语音信号处理中英文翻译
语音信号处理文献翻译
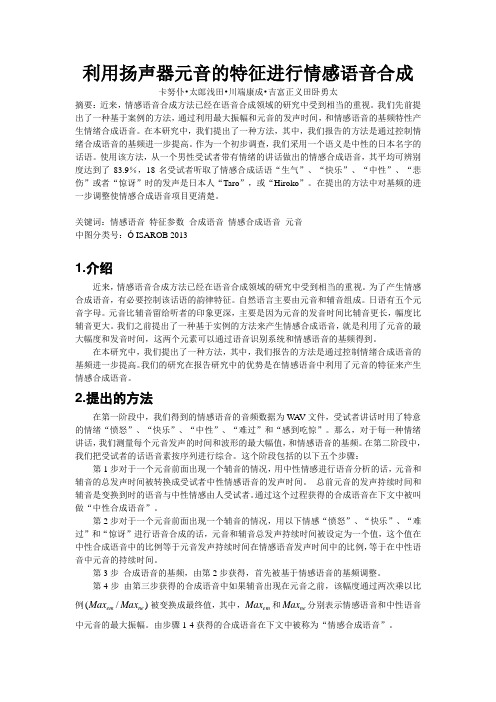
利用扬声器元音的特征进行情感语音合成卡努仆•太郎浅田•川端康成•吉富正义田卧勇太摘要:近来,情感语音合成方法已经在语音合成领域的研究中受到相当的重视。
我们先前提出了一种基于案例的方法,通过利用最大振幅和元音的发声时间,和情感语音的基频特性产生情绪合成语音。
在本研究中,我们提出了一种方法,其中,我们报告的方法是通过控制情绪合成语音的基频进一步提高。
作为一个初步调查,我们采用一个语义是中性的日本名字的话语。
使用该方法,从一个男性受试者带有情绪的讲话做出的情感合成语音,其平均可辨别度达到了83.9%,18名受试者听取了情感合成话语“生气”、“快乐”、“中性”、“悲伤”或者“惊讶”时的发声是日本人“Taro ”,或“Hiroko ”。
在提出的方法中对基频的进一步调整使情感合成语音项目更清楚。
关键词:情感语音 特征参数 合成语音 情感合成语音 元音中图分类号:Ó ISAROB 20131.介绍近来,情感语音合成方法已经在语音合成领域的研究中受到相当的重视。
为了产生情感合成语音,有必要控制该话语的韵律特征。
自然语言主要由元音和辅音组成。
日语有五个元音字母。
元音比辅音留给听者的印象更深,主要是因为元音的发音时间比辅音更长,幅度比辅音更大。
我们之前提出了一种基于实例的方法来产生情感合成语音,就是利用了元音的最大幅度和发音时间,这两个元素可以通过语音识别系统和情感语音的基频得到。
在本研究中,我们提出了一种方法,其中,我们报告的方法是通过控制情绪合成语音的基频进一步提高。
我们的研究在报告研究中的优势是在情感语音中利用了元音的特征来产生情感合成语音。
2.提出的方法在第一阶段中,我们得到的情感语音的音频数据为WA V 文件,受试者讲话时用了特意的情绪“愤怒”、“快乐”、“中性”、“难过”和“感到吃惊”。
那么,对于每一种情绪讲话,我们测量每个元音发声的时间和波形的最大幅值,和情感语音的基频。
在第二阶段中,我们把受试者的话语音素按序列进行综合。
语音信号处理

1950
第一台语音识 别机器的诞生
动态规划在语音 识别中的应用
1960 语音产生的声 学理论 1970 LPC在语音识 在语音识 别中的应用
DTW算法的 算法的 出现
1980
非特定人大词 汇量连续语音 识别的成熟
1990
HMM在语音 在语音 识别中的应用
语音识别发展历史中的重要事件
未来的语音识别技术必须具备的特点: 未来的语音识别技术必须具备的特点:
现在假设平均速度是每秒十个音素, 现在假设平均速度是每秒十个音素,并忽略 相邻音素之间的相关性, 相邻音素之间的相关性,这样就可以估计得语音 60比特 的平均信息速度为60比特/ 的平均信息速度为60比特/s. 换句话说,在正常的讲话速度下, 换句话说,在正常的讲话速度下,与话音等 效的书面文字含有60bit/s的信息 当然, 60bit/s的信息。 效的书面文字含有60bit/s的信息。当然,语音 实际”信息的低限远高于这一速度, 的“实际”信息的低限远高于这一速度,这是因 为 在上面的估计中我们对很多音素末加考虑。例如 在上面的估计中我们对很多音素末加考虑。 说话人的个性和情绪, 说话人的个性和情绪,说话的速度和语音的强弱 等。
Speech Signal processing ---Principles and Practice
语音信号处理---原理与应用 原理与应用
基础理论 声学原理 语音编码 语音增强 语音识别
第一章 绪论
内容:介绍语音信号处理的意义、 内容:介绍语音信号处理的意义、基础理 论和算法、处理硬件和实用系统、 论和算法、处理硬件和实用系统、发展历 史及其应用的概况。 史及其应用的概况。 要求:了解语音信号处理技术的总体概况。 要求:了解语音信号处理技术的总体概况。
15_Speech Signal Processing(语音信号处理)
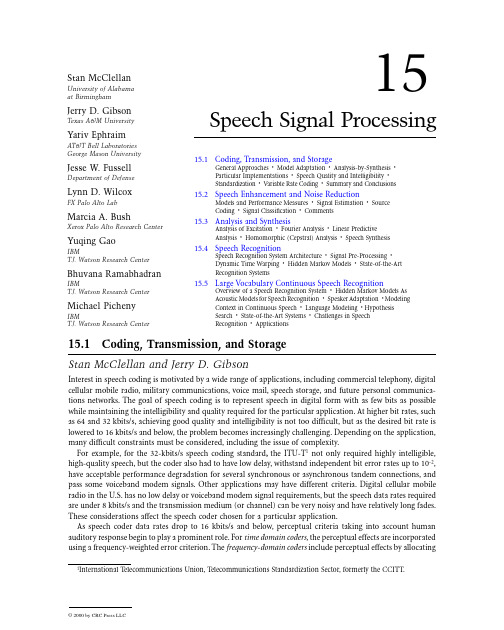
General Approaches
Time Domain Coders and Linear Prediction Linear Predictive Coding (LPC) is a modeling technique that has seen widespread application among timedomain speech coders, largely because it is computationally simple and applicable to the mechanisms involved in speech production. In LPC, general spectral characteristics are described by a parametric model based on estimates of autocorrelations or autocovariances. The model of choice for speech is the all-pole or autoregressive (AR) model. This model is particularly suited for voiced speech because the vocal tract can be well modeled by an all-pole transfer function. In this case, the estimated LPC model parameters correspond to an AR process which can produce waveforms very similar to the original speech segment. Differential Pulse Code Modulation (DPCM) coders (i.e., ITU-T G.721 ADPCM [CCITT, 1984]) and LPC vocoders (i.e., U.S. Federal Standard 1015 [National Communications System, 1984]) are examples of this class of time-domain predictive architecture. Code Excited Coders (i.e., ITU-T G728 [Chen, 1990] and U.S. Federal Standard 1016 [National Communications System, 1991]) also utilize LPC spectral modeling techniques.1 Based on the general spectral model, a predictive coder formulates an estimate of a future sample of speech based on a weighted combination of the immediately preceding samples. The error in this estimate (the prediction residual) typically comprises a significant portion of the data stream of the encoded speech. The residual contains information that is important in speech perception and cannot be modeled in a straightforward fashion. The most familiar form of predictive coder is the classical Differential Pulse Code Modulation (DPCM) system shown in Fig. 15.1. In DPCM, the predicted value at time instant k, ˆ s(k Έ k – 1), is subtracted from the input signal at time k, s(k), to produce the prediction error signal e(k). The prediction error is then approximated (quantized) and the quantized prediction error, eq(k), is coded (represented as a binary number) s(k Έ k – 1) to yield a for transmission to the receiver. Simultaneously with the coding, eq(k) is summed with ˆ s(k). Assuming no channel errors, an identical reconstruction, reconstructed version of the input sample, ˆ distorted only by the effects of quantization, is accomplished at the receiver. At both the transmitter and receiver, the predicted value at time instant k +1 is derived using reconstructed values up through time k, and the procedure is repeated. N ˆ (z) = 0 and Â(z) = The first DPCM systems had B a z -i , where {ai ,i = 1…N} are the LPC coefficients i =1 i –1 and z represents unit delay, so that the predicted value was a weighted linear combination of previous reconstructed valuesJ. Watson Research Center
混音常用英汉互译
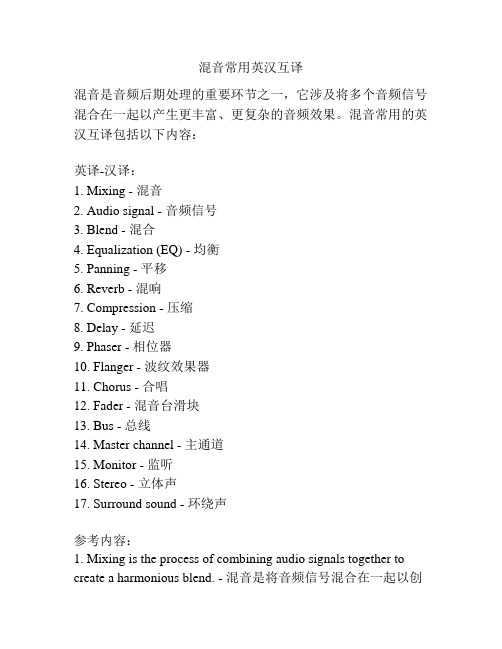
混音常用英汉互译混音是音频后期处理的重要环节之一,它涉及将多个音频信号混合在一起以产生更丰富、更复杂的音频效果。
混音常用的英汉互译包括以下内容:英译-汉译:1. Mixing - 混音2. Audio signal - 音频信号3. Blend - 混合4. Equalization (EQ) - 均衡5. Panning - 平移6. Reverb - 混响7. Compression - 压缩8. Delay - 延迟9. Phaser - 相位器10. Flanger - 波纹效果器11. Chorus - 合唱12. Fader - 混音台滑块13. Bus - 总线14. Master channel - 主通道15. Monitor - 监听16. Stereo - 立体声17. Surround sound - 环绕声参考内容:1. Mixing is the process of combining audio signals together to create a harmonious blend. - 混音是将音频信号混合在一起以创造和谐的混合声音的过程。
2. Equalization, or EQ, is a tool used in mixing to control the frequencies of audio signals. - 均衡是混音中用于控制音频信号频率的工具。
3. Panning refers to the technique of placing sounds in different positions within the stereo field. - 平移是指在立体声领域中将声音放置在不同位置的技术。
4. Reverb is an effect that simulates the natural reverberation of sound in different environments. - 混响是一种模拟不同环境下声音自然混响的效果。
201116910524苗云龙外文资料翻译
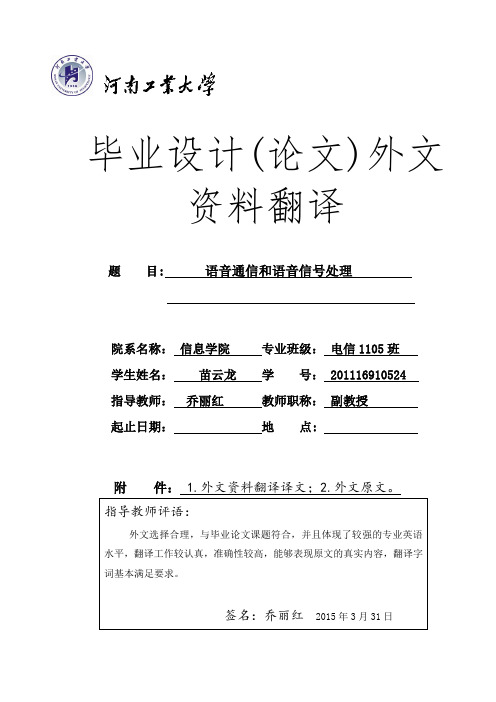
毕业设计(论文)外文资料翻译题目:语音通信和语音信号处理院系名称:信息学院专业班级:电信1105班学生姓名:苗云龙学号: 201116910524指导教师:乔丽红教师职称:副教授起止日期:地点:附件: 1.外文资料翻译译文;2.外文原文。
附件1:外文资料翻译译文语音通信和语音信号处理序言像语音所携带的信息一样,与一个机器在常规模式下进行交流不仅是一个科技性的挑战,而且还有我们对人们是如何如此不费吹灰之力进行沟通交流能力上的理解力的限制关键点在于去理解语音处理(看作是人们的沟通方式)和语音信号处理(看作是一种机制)之间的不同之处。
当人们听到语音的时候,他们会应用他们积累的语言知识与一种语言的关系来捕获信息。
在这个过程中,注意到用经过很长一段时间学得的知识资源进行有选择的处理那些输入语音信号是非常有趣的,例如良好的声音单员, 声学语音学、韵律、词汇、语法、语义和语用这些知识资源,这种处理过程因人不同而不同,并且,对于任何一个个人去准确有利的表达出他或她在处理输入语音信号这个过程中是用什么原理是非常困难的。
这也就使得通过写一段程序去通过机器来执行提取语音信号重的信息的任务变得比较困难。
应当被注意到的是,对于一种机器来说,在一个抽样序列的模式里,仅仅只有语音信号能够被提取到,而其他的一些包括在输入信号上的知识资源的鉴定以及对他们的调用都是一种科学上的挑战。
这样语音信号的处理过程就是很多非常有趣的挑战之一,以至于引起了报错很多不同科学小组的好奇,包括语言学家,语言学者,心理学或声学专家,电子工程师,计算机科学家,和应用工程师。
SADHANA的编辑文员会已经恰当的把这个主题认同为应当被定位为一个特殊的问题。
他们已经让我采取首创的自发精神来搜集引导科学小组的观点,和这个特殊问题的论文的文章形式。
我也的确非常幸运的已经能够劝说很多已经有很高成就的科学家,说服他们在他们的领域内致力于这个特殊的问题,对这个特殊的额问题多做文章。
语音识别中英文对照外文翻译文献
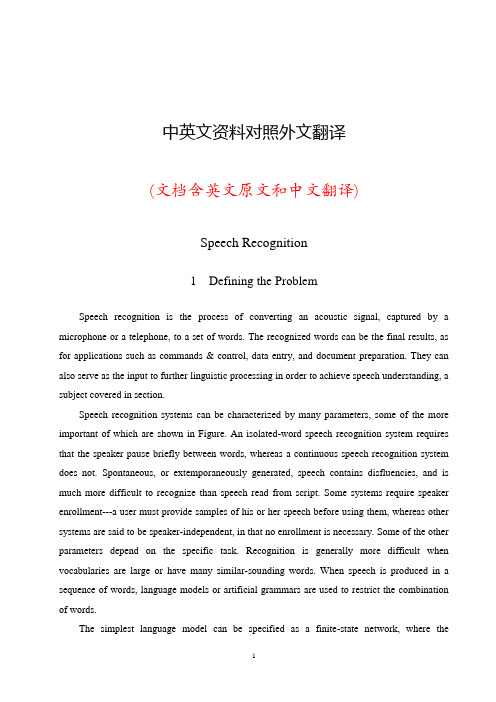
中英文资料对照外文翻译(文档含英文原文和中文翻译)Speech Recognition1 Defining the ProblemSpeech recognition is the process of converting an acoustic signal, captured by a microphone or a telephone, to a set of words. The recognized words can be the final results, as for applications such as commands & control, data entry, and document preparation. They can also serve as the input to further linguistic processing in order to achieve speech understanding, a subject covered in section.Speech recognition systems can be characterized by many parameters, some of the more important of which are shown in Figure. An isolated-word speech recognition system requires that the speaker pause briefly between words, whereas a continuous speech recognition system does not. Spontaneous, or extemporaneously generated, speech contains disfluencies, and is much more difficult to recognize than speech read from script. Some systems require speaker enrollment---a user must provide samples of his or her speech before using them, whereas other systems are said to be speaker-independent, in that no enrollment is necessary. Some of the other parameters depend on the specific task. Recognition is generally more difficult when vocabularies are large or have many similar-sounding words. When speech is produced in a sequence of words, language models or artificial grammars are used to restrict the combination of words.The simplest language model can be specified as a finite-state network, where the1permissible words following each word are given explicitly. More general language models approximating natural language are specified in terms of a context-sensitive grammar.One popular measure of the difficulty of the task, combining the vocabulary size and the language model, is perplexity, loosely defined as the geometric mean of the number of words that can follow a word after the language model has been applied (see section for a discussion of language modeling in general and perplexity in particular). Finally, there are some external parameters that can affect speech recognition system performance, including the characteristics of the environmental noise and the type and the placement of the microphone.Table: Typical parameters used to characterize the capability of speech recognition systems Speech recognition is a difficult problem, largely because of the many sources of variability associated with the signal. First, the acoustic realizations of phonemes, the smallest sound units of which words are composed, are highly dependent on the context in which they appear. These phonetic variabilities are exemplified by the acoustic differences of the phoneme,At word boundaries, contextual variations can be quite dramatic---making gas shortage sound like gash shortage in American English, and devo andare sound like devandare in Italian.Second, acoustic variabilities can result from changes in the environment as well as in the position and characteristics of the transducer. Third, within-speaker variabilities can result from changes in the speaker's physical and emotional state, speaking rate, or voice quality. Finally, differences in sociolinguistic background, dialect, and vocal tract size and shape can contribute to across-speaker variabilities.Figure shows the major components of a typical speech recognition system. The digitized speech signal is first transformed into a set of useful measurements or features at a fixed rate, typically once every 10--20 msec (see sectionsand 11.3 for signal representation and digital signal processing, respectively). These measurements are then used to search for the most likely word candidate, making use of constraints imposed by the acoustic, lexical, and language models. Throughout this process, training data are used to determine the values of the model parameters.Figure: Components of a typical speech recognition system.Speech recognition systems attempt to model the sources of variability described above in several ways. At the level of signal representation, researchers have developed representations that emphasize perceptually important speaker-independent features of the signal, and de-emphasize speaker-dependent characteristics. At the acoustic phonetic level, speaker variability is typically modeled using statistical techniques applied to large amounts of data. Speaker adaptation algorithms have also been developed that adapt speaker-independent acoustic models to those of the current speaker during system use, (see section). Effects of linguistic context at the acoustic phonetic level are typically handled by training separate models for phonemes in different contexts; this is called context dependent acoustic modeling.Word level variability can be handled by allowing alternate pronunciations of words in representations known as pronunciation networks. Common alternate pronunciations of words, as well as effects of dialect and accent are handled by allowing search algorithms to find alternate paths of phonemes through these networks. Statistical language models, based on estimates of the frequency of occurrence of word sequences, are often used to guide the searchthrough the most probable sequence of words.The dominant recognition paradigm in the past fifteen years is known as hidden Markov models (HMM). An HMM is a doubly stochastic model, in which the generation of the underlying phoneme string and the frame-by-frame, surface acoustic realizations are both represented probabilistically as Markov processes, as discussed in sections,and 11.2. Neural networks have also been used to estimate the frame based scores; these scores are then integrated into HMM-based system architectures, in what has come to be known as hybrid systems, as described in section 11.5.An interesting feature of frame-based HMM systems is that speech segments are identified during the search process, rather than explicitly. An alternate approach is to first identify speech segments, then classify the segments and use the segment scores to recognize words. This approach has produced competitive recognition performance in several tasks.2 State of the ArtComments about the state-of-the-art need to be made in the context of specific applications which reflect the constraints on the task. Moreover, different technologies are sometimes appropriate for different tasks. For example, when the vocabulary is small, the entire word can be modeled as a single unit. Such an approach is not practical for large vocabularies, where word models must be built up from subword units.Performance of speech recognition systems is typically described in terms of word error rate E, defined as:where N is the total number of words in the test set, and S, I, and D are the total number of substitutions, insertions, and deletions, respectively.The past decade has witnessed significant progress in speech recognition technology. Word error rates continue to drop by a factor of 2 every two years. Substantial progress has been made in the basic technology, leading to the lowering of barriers to speaker independence, continuous speech, and large vocabularies. There are several factors that have contributed to this rapid progress. First, there is the coming of age of the HMM. HMM is powerful in that, with the availability of training data, the parameters of the model can be trained automatically to giveoptimal performance.Second, much effort has gone into the development of large speech corpora for system development, training, and testing. Some of these corpora are designed for acoustic phonetic research, while others are highly task specific. Nowadays, it is not uncommon to have tens of thousands of sentences available for system training and testing. These corpora permit researchers to quantify the acoustic cues important for phonetic contrasts and to determine parameters of the recognizers in a statistically meaningful way. While many of these corpora (e.g., TIMIT, RM, ATIS, and WSJ; see section 12.3) were originally collected under the sponsorship of the U.S. Defense Advanced Research Projects Agency (ARPA) to spur human language technology development among its contractors, they have nevertheless gained world-wide acceptance (e.g., in Canada, France, Germany, Japan, and the U.K.) as standards on which to evaluate speech recognition.Third, progress has been brought about by the establishment of standards for performance evaluation. Only a decade ago, researchers trained and tested their systems using locally collected data, and had not been very careful in delineating training and testing sets. As a result, it was very difficult to compare performance across systems, and a system's performance typically degraded when it was presented with previously unseen data. The recent availability of a large body of data in the public domain, coupled with the specification of evaluation standards, has resulted in uniform documentation of test results, thus contributing to greater reliability in monitoring progress (corpus development activities and evaluation methodologies are summarized in chapters 12 and 13 respectively).Finally, advances in computer technology have also indirectly influenced our progress. The availability of fast computers with inexpensive mass storage capabilities has enabled researchers to run many large scale experiments in a short amount of time. This means that the elapsed time between an idea and its implementation and evaluation is greatly reduced. In fact, speech recognition systems with reasonable performance can now run in real time using high-end workstations without additional hardware---a feat unimaginable only a few years ago.One of the most popular, and potentially most useful tasks with low perplexity (PP=11) is the recognition of digits. For American English, speaker-independent recognition of digit strings spoken continuously and restricted to telephone bandwidth can achieve an error rate of 0.3% when the string length is known.One of the best known moderate-perplexity tasks is the 1,000-word so-called Resource Management (RM) task, in which inquiries can be made concerning various naval vessels in the Pacific ocean. The best speaker-independent performance on the RM task is less than 4%, using a word-pair language model that constrains the possible words following a given word (PP=60). More recently, researchers have begun to address the issue of recognizing spontaneously generated speech. For example, in the Air Travel Information Service (ATIS) domain, word error rates of less than 3% has been reported for a vocabulary of nearly 2,000 words and a bigram language model with a perplexity of around 15.High perplexity tasks with a vocabulary of thousands of words are intended primarily for the dictation application. After working on isolated-word, speaker-dependent systems for many years, the community has since 1992 moved towards very-large-vocabulary (20,000 words and more), high-perplexity (PP≈200), speaker-independent, continuous speech recognition. The best system in 1994 achieved an error rate of 7.2% on read sentences drawn from North America business news.With the steady improvements in speech recognition performance, systems are now being deployed within telephone and cellular networks in many countries. Within the next few years, speech recognition will be pervasive in telephone networks around the world. There are tremendous forces driving the development of the technology; in many countries, touch tone penetration is low, and voice is the only option for controlling automated services. In voice dialing, for example, users can dial 10--20 telephone numbers by voice (e.g., call home) after having enrolled their voices by saying the words associated with telephone numbers. AT&T, on the other hand, has installed a call routing system using speaker-independent word-spotting technology that can detect a few key phrases (e.g., person to person, calling card) in sentences such as: I want to charge it to my calling card.At present, several very large vocabulary dictation systems are available for document generation. These systems generally require speakers to pause between words. Their performance can be further enhanced if one can apply constraints of the specific domain such as dictating medical reports.Even though much progress is being made, machines are a long way from recognizing conversational speech. Word recognition rates on telephone conversations in the Switchboard corpus are around 50%. It will be many years before unlimited vocabulary, speaker-independentcontinuous dictation capability is realized.3 Future DirectionsIn 1992, the U.S. National Science Foundation sponsored a workshop to identify the key research challenges in the area of human language technology, and the infrastructure needed to support the work. The key research challenges are summarized in. Research in the following areas for speech recognition were identified:Robustness:In a robust system, performance degrades gracefully (rather than catastrophically) as conditions become more different from those under which it was trained. Differences in channel characteristics and acoustic environment should receive particular attention.Portability:Portability refers to the goal of rapidly designing, developing and deploying systems for new applications. At present, systems tend to suffer significant degradation when moved to a new task. In order to return to peak performance, they must be trained on examples specific to the new task, which is time consuming and expensive.Adaptation:How can systems continuously adapt to changing conditions (new speakers, microphone, task, etc) and improve through use? Such adaptation can occur at many levels in systems, subword models, word pronunciations, language models, etc.Language Modeling:Current systems use statistical language models to help reduce the search space and resolve acoustic ambiguity. As vocabulary size grows and other constraints are relaxed to create more habitable systems, it will be increasingly important to get as much constraint as possible from language models; perhaps incorporating syntactic and semantic constraints that cannot be captured by purely statistical models.Confidence Measures:Most speech recognition systems assign scores to hypotheses for the purpose of rank ordering them. These scores do not provide a good indication of whether a hypothesis is correct or not, just that it is better than the other hypotheses. As we move to tasks that require actions,we need better methods to evaluate the absolute correctness of hypotheses.Out-of-Vocabulary Words:Systems are designed for use with a particular set of words, but system users may not know exactly which words are in the system vocabulary. This leads to a certain percentage of out-of-vocabulary words in natural conditions. Systems must have some method of detecting such out-of-vocabulary words, or they will end up mapping a word from the vocabulary onto the unknown word, causing an error.Spontaneous Speech:Systems that are deployed for real use must deal with a variety of spontaneous speech phenomena, such as filled pauses, false starts, hesitations, ungrammatical constructions and other common behaviors not found in read speech. Development on the ATIS task has resulted in progress in this area, but much work remains to be done.Prosody:Prosody refers to acoustic structure that extends over several segments or words. Stress, intonation, and rhythm convey important information for word recognition and the user's intentions (e.g., sarcasm, anger). Current systems do not capture prosodic structure. How to integrate prosodic information into the recognition architecture is a critical question that has not yet been answered.Modeling Dynamics:Systems assume a sequence of input frames which are treated as if they were independent. But it is known that perceptual cues for words and phonemes require the integration of features that reflect the movements of the articulators, which are dynamic in nature. How to model dynamics and incorporate this information into recognition systems is an unsolved problem.语音识别一定义问题语音识别是指音频信号的转换过程,被电话或麦克风的所捕获的一系列的消息。
信号处理中英文对照外文翻译文献
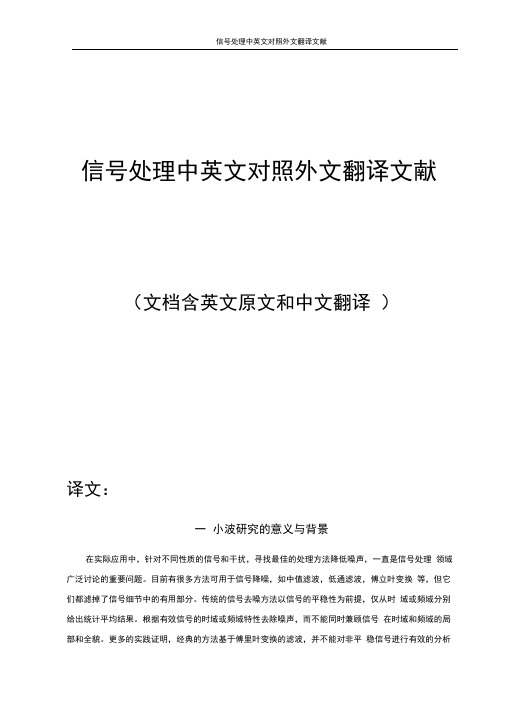
信号处理中英文对照外文翻译文献(文档含英文原文和中文翻译)译文:一小波研究的意义与背景在实际应用中,针对不同性质的信号和干扰,寻找最佳的处理方法降低噪声,一直是信号处理领域广泛讨论的重要问题。
目前有很多方法可用于信号降噪,如中值滤波,低通滤波,傅立叶变换等,但它们都滤掉了信号细节中的有用部分。
传统的信号去噪方法以信号的平稳性为前提,仅从时域或频域分别给出统计平均结果。
根据有效信号的时域或频域特性去除噪声,而不能同时兼顾信号在时域和频域的局部和全貌。
更多的实践证明,经典的方法基于傅里叶变换的滤波,并不能对非平稳信号进行有效的分析和处理,去噪效果已不能很好地满足工程应用发展的要求。
常用的硬阈值法则和软阈值法则采用设置高频小波系数为零的方法从信号中滤除噪声。
实践证明,这些小波阈值去噪方法具有近似优化特性,在非平稳信号领域中具有良好表现。
小波理论是在傅立叶变换和短时傅立叶变换的基础上发展起来的,它具有多分辨分析的特点,在时域和频域上都具有表征信号局部特征的能力,是信号时频分析的优良工具。
小波变换具有多分辨性、时频局部化特性及计算的快速性等属性,这使得小波变换在地球物理领域有着广泛的应用。
随着技术的发展,小波包分析 (Wavelet Packet Analysis) 方法产生并发展起来,小波包分析是小波分析的拓展,具有十分广泛的应用价值。
它能够为信号提供一种更加精细的分析方法,它将频带进行多层次划分,对离散小波变换没有细分的高频部分进一步分析,并能够根据被分析信号的特征,自适应选择相应的频带,使之与信号匹配,从而提高了时频分辨率。
小波包分析 (wavelet packet analysis) 能够为信号提供一种更加精细的分析方法,它将频带进行多层次划分,对小波分析没有细分的高频部分进一步分解,并能够根据被分析信号的特征,自适应地选择相应频带 , 使之与信号频谱相匹配,因而小波包具有更广泛的应用价值。
利用小波包分析进行信号降噪,一种直观而有效的小波包去噪方法就是直接对小波包分解系数取阈值,选择相关的滤波因子,利用保留下来的系数进行信号的重构,最终达到降噪的目的。
win7语音识别中英文对照语音指令

select when through Phrases 选择从 when到Phrases
new line/new pragraph 新起一行
go to the end of the document 到行末尾
go to the startof the document 到行首部
accessories 附件
double click... 双击
right click document Maxine mize 右击文本
show numbers 显示编码
scroll down 向下滑动
scroll up 向上滑动
scroll down 10 向下滑动10
maximize 最大化
restore down 还原
mousegrid 鼠标格子
press capital b 按下打下b
press c as in close 按下c作为结束
backspace 退格
press control home 按下 ctrl+home
press y 3 times 按下3次y
start 开始
all programs 所有程序
6. show speech recgnition 显示语音识别
7. what can I say 我能说什么
period 句号
exclamation mark 感叹号
question mark 问号
correct ... 改正...(改正某个单词)
undo/undo that/delete that 取消
scroll up 20 向上滑动20
09 语音信号处理(temp) _ New
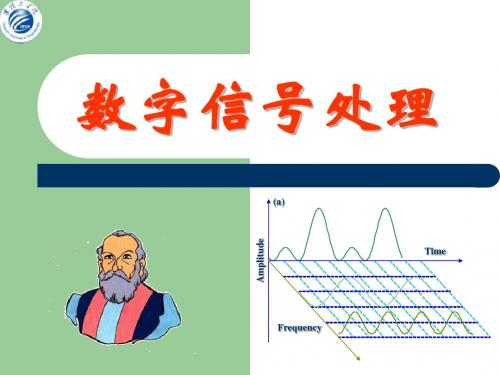
24/83
例 3:—— 短时平均能量
load chirp; % 加载鸟鸣信号
25/83
subplot(3,1,1),plot(y);
h1=linspace(1,1,64); En1=conv(h1,y.*y); subplot(3,1,2),plot(En1); legend('N= 64'); h2=linspace(1,1,512); En2=conv(h2,y.*y); subplot(3,1,3),plot(En2); %形成一个矩形窗,长度为N % 求y平方与h的卷积,从而短时能量函数En %形成一个矩形窗,长度为N % 求y平方与h的卷积,从而短时能量函数En
分帧函数:
测试代码: y=[1,2,-1,1,1,-1,-1,0,1,1,1,1,3,1,1,1,1,1,6,-1,1,1,1,-1,1,1,1,1,-1,1,2,3]; xn=enframe1(y,10,5); 结果如下: xn = 0.0800 -0.0800 0.0800 0.0800 0.0800 0.0800 0.3752 -0.1876 0.1876 0.1876 0.1876 0.1876 -0.4601 0 1.3804 0.4601 0.4601 0.4601 0.7700 0.7700 0.7700 4.6200 -0.7700 -0.7700 0.9723 0.9723 0.9723 -0.9723 0.9723 0.9723 -0.9723 0.9723 0.9723 0.9723 0.9723 1.9445 -0.7700 0.7700 0.7700 0.7700 0.7700 2.3100 0 1.3804 0.4601 0.4601 0.4601 0 0.1876 0.1876 1.1257 -0.1876 -0.1876 0 0.0800 0.0800 -0.0800 0.0800 0.0800 0
我收集到的最齐全的音频专业术语中英文对照表翻译交流

我收集到的最齐全的音频专业术语中英文对照表翻译交流AAAC automatic ampltiude control 自动幅度控制AB AB制立体声录音法Abeyancd 暂停,潜态A-B repeat A-B重复ABS absolute 绝对的,完全的,绝对时间ABS american bureau of standard 美国标准局ABSS auto blank secrion scanning 自动磁带空白部分扫描Abstime 绝对运行时间A.DEF audio defeat 音频降噪,噪声抑制,伴音静噪ADJ adjective 附属的,附件ADJ Adjust 调节ADJ acoustic delay line 声延迟线Admission 允许进入,供给ADP acoustic data processor 音响数据处理机ADP(T) adapter 延配器,转接器ADRES automatic dynamic range expansion system 动态范围扩展系统ADRM analog to digital remaster 模拟录音、数字处理数码唱盘ADS audio distribution system 音频分配系统A。
DUB audio dubbing 配音,音频复制,后期录音ADV advance 送入,提升,前置量ADV adversum 对抗ADV advancer 相位超前补偿器Adventure 惊险效果AE audio erasing 音频(声音)擦除AE auxiliary equipment 辅助设备Aerial 天线AES audio engineering society 美国声频工程协会AF audio fidelity 音频保真度AF audio frequency 音频频率AFC active field control 自动频率控制AFC automatic frequency control 声场控制Affricate 塞擦音AFL aside fade listen 衰减后(推子后)监听A-fader 音频衰减AFM advance frequency modulation 高级调频AFS acoustic feedback speaker 声反馈扬声器AFT automatic fine tuning 自动微调AFTAAS advanced fast time acoustic analysis system 高级快速音响分析系统After 转移部分文件Afterglow 余辉,夕照时分音响效果Against 以……为背景AGC automatic gain control 自动增益控制AHD audio high density 音频高密度唱片系统AI advanced integrated 预汇流AI amplifier input 放大器输入AI artificial intelligence 人工智能AI azimuth indicator 方位指示器A-IN 音频输入A-INSEL audio input selection 音频输入选择Alarm 警报器ALC automatic level control 自动电平控制ALC automatic load control自动负载控制Alford loop 爱福特环形天线Algorithm 演示Aliasing 量化噪声,频谱混叠Aliasing distortion 折叠失真Align alignment 校正,补偿,微调,匹配Al—Si—Fe alloy head 铁硅铝合金磁头Allegretto 小快板,稍快地Allegro 快板,迅速地Allocation 配置,定位All rating 全(音)域ALM audio level meter 音频电平表ALT alternating 震荡,交替的ALT alternator 交流发电机ALT altertue 转路ALT-CH alternate channel 转换通道,交替声道Alter 转换,交流电,变换器AM amperemeter 安培计,电流表AM amplitude modulation 调幅(广播)AM auxiliary memory 辅助存储器Ambience 临场感,环绕感ABTD automatic bulk tape degausser 磁带自动整体去磁电路Ambient 环境的Ambiophonic system 环绕声系统Ambiophony 现场混响,环境立体声AMLS automatic music locate system 自动音乐定位系统AMP ampere 安培AMP amplifier 放大器AMPL amplification 放大AMP amplitude 幅度,距离Amorphous head 非晶态磁头Abort 终止,停止(录制或播放)A-B TEST AB比较试听Absorber 减震器Absorption 声音被物体吸收ABX acoustic bass extension 低音扩展AC accumulator 充电电池AC adjustment caliration 调节—校准AC alternating current 交流电,交流AC audio coding 数码声,音频编码AC audio center 音频中心AC azimuth comprator 方位比较器AC—3 杜比数码环绕声系统AC—3 RF 杜比数码环绕声数据流(接口) ACC Acceleration 加速Accel 渐快,加速Accent 重音,声调Accentuator 预加重电路Access 存取,进入,增加,通路Accessory 附件(接口),配件Acryl 丙基酰基Accompaniment 伴奏,合奏,伴随Accord 和谐,调和Accordion 手风琴ACD automatic call distributor 自动呼叫分配器ACE audio control erasing 音频控制消磁A-Channel A(左)声道Acoumeter 测听计Acoustical 声的,声音的Acoustic coloring 声染色Acoustic image 声像Across 交叉,并行,跨接Across frequency 交叉频率,分频频率ACST access time 存取时间Active 主动的,有源的,有效的,运行的Active crossover 主动分频,电子分频,有源分频Active loudsperker 有源音箱Armstrong MOD 阿姆斯特朗调制ARP azimuth reference pulse 方位基准脉冲Arpeggio 琶音Articulation 声音清晰度,发音Artificial 仿……的,人工的,手动(控制) AAD active acoustic devide 有源声学软件ABC auto base and chord 自动低音合弦Architectural acoustics 建筑声学Arm motor 唱臂唱机Arpeggio single 琶音和弦,分解和弦ARL aerial 天线ASC automatic sensitivity control 自动灵敏度控制ASGN Assign 分配,指定,设定ASP audio signal processing 音频信号处理ASS assembly 组件,装配,总成ASSEM assemble 汇编,剪辑ASSEM Assembly 组件,装配,总成Assign 指定,转发,分配Assist 辅助(装置)ASSY accessory 组件,附件AST active servo techonology 有源伺服技术A Tempo 回到原速Astigmatism methord 象散法BB band 频带B Bit 比特,存储单元B Button 按钮Babble 多路感应的复杂失真Back 返回Back clamping 反向钳位Back drop 交流哼声,干扰声Background noise 背景噪声,本底噪声Backing copy 副版Backoff 倒扣,补偿Back tracking 补录Back up 磁带备份,支持,预备Backward 快倒搜索Baffle box 音箱BAL balance 平衡,立体声左右声道音量比例,平衡连接Balanced 已平衡的Balancing 调零装置,补偿,中和Balun 平衡=不平衡转换器Banana jack 香蕉插头Banana bin 香蕉插座Banana pin 香蕉插头Banana plug 香蕉插头Band 频段,Band pass 带通滤波器Bandwidth 频带宽,误差,范围Band 存储单元Bar 小节,拉杆BAR barye 微巴Bargraph 线条Barrier 绝缘(套)Base 低音Bass 低音,倍司(低音提琴)Bass tube 低音号,大号Bassy 低音加重BATT battery 电池Baud 波特(信息传输速率的单位)Bazooka 导线平衡转接器BB base band 基带BBD Bucket brigade device 戽链器件(效果器)B BAT Battery 电池BBE 特指BBE公司设计的改善较高次谐波校正程度的系统BC balanced current 平衡电流BC Broadcast control 广播控制BCH band chorus 分频段合唱BCST broadcast (无线电)广播BD board 仪表板Beat 拍,脉动信号Beat cancel switch 差拍干扰消除开关Bel 贝尔Below 下列,向下Bench 工作台Bend 弯曲,滑音Bender 滑音器BER bit error rate 信息差错率BF back feed 反馈BF Backfeed flanger 反馈镶边BF Band filter 带通滤波器BGM background music 背景音乐Bias 偏置,偏磁,偏压,既定程序Bidirectional 双向性的,8字型指向的Bifess Bi-feedback sound system 双反馈系统Big bottom 低音扩展,加重低音Bin 接收器,仓室BNG BNC连接器(插头、插座),卡口同轴电缆连接器Binaural effect 双耳效应,立体声Binaural synthesis 双耳合成法Bin go 意外现象Bit binary digit 字节,二进制数字,位Bitstream 数码流,比特流Bit yield 存储单元Bi-AMP 双(通道)功放系统Bi-wire 双线(传输、分音)Bi—Wring 双线BK break 停顿,间断BKR breaker 断电器Blamp 两路电子分音Blanking 关闭,消隐,断路Blaster 爆裂效果器Blend 融合(度)、调和、混合Block 分程序,联动,中断Block Repeat 分段重复Block up 阻塞Bloop (磁带的)接头噪声,消音贴片BNC bayonet connector 卡口电缆连接器Body mike 小型话筒Bond 接头,连接器Bongo 双鼓Boom 混响,轰鸣声Boomy 嗡嗡声(指低音过强)Boost 提升(一般指低音),放大,增强Booth 控制室,录音棚Bootstrap 辅助程序,自举电路Both sides play disc stereo system双面演奏式唱片立体声系统Bottoming 底部切除,末端切除Bounce 合并Bourclon 单调低音Bowl 碗状体育场效果BP bridge bypass 电桥旁路BY bypass 旁通BPC basic pulse generator 基准脉冲发生器。
英语语音翻译中文的功能

现在的AI技术发展很快,智能语音翻译机就是同声声控语音识别的,也就是说,你对着翻译界面的话筒说出一句话,就可以就可以及时翻译出对方懂的语言,同时有文字输出哦。
操作选用工具:在应用市场下载【录音转文字助手】
操作步骤:
第一步:首先我们在百度手机助手或者应用市场里面搜索:【录音转文字助手】找到以后进行下载并安装。
第二步:接着打开软件就可以看到【录音识别】、【文件识别】、【语音翻译】、【录音机】的四个功能,这里我们就举例说明下【语音翻译】。
第三步:点击橙色的【中文】按钮,开始说中文以后,下面就是翻译的英文。
第四步:点击蓝色的【English】按钮,开始说英文,就可以把你说的英语转成中文了。
以上就是语音翻译的操作步骤了,方法介绍到这里,希望可以对大家有帮助哦。
专业音频术语中英文对照T
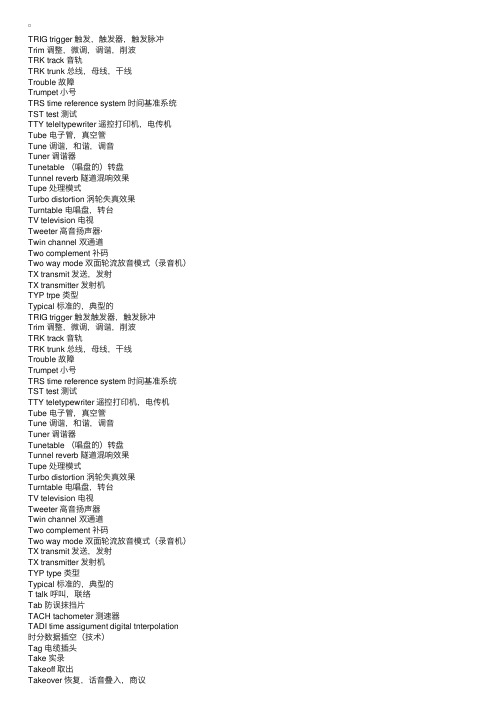
TRIG trigger 触发,触发器,触发脉冲Trim 调整,微调,调谐,削波TRK track ⾳轨TRK trunk 总线,母线,⼲线Trouble 故障Trumpet ⼩号TRS time reference system 时间基准系统TST test 测试TTY teleltypewriter 遥控打印机,电传机Tube 电⼦管,真空管Tune 调谐,和谐,调⾳Tuner 调谐器Tunetable (唱盘的)转盘Tunnel reverb 隧道混响效果Tupe 处理模式Turbo distortion 涡轮失真效果Turntable 电唱盘,转台TV television 电视Tweeter ⾼⾳扬声器·Twin channel 双通道Two complement 补码Two way mode 双⾯轮流放⾳模式(录⾳机)TX transmit 发送,发射TX transmitter 发射机TYP trpe 类型Typical 标准的,典型的TRIG trigger 触发触发器,触发脉冲Trim 调整,微调,调谐,削波TRK track ⾳轨TRK trunk 总线,母线,⼲线Trouble 故障Trumpet ⼩号TRS time reference system 时间基准系统TST test 测试TTY teletypewriter 遥控打印机,电传机Tube 电⼦管,真空管Tune 调谐,和谐,调⾳Tuner 调谐器Tunetable (唱盘的)转盘Tunnel reverb 隧道混响效果Tupe 处理模式Turbo distortion 涡轮失真效果Turntable 电唱盘,转台TV television 电视Tweeter ⾼⾳扬声器Twin channel 双通道Two complement 补码Two way mode 双⾯轮流放⾳模式(录⾳机)TX transmit 发送,发射TX transmitter 发射机TYP type 类型Typical 标准的,典型的T talk 呼叫,联络Tab 防误抹挡⽚TACH tachometer 测速器TADI time assigument digital tnterpolation时分数据插空(技术)Tag 电缆插头Take 实录Takeoff 取出Takeover 恢复,话⾳叠⼊,商议Talkback 对讲,联络Tally 播出,提⽰,插⼊Tap 电流输出,节拍Tape 带,磁带Tango 探⼽TB talkback 对讲回送TB terminal board 接线端⼦⼦板TB time base 时基TBC time base corrector 时基校正器TBK tallback 对讲TC telecine 电影电视机TC time code 时间码TC transmitter-tunning circuit 发射机调谐电路TC trim coil 微调线圈TCC tripl concentric cable 三芯同轴电缆TDE time domain equalizer 时域等化器TECH technique 技术,技能,技巧TED television disk 电视唱⽚TEL telescopic 拉杆天线TEMP temperature 温度Temp 节奏TEMOP temporary 中间(⼯作)单元TEMPO Tempo 节奏,连接,速度Terminal 终端,接线柱,引线,接头Tentelometer 张⼒表TER termination 终端Test 测试,试验,检验THD total harmonic distortion 总谐波失真Theater 剧场效果,现场Thermal noise 热噪声Thick 沈重,厚重度Thin 单薄声⾳Thinness 薄(打击乐)THR THRESH threshold 阈值,阈,门限Thresh thrash 多次反复Three dimension 3D⾳响,三维⽴体声⾳响系统Throat ⾼⾳号⾓的喉THRU through 通过,过桥,直接转送Trump 键击噪声,低频噪声,开机砰声Thrust 插⼊,强⾏加⼊THX tom holman"s eXpriment 汤·霍尔曼实验,家庭* TI temperatun indicator 温度指⽰器TIE terminal interface exchange 终端接⼝交换Tie 连接符号,馈线,通信Tierce 第三⾳,五倍⾳Tight 硬,紧,硬朗TIM transient intermodulation 瞬态互调失真Timber ⾳质,⾳⾊Timbre 声部Time 时间,倍,次,定时的Timer 定时器,计时器Tininess 单薄Tint ⾊调TIP terminal interface processor 终端接⼝处理机Tip 头端,热端Title 标题,字幕TK track ⾳轨TL track loss 轨迹丢失TLE trunk line equipment ⼲线设备TM trade mark 注册商标TMS transmission mesurement set 电平表TMT transmit 发送TN tuning unit 调谐装置TOC 节⽬⽬录Tone ⾳调,声调,纯⾳Tone burst 猝发⾳Tone color ⾳⾊Tone quality ⾳⾊,⾳品Tonic 律⾳TopTOS tape operating system 磁带操作系统Total 总,总共Total tune 整体协调,总调谐Touch 触,压,按Touch sens 键盘乐器指触的触感TPD turnout piece delay 分⽀延时TR tape recorder 磁带录⾳机TR telerecording 电视屏幕录像TR tracking 跟踪TR transfer 传输,转移TR trick 特技效果Track 曲⽬号,磁迹,⾳轨Tracking 寻迹,跟踪,统调Tracking monitor 调校监听Trad 陷波器,带阻滤波器Tramp 三通道功放系统Transfer 转印,转接Transformer 变压器Transpose 转调,变换器,移调Transport 运⾏,发送Transient 瞬态Transient distortion 瞬态失真Transient response 瞬态反应Transmit 发射Transistor 晶体管,三极管Transponder 转调器,变换器Transposer 变调器Transversal equalizers 横向均衡器Treble ⾼⾳,三倍的,三重的Tremold tremor 颤⾳Tremolo 震⾳Tremor 颤⾳,振⾳装置Triamp 三路电⼦分⾳Trick 特技Trig 修饰 Spatializer 声场定位技术。
Efficient voice activity detection algorithms using long-term speech information
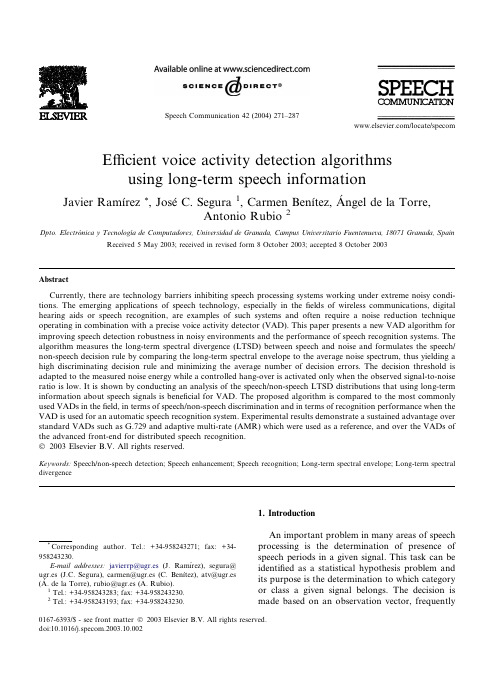
Efficient voice activity detection algorithmsusing long-term speech informationJavier Ram ırez *,Jos e C.Segura 1,Carmen Ben ıtez, Angel de la Torre,Antonio Rubio 2Dpto.Electr o nica y Tecnolog ıa de Computadores,Universidad de Granada,Campus Universitario Fuentenueva,18071Granada,SpainReceived 5May 2003;received in revised form 8October 2003;accepted 8October 2003AbstractCurrently,there are technology barriers inhibiting speech processing systems working under extreme noisy condi-tions.The emerging applications of speech technology,especially in the fields of wireless communications,digitalhearing aids or speech recognition,are examples of such systems and often require a noise reduction technique operating in combination with a precise voice activity detector (VAD).This paper presents a new VAD algorithm for improving speech detection robustness in noisy environments and the performance of speech recognition systems.The algorithm measures the long-term spectral divergence (LTSD)between speech and noise and formulates the speech/non-speech decision rule by comparing the long-term spectral envelope to the average noise spectrum,thus yielding a high discriminating decision rule and minimizing the average number of decision errors.The decision threshold is adapted to the measured noise energy while a controlled hang-over is activated only when the observed signal-to-noise ratio is low.It is shown by conducting an analysis of the speech/non-speech LTSD distributions that using long-term information about speech signals is beneficial for VAD.The proposed algorithm is compared to the most commonly used VADs in the field,in terms of speech/non-speech discrimination and in terms of recognition performance when the VAD is used for an automatic speech recognition system.Experimental results demonstrate a sustained advantage over standard VADs such as G.729and adaptive multi-rate (AMR)which were used as a reference,and over the VADs of the advanced front-end for distributed speech recognition.Ó2003Elsevier B.V.All rights reserved.Keywords:Speech/non-speech detection;Speech enhancement;Speech recognition;Long-term spectral envelope;Long-term spectral divergence1.IntroductionAn important problem in many areas of speech processing is the determination of presence of speech periods in a given signal.This task can be identified as a statistical hypothesis problem and its purpose is the determination to which category or class a given signal belongs.The decision is made based on an observation vector,frequently*Corresponding author.Tel.:+34-958243271;fax:+34-958243230.E-mail addresses:javierrp@ugr.es (J.Ram ırez),segura@ugr.es (J.C.Segura),carmen@ugr.es (C.Ben ıtez),atv@ugr.es ( A.de la Torre),rubio@ugr.es (A.Rubio).1Tel.:+34-958243283;fax:+34-958243230.2Tel.:+34-958243193;fax:+34-958243230.0167-6393/$-see front matter Ó2003Elsevier B.V.All rights reserved.doi:10.1016/j.specom.2003.10.002Speech Communication 42(2004)271–287/locate/specomcalled feature vector,which serves as the input to a decision rule that assigns a sample vector to one of the given classes.The classification task is often not as trivial as it appears since the increasing level of background noise degrades the classifier effec-tiveness,thus leading to numerous detection er-rors.The emerging applications of speech technolo-gies(particularly in mobile communications,ro-bust speech recognition or digital hearing aid devices)often require a noise reduction scheme working in combination with a precise voice activity detector(VAD)(Bouquin-Jeannes and Faucon,1994,1995).During the last decade numerous researchers have studied different strat-egies for detecting speech in noise and the influ-ence of the VAD decision on speech processing systems(Freeman et al.,1989;ITU,1996;Sohn and Sung,1998;ETSI,1999;Marzinzik and Kol-lmeier,2002;Sangwan et al.,2002;Karray and Martin,2003).Most authors reporting on noise reduction refer to speech pause detection when dealing with the problem of noise estimation.The non-speech detection algorithm is an important and sensitive part of most of the existing single-microphone noise reduction schemes.There exist well known noise suppression algorithms(Berouti et al.,1979;Boll,1979),such as Wienerfiltering (WF)or spectral subtraction,that are widely used for robust speech recognition,and for which,the VAD is critical in attaining a high level of per-formance.These techniques estimate the noise spectrum during non-speech periods in order to compensate its harmful effect on the speech signal. Thus,the VAD is more critical for non-stationary noise environments since it is needed to update the constantly varying noise statistics affecting a mis-classification error strongly to the system perfor-mance.In order to palliate the importance of the VAD in a noise suppression systems Martin pro-posed an algorithm(Martin,1993)that continu-ally updated the noise spectrum in order to prevent a misclassification of the speech signal causes a degradation of the enhanced signal.These tech-niques are faster in updating the noise but usually capture signal energy during speech periods,thus degrading the quality of the compensated speech signal.In this way,it is clearly better using an efficient VAD for most of the noise suppression systems and applications.VADs are employed in many areas of speech processing.Recently,various voice activity detec-tion procedures have been described in the litera-ture for several applications including mobile communication services(Freeman et al.,1989), real-time speech transmission on theInternet 272J.Ram ırez et al./Speech Communication42(2004)271–287(Sangwan et al.,2002)or noise reduction for dig-ital hearing aid devices(Itoh and Mizushima, 1997).Interest of research has focused on the development of robust algorithms,with special attention being paid to the study and derivation of noise robust features and decision rules.Sohn and Sung(1998)presented an algorithm that uses a novel noise spectrum adaptation employing soft decision techniques.The decision rule was derived from the generalized likelihood ratio test by assuming that the noise statistics are known a priori.An enhanced version(Sohn et al.,1999)of the original VAD was derived with the addition of a hang-over scheme which considers the previous observations of afirst-order Markov process modeling speech occurrences.The algorithm out-performed or at least was comparable to the G.729B VAD(ITU,1996)in terms of speech detection and false-alarm probabilities.Other researchers presented improvements over the algorithm proposed by Sohn et al.(1999).Cho et al.(2001a);Cho and Kondoz(2001)presented a smoothed likelihood ratio test to alleviate the detection errors,yielding better results than G.729B and comparable performance to adaptive multi-rate(AMR)option2.Cho et al.(2001b)also proposed a mixed decision-based noise adaptation yielding better results than the soft decision noise adaptation technique reported by Sohn and Sung (1998).Recently,a new standard incorporating noise suppression methods has been approved by the European Telecommunication Standards Institute(ETSI)for feature extraction and dis-tributed speech recognition(DSR).The so-called advanced front-end(AFE)(ETSI,2002)incorpo-rates an energy-based VAD(WF AFE VAD)for estimating the noise spectrum in Wienerfiltering speech enhancement,and a different VAD for non-speech frame dropping(FD AFE VAD).On the other hand,a VAD achieves silence compression in modern mobile telecommunication systems reducing the average bit rate by using the discontinuous transmission(DTX)mode.Many practical applications,such as the global system for mobile communications(GSM)telephony,use silence detection and comfort noise injection for higher coding efficiency.The International Tele-communication Union(ITU)adopted a toll-quality speech coding algorithm known as G.729 to work in combination with a VAD module in DTX mode.The recommendation G.729Annex B (ITU,1996)uses a feature vector consisting of the linear prediction(LP)spectrum,the full-band en-ergy,the low-band(0–1KHz)energy and the zero-crossing rate(ZCR).The standard was developed with the collaboration of researchers from France Telecom,the University of Sherbrooke,NTT and AT&T Bell Labs and the effectiveness of the VAD was evaluated in terms of subjective speech quality and bit rate savings(Benyassine et al.,1997). Objective performance tests were also conducted by hand-labelling a large speech database and assessing the correct identification of voiced,un-voiced,silence and transition periods.Another standard for DTX is the ETSI adaptive multi-rate speech coder(ETSI,1999)developed by the special mobile group for the GSM system.The standard specifies two options for the VAD to be used within the digital cellular telecommunications system.In option1,the signal is passed through a filterbank and the level of signal in each band is calculated.A measure of the signal-to-signal ratio (SNR)is used to make the VAD decision together with the output of a pitch detector,a tone detector and the correlated complex signal analysis module. An enhanced version of the original VAD is the AMR option2VAD.It uses parameters of the speech encoder being more robust against envi-ronmental noise than AMR1and G.729.These VADs have been used extensively in the open literature as a reference for assessing the perfor-mance of new algorithms.Marzinzik and Kol-lmeier(2002)proposed a new VAD algorithm for noise spectrum estimation based on tracking the power envelope dynamics.The algorithm was compared to the G.729VAD by means of the re-ceiver operating characteristic(ROC)curves showing a reduction in the non-speech false alarm rate together with an increase of the non-speech hit rate for a representative set of noises and condi-tions.Beritelli et al.(1998)proposed a fuzzy VAD with a pattern matching block consisting of a set of six fuzzy rules.The comparison was made using objective,psychoacoustic,and subjective parame-ters being G.729and AMR VADs used as a ref-erence(Beritelli et al.,2002).Nemer et al.(2001)J.Ram ırez et al./Speech Communication42(2004)271–287273presented a robust algorithm based on higher order statistics(HOS)in the linear prediction cod-ing coefficients(LPC)residual domain.Its perfor-mance was compared to the ITU-T G.729B VAD in various noise conditions,and quantified using the probability of correct and false classifications.The selection of an adequate feature vector for signal detection and a robust decision rule is a challenging problem that affects the performance of VADs working under noise conditions.Most algorithms are effective in numerous applications but often cause detection errors mainly due to the loss of discriminating power of the decision rule at low SNR levels(ITU,1996;ETSI,1999).For example,a simple energy level detector can work satisfactorily in high signal-to-noise ratio condi-tions,but would fail significantly when the SNR drops.Several algorithms have been proposed in order to palliate these drawbacks by means of the definition of more robust decision rules.This paper explores a new alternative towards improv-ing speech detection robustness in adverse envi-ronments and the performance of speech recognition systems.A new technique for speech/ non-speech detection(SND)using long-term information about the speech signal is studied.The algorithm is evaluated in the context of the AURORA project(Hirsch and Pearce,2000; ETSI,2000),and the recently approved Advanced Front-end standard(ETSI,2002)for distributed speech recognition.The quantifiable benefits of this approach are assessed by means of an exhaustive performance analysis conducted on the AURORA TIdigits(Hirsch and Pearce,2000)and SpeechDat-Car(SDC)(Moreno et al.,2000;No-kia,2000;Texas Instruments,2001)databases, with standard VADs such as the ITU G.729(ITU, 1996),ETSI AMR(ETSI,1999)and AFE(ETSI, 2002)used as a reference.2.VAD based on the long-term spectral divergenceVADs are generally characterized by the feature selection,noise estimation and classification methods.Various features and combinations of features have been proposed to be used in VAD algorithms(ITU,1996;Beritelli et al.,1998;Sohn and Sung,1998;Nemer et al.,2001).Typically, these features represent the variations in energy levels or spectral difference between noise and speech.The most discriminating parameters in speech detection are the signal energy,zero-cross-ing rates,periodicity measures,the entropy,or linear predictive coding coefficients.The proposed speech/non-speech detection algorithm assumes that the most significant information for detecting voice activity on a noisy speech signal remains on the time-varying signal spectrum magnitude. It uses a long-term speech window instead of instantaneous values of the spectrum to track the spectral envelope and is based on the estimation of the so-called long-term spectral envelope(LTSE). The decision rule is then formulated in terms of the long-term spectral divergence(LTSD)between speech and noise.The motivations for the pro-posed strategy will be clarified by studying the distributions of the LTSD as a function of the long-term window length and the misclassification errors of speech and non-speech segments.2.1.Definitions of the LTSE and LTSDLet xðnÞbe a noisy speech signal that is seg-mented into overlapped frames and,Xðk;lÞits amplitude spectrum for the k band at frame l.The N-order long-term spectral envelope is defined as LTSE Nðk;lÞ¼max f Xðk;lþjÞg j¼þNj¼ÀNð1ÞThe N-order long-term spectral divergence be-tween speech and noise is defined as the deviation of the LTSE respect to the average noise spec-trum magnitude NðkÞfor the k band,k¼0;1;...;NFFTÀ1,and is given byLTSD NðlÞ¼10log101NFFTXNFFTÀ1k¼0LTSE2ðk;lÞN2ðkÞ!ð2ÞIt will be shown in the rest of the paper that the LTSD is a robust feature defined as a long-term spectral distance measure between speech and noise.It will also be demonstrated that using long-term speech information increases the speech detection robustness in adverse environments and,274J.Ram ırez et al./Speech Communication42(2004)271–287when compared to VAD algorithms based on instantaneous measures of the SNR level,it will enable formulating noise robust decision rules with improved speech/non-speech discrimination.2.2.LTSD distributions of speech and silenceIn this section we study the distributions of the LTSD as a function of the long-term window length(N)in order to clarify the motivations for the algorithm proposed.A hand-labelled version of the Spanish SDC database was used in the analysis.This database contains recordings from close-talking and distant microphones at different driving conditions:(a)stopped car,motor run-ning,(b)town traffic,low speed,rough road and (c)high speed,good road.The most unfavourable noise environment(i.e.high speed,good road)was selected and recordings from the distant micro-phone were considered.Thus,the N-order LTSD was measured during speech and non-speech periods,and the histogram and probability distri-butions were built.The8kHz input signal was decomposed into overlapping frames with a10-ms window shift.Fig.1shows the LTSD distributions of speech and noise for N¼0,3,6and9.It is derived from Fig.1that speech and noise distri-butions are better separated when increasing the order of the long-term window.The noise is highly confined and exhibits a reduced variance,thus leading to high non-speech hit rates.This fact can be corroborated by calculating the classification error of speech and noise for an optimal Bayes classifier.Fig.2shows the classification errors as a function of the window length N.The speech classification error is approximately reduced by half from22%to9%when the order of the VAD is increased from0to6frames.This is motivated by the separation of the LTSD distributions that takes place when N is increased as shown in Fig.1. On the other hand,the increased speech detection robustness is only prejudiced by a moderate in-crease in the speech detection error.According to Fig.2,the optimal value of the order of the VADJ.Ram ırez et al./Speech Communication42(2004)271–287275would be N¼6.As a conclusion,the use of long-term spectral divergence is beneficial for VAD since it reduces importantly misclassification er-rors.2.3.Definition of the LTSD VAD algorithmAflowchart diagram of the proposed VADalgorithm is shown in Fig.3.The algorithm can be described as follows.During a short initialization period,the mean noise spectrum NðkÞ(k¼0;1;...;NFFTÀ1)is estimated by averaging the noise spectrum magnitude.After the initialization period,the LTSE VAD algorithm decomposes the input utterance into overlapped frames being their spectrum,namely Xðk;lÞ,processed by means of a ð2Nþ1Þ-frame window.The LTSD is obtained by computing the LTSE by means of Eq.(1).The VAD decision rule is based on the LTSD calcu-lated using Eq.(2)as the deviation of the LTSE with respect to the noise spectrum.Thus,the algorithm has an N-frame delay since it makes a decision for the l-th frame using a(2Nþ1)-frame window around the l-th frame.On the other hand, thefirst N frames of each utterance are assumed to be non-speech periods being used for the initiali-zation of the algorithm.The LTSD defined by Eq.(2)is a biased mag-nitude and needs to be compensated by a given offset.This value depends on the noise spectral variance and the order of the VAD and can be estimated during the initialization period or as-sumed to take afixed value.The VAD makes the SND by comparing the unbiased LTSD to an adaptive threshold c.The detection threshold is adapted to the observed noise energy E.It is as-sumed that the system will work at different noisy conditions characterized by the energy of the background noise.Optimal thresholds c0and c1 can be determined for the system working in the cleanest and noisiest conditions.These thresholds define a linear VAD calibration curve that is used during the initialization period for selecting an adequate threshold c as a function of the noise energy E:c¼cE6E0c0Àc1E0ÀE1Eþc0Àc0Àc11ÀE1=E0E0<E<E1c1E P E18><>:ð3Þwhere E0and E1are the energies of the back-ground noise for the cleanest and noisiest condi-276J.Ram ırez et al./Speech Communication42(2004)271–287tions that can be determined examining the speech databases being used.A high speech/non-speech discrimination is ensured with this model since silence detection is improved at high and medium SNR levels while maintaining a high precision detecting speech periods under high noise condi-tions.The VAD is defined to be adaptive to time-varying noise environments with the following algorithm for updating the noise spectrum NðkÞduring non-speech periods being used:Nðk;lÞ¼a Nðk;lÀ1Þþð1ÀaÞN KðkÞif speech pause is detectedNðk;lÀ1Þotherwise8>><>>:ð4Þwhere N K is the average spectrum magnitude over a K-frame neighbourhood:N KðkÞ¼12Kþ1X Kj¼ÀKXðk;lþjÞð5ÞFinally,a hangover was found to be beneficial to maintain a high accuracy detecting speech periods at low SNR levels.Thus,the VAD delays the speech to non-speech transition in order to prevent low-energy word endings being misclassified as silence.On the other hand,if the LTSD achieves a given threshold LTSD0the hangover mechanism is turned offto improve non-speech detection when the noise level is low.Thus,the LTSE VAD yields an excellent classification of speech and pause periods.Examples of the operation of the LTSE VAD on an utterance of the Spanish SDC data-base are shown in Fig.4a(N¼6)and Fig.4b (N¼0).The use of a long-term window for for-mulating the decision rule reports quantifiable benefits in speech/non-speech detection.It can be seen that using a6-frame window reduces the variability of the LTSD in the absence of speech, thus yielding to reduced noise variance and better speech/non-speech discrimination.Speech detec-tion is not affected by the smoothing process in-volved in the long-term spectral estimation algorithm and maintains good margins that cor-rectly separate speech and pauses.On the other hand,the inherent anticipation of the VAD deci-sion contributes to reduce speech clipping errors.3.Experimental frameworkSeveral experiments are commonly conducted to evaluate the performance of VAD algorithms. The analysis is normally focused on the deter-mination of misclassification errors at different SNR levels(Beritelli et al.,2002;Marzinzik and Kollmeier,2002),and the influence of the VAD zdecision on speech processing systems(Bouquin-Jeannes and Faucon,1995;Karray and Martin, 2003).The experimental framework and the objective performance tests conducted to evaluate the proposed algorithm are described in this sec-tion.3.1.Speech/non-speech discrimination analysisFirst,the proposed VAD was evaluated in terms of the ability to discriminate between speech and pause periods at different SNR levels.The original AURORA-2database(Hirsch and Pe-arce,2000)was used in this analysis since it uses the clean TIdigits database consisting of sequences of up to seven connected digits spoken by Ameri-can English talkers as source speech,and a selec-tion of eight different real-world noises that have been artificially added to the speech at SNRs of20, 15,10,5,0and)5dB.These noisy signals have been recorded at different places(suburban train, crowd of people(babble),car,exhibition hall, restaurant,street,airport and train station),and were selected to represent the most probable application scenarios for telecommunication ter-minals.In the discrimination analysis,the clean TIdigits database was used to manually label each utterance as speech or non-speech frames for ref-erence.Detection performance as a function of the SNR was assessed in terms of the non-speech hit-rate(HR0)and the speech hit-rate(HR1)defined as the fraction of all actual pause or speech frames that are correctly detected as pause or speech frames,respectively:HR0¼N0;0NHR1¼N1;1N1ð6Þwhere N refand N ref1are the number of real non-speech and speech frames in the whole database,J.Ram ırez et al./Speech Communication42(2004)271–287277respectively,while N0;0and N1;1are the number of non-speech and speech frames correctly classified.The LTSE VAD decomposes the input signal sample at8kHz into overlapping frames with a 10-ms shift.Thus,a13-frame long-term window and NFFT¼256was found to be good choices for the noise conditions being studied.Optimal detection threshold c0¼6dB and c1¼2:5dB278J.Ram ırez et al./Speech Communication42(2004)271–287were determined for clean and noisy conditions, respectively,while the threshold calibration curve was defined between E0¼30dB(low noise energy) and E1¼50dB(high noise energy).The hangover mechanism delays the speech to non-speech VAD transition during8frames while it is deactivated when the LTSD exceeds25dB.The offset isfixed and equal to5dB.Finally,it is used a forgotten factor a¼0:95,and a3-frame neighbourhood (K¼3)for the noise update algorithm.Fig.5provides the results of this analysis and compares the proposed LTSE VAD algorithm to standard G.729,AMR and AFE VADs in terms of non-speech hit-rate(Fig.5a)and speech hit-rate (Fig.5b)for clean conditions and SNR levels ranging from20to)5dB.Note that results for the two VADs defined in the AFE DSR standard (ETSI,2002)for estimating the noise spectrum in the Wienerfiltering stage and non-speech frame-dropping are provided.Note that the results shown in Fig.5are averaged values for the entire set of noises.Thus,the following conclusions can be derived from Fig.5about the behaviour of the different VADs analysed:(i)G.729VAD suffers poor speech detection accu-racy with the increasing noise level while non-speech detection is good in clean conditions (85%)and poor(20%)in noisy conditions. (ii)AMR1yields an extreme conservative behav-iour with high speech detection accuracy for the whole range of SNR levels but very poor non-speech detection results at increasing noise levels.Although AMR1seems to be well suited for speech detection at unfavourable noise conditions,its extremely conservative behaviour degrades its non-speech detection accuracy being HR0less than10%below10 dB,making it less useful in a practical speech processing system.(iii)AMR2leads to considerable improvements over G.729and AMR1yielding better non-speech detection accuracy while still suffering fast degradation of the speech detection abil-ity at unfavourable noisy conditions.(iv)The VAD used in the AFE standard for esti-mating the noise spectrum in the Wienerfilter-ing stage is based in the full energy band and yields a poor speech detection performance with a fast decay of the speech hit-rate at low SNR values.On the other hand,the VAD used in the AFE for frame-dropping achieves a high accuracy in speech detection but moderate results in non-speech detection. (v)LTSE achieves the best compromise among the different VADs tested.It obtains a good behaviour in detecting non-speech periods as well as exhibits a slow decay in performance at unfavourable noise conditions in speech detection.Table1summarizes the advantages provided by the LTSE-based VAD over the different VAD methods being evaluated by comparing them in terms of the average speech/non-speech hit-rates. LTSE yields a47.28%HR0average value,while the G.729,AMR1,AMR2,WF and FD AFE VADs yield31.77%,31.31%,42.77%,57.68%and 28.74%,respectively.On the other hand,LTSE attains a98.15%HR1average value in speechJ.Ram ırez et al./Speech Communication42(2004)271–287279detection while G.729,AMR1,AMR2,WF and FD AFE VADs provide93.00%,98.18%,93.76%, 88.72%and97.70%,respectively.Frequently VADs avoid losing speech periods leading to an extremely conservative behaviour in detecting speech pauses(for instance,the AMR1VAD). Thus,in order to correctly describe the VAD performance,both parameters have to be consid-ered.Thus,considering together speech and non-speech hit-rates,the proposed VAD yielded the best results when compared to the most represen-tative VADs analysed.3.2.Receiver operating characteristic curvesAn additional test was conducted to compare speech detection performance by means of the ROC curves(Madisetti and Williams,1999),a frequently used methodology in communications based on the hit and error detection probabilities (Marzinzik and Kollmeier,2002),that completely describes the VAD error rate.The AURORA subset of the original Spanish SDC database (Moreno et al.,2000)was used in this analysis. This database contains4914recordings using close-talking and distant microphones from more than160speakers.As in the whole SDC database, thefiles are categorized into three noisy condi-tions:quiet,low noisy and highly noisy conditions, which represent different driving conditions and average SNR values of12,9and5dB.The non-speech hit rate(HR0)and the false alarm rate(FAR0¼100)HR1)were determined in each noise condition for the proposed LTSE VAD and the G.729,AMR1,AMR2,and AFE VADs,which were used as a reference.For the calculation of the false-alarm rate as well as the hit rate,the‘‘real’’speech frames and‘‘real’’speech pauses were determined by hand-labelling the database on the close-talking microphone.The non-speech hit rate(HR0)as a function of the false alarm rate(FAR0¼100)HR1)for 0<c610dB is shown in Fig.6for recordings from the distant microphone in quiet,low and high noisy conditions.The working point of the adaptive LTSE,G.729,AMR and the recently approved AFE VADs(ETSI,2002)are also in-cluded.It can be derived from these plots that: (i)The working point of the G.729VAD shifts tothe right in the ROC space with decreasing SNR,while the proposed algorithm is less af-fected by the increasing level of background noise.(ii)AMR1VAD works on a low false alarm rate point of the ROC space but it exhibits poor non-speech hit rate.(iii)AMR2VAD yields clear advantages over G.729and AMR1exhibiting important reduction in the false alarm rate when com-pared to G.729and increase in the non-speech hit rate over AMR1.(iv)WF AFE VAD yields good non-speech detec-tion accuracy but works on a high false alarm rate point on the ROC space.It suffers rapid performance degradation when the driving conditions get noisier.On the other hand, FD AFE VAD has been planned to be conser-vative since it is only used in the DSR stan-dard for frame-dropping.Thus,it exhibits poor non-speech detection accuracy working on a low false alarm rate point of the ROC space.(v)LTSE VAD yields the lowest false alarm rate for afixed non-speech hit rate and also,the highest non-speech hit rate for a given false alarm rate.The ability of the adaptive LTSE VAD to tune the detection threshold by means the algorithm described in Eq.(3)en-ables working on the optimal point of the ROC curve for different noisy conditions.Thus,the algorithm automatically selects theTable1Average speech/non-speech hit rates for SNR levels ranging from clean conditions to)5dBVADG .729AMR1AMR2AFE(WF)AFE(FD)LTSEHR0(%)31.7731.3142.7757.6828.7447.28 HR1(%)93.0098.1893.7688.7297.7098.15 280J.Ram ırez et al./Speech Communication42(2004)271–287。
专业音频术语中英文对照

专业⾳频术语中英⽂对照专业⾳频术语中英⽂对照使⽤⽅法按下ctrl+F 即可进⾏查找AAC automatic ampltiude control ⾃动幅度控制AB AB制⽴体声录⾳法Abeyancd 暂停,潜态A-B repeat A-B重复ABS absolute 绝对的,完全的,绝对时间ABS american bureau of standard 美国标准局ABSS auto blank secrion scanning ⾃动磁带空⽩部分扫描Abstime 绝对运⾏时间A.DEF audio defeat ⾳频降噪,噪声抑制,伴⾳静噪ADJ adjective 附属的,附件ADJ Adjust 调节ADJ acoustic delay line 声延迟线Admission 允许进⼊,供给ADP acoustic data processor ⾳响数据处理机ADP(T) adapter 延配器,转接器ADRES automatic dynamic range expansion system动态范围扩展系统ADRM analog to digital remaster模拟录⾳、数字处理数码唱盘ADS audio distribution system ⾳频分配系统A.DUB audio dubbing 配⾳,⾳频复制,后期录⾳ADV advance 送⼊,提升,前置量ADV adversum 对抗ADV advancer 相位超前补偿器Adventure 惊险效果AE audio erasing ⾳频(声⾳)擦除AE auxiliary equipment 辅助设备Aerial 天线AES audio engineering society 美国声频⼯程协会AF audio fidelity ⾳频保真度AF audio frequency ⾳频频率AFC active field control ⾃动频率控制AFC automatic frequency control 声场控制Affricate 塞擦⾳AFL aside fade listen 衰减后(推⼦后)监听A-fader ⾳频衰减AFM advance frequency modulation ⾼级调频AFS acoustic feedback speaker 声反馈扬声器AFT automatic fine tuning ⾃动微调AFTAAS advanced fast time acoustic analysis system⾼级快速⾳响分析系统After 转移部分⽂件Afterglow 余辉,⼣照时分⾳响效果Against 以……为背景AGC automatic gain control ⾃动增益控制AHD audio high density ⾳频⾼密度唱⽚系统AI advanced integrated 预汇流AI amplifier input 放⼤器输⼊AI artificial intelligence ⼈⼯智能AI azimuth indicator ⽅位指⽰器A-IN ⾳频输⼊A-INSEL audio input selection ⾳频输⼊选择Alarm 警报器ALC automatic level control ⾃动电平控制ALC automatic load control⾃动负载控制Alford loop 爱福特环形天线Algorithm 演⽰Aliasing 量化噪声,频谱混叠Aliasing distortion 折叠失真Align alignment 校正,补偿,微调,匹配Al-Si-Fe alloy head 铁硅铝合⾦磁头Allegretto ⼩快板,稍快地Allegro 快板,迅速地Allocation 配置,定位All rating 全(⾳)域ALM audio level meter ⾳频电平表ALT alternating 震荡,交替的ALT alternator 交流发电机ALT altertue 转路ALT-CH alternate channel 转换通道,交替声道Alter 转换,交流电,变换器AM amperemeter 安培计,电流表AM amplitude modulation 调幅(⼴播)AM auxiliary memory 辅助存储器Ambience 临场感,环绕感ABTD automatic bulk tape degausser磁带⾃动整体去磁电路Ambient 环境的Ambiophonic system 环绕声系统Ambiophony 现场混响,环境⽴体声AMLS automatic music locate system⾃动⾳乐定位系统AMP ampere 安培AMP amplifier 放⼤器AMPL amplification 放⼤AMP amplitude 幅度,距离Amorphous head ⾮晶态磁头Abort 终⽌,停⽌(录制或播放)A-B TEST AB⽐较试听Absorber 减震器Absorption 声⾳被物体吸收ABX acoustic bass extension 低⾳扩展AC accumulator 充电电池AC adjustment caliration 调节-校准AC alternating current 交流电,交流AC audio coding 数码声,⾳频编码AC audio center ⾳频中⼼AC azimuth comprator ⽅位⽐较器AC-3 杜⽐数码环绕声系统AC-3 RF 杜⽐数码环绕声数据流(接⼝)ACC Acceleration 加速Accel 渐快,加速Accent 重⾳,声调Accentuator 预加重电路Access 存取,进⼊,增加,通路Accessory 附件(接⼝),配件Acryl 丙基酰基Accompaniment 伴奏,合奏,伴随Accord 和谐,调和Accordion ⼿风琴ACD automatic call distributor ⾃动呼叫分配器ACE audio control erasing ⾳频控制消磁A-Channel A(左)声道Acoumeter 测听计Acoustical 声的,声⾳的Acoustic coloring 声染⾊Acoustic image 声像Across 交叉,并⾏,跨接Across frequency 交叉频率,分频频率ACST access time 存取时间Active 主动的,有源的,有效的,运⾏的Active crossover 主动分频,电⼦分频,有源分频Active loudsperker 有源⾳箱Armstrong MOD 阿姆斯特朗调制ARP azimuth reference pulse ⽅位基准脉冲Arpeggio 琶⾳Articulation 声⾳清晰度,发⾳Artificial 仿……的,⼈⼯的,⼿动(控制)AAD active acoustic devide 有源声学软件ABC auto base and chord ⾃动低⾳合弦Architectural acoustics 建筑声学Arm motor 唱臂唱机Arpeggio single 琶⾳和弦,分解和弦ARL aerial 天线ASC automatic sensitivity control ⾃动灵敏度控制ASGN Assign 分配,指定,设定ASP audio signal processing ⾳频信号处理ASS assembly 组件,装配,总成ASSEM assemble 汇编,剪辑ASSEM Assembly 组件,装配,总成Assign 指定,转发,分配Assist 辅助(装置)ASSY accessory 组件,附件AST active servo techonology 有源伺服技术Automation:⾃动化A Tempo 回到原速Astigmatism methord 象散法B band 频带B Bit ⽐特,存储单元B Button 按钮Babble 多路感应的复杂失真Back 返回Back clamping 反向钳位Back drop 交流哼声,⼲扰声Background noise 背景噪声,本底噪声Backing copy 副版Backoff 倒扣,补偿Back tracking 补录Back up 磁带备份,⽀持,预备Backward 快倒搜索Baffle box ⾳箱BAL balance 平衡,⽴体声左右声道⾳量⽐例,平衡连接Balanced 已平衡的Balancing 调零装置,补偿,中和Balun 平衡=不平衡转换器Banana jack ⾹蕉插头Banana bin ⾹蕉插座Banana pin ⾹蕉插头Banana plug ⾹蕉插头Band 频段,Band pass 带通滤波器Bandwidth 频带宽,误差,范围Band 存储单元Bar ⼩节,拉杆BAR barye 微巴Bargraph 线条Barrier 绝缘(套)Base 低⾳Bass 低⾳,倍司(低⾳提琴)Bass tube 低⾳号,⼤号Bassy 低⾳加重BA TT battery 电池Baud 波特(信息传输速率的单位)Bazooka 导线平衡转接器BB base band 基带BBD Bucket brigade device 戽链器件(效果器)B BAT Battery 电池BBE 特指BBE公司设计的改善较⾼次谐波校正程度的系统BC balanced current 平衡电流BC Broadcast control ⼴播控制BCH band chorus 分频段合唱BCST broadcast (⽆线电)⼴播BD board 仪表板Beat 拍,脉动信号Beat cancel switch 差拍⼲扰消除开关Bel 贝尔Below 下列,向下Bench ⼯作台Bend 弯曲,滑⾳Bender 滑⾳器BER bit error rate 信息差错率BF back feed 反馈BF Backfeed flanger 反馈镶边BF Band filter 带通滤波器BGM background music 背景⾳乐Bias 偏置,偏磁,偏压,既定程序Bidirectional 双向性的,8字型指向的Bifess Bi-feedback sound system 双反馈系统Big bottom 低⾳扩展,加重低⾳Bin 接收器,仓室BNG BNC连接器(插头、插座),卡⼝同轴电缆连接器Binaural effect 双⽿效应,⽴体声Binaural synthesis 双⽿合成法Bin go 意外现象Bit binary digit 字节,⼆进制数字,位Bitstream 数码流,⽐特流Bit yield 存储单元Bi-AMP 双(通道)功放系统Bi-wire 双线(传输、分⾳)Bi-Wring 双线BK break 停顿,间断BKR breaker 断电器Blamp 两路电⼦分⾳Blanking 关闭,消隐,断路Blaster 爆裂效果器Blend 融合(度)、调和、混合Block 分程序,联动,中断Block Repeat 分段重复Bloop (磁带的)接头噪声,消⾳贴⽚BNC bayonet connector 卡⼝电缆连接器Body mike ⼩型话筒Bond 接头,连接器Bongo 双⿎Boom 混响,轰鸣声Boomy 嗡嗡声(指低⾳过强)Boost 提升(⼀般指低⾳),放⼤,增强Booth 控制室,录⾳棚Bootstrap 辅助程序,⾃举电路Both sides play disc stereo system 双⾯演奏式唱⽚⽴体声系统Bottoming 底部切除,末端切除Bounce 合并Bourclon 单调低⾳Bowl 碗状体育场效果BP bridge bypass 电桥旁路BY bypass 旁通BPC basic pulse generator 基准脉冲发⽣器BPF band pass filter 带通滤波器BPS band pitch shift 分频段变调节器BNC bayonet connector 卡⼝电缆连接器Body mike ⼩型话筒Bond 接头,连接器Bongo 双⿎Boom 混响,轰鸣声Boomy 嗡嗡声(指低⾳过强)Boost 提升(⼀般指低⾳),放⼤,增强Booth 控制室,录⾳棚Bootstrap 辅助程序,⾃举电路Bottoming 底部切除,末端切除Bounce 合并Bourclon 单调低⾳Bowl 碗状体育场效果BP bridge bypass 电桥旁路BPC basic pulse generator 基准脉冲发⽣器BPF band pass filter 带通滤波器BPS band pitch shift 分频段变调节器BR bregister 变址寄存器BR Bridge 电桥Break 中⽌(程序),减弱Breathing 喘息效应B.Reso base resolve 基本解析度Bridge 桥接,电桥,桥,(乐曲的)变奏过渡Bright 明亮(感)Brightness 明亮度,指中⾼⾳听⾳感觉Brilliance 响亮BRKRS breakers 断路器Broadcast ⼴播BTB bass tuba 低⾳⼤喇叭BTL balanced transformer-less 桥式推挽放⼤电路BTM bottom 最⼩,低⾳BU backup nuit 备⽤器件Bumper 减震器Bus 母线,总线Busbar 母线Buss 母线Busy 占线BUT button 按钮,旋钮BW band width 频带宽度,带度BYP bypass 旁路By path 旁路BZ buzzer 蜂⾳器C cathode 阴极,负极C Cell 电池C Center 中⼼C Clear 清除C Cold 冷(端)CA cable 电缆Cable 电缆Cabinet ⼩操纵台CAC coherent acoustic coding 相⼲声学编码Cache 缓冲存储器Cal calando 减⼩⾳量CAL Calendar 分类CAL Caliber ⼝径CAL Calibrate 标准化CAL Continuity accept limit 连续性接受极限Calibrate 校准,定标Call 取回,复出,呼出Can 监听⽿机,带盒CANCL cancel 删除CANCL Cancelling 消除Cancel 取消Cannon 卡侬接⼝Canon 规则Cap 电容Capacitance Mic 电容话筒Capacity 功率,电容量CAR carrier 载波,⽀座,鸡⼼夹头Card 程序单,插件板Cardioid ⼼型的CA TV cable television 有线电视Crispness 脆声Category 种类,类型Cartridge 软件卡,拾⾳头Carrkioid ⼼型话筒Carrier 载波器Cart 转运Cartridge 盒式存储器,盒式磁带Cascade 串联Cassette 卡式的,盒式的CA V constant angular velocity 恒⾓速度Caution 报警CBR circuit board rack 电路板架CC contour correction 轮廓校正CCD charge coupled device 电荷耦合器件CD compact disc 激光唱⽚CDA current dumping amplifier 电流放⼤器CD-E compact disc erasable 可抹式激光唱⽚CDG compact-disc plus graphic 带有静⽌图像的CD唱盘CD constant directional horn 恒定指向号⾓CDV compact disc with video 密纹声像唱⽚CE ceramic 陶瓷Clock enable 时钟启动Cell 电池,元件,单元Cellar club 地下俱乐部效果Cello ⼤提琴CEMA consumer electronics manufacturer?sassociation (美国)消费电⼦产品制造商协会CENELEC connector 欧洲标准21脚A V连接器Cent ⾳分Central earth 中⼼接地CES consumer electronic show(美国)消费电⼦产品展览会CF center frequency 中⼼频率Cross fade 软切换CH channel 声道,通道Chain 传输链,信道Chain play 连续演奏Chamber 密⾳⾳响效果,消声室CHAN channel 通道Change 交换Chapter 曲⽬Chaper skip 跳节CHAE character 字符,符号Characteristic curve 特性曲线Charge 充电Charger 充电器Chase 跟踪Check 校验CHC charge 充电CH - off 通道切断Choke 合唱Choose 选择Chromatic ⾊彩,半⾳Church 教堂⾳响效果CI cut in 切⼊CIC cross interleave code 交叉隔⾏编码CIRC circulate 循环Circuit 电路CL cancel 取消Classic 古典的Clean 净化CLR clear 归零Click 嘀哒声Clip 削波,限幅,接线柱CLK clock 时钟信号Close 关闭,停⽌CLS 控制室监听Cluster ⾳箱阵效果CLV ceiling limit value 上限值CMP compact 压缩CMPT compatibility 兼容性CMRR common mode rejection ratio 共模抑制⽐CNT count 记数,记数器CNTRL central 中⼼,中央CO carry out 定位输出Coarse 粗调Coax 同轴电缆Coaxial 数码同轴接⼝Code 码,编码Coefficient 系数Coincident 多信号同步Cold 冷的,单薄的Color 染⾊效果COM comb 梳状(滤波)COMB combination 组合⾳⾊COMBI combination 组合,混合COMBO combination 配合,组合Combining 集合,结合COMM communication 换向的,切换装置Command 指令,操作,信号COMMON 公共的,公共地端Communieation speed 通讯速度选择COMP comparator ⽐较器COMP compensate 补偿Compact 压缩Compander 压缩扩展器Compare ⽐拟Compatibility 兼容Compensate 补偿Complex 全套设备Copmoser 创意者,作曲者Compressor 压缩器COMP-EXP 压扩器Compromise (频率)平衡Computer 计算机,电脑CON concentric cable 同轴电缆CON console 操纵台CON controller 控制器Concentric 同轴的,同⼼的Concert ⾳乐厅效果Condenser Microphone 电容话筒Cone type 锥形(扬声器)CONFIG 布局,线路接法Connect 连接,联络CORR correct 校正,补偿,抵消Configuration 线路布局Confirmation 确认Consent 万能插座Console 调⾳台Consonant 辅⾳Constant 常数CONT continuous 连续的(⾳⾊特性)CONT control 控制,操纵Contact 接触器Content 内容Continue 连续,继续Continue button 两录⾳卡座连续放⾳键Contour 外形,轮廓,保持Contra 次⼋度Contrast 对⽐度Contribution 分配Controlled 可控的Controller 控制器CONV conventional 常规的CONV convert 变换CONV convertible 可转换的Copy 复制Correlation meter 相关表Coupler 耦合Cover 补偿Coverage 有效范围CP clock pulse 时钟脉冲CP control program 控制程序CPU 中央处理器CR card reader 卡⽚阅读机CRC cyclic redundancy check 循环冗余校验Create 建⽴,创造Crescendo 渐强或渐弱Crispness 清脆感CRM control room 控制室CROM control read only memory 控制只读存储器Crossfader 交叉渐变器Cross-MOD 交叉调制Crossover 分频器,换向,切断Cross talk 声道串扰,串⾳Crunch 摩擦⾳C/S cycle/second 周/秒CSS content scrambling system 内容加密系统CST case style tape 盒式磁带CT current 电流CTM close talking microphone 近讲话筒CU counting unit 计数单元Cue 提⽰,选听Cue clock 故障计时钟Cueing 提⽰,指出Cursor 指⽰器,光标Curve (特性)曲线Custom 常规CUT 切去,硬切换D double 双重的,对偶的D drum ⿎,磁⿎DA delayed action 延迟作⽤D/Adigital/analog 数字/模拟DAB digital audio broadcasting 数字⾳频⼴播Damp 阻尼DASH digital audio stationar head 数字固定磁头Dashpot 缓冲器,减震器DAT digital audio tape 数字⾳频磁带,数字录⾳机DA TA 数据DA TAtron 数据处理机DA TE ⽇期DB(dB) decibel 分贝DB distribution 分线盒DBA decibel asolute 绝对分贝DBA decibel adjusted 调整分贝DBB dynamic bass boost 动态低⾳提升DBK decibels referred to one kilowatt 千⽡分贝DBm decibel above one milliwatt in 600 ohms 毫⽡分贝DBS direct broadcast satellite 直播卫星DBX 压缩扩展式降噪系统DC distance controlled 遥控器DCA digital command assembly 数字指令装置DCE data circuit terminating equipment数据通讯线路终端设备DCF digital comb filter 数字梳状滤波器DCH decade chorus ⼗声部合唱DCP date central processor 数据中⼼处理器DD direct drive 直接驱动DD dolby digital 数字杜⽐DDC direct digital control 直接数字控制DDS digital dynamic sound 数字动态声DDT data definition table 数据定义表Dead 具有强吸声特性的房间的静寂DEC decay 衰减,渐弱,余⾳效果Decibel 分贝Deck 卡座,录⾳座,带⽀加的,⾛带机构Deemphasis 释放Deep reverb 纵深混响De-esser 去咝声器DEF defeat 消隐,静噪Delete 删除Delivery end 输⼊端DEMO demodulator 解调器Demo ⾃动演奏Demoder 解码器Density 密度,声⾳密度效果Detune ⾳⾼微调,去谐DepFin 纵深微调Depth 深度Denoiser 降噪器Design 设计Destroyer 抑制器DET detector 检波器Deutlichkeit 清晰度DEV device 装置,仪器DEX dynamic exciter 动态激励器DF damping factor 动态滤波器DFL dynamic filter 动态滤波DFS digital frequency synthesizer 数字频率合成器DI data input 数据输⼊Diagram 图形,原理图Dial 调节度盘Difference 不同,差别DIFF differential 差动Diffraction 衍射,绕射Diffuse 传播Diffusion 扩散DIG digit 数字式Digital 数字的,数字式,计数的Digitalyier 数字化装置DIM digital input module 数字输⼊模块DIM diminished 衰减,减半⾳Dimension 范围,密度,尺⼨,(空间)维,声像宽度Din 五芯插⼝(德国⼯业标准)DIN digital input 数字输⼊DIR direct 直接的,(调⾳台)直接输出,定向的Direct box 指令盒,控制盒Direct sound 直达声Directory ⽬录Direction 配置⽅式Directional ⽅向,指向的Directivity ⽅向性DIS display 显⽰器DISC disconnect 切断,开路DISC discriminator 鉴相器Disc 唱盘,唱⽚,碟Disc holder 唱⽚抽屉Disc recorder 盘⽚式录⾳机Dischage 释放,解除Disco 迪斯科,迪斯科⾳乐效果Discord 不谐和弦Disk 唱盘,碟DISP display 显⽰器,显⽰屏Dispersion 频散特性,声⾳分布Displacement 偏转,代换Distortion 失真,畸变DIST distance 距离,间距DIST district 区间Distributer 分配器,导向装置DITEC digital television camera 数字电视摄像机Dim 变弱,变暗,衰减DIV divergence 发散DIV division 分段DIV divisor 分配器Diversity 分集(接收)Divider 分配器Divx 美国数字视频快递公司开发的⼀种每次观看付费的DVDDJ Disc Jocker 唱⽚骑⼠DJ dust jacket 防尘罩DJ delay 延迟DLD dynamic linear drive 动态线性驱动DLLD direct linear loop detector 直接线性环路检波器DME digital multiple effector 数字综合效果器DMS date multiplexing system 数据多路传输系统DMS digital multiplexing synchronizer数字多路传输同步器DMX data multiplex 数据多路(传输)DNL dynamic noise limiter 动态噪声抑制器DNR dynamic noise reduction 动态降噪电路DO dolly out 后移DO dropout 信号失落DOB dolby 杜⽐DOL dynamic optimum loudness 动态最佳响度Dolby 杜⽐,杜⽐功能Dolby Hx Pro dolby Hx pro headroom extension system杜⽐Hx Pro动态余量扩展系统Dolby NR 杜⽐降噪Dolby Pro-logic 杜⽐定向逻辑Dolby SR-D dolby SR digital 杜⽐数字频谱记录Dolby Surround 杜⽐环绕Dome loudspeaker 球顶扬声器Dome type 球顶(扬声器)DOP doppler 多普勒(响应)Double 加倍,双,次⼋度Doubler 倍频器,加倍器Double speed 倍速复制D.OUT direct output 直接输出Down 向下,向下调整,下移,减少DPCM differential pulse code modulation 差动脉冲调制DPD direct pure MPX decoder 直接纯多路解调器DPL dolby pro logic 杜⽐定向逻辑DPL duplex 双⼯,双联DPLR doppler 多普勒(系统)D.Poher effect 德.波埃效应Dr displacement corrector 位移校准器,同步机DR distributor 分配器DR drum 磁⿎Drain 漏电,漏极DRAM direct read after write ⼀次性读写存储器Drama 剧场效果DRAW 只读追忆型光盘Dr.Beat 取字时间校准器DRCN dynamic range compression and normalization动态范围压缩和归⼀化Drive 驱动,激励Dr.Rhythm 节奏同步校准器DRPS digital random program selector数字式节⽬随机选择器DDrum ⿎Drum machine ⿎机Dry ⼲,⽆效果声,直达声DS distortion 失真DSC digital signal converter 数字信号转换器DSL dynamic super loudness 低⾳动态超响度,重低⾳恢复DSM dynamic scan modulation 动态扫描速度调制器DSP digital signal processor 数字信号处理器DSP display simulation program 显⽰模拟程序DSP digital sound processor 数字声⾳处理器DSP digital sound field processor 数字声场处理器DSP dynamic speaker 电动式扬声器DSS digital satellite system 数字卫星系统DT data terminal 数据终端DT data transmission 数据传输DTL direct to line 直接去线路DTS digital theater system 数字影剧院系统DTS digital tuning system 数字调谐系统DTV digital television 数字电视Dual 对偶,双重,双Dub 复制,配⾳,拷贝,转录磁带Dubbing mixer 混录调⾳台Duck 按⼊,进⼊Dummyload 假负载DUP Duplicate 复制(品)Duplicator 复制装置,增倍器Duration 持续时间,宽度Duty 负载,作⽤范围,功率Duty cycle 占空系数,频宽⽐DUX duplex 双⼯DV device 装置,器件DVC digital video cassette 数字录象带DVD digital video disc 数字激光视盘DX 天线收发开关,双重的,双向的DYN dynamic 电动式的,动态范围,动圈式的Dynamic filter 动态滤波(特殊效果处理)器Dynamic Microphone 动圈话筒Dynamic range 动态范围Dynode 电⼦倍增器电极early warning 预警E earth 真地,接地E error 错误,差错(故障显⽰)EA earth 地线,真地EAR early 早期(反射声)Earphone ⽿机Earth terminal 接地端EASE electro-acooustic simulators for engineers⼯程师⽤电声模拟器,计算机电声与声学设计软件Eat 收取信号EBU european broadcasting union 欧洲⼴播联盟EC error correction 误差校正ECD electrochomeric display 电致变⾊显⽰器Echo 回声,回声效果,混响ECL extension zcompact limitter 扩展压缩限制器ECM electret condenser microphone 驻极体话筒ECSL equivalent continuous sound level 等级连续声级ECT electronec controlled transmission 电控传输ED edit editor 编辑,编辑器Edit 编辑Edge tone 边棱⾳EDTV enhanced definition television增强清晰度电视(⼀种可兼容⾼清晰度电视)E-DRAW erasable direct after write 可存可抹读写存储器EE errors excepted 允许误差EFF effect efficiency 效果,作⽤Effector 操纵装置,效果器Effects generator 效果发⽣器EFM 8/14位调制法EFX effect 效果EG envelope generator 包络发⽣器EIA electronec industries association(美国)电⼦⼯业协会EIAJ electronic industries association Japan ⽇本电⼦⼯业协会EIN einstein 量⼦摩尔(能量单位)EIN equivalent input noise 等效输⼊噪声EIO error in operation 操作码错误Eject 弹起舱门,取出磁带(光盘),出盒EL electro luminescence 场致发光ELAC electroacoustic 电声(器件)ELEC electret 驻极体Electret condenser microphone 驻极体话筒ELF extremely low frequency 极低频ELEC electronec 电⼦的Electroacoustics 电声学EMI electro magnetic interference 电磁⼲扰Emission 发射EMP emphasispo 加重EMP empty 空载Emphasis 加重EMS emergency switch 紧急开关Emulator 模拟器,仿真设备EN enabling 启动Enable 赋能,撤消禁⽌指令Encoding 编码End 末端,结束,终⽌Ending 终端,端接法,镶边ENG engineering ⼯程Engine 运⾏,使⽤ENG land ⼯程接地Enhance 增强,提⾼,提升ENS ensemble 合奏ENS envelope sensation 群感Eensemble 合奏Eensemble 合奏ENT enter 记录Enter 记⼊,进⼊,回车Entering 插⼊,记录Entry 输⼊数据,进⼊ENV envelope 包络线Envelopment 环绕感EOP electronic overload protection 电⼦过载保护EOP end of program 程序结束EOP end output 末端输出EOT end of tape 磁带尾端EP extend playing record 多曲⽬唱⽚EP extended play 长时间放录,密录EPG edit pulse generator 编辑脉冲发⽣器EPS emergency power supply 应急电源EQ equalizer 均衡器,均衡EQ equalization 均衡EQL equalization 均衡Equal-loudness contour 等响曲线Equipped 准备好的,已装备Equitonic 全⾳Equivalence 等效值ER erect 设置ER error 错误,误差ERA earphone ⽿机Eraser 抹去,消除Erasing 擦除,清洗Erasure 抹⾳Erase 消除,消Er early 早期的ERCD extended resolution CD 扩展解析度CDEREQ erect equalizer均衡器(频点)位置(点频补偿电路的中点频率)调整ERF early reflection 早期反射(声)Ernumber 早期反射声量Error 错误,出错,不正确ES earth swith 接地开关ES electrical stimulation 点激励Escqpe 退出ETER eternity ⽆限Euroscart 欧洲标准21脚A V连接器Event 事件EVF envelope follower包络跟随器(⾳响合成装置功能单元)EX exciter 激励器EX exchange 交换EX expanding 扩展EXB expanded bass 低⾳增强EXC exciter 激励器EXCH exchange 转换Exclusive 专⽤的Excursion 偏移,偏转,漂移,振幅EXP expender 扩展器,动态扩展器EXP export 输出Exponential horn tweeter 指数型⾼⾳号⾓扬声器Expression pedal 表达踏板(⽤于控制乐器或效果器的脚踏装置)EXT extend 扩展EXT exterior 外接的(设备)EXT external 外部的,外接的EXT extra 超过EXTN extension 扩展,延伸(程控装置功能单元)Extract 轨道提出EXTSN extension 扩展,延伸(程控装置功能单元)F fast 快(速)F feedback 反馈F forward 向前F foot 脚踏(装置)F frequency 频率F function 功能Ffactor 因⼦,因素,系数,因数Fade 衰减(⾳量控制单元)Fade in-out 淡⼊淡出,慢转换Fader 衰减器Fade up 平滑上升Failure 故障Fall 衰落,斜度Faraday shield 法拉第屏蔽,静电屏蔽FAS full automatic search 全⾃动搜索Fast 快速(⾃动演奏装置的速度调整钮)Fastener 接线柱,闭锁Fat 浑厚(⾳争调整钮)Fattens out 平直输出Fault 故障,损坏Fader 衰减器,调⾳台推拉电位器(推⼦)Fading in 渐显Fading out 渐显False 错误Fancier ⾳响发烧友Far field 远场FatEr 丰满的早期反射FB feedback 反馈,声反馈FB fuse block 熔丝盒F.B fiver by 清晰FBO feedback outrigger 反馈延伸FCC federal communications commission(美国)联邦通信委员会FD fade depth 衰减深度FD feed 馈⼊信号FDR fader 衰减器FeCr 铁铬磁带Feed 馈给,馈⼊,输⼊Feeder 馈线Feed/Rewind spool 供带盘/倒带盘Ferrite head 铁氧体磁头F.&B. forward and back 前后FET field effect technology 场效应技术FF flip flop 触发器FF fast forward 快进FG flag generator 标志信号发⽣器FI fade in 渐进Field 声场Field pickup 实况拾⾳File ⽂件,存⼊,归档,数据集,(外)存储器Fill-in 填⼊FILT filter 滤波器Final 韵母Fine 微调Fingered 多指和弦Finger ⼿指,单指和弦FIN GND 接地⽚Finish 结束,修饰FIP digital frequency display panel 数字频率显⽰板FIR finite-furation impulse response 有限冲激响应(滤波器)Fire 启动Fix 确定,固定Fizz 嘶嘶声FL fluorescein 荧光效果Flange 法兰⾳响效果,镶边效果Flanger 镶边器Flanging 镶边Flash 闪光信号Flat 平坦,平直Flat noise ⽩噪声Flat tuning 粗调Flex 拐点FLEX flexible cord 软线,塞绳FLEX frequency level expander 频率扩展器FLEXWA VE flexible waveguide 可弯曲波导管FLG flanger 镶边器Flip 替换,调换Floating ⾮固定的,悬浮式的Floppy disc 软磁盘FLTR filter 滤波器Fluorescent display 荧光显⽰器Flute 长笛Flutter ⼀种放⾳失真,脉冲⼲扰,颤动FLW follow 跟踪,随动FL Y 均衡器FM fade margin 衰落设备FM frequency modulation 调频⼴播FM/SW telescopic rod aerial 调频/短波拉杆天线FO fade out 渐隐Focus 焦点,中⼼点Foldback 返送,监听Foot(board) 脚踏板(开关控制)Fomant 共振峰Force 过载,强⾏置⼊Format 格式,格式化,规格,(储存器中的)信息安排Forward 转送FPR floating point routine 浮点程序FPR full power response 全功率响应FR frequency 频率FR frequency response 频率响应Frame 画⾯,(电视的)帧Frames 帧数Free 剩余,⾃由Free echoes ⽆限回声(延时效果处理的⼀种)Free edge ⾃由折环(扬声器)FREEQ frequency 频率F.Rew fast rewind 快倒Freeze 凝固,声⾳骤停,静⽌Frequency divider 分频器Frequency shifter 移频器,变频器Fricative 擦⾳Front 前⾯的,正⾯的Front balance 前置平衡Front process 前声场处理FRU field replaceable unit 插件,可换部件FS frequency shift 频移,变调FS full short 全景FT facility terminal 设备(输出)端⼝FT fine tuning 微调FT foot 脚踏装置FT function tist 功能测试FT frequency tracke 频率跟踪器FTG fitting 接头,配件FTS faverate track selection 最佳声迹选择Full 丰满,饱和Full auto 全⾃动Full effect recording 全效果录⾳Full range 全⾳域,全频G gate 门(电路)G ground 接地GA general average 总平均值Gain 增益,提衰量Game 卡拉OK⾳响效果Gamut ⾳域Gap 间隔,通道Gate 噪声门,门,选通Gated Rev 选通混响(开门的时间内有混响效果)GB 吉字节Gear 风格,格调GEN generator (信号)发⽣器General 综合效果Generator 信号发⽣器GEQ graphie equalizier 图⽰均衡器GD ground 接地Girth 激励器的低⾳强度调节Glide strip 滑奏条(演奏装置)GLLS-sando 滑降(演奏的效果)Global 总体设计GM genertal MIDI 通⽤乐器数字接器GND ground 地线,接地端GP group 编组GPR general purpose receiver 通⽤接收机GPI general purpose interface 通⽤接⼝设备Govern 调整,控制,操作,运转GR group 组合Gramophone 留声机,唱机Graphic equalizer 图⽰均衡器,图表均衡器GRND ground 接地Groove 光盘螺旋道的槽Group 编组(调⾳台),组Growler 线圈短路测试仪GT gate 门,噪声门GT gauge template 样板GTE gate 门(电路)GTR gate reverb 门混响Guard 保护,防护装置GUI graphical user interface 图形⽤户接⼝Guitar 吉它Guy 拉线Gymnasium 体育馆效果Gyrator 回旋器HQAD high quality audio disc ⾼品位⾳频光盘HR handing room 操作室HR high resistance ⾼阻抗(信号端⼦的阻抗特性)HRTF head-related transfer function ⼈脑相关转换功能HS head set 头戴式⽿机HS hybrid system 混合系统HT home theater 家庭影院,家庭剧场Hubrid 混合⽹络,桥接岔路Hum 交流哼声,交流低频(50Hz)噪声Hum and Noise 哼杂声,交流噪声Humidity 湿度,湿⽓HUT homes using TV 家⽤电视HVDS Hi-visual dramatic sound ⾼保真现场感⾳响系统HX headroom extension 动态余量扩展(系统)(⼀种杜⽐降噪系统),净空延伸H horizonal ⽔平(状态)H hot 热(平衡信号端⼝的“热端”)Hall 厅堂效果Handle ⼿柄,控制HAR harmonec 谐波Hard knee 硬拐点(压限器)Harmonic 谐波Harmonic distortion 谐波失真Harmonic Generator 谐波发⽣器Harmonize (使)和谐,校⾳Harmony 和谐Harp 竖琴Hash 杂乱脉冲⼲扰Hass effect 哈斯效应HD harmonic distortion 谐波失真HDCD high definition compatible digital⾼分辨率兼容性数字技术HDTV hight definiton television ⾼清晰度电视Head 录⾳机磁头,前置的,唱头Head azimuth 磁头⽅位⾓Head gap 磁头缝隙Headroom 动态余量,动态范围上限,电平储备Headphone 头戴式⽿机Headset 头带式⽿机Heavy metel 重⾦属HeiFin 垂直微调Hearing 听到,听觉Heat sink 散热板Help (对程序的)解释HF high frequency ⾼频,⾼⾳Hi hign ⾼频,⾼⾳HI band ⾼频带Hi-end 最⾼品质,顶级Hi-BLEND ⾼频混合指⽰High cut ⾼切High pass ⾼通Highway 总线,信息通道Hi-Fi high fidelity ⾼保真,⾼保真⾳响Hiss 咝声Hi-Z ⾼阻抗HL half reverb ⼤厅混响Hoghorn 抛物⾯喇叭Hoisting 提升Hold 保持,⽆限延续,保持时间Holder ⽀架,固定架Hold-off 解除保持Home 家庭,实⽤Home theatre 家庭影院Horizontal ⽔平的,横向的Horn ⾼⾳号⾓,号筒,圆号Hornloaded 号⾓处理Hot 热端,⾼电位端Hour ⼩时Howling 啸叫声Howlround 啸叫H.P headphone 头戴式⽿机HPA haas pan allochthonous 哈斯声像漂移HPF high pass filter ⾼通滤波器HQ high quality ⾼质量,⾼品位Hyper Condenser 超⼼型的HZ hertz 赫兹H hard 硬的(⾳响效果特征)IC integrated circuit 集成电路ID identification 识别ID identify 标志Idle 空载的,⽆效果的IDTV improved definition television改进清晰度电视系统IEC international electrical commission国际电⼯委员会IEEE institute of electrical&electronic engineers电⽓及电⼦⼯程师学会IF intermidiate frequency 中频的I/F interface 接⼝IHF the institute of high fidelity ⾼保真学会IIR infinite-duration impulse response⽆限冲激响应IKA Interactive knee adapt互调拐点适配,软拐点I/O input/output 输⼊/输出IM impulse modulation 脉冲调剂IM image 影象IMD intermodulation distortion 互调失真IMP impedance 阻抗IMP impedence 阻抗IMP interface message processor 接⼝信息处理机Improper 错误的IN inductor 感应器IN input 输⼊IN inverter 反演器,倒相器Inactive 暂停,失效的INC incoming 引⼊线INC increase 增⾼INCOM intercom 内部通话(系统)In phase 同相IND index 索引,标志,指数IND indicator 指⽰器Indicator 显⽰器,指⽰器Indirect 间接Inductance 电感Induction 感应,引⼊INF infinite ⽆限⼤Infrared 红外线的Infra-red remote control 红外线遥控INH inhibit 抑制,禁⽌Initial 声母,初始化In/Out 加与不加选择(相当于旁路)开关,接通开关Infinite ⽆限的,⾮限定的Increase 增加Initial Delay 早期延时,初次延时Inject 注⼊,置⼊Inlead 引⼊线Inlet 引⼊线,插⼊In-line 串联的,在线的INP input 输⼊(端⼝)INV invertor 倒相器,翻转器,反相器,变换器Inverse 倒相Inverseve Rev 颠倒式混响效果Invert 轮流,反转I/O in/out输⼊/输出(接⼝),信号插⼊接⼝I/Oinstead of 替代IPE integrated parameter editing 综合参量编辑IR infrared sensor 红外线传感器IROA impulse response optimum algorithm脉冲响应最佳算法IS information separators 信息分隔字符IS in service 不中断服务ISO International Standardization Organization国际标准化组织J jack 插孔,插座,传动装置Jack socket 插孔Jaff 复⼲扰Jagg club 爵⼠乐俱乐部效果Jam 抑制,⼲扰Jamproof 抗⼲扰的Jazz 爵⼠JB junction box 接线盒JIS ⽇本⼯业标准Job 事件,作业指令,成品Jog 旋盘缓进,慢进,突然转向Joker 暗藏的不利因素,含混不清Joystick 控制⼿柄,操纵杆,摇杆JSS jet servo system 喷射伺服式重低⾳扬声器系统Jumper 跳线,条形接⽚Justify 调整Input 输⼊Indicator 显⽰器,指⽰灯INS insert 插⼊(信号),插⼊接⼝INSEL input select 输⼊选择INST instant 直接的,实时INST institution 建⽴,设置INST instrument 仪器,乐器Instrument 乐器Insulator 绝缘体INT intake 进⼊,⼊⼝INT intensity 强度,烈度INT interior 内部INT interrupter 断路器Integrated 组合的Integrated amplifier前置-功率放⼤器,综合功率放⼤器Intelligate 智能化噪声门Intelligibility 可懂度Interactie 相互作⽤,⼈机对话,软拐点Interval ⾳⾼差别Integrated 集成的,完全的Intercom 对讲,通话Interconnect 互相联系Inter cut 插播Interface 接⼝,对话装置Interference ⼲扰,⼲涉,串扰Interim 临时的,过渡特征Intermodulation 互调,内调制Intermodulation distortion 交越失真Internal 内存,对讲机Internally 在内部,内存Inter parameter 内部参数Interval ⾳⾼差别Interplay 相互作⽤,内部播放Interval shifter ⾳歇移相器Intimacy 亲切感Intonation 声调INTRO introduction 介绍,浏览,引⼊,(乐曲的)前奏INTRO sacn 曲头检索(节⽬搜索)INTRO sensor 曲头读出器(节⽬查询)Introskip 内移,内跳ISS insertion test signal 插⼊切换信号ISS interference suppression switch ⼲扰抑制开关ITS insertion test signal 插⼊测试信号IV interval 间隔搜索IV inverter 倒相器IWC interrupted wave 断续波IX index 标盘,指针,索引K key 按键Karaoke 卡拉OK,⽆⼈伴奏乐队KB key board 键盘,按钮Kerr 克⽿效应,(可读写光盘)磁光效应Kernelstreaming:内核流Key 键,按键,声调Keyboard 键盘,按钮Key control 键控,变调控制Keyed 键控Key EQ ⾳调均衡kHz Kiloherts 千赫兹Kikll 清除,消去,抑制,衰减,断开Killer 抑制器,断路器Kit 设定Knee 压限器拐点Knob 按钮,旋钮,调节器KP key pulse 键控脉冲KTV karaoke TV 拌唱电视(节⽬)KX key 键控Lesion 故障,损害Leslie 列斯利(⼀种调相效果处理⽅式)LEV level 电平LEVCON level control 电平控制Level 电平,⽔平,级LF low frequency 低频,低⾳LFB local feedback 本机反馈,局部反馈LFE lowfrequency response 低频响应LFO low frequency oscillation 低频振荡信号LGD long delay 长延时LH low high 低噪声⾼输出LH low noise high output 低噪声⾼输出磁带L.hall large hall ⼤厅效果Lift 提升(⼀种提升地电位的装置)Lift up 升起Labial 唇⾳L left 左(⽴体声系统的左声道)L line 线路L link 链路L long 长(时间)LA laser 激光(镭射)Lag 延迟,滞后Lamp 灯,照明灯Land 光盘螺旋道的肩,接地,真地Lap dissolve 慢转换Lapping SW 通断开关Large ⼤,⼤型Large hall ⼤厅混响Larigot 六倍⾳Laser 激光(镭射)Latency 空转,待机Launching 激励,发射Layer 层叠控制,多⾳⾊同步控制LCD liquid crystal display 液晶显⽰LCR left center right 左中右LD laser vision disc 激光视盘,影碟机LD load 负载LDP input 影碟输⼊LDTV low definition television低分辨率数字电视LCD projictor 液晶投影机Lead 通道,前置,输⼊Lead-in 引⼊线Leak 漏泄Learn 学习LED light emitting deivce 发光辐射器,发光器件M main 主信道M master 主控M memory 存储器M mix 混频M moderate 适中的M music ⾳乐Mac manchester auto code 曼切斯特⾃动码MADI musical audio digital interface ⾳频数字接⼝Main 主要的,主线,主通道,电源MAG magnet 磁铁Magnetic tape 磁带Magnetic type recorder 磁带录⾳机Main 电源,主要的Major chord ⼤三和弦Make 接通,闭合Makeup 接通,选配Male 插头,插件MAN manual ⼿动的,⼿控。
语音信号处理文献翻译(1)
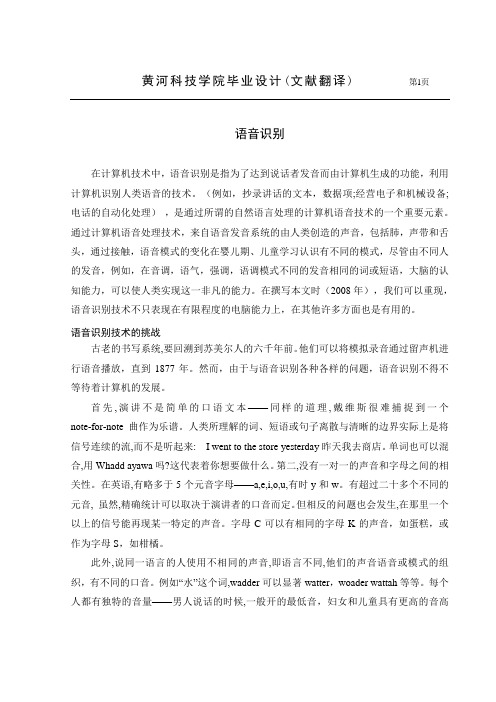
黄河科技学院毕业设计(文献翻译)第1页语音识别在计算机技术中,语音识别是指为了达到说话者发音而由计算机生成的功能,利用计算机识别人类语音的技术。
(例如,抄录讲话的文本,数据项;经营电子和机械设备;电话的自动化处理),是通过所谓的自然语言处理的计算机语音技术的一个重要元素。
通过计算机语音处理技术,来自语音发音系统的由人类创造的声音,包括肺,声带和舌头,通过接触,语音模式的变化在婴儿期、儿童学习认识有不同的模式,尽管由不同人的发音,例如,在音调,语气,强调,语调模式不同的发音相同的词或短语,大脑的认知能力,可以使人类实现这一非凡的能力。
在撰写本文时(2008年),我们可以重现,语音识别技术不只表现在有限程度的电脑能力上,在其他许多方面也是有用的。
语音识别技术的挑战古老的书写系统,要回溯到苏美尔人的六千年前。
他们可以将模拟录音通过留声机进行语音播放,直到1877年。
然而,由于与语音识别各种各样的问题,语音识别不得不等待着计算机的发展。
首先,演讲不是简单的口语文本——同样的道理,戴维斯很难捕捉到一个note-for-note曲作为乐谱。
人类所理解的词、短语或句子离散与清晰的边界实际上是将信号连续的流,而不是听起来: I went to the store yesterday昨天我去商店。
单词也可以混合,用Whadd ayawa吗?这代表着你想要做什么。
第二,没有一对一的声音和字母之间的相关性。
在英语,有略多于5个元音字母——a,e,i,o,u,有时y和w。
有超过二十多个不同的元音, 虽然,精确统计可以取决于演讲者的口音而定。
但相反的问题也会发生,在那里一个以上的信号能再现某一特定的声音。
字母C可以有相同的字母K的声音,如蛋糕,或作为字母S,如柑橘。
此外,说同一语言的人使用不相同的声音,即语言不同,他们的声音语音或模式的组织,有不同的口音。
例如―水‖这个词,wadder可以显著watter,woader wattah等等。
数字语音信号处理_01
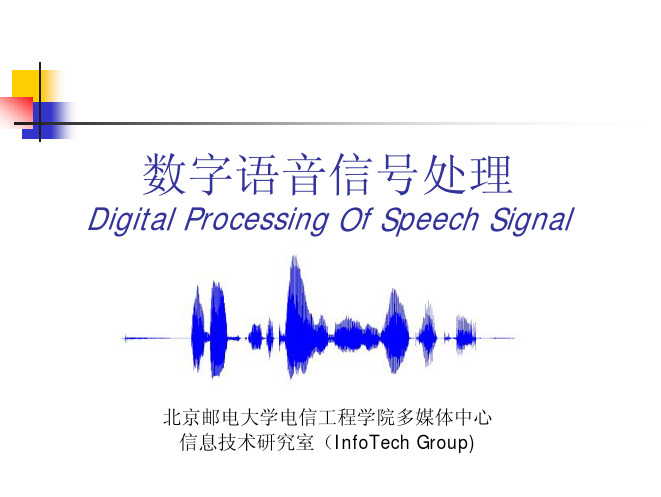
V1 V2 V3 V4 V5
Vi ( z ) =
1 − B i z −1 − C i z − 2
Ai
数字语音信号处理
V1 V2 V1 V2 V3 V4 V3 V4 V5
数字语音信号处理
ul(n)
线性系统 唇辐射R(z) Pl(z)=R(z)Ul(z) R(z)=R0(1-z-1)
基音周期TP
H( z ) =
1− ∑ dk z
k =1
随机噪声 q 发生器 −k
G
开关
k =1 声道V(z)
R(z)
AN
数字语音信号处理
语音的感知
听觉系统 耳的结构
数字语音信号处理
语音的感知
听觉系统 耳的结构
数字语音信号处理
语音的感知
听觉系统 耳蜗的作用
数字语音信号处理
数字语音信号处理
语音的产生
语音产生机理 发声器官 声带 基音频率
Fp(Hz) 男人 女人 小孩 60~200 150~300 200~400
数字语音信号处理
语音的产生
语音产生机理 发声器官 声道 频谱整形 共振峰频率
数字语音信号处理
语音的产生
根据声源的语音分类 浊音 清音 摩擦音 爆破音
pl(n)
数字语音信号处理
冲激序列 发生器 基音周期TP 声门脉冲 模型G(z) 清/浊音 开关 随机噪声 发生器
Av
线性系统 声道V(z) 辐射模型 R(z)
AN
数字语音信号处理
H( z ) = G( z )V ( z )R( z ) A 冲激序列 声门脉冲 v G 1 −1 发生器 H( z ) = 模型G(z) ⋅ R0 (1 − z ) ⋅ −cT −1 2 N ( 1 − e z ) 1 − ∑ a z −k 线性系统 清/浊音 辐射模型 k
- 1、下载文档前请自行甄别文档内容的完整性,平台不提供额外的编辑、内容补充、找答案等附加服务。
- 2、"仅部分预览"的文档,不可在线预览部分如存在完整性等问题,可反馈申请退款(可完整预览的文档不适用该条件!)。
- 3、如文档侵犯您的权益,请联系客服反馈,我们会尽快为您处理(人工客服工作时间:9:00-18:30)。
附录:中英文翻译15SpeechSignalProcessing15.3AnalysisandSynthesisJ esseW. FussellA fte r an acousti c spee ch s i gnal i s conve rte d to an ele ctri cal si gnal by a mi crophone, i t m ay be desi rable toanalyzetheelectricalsignaltoestimatesometime-varyingparameterswhichprovideinformationaboutamodel of the speech producti on me chanism. S peech a na ly sis i s the process of e stim ati ng such paramete rs. Simil arl y , g ive n some parametri c model of spee ch production and a se que nce of param eters for that m odel,speechsynthesis istheprocessofcreatinganelectricalsignalwhichapproximatesspeech.Whileanalysisandsynthesistechniques maybedoneeitheronthecontinuoussignaloronasampledversionofthesignal,mostmode rn anal y sis and sy nthesis methods are base d on di gital si gnal processing.Atypicalspeechproductionmodelisshownin Fig.15.6.Inthismodeltheoutputoftheexcitationfunctionisscaledbythegainparam eterandthenfilteredtoproducespeech.Allofthesefunctionsaretime-varying.F IGUR E 15 .6 A ge ne ra l spee ch productionmodel.F IGUR E 1 5 .7 W ave form of a spoken phone me /i/ as i nbeet.Formanymodels,theparametersarevariedataperiodicrate,typically50to100timespersecond.Mostspee ch inform ati on is containe d i n the porti on of the si gnal bel ow about 4 kHz.Theexcitationisusually modeledaseitheramixtureorachoiceofrandomnoiseandperiodicwaveform.For hum an spee ch, v oi ced e x citati on occurs w hen the vocal fol ds in the lary nx vibrate; unvoi ce d e x citati onoccurs at constri cti ons i n the vocal tract w hi ch cre ate turbulent a i r fl ow [Fl anagan, 1965] . The rel ati ve mi x ofthesetw o type s ofexcitationisterme d ‚v oicing.‛In addition,theperiodi c e xcitation i s characterizedby afundamentalfrequency,termed pitch orF0.Theexcitationisscaledbyafactordesignedtoproducetheproperampli tude or level of the spee ch si gnal . The scaled ex citati on function i s then fi ltere d to produce the properspe ctral characte risti cs. W hile the filter m ay be nonli near, i t i s usuall y m odele d as a li nearfunction.AnalysisofExcitationInasimplifiedform,theexcitationfunctionmaybeconsideredtobepurelyperiodic,forvoicedspeech,orpurel y random, for unvoi ce d. T hese tw o states correspond to voi ce d phoneti c cl asse s such as vow elsand nasalsandunvoicedsoundssuchasunvoicedfricatives.Thisbinaryvoicingmodelisanoversimplificationforsounds such as v oi ced fri cati ves, whi ch consist of a mi xture of peri odi c and random compone nts. Fi gure 15.7is an ex ample of a time w ave form of a spoke n /i/ phoneme , w hi ch is w ell m odeled by onl y pe riodi c e x citation.B oth ti me dom ai n and frequency dom ai n anal y s is te chni ques have bee n used to esti m ate the de greeofvoi ci ng for a short se gme nt or frame of spee ch. One ti me dom ain fe ature, te rme d the ze ro crossing rate,i sthenumberoftimesthesignalchangessigninashortinterval.AsshowninFig.15.7,thezerocrossingrateforvoicedsoundsisrelativ elylow.Sinceunvoicedspeechtypicallyhasalargerproportionofhigh-frequencyenergy than voi ce d spee ch, the ratio of high-fre que ncy to low -frequency e nergy is a fre que ncy dom aintechni que that provi des i nform ation on voi cing.A nothe r measure use d to estim ate the de gree of voi ci ng is the autocorrel ation functi on, w hi ch is de fine d fora sam pled speech se gment, S ,aswheres(n)isthevalueofthenthsamplewithinthesegmentoflengthN.Sincetheautocorrelationfunctionofa periodi c functi on is i tsel f pe ri odi c, voi ci ng can be e sti mated from the de gree of pe ri odi city oftheautocorrel ati on function. Fi gure 15. 8 i s a graph of the nonne gati ve te rms of the autocorrel ation functi on for a64 -ms frame of the w aveform of Fi g . 15. 7. Ex cept for the de cre ase i n amplitude w ith i ncre asi ng lag, whi chresultsfromtherectangularwindowfunctionwhichdelimitsthesegment,theautocorrelationfunctionisseento be quite pe riodi c for thi s voi ce dutterance.F IGUR E 1 5 .8 A utocorrel ati on functi on of one frame of /i/. Ifananalysisofthevoicingofthespeechsignalindicatesavoicedorperiodiccomponentispresent,another ste p i n the anal y si s process m ay be to estim ate the freque ncy ( or pe ri od) of the voi ce d component.Thereareanumberofwaysinwhichthismaybedone.Oneistomeasurethetimelapsebetweenpeaksinthetime dom ai n si gnal. For ex am ple i n Fi g . 15.7 the m aj or peaks are separate d by about 0. 00 71 s, for afundamentalfrequencyofabout141Hz.Note,itwouldbequitepossibletoerrintheestimateoffundamentalfre quency by mistaki ng the sm aller pe aks that occur betwee n the m a jor pe aks for the m aj or pe aks. Thesesmallerpeaksareproducedbyresonanceinthevocaltractwhich,inthisexample,happentobeatabouttwicethe ex citation fre quency . T his ty pe of e rror w ould re sult in an e sti m ate of pitch approxi m atel y tw i ce the corre ct fre quency.The di stance betw ee n m ajor pe ak s of the autocorrel ation functi on is a closel y rel ate d fe ature thatisfre quentl y use d to esti m ate the pitch pe ri od. In Fi g . 15. 8, the di stance between the m aj or peaks in the autocorrelationfunctionisabout0.0071s.Estimatesofpitchfromtheautocorrelationfunctionarealsosusce pti ble to mistaking the fi rst vocal track resonance for the g l ottal e x citati on frequency.The absol ute m agnitude di ffere nce functi on ( AM DF), de fi nedas,is another functi on w hi ch is often use d i n estim ating the pitch of voi ce d spee ch. A n ex ample of the AM DF isshownin Fig.15.9forthesame64-msframeofthe/i/phoneme.However,theminimaoftheAMDFisusedasanindicatorofthepitchperiod.TheAMDFhasbeenshownt obeagoodpitchperiodindicator[Rossetal.,19 74 ] and does not requi re multi pli cations.FourierAnalysisOne of the m ore comm on processe s for e stim ating the spe ctrum of a se gme nt of spee ch is the Fourie rtransform [ Oppenheim and S chafer, 1 97 5 ]. T he Fourie r transform of a seque nce is m athem ati call y de fine daswheres(n)representsthetermsofthesequence.Theshort-timeFouriertransformofasequenceisatimedependentfunction,definedasF IGUR E 1 5 .9 A bsolute m agnitude diffe rence functi on of one frame of /i/.wherethewindowfunctionw(n)isusuallyzeroexceptforsomefiniterange,andthevariablemisusedtoselectthesectionofthesequ enceforanalysis.ThediscreteFouriertransform(DFT)isobtainedbyuniformlysam pling the short-ti me Fourie r transform i n the fre quency dime nsi on. Thus an N-point DFT is computedusingEq.(15.14),wherethe setofNsamples,s(n),may have firstbeenmultiplied by a window function.Anexampleofthemagnitudeofa512-pointDFTofthewaveformofthe/i/from Fig.15.10isshowninFig.15.10.Noteforthisfi gure, the 512 poi nts in the se que nce have been m ulti plied by a Ham ming w i ndow de fi nedbyF IGUR E 1 5 .1 0 M agnitude of 51 2-point FFT of Ham mi ng window e d/i/.S ince the spe ctral characteristi cs of spee ch m ay change dram a ti call y in a fe w milli se conds, the le ngth, type,and l ocation of the wi ndow function are im portant consi derati ons. If the w indow is too long, changi ng spe ctralcharacteristicsmaycauseablurredresult;ifthewindowistooshort,spectralinaccuraciesresult.AHammingwi ndow of 16 to 32 m s durati on is com m onl y use d for spee ch analysis.S everal characte risti cs of a speech utte rance m ay be dete rmine d by ex amination of the DFT m agnitude. InFig.15.10,theDFTofavoicedutterancecontainsaseriesofsharppeaksinthefrequencydomain.Thesepeaks, caused by the peri odi c sampl ing acti on of the g lottal ex ci tation, are separated by the fundame ntalfrequencywhichisabout141Hz,inthisexample.Inaddition,broaderpeakscanbeseen,forexampleatabout300 Hz and at about 2300 Hz. T hese broad peaks, calle d formants, result from resonances in the vocaltract. LinearPredictiveAnalysisGivenasampled(discrete-time)signals(n),apowerfulandgeneralparametric modelfortimeseriesanalysisiswheres(n)istheoutputandu(n)istheinput(perhapsunknown).Themodelparametersare a(k)fork=1,p,b( l ) for l = 1, q, and G. b( 0) is assume d to be unity. Thi s m odel , describe d as an autore g ressi ve m ov ing average(ARM A)orpole-zeromodel,formsthefoundationfortheanalysismethodtermedlinearprediction.Anautoregressive(AR) orall-polemodel,forwhichallofthe‚b‛coe fficientsexceptb(0)arezero,isfrequentlyused for spee ch anal y si s [M arkel and Gray, 1976].In the standard A R formul ati on of li ne ar predi ction, the model paramete rs are sele cte d to mi ni mizethemean-squarederrorbetweenthemodelandthespeechdata.Inoneofthevariantsoflinearprediction,theautocorrelationmethod,themini mizationiscarriedoutforawindowedsegmentofdata.Intheautocorrelationmethod,minimizingthemean-squareerror of the time domain samples is equivalentto minimizing theintegratedratioofthesignalspectrumtothespectrumoftheall-polemodel.Thus,linearpredictiveanalysisisagoodmethod forspectralanalysiswheneverthesignalisproducedby an all-pole system.M ost speechsounds fi t thi s model w ell.One ke y consi deration for li near pre dicti ve anal y si s is the order of the model, p. For spee ch, if the orde ristoosmall,theformantstructureisnot well represented. If the orderis too large, pitch pulses as well asformantsbegintoberepresented.Tenth- or twelfth-order analysis is typical forspeech.Figures15.11 and15.12 provideexamplesof the spectrum produced by eighth-order and sixteenth-order linear predictiveanalysisofthe/i/waveformofFig.15.7.Figure15.11showstheretobethreeformantsatfrequenciesofabout30 0, 23 00, and 3200 Hz , whi ch are ty pi cal for an/i/.Homomorphic(Cepstral)AnalysisFor the speech m odel of Fi g. 15. 6, the e x citati on and filter i mpulse response are convol ved to produce thespeech.Oneoftheproblemsofspeechanalysisistoseparateordeconvolvethespeechintothesetw ocom ponents. Onesuch te chni que is called hom omorphi c filte ri ng [ Oppe nheim and S chafer, 1968 ]. Thecharacte risti c sy ste mfor a sy ste m for hom om orphi c deconvol ution conve rts a convolution operation to anadditi on ope ration. The output of such a characteristi c sy stem is calle d the com ple x cep str u m . The complexcepstrumisdefinedastheinverseFouriertransformofthecomplexlogarithmoftheFouriertransformoftheinput.Iftheinputseque nceisminimumphase(i.e.,thez-transformoftheinputsequencehasnopolesorzerosoutside the unit ci rcle), the se quence can be represe nted by the real portion of the transforms. Thus, the re alcepstrum can be com pute d by cal cul ati ng the inve rse Fourie r transform of the log- spe ctrum of theinput.FIGURE15.11Eighth-orderlinearpredictiveanalysisofan‚i‛.FIGURE15.12Sixteenth-orderlinearpredictiveanalysisofan‚i‛.Fi gure 1 5.1 3 show s an e x ample of the cepstrum for the voi ced /i/ utterance from Fi g. 15.7 . The cepstrum ofsuch a voi ce d utterance i s characte rized by rel ati vel y la rge v alues in the fi rst one or tw o milli se conds as w ellas。