevated in P and corrected by B.fragilis (BF)treatment.n =10/group.See also Figure S2and Table S2.1456Cell 155,1451–1463,December 19,2013ª2013Elsevier Inc.spent in the center of the arena,which is indicative of anxiety-like behavior (Figure 5A;compare saline [S]to poly(I:C)[P]).The prepulse inhibition (PPI)task measures the ability of an animal to inhibit its startle in response to an acoustic tone (‘‘pulse’’)when it is preceded by a lower-intensity stimulus (‘‘prepulse’’).Deficiencies in PPI are a measure of impaired sensorimotor gating and are observed in several neurodevelopmental disor-ders,including autism (Perry et al.,2007).MIA offspring exhibit decreased PPI in response to 5or 15db prepulses (Figure 5B).The marble burying test measures the propensity of mice to engage repetitively in a natural digging behavior that is not confounded by anxiety (Thomas et al.,2009).MIA offspring display increased stereotyped marble burying compared tocontrols (Figure 5C).Ultrasonic vocalizations are used to mea-sure communication by mice,wherein calls of varying types and motifs are produced in different social paradigms (Grimsley et al.,2011).MIA offspring exhibit deficits in communication,as indicated by reduced number and duration of ultrasonic vocali-zations produced in response to a social encounter (Figure 5D).Finally,the three-chamber social test is used to measure ASD-P P I (%)5 db 15 dbS P P+BF**S P P+BF 0255075D u r a t i o n p e r c a l l (m s )****T o t a l c a l l d u r a t i o n (s )****S P P+BF200400600T o t a l n u m b e r o f c a l l s**S P P+BF ****S PP+BF 010203040D i s t a n c e (m )S o c i a l p r e f e r e n c e :c h a m b e r d u r a t i o n (%)***S o c i a b i l i t y :c h a m b e r d u r a t i o n (%)*Anxiety and locomotion: Open field exploration Sensorimotor gating: Pre-pulse inhibitionStereotyped behavior: Marble burying Communication:Ultrasonic vocalizationsSocial Interaction:SociabilitySocial Interaction: Social preference BDEF Figure 5.B.fragilis Treatment Ameliorates Autism-Related Behavioral Abnormalities in MIA Offspring(A)Anxiety-like and locomotor behavior in the open field exploration assay.n =35–75/group.(B)Sensorimotor gating in the PPI assay.n =35–75/group.(C)Repetitive marble burying assay.n =16–45/group.(D)Ultrasonic vocalizations produced by adult male mice during social encounter.n =10/group.S =saline+vehicle,p =poly(I:C)+vehicle,P+BF =poly(I:C)+B.fragilis .Data were collected simul-taneously for poly(I:C)+B.fragilis D PSA and poly(I:C)+B.thetaiotaomicron treatment groups (See also Figures S3and S4).related impairments in social interaction (Silverman et al.,2010).MIA offspringexhibit deficits in both sociability,or pref-erence to interact with a novel mouseover a novel object,and social preference (social novelty),or preference to interact with an unfamiliar versus a familiar mouse(Figures 5E and 5F).Altogether,MIA offspring demonstrate a number of be-havioral abnormalities associated with ASD as well as others such as anxiety and deficient sensorimotor gating.Remarkably,oral treatment with B.fragilis ameliorates many of these be-haviors.B.fragilis -treated MIA offspring do not exhibit anxiety-like behavior in theopen field (Figure 5A;compare poly(I:C)[P]to poly(I:C)+B.fragilis [P+BF]),as shown by restoration in the number of center entries and duration of time spent in the center of the arena.B.fragilis im-proves sensorimotor gating in MIA offspring,as indicated by increased combined PPI in response to 5and 15db prepulses (Figure 5B),with no significant effect on the intensity of startle to the acoustic stimulus (data not shown).B.fragilis -treated mice also exhibit decreased levels of stereotyped marble burying and restored communicative behavior (Figures 5C and 5D).Interestingly,B.fragilis raises the duration per call by MIA offspring to levels exceeding those observed in saline controls (Figure 5D),suggesting that despite normalization of the pro-pensity to communicate (number of calls),there is a qualitative difference in the types of calls generated with enrichment of longer syllables.Although B.fragilis -treated MIA offspring exhibit improved communicative,repetitive,anxiety-like,and sensorimotor behavior,they retain deficits in sociability and social preference (Figure 5E).Selective amelioration of ASD-related behaviors is also seen with risperidone treatment of CNTNAP2knockoutmice,a genetic mouse model for ASD (Pen˜agarikano et al.,2011),wherein communicative and repetitive,but not social,behavior is corrected.These data suggest that there may beCell 155,1451–1463,December 19,2013ª2013Elsevier Inc.1457。
The Gut Microbiota and the Development of the Brain
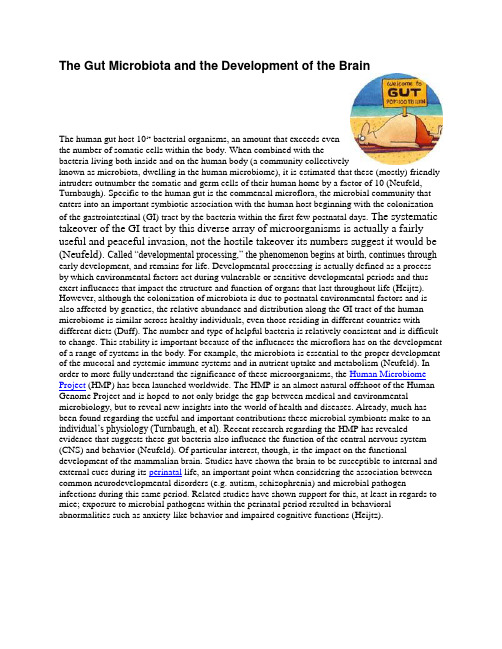
The Gut Microbiota and the Development of the BrainThe human gut host 1014 bacterial organisms, an amount that exceeds eventhe number of somatic cells within the body. When combined with thebacteria living both inside and on the human body (a community collectivelyknown as microbiota, dwelling in the human microbiome), it is estimated that these (mostly) friendlyintruders outnumber the somatic and germ cells of their human home by a factor of 10 (Neufeld, Turnbaugh). Specific to the human gut is the commensal microflora, the microbial community thatenters into an important symbiotic association with the human host beginning with the colonization of the gastrointestinal (GI) tract by the bacteria within the first few postnatal days. The systematic takeover of the GI tract by this diverse array of microorganisms is actually a fairlyuseful and peaceful invasion, not the hostile takeover its numbers suggest it would be(Neufeld). Called “developmental processing,” the phenomenon begins at birth, continues throughearly development, and remains for life. Developmental processing is actually defined as a process by which environmental factors act during vulnerable or sensitive developmental periods and thus exert influences that impact the structure and function of organs that last throughout life (Heijtz). However, although the colonization of microbiota is due to postnatal environmental factors and is also affected by genetics, the relative abundance and distribution along the GI tract of the human microbiome is similar across healthy individuals, even those residing in different countries with different diets (Duff). The number and type of helpful bacteria is relatively consistent and is difficult to change. This stability is important because of the influences the microflora has on the development of a range of systems in the body. For example, the microbiota is essential to the proper development of the mucosal and systemic immune systems and in nutrient uptake and metabolism (Neufeld). In order to more fully understand the significance of these microorganisms, the Human Microbiome Project (HMP) has been launched worldwide. The HMP is an almost natural offshoot of the Human Genome Project and is hoped to not only bridge the gap between medical and environmental microbiology, but to reveal new insights into the world of health and diseases. Already, much has been found regarding the useful and important contributions these microbial symbionts make to an individual’s physiology (Turnbaugh, et al). Rec ent research regarding the HMP has revealed evidence that suggests these gut bacteria also influence the function of the central nervous system (CNS) and behavior (Neufeld). Of particular interest, though, is the impact on the functional development of the mammalian brain. Studies have shown the brain to be susceptible to internal and external cues during its perinatal life, an important point when considering the association between common neurodevelopmental disorders (e.g. autism, schizophrenia) and microbial pathogen infections during this same period. Related studies have shown support for this, at least in regards to mice; exposure to microbial pathogens within the perinatal period resulted in behavioral abnormalities such as anxiety-like behavior and impaired cognitive functions (Heijtz).Gut FeelingsThe relationship between the gut microflora and the brain, the so-called gut-brain axis, has been an important component for many years in gastrointestinal research. It is especially influential in such functional bowel disorders as Irritable Bowel Syndrome (IBS) and may play a part in ulcers. However, as with IBS, most of the studies have focused on a brain-to-gut control, a type of top-down relationship that examines mainly the impact of the brain on the function of the gut. New research in this field has also revealed that there may be close ties between the microbiota of the gut and the development and function of the central nervous system (CNS). This, in turn, has led to a discovery of a link between early life stress, altered stress activity later in life, and a change in the landscape of the microflora, all examined using the top-down approach. Further studies demonstrated the influence of microbiota over energy balance and their integral connections to the pathophysiology of obesity. Energy balance and the intake of food are both centrally mediated processes, and although the direct link between the gut microbiome and the central feeding circuits has not been discovered yet, it reveals the presence of the alternative bottom-up control, where the microbiota have an influence over the development and functionality of the brain. This discovery of bottom-up controlswill be important in the research regarding the relationship between brain development and the establishment of the gut microbiota (Neufeld). It also brings into light the possible role of the human microbiome in the incidence of neurodevelopmental disorders (Heijtz)./image.axd?id=b2487c81-5d3e-4bdc-a4bb-8b7102af2b5e&t=634164293398800000Evidence in MiceIn 2006, immunologist Sven Pettersson of the Karolinska Institute in Stockholm developed an interest in the bottom-up approach to the gut-brain axis when he and genomicistShugui Wang of the Genome Institute of Singapore discovered that the key brain chemical was regulated by the microbes in the gut (Pennisi). This finding led him to collaboration with a neurobiologist at the Karolinska Institute, Rochellys Diaz Heijtz , in order to further study the effects of gut microbiota on brain development and function using mice (Heigtz).For the study, they engineered germ-free (GF) mice that had no commensal intestinal microflora and therefore an underdeveloped immune system and specific pathogen free (SPF) mice with normal gut microbiota and put them through a series of tests in order to observe their exploratory activity and anxiety. Such tests included placing the mice in an open field box and measuring their spontaneous motor activity for an hour. Another test that assessed whether nonpathogenic gut microbiota could affect anxiety-like behavior involved both a light-dark box test and an elevated plus maze. In every case, the GF mice what are considered riskier behaviors, spending more time in the light box and in the open arms or end of the open arms of the elevated plus maze than the SPF mice. They also generated more spontaneous activity, traveling a greater total distance with more exploration of the open field than the SPF mice in the open field box. However, when the same battery of tests were performed with adult conventionalized offspring (CON) (offspring of GF mice that were exposed to the microbial pathogens early in their development), the CON mice displayed similar tendencies to the SPF mice instead of their GF parents. In order to check that this normalization of behavior patterns takes place only during a sensitive or critical period wherein the effects of the normal gut microbiota can occur, Heijtz et. al. then conventionalized adult GF mice and retested them. The new findings of the conventionalized adult GF mice showed no significant changes, displaying a failure to normalize their behavior at this late stage (Heijtz). Dr. Heijtz inferred from this data “that there is a critical period early in l ife when gut microorganisms affect the brain and change behavior in later life” (Pennisi).nerve-cell (Pennisi, Heijtz).This study clearly supports the hypothesis that the gut microbiota can have a significant impacton normal brain development and behavioral functions during a critical period early in the postnatal growth that lasts throughout life. It suggests also that during the course of evolution, microbiota colonization became an integral part of the development and programming of the brain with its effects extending to motor control and anxiety-like behaviors (Heijtz). However, Pettersson cautions that “it is importa nt to note that this knowledge can be applied only to mice, and that it is too early to say anything about the effect of gut bacteria on the human brain” (“Bacteria in the gut…”).Evidence in AutismEven with such cautions, it is hard to ignore the implications to the world of neurodevelopmental disorders. In fact, many GI disorders have shown a high comorbidity with psychiatric illnesses (Neufeld). The most widely hoped for link is between the gut microbiota and schizophrenia or autism. Children with autistic spectrum disorders, particularly, demonstrate a high incidence of severe gastrointestinal problems, combined with an onset in early childhood, perhaps linking to the critical period discovered by Heijtz et al (Parracho). In addition to several bodily and behavioral changes and difficulties, there often is an inflammation of the intestinal lining, causing the gut to be more permeable. Some of these symptoms have even been linked to changes in the gut microbiota due to treatment with antibiotics (Duff).Several studies have been done hoping to find an association between the microbiota and autism in order to begin finding a cause and then a cure. One such study tested 86 children with autism and revealed a substantial change in the metabolism of amino acids and in the normal microorganisms that inhabit the gut of a healthy person. These major differences were mostly concerned with the aerobic bacteria Escherichia coli (E. coli) and Enterococcus, and with the anaerobic bacteria Bacteroides and Bifidobacteria. E. coli is the most common aerobe of the microflora, accounting for around 80-90% of all aerobic bacteria. The second, then,is Enterococcus which accounts for just 5% of aerobes. When interpreting the results of the Bioscreenfaecal microbiology, it was found that the average amount of E. coli had considerably decreased, accounting for only 56% instead of its usual 90%. In 18 of the children, the amount of E. coli was actually less than 10%. Conversely, the amount of Enterococcus had risen to 40%, with a complete swap of the two aerobes in 19% of the children, as Enterococcus accounted for 95% of the aerobic bacteria. Relatedly, the anaerobes changed as well. There was a measurable decreasein Bacteroides whereas the Bifidobacteria increased (Duff).This study, however, is very basic and does not take into account many variables that would have to be considered in further examination of the role of the gut microorganisms in autism. For example, repeated microbial use (such as those commonly taken for autism) could cause disruptions in the indigenous microflora of the gut, accounting for some of the discrepencies. As Pettersson stated, at this point in the game, it is far too early to make any assumptions about the significance of the microbiota in the development of the human brain. In the future, who knows what will be discovered? Perhaps we will just have to listento our guts.References1. Duff, Jacques. "Autism and ADHD: Intestinal Dysbiosis." ADHD,ADD, AttentionDeficit Hyperactivity Disorder. BehaviouralNeurotherapy Clinic. Web.<.au/Intestinal_Dysbiosis.htm>.2. Heijtz, Rochellys Diaz, Shugui Wang, FarhanaAnuar, Yu Qian, Britta Björkholm,Annika Samuelsson, Martin L. Hibberd, Hans Forssberg, and SvenPettersson."Normal gut microbiota modulates brain development andbehavior." PNAS 108.7 (2011): 3047-052. PNAS: Proceedings of the NationalAcademy of Sciences of the United States of America. National Academy of Sciences,31 Jan. 2011. Web.</content/early/2011/01/26/1010529108.full.pdf+html>.3. KarolinskaInstitutet. "Bacteria in the gut may influence braindevelopment." ScienceDaily, 1 Feb. 2011. Web.4. Neufeld, BSc, Karen-Anne, and Jane A. Foster, PhD. "Effects of gut microbiota onthe brain: implications for psychiatry." Journal of Psychiatry & Neuroscience 34.3 (2009): 230-31. PubMed Central. Canadian Medical Association, May 2009. Web.</pmc/articles/PMC2674977/>.5. Parracho, H. M. "Differences between the gut microflora of children with autisticspectrum disorders and that of healthy children." Journal of MedicalMicrobiology 54.10 (2005): 987-91. . National Center for Biotechnology Information, U.S. National Library of Medicine, Aug. 2005. Web.</pubmed/16157555>.6. Pennisi, Elizabeth. "Do Gut Bugs Practice Mind Control?" Science/AAAS | News - Upto the Minute News and Features from Science. American Association for theAdvancement of Science, 31 Jan. 2011. Web.</sciencenow/2011/01/do-gut-bugs-practice-mind-contro.html>.7. Turnbaugh, Peter J., Ruth E. Ley, Micah Hamady, Claire M. Fraser-Liggett, RobKnight, and Jeffrey I. Gordon. "The Human Microbiome Project." Nature 449.7164 (2007): 804-10. Nature. Nature Publishing Group, 17 Oct. 2007. Web.</nature/journal/v449/n7164/abs/nature06244.html>.。
Bifurcation in epidemic models
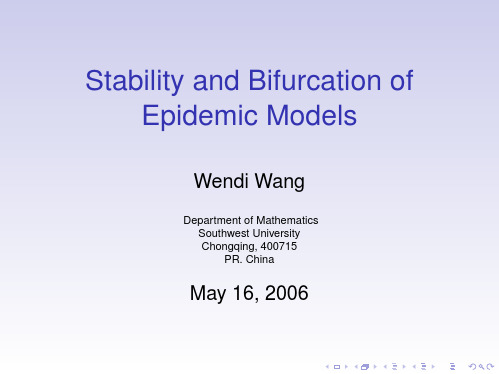
(7)
(8)
Bifurcations in an SIRS Model (cont.)
where p= α(d + ν) , k d +γ m= , d +ν A = N0 q= k , d +ν
γ . d +ν
Saddle-Node Bifurcation
(0, 0) is an equilibrium. This is a disease free equilibrium. It is always stable. According to the formula from van den Driessche and Watmough (Math. Biosci,2002), we can calculate the reproduction number of this model and obtain R0 = 0. we should know further details of dynamical behaviors: When is the disease persistent? When does the disease die out?
SN Continue (cont.)
I ’ = I2 (A − I − R)/(1 + p I2) − m I R’=qI−R A = 11.5 p = 0.2 q = 4.2 m=5
7
6
5
4 R 3 2 1 0 0 0.5 1 1.5 I 2 2.5 3
Hopf Bifurcation
E1 is a saddle, impossible for a Hopf bifurcation, E2 is a node or a focus, it is possible to have a Hopf bifurcation, a necessary condition is that the trace of the Jacobian matrix at E2 is zero. The sign of the trace is determined by
频闪视觉训练在体育运动中的应用现状及发展趋势研究
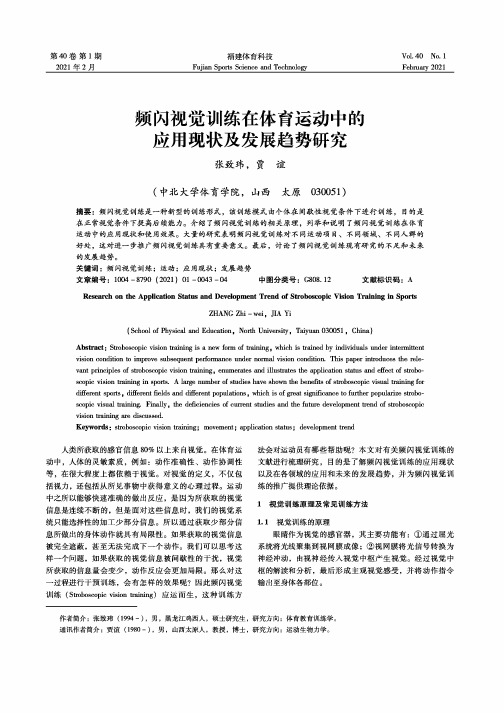
第40卷第1期2021年2月福建体育科技Fujian Sports Science and TechnologyVol.40No.1February2021频闪视觉训练在体育运动中的应用现状及发展趋势研究张致玮,贾谊(中北大学体育学院,山西太原030051)摘要:频闪视觉训练是一种新型的训练形式,该训练模式由个体在间歇性视觉条件下进行训练,目的是在正常视觉条件下提高后续能力。
介绍了频闪视觉训练的相关原理,列举和说明了频闪视觉训练在体育运动中的应用现状和使用效果。
大量的研究表明频闪视觉训练对不同运动项目、不同领域、不同人群的好处,这对进一步推广频闪视觉训练具有重要意义。
最后,讨论了频闪视觉训练现有研究的不足和未来的发展趋势。
关键词:频闪视觉训练;运动;应用现状;发展趋势文章编号:1004-8790(2021)01-0043-04中图分类号:G808.12文献标识码:A Research on the Application Status and Development Trend of Stroboscopic Vision Training in SportsZHANG Zhi-wei,JIA Yi(School of Physical and Education,North University,Taiyuan030051,China)Abstract:Stroboscopic vision training is a new form of training,which is trained by individuals under intermittent vision condition to improve subsequent performance under normal vision condition.This paper introduces the relevant principles of stroboscopic vision training,enumerates and illustrates the application status and effect of stroboscopic vision training in sports.A large number of studies have shown the benefits of stroboscopic visual training for different sports,different fields and different populations,which is of great significance to further popularize stroboscopic visual training.Finally,the deficiencies of current studies and the future development trend of stroboscopic vision training are discussed.Keywords:stroboscopic vision training;movement;application status;development trend人类所获取的感官信息80%以上来自视觉。
工业机器人刚度的辨识方法与性能分析
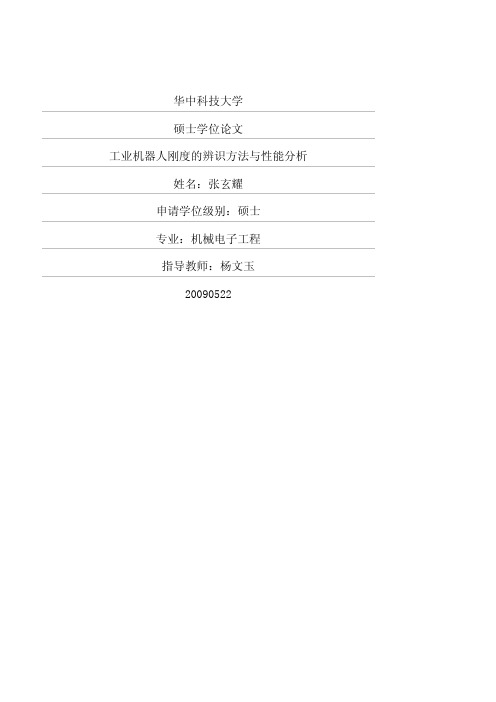
1
华中科技大学硕士学位论文
人的弹性变形主要体现在组成关节的传动部件上。 机器人关节变形的一个直接影响是引起末端的静态变形误差。在机器人的自重和外
部负载的作用下,各关节会发生相应的变形,而这种变形又会累积到机器人的末端。对 串联机器人,这种作用尤为明显。机器人关节变形的另一个影响是引起末端的动态变形 误差,即振荡,从而降低了机器人的动态性能。当机器人高速运行时,这种影响较为显 著。因此,若要提高机器人的位置精度和动态性能,必须减小关节变形的影响。
柔性机器人的研究初期,研究者们主要集中在柔性连杆的研究上。柔性连杆机器人 的建模方法主要分为三种:集中参数法、有限元法和假设模态法。
集中参数法[21,22]包括集中质量法和集中刚度法。集中质量模型将机械臂简化为集中 质量和无质量的弹性连杆,集中刚度模型则将机械臂简化为由弹簧连接的刚性杆。这两 种方法大大简化了计算,可以很方便地写出系统动力学方程。但由于简化过多,这两种 方法都很不完善,有待进一步的研究。
除了对位置精度和动态性能的不良影响之外,目前,弹性变形已在某些场合作为一 项有利因素加以应用。对于刚性机器人,其位置控制已经得到了充分的研究[4],但由于 实际中经常需要机器人与环境接触,而环境的位置往往具有不确定性,因此传统的位置 控制难以满足任务要求。以自动装配为例,当装配机器人与零件的容差大于装配间隙时, 装配将难以顺利完成。在这一场合,要求机器人在与装配零件的接触处受到作用力时, 能发生相应的变形,这种性能被称为顺应。顺应性的实现有两种方式,包括主动顺应和 被动顺应。通过在机器人的控制中采用一定的控制算法,实现机器人末端的操作刚度, 这种方式为主动顺应[5]。由于弹性是机械结构的固有特性,通过机械结构(包括连杆, 关节,末端执行器)的设计,可以使机器人的末端具有一定的操作刚度,这种方式称之 为被动顺应[6]。在主动顺应中,为使其控制更稳定,比如提高抗干扰能力[7]和稳定力反 馈环[8],往往会加入被动顺应[9]。
桦褐孔菌多糖的提取 功能特性及结构表征研究进展
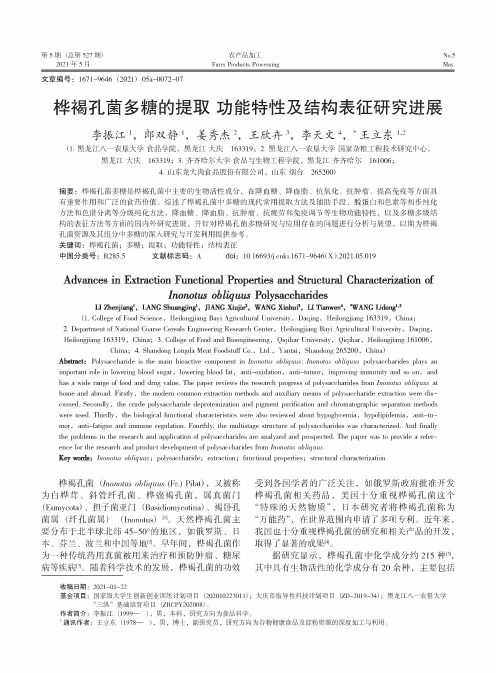
第5期(总第527期)2021年5月农产品加工Farm Products ProcessingNo.5May.文章编号:1671-9646(2021) 05a-0072-07桦褐孔菌多糖的提取功能特性及结构表征研究进展李振江1,郎双静蔦姜秀杰2,王欣卉3,李天文4, **王立东2收稿日期:2021-01-22基金项目:国家级大学生创新创业训练计划项目(202010223011);大庆市指导性科技计划项目(ZD-2019-34);黑龙江八一农垦大学"三纵”基础培育项目(ZRCPY202008)。
作者简介:李振江(1999—),男,本科,研究方向为食品科学。
*通讯作者:王立东(1978—),男,博士,副研究员,研究方向为谷物健康食品及淀粉资源的深度加工与利用。
(1.黑龙江八一农垦大学食品学院,黑龙江大庆163319; 2.黑龙江八一农垦大学国家杂粮工程技术研究中心,黑龙江大庆163319; 3.齐齐哈尔大学食品与生物工程学院,黑龙江齐齐哈尔161006;4.山东龙大肉食品股份有限公司,山东烟台265200)摘要:桦褐孔菌多糖是桦褐孔菌中主要的生物活性成分,在降血糖、降血脂、抗氧化、抗肿瘤、提高免疫等方面具有重要作用和广泛的食药价值。
综述了桦褐孔菌中多糖的现代常用提取方法及辅助手段,脱蛋白和色素等初步纯化方法和色谱分离等分级纯化方法,降血糖、降血脂、抗肿瘤、抗疲劳和免疫调节等生物功能特性,以及多糖多级结 构的表征方法等方面的国内外研究进展,并针对桦褐孔菌多糖研究与应用存在的问题进行分析与展望,以期为桦褐 孔菌资源及其组分中多糖的深入研究与开发利用提供参考。
关键词:桦褐孔菌;多糖;提取;功能特性;结构表征中图分类号:R285.5 文献标志码:A doi : 10.16693/ki.1671-9646(X ).2021.05.019Advances in Extraction Functional Properties and Structural Characterization ofInonotus obliquus PolysaccharidesLI Zhenjiang 1, LANG Shuangjing 1, JIANG Xiujie 2, WANG Xinhui 3, LI Tianwen 4, *W ANG Lidong 1,2(1. College of Food Science , Heilongjiang Bayi Agricultural University , Daqing , Heilongjiang 163319, China ;2. Department of National Coarse Cereals Engineering Research Center , Heilongjiang Bayi Agricultural University , Daqing , Heilongjiang 163319, China ; 3. College of Food and Bioengineering , Qiqihar University , Qiqihar , Heilongjiang 161006,China ; 4. Shandong Longda Meat Foodstuff Co., Ltd., Yantai , Shandong 265200, China)Abstract : Polysaccharide is the main bioactive component in Inonotus obliquus . Inonotus obliquus polysaccharides plays animportant role in lowering blood sugar , lowering blood fat , anti-oxidation , anti-tumor , improving immunity and so on , and has a wide range of food and drug value. The paper reviews the research progress of polysaccharides from Inonotus obliquus at home and abroad. Firstly , the modern common extraction methods and auxiliary means of polysaccharide extraction were dis cussed. Secondly , the crude polysaccharide deproteinization and pigment purification and chromatographic separation methods were used. Thirdly , the biological functional characteristics were also reviewed about hypoglycemia , hypolipidemia , anti-tu-mor , anti-fatigue and immune regulation. Fourthly, the multistage structure of polysaccharides was characterized. And finally the problems in the research and application of polysaccharides are analyzed and prospected. The paper was to provide a refer ence for the research and product development of polysaccharides from Inonotus obliquus .Key words : Inonotus obliquus ; polysaccharide ; extraction ; functional properties ; structural characterization桦褐孔菌(Inonotus obliquus (Fr.) Pilat),又被称 为白桦茸、斜管纤孔菌、桦癌褐孔菌,属真菌门 (Eumycota )、担子菌亚门(Basidiomycotina )、褐卧孔 菌属(纤孔菌属)(Inonotus )叫天然桦褐孔菌主要分布于北半球北纬45~50°的地区,如俄罗斯、日 本、芬兰、波兰和中国等地叫早年间,桦褐孔菌作 为一种传统药用真菌被用来治疗和预防肿瘤、糖尿 病等疾病叫随着科学技术的发展,桦褐孔菌的功效受到各国学者的广泛关注,如俄罗斯政府批准开发 桦褐孔菌相关药品,美国十分重视桦褐孔菌这个 “特殊的天然物质”,日本研究者将桦褐孔菌称为 “万能药”,在世界范围内申请了多项专利。
Gut Microbiota Imbalance
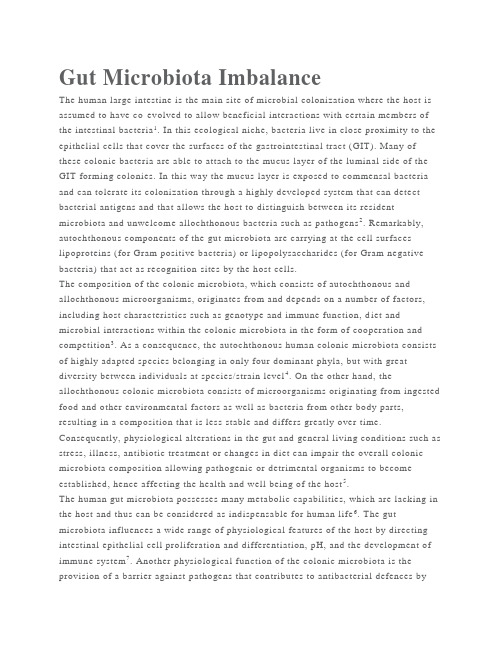
Gut Microbiota ImbalanceThe human large intestine is the main site of microbial colonization where the host is assumed to have co-evolved to allow beneficial interactions with certain members of the intestinal bacteria1. In this ecological niche, bacteria live in close proximity to the epithelial cells that cover the surfaces of the gastrointestinal tract (GIT). Many of these colonic bacteria are able to attach to the mucus layer of the luminal side of the GIT forming colonies. In this way the mucus layer is exposed to commensal bacteria and can tolerate its colonization through a highly developed system that can detect bacterial antigens and that allows the host to distinguish between its resident microbiota and unwelcome allochthonous bacteria such as pathogens2. Remarkably, autochthonous components of the gut microbiota are carrying at the cell surfaces lipoproteins (for Gram positive bacteria) or lipopolysaccharides (for Gram negative bacteria) that act as recognition sites by the host cells.The composition of the colonic microbiota, which consists of autochthonous and allochthonous microorganisms, originates from and depends on a number of factors, including host characteristics such as genotype and immune function, d iet and microbial interactions within the colonic microbiota in the form of cooperation and competition3. As a consequence, the autochthonous human colonic microbiota consists of highly adapted species belonging in only four dominant phyla, but with great diversity between individuals at species/strain level4. On the other hand, the allochthonous colonic microbiota consists of microorganisms originating from ingested food and other environmental factors as well as bacteria from other body parts, resulting in a composition that is less stable and differs greatly over time. Consequently, physiological alterations in the gut and general living conditions such as stress, illness, antibiotic treatment or changes in diet can impair the overall colonic microbiota composition allowing pathogenic or detrimental organisms to become established, hence affecting the health and well being of the host5.The human gut microbiota possesses many metabolic capabilities, which are lacking in the host and thus can be considered as indispensable for human life6. The gut microbiota influences a wide range of physiological features of the host by directing intestinal epithelial cell proliferation and differentiation, pH, and the development of immune system7. Another physiological function of the colonic microbiota is the provision of a barrier against pathogens that contributes to antibacterial defences byproducing antibacterial peptides8. Moreover, the gut microbiota is capable of modulating the intestinal epithelium of the host. In model systems involving human cell lines it has been noted that an appropriate balance of the microbiota induces mucin gene expression, that acts as a barrier and at the same time provides nutrients, like saccharides, for bacterial growth thereby promoting intestinal colonization of the adhering autochthonous bacteria, which helps reduce the chances of host invasion by pathogens9.Due to all the above-mentioned properties of the microbiota, there is a strong incentive for the host to control the shaping of the microbiota through the action of its immune system10. In fact, it has been demonstrated that an imbalance of the intestinal microbiota can lead to local and/or systemic disease state11. The interaction between the gut microbiota and host may therefore allow different kinds of relationships, such as commensalism, mutualism, symbiosis and pathogenicity.ReferencePalmer C, Bik EM, Digiulio DB, Relman DA, Brown PO (2007) Development of the Human Infant Intestinal Microbiota. PLoSBiol5:e177Ley RE, Peterson DA, Gordon JI (2006) Ecological and evolutionary forces shaping microbial diversity in the human intestine. Cell 124:837–848Xu J (2006) Microbial ecology in the age of genomics and metagenomics: concepts, tools, and recent advances. MolEcol 15:1713–1731Ventura M, Canchaya C, Tauch A, Chandra G, Fitzgerald GF, Chater KF, van Sinderen D (2007) Genomics of Actinobacteria: tracing the evolutionary history of an ancient phylum. MicrobiolMolBiol Rev 71:495–548Macpherson AJ, Harris NL. Interactions between commensal intestinal bacteria and the immune system. Nat Rev Immunol 4:478–485, 2004.Savage DC (2001) Microbial biota of the human intestine: a tribute to some pioneering scientists. Curr Issues IntestMicrobiol 2:1–15Backhed F, Ley RE, Sonnenburg JL, Peterson DA, Gordon JI (2005) Host-bacterial mutualism in the human intestine. Science 307:1915–1920Lievin-Le Moal V, Servin AL (2006) The front line of enteric host defense against unwelcome intrusion of harmful microorganisms: mucins, antimicrobial peptides, and microbiota. ClinMicrobiol Rev 19:315–337Destoumieux-Garzon D, Peduzzi J, Rebuffat S (2002) Focus on modified microcins: structural features and mechanisms of action. Biochimie 84:511–519Ley RE, Backhed F, Turnbaugh P, Lozupone CA, Knight RD, Gordon JI (2005) Obesity alters gut microbial ecology. Proc Natl AcadSci U S A 102:11070–11075Ley RE, Turnbaugh PJ, Klein S, Gordon JI (2006) Microbial ecology: human gut microbes associated with obesity. Nature 444:1022–1023。
高通量自动化类器官芯片研究进展
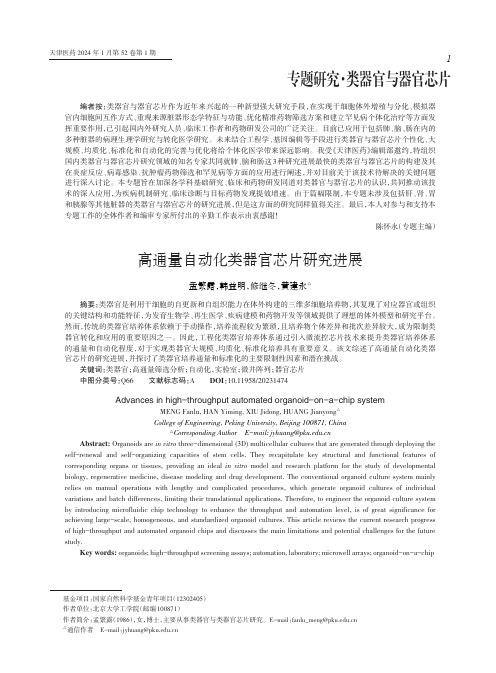
天津医药2024年1月第52卷第1期1专题研究·类器官与器官芯片编者按:类器官与器官芯片作为近年来兴起的一种新型强大研究手段,在实现干细胞体外增殖与分化、模拟器官内细胞间互作方式、重现来源脏器形态学特征与功能、优化精准药物筛选方案和建立罕见病个体化治疗等方面发挥重要作用,已引起国内外研究人员、临床工作者和药物研发公司的广泛关注。
目前已应用于包括肺、脑、肠在内的多种脏器的病理生理学研究与转化医学研究。
未来结合工程学、基因编辑等手段进行类器官与器官芯片个性化、大规模、均质化、标准化和自动化的完善与优化将给个体化医学带来深远影响。
我受《天津医药》编辑部邀约,特组织国内类器官与器官芯片研究领域的知名专家共同就肺、脑和肠这3种研究进展最快的类器官与器官芯片的构建及其在炎症反应、病毒感染、抗肿瘤药物筛选和罕见病等方面的应用进行阐述,并对目前关于该技术待解决的关键问题进行深入讨论。
本专题旨在加深各学科基础研究、临床和药物研发同道对类器官与器官芯片的认识,共同推动该技术的深入应用,为疾病机制研究、临床诊断与目标药物发现提效增速。
由于篇幅限制,本专题未涉及包括肝、肾、胃和胰腺等其他脏器的类器官与器官芯片的研究进展,但是这方面的研究同样值得关注。
最后,本人对参与和支持本专题工作的全体作者和编审专家所付出的辛勤工作表示由衷感谢!陈怀永(专题主编)高通量自动化类器官芯片研究进展孟繁露,韩益明,修继冬,黄建永△摘要:类器官是利用干细胞的自更新和自组织能力在体外构建的三维多细胞培养物,其复现了对应器官或组织的关键结构和功能特征,为发育生物学、再生医学、疾病建模和药物开发等领域提供了理想的体外模型和研究平台。
然而,传统的类器官培养体系依赖于手动操作,培养流程较为繁琐,且培养物个体差异和批次差异较大,成为限制类器官转化和应用的重要原因之一。
因此,工程化类器官培养体系通过引入微流控芯片技术来提升类器官培养体系的通量和自动化程度,对于实现类器官大规模、均质化、标准化培养具有重要意义。
银耳多糖对人软骨细胞的增殖效应和抗炎作用
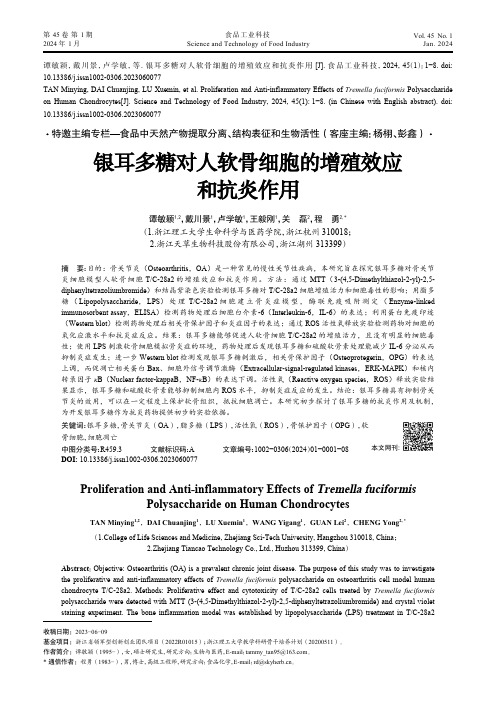
谭敏颖,戴川景,卢学敏,等. 银耳多糖对人软骨细胞的增殖效应和抗炎作用[J]. 食品工业科技,2024,45(1):1−8. doi:10.13386/j.issn1002-0306.2023060077TAN Minying, DAI Chuanjing, LU Xuemin, et al. Proliferation and Anti-inflammatory Effects of Tremella fuciformis Polysaccharide on Human Chondrocytes[J]. Science and Technology of Food Industry, 2024, 45(1): 1−8. (in Chinese with English abstract). doi:10.13386/j.issn1002-0306.2023060077· 特邀主编专栏—食品中天然产物提取分离、结构表征和生物活性(客座主编:杨栩、彭鑫) ·银耳多糖对人软骨细胞的增殖效应和抗炎作用谭敏颖1,2,戴川景1,卢学敏1,王毅刚1,关 磊2,程 勇2, *(1.浙江理工大学生命科学与医药学院,浙江杭州 310018;2.浙江天草生物科技股份有限公司,浙江湖州 313399)摘 要:目的:骨关节炎(Osteoarthritis ,OA )是一种常见的慢性关节性疾病,本研究旨在探究银耳多糖对骨关节炎细胞模型人软骨细胞T/C-28a2的增殖效应和抗炎作用。
方法:通过MTT (3-(4,5-Dimethylthiazol-2-yl)-2,5-diphenyltetrazoliumbromide )和结晶紫染色实验检测银耳多糖对T/C-28a2细胞增殖活力和细胞毒性的影响;用脂多糖(Lipopolysaccharide ,LPS )处理T/C-28a2细胞建立骨炎症模型,酶联免疫吸附测定(Enzyme-linked immunosorbent assay ,ELISA )检测药物处理后细胞白介素-6(Interleukin-6,IL-6)的表达;利用蛋白免疫印迹(Western blot )检测药物处理后相关骨保护因子和炎症因子的表达;通过ROS 活性氧释放实验检测药物对细胞的氧化应激水平和抗炎症反应。
Method and apparatus for computer modeling of the

专利名称:Method and apparatus for computermodeling of the interaction between andamong cortical and subcortical areas in thehuman brain for the purpose of predictingthe effect of drugs in psychiatric andcognitive diseases发明人:Hugo Geerts,Athan Spiros申请号:US13412626申请日:20120306公开号:US08332158B2公开日:20121211专利内容由知识产权出版社提供专利附图:摘要:A computer model of a diseased human brain includes inputs representing a drug and outputs representing the clinical effect of that drug on psychiatric and cognitive diseases. Diseases that can be modeled include psychiatric disorders, such as schizophrenia, bipolar disorder, major depression, ADHD, autism, obsessive-compulsive disorder, substance abuse and cognitive deficits therein and neurological disorders such as Alzheimer's disease, Mild Cognitive impairment, Parkinson's disease, stroke, vascular dementia, Huntington's disease, epilepsy and Down syndrome. The computer model preferably uses the biological state of interactions between and among cortico and subcortical areas of the human brain, to define the biological processes related to the biological state of the generic synapse model, the striatum, Locus Coeruleus, Dorsal raphe, hippocampus, amygdala and cortex, as well as certain mathematical relationships related to interactions among biological variables associated with the biological processes and to correlations between the biological variables and clinical effects on a clinical scale.申请人:Hugo Geerts,Athan Spiros地址:Berwyn PA US,Portland OR US 国籍:US,US代理机构:Berliner & Associates更多信息请下载全文后查看。
国际上著名的从事药剂学研究的专家

Intra Oral Delivery (口腔内传递)直接由口腔黏膜吸收,瞬间进入血液循环,有效成分不流失。
Universities, Departments,FacultiesResearchersButler University College of Pharmacy and Health Sciences Health Sciences USA Associate Professor Nandita G. DasMain focus on her research facilities are about peformulation, biopharmaceutics, drug targeting, anticancer drug delivery.Purdue University School of Pharmacy and Pharmacal Sciences Department of Industrial and Physical Pharmacy (IPPH) USA Professor Kinam ParkControlled Drug Delivery, Glucose-Sensitive Hydrogels for Self-Regulated Insulin Delivery, Superporous Hydrogel Composites, Oral Vaccination using Hydrogel Microparticles, Fractal Analysis of Pharmaceutical Solid Materials.St. John's University School of Pharmacy and Allied Health ProfessionsUSA Professor Parshotam L. MadanControlled and targeted drug delivery systems; Bio-erodible polymers as drug delivery systemsThe University of Iowa College of Dentistry Department of Oral Pathology, Radiology, and Medicine USA Professor Christopher A. Squierpermeability of skin, and oral mucosa to exogenous substances, including alcohol and tobacco, and drug deliveryThe University of Iowa College of Pharmacy Department of Pharmaceutics USA Associate Professor Maureen D. DonovanMucosal drug delivery especially via the nasal, gastrointestinal and vaginal epithelia; and mechanisms of drug absorption and disposition.The University of Texas at San Antonio College of Engineering Department of Biomedical Engineering USA Professor Jeffrey Y. ThompsonDental restorative materials and implantsThe University of Utah Pharmaceutics & Pharmaceutical Chemistry USA Professor John W. MaugerDr. Maugner is mainly focused on dissolution testing and coating technology of orally administered drug products with bitter taste about which he is one of the inventors of a filed patent.University of Kentucky College of Pharmacy Pharmaceutical Sciences USA Professor Peter CrooksDr. Crooks is internationally known for his research work in drug discovery, delivery, and development, which includes drug design and synthesis, pharmacophore development, drug biotransformation studies, prodrug design, and medicinal plant natural product research. His research also focuses on preclinical drug development, including drug metabolism and pharmacokinetics in animal models, dosage form development, and drug delivery assessment using both conventional and non-conventional routes, and preformulation/formulation studies.Associate Professor Russell MumperDr. Mumper's main research areas are thin-films and mucoadhesive gels for (trans)mucosal delivery of drugs, microbicides, and mucosal vaccines, and nanotemplate engineering of nano-based detection devices and cell-specific nanoparticles for tumor and brain targeting, gene therapy and vaccines.West Virginia University School of Pharmacy Department of Basic Pharmaceutical Sciences USA Associate Professor Paula Jo Meyer StoutDr. Stout's research areas are composed of dispersed pharmaceutical systems, sterile product formulation DDS for dental diseases and coating of sustained release formulations.Monash University Victorian College of Pharmacy Department of Pharmaceutics Australia Professor Barrie C. FinninTransdermal Drug Delivery. Physicochemical Characterisation of Drug Candidates. Topical Drug Delivery. Drug uptake by the buccal mucosaProfessor Barry L. ReedTransdermal Drug Delivery. Topical Drug Delivery. Formulation of Dental Pharmaceuticals.University of Gent Faculty of Pharmaceutical Sciences Department of Pharmaceutics Belgium Professor Chris Vervaet-Extrusion/spheronisation - Bioadhesion - Controlled release based on hot stage extrusion technology - Freeze-drying - Tabletting and - GranulationPh.D. Els AdriaensMucosal drug delivery (Vaginal and ocular) Nasal BioadhesionUniversity of Gent Faculty of Pharmaceutical SciencesLaboratory of Pharmaceutical Technology Belgium Professor Jean Paul Remonbioadhesive carriers, mucosal delivery, Ocular bioerodible minitablets, Compaction of enteric-coated pellets; matrix-in-cylinder system for sustained drug delivery; formulation of solid dosage forms; In-line monitoring of a pharmaceutical blending process using FT-Raman spectroscopy; hot-melt extruded mini-matricesDanish University of Pharmaceutical Sciences Department of Pharmaceutics Denmark Associate Professor Jette JacobsenLow soluble drugs ?in vitro lymphatic absorption Drug delivery to the oral cavity ?in vitro models (cell culture, diffusion chamber) for permeatbility and toxicity of drugs, in vivo human perfusion model, different formulation approaces, e.g. iontophoresis.。
Biopsychosocial model
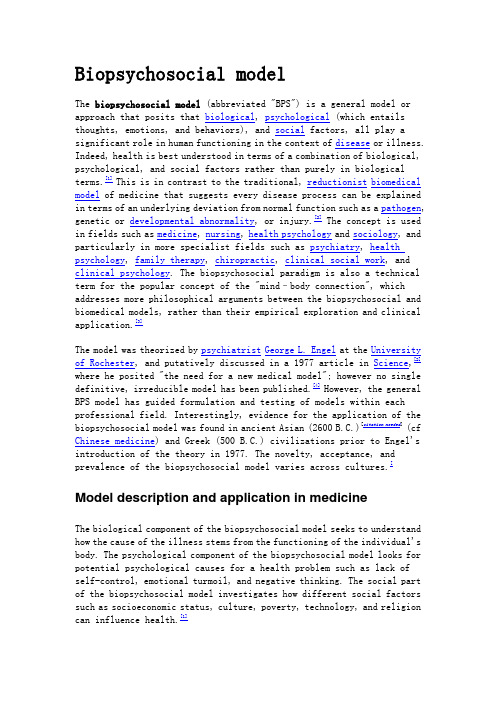
Biopsychosocial modelThe biopsychosocial model (abbreviated "BPS") is a general model or approach that posits that biological, psychological (which entails thoughts, emotions, and behaviors), and social factors, all play a significant role in human functioning in the context of disease or illness. Indeed, health is best understood in terms of a combination of biological, psychological, and social factors rather than purely in biological terms.[1]This is in contrast to the traditional, reductionist biomedical model of medicine that suggests every disease process can be explained in terms of an underlying deviation from normal function such as a pathogen, genetic or developmental abnormality, or injury.[2] The concept is used in fields such as medicine, nursing, health psychology and sociology, and particularly in more specialist fields such as psychiatry, health psychology, family therapy, chiropractic, clinical social work, and clinical psychology. The biopsychosocial paradigm is also a technical term for the popular concept of the "mind–body connection", which addresses more philosophical arguments between the biopsychosocial and biomedical models, rather than their empirical exploration and clinical application.[3]The model was theorized by psychiatrist George L. Engel at the University of Rochester, and putatively discussed in a 1977 article in Science,[2] where he posited "the need for a new medical model"; however no single definitive, irreducible model has been published.[4]However, the general BPS model has guided formulation and testing of models within each professional field. Interestingly, evidence for the application of the biopsychosocial model was found in ancient Asian (2600 B.C.)[citation needed](cf Chinese medicine) and Greek (500 B.C.) civilizations prior to Engel's introduction of the theory in 1977. The novelty, acceptance, and prevalence of the biopsychosocial model varies across cultures.[Model description and application in medicineThe biological component of the biopsychosocial model seeks to understand how the cause of the illness stems from the functioning of the individual's body. The psychological component of the biopsychosocial model looks for potential psychological causes for a health problem such as lack of self-control, emotional turmoil, and negative thinking. The social part of the biopsychosocial model investigates how different social factors such as socioeconomic status, culture, poverty, technology, and religion can influence health.[1]The biopsychosocial model of health is based in part on social cognitive theory. The biopsychosocial model implies that treatment of disease processes, for example type two diabetes and cancer, requires that the health care team address biological, psychological and social influences upon a patient's functioning. In a philosophical sense, the biopsychosocial model states that the workings of the body can affect the mind, and the workings of the mind can affect the body.[5]This means both a direct interaction between mind and body as well as indirect effects through intermediate factors.The biopsychosocial model presumes that it is important to handle the three together as a growing body of empirical literature suggests that patient perceptions of health and threat of disease, as well as barriers in a patient's social or cultural environment, appear to influence the likelihood that a patient will engage in health-promoting or treatment behaviors, such as medication taking, proper diet or nutrition, and engaging in physical activity.[6]While operating from a BPS framework requires that more information be gathered during a consultation, a growing trend in US healthcare (and already well-established in Europe such as in the U.K. & Germany) includes the integration of professional services through integrated disciplinary teams, to provide better care and address the patient's needs at all three levels.[7] As seen, for example in integrated primary care clinics, such as used in the U.K., Germany, U.S. Veteran's Administration, U.S. military, Kaiser Permanente, integrated teams may comprise physicians, nurses, health psychologists, social workers, and other specialties to address all three aspects of the BPS framework, allowing the physician to focus on predominantly biological mechanisms of the patient's complaints[7]See also[8]Psychosocial factors can cause a biological effect by predisposing the patient to risk factors. An example is that depression by itself may not cause liver problems, but a depressed person may be more likely to have alcohol problems, and therefore liver damage. Perhaps it is this increased risk-taking that leads to an increased likelihood of disease. Most diseases in BPS discussion are such behaviourally-moderated illnesses, with known high risk factors, or so-called "biopsychosocialillnesses/disorders".[9][10] An example of this is type 2 diabetes, which with the growing prevalence of obesity and physical inactivity, is on course to become a worldwide pandemic. For example, approximately 20 million Americans are estimated to have diabetes, with 90% to 95% considered type 2.[11]It is important to note that the biopsychosocial model does not provide a straightforward, testable model to explain the interactions or causal influences (that is, amount of variance accounted for) by each of the components (biological, psychological, or social). Rather, the model has been a general framework to guide theoretical and empirical exploration, which has amassed a great deal of research since Engel's 1977 article. One of the areas that has been greatly influenced is the formulation and testing of social-cognitive models of health behavior over the past 30 years.[12] While no single model has taken precedence, a large body of empirical literature has identified social-cognitive (the psycho-social aspect of Engel's model) variables that appear to influence engagement in healthy behaviors and adhere to prescribed medical regimens, such as self-efficacy, in chronic diseases such as type 2 diabetes, cardiovascular disease, etc.[13][14] These models include the Health Belief Model, Theory of Reasoned Action and Theory of Planned Behavior, Transtheoretical Model, the Relapse Prevention Model, Gollwitzer's implementation-intentions, the Precaution–Adoption Model, the Health Action Process Approach, etcCriticismSome critics point out this question of distinction and of determination of the roles of illness and disease runs against the growing concept of the patient–doctor partnership or patient empowerment, as "biopsychosocial" becomes one more disingenuous euphemism for psychosomatic illness.[20] This may be exploited by medical insurance companies or government welfare departments eager to limit or deny access to medical and social care.[21]Some psychiatrists see the BPS model as flawed, in either formulation or application. Epstein and colleagues describe six conflicting interpretations of what the model might be, and proposes that "...habits of mind may be the missing link between a biopsychosocial intent and clinical reality."[22] Psychiatrist Hamid Tavakoli argues that the BPS model should be avoided because it unintentionally promotes an artificial distinction between biology and psychology, and merely causes confusion in psychiatric assessments and training programs, and that ultimately it has not helped the cause of trying to destigmatize mental health.[23]Sociologist David Pilgrim suggests that a necessary pragmatism and a form of "mutual tolerance" (Goldie, 1977) has forced a co-existence of perspectives, rather than a genuine "theoretical integration as a shared BPS orthodoxy."[24] Pilgrim goes on to state that despite "scientific and ethical virtues," the BPS model "...has not been properly realised. Itseems to have been pushed into the shadows by a return to medicine and the re-ascendancy of a biomedical model."[25]However, a vocal philosophical critic of the BPS model, psychiatrist Niall McLaren,[26] writes:"Since the collapse of the 19th century models (psychoanalysis, biologism and behaviourism), psychiatrists have been in search of a model that integrates the psyche and the soma. So keen has been their search that they embraced the so-called 'biopsychosocial model' without ever bothering to check its details. If, at any time over the last three decades, they had done so, they would have found it had none. This would have forced them into the embarrassing position of having to acknowledge that modern psychiatry is operating in a theoretical vacuum."[27]The rationale for this theoretical vacuum is outlined in his 1998 paper[28] and more recently in his books, most notably Humanizing Psychiatrists.[29] Simply put, the purpose of a scientific model is to see if a scientific theory works and to actualize its logical consequences. In this sense, models are real and their material consequences can be measured, whereas theories are ideas and can no more be measured than daydreams.Model-building separates theories with a future from those that always remain dreams. An example of a true scientific model is longer necked giraffes reach more food, survive at higher rates, and pass on this longer neck trait to their progeny. This is a model (natural selection) of the theory of evolution. Therefore, from an epistemological stance there can be no model of mental disorder without first establishing a theory of the mind. Dr. McLaren does not say that the biopsychosocial model is devoid of merit, just that it does not fit the definition of a scientific model (or theory) and does not "reveal anything that would not be known (implicitly, if not explicitly) to any practitioner of reasonable sensitivity." He states that the biopsychosocial model should be seen in a historical context as bucking against the trend of biological reductionism, which was (and still is) overtaking psychiatry. Engel "has done a very great service to orthodox psychiatry in that he legitimised the concept of talking to people as people." In short, even though it is correct to say that sociology, psychology, and biology are factors in mental illness, simply stating this obvious fact does not make it a model in the scientific sense of the word.。
含有乳清蛋白水解物和磷脂的乳状液行为
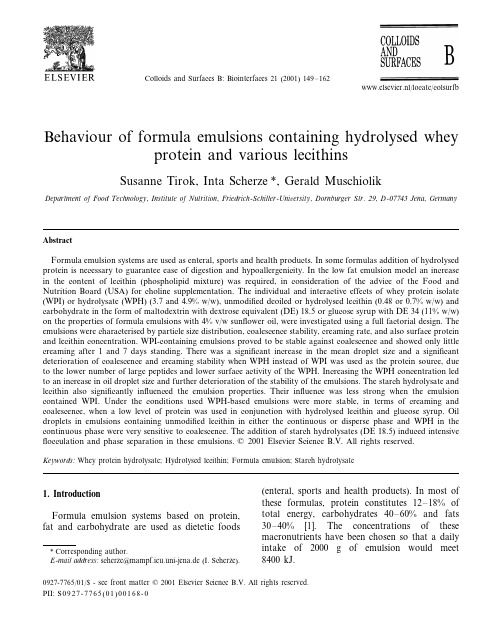
Colloids and Surfaces B:Biointerfaces21(2001)149–162Behaviour of formula emulsions containing hydrolysed wheyprotein and various lecithinsSusanne Tirok,Inta Scherze*,Gerald MuschiolikDepartment of Food Technology,Institute of Nutrition,Friedrich-Schiller-Uni6ersity,Dornburger Str.29,D-07743Jena,GermanyAbstractFormula emulsion systems are used as enteral,sports and health products.In some formulas addition of hydrolysed protein is necessary to guarantee ease of digestion and hypoallergenicity.In the low fat emulsion model an increase in the content of lecithin(phospholipid mixture)was required,in consideration of the advice of the Food and Nutrition Board(USA)for choline supplementation.The individual and interactive effects of whey protein isolate (WPI)or hydrolysate(WPH)(3.7and4.9%w/w),unmodified deoiled or hydrolysed lecithin(0.48or0.7%w/w)and carbohydrate in the form of maltodextrin with dextrose equivalent(DE)18.5or glucose syrup with DE34(11%w/w) on the properties of formula emulsions with4%v/w sunflower oil,were investigated using a full factorial design.The emulsions were characterised by particle size distribution,coalescence stability,creaming rate,and also surface protein and lecithin concentration.WPI-containing emulsions proved to be stable against coalescence and showed only little creaming after1and7days standing.There was a significant increase in the mean droplet size and a significant deterioration of coalescence and creaming stability when WPH instead of WPI was used as the protein source,due to the lower number of large peptides and lower surface activity of the WPH.Increasing the WPH concentration led to an increase in oil droplet size and further deterioration of the stability of the emulsions.The starch hydrolysate and lecithin also significantly influenced the emulsion properties.Their influence was less strong when the emulsion contained WPI.Under the conditions used WPH-based emulsions were more stable,in terms of creaming and coalescence,when a low level of protein was used in conjunction with hydrolysed lecithin and glucose syrup.Oil droplets in emulsions containing unmodified lecithin in either the continuous or disperse phase and WPH in the continuous phase were very sensitive to coalescence.The addition of starch hydrolysates(DE18.5)induced intensive flocculation and phase separation in these emulsions.©2001Elsevier Science B.V.All rights reserved. Keywords:Whey protein hydrolysate;Hydrolysed lecithin;Formula emulsion;Starch hydrolysatewww.elsevier.nl/locate/colsurfb1.IntroductionFormula emulsion systems based on protein, fat and carbohydrate are used as dietetic foods (enteral,sports and health products).In most of these formulas,protein constitutes12–18%of total energy,carbohydrates40–60%and fats 30–40%[1].The concentrations of these macronutrients have been chosen so that a daily intake of2000g of emulsion would meet 8400kJ.*Corresponding author.E-mail address:scherze@mampf.ieu.uni-jena.de(I.Scherze).0927-7765/01/$-see front matter©2001Elsevier Science B.V.All rights reserved. PII:S0927-7765(01)00168-0S.Tirok et al./Colloids and Surfaces B:Biointerfaces21(2001)149–162 150The carbohydrates are usually glucose polymers in the form of starch or its hydrolysates[1]. Starch hydrolysates are ideal,since they lead to no or only a small increase in viscosity,so that the emulsion stays drinkable or may be provided by feeding tubes.In this study,a commercial maltodextrin(DE=18.5)and dried glucose syrup (DE=34)were used to determine the influence of the degree of starch hydrolysis on the emulsion behaviour.It is sometimes desirable or even necessary to use proteins in a hydrolysed form to guarantee ease of digestion and hypoallergenicity.A degree of hydrolysis of23–29%,as in the chosen protein, represents a product mainly composed of di-and tripeptides.Peptides of this size have very low antigenicity[2]and therefore might be used for persons with milk protein allergy or to prevent development of milk allergy,especially in young children[2,3].Furthermore,extensively hy-drolysed protein is more easily digested because the constituent di-and tripeptides can be ab-sorbed without further enzymatic hydrolysis[4]. It is generally accepted that mild hydrolysis is beneficial and that extensive hydrolysis,due to production of many short peptides,is detrimental to emulsifying and stabilising properties[5].The use of an extensively hydrolysed protein as N-source in a formula emulsion is,therefore,not sufficient for stabilisation.Hence,a second sur-face active molecule has to be added to support emulsion formation.Lecithins(mixtures of phospholipids)are emulsifiers of natural origin that are widely used in pharmaceutical and food emulsion systems. Their high phosphatidylcholine content means that they represent a good source of choline. Choline is thought to be a vitamin-like substance which,according to recent research,may be essen-tial under certain circumstances[6,7].A lack of choline may lead to fat accumulation in the liver, necrosis of liver cells and even liver cancer[8–10]. When the formulation is the only nutrient source, an increase in the phospholipid content is re-quired in order to meet the requirements of the Food and Nutrition Board[11]for an adequate intake of choline.The choline content of the lecithin in the investigated formula emulsions is adequate to give about500mg choline in2000g of emulsion.Many studies have been carried out on the interaction and competition between lecithin and intact protein[12–14].Little is known though about systems containing extensively hydrolysed protein and modified lecithin(enriched with lyso-phospholipids).Recently,Agboola et al.[15]reported that the stability of emulsions formed using highly hy-drolysed whey proteins can be improved by addi-tion of hydroxylated lecithin.The aim of this work was to investigate the influence of unmodified and lyso-phospholipid-en-riched lecithin on the behaviour of dietetic for-mula emulsions in the presence of hydrolysed whey protein,taking into account the balance of macronutrient and an increased cholin content.2.Materials and methods2.1.Materials2.1.1.ProteinWhey protein isolate(WPI)was supplied by NZMP(Germany)GmbH and whey protein hy-drolysate(WPH)was obtained from Arla Foods Ingredients amba(Denmark)(see Table1for specification).2.1.2.Soy bean lecithinThe deoiled soybean lecithin(PL)and the hy-drolysed soybean lecithin(hPL—deoiled lecithin enriched with lyso-phospholipids)were con-tributed by Lucas Meyer GmbH(Germany).The composition is summarised in Table2.2.1.3.Starch hydrolysis productsThe two starch hydrolysates were provided by Cerestar Deutschland GmbH(Germany). Maltodextrin(dextrose equivalent DE18.5)con-tained84%higher saccharides,9%maltotriose, 6%maltose and1%dextrose.Glucose syrup(DE 34)consisted of52%higher saccharides,22% maltotriose,25%maltose and1%dextrose.Sunflower oil,containing4.8%polar lipids,was donated by W.Rau Lebensmittelwerke GmbH (Germany).S.Tirok et al./Colloids and Surfaces B:Biointerfaces21(2001)149–162151Table1Composition and molecular characteristics of the whey protein samples aCharacteristics WPHWPIProtein(%)Min.7693.4Carbohydrate(%)Max.7.5B1.0Max.0.2B1.0Fat(%)Max.4.7 Ash(%) 1.6Max.6.04.0Moisture(%)23–29 Degree of hydrolysis(%)––910Mean molecular weight(based onweight at214nm,Da)–Mean molecular weight(based on545 number at214nm,Da)Peptide distribution(based onnumber)1–3residues(%)49–32–4–6residues(%)–7–10residues(%)134–11–20residues(%)–\20residues(%)1a All data from technical bulletin.2.3.Analysis of the emulsions2.3.1.Measurement of particle sizesDroplet sizes d32(surface weighted average di-ameter)and d43(volume weighted average diame-ter)were measured in the freshly prepared emulsion,then after1and again after7days,by means of a laser diffraction particle analyser Coul-ter LS100(Micro Volume Module Cell,Coulter Electronics,USA).The measurement was repeated using a dissociating medium(0.2%w/v SDS, Merck-Schuchardt,Germany)which dispersed the aggregates formed during emulsification.It was verified that the use of SDS on unflocculated emulsions did not significantly affect the droplet size.Consequently,SDS only acted as a dissociat-ing agent of aggregates.This procedure was used to obtain the so-called apparent(measurement in water)and real(measurement in SDS-solution) mean droplet diameters[16].2.3.2.Coalescence stability of the oil droplets The coalescence was expressed as the ratio of oil droplet surface area calculated from d32measured in SDS-solution after1day and the oil droplet surface area of the freshly made emulsion,also measured in SDS-solution.2.3.3.Determination of creaming beha6iourA10-ml sample of each emulsion was poured into graduated tubes.The volume of the separated cream layer in each tube was recorded after24h storage at20°C,then after centrifugation(1000×g for10min)and also after heating(90°C for10 min).A second tube for each emulsion was stored at7°C for7days,after which time the volume of the separated cream layer was determined.2.2.Preparation of emulsionProtein,lecithin and the starch hydrolysate weredispersed together in water for least1h.To thisdispersion,0.02%w/w sodium azide(FlukaChemie AG,Switzerland)was added,in order toenhance storage stability.Oil-in-water emulsionscontaining4%v/w sunflower oil were prepared bypremixing all the ingredients with a shear mixerCAT X620(T17,8000rev.min−1,1min,M.Zipperer GmbH,Germany),followed by emulsifi-cation using a high pressure homogeniser(Emulsi-Flex C5,Avestin,Canada)at70MPa.Allemulsion experiments were performed in duplicate.Table2Composition(%)of the lecithin samplesLyso-lecithin b Sample Moisture aAcetone insolubles a Oil a PC b PI b PE b PA bMax.1.5Min.97 3.54 Deoiled lecithin 4.8116.513.221.8Max.1.514.33.3812.813.416.9Max.3Min.95Max.2Deoiled hydrolysed lecithina Data from technical bulletin.b Manufacturer’s data(NMR analysis,lyso-lecithin comprises LPC,LPI,LPE and LPA,data in weight%).S.Tirok et al./Colloids and Surfaces B:Biointerfaces21(2001)149–162152Table3Factors analysed in the experimental designLevel1(−)Level2(+)FactorHydrolysateX1IsolateWhey proteinX2Protein concentration a 3.7g/100g 4.9g/100gHydrolysedX3UnmodifiedLecithin0.48g/100gLecithin concentration a0.70g/100gX4Maltodextrin(DE18.5)X5Dried glucose syrup(DE34) Starch hydrolysatea Concentration per emulsion.2.3.4.Determination of surface protein concentrationAfter separation of cream from emulsions by centrifugation,the serum portion was carefully discarded and the cream layer was washed by resuspending in an imidazole buffer(20mM,pH 7.00)according to Sharma and Dalgleish[17].The resuspended cream was recentrifuged under the same conditions.The protein load,expressed as mg protein per m2fat surface area,was calculated from the amount of protein present in the washed cream layers after centrifugation of the emulsion, and the fat surface area.The fat surface area was calculated from d32measured using a Coulter LS 100with the Micro Volume Module Cell.Protein content was determined by the method of Lowry et al.[18]using bovine serum albumin as standard.2.3.5.Determination of surface phospholipid concentrationThe phospholipid content of the cream layer was determined by measuring the phosphorus content in the lipid extract[19].The fat was extracted from the cream layer with a2:1(by volume)chloroform/methanol solution.After evaporation and drying,5M sulphuric acid solu-tion was added to the fat.The whole mixture was then digested using an oven(4–6h,160°C).After treatment with hydrogen peroxide,the total phos-phorus oxidised to phosphate in the digest was measured at830nm using the colorimetric phos-phomolybdate reaction[20].This gave the phos-phorus content,from which the phospholipid content was calculated by multiplying the phos-phorus content by a factor of31.7,as recom-mended for soy lecithin[21].2.4.Experimental design and statistical analysisA full factorial design withfive factors at two levels(Table3)was used to investigate the influ-ence of different whey proteins,lecithins and starch products on the properties of the formula emulsion system.The data were analysed with commercial software(Trial Run1.0,SPSS).The regression procedure of the statistical program was used to generate regression coefficients,anal-ysis of variance and correlation coefficients for the model.The level of significance was defined at P50.05.3.Results3.1.Influence of the protein usedThe type of protein(X1—WPI or WPH) significantly influenced the properties of the emul-sions containing lecithin and carbohydrate(Ta-bles4and5).The use of WPI led to small oil droplets,which gave nearly normal distribution, with their maximum at1m m.The distribution did not change after storage,indicating coalescence stability of the oil droplets.Emulsions made with WPI showed very low cream layers( 1ml/100ml)after24h standing time,independent of the lecithin or carbohydrate used(Table6).Hence no coalescence was ob-served,the cream layers only slightly increasing after7days standing time.Emulsions made with WPI(3.7or 4.9%w/w)and maltodextrin,but without any lecithin,showed no significant differ-ence in oil droplet size,but displayed somewhat larger cream layers(data not shown).S .Tirok et al ./Colloids and Surfaces B :Biointerfaces 21(2001)149–162153Table 4Analysis of variance for responses of the mean oil droplet diameter (d 43),coalescence factor and creaming after 24h adfMean droplet diameter d 43Coalescence FactorCreaming after 24h Type I sum of squaresF -ratio P -value Type I sum of squares F -ratio P -value Type I sum of squares F -ratio P -value 1 1.8213.670.003X 10.3334.560.000 1.0182.780.00010.040.270.614X 20.1010.350.0070.1411.740.00510.030.200.6620.05X 3 4.920.047B 0.010.010.819X 410.20 1.490.2460.01 1.120.3100.000.00 1.000X 51 2.9522.140.0010.03 3.200.0990.07 5.940.0311 1.4010.490.0070.12X 1×X 212.400.0040.1915.650.0021 1.198.960.0110.1212.08X 1×X 30.0050.010.860.37310.070.520.4860.01X 1×X 4 1.060.3240.000.00 1.000X 1×X 51 1.168.740.0120.1211.960.0050.1613.420.003X 2×X 310.050.380.549B 0.010.070.8020.010.880.36810.16 1.180.300B 0.01X 2×X 40.260.6390.010.120.73810.16 1.230.2890.06 5.690.034X 2×X 50.07 5.940.0311 1.108.300.014B 0.01X 3×X 40.180.6760.000.00 1.000X 3×X 510.60 4.530.055B 0.010.020.9010.000.00 1.0001X 4×X 50.030.260.623B 0.010.100.7530.010.510.4870.8730.891r 20.920aStatistical analysis of the full factorial design with Trial Run 1.0(SPSS).All signi ficant effects (individual and interaction)are highlighted.The level of signi ficance was de fined at P 50.05.X 1,type of protein;X 2,protein concentration;X 3,type of lecithin;X 4,lecithin concentration;X 5,type of starch hydrolysate.S .Tirok et al ./Colloids and Surfaces B :Biointerfaces 21(2001)149–162154Emulsions made with 3.7or 4.9%w /w WPI formed a gel-like structure during heating at 90°C for 10min because of protein denaturation which was independent of the lecithin and carbohydrate used.In most cases,emulsions containing WPH showed signi ficantly larger oil droplets (d 32:1.5–2.5m m and d 43:2–4m m)than those with WPI (Fig.1).The use of WPH in emulsions resulted in increased cream layers after 24h standing time,compared to those with WPI (Table 6),and showed oiling-off,which was caused by the low coalescence stability (coalescence factor B 1,Fig.2).The statistical analysis showed that the protein load is signi ficantly reduced when using WPH in place of WPI.Fig.3demonstrates that there are only two exceptions.On the other hand,the lecithin load was signi ficantly higher in emulsions containing WPH compared to those made with WPI.3.2.In fluence of the protein concentrationThe concentration of the protein (X 2—3.7or 4.9%w /w)was found to have an important in flu-ence on the properties of the formula emulsions (Tables 4and 5).Increasing the WPI concentration from 3.7to 4.9%w /w signi ficantly reduced the mean oil droplet diameter (d 43)(Fig.1a,b)of emulsions containing the unmodi fied lecithin and led to higher protein load values in these emulsions (Fig.3a,b),but had no effect on the stability (creaming rate)(Table 6).Emulsions made with the low WPH concentra-tion gave rise to smaller cream layers (Table 6)and signi ficantly higher coalescence stability (Fig.2),in comparison to emulsions made with the high WPH concentration.Increasing the WPH concentration led to higher protein load values independent of the lecithin and carbohydrate used (Fig.3c,d).Table 5Analysis of variance for responses of the protein and lecithin load at the interface adfProtein load at the interface Lecithin load at the interfaceP -value F -ratio Type I sum of squares P -value F -ratio Type I sum of squares131.8532.14X 10.000237.5778.000.00010.96010.9211.020.006B 0.01X 2B 0.0130.430.0001X 30.960.970.34492.70 5.060.0441X 40.660.670.43115.410.1672.176.600.135X 52.572.5510.730.4101X 1×X 2 1.22 1.230.288 2.2217.187.24X 1×X 30.020139.9345.940.0000.0099.7329.650.943X 1×X 4B 0.01B 0.0111 5.08 5.13X 1×X 50.0430.720.240.6350.020.8821X 2×X 3 1.51 1.530.2400.071B 0.010.01X 2×X 40.913 1.610.530.481X 2×X 510.690.700.4190.170.050.8200.1402.502.481X 3×X 40.0118.9827.361 2.20X 3×X 50.7092.230.150.450.162X 4×X 510.540.4780.533.081.000.3360.938r 20.851aStatistical analysis of the full factorial design with Trial Run 1.0(SPSS).All signi ficant effects (individual and interaction)are highlighted.The level of signi ficance was de fined at P 50.05.X 1,type of protein;X 2,protein concentration;X 3,type of lecithin;X 4,lecithin concentration;X 5,type of starch hydrolysate.S.Tirok et al./Colloids and Surfaces B:Biointerfaces21(2001)149–162155 Table6Influence of the various whey proteins,lecithins and starch hydrolysis products on the creaming behaviour of the emulsions after 24h aProtein Creaming(ml/100ml emulsion)Starch hydrolysateType Concentration Unmodified lecithin(PL)Hydrolysed lecithin(hPL)0.48%w/w0.70%w/w0.48%w/w0.70%w/w WPI 3.7%w/w md111B1gs11114.9%w/w md B1B111gs1111md–bWPH–b3.7%w/w24gs4c2c3d2dmd–b–b4.9%w/w1010gs6c5c4d3da md,maltodextrin(DE18.5);gs,dried glucose syrup(DE34).b Breaking straight after preparation.c Breaking after2days.d Oiling-off.3.3.Influence of the lecithinThe type of lecithin(X3—deoiled unmodified or deoiled hydrolysed lecithin)was very important in determining the properties of the formula emul-sion studied.The lecithin concentration(X4—0.48%w/w and0.7%w/w)however was not an important influential factor on the emulsion be-haviour(Tables4and5).The significant influence of the interactive effect (X1×X3)indicates that the effect of factor X3 depends on the level of factor X1(type of protein). The type of lecithin had less effect on the WPI-stabilised emulsions.The use of an unmodified deoiled,rather than a hydrolysed,lecithin in WPI-stabilised emulsions only led to a slight increase in oil droplet size,especially at the lower protein concentration(3.7%w/w).Emulsions made with WPH were considerably less stable,with respect to coalescence and cream-ing,when unmodified rather than hydrolysed lecithin was added to the aqueous phase(Table 6).The use of unmodified lecithin under the con-ditions used,induced the breaking of emulsions containing WPH straight after preparation,when maltodextrin was the carbohydrate,or after2 days storage,when glucose syrup was used.Sup-plementary experiments showed that the stability could not be improved when the unmodified de-oiled lecithin was dispersed in the oil phase and the emulsification was performed at60°C(data not shown).3.4.Influence of the starch hydrolysateThe starch hydrolysates(X5—maltodextrin with DE18.5or glucose syrup with DE34)also strongly influenced the properties of the formula emulsions(Tables4and5).The significant influ-ence of the interactive effect(X1×X5)indicates that the effect of factor X5depends on the level of factor X1(type of protein).Under the conditions used,the type of starch hydrolysate had no significant effect on the parti-cle size(Fig.1a,b)and creaming behaviour(Table 6)of emulsions containing WPI with either lecithin.The protein load of emulsions made with WPI and unmodified lecithin(PL)was increased when maltodextrin was used instead of glucose syrup(Fig.3a,b).In contrast,WPH-containing emulsions were highly influenced,in terms of oil droplet size(Fig. 1c,d)and emulsion stability(i.e.creaming and oiling-off)(Table6)by the type of starch hy-S.Tirok et al./Colloids and Surfaces B:Biointerfaces21(2001)149–162 156drolysate pared to emulsions without polysaccharide,these emulsions showed increased oil droplets(Fig.4)and hence very strong cream-ing.Rapid phase separation by creaming,oiling-off andflocculation(interaction of components in the bulk aqueous phase,no dropletflocculation), due to the carbohydrate,was especially prevalent when unmodified lecithin was added.Flocculation was not observed when WPI was used in the emulsion.Fig.1.Mean oil droplet diameter(d43)in emulsions prepared with4%v/w sunflower oil and4.9%w/w( )or3.7%w/w( ) protein.(A)Emulsions containing WPI,dried glucose syrup(gs)and hydrolysed(hPL)or unmodified(PL)lecithin;(B)emulsions containing WPI,maltodextrin(md)and hydrolysed(hPL)or unmodified(PL)lecithin;(C)emulsions containing WPH,dried glucose syrup(gs)and hydrolysed(hPL)or unmodified(PL)lecithin;(D)emulsions containing WPH,maltodextrin(md)and hydrolysed (hPL)or unmodified(PL)lecithin.See Table3for lecithin and starch hydrolysate concentration.S.Tirok et al./Colloids and Surfaces B:Biointerfaces21(2001)149–162157 Fig.2.Coalescence factor of emulsions containing4%v/w sunflower oil,11%w/w starch hydrolysate(md,maltodextrin;gs,glucose syrup),whey protein hydrolysate(4.9%w/w WPH+,3.7%w/w WPH−)and lecithin.Hatchedfill pattern,0.48%w/w deoiled unmodified lecithin;nofill pattern,0.70%w/w deoiled unmodified lecithin;dottedfill;0.48%w/w hydrolysed lecithin;solidfill, 0.70%w/w hydrolysed lecithin.4.DiscussionThe results show that the properties of the investigated dietetic emulsions are highly depen-dent on the type of protein used and that WPH-containing emulsions are also influenced by the type of lecithin and carbohydrate used.Oil droplets in emulsions made with WPI proved to be stable against coalescence,indepen-dent of the lecithins and carbohydrates used. Since the model WPI emulsion had a small amount of oil droplets larger than1m m and a rather low viscosity,slight creaming was induced. The high percentage of protein in the emulsions with WPI led to a high protein load,indicating that multiple layers around the oil droplets were formed.The higher interface viscosity and elastic-ity,as has been described for b-lactoglobulin[22], a major component of WPI,enhanced the inter-face stability and prevented coalescence.Positive interactions between the WPI and the lecithin (plexation)at the interface can further increase the stabilising effect[23].In the case of emulsions containing WPH,the addition of lecithin was expected to compensate for the poor emulsifying capacity of this protein. Compared to emulsions prepared only with lecithin,however,the combination of unmodified or hydrolysed lecithin with WPH led to a deterio-ration of emulsion stability,giving emulsions with separated oil and increased cream layer.Due to its high degree of hydrolysis,the WPH mainly consists of short peptides,free amino acids and only a limited concentration of surface-active large peptides.The reasons behind the low emulsi-fying capacity of such a WPH are either due to the peptides having a high hydrophilicity,and so adsorb weakly or not at all at the interface,or due to such peptides not forming a sufficiently strong interfacialfilm[24].Although small peptides dif-fuse rapidly,they are less efficient in reducing the interfacial tension,because they cannot unfold and re-orientate at the interface[25].Similarity in molecular size means that lecithin and the pep-tides may reach the interface at the same time, thus suggesting that there is competitive adsorp-tion between these components at the oil droplet surface,so preventing an even lecithinfilm forma-tion.As the measurement of the protein and lecithin adsorption from emulsions containingS.Tirok et al./Colloids and Surfaces B:Biointerfaces21(2001)149–162 158WPH and unmodified or hydrolysed lecithin has shown,both the protein and lecithin were present at the surface of fat pared to WPI-stabilised emulsions,the lecithin load at the inter-face was significantly higher for WPH emulsions, whilst the protein load was much lower.The preferential adsorption of lecithin over WPH pep-tides may produce a reduction in electrostatic and steric repulsion,so promoting coalescence[15]. Adsorption and desorption processes possibly lead to a non-continuousfilm at the interface, which promotes re-coalescence of the oil droplets. In the presence of the starch hydrolysates, emulsions made with WPH and hydrolysed lecithin were slightly more stable than those with WPH and unmodified lecithin.Other studies us-Fig.3.Protein load at the interface of oil droplets in emulsions prepared with4%v/w sunflower oil and4.9%w/w( )or3.7%w/w ( )protein.See Fig.1for further composition.Fig.4.Influence of different carbohydrates on the oil droplet size distribution in emulsions containing4%v/w sunflower oil,3.7% w/w WPH and0.70%w/w hydrolysed lecithin:( )without starch hydrolysate;( )with11%w/w dried glucose syrup;( )with 11%w/w maltodextrin.ing WPH and lecithins also achieved better emul-sion stability with a more hydrophilic lecithin(in that case hydroxylated)[15,26].In contrast to our study,they did notfind any phospholipid at the interface when using the more hydrophilic lecithin.Increasing the WPH concentration in emulsions with added lecithin and carbohydrate,resulted in more unstable emulsions,although the protein surface load significantly increased because of the higher total number of surface-active substances. This contradicts other studies made with a com-parable extensively hydrolysed WPH[15,27],that found better coalescence stability with increasing WPH concentration,although it has to be taken into consideration that their emulsions did not contain lecithin and carbohydrate.In addition to the peptide chain length,the hydrophobicity of the resulting peptides,which is determined by the protease specificity,is an important factor that influences their emulsifying properties[28].Nega-tive interactions between the peptides and lecithin may also increase with higher WPH concentra-tions,thus hindering lecithin adsorbance at the interface.As reported recently,the mechanical stability of adsorbed layers,formed from such a whey protein hydrolysate with a high degree of hydrolysis,is weak,and cannot be stabilised by lyso-PC[29]. An excess of lyso-PC leads to a slight improve-ment,but only to reach the mechanical stability of layers for lyso-PC,which by itself is very small compared to b-lactoglobulin[29].When using a high concentration of small molecular surfactants as the emulsifier,the forma-tion of multilayers of a lamellar liquid crystalline phase may enhance stability[30].Although the lecithin concentration in the studied WPH emul-sions was high(12or17.5%w/v with regard to the oil phase),the WPH components adsorbed at the interface probably produced‘holes’in the lecithin layer,which therefore could not form an ordered structure.Adding the WPH after emul-sification could not prevent such instabilities,so suggesting that a lamellar layer of lecithin is either not formed,or is not strong enough to impede the penetration of the surface-active WPH components.The addition of maltodextrin(DE18.5)or glu-cose syrup(DE34)caused an increase in oil droplet size and strong creaming.This was partic-ularly noted where maltodextrin was the starch hydrolysate,where it is known that protein–maltodextrin interactions cause a lowering in the。
外骨骼可穿戴机器人 BLEEX
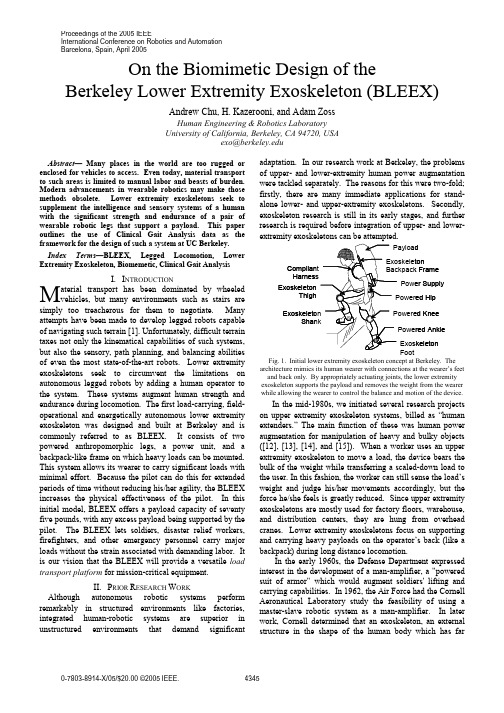
Human Engineering & Robotics Laboratory University of California, Berkeley, CA 94720, USA exo@
Abstract— Many places in the world are too rugged or enclosed for vehicles to access. Even today, material transport to such areas is limited to manual labor and beasts of burden. Modern advancements in wearable robotics may make those methods obsolete. Lower extremity exoskeletons seek to supplement the intelligence and sensory systems of a human with the significant strength and endurance of a pair of wearable robotic legs that support a payload. This paper outlines the use of Clinical Gait Analysis data as the framework for the design of such a system at UC Berkeley. Index Terms—BLEEX, Legged Locomotion, Lower Extremity Exoskeleton, Biomemetic, Clinical Gait Analysis
基于血清瘦素
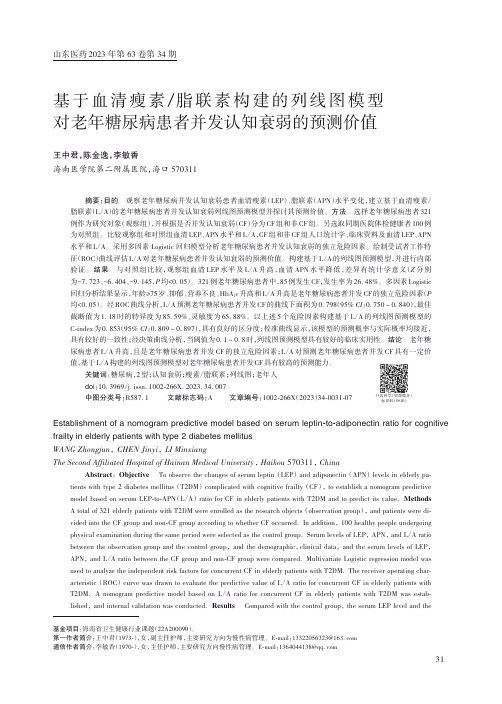
基于血清瘦素/脂联素构建的列线图模型对老年糖尿病患者并发认知衰弱的预测价值王中君,陈金逸,李敏香海南医学院第二附属医院,海口 570311摘要:目的 观察老年糖尿病并发认知衰弱患者血清瘦素(LEP )、脂联素(APN )水平变化,建立基于血清瘦素/脂联素(L /A )的老年糖尿病患者并发认知衰弱列线图预测模型并探讨其预测价值。
方法 选择老年糖尿病患者321例作为研究对象(观察组),并根据是否并发认知衰弱(CF )分为CF 组和非CF 组。
另选取同期医院体检健康者100例为对照组。
比较观察组和对照组血清LEP 、APN 水平和L /A ,CF 组和非CF 组人口统计学、临床资料及血清LEP 、APN 水平和L /A 。
采用多因素 Logistic 回归模型分析老年糖尿病患者并发认知衰弱的独立危险因素。
绘制受试者工作特征(ROC )曲线评估L /A 对老年糖尿病患者并发认知衰弱的预测价值。
构建基于L /A 的列线图预测模型,并进行内部验证。
结果 与对照组比较,观察组血清LEP 水平及L /A 升高,血清APN 水平降低,差异有统计学意义(Z 分别为-7.723、-6.404、-9.145,P 均<0.05)。
321例老年糖尿病患者中,85例发生CF ,发生率为26.48%。
多因素Logistic 回归分析结果显示,年龄≥75岁、抑郁、营养不良、HbA 1c 升高和L /A 升高是老年糖尿病患者并发CF 的独立危险因素(P 均<0.05)。
经ROC 曲线分析,L /A 预测老年糖尿病患者并发CF 的曲线下面积为0.798(95% CI :0.750~0.840),最佳截断值为1.18时的特异度为85.59%、灵敏度为65.88%。
以上述5个危险因素构建基于L /A 的列线图预测模型的C -index 为0.853(95% CI :0.809~0.897),具有良好的区分度;校准曲线显示,该模型的预测概率与实际概率均接近,具有较好的一致性;经决策曲线分析,当阈值为0.1~0.8时,列线图预测模型具有较好的临床实用性。
Lead paragraph
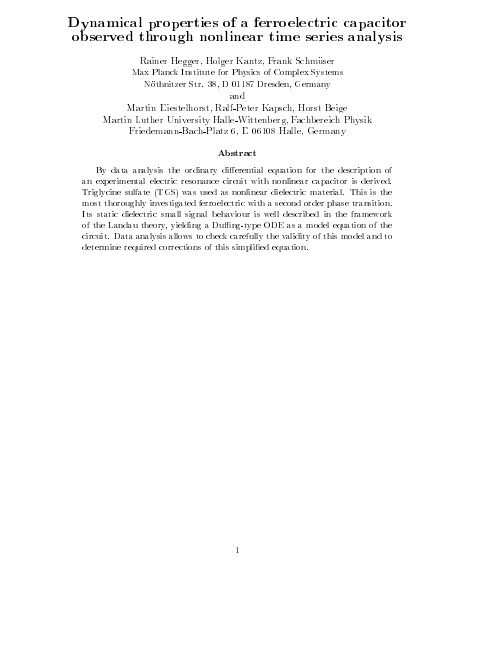
and Martin Diestelhorst, Ralf-Peter Kapsch, Horst Beige Martin Luther University Halle-Wittenberg, Fachbereich Physik Friedemann-Bach-Platz 6, D 06108 Halle, Germany
By data analysis the ordinary di erential equation for the description of an experimental electric resonance circuit with nonlinear capacitor is derived. Triglycine sulfate (TGS) was used as nonlinear dielectric material. This is the most thoroughly investigated ferroelectric with a second order phase transition. Its static dielectric small signal behaviour is well described in the framework of the Landau theory, yielding a Du ng-type ODE as a model equation of the circuit. Data analysis allows to check carefully the validity of this model and to determine required corrections of this simpli ed equation. Max Planck Institute for Physics of Complex Systems Nothnitzer Str. 38, D 01187 Dresden, Germany
Copper, zinc, cadmium and lead biosorption by Gymnogongrus torulosus. Thermodynamics and kinetics
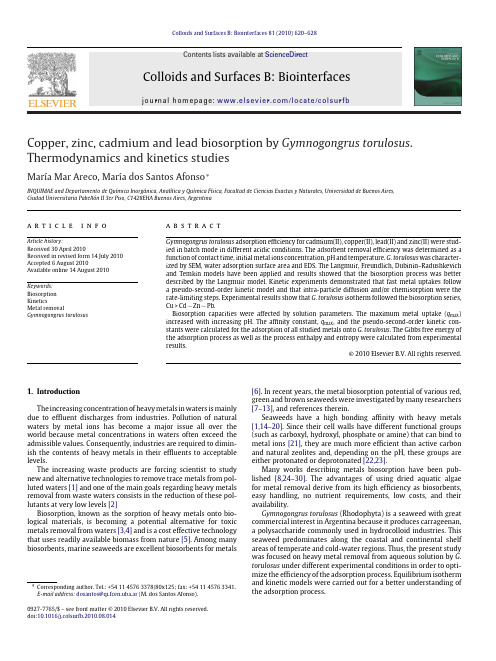
Colloids and Surfaces B:Biointerfaces 81 (2010) 620–628Contents lists available at ScienceDirectColloids and Surfaces B:Biointerfacesj o u r n a l h o m e p a g e :w w w.e l s e v i e r.c o m /l o c a t e /c o l s u r fbCopper,zinc,cadmium and lead biosorption by Gymnogongrus torulosus .Thermodynamics and kinetics studiesMaría Mar Areco,María dos Santos Afonso ∗INQUIMAE and Departamento de Química Inorgánica,Analítica y Química Física,Facultad de Ciencias Exactas y Naturales,Universidad de Buenos Aires,Ciudad Universitaria Pabellón II 3er Piso,C1428EHA Buenos Aires,Argentinaa r t i c l e i n f o Article history:Received 30April 2010Received in revised form 14July 2010Accepted 6August 2010Available online 14 August 2010Keywords:Biosorption KineticsMetal removalGymnogongrus torulosusa b s t r a c tGymnogongrus torulosus adsorption efficiency for cadmium(II),copper(II),lead(II)and zinc(II)were stud-ied in batch mode in different acidic conditions.The adsorbent removal efficiency was determined as a function of contact time,initial metal ions concentration,pH and temperature.G.torulosus was character-ized by SEM,water adsorption surface area and EDS.The Langmuir,Freundlich,Dubinin–Radushkevich and Temkin models have been applied and results showed that the biosorption process was better described by the Langmuir model.Kinetic experiments demonstrated that fast metal uptakes follow a pseudo-second-order kinetic model and that intra-particle diffusion and/or chemisorption were the rate-limiting steps.Experimental results show that G.torulosus isotherm followed the biosorption series,Cu >Cd ∼Zn ∼Pb.Biosorption capacities were affected by solution parameters.The maximum metal uptake (q max )increased with increasing pH.The affinity constant,q max ,and the pseudo-second-order kinetic con-stants were calculated for the adsorption of all studied metals onto G.torulosus .The Gibbs free energy of the adsorption process as well as the process enthalpy and entropy were calculated from experimental results.© 2010 Elsevier B.V. All rights reserved.1.IntroductionThe increasing concentration of heavy metals in waters is mainly due to effluent discharges from industries.Pollution of natural waters by metal ions has become a major issue all over the world because metal concentrations in waters often exceed the admissible values.Consequently,industries are required to dimin-ish the contents of heavy metals in their effluents to acceptable levels.The increasing waste products are forcing scientist to study new and alternative technologies to remove trace metals from pol-luted waters [1]and one of the main goals regarding heavy metals removal from waste waters consists in the reduction of these pol-lutants at very low levels [2]Biosorption,known as the sorption of heavy metals onto bio-logical materials,is becoming a potential alternative for toxic metals removal from waters [3,4]and is a cost effective technology that uses readily available biomass from nature [5].Among many biosorbents,marine seaweeds are excellent biosorbents for metals∗Corresponding author.Tel.:+541145763378/80x125;fax:+541145763341.E-mail address:dosantos@qi.fcen.uba.ar (M.dos Santos Afonso).[6].In recent years,the metal biosorption potential of various red,green and brown seaweeds were investigated by many researchers [7–13],and references therein.Seaweeds have a high bonding affinity with heavy metals [1,14–20].Since their cell walls have different functional groups (such as carboxyl,hydroxyl,phosphate or amine)that can bind to metal ions [21],they are much more efficient than active carbon and natural zeolites and,depending on the pH,these groups are either protonated or deprotonated [22,23].Many works describing metals biosorption have been pub-lished [8,24–30].The advantages of using dried aquatic algae for metal removal derive from its high efficiency as biosorbents,easy handling,no nutrient requirements,low costs,and their availability.Gymnogongrus torulosus (Rhodophyta)is a seaweed with great commercial interest in Argentina because it produces carrageenan,a polysaccharide commonly used in hydrocolloid industries.This seaweed predominates along the coastal and continental shelf areas of temperate and cold-water regions.Thus,the present study was focused on heavy metal removal from aqueous solution by G.torulosus under different experimental conditions in order to opti-mize the efficiency of the adsorption process.Equilibrium isotherm and kinetic models were carried out for a better understanding of the adsorption process.0927-7765/$–see front matter © 2010 Elsevier B.V. All rights reserved.doi:10.1016/j.colsurfb.2010.08.014M.M.Areco,M.dos Santos Afonso/Colloids and Surfaces B:Biointerfaces81 (2010) 620–628621 2.Materials and methods2.1.Biosorbent materialG.torulosus were collected in Cabo Corrientes at Mar del PlataCity,Buenos Aires Province,Argentina.The seaweed was washedthoroughly with deionized water,then dried overnight at60◦Candfinally stored in desiccators before being used.Afterwards,thedried seaweed was blended in a homogenizer intofiner particles.A stainless steel standard sieve was used to obtainfine particles(0.5–2mm)of seaweed,which were subsequently used for biosorp-tion experiments.The specific surface area was determined by water adsorption atroom temperature(S w=312.2m2g−1)[31,32].Seaweeds particleswere kept,until a constant weight was attained,in a desiccatorscontaining a saturated solution of Ca(NO3)2·6H2O that ensures arelative humidity of rh=0.56.Dehydrated raw and adsorbed metal samples werefixed to10mm metal mounts using carbon tape and spit coated with goldunder vacuum in an argon atmosphere.The surface morphology ofthe coated samples was visualized by afile emission gun scanningelectron microscope Zeiss(FEG-SEM Zeiss LEO982GEMINI)withcombined energy dispersive X-ray analyzer(EDS)at a voltage of3.0kV.SEM permitted the identification of interesting structuralfeatures on the seaweed surface with EDS.INCA software was usedto determine the elemental composition of the surface before andafter metal binding.2.2.Metal solutionsAll chemicals used were of analytical reagent grade and wereused without further purification.All solutions and algal sus-pensions were prepared using Milli-Q water with a resistivity of18.0M cm.Stock zinc(II),copper(II),cadmium(II)and lead(II)solutionsfor the experiments were prepared by dissolving ZnSO4·7H2O,CuSO4·5H2O,Cd(NO3)·4H2O and Pb(NO3)respectively.All solutionconcentrations ranged from25to500mg l−1of each metal.2.3.Experimental procedure2.3.1.Batch experimentsThe metal sorption performances for Cu(II),Zn(II),Cd(II)andPb(II)in batch mode by G.torulosus were compared.Batch sorp-tion experiments were performed with0.1g of biomass suspendedin100ml of Zn(II),Cu(II),Cd(II)or Pb(II)solutions in a concentra-tion range between25and500mg l−1of each metal.Suspensionswere kept in constant agitation at desired and constant pH andtemperature.Experiments were carried out by triplicate for eachconcentration.Algal biomass was separated from metal solutions byfiltrationwith cellulose nitrate membranefilters(0.22m pore).Afterwards,thefinal metal concentrations in thefiltered solutions were deter-mined and metal uptake(q)was calculated using the followingmass balance equation[7]q=(C i−C eq)×Vm(1)where C i is the initial metal concentration(mg l−1or mM),C eq the equilibrium metal concentration(mg l−1or mM),V the solution volume(l)and m is the dry seaweeds weight(g).The dissolved metal removal efficiency(RM,%)by both biosor-bents was determined by the following equation:RM=(C i−C eq)×100C i(2)Control experiments were carried out in the absence of adsorbents in order tofind out whether there is any adsorption on the container walls.2.3.2.Effect of pHThe pH dependence of metal uptake by G.torulosus was stud-ied through batch experiments in a pH range from1to6,with 1g l−1algae concentration and Pb(II),Cd(II),Cu(II)and Zn(II)initial concentration of0.21,0.45,0.77and0.90mM,respectively.The pH range was selected in order to avoid metal(hydr)oxides pre-cipitation,and to ensure that the decay of metal concentration is due to adsorption processes.The pH was adjusted using0.1M HCl or0.1M NaOH.The biosorption experiments were carried out by triplicate and there were no significant differences within each trip-licate.The expressed values represent the average of the obtained results.Resulting pH was measured using a Metrohm644pH-meter with a combined glass microelectrode.2.4.Metal quantificationCadmium(II)and lead(II)concentrations were determined by atomic absorption spectroscopy(AAS)using a Shimadzu6800 spectrophotometer with a GF-6501graphite oven,an ASC-6000 autosampler Hamamatshu and lead(II)and cadmium(II)cathode lamps.Zinc(II)concentrations were spectrophotometrically measured at620nm wavelength using Zincon(2-carboxy-2 -hydroxy-5 -sulfoformacylbenzol)as a chromogenic reagent.Zinc–Zincon blue complex is formed at pH values between8.5and9.5[33].Copper(II)concentrations were spectrophotometrically mea-sured using1g of Sodium Diethyldithiocarbamate(DDTC) dissolved in ethanol–water50%(v/v)and H2SO4(10−3M),as reagent[29].At low pH DDTC forms a yellow complex with cop-per(II).Absorbance of the corresponding solution was measured at 440nm.Absorbance measurements were made with a double beam UV–vis spectrophotometer Shimadzu-Pharmaspec UV-1700and a 1cm path length quartz cuvette.2.5.Reproducibility and data analysisUnless otherwise indicated,all data shown are the mean values from three replicate experiments.Standard deviations were below 5%.The statistical data analyses were made using the SigmaPlot software package.SigmaPlot uses the Durbin–Watson statis-tic to test residuals for their independence of each other.The Durbin–Watson statistic is a measure of serial correlation between the residuals.The residuals are often correlated when the indepen-dent variable is time,and the deviation between the observation and the regression line at one time is related to the deviation at the previous time.3.Results and discussion3.1.Biosorption kineticsEquilibrium analysis is fundamental in order to evaluate the affinity or capacity of a sorbent.However,it is important to assess how sorption rates vary with aqueous free metal concentrations, and how rates are affected by sorption capacity or by the sorbent622M.M.Areco,M.dos Santos Afonso /Colloids and Surfaces B:Biointerfaces81 (2010) 620–628Fig.1.Biosorption kinetics of metal uptake at pH 5.5and room temperature for ():Cu(II);():Zn(II);():Pb(II)and ():Cd(II)biosorbed onto G.torulosus .Solid lines were calculated using binding ligand kinetics model with parameters showed in Table 1.character in terms of kinetics [34].Fig.1shows the four metal uptakes by G.torulosus versus time at pH 5.5and room temper-ature.The initial metal concentrations were 0.99;0.83,0.360and 0.350mM of Zn(II),Cu(II),Cd(II)and Pb(II),respectively.The metal uptake was calculated using Eq.(1)and increases with the time of contact,it is relatively fast and in less than 5h the equilibrium plateau is reached.Thus,metal uptake was faster for the first 3h and afterwards the adsorption rates slowed until attaining equilibrium.The equilibration time for maximum uptake is reached in less than 5h and an equilibration period of 24h was selected for adsorp-tion experiments.The time profile of metal uptake is a single,smooth,and continuous curve leading to saturation,suggesting the possible monolayer coverage on the surface of the adsorbent.Three kinetic models,pseudo-first-order,pseudo-second-order and intra-particle diffusion were applied in order to interpret the experimental results.3.1.1.Kinetic modelsThe extent of biosorption is only dependent on the initial and final equilibrium states.The biosorption rate is dependent on the way that the process is performed between the initial and final steps.Sorption kinetics may be controlled by several independent processes such as transport from the bulk to the surface and chem-ical binding reactions of the sorbate.Surface complex formation with metal ions involves the coordi-nation of metal ions with oxygen donor atoms that constitute the biomasses cell walls,followed by the release of protons from thesurface.For example:SOH +Me 2+k fk b SOMe ++H +(3)where Me 2+is the concentration of sorbate in solution,SOH is the sorbent surface site which is susceptible of coordination,SOMe +is the sorbate concentration on the sorbent at any time,and k f and k b are the kinetic constants for the forward and backward reaction steps.Usually,the transport process in the bulk solution and the film diffusion through the boundary layer of bioadsorbent are consid-ered fast processes.Therefore,intra-particle diffusion or chemical binding reactions are the controlling steps of the adsorption mech-anism.3.1.1.1.Pseudo-first-order model.The most widely used kinetic model for biosorption is a first order reversible kinetic model that was previously reported by Bhattacharya and Venkobachar [35].They presented a model based on concentration solution and on cadmium(II)sorption by establishing a balance between liquid phase and solid biomass.The pseudo-first-order kinetics model was applied to many sys-tems,from organic compounds,such as dyes,to heavy metals adsorbed on biomaterials,biopolymers or on solid inorganic matri-ces [36].This kinetic model is based on a pseudo-first-order rate expression of Lagergren ln(q e −q t )=ln q e −k 1t(4)where k 1is the pseudo-first-order sorption rate constant,q e is the amount of metal ion adsorbed at equilibrium by the biomass and q t is the amount of metal ion adsorbed at any time t .Both,q e and q t were expressed in mmol g −1units.The overall rate constant k 1,in h −1,was calculated from the slope by plotting ln(q e −q t )versus t (Table 1).The correlation coefficients using the Lagergren model obtained at all studied initial concentrations were relatively low,ranging from 0.1393to 0.7280for all studied metals.Moreover,the experimental q e values did not agree with the calculated data.This suggests that the adsorption of lead(II),cadmium(II),zinc(II)and copper(II)onto G.torulosus can not be considered as a pseudo-first-order kinetic.3.1.1.2.Pseudo-second-order model.Since the pseudo-first-order model is not applicable for the understanding of the adsorption kinetics of heavy metals on G.torulosus biomass,the pseudo-second-order model was tested.In this model the rate at which adsorption sites are covered is proportional to the square of the number of unoccupied sites,and the number of occupied sites is proportional to the fraction of the metal ion adsorbed.Several systems respond to a second order kinetics model for sorptionTable 1Intra-particle diffusion,pseudo-first-order and pseudo-second-order kinetic parameters for four metals at room temperature and pH 5.5.q eq :maximum coverage concen-tration,k 1:pseudo-first-order constant,k 2:pseudo-second-order constant,k id :intra-particle diffusion constant.MeZn Cu Pb Cd Pseudo-first-orderq e (mmol g −1)0.090.080.110.11k 1(h −1)0.460.350.410.40R 20.13930.20400.72800.6185Pseudo-second-orderq e (mmol g −1)0.280.340.170.26k 2(g h −1mmol −1)87.8371.91142.1993.84R 20.99720.99890.99790.9991Intra-particle diffusionk id (mmol h −1g −1)0.420.380.210.32R 20.73200.95840.89180.7387M.M.Areco,M.dos Santos Afonso/Colloids and Surfaces B:Biointerfaces81 (2010) 620–628623Fig. 2.Pseudo-second-order kinetics model for the biosorption of(): Cu(II);():Zn(II);():Pb(II)and():Cd(II)onto G.torulosus.reactions,and are represented by the following equation[36]:dq tdt=k2(q e−q t)2(5) where k2is the rate of pseudo-second-order adsorption and q e and q t are the amount of metal ion adsorbed at equilibrium and the amount adsorbed at any time,respectively.The sorption rate can be calculated as the initial sorption rate when t approaches0.Inte-grating and rearranging,the pseudo-second-order equation can be written as:t q t =1k2q e+tq e(6)where k2is the rate constant of the pseudo-second-order sorption.The linear plot of t/q t against t was made in order to calculate the second order rate constant k2and the equilibrium adsorption capacity q e from the slope and intercept,respectively,as repre-sented in Fig.2.The solid lines in Figs.1and2were plotted using the binding ligand model(Eq.(7))and the pseudo second-order kinetics(Eq.(6)),respectively.The parameters obtained are listed in Table1. The binding ligand model is represented by Eq.(7):q=B max×t(k+t)(7)Eq.(7)is equivalent to Eq.(6)when B max=q e and k2−1=k×B max. Figs.1and2and Table1show significant agreement between the experimental and the calculated values.Thefitting parame-ters for both pseudo-first and pseudo-second-order equations are listed in Table1.The correlation factors of the pseudo-second-order kinetics model varied between0.9972and0.9991showing that pseudo-second-order model accurately represents the experimen-tal behavior.The analysis of the results shows that the correlation factors obtained are not so good for the pseudo-first-order model,while results obtained for the pseudo-second-order kinetic model were very good.Moreover,the values of q e calculated from the pseudo-first-order kinetics are not similar to the experimental values,while those calculated from second-order model are in good agreement with the experimental values in Fig.1.Thus,it is clear that experi-mental data are betterfitted by the second order equation than by the pseudo-first-order equation.The pseudo-second-order model has already beensuccessful applied to the description of Cd(II),Cu(II),Ni(II),Pb(II)biosorp-tion by different bioadsorbents.The obtained pseudo-second-order Fig. 3.Intra-particle diffusion kinetics model for the biosorption of(): Cu(II);():Zn(II);():Pb(II)and():Cd(II)onto G.torulosus.kinetic parameters are in accordance with those found in the liter-ature[34,36–38].3.1.1.3.Intra-particle diffusion model.The surfaces of an algae cell or a mineral particle suspended in water have interface boundaries that are characterized by a discontinuity of certain parameters such as density and chemical composition.The intrinsic adsorption is often faster than the transport to the interface.The thickness of the boundary layer surrounding the particle should be minimal when agitation speed will be fast enough and boundary layer resistance orfilm diffusion should not be a rate-controlling step.The rate constant for intra-particle diffusion(k id)is given byq t=k id(t)1/2(8) where k id is the internal diffusion coefficient,q t the amount of metal adsorbed at time t and t the sorption time.Plots of q ver-sus t1/2for lead(II),cadmium(II),zinc(II)and copper(II)are shown in Fig.3.From thisfigure,an initial steep-sloped portion at intra-particle diffusion is seen,followed by the plateau at equilibrium. The initial segment of the curve with a sharp slope(from0to1h)is attributed to surface adsorption,where the intra-particle diffusion is the rate-controlling step followed by a plateau that corresponds to equilibrium.The intra-particle diffusion rate was obtained from the slope of the linear part of the curve.The correlation coefficients were not so good(R2values ranged from0.7320to0.9584,see Table1).The straight lines obtained in the linear portion of the plot did not pass through the origin, indicating that pore diffusion was not the only controlling step. This deviation from the origin may be due to the difference in the rate of mass transfer in the initial andfinal stages of adsorption. This indicates some degree of boundary layer control and further show that the intra-particle diffusion was not the only rate-limiting step,chemical binding may also control the rate of sorption,or both process may operate simultaneously.The correlation coefficients for the pseudo-second-order kinetic model are greater than those for the intra-particle diffusion model (Table1),suggesting a chemical reaction mechanism[39].This would indicate that the metal cations sorption is a complex mix of surface chemisorption occurring on the boundary layer of the algal particle and intra-particle diffusion.3.1.1.4.Temperature effect.Temperature can influence the sorp-tion process rate.An increase in temperature from7to25◦C at pH5.5increases the pseudo-second-order kinetic constant by a factor of2.1,17.3,13.6and10.5for lead(II),cadmium(II),zinc(II)624M.M.Areco,M.dos Santos Afonso/Colloids and Surfaces B:Biointerfaces81 (2010) 620–628 and copper(II),respectively.Increasing the temperature from25to55◦C decreases the pseudo-second-order kinetic constant by afactor of0.1,0.2and0.6for lead(II),cadmium(II)and copper(II),respectively,and increases the constant by a factor of1.1for zinc(II)probably caused by a change in the texture of the sorbent and a lossin the sorption capacity due to material deterioration[5].3.2.Sorption modelsAdsorption isotherms are important criteria in optimizing theuse of adsorbents materials because they describe the nature ofthe interaction between sorbate and adsorbent.Thus,analysis ofexperimentally obtained equilibrium data by either theoretical orempirical equations is useful for practical design and operation ofadsorption systems.There are a great number of expressions that describeadsorption isotherms.Here the Langmuir,Freundlich,Dubinin–Radushkevich and Temkin models were applied tothe equilibrium data.ngmuir adsorption isothermsThe Langmuir isotherm is a well-known model that indicates areduction of the available interaction places as the metal ion con-centration increases.The Langmuir isotherm assumes monolayeradsorption and is determined by the following equation:q=q max×K L×C eq(1+K L×C eq)(9)where q max is the maximum metal uptake(in mg g−1or mmol g−1), meaning the maximum concentration on the solid phase,C eq is the metal concentration at the equilibrium on the aqueous media and K L is the Langmuir equilibrium constant which is related with the free energy of the reaction.Copper(II),zinc(II),cadmium(II)and lead(II)adsorption isotherms for G.torulosus are presented in Fig.4.The Langmuir isotherm,Eq.(9),was applied to estimate seaweed adsorption capacity.The Langmuir parameter and the correlation factor for the four metals are indicated in Table2.Affinity between sorbent and sorbate are represented by the constant K L.In general good biosorbents have a high q max and a high K L.G.torulosus have high saturation(q max)for all metals studied,except for lead(II).The maximum coverage,q max,follow the sequence Cu>Cd>Zn>Pb.The solid lines in Fig.4where plotted with the Langmuir model applied to metal uptake during the sorption process.The calculated lines and the experimental data match very well.The maximum adsorption capacities are one order in magnitude higher than those listed in[40]for differentbiosorbents.Fig.4.Adsorption isotherms at pH5.5for G.torulosus,():Cu(II);(): Zn(II);():Pb(II)and():Cd(II).Solid lines were calculated using Langmuir model.3.2.2.Freundlich adsorption isothermsThe Freundlich isotherm assumes a heterogeneous surface with a nonuniform distribution of heat of adsorption over the surface. Thus the Freundlich model describes the adsorption on an energet-ically heterogeneous surface on which the adsorbed molecules are interactive.This isotherm is represent by Eq.(10)q e=K F×C1/neq(10) where K F is the Freundlich constant indicating adsorption capacity and1/n is the adsorption intensity.Linear regression analysis was used for isotherm data treatment.The linear form of the Freundlich isotherm used wasln q e=ln K F+1n×ln C eqThe values of K F and1/n were calculated from the intercept and slope of the plot between ln q e versus ln C eq.K F,1/n and the corre-lation coefficient(R2)values of the Freundlich isotherm are given in Table2.For all cases,the Langmuir equationfits the experimental data better than the Freundlich equation.This isotherm does not pre-dict any saturation of the adsorbent by the sorbate.Instead,infinite surface coverage is predicted,indicating multilayer sorption on the surface.Table2Fitting parameters for Langmuir,Freundlich,Temkin and Dubinin–Radushkevich isotherm equations.Me Langmuir Freundlichq=q max K L C e(1+K L C e)q=K F C e1/nq max(mmol g−1)K L(l mmol−1)R2K F×10−031/n R2Zn0.68±0.056 1.93±0.390.9080 6.890.41±0.090.8013 Cu0.81±0.037 4.40±0.880.97169.120.40±0.070.8648 Pb0.68±0.230.90±0.400.922612.510.54±0.080.9035 Cd0.66±0.021 3.18±0.320.990412.810.49±0.100.8364Me Temkin Dubinin–Radushkevichq=B×ln K T+B×ln C e ln q=ln q max−ˇεo2K T(l mg−1)B(mg g−1)R2q max(mmol g−1)−ˇ×10−04R2Zn0.3099.31±1.860.83330.50488.0±150.8618 Cu0.9389.65±1.290.91680.6117.0±10.8609 Pb0.20016.2±3.90.76850.164 2.0±0.050.6470 Cd0.35714.1±1.10.97030.4037.0±10.8345M.M.Areco,M.dos Santos Afonso /Colloids and Surfaces B:Biointerfaces 81 (2010) 620–628625Table 3Thermodynamics parameters for the adsorption of Zn(II),Cu(II),Pb(II)and Cd(II)on G.torulosus at pH 5.5and room temperature.MeLangmuir Temkin Dubinin–Radushkevich Thermodynamic parameters G ads (kJ mol −1)b (J mol −1) G ads (kJ mol −1)E (kJ mol −1) G ◦(kJ mol −1) H ◦(kJ mol −1) S ◦(J mol −1)Zn −18.74266−24.567.54−10.4428129Cu −20.78257−27.2426.73−15.64−33−59Pb −16.86153−26.3350.00−15.25−24−32Cd−19.98175−26.2626.73−15.65−23−253.2.3.Dubinin–Radushkevich adsorption isothermsDubinin and Radushkevich proposed a well-liked equation for the analysis of isotherms in order to determine if the adsorption occurred by a physical or chemical process.The D–R equation is more general than the Langmuir model because it does not assume a homogeneous surface,a constant sorption potential nor absence of steric hindrance between adsorbed and incoming particles ln q e =ln q max −ˇε2(11)where q e is the amount of metal cations adsorbed per g of biomass,q max represents the maximum sorption capacity of the adsorbent,ˇis a constant related to sorption energy (D–R constant)and εis the Polanyi sorption potential calculated by Eq.(12)ε=RT ln1+1C eq(12)where R is the gas constant 8.314J mol −1K −1,T is the temper-ature in Kelvin and C eq is the metal equilibrium concentration.The Polanyi sorption approach assumes a fixed volume of sorption space close to the adsorbent surface and the existence of sorption potential over these spaces.The sorption space in the vicinity of a solid surface is characterized by a series of equipotential surfaces having the same sorption potential.This sorption potential is inde-pendent of the temperature but varies according to the nature of sorbent and sorbate.The values of ˇand q max were calculated from the slope and the intercept of the plot of ln q e versus ε2(Table 2).The mean free energy of sorption per molecule of sorbate required to transfer one mole of ion from the infinity in the solution to the surface of biomass and can be determined by the following Eq.(13)E =(−2ˇ)−1/2(13)The Dubinin–Radushkevich constants calculated for the adsorp-tion by G.torulosus of all metals studied are shown in Table 2.All energy values obtained (Table 3)have E >8kJ mol −1,which indicate that all metal cation adsorptions were chemical processes,since a physical adsorption process has an E <8kJ mol −1.The sorp-tion capacity was lower than the sorption capacity calculated using the Langmuir model,which may be attributed to different assump-tions taken into consideration.From comparing the correlation coefficient values,it was concluded that the sorption of lead(II),cad-mium(II),zinc(II)and copper(II)onto G.torulosus biomass followed the Langmuir model.3.2.4.Temkin adsorption isothermsThe Temkin isotherm is represented by the following equation:q e =RTbln(k T C e )=B ln(k T C e )(14)where constant B =RT /b is related to the heat of adsorption,R is the universal gas constant (J mol −1K −1),T is the temperature (K),b is the variation of adsorption energy (J mol −1)and K T is the equi-librium binding constant (l mg −1)corresponding to the maximum binding energy.The Temkin isotherm assumes that the heat of adsorption of all the molecules in a layer decreases linearly due to adsorbent–sorbate interactions and that adsorption is charac-terized by a uniform distribution of binding energies,up to some maximum binding energy.A plot of q e versus ln C e makes possi-ble the determination of the isotherm constants B and K T from the slope and the intercept,respectively.The calculated values for Temkin parameters are shown in Table 2.The correlation factors show that the Langmuir model approximation to the experimen-tal results is better than the Temkin model.Consequently,among the four isotherm models used,the Langmuir model offers the best correlation factors.Experimental results show that G.torulosus isotherm follows the biosorption series,Cu >Cd ∼Zn ∼Pb,while the sequence obtained for K L values (Table 2)is Cu >Cd >Zn >Pb.These resultsshow that copper has the highest affinity for cell wall functional groups.Studies carried out using Sargassum fluitans showed a decrease in metals uptake when carboxylic groups –COO –are blocked due to esterification processes [41].Similar results were also obtained for carboxyl groups of fresh water biomass such as Chlorella pyrenoi-dosa and Cyanidium caldarium [42].The hydroxyl groups are also present in all polysaccharides but they are less abundant at low pH values,increasing their contribution to metallic cation adsorption at basic pH.Scanning electron microscope (SEM)images were used for the surface analysis of G.torulosus as shown in Fig.5.The SEM imagesFig.5.G.torulosus Scanning Electron Microscopy (SEM)at different magnification (A:1000×and B:5000×)and 5.0kV.。
Biosensors and Bioelectronics
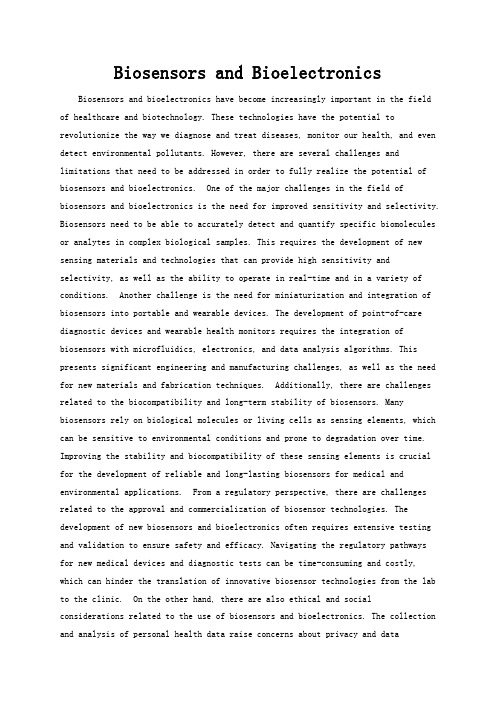
Biosensors and Bioelectronics Biosensors and bioelectronics have become increasingly important in the field of healthcare and biotechnology. These technologies have the potential to revolutionize the way we diagnose and treat diseases, monitor our health, and even detect environmental pollutants. However, there are several challenges and limitations that need to be addressed in order to fully realize the potential of biosensors and bioelectronics. One of the major challenges in the field of biosensors and bioelectronics is the need for improved sensitivity and selectivity. Biosensors need to be able to accurately detect and quantify specific biomolecules or analytes in complex biological samples. This requires the development of new sensing materials and technologies that can provide high sensitivity and selectivity, as well as the ability to operate in real-time and in a variety of conditions. Another challenge is the need for miniaturization and integration of biosensors into portable and wearable devices. The development of point-of-care diagnostic devices and wearable health monitors requires the integration of biosensors with microfluidics, electronics, and data analysis algorithms. This presents significant engineering and manufacturing challenges, as well as the need for new materials and fabrication techniques. Additionally, there are challenges related to the biocompatibility and long-term stability of biosensors. Many biosensors rely on biological molecules or living cells as sensing elements, which can be sensitive to environmental conditions and prone to degradation over time. Improving the stability and biocompatibility of these sensing elements is crucial for the development of reliable and long-lasting biosensors for medical and environmental applications. From a regulatory perspective, there are challenges related to the approval and commercialization of biosensor technologies. The development of new biosensors and bioelectronics often requires extensive testing and validation to ensure safety and efficacy. Navigating the regulatory pathwaysfor new medical devices and diagnostic tests can be time-consuming and costly, which can hinder the translation of innovative biosensor technologies from the lab to the clinic. On the other hand, there are also ethical and socialconsiderations related to the use of biosensors and bioelectronics. The collection and analysis of personal health data raise concerns about privacy and datasecurity. There is also a need to ensure that biosensor technologies are accessible and affordable for all populations, including underserved communities and developing countries. Despite these challenges, there are many exciting opportunities for the future of biosensors and bioelectronics. Advances in nanotechnology, materials science, and biotechnology are driving the development of new sensing platforms with unprecedented capabilities. The integration of biosensors with artificial intelligence and machine learning algorithms has the potential to revolutionize medical diagnostics and personalized medicine. In conclusion, biosensors and bioelectronics hold great promise for the future of healthcare and biotechnology, but there are significant challenges that need to be addressed. Improving sensitivity and selectivity, miniaturization and integration, stability and biocompatibility, regulatory approval, and ethical considerations are all important aspects that require attention. By addressing these challenges, we can unlock the full potential of biosensors and bioelectronics to improve human health and well-being.。
益生菌冲剂的体外模拟消化及抗菌活
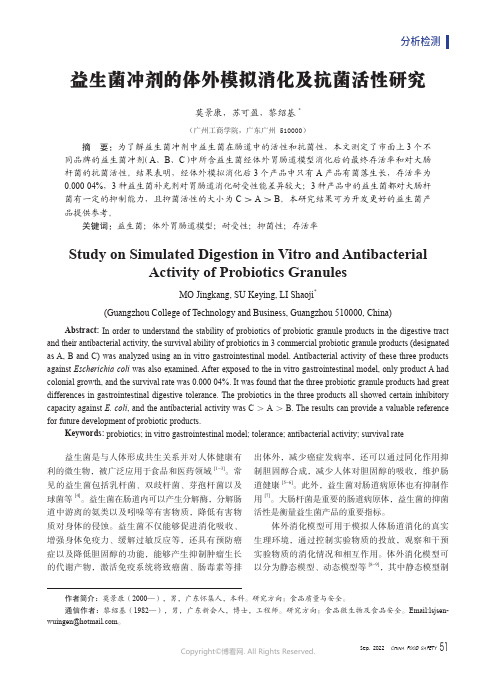
分析检测益生菌冲剂的体外模拟消化及抗菌活性研究莫景康,苏可盈,黎绍基*(广州工商学院,广东广州 510000)摘 要:为了解益生菌冲剂中益生菌在肠道中的活性和抗菌性,本文测定了市面上3个不同品牌的益生菌冲剂(A、B、C)中所含益生菌经体外胃肠道模型消化后的最终存活率和对大肠杆菌的抗菌活性。
结果表明,经体外模拟消化后3个产品中只有A产品有菌落生长,存活率为0.000 04%,3种益生菌补充剂对胃肠道消化耐受性能差异较大;3种产品中的益生菌都对大肠杆菌有一定的抑制能力,且抑菌活性的大小为C>A>B。
本研究结果可为开发更好的益生菌产品提供参考。
关键词:益生菌;体外胃肠道模型;耐受性;抑菌性;存活率Study on Simulated Digestion in Vitro and AntibacterialActivity of Probiotics GranulesMO Jingkang, SU Keying, LI Shaoji*(Guangzhou College of Technology and Business, Guangzhou 510000, China) Abstract: In order to understand the stability of probiotics of probiotic granule products in the digestive tract and their antibacterial activity, the survival ability of probiotics in 3 commercial probiotic granule products (designated as A, B and C) was analyzed using an in vitro gastrointestinal model. Antibacterial activity of these three products against Escherichia coli was also examined. After exposed to the in vitro gastrointestinal model, only product A had colonial growth, and the survival rate was 0.000 04%. It was found that the three probiotic granule products had great differences in gastrointestinal digestive tolerance. The probiotics in the three products all showed certain inhibitory capacity against E. coli, and the antibacterial activity was C>A>B. The results can provide a valuable reference for future development of probiotic products.Keywords: probiotics; in vitro gastrointestinal model; tolerance; antibacterial activity; survival rate益生菌是与人体形成共生关系并对人体健康有利的微生物,被广泛应用于食品和医药领域[1-3]。
- 1、下载文档前请自行甄别文档内容的完整性,平台不提供额外的编辑、内容补充、找答案等附加服务。
- 2、"仅部分预览"的文档,不可在线预览部分如存在完整性等问题,可反馈申请退款(可完整预览的文档不适用该条件!)。
- 3、如文档侵犯您的权益,请联系客服反馈,我们会尽快为您处理(人工客服工作时间:9:00-18:30)。
TCP
Several flavors of TCP: Tahoe: 4.3 BSD Tahoe (~ 1988) slow start, congestion avoidance, and fast retransmit (RFC 793, RFC 2001) Reno: 4.3 BSD Reno (~ 1990) slow start, congestion avoidance, fast retransmit, and fast recovery (RFC 2001, RFC 2581) NewReno (~ 1996) new fast recovery algorithm (RFC 2582) SACK (~ 1996, RFC 2018)
Stroboscopic model and bifurcations in TCP/RED
7
TCP Reno: fast retransmit and fast recovery
TCP sender three duplicate ACKs are 16 received 17 18 retransmit the packet 19 ssthresh = cwnd/2, 20 21 cwnd = ssthresh + 3 packets cwnd = cwnd + 1, for each additional duplicate ACK three duplicate ACKs17 transmit the new data, if cwnd allows cwnd = ssthresh, if ACK for new data is received TCP receiver
ISCAS: May 25, 2005, Kobe
Stroboscopic model and bifurcations in TCP/RED
10
RED
Random Early Detection Gateways for Congestion Avoidance Proposed by S. Floyd and V. Jacobson, LBN, 1993.
RED: Random Early Detection Gateways for Congestion Avoidance
ISCAS: May 25, 2005, Kobe
Stroboscopic model and bifurcations in TCP/RED
3
TCP
TCP: Transmission Control Protocol Fourth layer of the OSI model Connection oriented, reliable, and byte-stream service Employs window based flow and congestion control algorithms
ISCAS: May 25, 2005, Kobe
Stroboscopic model and bifurcations in TCP/RED
5
TCP Reno
CWND
SS: Slow Start CA: Congestion Avoidance TO: Timeout
TO
ssthresh 1 ssthresh2
ACK 16 ACK ACK ACK ACK 16 16 16 16
ISCAS: May 25, 2005, Kobe
Stroboscopic model and bifurcations in TCP/RED
8
TCP Reno: timeout
TCP maintains a retransmission timer The duration of the timer is called retransmission timeout Timeout occurs when the ACK for the delivered data is not received before the retransmission timer expires TCP sender retransmits the lost packet ssthresh = cwnd/2 cwnd = 1 or 2 packets
ISCAS: May 25, 2005, Kobe
Stroboscopic model and bifurcations in TCP/RED
12
RED algorithm
Calculate: average queue size for each packet arrival
q = (1 − wq ) ⋅ q + wq ⋅ q
Main concept: drop packets before the queue becomes full
ISCAS: May 25, 2005, Kobe
Stroboscopic model and bifurcations in TCP/RED
11
RED variables and parameters
pa = 0
Mark or drop the arriving packet with probability pa
ISCAS: May 25, 2005, Kobe
Stroboscopic model and bifurcations in TCP/RED
14
Modeling methodology
q − qmin pb = pmax × qmax − qmin
pa =
pb 1 − count × pb
count : number of packets that arrived since the last packet drop
else if (q > qmax )
pa = 1
else
(q < qmin )
Main variables and parameters: average queue size: q instantaneous queue size: q drop probability: pa queue weight: wq maximum drop probability: pmax queue thresholds: qmin and qmax
S. Floyd and V. Jacobson, “Random early detection gateways for congestion avoidance,” IEEE/ACM Trans. Networking, vol. 1, no. 4, pp. 397–413, Aug. 1993.
1ACK1 TO1 NhomakorabeaISCAS: May 25, 2005, Kobe
Stroboscopic model and bifurcations in TCP/RED
9
AQM: Active Queue Management
AQM (RFC 2309): reduces bursty packet drops in routers provides lower-delay interactive service avoids the “lock-out” problem reacts to the incipient congestion before buffers overflow AQM algorithms: RED (RFC 2309) ARED, CHOKe, BLUE, …
SS
CA CA Fast retransmit and fast recovery
SS
t
ISCAS: May 25, 2005, Kobe
Stroboscopic model and bifurcations in TCP/RED
6
TCP Reno: slow start and congestion avoidance
window size: 5 packets
6
5
4
3
2
1
OSI: Open System Interconnection reference model
ISCAS: May 25, 2005, Kobe Stroboscopic model and bifurcations in TCP/RED 4
drop probability
pb 1
pmax
qmin
qmax
q
13
ISCAS: May 25, 2005, Kobe
Stroboscopic model and bifurcations in TCP/RED
RED algorithm: drop probability
if (qmin < q < qmax )
Roadmap
Introduction Discrete-time model of TCP Reno with RED: TCP/RED: model with one state variables model validation Bifurcation diagrams Conclusion References
ISCAS: May 25, 2005, Kobe
Stroboscopic model and bifurcations in TCP/RED
2
Motivation
Modeling TCP Reno with RED: examine the interactions between TCP and RED understand and predict the dynamical network behavior analyze the impact of system parameters