数学建模美赛o奖论文
美国大学生数学建模竞赛优秀论文
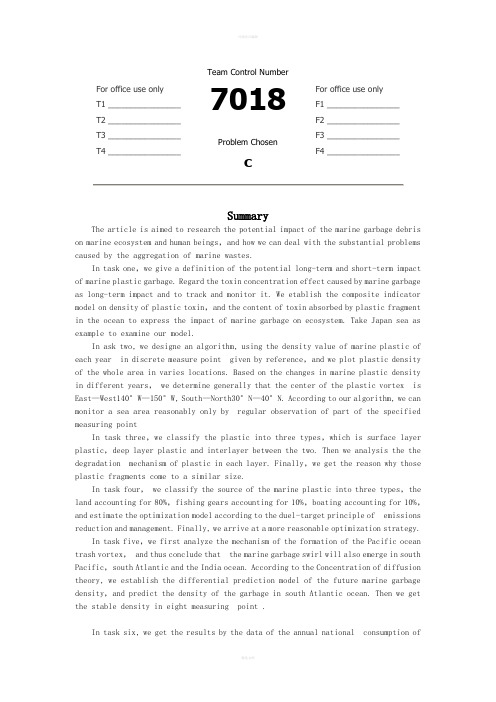
For office use onlyT1________________ T2________________ T3________________ T4________________Team Control Number7018Problem ChosencFor office use onlyF1________________F2________________F3________________F4________________ SummaryThe article is aimed to research the potential impact of the marine garbage debris on marine ecosystem and human beings,and how we can deal with the substantial problems caused by the aggregation of marine wastes.In task one,we give a definition of the potential long-term and short-term impact of marine plastic garbage. Regard the toxin concentration effect caused by marine garbage as long-term impact and to track and monitor it. We etablish the composite indicator model on density of plastic toxin,and the content of toxin absorbed by plastic fragment in the ocean to express the impact of marine garbage on ecosystem. Take Japan sea as example to examine our model.In ask two, we designe an algorithm, using the density value of marine plastic of each year in discrete measure point given by reference,and we plot plastic density of the whole area in varies locations. Based on the changes in marine plastic density in different years, we determine generally that the center of the plastic vortex is East—West140°W—150°W, South—North30°N—40°N. According to our algorithm, we can monitor a sea area reasonably only by regular observation of part of the specified measuring pointIn task three,we classify the plastic into three types,which is surface layer plastic,deep layer plastic and interlayer between the two. Then we analysis the the degradation mechanism of plastic in each layer. Finally,we get the reason why those plastic fragments come to a similar size.In task four, we classify the source of the marine plastic into three types,the land accounting for 80%,fishing gears accounting for 10%,boating accounting for 10%,and estimate the optimization model according to the duel-target principle of emissions reduction and management. Finally, we arrive at a more reasonable optimization strategy.In task five,we first analyze the mechanism of the formation of the Pacific ocean trash vortex, and thus conclude that the marine garbage swirl will also emerge in south Pacific,south Atlantic and the India ocean. According to the Concentration of diffusion theory, we establish the differential prediction model of the future marine garbage density,and predict the density of the garbage in south Atlantic ocean. Then we get the stable density in eight measuring point .In task six, we get the results by the data of the annual national consumption ofpolypropylene plastic packaging and the data fitting method, and predict the environmental benefit generated by the prohibition of polypropylene take-away food packaging in the next decade. By means of this model and our prediction,each nation will reduce releasing 1.31 million tons of plastic garbage in next decade.Finally, we submit a report to expediction leader,summarize our work and make some feasible suggestions to the policy- makers.Task 1:Definition:●Potential short-term effects of the plastic: the hazardeffects will be shown in the short term.●Potential long-term effects of the plastic: thepotential effects, of which hazards are great, willappear after a long time.The short- and long-term effects of the plastic on the ocean environment:In our definition, the short-term and long-term effects of the plastic on the ocean environment are as follows.Short-term effects:1)The plastic is eaten by marine animals or birds.2) Animals are wrapped by plastics, such as fishing nets, which hurt or even kill them.3)Deaden the way of the passing vessels.Long-term effects:1)Enrichment of toxins through the food chain: the waste plastic in the ocean has no natural degradation in theshort-term, which will first be broken down into tinyfragments through the role of light, waves,micro-organisms, while the molecular structure has notchanged. These "plastic sands", easy to be eaten byplankton, fish and other, are Seemingly very similar tomarine life’s food,causing the enrichment and delivery of toxins.2)Accelerate the greenhouse effect: after a long-term accumulation and pollution of plastics, the waterbecame turbid, which will seriously affect the marineplants (such as phytoplankton and algae) inphotosynthesis. A large number of plankton’s deathswould also lower the ability of the ocean to absorbcarbon dioxide, intensifying the greenhouse effect tosome extent.To monitor the impact of plastic rubbish on the marine ecosystem:According to the relevant literature, we know that plastic resin pellets accumulate toxic chemicals , such as PCBs、DDE , and nonylphenols , and may serve as a transport medium and soure of toxins to marine organisms that ingest them[]2. As it is difficult for the plastic garbage in the ocean to complete degradation in the short term, the plastic resin pellets in the water will increase over time and thus absorb more toxins, resulting in the enrichment of toxins and causing serious impact on the marine ecosystem.Therefore, we track the monitoring of the concentration of PCBs, DDE, and nonylphenols containing in the plastic resin pellets in the sea water, as an indicator to compare the extent of pollution in different regions of the sea, thus reflecting the impact of plastic rubbish on ecosystem.To establish pollution index evaluation model: For purposes of comparison, we unify the concentration indexes of PCBs, DDE, and nonylphenols in a comprehensive index.Preparations:1)Data Standardization2)Determination of the index weightBecause Japan has done researches on the contents of PCBs,DDE, and nonylphenols in the plastic resin pellets, we illustrate the survey conducted in Japanese waters by the University of Tokyo between 1997 and 1998.To standardize the concentration indexes of PCBs, DDE,and nonylphenols. We assume Kasai Sesside Park, KeihinCanal, Kugenuma Beach, Shioda Beach in the survey arethe first, second, third, fourth region; PCBs, DDE, andnonylphenols are the first, second, third indicators.Then to establish the standardized model:j j jij ij V V V V V min max min --= (1,2,3,4;1,2,3i j ==)wherej V max is the maximum of the measurement of j indicator in the four regions.j V min is the minimum of the measurement of j indicatorstandardized value of j indicator in i region.According to the literature [2], Japanese observationaldata is shown in Table 1.Table 1. PCBs, DDE, and, nonylphenols Contents in Marine PolypropyleneTable 1 Using the established standardized model to standardize, we have Table 2.In Table 2,the three indicators of Shioda Beach area are all 0, because the contents of PCBs, DDE, and nonylphenols in Polypropylene Plastic Resin Pellets in this area are the least, while 0 only relatively represents the smallest. Similarly, 1 indicates that in some area the value of a indicator is the largest.To determine the index weight of PCBs, DDE, and nonylphenolsWe use Analytic Hierarchy Process (AHP) to determine the weight of the three indicators in the general pollution indicator. AHP is an effective method which transforms semi-qualitative and semi-quantitative problems into quantitative calculation. It uses ideas of analysis and synthesis in decision-making, ideally suited for multi-index comprehensive evaluation.Hierarchy are shown in figure 1.Fig.1 Hierarchy of index factorsThen we determine the weight of each concentrationindicator in the generall pollution indicator, and the process are described as follows:To analyze the role of each concentration indicator, we haveestablished a matrix P to study the relative proportion.⎥⎥⎥⎦⎤⎢⎢⎢⎣⎡=111323123211312P P P P P P P Where mn P represents the relative importance of theconcentration indicators m B and n B . Usually we use 1,2,…,9 and their reciprocals to represent different importance. The greater the number is, the more important it is. Similarly, the relative importance of m B and n B is mn P /1(3,2,1,=n m ).Suppose the maximum eigenvalue of P is m ax λ, then theconsistency index is1max --=n nCI λThe average consistency index is RI , then the consistencyratio isRICI CR = For the matrix P of 3≥n , if 1.0<CR the consistency isthougt to be better, of which eigenvector can be used as the weight vector.We get the comparison matrix accoding to the harmful levelsof PCBs, DDE, and nonylphenols and the requirments ofEPA on the maximum concentration of the three toxins inseawater as follows:⎥⎥⎥⎦⎤⎢⎢⎢⎣⎡=165416131431P We get the maximum eigenvalue of P by MATLAB calculation0012.3max =λand the corresponding eigenvector of it is()2393.02975.09243.0,,=W1.0042.012.1047.0<===RI CI CR Therefore,we determine the degree of inconsistency formatrix P within the permissible range. With the eigenvectors of p as weights vector, we get thefinal weight vector by normalization ()1638.02036.06326.0',,=W . Defining the overall target of pollution for the No i oceanis i Q , among other things the standardized value of threeindicators for the No i ocean is ()321,,i i i i V V V V = and the weightvector is 'W ,Then we form the model for the overall target of marine pollution assessment, (3,2,1=i )By the model above, we obtained the Value of the totalpollution index for four regions in Japanese ocean in Table 3T B W Q '=In Table3, the value of the total pollution index is the hightest that means the concentration of toxins in Polypropylene Plastic Resin Pellets is the hightest, whereas the value of the total pollution index in Shioda Beach is the lowest(we point up 0 is only a relative value that’s not in the name of free of plastics pollution)Getting through the assessment method above, we can monitor the concentration of PCBs, DDE and nonylphenols in the plastic debris for the sake of reflecting the influence to ocean ecosystem.The highter the the concentration of toxins,the bigger influence of the marine organism which lead to the inrichment of food chain is more and more dramatic.Above all, the variation of toxins’ concentration simultaneously reflects the distribution and time-varying of marine litter. We can predict the future development of marine litter by regularly monitoring the content of these substances, to provide data for the sea expedition of the detection of marine litter and reference for government departments to make the policies for ocean governance.Task 2:In the North Pacific, the clockwise flow formed a never-ending maelstrom which rotates the plastic garbage. Over the years, the subtropical eddy current in North Pacific gathered together the garbage from the coast or the fleet, entrapped them in the whirlpool, and brought them to the center under the action of the centripetal force, forming an area of 3.43 million square kilometers (more than one-third of Europe) .As time goes by, the garbage in the whirlpool has the trend of increasing year by year in terms of breadth, density, and distribution. In order to clearly describe the variability of the increases over time and space, according to “Count Densities of Plastic Debris from Ocean Surface Samples North Pacific Gyre 1999—2008”, we analyze the data, exclude them with a great dispersion, and retain them with concentrated distribution, while the longitude values of the garbage locations in sampled regions of years serve as the x-coordinate value of a three-dimensional coordinates, latitude values as the y-coordinate value, the Plastic Count per cubic Meter of water of the position as the z-coordinate value. Further, we establish an irregular grid in the yx plane according to obtained data, and draw a grid line through all the data points. Using the inverse distance squared method with a factor, which can not only estimate the Plastic Count per cubic Meter of water of any position, but also calculate the trends of the Plastic Counts per cubic Meter of water between two original data points, we can obtain the unknown grid points approximately. When the data of all the irregular grid points are known (or approximately known, or obtained from the original data), we can draw the three-dimensional image with the Matlab software, which can fully reflect the variability of the increases in the garbage density over time and space.Preparations:First, to determine the coordinates of each year’s sampled garbage.The distribution range of garbage is about the East - West 120W-170W, South - North 18N-41N shown in the “Count Densities of Plastic Debris from Ocean Surface Samples North Pacific Gyre 1999--2008”, we divide a square in the picture into 100 grids in Figure (1) as follows:According to the position of the grid where the measuring point’s center is, we can identify the latitude and longitude for each point, which respectively serve as the x- and y- coordinate value of the three-dimensional coordinates.To determine the Plastic Count per cubic Meter of water. As the “Plastic Count per cubic Meter of water” provided by “Count Densities of P lastic Debris from Ocean Surface Samples North Pacific Gyre 1999--2008”are 5 density interval, to identify the exact values of the garbage density of one year’s different measuring points, we assume that the density is a random variable which obeys uniform distribution in each interval.Uniform distribution can be described as below:()⎪⎩⎪⎨⎧-=01a b x f ()others b a x ,∈We use the uniform function in Matlab to generatecontinuous uniformly distributed random numbers in each interval, which approximately serve as the exact values of the garbage density andz-coordinate values of the three-dimensional coordinates of the year’s measuring points.Assumptions(1)The data we get is accurate and reasonable.(2)Plastic Count per cubic Meter of waterIn the oceanarea isa continuous change.(3)Density of the plastic in the gyre is a variable by region.Density of the plastic in the gyre and its surrounding area is interdependent , However, this dependence decreases with increasing distance . For our discussion issue, Each data point influences the point of each unknown around and the point of each unknown around is influenced by a given data point. The nearer a given data point from the unknown point, the larger the role.Establishing the modelFor the method described by the previous,we serve the distributions of garbage density in the “Count Pensities of Plastic Debris from Ocean Surface Samples North Pacific Gyre 1999--2008”as coordinates ()z y,, As Table 1:x,Through analysis and comparison, We excluded a number of data which has very large dispersion and retained the data that is under the more concentrated the distribution which, can be seen on Table 2.In this way, this is conducive for us to get more accurate density distribution map.Then we have a segmentation that is according to the arrangement of the composition of X direction and Y direction from small to large by using x co-ordinate value and y co-ordinate value of known data points n, in order to form a non-equidistant Segmentation which has n nodes. For the Segmentation we get above,we only know the density of the plastic known n nodes, therefore, we must find other density of the plastic garbage of n nodes.We only do the sampling survey of garbage density of the north pacificvortex,so only understand logically each known data point has a certain extent effect on the unknown node and the close-known points of density of the plastic garbage has high-impact than distant known point.In this respect,we use the weighted average format, that means using the adverse which with distance squared to express more important effects in close known points. There're two known points Q1 and Q2 in a line ,that is to say we have already known the plastic litter density in Q1 and Q2, then speculate the plastic litter density's affects between Q1、Q2 and the point G which in the connection of Q1 and Q2. It can be shown by a weighted average algorithm22212221111121GQ GQ GQ Z GQ Z Z Q Q G +*+*=in this formula GQ expresses the distance between the pointG and Q.We know that only use a weighted average close to the unknown point can not reflect the trend of the known points, we assume that any two given point of plastic garbage between the changes in the density of plastic impact the plastic garbage density of the unknown point and reflecting the density of plastic garbage changes in linear trend. So in the weighted average formula what is in order to presume an unknown point of plastic garbage density, we introduce the trend items. And because the greater impact at close range point, and thus the density of plastic wastes trends close points stronger. For the one-dimensional case, the calculation formula G Z in the previous example modify in the following format:2212122212212122211111112121Q Q GQ GQ GQ Q Q GQ Z GQ Z GQ Z Z Q Q Q Q G ++++*+*+*=Among them, 21Q Q known as the separation distance of the known point, 21Q Q Z is the density of plastic garbage which is the plastic waste density of 1Q and 2Q for the linear trend of point G . For the two-dimensional area, point G is not on the line 21Q Q , so we make a vertical from the point G and cross the line connect the point 1Q and 2Q , and get point P , the impact of point P to 1Q and 2Q just like one-dimensional, and the one-dimensional closer of G to P , the distant of G to P become farther, the smaller of the impact, so the weighting factor should also reflect the GP in inversely proportional to a certain way, then we adopt following format:221212222122121222211111112121Q Q GQ GP GQ GQ Q Q GQ GP Z GQ Z GQ Z Z P Q Q Q Q G ++++++*+*+*=Taken together, we speculated following roles:(1) Each known point data are influence the density of plastic garbage of each unknown point in the inversely proportional to the square of the distance;(2) the change of density of plastic garbage between any two known points data, for each unknown point are affected, and the influence to each particular point of their plastic garbage diffuse the straight line along the two known particular point; (3) the change of the density of plastic garbage between any two known data points impact a specific unknown points of the density of plastic litter depends on the three distances: a. the vertical distance to a straight line which is a specific point link to a known point;b. the distance between the latest known point to a specific unknown point;c. the separation distance between two known data points.If we mark 1Q ,2Q ,…,N Q as the location of known data points,G as an unknown node, ijG P is the intersection of the connection of i Q ,j Q and the vertical line from G to i Q ,j Q()G Q Q Z j i ,,is the density trend of i Q ,j Q in the of plasticgarbage points and prescribe ()G Q Q Z j i ,,is the testing point i Q ’ s density of plastic garbage ,so there are calculation formula:()()∑∑∑∑==-==++++*=Ni N ij ji i ijGji i ijG N i Nj j i G Q Q GQ GPQ Q GQ GP G Q Q Z Z 11222222111,,Here we plug each year’s observational data in schedule 1 into our model, and draw the three-dimensional images of the spatial distribution of the marine garbage ’s density with Matlab in Figure (2) as follows:199920002002200520062007-2008(1)It’s observed and analyzed that, from 1999 to 2008, the density of plastic garbage is increasing year by year and significantly in the region of East – West 140W-150W, south - north 30N-40N. Therefore, we can make sure that this region is probably the center of the marine litter whirlpool. Gathering process should be such that the dispersed garbage floating in the ocean move with the ocean currents and gradually close to the whirlpool region. At the beginning, the area close to the vortex will have obviously increasable about plastic litter density, because of this centripetal they keeping move to the center of the vortex ,then with the time accumulates ,the garbage density in the center of the vortex become much bigger and bigger , at last it becomes the Pacific rubbish island we have seen today.It can be seen that through our algorithm, as long as the reference to be able to detect the density in an area which has a number of discrete measuring points,Through tracking these density changes ,we Will be able to value out all the waters of the density measurement through our models to determine,This will reduce the workload of the marine expedition team monitoring marine pollution significantly, and also saving costs .Task 3:The degradation mechanism of marine plasticsWe know that light, mechanical force, heat, oxygen, water, microbes, chemicals, etc. can result in the degradation of plastics . In mechanism ,Factors result in the degradation can be summarized as optical ,biological,and chemical。
2014年美国大学生数学建模MCM-B题O奖论文
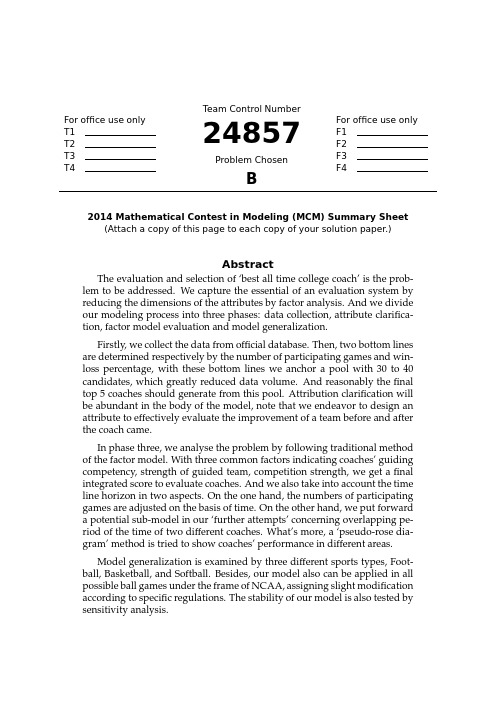
For office use only T1T2T3T4T eam Control Number24857Problem ChosenBFor office use onlyF1F2F3F42014Mathematical Contest in Modeling(MCM)Summary Sheet (Attach a copy of this page to each copy of your solution paper.)AbstractThe evaluation and selection of‘best all time college coach’is the prob-lem to be addressed.We capture the essential of an evaluation system by reducing the dimensions of the attributes by factor analysis.And we divide our modeling process into three phases:data collection,attribute clarifica-tion,factor model evaluation and model generalization.Firstly,we collect the data from official database.Then,two bottom lines are determined respectively by the number of participating games and win-loss percentage,with these bottom lines we anchor a pool with30to40 candidates,which greatly reduced data volume.And reasonably thefinal top5coaches should generate from this pool.Attribution clarification will be abundant in the body of the model,note that we endeavor to design an attribute to effectively evaluate the improvement of a team before and after the coach came.In phase three,we analyse the problem by following traditional method of the factor model.With three common factors indicating coaches’guiding competency,strength of guided team,competition strength,we get afinal integrated score to evaluate coaches.And we also take into account the time line horizon in two aspects.On the one hand,the numbers of participating games are adjusted on the basis of time.On the other hand,we put forward a potential sub-model in our‘further attempts’concerning overlapping pe-riod of the time of two different coaches.What’s more,a‘pseudo-rose dia-gram’method is tried to show coaches’performance in different areas.Model generalization is examined by three different sports types,Foot-ball,Basketball,and Softball.Besides,our model also can be applied in all possible ball games under the frame of NCAA,assigning slight modification according to specific regulations.The stability of our model is also tested by sensitivity analysis.Who’s who in College Coaching Legends—–A generalized Factor Analysis approach2Contents1Introduction41.1Restatement of the problem (4)1.2NCAA Background and its coaches (4)1.3Previous models (4)2Assumptions5 3Analysis of the Problem5 4Thefirst round of sample selection6 5Attributes for evaluating coaches86Factor analysis model106.1A brief introduction to factor analysis (10)6.2Steps of Factor analysis by SPSS (12)6.3Result of the model (14)7Model generalization15 8Sensitivity analysis189Strength and Weaknesses199.1Strengths (19)9.2Weaknesses (19)10Further attempts20 Appendices22 Appendix A An article for Sports Illustrated221Introduction1.1Restatement of the problemThe‘best all time college coach’is to be selected by Sports Illustrated,a magazine for sports enthusiasts.This is an open-ended problem—-no limitation in method of performance appraisal,gender,or sports types.The following research points should be noted:•whether the time line horizon that we use in our analysis make a difference;•the metrics for assessment are to be articulated;•discuss how the model can be applied in general across both genders and all possible sports;•we need to present our model’s Top5coaches in each of3different sports.1.2NCAA Background and its coachesNational Collegiate Athletic Association(NCAA),an association of1281institution-s,conferences,organizations,and individuals that organizes the athletic programs of many colleges and universities in the United States and Canada.1In our model,only coaches in NCAA are considered and ranked.So,why evaluate the Coaching performance?As the identity of a college football program is shaped by its head coach.Given their impacts,it’s no wonder high profile athletic departments are shelling out millions of dollars per season for the services of coaches.Nick Saban’s2013total pay was$5,395,852and in the same year Coach K earned$7,233,976in total23.Indeed,every athletic director wants to hire the next legendary coach.1.3Previous modelsTraditionally,evaluation in athletics has been based on the single criterion of wins and losses.Years later,in order to reasonably evaluate coaches,many reseachers have implemented the coaching evaluation model.Such as7criteria proposed by Adams:[1] (1)the coach in the profession,(2)knowledge of and practice of medical aspects of coaching,(3)the coach as a person,(4)the coach as an organizer and administrator,(5) knowledge of the sport,(6)public relations,and(7)application of kinesiological and physiological principles.1Wikipedia:/wiki/National_Collegiate_Athletic_ Association#NCAA_sponsored_sports2USAToday:/sports/college/salaries/ncaaf/coach/ 3USAToday:/sports/college/salaries/ncaab/coach/Such models relatively focused more on some subjective and difficult-to-quantify attributes to evaluate coaches,which is quite hard for sports fans to judge coaches. Therefore,we established an objective and quantified model to make a list of‘best all time college coach’.2Assumptions•The sample for our model is restricted within the scale of NCAA sports.That is to say,the coaches we discuss refers to those service for NCAA alone;•We do not take into account the talent born varying from one player to another, in this case,we mean the teams’wins or losses purely associate with the coach;•The difference of games between different Divisions in NCAA is ignored;•Take no account of the errors/amendments of the NCAA game records.3Analysis of the ProblemOur main goal is to build and analyze a mathematical model to choose the‘best all time college coach’for the previous century,i.e.from1913to2013.Objectively,it requires numerous attributes to judge and specify whether a coach is‘the best’,while many of the indicators are deemed hard to quantify.However,to put it in thefirst place, we consider a‘best coach’is,and supposed to be in line with several basic condition-s,which are the prerequisites.Those prerequisites incorporate attributes such as the number of games the coach has participated ever and the win-loss percentage of the total.For instance,under the conditions that either the number of participating games is below100,or the win-loss percentage is less than0.5,we assume this coach cannot be credited as the‘best’,ignoring his/her other facets.Therefore,an attempt was made to screen out the coaches we want,thus to narrow the range in ourfirst stage.At the very beginning,we ignore those whose guiding ses-sions or win-loss percentage is less than a certain level,and then we determine a can-didate pool for‘the best coach’of30-40in scale,according to merely two indicators—-participating games and win-loss percentage.It should be reasonably reliable to draw the top5best coaches from this candidate pool,regardless of any other aspects.One point worth mentioning is that,we take time line horizon as one of the inputs because the number of participating games is changing all the time in the previous century.Hence,it would be unfair to treat this problem by using absolute values, especially for those coaches who lived in the earlier ages when sports were less popular and games were sparse comparatively.4Thefirst round of sample selectionCollege Football is thefirst item in our research.We obtain data concerning all possible coaches since it was initiated,of which the coaches’tenures,participating games and win-loss percentage etc.are included.As a result,we get a sample of2053in scale.Thefirst10candidates’respective information is as below:Table1:Thefirst10candidates’information,here Pct means win-loss percentageCoach From To Years Games Wins Losses Ties PctEli Abbott19021902184400.5Earl Abell19281930328141220.536Earl Able1923192421810620.611 George Adams1890189233634200.944Hobbs Adams1940194632742120.185Steve Addazio20112013337201700.541Alex Agase1964197613135508320.378Phil Ahwesh19491949193600.333Jim Aiken19461950550282200.56Fred Akers19751990161861087530.589 ...........................Firstly,we employ Excel to rule out those who begun their coaching career earlier than1913.Next,considering the impact of time line horizon mentioned in the problem statement,we import our raw data into MATLAB,with an attempt to calculate the coaches’average games every year versus time,as delineated in the Figure1below.Figure1:Diagram of the coaches’average sessions every year versus time It can be drawn from thefigure above,clearly,that the number of each coach’s average games is related with the participating time.With the passing of time and the increasing popularity of sports,coaches’participating games yearly ascends from8to 12or so,that is,the maximum exceed the minimum for50%around.To further refinethe evaluation method,we make the following adjustment for coaches’participating games,and we define it as each coach’s adjusted participating games.Gi =max(G i)G mi×G iWhere•G i is each coach’s participating games;•G im is the average participating games yearly in his/her career;and•max(G i)is the max value in previous century as coaches’average participating games yearlySubsequently,we output the adjusted data,and return it to the Excel table.Obviously,directly using all this data would cause our research a mass,and also the economy of description is hard to achieved.Logically,we propose to employ the following method to narrow the sample range.In general,the most essential attributes to evaluate a coach are his/her guiding ex-perience(which can be shown by participating games)and guiding results(shown by win-loss percentage).Fortunately,these two factors are the ones that can be quantified thus provide feasibility for our modeling.Based on our common sense and select-ed information from sports magazines and associated programs,wefind the winning coaches almost all bear the same characteristics—-at high level in both the partici-pating games and the win-loss percentage.Thus we may arbitrarily enact two bottom line for these two essential attributes,so as to nail down a pool of30to40candidates. Those who do not meet our prerequisites should not be credited as the best in any case.Logically,we expect the model to yield insight into how bottom lines are deter-mined.The matter is,sports types are varying thus the corresponding features are dif-ferent.However,it should be reasonably reliable to the sports fans and commentators’perceptual intuition.Take football as an example,win-loss percentage that exceeds0.75 should be viewed as rather high,and college football coaches of all time who meet this standard are specifically listed in Wikipedia.4Consequently,we are able tofix upon a rational pool of candidate according to those enacted bottom lines and meanwhile, may tender the conditions according to the total scale of the coaches.Still we use Football to further articulate,to determine a pool of candidates for the best coaches,wefirst plot thefigure below to present the distributions of all the coaches.From thefigure2,wefind that once the games number exceeds200or win-loss percentage exceeds0.7,the distribution of the coaches drops significantly.We can thus view this group of coaches as outstanding comparatively,meeting the prerequisites to be the best coaches.4Wikipedia:/wiki/List_of_college_football_coaches_ with_a_.750_winning_percentageFigure2:Hist of the football coaches’number of games versus and average games every year versus games and win-loss percentageHence,we nail down the bottom lines for both the games number and the win-loss percentage,that is,0.7for the former and200for the latter.And these two bottom lines are used as the measure for ourfirst round selection.After round one,merely35 coaches are qualified to remain in the pool of candidates.Since it’s thefirst round sifting,rather than direct and ultimate determination,we hence believe the subjectivity to some extent in the opt of bottom lines will not cloud thefinal results of the best coaches.5Attributes for evaluating coachesThen anchored upon the35candidate selected,we will elaborate our coach evaluation system based on8attributes.In the indicator-select process,we endeavor to examine tradeoffs among the availability for data and difficulty for data quantification.Coaches’pay,for example,though serves as the measure for coaching evaluation,the corre-sponding data are limited.Situations are similar for attributes such as the number of sportsmen the coach ever cultivated for the higher-level tournaments.Ultimately,we determine the8attributes shown in the table below:Further explanation:•Yrs:guiding years of a coach in his/her whole career•G’:Gi =max(G i)G mi×G i see it at last section•Pct:pct=wins+ties/2wins+losses+ties•SRS:a rating that takes into account average point differential and strength of schedule.The rating is denominated in points above/below average,where zeroTable2:symbols and attributessymbol attributeYrs yearsG’adjusted overall gamesPct win-lose percentageP’Adjusted percentage ratioSRS Simple Rating SystemSOS Strength of ScheduleBlp’adjusted Bowls participatedBlw’adjusted Bowls wonis the average.Note that,the bigger for this value,the stronger for the team performance.•SOS:a rating of strength of schedule.The rating is denominated in points above/below average,where zero is the average.Noted that the bigger for this value,the more powerful for the team’s rival,namely the competition is more fierce.Sports-reference provides official statistics for SRS and SOS.5•P’is a new attribute designed in our model.It is the result of Win-loss in the coach’s whole career divided by the average of win-loss percentage(weighted by the number of games in different colleges the coach ever in).We bear in mind that the function of a great coach is not merely manifested in the pure win-loss percentage of the team,it is even more crucial to consider the improvement of the team’s win-loss record with the coach’s participation,or say,the gap between‘af-ter’and‘before’period of this team.(between‘after’and‘before’the dividing line is the day the coach take office)It is because a coach who build a comparative-ly weak team into a much more competitive team would definitely receive more respect and honor from sports fans.To measure and specify this attribute,we col-lect the key official data from sports-reference,which included the independent win-loss percentage for each candidate and each college time when he/she was in the team and,the weighted average of all time win-loss percentage of all the college teams the coach ever in—-regardless of whether the coach is in the team or not.To articulate this attribute,here goes a simple physical example.Ike Armstrong (placedfirst when sorted by alphabetical order),of which the data can be ob-tained from website of sports-reference6.We can easily get the records we need, namely141wins,55losses,15ties,and0.704for win-losses percentage.Fur-ther,specific wins,losses,ties for the team he ever in(Utab college)can also be gained,respectively they are602,419,30,0.587.Consequently,the P’value of Ike Armstrong should be0.704/0.587=1.199,according to our definition.•Bowl games is a special event in thefield of Football games.In North America,a bowl game is one of a number of post-season college football games that are5sports-reference:/cfb/coaches/6sports-reference:/cfb/coaches/ike-armstrong-1.htmlprimarily played by teams from the Division I Football Bowl Subdivision.The times for one coach to eparticipate Bowl games are important indicators to eval-uate a coach.However,noted that the total number of Bowl games held each year is changing from year to year,which should be taken into consideration in the model.Other sports events such as NCAA basketball tournament is also ex-panding.For this reason,it is irrational to use the absolute value of the times for entering the Bowl games (or NCAA basketball tournament etc.)and the times for winning as the evaluation measurement.Whereas the development history and regulations for different sports items vary from one to another (actually the differentiation can be fairly large),we here are incapable to find a generalized method to eliminate this discrepancy ,instead,in-dependent method for each item provide a way out.Due to the time limitation for our research and the need of model generalization,we here only do root extract of blp and blw to debilitate the differentiation,i.e.Blp =√Blp Blw =√Blw For different sports items,we use the same attributes,except Blp’and Blw’,we may change it according to specific sports.For instance,we can use CREG (Number of regular season conference championship won)and FF (Number of NCAA Final Four appearance)to replace Blp and Blw in basketball games.With all the attributes determined,we organized data and show them in the table 3:In addition,before forward analysis there is a need to preprocess the data,owing to the diverse dimensions between these indicators.Methods for data preprocessing are a lot,here we adopt standard score (Z score)method.In statistics,the standard score is the (signed)number of standard deviations an observation or datum is above the mean.Thus,a positive standard score represents a datum above the mean,while a negative standard score represents a datum below the mean.It is a dimensionless quantity obtained by subtracting the population mean from an individual raw score and then dividing the difference by the population standard deviation.7The standard score of a raw score x is:z =x −µσIt is easy to complete this process by statistical software SPSS.6Factor analysis model 6.1A brief introduction to factor analysisFactor analysis is a statistical method used to describe variability among observed,correlated variables in terms of a potentially lower number of unobserved variables called factors.For example,it is possible that variations in four observed variables mainly reflect the variations in two unobserved variables.Factor analysis searches for 7Wikipedia:/wiki/Standard_scoreTable3:summarized data for best college football coaches’candidatesCoach From To Yrs G’Pct Blp’Blw’P’SRS SOS Ike Armstrong19251949252810.70411 1.199 4.15-4.18 Dana Bible19151946313860.7152 1.73 1.0789.88 1.48 Bernie Bierman19251950242780.71110 1.29514.36 6.29 Red Blaik19341958252940.75900 1.28213.57 2.34 Bobby Bowden19702009405230.74 5.74 4.69 1.10314.25 4.62 Frank Broyles19571976202570.7 3.162 1.18813.29 5.59 Bear Bryant19451982385080.78 5.39 3.87 1.1816.77 6.12 Fritz Crisler19301947182080.76811 1.08317.15 6.67 Bob Devaney19571972162080.806 3.16 2.65 1.25513.13 2.28 Dan Devine19551980222800.742 3.16 2.65 1.22613.61 4.69 Gilmour Dobie19161938222370.70900 1.27.66-2.09 Bobby Dodd19451966222960.713 3.613 1.18414.25 6.6 Vince Dooley19641988253250.715 4.47 2.83 1.09714.537.12 Gus Dorais19221942192320.71910 1.2296-3.21 Pat Dye19741992192400.707 3.16 2.65 1.1929.68 1.51 LaVell Edwards19722000293920.716 4.69 2.65 1.2437.66-0.66 Phillip Fulmer19922008172150.743 3.87 2.83 1.08313.42 4.95 Woody Hayes19511978283290.761 3.32 2.24 1.03117.418.09 Frank Kush19581979222710.764 2.65 2.45 1.238.21-2.07 John McKay19601975162070.7493 2.45 1.05817.298.59 Bob Neyland19261952212860.829 2.65 1.41 1.20815.53 3.17 Tom Osborne19731997253340.8365 3.46 1.18119.7 5.49 Ara Parseghian19561974192250.71 2.24 1.73 1.15317.228.86 Joe Paterno19662011465950.749 6.08 4.9 1.08914.01 5.01 Darrell Royal19541976232970.7494 2.83 1.08916.457.09 Nick Saban19902013182390.748 3.74 2.83 1.12313.41 3.86 Bo Schembechler19631989273460.775 4.12 2.24 1.10414.86 3.37 Francis Schmidt19221942212670.70800 1.1928.490.16 Steve Spurrier19872013243160.733 4.363 1.29313.53 4.64 Bob Stoops19992013152070.804 3.74 2.65 1.11716.66 4.74 Jock Sutherland19191938202550.81221 1.37613.88 1.68 Barry Switzer19731988162090.837 3.61 2.83 1.16320.08 6.63 John Vaught19471973253210.745 4.24 3.16 1.33814.7 5.26 Wallace Wade19231950243070.765 2.24 1.41 1.34913.53 3.15 Bud Wilkinson19471963172220.826 2.83 2.45 1.14717.54 4.94 such joint variations in response to unobserved latent variables.The observed vari-ables are modelled as linear combinations of the potential factors,plus‘error’terms. The information gained about the interdependencies between observed variables can be used later to reduce the set of variables in a putationally this technique is equivalent to low rank approximation of the matrix of observed variables.8 Why carry out factor analyses?If we can summarise a multitude of measure-8Wikipedia:/wiki/Factor_analysisments with a smaller number of factors without losing too much information,we have achieved some economy of description,which is one of the goals of scientific investi-gation.It is also possible that factor analysis will allow us to test theories involving variables which are hard to measure directly.Finally,at a more prosaic level,factor analysis can help us establish that sets of questionnaire items(observed variables)are in fact all measuring the same underlying factor(perhaps with varying reliability)and so can be combined to form a more reliable measure of that factor.6.2Steps of Factor analysis by SPSSFirst we import the decided datasets of8attributes into SPSS,and the results can be obtained below after the software processing.[2-3]Figure3:Table of total variance explainedFigure4:Scree PlotThefirst table and scree plot shows the eigenvalues and the amount of variance explained by each successive factor.The remaining5factors have small eigenvalues value.Once the top3factors are extracted,it adds up to84.3%,meaning a great as the explanatory ability for the original information.To reflect the quantitative analysis of the model,we obtain the following factor loading matrix,actually the loadings are in corresponding to the weight(α1,α2 (i)the set ofx i=αi1f1+αi2f2+...+αim f j+εiAnd the relative strength of the common factors and the original attribute can also be manifested.Figure5:Rotated Component MatrixThen,with Rotated Component Matrix above,wefind the common factor F1main-ly expresses four attributes they are:G,Yrs,P,SRS,and logically,we define the com-mon factor generated from those four attributes as the guiding competency of the coach;similarly,the common factor F2mainly expresses two attributes,and they are: Pct and Blp,which can be de defined as the integrated strength of the guided team; while the common factor F3,mainly expresses two attributes:SOS and Blw,which can be summarized into a‘latent attribute’named competition strength.In order to obtain the quantitative relation,we get the following Component Score Coefficient Matrix processed by SPSS.Further,the function of common factors and the original attributes is listed as bel-low:F1=0.300x1+0.312x2+0.023x3+0.256x4+0.251x5+0.060x6−0.035x7−0.053x8F2=−0.107x1−0,054x2+0.572x3+0.103x4+0.081x5+0.280x6+0.372x7+0.142x8 F3=−0.076x1−0,098x2−0.349x3+0.004x4+0.027x5−0.656x6+0.160x7+0.400x8 Finally we calculate out the integrated factor scores,which should be the average score weighted by the corresponding proportion of variance contribution of each com-mon factor in the total variance contribution.And the function set should be:F=0.477F1+0.284F2+0.239F3Figure6:Component Score Coefficient Matrix6.3Result of the modelwe rank all the coaches in the candidate pool by integrated score represented by F.Seetable4:Table4:Integrated scores for best college football coach(show15data due to the limi-tation of space)Rank coaches F1F2F3Integrated factor1Joe Paterno 3.178-0.3150.421 1.3622Bobby Bowden 2.51-0.2810.502 1.1113Bear Bryant 2.1420.718-0.142 1.0994Tom Osborne0.623 1.969-0.2390.8205Woody Hayes0.140.009 1.6130.4846Barry Switzer-0.705 2.0360.2470.4037Darrell Royal0.0460.161 1.2680.4018Vince Dooley0.361-0.442 1.3730.3749Bo Schembechler0.4810.1430.3040.32910John Vaught0.6060.748-0.870.26511Steve Spurrier0.5180.326-0.5380.18212Bob Stoops-0.718 1.0850.5230.17113Bud Wilkinson-0.718 1.4130.1050.16514Bobby Dodd0.08-0.2080.7390.16215John McKay-0.9620.228 1.870.151Based on this model,we can make a scientific rank list for US college football coach-es,the Top5coaches of our model is Joe Paterno,Bobby Bowden,Bear Bryant,TomOsborne,Woody Hayes.In order to confirm our result,we get a official list of bestcollege football coaches from Bleacherreport99Bleacherreport:/articles/890705-college-football-the-top-50-coTable5:The result of our model in football,the last column is official college basketball ranking from bleacherreportRank Our model Integrated scores bleacherreport1Joe Paterno 1.362Bear Bryant2Bobby Bowden 1.111Knute Rockne3Bear Bryant 1.099Tom Osborne4Tom Osborne0.820Joe Paterno5Woody Hayes0.484Bobby Bowden By comparing thoes two ranking list,wefind that four of our Top5coaches ap-peared in the offical Top5list,which shows that our model is reasonable and effective.7Model generalizationOur coach evaluation system model,of which the feasibility of generalization is sat-isfying,can be accommodated to any possible NCAA sports concourses by assigning slight modification concerning specific regulations.Besides,this method has nothing to do with the coach’s gender,or say,both male and female coaches can be rationally evaluated by this system.And therefore we would like to generalize this model into softball.Further,we take into account the time line horizon,making corresponding adjust-ment for the indicator of number of participating games so as to stipulate that the evaluation measure for1913and2013would be the same.To further generalize the model,first let’s have a test in basketball,of which the data available is adequate enough as football.And the specific steps are as following:1.Obtain data from sports-reference10and rule out the coaches who begun theircoaching career earlier than1913.2.Calculate each coach’s adjusted number of participating games,and adjust theattribute—-FF(Number of NCAA Final Four appearance).3.Determine the bottom lines for thefirst round selection to get a pool of candidatesaccording to the coaches’participating games and win-loss percentage,and the ideal volumn of the pool should be from30to40.Hist diagrams are as below: We determine800as the bottom line for the adjusted participating games and0.7 for the win-loss percentage.Coincidently,we get a candidate pool of35in scale.4.Next,we collect the corresponding data of candidate coaches(P’,SRS,SOS etc.),as presented in the table6:5.Processed by z score method and factor analysis based on the8attributes anddata above,we get three common factors andfinal integrated scores.And among 10sports-reference:/cbb/coaches/Figure7:Hist of the basketball coaches’number of games versus and average gamesevery year versus games and win-loss percentagethe top5candidates,Mike Krzyzewski,Adolph Rupp,Dean SmithˇcˇnBob Knightare the same with the official statistics from bleacherreport.11We can say theeffectiveness of the model is pretty good.See table5.We also apply similar approach into college softball.Maybe it is because the popularity of the softball is not that high,the data avail-able is not adequate to employ ourfirst model.How can our model function in suchsituation?First and foremost,specialized magazines like Sports Illustrated,its com-mentators there would have more internal and confidential databases,which are notexposed publicly.On the one hand,as long as the data is adequate enough,we can saythe original model is completely feasible.While under the situation that there is datadeficit,we can reasonably simplify the model.The derivation of the softball data is NCAA’s official websites,here we only extractdata from All-Division part.12Softball is a comparatively young sports,hence we may arbitrarily neglect the re-stricted condition of‘100years’.Subsequently,because of the data deficit it is hard toadjust the number of participating games.We may as well determine10as the bottomline for participating games and0.74for win-loss percentage,producing a candidatepool of33in scaleAttributed to the inadequacy of the data for attributes,it is not convenient to furtheruse the factor analysis similarly as the assessment model.Therefore,here we employsolely two of the most important attributes to evaluate a coach and they are:partic-ipating games and win-loss percentage in the coach’s whole career.Specifically,wefirst adopt z score to normalize all the data because of the differentiation of various dimensions,and then the integrated score of the coach can be reached by the weighted11bleacherreport:/articles/1341064-10-greatest-coaches-in-ncaa-b 12NCAA softball Coaching Record:/Docs/stats/SB_Records/2012/coaches.pdf。
2016年美赛A题O奖论文
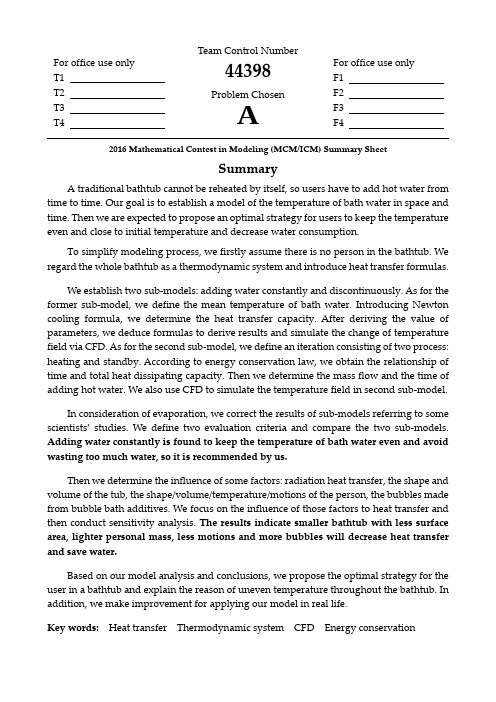
2016 Mathematical Contest in Modeling (MCM/ICM) Summary Sheet SummaryA traditional bathtub cannot be reheated by itself, so users have to add hot water from time to time. Our goal is to establish a model of the temperature of bath water in space and time. Then we are expected to propose an optimal strategy for users to keep the temperature even and close to initial temperature and decrease water consumption.To simplify modeling process, we firstly assume there is no person in the bathtub. We regard the whole bathtub as a thermodynamic system and introduce heat transfer formulas.We establish two sub-models: adding water constantly and discontinuously. As for the former sub-model, we define the mean temperature of bath water. Introducing Newton cooling formula, we determine the heat transfer capacity. After deriving the value of parameters, we deduce formulas to derive results and simulate the change of temperature field via CFD. As for the second sub-model, we define an iteration consisting of two process: heating and standby. According to energy conservation law, we obtain the relationship of time and total heat dissipating capacity. Then we determine the mass flow and the time of adding hot water. We also use CFD to simulate the temperature field in second sub-model.In consideration of evaporation, we correct the results of sub-models referring to some scientists’ studies. We define two evaluation criteria and compare the two sub-models. Adding water constantly is found to keep the temperature of bath water even and avoid wasting too much water, so it is recommended by us.Then we determine the influence of some factors: radiation heat transfer, the shape and volume of the tub, the shape/volume/temperature/motions of the person, the bubbles made from bubble bath additives. We focus on the influence of those factors to heat transfer and then conduct sensitivity analysis. The results indicate smaller bathtub with less surface area, lighter personal mass, less motions and more bubbles will decrease heat transfer and save water.Based on our model analysis and conclusions, we propose the optimal strategy for the user in a bathtub and explain the reason of uneven temperature throughout the bathtub. In addition, we make improvement for applying our model in real life.Key words: Heat transfer Thermodynamic system CFD Energy conservation For office use onlyT1 T2 T3 T4 For office use only F1 F2F3 F4 Team Control Number 44398 Problem Chosen AEnjoy a Cozy and Green BathContents1 Introduction (4)1.1 Background (4)1.2Literature Review (4)1.3Restatement of the Problem (4)2Assumptions and Justification (6)3Notations (7)4Model Overview (7)5 Sub-model I : Adding Water Continuously (8)5.1 Model Establishment (9)5.1.1 Control Equations and Boundary Conditions (9)5.1.2 Definition of the Mean Temperature (11)5.1.3 Determination of Heat Transfer Capacity (11)5.2 Results (13)5.2.1 Determination of Parameters (13)5.2.2 Calculating Results (14)5.2.3 Simulating Results (15)6 Sub-model II: Adding Water Discontinuously (18)6.1 Heating Model (18)6.1.1 Control Equations and Boundary Conditions (18)6.1.2 Determination of Inflow Time and Amount (19)6.2 Standby Model (20)6.2.1 Process Analysis (20)6.2.2 Calculation of Parameters (20)6.3 Results (21)6.3.1 Determination of Parameters (21)6.3.2 Calculating Results (23)6.3.3 Simulating Results (23)6.4 Conclusion (27)7 Correction and Contrast of Sub-Models (27)7.1 Correction with Evaporation Heat Transfer (27)7.1.1 Correction Principle (27)7.1.2 Correction Results (28)7.2 Contrast of Two Sub-Models (30)7.2.1 Evaluation Criteria (30)7.2.2 Determination of Water Consumption (30)7.2.3 Conclusion (31)8 Model Analysis and Sensitivity Analysis (31)8.1 The Influence of Different Bathtubs (32)8.1.1 Different Volumes of Bathtubs (32)8.1.2 Different Shapes of Bathtubs (34)8.2 The Influence of Person in Bathtub (36)8.2.1 When the Person Remains Static in a Bathtub (36)8.2.2 When the Person Moves in a Bathtub (37)8.2.3 Results Analysis and Sensitivity Analysis (38)8.3 The Influence of Bubble Bath Additives (42)8.4 The Influence of Radiation Heat Transfer (44)8.5 Conclusion (45)9 Further Discussion (45)9.1 Different Distribution of Inflow Faucets (45)9.2 Model Application (46)10 Strength and Weakness (47)10.1 Strength (47)10.2 Weakness (47)Report (49)Reference (50)。
建模美赛获奖范文

建模美赛获奖范文全文共四篇示例,供读者参考第一篇示例:近日,我校数学建模团队在全国大学生数学建模竞赛中荣获一等奖的喜讯传来,这是我校首次在该比赛中获得如此优异的成绩。
本文将从建模过程、团队合作、参赛经验等方面进行详细介绍,希望能为更多热爱数学建模的同学提供一些借鉴和参考。
让我们来了解一下比赛的背景和要求。
全国大学生数学建模竞赛是由中国工程院主办,旨在促进大学生对数学建模的兴趣和掌握数学建模的基本方法和技巧。
比赛通常会设置一些实际问题,参赛队伍需要在规定时间内通过建立数学模型、分析问题、提出解决方案等步骤来完成任务。
最终评选出的优胜队伍将获得一等奖、二等奖等不同级别的奖项。
在本次比赛中,我们团队选择了一道关于城市交通拥堵研究的题目,并从交通流理论、路网优化等角度进行建模和分析。
通过对城市交通流量、拥堵原因、路段限制等方面的研究,我们提出了一种基于智能交通系统的解决方案,有效缓解了城市交通拥堵问题。
在展示环节,我们通过图表、数据分析等方式清晰地呈现了我们的建模过程和成果,最终赢得了评委的认可。
在整个建模过程中,团队合作起着至关重要的作用。
每个成员都发挥了自己的专长和优势,在分析问题、建模求解、撰写报告等方面各司其职。
团队内部的沟通和协作非常顺畅,大家都能积极提出自己的想法和看法,达成共识后再进行实际操作。
通过团队合作,我们不仅完成了比赛的任务,也培养了团队精神和合作能力,这对我们日后的学习和工作都具有重要意义。
参加数学建模竞赛是一次非常宝贵的经历,不仅能提升自己的数学建模能力,也能锻炼自己的解决问题的能力和团队协作能力。
在比赛的过程中,我们学会了如何快速建立数学模型、如何分析和解决实际问题、如何展示自己的成果等,这些能力对我们未来的学习和工作都将大有裨益。
在未来,我们将继续努力,在数学建模领域不断学习和提升自己的能力,为更多的实际问题提供有效的数学解决方案。
我们也希望通过自己的经验和教训,为更多热爱数学建模的同学提供一些指导和帮助,共同进步,共同成长。
数学建模竞赛优秀大学生论文.doc
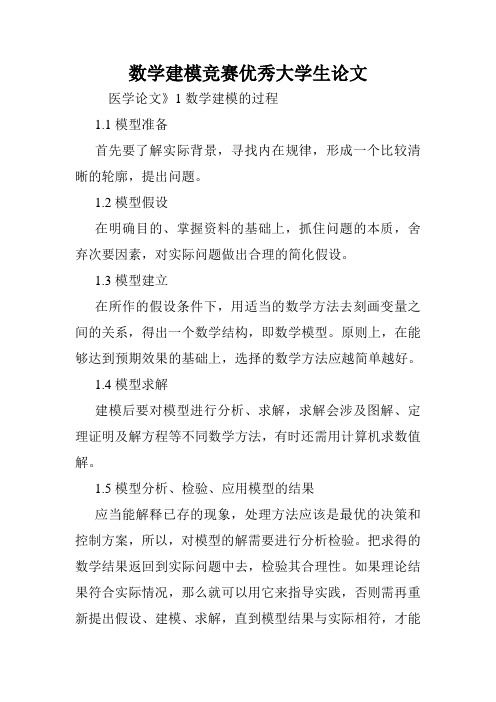
数学建模竞赛优秀大学生论文医学论文》1数学建模的过程1.1模型准备首先要了解实际背景,寻找内在规律,形成一个比较清晰的轮廓,提出问题。
1.2模型假设在明确目的、掌握资料的基础上,抓住问题的本质,舍弃次要因素,对实际问题做出合理的简化假设。
1.3模型建立在所作的假设条件下,用适当的数学方法去刻画变量之间的关系,得出一个数学结构,即数学模型。
原则上,在能够达到预期效果的基础上,选择的数学方法应越简单越好。
1.4模型求解建模后要对模型进行分析、求解,求解会涉及图解、定理证明及解方程等不同数学方法,有时还需用计算机求数值解。
1.5模型分析、检验、应用模型的结果应当能解释已存的现象,处理方法应该是最优的决策和控制方案,所以,对模型的解需要进行分析检验。
把求得的数学结果返回到实际问题中去,检验其合理性。
如果理论结果符合实际情况,那么就可以用它来指导实践,否则需再重新提出假设、建模、求解,直到模型结果与实际相符,才能进行实际应用。
总之,数学建模是一项富有创造性的工作,不可能用一些条条框框的规则规定的十分死板,只要是能够做到全面兼顾、能抓住问题的本质、最终检验结果合理,都是一个好的数学模型。
2数学建模在生物医学中的应用2.1DNA序列分类模型DNA分子是遗传信息存储的基本单位,许多生命科学中的重大问题都依赖于对这种特殊分子的深入了解。
因此,关于DNA分子结构与功能的问题,成为二十一世纪最重大的课题之一。
DNA序列分类问题是研究DNA分子结构的基础,它常用的方法是聚类分析法。
聚类分析是使用数据建模简化数据的一种方法,它将数据分成不同的类或者簇,同一个簇中的数据有很大的同质性,而不同的簇中的数据有很大的相异性。
在对DNA序列进行分类时,需首先引入样品变量,比如说单个碱基的丰度、两碱基丰度之比等;然后计算出每条DNA序列的样品变量值,存入到向量中;最后根据相似度度量原理,计算出所有序列两两之间的Lance与Williams距离,依据距离的远近进行分类。
美赛数模论文
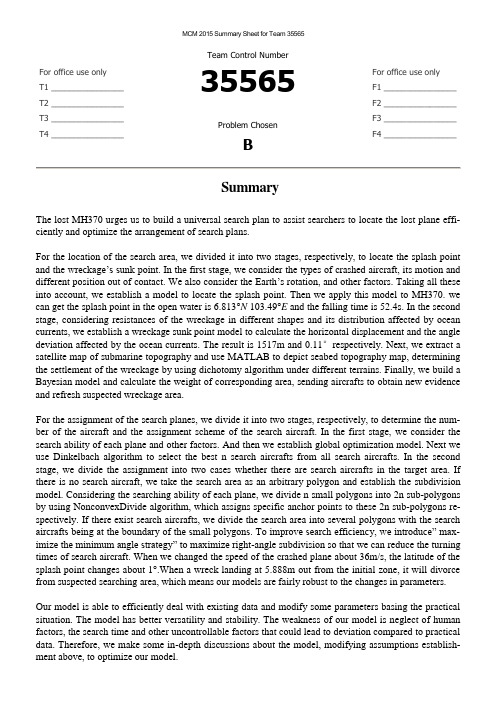
MCM 2015 Summary Sheet for Team 35565For office use onlyT1________________ T2________________ T3________________ T4________________Team Control Number35565Problem ChosenBFor office use onlyF1________________F2________________F3________________F4________________ SummaryThe lost MH370 urges us to build a universal search plan to assist searchers to locate the lost plane effi-ciently and optimize the arrangement of search plans.For the location of the search area, we divided it into two stages, respectively, to locate the splash point and the wreckage‟s sunk point. In the first stage, we consider the types of crashed aircraft, its motion and different position out of contact. We also consider the Earth‟s rotation, and other factors. Taking all these into account, we establish a model to locate the splash point. Then we apply this model to MH370. we can get the splash point in the open water is 6.813°N 103.49°E and the falling time is 52.4s. In the second stage, considering resistances of the wreckage in different shapes and its distribution affected by ocean currents, we establish a wreckage sunk point model to calculate the horizontal displacement and the angle deviation affected by the ocean currents. The result is 1517m and 0.11°respectively. Next, we extract a satellite map of submarine topography and use MATLAB to depict seabed topography map, determining the settlement of the wreckage by using dichotomy algorithm under different terrains. Finally, we build a Bayesian model and calculate the weight of corresponding area, sending aircrafts to obtain new evidence and refresh suspected wreckage area.For the assignment of the search planes, we divide it into two stages, respectively, to determine the num-ber of the aircraft and the assignment scheme of the search aircraft. In the first stage, we consider the search ability of each plane and other factors. And then we establish global optimization model. Next we use Dinkelbach algorithm to select the best n search aircrafts from all search aircrafts. In the second stage, we divide the assignment into two cases whether there are search aircrafts in the target area. If there is no search aircraft, we take the search area as an arbitrary polygon and establish the subdivision model. Considering the searching ability of each plane, we divide n small polygons into 2n sub-polygons by using NonconvexDivide algorithm, which assigns specific anchor points to these 2n sub-polygons re-spectively. If there exist search aircrafts, we divide the search area into several polygons with the search aircrafts being at the boundary of the small polygons. To improve search efficiency, we introduce” ma x-imize the minimum angle strategy” to maximize right-angle subdivision so that we can reduce the turning times of search aircraft. When we changed the speed of the crashed plane about 36m/s, the latitude of the splash point changes about 1°.When a wreck landing at 5.888m out from the initial zone, it will divorce from suspected searching area, which means our models are fairly robust to the changes in parameters. Our model is able to efficiently deal with existing data and modify some parameters basing the practical situation. The model has better versatility and stability. The weakness of our model is neglect of human factors, the search time and other uncontrollable factors that could lead to deviation compared to practical data. Therefore, we make some in-depth discussions about the model, modifying assumptions establish-Searching For a Lost PlaneControl#35565February 10, 2014Team # 35565 Page 3 of 47 Contents1 Introduction (5)1.1 Restatement of the Problem (5)1.2 Literature Review (6)2 Assumptions and Justifications (7)3 Notations (7)4 Model Overview (10)5 Modeling For Locating the Lost Plane (10)5.1 Modeling For Locating the Splash Poin t (11)5.1.1 Types of Planes (11)5.1.2 Preparation of the Model—Earth Rotation (12)5.1.3 Modeling (13)5.1.4 Solution of The Model (14)5.2 Modeling For Locating Wreckage (15)5.2.1 Assumptions of the Model (16)5.2.2 Preparation of the Model (16)5.2.3 Modeling (21)5.2.4 Solution of the Model (25)5.3 Verification of the Model (26)5.3.1 Verification of the Splash Point (26)5.3.2 Verification of the binary search algorithm (27)6 Modeling For Optimization of Search Plan (29)6.1 The Global Optimization Model (29)6.1.1 Preparation of the Model (29)6.1.2 Modeling (31)6.1.3 Solution of the Model (31)6.2 The Area Partition Algorithm (33)6.2.1 Preparation of the Model (33)6.2.2 Modeling (34)6.2.3 Solution of the Model (35)6.2.4 Improvement of the Model (36)7 Sensitivity Analysis (38)8 Further Discussions (39)9 Strengths and Weaknesses (41)9.1 Strengths (41)9.2 Weaknesses (42)10 Non-technical Paper (42)1 IntroductionAn airplane (informally plane) is a powered, fixed-wing aircraft that is propelled for-ward by thrust from a jet engine or propeller. Its main feature is fast and safe. Typi-cally, air travel is approximately 10 times safer than travel by car, rail or bus. Howev-er, when using the deaths per journey statistic, air travel is significantly more danger-ous than car, rail, or bus travel. In an aircraft crash, almost no one could survive [1]. Furthermore, the wreckage of the lost plane is difficult to find due to the crash site may be in the open ocean or other rough terrain.Thus, it will be exhilarating if we can design a model that can find the lost plane quickly. In this paper, we establish several models to find the lost plane in seawater and develop an op-timal scheme to assign search planes to model to locate the wreckage of the lost plane.1.1 Restatement of the ProblemWe are required to build a mathematical model to find the lost plane crashed in open water. We decompose the problem into three sub-problems:●Work out the position and distributions of the plane‟s wreckage●Arrange a mathematical scheme to schedule searching planesIn the first step, we seek to build a model with the inputs of altitude and other factors to locate the splash point on the sea-level. Most importantly, the model should reflect the process of the given plane. Then we can change the inputs to do some simulations. Also we can change the mechanism to apply other plane crash to our model. Finally, we can obtain the outputs of our model.In the second step, we seek to extend our model to simulate distribution of the plane wreckage and position the final point of the lost plane in the sea. We will consider more realistic factors such as ocean currents, characteristics of plane.We will design some rules to dispatch search planes to confirm the wreckage and de-cide which rule is the best.Then we attempt to adjust our model and apply it to lost planes like MH370. We also consider some further discussion of our model.1.2 Literature ReviewA model for searching the lost plane is inevitable to study the crashed point of the plane and develop a best scheme to assign search planes.According to Newton's second law, the simple types of projectile motion model can work out the splash point on the seafloor. We will analyze the motion state ofthe plane when it arrives at the seafloor considering the effect of the earth's rotation,After the types of projectile motion model was established, several scientists were devoted to finding a method to simulate the movement of wreckage. The main diffi-culty was to combine natural factors with the movement. Juan Santos-Echeandía introduced a differential equation model to simplify the difficulty [2]. Moreover,A. Boultif and D. Louër introduced a dichotomy iteration algorithm to circular compu-ting which can be borrowed to combine the motion of wreckage with underwater ter-rain [3]. Several conditions have to be fulfilled before simulating the movement: (1) Seawater density keeps unchanged despite the seawater depth. (2) The velocity of the wreck stay the same compared with velocity of the plane before it crashes into pieces.(3) Marine life will not affect our simulation. (4) Acting forceof seawater is a function of the speed of ocean currents.However the conclusion above cannot describe the wreckage zone accurately. This inaccuracy results from simplified conditions and ignoring the probability distribution of wreckage. In 1989, Stone et.al introduced a Bayesian search approach for searching problems and found the efficient search plans that maximize the probability of finding the target given a fixed time limit by maintaining an accurate target location probabil-ity density function, and by explicitly modeling the target‟s process model [4].To come up with a concrete dispatch plan. Xing Shenwei first simulated the model with different kinds of algorithm. [5] In his model, different searching planes are as-sessed by several key factors. Then based on the model established before, he use the global optimization model and an area partition algorithm to propose the number of aircrafts. He also arranged quantitative searching recourses according to the maxi-mum speed and other factors. The result shows that search operations can be ensured and effective.Further studies are carried out based on the comparison between model andreality.Some article illustrate the random error caused by assumptions.2 Assumptions and JustificationsTo simplify the problem, we make the following basic assumptions, each ofwhich is properly justified.●Utilized data is accuracy. A common modeling assumption.●We ignore the change of the gravitational acceleration. The altitude of anaircraft is less than 30 km [6]. The average radius of the earth is 6731.004km, which is much more than the altitude of an aircraft. The gravitational accele-ration changes weakly.●We assume that aeroengine do not work when a plane is out of contact.Most air crash resulted from engine failure caused by aircraft fault, bad weather, etc.●In our model, the angle of attack do not change in an air crash and thefuselage don’t wag from side to side. We neglect the impact of natural and human factors●We treat plane as a material point the moment it hit the sea-level. Thecrashing plane moves fast with a short time-frame to get into the water. The shape and volume will be negligible.●We assume that coefficient of air friction is a constant. This impact is neg-ligible compared with that of the gravity.●Planes will crash into wreckage instantly when falling to sea surface.Typically planes travel at highly speed and may happen explosion accident with water. So we ignore the short time.3 NotationsAll the variables and constants used in this paper are listed in Table 1 and Table 2.Table 1 Symbol Table–ConstantsSymbol DefinitionωRotational angular velocity of the earthg Gravitational accelerationr The average radius of the earthC D Coefficient of resistance decided by the angle of attack ρAtmospheric densityφLatitude of the lost contact pointμCoefficient of viscosityS0Area of the initial wrecking zoneS Area of the wrecking zoneS T Area of the searching zoneK Correction factorTable 2 Symbol Table-VariablesSymbol DefinitionF r Air frictionF g Inertial centrifugal forceF k Coriolis forceW Angular velocity of the crash planev r Relative velocity of the crash planev x Initial velocity of the surface layer of ocean currentsk Coefficient of fluid frictionF f Buoyancy of the wreckagef i Churning resistance of the wreckage from ocean currents f Fluid resistance opposite to the direction of motionG Gravity of the wreckageV Volume of the wreckageh Decent height of the wreckageH Marine depthS x Displacement of the wreckageS y Horizontal distance of S xα Deviation angle of factually final position of the wreckage s Horizontal distance between final point and splash point p Probability of a wreck in a given pointN The number of the searching planeTS ' The area of sea to be searched a i V ˆ The maximum speed of each planeai D The initial distance from sea to search planeai A The search ability of each plane is),(h T L i The maximum battery life of each plane isi L The mobilized times of each plane in the whole search )1(N Q Q a a ≤≤ The maximum number of search plane in the searching zone T(h) The time the whole action takes4 Model OverviewMost research for searching the lost plane can be classified as academic and practical. As practical methods are difficult to apply to our problem, we approach theproblem with academic techniques. Our study into the searching of the lost plane takes several approaches.Our basic model allows us to obtain the splash point of the lost plane. We focus on the force analysis of the plane. Then we We turn to simple types of projectile motion model. This model gives us critical data about the movement and serves as a stepping stone to our later study.The extended model views the problem based on the conclusion above. We run diffe-rential equation method and Bayesian search model to simulate the movement of wreckage. The essence of the model is the way to combine the effect of natural factors with distribution of the wreckage. Moreover, using distributing conditions, we treat size of the lost plane as “initial wreckage zone” so as to approximately describe the distribution. Thus, after considering the natural factors, we name the distribution of wreckage a “wreck zone” to minimize searching zone. While we name all the space needed to search “searching zone”.Our conclusive model containing several kinds of algorithm attempts to tackle a more realistic and more challenging problem. We add the global optimization model and an area partition algorithm to improve the efficiency of search aircrafts according to the area of search zone. An assessment of search planes consisting of search capabili-ties and other factors are also added. The Dinkelbach and NonConvexDivide algo-rithm for the solutions of the results are also added.We use the extended and conclusive model as a standard model to analyze the problem and all results have this two model at their cores.5 Modeling For Locating the Lost PlaneWe will start with the idea of the basic model. Then we present the Bayesian search model to get the position of the sinking point.5.1 Modeling For Locating the Splash PointThe basic model is a academic approach. A typical types of projectile behavior con-sists of horizontal and vertical motion. We also add another dimension consider-ing the effect of the earth's rotation. Among these actions, the force analysis is the most crucial part during descent from the point out of contact to the sea-level. Types of plane might impact trajectory of the crashing plane.5.1.1 Types of PlanesWe classify the planes into six groups [7]:●Helicopters: A helicopter is one of the most timesaving ways to transfer be-tween the city and airport, alternatively an easy way to reach remote destina-tions.●Twins Pistons: An economical aircraft range suitable for short distance flights.Aircraft seating capacity ranging from 3 to 8 passengers.●Turboprops: A wide range of aircraft suitable for short and medium distanceflights with a duration of up to 2-4 hours. Aircraft seating capacity ranging from 4 to 70 passengers.●Executive Jets:An Executive Jet is suitable for medium or long distanceflights. Aircraft seating capacity ranging from 4 to 16 passengers●Airliners:Large jet aircraft suitable for all kinds of flights. Aircraft seatingcapacity ranging from 50 to 400 passengers.●Cargo Aircrafts:Any type of cargo. Ranging from short notice flights carry-ing vital spare parts up to large cargo aircraft that can transport any volumin-ous goods.The lost plane may be one of these group. Then we extract the characteristics of planes into three essential factors: mass, maximum flying speed, volume. We use these three factors to abstract a variety of planes:●Mass: Planes of different product models have their own mass.●Maximum flying speed: Different planes are provided with kinds of me-chanical configuration, which will decide their properties such as flying speed.●Volume: Planes of distinct product models have different sizes and configura-tion, so the volume is definitive .5.1.2 Preparation of the Model —Earth RotationWhen considering the earth rotation, we should know that earth is a non-inertial run-ning system. Thus, mobile on the earth suffers two other non-inertial forces except air friction F r . They are inertial centrifugal force F g and Coriolis force F k . According to Newton ‟s second law of motion, the law of object relative motion to the earth is:Rotational angular velocity of the earth is very small, about .For a big mobile v r , it suffers far less inertial centrifugal force than Coriolis force, so we can ignore it. Thus, the equation can be approximated as follows:Now we establish a coordinate system: x axis z axis pointing to the east and south re-spectively, y axis vertical upward, then v r , ω and F r in the projection coordinate system are as follows:⎪⎪⎩⎪⎪⎨⎧++=⋅⋅-⋅⋅=++=kdt dz j dt dy i dt dx m v k j w kF j F i F F r rz ry rx r φωφωcos sinφis the latitude of the lost contact point of the lost plane. Put equation 1-3 and equa-tion 1-2 together, then the component of projectile movement in differential equation is:ma FF F k g r=++srad ⋅⨯=-5103.7ωmamv F r r =+ω2⎪⎪⎪⎩⎪⎪⎪⎨⎧+⋅=+⋅=+⎪⎭⎫ ⎝⎛+⋅-=m F dt dx w dt z d m F dt dx w dt y d m F dt dz dt dy w dtx d rz ry rx φφφφsin 2cos 2sin cos 22222225.1.3 ModelingConsidering the effect caused by earth rotation and air draught to plane when crashing to sea level, we analyze the force on the X axis by using Newton ‟s second law, the differential equation on x y and axis, we can conclude:In conclusion, we establish the earth rotation and types of projectile second order dif-ferential model:()⎪⎩⎪⎨⎧+-⋅'⋅⋅=''-⋅'+⋅'⋅⋅-=''-⋅'⋅⋅=''m gf y w m z m f z x w m y m f y w m x m obj 321cos 2cos sin 2sin 2.φφφφAccording to Coriolis theorem, we analyze the force of the plane on different direc-tions. By using the Newton ‟s laws of motion, we can work out the resultant accelera-tion on all directions:⎪⎪⎪⎪⎪⎪⎪⎪⎩⎪⎪⎪⎪⎪⎪⎪⎪⎨⎧'+'+'⋅'⋅⋅⨯+='+'+'⋅'⋅⋅⨯+='+'+'⋅'⋅⋅⨯+=⋅⋅-⋅⋅=⋅⨯=⋅'''⋅===-2222222225)()()(21)()()(21)()()(21cos sin 103.704.022z y x z c F f z y x y c F f z y x x c F f k j w s rad S y x F c D rz D ryD rx D ρρρφωφωωμφC D is the angle of attack of a plane flew in the best state, w is the angular speed of a moving object, vector j and k are the unit vector on y and z direction respectively,μisrx F y w m x m -⋅'⋅⋅⨯=''φsin 2()ry F z x w m y m -'+⋅'⋅⨯-=''φφcos sin 2mg F y w m z m rz +-⋅'⋅⋅⋅=''φcos 2the coefficient of viscosity of the object.5.1.4 Solution of the ModelWhen air flows through an object, only the air close to layer on the surface of the ob-ject in the laminar airflow is larger, whose air viscosity performance is more noticea-ble while the outer region has negligible viscous force [8]. Typically, to simplify cal-culation, we ignore the viscous force produced by plane surface caused by air resis-tance.Step 1: the examination of dimension in modelTo verify the validity of the model based on Newton ‟s second theorem, first, we standardize them respectively, turn them into the standardization of dimensionless data to diminish the influence of dimensional data. The standard equation is:Step 2: the confirmation of initial conditionsIn a space coordinate origin based on plane, we assume the earth's rotation direc-tion for the x axis, the plane's flight heading as y axis, the vertical downward di-rection for z axis. Space coordinate system are as follows:Figure 1 Space coordinate systemStep 3: the simplification and solutionAfter twice integrations of the model, ignoring some of the dimensionless in thesxx y i -=integral process, we can simplify the model and get the following:⎪⎪⎪⎩⎪⎪⎪⎨⎧+'⋅⋅⋅-⋅'⋅⨯='''-⋅⋅⋅-⋅'⋅⨯-=''⋅'⋅⨯=''g z m s c y w z y v m s c z w y y w x D D 220)(2cos 2)(2cos 2sin 2ρφρφφWe can calculate the corresponding xyz by putting in specific data to get the in-formation about the point of losing contact.Step 4: the solution of the coordinateThe distance of every latitude on the same longitude is 111km and the distance ofevery longitude on the same latitude is 111*cos (the latitude of this point) (km). Moreover, the latitude distance of two points on the same longitude is r ×cos(a ×pi/180) and the longitude distance of two points on the same latitude is: r ×sin(a ×pi/180)[9].We assume a as the clockwise angle starting with the due north direction and r as the distance between two points; X 、Y are the latitude and longitude coordinates of the known point P respectively; Lon , Lat are the latitude and longitude coordi-nates of the unknown point B respectively.Therefore, the longitude and latitude coordinates of the unknown point Q is:⎪⎪⎩⎪⎪⎨⎧⨯⨯+=⨯⨯⨯⨯+=111)180/cos()180/cos(111)180/sin(pi a r Y Lat pi Y pi a r X LonThus, we can get coordinates of the point of splash by putting in specific data.5.2 Modeling For Locating WreckageIn order to understand how the wreckage distributes in the sea, we have to understand the whole process beginning from the plane crashing into water to reaching the seaf-loor. One intuition for modeling the problem is to think of the ocean currents as astochastic process decided by water velocity. Therefore, we use a differential equation method to simulate the impact on wreckage from ocean currents.A Bayesian Searching model is a continuous model that computing a probability dis-tribution on the location of the wreckage (search object) in the presence of uncertain-ties and conflicting information that require the use of subjective probabilities. The model requires an initial searching zone and a set of the posterior distribution given failure of the search to plan the next increment of search. As the search proceeds, the subjective estimates of the detection will be more reliable.5.2.1 Assumptions of the ModelThe following general assumptions are made based on common sense and weuse them throughout our model.●Seawater density keeps unchanged despite the seawater depth.Seawater density is determined by water temperature, pressure, salinity etc.These factors are decided by or affected by the seawater density. Considering the falling height, the density changes slightly. To simplify the calculation, we consider it as a constant.●The velocity of the wreck stay the same compared with velocity of theplane before it crashes into pieces. The whole process will end quickly witha little loss of energy. Thus, we simplify the calculation.●Marine life will not affect our simulation.Most open coast habitats arefound in the deep ocean beyond the edge of the continental shelf, while the falling height of the plane cannot hit.●Acting force of seawater is a function of the speed and direction of oceancurrents. Ocean currents is a complicated element affected by temperature, wide direction, weather pattern etc. we focus on a short term of open sea.Acting force of seawater will not take this factors into consideration.5.2.2 Preparation of the Model●The resistance of objects of different shapes is different. Due to the continuityof the movement of the water, when faced with the surface of different shapes, the water will be diverted, resulting in the loss of partial energy. Thus the pressure of the surface of objects is changed. Based on this, we first consider the general object, and then revise the corresponding coefficients.●Ocean currents and influencing factorsOcean currents, also called sea currents, are large-scale seawater movements which have relatively stable speed and direction. Only in the land along the coast, due to tides, terrain, the injection of river water, and other factors, the speed and direction of ocean currents changes.Figure 2Distribution of world ocean currentsIt can be known from Figure 2 that warm and cold currents exist in the area where aircraft incidences happened. Considering the fact that the speed of ocean currents slows down as the increase of the depth of ocean, the velocity with depth sea surface currents gradually slowed down, v x is set as the initial speed of ocean currents in subsequent calculations.●Turbulent layerTurbulent flow is one kind of state of the fluid. When the flow rate is very low, the fluid is separated into different layers, called laminar flow, which do not mix with each other. As the flow speed increases, the flow line of the fluid begins to appear wavy swing. And the swing frequency and amplitude in-creases as the flow rate increases. This kind of stream regimen is called tran-sition flow. When the flow rate becomes great, the flow line is no longer clear and many small whirlpools, called turbulence, appeared in the flow field.Under the influence of ocean currents, the flow speed of the fluid changes as the water depth changes gradually, the speed and direction of the fluid is un-certain, and the density of the fluid density changes, resulting in uneven flow distribution. This indirectly causes the change of drag coefficient, and the re-sistance of the fluid is calculated as follows:2fkvGLCM texture of submarine topographyIn order to describe the impact of submarine topography, we choose a rectan-gular region from 33°33…W, 5°01…N to 31°42‟W , 3°37‟N. As texture is formed by repetitive distribution of gray in the spatial position, there is a cer-tain gray relation between two pixels which are separated by a certain dis-tance, which is space correlation character of gray in images. GLCM is a common way to describe the texture by studying the space correlation cha-racter of gray. We use correlation function of GLCM texture in MATLAB:I=imread ('map.jpg'); imshow(I);We arbitrarily select a seabed images and import seabed images to get the coordinate of highlights as follows:Table 1Coordinate of highlightsNO. x/km y/km NO. x/km y/km NO. x/km y/km1 154.59 1.365 13 91.2 22.71 25 331.42 16.632 151.25 8.19 14 40.04 18.12 26 235.77 13.93 174.6 14.02 15 117.89 14.89 27 240.22 17.754 172.38 19.23 16 74.51 12.29 28 331.42 24.455 165.71 24.82 17 45.6 8.56 29 102.32 19.486 215.75 26.31 18 103.43 5.58 30 229.1 18.247 262.46 22.96 19 48.934 3.51 31 176.83 9.188 331.42 22.34 20 212.42 2.85 32 123.45 3.239 320.29 27.55 21 272.47 2.48 33 32.252 11.7910 272.47 27.55 22 325.85 6.45 34 31.14 27.811 107.88 28.79 23 230.21 7.32 35 226.88 16.0112 25.579 27.05 24 280.26 9.93 36 291.38 5.46Then we use HDVM algorithm to get the 3D image of submarine topography, which can be simulated by MATLAB.Figure 3 3D image of submarine topographyObjects force analysis under the condition of currentsf is the resistance, f i is the disturbance resistance, F f is the buoyancy, G isgravity of object.Figure 4Force analysis of object under the conditions of currentsConsidering the impact of currents on the sinking process of objects, wheninterfered with currents, objects will sheer because of uneven force. There-。
2015年建模美赛(A题)O奖论文

For office use onlyT1________________ T2________________ T3________________ T4________________ Team Control Number32150Problem ChosenAFor office use onlyF1________________F2________________F3________________F4________________2015 Mathematical Contest in Modeling (MCM/ICM) Summary SheetHow to Eradicate Ebola?The breakout of Ebola in 2014 triggered global panic. How to control and eradicate Ebola has become a universal concern ever since.Firstly, we build up an epidemic model SEIHCR (CT) which takes the special features of Ebola into consideration. These are treatment from hospital, infectious corpses and intensified contact tracing. This model is developed from the traditional SEIR model. The model’s results(Fig.4,5,6), whose parameters are decided using computer simulation, match perfectly with the data reported by WHO, suggesting the validity of our improved model.Secondly, pharmaceutical intervention is studied thoroughly. The total quantity of the medicine needed is based on the cumulative number of individuals CUM(Fig.7). Results calculated from the WHO statistics and from the SEIHCR (CT) model show only minor discrepancy, further indicating the feasibility of our model. In designing the delivery system, we apply the weighted Fuzzy -c Means Clustering Algorithm and select 6 locations (Fig.10, Table.2) that should serve as the delivery centers for other cities. We optimize the delivery locations by each city’s location and needed medicine. The percentage each location shares is also figured out to facilitate future allocation (Table.3,4). The average speed of manufacturing should be no less than 106.2 unit dose per day and an increase in the manufacturing speed and the efficacy of medicine will reinforce the intervention effect.Thirdly, other critical factors besides those discussed early in the model, safer treatment of corpses, and earlier identification/isolation also prove to be relevant. By varying the value of parameters, we can project the future CUM. Results (Fig.12,13) show that these interventions will help reduce CUM to a lower plateau at a faster speed.We then analyze the factors for controlling and the time of eradication of Ebola. For example, when the rate of the infectious being isolated is 33% - 40%, the disease can be successfully controlled (Table.5). When the introduction time for treatment decreases from 210 to 145 days, the eradication of Ebola arrives over 200 days earlier.Finally, we select three parameters: the transmission rate, the incubation period and the fatality rate for sensitivity analysis.Key words: Ebola, epidemic model, cumulative cases, Clustering AlgorithmContents1. Introduction (1)1.1. Problem Background (1)1.2. Previous Research (2)1.3. Our Work (2)2. General Assumptions (3)3. Notations and Symbol Description (4)3.1. Notations (4)3.2. Symbol Description (4)4. Spread of Ebola (5)4.1. Traditional Epidemic Model (5)4.1.1. The SEIR Model (5)4.1.2. Outbreak Data (6)4.1.3. Results of the SEIR Model (7)4.2. Improved Model (8)4.2.1. The SEIHCR (CT) Model (8)4.2.2. Choosing Parameters (10)2.1.1. Results of the SEIHCR (CT) Model (11)5. Pharmaceutical Intervention (13)5.1. Total Quantity of the Medicine (13)5.1.1. Results from WHO Statistics (13)5.1.2. Results from the SEIHCR (CT) Model (15)5.2. Delivery System (15)5.2.1. Locations of Delivery (16)5.2.2. Amount of Delivery (19)5.3. Speed of Manufacturing (20)5.4. Medicine Efficacy (21)6. Other Important Interventions (21)6.1. Safer Treatment of Corpses (21)6.2. Intensified Contact Tracing and Earlier Isolation (22)6.3. Conclusion (24)7. Control and Eradication of Ebola (24)7.1. How Ebola Can Be Controlled (24)7.2. When Ebola Will Be Eradicated ...................................................... 26 8. Sensitivity Analysis (27)8.1. Impact of Transmission Rate I β (27)8.2. Impact of the Incubation Period 1/σ (28)8.3. Fluctuation of H δ ........................................................................... 28 9. Strengths and Weaknesses .. (29)9.1. Strengths (29)9.2. Weaknesses (30)9.3. Future Work (30)Letter to the World Medical Association (31)References (33)1.Introduction1.1.Problem BackgroundEbola virus disease (EVD), formerly known as Ebola haemorrhagic fever, is a severe, often fatal illness in humans.The current outbreak in West Africa, (first cases notified in March 2014), is the largest and most complex Ebola outbreak since the Ebola virus was first discovered in 1976. There have been more cases and deaths in this outbreak than all others combined. It started in Guinea and later spread across land borders to Sierra Leone and Liberia[1]. The current situation in the most affected countries can be seen clearly by the latest outbreak situation graph released by the World Health Organization (WHO).Figure 1. Ebola Outbreak Distribution Map in West Africa on 4th Feb, 2015/vhf/ebola/outbreaks/2014-west-africa/distribution-map.htmlEbola was first transmitted from fruit bats to the human population. It can now spread from human to human via direct contact with the blood, secretions, organs or other bodily fluids of infected people, and with surfaces and materials contaminated with these fluids.Burial ceremonies can also play a role in the transmission of Ebola because the virus can also be transmitted through the body of the deceased person[1].Control of outbreaks requires coordinated medical services, alongside a certain level of community engagement. The medical services include rapid detection of cases of disease, contact tracing of those who have come into contact with infected individuals, quick access to laboratory services, proper healthcare for those who areinfected, and proper disposal of the dead through cremation or burial[1],[2]. There are lots of different experimental vaccines and drug treatments for Ebola under development, tested both in the lab and in animal populations, but they have not yet been fully tested for safety or effectiveness[3], [4]. In the summer of 2014, the World Health Organization claimed fast-tracking testing was ethical in light of the epidemic[4]. In fact, the first batch of an experimental vaccine against Ebola have already been sent to Liberia in January 2015. According to the Dr Moncef Slaoui of British production company GlaxoSmithKline, t he initial phase is encouraging and encourages them to progress to the next phases of clinical testing[5].1.2.Previous ResearchThe analysis of the spread of epidemic has been a universal concern. Moreover, there have been a lot of research into the studies of the 2014 Ebola epidemic in West Africa[6]. For the spread of the disease, which is considered as an important factor in eradicating Ebola, there are a lot of previous research that can facilitate our understanding of the disease.For example, Fisman et al. used a two-parameter mathematical model to describe the epidemic growth and control[7]. Gomes et al. researched into the risk o f Ebola’s international spread along with its mobility data. His research reached a conclusion that the risk of Ebola’s international spread to outside Africa is relatively small compared to its expansion among the West African countries[8]. The Centers for Disease Control and Prevention used the traditional SIR model to extrapolate the Ebola epidemic and projected that Liberia and Sierra Leone will have had 1.4 million Ebola cases by Jan. 20, 2015[9]. Chowell et al. used the SEIR model and studied the effect of Ebola outbreaks in 1995 in Congo and in 2000 in Uganda[10]. The SEIR model takes the state of exposure into consideration, which is a special feature of Ebola because exposure to the virus will make individuals a lot more easily to be infected. Based on the SEIR model of Chowell et al[10], Althaus developed a model where the reproduction number is dependent on the time[11]. Valdez et al. developed a model and found that reducing population mobility had little effect on geographical containment of the disease and a rapid and early intervention that increases the hospitalization and reduces the disease transmission in hospitals and at funerals is the most important response to any possible re-emerging Ebola epidemic[12].1.3.Our WorkWe are asked to build a realistic, sensible, and useful model to optimize the eradication of Ebola or at least its current strain. Our model should not only considerthe spread of the disease, the quantity of the medicine needed, possible feasible delivery systems, locations of delivery, speed of manufacturing of the vaccine or drug, but also other critical factors that we consider to be necessary.To begin with, we searched a large number of papers that discuss the spread of Ebola to help us deepen the understanding of the problem. Chowell et al. provided a large amount of background information and their work[6]served as an important introduction. We found that a few of the papers used the traditional epidemic model to predict the transmission of the disease such as the SEIR model used by Althaus[11] to estimate the reproduction number of the virus during the 2014 outbreak. Therefore, we also applied the SEIR model in the early stage to predict the spread of Ebola. Later, we found out that the Ebola virus has some specific feathers that also needed to be considered and that are, the potential transmission threat posed by the highly infectious corpses, the improved infection control and reduced transmission rate if patients can be treated in hospitals, and the powerful intervention method: contact tracing. After taking all these critical factors into consideration, we improved our original epidemic model.Next, we deeply analyzed the pharmaceutical intervention. We first used the statistics provided by WHO[24] to help figure out the quantity of the needed medicine. We also used our improved model to predict the number of the patients and needed medicine in Guinea, Sierra Leone and Liberia. Two parameters: 1) the current cumulative number of patientsCUM and 2) the increasing rate of the disease topdecide the quantity of the medicine are set up as criteria.After the quantity of the medicine had been calculated, we sorted the affected cities of the three countries into several groups to determine the location of delivery. We applied the Fuzzy -c Means Clustering Algorithm and eventually selected 6 delivery points. These delivery points will be in charge of storing the medicine and transporting them to the set of cities surrounding them. Later, we took the speed of manufacturing and the efficacy of medicine into consideration and tested how these parameters could affect intervention.We then considered other important factors that would help the eradication of Ebola: 1) safer treatment of corpses and 2) intensified contact tracing and earlier isolation.After finishing analyzing all the relevant factors, we reached our final conclusion and predicted a time for Ebola’s eradication.In the last stage, we provide a non-technical letter for the world medical association to use in their announcement of inventing a new drug to stop Ebola and treat patients whose disease is not advanced.2.General Assumptions●The population of the new-born are not counted in the total population.●An individual who is exposed enters the incubation period and is not yetinfectious.●Recovered patients will not be infected again.●Medicine are only provided in hospitals.●Every location inside Guinea, Sierra Leone and Liberia can be selected as thedelivery center and delivery routes between cities are straight lines.●Medicine and vaccines can be delivered across borders.3.Notations and Symbol Description3.1.NotationsSusceptible individual[12]: A person with a clinical illness compatible with EVD and within 21 days before onset of illness, either:● a history of travel to the affected areas OR●direct contact with a probable or confirmed case OR●exposure to EVD-infected blood or other body fluids or tissues OR●direct handling of bats, rodents or primates, from Ebola-affected countries OR●preparation or consumption of bush meat from Ebola-affected countries.Exposed individual[14]: A person who has been infected by Ebola virus but are not yet infectious or symptomatic.Basic reproduction number[6]: The average number of secondary cases caused by a typical infected individual through its entire course of infection in a completely susceptible population and in the absence of control interventions.Contact tracing[15]: Find everyone who comes in direct contact with a sick Ebola patient. Contacts are watched for illness for 21 days from the last day they came in contact with the Ebola patient. If the contact develops a fever or other Ebola symptoms, they are immediately isolated, tested, provided care, and the cycle starts again-all of the new patient’s contacts are found and watched f or 21 days.3.2.Symbol DescriptionSymbol DescriptionR Basic reproduction numberN Size of total population()S t Number of suspected individuals at time tE t Number of exposed individuals at time t()I t Number of infectious individuals outside hospital at time t()()H t Number of hospitalized individuals at time tC t Number of contaminated deceased at time t()()R t Number of removed individuals at time t()CUM t Cumulative number of individuals at time t(),,I H C βββ Transmission rateα Rate of infectious individuals to be identified/isolated(),I H γγ Average time from symptoms onset to recovery (outside hospital, inside hospital)(),I H νν Average time from symptoms onset to death (outside hospital, inside hospital)(),I H δδ Fatality rate (outside hospital, inside hospital)1/ψ Average time until the deceased is properly handledκ Average number of contacts traced per identified/isolated infectious individual(),E I ππ Probability a contact traced individual (exposed, infectious) is isolated without causing a new case(),E I ωωRatio of probability that contact traced individual is (exposed,infectious) at time of originating case identification to theprobability a random individual in the population is (exposed,infectious) 4. Spread of Ebola4.1. Traditional Epidemic Model4.1.1. The SEIR ModelThe transmission of EBOV follows SEIR (susceptible-exposed-infectious-recovered) dynamics and can be described by the following set of ordinary differential equations [10]:()()()/,()()()/(),()()(),()(),()(),S t S t I t N E t S t I t N E t I t E t I t R t I t CUM t E t ββσσγγσ⎧=-⎪=-⎪⎪=-⎨⎪=⎪⎪=⎩ (4.1)where:()S t is the number of susceptible individuals at time t ,()E t is the number of exposed individuals at time t ,()I t is the number of infectious individuals at time t ,()R t is the number of removed individuals at time t ,()CUM t is the cumulative number of Ebola cases from symptoms onset,N is the size of total population,1/σ is the average duration of incubation,1/γ is the average duration of infectiousness.β is the transmission rate per person per day. β is constant in absence of control interventions. However, after control measures are introduced at time t τ≥, β is assumed to decay exponentially at rate k [16]. That is,0(1)0 ,() ,k t t e t τβτββτ--<⎧⎪=⎨≥⎪⎩ (4.2)4.1.2. Outbreak DataThe most seriously affected countries of the 2014 EBOV outbreak were Guinea, Sierra Leone and Liberia. Therefore, we mainly use the SEIR model to estimate the cumulative cases of these three countries. The data needed for the estimation can be obtained from the Centers for Disease Control and Prevention [17] which organizes the information provided in all the WHO situation reports [18] that are from March 1, 2014 to the most recent one on February 4, 2015. The data includes the numbers of reported total cases (confirmed, probable and suspected) and deaths.To test the validity of the SEIR model on the spread of the 2014 EBOV , we have to compare the actual data of cases and deaths in Guinea, Sierra Leone and Liberia with the calculated data from the model. And we need to first set the value for some of the parameters to carry on the calculation.Table 1. Parameter estimates for the 2014 EBOV outbreakParameterGuinea Sierra Leone Liberia Basic reproductionnumber, 0R1.512.53 1.59 Transmission rate withoutcontrol intervention 0β0.27 0.45 0.28 Reduce transmission, k0.048 0.048 0.048 Total population size, N 12,000,000 6,100,000 4,092,310The basic reproduction number 0R is given by 0/R βγ= where 1/ 5.61γ= day is the infectious duration from the study by Chowell et al [10]. Besides 1/ 5.61γ=, 1/ 5.3σ= is the average duration of the incubation period from a previous outbreak of the same EBOV in Congo in 1995[10]. Values of other parameters estimates [11] are listed in table 1.First, by applying the parameters into Equation (4.1), we are able to calculate the estimated number of cases and deaths for these three countries, that is, we will be able to predict the spread of the disease. Then, by comparing the calculated number with the reported data, we will be able to verify the validity of the SEIR model.4.1.3. Results of the SEIR ModelActual data of the cumulative numbers of infected cases and deaths are shown in the dotted curve, which are fitted against the data found in the WHO situation reports [18]. March 1, 2014 is defined as Day 1st and one cross in the figure represents one statistics of the cumulative numbers. Calculated data are obtained by solving Equation (4.1).GuineaSierra LeoneFigure 2. Cumulative number of individuals for Guinea, Sierra Leone and Liberia respectivelyFrom the figure above, we can see that the model fits the reported data of cases and deaths in these three countries very well. Therefore, SEIR can serve as a good tool for analyzing the spread of the 2014 EBOV outbreak.4.2. Improved Model4.2.1. The SEIHCR (CT) ModelSEIR is a basic model for predicting the spread of a disease. However, for the specific case of Ebola, we have to take other important factors into consideration. We mainly considered1) The potential threat posed by infectious corpses and the provision of hospitalcare;2) The involvement of contact tracing (CT).Figure 3 shows a schematic presentation of the improved model SEIHCR (CT), and indicates the compartmental states and the transition rates among the states.Figure 3.Compartmental flow of the SEIHCR (CT) modelLiberiaThe traditional model SEIR should be modified [20], [23] and the improved SEIHCR (CT) model can be written as()()()()()()()()()()()()()/()()/()()/,()()()/()()/()()/() /,()()()(1)()() /,()()(1)()I H C I H C E E I I I I I I H H H S t S t I t N S t H t N S t C t N E t S t I t N S t H t N S t C t N E t I t C t E t N I t E t I t I t I t I t C t I t N H t I t H t ββββββσκαψπωσαδγδνκαψπωαδγδ=---=++--+=-----+=---(),()()()(),()(1)()(1)()(),H I I H H I I H H H t C t I t H t C t R t I t H t C t νδνδνψδγδγψ⎧⎪⎪⎪⎪⎪⎪⎨⎪⎪⎪⎪=+-⎪⎪=-+-+⎩ (4.3)where:()S t is the number of susceptible individuals at time t ,()E t is the number of exposed individuals at time t ,()I t is the number of infectious individuals outside hospital at time t ,()H t is the number of hospitalized individuals at time t ,()C t is the number of contaminated deceased at time t ,()R t is the number of removed individuals at time t ,()CUM t is the cumulative number of Ebola cases from symptoms onset.I β is the transmission rate outside hospital, H β is the transmission rate inside hospital, C β is the transmission rate due to improper handling of deceased,and ()()()I H C I t H t C t N N Nλβββ=++, λ is the total transmission rate,1/σ is the average duration of incubation,α is the rate of infectious individuals to be identified/isolated,1/H γ is the average time from symptoms onset to recovery inside hospital,1/H ν is the average time from symptoms onset to death inside hospital,H δ is the fatality rate inside hospital, 1/I γ is the average time from symptoms onset to recovery outside hospital,1/I ν is the average time from symptoms onset to death outside hospital,I δ is the fatality rate outside hospital,1/ψ is the average time until deceased is properly handled.κ is the average number of contacts traced per identified/isolated infectious individual,E π is the probability a contact traced exposed individual is isolated without causing a new case,E ω is the ratio of probability that contact traced individual is exposed at time oforiginating case identification to the probability a random individual in the population is exposed,I π is the probability of a contact traced infectious individual is isolated withoutcausing a new case,I ω is the ratio probability that contact traced individual is infectious at time oforiginating case identification to the probability a random individual in the population is infectious.When we are to test the validity of the ultimate model, we should compare the calculated data with the actual data, just like what we have done in Section 4.1. The cumulative number of individuals can be obtained by()()().t tt CUM t t CUM t E s ds σ+∆+∆=+⎰ (4.4) Reasons for considering corpses, hospitals and contact tracingFirstly, the provision of hospital care [19] to affected populations could be used as a basis for treating patients and prevent the disease from spreading to a larger scale. Besides, funerals and burials [19] if regulated, will reduce the risk of being infected from improperly handled corpses of infected (contaminated deceased). Secondly, contact tracing is sometimes regarded as the key factor to stanch the Ebola outbreak in West Africa because if patients can be found earlier in their course of illness, their chances of exposure to family members and community health workers will reduce significantly [21].Equation (4.3) is improved from the original model SEIR and it is our ultimate model. We name it the SEIHCR (CT) model to illustrate its improvement and differences from the traditional SEIR model. The transition SEIR to SEIHCR (CT) shows how basic model can be greatly improved based on other factors and our own understanding toward this problem.4.2.2. Choosing Parameters1. Estimation of 0RIn order to obtain the reproduction number 0R , we use a method, following van den Driessche et al [22].()10,R FV ρ-= (4.5) where:()A ρ denotes the spectral radius of a matrix A ,F is the rate of appearance of new infections,V is the rate of transfer of individuals by all other means.F and V that are corresponded with the SEIHCR (CT) model are()//////0,000I H C I H C SI N SH N SC N SI N SH N SC N F ββββββ⎛-++⎫ ⎪++ ⎪ ⎪= ⎪ ⎪ ⎪ ⎪ ⎪⎝⎭0.I I H H I H I H E E I I I V I H H I H C I H C σσαγναγνννψγγψ⎛⎫ ⎪- ⎪ ⎪---= ⎪-- ⎪ ⎪+- ⎪ ⎪++⎝⎭ 2. Determination of other parametersThe values of the parameters I β, H β, C β, α and ψ are estimated for thethree countries using a least square curve fitting algorithm. The parameters σ, I γ, H γ, I ν, H ν, I δ and H δ are borrowed from existing references [11].The basic reproduction number of the SEIHCR (CT) model is given by the following formula, computed by the next-generation method: 0.(1)(1)C I H I I I I H H H H R βββαδγδνδγδνψ=+++-+-+ (4.6)Among all these parameters, I β is constant in absence of control interventions, just as β from the SEIR model. After control measures are introduced at time t τ≥, I β is assumed to decay exponentially at rate k [16]. That is,0(1)0 ,() ,I I k I t t e t τβτββτ--<⎧⎪=⎨≥⎪⎩ (4.7) Since k and H γ are both related to the efficacy of medicine, we assume their relationship to be,H k γθ= (4.8) where θ is assumed to be 3.2 based on experience.2.1.1. Results of the SEIHCR (CT) ModelAgain, we use the dotted line to represent the actual data of the cumulative numbers found in the WHO situation reports [18] and the solid line to represent our calculated number from Equation (4.3) of the SEIHCR (CT) model. The start day of the simulation is defined as Day 1st .Figure 4. Simulation of cumulative cases in Guinea from March 25th , 2014 to Feb 4th ,2015. The parameter values are 12,000,000N =,00.205I β=, 0.0001H β=,0.224C β=, 0.2α=, 1/32I γ=, 1/10H γ=, 1/8I υ=, 1/40H υ=,0.18ψ=, 0κ=, 0.03k =, 200τ=.Figure 5. Simulation of cumulative cases in Sierra Leone from May 27th , 2014 to Feb4th , 2015. The parameter values are 6,000,000N =,00.31I β=, 0.0001H β=,0.125C β=, 0.2α=, 1/30I γ=, 1/21H γ=, 1/8I υ=, 1/31H υ=,1/5.5ψ=, 0κ=, 0.015k =, 160τ=.Figure 6. Simulation of cumulative cases in Liberia from March 27th , 2014 to Feb 4th ,2015. The parameter values are 4,100,000N =,00.30I β=, 0.0001H β=,0.23C β=, 0.2α=, 1/32I γ=, 1/10H γ=, 1/8I υ=, 1/40H υ=,0.16ψ=, 0κ=, 0.03k =, 190τ=. These simulations are accomplished by varying combinations of parameters. Comparing these simulations with the ones based on the SEIR model, we can see that we obtain a better fit using the SEIHCR (CT) model, for the calculated data become more smoothly and closely approximate to the actual reported data with the simulation for Sierra Leone being the perfect approximation.5. Pharmaceutical InterventionIf medicine and vaccines can be available to patients and healthy people, the survival rate of the patients will increase and the situation of affected countries will improve to a great extent. Therefore, the manufacturing and delivery of medicine should remain an important factor in combating Ebola. Our tasks are to calculate the total amount of medicine, design a favorable delivery system and compare the intervention extent of different manufacturing speed and different level of medicine efficacy.5.1. Total Quantity of the Medicine5.1.1. Results from WHO StatisticsWhen medicine are about to be delivered to affected areas of Ebola, apparently, people should first analyze how serious the current situation is to decide the amount of medicine. However, just knowing the present situation is not enough. We should alsotake the speed of the spread of the virus into consideration because a large speed poses great potential threat. Therefore, when deciding the quantity of the medicine needed, we considered two important factors:1) The current cumulative number of patients p CUM ;2) The increasing rate of the disease υ.WHO has been releasing a weekly report of newly confirmed cases by district, which represent the sum of new patients [24] new SUM . Divide new SUM by the durationperiod T (7T = in this case since the report is released every week) and we will get the increasing rate of the disease v . Meanwhile, by adding the newly infected cases, we can know the current cumulative patients p CUM as well.By far, we have gathered all the information that we need to calculate the quantity of the medicine. By adding the current cumulative number of patients and the increasing rate of the disease (we place equal weights on these two factors), we can obtain the unit dose of the medicine needed every day D for each city and.p D CUM T υ=+⨯ (5.1) Due to the extremely large amount of statistics for both the number of cities (56 cities) and the duration of the reported data (57 weeks till Feb 1st , 2015), the concrete data and calculated value will not be presented. Instead, we drew a graph that illustrates the units of medicine needed by these three countries from Dec 30th , 2013 to Jan 31st , 2015.Figure 7. Unit dose of the medicine needed per day for Guinea, Liberia and Sierra LeoneIn the figure above, the blue, orange and green curve represents the unit dose of medicine needed per day by patients in Guinea, Liberia and Sierra Leone respectively. The grey area represents the combined doses needed by the three countries altogether.。
美国中学生数学建模竞赛获奖论文
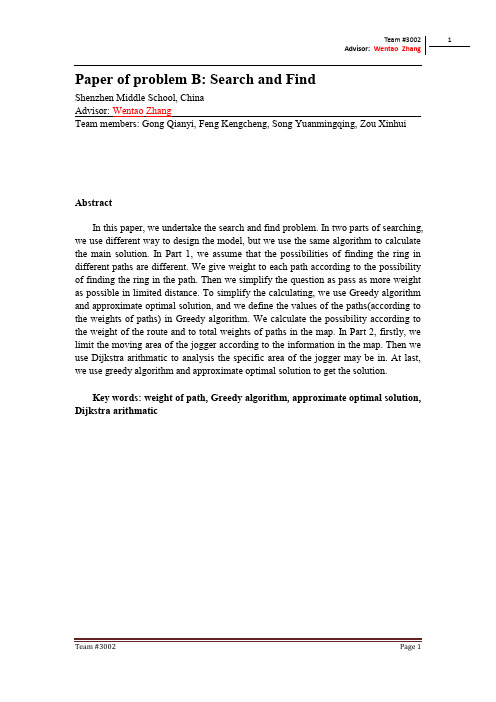
Abstract
In this paper, we undertake the search and find problem. In two parts of searching, we use different way to design the model, but we use the same algorithm to calculate the main solution. In Part 1, we assume that the possibilities of finding the ring in different paths are different. We give weight to each path according to the possibility of finding the ring in the path. Then we simplify the question as pass as more weight as possible in limited distance. To simplify the calculating, we use Greedy algorithm and approximate optimal solution, and we define the values of the paths(according to the weights of paths) in Greedy algorithm. We calculate the possibility according to the weight of the route and to total weights of paths in the map. In Part 2, firstly, we limit the moving area of the jogger according to the information in the map. Then we use Dijkstra arithmatic to analysis the specific area of the jogger may be in. At last, we use greedy algorithm and approximate optimal solution to get the solution.
数学建模美赛O奖论文
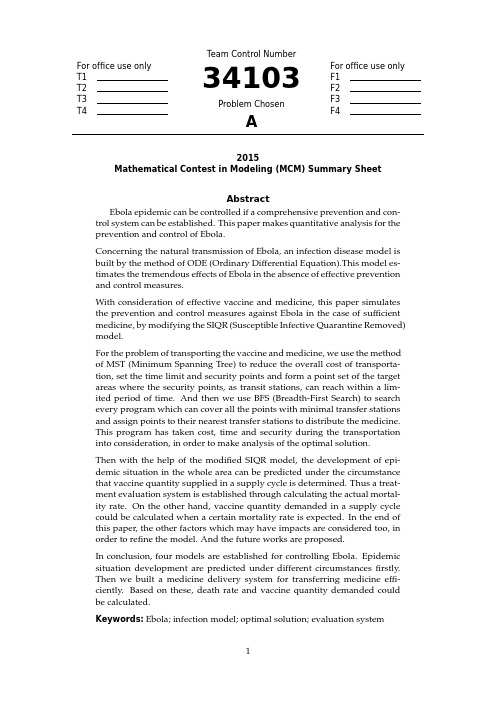
34103
Problem Chosen
For office use only F1 F2 F3 F4
A
2015 Mathematical Contest in Modeling (MCM) Summary Sheet
4.3
Model 3 . . . . . . . . . . . . . . . . . . . . . . . . . . . . . . . . . escription . . . . . . . . . . . . . . . . . . . . . . . . . . 4.3.2 4.3.3 4.3.4 4.3.5 4.3.6 Symbols . . . . . . . . . . . . . . . . . . . . . . . . . . . . . . . . . . Additional Assumptions . . . . . . . . . . . . . . . . . . . . . . . . . Model Establishment . . . . . . . . . . . . . . . . . . . . . . . . . . . Model Test . . . . . . . . . . . . . . . . . . . . . . . . . . . . . . . . . Model Analysis . . . . . . . . . . . . . . . . . . . . . . . . . . . . . .
Concerning the natural transmission of Ebola, an infection disease model is built by the method of ODE (Ordinary Differential Equation).This model estimates the tremendous effects of Ebola in the absence of effective prevention and control measures. With consideration of effective vaccine and medicine, this paper simulates the prevention and control measures against Ebola in the case of sufficient medicine, by modifying the SIQR (Susceptible Infective Quarantine Removed) model. For the problem of transporting the vaccine and medicine, we use the method of MST (Minimum Spanning Tree) to reduce the overall cost of transportation, set the time limit and security points and form a point set of the target areas where the security points, as transit stations, can reach within a limited period of time. And then we use BFS (Breadth-First Search) to search every program which can cover all the points with minimal transfer stations and assign points to their nearest transfer stations to distribute the medicine. This program has taken cost, time and security during the transportation into consideration, in order to make analysis of the optimal solution. Then with the help of the modified SIQR model, the development of epidemic situation in the whole area can be predicted under the circumstance that vaccine quantity supplied in a supply cycle is determined. Thus a treatment evaluation system is established through calculating the actual mortality rate. On the other hand, vaccine quantity demanded in a supply cycle could be calculated when a certain mortality rate is expected. In the end of this paper, the other factors which may have impacts are considered too, in order to refine the model. And the future works are proposed. In conclusion, four models are established for controlling Ebola. Epidemic situation development are predicted under different circumstances firstly. Then we built a medicine delivery system for transferring medicine efficiently. Based on these, death rate and vaccine quantity demanded could be calculated.
建模美赛获奖范文
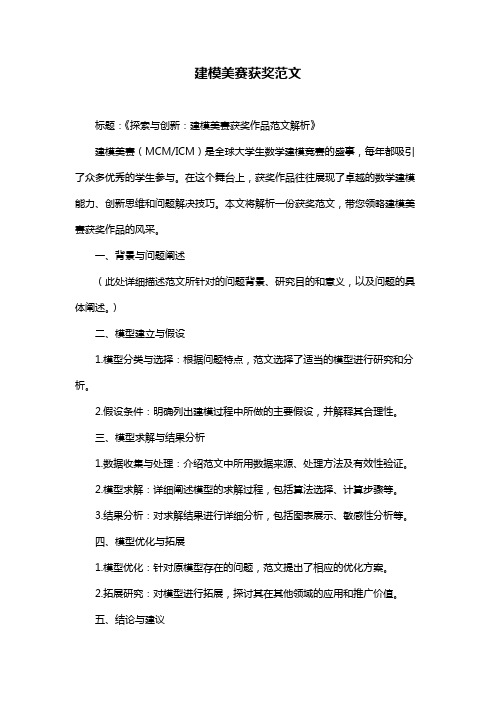
建模美赛获奖范文标题:《探索与创新:建模美赛获奖作品范文解析》建模美赛(MCM/ICM)是全球大学生数学建模竞赛的盛事,每年都吸引了众多优秀的学生参与。
在这个舞台上,获奖作品往往展现了卓越的数学建模能力、创新思维和问题解决技巧。
本文将解析一份获奖范文,带您领略建模美赛获奖作品的风采。
一、背景与问题阐述(此处详细描述范文所针对的问题背景、研究目的和意义,以及问题的具体阐述。
)二、模型建立与假设1.模型分类与选择:根据问题特点,范文选择了适当的模型进行研究和分析。
2.假设条件:明确列出建模过程中所做的主要假设,并解释其合理性。
三、模型求解与结果分析1.数据收集与处理:介绍范文中所用数据来源、处理方法及有效性验证。
2.模型求解:详细阐述模型的求解过程,包括算法选择、计算步骤等。
3.结果分析:对求解结果进行详细分析,包括图表展示、敏感性分析等。
四、模型优化与拓展1.模型优化:针对原模型存在的问题,范文提出了相应的优化方案。
2.拓展研究:对模型进行拓展,探讨其在其他领域的应用和推广价值。
五、结论与建议1.结论总结:概括范文的研究成果,强调其创新点和贡献。
2.实践意义:分析建模结果在实际问题中的应用价值和意义。
3.建议:针对问题解决,提出具体的建议和措施。
六、获奖亮点与启示1.创新思维:范文在模型选择、求解方法等方面展现出创新性。
2.严谨论证:文章结构清晰,逻辑严密,数据充分,论证有力。
3.团队合作:建模美赛强调团队协作,范文体现了成员间的紧密配合和分工合作。
总结:通过分析这份建模美赛获奖范文,我们可以学到如何从问题背景出发,建立合理的模型,进行严谨的求解和分析,以及如何优化和拓展模型。
同时,也要注重创新思维和团队合作,才能在建模美赛中脱颖而出。
美国大学生数学建模竞赛美赛--论文

Each team member must sign the statement below: (Failure to obtain signatures from each team member will result in disqualification of the entire team.)
2015 Mathematical Contest in Modeling (MCM/ICM) Control Sheet Please review this page before submitting your solution to ensure that all of the information is correct Do not make changes by hand to the information on this control sheet. If you need to change any of the information on this sheet, login via the Advisor Login link on the MCM web site, make the changes online, and print a new sheet. You may NOT photocopy this control sheet to give to a new team, nor may you assign any team a control number. Each team must have its own control number, obtained by registering via the MCM web site. Advisor Jinpeng Yu Name: Department: Control Engineering Institution: Qingdao University Address: 308 Ningxia Road,Shinan District,Qingdao,Shandong,China Qingdao, Shandong 266000 Phone: 18653250086 Fax: 053285953064 Email: zhanghaoran06@ Home Phone: 053285953064 The names of the team members will appear on your team's certificate exactly as they appear on this page, including all capitalization and punctuation, if any. Gender data is optional and will be used for statistical purposes only; it will not appear on the certificate. Team Member Haoran Zhang Yu Ma Guiying Dong Gender M M F Your team's control number is: 40906 (Place this control number on all pages of your solution paper and on any support material.) Problem Chosen: B
美国大学生数学建模比赛的论文格式
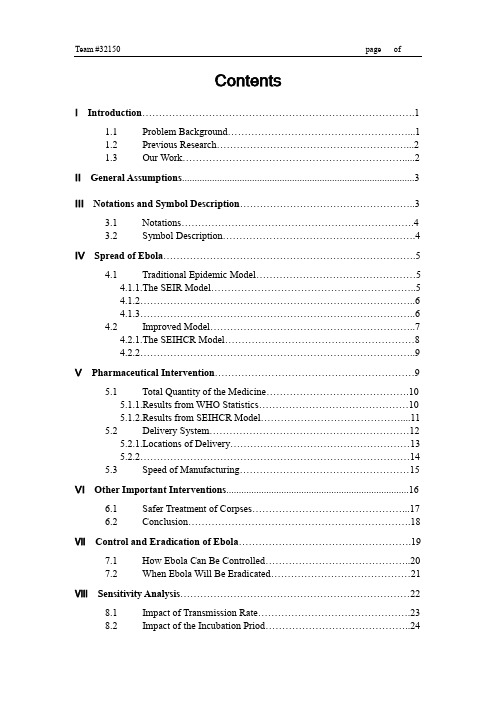
ContentsⅠIntroduction (1)1.1Problem Background (1)1.2Previous Research (2)1.3Our Work (2)ⅡGeneral Assumptions (3)ⅢNotations and Symbol Description (3)3.1 Notations (4)3.2 Symbol Description (4)ⅣSpread of Ebola (5)4.1 Traditional Epidemic Model (5)4.1.1.The SEIR Model (5)4.1.2 (6)4.1.3 (6)4.2 Improved Model (7)4.2.1.The SEIHCR Model (8)4.2.2 (9)ⅤPharmaceutical Intervention (9)5.1 Total Quantity of the Medicine (10)5.1.1.Results from WHO Statistics (10)5.1.2.Results from SEIHCR Model (11)5.2 Delivery System (12)5.2.1.Locations of Delivery (13)5.2.2 (14)5.3 Speed of Manufacturing (15)ⅥOther Important Interventions (16)6.1 Safer Treatment of Corpses (17)6.2 Conclusion (18)ⅦControl and Eradication of Ebola (19)7.1 How Ebola Can Be Controlled (20)7.2 When Ebola Will Be Eradicated (21)ⅧSensitivity Analysis (22)8.1 Impact of Transmission Rate (23)8.2 Impact of the Incubation Priod (24)ⅨStrengths and Weaknesses (25)9.1 Strengths (26)9.2 Weaknesses (27)9.3 Future Work (28)Letter to the World Medical Association (30)References (31)ⅠIntroduction1.1.Promblem Background1.2.Previous Research1.3.Our WorkⅡGeneral Assumptions●●ⅢNotations and Symbol Description3.1. Notataions3.2. Symbol DescriptionSymbol DescriptionⅣSpread of Ebola4.1. Traditional Epidemic Model4.1.1. The SEIR Model4.1.2. Outbreak Data4.1.3. Reslts of the SEIR Model4.2. Improved Model4.2.1. The SEIHCR Model4.2.2. Choosing paametersⅤPharmaceutical Intervention 5.1. Total Quantity of the Medicine 5.1.1. Results from WHO Statistics5.2. Delivery System5.2.1. Locations of Delivery5.2.2. Amount of Delivery5.3. Speed of Manufacturong5.4. Medicine EfficacyⅥOther Important Interventions 6.1. Safer Treatment of Corpses6.2. ConclusionⅦControl and Eradication of Ebola 7.1. How Ebola Can Be Controlled7.2. When Ebola Will Be EradicatedⅧSensitivity Analysis8.1. Impact of Transmission Rate8.2. Impact of Incubation PeriodⅨStrengths and Weaknesses 9.1. Strengths●●●9.2. Weaknesses●●●9.3.Future WorkLetter to the World Medical AssociationTo whom it may concern,Best regards,Team #32150References [1][2][3][4]。
历年美赛数学建模优秀论文大全
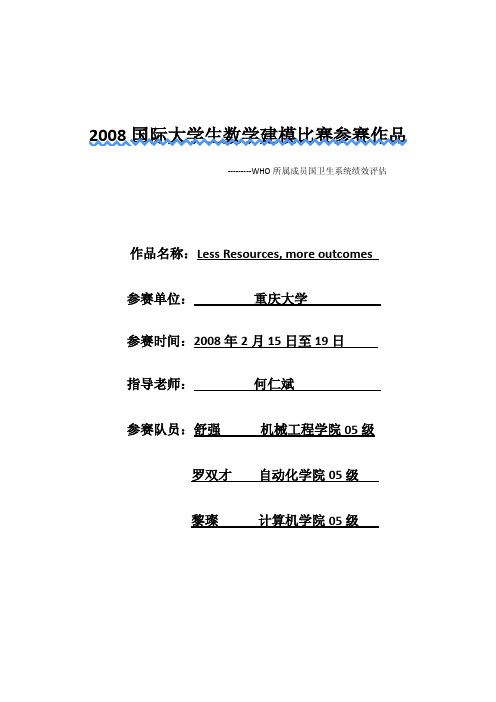
2008国际大学生数学建模比赛参赛作品---------WHO所属成员国卫生系统绩效评估作品名称:Less Resources, more outcomes参赛单位:重庆大学参赛时间:2008年2月15日至19日指导老师:何仁斌参赛队员:舒强机械工程学院05级罗双才自动化学院05级黎璨计算机学院05级ContentLess Resources, More Outcomes (4)1. Summary (4)2. Introduction (5)3. Key Terminology (5)4. Choosing output metrics for measuring health care system (5)4.1 Goals of Health Care System (6)4.2 Characteristics of a good health care system (6)4.3 Output metrics for measuring health care system (6)5. Determining the weight of the metrics and data processing (8)5.1 Weights from statistical data (8)5.2 Data processing (9)6. Input and Output of Health Care System (9)6.1 Aspects of Input (10)6.2 Aspects of Output (11)7. Evaluation System I : Absolute Effectiveness of HCS (11)7.1Background (11)7.2Assumptions (11)7.3Two approaches for evaluation (11)1. Approach A : Weighted Average Evaluation Based Model (11)2. Approach B: Fuzzy Comprehensive Evaluation Based Model [19][20] (12)7.4 Applying the Evaluation of Absolute Effectiveness Method (14)8. Evaluation system II: Relative Effectiveness of HCS (16)8.1 Only output doesn’t work (16)8.2 Assumptions (16)8.3 Constructing the Model (16)8.4 Applying the Evaluation of Relative Effectiveness Method (17)9. EAE VS ERE: which is better? (17)9.1 USA VS Norway (18)9.2 USA VS Pakistan (18)10. Less Resources, more outcomes (19)10.1Multiple Logistic Regression Model (19)10.1.1 Output as function of Input (19)10.1.2Assumptions (19)10.1.3Constructing the model (19)10.1.4. Estimation of parameters (20)10.1.5How the six metrics influence the outcomes? (20)10.2 Taking USA into consideration (22)10.2.1Assumptions (22)10.2.2 Allocation Coefficient (22)10.3 Scenario 1: Less expenditure to achieve the same goal (24)10.3.1 Objective function: (24)10.3.2 Constraints (25)10.3.3 Optimization model 1 (25)10.3.4 Solutions of the model (25)10.4. Scenario2: More outcomes with the same expenditure (26)10.4.1Objective function (26)10.4.2Constraints (26)10.4.3 Optimization model 2 (26)10.4.4Solutions to the model (27)15. Strengths and Weaknesses (27)Strengths (27)Weaknesses (27)16. References (28)Less Resources, More Outcomes1. SummaryIn this paper, we regard the health care system (HCS) as a system with input and output, representing total expenditure on health and its goal attainment respectively. Our goal is to minimize the total expenditure on health to archive the same or maximize the attainment under given expenditure.First, five output metrics and six input metrics are specified. Output metrics are overall level of health, distribution of health in the population,etc. Input metrics are physician density per 1000 population, private prepaid plans as % private expenditure on health, etc.Second, to evaluate the effectiveness of HCS, two evaluation systems are employed in this paper:●Evaluation of Absolute Effectiveness(EAE)This evaluation system only deals with the output of HCS,and we define Absolute Total Score (ATS) to quantify the effectiveness. During the evaluation process, weighted average sum of the five output metrics is defined as ATS, and the fuzzy theory is also employed to help assess HCS.●Evaluation of Relative Effectiveness(ERE)This evaluation system deals with the output as well as its input, and also we define Relative Total Score (RTS) to quantify the effectiveness. The measurement to ATS is units of output produced by unit of input.Applying the two kinds of evaluation system to evaluate HCS of 34 countries (USA included), we can find some countries which rank in a higher position in EAE get a relatively lower rank in ERE, such as Norway and USA, indicating that their HCS should have been able to archive more under their current resources .Therefore, taking USA into consideration, we try to explore how the input influences the output and archive the goal: less input, more output. Then three models are constructed to our goal:●Multiple Logistic RegressionWe model the output as function of input by the logistic equation. In more detains, we model ATS (output) as the function of total expenditure on health system. By curve fitting, we estimate the parameters in logistic equation, and statistical test presents us a satisfactory result.●Linear Optimization Model on minimizing the total expenditure on healthWe try to minimize the total expenditure and at the same time archive the same, that is to get a ATS of 0.8116. We employ software to solve the model, and by the analysis of the results. We cut it to 2023.2 billion dollars, compared to the original data 2109.8 billion dollars.●Linear Optimization Model on maximizing the attainment. We try to maximize the attainment (absolute total score) under the same total expenditure in2007.And we optimize the ATS to 0.8823, compared to the original data 0.8116.Finally, we discuss strengths and weaknesses of our models and make necessary recommendations to the policy-makers。
美赛O奖A题论文

For T1 T2 T3 T4
office use only ________________ ________________ ________________ ________________
2
Problem Chosen
A
Summary
For F1 F2 F3 F4
Team # 35532
1 / 34
Introduction
Problem background
A complex epidemic of Zaire Ebola virus (EBOV) has been affecting West Africa since approximately December 2013, with first cases likely occurring in southern Guinea [1] and facilitating several transmission chains to progress essentially unchecked in the region and to cross porous borders with neighboring Sierra Leone and Liberia and seed a limited outbreak in Nigeria via commercial airplane on 20 July 2014 [2]. Then the number of new cases appear an exponential growth. While public health interventions have been introduced in all affected countries, the numbers of infected cases and deaths from EBOV continue to increase due to the loss of effective medication. A total of 22,495 cases, with 8,981 deaths, have been reported to the World Health Organization as of 4 February 2015[3].
大学生美赛建模论文
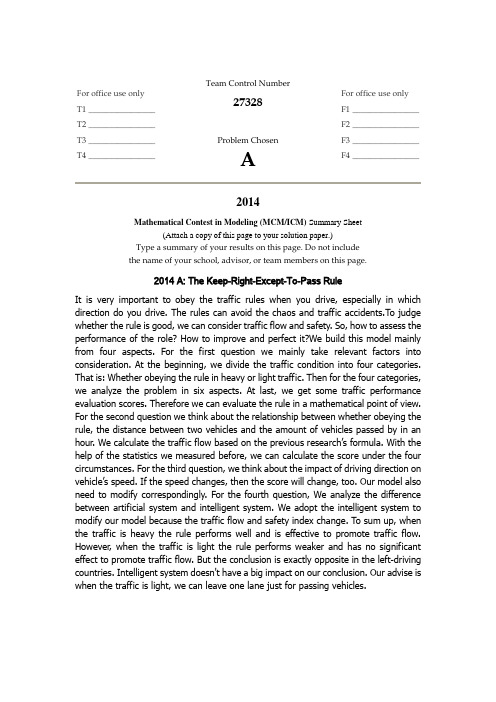
For office use onlyT1________________ T2________________ T3________________ T4________________ Team Control Number27328Problem ChosenAFor office use onlyF1________________F2________________F3________________F4________________ 2014Mathematical Contest in Modeling (MCM/ICM) Summary Sheet(Attach a copy of this page to your solution paper.)Type a summary of your results on this page. Do not includethe name of your school, advisor, or team members on this page.2014 A: The Keep-Right-Except-To-Pass RuleIt is very important to obey the traffic rules when you drive, especially in which direction do you drive. The rules can avoid the chaos and traffic accidents.To judge whether the rule is good, we can consider traffic flow and safety. So, how to assess the performance of the role? How to improve and perfect it?We build this model mainly from four aspects.For the first question we mainly take relevant factors into consideration. At the beginning, we divide the traffic condition into four categories. That is: Whether obeying the rule in heavy or light traffic. Then for the four categories, we analyze the problem in six aspects. At last,we get some traffic performance evaluation scores. Therefore we can evaluate the rule in a mathematical point of view. For the second question we think about the relationship between whether obeying the rule, the distance between two vehicles and the amount of vehicles passed by in an hour. We calculate the traffic fl ow based on the previous research’s formula. With the help of the statistics we measured before, we can calculate the score under the four circumstances. For the third question, we think about the impact of driving direction on vehicle’s speed. If the spee d changes, then the score will change, too. Our model also need to modify correspondingly. For the fourth question, We analyze the difference between artificial system and intelligent system. We adopt the intelligent system to modify our model because the traffic flow and safety index change. To sum up, when the traffic is heavy the rule performs well and is effective to promote traffic flow. However, when the traffic is light the rule performs weaker and has no significant effect to promote traffic flow. But the conclusion is exactly opposite in the left-driving countries. Intelligent system doesn't have a big impact on our conclusion. Our advise is when the traffic is light, we can leave one lane just for passing vehicles.COVERCOVER 0Summary (2)Key word (3)Problem background (3)Problem analysis (4)Assumptions (5)Symbols description (5)Model design and solving (6)Model 1: Introduction of vehicle length, weather, frictions and actual speed (6)Model 2: Calculating the distance between two vehicles (8)Model 3: Scores considering S(the traffic flow within an hour) and I(the number of traffic accident every 100000 kilometers) .. 9 Model 4: Improving traffic flow (13)Model 5: Can our new model be applied to driving-in-the-left countries ? (14)Model 6: Intelligent system model (14)Weaknesses and strengths of the model (15)Reference (15)Appendix (16)2014 A: The Keep-Right-Except-To-Pass RuleSummaryIt is very important to obey the traffic rules when you drive, especially in which direction do you drive. The rules can avoid the chaos and traffic accidents.To judge whether the rule is good, we can consider traffic flow and safety. So, how to assess the performance of the role? How to improve and perfect it?We build this model mainly from four aspects.For the first question we mainly take relevant factors into consideration. At the beginning, we divide the traffic condition into four categories. That is: Whether obeying the rule in heavy or light traffic. Then for the four categories, we analyze the problem in six aspects. At last,we get some traffic performance evaluation scores. Therefore we can evaluate the rule in a mathematical point of view. For the second question we think about the relationship between whether obeying the rule, the distance between two vehicles and the amount of vehicles passed by in an hour. We calculate the traffic flow based on the previous research’s formula. With the help of the statistics we measured before, we can calculate the score under the four circumstances. For the third question, we think about the impact of driving direction on vehicle’s speed. If the speed changes, then the score will change, too. Our model also need to modify correspondingly. For the fourth question, We analyze the difference between artificial system and intelligent system. We adopt the intelligent system to modify our model because the traffic flow and safety index change. To sum up, when the traffic is heavy the rule performs well and is effective to promote traffic flow. However, when the traffic is light the rule performs weaker and has no significant effect to promote traffic flow. But the conclusion is exactly opposite in the left-driving countries. Intelligent system doesn't have a big impact on our conclusion. Our advise is when the traffic is light, we can leave one lane just for passing vehicles.Key wordfactor analysis, optimization methods, traffic flow, the number of traffic accidents, scoreProblem backgroundIt is very important to obey the traffic rules when you drive, especially in which direction do you drive. The rules can avoid the chaos and traffic accidents. We can drive on the left or on the right.34% countries drive on the left and 66% countries drive on the right. The biggest advantage of driving on the left is human’s instinct to avoid its evils. In the case of fast movement, when you find it’s dangerous in the front, you will instinctively tilt to the left to protect your heart. The advantage of driving on the right is that drivers can take charge of the steering wheel by the left hand and can change the shift flexibly. Most of the countries adopt the rule that driving on the right. Drivers accustomed to the right don’t need to spare time on learning the left rules. At the same time, the seats drivers sit are usually on the left all over the world. If the car is the same, it is cheaper to buy the car that the seat is on the left than on the right. In our country, as the result of politics, economy and culture, we obey to the rule that drive on the right unless passing anther car.To judge whether the rule is good, we can consider traffic flow and safety. Generally speaking, the traffic rule will have good to promoting traffic flow, but when the vehicles are keeping increasing, the traffic must be heavy ,so the number of traffic accidents must increase, too. So, if we want to find a better way ,we must make the rules considering traffic flow and safety.So, regardless of the different driving directions, when we make the traffic rules, we must consider these factors. To ensure safety, some roads are under the control of intelligent systems. This can avoid driving after drinking, driving with tiredness. Theintelligent system can avoid some human judgment and forecast the traffic condition. The road will not be too crowded under the control of it. So this system can be good to the traffic.Based on this background, we build a mathematical model to check if the rule is rather good. At the same time ,we will answer the questions below:When we take safety, weather, road condition, traffic flow into consideration, how is the performance of this rule in light and heavy traffic?Is this rule effective in promoting better traffic flow? If not, how to check our model? Is our model applying to the driving on the left countries?If vehicle transportation on the same roadway was fully under the control of an intelligent system –either part of the road network or imbedded in the design of all vehicles using the roadway–to what extent would this change the results of your earlier analysis?Problem analysisAfter reading the question, we will split the problem into some small problems to solve.●Consider the road friction, weather, traffic condition, weather obey the rule’simpact on the vehicle’s speed.●Consider the relationship between the distance of two vehicles and speed.●Consider the relationship between traffic flow and the number of traffic accidents.We have two conditions: heavy traffic and light traffic. We calculate the score in different conditions when obeying the rule or not obeying the rule.●If our model can be applied to other countries?●Consider the intelligent system’s effect. Under the intelligent system, do we needto check our model?In question 1,2,3,4,we build a score to discuss the rule’s impact on traffic condition in heavy or light traffic. In the score, the traffic flow index takes up 50%,the safety index takes up 50%.In the same way, we can calculate the score when not obeying the rule.After preliminary analysis, when the traffic is light, if we don’t obey the rule, the score is higher. So, we take three road lanes for instance, when the traffic is heavy, we obey the rule. when the traffic is light, we don’t obey the rule. In question 5,We take human body’s inflexibility into consideration because it will affect the speed. In question 6,with the help of intelligent system, we can avoid heavy traffic and traffic accidents, we will have a new score and then check the rules.AssumptionsIn the optimization methods, we just think the road is straight to build a model.We set medium size vehicle as a standard. The stable speed of the vehicle in the free state(medium-car standard model)is X.Speed conversion coefficient under the standard of medium size car’s speed is A.We consider the vehicles all drive on the right, then we have four conditions, obey the rules or not, heavy traffic or light.The traffic flow is within an our. We take one normal day for instance. From 7a.m. to 9a.m and4:30p.m to 6p.m it is heavy traffic. From 23p.m to 4a.m it is light traffic. On this basis, we can build our model.Symbols descriptionModel design and solvingModel 1: Introduction of vehicle length, weather, frictions and actual speedIt covers 6 variable: vehicle length(c),the weather, road conditions and friction impact on the speed(K),the traffic impact on the speed(α),whether obeying rule impact on the speed(β),The stable speed of the vehicle in the free state(medium-car standard model)(X) and Speed conversion coefficient under the standard of medium size car’s speed(A).According to the research, we can know K and A.To K, we have five conditions:Level one: The road condition is good and vehicles can pass away normally.Level two: The road condition is slightly bad and vehicles can pass slowly.Level three: The road condition is bad and cars can pass slowly with a reasonable distance.Level four: The road condition is worse and vehicles can’t pass safely.Level five: The road condition is worst and no vehicles can pass.(Sheet 1)To A, we divide vehicles into 8 kinds. They are cars, van, motor coach, small trucks, freight train, large trucks, tractor and trailer. The stable speed of the vehicle in the free state(medium-car standard model) is X and Speed conversion coefficient under the standard of medium size car’s speed is A.Sheet 2 shows the vehicle speed Conversion Factor A:(Sheet 2)Considering these factors, the speed X is affected by α,β,A and K. According to the optimization methods, we just think these factors are proportional to X. Above all, we can define the actual speed of the vehicle.Model 2: Calculating the distance between two vehiclesWe think the least distance between two vehicles can ensure safety when braking.The vehicle brake deceleration process can be seen as linear motion.The acceleration is K ·g(g is acceleration due to gravity ). Then:22220122V kgt Vt kgt L V KXKX L gβαβα-=⎧⎪⎨-=⎪⎩=∴=Model 3: Scores considering S(the traffic fl ow within an hour) and I(thenumber of traffic accident every 100000 kilometers)Our model will give us a score considering S(the traffic flow within an hour) and I(thenumber of traffic accident every 100000 kilometers).If the score is big enough, thenthe rule is effective in promoting traffic condition.Firstly, we deduce the formula of the traffic flow. The most widely used formula in ourcountry is: time*V/(C+L).Considering heavy and light traffic, we just need to chooseone our in heavy time and light time.Secondly, we deduce the formula of the number of traffic accident every 100000kilometers(I).Obviously, the actual speed affects I. Here are some reasons below:(1)affecting eyesight. When speed is increasing, the drivers eyesight will be poorerthan the still eyesight.(2)affecting visual range. When speed is increasing, the visual range will be small andnarrow.(3)affecting recognizable sight. When driving, the driver needs to identify varioustraffic signs or traffic environment. The identification distance will be different under the different speed. When the speed is too high, the identification distance will be small. It will be hard to be aware of the road condition.(4)affecting judgment. It is according to the position change that drivers recognizethe object.When the vehicle is in a traveling state , the objects outside the vehicle change the position slowly and small.If the speed is high, then the change is slow and small. It is really hard to identify. Therefore, when the driver is driving, his ability to distinguish an object will fall.(5)impact on the braking distance and security region. As picture 3 shows,a vehiclewith the speed of 30km / h can stop his car in 13 meters. The passers-by will be safe outside the 13 meters. The damage is zero and this area is safe to passers-by.However, a vehicle with the speed of 50km / h can stop his car in 26 meters. If the passers-by still walk in 13metres,it will be dangerous. The safety index is zero.(picture 3)Sheet 4 is an ordinary road braking distances on different speed:Sheet 5 :stopping sight distance in a highway:In 2004, after analyzing the traffic accidents, Chinese scholars think accident rate increases when the standard deviation of speed increases. The bigger the standard deviation is, the higher the accident rate is. At the same time, research indicates that speed standard deviation and the accident rate are exponential relationship.With the improvement in the level of speed’s discrete, accident rate will be growing exponentially .Below are the picture 6:(picture 6)In 1993,Monash University Accident Research Centre summed a function model to tell the relationship between the speed level and traffic accidents rate.The function is :23=+∆+∆5000.80.014I V VIn the formula,I means the number of traffic accident every 100000 kilometers.-,Our model is to use this formula to calculate the number of V∆means V Xaccidents .At last, how do we calculate the score P ? That is the traffic flow index takes up 50% and the safety index takes up 50%.111V L + 1S )221V L +2S )331V L +S )10.8(V )X -(1I ) By linear regression, we can calculate αand β.Under the circumstance of heavy traffic, we take the scores when obeying the rule or not obeying the rule for comparison. We can have a conclusion that in this case the score is higher when obeying the rule. Similarly, under the circumstance of light traffic, we take the scoreswhen obeying the rule or not obeying the rule for comparison. We can have a conclusion that in this case the score is higher when not obeying the rule. Therefore, we can know the rule performs well in heavy traffic but performs bad in light traffic.Model 4: Improving traffic flowThe model here is similar the model above when calculating traffic flow./(C )S t V L =+.Similarly, we have to conditions. That is heavy traffic and light traffic, then we calculate the traffic flow when obeying the rule or not obeying rule to see if the rule is effective to promote the traffic flow.111V L +1S ) 221V L +2S ) 331V L +3S )According to the α、β ,we can calculate the traffic flow S easily.We find it obviously when the traffic is heavy, we will have a bigger traffic flow when obeying the rule. When the traffic is light, the traffic flow is bigger if not obeying the rule.Therefore, We promote When the traffic is heavy, we need to obey the previous rule--- drivers need to drive in the right-most lane unless they are passing another vehicle. When the traffic is light, we take two lanes in the right side for driving and the left one side for passing vehicles.(3 lanes for instance)At this time we control the vehicle’s speed in a reasonable range and then insureβ1βin a flexible range. Then the traffic flow is bigger when not obeying the and2previous rule.Model 5: Can our new model be applied to driving-in-the-left countries ? Whether our new model can be applied to driving-in-the-left country depends on the traffic flow and safety index. At this moment ,our model becomes that keep left when driving unless passing vehicles in heavy traffic, while in light traffic, we retained two lanes on the left as travel lanes and one left lane as a passing lane. Similarly, taking score into consideration, which is composed of traffic and security Index. In fact, it is easy to find that β1 and β2 exchanged themselves, so w e have enacted new rules about reciprocity. It is that we retained two lanes on the left as travel lanes and one remaining lane as a passing lane when the traffic is heavy. In addition, maintaining the previous rule that keep left except passing in light traffic.Model 6: Intelligent system modelIf the intelligent system replace the artificial system, the safety index will increase in a big extent. At the same time, the traffic flow will increase, too. However,βwill not change because this factor depends on human’s performance. So we need to modify our model partly. When the traffic is heavy, we keep the previous rule. When the traffic is light, we make one lane for passing vehicles. With the help of intelligent system, we can make two lanes in the most side for passing and the lanes left for driving.Weaknesses and strengths of the modelAt the very beginning, we analyze some factors that will affect the result. We just think the problem is not very complex, so we simplify our work. We give all the index a reasonable flexible range. We also consult some authority research.However, we also have some disadvantages. We don’t take the traffic lights and some more complex road condition into consideration.Time is urgent, we don’t find more statistics to test our model accurately. Reference[1] [J] The model of the relationship between vehicle speed and traffic flow, Wei Wang, Nan Jing ,Jiangsu province[2] [D] The research of intelligent system and traffic flow, Jianhui Dai, Tian Jin[3] Addison, Low Paul S, David J. Order and chaos in the dynamics of vehicle platoons[ J] . Traffic Engineering & Control,1996, 37( 7 8) : 456 -459.[4] Daganzo C F, Cassidy M J, Bertini R L. Possible explanations of phase transitions in highway traffic[ J] . Transportation Research , Part A, 1999, 33( 5) : 365- 379.[ 5] Jiang Y. Traffic capacity speed and queue- discharge rate of Indiana. s four- lane freeway work zones[ A] . In: Transportation Research Record 1657, TRB , National Research Council [ C] . Washington D C, 1999. 39 -44.[6] Schonfeld P, Chien S. Optimal work zone lengths for two lane highways [ J] . Journal of Transportation Engineering , Urban Transportation Division, ASCE , 1999, 125( 1) : 21-29.[ 7] Nam D D, Drew D R. Analyzing freeway traffic under congestion: traffic dynamics approach [ J] . Journal of Transportation Engineering, Urban Transportation Division, ASCE,1998, 124( 3) : 208 -212.Appendix[1]alpha1=0.56;alpha2=1.76;beta1=1.16;beta2=0.98;X=20;K=0.65;g=10;C=5;V1=beta1*alpha1*K*X;V2=beta1*alpha2*K*X;V3=beta2*alpha1*K*X;V4=beta2*alpha2*K*X;L1=((beta1)^2*(alpha1)^2*K*X^2)/(2*g);L2=((beta1)^2*(alpha2)^2*K*X^2)/(2*g);L3=((beta2)^2*(alpha1)^2*K*X^2)/(2*g);L4=((beta2)^2*(alpha2)^2*K*X^2)/(2*g);S1=((1*V1)/(C+L1))*3600S2=((1*V2)/(C+L2))*3600S3=((1*V3)/(C+L3))*3600S4=((1*V4)/(C+L4))*3600W1=S1/(S1+S3);W2=S2/(S2+S4);W3=S3/(S1+S3);W4=S4/(S2+S4);I1=500+0.8*(abs(V1-X))^2*3.6^2+0.014*(abs(V1-X))^3*3.6^3;I2=500+0.8*(abs(V2-X))^2*3.6^2+0.014*(abs(V2-X))^3*3.6^3;I3=500+0.8*(abs(V3-X))^2*3.6^2+0.014*(abs(V3-X))^3*3.6^3;I4=500+0.8*(abs(V4-X))^2*3.6^2+0.014*(abs(V4-X))^3*3.6^3;Q1=1-(I1/(I1+I3));Q2=1-(I2/(I2+I4));Q3=1-(I3/(I1+I3));Q4=1-(I4/(I2+I4));P1=(W1+Q1)/2P2=(W2+Q2)/2P3=(W3+Q3)/2P4=(W4+Q4)/2S1 =2.8993e+003S2 =1.6144e+003S3 =2.8809e+003S4 =1.8482e+003P1 =0.5283P2 =0.4011P3 =0.4717P4 = 0.5989[2]alpha1=0.56;alpha2=1.76;beta1=1.16;beta2=0.98;X=8;K=0.65;g=10;C=5; V1=beta1*alpha1*K*X;V2=beta1*alpha2*K*X;V3=beta2*alpha1*K*X;V4=beta2*alpha2*K*X;L1=((beta1)^2*(alpha1)^2*K*X^2)/(2*g);L2=((beta1)^2*(alpha2)^2*K*X^2)/(2*g);L3=((beta2)^2*(alpha1)^2*K*X^2)/(2*g);L4=((beta2)^2*(alpha2)^2*K*X^2)/(2*g);S1=((1*V1)/(C+L1))*3600S2=((1*V2)/(C+L2))*3600S3=((1*V3)/(C+L3))*3600S4=((1*V4)/(C+L4))*3600W1=S1/(S1+S3);W2=S2/(S2+S4);W3=S3/(S1+S3);W4=S4/(S2+S4);I1=500+0.8*(abs(V1-X))^2*3.6^2+0.014*(abs(V1-X))^3*3.6^3;I2=500+0.8*(abs(V2-X))^2*3.6^2+0.014*(abs(V2-X))^3*3.6^3;I3=500+0.8*(abs(V3-X))^2*3.6^2+0.014*(abs(V3-X))^3*3.6^3;I4=500+0.8*(abs(V4-X))^2*3.6^2+0.014*(abs(V4-X))^3*3.6^3;Q1=1-(I1/(I1+I3));Q2=1-(I2/(I2+I4));Q3=1-(I3/(I1+I3));Q4=1-(I4/(I2+I4));P1=(W1+Q1)/2P2=(W2+Q2)/2P3=(W3+Q3)/2P4=(W4+Q4)/2S1 =2.0689e+003S2 = 2.7959e+003S3 =1.8259e+003S4 =2.8860e+003P1 = 0.5274P2 = 0.4795P3 = 0.4726P4 = 0.5205[3]alpha1=0.56;alpha2=1.76;beta1=1.16;beta2=0.98;X=30;K=0.65;g=10;C=5;V1=beta1*alpha1*K*XV2=beta1*alpha2*K*XV3=beta2*alpha1*K*XV4=beta2*alpha2*K*XL1=((beta1)^2*(alpha1)^2*K*X^2)/(2*g)L2=((beta1)^2*(alpha2)^2*K*X^2)/(2*g)L3=((beta2)^2*(alpha1)^2*K*X^2)/(2*g)L4=((beta2)^2*(alpha2)^2*K*X^2)/(2*g)S1=((1*V1)/(C+L1))*3600S2=((1*V2)/(C+L2))*3600S3=((1*V3)/(C+L3))*3600S4=((1*V4)/(C+L4))*3600W1=S1/(S1+S3)W2=S2/(S2+S4)W3=S3/(S1+S3)W4=S4/(S2+S4)I1=500+0.8*(abs(V1-X))^2*3.6^2+0.014*(abs(V1-X))^3*3.6^3 I2=500+0.8*(abs(V2-X))^2*3.6^2+0.014*(abs(V2-X))^3*3.6^3 I3=500+0.8*(abs(V3-X))^2*3.6^2+0.014*(abs(V3-X))^3*3.6^3 I4=500+0.8*(abs(V4-X))^2*3.6^2+0.014*(abs(V4-X))^3*3.6^3 Q1=1-(I1/(I1+I3))Q2=1-(I2/(I2+I4))Q3=1-(I3/(I1+I3))Q4=1-(I4/(I2+I4))P1=(W1+Q1)/2P2=(W2+Q2)/2P3=(W3+Q3)/2P4=(W4+Q4)/2S1 =2.6294e+003S2 =1.1292e+003S3 =2.7898e+003S4 =1.3159e+003P1 =0.5243P2 =0.3510P3 =0.4757P4 =0.6490。
美赛数学建模优秀论文
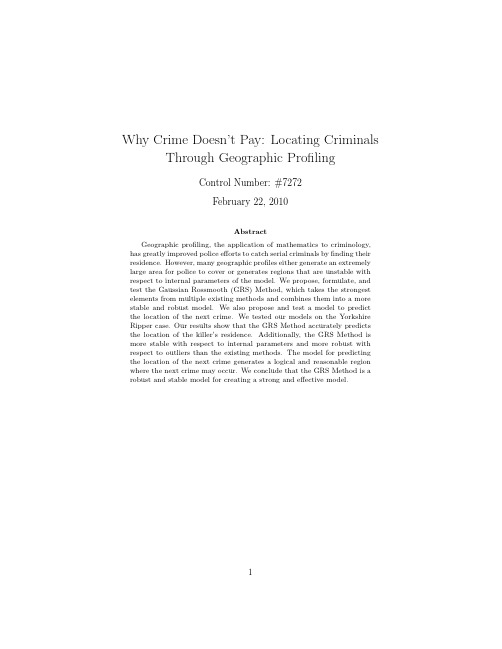
Why Crime Doesn’t Pay:Locating Criminals Through Geographic ProfilingControl Number:#7272February22,2010AbstractGeographic profiling,the application of mathematics to criminology, has greatly improved police efforts to catch serial criminals byfinding their residence.However,many geographic profiles either generate an extremely large area for police to cover or generates regions that are unstable with respect to internal parameters of the model.We propose,formulate,and test the Gaussian Rossmooth(GRS)Method,which takes the strongest elements from multiple existing methods and combines them into a more stable and robust model.We also propose and test a model to predict the location of the next crime.We tested our models on the Yorkshire Ripper case.Our results show that the GRS Method accurately predicts the location of the killer’s residence.Additionally,the GRS Method is more stable with respect to internal parameters and more robust with respect to outliers than the existing methods.The model for predicting the location of the next crime generates a logical and reasonable region where the next crime may occur.We conclude that the GRS Method is a robust and stable model for creating a strong and effective model.1Control number:#72722Contents1Introduction4 2Plan of Attack4 3Definitions4 4Existing Methods54.1Great Circle Method (5)4.2Centrography (6)4.3Rossmo’s Formula (8)5Assumptions8 6Gaussian Rossmooth106.1Properties of a Good Model (10)6.2Outline of Our Model (11)6.3Our Method (11)6.3.1Rossmooth Method (11)6.3.2Gaussian Rossmooth Method (14)7Gaussian Rossmooth in Action157.1Four Corners:A Simple Test Case (15)7.2Yorkshire Ripper:A Real-World Application of the GRS Method167.3Sensitivity Analysis of Gaussian Rossmooth (17)7.4Self-Consistency of Gaussian Rossmooth (19)8Predicting the Next Crime208.1Matrix Method (20)8.2Boundary Method (21)9Boundary Method in Action21 10Limitations22 11Executive Summary2311.1Outline of Our Model (23)11.2Running the Model (23)11.3Interpreting the Results (24)11.4Limitations (24)12Conclusions25 Appendices25 A Stability Analysis Images252Control number:#72723List of Figures1The effect of outliers upon centrography.The current spatial mean is at the red diamond.If the two outliers in the lower leftcorner were removed,then the center of mass would be locatedat the yellow triangle (6)2Crimes scenes that are located very close together can yield illog-ical results for the spatial mean.In this image,the spatial meanis located at the same point as one of the crime scenes at(1,1)..7 3The summand in Rossmo’s formula(2B=6).Note that the function is essentially0at all points except for the scene of thecrime and at the buffer zone and is undefined at those points..9 4The summand in smoothed Rossmo’s formula(2B=6,φ=0.5, and EPSILON=0.5).Note that there is now a region aroundthe buffer zone where the value of the function no longer changesvery rapidly (13)5The Four Corners Test Case.Note that the highest hot spot is located at the center of the grid,just as the mathematics indicates.15 6Crimes and residences of the Yorkshire Ripper.There are two residences as the Ripper moved in the middle of the case.Someof the crime locations are assaults and others are murders (16)7GRS output for the Yorkshire Ripper case(B=2.846).Black dots indicate the two residences of the killer (17)8GRS method run on Yorkshire Ripper data(B=2).Note that the major difference between this model and Figure7is that thehot zones in thisfigure are smaller than in the original run (18)9GRS method run on Yorkshire Ripper data(B=4).Note that the major difference between this model and Figure7is that thehot zones in thisfigure are larger than in the original run (19)10The boundary region generated by our Boundary Method.Note that boundary region covers many of the crimes committed bythe Sutcliffe (22)11GRS Method onfirst eleven murders in the Yorkshire Ripper Case25 12GRS Method onfirst twelve murders in the Yorkshire Ripper Case263Control number:#727241IntroductionCatching serial criminals is a daunting problem for law enforcement officers around the world.On the one hand,a limited amount of data is available to the police in terms of crimes scenes and witnesses.However,acquiring more data equates to waiting for another crime to be committed,which is an unacceptable trade-off.In this paper,we present a robust and stable geographic profile to predict the residence of the criminal and the possible locations of the next crime.Our model draws elements from multiple existing models and synthesizes them into a unified model that makes better use of certain empirical facts of criminology.2Plan of AttackOur objective is to create a geographic profiling model that accurately describes the residence of the criminal and predicts possible locations for the next attack. In order to generate useful results,our model must incorporate two different schemes and must also describe possible locations of the next crime.Addi-tionally,we must include assumptions and limitations of the model in order to ensure that it is used for maximum effectiveness.To achieve this objective,we will proceed as follows:1.Define Terms-This ensures that the reader understands what we aretalking about and helps explain some of the assumptions and limitations of the model.2.Explain Existing Models-This allows us to see how others have at-tacked the problem.Additionally,it provides a logical starting point for our model.3.Describe Properties of a Good Model-This clarifies our objectiveand will generate a sketelon for our model.With this underlying framework,we will present our model,test it with existing data,and compare it against other models.3DefinitionsThe following terms will be used throughout the paper:1.Spatial Mean-Given a set of points,S,the spatial mean is the pointthat represents the middle of the data set.2.Standard Distance-The standard distance is the analog of standarddeviation for the spatial mean.4Control number:#727253.Marauder-A serial criminal whose crimes are situated around his or herplace of residence.4.Distance Decay-An empirical phenomenon where criminal don’t traveltoo far to commit their crimes.5.Buffer Area-A region around the criminal’s residence or workplacewhere he or she does not commit crimes.[1]There is some dispute as to whether this region exists.[2]In our model,we assume that the buffer area exists and we measure it in the same spatial unit used to describe the relative locations of other crime scenes.6.Manhattan Distance-Given points a=(x1,y1)and b=(x2,y2),theManhattan distance from a to b is|x1−x2|+|y1−y2|.This is also known as the1−norm.7.Nearest Neighbor Distance-Given a set of points S,the nearestneighbor distance for a point x∈S ismin|x−s|s∈S−{x}Any norm can be chosen.8.Hot Zone-A region where a predictive model states that a criminal mightbe.Hot zones have much higher predictive scores than other regions of the map.9.Cold Zone-A region where a predictive model scores exceptionally low. 4Existing MethodsCurrently there are several existing methods for interpolating the position of a criminal given the location of the crimes.4.1Great Circle MethodIn the great circle method,the distances between crimes are computed and the two most distant crimes are chosen.Then,a great circle is drawn so that both of the points are on the great circle.The midpoint of this great circle is then the assumed location of the criminal’s residence and the area bounded by the great circle is where the criminal operates.This model is computationally inexpensive and easy to understand.[3]Moreover,it is easy to use and requires very little training in order to master the technique.[2]However,it has certain drawbacks.For example,the area given by this method is often very large and other studies have shown that a smaller area suffices.[4]Additionally,a few outliers can generate an even larger search area,thereby further slowing the police effort.5Control number:#727264.2CentrographyIn centrography ,crimes are assigned x and y coordinates and the “center of mass”is computed as follows:x center =n i =1x i ny center =n i =1y i nIntuitively,centrography finds the mean x −coordinate and the mean y -coordinate and associates this pair with the criminal’s residence (this is calledthe spatial mean ).However,this method has several flaws.First,it can be unstablewith respect to outliers.Consider the following set of points (shown in Figure 1:Figure 1:The effect of outliers upon centrography.The current spatial mean is at the red diamond.If the two outliers in the lower left corner were removed,then the center of mass would be located at the yellow triangle.Though several of the crime scenes (blue points)in this example are located in a pair of upper clusters,the spatial mean (red point)is reasonably far away from the clusters.If the two outliers are removed,then the spatial mean (yellow point)is located closer to the two clusters.A similar method uses the median of the points.The median is not so strongly affected by outliers and hence is a more stable measure of the middle.[3]6Control number:#72727 Alternatively,we can circumvent the stability problem by incorporating the 2-D analog of standard deviation called the standard distance:σSD=d center,iNwhere N is the number of crimes committed and d center,i is the distance from the spatial center to the i th crime.By incorporating the standard distance,we get an idea of how“close together”the data is.If the standard distance is small,then the kills are close together. However,if the standard distance is large,then the kills are far apart. Unfortunately,this leads to another problem.Consider the following data set (shown in Figure2):Figure2:Crimes scenes that are located very close together can yield illogical results for the spatial mean.In this image,the spatial mean is located at the same point as one of the crime scenes at(1,1).In this example,the kills(blue)are closely clustered together,which means that the centrography model will yield a center of mass that is in the middle of these crimes(in this case,the spatial mean is located at the same point as one of the crimes).This is a somewhat paradoxical result as research in criminology suggests that there is a buffer area around a serial criminal’s place of residence where he or she avoids the commission of crimes.[3,1]That is,the potential kill area is an annulus.This leads to Rossmo’s formula[1],another mathematical model that predicts the location of a criminal.7Control number:#727284.3Rossmo’s FormulaRossmo’s formula divides the map of a crime scene into grid with i rows and j columns.Then,the probability that the criminal is located in the box at row i and column j isP i,j=kTc=1φ(|x i−x c|+|y j−y c|)f+(1−φ)(B g−f)(2B−|x i−x c|−|y j−y c|)gwhere f=g=1.2,k is a scaling constant(so that P is a probability function), T is the total number of crimes,φputs more weight on one metric than the other,and B is the radius of the buffer zone(and is suggested to be one-half the mean of the nearest neighbor distance between crimes).[1]Rossmo’s formula incorporates two important ideas:1.Criminals won’t travel too far to commit their crimes.This is known asdistance decay.2.There is a buffer area around the criminal’s residence where the crimesare less likely to be committed.However,Rossmo’s formula has two drawbacks.If for any crime scene x c,y c,the equality2B=|x i−x c|+|y j−y c|,is satisfied,then the term(1−φ)(B g−f)(2B−|x i−x c|−|y j−y c|)gis undefined,as the denominator is0.Additionally,if the region associated withij is the same region as the crime scene,thenφi c j c is unde-fined by the same reasoning.Figure3illustrates this:This“delta function-like”behavior is disconcerting as it essentially states that the criminal either lives right next to the crime scene or on the boundary defined by Rossmo.Hence,the B-value becomes exceptionally important and needs its own heuristic to ensure its accuracy.A non-optimal choice of B can result in highly unstable search zones that vary when B is altered slightly.5AssumptionsOur model is an expansion and adjustment of two existing models,centrography and Rossmo’s formula,which have their own underlying assumptions.In order to create an effective model,we will make the following assumptions:1.The buffer area exists-This is a necessary assumption and is the basisfor one of the mathematical components of our model.2.More than5crimes have occurred-This assumption is importantas it ensures that we have enough data to make an accurate model.Ad-ditionally,Rossmo’s model stipulates that5crimes have occurred[1].8Control number:#72729Figure3:The summand in Rossmo’s formula(2B=6).Note that the function is essentially0at all points except for the scene of the crime and at the buffer zone and is undefined at those points3.The criminal only resides in one location-By this,we mean thatthough the criminal may change residence,he or she will not move toa completely different area and commit crimes there.Empirically,thisassumption holds,with a few exceptions such as David Berkowitz[1].The importance of this assumption is it allows us to adapt Rossmo’s formula and the centrography model.Both of these models implicitly assume that the criminal resides in only one general location and is not nomadic.4.The criminal is a marauder-This assumption is implicitly made byRossmo’s model as his spatial partition method only considers a small rectangular region that contains all of the crimes.With these assumptions,we present our model,the Gaussian Rossmooth method.9Control number:#7272106Gaussian Rossmooth6.1Properties of a Good ModelMuch of the literature regarding criminology and geographic profiling contains criticism of existing models for catching criminals.[1,2]From these criticisms, we develop the following criteria for creating a good model:1.Gives an accurate prediction for the location of the criminal-This is vital as the objective of this model is to locate the serial criminal.Obviously,the model cannot give a definite location of the criminal,but it should at least give law enforcement officials a good idea where to look.2.Provides a good estimate of the location of the next crime-Thisobjective is slightly harder than thefirst one,as the criminal can choose the location of the next crime.Nonetheless,our model should generate a region where law enforcement can work to prevent the next crime.3.Robust with respect to outliers-Outliers can severely skew predic-tions such as the one from the centrography model.A good model will be able to identify outliers and prevent them from adversely affecting the computation.4.Consitent within a given data set-That is,if we eliminate data pointsfrom the set,they do not cause the estimation of the criminal’s location to change excessively.Additionally,we note that if there are,for example, eight murders by one serial killer,then our model should give a similar prediction of the killer’s residence when it considers thefirstfive,first six,first seven,and all eight murders.5.Easy to compute-We want a model that does not entail excessivecomputation time.Hence,law enforcement will be able to get their infor-mation more quickly and proceed with the case.6.Takes into account empirical trends-There is a vast amount ofempirical data regarding serial criminals and how they operate.A good model will incorporate this data in order to minimize the necessary search area.7.Tolerates changes in internal parameters-When we tested Rossmo’sformula,we found that it was not very tolerant to changes of the internal parameters.For example,varying B resulted in substantial changes in the search area.Our model should be stable with respect to its parameters, meaning that a small change in any parameter should result in a small change in the search area.10Control number:#7272116.2Outline of Our ModelWe know that centrography and Rossmo’s method can both yield valuable re-sults.When we used the mean and the median to calculate the centroid of a string of murders in Yorkshire,England,we found that both the median-based and mean-based centroid were located very close to the home of the criminal. Additionally,Rossmo’s method is famous for having predicted the home of a criminal in Louisiana.In our approach to this problem,we adapt these methods to preserve their strengths while mitigating their weaknesses.1.Smoothen Rossmo’s formula-While the theory behind Rossmo’s for-mula is well documented,its implementation isflawed in that his formula reaches asymptotes when the distance away from a crime scene is0(i.e.point(x i,y j)is a crime scene),or when a point is exactly2B away froma crime scene.We must smoothen Rossmo’s formula so that idea of abuffer area is mantained,but the asymptotic behavior is removed and the tolerance for error is increased.2.Incorporate the spatial mean-Using the existing crime scenes,we willcompute the spatial mean.Then,we will insert a Gaussian distribution centered at that point on the map.Hence,areas near the spatial mean are more likely to come up as hot zones while areas further away from the spatial mean are less likely to be viewed as hot zones.This ensures that the intuitive idea of centrography is incorporated in the model and also provides a general area to search.Moreover,it mitigates the effect of outliers by giving a probability boost to regions close to the center of mass,meaning that outliers are unlikely to show up as hot zones.3.Place more weight on thefirst crime-Research indicates that crimi-nals tend to commit theirfirst crime closer to their home than their latter ones.[5]By placing more weight on thefirst crime,we can create a model that more effectively utilizes criminal psychology and statistics.6.3Our Method6.3.1Rossmooth MethodFirst,we eliminated the scaling constant k in Rossmo’s equation.As such,the function is no longer a probability function but shows the relative likelihood of the criminal living in a certain sector.In order to eliminate the various spikes in Rossmo’s method,we altered the distance decay function.11Control number:#727212We wanted a distance decay function that:1.Preserved the distance decay effect.Mathematically,this meant that thefunction decreased to0as the distance tended to infinity.2.Had an interval around the buffer area where the function values wereclose to each other.Therefore,the criminal could ostensibly live in a small region around the buffer zone,which would increase the tolerance of the B-value.We examined various distance decay functions[1,3]and found that the func-tions resembled f(x)=Ce−m(x−x0)2.Hence,we replaced the second term in Rossmo’s function with term of the form(1−φ)×Ce−k(x−x0)2.Our modified equation was:E i,j=Tc=1φ(|x i−x c|+|y j−y c|)f+(1−φ)×Ce−(2B−(|x i−x c|+|y j−y c|))2However,this maintained the problematic region around any crime scene.In order to eliminate this problem,we set an EPSILON so that any point within EPSILON(defined to be0.5spatial units)of a crime scene would have a weighting of a constant cap.This prevented the function from reaching an asymptote as it did in Rossmo’s model.The cap was defined asCAP=φEPSILON fThe C in our modified Rossmo’s function was also set to this cap.This way,the two maximums of our modified Rossmo’s function would be equal and would be located at the crime scene and the buffer zone.12Control number:#727213This function yielded the following curve (shown in in Figure4),which fit both of our criteria:Figure 4:The summand in smoothed Rossmo’s formula (2B =6,φ=0.5,and EPSILON =0.5).Note that there is now a region around the buffer zone where the value of the function no longer changes very rapidly.At this point,we noted that E ij had served its purpose and could be replaced in order to create a more intuitive idea of how the function works.Hence,we replaced E i,j with the following sum:Tc =1[D 1(c )+D 2(c )]where:D 1(c )=min φ(|x i −x c |+|y j −y c |),φEPSILON D 2(c )=(1−φ)×Ce −(2B −(|x i −x c |+|y j −y c |))2For equal weighting on both D 1(c )and D 2(c ),we set φto 0.5.13Control number:#7272146.3.2Gaussian Rossmooth MethodNow,in order to incorporate the inuitive method,we used centrography to locate the center of mass.Then,we generated a Gaussian function centered at this point.The Gaussian was given by:G=Ae −@(x−x center)22σ2x+(y−y center)22σ2y1Awhere A is the amplitude of the peak of the Gaussian.We determined that the optimal A was equal to2times the cap defined in our modified Rossmo’s equation.(A=2φEPSILON f)To deal with empirical evidence that thefirst crime was usually the closest to the criminal’s residence,we doubled the weighting on thefirst crime.However, the weighting can be represented by a constant,W.Hence,ourfinal Gaussian Rosmooth function was:GRS(x i,y j)=G+W(D1(1)+D2(1))+Tc=2[D1(c)+D2(c)]14Control number:#7272157Gaussian Rossmooth in Action7.1Four Corners:A Simple Test CaseIn order to test our Gaussain Rossmooth(GRS)method,we tried it against a very simple test case.We placed crimes on the four corners of a square.Then, we hypothesized that the model would predict the criminal to live in the center of the grid,with a slightly higher hot zone targeted toward the location of the first crime.Figure5shows our results,whichfits our hypothesis.Figure5:The Four Corners Test Case.Note that the highest hot spot is located at the center of the grid,just as the mathematics indicates.15Control number:#727216 7.2Yorkshire Ripper:A Real-World Application of theGRS MethodAfter the model passed a simple test case,we entered the data from the Yorkshire Ripper case.The Yorkshire Ripper(a.k.a.Peter Sutcliffe)committed a string of13murders and several assaults around Northern England.Figure6shows the crimes of the Yorkshire Ripper and the locations of his residence[1]:Figure6:Crimes and residences of the Yorkshire Ripper.There are two res-idences as the Ripper moved in the middle of the case.Some of the crime locations are assaults and others are murders.16Control number:#727217 When our full model ran on the murder locations,our data yielded the image show in Figure7:Figure7:GRS output for the Yorkshire Ripper case(B=2.846).Black dots indicate the two residences of the killer.In this image,hot zones are in red,orange,or yellow while cold zones are in black and blue.Note that the Ripper’s two residences are located in the vicinity of our hot zones,which shows that our model is at least somewhat accurate. Additionally,regions far away from the center of mass are also blue and black, regardless of whether a kill happened there or not.7.3Sensitivity Analysis of Gaussian RossmoothThe GRS method was exceptionally stable with respect to the parameter B. When we ran Rossmo’s model,we found that slight variations in B could create drastic variations in the given distribution.On many occassions,a change of 1spatial unit in B caused Rossmo’s method to destroy high value regions and replace them with mid-level value or low value regions(i.e.,the region would completely dissapper).By contrast,our GRS method scaled the hot zones.17Control number:#727218 Figures8and9show runs of the Yorkshire Ripper case with B-values of2and 4respectively.The black dots again correspond to the residence of the criminal. The original run(Figure7)had a B-value of2.846.The original B-value was obtained by using Rossmo’s nearest neighbor distance metric.Note that when B is varied,the size of the hot zone varies,but the shape of the hot zone does not.Additionally,note that when a B-value gets further away from the value obtained by the nearest neighbor distance metric,the accuracy of the model decreases slightly,but the overall search areas are still quite accurate.Figure8:GRS method run on Yorkshire Ripper data(B=2).Note that the major difference between this model and Figure7is that the hot zones in this figure are smaller than in the original run.18Control number:#727219Figure9:GRS method run on Yorkshire Ripper data(B=4).Note that the major difference between this model and Figure7is that the hot zones in this figure are larger than in the original run.7.4Self-Consistency of Gaussian RossmoothIn order to test the self-consistency of the GRS method,we ran the model on thefirst N kills from the Yorkshire Ripper data,where N ranged from6to 13,inclusive.The self-consistency of the GRS method was adversely affected by the center of mass correction,but as the case number approached11,the model stabilized.This phenomenon can also be attributed to the fact that the Yorkshire Ripper’s crimes were more separated than those of most marauders.A selection of these images can be viewed in the appendix.19Control number:#7272208Predicting the Next CrimeThe GRS method generates a set of possible locations for the criminal’s resi-dence.We will now present two possible methods for predicting the location of the criminal’s next attack.One method is computationally expensive,but more rigorous while the other method is computationally inexpensive,but more intuitive.8.1Matrix MethodGiven the parameters of the GRS method,the region analyzed will be a square with side length n spatial units.Then,the output from the GRS method can be interpreted as an n×n matrix.Hence,for any two runs,we can take the norm of their matrix difference and compare how similar the runs were.With this in mind,we generate the following method.For every point on the grid:1.Add crime to this point on the grid.2.Run the GRS method with the new set of crime points.pare the matrix generated with these points to the original matrix bysubtracting the components of the original matrix from the components of the new matrix.4.Take a matrix norm of this difference matrix.5.Remove the crime from this point on the grid.As a lower matrix norm indicates a matrix similar to our original run,we seek the points so that the matrix norm is minimized.There are several matrix norms to choose from.We chose the Frobenius norm because it takes into account all points on the difference matrix.[6]TheFrobenius norm is:||A||F=mi=1nj=1|a ij|2However,the Matrix Method has one serious drawback:it is exceptionally expensive to compute.Given an n×n matrix of points and c crimes,the GRS method runs in O(cn2).As the Matrix method runs the GRS method at each of n2points,we see that the Matrix Method runs in O(cn4).With the Yorkshire Ripper case,c=13and n=151.Accordingly,it requires a fairly long time to predict the location of the next crime.Hence,we present an alternative solution that is more intuitive and efficient.20Control number:#7272218.2Boundary MethodThe Boundary Method searches the GRS output for the highest point.Then,it computes the average distance,r,from this point to the crime scenes.In order to generate a resonable search area,it discards all outliers(i.e.,points that were several times further away from the high point than the rest of the crimes scenes.)Then,it draws annuli of outer radius r(in the1-norm sense)around all points above a certain cutoffvalue,defined to be60%of the maximum value. This value was chosen as it was a high enough percentage value to contain all of the hot zones.The beauty of this method is that essentially it uses the same algorithm as the GRS.We take all points on the hot zone and set them to“crime scenes.”Recall that our GRS formula was:GRS(x i,y j)=G+W(D1(1)+D2(1))+Tc=2[(D1(c)+D2(c))]In our boundary model,we only take the terms that involve D2(c).However, let D 2(c)be a modified D2(c)defined as follows:D 2(c)=(1−φ)×Ce−(r−(|x i−x c|+|y j−y c|))2Then,the boundary model is:BS(x i,y j)=Tc=1D 2(c)9Boundary Method in ActionThis model generates an outer boundary for the criminal’s next crime.However, our model does notfill in the region within the inner boundary of the annulus. This region should still be searched as the criminal may commit crimes here. Figure10shows the boundary generated by analyzing the Yorkshire Ripper case.21。
美赛o奖12149

Designing a Halfpipe for Advanced SnurfersTeam Control#12149February14,2011AbstractThe shape of a snowboarding halfpipe can be designed to maximize the vertical air a professional snowboarder will achieve;however,a practical course will take into account factors such as the number of cycles a snowboarder can make side to side as he descends the mountain,and the shape that yields the best launch for stunts.As a snowboarder descends a halfpipe the forces acting on him are dependent on the shape of the course.We will use Newton’s Second Law of Motion to sum the forces in each direction.Then we used a Runga-Kutta method computer simulation to deter-mine the acceleration of the snowboarder down the course.We tested three different functions that describe the shape of the half pipe,with three varying slopes for each. Using a computer simulation and physical testing the velocity of the snowboarder can be analyzed at the edge of the half pipe.The shapes that yield the largest vertical air and the most practical course will then be chosen as the best design.The forces taken into consideration in these models include:gravity,centrifugal, frictional,air resistance,and a constant force applied in the direction of travel that mimics the force from a professional snowboarder pumping his legs.These models do not take into account the force a professional snowboarder’s stylistic force would use to guide his path along the halfpipe.After running our three models we determined that out of the various shapes tested the best vertical height was optimized at a mountain slope of45degrees with a half pipe shape of the parabolaz(y)=.07y2−7The best practical model was found to be the same parabola at a mountain slope of25degrees.The Runge Kutta method approximation had an error of0.1%.Our best model is the comprehensive three dimensional force because it most realistically describes a snowboarder on a halfpipe.Team Control Number12149Page1of23 1IntroductionDeveloped in the1970’s,snowboarding has come a long way from the original snurfer (snow-surfer)which was an attempt by Shermann Poppen to bring surfing to the Rocky Mountains.Since then snowboarding has taken offwith perfecting ramps and slopes to enable a variety of tricks and stunts.Some of these stunts require a simple slope,others a rail,and for this problem we will look specifically at the half-pipe in order to optimize vertical air which can be defined as the distance a skilled snowboarder can obtain by being launched offthe edge of the halfpipe.1.1The ProblemA Basic HalfpipeA basic halfpipe consists of two concave ramps separated by a distance which totals to the width of the half pipe(figure10).The vertical side of the ramp,or the edge,is called a vertical,the curved part of the vertical is called the transition,and the distance between the two ramps is called theflat.In a basic halfpipe the vertical and transition allow for back and forth motion using the force of gravity to give the snowboarder a velocity.A snowboarder uses theflat to regain balance as well as a time to pump.Pumping adds work to the system and gives the boarder a greater velocity to make it up the opposite vertical and obtain a higher vertical air.Because theflat optimizes the angle that the force exerts on the snowboarder pumping happens primarily on theflat(see equation6).A Snowboarding HalfpipeA snowboarding halfpipe is a basic halfpipe that is built onto a hill(figure9).The slope of the hill gives a new form of energy that can be converted to velocity and used to maximize vertical air.The plane of the transition is oriented downhill at a slight grade to allow riders to use gravity in two directions to create a velocity both back and forth and down the slope to develop the optimal speed for stunts that require vertical air.The character of the half pipe depends mostly on the relationship between the transition angle and the height of each vertical.The higher the vertical the more difficult it is to land while a narrow stretch between the two verticals provides less time to recover as well as less opportunity to pump.So what is the best shape of a half pipe to optimize a skilled snowboarder’s vertical air?And what kind of options does the snowboarder have for a variety of stunts?And what changes could be made to develop a practical course?Our ApproachIn order tofind a snowboarder’s optimized vertical air we used both conservation of energy and Newton’s2nd Law to account for the various forces acting on a snowboarder while traveling down the half-pipe.We constructed models based on the law of conservationTeam Control Number12149Page2of23 of energy and Newton’s ing a function z(y)for the shape of the halfpipe we were able to set up a model that allows a function to be easily altered and evaluated for any shape.•Conservation of Energy Model:The energy model depends only on the change in height and gravity(fig1).Work can be added to this model in the form of a snowboarder pumping over theflat.This model gives an idea of how a more complex model might look.•Two Dimensional Force Model:We used Newton’s2nd Law in2D to minimize the energy lost through friction and air resistance by creating a system of ordinary differential equations that described the forces acting on the snowboarder(figure2).We evaluated a series of equations for the curve of the halfpipe,and evaluated the numerical results to determine which curve gave the best vertical air.This model acts on the assumption that the snowboarder is in a basic halfpipe which does not include the slope of the mountain.•Comprehensive Three Dimensional Force Model:Ourfinal and most compre-hensive model takes into account both the potential energy converted from the descent down the slope as well as friction from both air and snow.In order to model the loss in potential energy due to the decreasing height we developed a model that is based on not only the pitch of the two adjacent sides of the half-pipe but also the slope of the hill that the half-pipe is on.We continued using Newton’s2nd Law of motion as we did for the two dimensional model to determine the maximum height that can be reached by a snowboarder under the influence of gravity,pumping,air resistance,and the friction between the snow and the board.2Models2.1Conservation of EnergyHow It WorksThe conservation of energy model is our most intuitive analysis of the snowboarders speed. Looking at the conversion of potential energy to a new potential energy(through a change in height)we can determine analytically the kinetic energy used to launch a snowboarder offof the verticals and gain as much vertical air as possible(figure1).Team Control Number12149Page3of23Figure1:Energy Model DiagramKE i+P E i=KE f+P E f(1) Under the following conditions:v i=0(2)h i>h f(3) The conservation of energy results in the following equation.v=(2g(h i−h f))(4)Without adding any work the snowboarder will never go beyond the original height.In order to see the importance of pumping we added work to the system and the equation of velocity is changed by a factor of work(equation6).W=F applied•d=F applied d cosσ(5) F(applied)is the force applied by the snowboarder,d is the distance between the two verticals andσis the angle in which the force is applied.v=(2g(h i−h f)+2W)(6)A higher velocity means there is a higher vertical air.However,the angle of take offmust be taken into account as well.Considerθwhich is the angle between the horizontal axis andTeam Control Number12149Page4of23the direction of the velocity of the snowboarder(figure1).Whenθ=π2then our velocityis entirely in the z direction and our vertical air is maximized.Conversely we can also see that whenθ=0our velocity is entirely in the x direction and we have no vertical air.ConsequencesThe information provided by this analysis gives an intuitive look into what a snow-boarder’s motion would be neglecting all forms of friction in a two dimensional plane,with the option to add work.A closer look at the direction of the resulting velocity shows the importance of the takeoffangle.By choosing the angle of take off(angle between slope and horizon)to be as close as possible to90degrees a snowboarder can maximize vertical air.Strengths•This model gives information for the height difference necessary to give a snowboarder vertical air using only potential energy.•Simulations are quick,and it is easy to alter the height difference of the halfpipe verticals.•This model allows work to be added to the system.•The model only depends on the original andfinals height which means any path could be taken.Weaknesses•This model does not provide a way to solve for an optimal angle of either the half-pipe or the hill.•This model does not take into account any frictional forces.2.2Two Dimensional Force ModelHow It WorksIn order to model a snowboarder’s path down a two dimensional movement we used a set of linear ordinary differential equations for the forces acting on the snowboarder in both z and y directions(down and left to right).The gravitational,frictional and drag forces acting on the snowboarder are summed along the z and y axis(looking in the3-D z-y plane).F drag=12ρair ac d(v2x+v2y)m(7)N=g cos(θ)−(v2y+v2z)ρ(8)Team Control Number12149Page5of23Using Newton’s Second Law of MotionF=m a we can see that the force acting onthe snowboarder in the y-axis is equal to mass times acceleration along the y-axis.Applying this to both the y and z axis yields two second order differential equations(equations9and 10).dv ydt=N sinθ−µN cosθ−F dyz cosθ+ρcosθ(9)dv zdt=−g+N cosθ−µN sinθ+F dyz sinθ−ρsinθ(10) These two second order differential equations can be broken down into fourfirst order dif-ferential equations.(Further derivation of these equations can be reviewed in the appendix).Using a computer simulated Runga-Kutta method,the four differential equations are solved yielding the snowboarder’s path and velocity across the halfpipe.A force was added in the direction of travel that mimics the force of the snowboarder pumping his legs to add speed(equation5).ConsequencesThis model corrects for the drag force and frictional force acting on the snowboarder. These forces are dependent upon velocity across the halfpipe.This model shows how different shapes affect the amount of energy lost due to friction and drag.By adding the force of the snowboarder pumping his legs,the model shows the velocity in the z direction at the point the snowboarder leaves the halfpipe which is proportional to the vertical air the snowboarder achieves.With the added force being constant,and the dimensions similar,each shape can be analyzed side by side to determine the best cross-sectional shape for a halfpipe(figure2).Figure2:Two dimensional Force ModelTeam Control Number12149Page6of23•This method takes into account the frictional and drag forces that thefirst model neglected.•Offers a solution for optimizing the pitch of the halfpipe.•Simulations are quick,and it is easy to alter the height of the verticals or the width of the halfpipe.•The shape of the halfpipe can be changed into any function for z(y).Weaknesses•This model neglects the acceleration added from the halfpipe sloping down a hill.•Only the z-y cross-sectional dimensions are optimized.2.3Comprehensive Three Dimensional Force ModelIn order to model this problem in three dimensions we came up with a three dimensional force diagram.In this model we are taking into account the zx plane’s potential energy that is being converted into kinetic energy as the snowboarder descends the hill.For the sake of simplicity we shifted the axis used to define the zx plane so that the normal force is solely in the z direction(figure3).Figure3:zx plane for3D Force ModelAn important observation to take into account for this model is the fact that we redefinedTeam Control Number12149Page7of23 the coordinate system;this means that the force of gravity used in the2D model of the zy plane(figure2)is now gravity multiplied by the cos(φ).Whereφis the angle of the halfpipe on the hill.dv x=g sinφma−g cosφµ−F dx(11)dtdv y=N sinθ−µN cosθ−F dyz cosθ+ρcosθ(12)dtdv z=−g cosφ+N cosθ−µN sinθ+F dyz sinθ−ρsinθ(13) dtConsequencesWith the force caused by the ramp going down a slope added,this model allows the path and the velocity of the snowboarder side to side movement across the halfpipe to be evaluated as well as the velocity down the hill.This lets us see how the slope of the hill effects the vertical distance above the halfepipe the snowboarder is able to achieve.This model can be used to look at both variables;slope and the function of the cross-section,which allows us to choose the pair that yields the most desirable results. Strengths•This model takes into account the forces in all three directions by including the slope of the halfpipe down the hill.•This model allows different combinations of slope and cross-sectionals to be tested together.•This model offers a way to easily change the parameters of the system in question. Weaknesses•This model does not take into account the stylistic ways a snowboarder uses to slow himself down in the x direction.•This model does not take into account how a professional snowboarder will guide his path down the halfpipe2.4Assumptions•The snowboarder does not add force to the system causing a change in direction.•The force added in the two-dimensional model to mimic the snowboarder pumping his legs to add speed was added by adding a single constant force in the direction of travel.•In the three-dimensional model the added force is only taken into consideration in the z-y plane.Team Control Number12149Page8of23•No projectile motion is modeled for after the snowboarder leaves the halfpipe.3ResultsAfter evaluating each model we came to the conclusion that the best model is the three dimensional force model.It has the most broad spectra with the ability to easily change the slope of the mountain or the halfpipe verticals and still not lose the information needed to observe the snowboarder’s velocity in all directions.Both the conservation of energy modeland the two dimensional force model are necessary for evaluating how the slope changesa snowboarder’s velocity,as well as demonstrating the importance of strategies such as pumping,that add work to the system.3.1Conservation of Energy Model ResultsThis was an analytical model so there is no data to graph.However,a clear understandingof the model gives an intuitive look into why we choose to use second order differential equations to model the more complex systems.The information taken from this model isthat in order to maximize vertical air the take offangle must be as close as possible to90 degrees.Another less realistic suggestion to maximize velocity in the absence of work is to make one vertical shorter than the other in order to achieve vertical air from the left over converted potential energy(figure1).3.2Two Dimensional Force Model ResultsThe two dimensional force model is used to evaluate thefinal velocity of the snowboarderat the peak of the opposite vertical.By breaking the velocity into y and z components we were able to compare thefinal z velocity of three different functions.Function Z Velocity(m/s)Y Velocity(m/s)Line Color Initial Position(m)Center Parabola2 2.71546 1.357389Green(-10,0)(0,-10) Circle 2.6709210.139753Black(-10,0)(0,-10) Parabola1 3.54291 2.527488Red(-10,0)(0,-7)Where the functions used are:Shape Name Formula Parabola1z(y)=.07y2−7 Parabola2z(y)=.1y2−10 Circle z(y)=100−y2In order to be able to compare these three functions,all the functions should initially start at the same(z,y)and span the same y distance(width of the half pipe).The following graph is showing the motion of a snowboarder in the yz plane with friction and air resistance added.Team Control Number12149Page9of23Figure4:Dispacement in the YZ PlaneIn order to evaluate the affect of work done by the snowboarder a set value for the force added through work was added to the model.Because the snowboarder cannot reach a height greater than his original height using only potential energy he must use the pumping method to give him a greater velocity.This can be seen in the following graph of the snowboarder’s displacement.Team Control Number12149Page10of23Figure5:Dispacement in the YZ Plane Adding Work By modeling this further into a projectile motion problem we can verify that the function yielding the greatest velocity in the z direction will give the greatest vertical air.So,by comparing and contrasting the three different models we can extrapolate that:•Parabola1is23percent better than parabola2.•Parabola2is1.6percent better than the circle.3.3Comprehensive Three Dimensional Force Model ResultsThe comprehensive three dimensional force model allows the displacement in the x,y and the z direction to be graphed in a three dimensional graph(figure6).Team Control Number12149Page11of23Figure6:Ariel View of Three Dimensional Displacement To evaluate the best halfpipe shape we again looked at the velocities at the top of the vertical point on the halfpipe.Like we did for the two dimensional model we evaluate the velocities this time in both y and z directions.The x velocity is the speed that the snow boarder is traveling down the slope and because the model does not take into account the stylistic ways that snowboarders convert the x velocity we can ignore them for the optimization of vertical air.φShape Y Velocity Z Velocity(degrees)(m/s)(m/s)45Parabola1 2.981 4.23245Parabola2 1.639 3.30745Circle0.129 2.572By modeling this further into a projectile motion problem we can verify that the function yielding the greatest velocity in the z and y direction will give the greatest vertical air.So, by comparing and contrasting the three different models we can extrapolate that:•Parabola2is44percent better than parabola1for maximizing vertical air.•Parabola1is62percent better than the half circle for maximizing vertical air.3.4SolutionsMaximized Vertical Air Halfpipe•In the conservation of energy model we found that vertical air is maximized whenσis closest to90degrees.Team Control Number12149Page12of23•In the two dimensional force model the point of interest for velocity is at the top of the vertical where the snowboarder will achieve vertical air granted that enough speed is achieved.By modeling three different functions(half pipe slopes),each at three different z-slopes(mountain slope)we determined that for optimal vertical air to be reached,a parabola of the formy=.07x2−7(14) gives the greatest vertical air.•In the comprehensive force model we look at the top of the vertical for the velocities to be evaluated tofind that the mountain should have a slope of45degrees and indeed it is the parabola of the form of equation14that yields the greatest vertical air.Something to think about is that this is what the model tells us is the best shape but what the model does not take into account is that a snowboarder has various techniques to convert velocities in one direction to another such as carving and toe turns.A more shallow slope is needed for a generalized halfpipe.Best Practical HalfpipeWhen applying this model to a more realistic situation where a snowboarder converts most of the x-velocity(downward slope speed)into side to side velocity which can also be used for vertical air we might revaluate this conclusion.Taking into mind the fact that an even distribution of velocities with a max in the z direction is important we can look at the data listed in the appendix and see that it is in fact the same parabola(equation14)with a different mountain’s slope of25degrees that gives the best realistic shape for a half pipe that gives the best vertical air.4Testing Methods•Physical testing for the two and three dimensional force models.•Computer simulation with known values for the two and three dimensional force mod-els.•Analytical testing for the conservation of energy model.4.1Physical TestingFor the three dimensional force model we were able to set up a physical model and send a marble down the halfpipe to verify that our computer simulations were modeling the system correctly(figure7).We found that the path the marble followed was almost exactly mirrored the three dimensional graph of the snowboarder’s displacement(figure8).Team Control Number12149Page13of23Figure7:Physical Model TestingFigure8:3Dimensional Graph of Snowboarder’s Displacement4.2Computer Program Stability•In our two dimensional model,we were able to test its stability by changing the pa-rameters controlling it in order to obtain results we knew we should get given the conditions previously set.In our two dimensional model we tested it by:1.Taking away the frictional force and drag force applied to the snowboard andsnowboarder and checking to make sure that snowboarder continues perpetuallyin a pendulum like motion.Team Control Number12149Page14of232.By not adding work and checking that the snowboarder will continue with adamped motion,untilfinally coming to rest.By checking these two conditions,we were able to ensure that our two dimensional model successfully simulated a snowboarder limited to movement in2dimensions.•In our three dimensional model,we were able to test the stability in a similar way as was done for the two dimensional model.The stability tests that were run on the three dimension model were:1.Taking away the frictional force and drag force applied to the snowboard andsnowboarder,as well as settingφequal to0,and checking to make sure that ourthree dimensional model gave the same results as did our two dimensional modelunder the same conditions.2.By Settingφequal to0and making sure that our model gives the gives the sameresults as our two dimensional model.3.By not adding work and checking that the snowboarder will continue with adamped motion,untilfinally coming to rest.4.By running our full three dimensional model,and comparing it to the two di-mensional model using the same half-pipe shape,and checking that our threedimensional model gave a higher y velocity as greater Vertical Air,due to addedforce in the y and z directions due to the added x velocity.With this these methods for checking the stability of our three dimensional model,we were able to make sure that our transition from two dimensions to three,was consistent with the physics behind our model.Analytical TestingThe method for testing the conservation of energy model was an intuitive approach to the laws of conservation.Taking into mind the fact that the snowboarder only has as much energy as he starts with when no work is added we could see he would only be able to reach his original height.When adding in work we could verify that the only way that a snowboarder could reach any vertical height above the edge of the halfpipe would be if the snowboarder used pumping to give an additional force to the system.4.3Error AnalysisFor our two dimensional model,we computed the error in position due to the Runge Kutta approximation of our ODEs to be0.1%with a delta t of0.00001iterated for30 seconds.For our three dimensional model,we computed our error in the x,y,z direction to be0.1%error due to the factors mentioned above.We found that with the Runge Kutta approximation program we ran our simulations on,it is possible to achieve less than0.1%Team Control Number12149Page15of23 error by reducing our delta t;however for the given project0.1%error is a very acceptable percentage.5Future WorkA possible future model could be a half-pipe down the slope given by the equation of a cycloid.A cycloid is what Bernoulli found to be as the line of fastest line of descent.So,if the average velocity is maximized in the zx direction while holding basic half pipe function constant could potentially increase kinetic energy and maximize vertical air.Realistically this would not necessarily be the best model as the slope of a mountain is rarely in the cycloid equation form.Further consideration with respect to our model would be further testing with piecewise function as well as adding different types of work.Our model allowed work to be added in one direction.Also,finding some way to take into account the stylistic choices of a skilled snowboarder would be another factor we might add in.Team Control Number12149Page16of23 6Appendix/***************************************************************//*Runge Kutta for a set of first order differential equations*//***************************************************************/#include<stdio.h>#include<math.h>#include<iostream>using namespace std;/*************************************//*Parameters you may want to change*//*************************************/#define N6/*number of first order equations*/#define DELTA_T0.00001/*stepsize in t*/#define T_MAX30.0/*max for t*/#define INITIAL_Y00.0/*y*/#define INITIAL_Y20.0/*velocity y*/#define INITIAL_Y30.0/*velocity in z*/#define INITIAL_Y40.0/*possition in x*/#define INITIAL_Y50.0/*velocity in x*/ #define m75.0#define g9.80665#define mu0.04#define w6000.0#define hi3000.0#define rho_air1.225#define area0.87#define c_d0.7#define PI3.1415926#define phi15*PI/180/******************//*Define the ODE*//******************/double f(double x,double y[],int i){double theta,fdrag,n,rho,dzdy,dz2dy,fdragx,fy,fx,vx,vy,z;if(y[0]<=(20/3)){dzdy=0.5*y[0]-(10/3);dz2dy=0.5;}if(y[0]<=(40/3)){Team Control Number12149Page17of23dz2dy=0;}else{dzdy=0.5*y[0]-(20/3);dzdy=0.5;}theta=-atan(dzdy);fdrag=.5*rho_air*area*c_d*(y[2]*y[2]+y[3]*y[3])/m;fdragx=.5*rho_air*area*c_d*(y[5]*y[5])/m;rho=-sqrt((1+dzdy*dzdy)*(1+dzdy*dzdy)*(1+dzdy*dzdy))/dz2dy;n=g*cos(phi)*cos(theta)-(y[2]*y[2]+y[3]*y[3])/rho;if(y[0]==0){fx=(n*sin(theta))-(mu*n*cos(theta))-(fdrag*cos(theta));fy=-g*cos(phi)+(n*cos(theta))+(mu*n*sin(theta))+(fdrag*sin(theta));}else if((y[2]<0)){fy=-g*cos(phi)+(n*cos(theta))-(mu*n*sin(theta))-(fdrag*sin(theta));fx=(n*sin(theta))+(mu*n*cos(theta))+(fdrag*cos(theta));}//else if((y[2]<0)&(y[3]>0)&(0<=theta)&(theta<=PI/2))//{//fy=-g*cos(phi)+(n*cos(theta))+(mu*n*sin(theta))+(fdrag*sin(theta));//fx=(n*sin(theta))-(mu*n*cos(theta))-(fdrag*cos(theta)); //}else{fx=(n*sin(theta))-(mu*n*cos(theta))-(fdrag*cos(theta));fy=-g*cos(phi)+(n*cos(theta))+(mu*n*sin(theta))+(fdrag*sin(theta));}if(i==0)return(y[2]);if(i==1)return(y[3]);if(i==2)return(fx);if(i==3)return(fy);if(i==4)return(y[5]);if(i==5)return(g*sin(phi)-g*cos(phi)*mu-fdragx);cout<<fx;Team Control Number12149Page18of23}int main(void){double t,y[N],bob,count;int j,k;void runge4(double x,double y[],double step);/*Runge-Kutta function*/ y[0]=INITIAL_Y0;/*initial Y[0]*/if(y[0]<=(20/3)){y[1]=(1/4)*((y[0]-(20/3))*(y[0]-(20/3)));}else if(y[0]<=(40/3)){y[1]=0;}else{y[1]=(1/4)*((y[0]-(40/3))*(y[0]-(40/3)));}y[2]=INITIAL_Y2;y[3]=0;/*initial Y[1]*/count=-1;printf("%lf\t%lf\t%lf\n",INITIAL_Y0,y[1],y[5]);/*************//*Time Loop*//*************/for(t=0;y[4]<=175;t+=DELTA_T){runge4(t,y,DELTA_T);count++;if(y[0]<=(20/3)){。
2020美赛o奖论文使用模型
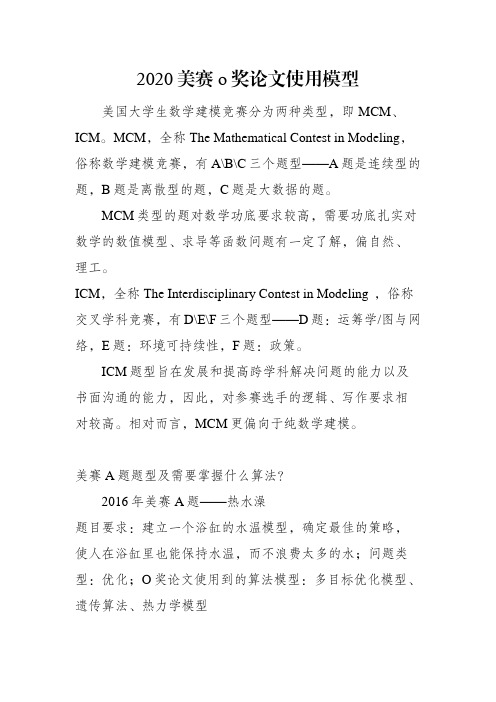
2020美赛o奖论文使用模型美国大学生数学建模竞赛分为两种类型,即MCM、ICM。
MCM,全称The Mathematical Contest in Modeling,俗称数学建模竞赛,有A\B\C三个题型——A题是连续型的题,B题是离散型的题,C题是大数据的题。
MCM类型的题对数学功底要求较高,需要功底扎实对数学的数值模型、求导等函数问题有一定了解,偏自然、理工。
ICM,全称The Interdisciplinary Contest in Modeling ,俗称交叉学科竞赛,有D\E\F三个题型——D题:运筹学/图与网络,E题:环境可持续性,F题:政策。
ICM题型旨在发展和提高跨学科解决问题的能力以及书面沟通的能力,因此,对参赛选手的逻辑、写作要求相对较高。
相对而言,MCM更偏向于纯数学建模。
美赛A题题型及需要掌握什么算法?2016年美赛A题——热水澡题目要求:建立一个浴缸的水温模型,确定最佳的策略,使人在浴缸里也能保持水温,而不浪费太多的水;问题类型:优化;O奖论文使用到的算法模型:多目标优化模型、遗传算法、热力学模型2017年美赛A题——管理赞比西河题目要求:评估修复、重建、拆除Kariba水坝潜在成本和收益、支持关于修建赞比西河新坝的数量和位置的建议;问题类型:评估预测;O奖论文使用到的算法模型:成本效益预测模型、k-NN、随机森林2018年美赛A题——高频无线电传播题目要求:为海洋信号反射建立一个数学模型;问题类型:评价决策;O奖论文使用到的算法模型:船舶摇摆模型、菲涅耳反射系数方程2019年美赛A题——权利的游戏题目要求:分析龙的特征,行为,习惯,饮食以及与环境的相互影响;问题类型:评价;O奖论文使用到的算法模型:渐近S形曲线,von Bertalanffy方程、n-种群元种群模型、增长模型2020年美赛A题——向北移动题目要求:建立一个数学模型,以识别未来50年内这两种鱼类最可能的位置,根据您的预测分析,这些小型捕捞公司是否应该改变其经营方式;问题类型:评估预测;O奖论文使用到的算法模型:时间序列分析法、K-Means聚类算法、线性预测、模糊综合评价法。
- 1、下载文档前请自行甄别文档内容的完整性,平台不提供额外的编辑、内容补充、找答案等附加服务。
- 2、"仅部分预览"的文档,不可在线预览部分如存在完整性等问题,可反馈申请退款(可完整预览的文档不适用该条件!)。
- 3、如文档侵犯您的权益,请联系客服反馈,我们会尽快为您处理(人工客服工作时间:9:00-18:30)。
For office use onlyT1________________ T2________________ T3________________ T4________________Team Control Number55069Problem ChosenAFor office use onlyF1________________F2________________F3________________F4________________ 2017 MCM/ICM Summary SheetThe Rehabilitation of the Kariba Dam Recently, the Institute of Risk Management of South Africa has just warned that the Kariba dam is in desperate need of rehabilitation, otherwise the whole dam would collapse, putting 3.5 million people at risk. Aimed to look for the best strategy with the three options listed to maintain the dam, we employ AHP model to filter factors and determine two most influential criteria, including potential costs and benefits. With the weight of each criterion worked out, our model demonstrates that option 3is the optimal choice.According to our choice, we are required to offer the recommendation as to the number and placement of the new dams. Regarding it as a set covering problem, we develop a multi-objective optimization model to minimize the number of smaller dams while improving the water resources management capacity. Applying TOPSIS evaluation method to get the demand of the electricity and water, we solve this problem with genetic algorithm and get an approximate optimal solution with 12 smaller dams and determine the location of them.Taking the strategy for modulating the water flow into account, we construct a joint operation of dam system to simulate the relationship among the smaller dams with genetic algorithm approach. We define four kinds of year based on the Kariba’s climate data of climate, namely, normal flow year, low flow year, high flow year and differential year. Finally, these statistics could help us simulate the water flow of each month in one year, then we obtain the water resources planning and modulating strategy.The sensitivity analysis of our model has pointed out that small alteration in our constraints (including removing an important city of the countries and changing the measurement of the economic development index etc.) affects the location of some of our dams slightly while the number of dams remains the same. Also we find that the output coefficient is not an important factor for joint operation of the dam system, for the reason that the discharge index and the capacity index would not change a lot with the output coefficient changing.Contents1Overview (1)1.1Background (1)1.2 Restatement of the Problem (1)1.3 Literature Review (2)2Assumptions and Justifications (2)3Notation (3)4Model Overview (4)5Model Theory (5)5.1Determination of the Number and Location of the Dams (5)5.2 Joint operation of dam system model (9)6Model Implementation and Results (11)6.1The Number and Location (11)6.2The Strategy of Modulating Water Flow (14)7 Sensitivity Analysis (15)7.1 The Model of Determination of the Number and Location (15)7.2 The Model of Modulating Water Flow (17)8 Further discussion (17)9 Strengths and Weaknesses (18)9.1 Strengths (18)9.2 Weaknesses (19)10 Conclusion (19)11 The Evaluation of Three Options (20)11.1 Establish a Hierarchical Model (20)11.2 Analysis and Results (21)1 Overview1.1 BackgroundA Zambezi River Authority conference was held in March 2014, engineers warned that the foundations of the dam had weakened and there was a possibility of dam failure unless repairs were made.On 3 October 2014 the BBC reported t hat “The Kariba Dam is in a dangerous state. Opened in 1959, it was built on a seemingly solid bed of basalt. However, in the past 50 years, the torrents from the spillway have eroded that bedrock, carving a vast crater that has undercut the dam’s foundations, engineers are now warning that without urgent repairs, the whole dam will collapse. If that happened, a tsunami-like wall of water would rip through the Zambezi valley, reaching the Mozambique border within eight hours. The torrent would overwhelm Moz ambique’s Cahora Bassa Dam and knock out 40% of southern Africa’s hydroelectric capacity. Along with the devastation of wildlife in the valley, the Zambezi River Authority estimates that the live of 3.5 million people are at risk.”On February 2015, Engineers have started on a R3.3bn rescue marathon to prevent the “catastrophic failure” of the Kariba Dam. According to a World Bank special repor t on the beleaguered structure-one of the biggest man-made dams in the world-a potential wall collapse threatens the lives of about 3-million people living on the Zambezi River floodplain between the hydro scheme on the Zambia-Zimbabwe border and the Mozambique coast. [1]1.2 Restatement of the ProblemWe are required to provide an overview of potential costs and benefits with the three options already listed. Then we need to establish a model to determine the number and placement of the new dams when removing the Kariba dam along the Zambezi River. The same overall water management capabilities are also needed. In addition, we should consider emergency water flow situations and restrictions regarding the locations and time, so that we could give out the strategy for modulating the water flow through our new multiple dam system.In order to solve those problems, we will proceed as follows:●Build a model to determine the number and location of the multiple dams.●Give the corresponding strategy of modulating water flow in different conditions.In our model, we first establish a multi-objective model and use genetic algorithm determine the number and location of the multiple dams. There are two goals improving the water resources management capacity and reducing the cost. Besides, we add some constraints such as water balance, water level, safety and water protection. We choose twenty suitable dam sites and employ the genetic algorithm to solve the optimal problem to determine the number and the location.After determining the number and location of the dams, we construct our joint operation of damsystem model and employ the genetic algorithm to solve the problem based on the thought of dynamic programming. According to the Kariba’s climate data for about 30 years, we abstract normal flow year, low flow year, high flow year and differential year. We use them to work out the water resources planning and scheduling strategy. The construction of the discharge index and the capacity index benefits an analysis and evaluation for joint operation of the dam system’s performance in different month and year.1.3 Literature ReviewDating back to 2004, the United States removed 72 dams in total, which created a historical record. Therefore, it is high time for us to focus on the construction of dams concerning their number and placement. Plenty of researchers have already made a number of notable papers to address these problemsAlfer Weber (1909) first proposed a framework for location problem, which is an allocation question with respect to space resource. Among the three classical location model, set covering problem is a significant branch of siting issues. They explored a multi-objective location model to tackle problems with siting optimal points. In their model, maximizing coverage rate in order to satis fy every place’s need is the target function, the concentric point and the capacity restrictions are constraint conditions. Thus, they could convert the optimization problem to the mixed integer linear programming question.After the set covering model was established, we can optimize our choice of siting the dams. Then several scientists were devoted to building an optimal operation model to provide a reasonable balance between safety and balance. They begin to figure out how the multiple dam system would benefit or affect each other within its system. Masse(1940) first illustrated the concept of it; their main computing method was to optimize water modulating strategy during dispatching period.Further studies are carried out about different methods to investigate the optimal operation model, including dynamic programming algorithm and neural network algorithm based on improved computer technique. Also, there is much theoretical analysis about location problem since 1990. John Current and Morton O'Kelly(1992) suggested using a modified version of the set covering location model, which still didn’t take the reality into account.2 Assumptions and JustificationsTo simplify our problems, we make the following basic assumptions, each of which is properly justified.●The dam system is built downstream in the valley of the Kariba Dam. Because it’smore convenient to build and also with less cost, which can be easily implemented.●The cost of the dam is mostly the same. Owing to the fact that the length of the canyonis not large(24km),geological conditions and climate conditions are mostly the same.● Each dam’s water supply is mostly the same. Taking into account of safe operation ofthe entire multi-dam system, we should make the burden of each dam to be the same as much as possible.● The water quality of the dam system is the average of the water quality between thetwo reservoirs. The river is flowing, so the water quality is mostly similar.● Water of the dams downstream only comes from dams upstream and naturalprecipitation. According to Google Maps, there are no tributaries near the canyon. Also with the principle of conservation of water, the formula should be maintained.3 NotationAbbreviation Description{}12,,m Y y y y = The set of cities{}12=,,n X x x x The set of dams(,)i n d y X The distance from the th i city to the nearest smaller dami Ele The electricity demand of th i cityi Wat The water demand of th i city(,)W t i The discharge amount of the th i dam at the end of period t,(,)Z t i The total amount of water released during period t(,)T t i The amount of natural water in period tij V The volume in the th j period of the th i damij Q The inflow in the th j period of the th i damij q The discharge volume in the th j period of the th i damij QJ The runoff volume between the th i dam and the (1)th i -damij S The actual water supply th i dam in the th j periodij D The planned water supply of the th i dam in the th j periodij H The hydraulic head of th i dam in the th j periodi K The fore-voltage factor of the th i damt λ The discharge flow indicators in the th t periodt μ The storage capacity indicators in the th t period4 Model OverviewTo provide a detailed analysis of the option (3)-removing the Kariba dam and newly building ten to twenty smaller dams. We need to determine the number and location of the multiple dams first. And on that basis we must establish a model to modulate the water flow through the multiple dam system to adapt to different situations. A reasonable balance between safety and cost of our strategy is also needed.Our first model allows us to determine the number and the location of the multiple dams. We regard the optimal problem as a set covering problem and establish a multi-objective model to solve the problem. There are two goals, namely improving water resources management capacity and reducing the cost. And there are also some constraints including water balance, water level, safety, water resources protection and number constraints. On the account that the optimal problem is difficult to solve in polynomial time, so we use genetic algorithm to get the solution.After determining the numbers and the location, we establish a joint operation of dam system model to gain a strategy about modulating water flow in different condition. Though it’s also a multi-objective problem, it is different from the previous model. We use the maximization of economic and social benefits as the objective and set some constraints, such as water balance, reservoir capacity and discharge flow constraints. We use genetic algorithm to get themodulating strategy in different conditions.In conclusion, we use programming and heuristic algorithm to solve the problem of building dams and the modulating strategy. It’s relatively easy to achieve and it has a significant guidance for the reality.5 Model Theory5.1 Determination of the Number and Location of the Dams5.1.1 Establishment of the modelThe construction of dams needs to consider many aspects, while at the same time it is subject to economic, social, environmental constraints and other factors. In order to obtain the proper number of dams and their location, we establish a multi-objective model.The Objective● Improve Water Resources Management CapacityThe purpose of building smaller dams instead of larger dams is to manage water resources better, mainly to satisfy dweller’s demand for the electricity consumption and water consumption (Including agricultural, industrial and domestic water) of the neighboring cities. Demand may vary between cities, but it is clear that for cities with greater demand, the dam should be built closer to them. so we get that1min (,)mi i n i Ele d y X =∑1min (,)mi i n i Wat d y X =∑● Reduce the CostOn the basis of ensuring the water supply and power supply, we should minimize the cost of our plan. The whole cost consists of the removal of the Kariba dam and building new smaller dams. Since the cost of removal is fixed, so we only consider the variable cost(building cost), which is only related to the number of dams. So we should minimize the quantity of the smaller dams.min nThe Constraints● Water Balance()(,)(1,)(,)(,)k i W t i W t i Z t k T t i π∈=-++∑(,)W t i ,(,)Z t i and (,)T t i represent the discharge amount of the th i dam at the end of periodt, the total amount of water released during period t and the amount of natural water in period t respectively.()i π denotes the set of all higher dams of th i dam. If ()i π is empty, the corresponding summation is zero.[2]● Water LevelThe water level in the dam area should be kept between the dead water level and the limited water level in the flood season. Dead Water Level, namely the lowest water level that allows a reservoir to fall off under normal operating conditions. Flood limited water level is the requirements of control over flood to limit reservoirs’ water storage.DWL WL FWL ≤≤● SafetyThe construction of multiple smaller dams is at least as safe as the original larger dams. The safety considerations for dam construction mainly include reducing the probability of dam failure, thereby reducing damage to dams downstream and enhancing resistance ability to extreme weather.sd Bd Safety Safety ≥sd Safety represents the safety of multiple dams system, while Bd Safety denotes the safety ofthe existing Kariba dam.● Water Resources ProtectionThe construction of smaller dams should require a higher degree of protection for the water resources as the replacement of the existing dam.sd Bd WRP WRP ≥sd WRP represents the environment protection of the multiple dams system, while Bd WRP denotes the environment protection of the existing Kariba dam.● NumberThe number of small dams should be greater than ten and less than twenty. Option 3 is replacing the Kariba dam with a series of ten to twenty smaller dams.To ensure the continuity of the model establishment, the parameters of the constraints will be described in the next part. And in the sixth section, we will clarify how to calculate the demand of electricity(i Ele ),the demand of the water(i Wat ),the safety of different dams and theenvironment protection of different dams.To sum up, we model the problem about smaller dams’ number and location decision with multi-objective optimization. The formulas of this model is1min (,)mi i n i Ele d y X =∑1min (,)m i i n i Wat d y X =∑min n..S t ()(,)(1,)(,)(,)k i W t i W t i Z t k T t i π∈=-++∑ DWL WL FWL ≤≤sd Bd Safety Safety ≥sd Bd WRP WRP ≥1020n ≤≤(n=10, 11, 12…..20)5.1.2 The Parameter of the Constraints● The Demand of ElectricityThe power demand of a city is mainly related to its economic and demographic factors. Our paper uses the city's latest available GDP data as the representative of its economic factors, and the total urban population as the representative of its demographic factor.The GDP of the th i city is i GDP .The population of the th i city is i pop .● The Demand of WaterThe water consumption of residents is mainly affected by residents' income, water price and other factors. Since within a country, the price difference is relatively small, so here we ignore the price difference.. Therefore, the water consumption of urban residents is mainly affected by the income of residents, We use the city's GNI to represent its urban residents. The GNI of the th i city is i GNI .● The Safety of Dams and the Kariba Dam [3]For the safety of dam construction, our paper mainly consider the index of risk loss as the agent of its safety indicators.For a single Big dam, its Risk Loss Index(RLI) Bd R equals to P multiply N . P is the failure probability of the dam beyond the current code standard. For example, the current code specifies that if a dam's seismic safety standard is 50 years beyond 10%, the probability offailure exceeding the current code specification is 0.1 / 50 = 0.002.N is the life loss of dam failure (we can convert economic loss into life loss in a certain percentage). We use the Dekay & McClelland method to calculate the loss. Thus, the RLI of the Kariba dam is()()Bd R P K N K =⋅.For multiple dam system, the RLI of the th j dam is1()()(|)()()nj l j R P j N j P l j P j N l =+=⋅+⋅⋅∑and the RLI of the multiple dam system is 1nsdsdj j R R ==∑ The safety of the multiple dam system should be greater than or at least equal to the safety of the existing Kariba dam. So we get that sd BdR R ≤, that is to say 1()()nsd i i R P K N K =≤⋅∑.And n is an integer greater than ten and less than twenty● The water resources protection of damsAccording to the International Commission On Large Dams(ICOLD), there are three goals of water resources management [4]✓ Improved management of the water supply✓ Improved water quality in our rivers✓ Improved environmental conditions in the watershedSince there are few branches in the Kariba Gorge, we mainly consider the management of the water supply and the water quality.According to the constraints, the water supply of the multiple-dam system should be greater than the big dam. That is to saysd Bd WS WS ≥sd WS equals to the number of the smaller dams multiply the water supply of a smaller dam,while Bd WS represents the water supply of the Kariba Dam.Besides, the water quality of the multiple-dam system should be greater than the Kariba dam.sd Bd WQ WQ ≥,sd WQ represents the expected average water quality of the Lake Kariba if the authority adoptthe option three, while Bd WQ represents the present water quality of the Lake Kariba.5.2 Joint Operation of Dam System ModelJoint operation of dam system can utilize the differences between dam capacity and hydrologic condition, so that we develop a joint compensation effect for the dam group and make full use of water resources. Compared with the traditional single large dam, the dam group establish on the basis of multi –dam system, which could help us take advantage of water resources for our social benefits, including economic and social benefits. Therefore, it is necessary to establish an effective water resources dispatching scheme.In order to better express our model ,we let 1...i n =indicates the number of dams , 1...j T =denotes the cycle of water dispatching ,ij V illustrates the th i dam ’s water storage during theth j period.[5]The ObjectiveThe hydropower station mainly bring about economic and social benefits. But obviously, hydropower station can bring magnificent economic benefits. Among them, the most direct indication is the dam power generation. Therefore, taking the reservoir power generation as the revelation of economic benefit.11***n Te i ij ij i j I K q H t ===∆∑∑ij q is the discharge flow of the th i dam in the th j period ,ij H is the hydraulic head of th i dam in the th j period ,i K is the force-voltage factor of the th i dam ,t ∆is the time period.In addition to creating enormous economic benefits, a large dam can also bring huge social benefits. Through the construction of dams, it can meet the need of a large number of industrial and agricultural water, also the domestic water.11()n Ts ij ij i i I S D ===-∑∑ij S is the actual water supply th i dam in the th t stage ,ij D is water demand or planned water supply of the th i dam in the th t stage.Therefore, we should make full use of water resources so as to make the economic and social benefits as large as possible.[6]max()e s I I I =+The Constraints● Water Balance,(1)()i t it it it it V V Q q t L +=+-⋅∆-,(1)(1),i t i t it Q q QJ +-=+it Q 、ij q are the average inflow into the dam and discharge volume in the th t period of the th i dam,it L is the evaporation and leakage loss.it QJ is the runoff volume between the th i dam and the th j dam.● Capacity of the DamTo prevent floods and droughts ,The capacity of each dam must be limited.min max i it i V V V ≤≤min i V 、max i V are the upper and lower limit of the th i dam’s water storage ,namely the dead capacity and the flood control capacity.● Discharge Flow ConstraintIn order to keep the normal life standard and to maintain the downstream ecological water use, we must ensure the certain discharge of water, we employ min i q to indicate the minimal discharge of water. Because of the limitation of the dam itself and the purpose of flood control, there need to be a maximal discharge capacity constraint max i qm max i it i q in q q ≤≤In conclusion, in order to make economic benefit and social benefit as large as possible while satisfying the constraint conditions, we need to develop water resources optimized dispatching scheme.1111max ***()n T n Ti ij ij ij ij i j i i I K q H t S D =====∆+-∑∑∑∑,(1),(1)(1),()*..min max m max i t it it it iti t i t it i it i i it i V V Q q t L Q q QJ s t V V V q in q q ++-=+-∆-⎧⎪=+⎪⎨≤≤⎪⎪≤≤⎩it V 、it q are the interval-type variable ,min i V 、min i q correspond to meet the basic industrial and agricultural water 、domestic water and ecological water ,they are the dead water level. For safety considerations such as flood control ,max i V 、max i q are the maximal water level. We let [,]i i Vf VF to be the of it V ,[,]i i qf qF is the optimum interval of it q .[7]1()/(min ),min 1,1()/(max ),max 0,i it i i i it ii it i it it i i i i it iVf V Vf V V V Vf Vf V VF V VF V VF VF V V else λ---≤≤⎧⎪≤≤⎪=⎨---≤≤⎪⎪⎩ 11N t it i N λλ==∑ 1()/(min ),min 1,1()/(max ),max 0,i it i i i it ii it i it it i i i i it i qf q qf q q q qf qf q qF q qF q qF qF q q else μ---≤≤⎧⎪≤≤⎪=⎨---≤≤⎪⎪⎩ 11N t it i N μμ==∑ 6 Model Implementation and Results6.1 The Number and LocationMeasuring the demand of the nearby cityTOPSIS (Technique for Order Preference by Similarity to an Ideal Solution) is a commonly used and effective method in multi-objective decision analysis. Hence ,we employ this model and then investigate extended TOPSIS to evaluate the demand of electricity and water of each city.Then we use the data to calculate the demand of the electricity and water with TOPSIS, and part of the results is shown in Table 1.Table 1 The Demand of Electricity and WaterCalculate the shortest distance from each dam to each cityThe dam should be built in the valley of the river fully, because the project is small with low cost. Moreover, the construction of small dams is mainly to replace the larger dam, so the small dam should be constructed mainly in the downstream of the Kariba dam in the canyon. Figure 1 is the suitable site for building dams.Figure 1 The Suitable Gorge for Building DamsWith respect to the option 3 that we need to construct 10 to 20 small dams, considering the geological factors around the dam, we first select the suitable location of the 20 dams.According to our model, we use the distance from the dam to the center of the city as a representative of the distance to the city. A total of 25 cities are selected close to the Kariba gorge, including 15 cities in Zambia, 10 cities in Zimbabwe. We search and calculate the shortest distance and path of each dam to the city through Google maps, and the results of some small dams to the cities are shown in Table 2.Table 2 The Distance Between the Dams and the City(Part)● The Data of Other FactorsAt the same time, we collect the data of other related factors through the Zambia National Bureau of Statistics, the World Bank, the World Commission on Dams and other institutions to gain the relevant statistics.Table 3 The Data of Other FactorsDWL NWL FWL BFWL RLI WS WQ Kariba 475.5 484.6 490 476.7 9000 608.5 19 Multiple 382 408 412 385 1n sd i i R=∑ e WS n ⋅ e WQUnder normal circumstances the water level should be higher than the dead water level, and lower than the normal position. But before the flood season, the water level is lower than the limit of water level.● The numbers and the locationThis optimal problem belongs to the NP-hard problem. We can’t solve it in polynomial time. So we can only use heuristic algorithms such as artificial neural networks, genetic algorithm, simulated annealing to obtain the approximate solution. In this paper, we select the genetic algorithm to solve the problem.We run the program repeatedly, and finally get a relatively good result and determine our number and location of the dams. There should be 12 smaller dams in the Kariba Gorge. Besides, we select the optimal 12 dam location to maximize our objective function from 20 appropriatedam sites. The sixth and twelfth site locations are listed in the Table 4Table 4 The Location of the DamAt the same time, we will also mark the location of the small dam on the map, we put the location of the sixth and twelfth dam on the map. We can see it on the Figure 2 andFigure 3Figure 2 The Location of the 6th Dam Figure 3 The Location of the 12th Dam 6.2 The Strategy of Modulating Water FlowTraditional optimization algorithm mostly belongs to the convex optimization category. It demands a unique global optimal solution, which is often used in practical applications. A number of heuristic intelligent algorithm, for example genetic algorithm etc.These methods show that the algorithm can work out satisfactory results for solving the optimization problem. Employing joint operation of dam system model, we use genetic algorithm and Matlab2014 for the solution.[8]By running the genetic algorithm and adjusting the parameters, we obtain a relatively satisfactory result, and then develop a joint operation of dam system model.(The picture of the results is shown in the appendix part four.)Normal Flow Year:Take the climate and hydrological conditions of the average of nearly5 years to simulate normal climatic conditions.In normal years, the water resources dispatching of the dam system shows obvious seasonal difference. In the dry season from June to September, the discharge of each reservoir reaches the bottom line. Compared with the middle and lower reaches of the river, upper and middlereaches of water resources and reserves are relatively abundant, therefore, still a lot of water could be scheduled downstream. But until the rainy season from November to March, the precipitation intensity and the discharge of each dam are very large. The results show that the discharge index and storage capacity index perform better in May and October, which are in the optimum range, while the other month are all above 0.7, this is a satisfactory result.●Low Flow Year: The monthly precipitation is taken as the highest value in the past 30years, simulating the more serious flood threat..When the annual precipitation decreases, each dam discharge is significantly lower, but the discharge index and the capacity index is still above 0.7, which reflects the joint operation of dam system still has obvious effect to resist abnormal weather like the drought condition.●High Flow Year:The monthly precipitation is taken as the highest value inthe past 30years, simulating the more serious flood threat.When the annual precipitation has increased significantly, then the reservoir’s discharge would increase. While the discharge of the rainy season increases a lot, but in dry season the increase range is smaller, which makes the discharge capacity index and volume index increase significantly from May to September. Although the index decreases significantly in November and February the figure are more than 0.7, is still relatively satisfactory.●Differentiation Year:The precipitation from May to September is taken as the lowestvalue in the past 30 years, the precipitation from October to April was taken as the highest value in the past 30 years. So that we could simulate drought, flood significantly differentiated condition..When confronted with the differentiation year where there comes rainy season when the precipitation increases and the dry season when the precipitation decreases all come about in one year. The discharge flow of each reservoir expands seasonally, which makes the discharge index and storage capacity index of each month decrease, and it is more obvious in the rainy season. Which can not be ignored is that the discharge flow indicators and storage capacity indicators remained at 0.7 or more.[9]7. Sensitivity Analysis7.1 The Model of Determination of the Number and Location●Remove an important city of both countriesIn the questions we discussed above,we have selected 15 cities in Zambia,10 cities in Zimbabwe to specify the number and placement of the newly built smaller dams. Among the sensitivity analysis, we remove important cities of the two countries, thus, we could see whether this movement would influence the number and placement of constructing dams.In our analysis, we leave out Lusaka (The capital city of Zambia) and Harare(The capital city of Zimbabwe) from our original cities, yet, we still use the multi objective optimization model and genetic algorithm we already established to calculate the number and placement of smaller dams. We discover that the optimal number of constructing dams is also 12, but the location of each dam is slightly changed. Just the fourth and the eleventh dam’s place changes a lot, while other dam’s place just changes a little. Therefore, we list the location of the fourth and the eleventh dam’s site in Table 5.。