Asymptotic enumeration of sparse nonnegative integer matrices with specified row and column
pp2反义词
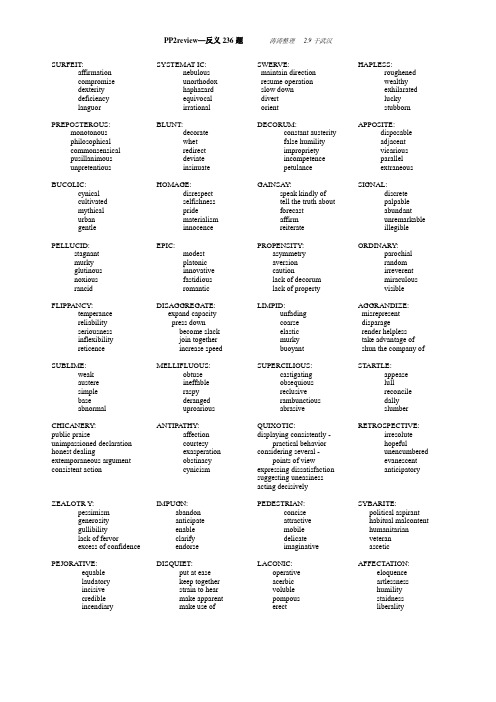
SURFEIT:affirmationcompromisedexteritydeficiencylanguor SYSTEMA T IC:nebulousunorthodoxhaphazardequivocalirrationalSWERVE:maintain directionresume operationslow downdivertorientHAPLESS:roughenedwealthyexhilaratedluckystubbornPREPOSTEROUS:monotonousphilosophicalcommonsensicalpusillanimousunpretentious BLUNT:decoratewhetredirectdeviateinsinuateDECORUM:constant austerityfalse humilityimproprietyincompetencepetulanceAPPOSITE:disposableadjacentvicariousparallelextraneousBUCOLIC:cynicalcultivatedmythicalurbangentle HOMAGE:disrespectselfishnesspridematerialisminnocenceGAINSAY:speak kindly oftell the truth aboutforecastaffirmreiterateSIGNAL:discretepalpableabundantunremarkableillegiblePELLUCID:stagnantmurkyglutinousnoxiousrancid EPIC:modestplatonicinnovativefastidiousromanticPROPENSITY:asymmetryaversioncautionlack of decorumlack of propertyORDINARY:parochialrandomirreverentmiraculousvisibleFLIPPANCY:temperancereliabilityseriousnessinflexibilityreticence DISAGGREGA TE:expand capacitypress downbecome slackjoin togetherincrease speedLIMPID:unfadingcoarseelasticmurkybuoyantAGGRANDIZE:misrepresentdisparagerender helplesstake advantage ofshun the company ofSUBLIME:weakausteresimplebaseabnormal MELLIFLUOUS:obtuseineffableraspyderangeduproariousSUPERCILIOUS:castigatingobsequiousreclusiverambunctiousabrasiveSTARTLE:appeaselullreconciledallyslumberCHICANERY:public praise unimpassioned declaration honest dealing extemporaneous argument consistent action ANTIPA THY:affectioncourtesyexasperationobstinacycynicismQUIXOTIC:displaying consistently -practical behaviorconsidering several -points of viewexpressing dissatisfactionsuggesting uneasinessacting decisivelyRETROSPECTIVE:irresolutehopefulunencumberedevanescentanticipatoryZEALOTR Y:pessimismgenerositygullibilitylack of fervorexcess of confidence IMPUGN:abandonanticipateenableclarifyendorsePEDESTRIAN:conciseattractivemobiledelicateimaginativeSYBARITE:political aspiranthabitual malcontenthumanitarianveteranasceticPEJORA TIVE:equablelaudatoryincisivecredibleincendiary DISQUIET:put at easekeep togetherstrain to hearmake apparentmake use ofLACONIC:operativeacerbicvolublepompouserectAFFECTA TION:eloquenceartlessnesshumilitystaidnessliberalitySUMMARY:tenuousextenuatingnominalaustereprotracted BUNGLE:bring offbail outgive inpull throughput togetherVIGILANT:reluctanthaphazardgullibleignorantobliviousENCOMIUM:biased evaluationpolite responsevague descriptionharsh criticismsorrowful expressionREIN:prodassessengulfcommendaffirm STODGY:nervousincisiveexcitingtalkativehappySATURNINE:helpfulintelligentrestrainedmodestjovialSERE:pungentacutemeandamphermeticVICISSITUDINOUS:charitableprosaicingratiatingintrinsicunchanging TRACTABLE:indefatigableincorrigibleinsatiableimpracticalimpetuousPONDEROUS:livelyhaphazardcongruousaccessiblefamiliarRESCISSION:expansionenactmentinstigationstimulationabdicationSPURIOUSNESS:eloquenceantiquityauthenticitypartial effectivenessblatant disregard HYBRID:inferiordestructiveunchangeableof unmixed extractionof known derivationFORTHRIGHT:unkindmistakenfurtiveinelegantinsensitiveREFINE:loosenobscuredestabilizedecrease sizereduce purityFASTIDIOUS:coarsedestructivewillingcollectivesecret VORACIT Y:lack of appetitelack of progressaccepting no apologystraightnesstediousnessEMBED:induceextendextractreceivedivergeBLANDISHMENT:mistakeparodyconceitthreatcureCOMPRESSION:increase in volumechange of altitudeloss of stabilityabsence of matterlack of motion BOMBAST:kindnessnonthreatening motiongreat effortdown-to-earth languagegood-natured approvalCUMBERSOME:likely to succeedreasonable to trustvaluable to haveeasy to handleimportant to knowPERTINACITY:liabilitysimplicityvacillationeccentricitymisrepresentationLACONISM:temerityvacuitydishonestyimmaturityverbosity RUEFUL:secretly enviousgravely thoughtfulopenly ambitiousimpenitentvengefulARTIFICE:contentmentself-assurancecandortemerityreticenceVI RTUOSITY:immoralitymediocritytimidityinconstancyvirulenceFIASCO:a realistic goala notable successa strong premonitiona conciliatory announcement an unexpected development PROVIDENT:unscrupulousunyieldingprofligatesombershallowMINGLE:obstructconfineseparatebranch outspread thinENERV ATE:inculcateinaugurateinvigoratefabricategenerateLUCIDITY:conventionalitydissimilaritystridencyconsonanceobscurity SEMINAL:obliqueprovincialincompletefluctuatingderivativeAMBIGUOUS:integratedstationarystraightforwardstrikingthoroughCOSSET:inspireacquitdislikeintercedeslightALLAY:replaceruptureassume responsibility for increase the intensity of attract the notice of SPLEEN:submissivenessvolubilitygoodwillsophisticationindecisionRESTIVENESS:contentmentheartinesssmugnessbashfulnessurbanityVERSATILE:challenging accepted beliefshaving limited applicationsmaking clear distinctionsexcessiveunreliableLIMPID:irrelevantcausticinformalunsubstantiatedunclear RECORD:postponedispersedeletedelaydeviseSANGUINE:morosepuzzledgiftedwittypersistentMOLLYCODDLE:talk boastfullyflee swiftlytreat harshlydemand suddenlyadjust temporarilySYNTHESIZE:break into partsreconsider an optionreinforce a foundationalter superficiallycause confusion BESMIRCH:establishpublicizeinspirerecanthonorREFRACTORY:activeproductiveenergeticresponsivepowerfulDAUNTLESS:easily discouragedopen to persuasionfree of prejudicesalvageableetherealINTRANSIGENT:accustomed to commandqualified to arbitrateopen to compromiseresigned to conflictopposed to violence FACETIOUS:uncomplicatedpridefulearnestlaconicforbearingDEFINITIVE:prosaicconvolutedunusualprovisionalvastDIFFIDENT:discerningvigorousbrassycooperativegenerousHASTY :unpalatablelatentcharitableunable to decide characterized by deliberation IGNITE:terminatepreventextinguishhinderretardHAUTEUR:mercyhumilityobedienceindulgenceselflessnessGALLING:submissiveenlighteningpraiseworthyaffirmingplacatingMUNIFICENT:commercialnoncommittalparsimoniouslaconicintrinsic GARBLE:abridgebelaborelucidatereorganize thoroughlyestablish conclusivelyRAIL:thrillwheedleextolflourishlingerLASH :disembarkdischargeuncoverunburdenunbindT RANSITORY:distinctivegradualpersistentdistantimplicit UNSEEMLY:predictableearnestexactingdecorousdiligentACCOLADE:prevaricationsuperficialitydigressiondenunciationretractionMYOPIC:contradictoryfarseeingterrifyingconfidentauthoritativePENCHANT:retreataversiontravestybetrayalprodigality CURSE:exonerationuntruthredressbenedictionseparationABDICA TE:assumeresistdefendproposedictateBOON:misfortuneimbrogliohopeless situationacrimonious debatecallous behaviorINIMITABLE:enviablereparableamicableunwieldycommonplace RECIPROCA TING:releasing slowlycalculating approximatelyaccepting provisionallymoving unidirectionallymixing thoroughlyPA THOLOGICAL:acutenormaladequatevariabletemporaryDOGMA:heresyindecencyself-interestmythical talehumorous gestureRESIST:acquiescedisqualifyemergecollectsustain CRUCIAL:vivaciouspraiseworthyinvalidintrepidinconsequentialGLUTINOUS:nonviscousnonporousantitoxiccatalyticalkalineHUSKY:wilycompliantflexibleswiftdelicateEXTINCTION:immunitymutationinhibitionformulationperpetuation WHOLESOME:deleterioussubmissiveprovokingmonotonousrigorousCREDULITY:comprehensionoriginalityincompetenceinaccuracyskepticismALLEVIATE:transposerectifydiagnoseintensifyderogateUNTOWARD:directfortunatetangentialdecisiveeffective VOLITION:ineligibility to negotiateinability to chooseindifference to shockindignity of frustrationinadequacy in socializingANOMALY:derivation from estimatesconformity to normsreturn to originsadaptation to stressesrepression of traitsCONCENTRA TE:particleliquidadditiverepellent forcedilute solutionUNTUTORED:polishedspecifichostiledisarmingcliched TOXIC:anaerobicbeneficialmoribundfar-rangingbarely perceptibleSERE:lushobstinateimmersedfortunateantiquatedEXOTIC:unessentialimplicitreticentimmutableindigenousDEACTIV AT E:palpatealleviateinhalearticulatepotentiate COALESCE:ossifydislocatemultiplydisaggregateenervateAMELIORA TE:preserveparticipateaggravateimplementimplicateINDUCEMENT:reproofdeterrentpartialitydistinctionconsiderationBALK:extendderiveobserveplan ahead carefullymove ahead willingly TETHER:detachabsorbassertdismantledesertTORPOR:competencezealopennesssuitabilitysatisfactionTORRID:somberarcticsanctionedinertdryCEMENT:purifyglazeoutmaneuversequesterfracture TEMPERANCE:tardinesspusillanimityabsentmindednesspedantryprofligacyMISREAD:refocusapprovepredictexplain succinctlyinterpret correctlyQUOTIDIAN:remarkablecursoryinappropriateengagingstressfulFLACCIDITY :firmnesscolorfulnessprecisionaggressionintuition DECREPIT:vigorouscapriciousmagnanimoussedulousaudaciousGREGARIOUS:feeblealooftactfulstingyinattentiveDIRE:pleasantplentifulmisdirectedsaturatedprotractedUNSUBSTANTIA TED: having unknown consequences carefully donemodifiedminimizedverified ENUNCIA TE:mumbledisclaimdissuadeblustercommendCOUNTENANCE:neglect to informrefuse to approvefail to appreciateavoid conflictdisobey ordersV ACUOUS:courteousexhilaratedmodestintelligentemergentABSOLUTE:inferiortolerantqualifiedpreliminaryimmeasurable JEJUNE:confidence-buildingwell-roundedhighly acclaimedwell-respectedthought-provokingPALLIA T E:delineatefrustrateoriginatefascinateexacerbateWAX:disconnectdecreasesupplementloosensquanderTAUTEN:rarefycoarsenforceloosenconstrain MIGRA TORY:speculativetransitoryrootedhardenedconvergentVOLUBILITY :gullibilityimmaturitytaciturnityscarcityimpassivityAWASH:fouledquenchedrigiddrysturdyDEBILITA TE:implicateinvigorateobfuscaterealignencumber INEPT:consideratediscreetinsightfulattentiveskilledABJECT:independentimpulsivefascinatingapprovingexultantHAPLESS:excitedelatedfortunatecompletely self-reliantassured of successFLUSTERED:mutecalmheavyingrainedcourageous POSTURE:walk clumsilybehave naturallyimpose arbitrarilypublicize widelyexplain carefullyA VER:collectaugmentplacatedenyencourageSTRATIFY:abridgeduplicatehomogenizeelevatedevelopOFFHAND:accurateuniversalappropriatepremeditateddisputatious EXCRETE:choose selectivelycoalesce tightlyingestimpairrestrainVIABILITY:inability to changeinability to breatheinability to livestate of frivolitystate of immobilityDISGORGE:interswallowsolidifyreplaceincludeALLY:mediatorfelonadversaryinventorconspirator ACCELERATE:swervearousedispelrejectretardGIST:artificial mannertrivial pointinformal procedureeccentric methodsingular eventJABBER:ticklearguestrokespeak slowlyjoke inaptlyGOSSAMER:sinceretaciturnintenseawkwardponderous ENDORSE:provoke criticismreceive paymentsubmit unwillinglyoppose publiclyperform quicklyWATERPROOF:soggynaturalunglazedviscouspermeableMOROSE:overawedagitatedcherubicdecisivecheerfulDISSONANCE:concordconfederacycollusionconsentcontract SEDULOUS:presumptuousponderoustreacherouscarelessuselessBREACH:garnersolderkeep silentmove forwardgive approvalFLUENT:insensitiveinappropriatederogatoryhaltinghypocriticalTRACTABILI T Y:infertilityimplausibilityincorrigibilityimpenetrabilityindefatigability ABROGATE:upholddeferdiscuss secretlyadmit willinglyread thoroughlyFACETIOUS:lugubriouscontentiousingenuousprodigiousaudaciousNOISOME:attractively fragrantsubtly flatteringconsistently patientsoftly glowinggradually diminishingPUNGENCY:boredomredundancyunresponsivenessblandnessinsignificance ANARCHY:courtesyhopeorderneutralityimportanceMETAMORPHOSE:move aheadremain unaltereddescend slowlyexamine in hasteprepare in advanceBROACH:keep track oflay claim toclose offsoothesimplifyAMALGAMA TE:separatefixateterminatecalibratecorrelate CRA VEN:indifferentpresumptuousvalorousscrupulouspetulantAWE:compassioneclecticismirreverenceguiltnervousnessMETAMORPHOSIS:relief from straincontinuation without changecyclical motiondogmatic persistencevaried activityWARMONGER:commentatorliaisonlobbyistemissarypacifist INSULARITY:overzealousnesscosmopolitanismsusceptibilitywilling hospitalityknowledgeable considerationBOISTEROUS:gratefulangrycleverfrightenedquietEMIT:absorbdemandmistakepreventrequireFERMENT:soliditypurityluciditytransparencytranquillity PERSEVERE:put intosend outtake awaygive upbring forwardAGGREGATE:belittlereturndisperseconfusealleviatePLETHORA:vacuousnessnarrownesschoicedearthconfusionEXTANT:extensiveextraneousextricableextinctextra DIVESTITURE:acquisitionpromotionsubsidizationconsultationmonopolizationINCURSION:loss of respectlack of resolvereparationrelapseretreatINDELIBILITY:availabilitycomprehensibilitydecidabilityerasabilityretrctabilitySPIRITUAL:eclecticfigurativeephemeralimmoralcorporeal DISPATCH:serenityleisurelinessheedlessnessirregularityaversionTOR TUOUS:enduringinformalcautioussimplisticstraightforwardEXPIRE:evolvestabilizecome to lifegrow to fruitionbring to lightSAV ANT:flattererboreunlearned personunprincipled individualmisunderstood advisor CURSORY:extremely delicateunusually brilliantcompletely naturalpainstakingly thoroughoverwhelmingly emotionalCIRCUMVENTION:plain languageneeded actioncareless inattentionprimary meansdirect encounterWITHHOLD:conjoinacceptcollaborategrantconsignIMMEMORIAL:recentdevouthastycrowdedcelebrated PANEGYRIC:covenantrecantationenigmaterminationanathemaVESTIGIAL:uniform in shapefully developedturgidmoribundmalleableHARBOR:evictdisenchanttake note ofbe surprised byhave doubts aboutCULPABLE:arcaneforgetfulconciliatorycontradictoryinnocent PERSISTENCE:affabilitytransienceturmoilsoundnesspluralityPANACHE:unremarkable behaviorpartisan stanceflexible attitudetraditionalismgullibilityPALPABLE:calmconstrainedsubtlelistlessdilatoryFACTIONAL:excessiveundistinguisheddisdainfuldisinteresteddisparate ESCHEW:embraceduplicatemollifymasticateglamorizeAMPLITUDE:brittlenessvaguenessmeagernesscoarsenessacutenessAIMLESS:connectednarrowedcentralpurposefulexploratorySAGE:victimfoolalienpauperaddict FERTILIZE:animatetranquilizemake humidrender barreneffect changeCONDENSE:make less compactgrow frigidmake malleablemake more variedincrease the volatility ofCOGNIZANT:elementaryfallaciousunawareretaliatoryunrelatedSEPULCHRAL:urbaneserenefrankmerrykind DILATE:absorbconsumededucecontractdischargePROSAIC:appropriatepropitiousambiguousmodernextraordinarySPATE:dearthtangentpresentimentinceptionresolutionCOUNTENANCE:feign reluctancedecide impetuouslycondemnaffrontdivert MOLLIFY:rouserefuterenounceopposecriticizeDISTRAUGHT:vigorouscomposedaccommodatingdeferentialcandidPOSEUR:sincere personingratiating individualapprenticeconfidantedullardPOLARIZE:forgiveconvertbefriendimpedeunify RAZE:complimentrepaycontainbuildcoddleMYOPIC:eagerdiscerningtrustworthyoften disengagedeasily convincedDISSIPATE:gatherseetherelaxexertinciteAnswer:DCAD CBCE DADD BABD CDDB DCBB CAAE DEEE BACB EAED ACED EBAB CDCE AACD ADDC EDCB BCCC EECE DCAB ECAC AEDA CCDC ECBE CCCE CDDB BDAA EDBA AEAE EAED BBBE ABAE EDCB EABB EEEA AABA EABD CEEB DCCD BEEC BBDC DCCB CEBD EDEE ADBD CAAA DCBC ACCB EBEA EDCD DAED EBEC CDED AEBA EBAC DACD BDAC DDEA CABA EDBA。
T.W. ANDERSON (1971). The Statistical Analysis of Time Series. Series in Probability and Ma

425 BibliographyH.A KAIKE(1974).Markovian representation of stochastic processes and its application to the analysis of autoregressive moving average processes.Annals Institute Statistical Mathematics,vol.26,pp.363-387. B.D.O.A NDERSON and J.B.M OORE(1979).Optimal rmation and System Sciences Series, Prentice Hall,Englewood Cliffs,NJ.T.W.A NDERSON(1971).The Statistical Analysis of Time Series.Series in Probability and Mathematical Statistics,Wiley,New York.R.A NDRE-O BRECHT(1988).A new statistical approach for the automatic segmentation of continuous speech signals.IEEE Trans.Acoustics,Speech,Signal Processing,vol.ASSP-36,no1,pp.29-40.R.A NDRE-O BRECHT(1990).Reconnaissance automatique de parole`a partir de segments acoustiques et de mod`e les de Markov cach´e s.Proc.Journ´e es Etude de la Parole,Montr´e al,May1990(in French).R.A NDRE-O BRECHT and H.Y.S U(1988).Three acoustic labellings for phoneme based continuous speech recognition.Proc.Speech’88,Edinburgh,UK,pp.943-950.U.A PPEL and A.VON B RANDT(1983).Adaptive sequential segmentation of piecewise stationary time rmation Sciences,vol.29,no1,pp.27-56.L.A.A ROIAN and H.L EVENE(1950).The effectiveness of quality control procedures.Jal American Statis-tical Association,vol.45,pp.520-529.K.J.A STR¨OM and B.W ITTENMARK(1984).Computer Controlled Systems:Theory and rma-tion and System Sciences Series,Prentice Hall,Englewood Cliffs,NJ.M.B AGSHAW and R.A.J OHNSON(1975a).The effect of serial correlation on the performance of CUSUM tests-Part II.Technometrics,vol.17,no1,pp.73-80.M.B AGSHAW and R.A.J OHNSON(1975b).The influence of reference values and estimated variance on the ARL of CUSUM tests.Jal Royal Statistical Society,vol.37(B),no3,pp.413-420.M.B AGSHAW and R.A.J OHNSON(1977).Sequential procedures for detecting parameter changes in a time-series model.Jal American Statistical Association,vol.72,no359,pp.593-597.R.K.B ANSAL and P.P APANTONI-K AZAKOS(1986).An algorithm for detecting a change in a stochastic process.IEEE rmation Theory,vol.IT-32,no2,pp.227-235.G.A.B ARNARD(1959).Control charts and stochastic processes.Jal Royal Statistical Society,vol.B.21, pp.239-271.A.E.B ASHARINOV andB.S.F LEISHMAN(1962).Methods of the statistical sequential analysis and their radiotechnical applications.Sovetskoe Radio,Moscow(in Russian).M.B ASSEVILLE(1978).D´e viations par rapport au maximum:formules d’arrˆe t et martingales associ´e es. Compte-rendus du S´e minaire de Probabilit´e s,Universit´e de Rennes I.M.B ASSEVILLE(1981).Edge detection using sequential methods for change in level-Part II:Sequential detection of change in mean.IEEE Trans.Acoustics,Speech,Signal Processing,vol.ASSP-29,no1,pp.32-50.426B IBLIOGRAPHY M.B ASSEVILLE(1982).A survey of statistical failure detection techniques.In Contribution`a la D´e tectionS´e quentielle de Ruptures de Mod`e les Statistiques,Th`e se d’Etat,Universit´e de Rennes I,France(in English). M.B ASSEVILLE(1986).The two-models approach for the on-line detection of changes in AR processes. In Detection of Abrupt Changes in Signals and Dynamical Systems(M.Basseville,A.Benveniste,eds.). Lecture Notes in Control and Information Sciences,LNCIS77,Springer,New York,pp.169-215.M.B ASSEVILLE(1988).Detecting changes in signals and systems-A survey.Automatica,vol.24,pp.309-326.M.B ASSEVILLE(1989).Distance measures for signal processing and pattern recognition.Signal Process-ing,vol.18,pp.349-369.M.B ASSEVILLE and A.B ENVENISTE(1983a).Design and comparative study of some sequential jump detection algorithms for digital signals.IEEE Trans.Acoustics,Speech,Signal Processing,vol.ASSP-31, no3,pp.521-535.M.B ASSEVILLE and A.B ENVENISTE(1983b).Sequential detection of abrupt changes in spectral charac-teristics of digital signals.IEEE rmation Theory,vol.IT-29,no5,pp.709-724.M.B ASSEVILLE and A.B ENVENISTE,eds.(1986).Detection of Abrupt Changes in Signals and Dynamical Systems.Lecture Notes in Control and Information Sciences,LNCIS77,Springer,New York.M.B ASSEVILLE and I.N IKIFOROV(1991).A unified framework for statistical change detection.Proc.30th IEEE Conference on Decision and Control,Brighton,UK.M.B ASSEVILLE,B.E SPIAU and J.G ASNIER(1981).Edge detection using sequential methods for change in level-Part I:A sequential edge detection algorithm.IEEE Trans.Acoustics,Speech,Signal Processing, vol.ASSP-29,no1,pp.24-31.M.B ASSEVILLE, A.B ENVENISTE and G.M OUSTAKIDES(1986).Detection and diagnosis of abrupt changes in modal characteristics of nonstationary digital signals.IEEE rmation Theory,vol.IT-32,no3,pp.412-417.M.B ASSEVILLE,A.B ENVENISTE,G.M OUSTAKIDES and A.R OUG´E E(1987a).Detection and diagnosis of changes in the eigenstructure of nonstationary multivariable systems.Automatica,vol.23,no3,pp.479-489. M.B ASSEVILLE,A.B ENVENISTE,G.M OUSTAKIDES and A.R OUG´E E(1987b).Optimal sensor location for detecting changes in dynamical behavior.IEEE Trans.Automatic Control,vol.AC-32,no12,pp.1067-1075.M.B ASSEVILLE,A.B ENVENISTE,B.G ACH-D EVAUCHELLE,M.G OURSAT,D.B ONNECASE,P.D OREY, M.P REVOSTO and M.O LAGNON(1993).Damage monitoring in vibration mechanics:issues in diagnos-tics and predictive maintenance.Mechanical Systems and Signal Processing,vol.7,no5,pp.401-423.R.V.B EARD(1971).Failure Accommodation in Linear Systems through Self-reorganization.Ph.D.Thesis, Dept.Aeronautics and Astronautics,MIT,Cambridge,MA.A.B ENVENISTE and J.J.F UCHS(1985).Single sample modal identification of a nonstationary stochastic process.IEEE Trans.Automatic Control,vol.AC-30,no1,pp.66-74.A.B ENVENISTE,M.B ASSEVILLE and G.M OUSTAKIDES(1987).The asymptotic local approach to change detection and model validation.IEEE Trans.Automatic Control,vol.AC-32,no7,pp.583-592.A.B ENVENISTE,M.M ETIVIER and P.P RIOURET(1990).Adaptive Algorithms and Stochastic Approxima-tions.Series on Applications of Mathematics,(A.V.Balakrishnan,I.Karatzas,M.Yor,eds.).Springer,New York.A.B ENVENISTE,M.B ASSEVILLE,L.E L G HAOUI,R.N IKOUKHAH and A.S.W ILLSKY(1992).An optimum robust approach to statistical failure detection and identification.IFAC World Conference,Sydney, July1993.B IBLIOGRAPHY427 R.H.B ERK(1973).Some asymptotic aspects of sequential analysis.Annals Statistics,vol.1,no6,pp.1126-1138.R.H.B ERK(1975).Locally most powerful sequential test.Annals Statistics,vol.3,no2,pp.373-381.P.B ILLINGSLEY(1968).Convergence of Probability Measures.Wiley,New York.A.F.B ISSELL(1969).Cusum techniques for quality control.Applied Statistics,vol.18,pp.1-30.M.E.B IVAIKOV(1991).Control of the sample size for recursive estimation of parameters subject to abrupt changes.Automation and Remote Control,no9,pp.96-103.R.E.B LAHUT(1987).Principles and Practice of Information Theory.Addison-Wesley,Reading,MA.I.F.B LAKE and W.C.L INDSEY(1973).Level-crossing problems for random processes.IEEE r-mation Theory,vol.IT-19,no3,pp.295-315.G.B ODENSTEIN and H.M.P RAETORIUS(1977).Feature extraction from the encephalogram by adaptive segmentation.Proc.IEEE,vol.65,pp.642-652.T.B OHLIN(1977).Analysis of EEG signals with changing spectra using a short word Kalman estimator. Mathematical Biosciences,vol.35,pp.221-259.W.B¨OHM and P.H ACKL(1990).Improved bounds for the average run length of control charts based on finite weighted sums.Annals Statistics,vol.18,no4,pp.1895-1899.T.B OJDECKI and J.H OSZA(1984).On a generalized disorder problem.Stochastic Processes and their Applications,vol.18,pp.349-359.L.I.B ORODKIN and V.V.M OTTL’(1976).Algorithm forfinding the jump times of random process equation parameters.Automation and Remote Control,vol.37,no6,Part1,pp.23-32.A.A.B OROVKOV(1984).Theory of Mathematical Statistics-Estimation and Hypotheses Testing,Naouka, Moscow(in Russian).Translated in French under the title Statistique Math´e matique-Estimation et Tests d’Hypoth`e ses,Mir,Paris,1987.G.E.P.B OX and G.M.J ENKINS(1970).Time Series Analysis,Forecasting and Control.Series in Time Series Analysis,Holden-Day,San Francisco.A.VON B RANDT(1983).Detecting and estimating parameters jumps using ladder algorithms and likelihood ratio test.Proc.ICASSP,Boston,MA,pp.1017-1020.A.VON B RANDT(1984).Modellierung von Signalen mit Sprunghaft Ver¨a nderlichem Leistungsspektrum durch Adaptive Segmentierung.Doctor-Engineer Dissertation,M¨u nchen,RFA(in German).S.B RAUN,ed.(1986).Mechanical Signature Analysis-Theory and Applications.Academic Press,London. L.B REIMAN(1968).Probability.Series in Statistics,Addison-Wesley,Reading,MA.G.S.B RITOV and L.A.M IRONOVSKI(1972).Diagnostics of linear systems of automatic regulation.Tekh. Kibernetics,vol.1,pp.76-83.B.E.B RODSKIY and B.S.D ARKHOVSKIY(1992).Nonparametric Methods in Change-point Problems. Kluwer Academic,Boston.L.D.B ROEMELING(1982).Jal Econometrics,vol.19,Special issue on structural change in Econometrics. L.D.B ROEMELING and H.T SURUMI(1987).Econometrics and Structural Change.Dekker,New York. D.B ROOK and D.A.E VANS(1972).An approach to the probability distribution of Cusum run length. Biometrika,vol.59,pp.539-550.J.B RUNET,D.J AUME,M.L ABARR`E RE,A.R AULT and M.V ERG´E(1990).D´e tection et Diagnostic de Pannes.Trait´e des Nouvelles Technologies,S´e rie Diagnostic et Maintenance,Herm`e s,Paris(in French).428B IBLIOGRAPHY S.P.B RUZZONE and M.K AVEH(1984).Information tradeoffs in using the sample autocorrelation function in ARMA parameter estimation.IEEE Trans.Acoustics,Speech,Signal Processing,vol.ASSP-32,no4, pp.701-715.A.K.C AGLAYAN(1980).Necessary and sufficient conditions for detectability of jumps in linear systems. IEEE Trans.Automatic Control,vol.AC-25,no4,pp.833-834.A.K.C AGLAYAN and R.E.L ANCRAFT(1983).Reinitialization issues in fault tolerant systems.Proc.Amer-ican Control Conf.,pp.952-955.A.K.C AGLAYAN,S.M.A LLEN and K.W EHMULLER(1988).Evaluation of a second generation reconfigu-ration strategy for aircraftflight control systems subjected to actuator failure/surface damage.Proc.National Aerospace and Electronic Conference,Dayton,OH.P.E.C AINES(1988).Linear Stochastic Systems.Series in Probability and Mathematical Statistics,Wiley, New York.M.J.C HEN and J.P.N ORTON(1987).Estimation techniques for tracking rapid parameter changes.Intern. Jal Control,vol.45,no4,pp.1387-1398.W.K.C HIU(1974).The economic design of cusum charts for controlling normal mean.Applied Statistics, vol.23,no3,pp.420-433.E.Y.C HOW(1980).A Failure Detection System Design Methodology.Ph.D.Thesis,M.I.T.,L.I.D.S.,Cam-bridge,MA.E.Y.C HOW and A.S.W ILLSKY(1984).Analytical redundancy and the design of robust failure detection systems.IEEE Trans.Automatic Control,vol.AC-29,no3,pp.689-691.Y.S.C HOW,H.R OBBINS and D.S IEGMUND(1971).Great Expectations:The Theory of Optimal Stop-ping.Houghton-Mifflin,Boston.R.N.C LARK,D.C.F OSTH and V.M.W ALTON(1975).Detection of instrument malfunctions in control systems.IEEE Trans.Aerospace Electronic Systems,vol.AES-11,pp.465-473.A.C OHEN(1987).Biomedical Signal Processing-vol.1:Time and Frequency Domain Analysis;vol.2: Compression and Automatic Recognition.CRC Press,Boca Raton,FL.J.C ORGE and F.P UECH(1986).Analyse du rythme cardiaque foetal par des m´e thodes de d´e tection de ruptures.Proc.7th INRIA Int.Conf.Analysis and optimization of Systems.Antibes,FR(in French).D.R.C OX and D.V.H INKLEY(1986).Theoretical Statistics.Chapman and Hall,New York.D.R.C OX and H.D.M ILLER(1965).The Theory of Stochastic Processes.Wiley,New York.S.V.C ROWDER(1987).A simple method for studying run-length distributions of exponentially weighted moving average charts.Technometrics,vol.29,no4,pp.401-407.H.C S¨ORG¨O and L.H ORV´ATH(1988).Nonparametric methods for change point problems.In Handbook of Statistics(P.R.Krishnaiah,C.R.Rao,eds.),vol.7,Elsevier,New York,pp.403-425.R.B.D AVIES(1973).Asymptotic inference in stationary gaussian time series.Advances Applied Probability, vol.5,no3,pp.469-497.J.C.D ECKERT,M.N.D ESAI,J.J.D EYST and A.S.W ILLSKY(1977).F-8DFBW sensor failure identification using analytical redundancy.IEEE Trans.Automatic Control,vol.AC-22,no5,pp.795-803.M.H.D E G ROOT(1970).Optimal Statistical Decisions.Series in Probability and Statistics,McGraw-Hill, New York.J.D ESHAYES and D.P ICARD(1979).Tests de ruptures dans un mod`e pte-Rendus de l’Acad´e mie des Sciences,vol.288,Ser.A,pp.563-566(in French).B IBLIOGRAPHY429 J.D ESHAYES and D.P ICARD(1983).Ruptures de Mod`e les en Statistique.Th`e ses d’Etat,Universit´e deParis-Sud,Orsay,France(in French).J.D ESHAYES and D.P ICARD(1986).Off-line statistical analysis of change-point models using non para-metric and likelihood methods.In Detection of Abrupt Changes in Signals and Dynamical Systems(M. Basseville,A.Benveniste,eds.).Lecture Notes in Control and Information Sciences,LNCIS77,Springer, New York,pp.103-168.B.D EVAUCHELLE-G ACH(1991).Diagnostic M´e canique des Fatigues sur les Structures Soumises`a des Vibrations en Ambiance de Travail.Th`e se de l’Universit´e Paris IX Dauphine(in French).B.D EVAUCHELLE-G ACH,M.B ASSEVILLE and A.B ENVENISTE(1991).Diagnosing mechanical changes in vibrating systems.Proc.SAFEPROCESS’91,Baden-Baden,FRG,pp.85-89.R.D I F RANCESCO(1990).Real-time speech segmentation using pitch and convexity jump models:applica-tion to variable rate speech coding.IEEE Trans.Acoustics,Speech,Signal Processing,vol.ASSP-38,no5, pp.741-748.X.D ING and P.M.F RANK(1990).Fault detection via factorization approach.Systems and Control Letters, vol.14,pp.431-436.J.L.D OOB(1953).Stochastic Processes.Wiley,New York.V.D RAGALIN(1988).Asymptotic solutions in detecting a change in distribution under an unknown param-eter.Statistical Problems of Control,Issue83,Vilnius,pp.45-52.B.D UBUISSON(1990).Diagnostic et Reconnaissance des Formes.Trait´e des Nouvelles Technologies,S´e rie Diagnostic et Maintenance,Herm`e s,Paris(in French).A.J.D UNCAN(1986).Quality Control and Industrial Statistics,5th edition.Richard D.Irwin,Inc.,Home-wood,IL.J.D URBIN(1971).Boundary-crossing probabilities for the Brownian motion and Poisson processes and techniques for computing the power of the Kolmogorov-Smirnov test.Jal Applied Probability,vol.8,pp.431-453.J.D URBIN(1985).Thefirst passage density of the crossing of a continuous Gaussian process to a general boundary.Jal Applied Probability,vol.22,no1,pp.99-122.A.E MAMI-N AEINI,M.M.A KHTER and S.M.R OCK(1988).Effect of model uncertainty on failure detec-tion:the threshold selector.IEEE Trans.Automatic Control,vol.AC-33,no12,pp.1106-1115.J.D.E SARY,F.P ROSCHAN and D.W.W ALKUP(1967).Association of random variables with applications. Annals Mathematical Statistics,vol.38,pp.1466-1474.W.D.E WAN and K.W.K EMP(1960).Sampling inspection of continuous processes with no autocorrelation between successive results.Biometrika,vol.47,pp.263-280.G.F AVIER and A.S MOLDERS(1984).Adaptive smoother-predictors for tracking maneuvering targets.Proc. 23rd Conf.Decision and Control,Las Vegas,NV,pp.831-836.W.F ELLER(1966).An Introduction to Probability Theory and Its Applications,vol.2.Series in Probability and Mathematical Statistics,Wiley,New York.R.A.F ISHER(1925).Theory of statistical estimation.Proc.Cambridge Philosophical Society,vol.22, pp.700-725.M.F ISHMAN(1988).Optimization of the algorithm for the detection of a disorder,based on the statistic of exponential smoothing.In Statistical Problems of Control,Issue83,Vilnius,pp.146-151.R.F LETCHER(1980).Practical Methods of Optimization,2volumes.Wiley,New York.P.M.F RANK(1990).Fault diagnosis in dynamic systems using analytical and knowledge based redundancy -A survey and new results.Automatica,vol.26,pp.459-474.430B IBLIOGRAPHY P.M.F RANK(1991).Enhancement of robustness in observer-based fault detection.Proc.SAFEPRO-CESS’91,Baden-Baden,FRG,pp.275-287.P.M.F RANK and J.W¨UNNENBERG(1989).Robust fault diagnosis using unknown input observer schemes. In Fault Diagnosis in Dynamic Systems-Theory and Application(R.Patton,P.Frank,R.Clark,eds.). International Series in Systems and Control Engineering,Prentice Hall International,London,UK,pp.47-98.K.F UKUNAGA(1990).Introduction to Statistical Pattern Recognition,2d ed.Academic Press,New York. S.I.G ASS(1958).Linear Programming:Methods and Applications.McGraw Hill,New York.W.G E and C.Z.F ANG(1989).Extended robust observation approach for failure isolation.Int.Jal Control, vol.49,no5,pp.1537-1553.W.G ERSCH(1986).Two applications of parametric time series modeling methods.In Mechanical Signature Analysis-Theory and Applications(S.Braun,ed.),chap.10.Academic Press,London.J.J.G ERTLER(1988).Survey of model-based failure detection and isolation in complex plants.IEEE Control Systems Magazine,vol.8,no6,pp.3-11.J.J.G ERTLER(1991).Analytical redundancy methods in fault detection and isolation.Proc.SAFEPRO-CESS’91,Baden-Baden,FRG,pp.9-22.B.K.G HOSH(1970).Sequential Tests of Statistical Hypotheses.Addison-Wesley,Cambridge,MA.I.N.G IBRA(1975).Recent developments in control charts techniques.Jal Quality Technology,vol.7, pp.183-192.J.P.G ILMORE and R.A.M C K ERN(1972).A redundant strapdown inertial reference unit(SIRU).Jal Space-craft,vol.9,pp.39-47.M.A.G IRSHICK and H.R UBIN(1952).A Bayes approach to a quality control model.Annals Mathematical Statistics,vol.23,pp.114-125.A.L.G OEL and S.M.W U(1971).Determination of the ARL and a contour nomogram for CUSUM charts to control normal mean.Technometrics,vol.13,no2,pp.221-230.P.L.G OLDSMITH and H.W HITFIELD(1961).Average run lengths in cumulative chart quality control schemes.Technometrics,vol.3,pp.11-20.G.C.G OODWIN and K.S.S IN(1984).Adaptive Filtering,Prediction and rmation and System Sciences Series,Prentice Hall,Englewood Cliffs,NJ.R.M.G RAY and L.D.D AVISSON(1986).Random Processes:a Mathematical Approach for Engineers. Information and System Sciences Series,Prentice Hall,Englewood Cliffs,NJ.C.G UEGUEN and L.L.S CHARF(1980).Exact maximum likelihood identification for ARMA models:a signal processing perspective.Proc.1st EUSIPCO,Lausanne.D.E.G USTAFSON, A.S.W ILLSKY,J.Y.W ANG,M.C.L ANCASTER and J.H.T RIEBWASSER(1978). ECG/VCG rhythm diagnosis using statistical signal analysis.Part I:Identification of persistent rhythms. Part II:Identification of transient rhythms.IEEE Trans.Biomedical Engineering,vol.BME-25,pp.344-353 and353-361.F.G USTAFSSON(1991).Optimal segmentation of linear regression parameters.Proc.IFAC/IFORS Symp. Identification and System Parameter Estimation,Budapest,pp.225-229.T.H¨AGGLUND(1983).New Estimation Techniques for Adaptive Control.Ph.D.Thesis,Lund Institute of Technology,Lund,Sweden.T.H¨AGGLUND(1984).Adaptive control of systems subject to large parameter changes.Proc.IFAC9th World Congress,Budapest.B IBLIOGRAPHY431 P.H ALL and C.C.H EYDE(1980).Martingale Limit Theory and its Application.Probability and Mathemat-ical Statistics,a Series of Monographs and Textbooks,Academic Press,New York.W.J.H ALL,R.A.W IJSMAN and J.K.G HOSH(1965).The relationship between sufficiency and invariance with applications in sequential analysis.Ann.Math.Statist.,vol.36,pp.576-614.E.J.H ANNAN and M.D EISTLER(1988).The Statistical Theory of Linear Systems.Series in Probability and Mathematical Statistics,Wiley,New York.J.D.H EALY(1987).A note on multivariate CuSum procedures.Technometrics,vol.29,pp.402-412.D.M.H IMMELBLAU(1970).Process Analysis by Statistical Methods.Wiley,New York.D.M.H IMMELBLAU(1978).Fault Detection and Diagnosis in Chemical and Petrochemical Processes. Chemical Engineering Monographs,vol.8,Elsevier,Amsterdam.W.G.S.H INES(1976a).A simple monitor of a system with sudden parameter changes.IEEE r-mation Theory,vol.IT-22,no2,pp.210-216.W.G.S.H INES(1976b).Improving a simple monitor of a system with sudden parameter changes.IEEE rmation Theory,vol.IT-22,no4,pp.496-499.D.V.H INKLEY(1969).Inference about the intersection in two-phase regression.Biometrika,vol.56,no3, pp.495-504.D.V.H INKLEY(1970).Inference about the change point in a sequence of random variables.Biometrika, vol.57,no1,pp.1-17.D.V.H INKLEY(1971).Inference about the change point from cumulative sum-tests.Biometrika,vol.58, no3,pp.509-523.D.V.H INKLEY(1971).Inference in two-phase regression.Jal American Statistical Association,vol.66, no336,pp.736-743.J.R.H UDDLE(1983).Inertial navigation system error-model considerations in Kalmanfiltering applica-tions.In Control and Dynamic Systems(C.T.Leondes,ed.),Academic Press,New York,pp.293-339.J.S.H UNTER(1986).The exponentially weighted moving average.Jal Quality Technology,vol.18,pp.203-210.I.A.I BRAGIMOV and R.Z.K HASMINSKII(1981).Statistical Estimation-Asymptotic Theory.Applications of Mathematics Series,vol.16.Springer,New York.R.I SERMANN(1984).Process fault detection based on modeling and estimation methods-A survey.Auto-matica,vol.20,pp.387-404.N.I SHII,A.I WATA and N.S UZUMURA(1979).Segmentation of nonstationary time series.Int.Jal Systems Sciences,vol.10,pp.883-894.J.E.J ACKSON and R.A.B RADLEY(1961).Sequential and tests.Annals Mathematical Statistics, vol.32,pp.1063-1077.B.J AMES,K.L.J AMES and D.S IEGMUND(1988).Conditional boundary crossing probabilities with appli-cations to change-point problems.Annals Probability,vol.16,pp.825-839.M.K.J EERAGE(1990).Reliability analysis of fault-tolerant IMU architectures with redundant inertial sen-sors.IEEE Trans.Aerospace and Electronic Systems,vol.AES-5,no.7,pp.23-27.N.L.J OHNSON(1961).A simple theoretical approach to cumulative sum control charts.Jal American Sta-tistical Association,vol.56,pp.835-840.N.L.J OHNSON and F.C.L EONE(1962).Cumulative sum control charts:mathematical principles applied to their construction and use.Parts I,II,III.Industrial Quality Control,vol.18,pp.15-21;vol.19,pp.29-36; vol.20,pp.22-28.432B IBLIOGRAPHY R.A.J OHNSON and M.B AGSHAW(1974).The effect of serial correlation on the performance of CUSUM tests-Part I.Technometrics,vol.16,no.1,pp.103-112.H.L.J ONES(1973).Failure Detection in Linear Systems.Ph.D.Thesis,Dept.Aeronautics and Astronautics, MIT,Cambridge,MA.R.H.J ONES,D.H.C ROWELL and L.E.K APUNIAI(1970).Change detection model for serially correlated multivariate data.Biometrics,vol.26,no2,pp.269-280.M.J URGUTIS(1984).Comparison of the statistical properties of the estimates of the change times in an autoregressive process.In Statistical Problems of Control,Issue65,Vilnius,pp.234-243(in Russian).T.K AILATH(1980).Linear rmation and System Sciences Series,Prentice Hall,Englewood Cliffs,NJ.L.V.K ANTOROVICH and V.I.K RILOV(1958).Approximate Methods of Higher Analysis.Interscience,New York.S.K ARLIN and H.M.T AYLOR(1975).A First Course in Stochastic Processes,2d ed.Academic Press,New York.S.K ARLIN and H.M.T AYLOR(1981).A Second Course in Stochastic Processes.Academic Press,New York.D.K AZAKOS and P.P APANTONI-K AZAKOS(1980).Spectral distance measures between gaussian pro-cesses.IEEE Trans.Automatic Control,vol.AC-25,no5,pp.950-959.K.W.K EMP(1958).Formula for calculating the operating characteristic and average sample number of some sequential tests.Jal Royal Statistical Society,vol.B-20,no2,pp.379-386.K.W.K EMP(1961).The average run length of the cumulative sum chart when a V-mask is used.Jal Royal Statistical Society,vol.B-23,pp.149-153.K.W.K EMP(1967a).Formal expressions which can be used for the determination of operating character-istics and average sample number of a simple sequential test.Jal Royal Statistical Society,vol.B-29,no2, pp.248-262.K.W.K EMP(1967b).A simple procedure for determining upper and lower limits for the average sample run length of a cumulative sum scheme.Jal Royal Statistical Society,vol.B-29,no2,pp.263-265.D.P.K ENNEDY(1976).Some martingales related to cumulative sum tests and single server queues.Stochas-tic Processes and Appl.,vol.4,pp.261-269.T.H.K ERR(1980).Statistical analysis of two-ellipsoid overlap test for real time failure detection.IEEE Trans.Automatic Control,vol.AC-25,no4,pp.762-772.T.H.K ERR(1982).False alarm and correct detection probabilities over a time interval for restricted classes of failure detection algorithms.IEEE rmation Theory,vol.IT-24,pp.619-631.T.H.K ERR(1987).Decentralizedfiltering and redundancy management for multisensor navigation.IEEE Trans.Aerospace and Electronic systems,vol.AES-23,pp.83-119.Minor corrections on p.412and p.599 (May and July issues,respectively).R.A.K HAN(1978).Wald’s approximations to the average run length in cusum procedures.Jal Statistical Planning and Inference,vol.2,no1,pp.63-77.R.A.K HAN(1979).Somefirst passage problems related to cusum procedures.Stochastic Processes and Applications,vol.9,no2,pp.207-215.R.A.K HAN(1981).A note on Page’s two-sided cumulative sum procedures.Biometrika,vol.68,no3, pp.717-719.B IBLIOGRAPHY433 V.K IREICHIKOV,V.M ANGUSHEV and I.N IKIFOROV(1990).Investigation and application of CUSUM algorithms to monitoring of sensors.In Statistical Problems of Control,Issue89,Vilnius,pp.124-130(in Russian).G.K ITAGAWA and W.G ERSCH(1985).A smoothness prior time-varying AR coefficient modeling of non-stationary covariance time series.IEEE Trans.Automatic Control,vol.AC-30,no1,pp.48-56.N.K LIGIENE(1980).Probabilities of deviations of the change point estimate in statistical models.In Sta-tistical Problems of Control,Issue83,Vilnius,pp.80-86(in Russian).N.K LIGIENE and L.T ELKSNYS(1983).Methods of detecting instants of change of random process prop-erties.Automation and Remote Control,vol.44,no10,Part II,pp.1241-1283.J.K ORN,S.W.G ULLY and A.S.W ILLSKY(1982).Application of the generalized likelihood ratio algorithm to maneuver detection and estimation.Proc.American Control Conf.,Arlington,V A,pp.792-798.P.R.K RISHNAIAH and B.Q.M IAO(1988).Review about estimation of change points.In Handbook of Statistics(P.R.Krishnaiah,C.R.Rao,eds.),vol.7,Elsevier,New York,pp.375-402.P.K UDVA,N.V ISWANADHAM and A.R AMAKRISHNAN(1980).Observers for linear systems with unknown inputs.IEEE Trans.Automatic Control,vol.AC-25,no1,pp.113-115.S.K ULLBACK(1959).Information Theory and Statistics.Wiley,New York(also Dover,New York,1968). K.K UMAMARU,S.S AGARA and T.S¨ODERSTR¨OM(1989).Some statistical methods for fault diagnosis for dynamical systems.In Fault Diagnosis in Dynamic Systems-Theory and Application(R.Patton,P.Frank,R. Clark,eds.).International Series in Systems and Control Engineering,Prentice Hall International,London, UK,pp.439-476.A.K USHNIR,I.N IKIFOROV and I.S AVIN(1983).Statistical adaptive algorithms for automatic detection of seismic signals-Part I:One-dimensional case.In Earthquake Prediction and the Study of the Earth Structure,Naouka,Moscow(Computational Seismology,vol.15),pp.154-159(in Russian).L.L ADELLI(1990).Diffusion approximation for a pseudo-likelihood test process with application to de-tection of change in stochastic system.Stochastics and Stochastics Reports,vol.32,pp.1-25.T.L.L A¨I(1974).Control charts based on weighted sums.Annals Statistics,vol.2,no1,pp.134-147.T.L.L A¨I(1981).Asymptotic optimality of invariant sequential probability ratio tests.Annals Statistics, vol.9,no2,pp.318-333.D.G.L AINIOTIS(1971).Joint detection,estimation,and system identifirmation and Control, vol.19,pp.75-92.M.R.L EADBETTER,G.L INDGREN and H.R OOTZEN(1983).Extremes and Related Properties of Random Sequences and Processes.Series in Statistics,Springer,New York.L.L E C AM(1960).Locally asymptotically normal families of distributions.Univ.California Publications in Statistics,vol.3,pp.37-98.L.L E C AM(1986).Asymptotic Methods in Statistical Decision Theory.Series in Statistics,Springer,New York.E.L.L EHMANN(1986).Testing Statistical Hypotheses,2d ed.Wiley,New York.J.P.L EHOCZKY(1977).Formulas for stopped diffusion processes with stopping times based on the maxi-mum.Annals Probability,vol.5,no4,pp.601-607.H.R.L ERCHE(1980).Boundary Crossing of Brownian Motion.Lecture Notes in Statistics,vol.40,Springer, New York.L.L JUNG(1987).System Identification-Theory for the rmation and System Sciences Series, Prentice Hall,Englewood Cliffs,NJ.。
The University of Florida Sparse Matrix Collection
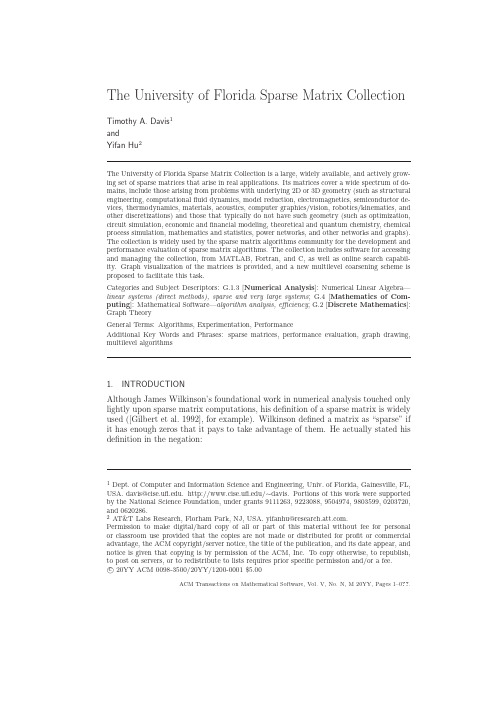
The University of Florida Sparse Matrix CollectionTimothy A.Davis1andYifan Hu2The University of Florida Sparse Matrix Collection is a large,widely available,and actively grow-ing set of sparse matrices that arise in real applications.Its matrices cover a wide spectrum of do-mains,include those arising from problems with underlying2D or3D geometry(such as structural engineering,computationalfluid dynamics,model reduction,electromagnetics,semiconductor de-vices,thermodynamics,materials,acoustics,computer graphics/vision,robotics/kinematics,and other discretizations)and those that typically do not have such geometry(such as optimization, circuit simulation,economic andfinancial modeling,theoretical and quantum chemistry,chemical process simulation,mathematics and statistics,power networks,and other networks and graphs). The collection is widely used by the sparse matrix algorithms community for the development and performance evaluation of sparse matrix algorithms.The collection includes software for accessing and managing the collection,from MATLAB,Fortran,and C,as well as online search capabil-ity.Graph visualization of the matrices is provided,and a new multilevel coarsening scheme is proposed to facilitate this task.Categories and Subject Descriptors:G.1.3[Numerical Analysis]:Numerical Linear Algebra—linear systems(direct methods),sparse and very large systems;G.4[Mathematics of Com-puting]:Mathematical Software—algorithm analysis,efficiency;G.2[Discrete Mathematics]: Graph TheoryGeneral Terms:Algorithms,Experimentation,PerformanceAdditional Key Words and Phrases:sparse matrices,performance evaluation,graph drawing, multilevel algorithms1.INTRODUCTIONAlthough James Wilkinson’s foundational work in numerical analysis touched only lightly upon sparse matrix computations,his definition of a sparse matrix is widely used([Gilbert et al.1992],for example).Wilkinson defined a matrix as“sparse”if it has enough zeros that it pays to take advantage of them.He actually stated his definition in the negation:1Dept.of Computer and Information Science and Engineering,Univ.of Florida,Gainesville,FL, USA.davis@cise.ufl.edu.http://www.cise.ufl.edu/∼davis.Portions of this work were supported by the National Science Foundation,under grants9111263,9223088,9504974,9803599,0203720, and0620286.2AT&T Labs Research,Florham Park,NJ,USA.yifanhu@.Permission to make digital/hard copy of all or part of this material without fee for personal or classroom use provided that the copies are not made or distributed for profit or commercial advantage,the ACM copyright/server notice,the title of the publication,and its date appear,and notice is given that copying is by permission of the ACM,Inc.To copy otherwise,to republish, to post on servers,or to redistribute to lists requires prior specific permission and/or a fee.c 20YY ACM0098-3500/20YY/1200-0001$5.00ACM Transactions on Mathematical Software,Vol.V,No.N,M20YY,Pages1–0??.2·T.A.Davis and Y.HuThe matrix may be sparse,either with the non-zero elements concen-trated on a narrow band centered on the diagonal or alternatively theymay be distributed in a less systematic manner.We shall refer to amatrix as dense if the percentage of zero elements or its distributionis such as to make it uneconomic to take advantage of their presence.[Wilkinson1971]In other words,if you can save time or memory(usually both)by exploiting the zeros,then the matrix is sparse.An interesting aspect of this definition is that it is dependent not only on the matrix,but on the algorithm used on the matrix.For example,a matrix may be“sparse”for an iterative method for solving linear systems or for a graph theoretic algorithm,but not for a sparse factorization method.This article describes the University of Florida Sparse Matrix Collection(here-after referred to as the UF Collection),which contains sparse matrices arising in a wide range of applications.Section2gives the motivation for collecting sparse matrices from real applications and making them widely available.Section3de-scribes the current state of the collection and the breadth of problems the matrices represent.Section4describes the algorithm used,and new techniques developed, for visualizing the matrices.Section5describes the three data formats used for storing the matrices,and the kinds of auxiliary information available for each ma-trix.Section6describes the four methods for searching and downloading matrices of interest:a MATLAB interface(UFget),a Java interface(UFgui),the matrix web pages(via a standard browser and a web-based search tool),and Amazon Web Services TM.Examples of how the UF Collection can be used for performance eval-uation are given in Section7.The future of the UF Collection depends critically upon the submission of new matrices,as discussed in Section8.Finally,Section9 summarizes the contributions of the UF Collection and its associated software.In this paper,a graph or mesh is said to have2D or3D geometry if its vertices have a position on an xy or xyz plane that naturally arises from the problem being solved.A sparse matrix is said to have2D or3D geometry if its nonzero pattern is the adjacency matrix of such a graph.The notation|A|refers to the number of nonzeros in a matrix.2.MOTIVATIONThe role of sparse matrices from real applications in the development,testing, and performance evaluation of sparse matrix algorithms has long been recognized. Thefirst established collection was started by Duffand Reid(1970to1979,[Duffand Reid1979]),and then compiled into the Harwell-Boeing collection by Duff, Grimes,and Lewis[Duffet al.1989].This collection provided the starting point of University of Florida Sparse Matrix Collection.Since the start of our collection in 1992,additional matrices have been added over the years,and other collections have been made,many of which have also been incorporated into the UF Collection(such as[Bai et al.1996;2008;Batagelj and Mrvar2008;Boisvert et al.2008;Boisvert et al.1997;Dumas2008;Gay2008;Gould et al.2008;Koster2008;M´e sz´a ros2008; Mittelmann2008;Resende et al.1995;Rudnyi2008;Rudnyi et al.2006;Saad2008; Schenk2008]).ACM Transactions on Mathematical Software,Vol.V,No.N,M20YY.University of Florida sparse matrix collection·3 The Matrix Market[Boisvert et al.1997]is the most similar collection to the UF Collection.Both collections include a search tool,and both categorize the matrices by application domain and problem source.Both provide matrices in similarfile formats.Both provide a web page for each matrix,with basic statistics andfigures. They differ in size,with the UF Collection containing much larger matrices and 4.5times as many matrices.The latest matrix added to the Matrix Market was in 2000,whereas UF collection is constantly being updated with new matrices.The largest matrix in the Matrix Market has dimension90,449with2.5million nonzeros, whereas the largest matrix in the UF Collection has a dimension of28million with 760million nonzeros.Nearly every matrix in the Matrix Market is also included in the UF Collection.However,the Matrix Market does include matrix generators; the UF Collection has no matrix generators.Nearly all research articles that include a section on the performance analysis of a sparse matrix algorithm include results on matrices from real applications or parametrized matrices that mimic those that could arise in practice.Since maintaining a large collection of matrices from real applications is not trivial,an alternative isfirst considered,namely,parametrized and random matrix generators.2.1Parameterized and random matricesRandomized or repeatable parametrized sequences of matrices can be easily con-structed via simple matrix generators.Examples are listed below,as a comparison and contrast with the UF Collection,which does not include any matrix generators.(1)Simple discretizations of the Laplace operator on square2D and3D meshes.(2)The L-shaped meshes of[George and Liu1981].(3)Least-squares problems from square2Dfinite-element meshes[George et al.1983].(4)The LAPACK test matrix generators[Anderson et al.1999],which can generatebanded matrices and sparse matrices with random nonzero pattern.These also appear in the Matrix Market.(5)The Non-Hermitian Eigenvalue Problem(NEP)matrix generators[Bai et al.1996;2008].These include regular2D meshes and random patterns;additional matrices are made available only as operators(y=Ax,where A is not explicitly represented).(6)Zlatev’s matrix generators,which create a sparse Toeplitz structure(whereselected diagonals are all nonzero)[Zlatev1991].(7)Higham’s Matrix Computational Toolbox[Higham2002],part of which appearsas the gallery function in MATLAB.The gallery contains three functions that generate parametrized sparse matrices from regular2D meshes(neumann, poisson,and wathen)and two that generate banded matrices(toeppen and tridiag).No randomization is used,except in the values(but not pattern) of the wathen matrices.Many of these matrix generators also appear in the Matrix Market.(8)Matrices with purely random nonzero patterns[Erd¨o s and R´e nyi1959;Gilbert1959].They can be generated by sprand,sprand,and sprandsym in MATLAB [Gilbert et al.1992].ACM Transactions on Mathematical Software,Vol.V,No.N,M20YY.4·T.A.Davis and Y.Hu(9)The YM11subroutine in HSL can generate random sparse matrices,with op-tions for ensuring structural nonsingularity and bandedness(a matrix is struc-turally nonsingular if there exists a permutation so that the matrix has a zero-free diagonal)[Duff2001].(10)In between the purely-random and purely-parametrized classes of matricesare partially randomized matrices with specific structure,as exemplified by the CONTEST toolbox[Taylor and Higham2009].They provide a set of graph generators,some of which use an underlying2D or3D geometry and some that do not.For example,they include an implementation of the small-world graphs of[Kleinberg2000],which are2D meshes with additional randomized edges to distant vertices in the work generators in CONTEST that do not have geometry include the scale-free graph models of[Barab´a si and Albert1999].The prime advantage of random and parametrized matrices is that they are very easy to generate in any size desired.Matrices from real applications are very difficult to generate and it is hard to vary them in size.Another key advantage of random and parametrized matrices is that asymptotic results can sometimes be derived.For example,the nested dissection ordering applied to a2D s-by-s mesh leads to an asymptotically optimal ordering for sparse Cholesky factorization,with31(n log2n)/8+O(n)nonzeros in the Cholesky factor L,and requiring829(n3/2)/84+O(n log n)floating point operations to compute, where n=s2is the dimension of the matrix[George and Liu1981].Purely random matrices may be useful for testing some sparse matrix applica-tions.For example,they can be useful for testing the convergence of iterative methods,assuming the generator has some control over the eigenvalue spectra(as is the case for sprandsym,for example).However,under Wilkinson’s definition they are not truly sparse when factorized by direct methods.With modest assumptions, purely random n-by-n matrices with O(n)nonzero entries require O(n3)time and O(n2)memory to factorize,because of catastrophicfill-in[Duff1974].Catastrophic fill-in very rarely occurs in matrices arising in real applications,and even when it does it is an indication that direct factorization methods are not applicable to that problem.Thus,performance obtained on purely random matrices will not indicate how well a sparse matrix factorization method will work on matrices from real ap-plications.Purely random networks also do not accurately model the characteristics of real networks[Watts and Strogatz1998].In between the two extremes of highly structured matrices(banded,or square 2D and3D meshes,for example)and purely randomized matrices is another class of matrix generators that can create matrices with some regular structure and a carefully selected random structure.The small-world graphs from the CONTEST toolbox are one such example.These are constructed to capture essential properties of large networks,such as the small-world property,scale-free degree distribution, motifs,and graphlet frequency[Taylor and Higham2009].These models are very useful for network algorithms,but they have not yet been shown to mimic the per-formance of sparse matrix factorization methods on matrices from real applications. ACM Transactions on Mathematical Software,Vol.V,No.N,M20YY.University of Florida sparse matrix collection·5Fig.1.Sparse matrix pattern of a RAH-66Comanche helicopter2.2Sparse matrices from real applicationsWhile useful,random and parametrized matrices have their limitations.This mo-tivates the development of the UF Collection,which focuses on matrices from real applications1.One of the matrices in the UF Collection is a complete representation of a Boe-ing/Sikorsky RAH-66Comanche helicopter,with3D geometry(obtained from Alex Pothen).Figure1shows the sparsity pattern of the matrix.Nested dissection is still useful for this problem,but asymptotic results cannot be derived for a complex structure such as this one.A simple square3D mesh would not be a good approxi-mation to this graph,since it would not capture the properties of the long helicopter rotors,or the topology of the hole where the rear rotor is located.This matrix is one of the few in the collection where the3D coordinates of the vertices are given;a picture of the graph drawing when these coordinates are used is shown in Figure2, on the left.For comparison,a force-directed graph drawing[Hu2005],which recre-ates these coordinates based only on the connectivity of the graph,is shown on the right.The method for creating thisfigure is discussed in Section4.The structure is warped,with the tail rotor twisted out of its housing(to the right)and itsfive main rotors shrunken(on the top),but it is still clear that the graph represents some kind of3D problem.Edge colors in the force-directed graphs represent the distance between two vertices.Figure3is the nonzero pattern of matrix arising from circuit simulation(left)and its force-directed graph drawing(right).The graphs of the Comanche helicopter and this electronic circuit indicate that one is a3D problem and the other is a network with no2D or3D geometry.This difference is not clear by merely comparing the nonzero patterns of their matrices.Parameterized matrices can capture many key properties of matrices from real applications,such as the regularity of a3D mesh,or the small-world properties of a network,but they cannot capture the rich and complex structure of matrices such1The UF Collection does include a handful of random matrices,which remain in the collection for historical reasons.ACM Transactions on Mathematical Software,Vol.V,No.N,M20YY.6·T.A.Davis and Y.HuFig.2.Graph of a RAH-66Comanche helicopter,using given3D coordinates(left)and its force-directed graph drawing(right)Fig.3.Sparse matrix pattern of an electronic circuit from Steve Hamm,Motorola(left)and its force-directed graph(right)as those presented above.2.3Collecting the matricesThe ideal matrix collection would be informally representative of matrices that arise in practice.It should cast a broad net so as to capture matrices from every applica-tion domain that relies on sparse matrix methods.For example,matrices arising in circuit simulation(and other network-related domains)differ greatly from matrices arising from the discretization of2D and3D physical domains;this can be clearly seen in the precedingfiputationalfluid dynamics matrices differ from structural engineering matrices,and both are vastly different from matrices arising in linear programming orfinancial portfolio optimization.The collection should be kept up to date,since matrices of interest grow in size each year as computer mem-ories get larger.New application domains also appear,such as eigenvalue problems arising in web connectivity matrices[Kamvar2008;Kamvar et al.2004;Page et al. 1998],which have existed only since the mid1990’s.ACM Transactions on Mathematical Software,Vol.V,No.N,M20YY.University of Florida sparse matrix collection·7 Sparse matrix algorithm developers use the matrices in the UF Collection to develop their methods,since theoretical asymptotic time/memory complexity anal-ysis only goes so far.If there are no matrices available to developers from a given application domain,it is quite possible that when their methods are used in that domain,the performance results will be disappointing.This provides a strong mo-tivation for computational scientists to submit their matrices to a widely available collection such as this one,so that gaps can be avoided.Thus,new application areas are always being added to the collection.Our strategy for adding matrices to the collection is simple,although admittedly ad hoc.Thefirst author maintains a collection of codes for the direct solution of sparse linear systems.End-users of this software are uniformly requested to submit matrices to the collection.Additional matrices are requested when they are found cited in articles and conference presentations,which includes a wider range of matrices(such as graphs arising in network analysis).Any matrices received are included,unless they are clear duplications of matrices already in the collection. Small matrices are not included,unless they are subsets of a larger collection(such as the Pajek data set).This strategy does introduce an unavoidable source of bias,but we have at-tempted to avoid this bias by relying on matrices collected by others.The UF Collection includes many sets of matrices collected in this way,such as those col-lected by Saad,who develops iterative methods for sparse linear systems[Saad 2003].Not all matrices in the collection arise from a sparse linear system.For example,many linear programming problems have been included in the UF Collec-tion[Gay2008;M´e sz´a ros2008;Mittelmann2008;Resende et al.1995].Network and combinatorial problems are also included,such as a matrix of prime numbers from Problem7of Trefethen’s100-digit challenge[Trefethen2002].Once a matrix is collected,its maintenance is the responsibility of thefirst author. This guards against arbitrary modifications in the matrices,which can occur when the matrix submitters modify their matrix problems.Repeatability of experiments based on the UF Collection is thus ensured.3.DESCRIPTION OF THE COLLECTIONAs of March2010the UF Sparse Matrix Collection consists of2272problems,each of which contains at least one sparse matrix(typically just one).It often represents a sparse linear system of the form Ax=b,where b is sometimes provided as well as the sparse matrix A.Many other problems are eigenvalue problems,and many matrices come from unstructured graphs and networks with no associated linear system.In some cases,a problem consists of a sequence of closely related matrices, such as Jacobian matrices arising in the solution of a nonlinear system.Some problems include two sparse matrices,such as a stiffness matrix and a mass matrix from a structural engineering eigenvalue problem.In this case,A is the stiffness matrix,and the mass matrix appears as an auxiliary matrix in the problem.3.1Application areasThe collection is divided into157different matrix groups,with more groups added as new matrices are submitted to the collection.A complete list of these groups is too long to include here;details are given on the collection’s web site.Each groupACM Transactions on Mathematical Software,Vol.V,No.N,M20YY.8·T.A.Davis and Y.HuTable I.Partial list of sources of matricesNon-Hermitian Eigenvalue Problems[Bai et al.1996]Pajek Networks[Batagelj and Mrvar2008]Multistage stochasticfinancial modeling[Berger et al.1995]The Matrix Market(collection)[Boisvert et al.1997]Univ.of Utrecht circuit simulation[Bomhof and van der Vorst2000]MRI reconstruction M.Bydder,UCSDHarwell-Boeing Collection[Duffet al.1989]Combinatorial problems[Dumas2008]Frequency domain,nonlinear analog circuits[Feldmann et al.1996]NETLIB Linear Programming Test Problems[Gay2008]Symmetric sparse matrix benchmarks[Gould et al.2008]Linear programming problems[Gupta1996]Stanford/Berkeley Web Matrices[Kamvar et al.2004]Xyce circuit simulation matrices[Keiter et al.2003]Computer vision problems[Kemelmacher2005]PARASOL Matrix Collection[Koster2008]Linear programming test set[M´e sz´a ros2008]2D and3D semiconductor physics[Miller and Wang1991]Linear programming test set[Mittelmann2008]QAPLIB,quadratic assignment[Resende et al.1995]Oberwolfach Model Reduction Benchmarks[Rudnyi et al.2006]SPARSKIT matrix collection[Saad2008]Univ.of Basel Collection[Schenk2008]DNA electrophoresis matrices[van Heukelum et al.2002]Chemical engineering problems[Zitney1992;Zitney et al.1996]typically consists of a set of matrices from a single source.A few of the matrix groups in the UF Collection consist of entire sets of matrices from another sparse matrix collection.In this case,the group may consist of matrices from very different application areas.For example,the Harwell-Boeing collection forms a single group [Duffand Reid1979;Duffet al.1989].Sources(papers and web sites)for some of the matrix groups are listed in Table I,which gives aflavor of the range of problems the collection contains.The group of a problem forms part of the full name of the problem.For exam-ple,the full name of the west0479problem in the Harwell-Boeing collection(the HB group in the UF sparse matrix collection)is HB/west0479.In addition,all problems are tagged with a string,kind,which indicates the application domain of the prob-lem.The group and kind of a problem are not the same.Some groups have many different kinds of problems,and problems of the same kind can appear in different groups.For example,the HB/west0479problem is in the HB group,and its kind is tagged as a“chemical process simulation problem.”Five other groups include chemical process simulation problems,namely,the Bai,Grund,Mallya,VanVelzen, and Zitney groups,all of which are named after the person from whom the matrices were obtained.A complete list of the kind strings for all problems is given in Table II.The table is split into two categories:problems with no underlying geometry and problems with2D or3D geometry.The collection contains68matrices with random nonzero pattern.They appear in the collection only because they already occur as widely-used test problems in ACM Transactions on Mathematical Software,Vol.V,No.N,M20YY.University of Florida sparse matrix collection·9 Table II.Summary of Problem.kind for all2272problems1516problems with no2D/3D geometry70chemical process simulation problem251circuit simulation problem299combinatorial problem11counter-example problem68economic problem4frequency-domain circuit simulation problem23least squares problem342linear programming problem135optimization problem56power network problem10statistical/mathematical problem61theoretical/quantum chemistry problem88directed graph8bipartite graph23undirected graph68random graph756problems with2D/3D geometry13acoustics problem166computationalfluid dynamics problem12computer graphics/vision problem44electromagnetics problem28materials problem42model reduction problem3robotics problem35semiconductor device problem288structural problem31thermal problem942D/3D problem(other than those listed above)another collection that was subsequently added to the UF sparse matrix collection. In retrospect,their inclusion in the UF Collection was a mistake,but matrices are never removed once added,since this would cause confusion when replicating results that use the collection.We guarantee statements such as“all matrices with property X in the collection as of date Y”always give a single consistent answer.3.2Matrix statisticsThe2272matrices in the collection come from359different authors and50different editors.A matrix editor is the one who collected the matrix for inclusion into any established collection(not just the UF Collection2,but also for others such as the Harwell/Boeing collection3,the Matrix Market4,or the GRID/TSLE collection5).A matrix author is the one who created the matrix.Each matrix has an editor and an author(sometimes the same person).Editors contributing at least10matrices are listed in Table III(sorted by the number of matrices collected).Figures4and5plot the matrix size(dimension and number of nonzeros)versus 2UF Sparse Matrix Collection:http://www.cise.ufl.edu/research/sparse/matrices3Harwell/Boeing collection:/nag/hb/hb.shtml4Matrix Market:/MatrixMarket5GRID/TLSE collection:http://gridtlse.enseeiht.fr:8080/websolve/ACM Transactions on Mathematical Software,Vol.V,No.N,M20YY.10·T.A.Davis and Y.HuFig.4.Matrix dimension(the largest of row/column dimension if rectangular)versus year created. The solid line is the cumulative sum.Fig.5.Number of nonzeros in each matrix versus year created.The solid line is the cumulative sum.ACM Transactions on Mathematical Software,Vol.V,No.N,M20YY.University of Florida sparse matrix collection·11 Table III.Primary editors of the UF Collection#matrices editor720T.Davis293J.-G.Dumas255I.Duff,R.Grimes,J.Lewis166 C.Meszaros103O.Schenk78Z.Bai,D.Day,J.Demmel,J.Dongarra72V.Batagelj67Y.Ye61 A.Baggag,Y.Saad48 D.Gay43R.Fourer39N.Gould,Y.Hu,J.Scott38 E.Rudnyi35G.Kumfert,A.Pothen34 A.Curtis,I.Duff,J.Reid28J.Chinneck27N.Gould25H.Mittelmann23R.Boisvert,R.Pozo,K.Remington,ler,R.Lipman,R.Barrett,J.Dongarra23 F.Grund22J.Koster13I.Lustig12H.Simon11M.ResendeFig.6.Overall histograms of matrix dimensions and nonzerosACM Transactions on Mathematical Software,Vol.V,No.N,M20YY.12·T.A.Davis and Y.Huthe year in which the matrices were created.The solid line in thefigures is the cumulative sum of the data plotted.Bothfigures show an exponential growth in problem sizes,similar to how computer memory sizes have grown since1970. Note that small problems are still being added to the collection.This is because a matrix group often includes a range of related problems,from small test cases to the largest problems of interest.The outlier matrix in1971is from the Edinburgh Associative Thesaurus,located at and obtained from the Pajek data set[Batagelj and Mrvar 2008].It is a graph with23,219vertices and325,592edges that wasfirst constructed in1971[Kiss et al.1973].Figure6plots two histograms of the overall distribution of matrices in the col-lection.4.VISUALIZING THE COLLECTIONMany basic facts,including symmetry,structural rank,ordering statistics,as well as a plot of the sparsity pattern,are given for each matrix in the collection.However these facts alone do not always give sufficient information about the matrix.For example,does this matrix come from an application involving2D or3D mesh?Or from a small-world network?Are there other structures in the applications that are not discernible from a plot of the sparsity pattern?To help answer these questions,a visualization of each matrix in the form of graph drawing is provided.If the matrix is structurally symmetric,it is taken as the adjacency matrix of an undirected graph,where two vertices i and j are connected if the(i,j)-th entry of the matrix is nonzero.Rectangular or structurally unsymmetric matrices are treated as bipartite graphs.More specifically,the augmented matrix0A A T0is used as the adjacency matrix of an undirected bipartite graph whose vertices are composed of the rows and columns of the matrix.We provide two graphs for square matrices with unsymmetric structure:the graph of A+A T and the augmented matrix above.The graph drawings depend only on the nonzero pattern,not the values.The basic algorithm used for drawing the graphs is a multilevel force-directed algorithm[Hu2005].However this algorithm fails to produce informative drawings for some matrices.We propose here a new coarsening scheme to deal with these cases.In the following we briefly describe the basic algorithm,followed by the new coarsening scheme.4.1The graph drawing algorithmThe basic algorithm[Hu2005]employs a force-directed model[Fruchterman and Reingold1991].Vertices are treated as particles with electrical charges that push each other away.This repulsive force F r(i,j)between any two vertices i and j is proportional to the inverse of their distance,F r(i,j)=K2x i−x j ,i=j.ACM Transactions on Mathematical Software,Vol.V,No.N,M20YY.。
Nonlinear Dynamics and Systems Theory, 7(4) (2007) 399–408 Observer Design for a Class of
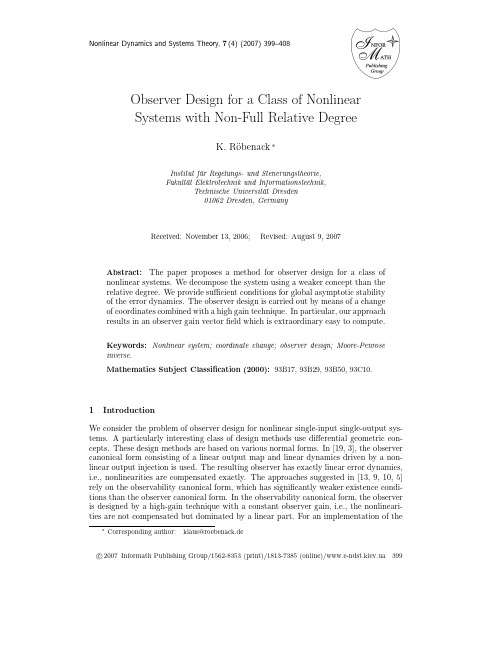
1
Introduction
We consider the problem of observer design for nonlinear single-input single-output systems. A particularly interesting class of design methods use differential geometric concepts. These design methods are based on various normal forms. In [19, 3], the observer canonical form consisting of a linear output map and linear dynamics driven by a nonlinear output injection is used. The resulting observer has exactly linear error dynamics, i.e., nonlinearities are compensated exactly. The approaches suggested in [13, 9, 10, 5] rely on the observability canonical form, which has significantly weaker existence conditions than the observer canonical form. In the observability canonical form, the observer is designed by a high-gain technique with a constant observer gain, i.e., the nonlinearities are not compensated but dominated by a linear part. For an implementation of the
扩展巴科斯范式(转自维基)
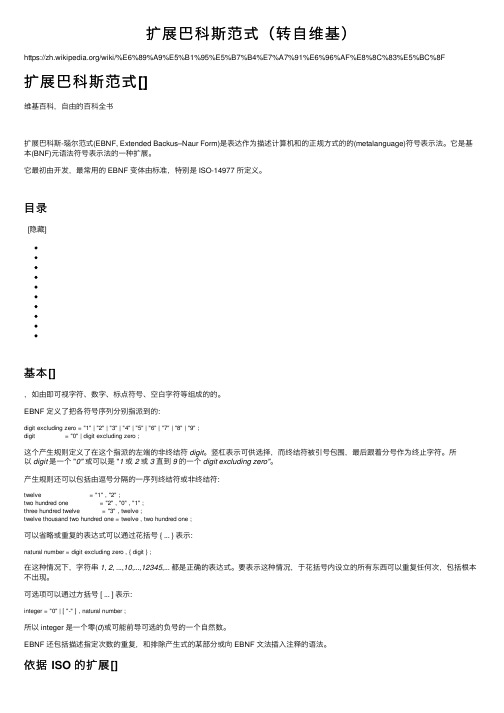
扩展巴科斯范式(转⾃维基)https:///wiki/%E6%89%A9%E5%B1%95%E5%B7%B4%E7%A7%91%E6%96%AF%E8%8C%83%E5%BC%8F扩展巴科斯范式[]维基百科,⾃由的百科全书扩展巴科斯-瑙尔范式(EBNF, Extended Backus–Naur Form)是表达作为描述计算机和的正规⽅式的的(metalanguage)符号表⽰法。
它是基本(BNF)元语法符号表⽰法的⼀种扩展。
它最初由开发,最常⽤的 EBNF 变体由标准,特别是 ISO-14977 所定义。
⽬录[隐藏]基本[],如由即可视字符、数字、标点符号、空⽩字符等组成的的。
EBNF 定义了把各符号序列分别指派到的:digit excluding zero = "1" | "2" | "3" | "4" | "5" | "6" | "7" | "8" | "9" ;digit = "0" | digit excluding zero ;这个产⽣规则定义了在这个指派的左端的⾮终结符digit。
竖杠表⽰可供选择,⽽终结符被引号包围,最后跟着分号作为终⽌字符。
所以digit是⼀个 "0"或可以是 "1或2或3直到9的⼀个digit excluding zero"。
产⽣规则还可以包括由逗号分隔的⼀序列终结符或⾮终结符:twelve = "1" , "2" ;two hundred one = "2" , "0" , "1" ;three hundred twelve = "3" , twelve ;twelve thousand two hundred one = twelve , two hundred one ;可以省略或重复的表达式可以通过花括号 { ... } 表⽰:natural number = digit excluding zero , { digit } ;在这种情况下,字符串1, 2, ...,10,...,12345,... 都是正确的表达式。
甘氨酸十二烷基磺电泳缓冲液酸钠。...
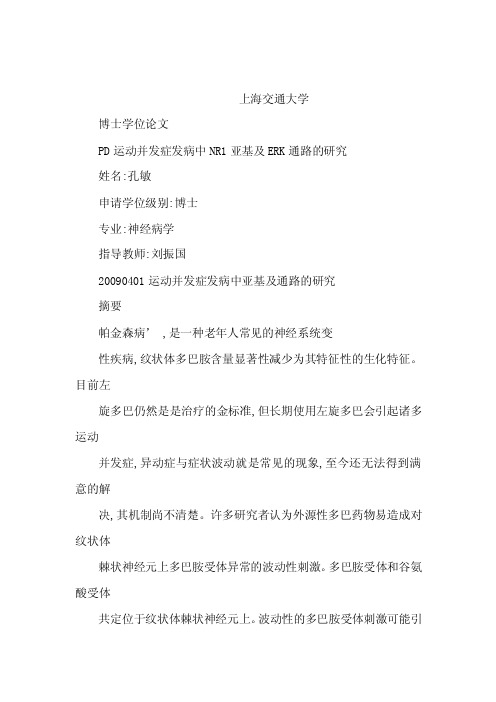
上海交通大学博士学位论文PD运动并发症发病中NR1亚基及ERK通路的研究姓名:孔敏申请学位级别:博士专业:神经病学指导教师:刘振国20090401运动并发症发病中亚基及通路的研究摘要帕金森病’ ,是一种老年人常见的神经系统变性疾病,纹状体多巴胺含量显著性减少为其特征性的生化特征。
目前左旋多巴仍然是是治疗的金标准,但长期使用左旋多巴会引起诸多运动并发症,异动症与症状波动就是常见的现象,至今还无法得到满意的解决,其机制尚不清楚。
许多研究者认为外源性多巴药物易造成对纹状体棘状神经元上多巴胺受体异常的波动性刺激。
多巴胺受体和谷氨酸受体共定位于纹状体棘状神经元上。
波动性的多巴胺受体刺激可能引神经元信号转导通路的变化,进而影响谷氨酸受体的状态及突触传递效率。
纹状体神经元上反应性改变导致了左旋多巴诱发的运动并发症的出现。
是促离子型谷氨酸受体中的一种,其特性取决于受体的亚单位组成及磷酸化状态。
受体拮抗剂可减轻左旋多巴诱发的运动并发症,这说明受体与运动并发症的发生相关。
最近研究表明, 左旋多巴长期刺激确实改变了的特性。
因此,本课题首先对受体通道的基本亚单位的特性进行研究。
同时,左旋多巴撤药后,运动反应改变会逐渐缓和,但再次使用左旋多巴后这些运动反应现象又很快出现。
短期使用左旋多巴则不会出现上述运动反应改变。
这提示我们可通过比较的方法,观察急性与长期左旋多巴作用后撤药对大鼠行为学与特性改变的影响,以期探讨它们之间的关系。
此外,目前迫切需要寻找和研发新型的抗药物,以替代和弥补已有药物的缺陷和不足。
研究发现长期非生理性的多巴胺波动性刺激激活了纹状体棘状神经元内多种激酶,并导致谷氨酸受体磷酸化。
故而,我们设想通过抑白激酶活性来减轻受体磷酸化而达到防止运动并发症的目的。
近年来以受体激活为起点,细胞内信号转导通路为主线,构成运动并发症研究的重点。
细胞外信号调节激酶是丝裂原激活的蛋白激酶家族中的一个,被认为是从细胞表面到细胞核信号转导的重要调制点。
基于自转一阶非连续式微球双平盘研磨的运动学分析与实验研究
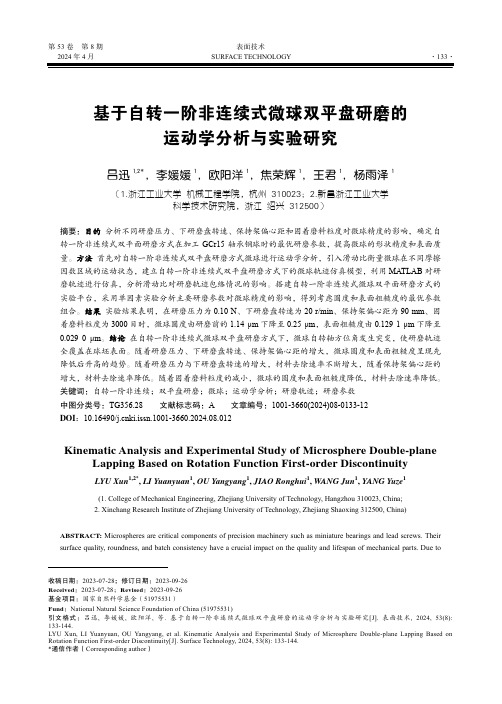
第53卷第8期表面技术2024年4月SURFACE TECHNOLOGY·133·基于自转一阶非连续式微球双平盘研磨的运动学分析与实验研究吕迅1,2*,李媛媛1,欧阳洋1,焦荣辉1,王君1,杨雨泽1(1.浙江工业大学 机械工程学院,杭州 310023;2.新昌浙江工业大学科学技术研究院,浙江 绍兴 312500)摘要:目的分析不同研磨压力、下研磨盘转速、保持架偏心距和固着磨料粒度对微球精度的影响,确定自转一阶非连续式双平面研磨方式在加工GCr15轴承钢球时的最优研磨参数,提高微球的形状精度和表面质量。
方法首先对自转一阶非连续式双平盘研磨方式微球进行运动学分析,引入滑动比衡量微球在不同摩擦因数区域的运动状态,建立自转一阶非连续式双平盘研磨方式下的微球轨迹仿真模型,利用MATLAB对研磨轨迹进行仿真,分析滑动比对研磨轨迹包络情况的影响。
搭建自转一阶非连续式微球双平面研磨方式的实验平台,采用单因素实验分析主要研磨参数对微球精度的影响,得到考虑圆度和表面粗糙度的最优参数组合。
结果实验结果表明,在研磨压力为0.10 N、下研磨盘转速为20 r/min、保持架偏心距为90 mm、固着磨料粒度为3000目时,微球圆度由研磨前的1.14 μm下降至0.25 μm,表面粗糙度由0.129 1 μm下降至0.029 0 μm。
结论在自转一阶非连续式微球双平盘研磨方式下,微球自转轴方位角发生突变,使研磨轨迹全覆盖在球坯表面。
随着研磨压力、下研磨盘转速、保持架偏心距的增大,微球圆度和表面粗糙度呈现先降低后升高的趋势。
随着研磨压力与下研磨盘转速的增大,材料去除速率不断增大,随着保持架偏心距的增大,材料去除速率降低。
随着固着磨料粒度的减小,微球的圆度和表面粗糙度降低,材料去除速率降低。
关键词:自转一阶非连续;双平盘研磨;微球;运动学分析;研磨轨迹;研磨参数中图分类号:TG356.28 文献标志码:A 文章编号:1001-3660(2024)08-0133-12DOI:10.16490/ki.issn.1001-3660.2024.08.012Kinematic Analysis and Experimental Study of Microsphere Double-plane Lapping Based on Rotation Function First-order DiscontinuityLYU Xun1,2*, LI Yuanyuan1, OU Yangyang1, JIAO Ronghui1, WANG Jun1, YANG Yuze1(1. College of Mechanical Engineering, Zhejiang University of Technology, Hangzhou 310023, China;2. Xinchang Research Institute of Zhejiang University of Technology, Zhejiang Shaoxing 312500, China)ABSTRACT: Microspheres are critical components of precision machinery such as miniature bearings and lead screws. Their surface quality, roundness, and batch consistency have a crucial impact on the quality and lifespan of mechanical parts. Due to收稿日期:2023-07-28;修订日期:2023-09-26Received:2023-07-28;Revised:2023-09-26基金项目:国家自然科学基金(51975531)Fund:National Natural Science Foundation of China (51975531)引文格式:吕迅, 李媛媛, 欧阳洋, 等. 基于自转一阶非连续式微球双平盘研磨的运动学分析与实验研究[J]. 表面技术, 2024, 53(8): 133-144.LYU Xun, LI Yuanyuan, OU Yangyang, et al. Kinematic Analysis and Experimental Study of Microsphere Double-plane Lapping Based on Rotation Function First-order Discontinuity[J]. Surface Technology, 2024, 53(8): 133-144.*通信作者(Corresponding author)·134·表面技术 2024年4月their small size and light weight, existing ball processing methods are used to achieve high-precision machining of microspheres. Traditional concentric spherical lapping methods, with three sets of circular ring trajectories, result in poor lapping accuracy. To achieve efficient and high-precision processing of microspheres, the work aims to propose a method based on the first-order discontinuity of rotation for double-plane lapping of microspheres. Firstly, the principle of the first-order discontinuity of rotation for double-plane lapping of microspheres was analyzed, and it was found that the movement of the microsphere changed when it was in different regions of the upper variable friction plate, resulting in a sudden change in the microsphere's rotational axis azimuth and expanding the lapping trajectory. Next, the movement of the microsphere in the first-order discontinuity of rotation for double-plane lapping method was analyzed, and the sliding ratio was introduced to measure the motion state of the microsphere in different friction coefficient regions. It was observed that the sliding ratio of the microsphere varied in different friction coefficient regions. As a result, when the microsphere passed through the transition area between the large and small friction regions of the upper variable friction plate, the sliding ratio changed, causing a sudden change in the microsphere's rotational axis azimuth and expanding the lapping trajectory. The lapping trajectory under different sliding ratios was simulated by MATLAB, and the results showed that with the increase in simulation time, the first-order discontinuity of rotation for double-plane lapping method could achieve full coverage of the microsphere's lapping trajectory, making it more suitable for precision machining of microspheres. Finally, based on the above research, an experimental platform for the first-order discontinuity of rotation for double-plane lapping of microsphere was constructed. With 1 mm diameter bearing steel balls as the processing object, single-factor experiments were conducted to study the effects of lapping pressure, lower plate speed, eccentricity of the holding frame, and grit size of fixed abrasives on microsphere roundness, surface roughness, and material removal rate. The experimental results showed that under the first-order discontinuity of rotation for double-plane lapping, the microsphere's rotational axis azimuth underwent a sudden change, leading to full coverage of the lapping trajectory on the microsphere's surface. Under the lapping pressure of 0.10 N, the lower plate speed of 20 r/min, the eccentricity of the holder of 90 mm, and the grit size of fixed abrasives of 3000 meshes, the roundness of the microsphere decreased from 1.14 μm before lapping to 0.25 μm, and the surface roughness decreased from 0.129 1 μm to 0.029 0 μm. As the lapping pressure and lower plate speed increased, the microsphere roundness and surface roughness were firstly improved and then deteriorated, while the material removal rate continuously increased. As the eccentricity of the holding frame increased, the roundness was firstly improved and then deteriorated, while the material removal rate decreased. As the grit size of fixed abrasives decreased, the microsphere's roundness and surface roughness were improved, and the material removal rate decreased. Through the experiments, the optimal parameter combination considering roundness and surface roughness is obtained: lapping pressure of 0.10 N/ball, lower plate speed of 20 r/min, eccentricity of the holder of 90 mm, and grit size of fixed abrasives of 3000 meshes.KEY WORDS: rotation function first-order discontinuity; double-plane lapping; microsphere; kinematic analysis; lapping trajectory; lapping parameters随着机械产品朝着轻量化、微型化的方向发展,微型电机、仪器仪表等多种工业产品对微型轴承的需求大量增加。
离散数学中英文名词对照表
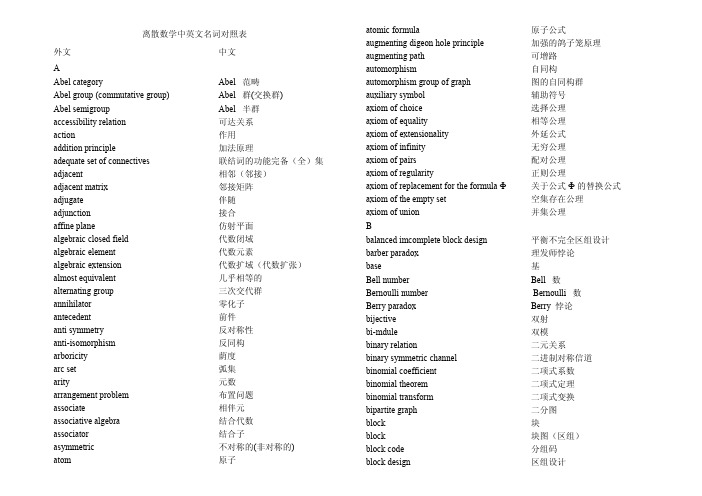
离散数学中英文名词对照表外文中文AAbel category Abel 范畴Abel group (commutative group) Abel 群(交换群)Abel semigroup Abel 半群accessibility relation 可达关系action 作用addition principle 加法原理adequate set of connectives 联结词的功能完备(全)集adjacent 相邻(邻接)adjacent matrix 邻接矩阵adjugate 伴随adjunction 接合affine plane 仿射平面algebraic closed field 代数闭域algebraic element 代数元素algebraic extension 代数扩域(代数扩张)almost equivalent 几乎相等的alternating group 三次交代群annihilator 零化子antecedent 前件anti symmetry 反对称性anti-isomorphism 反同构arboricity 荫度arc set 弧集arity 元数arrangement problem 布置问题associate 相伴元associative algebra 结合代数associator 结合子asymmetric 不对称的(非对称的)atom 原子atomic formula 原子公式augmenting digeon hole principle 加强的鸽子笼原理augmenting path 可增路automorphism 自同构automorphism group of graph 图的自同构群auxiliary symbol 辅助符号axiom of choice 选择公理axiom of equality 相等公理axiom of extensionality 外延公式axiom of infinity 无穷公理axiom of pairs 配对公理axiom of regularity 正则公理axiom of replacement for the formula Ф关于公式Ф的替换公式axiom of the empty set 空集存在公理axiom of union 并集公理Bbalanced imcomplete block design 平衡不完全区组设计barber paradox 理发师悖论base 基Bell number Bell 数Bernoulli number Bernoulli 数Berry paradox Berry 悖论bijective 双射bi-mdule 双模binary relation 二元关系binary symmetric channel 二进制对称信道binomial coefficient 二项式系数binomial theorem 二项式定理binomial transform 二项式变换bipartite graph 二分图block 块block 块图(区组)block code 分组码block design 区组设计Bondy theorem Bondy 定理Boole algebra Boole 代数Boole function Boole 函数Boole homomorophism Boole 同态Boole lattice Boole 格bound occurrence 约束出现bound variable 约束变量bounded lattice 有界格bridge 桥Bruijn theorem Bruijn 定理Burali-Forti paradox Burali-Forti 悖论Burnside lemma Burnside 引理Ccage 笼canonical epimorphism 标准满态射Cantor conjecture Cantor 猜想Cantor diagonal method Cantor 对角线法Cantor paradox Cantor 悖论cardinal number 基数Cartesion product of graph 图的笛卡儿积Catalan number Catalan 数category 范畴Cayley graph Cayley 图Cayley theorem Cayley 定理center 中心characteristic function 特征函数characteristic of ring 环的特征characteristic polynomial 特征多项式check digits 校验位Chinese postman problem 中国邮递员问题chromatic number 色数chromatic polynomial 色多项式circuit 回路circulant graph 循环图circumference 周长class 类classical completeness 古典完全的classical consistent 古典相容的clique 团clique number 团数closed term 闭项closure 闭包closure of graph 图的闭包code 码code element 码元code length 码长code rate 码率code word 码字coefficient 系数coimage 上象co-kernal 上核coloring 着色coloring problem 着色问题combination number 组合数combination with repetation 可重组合common factor 公因子commutative diagram 交换图commutative ring 交换环commutative seimgroup 交换半群complement 补图(子图的余) complement element 补元complemented lattice 有补格complete bipartite graph 完全二分图complete graph 完全图complete k-partite graph 完全k-分图complete lattice 完全格composite 复合composite operation 复合运算composition (molecular proposition) 复合(分子)命题composition of graph (lexicographic product)图的合成(字典积)concatenation (juxtaposition) 邻接运算concatenation graph 连通图congruence relation 同余关系conjunctive normal form 正则合取范式connected component 连通分支connective 连接的connectivity 连通度consequence 推论(后承)consistent (non-contradiction) 相容性(无矛盾性)continuum 连续统contraction of graph 图的收缩contradiction 矛盾式(永假式)contravariant functor 反变函子coproduct 上积corank 余秩correct error 纠正错误corresponding universal map 对应的通用映射countably infinite set 可列无限集(可列集)covariant functor (共变)函子covering 覆盖covering number 覆盖数Coxeter graph Coxeter 图crossing number of graph 图的叉数cuset 陪集cotree 余树cut edge 割边cut vertex 割点cycle 圈cycle basis 圈基cycle matrix 圈矩阵cycle rank 圈秩cycle space 圈空间cycle vector 圈向量cyclic group 循环群cyclic index 循环(轮转)指标cyclic monoid 循环单元半群cyclic permutation 圆圈排列cyclic semigroup 循环半群DDe Morgan law De Morgan 律decision procedure 判决过程decoding table 译码表deduction theorem 演绎定理degree 次数,次(度)degree sequence 次(度)序列derivation algebra 微分代数Descartes product Descartes 积designated truth value 特指真值detect errer 检验错误deterministic 确定的diagonal functor 对角线函子diameter 直径digraph 有向图dilemma 二难推理direct consequence 直接推论(直接后承)direct limit 正向极限direct sum 直和directed by inclution 被包含关系定向discrete Fourier transform 离散 Fourier 变换disjunctive normal form 正则析取范式disjunctive syllogism 选言三段论distance 距离distance transitive graph 距离传递图distinguished element 特异元distributive lattice 分配格divisibility 整除division subring 子除环divison ring 除环divisor (factor) 因子domain 定义域Driac condition Dirac 条件dual category 对偶范畴dual form 对偶式dual graph 对偶图dual principle 对偶原则(对偶原理) dual statement 对偶命题dummy variable 哑变量(哑变元)Eeccentricity 离心率edge chromatic number 边色数edge coloring 边着色edge connectivity 边连通度edge covering 边覆盖edge covering number 边覆盖数edge cut 边割集edge set 边集edge-independence number 边独立数eigenvalue of graph 图的特征值elementary divisor ideal 初等因子理想elementary product 初等积elementary sum 初等和empty graph 空图empty relation 空关系empty set 空集endomorphism 自同态endpoint 端点enumeration function 计数函数epimorphism 满态射equipotent 等势equivalent category 等价范畴equivalent class 等价类equivalent matrix 等价矩阵equivalent object 等价对象equivalent relation 等价关系error function 错误函数error pattern 错误模式Euclid algorithm 欧几里德算法Euclid domain 欧氏整环Euler characteristic Euler 特征Euler function Euler 函数Euler graph Euler 图Euler number Euler 数Euler polyhedron formula Euler 多面体公式Euler tour Euler 闭迹Euler trail Euler 迹existential generalization 存在推广规则existential quantifier 存在量词existential specification 存在特指规则extended Fibonacci number 广义 Fibonacci 数extended Lucas number 广义Lucas 数extension 扩充(扩张)extension field 扩域extension graph 扩图exterior algebra 外代数Fface 面factor 因子factorable 可因子化的factorization 因子分解faithful (full) functor 忠实(完满)函子Ferrers graph Ferrers 图Fibonacci number Fibonacci 数field 域filter 滤子finite extension 有限扩域finite field (Galois field ) 有限域(Galois 域)finite dimensional associative division algebra有限维结合可除代数finite set 有限(穷)集finitely generated module 有限生成模first order theory with equality 带符号的一阶系统five-color theorem 五色定理five-time-repetition 五倍重复码fixed point 不动点forest 森林forgetful functor 忘却函子four-color theorem(conjecture) 四色定理(猜想)F-reduced product F-归纳积free element 自由元free monoid 自由单元半群free occurrence 自由出现free R-module 自由R-模free variable 自由变元free-Ω-algebra 自由Ω代数function scheme 映射格式GGalileo paradox Galileo 悖论Gauss coefficient Gauss 系数GBN (Gödel-Bernays-von Neumann system)GBN系统generalized petersen graph 广义 petersen 图generating function 生成函数generating procedure 生成过程generator 生成子(生成元)generator matrix 生成矩阵genus 亏格girth (腰)围长Gödel completeness theorem Gödel 完全性定理golden section number 黄金分割数(黄金分割率)graceful graph 优美图graceful tree conjecture 优美树猜想graph 图graph of first class for edge coloring 第一类边色图graph of second class for edge coloring 第二类边色图graph rank 图秩graph sequence 图序列greatest common factor 最大公因子greatest element 最大元(素)Grelling paradox Grelling 悖论Grötzsch graph Grötzsch 图group 群group code 群码group of graph 图的群HHajós conjecture Hajós 猜想Hamilton cycle Hamilton 圈Hamilton graph Hamilton 图Hamilton path Hamilton 路Harary graph Harary 图Hasse graph Hasse 图Heawood graph Heawood 图Herschel graph Herschel 图hom functor hom 函子homemorphism 图的同胚homomorphism 同态(同态映射)homomorphism of graph 图的同态hyperoctahedron 超八面体图hypothelical syllogism 假言三段论hypothese (premise) 假设(前提)Iideal 理想identity 单位元identity natural transformation 恒等自然变换imbedding 嵌入immediate predcessor 直接先行immediate successor 直接后继incident 关联incident axiom 关联公理incident matrix 关联矩阵inclusion and exclusion principle 包含与排斥原理inclusion relation 包含关系indegree 入次(入度)independent 独立的independent number 独立数independent set 独立集independent transcendental element 独立超越元素index 指数individual variable 个体变元induced subgraph 导出子图infinite extension 无限扩域infinite group 无限群infinite set 无限(穷)集initial endpoint 始端initial object 初始对象injection 单射injection functor 单射函子injective (one to one mapping) 单射(内射)inner face 内面inner neighbour set 内(入)邻集integral domain 整环integral subdomain 子整环internal direct sum 内直和intersection 交集intersection of graph 图的交intersection operation 交运算interval 区间invariant factor 不变因子invariant factor ideal 不变因子理想inverse limit 逆向极限inverse morphism 逆态射inverse natural transformation 逆自然变换inverse operation 逆运算inverse relation 逆关系inversion 反演isomorphic category 同构范畴isomorphism 同构态射isomorphism of graph 图的同构join of graph 图的联JJordan algebra Jordan 代数Jordan product (anti-commutator) Jordan乘积(反交换子)Jordan sieve formula Jordan 筛法公式j-skew j-斜元juxtaposition 邻接乘法Kk-chromatic graph k-色图k-connected graph k-连通图k-critical graph k-色临界图k-edge chromatic graph k-边色图k-edge-connected graph k-边连通图k-edge-critical graph k-边临界图kernel 核Kirkman schoolgirl problem Kirkman 女生问题Kuratowski theorem Kuratowski 定理Llabeled graph 有标号图Lah number Lah 数Latin rectangle Latin 矩形Latin square Latin 方lattice 格lattice homomorphism 格同态law 规律leader cuset 陪集头least element 最小元least upper bound 上确界(最小上界)left (right) identity 左(右)单位元left (right) invertible element 左(右)可逆元left (right) module 左(右)模left (right) zero 左(右)零元left (right) zero divisor 左(右)零因子left adjoint functor 左伴随函子left cancellable 左可消的left coset 左陪集length 长度Lie algebra Lie 代数line- group 图的线群logically equivanlent 逻辑等价logically implies 逻辑蕴涵logically valid 逻辑有效的(普效的)loop 环Lucas number Lucas 数Mmagic 幻方many valued proposition logic 多值命题逻辑matching 匹配mathematical structure 数学结构matrix representation 矩阵表示maximal element 极大元maximal ideal 极大理想maximal outerplanar graph 极大外平面图maximal planar graph 极大平面图maximum matching 最大匹配maxterm 极大项(基本析取式)maxterm normal form(conjunctive normal form) 极大项范式(合取范式)McGee graph McGee 图meet 交Menger theorem Menger 定理Meredith graph Meredith 图message word 信息字mini term 极小项minimal κ-connected graph 极小κ-连通图minimal polynomial 极小多项式Minimanoff paradox Minimanoff 悖论minimum distance 最小距离Minkowski sum Minkowski 和minterm (fundamental conjunctive form) 极小项(基本合取式)minterm normal form(disjunctive normal form)极小项范式(析取范式)Möbius function Möbius 函数Möbius ladder Möbius 梯Möbius transform (inversion) Möbius 变换(反演)modal logic 模态逻辑model 模型module homomorphism 模同态(R-同态)modus ponens 分离规则modus tollens 否定后件式module isomorphism 模同构monic morphism 单同态monoid 单元半群monomorphism 单态射morphism (arrow) 态射(箭)Möbius function Möbius 函数Möbius ladder Möbius 梯Möbius transform (inversion) Möbius 变换(反演)multigraph 多重图multinomial coefficient 多项式系数multinomial expansion theorem 多项式展开定理multiple-error-correcting code 纠多错码multiplication principle 乘法原理mutually orthogonal Latin square 相互正交拉丁方Nn-ary operation n-元运算n-ary product n-元积natural deduction system 自然推理系统natural isomorphism 自然同构natural transformation 自然变换neighbour set 邻集next state 下一个状态next state transition function 状态转移函数non-associative algebra 非结合代数non-standard logic 非标准逻辑Norlund formula Norlund 公式normal form 正规形normal model 标准模型normal subgroup (invariant subgroup) 正规子群(不变子群)n-relation n-元关系null object 零对象nullary operation 零元运算Oobject 对象orbit 轨道order 阶order ideal 阶理想Ore condition Ore 条件orientation 定向orthogonal Latin square 正交拉丁方orthogonal layout 正交表outarc 出弧outdegree 出次(出度)outer face 外面outer neighbour 外(出)邻集outerneighbour set 出(外)邻集outerplanar graph 外平面图Ppancycle graph 泛圈图parallelism 平行parallelism class 平行类parity-check code 奇偶校验码parity-check equation 奇偶校验方程parity-check machine 奇偶校验器parity-check matrix 奇偶校验矩阵partial function 偏函数partial ordering (partial relation) 偏序关系partial order relation 偏序关系partial order set (poset) 偏序集partition 划分,分划,分拆partition number of integer 整数的分拆数partition number of set 集合的划分数Pascal formula Pascal 公式path 路perfect code 完全码perfect t-error-correcting code 完全纠-错码perfect graph 完美图permutation 排列(置换)permutation group 置换群permutation with repetation 可重排列Petersen graph Petersen 图p-graph p-图Pierce arrow Pierce 箭pigeonhole principle 鸽子笼原理planar graph (可)平面图plane graph 平面图Pólya theorem Pólya 定理polynomail 多项式polynomial code 多项式码polynomial representation 多项式表示法polynomial ring 多项式环possible world 可能世界power functor 幂函子power of graph 图的幂power set 幂集predicate 谓词prenex normal form 前束范式pre-ordered set 拟序集primary cycle module 准素循环模prime field 素域prime to each other 互素primitive connective 初始联结词primitive element 本原元primitive polynomial 本原多项式principal ideal 主理想principal ideal domain 主理想整环principal of duality 对偶原理principal of redundancy 冗余性原则product 积product category 积范畴product-sum form 积和式proof (deduction) 证明(演绎)proper coloring 正常着色proper factor 真正因子proper filter 真滤子proper subgroup 真子群properly inclusive relation 真包含关系proposition 命题propositional constant 命题常量propositional formula(well-formed formula,wff)命题形式(合式公式)propositional function 命题函数propositional variable 命题变量pullback 拉回(回拖) pushout 推出Qquantification theory 量词理论quantifier 量词quasi order relation 拟序关系quaternion 四元数quotient (difference) algebra 商(差)代数quotient algebra 商代数quotient field (field of fraction) 商域(分式域)quotient group 商群quotient module 商模quotient ring (difference ring , residue ring) 商环(差环,同余类环)quotient set 商集RRamsey graph Ramsey 图Ramsey number Ramsey 数Ramsey theorem Ramsey 定理range 值域rank 秩reconstruction conjecture 重构猜想redundant digits 冗余位reflexive 自反的regular graph 正则图regular representation 正则表示relation matrix 关系矩阵replacement theorem 替换定理representation 表示representation functor 可表示函子restricted proposition form 受限命题形式restriction 限制retraction 收缩Richard paradox Richard 悖论right adjoint functor 右伴随函子right cancellable 右可消的right factor 右因子right zero divison 右零因子ring 环ring of endomorphism 自同态环ring with unity element 有单元的环R-linear independence R-线性无关root field 根域rule of inference 推理规则Russell paradox Russell 悖论Ssatisfiable 可满足的saturated 饱和的scope 辖域section 截口self-complement graph 自补图semantical completeness 语义完全的(弱完全的)semantical consistent 语义相容semigroup 半群separable element 可分元separable extension 可分扩域sequent 矢列式sequential 序列的Sheffer stroke Sheffer 竖(谢弗竖)simple algebraic extension 单代数扩域simple extension 单扩域simple graph 简单图simple proposition (atomic proposition) 简单(原子)命题simple transcental extension 单超越扩域simplication 简化规则slope 斜率small category 小范畴smallest element 最小元(素)Socrates argument Socrates 论断(苏格拉底论断)soundness (validity) theorem 可靠性(有效性)定理spanning subgraph 生成子图spanning tree 生成树spectra of graph 图的谱spetral radius 谱半径splitting field 分裂域standard model 标准模型standard monomil 标准单项式Steiner triple Steiner 三元系大集Stirling number Stirling 数Stirling transform Stirling 变换subalgebra 子代数subcategory 子范畴subdirect product 子直积subdivison of graph 图的细分subfield 子域subformula 子公式subdivision of graph 图的细分subgraph 子图subgroup 子群sub-module 子模subrelation 子关系subring 子环sub-semigroup 子半群subset 子集substitution theorem 代入定理substraction 差集substraction operation 差运算succedent 后件surjection (surjective) 满射switching-network 开关网络Sylvester formula Sylvester公式symmetric 对称的symmetric difference 对称差symmetric graph 对称图symmetric group 对称群syndrome 校验子syntactical completeness 语法完全的(强完全的)Syntactical consistent 语法相容system Ł3 , Łn , Łא0 , Łא系统Ł3 , Łn , Łא0 , Łאsystem L 公理系统 Lsystem Ł公理系统Łsystem L1 公理系统 L1system L2 公理系统 L2system L3 公理系统 L3system L4 公理系统 L4system L5 公理系统 L5system L6 公理系统 L6system Łn 公理系统Łnsystem of modal prepositional logic 模态命题逻辑系统system Pm 系统 Pmsystem S1 公理系统 S1system T (system M) 公理系统 T(系统M)Ttautology 重言式(永真公式)technique of truth table 真值表技术term 项terminal endpoint 终端terminal object 终结对象t-error-correcing BCH code 纠 t -错BCH码theorem (provable formal) 定理(可证公式)thickess 厚度timed sequence 时间序列torsion 扭元torsion module 扭模total chromatic number 全色数total chromatic number conjecture 全色数猜想total coloring 全着色total graph 全图total matrix ring 全方阵环total order set 全序集total permutation 全排列total relation 全关系tournament 竞赛图trace (trail) 迹tranformation group 变换群transcendental element 超越元素transitive 传递的tranverse design 横截设计traveling saleman problem 旅行商问题tree 树triple system 三元系triple-repetition code 三倍重复码trivial graph 平凡图trivial subgroup 平凡子群true in an interpretation 解释真truth table 真值表truth value function 真值函数Turán graph Turán 图Turán theorem Turán 定理Tutte graph Tutte 图Tutte theorem Tutte 定理Tutte-coxeter graph Tutte-coxeter 图UUlam conjecture Ulam 猜想ultrafilter 超滤子ultrapower 超幂ultraproduct 超积unary operation 一元运算unary relation 一元关系underlying graph 基础图undesignated truth value 非特指值undirected graph 无向图union 并(并集)union of graph 图的并union operation 并运算unique factorization 唯一分解unique factorization domain (Gauss domain) 唯一分解整域unique k-colorable graph 唯一k着色unit ideal 单位理想unity element 单元universal 全集universal algebra 泛代数(Ω代数)universal closure 全称闭包universal construction 通用结构universal enveloping algebra 通用包络代数universal generalization 全称推广规则universal quantifier 全称量词universal specification 全称特指规则universal upper bound 泛上界unlabeled graph 无标号图untorsion 无扭模upper (lower) bound 上(下)界useful equivalent 常用等值式useless code 废码字Vvalence 价valuation 赋值Vandermonde formula Vandermonde 公式variery 簇Venn graph Venn 图vertex cover 点覆盖vertex set 点割集vertex transitive graph 点传递图Vizing theorem Vizing 定理Wwalk 通道weakly antisymmetric 弱反对称的weight 重(权)weighted form for Burnside lemma 带权形式的Burnside引理well-formed formula (wff) 合式公式(wff)word 字Zzero divison 零因子zero element (universal lower bound) 零元(泛下界)ZFC (Zermelo-Fraenkel-Cohen) system ZFC系统form)normal(Skolemformnormalprenex-存在正则前束范式(Skolem 正则范式)3-value proposition logic 三值命题逻辑。
Discriminatively Trained Sparse Code Gradients for Contour Detection
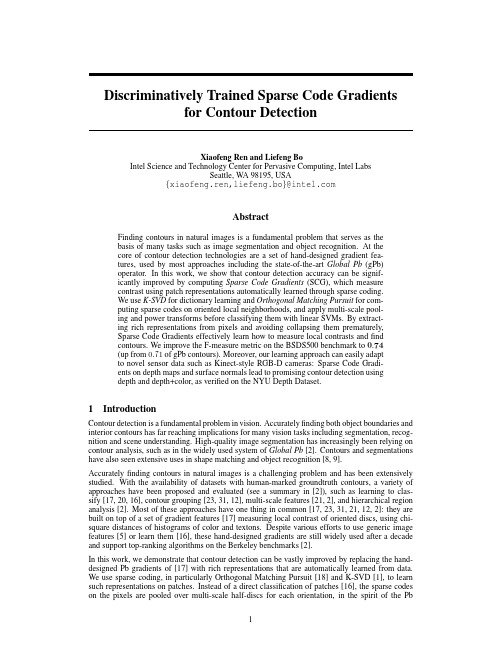
Discriminatively Trained Sparse Code Gradientsfor Contour DetectionXiaofeng Ren and Liefeng BoIntel Science and Technology Center for Pervasive Computing,Intel LabsSeattle,W A98195,USA{xiaofeng.ren,liefeng.bo}@AbstractFinding contours in natural images is a fundamental problem that serves as thebasis of many tasks such as image segmentation and object recognition.At thecore of contour detection technologies are a set of hand-designed gradient fea-tures,used by most approaches including the state-of-the-art Global Pb(gPb)operator.In this work,we show that contour detection accuracy can be signif-icantly improved by computing Sparse Code Gradients(SCG),which measurecontrast using patch representations automatically learned through sparse coding.We use K-SVD for dictionary learning and Orthogonal Matching Pursuit for com-puting sparse codes on oriented local neighborhoods,and apply multi-scale pool-ing and power transforms before classifying them with linear SVMs.By extract-ing rich representations from pixels and avoiding collapsing them prematurely,Sparse Code Gradients effectively learn how to measure local contrasts andfindcontours.We improve the F-measure metric on the BSDS500benchmark to0.74(up from0.71of gPb contours).Moreover,our learning approach can easily adaptto novel sensor data such as Kinect-style RGB-D cameras:Sparse Code Gradi-ents on depth maps and surface normals lead to promising contour detection usingdepth and depth+color,as verified on the NYU Depth Dataset.1IntroductionContour detection is a fundamental problem in vision.Accuratelyfinding both object boundaries and interior contours has far reaching implications for many vision tasks including segmentation,recog-nition and scene understanding.High-quality image segmentation has increasingly been relying on contour analysis,such as in the widely used system of Global Pb[2].Contours and segmentations have also seen extensive uses in shape matching and object recognition[8,9].Accuratelyfinding contours in natural images is a challenging problem and has been extensively studied.With the availability of datasets with human-marked groundtruth contours,a variety of approaches have been proposed and evaluated(see a summary in[2]),such as learning to clas-sify[17,20,16],contour grouping[23,31,12],multi-scale features[21,2],and hierarchical region analysis[2].Most of these approaches have one thing in common[17,23,31,21,12,2]:they are built on top of a set of gradient features[17]measuring local contrast of oriented discs,using chi-square distances of histograms of color and textons.Despite various efforts to use generic image features[5]or learn them[16],these hand-designed gradients are still widely used after a decade and support top-ranking algorithms on the Berkeley benchmarks[2].In this work,we demonstrate that contour detection can be vastly improved by replacing the hand-designed Pb gradients of[17]with rich representations that are automatically learned from data. We use sparse coding,in particularly Orthogonal Matching Pursuit[18]and K-SVD[1],to learn such representations on patches.Instead of a direct classification of patches[16],the sparse codes on the pixels are pooled over multi-scale half-discs for each orientation,in the spirit of the Pbimage patch: gray, abdepth patch (optional):depth, surface normal…local sparse coding multi-scale pooling oriented gradients power transformslinear SVM+ - …per-pixelsparse codes SVMSVMSVM … SVM RGB-(D) contoursFigure 1:We combine sparse coding and oriented gradients for contour analysis on color as well as depth images.Sparse coding automatically learns a rich representation of patches from data.With multi-scale pooling,oriented gradients efficiently capture local contrast and lead to much more accurate contour detection than those using hand-designed features including Global Pb (gPb)[2].gradients,before being classified with a linear SVM.The SVM outputs are then smoothed and non-max suppressed over orientations,as commonly done,to produce the final contours (see Fig.1).Our sparse code gradients (SCG)are much more effective in capturing local contour contrast than existing features.By only changing local features and keeping the smoothing and globalization parts fixed,we improve the F-measure on the BSDS500benchmark to 0.74(up from 0.71of gPb),a sub-stantial step toward human-level accuracy (see the precision-recall curves in Fig.4).Large improve-ments in accuracy are also observed on other datasets including MSRC2and PASCAL2008.More-over,our approach is built on unsupervised feature learning and can directly apply to novel sensor data such as RGB-D images from Kinect-style depth ing the NYU Depth dataset [27],we verify that our SCG approach combines the strengths of color and depth contour detection and outperforms an adaptation of gPb to RGB-D by a large margin.2Related WorkContour detection has a long history in computer vision as a fundamental building block.Modern approaches to contour detection are evaluated on datasets of natural images against human-marked groundtruth.The Pb work of Martin et.al.[17]combined a set of gradient features,using bright-ness,color and textons,to outperform the Canny edge detector on the Berkeley Benchmark (BSDS).Multi-scale versions of Pb were developed and found beneficial [21,2].Building on top of the Pb gradients,many approaches studied the globalization aspects,i.e.moving beyond local classifica-tion and enforcing consistency and continuity of contours.Ren et.al.developed CRF models on superpixels to learn junction types [23].Zhu ed circular embedding to enforce orderings of edgels [31].The gPb work of Arbelaez puted gradients on eigenvectors of the affinity graph and combined them with local cues [2].In addition to Pb gradients,Dollar et.al.[5]learned boosted trees on generic features such as gradients and Haar wavelets,Kokkinos used SIFT features on edgels [12],and Prasad et.al.[20]used raw pixels in class-specific settings.One closely related work was the discriminative sparse models of Mairal et al [16],which used K-SVD to represent multi-scale patches and had moderate success on the BSDS.A major difference of our work is the use of oriented gradients:comparing to directly classifying a patch,measuring contrast between oriented half-discs is a much easier problem and can be effectively learned.Sparse coding represents a signal by reconstructing it using a small set of basis functions.It has seen wide uses in vision,for example for faces [28]and recognition [29].Similar to deep network approaches [11,14],recent works tried to avoid feature engineering and employed sparse coding of image patches to learn features from “scratch”,for texture analysis [15]and object recognition [30,3].In particular,Orthogonal Matching Pursuit [18]is a greedy algorithm that incrementally finds sparse codes,and K-SVD is also efficient and popular for dictionary learning.Closely related to our work but on the different problem of recognition,Bo ed matching pursuit and K-SVD to learn features in a coding hierarchy [3]and are extending their approach to RGB-D data [4].Thanks to the mass production of Kinect,active RGB-D cameras became affordable and were quickly adopted in vision research and applications.The Kinect pose estimation of Shotton et. ed random forests to learn from a huge amount of data[25].Henry ed RGB-D cam-eras to scan large environments into3D models[10].RGB-D data were also studied in the context of object recognition[13]and scene labeling[27,22].In-depth studies of contour and segmentation problems for depth data are much in need given the fast growing interests in RGB-D perception.3Contour Detection using Sparse Code GradientsWe start by examining the processing pipeline of Global Pb(gPb)[2],a highly influential and widely used system for contour detection.The gPb contour detection has two stages:local contrast estimation at multiple scales,and globalization of the local cues using spectral grouping.The core of the approach lies within its use of local cues in oriented gradients.Originally developed in [17],this set of features use relatively simple pixel representations(histograms of brightness,color and textons)and similarity functions(chi-square distance,manually chosen),comparing to recent advances in using rich representations for high-level recognition(e.g.[11,29,30,3]).We set out to show that both the pixel representation and the aggregation of pixel information in local neighborhoods can be much improved and,to a large extent,learned from and adapted to input data. For pixel representation,in Section3.1we show how to use Orthogonal Matching Pursuit[18]and K-SVD[1],efficient sparse coding and dictionary learning algorithms that readily apply to low-level vision,to extract sparse codes at every pixel.This sparse coding approach can be viewed similar in spirit to the use offilterbanks but avoids manual choices and thus directly applies to the RGB-D data from Kinect.We show learned dictionaries for a number of channels that exhibit different characteristics:grayscale/luminance,chromaticity(ab),depth,and surface normal.In Section3.2we show how the pixel-level sparse codes can be integrated through multi-scale pool-ing into a rich representation of oriented local neighborhoods.By computing oriented gradients on this high dimensional representation and using a double power transform to code the features for linear classification,we show a linear SVM can be efficiently and effectively trained for each orientation to classify contour vs non-contour,yielding local contrast estimates that are much more accurate than the hand-designed features in gPb.3.1Local Sparse Representation of RGB-(D)PatchesK-SVD and Orthogonal Matching Pursuit.K-SVD[1]is a popular dictionary learning algorithm that generalizes K-Means and learns dictionaries of codewords from unsupervised data.Given a set of image patches Y=[y1,···,y n],K-SVD jointlyfinds a dictionary D=[d1,···,d m]and an associated sparse code matrix X=[x1,···,x n]by minimizing the reconstruction errorminY−DX 2F s.t.∀i, x i 0≤K;∀j, d j 2=1(1) D,Xwhere · F denotes the Frobenius norm,x i are the columns of X,the zero-norm · 0counts the non-zero entries in the sparse code x i,and K is a predefined sparsity level(number of non-zero en-tries).This optimization can be solved in an alternating manner.Given the dictionary D,optimizing the sparse code matrix X can be decoupled to sub-problems,each solved with Orthogonal Matching Pursuit(OMP)[18],a greedy algorithm forfinding sparse codes.Given the codes X,the dictionary D and its associated sparse coefficients are updated sequentially by singular value decomposition. For our purpose of representing local patches,the dictionary D has a small size(we use75for5x5 patches)and does not require a lot of sample patches,and it can be learned in a matter of minutes. Once the dictionary D is learned,we again use the Orthogonal Matching Pursuit(OMP)algorithm to compute sparse codes at every pixel.This can be efficiently done with convolution and a batch version of the OMP algorithm[24].For a typical BSDS image of resolution321x481,the sparse code extraction is efficient and takes1∼2seconds.Sparse Representation of RGB-D Data.One advantage of unsupervised dictionary learning is that it readily applies to novel sensor data,such as the color and depth frames from a Kinect-style RGB-D camera.We learn K-SVD dictionaries up to four channels of color and depth:grayscale for luminance,chromaticity ab for color in the Lab space,depth(distance to camera)and surface normal(3-dim).The learned dictionaries are visualized in Fig.2.These dictionaries are interesting(a)Grayscale (b)Chromaticity (ab)(c)Depth (d)Surface normal Figure 2:K-SVD dictionaries learned for four different channels:grayscale and chromaticity (in ab )for an RGB image (a,b),and depth and surface normal for a depth image (c,d).We use a fixed dictionary size of 75on 5x 5patches.The ab channel is visualized using a constant luminance of 50.The 3-dimensional surface normal (xyz)is visualized in RGB (i.e.blue for frontal-parallel surfaces).to look at and qualitatively distinctive:for example,the surface normal codewords tend to be more smooth due to flat surfaces,the depth codewords are also more smooth but with speckles,and the chromaticity codewords respect the opponent color pairs.The channels are coded separately.3.2Coding Multi-Scale Neighborhoods for Measuring ContrastMulti-Scale Pooling over Oriented Half-Discs.Over decades of research on contour detection and related topics,a number of fundamental observations have been made,repeatedly:(1)contrast is the key to differentiate contour vs non-contour;(2)orientation is important for respecting contour continuity;and (3)multi-scale is useful.We do not wish to throw out these principles.Instead,we seek to adopt these principles for our case of high dimensional representations with sparse codes.Each pixel is presented with sparse codes extracted from a small patch (5-by-5)around it.To aggre-gate pixel information,we use oriented half-discs as used in gPb (see an illustration in Fig.1).Each orientation is processed separately.For each orientation,at each pixel p and scale s ,we define two half-discs (rectangles)N a and N b of size s -by-(2s +1),on both sides of p ,rotated to that orienta-tion.For each half-disc N ,we use average pooling on non-zero entries (i.e.a hybrid of average and max pooling)to generate its representationF (N )= i ∈N |x i 1| i ∈N I |x i 1|>0,···, i ∈N |x im | i ∈NI |x im |>0 (2)where x ij is the j -th entry of the sparse code x i ,and I is the indicator function whether x ij is non-zero.We rotate the image (after sparse coding)and use integral images for fast computations (on both |x ij |and |x ij |>0,whose costs are independent of the size of N .For two oriented half-dics N a and N b at a scale s ,we compute a difference (gradient)vector DD (N a s ,N b s )= F (N a s )−F (N b s ) (3)where |·|is an element-wise absolute value operation.We divide D (N a s ,N b s )by their norms F (N a s ) + F (N b s ) + ,where is a positive number.Since the magnitude of sparse codes variesover a wide range due to local variations in illumination as well as occlusion,this step makes the appearance features robust to such variations and increases their discriminative power,as commonly done in both contour detection and object recognition.This value is not hard to set,and we find a value of =0.5is better than,for instance, =0.At this stage,one could train a classifier on D for each scale to convert it to a scalar value of contrast,which would resemble the chi-square distance function in gPb.Instead,we find that it is much better to avoid doing so separately at each scale,but combining multi-scale features in a joint representation,so as to allow interactions both between codewords and between scales.That is,our final representation of the contrast at a pixel p is the concatenation of sparse codes pooled at all thescales s ∈{1,···,S }(we use S =4):D p = D (N a 1,N b 1),···,D (N a S ,N b S );F (N a 1∪N b 1),···,F (N a S ∪N b S ) (4)In addition to difference D ,we also include a union term F (N a s ∪N b s ),which captures the appear-ance of the whole disc (union of the two half discs)and is normalized by F (N a s ) + F (N b s ) + .Double Power Transform and Linear Classifiers.The concatenated feature D p (non-negative)provides multi-scale contrast information for classifying whether p is a contour location for a partic-ular orientation.As D p is high dimensional (1200and above in our experiments)and we need to do it at every pixel and every orientation,we prefer using linear SVMs for both efficient testing as well as training.Directly learning a linear function on D p ,however,does not work very well.Instead,we apply a double power transformation to make the features more suitable for linear SVMs D p = D α1p ,D α2p (5)where 0<α1<α2<1.Empirically,we find that the double power transform works much better than either no transform or a single power transform α,as sometimes done in other classification contexts.Perronnin et.al.[19]provided an intuition why a power transform helps classification,which “re-normalizes”the distribution of the features into a more Gaussian form.One plausible intuition for a double power transform is that the optimal exponent αmay be different across feature dimensions.By putting two power transforms of D p together,we allow the classifier to pick its linear combination,different for each dimension,during the stage of supervised training.From Local Contrast to Global Contours.We intentionally only change the local contrast es-timation in gPb and keep the other steps fixed.These steps include:(1)the Savitzky-Goley filter to smooth responses and find peak locations;(2)non-max suppression over orientations;and (3)optionally,we apply the globalization step in gPb that computes a spectral gradient from the local gradients and then linearly combines the spectral gradient with the local ones.A sigmoid transform step is needed to convert the SVM outputs on D p before computing spectral gradients.4ExperimentsWe use the evaluation framework of,and extensively compare to,the publicly available Global Pb (gPb)system [2],widely used as the state of the art for contour detection 1.All the results reported on gPb are from running the gPb contour detection and evaluation codes (with default parameters),and accuracies are verified against the published results in [2].The gPb evaluation includes a number of criteria,including precision-recall (P/R)curves from contour matching (Fig.4),F-measures computed from P/R (Table 1,2,3)with a fixed contour threshold (ODS)or per-image thresholds (OIS),as well as average precisions (AP)from the P/R curves.Benchmark Datasets.The main dataset we use is the BSDS500benchmark [2],an extension of the original BSDS300benchmark and commonly used for contour evaluation.It includes 500natural images of roughly resolution 321x 481,including 200for training,100for validation,and 200for testing.We conduct both color and grayscale experiments (where we convert the BSDS500images to grayscale and retain the groundtruth).In addition,we also use the MSRC2and PASCAL2008segmentation datasets [26,6],as done in the gPb work [2].The MSRC2dataset has 591images of resolution 200x 300;we randomly choose half for training and half for testing.The PASCAL2008dataset includes 1023images in its training and validation sets,roughly of resolution 350x 500.We randomly choose half for training and half for testing.For RGB-D contour detection,we use the NYU Depth dataset (v2)[27],which includes 1449pairs of color and depth frames of resolution 480x 640,with groundtruth semantic regions.We choose 60%images for training and 40%for testing,as in its scene labeling setup.The Kinect images are of lower quality than BSDS,and we resize the frames to 240x 320in our experiments.Training Sparse Code Gradients.Given sparse codes from K-SVD and Orthogonal Matching Pur-suit,we train the Sparse Code Gradients classifiers,one linear SVM per orientation,from sampled locations.For positive data,we sample groundtruth contour locations and estimate the orientations at these locations using groundtruth.For negative data,locations and orientations are random.We subtract the mean from the patches in each data channel.For BSDS500,we typically have 1.5to 21In this work we focus on contour detection and do not address how to derive segmentations from contours.pooling disc size (pixel)a v e r a g e p r e c i s i o na v e r a g e p r e c i s i o nsparsity level a v e r a g e p r e c i s i o n (a)(b)(c)Figure 3:Analysis of our sparse code gradients,using average precision of classification on sampled boundaries.(a)The effect of single-scale vs multi-scale pooling (accumulated from the smallest).(b)Accuracy increasing with dictionary size,for four orientation channels.(c)The effect of the sparsity level K,which exhibits different behavior for grayscale and chromaticity.BSDS500ODS OIS AP l o c a l gPb (gray).67.69.68SCG (gray).69.71.71gPb (color).70.72.71SCG (color).72.74.75g l o b a l gPb (gray).69.71.67SCG (gray).71.73.74gPb (color).71.74.72SCG (color).74.76.77Table 1:F-measure evaluation on the BSDS500benchmark [2],comparing to gPb on grayscaleand color images,both for local contour detec-tion as well as for global detection (-bined with the spectral gradient analysis in [2]).Recall P r e c i s i o n Figure 4:Precision-recall curves of SCG vs gPb on BSDS500,for grayscale and color images.We make a substantial step beyondthe current state of the art toward reachinghuman-level accuracy (green dot).million data points.We use 4spatial scales,at half-disc sizes 2,4,7,25.For a dictionary size of 75and 4scales,the feature length for one data channel is 1200.For full RGB-D data,the dimension is 4800.For BSDS500,we train only using the 200training images.We modify liblinear [7]to take dense matrices (features are dense after pooling)and single-precision floats.Looking under the Hood.We empirically analyze a number of settings in our Sparse Code Gradi-ents.In particular,we want to understand how the choices in the local sparse coding affect contour classification.Fig.3shows the effects of multi-scale pooling,dictionary size,and sparsity level (K).The numbers reported are intermediate results,namely the mean of average precision of four oriented gradient classifier (0,45,90,135degrees)on sampled locations (grayscale unless otherwise noted,on validation).As a reference,the average precision of gPb on this task is 0.878.For multi-scale pooling,the single best scale for the half-disc filter is about 4x 8,consistent with the settings in gPb.For accumulated scales (using all the scales from the smallest up to the current level),the accuracy continues to increase and does not seem to be saturated,suggesting the use of larger scales.The dictionary size has a minor impact,and there is a small (yet observable)benefit to use dictionaries larger than 75,particularly for diagonal orientations (45-and 135-deg).The sparsity level K is a more intriguing issue.In Fig.3(c),we see that for grayscale only,K =1(normalized nearest neighbor)does quite well;on the other hand,color needs a larger K ,possibly because ab is a nonlinear space.When combining grayscale and color,it seems that we want K to be at least 3.It also varies with orientation:horizontal and vertical edges require a smaller K than diagonal edges.(If using K =1,our final F-measure on BSDS500is 0.730.)We also empirically evaluate the double power transform vs single power transform vs no transform.With no transform,the average precision is 0.865.With a single power transform,the best choice of the exponent is around 0.4,with average precision 0.884.A double power transform (with exponentsMSRC2ODS OIS APgPb.37.39.22SCG.43.43.33PASCAL2008ODS OIS APgPb.34.38.20SCG.37.41.27Table2:F-measure evaluation comparing our SCG approach to gPb on two addi-tional image datasets with contour groundtruth: MSRC2[26]and PASCAL2008[6].RGB-D(NYU v2)ODS OIS AP gPb(color).51.52.37 SCG(color).55.57.46gPb(depth).44.46.28SCG(depth).53.54.45gPb(RGB-D).53.54.40SCG(RGB-D).62.63.54Table3:F-measure evaluation on RGB-D con-tour detection using the NYU dataset(v2)[27].We compare to gPb on using color image only,depth only,as well as color+depth.Figure5:Examples from the BSDS500dataset[2].(Top)Image;(Middle)gPb output;(Bottom) SCG output(this work).Our SCG operator learns to preservefine details(e.g.windmills,faces,fish fins)while at the same time achieving higher precision on large-scale contours(e.g.back of zebras). (Contours are shown in double width for the sake of visualization.)0.25and0.75,which can be computed through sqrt)improves the average precision to0.900,which translates to a large improvement in contour detection accuracy.Image Benchmarking Results.In Table1and Fig.4we show the precision-recall of our Sparse Code Gradients vs gPb on the BSDS500benchmark.We conduct four sets of experiments,using color or grayscale images,with or without the globalization component(for which we use exactly the same setup as in gPb).Using Sparse Code Gradients leads to a significant improvement in accuracy in all four cases.The local version of our SCG operator,i.e.only using local contrast,is already better(F=0.72)than gPb with globalization(F=0.71).The full version,local SCG plus spectral gradient(computed from local SCG),reaches an F-measure of0.739,a large step forward from gPb,as seen in the precision-recall curves in Fig.4.On BSDS300,our F-measure is0.715. We observe that SCG seems to pick upfine-scale details much better than gPb,hence the much higher recall rate,while maintaining higher precision over the entire range.This can be seen in the examples shown in Fig.5.While our scale range is similar to that of gPb,the multi-scale pooling scheme allows theflexibility of learning the balance of scales separately for each code word,which may help detecting the details.The supplemental material contains more comparison examples.In Table2we show the benchmarking results for two additional datasets,MSRC2and PAS-CAL2008.Again we observe large improvements in accuracy,in spite of the somewhat different natures of the scenes in these datasets.The improvement on MSRC2is much larger,partly because the images are smaller,hence the contours are smaller in scale and may be over-smoothed in gPb. As for computational cost,using integral images,local SCG takes∼100seconds to compute on a single-thread Intel Core i5-2500CPU on a BSDS image.It is slower than but comparable to the highly optimized multi-thread C++implementation of gPb(∼60seconds).Figure6:Examples of RGB-D contour detection on the NYU dataset(v2)[27].Thefive panels are:input image,input depth,image-only contours,depth-only contours,and color+depth contours. Color is good picking up details such as photos on the wall,and depth is useful where color is uniform(e.g.corner of a room,row1)or illumination is poor(e.g.chair,row2).RGB-D Contour Detection.We use the second version of the NYU Depth Dataset[27],which has higher quality groundtruth than thefirst version.A medianfiltering is applied to remove double contours(boundaries from two adjacent regions)within3pixels.For RGB-D baseline,we use a simple adaptation of gPb:the depth values are in meters and used directly as a grayscale image in gPb gradient computation.We use a linear combination to put(soft)color and depth gradients together in gPb before non-max suppression,with the weight set from validation.Table3lists the precision-recall evaluations of SCG vs gPb for RGB-D contour detection.All the SCG settings(such as scales and dictionary sizes)are kept the same as for BSDS.SCG again outperforms gPb in all the cases.In particular,we are much better for depth-only contours,for which gPb is not designed.Our approach learns the low-level representations of depth data fully automatically and does not require any manual tweaking.We also achieve a much larger boost by combining color and depth,demonstrating that color and depth channels contain complementary information and are both critical for RGB-D contour detection.Qualitatively,it is easy to see that RGB-D combines the strengths of color and depth and is a promising direction for contour and segmentation tasks and indoor scene analysis in general[22].Fig.6shows a few examples of RGB-D contours from our SCG operator.There are plenty of such cases where color alone or depth alone would fail to extract contours for meaningful parts of the scenes,and color+depth would succeed. 5DiscussionsIn this work we successfully showed how to learn and code local representations to extract contours in natural images.Our approach combined the proven concept of oriented gradients with powerful representations that are automatically learned through sparse coding.Sparse Code Gradients(SCG) performed significantly better than hand-designed features that were in use for a decade,and pushed contour detection much closer to human-level accuracy as illustrated on the BSDS500benchmark. Comparing to hand-designed features(e.g.Global Pb[2]),we maintain the high dimensional rep-resentation from pooling oriented neighborhoods and do not collapse them prematurely(such as computing chi-square distance at each scale).This passes a richer set of information into learn-ing contour classification,where a double power transform effectively codes the features for linear paring to previous learning approaches(e.g.discriminative dictionaries in[16]),our uses of multi-scale pooling and oriented gradients lead to much higher classification accuracies. Our work opens up future possibilities for learning contour detection and segmentation.As we il-lustrated,there is a lot of information locally that is waiting to be extracted,and a learning approach such as sparse coding provides a principled way to do so,where rich representations can be automat-ically constructed and adapted.This is particularly important for novel sensor data such as RGB-D, for which we have less understanding but increasingly more need.。
不对称自由基反应英文
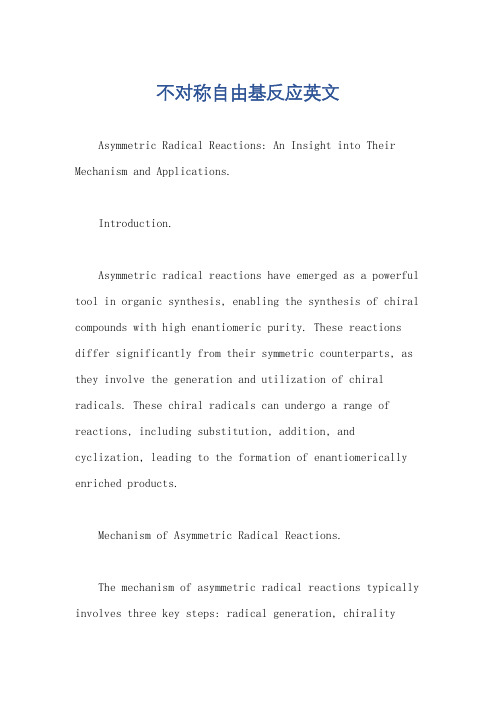
不对称自由基反应英文Asymmetric Radical Reactions: An Insight into Their Mechanism and Applications.Introduction.Asymmetric radical reactions have emerged as a powerful tool in organic synthesis, enabling the synthesis of chiral compounds with high enantiomeric purity. These reactions differ significantly from their symmetric counterparts, as they involve the generation and utilization of chiral radicals. These chiral radicals can undergo a range of reactions, including substitution, addition, and cyclization, leading to the formation of enantiomerically enriched products.Mechanism of Asymmetric Radical Reactions.The mechanism of asymmetric radical reactions typically involves three key steps: radical generation, chiralitytransfer, and radical termination.Radical Generation.The first step involves the generation of a radical species. This can be achieved through various methods, such as photolysis, thermal decomposition, or redox reactions. The generated radical can be chiral or achiral, depending on the starting materials and the conditions used.Chirality Transfer.The second step involves the transfer of chirality from a chiral auxiliary or catalyst to the radical species. This chirality transfer can occur through covalent or non-covalent interactions between the catalyst/auxiliary and the radical. The nature of these interactions determines the stereoselectivity of the reaction.Radical Termination.The final step involves the termination of the radicalspecies, leading to the formation of the desired product. This termination can occur through various mechanisms, such as coupling with another radical species, hydrogen atom abstraction, or disproportionation.Applications of Asymmetric Radical Reactions.Asymmetric radical reactions have found widespread applications in various fields of organic synthesis, including the synthesis of natural products, pharmaceuticals, and functional materials.Synthesis of Natural Products.Natural products often possess complex chiral structures, making their synthesis challenging. Asymmetric radical reactions have proven to be effective tools for the synthesis of such chiral natural products. For example, the use of chiral radicals generated from appropriate precursors has enabled the enantioselective synthesis of alkaloids, terpenes, and amino acids.Pharmaceutical Applications.The enantiomers of chiral drugs often differ significantly in their biological activities, making it crucial to control their enantiomeric purity. Asymmetric radical reactions can be used to synthesize enantiomerically enriched chiral drugs with high selectivity. This approach has been successfully applied to the synthesis of various drugs, including anti-inflammatory agents, anticancer agents, and antiviral agents.Functional Materials.Chiral materials possess unique physical and chemical properties that make them useful in various applications, such as displays, sensors, and catalysts. Asymmetricradical reactions can be used to synthesize chiral building blocks for the preparation of such materials. For instance, chiral polymers can be synthesized by utilizing asymmetric radical polymerization reactions, leading to the formation of materials with controlled chirality and tailored properties.Conclusion.Asymmetric radical reactions have emerged as powerful tools for the synthesis of enantiomerically enriched chiral compounds. Their unique mechanism, involving chirality transfer from a chiral catalyst/auxiliary to the radical species, enables high selectivity and enantiopurity in the product. The widespread applications of asymmetric radical reactions in organic synthesis, particularly in the synthesis of natural products, pharmaceuticals, and functional materials, highlight their importance in modern chemistry.Future Perspectives.Despite the significant progress made in the field of asymmetric radical reactions, there are still numerous challenges and opportunities for further exploration.Improving Selectivity and Efficiency.One of the key challenges in asymmetric radical reactions is achieving high selectivity and efficiency. While significant progress has been made in this area, there is still room for improvement. Future research could focus on developing new chiral catalysts/auxiliaries that can promote asymmetric radical reactions with higher selectivity and efficiency.Expanding the Scope of Reactions.Currently, the scope of asymmetric radical reactions is limited by the availability of suitable precursors and the reactivity of the generated radicals. Future research could aim to expand the scope of these reactions by developing new methods for generating radicals with desired functionalities and reactivities.Applications in Sustainable Chemistry.In the context of sustainable chemistry, asymmetric radical reactions offer an attractive alternative to traditional synthetic methods. By utilizing renewableresources and mild reaction conditions, asymmetric radical reactions could contribute to the development of more sustainable synthetic routes for the preparation of chiral compounds.Integration with Other Techniques.The integration of asymmetric radical reactions with other techniques, such as photocatalysis, electrochemistry, and microfluidics, could lead to the development of new and innovative synthetic methods. By combining the advantages of these techniques, it may be possible to achieve even higher selectivity, efficiency, and scalability in asymmetric radical reactions.In conclusion, asymmetric radical reactions have emerged as powerful tools for the synthesis of enantiomerically enriched chiral compounds. While significant progress has been made in this area, there are still numerous opportunities for further exploration and development. Future research in this field could lead tothe discovery of new and innovative synthetic methods with improved selectivity, efficiency, and sustainability.。
基于周期采样的分布式动态事件触发优化算法
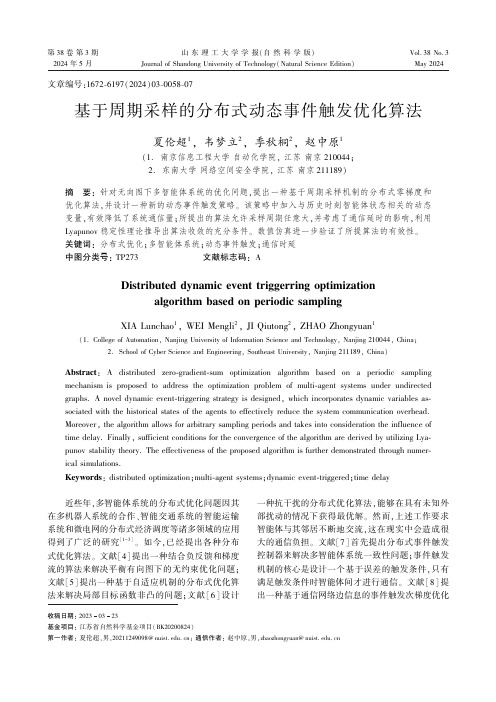
第38卷第3期2024年5月山东理工大学学报(自然科学版)Journal of Shandong University of Technology(Natural Science Edition)Vol.38No.3May 2024收稿日期:20230323基金项目:江苏省自然科学基金项目(BK20200824)第一作者:夏伦超,男,20211249098@;通信作者:赵中原,男,zhaozhongyuan@文章编号:1672-6197(2024)03-0058-07基于周期采样的分布式动态事件触发优化算法夏伦超1,韦梦立2,季秋桐2,赵中原1(1.南京信息工程大学自动化学院,江苏南京210044;2.东南大学网络空间安全学院,江苏南京211189)摘要:针对无向图下多智能体系统的优化问题,提出一种基于周期采样机制的分布式零梯度和优化算法,并设计一种新的动态事件触发策略㊂该策略中加入与历史时刻智能体状态相关的动态变量,有效降低了系统通信量;所提出的算法允许采样周期任意大,并考虑了通信延时的影响,利用Lyapunov 稳定性理论推导出算法收敛的充分条件㊂数值仿真进一步验证了所提算法的有效性㊂关键词:分布式优化;多智能体系统;动态事件触发;通信时延中图分类号:TP273文献标志码:ADistributed dynamic event triggerring optimizationalgorithm based on periodic samplingXIA Lunchao 1,WEI Mengli 2,JI Qiutong 2,ZHAO Zhongyuan 1(1.College of Automation,Nanjing University of Information Science and Technology,Nanjing 210044,China;2.School of Cyber Science and Engineering,Southeast University,Nanjing 211189,China)Abstract :A distributed zero-gradient-sum optimization algorithm based on a periodic sampling mechanism is proposed to address the optimization problem of multi-agent systems under undirected graphs.A novel dynamic event-triggering strategy is designed,which incorporates dynamic variables as-sociated with the historical states of the agents to effectively reduce the system communication overhead.Moreover,the algorithm allows for arbitrary sampling periods and takes into consideration the influence oftime delay.Finally,sufficient conditions for the convergence of the algorithm are derived by utilizing Lya-punov stability theory.The effectiveness of the proposed algorithm is further demonstrated through numer-ical simulations.Keywords :distributed optimization;multi-agent systems;dynamic event-triggered;time delay ㊀㊀近些年,多智能体系统的分布式优化问题因其在多机器人系统的合作㊁智能交通系统的智能运输系统和微电网的分布式经济调度等诸多领域的应用得到了广泛的研究[1-3]㊂如今,已经提出各种分布式优化算法㊂文献[4]提出一种结合负反馈和梯度流的算法来解决平衡有向图下的无约束优化问题;文献[5]提出一种基于自适应机制的分布式优化算法来解决局部目标函数非凸的问题;文献[6]设计一种抗干扰的分布式优化算法,能够在具有未知外部扰动的情况下获得最优解㊂然而,上述工作要求智能体与其邻居不断地交流,这在现实中会造成很大的通信负担㊂文献[7]首先提出分布式事件触发控制器来解决多智能体系统一致性问题;事件触发机制的核心是设计一个基于误差的触发条件,只有满足触发条件时智能体间才进行通信㊂文献[8]提出一种基于通信网络边信息的事件触发次梯度优化㊀算法,并给出了算法的指数收敛速度㊂文献[9]提出一种基于事件触发机制的零梯度和算法,保证系统状态收敛到最优解㊂上述事件触发策略是静态事件触发策略,即其触发阈值仅与智能体的状态相关,当智能体的状态逐渐收敛时,很容易满足触发条件并将生成大量不必要的通信㊂因此,需要设计更合理的触发条件㊂文献[10]针对非线性系统的增益调度控制问题,提出一种动态事件触发机制的增益调度控制器;文献[11]提出一种基于动态事件触发条件的零梯度和算法,用于有向网络的优化㊂由于信息传输的复杂性,时间延迟在实际系统中无处不在㊂关于考虑时滞的事件触发优化问题的文献很多㊂文献[12]研究了二阶系统的凸优化问题,提出时间触发算法和事件触发算法两种分布式优化算法,使得所有智能体协同收敛到优化问题的最优解,并有效消除不必要的通信;文献[13]针对具有传输延迟的多智能体系统,提出一种具有采样数据和时滞的事件触发分布式优化算法,并得到系统指数稳定的充分条件㊂受文献[9,14]的启发,本文提出一种基于动态事件触发机制的分布式零梯度和算法,与使用静态事件触发机制的文献[15]相比,本文采用动态事件触发机制可以避免智能体状态接近最优值时频繁触发造成的资源浪费㊂此外,考虑到进行动态事件触发判断需要一定的时间,使用当前状态值是不现实的,因此,本文使用前一时刻状态值来构造动态事件触发条件,更符合逻辑㊂由于本文采用周期采样机制,这进一步降低了智能体间的通信频率,但采样周期过长会影响算法收敛㊂基于文献[14]的启发,本文设计的算法允许采样周期任意大,并且对于有时延的系统,只需要其受采样周期的限制,就可得到保证多智能体系统达到一致性和最优性的充分条件㊂最后,通过对一个通用示例进行仿真,验证所提算法的有效性㊂1㊀预备知识及问题描述1.1㊀图论令R表示实数集,R n表示向量集,R nˑn表示n ˑn实矩阵的集合㊂将包含n个智能体的多智能体系统的通信网络用图G=(V,E)建模,每个智能体都视为一个节点㊂该图由顶点集V={1,2, ,n}和边集E⊆VˑV组成㊂定义A=[a ij]ɪR nˑn为G 的加权邻接矩阵,当a ij>0时,表明节点i和节点j 间存在路径,即(i,j)ɪE;当a ij=0时,表明节点i 和节点j间不存在路径,即(i,j)∉E㊂D=diag{d1, ,d n}表示度矩阵,拉普拉斯矩阵L等于度矩阵减去邻接矩阵,即L=D-A㊂当图G是无向图时,其拉普拉斯矩阵是对称矩阵㊂1.2㊀凸函数设h i:R nңR是在凸集ΩɪR n上的局部凸函数,存在正常数φi使得下列条件成立[16]:h i(b)-h i(a)- h i(a)T(b-a)ȡ㊀㊀㊀㊀φi2 b-a 2,∀a,bɪΩ,(1)h i(b)- h i(a)()T(b-a)ȡ㊀㊀㊀㊀φi b-a 2,∀a,bɪΩ,(2) 2h i(a)ȡφi I n,∀aɪΩ,(3)式中: h i为h i的一阶梯度, 2h i为h i的二阶梯度(也称黑塞矩阵)㊂1.3㊀问题描述考虑包含n个智能体的多智能体系统,假设每个智能体i的成本函数为f i(x),本文的目标是最小化以下的优化问题:x∗=arg minxɪΩðni=1f i(x),(4)式中:x为决策变量,x∗为全局最优值㊂1.4㊀主要引理引理1㊀假设通信拓扑图G是无向且连通的,对于任意XɪR n,有以下关系成立[17]:X T LXȡαβX T L T LX,(5)式中:α是L+L T2最小的正特征值,β是L T L最大的特征值㊂引理2(中值定理)㊀假设局部成本函数是连续可微的,则对于任意实数y和y0,存在y~=y0+ω~(y -y0),使得以下不等式成立:f i(y)=f i(y0)+∂f i∂y(y~)(y-y0),(6)式中ω~是正常数且满足ω~ɪ(0,1)㊂2㊀基于动态事件触发机制的分布式优化算法及主要结果2.1㊀考虑时延的分布式动态事件触发优化算法本文研究具有时延的多智能体系统的优化问题㊂为了降低智能体间的通信频率,提出一种采样周期可任意设计的分布式动态事件触发优化算法,95第3期㊀㊀㊀㊀㊀㊀㊀㊀㊀㊀㊀㊀夏伦超,等:基于周期采样的分布式动态事件触发优化算法其具体实现通信优化的流程图如图1所示㊂首先,将邻居和自身前一触发时刻状态送往控制器(本文提出的算法),得到智能体的状态x i (t )㊂然后,预设一个固定采样周期h ,使得所有智能体在同一时刻进行采样㊂同时,在每个智能体上都配置了事件检测器,只在采样时刻检查是否满足触发条件㊂接着,将前一采样时刻的智能体状态发送至构造的触发器中进行判断,当满足设定的触发条件时,得到触发时刻的智能体状态x^i (t )㊂最后,将得到的本地状态x^i (t )用于更新自身及其邻居的控制操作㊂由于在实际传输中存在时延,因此需要考虑满足0<τ<h 的时延㊂图1㊀算法实现流程图考虑由n 个智能体构成的多智能体系统,其中每个智能体都能独立进行计算和相互通信,每个智能体i 具有如下动态方程:x ㊃i (t )=-1h2f i (x i )()-1u i (t ),(7)式中u i (t )为设计的控制算法,具体为u i (t )=ðnj =1a ij x^j (t -τ)-x ^i (t -τ)()㊂(8)㊀㊀给出设计的动态事件触发条件:θi d i e 2i (lh )-γq i (lh -h )()ɤξi (lh ),(9)q i (t )=ðnj =1a ij x^i (t -τ)-x ^j (t -τ)()2,(10)㊀㊀㊀ξ㊃i (t )=1h[-μi ξi (lh )+㊀㊀㊀㊀㊀δi γq i (lh -h )-d i e 2i (lh )()],(11)式中:d i 是智能体i 的入度;γ是正常数;θi ,μi ,δi 是设计的参数㊂令x i (lh )表示采样时刻智能体的状态,偏差变量e i (lh )=x i (lh )-x^i (lh )㊂注释1㊀在进行动态事件触发条件设计时,可以根据不同的需求为每个智能体设定不同的参数θi ,μi ,δi ,以确保其能够在特定的情境下做出最准确的反应㊂本文为了方便分析,选择为每个智能体设置相同的θi ,μi ,δi ,以便更加清晰地研究其行为表现和响应能力㊂2.2㊀主要结果和分析由于智能体仅在采样时刻进行事件触发条件判断,并在达到触发条件后才通信,因此有x ^i (t -τ)=x^i (lh )㊂定理1㊀假设无向图G 是连通的,对于任意i ɪV 和t >0,当满足条件(12)时,在算法(7)和动态事件触发条件(9)的作用下,系统状态趋于优化解x ∗,即lim t ңx i (t )=x ∗㊂12-β2φm α-τβ2φm αh -γ>0,μi+δi θi <1,μi-1-δi θi >0,ìîíïïïïïïïï(12)式中φm =min{φ1,φ2}㊂证明㊀对于t ɪ[lh +τ,(l +1)h +τ),定义Lyapunov 函数V (t )=V 1(t )+V 2(t ),其中:V 1(t )=ðni =1f i (x ∗)-f i (x i )-f ᶄi (x i )(x ∗-x i )(),V 2(t )=ðni =1ξi (t )㊂令E (t )=e 1(t ), ,e n (t )[]T ,X (t )=x 1(t ), ,x n (t )[]T ,X^(t )=x ^1(t ), ,x ^n (t )[]T ㊂对V 1(t )求导得V ㊃1(t )=1h ðni =1u i (t )x ∗-x i (t )(),(13)由于ðni =1ðnj =1a ij x ^j (t -τ)-x ^i (t -τ)()㊃x ∗=0成立,有V ㊃1(t )=-1hX T (t )LX ^(lh )㊂(14)6山东理工大学学报(自然科学版)2024年㊀由于㊀㊀X (t )=X (lh +τ)-(t -lh -τ)X ㊃(t )=㊀㊀㊀㊀X (lh )+τX ㊃(lh )+t -lh -τhΓ1LX^(lh )=㊀㊀㊀㊀X (lh )-τh Γ2LX^(lh -h )+㊀㊀㊀㊀(t -lh -τ)hΓ1LX^(lh ),(15)式中:Γ1=diag (f i ᶄᶄ(x ~11))-1, ,(f i ᶄᶄ(x ~1n ))-1{},Γ2=diag (f i ᶄᶄ(x ~21))-1, ,(f i ᶄᶄ(x ~2n))-1{},x ~1iɪ(x i (lh +τ),x i (t )),x ~2i ɪ(x i (lh ),x i (lh+τ))㊂将式(15)代入式(14)得㊀V ㊃1(t )=-1h E T (lh )LX ^(lh )-1hX ^T (lh )LX ^(lh )+㊀㊀㊀τh2Γ2X ^T (lh -h )L T LX ^(lh )+㊀㊀㊀(t -lh -τ)h2Γ1X ^T (lh )L T LX ^(lh )㊂(16)根据式(3)得(f i ᶄᶄ(x ~i 1))-1ɤ1φi,i =1, ,n ㊂即Γ1ɤ1φm I n ,Γ2ɤ1φmI n ,φm =min{φ1,φ2}㊂首先对(t -lh -τ)h2Γ1X ^T (lh )L T LX ^(lh )项进行分析,对于t ɪ[lh +τ,(l +1)h +τ),基于引理1和式(3)有(t -lh -τ)h2Γ1X ^T (lh )L T LX ^(lh )ɤβhφm αX ^T (lh )LX ^(lh )ɤβ2hφm αðni =1q i(lh ),(17)式中最后一项根据X^T (t )LX ^(t )=12ðni =1q i(t )求得㊂接着分析τh2Γ2X ^(lh -h )L T LX ^(lh ),根据引理1和杨式不等式有:τh2Γ2X ^T (lh -h )L T LX ^(lh )ɤ㊀㊀㊀㊀τβ2h 2φm αX ^T (lh -h )LX ^(lh -h )+㊀㊀㊀㊀τβ2h 2φm αX ^T (lh )LX ^(lh )ɤ㊀㊀㊀㊀τβ4h 2φm αðni =1q i (lh -h )+ðni =1q i (lh )[]㊂(18)将式(17)和式(18)代入式(16)得㊀V ㊃1(t )ɤβ2φm α+τβ4φm αh -12()1h ðni =1q i(lh )+㊀㊀㊀τβ4φm αh ðni =1q i (lh -h )+1h ðni =1d i e 2i(lh )㊂(19)根据式(11)得V ㊃2(t )=-ðni =1μih ξi(lh )+㊀㊀㊀㊀ðni =1δihγq i (lh -h )-d i e 2i (lh )()㊂(20)结合式(19)和式(20)得V ㊃(t )ɤ-12-β2φm α-τβ4φm αh ()1h ðni =1q i (lh )+㊀㊀㊀㊀τβ4φm αh 2ðn i =1q i (lh -h )+γh ðni =1q i (lh -h )-㊀㊀㊀㊀1h ðni =1(μi -1-δi θi)ξi (lh ),(21)因此根据李雅普诺夫函数的正定性以及Squeeze 定理得㊀V (l +1)h +τ()-V (lh +τ)ɤ㊀㊀㊀-12-β2φm α-τβ4φm αh()ðni =1q i(lh )+㊀㊀㊀τβ4φm αh ðni =1q i (lh -h )+γðni =1q i (lh -h )-㊀㊀㊀ðni =1(μi -1-δiθi)ξi (lh )㊂(22)对式(22)迭代得V (l +1)h +τ()-V (h +τ)ɤ㊀㊀-12-β2φm α-τβ2φm αh-γ()ðl -1k =1ðni =1q i(kh )+㊀㊀τβ4φm αh ðni =1q i (0h )-㊀㊀12-β2φm α-τβ4φm αh()ðni =1q i(lh )-㊀㊀ðlk =1ðni =1μi -1-δiθi()ξi (kh ),(23)进一步可得㊀lim l ңV (l +1)h -V (h )()ɤ㊀㊀㊀τβ4φm αh ðni =1q i(0h )-16第3期㊀㊀㊀㊀㊀㊀㊀㊀㊀㊀㊀㊀夏伦超,等:基于周期采样的分布式动态事件触发优化算法㊀㊀㊀ðni =1(μi -1-δi θi )ðl =1ξi (lh )-㊀㊀㊀12-β2φm α-τβ2φm αh-γ()ð l =1ðni =1q i(lh )㊂(24)由于q i (lh )ȡ0和V (t )ȡ0,由式(24)得lim l ң ðni =1ξi (lh )=0㊂(25)基于ξi 的定义和拉普拉斯矩阵的性质,可以得到每个智能体的最终状态等于相同的常数,即lim t ңx 1(t )= =lim t ңx n (t )=c ㊂(26)㊀㊀由于目标函数的二阶导数具有以下性质:ðni =1d f ᶄi (x i (t ))()d t =㊀㊀㊀㊀-ðn i =1ðnj =1a ij x ^j (t )-x ^i (t )()=㊀㊀㊀㊀-1T LX^(t )=0,(27)式中1=[1, ,1]n ,所以可以得到ðni =1f i ᶄ(x i (t ))=ðni =1f i ᶄ(x ∗i )=0㊂(28)联立式(26)和式(28)得lim t ңx 1(t )= =lim t ңx n (t )=c =x ∗㊂(29)㊀㊀定理1证明完成㊂当不考虑通信时延τ时,可由定理1得到推论1㊂推论1㊀假设通信图G 是无向且连通的,当不考虑时延τ时,对于任意i ɪV 和t >0,若条件(30)成立,智能体状态在算法(7)和触发条件(9)的作用下趋于最优解㊂14-n -1φm -γ>0,μi+δi θi <1,μi-1-δi θi >0㊂ìîíïïïïïïïï(30)㊀㊀证明㊀该推论的证明过程类似定理1,由定理1结果可得14-β2φm α-γ>0㊂(31)令λn =βα,由于λn 是多智能体系统的全局信息,因此每个智能体很难获得,但其上界可以根据以下关系来估计:λn ɤ2d max ɤ2(n -1),(32)式中d max =max{d i },i =1, ,n ㊂因此得到算法在没有时延情况下的充分条件:14-n -1φm -γ>0㊂(33)㊀㊀推论1得证㊂注释2㊀通过定理1得到的稳定性条件,可以得知当采样周期h 取较小值时,由于0<τ<h ,因此二者可以抵消,从而稳定性不受影响;而当采样周期h 取较大值时,τβ2φm αh项可以忽略不计,因此从理论分析可以得出允许采样周期任意大的结论㊂从仿真实验方面来看,当采样周期h 越大,需要的收剑时间越长,但最终结果仍趋于优化解㊂然而,在文献[18]中,采样周期过大会导致稳定性条件难以满足,即算法最终难以收敛,无法达到最优解㊂因此,本文提出的算法允许采样周期任意大,这一创新点具有重要意义㊂3㊀仿真本文对一个具有4个智能体的多智能体网络进行数值模拟,智能体间的通信拓扑如图2所示㊂采用4个智能体的仿真网络仅是为了初步验证所提算法的有效性㊂值得注意的是,当多智能体的数量增加时,算法的时间复杂度和空间复杂度会增加,但并不会影响其有效性㊂因此,该算法在更大规模的多智能体网络中同样适用㊂成本函数通常选择凸函数㊂例如,在分布式传感器网络中,成本函数为z i -x 2+εi x 2,其中x 表示要估计的未知参数,εi 表示观测噪声,z i 表示在(0,1)中均匀分布的随机数;在微电网中,成本函数为a i x 2+b i x +c i ,其中a i ,b i ,c i 是发电机成本参数㊂这两种情境下的成本函数形式不同,但本质上都是凸函数㊂本文采用论文[19]中的通用成本函数(式(34)),用于证明本文算法在凸函数上的可行性㊂此外,通信拓扑图结构并不会影响成本函数的设计,因此,本文的成本函数在分布式网络凸优化问题中具有通用性㊂g i (x )=(x -i )4+4i (x -i )2,i =1,2,3,4㊂(34)很明显,当x i 分别等于i 时,得到最小局部成本函数,但是这不是全局最优解x ∗㊂因此,需要使用所提算法来找到x ∗㊂首先设置重要参数,令φm =16,γ=0.1,θi =1,ξi (0)=5,μi =0.2,δi =0.2,26山东理工大学学报(自然科学版)2024年㊀图2㊀通信拓扑图x i (0)=i ,i =1,2,3,4㊂图3为本文算法(7)解决优化问题(4)时各智能体的状态,其中设置采样周期h =3,时延τ=0.02㊂智能体在图3中渐进地达成一致,一致值为全局最优点x ∗=2.935㊂当不考虑采样周期影响时,即在采样周期h =3,时延τ=0.02的条件下,采用文献[18]中的算法(10)时,各智能体的状态如图4所示㊂显然,在避免采样周期的影响后,本文算法具有更快的收敛速度㊂与文献[18]相比,由于只有当智能体i 及其邻居的事件触发判断完成,才能得到q i (lh )的值,因此本文采用前一时刻的状态值构造动态事件触发条件更符合逻辑㊂图3㊀h =3,τ=0.02时算法(7)的智能体状态图4㊀h =3,τ=0.02时算法(10)的智能体状态为了进一步分析采样周期的影响,在时延τ不变的情况下,选择不同的采样周期h ,其结果显示在图5中㊂对比图3可以看出,选择较大的采样周期则收敛速度减慢㊂事实上,这在算法(7)中是很正常的,因为较大的h 会削弱反馈增益并减少固定有限时间间隔中的控制更新次数,具体显示在图6和图7中㊂显然,当选择较大的采样周期时,智能体的通信频率显著下降,同时也会导致收敛速度减慢㊂因此,虽然采样周期允许任意大,但在收敛速度和通信频率之间需要做出权衡,以选择最优的采样周期㊂图5㊀h =1,τ=0.02时智能体的状态图6㊀h =3,τ=0.02时的事件触发时刻图7㊀h =1,τ=0.02时的事件触发时刻最后,固定采样周期h 的值,比较τ=0.02和τ=2时智能体的状态,结果如图8所示㊂显然,时延会使智能体找到全局最优点所需的时间更长,但由于其受采样周期的限制,最终仍可以对于任意有限延迟达成一致㊂图8㊀h =3,τ=2时智能体的状态36第3期㊀㊀㊀㊀㊀㊀㊀㊀㊀㊀㊀㊀夏伦超,等:基于周期采样的分布式动态事件触发优化算法4 结束语本文研究了无向图下的多智能体系统的优化问题,提出了一种基于动态事件触发机制的零梯度和算法㊂该机制中加入了与前一时刻智能体状态相关的动态变量,避免智能体状态接近最优值时频繁触发产生的通信负担㊂同时,在算法和触发条件设计中考虑了采样周期的影响,在所设计的算法下,允许采样周期任意大㊂对于有时延的系统,在最大允许传输延迟小于采样周期的情况下,给出了保证多智能体系统达到一致性和最优性的充分条件㊂今后拟将本算法向有向图和切换拓扑图方向推广㊂参考文献:[1]杨洪军,王振友.基于分布式算法和查找表的FIR滤波器的优化设计[J].山东理工大学学报(自然科学版),2009,23(5):104-106,110.[2]CHEN W,LIU L,LIU G P.Privacy-preserving distributed economic dispatch of microgrids:A dynamic quantization-based consensus scheme with homomorphic encryption[J].IEEE Transactions on Smart Grid,2022,14(1):701-713.[3]张丽馨,刘伟.基于改进PSO算法的含分布式电源的配电网优化[J].山东理工大学学报(自然科学版),2017,31(6):53-57.[4]KIA S S,CORTES J,MARTINEZ S.Distributed convex optimization via continuous-time coordination algorithms with discrete-time communication[J].Automatica,2015,55:254-264.[5]LI Z H,DING Z T,SUN J Y,et al.Distributed adaptive convex optimization on directed graphs via continuous-time algorithms[J]. IEEE Transactions on Automatic Control,2018,63(5):1434 -1441.[6]段书晴,陈森,赵志良.一阶多智能体受扰系统的自抗扰分布式优化算法[J].控制与决策,2022,37(6):1559-1566. [7]DIMAROGONAS D V,FRAZZOLI E,JOHANSSON K H.Distributed event-triggered control for multi-agent systems[J].IEEE Transactions on Automatic Control,2012,57(5):1291-1297.[8]KAJIYAMA Y C,HAYASHI N K,TAKAI S.Distributed subgradi-ent method with edge-based event-triggered communication[J]. IEEE Transactions on Automatic Control,2018,63(7):2248 -2255.[9]LIU J Y,CHEN W S,DAI H.Event-triggered zero-gradient-sum distributed convex optimisation over networks with time-varying topol-ogies[J].International Journal of Control,2019,92(12):2829 -2841.[10]COUTINHO P H S,PALHARES R M.Codesign of dynamic event-triggered gain-scheduling control for a class of nonlinear systems [J].IEEE Transactions on Automatic Control,2021,67(8): 4186-4193.[11]CHEN W S,REN W.Event-triggered zero-gradient-sum distributed consensus optimization over directed networks[J].Automatica, 2016,65:90-97.[12]TRAN N T,WANG Y W,LIU X K,et al.Distributed optimization problem for second-order multi-agent systems with event-triggered and time-triggered communication[J].Journal of the Franklin Insti-tute,2019,356(17):10196-10215.[13]YU G,SHEN Y.Event-triggered distributed optimisation for multi-agent systems with transmission delay[J].IET Control Theory& Applications,2019,13(14):2188-2196.[14]LIU K E,JI Z J,ZHANG X F.Periodic event-triggered consensus of multi-agent systems under directed topology[J].Neurocomputing, 2020,385:33-41.[15]崔丹丹,刘开恩,纪志坚,等.周期事件触发的多智能体分布式凸优化[J].控制工程,2022,29(11):2027-2033. [16]LU J,TANG C Y.Zero-gradient-sum algorithms for distributed con-vex optimization:The continuous-time case[J].IEEE Transactions on Automatic Control,2012,57(9):2348-2354. [17]LIU K E,JI Z J.Consensus of multi-agent systems with time delay based on periodic sample and event hybrid control[J].Neurocom-puting,2016,270:11-17.[18]ZHAO Z Y.Sample-baseddynamic event-triggered algorithm for op-timization problem of multi-agent systems[J].International Journal of Control,Automation and Systems,2022,20(8):2492-2502.[19]LIU J Y,CHEN W S.Distributed convex optimisation with event-triggered communication in networked systems[J].International Journal of Systems Science,2016,47(16):3876-3887.(编辑:杜清玲)46山东理工大学学报(自然科学版)2024年㊀。
On the asymptotic expansion of the solutions of the separated nonlinear Schroedinger equati

a r X i v :n l i n /0012025v 3 [n l i n .S I ] 10 M a y 2001On the Asymptotic Expansion of the Solutions of the Separated Nonlinear Schr¨o dinger EquationA.A.Kapaev,St Petersburg Department of Steklov Mathematical Institute,Fontanka 27,St Petersburg 191011,Russia,V.E.Korepin,C.N.Yang Institute for Theoretical Physics,State University of New York at Stony Brook,Stony Brook,NY 11794-3840,USAAbstractNonlinear Schr¨o dinger equation with the Schwarzian initial data is important in nonlinear optics,Bose condensation and in the theory of strongly correlated electrons.The asymptotic solutions in the region x/t =O (1),t →∞,can be represented as a double series in t −1and ln t .Our current purpose is the description of the asymptotics of the coefficients of the series.MSC 35A20,35C20,35G20Keywords:integrable PDE,long time asymptotics,asymptotic expansion1IntroductionA coupled nonlinear dispersive partial differential equation in (1+1)dimension for the functions g +and g −,−i∂t g +=12∂2x g −+4g 2−g +,(1)called the separated Nonlinear Schr¨o dinger equation (sNLS),contains the con-ventional NLS equation in both the focusing and defocusing forms as g +=¯g −or g +=−¯g −,respectively.For certain physical applications,e.g.in nonlin-ear optics,Bose condensation,theory of strongly correlated electrons,see [1]–[9],the detailed information on the long time asymptotics of solutions with initial conditions rapidly decaying as x →±∞is quite useful for qualitative explanation of the experimental phenomena.Our interest to the long time asymptotics for the sNLS equation is inspired by its application to the Hubbard model for one-dimensional gas of strongly correlated electrons.The model explains a remarkable effect of charge and spin separation,discovered experimentally by C.Kim,Z.-X.M.Shen,N.Motoyama,H.Eisaki,hida,T.Tohyama and S.Maekawa [19].Theoretical justification1of the charge and spin separation include the study of temperature dependent correlation functions in the Hubbard model.In the papers[1]–[3],it was proven that time and temperature dependent correlations in Hubbard model can be described by the sNLS equation(1).For the systems completely integrable in the sense of the Lax representa-tion[10,11],the necessary asymptotic information can be extracted from the Riemann-Hilbert problem analysis[12].Often,the fact of integrability implies the existence of a long time expansion of the generic solution in a formal series, the successive terms of which satisfy some recurrence relation,and the leading order coefficients can be expressed in terms of the spectral data for the associ-ated linear system.For equation(1),the Lax pair was discovered in[13],while the formulation of the Riemann-Hilbert problem can be found in[8].As t→∞for x/t bounded,system(1)admits the formal solution given byg+=e i x22+iν)ln4t u0+∞ n=12n k=0(ln4t)k2t −(1t nv nk ,(2)where the quantitiesν,u0,v0,u nk and v nk are some functions ofλ0=−x/2t.For the NLS equation(g+=±¯g−),the asymptotic expansion was suggested by M.Ablowitz and H.Segur[6].For the defocusing NLS(g+=−¯g−),the existence of the asymptotic series(2)is proven by P.Deift and X.Zhou[9] using the Riemann-Hilbert problem analysis,and there is no principal obstacle to extend their approach for the case of the separated NLS equation.Thus we refer to(2)as the Ablowitz-Segur-Deift-Zhou expansion.Expressions for the leading coefficients for the asymptotic expansion of the conventional NLS equation in terms of the spectral data were found by S.Manakov,V.Zakharov, H.Segur and M.Ablowitz,see[14]–[16].The general sNLS case was studied by A.Its,A.Izergin,V.Korepin and G.Varzugin[17],who have expressed the leading order coefficients u0,v0andν=−u0v0in(2)in terms of the spectral data.The generic solution of the focusing NLS equation contains solitons and radiation.The interaction of the single soliton with the radiation was described by Segur[18].It can be shown that,for the generic Schwarzian initial data and generic bounded ratio x/t,|c−xthese coefficients as well as for u n,2n−1,v n,2n−1,wefind simple exact formulaeu n,2n=u0i n(ν′)2n8n n!,(3)and(20)below.We describe coefficients at other powers of ln t using the gener-ating functions which can be reduced to a system of polynomials satisfying the recursion relations,see(24),(23).As a by-product,we modify the Ablowitz-Segur-Deift-Zhou expansion(2),g+=exp i x22+iν)ln4t+i(ν′)2ln24t2] k=0(ln4t)k2t −(18t∞n=02n−[n+1t n˜v n,k.(4)2Recurrence relations and generating functions Substituting(2)into(1),and equating coefficients of t−1,wefindν=−u0v0.(5) In the order t−n,n≥2,equating coefficients of ln j4t,0≤j≤2n,we obtain the recursion−i(j+1)u n,j+1+inu n,j=νu n,j−iν′′8u n−1,j−2−−iν′8u′′n−1,j+nl,k,m=0l+k+m=nα=0, (2)β=0, (2)γ=0, (2)α+β+γ=ju l,αu k,βv m,γ,(6) i(j+1)v n,j+1−inv n,j=νv n,j+iν′′8v n−1,j−2++iν′8v′′n−1,j+nl,k,m=0l+k+m=nα=0, (2)β=0, (2)γ=0, (2)α+β+γ=ju l,αv k,βv m,γ,(7)where the prime means differentiation with respect toλ0=−x/(2t).Master generating functions F(z,ζ),G(z,ζ)for the coefficients u n,k,v n,k are defined by the formal seriesF(z,ζ)= n,k u n,k z nζk,G(z,ζ)= n,k v n,k z nζk,(8)3where the coefficients u n,k,v n,k vanish for n<0,k<0and k>2n.It is straightforward to check that the master generating functions satisfy the nonstationary separated Nonlinear Schr¨o dinger equation in(1+2)dimensions,−iFζ+izF z= ν−iν′′8zζ2 F−iν′8zF′′+F2G,iGζ−izG z= ν+iν′′8zζ2 G+iν′8zG′′+F G2.(9) We also consider the sectional generating functions f j(z),g j(z),j≥0,f j(z)=∞n=0u n,2n−j z n,g j(z)=∞n=0v n,2n−j z n.(10)Note,f j(z)≡g j(z)≡0for j<0because u n,k=v n,k=0for k>2n.The master generating functions F,G and the sectional generating functions f j,g j are related by the equationsF(zζ−2,ζ)=∞j=0ζ−j f j(z),G(zζ−2,ζ)=∞j=0ζ−j g j(z).(11)Using(11)in(9)and equating coefficients ofζ−j,we obtain the differential system for the sectional generating functions f j(z),g j(z),−2iz∂z f j−1+i(j−1)f j−1+iz∂z f j==νf j−z iν′′8f j−ziν′8f′′j−2+jk,l,m=0k+l+m=jf k f lg m,2iz∂z g j−1−i(j−1)g j−1−iz∂z g j=(12)=νg j+z iν′′8g j+ziν′8g′′j−2+jk,l,m=0k+l+m=jf kg l g m.Thus,the generating functions f0(z),g0(z)for u n,2n,v n,2n solve the systemiz∂z f0=νf0−z (ν′)28g0+f0g20.(13)The system implies that the product f0(z)g0(z)≡const.Since f0(0)=u0and g0(0)=v0,we obtain the identityf0g0(z)=−ν.(14) Using(14)in(13),we easilyfindf0(z)=u0e i(ν′)28n n!z n,4g0(z)=v0e−i(ν′)28n n!z n,(15)which yield the explicit expressions(3)for the coefficients u n,2n,v n,2n.Generating functions f1(z),g1(z)for u n,2n−1,v n,2n−1,satisfy the differential system−2iz∂z f0+iz∂z f1=νf1−z iν′′8f1−ziν′8g0−z(ν′)24g′0+f1g20+2f0g0g1.(16)We will show that the differential system(16)for f1(z)and g1(z)is solvable in terms of elementary functions.First,let us introduce the auxiliary functionsp1(z)=f1(z)g0(z).These functions satisfy the non-homogeneous system of linear ODEs∂z p1=iν4−ν′′4f′0z(p1+q1)−i(ν′)28−ν′g0,(17)so that∂z(q1+p1)=−(ν2)′′8z,p1(z)= −iνν′′8−ν′u′032z2,g1(z)=q1(z)g0(z),g0(z)=v0e−i(ν′)24−ν′′4v0 z+i(ν′)2ν′′4−ν′′4u0 ,v1,1=v0 iνν′′8−ν′v′0u n,2n −1=−2u 0i n −1(ν′)2(n −1)n −1ν′′u 0,n ≥2,v n,2n −1=−2v 0(−i )n −1(ν′)2(n −1)n −1ν′′v 0,n ≥2.Generating functions f j (z ),g j (z )for u n,2n −j ,v n,2n −j ,j ≥2,satisfy the differential system (12).Similarly to the case j =1above,let us introduce the auxiliary functions p j and q j ,p j =f jg 0.(21)In the terms of these functions,the system (12)reads,∂z p j =iνz(p j +q j )+b j ,(22)wherea j =2∂z p j −1+i (ν′)28−j −14(p j −1f 0)′8f 0+iν4−ν′′zq j −1−−ν′g 0+i(q j −2g 0)′′zj −1 k,l,m =0k +l +m =jp k q l q m .(23)With the initial condition p j (0)=q j (0)=0,the system is easily integrated and uniquely determines the functions p j (z ),q j (z ),p j (z )= z 0a j (ζ)dζ+iνzdζζζdξ(a j (ξ)+b j (ξ)).(24)These equations with expressions (23)together establish the recursion relationfor the functions p j (z ),q j (z ).In terms of p j (z )and q j (z ),expansion (2)readsg +=ei x22+iν)ln 4t +i(ν′)2ln 24tt2t−(18tv 0∞ j =0q j ln 24tln j 4t.(25)6Let a j (z )and b j (z )be polynomials of degree M with the zero z =0of multiplicity m ,a j (z )=M k =ma jk z k,b j (z )=Mk =mb jk z k .Then the functions p j (z )and q j (z )(24)arepolynomials of degree M +1witha zero at z =0of multiplicity m +1,p j (z )=M +1k =m +11k(a j,k −1+b j,k −1)z k ,q j (z )=M +1k =m +11k(a j,k −1+b j,k −1) z k.(26)On the other hand,a j (z )and b j (z )are described in (23)as the actions of the differential operators applied to the functions p j ′,q j ′with j ′<j .Because p 0(z )=q 0(z )≡1and p 1(z ),q 1(z )are polynomials of the second degree and a single zero at z =0,cf.(19),it easy to check that a 2(z )and b 2(z )are non-homogeneous polynomials of the third degree such thata 2,3=−(ν′)4(ν′′)2210(2+iν),(27)a 2,0=−iνν′′8−ν′u ′08u 0,b 2,0=iνν′′8−ν′v ′08v 0.Thus p 2(z )and q 2(z )are polynomials of the fourth degree with a single zero at z =0.Some of their coefficients arep 2,4=q 2,4=−(ν′)4(ν′′)24−(1+2iν)ν′′8u 0−ν(u ′0)24−(1−2iν)ν′′8v 0−ν(v ′0)22.Proof .The assertion holds true for j =0,1,2.Let it be correct for ∀j <j ′.Then a j ′(z )and b j ′(z )are defined as the sum of polynomials.The maximal de-grees of such polynomials are deg (p j ′−1f 0)′/f 0 =2j ′−1,deg (q j ′−1g 0)′/g 0 =72j′−1,anddeg 1z j′−1 α,β,γ=0α+β+γ=j′pαqβqγ =2j′−1. Thus deg a j′(z)=deg b j′(z)≤2j′−1,and deg p j′(z)=deg q j′(z)≤2j′.Multiplicity of the zero at z=0of a j′(z)and b j′(z)is no less than the min-imal multiplicity of the summed polynomials in(23),but the minor coefficients of the polynomials2∂z p j′−1and−(j−1)p j′−1/z,as well as of2∂z q j′−1and −(j−1)q j′−1/z may cancel each other.Let j′=2k be even.Thenm j′=min m j′−1;m j′−2+1;minα,β,γ=0,...,j′−1α+β+γ=j′mα+mβ+mγ =j′2 . Let j′=2k−1be odd.Then2m j′−1−(j′−1)=0,andm j′=min m j′−1+1;m j′−2+1;minα,β,γ=0,...,j′−1α+β+γ=j′mα+mβ+mγ =j′+12]p j,k z k,q j(z)=2jk=[j+12]z nn−[j+18k k!,g j(z)=v0∞n=[j+12]k=max{0;n−2j}q j,n−k(−i)k(ν′)2k2]k=max{0;n−2j}p j,n−ki k(ν′)2k2]k=max{0;n−2j}q j,n−k(−i)k(ν′)2kIn particular,the leading asymptotic term of these coefficients as n→∞and j fixed is given byu n,2n−j=u0p j,2j i n−2j(ν′)2(n−2j)n) ,v n,2n−j=v0q j,2j (−i)n−2j(ν′)2(n−2j)n) .(32)Thus we have reduced the problem of the evaluation of the asymptotics of the coefficients u n,2n−j v n,2n−j for large n to the computation of the leading coefficients of the polynomials p j(z),q j(z).In fact,using(24)or(26)and(23), it can be shown that the coefficients p j,2j,q j,2j satisfy the recurrence relationsp j,2j=−i (ν′)2ν′′2jj−1k,l,m=0k+l+m=jp k,2k p l,2l q m,2m++ν(ν′)2ν′′4j2j−1k,l,m=0k+l+m=jp k,2k(p l,2l−q l,2l)q m,2m,q j,2j=i (ν′)2ν′′2jj−1k,l,m=0k+l+m=jp k,2k q l,2l q m,2m−(33)−ν(ν′)2ν′′4j2j−1k,l,m=0k+l+m=jp k,2k(p l,2l−q l,2l)q m,2m.Similarly,the coefficients u n,0,v n,0for the non-logarithmic terms appears from(31)for j=2n,and are given simply byu n,0=u0p2n,n,v n,0=v0q2n,n.(34) Thus the problem of evaluation of the asymptotics of the coefficients u n,0,v n,0 for n large is equivalent to computation of the asymptotics of the minor coeffi-cients in the polynomials p j(z),q j(z).However,the last problem does not allow a straightforward solution because,according to(8),the sectional generating functions for the coefficients u n,0,v n,0are given byF(z,0)=∞n=0u n,0z n,G(z,0)=∞n=0v n,0z n,and solve the separated Nonlinear Schr¨o dinger equation−iFζ+izF z=νF+18zG′′+F G2.(35)93DiscussionOur consideration based on the use of generating functions of different types reveals the asymptotic behavior of the coefficients u n,2n−j,v n,2n−j as n→∞and jfixed for the long time asymptotic expansion(2)of the generic solution of the sNLS equation(1).The leading order dependence of these coefficients on n is described by the ratio a n2+d).The investigation of theRiemann-Hilbert problem for the sNLS equation yielding this estimate will be published elsewhere.Acknowledgments.We are grateful to the support of NSF Grant PHY-9988566.We also express our gratitude to P.Deift,A.Its and X.Zhou for discussions.A.K.was partially supported by the Russian Foundation for Basic Research under grant99-01-00687.He is also grateful to the staffof C.N.Yang Institute for Theoretical Physics of the State University of New York at Stony Brook for hospitality during his visit when this work was done. References[1]F.G¨o hmann,V.E.Korepin,Phys.Lett.A260(1999)516.[2]F.G¨o hmann,A.R.Its,V.E.Korepin,Phys.Lett.A249(1998)117.[3]F.G¨o hmann,A.G.Izergin,V.E.Korepin,A.G.Pronko,Int.J.Modern Phys.B12no.23(1998)2409.[4]V.E.Zakharov,S.V.Manakov,S.P.Novikov,L.P.Pitaevskiy,Soli-ton theory.Inverse scattering transform method,Moscow,Nauka,1980.[5]F.Calogero,A.Degasperis,Spectral transforms and solitons:toolsto solve and investigate nonlinear evolution equations,Amsterdam-New York-Oxford,1980.[6]M.J.Ablowitz,H.Segur,Solitons and the inverse scattering trans-form,SIAM,Philadelphia,1981.10[7]R.K.Dodd,J.C.Eilbeck,J.D.Gibbon,H.C.Morris,Solitons andnonlinear wave equations,Academic Press,London-Orlando-San Diego-New York-Toronto-Montreal-Sydney-Tokyo,1982.[8]L.D.Faddeev,L.A.Takhtajan,Hamiltonian Approach to the Soli-ton Theory,Nauka,Moscow,1986.[9]P.Deift,X.Zhou,Comm.Math.Phys.165(1995)175.[10]C.S.Gardner,J.M.Greene,M.D.Kruskal,R.M.Miura,Phys.Rev.Lett.19(1967)1095.[11]x,Comm.Pure Appl.Math.21(1968)467.[12]V.E.Zakharov,A.B.Shabat,Funkts.Analiz Prilozh.13(1979)13.[13]V.E.Zakharov,A.B.Shabat,JETP61(1971)118.[14]S.V.Manakov,JETP65(1973)505.[15]V.E.Zakharov,S.V.Manakov,JETP71(1973)203.[16]H.Segur,M.J.Ablowitz,J.Math.Phys.17(1976)710.[17]A.R.Its,A.G.Izergin,V.E.Korepin,G.G.Varzugin,Physica D54(1992)351.[18]H.Segur,J.Math.Phys.17(1976)714.[19]C.Kim,Z.-X.M.Shen,N.Motoyama,H.Eisaki,hida,T.To-hyama and S.Maekawa Phys Rev Lett.82(1999)802[20]A.R.Its,SR Izvestiya26(1986)497.11。
皂苷 生合成

ReviewMolecular activities,biosynthesis and evolution of triterpenoid saponinsJörg M.Augustin a ,Vera Kuzina a ,Sven B.Andersen b ,Søren Bak a ,⇑aPlant Biochemistry Laboratory,Department of Plant Biology and Biotechnology,Center for Synthetic Biology,VKR Research Centre ‘‘Pro-Active Plants’’,Faculty of Life Sciences,University of Copenhagen,Thorvaldsensvej 40,DK-1871Frederiksberg C,Copenhagen,Denmark bDepartment of Agriculture and Ecology,Faculty of Life Sciences,University of Copenhagen,Thorvaldsensvej 40,DK-1871Frederiksberg C,Copenhagen,Denmarka r t i c l e i n f o Article history:Received 30September 2010Received in revised form 7January 2011Available online 16February 2011Keywords:SaponinsHemolytic activity TriterpenesOxidosqualene cyclases Cytochromes P450UDP-gylcosyltransferases PhylogenyGene discoverya b s t r a c tSaponins are bioactive compounds generally considered to be produced by plants to counteract patho-gens and herbivores.Besides their role in plant defense,saponins are of growing interest for drug research as they are active constituents of several folk medicines and provide valuable pharmacological properties.Accordingly,much effort has been put into unraveling the modes of action of saponins,as well as in exploration of their potential for industrial processes and pharmacology.However,the exploitation of saponins for bioengineering crop plants with improved resistances against pests as well as circumven-tion of laborious and uneconomical extraction procedures for industrial production from plants is hampered by the lack of knowledge and availability of genes in saponin biosynthesis.Although the ability to produce saponins is rather widespread among plants,a complete synthetic pathway has not been elucidated in any single species.Current conceptions consider saponins to be derived from intermediates of the phytosterol pathway,and predominantly enzymes belonging to the multigene families of oxidosqualene cyclases (OSCs),cytochromes P450(P450s)and family 1UDP-glycosyltransferases (UGTs)are thought to be involved in their biosynthesis.Formation of unique structural features involves additional biosynthetical enzymes of diverse phylogenetic background.As an example of this,a serine carboxypeptidase-like acyltransferase (SCPL)was recently found to be involved in synthesis of triterpenoid saponins in oats.However,the total number of identified genes in saponin biosynthesis remains low as the complexity and diversity of these multigene families impede gene discovery based on sequence analysis and phylogeny.This review summarizes current knowledge of triterpenoid saponin biosynthesis in plants,molecular activities,evolutionary aspects and perspectives for further gene discovery.Ó2011Elsevier Ltd.All rights reserved.Contents 1.Introduction .........................................................................................................4362.Structural aspects and nomenclature .....................................................................................4373.Molecular activities on membranes ......................................................................................4384.Biosynthesis of triterpenoid saponins.....................................................................................4404.1.From acetyl-CoA to 2,3-oxidosqualene –common biosynthetic origin with phytosterols......................................4404.2.Cyclization of 2,3-oxidosqualene –emergence of sapogenin heterogeneity .................................................4414.3.Decoration of the cyclization product –elaboration of sapogenin diversity.................................................4464.4.Sapogenin glycosylation –conferring of biological activity ..............................................................4485.Conclusions..........................................................................................................451Acknowledgments ....................................................................................................452References ..........................................................................................................4520031-9422/$-see front matter Ó2011Elsevier Ltd.All rights reserved.doi:10.1016/j.phytochem.2011.01.015⇑Corresponding author.Tel.:+4535333346.E-mail addresses:joma@life.ku.dk (J.M.Augustin),verakuzina@ (V.Kuzina),sba@life.ku.dk (S.B.Andersen),bak@life.ku.dk (S.Bak).1.IntroductionThe term‘saponin’defines a group of natural compounds that consist of an isoprenoidal-derived aglycone,designated genin or sapogenin,covalently linked to one or more sugar moieties.The name is deduced from the Latin word sapo(Engl.:soap)reflecting their wide spread ability to form stable soap-like foams in aqueous solutions.In fact,many plant extracts(e.g.from soapwort–Saponaria officinalis,soapbark–Quillaja saponaria,etc.,Fig.1) previously used for their soap-resembling properties often gained this ability due to saponins(Hostettmann and Marston,1995).This characteristic trait is caused by the amphiphilic nature of saponins due to linkage of the lipophilic sapogenin to hydrophilic saccharide side chains.Most known saponins are plant-derived secondary metabolites, though several saponins are also found in marine animals such as sea cucumbers(Holothuroidea)(e.g.Van Dyck et al.,2010)and starfish(Asteroidea)(e.g.Liu et al.,2008).The ability to synthesize saponins is rather widespread among plants belonging to the divi-sion of Magnoliophyta,covering both dicotyledons and monocoty-ledons.However,the majority of saponin-producing species has been found within dicotyledons(Vincken et al.,2007).The biological role of saponins is not completely understood.In plants,they are generally considered to be part of defense systems due to anti-microbial,fungicidal,allelopathic,insecticidal and mol-luscicidal,etc.activities(reviewed in Francis et al.,2002;Sparg et al.,2004).Saponin-producing plants generally accumulate saponins as part of their normal development.However,saponin accumulation is also known to be influenced by several environmental factors such as nutrient and water availability,light irradiation or com-bined effects(reviewed in Szakiel et al.,in press).In addition,sapo-nin distribution has been found to vary greatly in individual plant organs or tissues during ontogenesis or to show seasonalfluctua-tions.Variations in saponin distribution and levels have been sug-gested to represent varying needs for protection and to target specific herbivores and pests,respectively.For example,Ndamba et al.(1994)propose that maximal saponin accumulation in early stages of Phytolacca dodecandra(soapberry)berry development is to prevent fruit loss and to assure seed maturation.Lin et al. (2009)discuss that the highest saponin accumulation among all organs of Dioscorea pseudojaponica Yamamoto(yam)is in tubers to provide protection for this reproductive stly,specific accumulation of saponins in the root epidermis of Avenae spp. (oats)has been demonstrated to counteract soil-borne fungi (Papadopoulou et al.,1999).Saponin levels are often increased in response to treatment with elicitors such as yeast extract or jasm-onate derivatives(reviewed in Yendo et al.,2010).As particularly jasmonates are well known for triggering plant defense responses to herbivory(reviewed in Howe and Jander,2008)this further cor-roborates the assumption of saponins being involved in plant defense.Due to their chemical properties and abilities as foaming agents, saponins or saponin-containing plant extracts are exploited by industry as additives to foods and cosmetics.They have the poten-tial to be used for further industrial applications as,for instance,as preservatives,flavor modifiers and agents for removal of choles-terol from dairy products(reviewed in Güçlü-Üstündag˘and Mazza, 2007;San Martín and Briones,1999).Saponin-rich byproducts from tea oil production have also been explored as botanical ver-micide for selective managing of earthworm casts on golf courses and sportfields(Potter et al.,2010).Saponins are also known to be major constituents of many traditional folk medicines(e.g.ex-tracts of liquorice–Glycyrrhiza sp.or ginseng–Panax sp.). Although health beneficial effects of these herbal extracts have not unequivocally been confirmed in clinical studies(GlycyrrhizaSaponaria officinalis436J.M.Augustin et al./Phytochemistry72(2011)435–457sp.:reviewed in Asl and Hosseinzadeh,2008;Panax ginseng :re-viewed in Xiang et al.,2008),several of the saponins exhibit phar-macological activities and thus,attract attention as target for drug discovery.For example,several sapogenins (oleanolic acid:Sultana and Ata,2008)and saponins are considered to possess activities such as anti-inflammatory (e.g.Sun et al.,2010b;Tapondjou et al.,2008),anti-cancerogenic (e.g.Musende et al.,2009;further reviewed in Man et al.,2010),anti-bacterial (e.g.De Leo et al.,2006;further reviewed in Saleem et al.,2010),anti-fungal (e.g.Coleman et al.,2010;Zhang et al.,2005)and anti-viral (e.g.Cinatl et al.,2003;Rattanathongkom et al.,2009;Zhao et al.,2008)ef-fects.Saponins are also of interest as valuable adjuvants and the first saponin-based vaccines are introduced commercially (re-viewed in Sun et al.,2009).Present availability of saponins depends on their extractability from plants.Extraction procedures may be effective for saponins that occur in high concentrations in easily cultivable plants,and for applications that do not demand absolute purity.However,a steady supply of sufficient amounts of specific saponins from plants that accumulate mixtures of structurally related compounds is not feasible (Adams et al.,2010).Synthetic chemistry mainly at-tempts to address these issues by chemically linking desired side chains to extracted sapogenins (reviewed in Yu and Sun,2009).However,the availability of the corresponding sapogenins,which may demand sophisticated modifications to derive from more common precursors,still constitutes bottlenecks.Biotechnological production of either complete saponins,or of saponin pathway intermediates that are not readily accessible,may circumvent the limitation of natural saponin availability.Bioengineering of crop plants with improved resistance towards specific pests as well as saponin reduction in other plants for increased food and feed qual-ity (Dixon and Sumner,2003)are additional motivations for eluci-dation of saponin biosynthesis.Substantial efforts have been made to unravel saponin biosyn-thesis in plants.Although a general consensus on the basic orga-nization of pathways and enzyme classes involved in saponin anabolism has been reached,discovery of individual genes in saponin biosynthesis has so far occurred rather sporadically.However,considerable progress has been accomplished since tri-terpenoid saponin biosynthesis was last reviewed (Haralampidis et al.,2002).The aim of this review is to summarize this progress and pro-vide an updated overview on the current knowledge of the enzymes involved in triterpenoid saponin biosynthesis.In addition,general aspects of molecular activities of saponins,with particular focus on their mode of action towards membranes,are outlined.Finally,the phylogeny of known enzymes in saponin pathways is reviewed and conclusions about evolution of saponin biosynthesis and consequences for further gene discovery are discussed.2.Structural aspects and nomenclatureThe commonly used nomenclature for saponins distinguishes between triterpenoid (also:triterpene)and steroidal saponins(Fig.2).This differentiation is based on the structure and biochem-ical background of their aglycones.Both sapogenin types are thought to derive from 2,3-oxidosqualene,a central metabolite in sterol biosynthesis.In phytosterol anabolism,2,3-oxidosqualene is mainly cyclized into cycloartenol.Triterpenoid sapogenins branch off the phytosterol pathway by alternative cyclization of 2,3-oxidosqualene,while steroidal sapogenins are thought to de-rive from intermediates in the phytosterol pathway downstream of cycloartenol formation (Haralampidis et al.,2002;Kalinowska et al.,2005,Fig.7).A more detailed classification of saponins based on sapogenin structure with 11main classes and 16subclasses has been proposed by Vincken et al.(2007).Steroidal glycoalkaloids (Fig.3)are sometimes considered a third type of saponins (Haralampidis et al.,2002;Hostettmann and Marston,1995;Kalinowska et al.,2005).This may be tempting as the structural characteristics suggest a similar biosynthetic ori-gin and biological activities as proposed for steroidal saponins.However,since neither the biosynthesis of steroidal saponins nor steroidal glycoalkaloids has been fully clarified,a common biosyn-thetic background cannot be confirmed.From a structural point of view,Vincken et al.(2007)suggested to omit steroidal glycoalka-loids from the class of saponins due to the occurrence of the char-acteristic nitrogen atom in the aglycone backbone.In this review,molecular activities of steroidal glycoalkaloids towards mem-branes are discussed together with saponins even though the alter-able protonation state of the nitrogen enhances the effect of pH on the biological activities of steroidal glycoalkaloids as compared to triterpenoid and steroidal saponins.Saponins are also classified based on the number of attached saccharide side chains.Most known saponins are monodesmosidic saponins which means that only one position of the aglycone is glycosylated (Fig.4A).In most monodesmosidic saponins this sac-charide chain is attached by an ether linkage to the C3hydroxy group present in the majority of sapogenins (Vincken et al.,2007).These saccharide side groups can be branched and in most cases comprise 2–5monosaccharide units,which may increase up to 11units (Hostettmann and Marston,1995).Saponins with two saccharide chains are designated bidesmosidic (Fig.4B).In tri-terpenoid saponins such second chains are most often attached by an ester linkage to the C28carboxy group.Finally,in the rather rare tridesmosidic saponins,three saccharide chains are attached to the aglycone at different positions (Schwarz,2000;Vincken et al.,2007).J.M.Augustin et al./Phytochemistry 72(2011)435–457437In addition to sugars,other substituents such as small aliphatic and aromatic acids,monoterpenoidal derived compounds and acyl groups are occasionally attached to sapogenins(see Vincken et al., 2007for more details).3.Molecular activities on membranesPlant-derived saponins are mainly considered to be part of plant defense systems against pathogens and herbivores.Numerous re-ports emphasize the fungicidal(Lee et al.,2001;Morrissey and Osbourn,1999;Saha et al.,2010;Sung and Lee,2008a),anti-micro-bial(Avato et al.,2006;Sung and Lee,2008b),allelopathic(Waller et al.,1993),insecticidal(Sandermann and Funke,1970;Shinoda et al.,2002;Kuzina et al.,2009;Nielsen et al.,2010a)and mollus-cicidal(Aladesanmi,2007;Gopalsamy et al.,1990;Hostettmann, 1980;Huang et al.,2003)activity of various saponins.However, the molecular mechanisms behind these deterrent and toxic effects are not completely elucidated.The most intensively studied effect of several saponins and many steroidal glycoalkaloids is their ability to cause membrane perturbation.This property is often referred to as the‘hemolytic activity of saponins’,as it is thought to be the molecular basis for the often observed ability of saponins to cause lysis of mammalian erythrocytes(Baumann et al.,2000;Chwalek et al.,2006;Seeman et al.,1973).This effect towards membranes wasfirst described by Dourmashkin et al.(1962)in their study on saponin-inactivated Rous sarcoma virus particles by electron microscopy.The observed formation of pores(or pits)in the viral membrane coat as a conse-quence of the saponin treatment inspired Bangham and Horne (1962)and Glauert et al.(1962)to pursue additional experiments in this direction.Both groups concurrently reported the occurrence of cholesterol in the target membrane to be essential for saponin-induced pore formation.Since then,several research groups further elucidated the molecular basis behind the membrane pen-etration activity by studying various sets of saponins or steroidal glycoalkaloids on natural occurring or artificial membrane sys-tems.The results of these studies confirmed the influence of membrane composition,especially in regards to concentration lev-els as well as the structure of incorporated membrane sterols,on the ability of saponins and steroidal glycoalkaloids to cause mem-brane perturbation(Armah et al.,1999;Gögelein and Hüby,1984; Keukens et al.,1992,1995;Nishikawa et al.,1984;Rosenqvist et al.,1980;Steel and Drysdale,1988;Stine et al.,2006;Walker et al.,2008;Yu and Jo,1984).In addition,it became obvious that the overall hemolytic potential of saponins and steroidal glycoalka-loids is affected by virtually all characteristics of their chemical composition,including structure of the aglycone(Gauthier et al., 2009a,b;Oda et al.,2000;Takechi et al.,2003;Ullah et al.,2000; Voutquenne et al.,2002;Yu and Jo,1984),number of saccharide side chains(Hase et al.,1981;Hu et al.,1996;Voutquenne et al., 2002;Woldemichael and Wink,2001),length of these side chains (Armah et al.,1999;Chwalek et al.,2006;Keukens et al.,1995; Nishikawa et al.,1984;Oda et al.,2000;Pléet al.,2004;Seebacher et al.,1999b;Voutquenne et al.,2002)as well as the types and link-age variants of the incorporated sugar units(Chwalek et al.,2006; Hu et al.,1996;Seebacher et al.,1999a,b,2000;Takechi et al., 2003).Combinatory effects when applying mixtures of different steroidal glycoalkaloids or saponins were also reported by Keukens et al.(1995),Smith et al.(2001and references herein)and Voutquenne et al.(2002).However,due to the high number of structural characteristics found to modulate the hemolytic activity, the diversity in experimental setups and the partially conflicting conclusions,an exhaustive relationship between saponin structure and their membrane perturbation activity has not been estab-lished.Consequently,the predictability of the hemolytic potential of uncharacterized saponins often remains low.Based on their observations,Glauert et al.(1962)proposed the first model of saponin action towards membranes.According to this model,spontaneous formation of complexes between sapo-nins and cholesterol in membranes is followed by association of these complexes into‘two-dimensional micellar-type structures’within the membrane.The hydrophilic sugar chains of the sapo-nins,which are thought to be centrally orientated in the micel-lar-like complex,lead to formation of an aqueous pore.Such pores would cause an increase in membrane permeability enabling438J.M.Augustin et al./Phytochemistry72(2011)435–457ions and macromolecules up to proteins to pass the membrane bi-layer.More recent models of the molecular mechanism behind the membrane permeabilizing activity of saponins and steroidal gly-coalkaloids(Armah et al.,1999;Keukens et al.,1995;Nishikawa et al.,1984;Fig.5)expand this initial hypothesis.Thefirst step in these modes of action is the incorporation of saponins or steroi-dal glycoalkaloids into the facing membrane monolayer.This step occurs spontaneously,driven by the lipophilic character of the aglycone as it is expected to favor the hydrophobic conditions of membrane layers over aqueous surroundings.Within the mem-branes,saponins and steroidal glycoalkaloids then assemble into 1:1-complexes with membrane sterols as,e.g.,cholesterol in mam-malian cell membranes.Subsequently,the saponin–sterol or gly-coalkaloid–sterol complexes accumulate into matrices or plaques by processes whose driving force is not currently understood. Armah et al.(1999)and Keukens et al.(1995)proposed interac-tions between the sugar moieties of the incorporated saponins/ glycoalkaloids to cause these phase-separation phenomena.Final-ly,as a consequence of such accumulation,the sterical properties of saponins and steroidal glycoalkaloids may cause membrane cur-vature as afinal step in the membrane perturbation mechanism. This curvature could result in the formation of either pores(Armah et al.,1999;Fig.5A)within these saponin/sterol plaques,or hemi-tubular protuberances that may eventually lead to sterol extrac-tion via vesiculation(Keukens et al.,1995;Fig.5B).The observation of pore-like structures and holes in electron micros-copy images of membranes and cells treated with saponins (Bangham and Horne,1962;Baumann et al.,2000;Dourmashkin et al.,1962;Glauert et al.,1962;Mazzucchelli et al.,2008;Seeman et al.,1973)supports the concept of pore formation.In addition, formation of pores may provide the simplest explanation for the observed changes in ion conductivity(e.g.Armah et al.,1999; Gögelein and Hüby,1984)and protein mobility through the mem-brane(e.g.Krawczyk et al.,2010;Mazzucchelli et al.,2008).How-ever,microscopy studies based on membranes treated with steroidal glycoalkaloids did not reveal similar pores(Keukens et al.,1992,1995;Stine et al.,2006;Walker et al.,2008).Absence of pore-resembling structures was also reported by DourmashkinJ.M.Augustin et al./Phytochemistry72(2011)435–457439et al.(1962)when membranes were treated with the steroidal saponin digitonin instead of the saponin extract previously found to cause pore formation.Moreover,pretreatment with digitonin in this study appeared to even prevent formation of pores during subsequent exposure to pore-inducing saponins.Further studies on the activity of digitonin towards membranes(Elias et al., 1978;Miller,1984)reported hemitubular alterations similar to the observation made by Keukens et al.(1992,1995)for glycoal-kaloids.Finally,computational simulations based on the steroidal saponin dioscin(Lin and Wang,2010)also suggest membrane curvature,resembling the formation of hemitubular alterations, as a consequence of increasing accumulation of saponin mole-cules in confined areas of the membrane.Pore formation and tubular alteration/vesiculation may therefore exist in parallel, and the chemical properties of the saponin or glycoalkaloid determine which perturbation type predominates.Such diversity in the ability to cause membrane permeabilization by different saponins was recently demonstrated by Krawczyk et al.(2010). In this study,digitonin only permeabilized plasma membranes, while a saponin extract from Q.saponaria,which accumulates a vast array of diverse triterpenoid saponins(Fig.1),caused addi-tional perturbation of intra-cellular membrane systems.An alternative model of saponin activity towards membranes originates from coarse-grained molecular dynamics simulations (Lin and Wang,2010;Fig.5C).In this model,saponins migrate in the membrane to sphingomyelin and cholesterol enriched mem-brane domains,the so-called lipid rafts(Brown and London, 2000;Fantini et al.,2002),and complex with the cholesterols therein.Such saponin interaction with specific membrane domains is supported by recent studies on saponin-induced lipid raft dis-ruption(Xu et al.,2009;Yi et al.,2009;Zhuang et al.,2002)and microdomain alteration(Naruse et al.,2010).Consequently,the earlier indicated plaque formation may originate from complex formation following preexisting phase separation rather than resulting from membrane reorganization processes triggered by saponin–sterol complex accumulation.There is a general lack of knowledge on intracellular transport and subcellular storage of saponins and steroidal glycoalkaloids as well as how plants prevent disruption of endogenous mem-branes.Cell fractionation studies by Kesselmeier and Urban (1983)and Urban et al.(1983)on steroidal avenacosides from areal tissues of oat(Avena spp.),and confocal microscopy by Mylona et al.(2008)on oat root triterpenoid avenacins,suggest that sapo-nins are mainly stored in vacuoles.That steriodal glycoalkaloids in tomato(Solanum lycopersicum)and potato(Solanum tuberosum) mainly occur in the soluble phase after cell fractionation further indicates that steroidal glycoalkaloids are stored in a solubilized form rather than by incorporation into host cell membranes (Roddick,1977).Plants have developed different strategies to pro-tect themselves against their own saponins or steroidal glycoalka-loids.An example of this is that avenacosides are known to be stored in an inactive bidesmosidic form,and are transformed into the biologically active monodesmosides by specific b-glucosidases upon tissue disruption(reviewed in Morant et al.,2008).A similar mechanism has been described for bidesmosidic saponins in P.dodecandra berries(Parkhurst et al.,1989).However,most sapo-nins are presumably stored in biologically active forms and little is known about how plants protect the integrity of their cell mem-branes against them.Steel and Drysdale(1988)proposed that the increased resistance of steroidal glycoalkaloid-producing plants to-wards their own defense compounds results from reduced amounts of sterols in cell membranes or their substitution with sterol derivatives with reduced affinity to steroidal glycoalkaloids. Consistent with this,recently reported resistance of membranes of the amoeba Dictyostelium discoideum towards permeabilization with Q.saponaria saponin extracts(Mercanti and Cosson,2010)has been suggested to be due to their plant-resembling sterol composition.Although interference with the integrity of biological mem-branes is probably the most abundant effect of saponins,their activities are not limited to this type of action,and not all saponins show significant hemolytic activity(e.g.Chwalek et al.,2006; Gauthier et al.,2009a,b;Nakamura et al.,1979).Several struc-ture–activity relation studies further emphasized that the ability to cause hemolysis often does not correlate with other known activities of saponins such as their anti-fungal and cytotoxic prop-erties or their applicability as adjuvants(Adams et al.,2010; Chwalek et al.,2006;Gauthier et al.,2009a,b;Oda et al.,2000; Santos et al.,1997;Takechi et al.,2003).Induction of cytotoxicity may in some cases also involve disruption of lipid rafts(Xu et al., 2009;Yi et al.,2009).In addition,some saponins have been shown to influence the properties of different types of membrane proteins such as Ca2+channels and Na+–K+ATPases(Chen et al.,2009;Choi et al.,2001;Haruna et al.,1995;Takechi et al.,2003).However,it is not yet clear,whether these effects are caused by agonistic or antagonistic binding to effector sites and changes in protein topol-ogy or as a result from changes influidity of the surrounding mem-brane layer.Another intriguing mode of action for some saponins is the ability to bind to glucocorticoid receptors.Glucocorticoids rep-resent a class of steroidal hormones known to be involved in reg-ulation of a manifold of physiological processes.In mammals, they play an important role in regulation of development,metab-olism,neurobiology and apoptosis,etc.(reviewed in Yudt and Cidlowski,2002).Due to the structural resemblance of saponin aglycones and steroids,it is therefore not surprising that several pharmacological activities of saponins such as anti-inflammatory (Giner et al.,2000)and neuroprotective(Zhang et al.,2008)effects as well as induction of adipogenesis(Niu et al.,2009)or apoptosis (Jia et al.,2004),are linked to interaction with receptors of gluco-corticoid hormones.An intriguing reversion of steroidal glycoalkaloid based plant defense mechanisms by plant pathogens has been reported for a-tomatine from S.lycopersicum(tomato).Thus,fungal tomato pests such as Septoria lycopersici and Fusarium oxysporum have been found to produce extracellular enzymes that hydrolyze different glycosidic bonds within the saccharide chain of a-tomatine.Sur-prisingly,the hydrolyzed products not only exhibit reduced anti-fungal activity but also cause suppression of induced plant defense mechanisms such as hypersensitive response and oxidative burst (Bouarab et al.,2002;Ito et al.,2004).Yet,the mechanism behind this phenomenon is unclear and similar effects have not been re-ported for other steroidal glycoalkaloids or saponins.Taken together,saponin activities are as multifarious as their chemical compositions,and solely based on structure their activi-ties are often unpredictable.As many saponin activities and in par-ticular the ability to cause membrane permeabilization,are considered to depend on their three-dimensional shape,computa-tional modeling combined with experimental observations may provide a key to gain further insights into the corresponding molecular backgrounds.4.Biosynthesis of triterpenoid saponins4.1.From acetyl-CoA to2,3-oxidosqualene–common biosynthetic origin with phytosterolsCurrent ideas of saponin biosynthesis in plants,consider them to be derived from metabolites of phytosterol anabolism.This assump-tion is supported by the reported concurrent upregulation of sterols and saponins in ginseng plants(P.ginseng)over-expressing squalene synthase(Lee et al.,2004),an enzyme catalyzing a step440J.M.Augustin et al./Phytochemistry72(2011)435–457。
数据结构与算法模拟题2
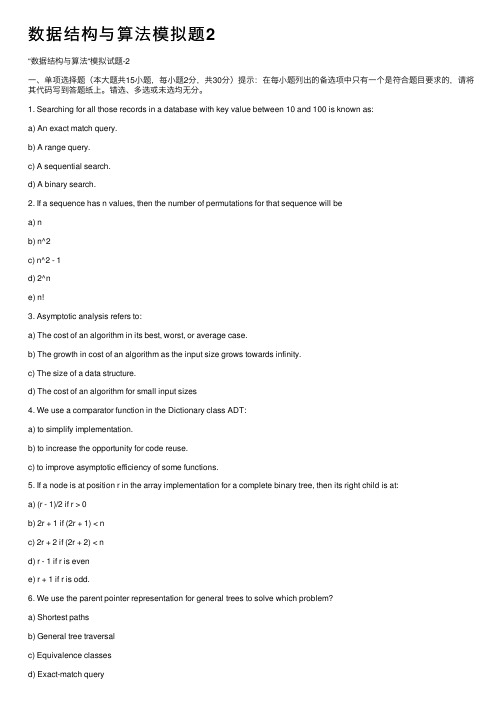
数据结构与算法模拟题2“数据结构与算法“模拟试题-2⼀、单项选择题(本⼤题共15⼩题,每⼩题2分,共30分)提⽰:在每⼩题列出的备选项中只有⼀个是符合题⽬要求的,请将其代码写到答题纸上。
错选、多选或未选均⽆分。
1. Searching for all those records in a database with key value between 10 and 100 is known as:a) An exact match query.b) A range query.c) A sequential search.d) A binary search.2. If a sequence has n values, then the number of permutations for that sequence will bea) nb) n^2c) n^2 - 1d) 2^ne) n!3. Asymptotic analysis refers to:a) The cost of an algorithm in its best, worst, or average case.b) The growth in cost of an algorithm as the input size grows towards infinity.c) The size of a data structure.d) The cost of an algorithm for small input sizes4. We use a comparator function in the Dictionary class ADT:a) to simplify implementation.b) to increase the opportunity for code reuse.c) to improve asymptotic efficiency of some functions.5. If a node is at position r in the array implementation for a complete binary tree, then its right child is at:a) (r - 1)/2 if r > 0b) 2r + 1 if (2r + 1) < nc) 2r + 2 if (2r + 2) < nd) r - 1 if r is evene) r + 1 if r is odd.6. We use the parent pointer representation for general trees to solve which problem?a) Shortest pathsb) General tree traversalc) Equivalence classesd) Exact-match query7. When sorting n records, Selection sort will perform how many swaps in the worst case?a) O(log n).b) O(n).c) O(n log n).d) O(n^2)e) O(n!)f) None of the above.8. The basic unit of I/O when accessing a disk drive is:a) A byte.b) A sector.c) A cluster.d) A track.e) An extent.9. When properly implemented, which search method is generally the most efficient for exact-match queries?a) Sequential search.b) Binary search.c) Dictionary search.d) Search in self-organizing listse) Hashing10. The primary difference between a B+-tree and a B*-tree is:a) The B+-tree store records only at the leaf nodes.b) The B+-tree has a higher branching factor.c) The B+-tree is hight balanced.d) The B+-tree is smaller.11. Dijkstra's algorithm requires that vertices be visited in:a) Depth-first order.b) Breadth-first order.c) Order of distance from the source vertex.d) No particular order.12. If R is a binary relation over set S, then R is transitive ifa) aRa for all a in S.b) whenever aRb, then bRa, for all a, b in S.c) whenever aRb and bRa, then a = b, for all a, b in S.d) whenever aRb and aRc, then aRc, for all a, b, c in S.13. When we wish to describe the upper bound for a problem we use:a) The upper bound of the best algorithm we know.b) The lower bound of the best algorithm we know.c) We can't talk about the upper bound of a problem because there can always be an arbitrarily slow algorithm.14. A full binary tree is one in which:a) Every internal node has two non-empty children.b) all of the levels, except possibly the bottom level, are filled.15. An entry-sequenced file stores records sorted by:a) Primary key value.b) Secondary key value.c) Order of arrival.d) Frequency of access.⼆、名词解释题(本⼤题共3⼩题,每⼩题4分,共12分)提⽰:对题⽬名词进⾏解释,英⽂缩写的需要给出全称并解释。
asymptomatic数学

asymptomatic数学摘要:一、引言1.概述asymptomatic 的概念2.强调asymptomatic 在数学领域的重要性二、asymptomatic 的数学定义1.定义asymptomatic 函数2.介绍渐进式和无穷级数3.讲解asymptomatic 收敛的概念三、asymptomatic 在数学领域中的应用1.分析连续函数的性质2.研究级数的收敛性3.解决微积分中的极限问题四、举例说明asymptomatic 的应用1.泰勒级数的例子2.洛必达法则的例子3.利用asymptomatic 求解极限的例子五、总结1.重申asymptomatic 在数学中的重要性2.展望asymptomatic 在未来数学研究的发展趋势正文:在数学领域,asymptomatic 是一个重要的概念,它涉及到函数、级数收敛以及极限等问题。
本文将首先介绍asymptomatic 的数学定义,然后重点讲述它在数学领域中的应用,并通过实例详细说明如何利用asymptomatic 来解决问题。
首先,我们需要了解asymptomatic 的数学定义。
在数学中,asymptomatic 通常用于描述一种渐进式,可以表示为:f(x) ≈ f(a) + f"(a)(x - a) + ...+ R_n(x)其中,f(x) 是一个函数,f"(a) 是f(x) 在点a 处的导数,R_n(x) 是余项,表示f(x) 与f(a) 之间的误差。
当x 趋近于a 时,f(x) 接近于f(a),这就是asymptomatic 的概念。
此外,asymptomatic 还与无穷级数有关。
一个级数如果满足某种条件,那么我们可以用asymptomatic 收敛来描述它。
例如,如果一个级数满足以下两个条件:1.各项绝对值单调递减2.极限为零那么我们可以说这个级数是asymptomatic 收敛的。
在数学领域,asymptomatic 有着广泛的应用。
免疫算法介绍
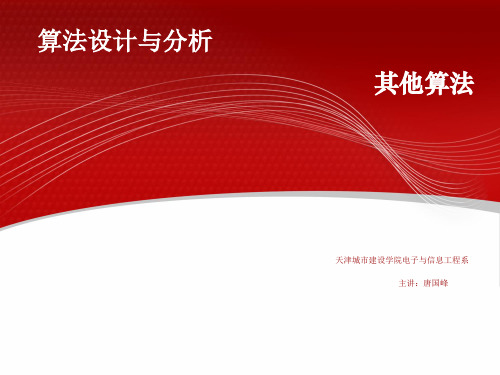
该过程重复进行,直到所需数量的监测器被产生出来 。通常用概率分析方法来估算为了满足一定的可靠性 所应有的监测器的数目。
9
免疫算法 免疫识别过程同时也是一个学习的过程,学习的结果 是免疫细胞的个体亲和度提高、群体规模扩大,并且 最优个体以免疫记忆的形式得到保存。 当机体重复遇到同一抗原时,由于免疫记忆机制的作 用,免疫系统对该抗原的应答速度大大提高,并且产 生高亲和度的抗体去除病原,这个过程是一个增强式 学习过程。而且可以对结构类似的抗原进行识别。
约束搜索优化问题和多判据设计问题;
32
扩展:人工免疫系统
AIS在网络安全的应用
数据检测(Forrest );
病毒检测( Kephart);
UNIX过程监控( Forrest)。
33
扩展:人工免疫系统
国际研究新动向之一
以开发新型的智能系统方法为背景,研究基于生物免 疫系统机理的智能系统理论和技术,同时将AIS与模糊 系统、神经网络和遗传算法等软计算技术进行集成, 并给出其应用方法。
免疫算法
Procedure
阴性选择算法
Begin
随机生成大量的候选检测器(即免疫细胞) /*初始化*/ While一个给定大小的检测器集合还没有被产生do/*耐受*/
Begin
计算出每一个自体元素和一个候选检测器之间的亲和力; If这个候选的检测器识别出了自体集合中的任何一个元素 Then这个检测器就要被消除掉; Else把这个检测器放入检测器集合里面; /*该检测器成熟*/ 利用经过耐受的检测器集合,检测系统以找出变种;
7
免疫算法
(1)定义自己(self)为一个字符串集合S,每个字符 串由n个字母组成,字符串可以是一个网络数据包 ,电子邮件特征向量或程序的一般行为模式。 (2)产生一个初始监测器集合R。
- 1、下载文档前请自行甄别文档内容的完整性,平台不提供额外的编辑、内容补充、找答案等附加服务。
- 2、"仅部分预览"的文档,不可在线预览部分如存在完整性等问题,可反馈申请退款(可完整预览的文档不适用该条件!)。
- 3、如文档侵犯您的权益,请联系客服反馈,我们会尽快为您处理(人工客服工作时间:9:00-18:30)。
≤ a log n.
(1.1)
M (m, s; n, t) =
(n−1)/2
1 + exp − 2
∆(m, s; n, t) . m+n
Then ∆(m, s; n, t) = O (n−b)(m + n) as m, n → ∞. Canfield and McKay conjectured that in fact 0 < ∆(m, s; n, t) < 2 for all s, t ≥ 1. The results in the present paper establish that conjecture for sufficiently large m, n in the case st = o (mn)1/5 . (See Corollary 4.2.) 2
School of Mathematics and Statistics The University of New South Wales Sydney NSW 2052, Australia csg@.au
Brendan D. McKay
Department of Computer Science Australian National University Canberra, ACT 0200, Australia bdm@.au
Asymptotic enumeration of sparse nonnegative integer matrices with specified row and column sums
arXiv:0707.0340v2 [math.CO] 14 Feb 2008
Catherine Greenhill
Keywords: asymptotic enumeration, non-negative integer matrices, contingency tables, switchings MSC 2000: 05A16, 05C50, 62H17
Abstract Let s = (s1 , . . . , sm ) and t = (t1 , . . . , tn ) be vectors of nonnegative integer-valued n functions of m, n with equal sum S = m i=1 si = j =1 tj . Let M (s, t) be the number of m × n matrices with nonnegative integer entries such that the ith row has row sum si and the j th column has column sum tj for all i, j . Such matrices occur in many different settings, an important example being the contingency tables (also called frequency tables) important in statistics. Define s = maxi si and t = maxj tj . Previous work has established the asymptotic value of M (s, t) as m, n → ∞ with s and t bounded (various authors independently, 1971–1974), and when all entries of s equal s, all entries of t equal t, and m/n, n/m, s/n ≥ c/ log n for sufficiently large c (Canfield and McKay, 2007). In this paper we extend the sparse range to the case st = o(S 2/3 ). The proof in part follows a previous asymptotic enumeration of 0-1 matrices under the same conditions (Greenhill, McKay and Wang, 2006). We also generalise the enumeration to matrices over any subset of the nonnegative integers that includes 0 and 1.
i=1
[si ]k ,
Tk =
j =1
[tj ]k
for k ≥ 1. Note that S1 = T1 = S . Theorem 1.2 ([11, Corollary 5.1]). Let s = s(m, n) = (s1 , . . . , sm ) and t = t(m, n) = n m (t1 , . . . , tn ) be vectors of nonnegative integers with equal sum S = j =1 tj . i=1 si = 2/3 Suppose that m, n → ∞, S → ∞ and 1 ≤ st = o(S ). Then N (s, t) =
The main result in this paper is the asymptotic value of M (s, t) for st = o(S 2/3 ). Our proof uses the method of switchings in a number of different ways. In several aspects our approach is parallel to that which provided our previous asymptotic estimate of N (s, t), the number of 0-1 matrices in the class M(s, t). We now restate that result for convenience. For any x, define [x]0 = 1 and for a positive integer k , [x]k = x(x − 1) · · · (x − k +1). Also define n m Sk =
1
Introduction
Let s = s(m, n) = (s1 , . . . , sm ) and t = t(m, n) = (t1 , . . . , tn ) be vectors of nonnegative n integers with equal sum S = m j =1 tj . Let M(s, t) be the set of all m × n i=1 si = matrices with nonnegative integer entries such that the ith row has row sum si and the 1
j th column has column sum tj for each i, j . Then define M (s, t) = |M(s, t)| to be the number of such matrices. Our task in this paper is to determine the asymptotic value of M (s, t) as m, n → ∞ under suitable conditions on s and t. The matrices M(s, t) appear in many combinatorial contexts; see Stanley [15, Chapter 1] for a brief history. A large body of statistical literature is devoted to them under the name of contingency tables or frequency tables ; see [6, 7] for a partial survey. In theoretical computer science there has been interest in efficient algorithms for the problem of generating contingency tables with prescribed margins at random, and for approximately counting these tables. See for example [1, 8, 13]. The history of the enumeration problem for nonnegative integer matrices is surveyed in [5], while a history for the corresponding problem for 0-1 matrices is given in [11]. Here we recall only the few previous exact results on asymptotic enumeration for nonnegative integer matrices. Define s = maxi si and t = maxj tj . The first non-trivial case s1 = · · · = sm = t1 = · · · = tn = 3 was solved by Read [14] in 1958. During the period 1971–74, this was generalised to bounded s, t by three independent groups: B´ ek´ essy, B´ ek´ essy and Koml´ os [2], Bender [3], and Everett and Stein [9], under slightly different conditions. In the case of denser matrices, the only precise asymptotics were found by Canfield and McKay [5] in the case that the row sums are all the same and the column sums are all the same. Let M (m, s; n, t) = M ((s, s, . . . , s), (t, t, . . . , t)), where the vectors have length m, n, respectively, and ms = nt. Theorem 1.1 ([5, Theorem 1]). Let s = s(m, n), t = t(m, n) be positive integers satisfying 1 ms = nt. Define λ = s/n = t/m. Let a, b > 0 be constants such that a + b < 2 . Suppose that m, n → ∞ in such a way that 5m 5n (1 + 2λ)2 1+ + 4λ(1 + λ) 6n 6m Define ∆(m, s; n, t) by m+t−1 n+s−1 t s mn + λmn − 1 λmn m + 1 (m−1)/2 n + 1 × m n