4_SeisClass_Workflow(GeoFrame聚类分析)
人工智能领域中英文专有名词汇总
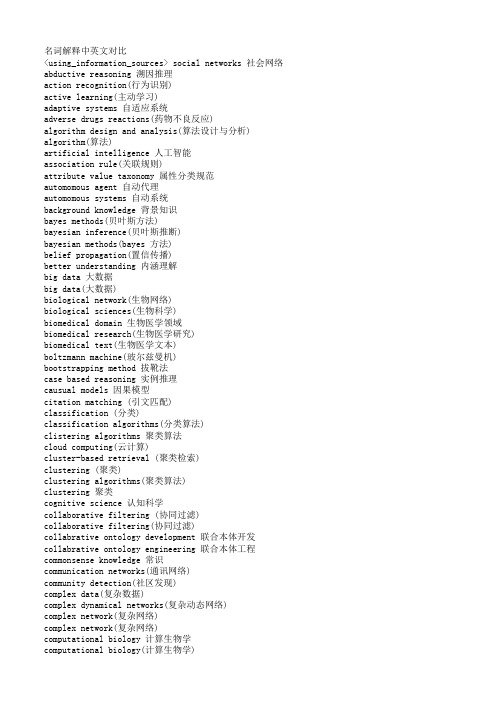
名词解释中英文对比<using_information_sources> social networks 社会网络abductive reasoning 溯因推理action recognition(行为识别)active learning(主动学习)adaptive systems 自适应系统adverse drugs reactions(药物不良反应)algorithm design and analysis(算法设计与分析) algorithm(算法)artificial intelligence 人工智能association rule(关联规则)attribute value taxonomy 属性分类规范automomous agent 自动代理automomous systems 自动系统background knowledge 背景知识bayes methods(贝叶斯方法)bayesian inference(贝叶斯推断)bayesian methods(bayes 方法)belief propagation(置信传播)better understanding 内涵理解big data 大数据big data(大数据)biological network(生物网络)biological sciences(生物科学)biomedical domain 生物医学领域biomedical research(生物医学研究)biomedical text(生物医学文本)boltzmann machine(玻尔兹曼机)bootstrapping method 拔靴法case based reasoning 实例推理causual models 因果模型citation matching (引文匹配)classification (分类)classification algorithms(分类算法)clistering algorithms 聚类算法cloud computing(云计算)cluster-based retrieval (聚类检索)clustering (聚类)clustering algorithms(聚类算法)clustering 聚类cognitive science 认知科学collaborative filtering (协同过滤)collaborative filtering(协同过滤)collabrative ontology development 联合本体开发collabrative ontology engineering 联合本体工程commonsense knowledge 常识communication networks(通讯网络)community detection(社区发现)complex data(复杂数据)complex dynamical networks(复杂动态网络)complex network(复杂网络)complex network(复杂网络)computational biology 计算生物学computational biology(计算生物学)computational complexity(计算复杂性) computational intelligence 智能计算computational modeling(计算模型)computer animation(计算机动画)computer networks(计算机网络)computer science 计算机科学concept clustering 概念聚类concept formation 概念形成concept learning 概念学习concept map 概念图concept model 概念模型concept modelling 概念模型conceptual model 概念模型conditional random field(条件随机场模型) conjunctive quries 合取查询constrained least squares (约束最小二乘) convex programming(凸规划)convolutional neural networks(卷积神经网络) customer relationship management(客户关系管理) data analysis(数据分析)data analysis(数据分析)data center(数据中心)data clustering (数据聚类)data compression(数据压缩)data envelopment analysis (数据包络分析)data fusion 数据融合data generation(数据生成)data handling(数据处理)data hierarchy (数据层次)data integration(数据整合)data integrity 数据完整性data intensive computing(数据密集型计算)data management 数据管理data management(数据管理)data management(数据管理)data miningdata mining 数据挖掘data model 数据模型data models(数据模型)data partitioning 数据划分data point(数据点)data privacy(数据隐私)data security(数据安全)data stream(数据流)data streams(数据流)data structure( 数据结构)data structure(数据结构)data visualisation(数据可视化)data visualization 数据可视化data visualization(数据可视化)data warehouse(数据仓库)data warehouses(数据仓库)data warehousing(数据仓库)database management systems(数据库管理系统)database management(数据库管理)date interlinking 日期互联date linking 日期链接Decision analysis(决策分析)decision maker 决策者decision making (决策)decision models 决策模型decision models 决策模型decision rule 决策规则decision support system 决策支持系统decision support systems (决策支持系统) decision tree(决策树)decission tree 决策树deep belief network(深度信念网络)deep learning(深度学习)defult reasoning 默认推理density estimation(密度估计)design methodology 设计方法论dimension reduction(降维) dimensionality reduction(降维)directed graph(有向图)disaster management 灾害管理disastrous event(灾难性事件)discovery(知识发现)dissimilarity (相异性)distributed databases 分布式数据库distributed databases(分布式数据库) distributed query 分布式查询document clustering (文档聚类)domain experts 领域专家domain knowledge 领域知识domain specific language 领域专用语言dynamic databases(动态数据库)dynamic logic 动态逻辑dynamic network(动态网络)dynamic system(动态系统)earth mover's distance(EMD 距离) education 教育efficient algorithm(有效算法)electric commerce 电子商务electronic health records(电子健康档案) entity disambiguation 实体消歧entity recognition 实体识别entity recognition(实体识别)entity resolution 实体解析event detection 事件检测event detection(事件检测)event extraction 事件抽取event identificaton 事件识别exhaustive indexing 完整索引expert system 专家系统expert systems(专家系统)explanation based learning 解释学习factor graph(因子图)feature extraction 特征提取feature extraction(特征提取)feature extraction(特征提取)feature selection (特征选择)feature selection 特征选择feature selection(特征选择)feature space 特征空间first order logic 一阶逻辑formal logic 形式逻辑formal meaning prepresentation 形式意义表示formal semantics 形式语义formal specification 形式描述frame based system 框为本的系统frequent itemsets(频繁项目集)frequent pattern(频繁模式)fuzzy clustering (模糊聚类)fuzzy clustering (模糊聚类)fuzzy clustering (模糊聚类)fuzzy data mining(模糊数据挖掘)fuzzy logic 模糊逻辑fuzzy set theory(模糊集合论)fuzzy set(模糊集)fuzzy sets 模糊集合fuzzy systems 模糊系统gaussian processes(高斯过程)gene expression data 基因表达数据gene expression(基因表达)generative model(生成模型)generative model(生成模型)genetic algorithm 遗传算法genome wide association study(全基因组关联分析) graph classification(图分类)graph classification(图分类)graph clustering(图聚类)graph data(图数据)graph data(图形数据)graph database 图数据库graph database(图数据库)graph mining(图挖掘)graph mining(图挖掘)graph partitioning 图划分graph query 图查询graph structure(图结构)graph theory(图论)graph theory(图论)graph theory(图论)graph theroy 图论graph visualization(图形可视化)graphical user interface 图形用户界面graphical user interfaces(图形用户界面)health care 卫生保健health care(卫生保健)heterogeneous data source 异构数据源heterogeneous data(异构数据)heterogeneous database 异构数据库heterogeneous information network(异构信息网络) heterogeneous network(异构网络)heterogenous ontology 异构本体heuristic rule 启发式规则hidden markov model(隐马尔可夫模型)hidden markov model(隐马尔可夫模型)hidden markov models(隐马尔可夫模型) hierarchical clustering (层次聚类) homogeneous network(同构网络)human centered computing 人机交互技术human computer interaction 人机交互human interaction 人机交互human robot interaction 人机交互image classification(图像分类)image clustering (图像聚类)image mining( 图像挖掘)image reconstruction(图像重建)image retrieval (图像检索)image segmentation(图像分割)inconsistent ontology 本体不一致incremental learning(增量学习)inductive learning (归纳学习)inference mechanisms 推理机制inference mechanisms(推理机制)inference rule 推理规则information cascades(信息追随)information diffusion(信息扩散)information extraction 信息提取information filtering(信息过滤)information filtering(信息过滤)information integration(信息集成)information network analysis(信息网络分析) information network mining(信息网络挖掘) information network(信息网络)information processing 信息处理information processing 信息处理information resource management (信息资源管理) information retrieval models(信息检索模型) information retrieval 信息检索information retrieval(信息检索)information retrieval(信息检索)information science 情报科学information sources 信息源information system( 信息系统)information system(信息系统)information technology(信息技术)information visualization(信息可视化)instance matching 实例匹配intelligent assistant 智能辅助intelligent systems 智能系统interaction network(交互网络)interactive visualization(交互式可视化)kernel function(核函数)kernel operator (核算子)keyword search(关键字检索)knowledege reuse 知识再利用knowledgeknowledgeknowledge acquisitionknowledge base 知识库knowledge based system 知识系统knowledge building 知识建构knowledge capture 知识获取knowledge construction 知识建构knowledge discovery(知识发现)knowledge extraction 知识提取knowledge fusion 知识融合knowledge integrationknowledge management systems 知识管理系统knowledge management 知识管理knowledge management(知识管理)knowledge model 知识模型knowledge reasoningknowledge representationknowledge representation(知识表达) knowledge sharing 知识共享knowledge storageknowledge technology 知识技术knowledge verification 知识验证language model(语言模型)language modeling approach(语言模型方法) large graph(大图)large graph(大图)learning(无监督学习)life science 生命科学linear programming(线性规划)link analysis (链接分析)link prediction(链接预测)link prediction(链接预测)link prediction(链接预测)linked data(关联数据)location based service(基于位置的服务) loclation based services(基于位置的服务) logic programming 逻辑编程logical implication 逻辑蕴涵logistic regression(logistic 回归)machine learning 机器学习machine translation(机器翻译)management system(管理系统)management( 知识管理)manifold learning(流形学习)markov chains 马尔可夫链markov processes(马尔可夫过程)matching function 匹配函数matrix decomposition(矩阵分解)matrix decomposition(矩阵分解)maximum likelihood estimation(最大似然估计)medical research(医学研究)mixture of gaussians(混合高斯模型)mobile computing(移动计算)multi agnet systems 多智能体系统multiagent systems 多智能体系统multimedia 多媒体natural language processing 自然语言处理natural language processing(自然语言处理) nearest neighbor (近邻)network analysis( 网络分析)network analysis(网络分析)network analysis(网络分析)network formation(组网)network structure(网络结构)network theory(网络理论)network topology(网络拓扑)network visualization(网络可视化)neural network(神经网络)neural networks (神经网络)neural networks(神经网络)nonlinear dynamics(非线性动力学)nonmonotonic reasoning 非单调推理nonnegative matrix factorization (非负矩阵分解) nonnegative matrix factorization(非负矩阵分解) object detection(目标检测)object oriented 面向对象object recognition(目标识别)object recognition(目标识别)online community(网络社区)online social network(在线社交网络)online social networks(在线社交网络)ontology alignment 本体映射ontology development 本体开发ontology engineering 本体工程ontology evolution 本体演化ontology extraction 本体抽取ontology interoperablity 互用性本体ontology language 本体语言ontology mapping 本体映射ontology matching 本体匹配ontology versioning 本体版本ontology 本体论open government data 政府公开数据opinion analysis(舆情分析)opinion mining(意见挖掘)opinion mining(意见挖掘)outlier detection(孤立点检测)parallel processing(并行处理)patient care(病人医疗护理)pattern classification(模式分类)pattern matching(模式匹配)pattern mining(模式挖掘)pattern recognition 模式识别pattern recognition(模式识别)pattern recognition(模式识别)personal data(个人数据)prediction algorithms(预测算法)predictive model 预测模型predictive models(预测模型)privacy preservation(隐私保护)probabilistic logic(概率逻辑)probabilistic logic(概率逻辑)probabilistic model(概率模型)probabilistic model(概率模型)probability distribution(概率分布)probability distribution(概率分布)project management(项目管理)pruning technique(修剪技术)quality management 质量管理query expansion(查询扩展)query language 查询语言query language(查询语言)query processing(查询处理)query rewrite 查询重写question answering system 问答系统random forest(随机森林)random graph(随机图)random processes(随机过程)random walk(随机游走)range query(范围查询)RDF database 资源描述框架数据库RDF query 资源描述框架查询RDF repository 资源描述框架存储库RDF storge 资源描述框架存储real time(实时)recommender system(推荐系统)recommender system(推荐系统)recommender systems 推荐系统recommender systems(推荐系统)record linkage 记录链接recurrent neural network(递归神经网络) regression(回归)reinforcement learning 强化学习reinforcement learning(强化学习)relation extraction 关系抽取relational database 关系数据库relational learning 关系学习relevance feedback (相关反馈)resource description framework 资源描述框架restricted boltzmann machines(受限玻尔兹曼机) retrieval models(检索模型)rough set theroy 粗糙集理论rough set 粗糙集rule based system 基于规则系统rule based 基于规则rule induction (规则归纳)rule learning (规则学习)rule learning 规则学习schema mapping 模式映射schema matching 模式匹配scientific domain 科学域search problems(搜索问题)semantic (web) technology 语义技术semantic analysis 语义分析semantic annotation 语义标注semantic computing 语义计算semantic integration 语义集成semantic interpretation 语义解释semantic model 语义模型semantic network 语义网络semantic relatedness 语义相关性semantic relation learning 语义关系学习semantic search 语义检索semantic similarity 语义相似度semantic similarity(语义相似度)semantic web rule language 语义网规则语言semantic web 语义网semantic web(语义网)semantic workflow 语义工作流semi supervised learning(半监督学习)sensor data(传感器数据)sensor networks(传感器网络)sentiment analysis(情感分析)sentiment analysis(情感分析)sequential pattern(序列模式)service oriented architecture 面向服务的体系结构shortest path(最短路径)similar kernel function(相似核函数)similarity measure(相似性度量)similarity relationship (相似关系)similarity search(相似搜索)similarity(相似性)situation aware 情境感知social behavior(社交行为)social influence(社会影响)social interaction(社交互动)social interaction(社交互动)social learning(社会学习)social life networks(社交生活网络)social machine 社交机器social media(社交媒体)social media(社交媒体)social media(社交媒体)social network analysis 社会网络分析social network analysis(社交网络分析)social network(社交网络)social network(社交网络)social science(社会科学)social tagging system(社交标签系统)social tagging(社交标签)social web(社交网页)sparse coding(稀疏编码)sparse matrices(稀疏矩阵)sparse representation(稀疏表示)spatial database(空间数据库)spatial reasoning 空间推理statistical analysis(统计分析)statistical model 统计模型string matching(串匹配)structural risk minimization (结构风险最小化) structured data 结构化数据subgraph matching 子图匹配subspace clustering(子空间聚类)supervised learning( 有support vector machine 支持向量机support vector machines(支持向量机)system dynamics(系统动力学)tag recommendation(标签推荐)taxonmy induction 感应规范temporal logic 时态逻辑temporal reasoning 时序推理text analysis(文本分析)text anaylsis 文本分析text classification (文本分类)text data(文本数据)text mining technique(文本挖掘技术)text mining 文本挖掘text mining(文本挖掘)text summarization(文本摘要)thesaurus alignment 同义对齐time frequency analysis(时频分析)time series analysis( 时time series data(时间序列数据)time series data(时间序列数据)time series(时间序列)topic model(主题模型)topic modeling(主题模型)transfer learning 迁移学习triple store 三元组存储uncertainty reasoning 不精确推理undirected graph(无向图)unified modeling language 统一建模语言unsupervisedupper bound(上界)user behavior(用户行为)user generated content(用户生成内容)utility mining(效用挖掘)visual analytics(可视化分析)visual content(视觉内容)visual representation(视觉表征)visualisation(可视化)visualization technique(可视化技术) visualization tool(可视化工具)web 2.0(网络2.0)web forum(web 论坛)web mining(网络挖掘)web of data 数据网web ontology lanuage 网络本体语言web pages(web 页面)web resource 网络资源web science 万维科学web search (网络检索)web usage mining(web 使用挖掘)wireless networks 无线网络world knowledge 世界知识world wide web 万维网world wide web(万维网)xml database 可扩展标志语言数据库附录 2 Data Mining 知识图谱(共包含二级节点15 个,三级节点93 个)间序列分析)监督学习)领域 二级分类 三级分类。
GF4_G&G_evaluation油藏描述系统
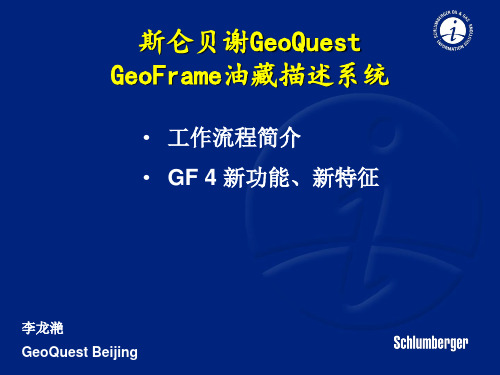
裂缝指 示器
离散 裂缝 模型 裂缝 模型 基质 模型
单井属性
储层物性总模型 及基质模型
溶孔 模型
基质 模型
Merak
Economic evaluation and decision Oil/Gas pipe transmission system analysis Development plan decision
指示变换
分析油、气 水剖面分布
手工或随 机方法
构造模型
约束
约束
沉积相模型
约束
储层参数模型
约束
成岩模型
地球化学模型
粗化 油藏数值模拟
油藏地质模型 E2G
地震数据
Synthetics SeisTie
地质数据
测井数据 WellEdit/ Preplus
IESX
Indepth
FrameWork(T) FrameWork(D)
地质标定
CSA
ResSum
Elan/PVP/F RockCell/ MI/DipFan RockClass
指示变换
WellPix
Cross Section
Seismic Drop
LPM
SeisClass
分析油、气 水剖面分布
约束 Properties/ GeoObject
Assembly
约束
SISim.
Display Results
Refine Interpretation
多种手段进行地球物理解释及分析
断面网格
平面断点链式追踪
断裂走向链式追踪
断 层 链 式 追 踪 : 搜 索 半 径 和搜 索 角 设 定 沿 特 定 方 向 追 踪 在 优 势 方 向 上 断 点 的 自 动连 结 编 辑 与 修 改 多 边 形 拾 取 : 拾 取 容 忍 限 设 定 对 模 糊 点 只 需 少 量 的 人 工 干 预
Geomodeling_VVA 软件技术附件
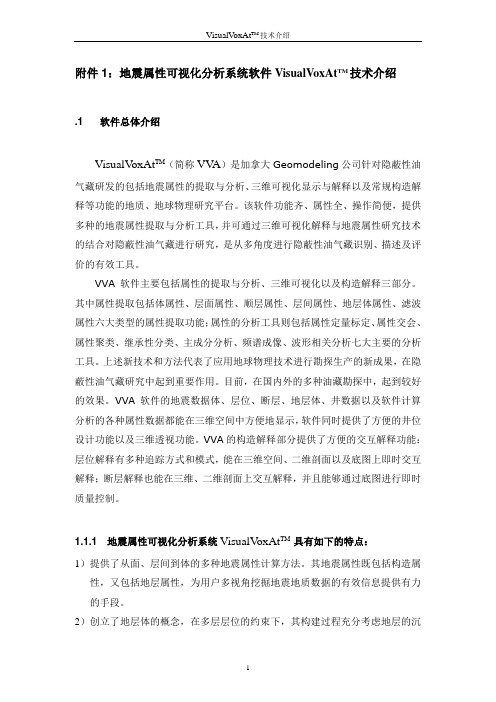
附件1:地震属性可视化分析系统软件VisualVoxAt TM技术介绍.1 软件总体介绍VisualV oxAt TM(简称VV A)是加拿大Geomodeling公司针对隐蔽性油气藏研发的包括地震属性的提取与分析、三维可视化显示与解释以及常规构造解释等功能的地质、地球物理研究平台。
该软件功能齐、属性全、操作简便,提供多种的地震属性提取与分析工具,并可通过三维可视化解释与地震属性研究技术的结合对隐蔽性油气藏进行研究,是从多角度进行隐蔽性油气藏识别、描述及评价的有效工具。
VVA软件主要包括属性的提取与分析、三维可视化以及构造解释三部分。
其中属性提取包括体属性、层面属性、顺层属性、层间属性、地层体属性、滤波属性六大类型的属性提取功能;属性的分析工具则包括属性定量标定、属性交会、属性聚类、继承性分类、主成分分析、频谱成像、波形相关分析七大主要的分析工具。
上述新技术和方法代表了应用地球物理技术进行勘探生产的新成果,在隐蔽性油气藏研究中起到重要作用。
目前,在国内外的多种油藏勘探中,起到较好的效果。
VVA软件的地震数据体、层位、断层、地层体、井数据以及软件计算分析的各种属性数据都能在三维空间中方便地显示,软件同时提供了方便的井位设计功能以及三维透视功能。
VVA的构造解释部分提供了方便的交互解释功能:层位解释有多种追踪方式和模式,能在三维空间、二维剖面以及底图上即时交互解释;断层解释也能在三维、二维剖面上交互解释,并且能够通过底图进行即时质量控制。
1.1.1地震属性可视化分析系统VisualV oxAt TM具有如下的特点:1)提供了从面、层间到体的多种地震属性计算方法。
其地震属性既包括构造属性,又包括地层属性,为用户多视角挖掘地震地质数据的有效信息提供有力的手段。
2)创立了地层体的概念,在多层层位的约束下,其构建过程充分考虑地层的沉积在平面和空间上的变化。
地层体切片比时间切片更好地反映地质规律。
各种属性以及分析方法都可以应用到地层体中,使得对隐蔽性油气藏识别、描述和评价更具目的性。
geoframe地震属性基本提取步骤
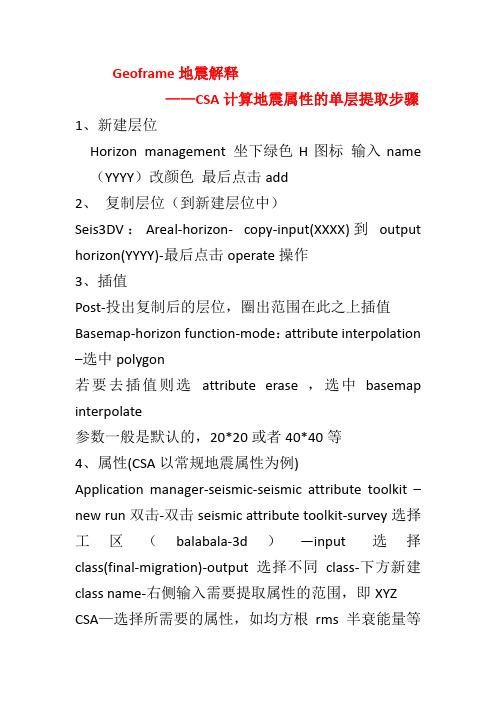
Geoframe地震解释——CSA计算地震属性的单层提取步骤1、新建层位Horizon management 坐下绿色H图标输入name (YYYY)改颜色最后点击add2、复制层位(到新建层位中)Seis3DV:Areal-horizon- copy-input(XXXX)到output horizon(YYYY)-最后点击operate操作3、插值Post-投出复制后的层位,圈出范围在此之上插值Basemap-horizon function-mode:attribute interpolation –选中polygon若要去插值则选attribute erase ,选中basemap interpolate参数一般是默认的,20*20或者40*40等4、属性(CSA以常规地震属性为例)Application manager-seismic-seismic attribute toolkit –new run双击-双击seismic attribute toolkit-survey选择工区(balabala-3d)—input选择class(final-migration)-output选择不同class-下方新建class name-右侧输入需要提取属性的范围,即XYZ CSA—选择所需要的属性,如均方根rms 半衰能量等-single horizon沿单层提取-reference horizon参考层位-选择start relative to –above 向上开时窗- 一般选none 不确定极性是红轴(正)还是黑轴(负)-时间10ms Output-输出层位(YYYY)最后点击run5、投影到basemapPost-interpretation-horizon-(YYYY)-attributes-rms_ampli tude&final_migration-~~~~~~~~~下面是一些基础学习资料~~~~~~~~~~~~ 1、常规地震属性(CSA) 常规地震属性参数可以使用默认CSA参数功能来设置。
Workflow mining A survey of issues and approaches
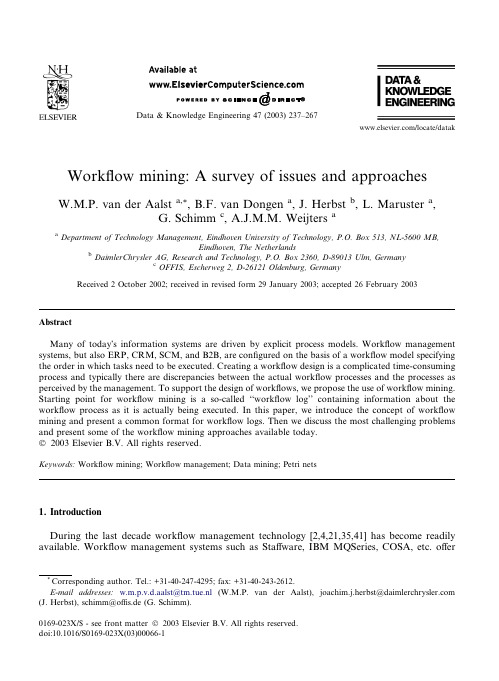
Workflow mining:A survey of issues and approachesW.M.P.van der Aalst a,*,B.F.van Dongen a ,J.Herbst b ,L.Maruster a ,G.Schimm c ,A.J.M.M.Weijters aaDepartment of Technology Management,Eindhoven University of Technology,P.O.Box 513,NL-5600MB,Eindhoven,The NetherlandsbDaimlerChrysler AG,Research and Technology,P.O.Box 2360,D-89013Ulm,GermanycOFFIS,Escherweg 2,D-26121Oldenburg,GermanyReceived 2October 2002;received in revised form 29January 2003;accepted 26February 2003AbstractMany of today Õs information systems are driven by explicit process models.Workflow management systems,but also ERP,CRM,SCM,and B2B,are configured on the basis of a workflow model specifying the order in which tasks need to be executed.Creating a workflow design is a complicated time-consuming process and typically there are discrepancies between the actual workflow processes and the processes as perceived by the management.To support the design of workflows,we propose the use of workflow mining.Starting point for workflow mining is a so-called ‘‘workflow log’’containing information about the workflow process as it is actually being executed.In this paper,we introduce the concept of workflow mining and present a common format for workflow logs.Then we discuss the most challenging problems and present some of the workflow mining approaches available today.Ó2003Elsevier B.V.All rights reserved.Keywords:Workflow mining;Workflow management;Data mining;Petri nets1.IntroductionDuring the last decade workflow management technology [2,4,21,35,41]has become readily available.Workflow management systems such as Staffware,IBM MQSeries,COSA,etc.offer*Corresponding author.Tel.:+31-40-247-4295;fax:+31-40-243-2612.E-mail addresses:w.m.p.v.d.aalst@tm.tue.nl (W.M.P.van der Aalst),joachim.j.herbst@ (J.Herbst),schimm@offis.de (G.Schimm).0169-023X/$-see front matter Ó2003Elsevier B.V.All rights reserved.doi:10.1016/S0169-023X(03)00066-1/locate/datakData &Knowledge Engineering 47(2003)237–267238W.M.P.van der Aalst et al./Data&Knowledge Engineering47(2003)237–267generic modeling and enactment capabilities for structured business processes.By making process definitions,i.e.,models describing the life-cycle of a typical case(workflow instance)in isolation, one can configure these systems to support business processes.These process definitions need to be executable and are typically graphical.Besides pure workflow management systems many other software systems have adopted workflow technology.Consider for example Enterprise Resource Planning(ERP)systems such as SAP,PeopleSoft,Baan and Oracle,Customer Relationship Management(CRM)software,Supply Chain Management(SCM)systems,Business to Business (B2B)applications,etc.which embed workflow technology.Despite its promise,many problems are encountered when applying workflow technology.One of the problems is that these systems require a workflow design,i.e.,a designer has to construct a detailed model accurately describing the routing of work.Modeling a workflow is far from trivial:It requires deep knowledge of the business process at hand(i.e.,lengthy discussions with the workers and management are needed) and the workflow language being used.To compare workflow mining with the traditional approach towards workflow design and enactment,consider the workflow life cycle shown in Fig.1.The workflow life cycle consists of four phases:(A)workflow design,(B)workflow configuration,(C)workflow enactment,and(D) workflow diagnosis.In the traditional approach the design phase is used for constructing a workflow model.This is typically done by a business consultant and is driven by ideas of man-agement on improving the business processes at hand.If the design isfinished,the workflow system(or any other system that is‘‘process aware’’)is configured as specified in the design phase. In the configuration phases one has to deal with limitation and particularities of the workflow management system being used(cf.[5,65]).In the enactment phase,cases(i.e.,workflow instances) are handled by the workflow system as specified in the design phase and realized in the configu-ration phase.Based on a running workflow,it is possible to collect diagnostic information which is analyzed in the diagnosis phase.The diagnosis phase can again provide input for the design phase thus completing the workflow life cycle.In the traditional approach the focus is on the design and configuration phases.Less attention is paid to the enactment phase and few organi-zations systematically collect runtime data which is analyzed as input for redesign(i.e.,the diag-nosis phase is typically missing).The goal of workflow mining is to reverse the process and collect data at runtime to support workflow design and analysis.Note that in most cases,prior to the deployment of a workflowW.M.P.van der Aalst et al./Data&Knowledge Engineering47(2003)237–267239 system,the workflow was already there.Also note that in most information systems transactional data is registered(consider for example the transaction logs of ERP systems like SAP).The in-formation collected at run-time can be used to derive a model explaining the events recorded. Such a model can be used in both the diagnosis phase and the(re)design phase.Modeling an existing process is influenced by perceptions,e.g.,models are often normative in the sense that they state what‘‘should’’be done rather than describing the actual process.As a result models tend to be rather subjective.A more objective way of modeling is to use data related to the actual events that took place.Note that workflow mining is not biased by perceptions or normative behavior.However,if people bypass the system doing things differently,the log can still deviate from the actual work being done.Nevertheless,it is useful to confront man-made models with models discovered through workflow mining.Closely monitoring the events taking place at runtime also enables Delta analysis,i.e.,detecting discrepancies between the design constructed in the design phase and the actual execution regis-tered in the enactment phase.Workflow mining results in an‘‘a posteriori’’process model which can be compared with the‘‘a priori’’model.Workflow technology is moving into the direction of more operationalflexibility to deal with workflow evolution and workflow exception handling [2,7,10,13,20,30,39,40,64].As a result workers can deviate from the prespecified workflow design. Clearly one wants to monitor these deviations.For example,a deviation may become common practice rather than being a rare exception.In such a case,the added value of a workflow system becomes questionable and an adaptation is required.Clearly,workflow mining techniques can be used to create a feedback loop to adapt the workflow model to changing circumstances and detect imperfections of the design.The topic of workflow mining is related to management trends such as Business Process Re-engineering(BPR),Business Intelligence(BI),Business Process Analysis(BPA),Continuous Process Improvement(CPI),and Knowledge Management(KM).Workflow mining can be seen as part of the BI,BPA,and KM trends.Moreover,workflow mining can be used as input for BPR and CPI activities.Note that workflow mining seems to be more appropriate for BPR than for CPI.Recall that one of the basic elements of BPR is that it is radical and should not be restricted by the existing situation[23].Also note that workflow mining is not a tool to(re)design processes. The goal is to understand what is really going on as indicated in Fig.1.Despite the fact that workflow mining is not a tool for designing processes,it is evident that a good understanding of the existing processes is vital for any redesign effort.This paper is a joint effort of a number of researchers using different approaches to workflow mining and is a spin-offof the‘‘Workflow Mining Workshop’’.1The goal of this paper is to introduce the concept of workflow mining,to identify scientific and practical problems,to present a common format to store workflow logs,to provide an overview of existing approaches,and to present a number of mining techniques in more detail.The remainder of this paper is organized as follows.First,we summarize related work.In Section3we define workflow mining and present some of the challenging problems.In Section 4we propose a common XML-based format for storing and exchanging workflow logs.This format is used by the mining tools developed by the authors and interfaces with some of the 1This workshop took place on May22nd and23rd2002in Eindhoven,The Netherlands.240W.M.P.van der Aalst et al./Data&Knowledge Engineering47(2003)237–267leading workflow management systems(Staffware,MQSeries Workflow,and InConcert).Sec-tions5–9introducefive approaches to workflow mining focusing on different aspects.These sections give an overview of some of the ongoing work on workflow mining.Section10 compares the various approaches and list a number of open problems.Section11concludes the paper.2.Related workThe idea of process mining is not new[8,11,15–17,24–29,42–44,53–57,61–63].Cook and Wolf have investigated similar issues in the context of software engineering processes.In[15]they describe three methods for process discovery:one using neural networks,one using a purely algorithmic approach,and one Markovian approach.The authors consider the latter two the most promising approaches.The purely algorithmic approach builds afinite state machine (FSM)where states are fused if their futures(in terms of possible behavior in the next k steps) are identical.The Markovian approach uses a mixture of algorithmic and statistical methods and is able to deal with noise.Note that the results presented in[6]are limited to sequential behavior.Cook and Wolf extend their work to concurrent processes in[16].They propose specific metrics(entropy,event type counts,periodicity,and causality)and use these metrics to discover models out of event streams.However,they do not provide an approach to generate explicit process models.Recall that thefinal goal of the approach presented in this paper is to find explicit representations for a broad range of process models,i.e.,we want to be able to generate a concrete Petri net rather than a set of dependency relations between events.In[17] Cook and Wolf provide a measure to quantify discrepancies between a process model and the actual behavior as registered using event-based data.The idea of applying process mining in the context of workflow management wasfirst introduced in[11].This work is based on workflow graphs,which are inspired by workflow products such as IBM MQSeries workflow(formerly known as Flowmark)and InConcert.In this paper,two problems are defined.Thefirst problem is tofind a workflow graph generating events appearing in a given workflow log.The second problem is tofind the definitions of edge conditions.A concrete algorithm is given for tackling thefirst problem.The approach is quite different from other approaches:Because the nature of workflow graphs there is no need to identify the nature(AND or OR)of joins and splits.As shown in[37],workflow graphs use true and false tokens which do not allow for cyclic graphs. Nevertheless,[11]partially deals with iteration by enumerating all occurrences of a given task and then folding the graph.However,the resulting conformal graph is not a complete model.In [44],a tool based on these algorithms is presented.Schimm[53,54,57]has developed a mining tool suitable for discovering hierarchically structured workflow processes.This requires all splits and joins to be balanced.Herbst and Karagiannis also address the issue of process mining in the context of workflow management[24–29]using an inductive approach.The work presented in[27,29]is limited to sequential models.The approach described in[24–26,28]also allows for concurrency.It uses stochastic task graphs as an intermediate representation and it generates a workflow model described in the ADONIS modeling language.In the induction step task nodes are merged and split in order to discover the underlying process.A notable difference with other approaches is that the same task can appear multiple times in the workflow model.The graphW.M.P.van der Aalst et al./Data&Knowledge Engineering47(2003)237–267241 generation technique is similar to the approach of[11,44].The nature of splits and joins(i.e., AND or OR)is discovered in the transformation step,where the stochastic task graph is transformed into an ADONIS workflow model with block-structured splits and joins.In con-trast to the previous papers,the work in[8,42,43,61,62]is characterized by the focus on workflow processes with concurrent behavior(rather than adding ad hoc mechanisms to capture parallelism).In[61,62]a heuristic approach using rather simple metrics is used to construct so-called‘‘dependency/frequency tables’’and‘‘dependency/frequency graphs’’.In[42]another variant of this technique is presented using examples from the health-care domain.The pre-liminary results presented in[42,61,62]only provide heuristics and focus on issues such as noise. The approach described in[8]differs from these approaches in the sense that for the a algorithm it is proven that for certain subclasses it is possible tofind the right workflow model.In[3]the a algorithm is extended to incorporate timing information.Process mining can be seen as a tool in the context of Business(Process)Intelligence(BPI). In[22,52]a BPI toolset on top of HPs Process Manager is described.The BPI tools set includes a so-called‘‘BPI Process Mining Engine’’.However,this engine does not provide any techniques as discussed before.Instead it uses generic mining tools such as SAS Enterprise Miner for the generation of decision trees relating attributes of cases to information about execution paths(e.g., duration).In order to do workflow mining it is convenient to have a so-called‘‘process data warehouse’’to store audit trails.Such as data warehouse simplifies and speeds up the queries needed to derive causal relations.In[19,46–48]the design of such warehouse and related issues are discussed in the context of workflow logs.Moreover,[48]describes the PISA tool which can be used to extract performance metrics from workflow logs.Similar diagnostics are provided by the ARIS Process Performance Manager(PPM)[34].The later tool is commercially available and a customized version of PPM is the Staffware Process Monitor(SPM)[59]which is tailored towards mining Staffware logs.Note that none of the latter tools is extracting the process model.The main focus is on clustering and performance analysis rather than causal relations as in[8,11,15–17,24–29,42–44,53–57,61–63].Much of the work mentioned above will be discussed in more detail in Sections5–9.Before doing so,wefirst look at workflow mining in general and introduce a common XML-based format for storing and exchanging workflow logs.3.Workflow miningThe goal of workflow mining is to extract information about processes from transaction logs. Instead of starting with a workflow design,we start by gathering information about the workflow processes as they take place.We assume that it is possible to record events such that(i)each event refers to a task(i.e.,a well-defined step in the workflow),(ii)each event refers to a case(i.e.,a workflow instance),and(iii)events are totally ordered.Any information system using transac-tional systems such as ERP,CRM,or workflow management systems will offer this information in some form.Note that we do not assume the presence of a workflow management system.The only assumption we make,is that it is possible to collect workflow logs with event data.These workflow logs are used to construct a process specification which adequately models the behavior registered. The term process mining refers to methods for distilling a structured process description from a set242W.M.P.van der Aalst et al./Data&Knowledge Engineering47(2003)237–267of real executions.Because these methods focus on so-called case-driven process that are sup-ported by contemporary workflow management systems,we also use the term workflow mining.Table1shows a fragment of a workflow log generated by the Staffware system.In Staffware events are grouped on a case-by-case basis.Thefirst column refers to the task(description),the second to the type of event,the third to the user generating the event(if any),and the last column shows a time stamp.The corresponding Staffware model is shown in Fig.2.Case10shown in Table1follows the scenario wherefirst task Register is executed followed Send questionnaire, Receive questionnaire,and Evaluate.Based on the Evaluation,the decision is made to directly archive(task Archive)the case without further processing.For Case9further processing is nee-ded,while Case8involves a timeout and the repeated execution of some tasks.Someone familiar with Staffware will be able to decide that the three cases indeed follow a scenario possible in the Staffware model shown in Fig.2.However,three cases are not sufficient to automatically derive the model of Fig.2.Note that there are more Staffware models enabling the three scenarios shown in Table1.The challenge of workflow mining is to derive‘‘good’’workflow models with as little information as possible.To illustrate the principle of process mining in more detail,we consider the workflow log shown in Table2.This log abstracts from the time,date,and event type,and limits the information to the order in which tasks are being executed.The log shown in Table2contains information aboutfive cases(i.e.,workflow instances).The log shows that for four cases(1,2,3,and4)the tasks A,B,C, and D have been executed.For thefifth case only three tasks are executed:tasks A,E,and D. Each case starts with the execution of A and ends with the execution of D.If task B is executed, then also task C is executed.However,for some cases task C is executed before task B.Based on the information shown in Table2and by making some assumptions about the completeness of the log(i.e.,assuming that the cases are representative and a sufficient large subset of possible be-haviors is observed),we can deduce for example the process model shown in Fig.3.The model is represented in terms of a Petri net[50].The Petri net starts with task A andfinishes with task D. These tasks are represented by transitions.After executing A there is a choice between either executing B and C in parallel or just executing task E.To execute B and C in parallel two non-observable tasks(AND-split and AND-join)have been added.These tasks have been added for routing purposes only and are not present in the workflow log.Note that for this example we assume that two tasks are in parallel if they appear in any order.By distinguishing between start events and complete events for tasks it is possible to explicitly detect parallelism(cf.Section4).Table2contains the minimal information we assume to be present.In many applications,the workflow log contains a time stamp for each event and this information can be used to extract additional causality information.In addition,a typical log also contains information about the type of event,e.g.,a start event(a person selecting an task from a worklist),a complete event(the completion of a task),a withdraw event(a scheduled task is removed),etc.Moreover,we are also interested in the relation between attributes of the case and the actual route taken by a particular case.For example,when handling traffic violations:Is the make of a car relevant for the routing of the corresponding traffic violation?(For example,People driving a Ferrari always pay theirfines in time.)For this simple example(i.e.,Table2),it is quite easy to construct a process model that is able to regenerate the workflow log(e.g.,Fig.3).For more realistic situations there are however a number of complicating factors:W.M.P.van der Aalst et al./Data&Knowledge Engineering47(2003)237–267243 Table1A Staffware logDirective description Event User yyyy/mm/dd hh:mmCase10Start bvdongen@staffw_e2002/06/1912:58Register Processed to bvdongen@staffw_e2002/06/1912:58Register Released by bvdongen@staffw_e2002/06/1912:58Send questionnaire Processed to bvdongen@staffw_e2002/06/1912:58Evaluate Processed to bvdongen@staffw_e2002/06/1912:58Send questionnaire Released by bvdongen@staffw_e2002/06/1913:00Receive questionnaire Processed to bvdongen@staffw_e2002/06/1913:00Receive questionnaire Released by bvdongen@staffw_e2002/06/1913:00Evaluate Released by bvdongen@staffw_e2002/06/1913:00Archive Processed to bvdongen@staffw_e2002/06/1913:00Archive Released by bvdongen@staffw_e2002/06/1913:00Terminated2002/06/1913:00Case9Start bvdongen@staffw_e2002/06/1912:36Register Processed to bvdongen@staffw_e2002/06/1912:36Register Released by bvdongen@staffw_e2002/06/1912:35Send questionnaire Processed to bvdongen@staffw_e2002/06/1912:36Evaluate Processed to bvdongen@staffw_e2002/06/1912:36Send questionnaire Released by bvdongen@staffw_e2002/06/1912:36Receive questionnaire Processed to bvdongen@staffw_e2002/06/1912:36Receive questionnaire Released by bvdongen@staffw_e2002/06/1912:36Evaluate Released by bvdongen@staffw_e2002/06/1912:37Process complaint Processed to bvdongen@staffw_e2002/06/1912:37Process complaint Released by bvdongen@staffw_e2002/06/1912:37Check processing Processed to bvdongen@staffw_e2002/06/1912:37Check processing Released by bvdongen@staffw_e2002/06/1912:38Archive Processed to bvdongen@staffw_e2002/06/1912:38Archive Released by bvdongen@staffw_e2002/06/1912:38Terminated2002/06/1912:38Case8Start bvdongen@staffw_e2002/06/1912:36Register Processed to bvdongen@staffw_e2002/06/1912:36Register Released by bvdongen@staffw_e2002/06/1912:35Send questionnaire Processed to bvdongen@staffw_e2002/06/1912:36Evaluate Processed to bvdongen@staffw_e2002/06/1912:36Send questionnaire Released by bvdongen@staffw_e2002/06/1912:36Receive questionnaire Processed to bvdongen@staffw_e2002/06/1912:36Receive questionnaire Expired bvdongen@staffw_e2002/06/1912:37Receive questionnaire Withdrawn bvdongen@staffw_e2002/06/1912:37Receive timeout Processed to bvdongen@staffw_e2002/06/1912:37Receive timeout Released by bvdongen@staffw_e2002/06/1912:37Evaluate Released by bvdongen@staffw_e2002/06/1912:37Process complaint Processed to bvdongen@staffw_e2002/06/1912:37Process complaint Released by bvdongen@staffw_e2002/06/1912:37Check processing Processed to bvdongen@staffw_e2002/06/1912:37(continued on next page)Table 2A workflow log Case identifier Task identifier Case 1Task A Case 2Task A Case 3Task A Case 3TaskB Case 1Task B Case 1TaskC Case 2Task C Case 4Task A Case 2Task B Case 2TaskD Case 5Task A Case 4Task C Case 1Task D Case 3Task C Case 3Task D Case 4Task B Case 5TaskE Case 5Task D Case4TaskDFig.2.The staffware model.Table 1(continued )Directive description Event Useryyyy/mm/dd hh:mm Check processing Released by bvdongen@staffw_e 2002/06/1912:38Process complaint Processed to bvdongen@staffw_e 2002/06/1912:37Process complaint Released by bvdongen@staffw_e 2002/06/1912:37Check processing Processed to bvdongen@staffw_e 2002/06/1912:37Check processing Released by bvdongen@staffw_e 2002/06/1912:38Archive Processed to bvdongen@staffw_e 2002/06/1912:38ArchiveReleased by bvdongen@staffw_e2002/06/1912:38Terminated2002/06/1912:38244W.M.P.van der Aalst et al./Data &Knowledge Engineering 47(2003)237–267W.M.P.van der Aalst et al./Data&Knowledge Engineering47(2003)237–267245•For larger workflow models mining is much more difficult.For example,if the model exhibits alternative and parallel routing,then the workflow log will typically not contain all possible combinations.Consider10tasks which can be executed in parallel.The total number of inter-leavings is10!¼3628800.It is not realistic that each interleaving is present in the log.More-over,certain paths through the process model may have a low probability and therefore remain undetected.•Workflow logs will typically contain noise,i.e.,parts of the log can be incorrect,incomplete,or refer to exceptions.Events can be logged incorrectly because of human or technical errors.Events can be missing in the log if some of the tasks are manual or handled by another sys-tem/organizational unit.Events can also refer to rare or undesired events.Consider for example the workflow in a hospital.If due to time pressure the order of two events(e.g.,make X-ray and remove drain)is reversed,this does not imply that this would be part of the regular medical protocol and should be supported by the hospitalÕs workflow system.Also two causally unre-lated events(e.g.,take blood sample and death of patient)may happen next to each other with-out implying a causal relation(i.e.,taking a sample did not result in the death of the patient;it was sheer coincidence).Clearly,exceptions which are recorded only once should not automat-ically become part of the regular workflow.•Table2only shows the order of events without giving information about the type of event,the time of the event,and attributes of the event(i.e.,data about the case and/or task).Clearly,it isa challenge to exploit such additional information.Sections5–9will present different approaches to some of these problems.To conclude this section,we point out legal issues relevant when mining(timed)workflow logs. Clearly,workflow logs can be used to systematically measure the performance of employees.The legislation with respect to issues such as privacy and protection of personal data differs from country to country.For example,Dutch companies are bound by the Personal Data Protection Act (Wet Bescherming Persoonsgegeven)which is based on a directive from the European Union.The practical implications of this for the Dutch situation are described in[14,31,51].Workflow logs are not restricted by these laws as long as the information in the log cannot be traced back to indi-viduals.If information in the log can be traced back to a specific employee,it is important that the employee is aware of the fact that her/his activities are logged and the fact that this logging is used to monitor her/his performance.Note that in a timed workflow log we can abstract from information about the workers executing tasks and still mine the process.Therefore,it is possible to avoid collecting information on the productivity of individual workers and legislation such as the Personal246W.M.P.van der Aalst et al./Data&Knowledge Engineering47(2003)237–267Data Protection Act does not apply.Nevertheless,the logs of most workflow systems contain information about individual workers,and therefore,this issue should be considered carefully.4.Workflow logs:A common XML formatIn this section we focus on the syntax and semantics of the information stored in the workflow log.We will do this by presenting a tool independent XML format that is used by each of the mining approaches/tools described in the remainder.Fig.4shows that this XML format connects transactional systems such as workflow management systems,ERP systems,CRM systems,and case handling systems.In principle,any system that registers events related to the execution of tasks for cases can use this tool independent format to store and exchange logs.The XML format is used as input for the analysis tools presented in Sections5–9.The goal of using a single format is to reduce the implementation effort and to promote the use of these mining techniques in multiple contexts.Table3shows the Document Type Definition(DTD)[12]for workflow logs.This DTD specifies the syntax of a workflow log.A workflow log is a consistent XML document,i.e.,a well-formed and valid XMLfile with top element WorkFlow_log(see Table3).As shown,a workflow log consists of(optional)information about the source program and information about one or more workflow processes.Each workflow process(element process)consists of a sequence of cases (element case)and each case consists of a sequence of log lines(element log_line).Both processes and cases have an id and a description.Each line in the log contains the name of a task(element task_name).In addition,the line may contain information about the task instance(element task_instance),the type of event(element event),the date(element date),and the time of the event (element time).It is advised to make sure that the process description and the case description are unique for each process or case respectively.The task name should be a unique identifier for a task within a process.If there are two or more tasks in a process with the same task name,they are as-。
Geoframe软件操作-数据加载和时深转换
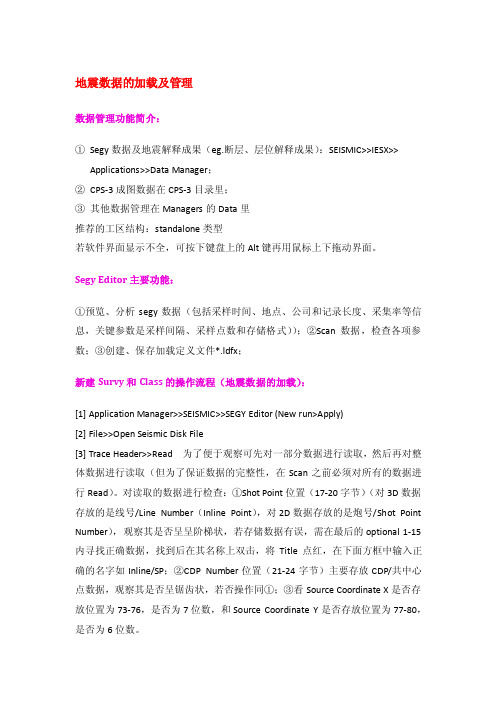
地震数据的加载及管理数据管理功能简介:①Segy数据及地震解释成果(eg.断层、层位解释成果):SEISMIC>>IESX>>Applications>>Data Manager;②CPS-3成图数据在CPS-3目录里;③其他数据管理在Managers的Data里推荐的工区结构:standalone类型若软件界面显示不全,可按下键盘上的Alt键再用鼠标上下拖动界面。
Segy Editor主要功能:①预览、分析segy数据(包括采样时间、地点、公司和记录长度、采集率等信息,关键参数是采样间隔、采样点数和存储格式));②Scan数据,检查各项参数;③创建、保存加载定义文件*.ldfx;新建Survy和Class的操作流程(地震数据的加载):[1] Application Manager>>SEISMIC>>SEGY Editor (New run>Apply)[2] File>>Open Seismic Disk File[3] Trace Header>>Read 为了便于观察可先对一部分数据进行读取,然后再对整体数据进行读取(但为了保证数据的完整性,在Scan之前必须对所有的数据进行Read)。
对读取的数据进行检查:①Shot Point位置(17-20字节)(对3D数据存放的是线号/Line Number(Inline Point),对2D数据存放的是炮号/Shot Point Number),观察其是否呈呈阶梯状,若存储数据有误,需在最后的optional 1-15内寻找正确数据,找到后在其名称上双击,将Title点红,在下面方框中输入正确的名字如Inline/SP;②CDP Number位置(21-24字节)主要存放CDP/共中心点数据,观察其是否呈锯齿状,若否操作同①;③看Source Coordinate X是否存放位置为73-76,是否为7位数,和Source Coordinate Y是否存放位置为77-80,是否为6位数。
Geoframe软件基本知识
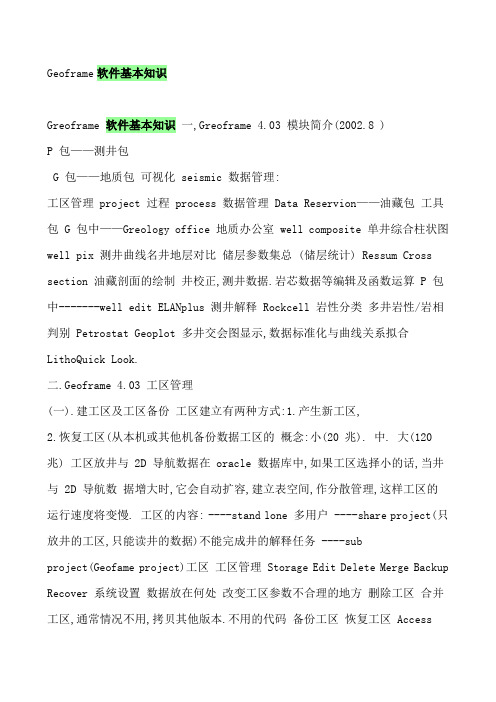
Geoframe软件基本知识Greoframe 软件基本知识一,Greoframe 4.03 模块简介(2002.8 )P 包——测井包G 包——地质包可视化 seismic 数据管理:工区管理 project 过程 process 数据管理 Data Reservion——油藏包工具包 G 包中——Greology office 地质办公室 well composite 单井综合柱状图well pix 测井曲线名井地层对比储层参数集总 (储层统计) Ressum Cross section 油藏剖面的绘制井校正,测井数据.岩芯数据等编辑及函数运算 P 包中-------well edit ELANplus 测井解释 Rockcell 岩性分类多井岩性/岩相判别 Petrostat Geoplot 多井交会图显示,数据标准化与曲线关系拟合LithoQuick Look.二.Geoframe 4.03 工区管理(一).建工区及工区备份工区建立有两种方式:1.产生新工区,2.恢复工区(从本机或其他机备份数据工区的概念:小(20 兆). 中. 大(120 兆) 工区放井与 2D 导航数据在 oracle 数据库中,如果工区选择小的话,当井与 2D 导航数据增大时,它会自动扩容,建立表空间,作分散管理,这样工区的运行速度将变慢. 工区的内容: ----stand lone 多用户 ----share project(只放井的工区,只能读井的数据)不能完成井的解释任务 ----subproject(Geofame project)工区工区管理 Storage Edit Delete Merge Backup Recover 系统设置数据放在何处改变工区参数不合理的地方删除工区合并工区,通常情况不用,拷贝其他版本.不用的代码备份工区恢复工区 Accessrights Rebuild zndexes 自动做整个工区,提高速度,一般先要作备份工区备份 Backup-----Acrchive*.gfa 完全备份产生的*log 文件有些有用的信息Fast-backup*.gfb 增量备份 recover 恢复增量备份恢复完全备份选择性备份 1 解释输出(在 Seismic 中 Data manage) 2 Data Save(well Data)将整个油田井数据备份 3 2D Seismic 校正好的 Class 包括加载定义一起备份 4 在CP 备份整个 CP 目录,作一个 tar 文件(包括网格文件)从工区输入 (CP 是个单独模块,可以放在任何一个工区下运行) 使用命令备份remoto—gen---save.csh 选择性备份 restore:remite—recover.csh 关于基准面的问题: (Data Manager Users Guide---AppendixA) ER (elevation Reference) EIT (elebation at Time zere) 建工区给工区名——确认口令——从 DBA 选工区的大小. 类型——DBA 注册——连接数据库分配空间分开管理(解释地震速度 CPS 缺省)——创建工区的参数(米制) 地震基准面 North American 27 clake1866 zone 代理很重要 OTM zone number——19(二)地震数据的加载与备份 1 SEGX Dump: 带是否可读测线名字道头位置shotpoint 的数及在道头位置,增量是正是负采样率是否正确(必须是用微秒) 样点数是否正确有无坐标及在道头位置 EBCDIC (Extended Binary coded Decimal znterchange code)计算机对符号的代码 3200bytes reel header 卷头400bytes 240 240…… line 头 trace1 trace2 二进制二进制道头 one at the end of each file two EOT's mark on the end of tape End of File mark End of Tape mark (EOT) 2 SEGY formate line header 1 Sample rate 4000 microseconds=4ms 2 Number of Sample pertrace 3 Data Sample formate code 1—IBM32—bit 浮点 (4byte per sample) 2—IBM32—bit 整数 (4byte persample) 3—IBM16—bit 整数 (2byte per sample) 4—32bit point with gain(4byte per sample) Sample rate(ms) *(number of Sample)=length of the trace 4 1000 4000 Full Dump (完全 Dump) 2D 定义 * loc line CDP x y 3D 定义 3 个点面元大小 3 在 seismic 中 Data Manager (详细见中的数据管理) 1 地震 seY 输入输出 2 删除.拷贝.层位.断层输入输出 (缺省格式) 3 产生.改变 class,重新命名测线,产生 Time.Slice 切片 4 二维测站,删除等5 surface 管理可以转换为层位提供 IESX—UTIL 恢复工区 cd IESXPROG 定义格式 line read 表达式 expression trace read6 2D 测线加入中带桩号(找到对应关系) (三)数据加载及备份井数据 name checkshots OWI marker API log dat bottom location hole condition index elevation information flow direction deviation surrey 3 井数据加载井数据备份 ASCII 加载 Data Load 在井的各数管理中备份(打印输出) Data Save 1 关于 KB 的问题 KB 不是补正高,它是井到 project reference 之间 SCB Checkshot depth datumn USP 处理针对哪一个面做的加井时 well—Borehol—加 elevation—Reference ASCII 加载 (控制文件 Data——ASCII Load 批加载) Wu-asciiWu-galoaden*.ctl(放在缺省目录下) 2 (1) 井位加载文件: 井名 x ywell.ctl g25 Name well 1 OWI Borehole 1 Location x 2 Location y 3 (单井加入) (2) 井曲线 (log data) 原文件: 井深 las1 las2 控制文件 log.ctl DT us/m 2 RHOB g/cm3 3 GR gAPI 4 (3) 井分层 (well marker data ) (多井加入) 原文件 : 井名分层测深 g23 23 ^^^^^ g23 32 ^^^^^ g24 23 ^^^^^ g32 32 ^^^^^ 控制文件: (marker.ctl): Borhole-name start-wam Depth/Time Name (4) 井斜 (well deviation survey) 原文件: 井名 MD 垂深东分北分控制文件 MD m1 MD m2 DX m3 (单井加入) DY m4 1 2 3 (marker ram) MD TVD DX DY m1 m2 m3 m4 在 Borehole UIM (1)多井装入 (5) 井的 Checkshot (well checkshot survey) 原文件 TVD Time(s) 控制文件 TVD m (checkshot.ctl) TWOTIM s 装入给出是给出井名 (一个工区允许有多个 checkshot,也可把速度按这种方式装入) (6) 离散文件装入 (Scatter set Data) 原文件 X Y Z (如平均速度) 控制文件 X 1 Y 2 Property 3 UWI (Unique well Identifier) 井名(最长 40) API (the American Petroleum Institute) Well name 属性用于区分多个版本,别各针对井,也用于家载 DLIS 数据 DLIS (Digital Log Interchange Standard) LAS (Log Interchange Standard) Well composed of Multiple Boreholes Elevation 是到参考面 datumis 垂直距离 MD (Measured Depth) True Vertical Depth (TVD) Two way Time (TWT) (需要做校正) Borehole working Datum Wellcheckshot Datum Well Deviation Datum Well Marker Datum Depth Grid 工区工作 datum 3.井数据 Data Load 加载 Data Load *.gf66 *.Lis *.Dlis 对油田井打包后加入,加的是二进制的文件,只看到文件的头, (preview 可以查看) 如果想选择性加入一些曲线,则——Library Filter option 1 不过滤. 单个文件 DLIS 4. 井数据备份 (井的数据直接在数据库) 1 将井的各种数据在各数据相应的管理窗口备份选择所要输出内容 (小打印机)输出选上 well symbol (SPACE)空格 5 2 Data save——打包 Archive *.gf66 所有井数据二进制测井曲线 DLIS ASCII x . y . marker 在 Data—general—右键—弹出油田—弹出油田有关井信息打开 TTC 转输在 Data save 下井数据会自动显示如果选 ASCII—no Depth index muti borehole and producer 在LIS 格式下 File number 1 代表每口井的数 2 3 每口井输出后需要 Apply 单口井输出 marker—ASCII 中—well marker 3 在 CPS 中——井数据可以打包(四)常用一些命令 (gf-db-admin.fm) 1. 在 Geoframe xterm window 启动proman Q 2. $CL-f 1 $CL-f-project name 查看谁在用计算机解锁 2 $CL-f project project password 3. gf-users 查看本机工区运行情况 client___ID process 4. gf__accounts 查看机本机名字,分配的 oracle gf__accounts-o (每个工区的所有元) gf__project-users free space project__passproj-changepasswd 允许 DBA 或用户来改变它们工区的口令 proj__delete工区工区口令 __update 三合成记录制作,时深关系建立 (synthetrcs) seismic---IESX---Application Synthetics seismic---synthetic sonic logs 选井---post---Time/Depth check shots 声波慢度两者任选其一 velocity 给初始速度值 coefficient 1 不用 checkshot,时深关系从声波中提取,可用correction 2 用 checkshot. (校正声波用 checkshot)选 checkshotpost---sonic scale marker Reflection 曲线刻度分层 wavelet seismic correlation Auxiliary log 子波地震(井旁道) 其中子波可以在 Tools---通过井旁道提,对子波编辑,分析,输入输出 Tools---漂移及局部拉伸在子波分析---Time varying 在面版 seismic 右键弹出选 borehole---在井柱上选---appearance---出现窗口 borehole appearance 可贴上合成记录(直接在合成记录的窗口) Tools—Backshif 在声波,marker 上漂.虽在时域工作互校正了时深关系 (整体漂移) stretch-sqeeze 局部拉伸选停泊点 anchor 停泊 2 键选点 1 键拉伸 relocity surey 编辑 checkshot 后校正声波曲线 wavelet Extract Analytical Edit Time varying 产生时变子波 Import/export 合成记录完成后三键---Save 存在合成记录---在地震解释在 Boreholeappearance 中完成合成记录定义---update well (更新井集)再贴在剖面上(表现一道或多道) 在 Tools---Synthetic---上下键记住时间值,回到合成记录中,重新做合成记录四 .地震资料综合解释 Interpretation: 2D/3D 综合解释,底图 Basemap Data Manager: 数据管理 Computation Manager 属性计算,方差体 Mistie Analysis 自动追踪 Automatic Picking IESX Surface Slice Synthetics 合成记录制作 Geoviz 可视化 Geoviz expore Basic TD 时深转换Interpetation Model Manager 解释模型管理 (一) 解释模型管理启动Interpretation ----选 Model 创建 Model Model Manager ----Populate 合并 7 ----两种方式 1 Assign 全拷(会覆盖相同的层位) 2 Clone 拷贝(选择性拷贝,不会覆盖相同的层位) 选 Source Model 对要拷贝层位,断层选择 (二)自动追踪 Automatic Picking---ASAP AutoPix 算法不一样 ASAP(Automatic Seismic Area Picken) ----在 Edit 定义一个 Seismic Area Path 多边形 ----数据种子类 Tracking option Area 断层边界追踪方法----先作 Advanced 追踪的数据在 Horizon Function----Attrebute Erase 删除最好先备份在解释窗口 Areal----Horizon Copy 备份下换成另一个名字,再做自动追踪或删除(三)数据管理 Data Manage 在 IESX 放的数据 3D/2D 测线工线 Surface(层位,断层) 解释(属性,断层 Cuts, Contacts) 网格文件在 Geoframe 中所有的3D 层位属性存在数据库以网格形式 2D 层位属性以线数据存在 Geoframe 下列属性自动产生:Time Snap-Criteria Interpretation-origin Pick-Quality (地震解释,ASAP,AutoPix) 逆断层由 Horizon Patches 进行管理(1,2,3)LCS( Local Coordinate System) IESX ing-f/dev/rmt/on fsf/ 绕过另一个文件解锁 $CL 大写 $IESXPROG/Da-clear 全称作切割线输出,先产生2D,后输出工具 IESX cd $IESXPROG ies_Util 1 12 22 42 44 2 终止退出其中有的恢复工区 Path----定义多边形----输出层位(控制范围) 收敛 (四)属性,方差体产生选测站,选要运行的程序----输出文件放在 DataManangers----Interpretation Data 找到可以删除删除 (五)常见问题 1. 优先级管理 ---Baspman---user---preferme---presence1,2,3---3—2—1切 ----surveys 2. 手工加的 marker 显示不出来.在 Source 中加入GF-Loader 3. 输入层位必须是缺省 5 项记录 X Y line CDP Time 4. 将 IESX 中的层位按 X,Y,Z 输出,作为离散文件加入,将离散文件网格 Gridding,再将网格文件还原成 Surface (Data Manage---解释---Surface 完成 5. 属性产生与删除, 在数据管理下解释数据中删除 6. 移动底图上粘贴顺序 7. 斜线的切割 8. 数据加载中 Raviable fix 9. 计算井斜-----倾角问题 10. 加入深度域的地震数据,在剖面显示中 User—改变显示状态 Metric 缺省的是 11.User----refrenence----Fault(从 Segment---(变成) Symbol)能把断层的闭合点显示大一些五.Geoviz. 可视化 (相干数据体) Geoviz explore Data Manage—Geoviz—只有显示功能 Visualization File—Volume Load (三维数据体加载) 看有没有空洞,颜色是否均匀去掉不要的东西—点黑如 Box Map 等对地震 ----在 Tools—Modify 下修改颜色网格层位/断层体Tools—Volume—Pan—解释(如加断层)必须在+状态解释右键退出解释状态Fault Surface/Volume (产生断层面) 解释完后可以可以产生断层面,以后的断层解释可用 XN GT R O 外推限制不限制 Shift+P 去掉立体框 Voxel Picking—种子体检测 Shift+1 键点种子点 Covert Detected Volume—产生层位体—输出层面存顶,底 1.井为空井 (0,0) 2.空 2D,3D Surface 3.2D 导航数据出错出现故障而不能找到数据 4.速度异常 Tool—Modif—修改层位—Coloning—Chang Attribute—选 Grid ( 产生各种属性数据体的时间网)--Modify Object—Modify Surface—Coloning Grid—改变颜色 9 Geofram 4.0 版本软件使用手册第一部分基础知识一,系统介绍 Geofram 4.0 系统是schlumber 公司针对解决测井,地震,油藏, 公司针对解决测井,地震,油藏, 地质以及综合研究等问题开发的较为完整的集成软件. 所示, 地质以及综合研究等问题开发的较为完整的集成软件.如图 1-1 所示,本等问题开发的较为完整的集成软件软件共划分井眼地质 ( geolog ) 岩石物理 ,油藏描述(reservoir) (petrophysics) 油藏描述(reservoir),地震 petrophysics)油藏描述(reservoir), , seismic) 可视化地震(visualization) ,可视化地震(visualization)和工 ( seismic ) 可视化地震 (visualization) 和工 , 具(utility)等六个部分.系统管理配备 3 个基 (utility)等六个部分. 等六个部分本管理工具:项目管理工具( mandge) 本管理工具:项目管理工具(project mandge) , 工作流程管理器( manage) 工作流程管理器(process manage)及数据管理 manage) 该系统具有如下特色: .该系统具有如下特色器(data manage) 该系统具有如下特色: . 关系型数据库管理: *基于 Oracle 关系型数据库管理:使得解释参数,描述信息及离散,连续采样的信息数据, 参数,描述信息及离散,连续采样的信息数据, 样的信息数据均以数据指针信息存放在项目数据库的目录中. 均以数据指针信息存放在项目数据库的目录中. 数据入库后不需知道数据以什么样格式存放再什么地方,只进行聚焦即可得到快速查询. 什么地方,只进行聚焦即可得到快速查询. 可靠的安全性:设置了用户存储权限, *可靠的安全性:设置了用户存储权限,使得数据管理更为方便; 得数据管理更为方便;图 1-1 *极大提高工作效率:合理的项目配置,减少不必要的数据重复,使数极大提高工作效率:合理的项目配置,减少不必要的数据重复, 据深度,单位,坐标系统等转换更为方便,省时; 据深度,单位,坐标系统等转换更为方便,省时; *强大的可操作性:所有程序军采用窗口,菜单选项交互运行,加大可强大的可操作性:所有程序军采用窗口,菜单选项交互运行, 视化程序. 视化程序. 二,系统登陆当在界面敲入用户名和密码之后, 所视界面, 当在界面敲入用户名和密码之后 , 出现如图 1-2 所视界面 , 选择版本系统, 所示界面, GeoFram 4.0.3 进入 GeoFram4.0 版本系统,出现图 1-3 所示界面,选择其中一个用户,敲入密码 ( pas sword 图 1-2 图 1-3 ),当系统确认后在单击Application manager 即可完成系统的完全登陆. 即可完成系统的完全登陆. 所示界面.可选择进行下一步工作. 出现如图 1-4 所示界面.可选择进行下一步工作. 11 三,数据加载进入系统后, 管理器, 进入系统后,单击系统界面中的 Data manager 管理器,出现数据管图 1-4 图 1-5 理主窗口(如图 1-4) 理主窗口( . 窗口, 选择 loaders and unloaders 出现如图 1-5 窗口, 其中ASCIL Load 表数据加载; 数据加载; 表示数据存示 ASCIL 数据加载;data load 为 Dlis 数据加载;data save 表示数据存储.其具体操作方法如下: 其具体操作方法如下: a,ASCII LOAD ASCII load 模块主要用来加载文本数据,具体步骤如下: 具体步骤如下: 将 ASCII LOAD 模块调入工作流程管理器中,单击鼠标左键,则可掉入主窗口( 器中,单击鼠标左键,则可掉入主窗口(如图1-6) : 图 1-6 1) 2) 3) 中选择所加数据文件名称; 在 input file 中选择所加数据文件名称; 中填入控制文件名; 在 control file 中填入控制文件名; 在 create file 中可编写所加载的数据.选择所需要的曲线; 载的数据.选择所需要的曲线; 4) 按 run 键即可完成 ascii 数据的加载. 的加载. 模块. b , data load 模块 . 本模块主要用 LIS,DLIS,BIT, 等格式的途是将LIS,DLIS,BIT,LA716 等格式的数据加入数据库中.具体步骤如下: 数据加入数据库中.具体步骤如下: 在 Geofram 的 P 包中,将 Data load 包中, 图 1-7 模块调入工作流程管理器,并用鼠标左键双击, 模块调入工作流程管理器,并用鼠标左键双击,则可调入 Data load 主窗口(如图 1-5) : ,在对话框中假如你想要加入的数据文件名称(LIS, 1) 在 input file 对话框中假如你想要加入的数据文件名称(LIS, , DLIS,BIT, 等格式) DLIS,BIT,LA716 等格式) ; 2) 在targetr field 中假如油田名称; ,在 , 中假如油田名称; ,在中输入井名; 3) 在 target well 中输入井名; , ,在中输入井眼名称; 4) 在 target borehole 中输入井眼名称; , ,在中输入公司名(可选) 5) 在 producer 中输入公司名(可选) , ; ,点击按纽,即可将所要数据加入数据库中. 6) 点击 run 按纽,即可将所要数据加入数据库中. , 13 资料预处理( edit) 第二部分资料预处理(well edit) 因为各种井况,仪器,人为等因素造成测井原始资料深度不统一,因为各种井况,仪器,人为等因素造成测井原始资料深度不统一, 局部出现畸点等,因此在室内对其进行修改和编辑非常重要, 局部出现畸点等,因此在室内对其进行修改和编辑非常重要,Geofram 系统软件就提供这样一个方便快洁的编辑模块——well 模块, 统软件就提供这样一个方便快洁的编辑模块——well edit 模块,我门可——以利用它来队原始数据资料进行处理.其工作步骤如下: 以利用它来队原始数据资料进行处理.其工作步骤如下: 一, 进入编辑窗口, 如图 2-1 所示进入 p 包后直接选择 Welledit 进入编辑窗口, 模块即可. 模块即可. 窗口. 二,点击 Welledit 右键出现图 2-2 窗口.其中 inspect 表示聚焦选择井文件, 表示运行程序表示退出该模块, 井文件,run 表示运行程序,exit 表示退出该模块,abort 表示中断目前运行方式. 运行方式. 图2-1 图 2-2 图 2-3 编辑模块主界面. 所示, 三,聚焦数据后即可进入welledit 编辑模块主界面.如图 2-3 所示, 模块, 然后根据需要分别点击Edit ,depth match 和 splice 模块,可以根据需求进行井编辑,其中部分快捷功能键用途说明如下: 求进行井编辑,其中部分快捷功能键用途说明如下: 各快洁键使用说明如下: 各快洁键使用说明如下: 第三部分 Utility PlotUtility Plot 是 Geofram 系统中的一个交会图工具模块,主要功能是进行 XY 交会 XY- 值图,直方图, 图,XY-Z 值图,直方图,玫瑰图等, 瑰图等,主要窗口如图 3-1. 该模块使用方法如下: 该模块使用方法如下: 1, 点击 Data Focus 模块进行数据聚焦,即选择交会曲线所在位置; 模块进行数据聚焦,即选择交会曲线所在位置; 15 图 3-1 选项中分别输入所交会曲线的起止深度; 2, 在 Top 和 Bottom 选项中分别输入所交会曲线的起止深度; 选项中选择所需要图形类别, 3, 在 Application Type 选项中选择所需要图形类别, 分别为 Cross Plot 值图) Historm(直方图 Polar(极坐标图直方图) 极坐标图) (交会图),Z Plot (Z 值图),Historm(直方图),Polar(极坐标图)等, 交会图) 现以交会图为例进行说明. 现以交会图为例进行说明. 4, 单击 Presentation File 按钮所示图版, 出现图 3-2 所示图版,依据需要选择所需要的类型; 要选择所需要的类型; 5, 单击 Overlay File 进入图 3-3 所示图版, 所示图版,依据需要选择所需要的类型; 要的类型; 所对应曲线; 6, 选择 X,Y 所对应曲线; Run,进入图形显示窗口( ,即可完成本次交会工作 7, 单击 Run,进入图形显示窗口(图 3-4) 即可完成本次交会工作. ,即可完成本次交会工作. 注:完成以上步骤后可以根据需要对所选择曲线进行优选编辑, 需要对所选择曲线进行优选编辑, 回归. 回归. 图 3-2 图 3-3 图 3-4 第四部分常规测井资料处理技术(PVP) 常规测井资料处理技术(PVP) 对于常规资料处理模块, 两种, 对于常规资料处理模块,目前只有 PVP 和 ElanPlus 两种,在这两种模块之中,前者所需参数相对较少,应用层次明显,做法相对简单; 块之中,前者所需参数相对较少,应用层次明显,做法相对简单;而后者所需参数多,计算复杂,因此, 处理模块进行说明. 所需参数多,计算复杂,因此,本文主要对 PVP 处理模块进行说明. 第五部分一电成像的处理流程特殊资料处理(声,电成像) 特殊资料处理( 电成像) 该套软件可以处理三大测井公司的多系列电成像及井周成像资料, 电成该套软件可以处理三大测井公司的多系列电成像及井周成像资料, FMI,ARI,STARII,EMI, USI,CBIL,CAST. 像包括 FMI,ARI,STARII,EMI,井周成像包括 USI,CBIL,CAST.对于这些测井系列,其处理原理和过程基本一致, 些测井系列,其处理原理和过程基本一致,其处理框图见图 1. 17 数据的转换与加载对于斯伦贝谢的测井资料是先数据加载, 对于斯伦贝谢的测井资料是先数据加载,再通过 BHGeol Formatter 将原始数据包解压,快慢道数据分开,检查数据的完整性. 原始数据包解压,快慢道数据分开,检查数据的完整性.而对于另外两家 Converter 进行数据转换, 测井公司的资料则是先利用 BHGeol Converter 进行数据转换,再将数据加载到数据库中. 载到数据库中. 磁场及加速度数据的质量检查, 2) GPIT Survey 磁场及加速度数据的质量检查,GPIT 的正确与否是进行余下处理的关键, 进行余下处理的关键,正确的磁场资料和加速度资料其 X 和 Y 轴的分量应为中心的圆或弧. 是以 O 为中心的圆或弧. 对数据进行预处理, BorEID 对数据进行预处理,并形成静态图象资料对数据进行环境,加速度及死电极的校正, 对数据进行环境,加速度及死电极的校正,校正后的数据进行均衡处理,输出静态平衡的图象, 输出静态平衡的图象,该图象反映了整个测量井段的微电阻率的变化. 该图象反映了整个测量井段的微电阻率的变化. BorScal 微电阻率成像测井资料的刻度成像测井的微电阻率与微球测的浅电阻有一定的差别, 用浅电阻率冲 ( 成像测井的微电阻率与微球测的浅电阻有一定的差别, 洗带)刻度成像的微电阻率,使其比较准确的代表井眼周围的地层.该模刻度成像的微电阻率,使其比较准确的代表井眼周围的地层. 块只对定量计算裂缝参数时才有用, 且只适用于斯伦贝谢的成像测井资料. 块只对定量计算裂缝参数时才有用, 且只适用于斯伦贝谢的成像测井资料. BorDip 自动计算构造倾角或沉积倾角用相关对比法自动计算地层倾角.可以改变计算的窗长和步长分别求用相关对比法自动计算地层倾角. 取构造倾角和沉积倾角. 取构造倾角和沉积倾角. BorNor 图象的动态加强输出的静态图象是全井段大范围电阻率变化之间的颜色刻度, 输出的静态图象是全井段大范围电阻率变化之间的颜色刻度,由于有限的颜色资源,使静态图象不能清晰的反映井眼地质特征. 限的颜色资源,使静态图象不能清晰的反映井眼地质特征.图象的动态加强是在每0 . 5 米配一次色,这种图象可用于识别岩层中各种细微的特征米配一次色, 和构造变化. 和构造变化. BorView BorView 人机交互解释这是微电阻率扫描成像测井最关键的一步,通过人机交互解释, 关键的一步,通过人机交互解释,识别出资料上反映的各种地质特征如沉积构造,裂缝等特征, 沉积构造,裂缝等特征,并进行详细的计算及统计. 的计算及统计. 8)Data Save 数据输出将处理解释的各项结果存盘输出,并对裂缝进行定量计算. 并对裂缝进行定量计算. 19 二偶极子横波的处理流程该软件只处理斯伦贝谢的偶极子横波资料.该软件只处理斯伦贝谢的偶极子横波资料.其处理流程比较复杂, 其处理流程比较复杂,包括资料的预处理和解释处理. 资料的预处理和解释处理. (一)资料的预处理其预处理流程见图 2 主要分以下几个步骤: 主要分以下几个步骤: 1,DataLoad 数据的加载读取声波波形数据,对输入的数据进行解压, 处理,2,SWP 读取声波波形数据,对输入的数据进行解压,预处理,输出一些后处理所必需的输入参数,产生波形数据集; 些后处理所必需的输入参数,产生波形数据集; 计算出时差/时间平面上的所有相似系数值, 3,STC 计算出时差/时间平面上的所有相似系数值,通过峰值的最大相关性在平面上形成一个等高线图,在等高线图上最大相关峰值给出了时差关性在平面上形成一个等高线图, 和时间. 和时间. Sonic Labelling 声波标定计算输出的峰值矩阵中进行峰值标定,求出相应的纵, 在 STC 计算输出的峰值矩阵中进行峰值标定,求出相应的纵,横及斯通利波时差. 通利波时差. Label Editor 标定声波的编辑对提取的声波时差进行编辑. 对提取的声波时差进行编辑. 对提取的纵波,横波及斯通利波时差进行井眼补偿校正. 6,MBHC 对提取的纵波,横波及斯通利波时差进行井眼补偿校正. Vp/Vs,泊松比以及纵波,横波及斯通利波的传播时 7,SPP 用来计算 Vp/Vs,泊松比以及纵波,横波及斯通利波的传播时间. (二)解释处理偶极横波资料可以用来进行地层评价, 偶极横波资料可以用来进行地层评价,进行地层各向异性分析, 进行地层各向异性分析,岩石机械强度分析,目前我们主要有以下几个方面的应用. 械强度分析,目前我们主要有以下几个方面的应用. 斯通利波的处理应用流程具体的流程见图 3. Sonic Fracture 利用斯通利波指示裂缝的张开度斯通利波在遇到裂缝时, 斯通利波在遇到裂缝时,其透射波和反射波会发生变化, 其透射波和反射波会发生变化,利用计算的透射系数与反射系数的结合, 的结合,可以提高裂缝评价的效果, 缝评价的效果,反映裂缝的有效性,反射裂缝的有效性, 系数的大小在一定程度上反映了裂缝的张开度, 的张开度,但目前还没有岩心资料来标定,因此还只是半定量的分析. 量的分析. permeability 2) Stoneley permeability 利用斯通利波计算地层渗透率斯通利波的传播受各种因素的影响, 其中包括骨架渗透率和开口裂缝. 斯通利波的传播受各种因素的影响, 其中包括骨架渗透率和开口裂缝. 因此斯通利波的时差, 幅度衰减等异常往往和地层渗透性有很好的相关性. 因此斯通利波的时差, 幅度衰减等异常往往和地层渗透性有很好的相关性. 根据毕奥模型,利用测量的斯通利波时差与计算的弹性的斯通利波的时差根据毕奥模型, 21 来计算渗透率, 来计算渗透率,在计算弹性斯通利波时差时关键的参数是泥浆时差. 在计算弹性斯通利波时差时关键的参数是泥浆时差.另外, 另外, 利用纵波, 横波时差与地层密度合成的理论上的斯通利波时差(DTSTE)与实利用纵波, 横波时差与地层密度合成的理论上的斯通利波时差(DTSTE)与实(DTSTE) 测的斯通利波时差的差值,可以作为流体移动的指数. 测的斯通利波时差的差值,可以作为流体移动的指数. 3)Sonic Waveform Energy 利用该模块计算斯通利波的微差能量判断地层裂缝的有效性.当井壁有有效裂缝存在时,则钻井液沿着裂缝判断地层裂缝的有效性.当井壁有有效裂缝存在时, 流进或流出,从而消耗斯通利波的能量,使其幅度降低, 流进或流出,从而消耗斯通利波的能量,使其幅度降低,反之在无效缝处就不会产生能量衰减, 就不会产生能量衰减,但还要注意泥饼的影响, 泥饼的影响,因泥饼要阻止流体在裂缝中流动,微差能量越大, 裂缝中流动,微差能量越大,说明裂缝的有效性越好. 裂缝的有效性越好. 偶极横波的处理应用流程( 偶极横波的处理应用流程 ( 见。
Geoframe2012宣传册

二维地震线快速批量加载器 Easy Loader 2d
GeoViz 三维可视化解释 多层位断层混合自动解释(AVI)模式 GIS 数据显示 叠前数据显示 全新 ASAP 自动追踪算法 大数据体操作( >200GB) 多数据体联合解释 特殊体 Geobody 解释及显示 可以直接从 Seis%DV 剖面上启动
GeoFrame 数据管理功能
GeoFrame 提供了安全、快捷的数据管理功能。数据的 访问权限按照系统管理员、 工区所有者、 数据所有者三级分 别进行授权。 用户可以根据自己的身份设置工区、 数据的安 全级别。 对同一工区, 系统允许解释用户建立多个解释模型, 不同的解释员可以有不同的解释模型, 他们之间可以共享解 释方案, 也可为自己设定多套解释方案, 尤其是对于一些地 震资料解释方案具有多解性的地区, 便于解释员使用多个方 案进行优选对比。
AVI 解释模式
叠前数据显示
Geobody 三维显示
地震地质综合解释 Basemap 书签 新 Seis%DV 界面 十字光标和光标追踪范围定义 快速选到有解释数据的地震线 Geobody 及多属性体融合显示 子体及井沿井数据体交汇分析 工区底图中选择井数据、地震数据输出 InDepth 新的 Velocity set 界面
速度体与振幅体融合显示 IESX 地震综合解释平台
AVO 属性及入射角显示
叠前数据显示和交会分析
十字光标和光标追踪范围定义,方便解释方案闭合检查和对比
Basemap Plus 工区底图 底图书签 数据综合平面显示 解释数据内插 快速平面网格化作图功能 层位解释数据快速质控 底图数据快速查询
Geoframe应用教程
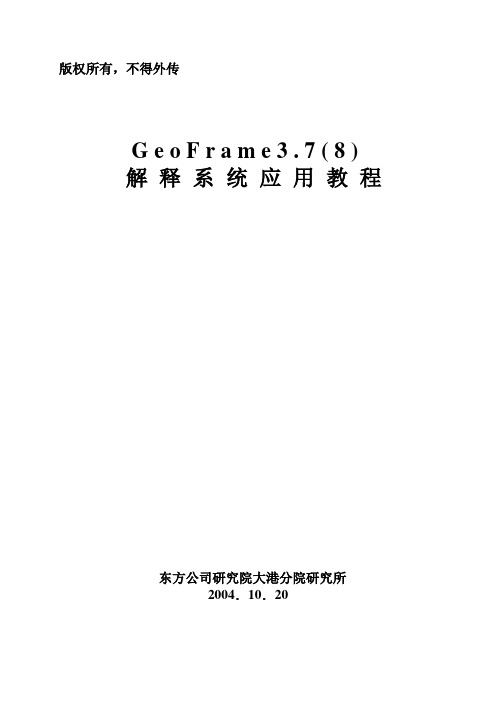
版权所有,不得外传G e o F r a m e3.7(8)解释系统应用教程东方公司研究院大港分院研究所2004.10.20目录1.剖面 (1)2.底图 (2)3.井 (2)4.地震解释 (3)5.剖面上显示和标注的补充 (5)6.有关项目的操作(在项目管理窗口) (6)7.关于数据的输入输出与管理 (7)8.合成地震记录的制作 (9)9.时间切片的产生与显示 (11)10.底图快速成图Gridding (11)1.剖面1)选择剖面:①底图上直接选;②指定线号Display→Seismic line…;③滚线:Display→Roll(往大号方向,往小号),按一定角度滚动剖面;④折线:Display→Fold vertical 折剖面(垂直方向);Display→Fold horizontal 剖面与时间切片(水平方向);⑤从底图上抽取任意线或底图上Edit→Seismic Traverse path→Simple;⑥从底图上抽取多工区任意线或底图上Edit→Seismic Traverse path→composite;⑦剖面上的切换:切相交线或剖面上Display→Intersect→;切水平切片(保证已有切片数据体)或Display→Intersect→;⑧滚动参数的设定:User→Display→Roll(可设定主测线,联络线的滚动步长;任意线的滚动方向及滚动步长。
)。
2)展剖面及剖面上的操作(剖面显示):①展剖面的标注:(包括时间线,时间标注,主测线,联络线号,道号及二维线交点等)Define→Annotation或;②设置剖面显示参数:(包括左下角标注,增益大小,时间切片的方向,地震道及充填相位的颜色等)Define→Display spec或;③剖面的放大和缩小View→Zoom和Unzoom或和;④剖面的刷新Display→Redisplay或;⑤剖面的反转(左右互调)Display→Reverse path或;⑥任意线剖面的自动延展(到工区边界)Display→Extend Path或;⑦多窗口显示Define→Layout或;⑧显示图例View→Lagend;⑨剖面的输出(cgm文件)File→Scaled plot…...;⑩剖面上展解释结果Post→Interpretation…..或。
GeoFrame模块详细技术说明
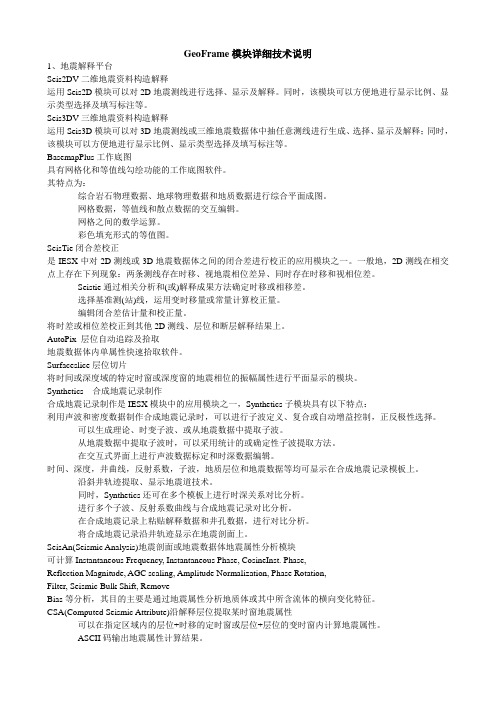
GeoFrame模块详细技术说明1、地震解释平台Seis2DV二维地震资料构造解释运用Seis2D模块可以对2D地震测线进行选择、显示及解释。
同时,该模块可以方便地进行显示比例、显示类型选择及填写标注等。
Seis3DV三维地震资料构造解释运用Seis3D模块可以对3D地震测线或三维地震数据体中抽任意测线进行生成、选择、显示及解释;同时,该模块可以方便地进行显示比例、显示类型选择及填写标注等。
BasemapPlus工作底图具有网格化和等值线勾绘功能的工作底图软件。
其特点为:综合岩石物理数据、地球物理数据和地质数据进行综合平面成图。
网格数据,等值线和散点数据的交互编辑。
网格之间的数学运算。
彩色填充形式的等值图。
SeisTie闭合差校正是IESX中对2D测线或3D地震数据体之间的闭合差进行校正的应用模块之一。
一般地,2D测线在相交点上存在下列现象:两条测线存在时移、视地震相位差异、同时存在时移和视相位差。
Seistie通过相关分析和(或)解释成果方法确定时移或相移差。
选择基准测(站)线,运用变时移量或常量计算校正量。
编辑闭合差估计量和校正量。
将时差或相位差校正到其他2D测线、层位和断层解释结果上。
AutoPix 层位自动追踪及拾取地震数据体内单属性快速拾取软件。
Surfaceslice层位切片将时间或深度域的特定时窗或深度窗的地震相位的振幅属性进行平面显示的模块。
Synthetics 合成地震记录制作合成地震记录制作是IESX模块中的应用模块之一,Synthetics子模块具有以下特点:利用声波和密度数据制作合成地震记录时,可以进行子波定义、复合或自动增益控制,正反极性选择。
可以生成理论、时变子波、或从地震数据中提取子波。
从地震数据中提取子波时,可以采用统计的或确定性子波提取方法。
在交互式界面上进行声波数据标定和时深数据编辑。
时间、深度,井曲线,反射系数,子波,地质层位和地震数据等均可显示在合成地震记录模板上。
层次聚类模型的原理

层次聚类模型是一种基于数据之间相似度进行聚类的算法,其基本原理层次聚类模型是一种基于数据之间相似度进行聚类的算法,其基本原理是通过计算不同类别数据点间的相似度来创建一棵有层次的嵌套聚类树。
该模型有两种主要的划分策略:自底向上的聚合(agglomerative)策略和自顶向下的分拆(divisive)策略。
在实际应用中,我们可以根据数据集的特性和需求选择适合的策略进行聚类分析。
聚合策略的基本思想是将每个样本视为一个簇,然后将最相似的两个簇合并为一个新的簇,如此反复执行,直到所有的样本都合并到一个簇中。
而分拆策略则是从所有样本都在同一个簇开始,然后逐渐将簇拆分成更小的簇,直到每个样本都在一个单独的簇中为止。
这两种策略都会生成一种树形的聚类结构,称为聚类树或质心树。
这种树形结构可以帮助我们更好地理解数据的结构和关系,同时也可以用于可视化展示和决策支持。
Geoframe使用手册_个人记录实战手册喷血奉献
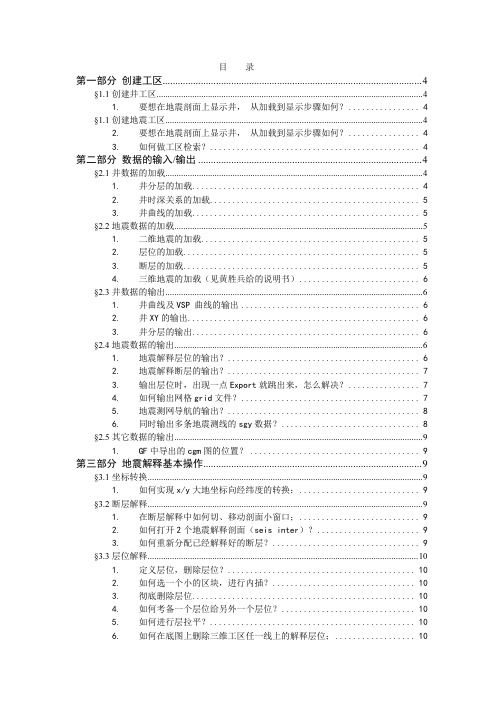
目录第一部分创建工区 (4)§1.1创建井工区 (4)1.要想在地震剖面上显示井,从加载到显示步骤如何? (4)§1.1创建地震工区 (4)2.要想在地震剖面上显示井,从加载到显示步骤如何? (4)3.如何做工区检索? (4)第二部分数据的输入/输出 (4)§2.1井数据的加载 (4)1.井分层的加载 (4)2.井时深关系的加载 (5)3.井曲线的加载 (5)§2.2地震数据的加载 (5)1.二维地震的加载 (5)2.层位的加载 (5)3.断层的加载 (5)4.三维地震的加载(见黄胜兵给的说明书) (6)§2.3井数据的输出 (6)1.井曲线及VSP 曲线的输出 (6)2.井XY的输出 (6)3.井分层的输出 (6)§2.4地震数据的输出 (6)1.地震解释层位的输出? (6)2.地震解释断层的输出? (7)3.输出层位时,出现一点Export就跳出来,怎么解决? (7)4.如何输出网格grid文件? (7)5.地震测网导航的输出? (8)6.同时输出多条地震测线的sgy数据? (8)§2.5其它数据的输出 (9)1.GF中导出的cgm图的位置? (9)第三部分地震解释基本操作 (9)§3.1坐标转换 (9)1.如何实现x/y大地坐标向经纬度的转换: (9)§3.2断层解释 (9)1.在断层解释中如何切、移动剖面小窗口; (9)2.如何打开2个地震解释剖面(seis inter)? (9)3.如何重新分配已经解释好的断层? (9)§3.3层位解释 (10)1.定义层位,删除层位? (10)2.如何选一个小的区块,进行内插? (10)3.彻底删除层位 (10)4.如何考备一个层位给另外一个层位? (10)5.如何进行层拉平? (10)6.如何在底图上删除三维工区任一线上的解释层位; (10)7.如何在底图局部删除内插层位,恢复原来网格状态; (10)§3.4井的基本操作 (10)1.要想在地震剖面上显示井,从加载到显示步骤如何? (10)2.如何看井的基本信息: (11)3.如何在basemap 上查找一口井 (11)4.如何使距离地震剖面较远的井投到地震剖面上? (11)5.如何定义井列表及井的显示及井层位的显示? (11)6.如何将井上的层位,在地震剖面上显示出来? (11)§3.5断层多边形的操作? (11)1.如何在basemap 上投断层的投影点: (11)2.如何画多边形断层 (11)3.利用断层在底图上的投影绘制断层多边形?填充着色及修改删除操作? 124.如何复制断层多边形 (12)5.将组合的断层转换到另一条断层之下 (12)§3.6地震解释技巧 (12)1.如何解决地震Path出现有的剖面空白的情况? (12)2.如何将三维中的path线存成二维线; (12)3.在三维解释中,如何定义滚动的线数? (12)4.在变面积剖面上,如何调显示振幅? (13)5.如何在底图上读长度,算面积? (13)6.如何调整底图显示的信息项(如地震path 名称等)? (13)7.解释时放大镜的使用? (13)8.如何拉连井剖面,存成文件名,如何再调用? (13)9.如何在地震剖面上把井的曲线加上去? (13)10.Bookmark的使用? (13)§3.7制作合成地震记录 (14)1.制作过程 (14)2.在做合成记录的过程中,如果出现调动后,层位不动怎么办? (14)§3.8构造图的编制 (14)3.编制等T0图: (14)4.编制深度域构造图: (14)5.如何编辑简单的厚度等值线图?如何圈定等值线的范围? (15)§3.9对数据体的操作 (16)1.如何制作层拉平体及沿层切片? (16)2.如何进行沿层相干切片的制作? (16)第四部分地震数据的操作管理 (16)§4.1工区数据的共享 (16)1.如何进行其它工区的数据共享工作; (16)§4.2二维测线survey的管理 (17)1.如何进行地震二维数据测线的survey的管理 (17)2.如何把已存在的要seismic path地震测线删除? (17)3.在basemap中如何锁定地震测网或图层 (17)4.如何更改数据的Class; (17)5.在Basemap如何删除某个survey的地震测网或图层; (17)第五部分GeoFrame 中的三维立体显示 (17)如何将属性、地震数据及解释层位文体显示在一张图上。
1_2_DM_Syn_IESX(斯伦贝谢软件地震模块)
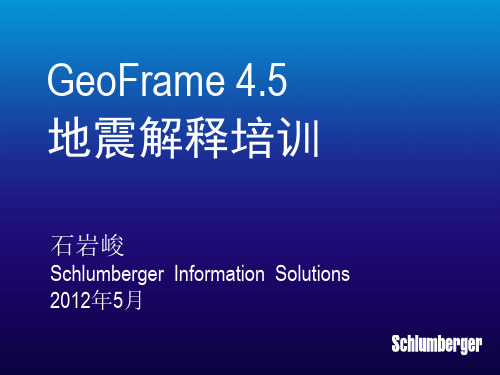
。
时变子波
不同的时间段(时窗)内选择不同的子波
Tools → Wavelet→ Time Varying…
时变子波
合成记录
Post → Synthetics…
显示井旁地震道
Post → Seismic…
时间范围
选道方式
选工区
道范围
选属性 显示顺序
保存合成记录
Data → General Data Manager…
3D数据关键是线号/line number和共中心点号/CDP所在字节; 2D数据关键是炮号/SP number和共中心点号/CDP所在字节。
数据实例:
Crossline
Inline
扫描数据
坐标 坐标 输入实际保存 位置。
创建加载定义文件:*.ldfx
名词释义
Survey:地震测网,定义了地震采集在地表上分布 的位置、范围等信息; Class:地震数据体的名字,一般可以标识数据的位 数(8,16,32)、属性(amplitude,variance)、处 理方法(stack,migration,etc)等信息。 一个工区/project里可有多个survey,但不能重名; 一个survey里可以有多个class,不能重名; 一个class名字也可以被多个survey使用。
全球客户自助平台:https:///
SIS中文网站:/
课程安排
Day
1 2 3
Application Module
Description
Data Loading & Management
数据加载及数据管理
Synthetics
(t ) t 1 2 2 3 1
Geomodeling_VVA 软件技术附件
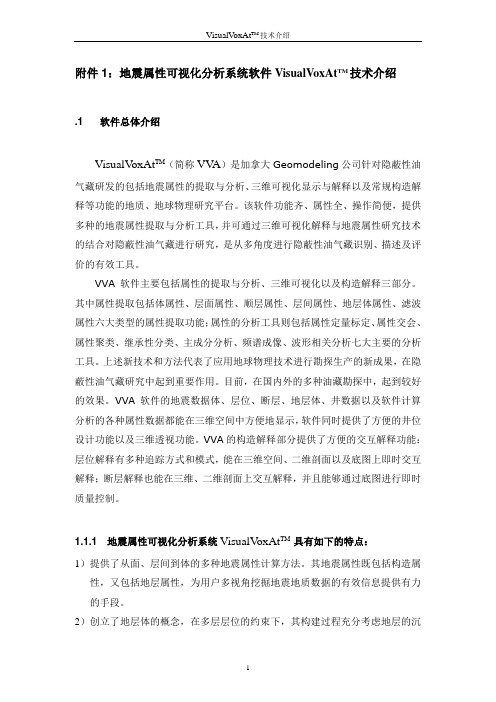
附件1:地震属性可视化分析系统软件VisualVoxAt TM技术介绍.1 软件总体介绍VisualV oxAt TM(简称VV A)是加拿大Geomodeling公司针对隐蔽性油气藏研发的包括地震属性的提取与分析、三维可视化显示与解释以及常规构造解释等功能的地质、地球物理研究平台。
该软件功能齐、属性全、操作简便,提供多种的地震属性提取与分析工具,并可通过三维可视化解释与地震属性研究技术的结合对隐蔽性油气藏进行研究,是从多角度进行隐蔽性油气藏识别、描述及评价的有效工具。
VVA软件主要包括属性的提取与分析、三维可视化以及构造解释三部分。
其中属性提取包括体属性、层面属性、顺层属性、层间属性、地层体属性、滤波属性六大类型的属性提取功能;属性的分析工具则包括属性定量标定、属性交会、属性聚类、继承性分类、主成分分析、频谱成像、波形相关分析七大主要的分析工具。
上述新技术和方法代表了应用地球物理技术进行勘探生产的新成果,在隐蔽性油气藏研究中起到重要作用。
目前,在国内外的多种油藏勘探中,起到较好的效果。
VVA软件的地震数据体、层位、断层、地层体、井数据以及软件计算分析的各种属性数据都能在三维空间中方便地显示,软件同时提供了方便的井位设计功能以及三维透视功能。
VVA的构造解释部分提供了方便的交互解释功能:层位解释有多种追踪方式和模式,能在三维空间、二维剖面以及底图上即时交互解释;断层解释也能在三维、二维剖面上交互解释,并且能够通过底图进行即时质量控制。
1.1.1地震属性可视化分析系统VisualV oxAt TM具有如下的特点:1)提供了从面、层间到体的多种地震属性计算方法。
其地震属性既包括构造属性,又包括地层属性,为用户多视角挖掘地震地质数据的有效信息提供有力的手段。
2)创立了地层体的概念,在多层层位的约束下,其构建过程充分考虑地层的沉积在平面和空间上的变化。
地层体切片比时间切片更好地反映地质规律。
各种属性以及分析方法都可以应用到地层体中,使得对隐蔽性油气藏识别、描述和评价更具目的性。
系统聚类类平均法-概述说明以及解释

系统聚类类平均法-概述说明以及解释1.引言1.1 概述概述部分的内容可以介绍系统聚类类平均法的基本概念和一些相关背景知识。
下面是一个概述部分的示例:在数据分析和机器学习领域,聚类是一种常见的数据分析方法,用于将一组数据样本划分为相似的子集或群集。
系统聚类类平均法(hierarchical clustering)是聚类的一种常用方法之一。
它的主要目标是通过测量不同数据样本之间的相似度或距离来构建层级结构的聚类结果。
系统聚类类平均法的基本思想是通过合并或分割数据样本来构建聚类层级。
聚类的过程可以看作是在一个树状结构上不断合并最相似的样本或群集,直到所有的样本都合并到一个群集中或满足某个停止准则为止。
这样就能得到一个层次化的聚类结果,可以直观地展示数据样本之间的相似度和差异。
系统聚类类平均法有两种常见的形式:凝聚(agglomerative)和分裂(divisive)。
凝聚聚类从每个数据样本开始,逐步合并相似的样本或群集,直到所有样本都合并为一个群集。
分裂聚类则从一个包含所有数据样本的群集开始,逐步将其分割成更小的子群集,直到每个样本都是一个单独的群集。
系统聚类类平均法具有一些较强的特点,例如灵活性、可解释性和可视化效果好等。
由于它能够提供一个层次化的聚类结果,研究人员可以根据需要选择不同层次的聚类结果进行数据分析和模式发现。
此外,在大规模数据处理时,系统聚类类平均法的计算效率也比较高。
本文将深入探讨系统聚类类平均法的原理和应用,并通过实例解释其具体操作步骤。
希望通过阅读本文,读者能够更好地理解系统聚类类平均法,并在实际应用中灵活运用。
1.2 文章结构文章结构部分应该包含以下内容:文章结构部分的目的是为读者提供一个关于整篇文章的概览,明确每个部分的主题和内容,以帮助读者更好地理解和组织所阐述的内容。
在本篇文章中,主要分为以下几个部分来组织论述:第一部分是引言。
引言是整篇文章的开端,通过概述系统聚类类平均法的背景和意义来引入该主题。
squeeze-excitation结构
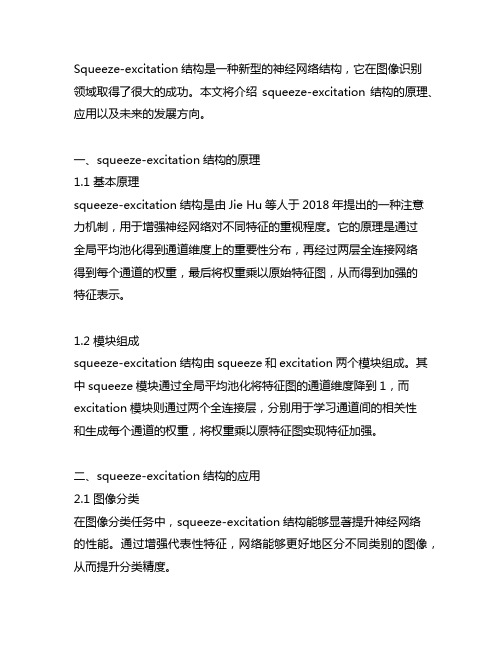
Squeeze-excitation结构是一种新型的神经网络结构,它在图像识别领域取得了很大的成功。
本文将介绍squeeze-excitation结构的原理、应用以及未来的发展方向。
一、squeeze-excitation结构的原理1.1 基本原理squeeze-excitation结构是由Jie Hu等人于2018年提出的一种注意力机制,用于增强神经网络对不同特征的重视程度。
它的原理是通过全局平均池化得到通道维度上的重要性分布,再经过两层全连接网络得到每个通道的权重,最后将权重乘以原始特征图,从而得到加强的特征表示。
1.2 模块组成squeeze-excitation结构由squeeze和excitation两个模块组成。
其中squeeze模块通过全局平均池化将特征图的通道维度降到1,而excitation模块则通过两个全连接层,分别用于学习通道间的相关性和生成每个通道的权重,将权重乘以原特征图实现特征加强。
二、squeeze-excitation结构的应用2.1 图像分类在图像分类任务中,squeeze-excitation结构能够显著提升神经网络的性能。
通过增强代表性特征,网络能够更好地区分不同类别的图像,从而提升分类精度。
2.2 目标检测在目标检测任务中,squeeze-excitation结构也取得了不俗的表现。
通过引入注意力机制,网络能够更加关注重要的目标特征,从而提升目标检测的准确性和鲁棒性。
2.3 语义分割在语义分割任务中,squeeze-excitation结构能够帮助神经网络更好地理解图像内容,从而提升分割的准确性和细节性。
三、squeeze-excitation结构的未来发展方向3.1 结合其他模块未来,可以考虑将squeeze-excitation结构与其他模块相结合,提升神经网络的性能。
比如结合注意力机制、残差连接等,构建更加强大的神经网络结构。
3.2 应用于更多领域除了图像识别领域,squeeze-excitation结构还可以应用于自然语言处理、医学影像分析等领域。
Geoframe属性(word文档良心出品)
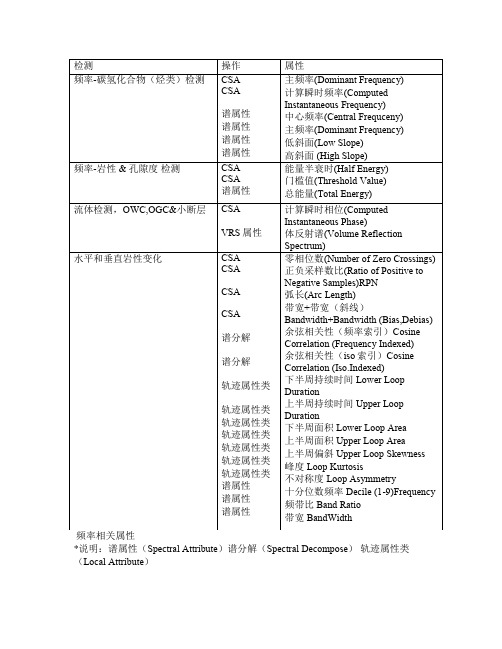
*说明:谱属性(Spectral Attribute)谱分解(Spectral Decompose)轨迹属性类(Local Attribute)*瞬时频率(Inst Frequency ):定义为瞬时相位对时间的导数,用Hz 表示。
经常用来估计地震振幅的衰减,往往油气的存在引起高频成分的衰减,可用这一属性检测油气。
瞬时相位(Inst Phase ):表示在所选样点上各道的相位值,以度或弧度表示。
主要用于增强油藏内弱同相轴,对噪音也有放大作用,最终成图的彩色色标应考虑到结果的周期性,即:由于油气的存在经常引起相位的局部变化,所以这一属性常和其它属性一起用作油气检测的指标之一,也可用于测定薄层的相位特征,其横向变反射强度(Reflection Magnitudes ):反映了岩性差异、地层连续、地层空间、孔隙度的变化。
反(负)二阶微商变换(Negative of Second Derivative ) :显著地提升了连续性,有助于更快、更准确的层位解释。
道积分(Integrated Seismic Trace ):能起到伪波阻抗剖面的作用. 并不是说用它替代反演, 它可以起到快速指示孔隙度变化的作用.谱分解技术(Spectral Decomposition )—— 分频:用于揭示薄层岩性横向的变化,指示可能的含烃地层圈闭。
最后分频属性和井砂岩厚度结合作出目标层段的砂岩厚度图。
由于不同频率段所看到的东西是有区别的,所以分频还可以观察到河道的形状更清晰,河道内的岩性细节变化。
砂岩厚度图流程图:Find the Power Spectrum usingSYNTHETICS Extract Tuning FrequencySATK Run Spectral DecompositionSATK Net Thickness DeterminationCorrelate using LPM等频体(Iso Frequency):结果是一个某一特定频率的相关数据体。
petrel地质建模软件的wrkflows教程
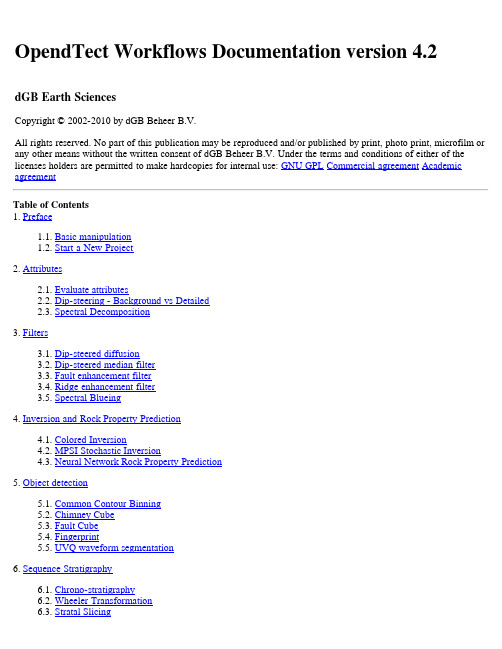
This document describes various work flows in OpendTect + plugins. We describe the purpose, what software is needed (OpendTect only or commercial plugins), and how to do it. The workflows are given in the form of a bullet list describing the sequential steps to be taken. Where possible, links to the User doc and the OpendTect and dGB websites are given with further information. For the latter links to work, you need access to the Internet.For more info, see this Tutorial video: Basic interaction (flash video)For more info, see this Start new project (flash video)Attribute analysis is one of the key functionalities in OpendTect. Attributes are used for two purposes:1. Visualization: you create a new view of the data that may lead to new geologic insight.2. Integration of informationTo use attributes, you must define an attribute set (For more info, see this Evaluate attributes (flash video)Filters in OpendTect are implemented as Attributes that need to be defined in the attribute set window. Filters with a user interface are grouped under Filters. This group includes convolution, frequency filters, gap decon, and velocity fan filters. Filters without a user interface are filters that are constructed from (chains of) attributes. For example, using Reference Shift, Volume Statistics, and Mathematics, you can extract and manipulate data to construct your own filters. This group of filters contains, among others: dip-steered median filter, dip-steered diffusion filter, fault enhancement filter, and ridge-enhancement filter. A number of these filters are delivered with the system asFor more info, see thisDip steered median filter (flash video)For more info, see thisSpectral Blueing and Colored Inversion (flash video)OpendTect offers various plugins for quantitative seismic interpretation.fast way to convert seismic data to band-limited acoustic impedance. Full-bandwidthinversion is offered in plugins with the same names by Earthworks and Ark cls. Using Neural Networks, it is possible to convert seismic information (e.g. acoustic and/or elastic impedance) to rock properties (e.g. Porosity, Vshale etc). The supervised network is trained along well tracks to find the (non-linear) relationship between seismic and well logs.For more info, see thisMPSI stochastic inversion (flash video)Seismic attributes are used to visualize data such that relevant information becomes easier to interpret. However, calculating many attributes leads to a data explosion and confusion:interesting attributes into one output representing the optimal view?attributes that are combined in an intelligent way. In OpendTect you can create meta-attributes using math and logic (Mathematics attribute in OpendTect), neural networks (commercial plugin), and using the fingerprint attribute (OpendTect).For more info, see thisCommon Contour Binning (flash video)For more info, see this Chimney Cube (flash video)Seismic sequence stratigraphic interpretationand (2) predicting potential stratigraphic trapsthis domain. In SSIS all possible horizons are tracked (data-driven mode) or modeled (interpolated between mapped horizons, or shifted parallel to upper or lower bounding surface). Each horizon is a chrono-stratigraphic event that can be used to reconstruct the depositional history (chrono-strat slider), to flatten seismic data and attributes (Wheeler transform), and to interpret system tracts (relating units to the relative sea level curve).For more info, see this Chrono-stratigraphy (flash video)For more info, see thisSSIS interpretation (flash video)。
Geoeast属性分析说明书
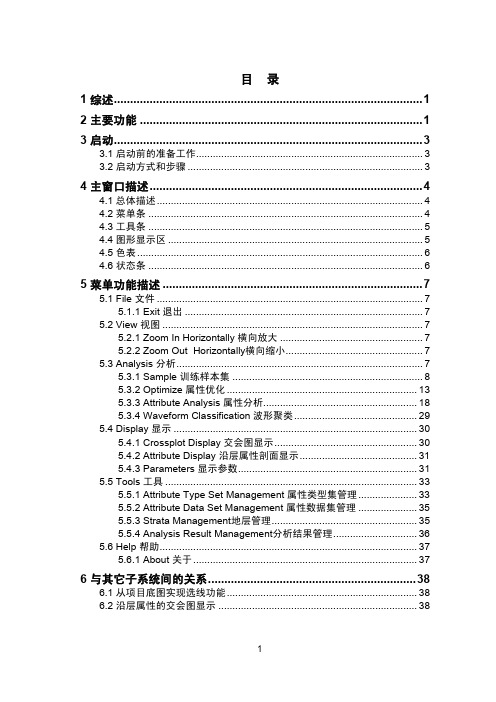
体属性处理
层属性提取
属性相关对比
层属性优化处理
无样本分析
综合分析
有别
BP 神经网络
地质资料 岩性物性 交会图转换图板
参数物性转换
平面显示
剖面显示
图 2-1
2
3 启动
GeoAttributeAnalysis 地震属性分析
3.1 启动前的准备工作
在启动三维地震属性分析子系统前,需要做以下准备工作:建立项目、建立工 区、加载测量成果数据、地震数据、完成层位、断层解释和属性提取。
本子系统将采用监督条件下和非监督条件下的模式识别、人工神经网络等先进技 术,配以属性交会图等工具的支持,可实现多参数分析、多目标优化等功能,可以实 现在只有较少井资料的控制下,主要靠三维地震数据中的信息进行全工区的储层分析 和预测,尤其适用于油藏描述的早期,充分利用地震资料,获取对油藏较准确的认 识,对缩短勘探周期,提高探井成功率,降低勘探风险和成本具有重要作用。
5.4 Display 显示 ....................................................................................... 30 5.4.1 Crossplot Display 交会图显示................................................... 30 5.4.2 Attribute Display 沿层属性剖面显示.......................................... 31 5.4.3 Parameters 显示参数................................................................ 31
- 1、下载文档前请自行甄别文档内容的完整性,平台不提供额外的编辑、内容补充、找答案等附加服务。
- 2、"仅部分预览"的文档,不可在线预览部分如存在完整性等问题,可反馈申请退款(可完整预览的文档不适用该条件!)。
- 3、如文档侵犯您的权益,请联系客服反馈,我们会尽快为您处理(人工客服工作时间:9:00-18:30)。
非约束聚类算法
K-Means lustering Method
K-Means 聚类是一种统计学算法,首先在属 性空间内根据用户定义的分类数目任意的为 每一类选择一个聚类中心。由于开始聚类中 心选择的任意性,因此该计算不能总是收敛 于一个解决方案,但对于输入数据相同的多 个操作,其最终结果是非常相似的。 Error Limit是可供选择的唯一的一个控制参 数,当聚类中心间的平均值小于该参数时, 聚类完成。当将Error Limit参数设置较低时, 会提高聚类的精度,但会增加计算的时间。
Learning Rate:控制神经网络调整权重的速度,该权重是将数据点归到其合适类中的参数。 学习速率高,权重更新的量就大。在聚类过程的早期是可行的,但在后期会造成稳定性问 题。在聚类过程后期,计算接近于最终结果,权重的大幅波动会导致不稳定。 Beta :是用于限制或避免conscience参数随机波动的参数。
地震相分析工作流程
Exercise
谢谢大家!
Bayesian Method
Bayesian方法也是一种统计学聚类方法。该方法需要所有数据点为高斯分布, 每类数据具有不同的协方差矩阵。基于属性空间中的向量计算每个点属于某一 类的概率。具有较高所属概率的点分配给该类。Bayesian算法不需要参数设置。 但在Classes文件夹中可以设置聚类过程中用于训练的训练数据的比例,以及用 于计算网络误差的比例。
SeisClass 地震相聚类分析
Schlumberger Information Solutions 2011年12月
聚类算法
SeisClass中包括两类聚类算法:非约束聚类 和约束聚类。 非约束聚类包括两种方法: • K-Means Clustering; • Competitive Learning。 该聚类方法基于属性空间内全部数据集, 其结果没有依靠对数据的先验认识。 约束聚类包括两种方法: • Fisher’s Discriminate ; • Bayesian; • Back Propagation。 对数据的先验知识是该聚类方法的基础。 在约束聚类中,使用从井得到的信息来指 导离井较远地区的聚类。
非约束聚类算法
Competitive Learning Method
Competitive Learning聚类是一种神经网络算法。与K-Means 算法类似,该算法不需要对数 据有先验认识,用户只需定义需要创建的类别数目。使用Competitive这个词是因为在该算 法中聚类中心需要对所属数据点进行竞争。聚类中心的最初位置并不像K-Means 算法一样 是任意给定的,而是通过算法来选择的。 Number of Iterations:控制迭代次数。缺省设置为100,最大为2000。迭代次数过高虽然可 时算法更精确,但会损害聚类的总体面貌。当迭代次数太高时,对于大数据其运行时间会 很长。
Conscience:参数用于阻止输出的某一类将太多的数据划给自己。因此占有太多输入数据 点的类别会被给予较差的conscience,并且暂时退出对新数据点的竞争。目的是在聚类结果 的网格中输出数据点产生一个较均衡的分布,避免一类赢得太多数据。高值将会降低聚类 的过程。
约束聚类算法
Fisher’s Discriminant Method
Fisher判别方法是一种统计学聚类方法。该方法不需要设置参数,是约束聚类方 法中最快的算法。该方法假定所有类具有相同的协方差矩阵,且在属性空间内 其最初分布是相等的。该算法的目的是通过将N维(N为分类数)数据投影到一 维平面上,从而使不同类别间的距离最大化,然后所有数据点分配给离其最近 的类。该方法对于每类数据分布距离相等情况下效果较好 。
约束聚类算法
Back Error Propagation Method
Back Error Propagation方法是一种神经网络聚类方法。该方法对输入数据未做假定,而是试图通过用户 定义的训练数据不断减小实际网络与输出层(分类)之间的不匹配。该方法使用梯度下降方法来减小 误差函数,避免产生局部极小值相关的问题。误差通过网络反向传播,权重系数不断更新。 参数设置包括: • Max Epochs : 每一输入/输出数据对权重更新后,一个间隔就完成了。换言之,当所有训练数据提供 给网络一次,一个间隔就完成了。有效的反响传播需要进行提供多次数据。缺省设置为100,最大值为 1000。 • Error Limit :定义输出网络中均方差误差的最小容忍值,用于中止训练状态。 • Update Interval:在权重系数更新前,提供给网络的训练数据个数。缺省设置为100,最大值为1000。 低值会降低学习时间,但存在训练结果不稳定的风险。 • Flexibility:在0和1之间变化,缺省设置为0.5。该参数控制了隐藏层中节点的个数。其数值小时,隐 藏层节点少,将从输入数据中获得一个总体的趋势。高值将使网络变得更细化。如果有多种属性及多 个类别,将该参数设为低值,反之亦然。 • TestData:在0和50%之间变化,缺省设置为0。该参数与Bayesian方法中的比例参数类似。如果该参数 大于0,则有部分输入数据不参与训练,而用于计算网格误差。训练完成后,测试数据中最低误差的网 个数据用于聚类计算。