北京大学实证金融学讲义 7 volatility
金融学专业名词解析
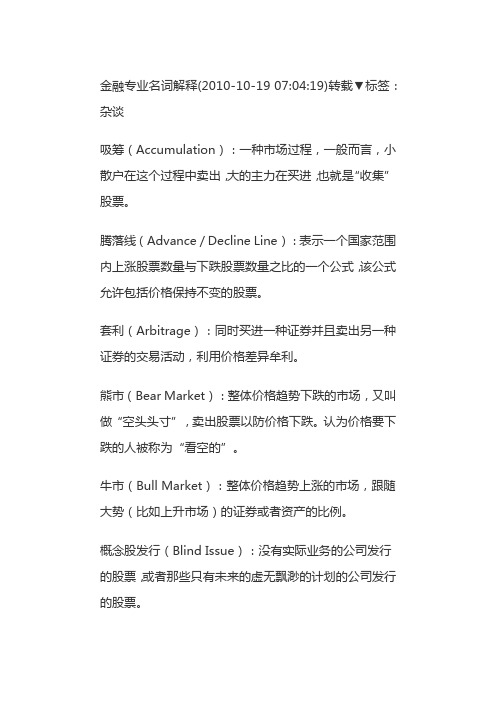
金融专业名词解释(2010-10-19 07:04:19)转载▼标签:杂谈吸筹(Accumulation):一种市场过程,一般而言,小散户在这个过程中卖出,大的主力在买进,也就是“收集”股票。
腾落线(Advance / Decline Line):表示一个国家范围内上涨股票数量与下跌股票数量之比的一个公式,该公式允许包括价格保持不变的股票。
套利(Arbitrage):同时买进一种证券并且卖出另一种证券的交易活动,利用价格差异牟利。
熊市(Bear Market):整体价格趋势下跌的市场,又叫做“空头头寸”,卖出股票以防价格下跌。
认为价格要下跌的人被称为“看空的”。
牛市(Bull Market):整体价格趋势上涨的市场,跟随大势(比如上升市场)的证券或者资产的比例。
概念股发行(Blind Issue):没有实际业务的公司发行的股票,或者那些只有未来的虚无飘渺的计划的公司发行的股票。
泡沫头部(Blow-off Top):主要的牛市的头部,由股价的加速上行所形成的。
面值(Book Value):表示每股资本的净资产大小,由股价的加速上升所形成。
交易所(Bourse):进行证券(股票交易)、商品、贵金属和外汇交易的市场。
世界上最早的交易所是16世纪的荷兰的范德伯尔斯家族位于布鲁日的房子。
广度(Breadth):在某个具体的金融市场,跟随大势(比如上升或者下降市场)的证券或者资产的比例。
突破(Breakout):价格变化突破某个成交密集区域。
发散形态(Broadening Formation):一种技术形态,价格在越来越宽广的范围内震荡。
经纪人(Broker):收取佣金、代人买卖证券的自然人或者公司。
蝴蝶效应(Butterfly Effect):系统对初始条件的极端敏感性。
正向套利(Cash and Carry):同时买进某种证券、卖出其期货(或者进行相反的操作),利用价格差异牟取利润的交易行为。
收盘价(Close Price):交易所(或者外汇市场)的收盘价。
2017北大经院金融专业考研辅导讲义
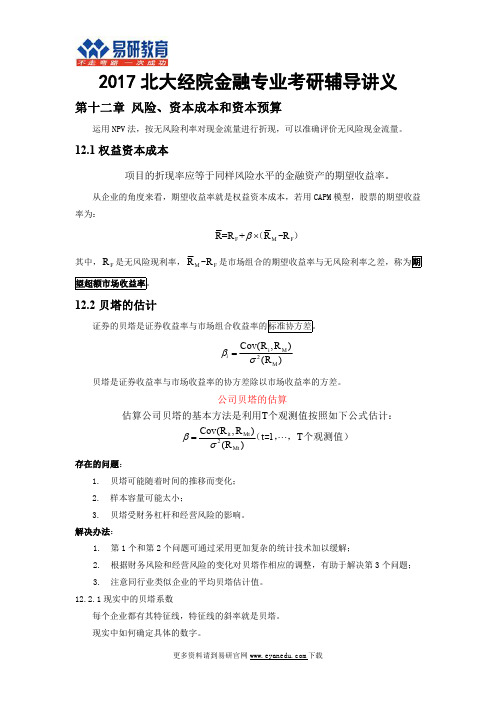
有效市场 过度反映后修正 延迟反映
13.3 有效资本市场的类型
13.3.1 弱型有效市场 如果某一资本市场上证券的价格充分地包含和反映了其历史价格的信息,那么,资本 市场就达到了“弱型有效”,或者说满足“弱型有效假说”。 弱型效率(公式): Pt Pt 1 期望收益 随机误差t 弱型效率是资本市场所能表现出来的最低形本估计 12.6 降低资本成本
期望收益和资本成本均与流动性负相关。 12.6.1 流动性 1. 佣金 2. 买卖价差——专营商 3. 市场引致的成本——有大额销售导致的价格下跌和由大额购买导致的价格上升称 为市场引致的成本。 12.6.2 流动性、期望收益和资本成本 流动性较差的股票的交易费用会降低投资者获得的总报酬。 12.6.3 流动性和逆向选择 股票交易中的知情者将提升权益的必要报酬率,从而提升资本成本。 12.6.4 公司能做什么? 公司有动机降低交易成本以降低资本成本。两种策略: 1. 试图引进更多的非知情的投资者——如股票拆细; 2. 公司可以披露更多的信息——这缩小了知情者与不知情者之间的距离。 研究表明,当公司发布这些预测时,公司股票的买卖价差缩小。
公司贝塔的估算 估算公司贝塔的基本方法是利用T个观测值按照如下公式估计: Cov(R it , R Mt ) (t =1, ,T 个观测值) 2 (R Mt )
存在的问题: 1. 2. 3.
贝塔可能随着时间的推移而变化; 样本容量可能太小; 贝塔受财务杠杆和经营风险的影响。
解决办法: 1. 第 1 个和第 2 个问题可通过采用更加复杂的统计技术加以缓解; 2. 根据财务风险和经营风险的变化对贝塔作相应的调整,有助于解决第 3 个问题; 3. 注意同行业类似企业的平均贝塔估计值。 12.2.1 现实中的贝塔系数 每个企业都有其特征线,特征线的斜率就是贝塔。 现实中如何确定具体的数字。
北大经济金融课件_本科生证券投资学讲义(光华)_证券投资学第10章
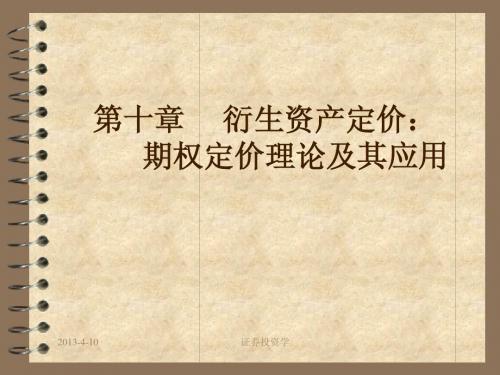
2012-8-20
证券投资学
期权定价的技巧被广泛的应用到许多金融领域
和非金融领域,包括各种衍生证券定价、公司 投资决策等。 学术领域内的巨大进步带来了实际领域的飞速 发展。期权定价的技巧对产生全球化的金融产 品和金融市场起着最基本的作用。 近年来,从事金融产品的创造及定价的行业蓬 勃发展,从而使得期权定价理论得到不断的改 进和拓展。
证券投资学
2012-8-20
p
K
2012-8-20 证券投资学 • 图2 看跌期权在到期日的收益
ST
注意,看跌期权在 T 时的价值是有界的,
而看涨期权在 T 时的价格是无界的。相 反,当写一份看涨期权时,可能的损失 是无界的。
ห้องสมุดไป่ตู้
2012-8-20
证券投资学
期权的写者的收益
– 看涨期权的写者在到期日的收益
既然期权的持有者获得的是权利而不需
要承担什么义务,他就必须花钱购买这 个权利,那么,公平的价格应该是多少? 这是证券投资学研究的重要内容。
2012-8-20
证券投资学
2 影响欧式期权价格的因素
本章的主要目的:如何确定以金融证券
为标的物的欧式期权的价格。 在整个一章中假设:如果无特殊说明, 标的物在到期日以前不支付红利。
• 如果在到期日之前的任何时间以及到期日都能执行,我们称这种 期权为美式期权。如果只能在到期日执行,称为欧式期权。 • 美式和欧式这两个名词曾代表了以股票为标的物的期权在美洲和 欧洲的结构形式。但是现在,它们已成为反映两种不同结构的期 权的标准名词,而不管期权是在哪儿发行的。
2012-8-20
证券投资学
第七章 期权市场与期权定价
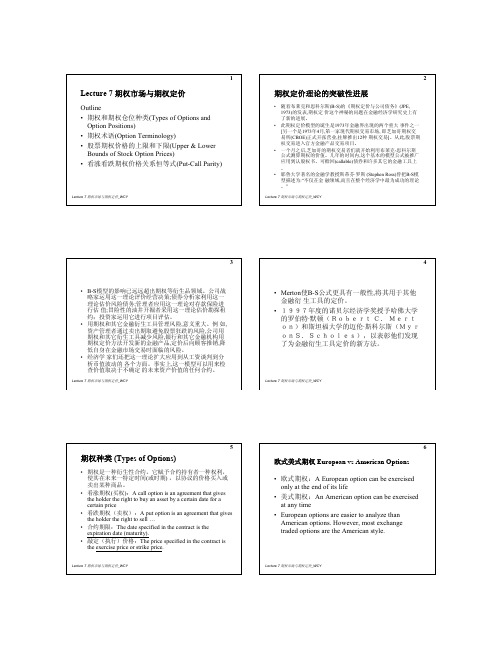
2
期权定价理论的突破性进展
• 随着布莱克和思科尔斯(B-S)的《期权定价与公司债务》(JPE, 1973)的发表,期权定 价这个神秘的问题在金融经济学研究史上有 了新的进展。
• 此期权定价模型的诞生是1973年金融界出现的两个重大 事件之一 [另一个是1973年4月,第一家现代期权交易市场, 即芝加哥期权交 易所(CBOE)正式开张营业,挂牌推出12种 期权交易]。从此,股票期 权交易进入官方金融产品交易项目。
flows result (S0 >X for a call, S0 <X for a put)- the option is an in-the-money (价内)option. • Negative moneyness: if an option is exercised, negative cash flows result (S0 <X call, S0 >X for put) – option is out-of-the-money(价外). • If S0 =X, option is at-the-money(价平).
16
货币性(Moneyness)
• Moneyness of an option 是立即执行期权所实现的收入 ( 假定执行期权是可行的).
• Moneyness is S0 –X for a call, X- S0 for a put • Positive moneyness: if an option is exercised, positive cash
• 敲定(执行)价格:The price specified in the contract is the exercise price or strike price.
金融幂律
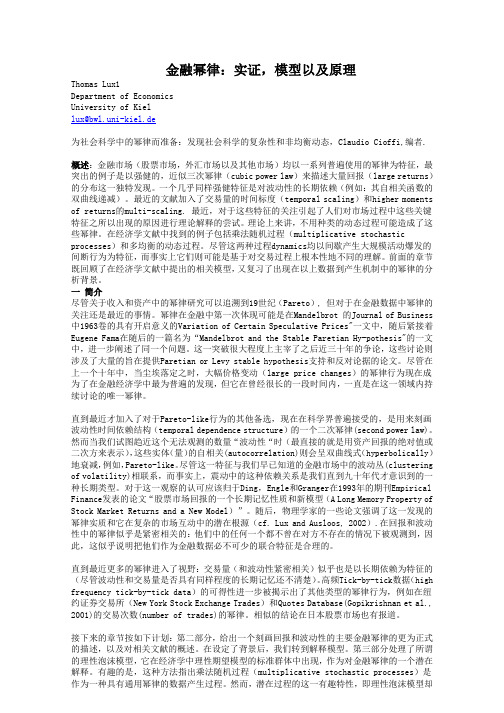
金融幂律:实证,模型以及原理Thomas Lux1Department of EconomicsUniversity of Kiellux@bwl.uni-kiel.de为社会科学中的幂律而准备:发现社会科学的复杂性和非均衡动态,Claudio Cioffi,编者.概述:金融市场(股票市场,外汇市场以及其他市场)均以一系列普遍使用的幂律为特征,最突出的例子是以强健的,近似三次幂律(cubic power law)来描述大量回报(large returns)的分布这一独特发现。
一个几乎同样强健特征是对波动性的长期依赖(例如:其自相关函数的双曲线递减)。
最近的文献加入了交易量的时间标度(temporal scaling)和higher moments of returns的multi-scaling. 最近,对于这些特征的关注引起了人们对市场过程中这些关键特征之所以出现的原因进行理论解释的尝试。
理论上来讲,不用种类的动态过程可能造成了这些幂律。
在经济学文献中找到的例子包括乘法随机过程(multiplicative stochastic processes)和多均衡的动态过程。
尽管这两种过程dynamics均以间歇产生大规模活动爆发的间断行为为特征,而事实上它们则可能是基于对交易过程上根本性地不同的理解。
前面的章节既回顾了在经济学文献中提出的相关模型,又复习了出现在以上数据到产生机制中的幂律的分析背景。
一简介尽管关于收入和资产中的幂律研究可以追溯到19世纪(Pareto), 但对于在金融数据中幂律的关注还是最近的事情。
幂律在金融中第一次体现可能是在Mandelbrot 的Journal of Business 中1963卷的具有开启意义的Variation of Certain Speculative Prices"一文中,随后紧接着Eugene Fama在随后的一篇名为“Mandelbrot and the Stable Paretian Hy-pothesis"的一文中,进一步阐述了同一个问题。
北大金融经济学 (7)
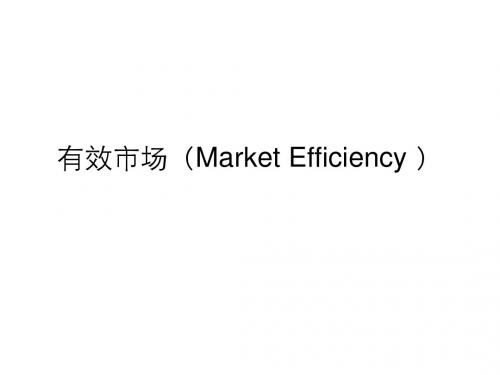
弱式有效检验:经验证据
Park and Irwin (2004): • “Early studies indicated that technical trading strategies were profitable in foreign exchange markets and futures markets, but not in stock markets before the 1980s. Modern studies indicated that technical trading strategies consistently generated economic profits in a variety of speculative markets at least until the early 1990s. Among a total of 92 modern studies, 58 studies found positive results regarding technical trading strategies, while 24 studies obtained negative results. Ten studies indicated mixed results. • Despite the positive evidence on the profitability of technical trading strategies, it appears that most empirical studies are subject to various problems in their testing procedures, e.g., data snooping, ex post selection of trading rules or search technologies, and difficulties in estimation of risk and transaction costs.”
W07_lecture07_面板数据 北大 光华 计量

•
• • •
在小白鼠的药物试验中,如何解决这个问题? – 几乎完全一样的一群小白鼠,一半用作treatment,一半用作control。 – 政策评估过程中,最理想的是treatment group 和control group完全一样。四组 (前后、有无受影响)除了政策影响外,没有任何差别 而我们的实证金融中,没有试验,有的是quasi-experiment,没法选择谁做treatment, 谁作control。 这时除了尽量选择接近的两组样本外,就是用控制变量,即把那些因素加入到回归中 注意,控制变量只能在一定程度上解决这个问题( treatment group 和control group 的差别)。比如 – 房价问题中,两组内部的大小可以有变化,但两组之间的size几乎无交集。 – 这时回归中尽管控制了size,但在回归中size的影响是线性的,但78年到81年大 房子涨价更多,是指正好size的影响在两组之间的size差别之处有突变,即非线 性的 • 那用非线性的size能否控制? • 有共线性问题:非线性正好发生在垃圾场附近与否之间,因此,这个非线性 项与nearinc高度相关。
4
•
一个可能的原因是: – 78年的数据中,垃圾场附近的多为新房子;而81年的数据中,垃圾场附近多为老 房子。这样81年垃圾场附近的房价相对偏低是由于房子抽样导致的。 – 或者其它这样的抽样房子特点的不同:大小、房间个数等等 – 所以在回归模型中,我们要控制这些因素。
– 现在78年抽 • 78年数据抽样的垃圾场附近房子的外观和内部结构分布很好,而81年抽样的 则很差。 • 而外观和内部结构都是“不可观测(衡量)因素”,没法控制。 • 如果我们能保证78年和81年抽样的房子是同一栋房子,就没有这个问题了。
北京大学金融专业考研《计量经济学》辅导讲义4

(三)重点掌握阶段(7 月初——10 月上旬) : 关键词:分清重点、地毯式全面记忆、不断循环巩固、检测督促
这一阶段最重要的任务是抓住重点、掌握重点。要抓住重点,一是要分析真题;二是要 专业化辅导;三是内部资料,如出题老师的论文、讲义、当前学术热点等。在此基础上坚持 专业课复习的 80/20 法则,对核心概念、基础概念、重要知识点、要点、常见公式一定要地 毯式全面记忆,并反复强化,达到永久记忆。提醒广大考生要自我检测或者让专业课辅导老 师及时检测,不断督促,有压力才能保障效果。
这一阶段最重要的任务是:全面的自我分析基础上,定下自己的目标院校和专业,并进 一步明确自己报考专业的参考书目、报考人数、招生人数、复试分数线、该专业必备考研资 料。提醒广大考生:选择院校和专业要综合考虑兴趣、专业课基础、外语水平、未来职业规 划、 报考专业的就业前景等因素。 考研就是给自己一次机会, 无论跨考与否, 报考名校与否, 择校、择专业一定要要建立在全面自我分析的基础上。一旦决定,要抱定信念,切勿轻易中 途换学校、转专业!中途换院校和专业会极大浪费有限的备考时间和精力。
(四)框架专题阶段(10 月上旬——11 月上旬) : 关键词:将知识系统化、体系化,建立知识结构树
这一阶段最重要的任务是将知识体系化,系统化。知识点掌握的零散,不体系化,会造成 只见树木不见森林,思路狭隘,影响答题发挥,尤其是做大题的时候。必须要按照参考书的 章节架构或者通过总结专题将知识体系化,系统化。对参考书做到提纲挈领,纲举目张。总 结了全国各学校专业课的专题和章节联系, 能在这一阶段帮助广大考生建立系统化的知识体
1 高中 D2 0 否则
1 大学及以上 D3 否则 0
三、包含一个定量变量 X, 一个多分定性变量 D 的回归 • 若性质有 m 个类别,应引入 m-1 个虚拟变量 D • 注意区分哪个类别是基底 例:P219 表 10-4 学校教师薪水的地区差异 四、包含一个定量变量 X, 多个定性变量 D 的回归 • 之前:方程中只考虑某一种属性的出现与否,即只有一个 m 分定性变量,需要 m-1 个虚拟变量。 (如性别,2 分,需 1 个 D) • 现在:方程中同时考虑几种属性出现与否,即需要多个 m 分定性变量(如性别、肤 色都考虑) • P221 例子不好,另给一例方便理解 ★ 重要规律: 关于虚拟变量个数的确定
北京大学金融硕士考研博迪《投资学》辅导讲义.
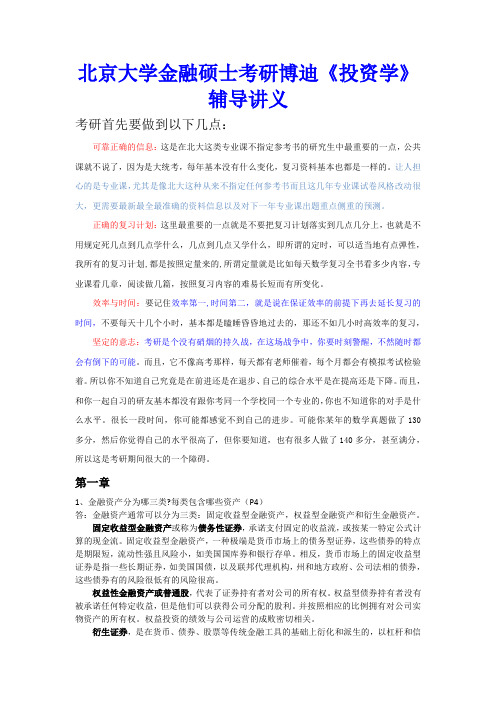
北京大学金融硕士考研博迪《投资学》辅导讲义考研首先要做到以下几点:可靠正确的信息:这是在北大这类专业课不指定参考书的研究生中最重要的一点,公共课就不说了,因为是大统考,每年基本没有什么变化,复习资料基本也都是一样的。
让人担心的是专业课,尤其是像北大这种从来不指定任何参考书而且这几年专业课试卷风格改动很大,更需要最新最全最准确的资料信息以及对下一年专业课出题重点侧重的预测。
正确的复习计划:这里最重要的一点就是不要把复习计划落实到几点几分上,也就是不用规定死几点到几点学什么,几点到几点又学什么,即所谓的定时,可以适当地有点弹性,我所有的复习计划,都是按照定量来的,所谓定量就是比如每天数学复习全书看多少内容,专业课看几章,阅读做几篇,按照复习内容的难易长短而有所变化。
效率与时间:要记住效率第一,时间第二,就是说在保证效率的前提下再去延长复习的时间,不要每天十几个小时,基本都是瞌睡昏昏地过去的,那还不如几小时高效率的复习,坚定的意志:考研是个没有硝烟的持久战,在这场战争中,你要时刻警醒,不然随时都会有倒下的可能。
而且,它不像高考那样,每天都有老师催着,每个月都会有模拟考试检验着。
所以你不知道自己究竟是在前进还是在退步、自己的综合水平是在提高还是下降。
而且,和你一起自习的研友基本都没有跟你考同一个学校同一个专业的,你也不知道你的对手是什么水平。
很长一段时间,你可能都感觉不到自己的进步。
可能你某年的数学真题做了130多分,然后你觉得自己的水平很高了,但你要知道,也有很多人做了140多分,甚至满分,所以这是考研期间很大的一个障碍。
第一章1、金融资产分为哪三类?每类包含哪些资产(P4)答:金融资产通常可以分为三类:固定收益型金融资产,权益型金融资产和衍生金融资产。
固定收益型金融资产或称为债务性证券,承诺支付固定的收益流,或按某一特定公式计算的现金流。
固定收益型金融资产,一种极端是货币市场上的债务型证券,这些债券的特点是期限短,流动性强且风险小,如美国国库券和银行存单。
北大中级微观经济学课件ch7
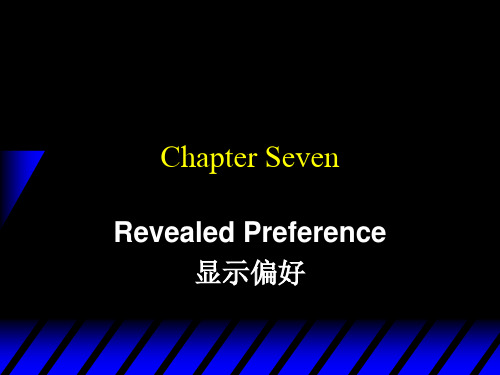
D
p
Indirect Preference Revelation
Suppose x is revealed directly preferred to y, and y is revealed directly preferred to z. Then, by transitivity, x is indirectly revealed preferred to z. Write this as
indirectly) as preferred to the bundle
y and x y, then it is never the case
that the y is revealed (directly or
indirectly) as preferred to x; i.e.
p
p
x bundle x* is chosen when the bundle y is affordable. Then x* is directly revealed preferred to y (otherwise y would have been chosen). x*直接显示偏好于y
Direct Preference Revelation
x2
The chosen bundle x* is
directly revealed preferred
to the bundles y and z.
x*
yz
x1
Direct Preference Revelation
That x is directly revealed preferred to y will be written as
光华管理学院本科生金融计量经济学讲义(1)

1.1 什么是金融计量经济学
当一家金融机构,面临某种制度(例如,引入独立董事制度、融资融券业务的推出,再 融资标准的变化,交易制度的引入或限制某种交易行为等)的引入时,需要考虑引入制度对 公司价值的提升作用或交易环境变化的影响进行相应决策。 假定你在一家投资银行工作, 你 可能需要研究几种不同的交易策略对所准备投资证券的收益影响。 假定你是有志作为一名学 术研究者,你需要验证市场上的各种变量之间的关系(例如长短期利率变化,汇率波动与净 出口贸易, 货币政策的变化对那些类别的资产价格会产生影响等) 来确定它们是否遵从相关 的金融理论和模型假设。 假若你是一个市场中的投资者, 你可能需要根据各种影响证券价值 变化的因素来选择投资的对象和买卖的时机。 要完成上述这些任务中的任意一项, 如果你希 望你的行为和决定是有一定科学依据的, 而不愿意象一个赌徒那样接受随机发生的结果, 你 就需要使用金融计量方法,对各种变量之间的关系通过统计方法进行分析和检验。为此,你 将要面对和使用大量的统计模型和计量方法。 金融计量经济学中将会大量使用统计模型和方法解决金融相关问题, 是不是金融计量就 等同于统计方法呢,尽管二者之间存在许多密不可分的联系,但答案仍然是否定的。为此金 融计量与统计之间的关系如何就是一个首先需要明确的问题。 金融学家最初所使用的方法大 多是基于模型的统计推断方法。然而,金融市场的发展日新月异,很对的金融问题已经没有 统计工具来帮助解决,因此,金融计量经济学是相对独立于数理统计而发展的。另一方面, 数理统计更多的是基于假设和可以重复实验, 而计量金融学主要集中在对不可重复试验数据
y f ( x1, x2 , x3 , x4 , x5 )
的特性只能从所获取的观测数据中去寻找。
(1.2)
模型中的干扰项 所具有的特征及其对结果的影响将是计量分析的重要内容。 而干扰项 为此, 接下来就要去收集相关的数据并通过计量方法进行验证。 因为没有通过验证的任 何理论,在经济金融领域只能算是一个假设或猜想,甚至是一个误认为。在后面的内容中再 详细讨论第二、 三两个步骤所涉及的相关内容。 在此, 我们要先来熟悉一下数据是什么样的, 常见都有那些数据类型?
北京大学实证金融学讲义 4 VAR介绍
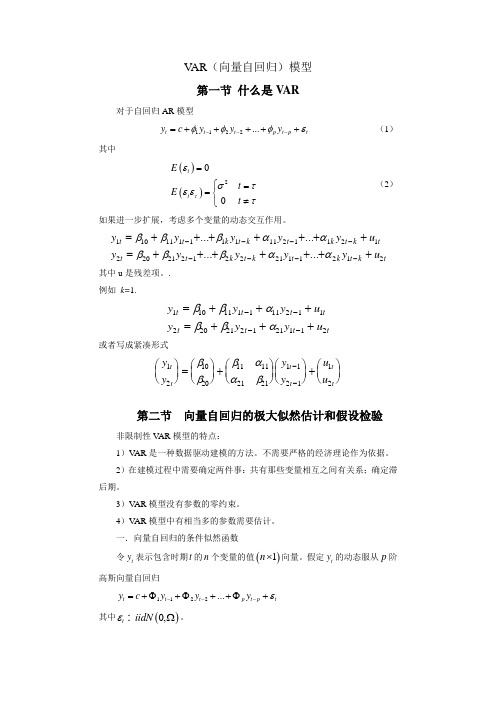
V AR (向量自回归)模型第一节 什么是V AR对于自回归AR 模型1122...t t t p t p t y c y y y φφφε---=+++++ (1)其中()()20t t E t E t τεστεετ=⎧==⎨≠⎩ (2) 如果进一步扩展,考虑多个变量的动态交互作用。
y y y y y u y y y y y u t t k t k t k t k t t t k t k t k t k t 11011111111211212202121222111212=+++++++=+++++++--------βββααβββαα............ 其中u 是残差项。
.例如 k=1.y y y u y y y u t t t tt t t t 110111111211220212121112=+++=+++----ββαββα或者写成紧凑形式y y y y u u t t t t t t 12102011112121112112⎛⎝ ⎫⎭⎪=⎛⎝ ⎫⎭⎪+⎛⎝ ⎫⎭⎪⎛⎝ ⎫⎭⎪+⎛⎝ ⎫⎭⎪--βββααβ第二节 向量自回归的极大似然估计和假设检验 非限制性V AR 模型的特点:1)V AR 是一种数据驱动建模的方法。
不需要严格的经济理论作为依据。
2)在建模过程中需要确定两件事:共有那些变量相互之间有关系;确定滞后期。
3)V AR 模型没有参数的零约束。
4)V AR 模型中有相当多的参数需要估计。
一.向量自回归的条件似然函数令t y 表示包含时期t 的n 个变量的值()1n ⨯向量。
假定t y 的动态服从p 阶高斯向量自回归1122...t t t p t p t y c y y y ε---=+Φ+Φ++Φ+ 其中()0,t iidN εΩ。
假定已经观测到()T P +个时期n 各变量中的每个变量的值。
则以前p 个观测为条件,根据后T 各观察值形成估计,这就是条件似然函数:()110111101,,....,,,....,1,,....,,,....,T T p T T Y Y Y Y Y Y p f y y y y y y ---+---+ 并对θ求最大值,这里θ包含12,,,...,p c ΦΦΦ和Ω种元素的向量。
第七章利率期权(固定收益证券-北大姚长辉)
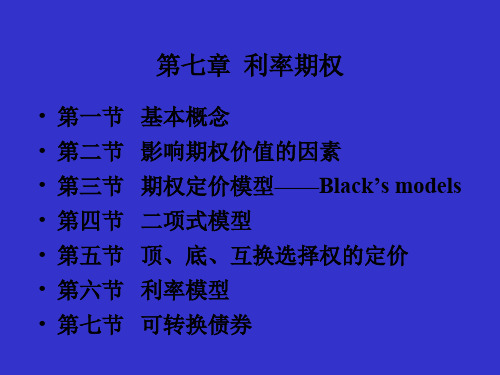
2. 短期利率不变
短期利率变化!
3. 价格波动率不变
债券价格的波动率在接近偿还 期时会降低。
价格波动率:股票与债券
•
股票
•
债券
•
时间
Black's Model
• 尽管存在着以上问题,Black-Scholes 的变形, 叫做Black’s Model, 也还经常被使用,条 件是:
rU=4.976%
V=101.333 C=5.25
rd=4.074%
V=98.588 C=5.25
ruu=6.757%
V=99.732 C=5.25
rud=5.532%
V=100.689 C=5.25
rdd=4.53%
V=100 C=5.25
V=100 C=5.25
V=100 C=5.25
V=100 C=5.25
Short a call
• profit
期权的盈亏
profit
•
Long a put
Short a put
第二节 影响期权价值的因素
因素
标的证券的价格 执行价格 到期时间 利率波动率 短期利率 利息支付
Call 的价格
上升 下降 上升 上升 上升 下降
Put 的价格
下降 上升 上升 上升 下降 上升
9.5%,10个月的无风险利率为10% (以年为基础, 连续利率) – 债券价格的波动率为年9%
例 7.2: 应用 Black's Model
• 求解
• 第一步: 找到远期价格
P0 960 50 e0.09(.25) 50 e0.09(.75) F e0.09(.8333) F 939 .86
idiosyncratic volatility计算 概述及解释说明
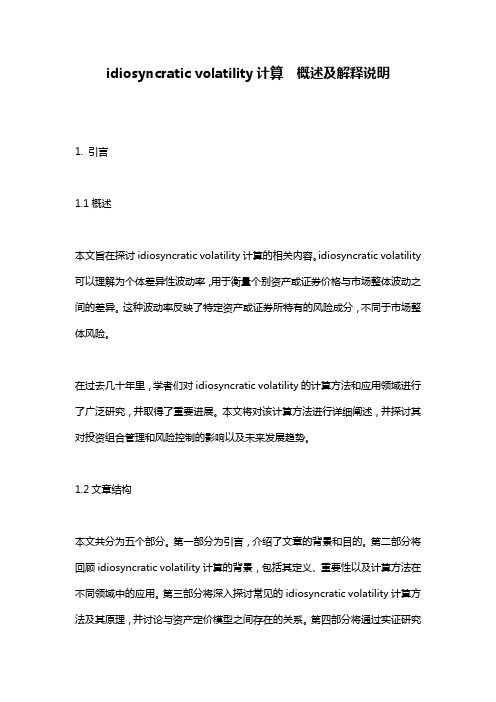
idiosyncratic volatility计算概述及解释说明1. 引言1.1概述本文旨在探讨idiosyncratic volatility计算的相关内容。
idiosyncratic volatility 可以理解为个体差异性波动率,用于衡量个别资产或证券价格与市场整体波动之间的差异。
这种波动率反映了特定资产或证券所特有的风险成分,不同于市场整体风险。
在过去几十年里,学者们对idiosyncratic volatility的计算方法和应用领域进行了广泛研究,并取得了重要进展。
本文将对该计算方法进行详细阐述,并探讨其对投资组合管理和风险控制的影响以及未来发展趋势。
1.2文章结构本文共分为五个部分。
第一部分为引言,介绍了文章的背景和目的。
第二部分将回顾idiosyncratic volatility计算的背景,包括其定义、重要性以及计算方法在不同领域中的应用。
第三部分将深入探讨常见的idiosyncratic volatility计算方法及其原理,并讨论与资产定价模型之间存在的关系。
第四部分将通过实证研究和案例分析,进一步验证并说明idiosyncratic volatility计算方法的实际应用。
最后,第五部分总结文章主要内容,并展望未来idiosyncratic volatility计算研究的发展趋势。
1.3目的本文旨在提供对读者关于idiosyncratic volatility计算的综合了解,并帮助读者理解该概念在投资组合管理和风险控制中的重要性。
通过详细解释计算方法和模型,以及实证研究和案例分析,本文将为读者提供一个全面且深入的认识,使其能够更好地应用该概念于实际投资决策中。
最后,在文章结论中,我们将对整个主题进行总结回顾,并提出进一步研究或建议等。
2. idiosyncratic volatility计算的背景:2.1 什么是idiosyncratic volatility:Idiosyncratic volatility是指公司个别股票的价格波动相对于整个市场的不确定性。
《香帅的北大金融学课》证券选择:金融资产的定价因子
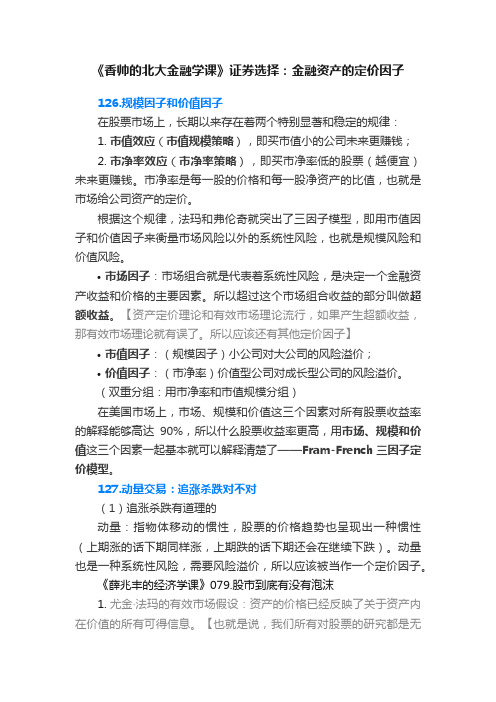
《香帅的北大金融学课》证券选择:金融资产的定价因子126.规模因子和价值因子在股票市场上,长期以来存在着两个特别显著和稳定的规律:1.市值效应(市值规模策略),即买市值小的公司未来更赚钱;2.市净率效应(市净率策略),即买市净率低的股票(越便宜)未来更赚钱。
市净率是每一股的价格和每一股净资产的比值,也就是市场给公司资产的定价。
根据这个规律,法玛和弗伦奇就突出了三因子模型,即用市值因子和价值因子来衡量市场风险以外的系统性风险,也就是规模风险和价值风险。
•市场因子:市场组合就是代表着系统性风险,是决定一个金融资产收益和价格的主要因素。
所以超过这个市场组合收益的部分叫做超额收益。
【资产定价理论和有效市场理论流行,如果产生超额收益,那有效市场理论就有误了。
所以应该还有其他定价因子】•市值因子:(规模因子)小公司对大公司的风险溢价;•价值因子:(市净率)价值型公司对成长型公司的风险溢价。
(双重分组:用市净率和市值规模分组)在美国市场上,市场、规模和价值这三个因素对所有股票收益率的解释能够高达90%,所以什么股票收益率更高,用市场、规模和价值这三个因素一起基本就可以解释清楚了——Fram-French三因子定价模型。
127.动量交易:追涨杀跌对不对(1)追涨杀跌有道理的动量:指物体移动的惯性,股票的价格趋势也呈现出一种惯性(上期涨的话下期同样涨,上期跌的话下期还会在继续下跌)。
动量也是一种系统性风险,需要风险溢价,所以应该被当作一个定价因子。
《薛兆丰的经济学课》079.股市到底有没有泡沫1.尤金·法玛的有效市场假设:资产的价格已经反映了关于资产内在价值的所有可得信息。
【也就是说,我们所有对股票的研究都是无用的,参考《漫步华尔街》】2.罗伯特·希勒的市场泡沫论:市场并不是那么有效,资产的定价有时候过高,有时候过低,人们的反应有时候不足,有时候又过激。
3.资产价格与博傻行为:有一派认为资产价格取决于人们认为它是否有价值。
- 1、下载文档前请自行甄别文档内容的完整性,平台不提供额外的编辑、内容补充、找答案等附加服务。
- 2、"仅部分预览"的文档,不可在线预览部分如存在完整性等问题,可反馈申请退款(可完整预览的文档不适用该条件!)。
- 3、如文档侵犯您的权益,请联系客服反馈,我们会尽快为您处理(人工客服工作时间:9:00-18:30)。
• There are many types of non-linear models, e.g. - ARCH / GARCH - switching models - bilinear models
„Introductory Econometrics for Finance‟ © Chris Brooks 2002
• What could the current value of the variance of the errors plausibly depend upon? – Previous squared error terms. • This leads to the autoregressive conditionally heteroscedastic model for the variance of the errors: t2 = 0 + 1 u2 t 1 • This is known as an ARCH(1) model.
„Introductory Econometrics for Finance‟ © Chris Brooks 2002
2
A Sample Financial Asset Returns Time Series
Daily S&P 500 Returns for January 1990 – December 1999
• Campbell, Lo and MacKinlay (1997) define a non-linear data generating process as one that can be written yt = f(ut, ut-1, ut-2, …) where ut is an iid error term and f is a non-linear function. • They also give a slightly more specific definition as yt = g(ut-1, ut-2, …)+ ut2(ut-1, ut-2, …) where g is a function of past error terms only and 2 is a variance term. • Models with nonlinear g(•) are “non-linear in mean”, while those with nonlinear 2(•) are “non-linear in variance”.
ut 0 1 yt2 2 yt3 ... p 1 ytp vt
• Many other non-linearity tests are available, e.g. the “BDS test” and the bispectrum test. • One particular non-linear model that has proved very useful in finance is the ARCH model due to Engle (1982).
„Introductory Econometrics for Finance‟ © Chris Brooks 2002 7
Autoregressive Conditionally Heteroscedastic (ARCH) Models
• So use a model which does not assume that the variance is constant. • Recall the definition of the variance of ut: t2 = Var(ut ut-1, ut-2,...) = E[(ut-E(ut))2 ut-1, ut-2,...] We usually assume that E(ut) = 0 so t2 = Var(ut ut-1, ut-2,...) = E[ut2 ut-1, ut-2,...].
t 0 1ut21
,
vt N(0,1)
• The two are different ways of expressing exactly the same model. The first form is easier to understand while the second form is required for simulating from an ARCH model, for example.
„Introductory Econometrics for Finance‟ © Chris Brooks 2002 4
Types of non-linear models
• The linear paradigm is a useful one. Many apparently non-linear relationships can be made linear by a suitable transformation. On the other hand, it is likely that many relationships in finance are intrinsically non-linear.
„Introductory Econometrics for Finance‟ © Chris Brooks 2002
10
Testing for “ARCH Effects”
1. First, run any postulated linear regression of the form given in the equation above, e.g. yt = 1 + 2x2t + ... + kxkt + ut ˆ saving the residuals, ut . 2. Then square the residuals, and regress them on q own lags to test for ARCH of order q, i.e. run the regression ˆ ˆ ˆ ˆ ut2 0 1ut21 2ut2 2 ... qut2 q vt where vt is iid. Obtain R2 from this regression
• What if the variance of the errors is not constant? - heteroscedasticity - would imply that standard error estimates could be wrong. • Is the variance of the errors likely to be constant over time? Not for financial data.
„Introductory Econometrics for Finance‟ © Chris Brooks 2002 8
Autoregressive Conditionally Heteroscedastic (ARCH) Models (cont’d)
• The full model would be yt = 1 + 2x2t + ... + kxkt + ut , ut N(0, t2) where t2 = 0 + 1 ut21 • We can easily extend this to the general case where the error variance depends on q lags of squared errors: 2 2 u2 t2 = 0 + 1 ut 1 +2 ut 2 +...+q t q • This is an ARCH(q) model. • Instead of calling the variance t2, in the literature it is usually called ht, so the model is yt = 1 + 2x2t + ... + kxkt + ut , ut N(0,ht) where ht = 0 + 1 ut21 +2 ut2 2 +...+q ut2 q
• Our “traditional” structural model could be something like: yt = 1 + 2x2t + ... + kxkt + ut, or more compactly y = X + u. • We also assumed ut N(0,2).
„Introductory Econometrics for Finance‟ © Chris Brooks 2002 6
Heteroscedasticity Revisited
• An example of a structural model is yt = 1 + 2x2t + 3x3t + 4x4t + u t 2 with ut N(0, u ). • The assumption that the variance of the errors is constant is known as 2 homoscedasticity, i.e. Var (ut) = u .
5
Testing for Non-linearity
• The “traditional” tools of time series analysis (acf‟s, spectral analysis) may find no evidence that we could use a linear model, but the data may still not be independent. • Portmanteau tests for non-linear dependence have been developed. The simplest is Ramsey‟s RESET test, which took the form: