A multi-interval chebyshev collocation method for efficient high-accuracy rf circuit simula
统计学专业术语英汉词典
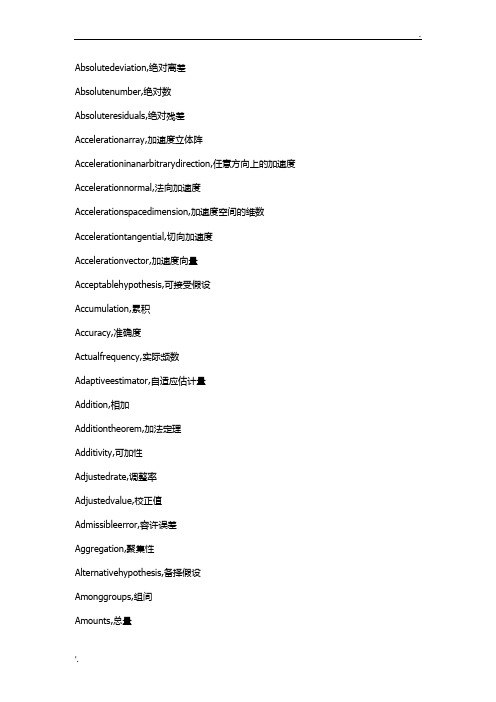
Absolutedeviation,绝对离差Absolutenumber,绝对数Absoluteresiduals,绝对残差Accelerationarray,加速度立体阵Accelerationinanarbitrarydirection,任意方向上的加速度Accelerationnormal,法向加速度Accelerationspacedimension,加速度空间的维数Accelerationtangential,切向加速度Accelerationvector,加速度向量Acceptablehypothesis,可接受假设Accumulation,累积Accuracy,准确度Actualfrequency,实际频数Adaptiveestimator,自适应估计量Addition,相加Additiontheorem,加法定理Additivity,可加性Adjustedrate,调整率Adjustedvalue,校正值Admissibleerror,容许误差Aggregation,聚集性Alternativehypothesis,备择假设Amonggroups,组间Amounts,总量Analysisofcorrelation,相关分析Analysisofcovariance,协方差分析Analysisofregression,回归分析Analysisoftimeseries,时间序列分析Analysisofvariance,方差分析Angulartransformation,角转换ANOVA(analysisofvariance),方差分析ANOVAModels,方差分析模型Arcing,弧/弧旋Arcsinetransformation,反正弦变换Areaunderthecurve,曲线面积AREG,评估从一个时间点到下一个时间点回归相关时的误差ARIMA,季节和非季节性单变量模型的极大似然估计Arithmeticgridpaper,算术格纸Arithmeticmean,算术平均数Arrheniusrelation,艾恩尼斯关系Assessingfit,拟合的评估Associativelaws,结合律Asymmetricdistribution,非对称分布Asymptoticbias,渐近偏倚Asymptoticefficiency,渐近效率Asymptoticvariance,渐近方差Attributablerisk,归因危险度Attributedata,属性资料Attribution,属性Autocorrelation,自相关Autocorrelationofresiduals,残差的自相关Average,平均数Averageconfidenceintervallength,平均置信区间长度Averagegrowthrate,平均增长率Barchart,条形图Bargraph,条形图Baseperiod,基期Bayes'theorem,Bayes定理Bell-shapedcurve,钟形曲线Bernoullidistribution,伯努力分布Best-trimestimator,最好切尾估计量Bias,偏性Binarylogisticregression,二元逻辑斯蒂回归Binomialdistribution,二项分布Bisquare,双平方BivariateCorrelate,二变量相关Bivariatenormaldistribution,双变量正态分布Bivariatenormalpopulation,双变量正态总体Biweightinterval,双权区间BiweightM-estimator,双权M估计量Block,区组/配伍组BMDP(Biomedicalcomputerprograms),BMDP统计软件包Boxplots,箱线图/箱尾图Breakdownbound,崩溃界/崩溃点Canonicalcorrelation,典型相关Caption,纵标目Case-controlstudy,病例对照研究Categoricalvariable,分类变量Catenary,悬链线Cauchydistribution,柯西分布Cause-and-effectrelationship,因果关系Cell,单元Censoring,终检Centerofsymmetry,对称中心Centeringandscaling,中心化和定标Centraltendency,集中趋势Centralvalue,中心值CHAID-χ2AutomaticInteractionDetector,卡方自动交互检测Chance,机遇Chanceerror,随机误差Chancevariable,随机变量Characteristicequation,特征方程Characteristicroot,特征根Characteristicvector,特征向量Chebshevcriterionoffit,拟合的切比雪夫准则Chernofffaces,切尔诺夫脸谱图Chi-squaretest,卡方检验/χ2检验Choleskeydecomposition,乔洛斯基分解Circlechart,圆图Classinterval,组距Classmid-value,组中值Classupperlimit,组上限Classifiedvariable,分类变量Clusteranalysis,聚类分析Clustersampling,整群抽样Code,代码Codeddata,编码数据Coding,编码Coefficientofcontingency,列联系数Coefficientofdetermination,决定系数Coefficientofmultiplecorrelation,多重相关系数Coefficientofpartialcorrelation,偏相关系数Coefficientofproduction-momentcorrelation,积差相关系数Coefficientofrankcorrelation,等级相关系数Coefficientofregression,回归系数Coefficientofskewness,偏度系数Coefficientofvariation,变异系数Cohortstudy,队列研究Column,列Columneffect,列效应Columnfactor,列因素Combinationpool,合并Combinativetable,组合表Commonfactor,共性因子Commonregressioncoefficient,公共回归系数Commonvalue,共同值Commonvariance,公共方差Commonvariation,公共变异Communalityvariance,共性方差Comparability,可比性Comparisonofbathes,批比较Comparisonvalue,比较值Compartmentmodel,分部模型Compassion,伸缩Complementofanevent,补事件Completeassociation,完全正相关Completedissociation,完全不相关Completestatistics,完备统计量Completelyrandomizeddesign,完全随机化设计Compositeevent,联合事件Compositeevents,复合事件Concavity,凹性Conditionalexpectation,条件期望Conditionallikelihood,条件似然Conditionalprobability,条件概率Conditionallylinear,依条件线性Confidenceinterval,置信区间Confidencelimit,置信限Confidencelowerlimit,置信下限Confidenceupperlimit,置信上限ConfirmatoryFactorAnalysis,验证性因子分析Confirmatoryresearch,证实性实验研究Confoundingfactor,混杂因素Conjoint,联合分析Consistency,相合性Consistencycheck,一致性检验Consistentasymptoticallynormalestimate,相合渐近正态估计Consistentestimate,相合估计Constrainednonlinearregression,受约束非线性回归Constraint,约束Contaminateddistribution,污染分布ContaminatedGausssian,污染高斯分布Contaminatednormaldistribution,污染正态分布Contamination,污染Contaminationmodel,污染模型Contingencytable,列联表Contour,边界线Contributionrate,贡献率Control,对照Controlledexperiments,对照实验Conventionaldepth,常规深度Convolution,卷积Correctedfactor,校正因子Correctedmean,校正均值Correctioncoefficient,校正系数Correctness,正确性Correlationcoefficient,相关系数Correlationindex,相关指数Correspondence,对应Counting,计数Counts,计数/频数Covariance,协方差Covariant,共变CoxRegression,Cox回归Criteriaforfitting,拟合准则Criteriaofleastsquares,最小二乘准则Criticalratio,临界比Criticalregion,拒绝域Criticalvalue,临界值Cross-overdesign,交叉设计Cross-sectionanalysis,横断面分析Cross-sectionsurvey,横断面调查Crosstabs,交叉表Cross-tabulationtable,复合表Cuberoot,立方根Cumulativedistributionfunction,分布函数Cumulativeprobability,累计概率Curvature,曲率/弯曲Curvature,曲率Curvefit,曲线拟和Curvefitting,曲线拟合Curvilinearregression,曲线回归Curvilinearrelation,曲线关系Cut-and-trymethod,尝试法Cycle,周期Cyclist,周期性Dtest,D检验Dataacquisition,资料收集Databank,数据库Datacapacity,数据容量Datadeficiencies,数据缺乏Datahandling,数据处理Datamanipulation,数据处理Dataprocessing,数据处理Datareduction,数据缩减Dataset,数据集Datasources,数据来源Datatransformation,数据变换Datavalidity,数据有效性Data-in,数据输入Data-out,数据输出Deadtime,停滞期Degreeoffreedom,自由度Degreeofprecision,精密度Degreeofreliability,可靠性程度Degression,递减Densityfunction,密度函数Densityofdatapoints,数据点的密度Dependentvariable,应变量/依变量/因变量Dependentvariable,因变量Depth,深度Derivativematrix,导数矩阵Derivative-freemethods,无导数方法Design,设计Determinacy,确定性Determinant,行列式Determinant,决定因素Deviation,离差Deviationfromaverage,离均差Diagnosticplot,诊断图Dichotomousvariable,二分变量Differentialequation,微分方程Directstandardization,直接标准化法Discretevariable,离散型变量DISCRIMINANT,判断Discriminantanalysis,判别分析Discriminantcoefficient,判别系数Discriminantfunction,判别值Dispersion,散布/分散度Disproportional,不成比例的Disproportionatesub-classnumbers,不成比例次级组含量Distributionfree,分布无关性/免分布Distributionshape,分布形状Distribution-freemethod,任意分布法Distributivelaws,分配律Disturbance,随机扰动项Doseresponsecurve,剂量反应曲线Doubleblindmethod,双盲法Doubleblindtrial,双盲试验Doubleexponentialdistribution,双指数分布Doublelogarithmic,双对数Downwardrank,降秩Dual-spaceplot,对偶空间图DUD,无导数方法Duncan'snewmultiplerangemethod,新复极差法/Duncan新法Effect,实验效应Eigenvalue,特征值Eigenvector,特征向量Ellipse,椭圆Empiricaldistribution,经验分布Empiricalprobability,经验概率单位Enumerationdata,计数资料Equalsun-classnumber,相等次级组含量Equallylikely,等可能Equivariance,同变性Error,误差/错误Errorofestimate,估计误差ErrortypeI,第一类错误ErrortypeII,第二类错误Estimand,被估量Estimatederrormeansquares,估计误差均方Estimatederrorsumofsquares,估计误差平方和Euclideandistance,欧式距离Event,事件Event,事件Exceptionaldatapoint,异常数据点Expectationplane,期望平面Expectationsurface,期望曲面Expectedvalues,期望值Experiment,实验Experimentalsampling,试验抽样Experimentalunit,试验单位Explanatoryvariable,说明变量Exploratorydataanalysis,探索性数据分析ExploreSummarize,探索-摘要Exponentialcurve,指数曲线Exponentialgrowth,指数式增长EXSMOOTH,指数平滑方法Extendedfit,扩充拟合Extraparameter,附加参数Extrapolation,外推法Extremeobservation,末端观测值Extremes,极端值/极值Fdistribution,F分布Ftest,F检验Factor,因素/因子Factoranalysis,因子分析FactorAnalysis,因子分析Factorscore,因子得分Factorial,阶乘Factorialdesign,析因试验设计Falsenegative,假阴性Falsenegativeerror,假阴性错误Familyofdistributions,分布族Familyofestimators,估计量族Fanning,扇面Fatalityrate,病死率Fieldinvestigation,现场调查Fieldsurvey,现场调查Finitepopulation,有限总体Finite-sample,有限样本Firstderivative,一阶导数Firstprincipalcomponent,第一主成分Firstquartile,第一四分位数Fisherinformation,费雪信息量Fittedvalue,拟合值Fittingacurve,曲线拟合Fixedbase,定基Fluctuation,随机起伏Forecast,预测Fourfoldtable,四格表Fourth,四分点Fractionblow,左侧比率Fractionalerror,相对误差Frequency,频率Frequencypolygon,频数多边图Frontierpoint,界限点Functionrelationship,泛函关系Gammadistribution,伽玛分布Gaussincrement,高斯增量Gaussiandistribution,高斯分布/正态分布Gauss-Newtonincrement,高斯-牛顿增量Generalcensus,全面普查GENLOG(Generalizedlinermodels),广义线性模型Geometricmean,几何平均数Gini'smeandifference,基尼均差GLM(Generallinermodels),通用线性模型Goodnessoffit,拟和优度/配合度Gradientofdeterminant,行列式的梯度Graeco-Latinsquare,希腊拉丁方Grandmean,总均值Grosserrors,重大错误Gross-errorsensitivity,大错敏感度Groupaverages,分组平均Groupeddata,分组资料Guessedmean,假定平均数Half-life,半衰期HampelM-estimators,汉佩尔M估计量Happenstance,偶然事件Harmonicmean,调和均数Hazardfunction,风险均数Hazardrate,风险率Heading,标目Heavy-taileddistribution,重尾分布Hessianarray,海森立体阵Heterogeneity,不同质Heterogeneityofvariance,方差不齐Hierarchicalclassification,组内分组Hierarchicalclusteringmethod,系统聚类法High-leveragepoint,高杠杆率点HILOGLINEAR,多维列联表的层次对数线性模型Hinge,折叶点Histogram,直方图Historicalcohortstudy,历史性队列研究Holes,空洞HOMALS,多重响应分析Homogeneityofvariance,方差齐性Homogeneitytest,齐性检验HuberM-estimators,休伯M估计量Hyperbola,双曲线Hypothesistesting,假设检验Hypotheticaluniverse,假设总体Impossibleevent,不可能事件Independence,独立性Independentvariable,自变量Index,指标/指数Indirectstandardization,间接标准化法Individual,个体Inferenceband,推断带Infinitepopulation,无限总体Infinitelygreat,无穷大Infinitelysmall,无穷小Influencecurve,影响曲线Informationcapacity,信息容量Initialcondition,初始条件Initialestimate,初始估计值Initiallevel,最初水平Interaction,交互作用Interactionterms,交互作用项Intercept,截距Interpolation,内插法Interquartilerange,四分位距Intervalestimation,区间估计Intervalsofequalprobability,等概率区间Intrinsiccurvature,固有曲率Invariance,不变性Inversematrix,逆矩阵Inverseprobability,逆概率Inversesinetransformation,反正弦变换Iteration,迭代Jacobiandeterminant,雅可比行列式Jointdistributionfunction,分布函数Jointprobability,联合概率Jointprobabilitydistribution,联合概率分布Kmeansmethod,逐步聚类法Kaplan-Meier,评估事件的时间长度Kaplan-Merierchart,Kaplan-Merier图Kendall'srankcorrelation,Kendall等级相关Kinetic,动力学Kolmogorov-Smirnovetest,柯尔莫哥洛夫-斯米尔诺夫检验KruskalandWallistest,Kruskal及Wallis检验/多样本的秩和检验/H检验Kurtosis,峰度Lackoffit,失拟Ladderofpowers,幂阶梯Lag,滞后Largesample,大样本Largesampletest,大样本检验Latinsquare,拉丁方Latinsquaredesign,拉丁方设计Leakage,泄漏Leastfavorableconfiguration,最不利构形Leastfavorabledistribution,最不利分布Leastsignificantdifference,最小显著差法Leastsquaremethod,最小二乘法Least-absolute-residualsestimates,最小绝对残差估计Least-absolute-residualsfit,最小绝对残差拟合Least-absolute-residualsline,最小绝对残差线Legend,图例L-estimator,L估计量L-estimatoroflocation,位置L估计量L-estimatorofscale,尺度L估计量Level,水平Lifeexpectance,预期期望寿命Lifetable,寿命表Lifetablemethod,生命表法Light-taileddistribution,轻尾分布Likelihoodfunction,似然函数Likelihoodratio,似然比linegraph,线图Linearcorrelation,直线相关Linearequation,线性方程Linearprogramming,线性规划Linearregression,直线回归LinearRegression,线性回归Lineartrend,线性趋势Loading,载荷Locationandscaleequivariance,位置尺度同变性Locationequivariance,位置同变性Locationinvariance,位置不变性Locationscalefamily,位置尺度族Logranktest,时序检验Logarithmiccurve,对数曲线Logarithmicnormaldistribution,对数正态分布Logarithmicscale,对数尺度Logarithmictransformation,对数变换Logiccheck,逻辑检查Logisticdistribution,逻辑斯特分布Logittransformation,Logit转换LOGLINEAR,多维列联表通用模型Lognormaldistribution,对数正态分布Lostfunction,损失函数Lowcorrelation,低度相关Lowerlimit,下限Lowest-attainedvariance,最小可达方差LSD,最小显著差法的简称Lurkingvariable,潜在变量Main effect,主效应Major heading,主辞标目Marginal density function,边缘密度函数Marginal probability,边缘概率Marginal probability distribution,边缘概率分布Matched data,配对资料Matched distribution,匹配过分布Matching of distribution,分布的匹配Matching of transformation,变换的匹配Mathematical expectation,数学期望Mathematical model,数学模型Maximum L-estimator,极大极小L 估计量Maximum likelihood method,最大似然法Mean,均数Mean squares between groups,组间均方Mean squares within group,组内均方Means (Compare means),均值-均值比较Median,中位数Median effective dose,半数效量Median lethal dose,半数致死量Median polish,中位数平滑Median test,中位数检验Minimal sufficient statistic,最小充分统计量Minimum distance estimation,最小距离估计Minimum effective dose,最小有效量Minimum lethal dose,最小致死量Minimum variance estimator,最小方差估计量MINITAB,统计软件包Minor heading,宾词标目Missing data,缺失值Model specification,模型的确定Modeling Statistics ,模型统计Models for outliers,离群值模型Modifying the model,模型的修正Modulus of continuity,连续性模Morbidity,发病率Most favorable configuration,最有利构形Multidimensional Scaling (ASCAL),多维尺度/多维标度Multinomial Logistic Regression ,多项逻辑斯蒂回归Multiple comparison,多重比较Multiple correlation ,复相关Multiple covariance,多元协方差Multiple linear regression,多元线性回归Multiple response ,多重选项Multiple solutions,多解Multiplication theorem,乘法定理Multiresponse,多元响应Multi-stage sampling,多阶段抽样Multivariate T distribution,多元T分布Mutual exclusive,互不相容Mutual independence,互相独立Natural boundary,自然边界Natural dead,自然死亡Natural zero,自然零Negative correlation,负相关Negative linear correlation,负线性相关Negatively skewed,负偏Newman-Keuls method,q检验NK method,q检验No statistical significance,无统计意义Nominal variable,名义变量Nonconstancy of variability,变异的非定常性Nonlinear regression,非线性相关Nonparametric statistics,非参数统计Nonparametric test,非参数检验Nonparametric tests,非参数检验Normal deviate,正态离差Normal distribution,正态分布Normal equation,正规方程组Normal ranges,正常范围Normal value,正常值Nuisance parameter,多余参数/讨厌参数Null hypothesis,无效假设Numerical variable,数值变量Objective function,目标函数Observation unit,观察单位Observed value,观察值One sided test,单侧检验One-way analysis of variance,单因素方差分析Oneway ANOVA ,单因素方差分析Open sequential trial,开放型序贯设计Optrim,优切尾Optrim efficiency,优切尾效率Order statistics,顺序统计量Ordered categories,有序分类Ordinal logistic regression ,序数逻辑斯蒂回归Ordinal variable,有序变量Orthogonal basis,正交基Orthogonal design,正交试验设计Orthogonality conditions,正交条件ORTHOPLAN,正交设计Outlier cutoffs,离群值截断点Outliers,极端值OVERALS ,多组变量的非线性正规相关Overshoot,迭代过度Paired design,配对设计Paired sample,配对样本Pairwise slopes,成对斜率Parabola,抛物线Parallel tests,平行试验Parameter,参数Parametric statistics,参数统计Parametric test,参数检验Partial correlation,偏相关Partial regression,偏回归Partial sorting,偏排序Partials residuals,偏残差Pattern,模式Pearson curves,皮尔逊曲线Peeling,退层Percent bar graph,百分条形图Percentage,百分比Percentile,百分位数Percentile curves,百分位曲线Periodicity,周期性Permutation,排列P-estimator,P估计量Pie graph,饼图Pitman estimator,皮特曼估计量Pivot,枢轴量Planar,平坦Planar assumption,平面的假设PLANCARDS,生成试验的计划卡Point estimation,点估计Poisson distribution,泊松分布Polishing,平滑Polled standard deviation,合并标准差Polled variance,合并方差Polygon,多边图Polynomial,多项式Polynomial curve,多项式曲线Population,总体Population attributable risk,人群归因危险度Positive correlation,正相关Positively skewed,正偏Posterior distribution,后验分布Power of a test,检验效能Precision,精密度Predicted value,预测值Preliminary analysis,预备性分析Principal component analysis,主成分分析Prior distribution,先验分布Prior probability,先验概率Probabilistic model,概率模型probability,概率Probability density,概率密度Product moment,乘积矩/协方差Profile trace,截面迹图Proportion,比/构成比Proportion allocation in stratified random sampling,按比例分层随机抽样Proportionate,成比例Proportionate sub-class numbers,成比例次级组含量Prospective study,前瞻性调查Proximities,亲近性Pseudo F test,近似F检验Pseudo model,近似模型Pseudosigma,伪标准差Purposive sampling,有目的抽样QR decomposition,QR分解Quadratic approximation,二次近似Qualitative classification,属性分类Qualitative method,定性方法Quantile-quantile plot,分位数-分位数图/Q-Q图Quantitative analysis,定量分析Quartile,四分位数Quick Cluster,快速聚类Radix sort,基数排序Random allocation,随机化分组Random blocks design,随机区组设计Random event,随机事件Randomization,随机化Range,极差/全距Rank correlation,等级相关Rank sum test,秩和检验Rank test,秩检验Ranked data,等级资料Rate,比率Ratio,比例Raw data,原始资料Raw residual,原始残差Rayleigh's test,雷氏检验Rayleigh's Z,雷氏Z值Reciprocal,倒数Reciprocal transformation,倒数变换Recording,记录Redescending estimators,回降估计量Reducing dimensions,降维Re-expression,重新表达Reference set,标准组Region of acceptance,接受域Regression coefficient,回归系数Regression sum of square,回归平方和Rejection point,拒绝点Relative dispersion,相对离散度Relative number,相对数Reliability,可靠性Reparametrization,重新设置参数Replication,重复Report Summaries,报告摘要Residual sum of square,剩余平方和Resistance,耐抗性Resistant line,耐抗线Resistant technique,耐抗技术R-estimator of location,位置R估计量R-estimator of scale,尺度R估计量Retrospective study,回顾性调查Ridge trace,岭迹Ridit analysis,Ridit分析Rotation,旋转Rounding,舍入Row,行Row effects,行效应Row factor,行因素RXC table,RXC表Sample,样本Sample regression coefficient,样本回归系数Sample size,样本量Sample standard deviation,样本标准差Sampling error,抽样误差SAS(Statistical analysis system ),SAS统计软件包Scale,尺度/量表Scatter diagram,散点图Schematic plot,示意图/简图Score test,计分检验Screening,筛检SEASON,季节分析Second derivative,二阶导数Second principal component,第二主成分SEM (Structural equation modeling),结构化方程模型Semi-logarithmic graph,半对数图Semi-logarithmic paper,半对数格纸Sensitivity curve,敏感度曲线Sequential analysis,贯序分析Sequential data set,顺序数据集Sequential design,贯序设计Sequential method,贯序法Sequential test,贯序检验法Serial tests,系列试验Short-cut method,简捷法Sigmoid curve,S形曲线Sign function,正负号函数Sign test,符号检验Signed rank,符号秩Significance test,显著性检验Significant figure,有效数字Simple cluster sampling,简单整群抽样Simple correlation,简单相关Simple random sampling,简单随机抽样Simple regression,简单回归simple table,简单表Sine estimator,正弦估计量Single-valued estimate,单值估计Singular matrix,奇异矩阵Skewed distribution,偏斜分布Skewness,偏度Slash distribution,斜线分布Slope,斜率Smirnov test,斯米尔诺夫检验Source of variation,变异来源Spearman rank correlation,斯皮尔曼等级相关Specific factor,特殊因子Specific factor variance,特殊因子方差Spectra ,频谱Spherical distribution,球型正态分布Spread,展布SPSS(Statistical package for the social science),SPSS统计软件包Spurious correlation,假性相关Square root transformation,平方根变换Stabilizing variance,稳定方差Standard deviation,标准差Standard error,标准误Standard error of difference,差别的标准误Standard error of estimate,标准估计误差Standard error of rate,率的标准误Standard normal distribution,标准正态分布Standardization,标准化Starting value,起始值Statistic,统计量Statistical control,统计控制Statistical graph,统计图Statistical inference,统计推断Statistical table,统计表Steepest descent,最速下降法Stem and leaf display,茎叶图Step factor,步长因子Stepwise regression,逐步回归Storage,存Strata,层(复数)Stratified sampling,分层抽样Stratified sampling,分层抽样Strength,强度Stringency,严密性Structural relationship,结构关系Studentized residual,学生化残差/t化残差Sub-class numbers,次级组含量Subdividing,分割Sufficient statistic,充分统计量Sum of products,积和Sum of squares,离差平方和Sum of squares about regression,回归平方和Sum of squares between groups,组间平方和Sum of squares of partial regression,偏回归平方和Sure event,必然事件Survey,调查Survival,生存分析Survival rate,生存率Suspended root gram,悬吊根图Symmetry,对称Systematic error,系统误差Systematic sampling,系统抽样Tags,标签Tail area,尾部面积Tail length,尾长Tail weight,尾重Tangent line,切线Target distribution,目标分布Taylor series,泰勒级数Tendency of dispersion,离散趋势Testing of hypotheses,假设检验Theoretical frequency,理论频数Time series,时间序列Tolerance interval,容忍区间Tolerance lower limit,容忍下限Tolerance upper limit,容忍上限Torsion,扰率Total sum of square,总平方和Total variation,总变异Transformation,转换Treatment,处理Trend,趋势Trend of percentage,百分比趋势Trial,试验Trial and error method,试错法Tuning constant,细调常数Two sided test,双向检验Two-stage least squares,二阶最小平方Two-stage sampling,二阶段抽样Two-tailed test,双侧检验Two-way analysis of variance,双因素方差分析Two-way table,双向表Type I error,一类错误/α错误Type II error,二类错误/β错误UMVU,方差一致最小无偏估计简称Unbiased estimate,无偏估计Unconstrained nonlinear regression ,无约束非线性回归Unequal subclass number,不等次级组含量Ungrouped data,不分组资料Uniform coordinate,均匀坐标Uniform distribution,均匀分布Uniformly minimum variance unbiased estimate,方差一致最小无偏估计Unit,单元Unordered categories,无序分类Upper limit,上限Upward rank,升秩Vague concept,模糊概念Validity,有效性VARCOMP (Variance component estimation),方差元素估计Variability,变异性Variable,变量Variance,方差Variation,变异Varimax orthogonal rotation,方差最大正交旋转Volume of distribution,容积W test,W检验Weibull distribution,威布尔分布Weight,权数Weighted Chi-square test,加权卡方检验/Cochran检验Weighted linear regression method,加权直线回归Weighted mean,加权平均数Weighted mean square,加权平均方差Weighted sum of square,加权平方和Weighting coefficient,权重系数Weighting method,加权法W-estimation,W估计量W-estimation of location,位置W估计量Width,宽度Wilcoxon paired test,威斯康星配对法/配对符号秩和检验Wild point,野点/狂点Wild value,野值/狂值Winsorized mean,缩尾均值Withdraw,失访Youden's index,尤登指数Z test,Z检验Zero correlation,零相关Z-transformation,Z变换。
Improved algorithm for estimating pulse repetition intervals
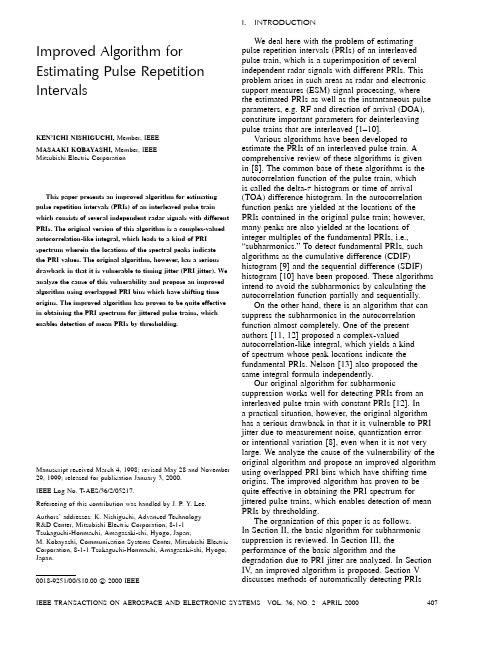
Improved Algorithm for Estimating Pulse Repetition IntervalsKEN’ICHI NISHIGUCHI,Member,IEEEMASAAKI KOBAYASHI,Member,IEEEMitsubishi Electric CorporationThis paper presents an improved algorithm for estimating pulse repetition intervals(PRIs)of an interleaved pulse train which consists of several independent radar signals with different PRIs.The original version of this algorithm is a complex-valued autocorrelation-like integral,which leads to a kind of PRI spectrum wherein the locations of the spectral peaks indicatethe PRI values.The original algorithm,however,has a serious drawback in that it is vulnerable to timing jitter(PRI jitter).We analyze the cause of this vulnerability and propose an improved algorithm using overlapped PRI bins which have shifting time origins.The improved algorithm has proven to be quite effective in obtaining the PRI spectrum for jittered pulse trains,which enables detection of mean PRIs by thresholding.Manuscript received March4,1998;revised May28and November 29,1999;released for publication January3,2000.IEEE Log No.T-AES/36/2/05217.Refereeing of this contribution was handled by J.P.Y.Lee. Authors’addresses:K.Nishiguchi,Advanced TechnologyR&D Center,Mitsubishi Electric Corporation,8-1-1Tsukaguchi-Honmachi,Amagasaki-shi,Hyogo,Japan;M.Kobayashi,Communication Systems Center,Mitsubishi Electric Corporation,8-1-1Tsukaguchi-Honmachi,Amagasaki-shi,Hyogo, Japan.0018-9251/00/$10.00c°2000IEEE I.INTRODUCTIONWe deal here with the problem of estimating pulse repetition intervals(PRIs)of an interleaved pulse train,which is a superimposition of several independent radar signals with different PRIs.This problem arises in such areas as radar and electronic support measures(ESM)signal processing,wherethe estimated PRIs as well as the instantaneous pulse parameters,e.g.RF and direction of arrival(DOA), constitute important parameters for deinterleaving pulse trains that are interleaved[1—10].Various algorithms have been developed to estimate the PRIs of an interleaved pulse train.A comprehensive review of these algorithms is givenin[8].The common base of these algorithms is the autocorrelation function of the pulse train,whichis called the delta-¿histogram or time of arrival (TOA)difference histogram.In the autocorrelation function peaks are yielded at the locations of the PRIs contained in the original pulse train;however, many peaks are also yielded at the locations of integer multiples of the fundamental PRIs,i.e.,“subharmonics.”To detect fundamental PRIs,such algorithms as the cumulative difference(CDIF) histogram[9]and the sequential difference(SDIF) histogram[10]have been proposed.These algorithms intend to avoid the subharmonics by calculating the autocorrelation function partially and sequentially.On the other hand,there is an algorithm that can suppress the subharmonics in the autocorrelation function almost completely.One of the present authors[11,12]proposed a complex-valued autocorrelation-like integral,which yields a kindof spectrum whose peak locations indicate the fundamental PRIs.Nelson[13]also proposed the same integral formula independently.Our original algorithm for subharmonic suppression works well for detecting PRIs from an interleaved pulse train with constant PRIs[12].Ina practical situation,however,the original algorithm has a serious drawback in that it is vulnerable to PRI jitter due to measurement noise,quantization erroror intentional variation[8],even when it is not very large.We analyze the cause of the vulnerability of the original algorithm and propose an improved algorithm using overlapped PRI bins which have shifting time origins.The improved algorithm has proven to be quite effective in obtaining the PRI spectrum for jittered pulse trains,which enables detection of mean PRIs by thresholding.The organization of this paper is as follows.In Section II,the basic algorithm for subharmonic suppression is reviewed.In Section III,the performance of the basic algorithm and the degradation due to PRI jitter are analyzed.In Section IV,an improved algorithm is proposed.Section V discusses methods of automatically detecting PRIsfrom the spectrum of the improved algorithm as well as the detection performance of the algorithm.Finally, Section VI draws some conclusions.II.PRI TRANSFORMIn this section we review the basic algorithm for subharmonic suppression,which we refer to as the PRI transform[12].A.Definition and PrincipleLet t n,n=0,:::,N¡1be pulse arrival times, where N is the number of pulses.If we consider the TOA as the only parameter of each pulse,the pulse train can be modeled as a sum of unit impulses,g(t)=N¡1Xn=0±(t¡t n)(1)where±(¢)is the Dirac delta function.We consider the following integral transformation of g(t)[8,11—13], D(¿)=Z1¡1g(t)g(t+¿)exp(2¼it=¿)dt(2)where the domain of¿is¿>0.This integral is referred to as the harmonics rejecting correlation function in[8]or as the Nelson TDOA histogram in [14].However we give it the brief name of the PRI transform since its absolute value gives a kind of PRI spectrum wherein the locations of the spectral peaks indicate the PRI values[11,12].The PRI transformis similar in its form to the autocorrelation function defined byC(¿)=Z1¡1g(t)g(t+¿)dt(3)and also similar to the Fourier transform F[g](¡1=¿) =R11g(t)exp(2¼it=¿)dt.Substituting(1)into(2)and (3)yieldsD(¿)=N¡1Xn=1n¡1Xm=0±(¿¡t n+t m)exp[2¼it n=(t n¡t m)](4)C(¿)=N¡1Xn=1n¡1Xm=0±(¿¡t n+t m):(5)The difference between the PRI transform andthe autocorrelation function is that the former has the phase factor exp(2¼it=¿)or exp[2¼it n=(t n¡t m)], and this factor plays an important role in suppressing the subharmonics which appear in the autocorrelation function.To explain the effect of the phase factor of the PRI transform,let us define the phase of a pulse train. The pulse arrival times of a pulse train with a single PRI,which we refer to as a single pulse train,can be written ast n=(n+´)p,n=0,1,2,:::(6) where p is the PRI and´is a constant.We define the phase of the pulse train byµ=2¼´mod2¼:(7) Two phases,µ1andµ2,are equivalent if they satisfyµ1=µ2mod2¼,or exp(iµ1)=exp(iµ2).In symbols we writeµ1´µ2.The phase of a single pulse train with the PRI p can also be obtained byµ´2¼t n=p=2¼t n=(t n¡t n¡1)(8) for all t n,n=1,2,:::.Therefore,the phase is calculated in terms of every two adjacent pulses.Next,we consider the autocorrelation function of a single pulse train.Substituting(6)into(5),we obtainC(¿)=N¡1Xl=1(N¡l)±(¿¡lp):(9)Although the impulses located at¿=lp,l=2,3,::: are the subharmonics of the PRI p,from another viewpoint these impulses can be considered indicators of the pulse trains with PRI lp.Actually,the single pulse train with pulse TOAs given by(6)can be decomposed to l single pulse trains with the samePRI lp as shown in Fig.1(a).By definition,the phases of these l pulse trains becomeµ1=µ=l,µ2= (µ+2¼)=l,:::,µl=(µ+2¼(l¡1))=l,whereµ´2¼´, 0·µ<2¼.If we represent these phases by points on the unit circle as in Fig.1(b),the vector sum of these points become zero except when l=1.The phase of the pulse train that includes the pulse pair(t m,t n)as adjacent pulses is given by2¼t n=(t n¡t m).This implies that if we multiply each term on the right-hand side (RHS)of(5)by the phase factor exp[2¼it n=(t n¡t m)] and take the summation as in(4),the subharmonics appearing in the autocorrelation function would be suppressed.B.Discrete PRI TransformThe PRI transform defined by(2)or(4)has the form of the sum of the impulses,and hence it is inappropriate to calculate it numerically.We must obtain a discrete version of the PRI transform,which takes some finite values at discrete points on the¿-axis.Let[¿min,¿max]be the range of the PRI to be investigated.We separate this range into K small intervals,which we refer to as PRI bins(see Fig.2). The width of a PRI bin is b=(¿max¡¿min)=K,and its center is¿k=(k¡1=2)b+¿min,k=1,2,:::,K:(10)Fig.1.Subharmonic components of pulse train.(a)Decomposition.Single pulse train with PRI =p can be decomposed into l subharmonic components with PRI =lp .(b)Phases of l subharmonic components.µ1=µ=l ,µ2=(µ+2¼)=l ,:::,µl =(µ+(l ¡1)¼)=l.Fig.2.PRI bins.We define the discrete PRI transform as follows:D k =Z ¿k +b=2¿k ¡b=2D (¿)d¿=Xf (m ,n );¿k ¡b=2<t n ¡t m ·¿k +b=2gexp ·2¼it nn m¸:(11)Further we define a PRI spectrum by j D k j .We note that if b !0,then D k =b !D (¿)in the sense of distribution.For the sake of comparison,we calculate a discrete version of the autocorrelation function (4)as follows:C k =Z¼k +b=2¿k ¡b=2C (¿)d¿=number of pairs (t m ,t n )that satisfy ¿k ¡b=2<t n ¡t m ·¿k +b=2,k =1,2,:::,K:(12)Obviously,the following inequality holds for every kj D k j ·C k :(13)The discrete PRI transform is easily calculated by the following procedure.1)Initialization.Let D k =0for 1·k ·K and let n =1.2)Let m =n ¡1.3)Let ¿=t n ¡t m .If ¿·¿min go to 5.Else,if ¿>¿max go to 6.4)Processing for each pair (m ,n ).a)Choose k such that ¿k ¡b=2<¿·¿k +b=2.b)Update the PRI transform.D k =D k +exp(2¼it n =¿).5)Substitute m =m ¡1.If m <0go to 6.Else,go to 3.6)Substitute n =n +1.If n >N ¡1stop.Else,go to 2.III.PERFORMANCE OF ORIGINAL PRI TRANSFORM A.Application to Single Pulse Train with Constant PRILet us calculate the PRI transform of a single pulse train.Substituting (6)into (4)yields D (¿)=N ¡1X n =1n ¡1X m =0±(¿¡(n ¡m )p )exp2¼i (´+n )=N ¡1X l =1±(¿¡lp )exp 2¼i´N ¡l ¡1X n =0exp2¼in=(N ¡1)±(¿¡p )exp(2¼i´)+N ¡1X l =2±(¿¡lp )sin(N¼=l )exp ¼i (N ¡1+2´):(14)Similarly,the autocorrelation function of g (t )is obtained by substituting (6)into (5)as follows:C (¿)=(N ¡1)±(¿¡p )+N ¡1X l =2(N ¡l )±(¿¡lp ):(15)The first term on the RHS of (14)represents an impulse located at ¿=p ,and the absolute value of its coefficient is N ¡1,which is the same as that of the autocorrelation function.The second term on the RHS of (14)is the sum of impulses located at ¿=lp ,l =2,:::,N ¡1,and the absolute value of each coefficient is evaluated by¯¯¯¯sin(N¼=l )sin(¼=l )¯¯¯¯·1sin(¼=l )·l 2=¿2p :(16)We note that the RHS does not depend on N ,so that the ratio between the peak level at the locationsparison between PRI spectrum and autocorrelation function for pulse train with constant PRIs.(a)Input pulse train,which is superimposition of3single pulse trains with PRIs1,p and p(b)PRI spectrum.(c)Autocorrelation function.corresponding to the PRI and the noise level decreasesas N becomes larger.B.Application to Interleaved Pulse Train with ConstantPRIsIn order to analyze the PRI transform of aninterleaved pulse train,we represent the interleavedpulse train as follows:g(t)=MX¹=1g¹(t)(17)whereg¹(t)=N¹¡1Xn¹=1±(t¡t n¹),¹=1,:::,M(18)are single pulse trains with PRI p¹,¹=1,:::,M.The PRI transform of(17)becomesD(¿)=MX¹=1D¹¹(¿)+MX¹=1MXº=1(º=¹)D¹º(¿)(19)whereD¹º(¿)=Z1¡1g¹(t)gº(t+¿)exp(2¼it=¿)dt:(20)The first term of the RHS of(19)is the sum of the PRI transforms of single pulse trains with constant PRIs.Hence,if the PRIs are different,this term has notable peaks only at the locations corresponding to the PRIs of the single pulse trains as in the case of a single pulse train described in the previous section.The second term on the RHS of(19)is causedby the mutual interference between different single pulse trains.This interference gives rise to a noise-like spectral shape in the PRI transform and its level can be evaluated probabilistically using the Poisson arrivalTABLE IParameters of PRI Transform(Figs.3and5)model,shown in the Appendix.As a result,the value of the discrete PRI transform at the PRI bins thatdo not correspond to any true PRIs or their integer multiples are evaluated byq hj D k j2i<p(21) where h¢i means the sample average,and½denotes the pulse density.Fig.3shows an example of the PRI spectrum of an interleaved pulse train,where the PRIs of the input pulse train are1,p2,and p5.The parameters used are shown in Table I.As is apparent from the figure, the subharmonics that appeared in the autocorrelation function are suppressed almost completely by the PRI transform.C.Application to Jittered Pulse TrainsWe assume that the input pulse train is a single pulse train and the TOAs are represented ast0=0(22)t n=t n¡1+p(1+²n),n=1,2,:::,N¡1(23) where p is the average PRI and²n is the relative deviation of the adjacent pulse interval fromthe average PRI.We further assume that²n s are independently and identically distributed random variables with mean0and the standard deviation¾=p n Under these assumptions,the phase of the pairs of adjacent pulses is given byµn =2¼t n =(t n ¡t n ¡1)=2¼n +²1+¢¢¢+²n1+²n´2¼²1+¢¢¢+²n ¡n²nn+2¼(²1+¢¢¢+²n ¡n²n ):(24)When n is large ²1+¢¢¢+²n is the order of pn while n²n is the order of n ,so that the latter is significant and the phase is approximated byµn +¡2¼n²n(25)which means that the phase error increases in proportion to n .We suppose that all the pulse pairs (t n ¡1,t n ),n =1,2,:::,N ¡1are gathered into the k th PRI bin.Then the PRI transform at the bin is given byD k =N ¡1X n =1eiµn+N ¡1X n =1e ¡2¼in²n :(26)Let q (²)be the probability density function of ²n ,so the expectation of D k can be written ash D k i +N Xn =2Z 1¡1e ¡2¼in²q (²)d²:(27)We calculate this expectation for the following twocases.1)Uniform distribution.If q (²)is given byq (²)=½1=2a ,¡a ·²·a ,0,otherwise,(28)where 0<a <1=2,then h D k i =N ¡1X n =1Z a ¡acos2¼n²¢12a d²=N ¡1X n =112¼nasin2¼na !1¡1,as N !1(29)(see Fig.4(a)).When a is sufficiently small h D k i can be approximated byh D k i +1Z 2¼aN 0sin µdµ(30)which takes the maximum value (1=2¼a )R ¼0(1=µ)£sin µdµ=0:295=a at N =1=2a .2)Gaussian distribution.If q (²)is given byq (²)=p 2¼¾2¡²2=2¾2(31)Fig.4.h D k i versus N .(a)In the case of uniform distribution.(b)In the case of Gaussian distribution.thenh D k i =N ¡1X n =1Z a ¡acos2p ¡²2=2¾2d²=N ¡1X n =1e ¡(2¼n¾)2=2(32)which is a monotonically increasing function of N(see Fig.4(b)).When ¾is sufficiently small h D k i can be approximated byh D k i +1Z 2¼¾N 0e ¡µ2=2dµ(33)which approaches 1=2p2¼¾=0:1995=¾as N !1.As is seen by the above examples,though h D k i increases in proportion to N when N is small,it does not exceed an upper bound.The upper bound decreases as the PRI jitter becomes larger.On the other hand the noise level of the PRI transform increases in proportion to p N ,so that the ratio between the peak level at the locationscorresponding to the PRI and the noise level decreases as N becomes larger.In Fig.5the results of the PRI transform applied to an interleaved pulse train which includes 3single pulse trains with PRI jitter are shown.The parameters used are shown in Table I.As is shown in the figure,the spectral peaks corresponding to true PRIs were submerged in the noise even in the case of a =0:01(Fig.5(b)).Fig.5.PRI spectrum by PRI transform.Input data is an interleaved pulse train with PRI jitter.Mean PRIs are 1,p and pandjitter follows uniform distribution with width 2a as in(28).(a)a =0:001.(b)a =0:01.(c)a =0:1.IV .MODIFICATION OF PRI TRANSFORMIn the previous section we saw that the peaks of the PRI spectrum derived from the original PRI transform are reduced in the case of jittered pulse trains.There are two factors that cause this reduction.One is that the phase error of the phase factor of the PRI transform is enlarged as the TOAs grow apart from the time origin.The other is that the pulse pairs,concentrated in a PRI bin when the PRI is constant,are distributed in several bins around the average PRI.In this section we describe a modified algorithm of the PRI transform to overcome these drawbacks.A.Shifting Time OriginsTo avoid the enlargement of the phase error caused by the TOAs becoming large,we may change thetimeFig.6.Subharmonic components of PRI.origin.The role of the phase factor exp[2¼it n =(t n ¡t m )]in the PRI transform (4)is to suppress thesubharmonics which appeared in the autocorrelation function,while keeping the peak levels at the PRIs.To do so,it is not necessary that the phases of all pulse pairs are determined using a common time origin.For example,in the case of Fig.6,it is sufficient that the phases of 3pairs (t 1,t 4),(t 2,t 5),(t 3,t 6)differ from each other by 2¼=3.Consequently,we may update the time origin in the period 3£PRI.In general to eliminate the k £PRI components,we may update the time origin in the period k £PRI.On the other hand to keep the peak levels at the PRIs we may update the time origin in the period of a PRI.Hence,in all cases we may update the time origin in the period ¿k in the k th PRI bin;therefore,a different time origin is necessary for each PRI bin.If there are no missing pulses,the condition of the time shift is that t m of the pair (t m ,t n )agrees with the previous time origin.However,we have to consider the missing pulses which occur in practical situations.It is also necessary to shift the time origin whent m ¡O k takes a value near some integer multiple of ¿k .Considering the above,we shift the time origins as follows.First we calculate a preliminary phase by´0=t n ¡O kk(34)where O k denotes the previous time origin of the k th PRI bin.Here we use ¿k instead of t n ¡t m toaccommodate the influence of the PRI jitter.Then we decompose the phase as´0=º(1+³)(35)where ºis an integer and ³is a real number such that ¡1=2<³<1=2.Finally we decide whether to shift the time origin or not according to the following conditions:1)when º=0,do not shift the time origin,2)when º=1,if t m =O k ,then let t n be the new time origin,3)when º¸2,if j ³j ·³0,then let t n be the new time origin,where ³0is a positive parameter that determines the mobility of the time origins.In Fig.7examples of the shift of time origins are shown.Fig.7.Shift of time origins.(a)When PRI bin includes PRI component.(b)When PRI bin includes subharmonic componentofPRI.Fig.8.PRI bins for modified PRI transform.B.Overlapped PRI BinsTo avoid the reduction of the peaks by the distribution of the pulse pairs,the width of the PRI bins must be greater than the width of the PRI jitter.However this causes the degradation of theresolution of the estimated PRIs and makes it difficult to deinterleave an interleaved pulse train.To resolve this dilemma we may use overlapped bins (see Fig.8).Let ²be the upper limit of the PRI jitter.Let K be the number of PRI bins.We determine the center of each PRI bin in the same way as before,i.e.,¿=k ¡1=2(¿max ¡¿min )+¿min ,k =1,2,:::,K(36)where [¿min ,¿max ]is the range of PRI to beinvestigated and K is the number of PRI bins.Then the width of the PRI bin may be set asb k =2²¿k :(37)C.Modified PRI TransformBy combining shifting time origins and overlapped PRI bins we obtain the following modified PRI transform algorithm.1)Initialization.Let D k =0for 1·k ·K and let n =2.2)Let m =n ¡1.3)Let ¿=t n ¡t m .If ¿·(1¡²)¿min go to 5.Else,if ¿>(1+²)¿max go to 6.4)Calculate the range of PRI bins:k 1=·³¿1+²¡¿min´Á¢¿¸+1,k 2=·µ¿1¡²¡¿min¶Á¢¿¸+1where ¢¿=(¿max ¡¿min )=K .5)Repeat the next 5steps (from 6to 10)for k =k 1,:::,k 2.6)Initialization of the time origin.If the k th PRI bin is used for the first time,then let O k =t n .7)Calculate the preliminary phase and decompose it:´0=(t n ¡O k )=¿k ,º=[´0+0:4999:::],³=´0=º¡1:8)Shift of the time origin.If either of the following conditions are satisfied then let O k =t n .a)º=1and t m =O k .b)º¸2and j ³j ·³0.9)Calculate the phase:´=(t n ¡O k )=¿k .10)Update the PRI transform.D k =D k +exp(2¼i´).11)Substitute m =m ¡1.If m <1go to 12.Else,go to 3.12)Substitute n =n +1.If n >N stop.Else,go to 2.In Fig.9the results of the modified PRI transform are applied to the same pulse train as in Fig.5.The parameters used are shown in Table II.As is apparent from the figure,the spectral peaks corresponding to the true PRIs are recovered.Although the number of all pulse pairs (t m ,t n )s is N (N ¡1),only those that satisfy ¿min ·t n ¡t m ·¿max are processed by the modified PRI transform,so that the processing time of the modified PRI transform is proportional to N½(¿max ¡¿min ),where ½is the pulse density.Fig.10shows the CPU time of the modified PRI transform on an Intel Pentium IIITABLE IIParameters of Modified PRI Transform (Figs.9—15)Fig.9.PRI spectrum by modified PRI transform.Input data is same as in Fig.5.(Values of mean PRIs are 1,p and pand jitter follows uniform distribution with width 2a as in (28).)(a)a =0:001.(b)a =0:01.(c)a =0:1.Fig.10.Processing time of modified PRI transform as measured on Intel Pentium III 550MHz processor (densities ½=1:0,2.150,and 2.695correspond to 1,3,and 5emitters,respectively).550MHz processor.The parameters are shown in Table II.Fig.10exhibits the linear dependence of the computational load on N ,which is common to a broad class of pulse deinterleaving algorithms [15].V .DETECTION OF PRIS USING MODIFIED PRITRANSFORM A.Threshold for Detection of PRIsTo detect PRIs from the result of the modified PRI transform,the PRI bins that correspond to the correct PRIs must be distinguished from the other PRI bins.This discrimination can be achieved by using three criteria:a criterion by observation time,a criterion for eliminating subharmonics,and a criterion for eliminating noise.The threshold used to detect PRIs can be established by these criteria.Criterion by Observation Time :If a single pulse train with a PRI ¿k exists in the entire observation time T ,then the number of pulses is T=¿k .On the other hand,j D k j denotes the number of pulses of the pulse train with a PRI ¿k ,and thus ideally it becomes j D k j =T=¿k .In actual situations,each pulse train doesnot always exist throughout the entire observation time and there are some missing pulses;accordingly, we make the following criterion with a margin:j D k j¸®T¿k(38)where®is a tunable parameter.Criterion for Eliminating Subharmonics:If¿k is the PRI of a pulse train,then ideally j D k j=C k+number of pulses of the single pulse train. Otherwise,if¿k is the subharmonics of the PRI of some single pulse train,then jD k j¿C k.Therefore, we can judge whether¿k is a PRI or its subharmonics by the criterion:j D k j¸¯C k(39) where¯is a tunable parameter.This criterion is effective for jittered pulse trains,which has incomplete suppression of the subharmonics by the modified PRI transform.Criterion for Eliminating Noise:To detect PRIs from the result of the PRI transform,it is necessary that the levels of the PRI bins that correspond to the correct PRIs are much larger than the noise level,i.e., the level of the PRI bins other than those including the PRIs or their integer multiples.In the case of the modified PRI transform,however,it is not easy to estimate the noise level because of the shifting time origins.Therefore,we have devised a criterion that uses the estimate of the noise level of the original PRI transform.As is shown in the Appendix,if D k is a noise component of the original PRI transform,then the variance of j D k j is less than T½2b k,where½is the pulse density and b k is the width of the k th PRI bin. Using this variance,we can judge that the k th PRIbin includes some component other than noise by the following criterion:j D k j¸°q T½2b k(40)where°is a tunable parameter.If D k is the value of the original PRI transform,then°=3is adequate by the“three-¾criterion.”Since the noise level of the modified PRI transform is greater than that of the original PRI transform,it is necessary to choose a value of°not less than3.Combining the above three criteria we canestablish the threshold as follows:A k=max½®T¿k,¯C k,°q T½2b k ¾(41)where three tunable parameters are®,¯,and°.We tuned the values of these parameters through simulations under various conditions to increase the detection probabilities and to reduce the false alarm probability.All numerical examples in this paper were calculated by the following common values:®=0:3,¯=0:15,°=3:Fig.11.Determination of threshold and detection of PRIs. (a)Components of threshold(RHS of(41)with®=0:3,¯=0:15,°=3).(b)Detection of PRIs based on threshold.In Fig.11,an example of the components of the above threshold and the detection by the thresholdis shown.As the figure clearly shows,we can easily detect correct PRIs by finding the peaks that exceed the threshold.B.Detection PerformanceThere are mainly three factors that affect the detection performance:number of input pulses, number of emitters(single pulse trains),and jitter width.To investigate the influence of these factors, computer simulation was performed under various conditions.The input data was generated by the superimposition of all or part of five emitters with average PRIs of1,p p p and p All emitters obey the uniform jitter with the same peak-to-peak jitter width of10%,20%,or30%.Figs.12—14show the PRI spectrum and the detection results using the threshold described in the preceding section.The parameters of the modified PRI transform are shown in Table II.The detection results shown in Figs.12—14as well as others are summarized in Table III.When the number of input pulses is1000(Fig.12),up to5emitters with a10%PRI jitter can be detected.If the jitter width is expanded to30%, the number of detected emitters is reduced to threeor four.In some cases,there are false detections.It seems,however,that these false detections are caused by the nonoptimality of the current threshold.。
SPSS词汇(中英文对照)
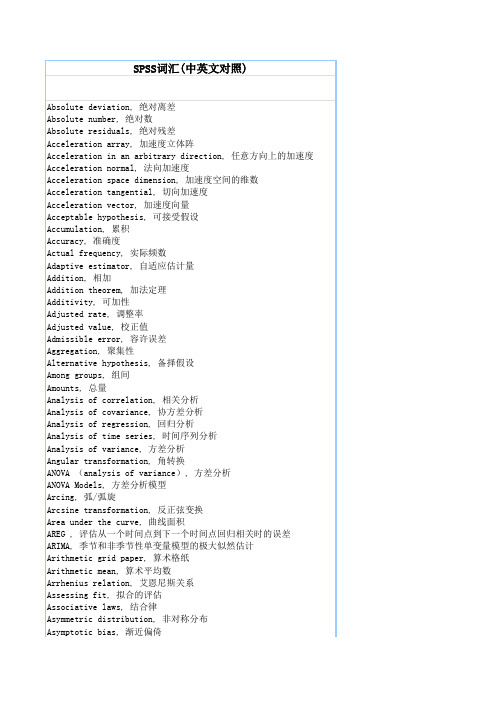
Asymptotic efficiency, 渐近效率Asymptotic variance, 渐近方差Attributable risk, 归因危险度Attribute data, 属性资料Attribution, 属性Autocorrelation, 自相关Autocorrelation of residuals, 残差的自相关Average, 平均数Average confidence interval length, 平均置信区间长度Average growth rate, 平均增长率Bar chart, 条形图Bar graph, 条形图Base period, 基期Bayes' theorem , Bayes定理Bell-shaped curve, 钟形曲线Bernoulli distribution, 伯努力分布Best-trim estimator, 最好切尾估计量Bias, 偏性Binary logistic regression, 二元逻辑斯蒂回归Binomial distribution, 二项分布Bisquare, 双平方Bivariate Correlate, 二变量相关Bivariate normal distribution, 双变量正态分布Bivariate normal population, 双变量正态总体Biweight interval, 双权区间Biweight M-estimator, 双权M估计量Block, 区组/配伍组BMDP(Biomedical computer programs), BMDP统计软件包Boxplots, 箱线图/箱尾图Breakdown bound, 崩溃界/崩溃点Canonical correlation, 典型相关Caption, 纵标目Case-control study, 病例对照研究Categorical variable, 分类变量Catenary, 悬链线Cauchy distribution, 柯西分布Cause-and-effect relationship, 因果关系Cell, 单元Censoring, 终检Center of symmetry, 对称中心Centering and scaling, 中心化和定标Central tendency, 集中趋势Central value, 中心值CHAID -χ2 Automatic Interaction Detector, 卡方自动交互检测Chance, 机遇Chance error, 随机误差Chance variable, 随机变量Characteristic equation, 特征方程Characteristic root, 特征根Characteristic vector, 特征向量Chebshev criterion of fit, 拟合的切比雪夫准则Chernoff faces, 切尔诺夫脸谱图Chi-square test, 卡方检验/χ2检验Choleskey decomposition, 乔洛斯基分解Circle chart, 圆图Class interval, 组距Class mid-value, 组中值Class upper limit, 组上限Classified variable, 分类变量Cluster analysis, 聚类分析Cluster sampling, 整群抽样Code, 代码Coded data, 编码数据Coding, 编码Coefficient of contingency, 列联系数Coefficient of determination, 决定系数Coefficient of multiple correlation, 多重相关系数Coefficient of partial correlation, 偏相关系数Coefficient of production-moment correlation, 积差相关系数Coefficient of rank correlation, 等级相关系数Coefficient of regression, 回归系数Coefficient of skewness, 偏度系数Coefficient of variation, 变异系数Cohort study, 队列研究Column, 列Column effect, 列效应Column factor, 列因素Combination pool, 合并Combinative table, 组合表Common factor, 共性因子Common regression coefficient, 公共回归系数Common value, 共同值Common variance, 公共方差Common variation, 公共变异Communality variance, 共性方差Comparability, 可比性Comparison of bathes, 批比较Comparison value, 比较值Compartment model, 分部模型Compassion, 伸缩Complement of an event, 补事件Complete association, 完全正相关Complete dissociation, 完全不相关Complete statistics, 完备统计量Completely randomized design, 完全随机化设计Composite event, 联合事件Composite events, 复合事件Concavity, 凹性Conditional expectation, 条件期望Conditional likelihood, 条件似然Conditional probability, 条件概率Conditionally linear, 依条件线性Confidence interval, 置信区间Confidence limit, 置信限Confidence lower limit, 置信下限Confidence upper limit, 置信上限Confirmatory Factor Analysis , 验证性因子分析Confirmatory research, 证实性实验研究Confounding factor, 混杂因素Conjoint, 联合分析Consistency, 相合性Consistency check, 一致性检验Consistent asymptotically normal estimate, 相合渐近正态估计Consistent estimate, 相合估计Constrained nonlinear regression, 受约束非线性回归Constraint, 约束Contaminated distribution, 污染分布Contaminated Gausssian, 污染高斯分布Contaminated normal distribution, 污染正态分布Contamination, 污染Contamination model, 污染模型Contingency table, 列联表Contour, 边界线Contribution rate, 贡献率Control, 对照Controlled experiments, 对照实验Conventional depth, 常规深度Convolution, 卷积Corrected factor, 校正因子Corrected mean, 校正均值Correction coefficient, 校正系数Correctness, 正确性Correlation coefficient, 相关系数Correlation index, 相关指数Correspondence, 对应Counting, 计数Counts, 计数/频数Covariance, 协方差Covariant, 共变Cox Regression, Cox回归Criteria for fitting, 拟合准则Criteria of least squares, 最小二乘准则Critical ratio, 临界比Critical region, 拒绝域Critical value, 临界值Cross-over design, 交叉设计Cross-section analysis, 横断面分析Cross-section survey, 横断面调查Crosstabs , 交叉表Cross-tabulation table, 复合表Cube root, 立方根Cumulative distribution function, 分布函数Cumulative probability, 累计概率Curvature, 曲率/弯曲Curvature, 曲率Curve fit , 曲线拟和Curve fitting, 曲线拟合Curvilinear regression, 曲线回归Curvilinear relation, 曲线关系Cut-and-try method, 尝试法Cycle, 周期Cyclist, 周期性D test, D检验Data acquisition, 资料收集Data bank, 数据库Data capacity, 数据容量Data deficiencies, 数据缺乏Data handling, 数据处理Data manipulation, 数据处理Data processing, 数据处理Data reduction, 数据缩减Data set, 数据集Data sources, 数据来源Data transformation, 数据变换Data validity, 数据有效性Data-in, 数据输入Data-out, 数据输出Dead time, 停滞期Degree of freedom, 自由度Degree of precision, 精密度Degree of reliability, 可靠性程度Degression, 递减Density function, 密度函数Density of data points, 数据点的密度Dependent variable, 应变量/依变量/因变量Dependent variable, 因变量Depth, 深度Derivative matrix, 导数矩阵Derivative-free methods, 无导数方法Design, 设计Determinacy, 确定性Determinant, 行列式Determinant, 决定因素Deviation, 离差Deviation from average, 离均差Diagnostic plot, 诊断图Dichotomous variable, 二分变量Differential equation, 微分方程Direct standardization, 直接标准化法Discrete variable, 离散型变量DISCRIMINANT, 判断Discriminant analysis, 判别分析Discriminant coefficient, 判别系数Discriminant function, 判别值Dispersion, 散布/分散度Disproportional, 不成比例的Disproportionate sub-class numbers, 不成比例次级组含量Distribution free, 分布无关性/免分布Distribution shape, 分布形状Distribution-free method, 任意分布法Distributive laws, 分配律Disturbance, 随机扰动项Dose response curve, 剂量反应曲线Double blind method, 双盲法Double blind trial, 双盲试验Double exponential distribution, 双指数分布Double logarithmic, 双对数Downward rank, 降秩Dual-space plot, 对偶空间图DUD, 无导数方法Duncan's new multiple range method, 新复极差法/Duncan新法Effect, 实验效应Eigenvalue, 特征值Eigenvector, 特征向量Ellipse, 椭圆Empirical distribution, 经验分布Empirical probability, 经验概率单位Enumeration data, 计数资料Equal sun-class number, 相等次级组含量Equally likely, 等可能Equivariance, 同变性Error, 误差/错误Error of estimate, 估计误差Error type I, 第一类错误Error type II, 第二类错误Estimand, 被估量Estimated error mean squares, 估计误差均方Estimated error sum of squares, 估计误差平方和Euclidean distance, 欧式距离Event, 事件Event, 事件Exceptional data point, 异常数据点Expectation plane, 期望平面Expectation surface, 期望曲面Expected values, 期望值Experiment, 实验Experimental sampling, 试验抽样Experimental unit, 试验单位Explanatory variable, 说明变量Exploratory data analysis, 探索性数据分析Explore Summarize, 探索-摘要Exponential curve, 指数曲线Exponential growth, 指数式增长EXSMOOTH, 指数平滑方法Extended fit, 扩充拟合Extra parameter, 附加参数Extrapolation, 外推法Extreme observation, 末端观测值Extremes, 极端值/极值F distribution, F分布F test, F检验Factor, 因素/因子Factor analysis, 因子分析Factor Analysis, 因子分析Factor score, 因子得分Factorial, 阶乘Factorial design, 析因试验设计False negative, 假阴性False negative error, 假阴性错误Family of distributions, 分布族Family of estimators, 估计量族Fanning, 扇面Fatality rate, 病死率Field investigation, 现场调查Field survey, 现场调查Finite population, 有限总体Finite-sample, 有限样本First derivative, 一阶导数First principal component, 第一主成分First quartile, 第一四分位数Fisher information, 费雪信息量Fitted value, 拟合值Fitting a curve, 曲线拟合Fixed base, 定基Fluctuation, 随机起伏Forecast, 预测Four fold table, 四格表Fourth, 四分点Fraction blow, 左侧比率Fractional error, 相对误差Frequency, 频率Frequency polygon, 频数多边图Frontier point, 界限点Function relationship, 泛函关系Gamma distribution, 伽玛分布Gauss increment, 高斯增量Gaussian distribution, 高斯分布/正态分布Gauss-Newton increment, 高斯-牛顿增量General census, 全面普查GENLOG (Generalized liner models), 广义线性模型Geometric mean, 几何平均数Gini's mean difference, 基尼均差GLM (General liner models), 一般线性模型Goodness of fit, 拟和优度/配合度Gradient of determinant, 行列式的梯度Graeco-Latin square, 希腊拉丁方Grand mean, 总均值Gross errors, 重大错误Gross-error sensitivity, 大错敏感度Group averages, 分组平均Grouped data, 分组资料Guessed mean, 假定平均数Half-life, 半衰期Hampel M-estimators, 汉佩尔M估计量Happenstance, 偶然事件Harmonic mean, 调和均数Hazard function, 风险均数Hazard rate, 风险率Heading, 标目Heavy-tailed distribution, 重尾分布Hessian array, 海森立体阵Heterogeneity, 不同质Heterogeneity of variance, 方差不齐Hierarchical classification, 组内分组Hierarchical clustering method, 系统聚类法High-leverage point, 高杠杆率点HILOGLINEAR, 多维列联表的层次对数线性模型Hinge, 折叶点Histogram, 直方图Historical cohort study, 历史性队列研究Holes, 空洞HOMALS, 多重响应分析Homogeneity of variance, 方差齐性Homogeneity test, 齐性检验Huber M-estimators, 休伯M估计量Hyperbola, 双曲线Hypothesis testing, 假设检验Hypothetical universe, 假设总体Impossible event, 不可能事件Independence, 独立性Independent variable, 自变量Index, 指标/指数Indirect standardization, 间接标准化法Individual, 个体Inference band, 推断带Infinite population, 无限总体Infinitely great, 无穷大Infinitely small, 无穷小Influence curve, 影响曲线Information capacity, 信息容量Initial condition, 初始条件Initial estimate, 初始估计值Initial level, 最初水平Interaction, 交互作用Interaction terms, 交互作用项Intercept, 截距Interpolation, 内插法Interquartile range, 四分位距Interval estimation, 区间估计Intervals of equal probability, 等概率区间Intrinsic curvature, 固有曲率Invariance, 不变性Inverse matrix, 逆矩阵Inverse probability, 逆概率Inverse sine transformation, 反正弦变换Iteration, 迭代Jacobian determinant, 雅可比行列式Joint distribution function, 分布函数Joint probability, 联合概率Joint probability distribution, 联合概率分布K means method, 逐步聚类法Kaplan-Meier, 评估事件的时间长度Kaplan-Merier chart, Kaplan-Merier图Kendall's rank correlation, Kendall等级相关Kinetic, 动力学Kolmogorov-Smirnove test, 柯尔莫哥洛夫-斯米尔诺夫检验Kruskal and Wallis test, Kruskal及Wallis检验/多样本的秩和检验/H检验Kurtosis, 峰度Lack of fit, 失拟Ladder of powers, 幂阶梯Lag, 滞后Large sample, 大样本Large sample test, 大样本检验Latin square, 拉丁方Latin square design, 拉丁方设计Leakage, 泄漏Least favorable configuration, 最不利构形Least favorable distribution, 最不利分布Least significant difference, 最小显著差法Least square method, 最小二乘法Least-absolute-residuals estimates, 最小绝对残差估计Least-absolute-residuals fit, 最小绝对残差拟合Least-absolute-residuals line, 最小绝对残差线Legend, 图例L-estimator, L估计量L-estimator of location, 位置L估计量L-estimator of scale, 尺度L估计量Level, 水平Life expectance, 预期期望寿命Life table, 寿命表Life table method, 生命表法Light-tailed distribution, 轻尾分布Likelihood function, 似然函数Likelihood ratio, 似然比line graph, 线图Linear correlation, 直线相关Linear equation, 线性方程Linear programming, 线性规划Linear regression, 直线回归Linear Regression, 线性回归Linear trend, 线性趋势Loading, 载荷Location and scale equivariance, 位置尺度同变性Location equivariance, 位置同变性Location invariance, 位置不变性Location scale family, 位置尺度族Log rank test, 时序检验Logarithmic curve, 对数曲线Logarithmic normal distribution, 对数正态分布Logarithmic scale, 对数尺度Logarithmic transformation, 对数变换Logic check, 逻辑检查Logistic distribution, 逻辑斯特分布Logit transformation, Logit转换LOGLINEAR, 多维列联表通用模型Lognormal distribution, 对数正态分布Lost function, 损失函数Low correlation, 低度相关Lower limit, 下限Lowest-attained variance, 最小可达方差LSD, 最小显著差法的简称Lurking variable, 潜在变量Main effect, 主效应Major heading, 主辞标目Marginal density function, 边缘密度函数Marginal probability, 边缘概率Marginal probability distribution, 边缘概率分布Matched data, 配对资料Matched distribution, 匹配过分布Matching of distribution, 分布的匹配Matching of transformation, 变换的匹配Mathematical expectation, 数学期望Mathematical model, 数学模型Maximum L-estimator, 极大极小L 估计量Maximum likelihood method, 最大似然法Mean, 均数Mean squares between groups, 组间均方Mean squares within group, 组内均方Means (Compare means), 均值-均值比较Median, 中位数Median effective dose, 半数效量Median lethal dose, 半数致死量Median polish, 中位数平滑Median test, 中位数检验Minimal sufficient statistic, 最小充分统计量Minimum distance estimation, 最小距离估计Minimum effective dose, 最小有效量Minimum lethal dose, 最小致死量Minimum variance estimator, 最小方差估计量MINITAB, 统计软件包Minor heading, 宾词标目Missing data, 缺失值Model specification, 模型的确定Modeling Statistics , 模型统计Models for outliers, 离群值模型Modifying the model, 模型的修正Modulus of continuity, 连续性模Morbidity, 发病率Most favorable configuration, 最有利构形Multidimensional Scaling (ASCAL), 多维尺度/多维标度Multinomial Logistic Regression , 多项逻辑斯蒂回归Multiple comparison, 多重比较Multiple correlation , 复相关Multiple covariance, 多元协方差Multiple linear regression, 多元线性回归Multiple response , 多重选项Multiple solutions, 多解Multiplication theorem, 乘法定理Multiresponse, 多元响应Multi-stage sampling, 多阶段抽样Multivariate T distribution, 多元T分布Mutual exclusive, 互不相容Mutual independence, 互相独立Natural boundary, 自然边界Natural dead, 自然死亡Natural zero, 自然零Negative correlation, 负相关Negative linear correlation, 负线性相关Negatively skewed, 负偏Newman-Keuls method, q检验NK method, q检验No statistical significance, 无统计意义Nominal variable, 名义变量Nonconstancy of variability, 变异的非定常性Nonlinear regression, 非线性相关Nonparametric statistics, 非参数统计Nonparametric test, 非参数检验Nonparametric tests, 非参数检验Normal deviate, 正态离差Normal distribution, 正态分布Normal equation, 正规方程组Normal ranges, 正常范围Normal value, 正常值Nuisance parameter, 多余参数/讨厌参数Null hypothesis, 无效假设Numerical variable, 数值变量Objective function, 目标函数Observation unit, 观察单位Observed value, 观察值One sided test, 单侧检验One-way analysis of variance, 单因素方差分析Oneway ANOVA , 单因素方差分析Open sequential trial, 开放型序贯设计Optrim, 优切尾Optrim efficiency, 优切尾效率Order statistics, 顺序统计量Ordered categories, 有序分类Ordinal logistic regression , 序数逻辑斯蒂回归Ordinal variable, 有序变量Orthogonal basis, 正交基Orthogonal design, 正交试验设计Orthogonality conditions, 正交条件ORTHOPLAN, 正交设计Outlier cutoffs, 离群值截断点Outliers, 极端值OVERALS , 多组变量的非线性正规相关Overshoot, 迭代过度Paired design, 配对设计Paired sample, 配对样本Pairwise slopes, 成对斜率Parabola, 抛物线Parallel tests, 平行试验Parameter, 参数Parametric statistics, 参数统计Parametric test, 参数检验Partial correlation, 偏相关Partial regression, 偏回归Partial sorting, 偏排序Partials residuals, 偏残差Pattern, 模式Pearson curves, 皮尔逊曲线Peeling, 退层Percent bar graph, 百分条形图Percentage, 百分比Percentile, 百分位数Percentile curves, 百分位曲线Periodicity, 周期性Permutation, 排列P-estimator, P估计量Pie graph, 饼图Pitman estimator, 皮特曼估计量Pivot, 枢轴量Planar, 平坦Planar assumption, 平面的假设PLANCARDS, 生成试验的计划卡Point estimation, 点估计Poisson distribution, 泊松分布Polishing, 平滑Polled standard deviation, 合并标准差Polled variance, 合并方差Polygon, 多边图Polynomial, 多项式Polynomial curve, 多项式曲线Population, 总体Population attributable risk, 人群归因危险度Positive correlation, 正相关Positively skewed, 正偏Posterior distribution, 后验分布Power of a test, 检验效能Precision, 精密度Predicted value, 预测值Preliminary analysis, 预备性分析Principal component analysis, 主成分分析Prior distribution, 先验分布Prior probability, 先验概率Probabilistic model, 概率模型probability, 概率Probability density, 概率密度Product moment, 乘积矩/协方差Profile trace, 截面迹图Proportion, 比/构成比Proportion allocation in stratified random sampling, 按比例分层随机抽样Proportionate, 成比例Proportionate sub-class numbers, 成比例次级组含量Prospective study, 前瞻性调查Proximities, 亲近性Pseudo F test, 近似F检验Pseudo model, 近似模型Pseudosigma, 伪标准差Purposive sampling, 有目的抽样QR decomposition, QR分解Quadratic approximation, 二次近似Qualitative classification, 属性分类Qualitative method, 定性方法Quantile-quantile plot, 分位数-分位数图/Q-Q图Quantitative analysis, 定量分析Quartile, 四分位数Quick Cluster, 快速聚类Radix sort, 基数排序Random allocation, 随机化分组Random blocks design, 随机区组设计Random event, 随机事件Randomization, 随机化Range, 极差/全距Rank correlation, 等级相关Rank sum test, 秩和检验Rank test, 秩检验Ranked data, 等级资料Rate, 比率Ratio, 比例Raw data, 原始资料Raw residual, 原始残差Rayleigh's test, 雷氏检验Rayleigh's Z, 雷氏Z值Reciprocal, 倒数Reciprocal transformation, 倒数变换Recording, 记录Redescending estimators, 回降估计量Reducing dimensions, 降维Re-expression, 重新表达Reference set, 标准组Region of acceptance, 接受域Regression coefficient, 回归系数Regression sum of square, 回归平方和Rejection point, 拒绝点Relative dispersion, 相对离散度Relative number, 相对数Reliability, 可靠性Reparametrization, 重新设置参数Replication, 重复Report Summaries, 报告摘要Residual sum of square, 剩余平方和Resistance, 耐抗性Resistant line, 耐抗线Resistant technique, 耐抗技术R-estimator of location, 位置R估计量R-estimator of scale, 尺度R估计量Retrospective study, 回顾性调查Ridge trace, 岭迹Ridit analysis, Ridit分析Rotation, 旋转Rounding, 舍入Row, 行Row effects, 行效应Row factor, 行因素RXC table, RXC表Sample, 样本Sample regression coefficient, 样本回归系数Sample size, 样本量Sample standard deviation, 样本标准差Sampling error, 抽样误差SAS(Statistical analysis system ), SAS统计软件包Scale, 尺度/量表Scatter diagram, 散点图Schematic plot, 示意图/简图Score test, 计分检验Screening, 筛检SEASON, 季节分析Second derivative, 二阶导数Second principal component, 第二主成分SEM (Structural equation modeling), 结构化方程模型Semi-logarithmic graph, 半对数图Semi-logarithmic paper, 半对数格纸Sensitivity curve, 敏感度曲线Sequential analysis, 贯序分析Sequential data set, 顺序数据集Sequential design, 贯序设计Sequential method, 贯序法Sequential test, 贯序检验法Serial tests, 系列试验Short-cut method, 简捷法Sigmoid curve, S形曲线Sign function, 正负号函数Sign test, 符号检验Signed rank, 符号秩Significance test, 显著性检验Significant figure, 有效数字Simple cluster sampling, 简单整群抽样Simple correlation, 简单相关Simple random sampling, 简单随机抽样Simple regression, 简单回归simple table, 简单表Sine estimator, 正弦估计量Single-valued estimate, 单值估计Singular matrix, 奇异矩阵Skewed distribution, 偏斜分布Skewness, 偏度Slash distribution, 斜线分布Slope, 斜率Smirnov test, 斯米尔诺夫检验Source of variation, 变异来源Spearman rank correlation, 斯皮尔曼等级相关Specific factor, 特殊因子Specific factor variance, 特殊因子方差Spectra , 频谱Spherical distribution, 球型正态分布Spread, 展布SPSS(Statistical package for the social science), SPSS统计软件包Spurious correlation, 假性相关Square root transformation, 平方根变换Stabilizing variance, 稳定方差Standard deviation, 标准差Standard error, 标准误Standard error of difference, 差别的标准误Standard error of estimate, 标准估计误差Standard error of rate, 率的标准误Standard normal distribution, 标准正态分布Standardization, 标准化Starting value, 起始值Statistic, 统计量Statistical control, 统计控制Statistical graph, 统计图Statistical inference, 统计推断Statistical table, 统计表Steepest descent, 最速下降法Stem and leaf display, 茎叶图Step factor, 步长因子Stepwise regression, 逐步回归Storage, 存Strata, 层(复数)Stratified sampling, 分层抽样Stratified sampling, 分层抽样Strength, 强度Stringency, 严密性Structural relationship, 结构关系Studentized residual, 学生化残差/t化残差Sub-class numbers, 次级组含量Subdividing, 分割Sufficient statistic, 充分统计量Sum of products, 积和Sum of squares, 离差平方和Sum of squares about regression, 回归平方和Sum of squares between groups, 组间平方和Sum of squares of partial regression, 偏回归平方和Sure event, 必然事件Survey, 调查Survival, 生存分析Survival rate, 生存率Suspended root gram, 悬吊根图Symmetry, 对称Systematic error, 系统误差Systematic sampling, 系统抽样Tags, 标签Tail area, 尾部面积Tail length, 尾长Tail weight, 尾重Tangent line, 切线Target distribution, 目标分布Taylor series, 泰勒级数Tendency of dispersion, 离散趋势Testing of hypotheses, 假设检验Theoretical frequency, 理论频数Time series, 时间序列Tolerance interval, 容忍区间Tolerance lower limit, 容忍下限Tolerance upper limit, 容忍上限Torsion, 扰率Total sum of square, 总平方和Total variation, 总变异Transformation, 转换Treatment, 处理Trend, 趋势Trend of percentage, 百分比趋势Trial, 试验Trial and error method, 试错法Tuning constant, 细调常数Two sided test, 双向检验Two-stage least squares, 二阶最小平方Two-stage sampling, 二阶段抽样Two-tailed test, 双侧检验Two-way analysis of variance, 双因素方差分析Two-way table, 双向表Type I error, 一类错误/α错误Type II error, 二类错误/β错误UMVU, 方差一致最小无偏估计简称Unbiased estimate, 无偏估计Unconstrained nonlinear regression , 无约束非线性回归Unequal subclass number, 不等次级组含量Ungrouped data, 不分组资料Uniform coordinate, 均匀坐标Uniform distribution, 均匀分布Uniformly minimum variance unbiased estimate, 方差一致最小无偏估计Unit, 单元Unordered categories, 无序分类Upper limit, 上限Upward rank, 升秩Vague concept, 模糊概念Validity, 有效性VARCOMP (Variance component estimation), 方差元素估计Variability, 变异性Variable, 变量Variance, 方差Variation, 变异Varimax orthogonal rotation, 方差最大正交旋转Volume of distribution, 容积W test, W检验Weibull distribution, 威布尔分布Weight, 权数Weighted Chi-square test, 加权卡方检验/Cochran检验Weighted linear regression method, 加权直线回归Weighted mean, 加权平均数Weighted mean square, 加权平均方差Weighted sum of square, 加权平方和Weighting coefficient, 权重系数Weighting method, 加权法W-estimation, W估计量W-estimation of location, 位置W估计量Width, 宽度Wilcoxon paired test, 威斯康星配对法/配对符号秩和检验Wild point, 野点/狂点Wild value, 野值/狂值Winsorized mean, 缩尾均值Withdraw, 失访Youden's index, 尤登指数Z test, Z检验Zero correlation, 零相关Z-transformation, Z变换。
RH问题
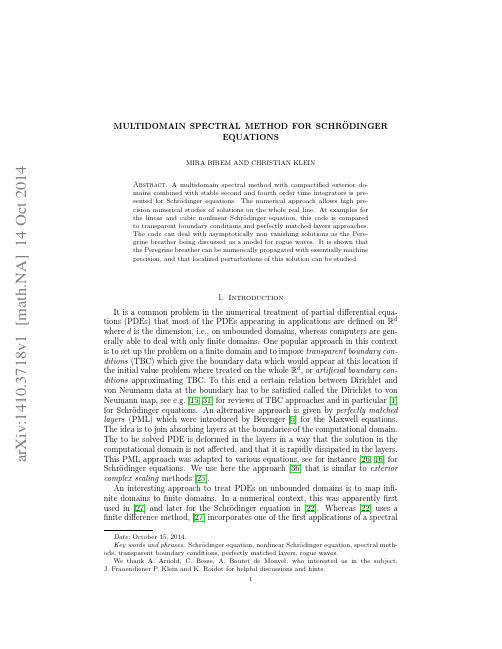
1. Introduction It is a common problem in the numerical treatment of partial differential equations (PDEs) that most of the PDEs appearing in applications are defined on Rd where d is the dimension, i.e., on unbounded domains, whereas computers are generally able to deal with only finite domains. One popular approach in this context is to set up the problem on a finite domain and to impose transparent boundary conditions (TBC) which give the boundary data which would appear at this location if the initial value problem where treated on the whole Rd , or artificial boundary conditions approximating TBC. To this end a certain relation between Dirichlet and von Neumann data at the boundary has to be satisfied called the Dirichlet to von Neumann map, see e.g. [15, 31] for reviews of TBC approaches and in particular [1] for Schrödinger equations. An alternative approach is given by perfectly matched layers (PML) which were introduced by Bérenger [5] for the Maxwell equations. The idea is to join absorbing layers at the boundaries of the computational domain. The to be solved PDE is deformed in the layers in a way that the solution in the computational domain is not affected, and that it is rapidly dissipated in the layers. This PML approach was adapted to various equations, see for instance [26, 16] for Schrödinger equations. We use here the approach [36] that is similar to exterior complex scaling methods [25]. An interesting approach to treat PDEs on unbounded domains is to map infinite domains to finite domains. In a numerical context, this was apparently first used in [27] and later for the Schrödinger equation in [22]. Whereas [22] uses a finite difference method, [27] incorporates one of the first applications of a spectral
Handbook of Numerical Analysis. Volume V. Techniques of Scientific Computing. (Part 2).

By P. G. Ciarlet and J. L. Lions. Elsevier, Inc., oretically oriented. The authors analyze the basic Amsterdam, 1997. 818 pp., hardcover. ISBN 0-444- spectral methods on model problems (Dirichlet and 82278-X. Neumann problems the Laplace and bilaplacian operators) for tensorized domains (intervals, squares, and This book contains ve solicited articles by experts cubes). This allows them to carry on a very deliin techniques of scienti c computing. These articles cate analysis which is not readily available in other are written mainly for researchers in related elds, sources. The usage of the tensorized bases of polytherefore, some preliminary knowledge is required. nomials is intended to take advantage of the properties of one-dimensional operators when possible, and, Numerical Path Following by E. L. Allgower and K. hence, to reduce the computational cost by some fast Georg is an extended and updated version of the au- algorithms. thors 1993 survey article 2]. However, the contents of Collocation method is the main emphasis and the the present article are substantially increased. This Galerkin method is discussed for the theoretical con200-page article provides more than 400 references venient. In some cases, Galerkin methods with indating to 1994. More detailed material up to 1990 can tegrals replaced by appropriate quadrature formube found in the authors' earlier book 1] where 900 las coincide with collocation methods. Two topics references are listed. Also, for numerical solutions of which are not considered in this article are the Tau multivariate polynomial systems by homotopy con- method and the Fourier series. The Legendre spectinuation methods, see the recent survey article by tral methods are extensively analyzed. The analysis is carried out on Sobolev spaces with a Jacobi type Li 5]. In the literature, \numerical path following" and weight which includes both Legendre and Chebyshev \numerical continuation method" are used inter- approximations in a uni ed framework. However, a changeably. Numerical continuation methods are number of new and important results are only stated techniques for numerically approximating a solution or proven for the Legendre techniques. More than a curve C which is implicitly de ned by an underdeter- quarter of the contents are devoted to spectral discretizations of the Navier-Stokes equations. The apmined system of equations. This article gives an introduction to the main ideas plication to hyperbolic systems of conservation laws of numerical path following and presents some ad- is brie y discussed. vances in the subject regarding adaptations, appli- Although the spectral method is closely related to cations, analysis of e ciency, and complexity. Both the p-version nite element method, only a few works theoretical and implementing aspects of the predic- on the p-version are quoted. About one quarter of 125 tor corrector path following methods are thoroughly references are the authors' own works. The newest discussed. Piecewise linear methods are also studied. reference is dated 1994. For the literature before At the end, a list for some available software related 1987, the reader is referred to 3] where more than 500 references are listed; additionally, more than 200 to path following is provided. supplemental references from 1986 to 1991 can be Spectral Methods by C. Bernardi and Y. Maday is found in the second and third printings of 3]. the longest article in Volume V (270 pages) which The article, which provides a solid theoretical founis based on the authors' 1992 book in French with dation for spectral methods by rigorous mathematia slight extension. Although the main advantage of cal arguments, is well organized and clearly written. spectral methods is in computational uid dynamics, For the readers' convenience, the authors even prothis article concerns mainly elliptic problems where vide a List of Symbols with the page numbers at the the theory is more complete and appealing. Com- end. The article is surely an important addition to pared with an early book by Canuto, Hussaini, Quar- the literature.
统计学专业英语翻译

汉译英Population 总体,样本总体sample 样本,标本parameter 限制因素median 中位数odd 奇数,单数even 偶数range 极差variance 方差standard deviation 标准差Covariance 协方差empty event 空事件product event 积事件conditional probability 条件概率Random variable 随机变量binominal distribution 二项式分布uniform distribution 均匀分布Poisson distribution 泊松分布residual 残差central limit theorem 中心极限定律英译汉descriptive statistics 描述统计学mathematical statistics 数理统计学inductive statistics 归纳统计学Inferential statistics 推断统计学dimension 维,维数continuous variable 连续变量ordinal variable 有序变量nominal variable 名义变量dichotomous 两分的;二歧的discrete variable 离散变量categorical variable 分类变量location 定位,位置,场所dispersion 分散mean 均值unimodal单峰的multimodal 多峰的chaotic 无秩序的grouped data 分组数据frequency distribution频数分布cumulative frequency 累加频数tallying 计算Uniformly distribution 均匀分布histogram 直方图frequency polygon 频率多边图rectangle 矩形Percentile 百分位数quartile 四分位数interquartile range 四分位数间距simple event 简单事件Compound event 复合事件mutually exclusive 互斥的,互补相交的complementary event 对立事件Independent 独立的joint probability function 联合概率函数jacobian雅克比行列式Law of large numbers大数定律point estimate 点估计estimate 估计值statistic 统计量optimality 最优性Unbiased estimate 无偏估计量efficient estimate 有偏估计量unbiasedness无偏性efficience有效性Consistent estimate 一致估计量asymptotic properties 渐近性质Confidence interval 置信区间interval estimation 区间估计null hypothesis 原假设alternative hypothesis 备择假设significance level 显著性水平power function 幂函数testing procedures 检验方法test statistic 检验统计量rejection region 拒绝区域acceptance region 接受区域critical region 临界区域first-derivatives 一阶导数second-derivatives 二阶导数Likelihood ratio 似然比dependent variable因变量unexplanatory variable未解释变量independent variable自变量Error term 误差项regression coefficients 回归系数Sum of squared residuals 残差平方和Marginal probability function 边际概率函数joint probability density function 联合概率密度函数Marginal probability density function边际概率密度函数stochastically independent 随机独立的Mutually independently distribution 相互独立的分布independently and identically distribution 独立同分布的likelihood function 似然函数maximum likelihood estimator 最大似然估计量maximum likelihood estimate 最大似然估计值log-likelihood function 对数似然函数ordinary least squares estimation/estimate/estimator 普通最小二乘估计/估计值/估计量linear unbiased estimator 线性无偏估计第三章、概念与符号[An index]把指数定义成是对一组相关变量之中变化进行测算的一个实数。
统计学术语中英文对照
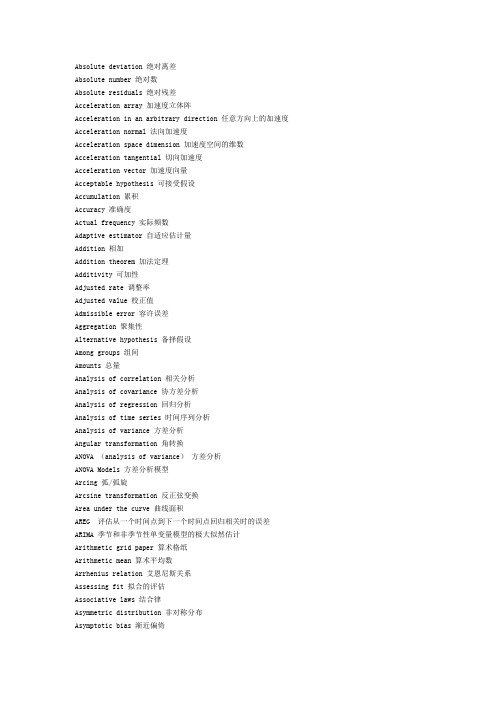
Absolute deviation 绝对离差Absolute number 绝对数Absolute residuals 绝对残差Acceleration array 加速度立体阵Acceleration in an arbitrary direction 任意方向上的加速度Acceleration normal 法向加速度Acceleration space dimension 加速度空间的维数Acceleration tangential 切向加速度Acceleration vector 加速度向量Acceptable hypothesis 可接受假设Accumulation 累积Accuracy 准确度Actual frequency 实际频数Adaptive estimator 自适应估计量Addition 相加Addition theorem 加法定理Additivity 可加性Adjusted rate 调整率Adjusted value 校正值Admissible error 容许误差Aggregation 聚集性Alternative hypothesis 备择假设Among groups 组间Amounts 总量Analysis of correlation 相关分析Analysis of covariance 协方差分析Analysis of regression 回归分析Analysis of time series 时间序列分析Analysis of variance 方差分析Angular transformation 角转换ANOVA (analysis of variance)方差分析ANOVA Models 方差分析模型Arcing 弧/弧旋Arcsine transformation 反正弦变换Area under the curve 曲线面积AREG 评估从一个时间点到下一个时间点回归相关时的误差ARIMA 季节和非季节性单变量模型的极大似然估计Arithmetic grid paper 算术格纸Arithmetic mean 算术平均数Arrhenius relation 艾恩尼斯关系Assessing fit 拟合的评估Associative laws 结合律Asymmetric distribution 非对称分布Asymptotic bias 渐近偏倚Asymptotic efficiency 渐近效率Asymptotic variance 渐近方差Attributable risk 归因危险度Attribute data 属性资料Attribution 属性Autocorrelation 自相关Autocorrelation of residuals 残差的自相关Average 平均数Average confidence interval length 平均置信区间长度Average growth rate 平均增长率Bar chart 条形图Bar graph 条形图Base period 基期Bayes' theorem Bayes定理Bell-shaped curve 钟形曲线Bernoulli distribution 伯努力分布Best-trim estimator 最好切尾估计量Bias 偏性Binary logistic regression 二元逻辑斯蒂回归Binomial distribution 二项分布Bisquare 双平方Bivariate Correlate 二变量相关Bivariate normal distribution 双变量正态分布Bivariate normal population 双变量正态总体Biweight interval 双权区间Biweight M-estimator 双权M估计量Block 区组/配伍组BMDP(Biomedical computer programs) BMDP统计软件包Boxplots 箱线图/箱尾图Breakdown bound 崩溃界/崩溃点Canonical correlation 典型相关Caption 纵标目Case-control study 病例对照研究Categorical variable 分类变量Catenary 悬链线Cauchy distribution 柯西分布Cause-and-effect relationship 因果关系Cell 单元Censoring 终检Center of symmetry 对称中心Centering and scaling 中心化和定标Central tendency 集中趋势Central value 中心值CHAID -χ2 Automatic Interaction Detector 卡方自动交互检测Chance 机遇Chance error 随机误差Chance variable 随机变量Characteristic equation 特征方程Characteristic root 特征根Characteristic vector 特征向量Chebshev criterion of fit 拟合的切比雪夫准则Chernoff faces 切尔诺夫脸谱图Chi-square test 卡方检验/χ2检验Choleskey decomposition 乔洛斯基分解Circle chart 圆图Class interval 组距Class mid-value 组中值Class upper limit 组上限Classified variable 分类变量Cluster analysis 聚类分析Cluster sampling 整群抽样Code 代码Coded data 编码数据Coding 编码Coefficient of contingency 列联系数Coefficient of determination 决定系数Coefficient of multiple correlation 多重相关系数Coefficient of partial correlation 偏相关系数Coefficient of production-moment correlation 积差相关系数Coefficient of rank correlation 等级相关系数Coefficient of regression 回归系数Coefficient of skewness 偏度系数Coefficient of variation 变异系数Cohort study 队列研究Column 列Column effect 列效应Column factor 列因素Combination pool 合并Combinative table 组合表Common factor 共性因子Common regression coefficient 公共回归系数Common value 共同值Common variance 公共方差Common variation 公共变异Communality variance 共性方差Comparability 可比性Comparison of bathes 批比较Comparison value 比较值Compartment model 分部模型Compassion 伸缩Complement of an event 补事件Complete association 完全正相关Complete dissociation 完全不相关Complete statistics 完备统计量Completely randomized design 完全随机化设计Composite event 联合事件Composite events 复合事件Concavity 凹性Conditional expectation 条件期望Conditional likelihood 条件似然Conditional probability 条件概率Conditionally linear 依条件线性Confidence interval 置信区间Confidence limit 置信限Confidence lower limit 置信下限Confidence upper limit 置信上限Confirmatory Factor Analysis 验证性因子分析Confirmatory research 证实性实验研究Confounding factor 混杂因素Conjoint 联合分析Consistency 相合性Consistency check 一致性检验Consistent asymptotically normal estimate 相合渐近正态估计Consistent estimate 相合估计Constrained nonlinear regression 受约束非线性回归Constraint 约束Contaminated distribution 污染分布Contaminated Gausssian 污染高斯分布Contaminated normal distribution 污染正态分布Contamination 污染Contamination model 污染模型Contingency table 列联表Contour 边界线Contribution rate 贡献率Control 对照Controlled experiments 对照实验Conventional depth 常规深度Convolution 卷积Corrected factor 校正因子Corrected mean 校正均值Correction coefficient 校正系数Correctness 正确性Correlation coefficient 相关系数Correlation index 相关指数Correspondence 对应Counting 计数Counts 计数/频数Covariance 协方差Covariant 共变Cox Regression Cox回归Criteria for fitting 拟合准则Criteria of least squares 最小二乘准则Critical ratio 临界比Critical region 拒绝域Critical value 临界值Cross-over design 交叉设计Cross-section analysis 横断面分析Cross-section survey 横断面调查Crosstabs 交叉表Cross-tabulation table 复合表Cube root 立方根Cumulative distribution function 分布函数Cumulative probability 累计概率Curvature 曲率/弯曲Curvature 曲率Curve fit 曲线拟和Curve fitting 曲线拟合Curvilinear regression 曲线回归Curvilinear relation 曲线关系Cut-and-try method 尝试法Cycle 周期Cyclist 周期性D test D检验Data acquisition 资料收集Data bank 数据库Data capacity 数据容量Data deficiencies 数据缺乏Data handling 数据处理Data manipulation 数据处理Data processing 数据处理Data reduction 数据缩减Data set 数据集Data sources 数据来源Data transformation 数据变换Data validity 数据有效性Data-in 数据输入Data-out 数据输出Dead time 停滞期Degree of freedom 自由度Degree of precision 精密度Degree of reliability 可靠性程度Degression 递减Density function 密度函数Density of data points 数据点的密度Dependent variable 应变量/依变量/因变量Dependent variable 因变量Depth 深度Derivative matrix 导数矩阵Derivative-free methods 无导数方法Design 设计Determinacy 确定性Determinant 行列式Determinant 决定因素Deviation 离差Deviation from average 离均差Diagnostic plot 诊断图Dichotomous variable 二分变量Differential equation 微分方程Direct standardization 直接标准化法Discrete variable 离散型变量DISCRIMINANT 判断Discriminant analysis 判别分析Discriminant coefficient 判别系数Discriminant function 判别值Dispersion 散布/分散度Disproportional 不成比例的Disproportionate sub-class numbers 不成比例次级组含量Distribution free 分布无关性/免分布Distribution shape 分布形状Distribution-free method 任意分布法Distributive laws 分配律Disturbance 随机扰动项Dose response curve 剂量反应曲线Double blind method 双盲法Double blind trial 双盲试验Double exponential distribution 双指数分布Double logarithmic 双对数Downward rank 降秩Dual-space plot 对偶空间图DUD 无导数方法Duncan's new multiple range method 新复极差法/Duncan新法Effect 实验效应Eigenvalue 特征值Eigenvector 特征向量Ellipse 椭圆Empirical distribution 经验分布Empirical probability 经验概率单位Enumeration data 计数资料Equal sun-class number 相等次级组含量Equally likely 等可能Equivariance 同变性Error 误差/错误Error of estimate 估计误差Error type I 第一类错误Error type II 第二类错误Estimand 被估量Estimated error mean squares 估计误差均方Estimated error sum of squares 估计误差平方和Euclidean distance 欧式距离Event 事件Event 事件Exceptional data point 异常数据点Expectation plane 期望平面Expectation surface 期望曲面Expected values 期望值Experiment 实验Experimental sampling 试验抽样Experimental unit 试验单位Explanatory variable 说明变量Exploratory data analysis 探索性数据分析Explore Summarize 探索-摘要Exponential curve 指数曲线Exponential growth 指数式增长EXSMOOTH 指数平滑方法Extended fit 扩充拟合Extra parameter 附加参数Extrapolation 外推法Extreme observation 末端观测值Extremes 极端值/极值F distribution F分布F test F检验Factor 因素/因子Factor analysis 因子分析Factor Analysis 因子分析Factor score 因子得分Factorial 阶乘Factorial design 析因试验设计False negative 假阴性False negative error 假阴性错误Family of distributions 分布族Family of estimators 估计量族Fanning 扇面Fatality rate 病死率Field investigation 现场调查Field survey 现场调查Finite population 有限总体Finite-sample 有限样本First derivative 一阶导数First principal component 第一主成分First quartile 第一四分位数Fisher information 费雪信息量Fitted value 拟合值Fitting a curve 曲线拟合Fixed base 定基Fluctuation 随机起伏Forecast 预测Four fold table 四格表Fourth 四分点Fraction blow 左侧比率Fractional error 相对误差Frequency 频率Frequency polygon 频数多边图Frontier point 界限点Function relationship 泛函关系Gamma distribution 伽玛分布Gauss increment 高斯增量Gaussian distribution 高斯分布/正态分布Gauss-Newton increment 高斯-牛顿增量General census 全面普查GENLOG (Generalized liner models) 广义线性模型Geometric mean 几何平均数Gini's mean difference 基尼均差GLM (General liner models) 通用线性模型Goodness of fit 拟和优度/配合度Gradient of determinant 行列式的梯度Graeco-Latin square 希腊拉丁方Grand mean 总均值Gross errors 重大错误Gross-error sensitivity 大错敏感度Group averages 分组平均Grouped data 分组资料Guessed mean 假定平均数Half-life 半衰期Hampel M-estimators 汉佩尔M估计量Happenstance 偶然事件Harmonic mean 调和均数Hazard function 风险均数Hazard rate 风险率Heading 标目Heavy-tailed distribution 重尾分布Hessian array 海森立体阵Heterogeneity 不同质Heterogeneity of variance 方差不齐Hierarchical classification 组内分组Hierarchical clustering method 系统聚类法High-leverage point 高杠杆率点HILOGLINEAR 多维列联表的层次对数线性模型Hinge 折叶点Histogram 直方图Historical cohort study 历史性队列研究Holes 空洞HOMALS 多重响应分析Homogeneity of variance 方差齐性Homogeneity test 齐性检验Huber M-estimators 休伯M估计量Hyperbola 双曲线Hypothesis testing 假设检验Hypothetical universe 假设总体Impossible event 不可能事件Independence 独立性Independent variable 自变量Index 指标/指数Indirect standardization 间接标准化法Individual 个体Inference band 推断带Infinite population 无限总体Infinitely great 无穷大Infinitely small 无穷小Influence curve 影响曲线Information capacity 信息容量Initial condition 初始条件Initial estimate 初始估计值Initial level 最初水平Interaction 交互作用Interaction terms 交互作用项Intercept 截距Interpolation 内插法Interquartile range 四分位距Interval estimation 区间估计Intervals of equal probability 等概率区间Intrinsic curvature 固有曲率Invariance 不变性Inverse matrix 逆矩阵Inverse probability 逆概率Inverse sine transformation 反正弦变换Iteration 迭代Jacobian determinant 雅可比行列式Joint distribution function 分布函数Joint probability 联合概率Joint probability distribution 联合概率分布K means method 逐步聚类法Kaplan-Meier 评估事件的时间长度Kaplan-Merier chart Kaplan-Merier图Kendall's rank correlation Kendall等级相关Kinetic 动力学Kolmogorov-Smirnove test 柯尔莫哥洛夫-斯米尔诺夫检验Kruskal and Wallis test Kruskal及Wallis检验/多样本的秩和检验/H检验Kurtosis 峰度Lack of fit 失拟Ladder of powers 幂阶梯Lag 滞后Large sample 大样本Large sample test 大样本检验Latin square 拉丁方Latin square design 拉丁方设计Leakage 泄漏Least favorable configuration 最不利构形Least favorable distribution 最不利分布Least significant difference 最小显著差法Least square method 最小二乘法Least-absolute-residuals estimates 最小绝对残差估计Least-absolute-residuals fit 最小绝对残差拟合Least-absolute-residuals line 最小绝对残差线Legend 图例L-estimator L估计量L-estimator of location 位置L估计量L-estimator of scale 尺度L估计量Level 水平Life expectance 预期期望寿命Life table 寿命表Life table method 生命表法Light-tailed distribution 轻尾分布Likelihood function 似然函数Likelihood ratio 似然比line graph 线图Linear correlation 直线相关Linear equation 线性方程Linear programming 线性规划Linear regression 直线回归Linear Regression 线性回归Linear trend 线性趋势Loading 载荷Location and scale equivariance 位置尺度同变性Location equivariance 位置同变性Location invariance 位置不变性Location scale family 位置尺度族Log rank test 时序检验Logarithmic curve 对数曲线Logarithmic normal distribution 对数正态分布Logarithmic scale 对数尺度Logarithmic transformation 对数变换Logic check 逻辑检查Logistic distribution 逻辑斯特分布Logit transformation Logit转换LOGLINEAR 多维列联表通用模型Lognormal distribution 对数正态分布Lost function 损失函数Low correlation 低度相关Lower limit 下限Lowest-attained variance 最小可达方差LSD 最小显著差法的简称Lurking variable 潜在变量Main effect 主效应Major heading 主辞标目Marginal density function 边缘密度函数Marginal probability 边缘概率Marginal probability distribution 边缘概率分布Matched data 配对资料Matched distribution 匹配过分布Matching of distribution 分布的匹配Matching of transformation 变换的匹配Mathematical expectation 数学期望Mathematical model 数学模型Maximum L-estimator 极大极小L 估计量Maximum likelihood method 最大似然法Mean 均数Mean squares between groups 组间均方Mean squares within group 组内均方Means (Compare means) 均值-均值比较Median 中位数Median effective dose 半数效量Median lethal dose 半数致死量Median polish 中位数平滑Median test 中位数检验Minimal sufficient statistic 最小充分统计量Minimum distance estimation 最小距离估计Minimum effective dose 最小有效量Minimum lethal dose 最小致死量Minimum variance estimator 最小方差估计量MINITAB 统计软件包Minor heading 宾词标目Missing data 缺失值Model specification 模型的确定Modeling Statistics 模型统计Models for outliers 离群值模型Modifying the model 模型的修正Modulus of continuity 连续性模Morbidity 发病率Most favorable configuration 最有利构形Multidimensional Scaling (ASCAL) 多维尺度/多维标度Multinomial Logistic Regression 多项逻辑斯蒂回归Multiple comparison 多重比较Multiple correlation 复相关Multiple covariance 多元协方差Multiple linear regression 多元线性回归Multiple response 多重选项Multiple solutions 多解Multiplication theorem 乘法定理Multiresponse 多元响应Multi-stage sampling 多阶段抽样Multivariate T distribution 多元T分布Mutual exclusive 互不相容Mutual independence 互相独立Natural boundary 自然边界Natural dead 自然死亡Natural zero 自然零Negative correlation 负相关Negative linear correlation 负线性相关Negatively skewed 负偏Newman-Keuls method q检验NK method q检验No statistical significance 无统计意义Nominal variable 名义变量Nonconstancy of variability 变异的非定常性Nonlinear regression 非线性相关Nonparametric statistics 非参数统计Nonparametric test 非参数检验Nonparametric tests 非参数检验Normal deviate 正态离差Normal distribution 正态分布Normal equation 正规方程组Normal ranges 正常范围Normal value 正常值Nuisance parameter 多余参数/讨厌参数Null hypothesis 无效假设Numerical variable 数值变量Objective function 目标函数Observation unit 观察单位Observed value 观察值One sided test 单侧检验One-way analysis of variance 单因素方差分析Oneway ANOVA 单因素方差分析Open sequential trial 开放型序贯设计Optrim 优切尾Optrim efficiency 优切尾效率Order statistics 顺序统计量Ordered categories 有序分类Ordinal logistic regression 序数逻辑斯蒂回归Ordinal variable 有序变量Orthogonal basis 正交基Orthogonal design 正交试验设计Orthogonality conditions 正交条件ORTHOPLAN 正交设计Outlier cutoffs 离群值截断点Outliers 极端值OVERALS 多组变量的非线性正规相关Overshoot 迭代过度Paired design 配对设计Paired sample 配对样本Pairwise slopes 成对斜率Parabola 抛物线Parallel tests 平行试验Parameter 参数Parametric statistics 参数统计Parametric test 参数检验Partial correlation 偏相关Partial regression 偏回归Partial sorting 偏排序Partials residuals 偏残差Pattern 模式Pearson curves 皮尔逊曲线Peeling 退层Percent bar graph 百分条形图Percentage 百分比Percentile 百分位数Percentile curves 百分位曲线Periodicity 周期性Permutation 排列P-estimator P估计量Pie graph 饼图Pitman estimator 皮特曼估计量Pivot 枢轴量Planar 平坦Planar assumption 平面的假设PLANCARDS 生成试验的计划卡Point estimation 点估计Poisson distribution 泊松分布Polishing 平滑Polled standard deviation 合并标准差Polled variance 合并方差Polygon 多边图Polynomial 多项式Polynomial curve 多项式曲线Population 总体Population attributable risk 人群归因危险度Positive correlation 正相关Positively skewed 正偏Posterior distribution 后验分布Power of a test 检验效能Precision 精密度Predicted value 预测值Preliminary analysis 预备性分析Principal component analysis 主成分分析Prior distribution 先验分布Prior probability 先验概率Probabilistic model 概率模型probability 概率Probability density 概率密度Product moment 乘积矩/协方差Profile trace 截面迹图Proportion 比/构成比Proportion allocation in stratified random sampling 按比例分层随机抽样Proportionate 成比例Proportionate sub-class numbers 成比例次级组含量Prospective study 前瞻性调查Proximities 亲近性Pseudo F test 近似F检验Pseudo model 近似模型Pseudosigma 伪标准差Purposive sampling 有目的抽样QR decomposition QR分解Quadratic approximation 二次近似Qualitative classification 属性分类Qualitative method 定性方法Quantile-quantile plot 分位数-分位数图/Q-Q图Quantitative analysis 定量分析Quartile 四分位数Quick Cluster 快速聚类Radix sort 基数排序Random allocation 随机化分组Random blocks design 随机区组设计Random event 随机事件Randomization 随机化Range 极差/全距Rank correlation 等级相关Rank sum test 秩和检验Rank test 秩检验Ranked data 等级资料Rate 比率Ratio 比例Raw data 原始资料Raw residual 原始残差Rayleigh's test 雷氏检验Rayleigh's Z 雷氏Z值Reciprocal 倒数Reciprocal transformation 倒数变换Recording 记录Redescending estimators 回降估计量Reducing dimensions 降维Re-expression 重新表达Reference set 标准组Region of acceptance 接受域Regression coefficient 回归系数Regression sum of square 回归平方和Rejection point 拒绝点Relative dispersion 相对离散度Relative number 相对数Reliability 可靠性Reparametrization 重新设置参数Replication 重复Report Summaries 报告摘要Residual sum of square 剩余平方和Resistance 耐抗性Resistant line 耐抗线Resistant technique 耐抗技术R-estimator of location 位置R估计量R-estimator of scale 尺度R估计量Retrospective study 回顾性调查Ridge trace 岭迹Ridit analysis Ridit分析Rotation 旋转Rounding 舍入Row 行Row effects 行效应Row factor 行因素RXC table RXC表Sample 样本Sample regression coefficient 样本回归系数Sample size 样本量Sample standard deviation 样本标准差Sampling error 抽样误差SAS(Statistical analysis system ) SAS统计软件包Scale 尺度/量表Scatter diagram 散点图Schematic plot 示意图/简图Score test 计分检验Screening 筛检SEASON 季节分析Second derivative 二阶导数Second principal component 第二主成分SEM (Structural equation modeling) 结构化方程模型Semi-logarithmic graph 半对数图Semi-logarithmic paper 半对数格纸Sensitivity curve 敏感度曲线Sequential analysis 贯序分析Sequential data set 顺序数据集Sequential design 贯序设计Sequential method 贯序法Sequential test 贯序检验法Serial tests 系列试验Short-cut method 简捷法Sigmoid curve S形曲线Sign function 正负号函数Sign test 符号检验Signed rank 符号秩Significance test 显著性检验Significant figure 有效数字Simple cluster sampling 简单整群抽样Simple correlation 简单相关Simple random sampling 简单随机抽样Simple regression 简单回归simple table 简单表Sine estimator 正弦估计量Single-valued estimate 单值估计Singular matrix 奇异矩阵Skewed distribution 偏斜分布Skewness 偏度Slash distribution 斜线分布Slope 斜率Smirnov test 斯米尔诺夫检验Source of variation 变异来源Spearman rank correlation 斯皮尔曼等级相关Specific factor 特殊因子Specific factor variance 特殊因子方差Spectra 频谱Spherical distribution 球型正态分布Spread 展布SPSS(Statistical package for the social science) SPSS统计软件包Spurious correlation 假性相关Square root transformation 平方根变换Stabilizing variance 稳定方差Standard deviation 标准差Standard error 标准误Standard error of difference 差别的标准误Standard error of estimate 标准估计误差Standard error of rate 率的标准误Standard normal distribution 标准正态分布Standardization 标准化Starting value 起始值Statistic 统计量Statistical control 统计控制Statistical graph 统计图Statistical inference 统计推断Statistical table 统计表Steepest descent 最速下降法Stem and leaf display 茎叶图Step factor 步长因子Stepwise regression 逐步回归Storage 存Strata 层(复数)Stratified sampling 分层抽样Stratified sampling 分层抽样Strength 强度Stringency 严密性Structural relationship 结构关系Studentized residual 学生化残差/t化残差Sub-class numbers 次级组含量Subdividing 分割Sufficient statistic 充分统计量Sum of products 积和Sum of squares 离差平方和Sum of squares about regression 回归平方和Sum of squares between groups 组间平方和Sum of squares of partial regression 偏回归平方和Sure event 必然事件Survey 调查Survival 生存分析Survival rate 生存率Suspended root gram 悬吊根图Symmetry 对称Systematic error 系统误差Systematic sampling 系统抽样Tags 标签Tail area 尾部面积Tail length 尾长Tail weight 尾重Tangent line 切线Target distribution 目标分布Taylor series 泰勒级数Tendency of dispersion 离散趋势Testing of hypotheses 假设检验Theoretical frequency 理论频数Time series 时间序列Tolerance interval 容忍区间Tolerance lower limit 容忍下限Tolerance upper limit 容忍上限Torsion 扰率Total sum of square 总平方和Total variation 总变异Transformation 转换Treatment 处理Trend 趋势Trend of percentage 百分比趋势Trial 试验Trial and error method 试错法Tuning constant 细调常数Two sided test 双向检验Two-stage least squares 二阶最小平方Two-stage sampling 二阶段抽样Two-tailed test 双侧检验Two-way analysis of variance 双因素方差分析Two-way table 双向表Type I error 一类错误/α错误Type II error 二类错误/β错误UMVU 方差一致最小无偏估计简称Unbiased estimate 无偏估计Unconstrained nonlinear regression 无约束非线性回归Unequal subclass number 不等次级组含量Ungrouped data 不分组资料Uniform coordinate 均匀坐标Uniform distribution 均匀分布Uniformly minimum variance unbiased estimate 方差一致最小无偏估计Unit 单元Unordered categories 无序分类Upper limit 上限Upward rank 升秩Vague concept 模糊概念Validity 有效性VARCOMP (Variance component estimation) 方差元素估计Variability 变异性Variable 变量Variance 方差Variation 变异Varimax orthogonal rotation 方差最大正交旋转Volume of distribution 容积W test W检验Weibull distribution 威布尔分布Weight 权数Weighted Chi-square test 加权卡方检验/Cochran检验Weighted linear regression method 加权直线回归Weighted mean 加权平均数Weighted mean square 加权平均方差Weighted sum of square 加权平方和Weighting coefficient 权重系数Weighting method 加权法W-estimation W估计量W-estimation of location 位置W估计量Width 宽度Wilcoxon paired test 威斯康星配对法/配对符号秩和检验Wild point 野点/狂点Wild value 野值/狂值Winsorized mean 缩尾均值Withdraw 失访Youden's index 尤登指数Z test Z检验Zero correlation 零相关Z-transformation Z变换。
Measuring Progress towards a Goal Estimating Teacher Productivity using a Multivariate Mult

Measuring Progress towards a Goal:Estimating Teacher Productivity using a Multivariate Multilevel Model for Value-Added AnalysisYeow Meng ThumGraduate School of Education and Information Studies,University of California,Los AngelesFINAL DRAFTAbstractThis paper develops a procedure for measuring how much is gained,and at what precision,by students in a pre-test and post-test situation againsta target score on the post-test.We define our productivity index,M j,forteacher j as the ratio of estimated gains to an estimated standard that is the distance between an estimate of the pre-test score and the target ing language,mathematics,and reading scores on the SAT9for 1999and2000from75public elementary classrooms(grades3,4,5,and 6in2000),we employ a Bayesian implementation of a multivariate mixed model for repeated test scores from individual students who in turn are nested within teachers.Our analysis point to statistically significant gains on the whole for grades3,4,and6.The strength of the approach lies in a straightforward estimation of the productivity ing the simulated sampling distribution of the posterior mean of the productivity index, we introduce a fuller depiction of progress in the productivity curve,or productivity profile,by calculating the probability that the index exceeds set proportions of the estimated standard.The basic model employed in this study thus contributes three essential components for sound accountability decisions.First,it estimates correlated measurement errors when using multiple measures.In doing so,we take full advantage of the informational redundancy in the measures.Second,it estimates initial status and value-added gains stly,it proposes a productivity index along with new procedures for representing the uncertainty in individual productivity estimates in the form of a productivity profile.This approach also facilitates a Bayesian effect-size analysis free from frequentist appeals to non-central t−or F−distributions.Keywords.Accountability models;Bayesian Effect size;Covariate ad-justment;Gain score;Hierarchical Bayes;Measuring change;Markov chain Monte Carlo;Multivariate multilevel modeling;Longitudinal data;Produc-tivity index;Productivity profile;Teacher productivity;Value-added.IntroductionThis article presents a general procedure for measuring teacher or school effectiveness based on student assessment.We begin with some caveats.By“assessment”we do not simply mean standardized test scores,grades,or the like,but the broader set of attainment,behavioral, attitudinal outcomes that might be relevant to our accountability concerns and that have the psychometric properties favorable to longitudinal analysis.The general approach does not rely on the use of test scores.Furthermore,the procedure is wholly concerned with characterizing the change observed even when the use of terms such as“effectiveness”or“productivity”does presume at least a palpable link between student learning and instructional quality.The procedure will accommodate stronger theories of student performance when they are available, such as those involving explicit notions of instructional quality.Finally,although our specific illustration consists of a pre-test score and a post-test score for a student,the model we present is generally suited for longitudinal or doubly multivariate analysis(Bock,1975).When compared with a classical analysis for the same model,the strengths of our Bayesian approach are evident.There are many accountability systems now in place.The specific form that each takes is a function of a host of factors that include data quality and technical constraints against the backdrop of a particular set of objectives for an accountability system.We limit ourselves to just two of these,namely,data and model.In the next sections of this paper,wefirst summarize the reasons for rejecting the usual residual gain score for measuring change in favor of treating both pre-test and post-test scores as outcomes in a multivariate mixed model.We then motivate the use of a norm-adjusted pre-test score for measuring change.A description of the data and the development of our model are taken up next.The analysis of our data is followed,lastly,with an illustration of the essential features of our productivity analysis based on the results of our model.Measuring Progress with Accountability Models:A SynopsisIn a recent review of assessment-based school reform in the United States,Linn(2000; 2001)concluded that many questions regarding the quality of assessment data and accountability models have remained.These problems have persisted due to our failure to adequately resolve the following related issues when we design and implement accountability systems for monitoring progress:1.Should attainment status be the focus,or should we focus on change?2.What is the right way to measure change?Do we use a regression-based adjustment or the gain score?This study was prepared for the Milken Family Foundation.The paper has benefited from close readings by Tony Bryk,Bill Sanders,Mike Seltzer,Emir Shuford,and Doug Willms.Sincere thanks to Dr.John Schacter of the Milken Family Foundation who managed this study,provided clarifications,insights and ments and suggestions from the editor and two anonymous reviewers significantly improved the paper.Opinions expressed in this paper are however the sole responsibility of this author,as are all remaining errors.Please direct all correspondence to Y.M. Thum,at thum@.3.How should student level information be aggregated for accountability use at the teacher, classroom,school,or district levels?Two recent developments in educational research have clarified the choices and their consequences for the questions above.Due to space limitations,only a brief synopsis is possible.Thefirst is a stream of work by Bryk and Weisberg(1976),Rogosa,Brandt,and Zimowski (1982),Rogosa and Willett(1985),Rogosa(1995),among others,that dispels the notion that gain scores are inherently unreliable(see,for example,the volume by Harris(1963)).Treating gains within a broader growth modeling framework for understanding individual change,Rogosa and his colleagues show that,first of all,the gain score is not inherently unreliable.They further demonstrate that the correlation between gain and initial status,long misconstrued as another inherent problem when using the gain score,can take many forms and that its magnitude is merely an artifact of the design on time and the joint distribution of initial status and gain.The nature of the correlation is the outcome of the parameterization we choose for the growth factors and,as a consequence,the meaning we attach to them(see Schuster and von Eye(1998)).(This is also sometimes known as the“centering problem.”)To fully describe development,we need to simultaneously describe both the level of performance(initial orfinal status)as well as evidence of improvement(gains).It is also pointed out that,conceptually,the true gain score,of which the observed gain score is an unbiased estimator,is exactly the quantity we wish to study in many educational studies of change.Nevertheless,researchers have frequently regressed the post-test score on the pre-test score,motivated by some notions of“fairness”in order to“level the playingfield”(e.g., Good,Biddle,and Brody(1975);Linn and Slinde(1977)).Regression residuals then seem to provide some“relative measure of gain,”but it cannot be considered a“corrected”measure of gain.It simply fails to tell us how much an individual has changed on an attribute(Cronbach& Furby,1970).Figure1depicts how the gain score is distinct from the residual gain score.Employing the regression metaphor,gains are also“residuals”when we set the intercept to be0and the slope at 1,or we simply set y i2−y i1.The regression of y i2on y i1produces E(y i2|y i1),with residualsy i2−E(y i2|y i1).When taken as a group,an average of individual gains can be different from zero whereas the residual gains score sums to zero by construction,so that a group mean of residual gain scores is not a particularly helpful quantity if one asks“How did the program impact the students as a group?”According to Willett(1988),who offered perhaps the most cogent defense of the gain score in a single source,additional technical problems attend the use of the residual gain score.Just like the post-test,the pre-test is measured with error so that a regression of post-test on pre-test score violates the basic regression assumption that covariates are error free.The result is that the residual gain score is an inefficient and inconsistent estimator of true residual change.Linear regression is also sensitive to the particular make-up of the sample.Outlying cases can exert undue influence on the solution.Thus the residual gain estimated for a subject may be heavily0246810-2.50.02.55.07.510.0y 2y 1Figure 1.Gains (Y i 2−Y i 1)vs.Residual Gain Score (Y i 2−E (Y i 2|Y i 1)).Observations on the identity line (dashes)indicate not change.impacted by whoever else contributes to determining the regression relationship.Finally,it iscurious why the exact rationale for adjusting the post-test does not apply to the pre-test itself.It is reasonable to expect that the same set of factors affecting the pre-test would affect thepost-test as well,perhaps even with about the same force.In contrast,the repeated measures approach we adopt in this report places the pre-test and the post-test on an equal footing in that both serve as outcomes in our models.On these issues,Allison (1990)arrived at essentiallyidentical conclusions.The “sea change”with respect to the clear preference for using gain scores in measuring change is evident in recent research (Collins,1996;Maassen,2000;Maris,1998;Mellenbergh,1999;Raykov,1999;Williams &Zimmerman,1996).The second development,variously known as “hierarchical”,“multilevel”or “mixed-effects”modeling,provided the methodological complement for a proper treatment of growth or change data in stratified sampling designs that are common in educational research settings –repeated measures on student over time,students nested with schools in the district,etc.One distinct advantage of this approach is that it is flexible with respect to the time structure of the observations.Equally important is that multilevel models further facilitate the study of theeffects of covariates on development at every level of nesting(Laird&Ware,1982;Bryk& Raudenbush,1992;Goldstein,1995;Snijders&Bosker,1999).Many current accountability systems employ some form of the multilevel model(Willms,1992;Willms&Raudenbush,1989; Sanders&Horn,1994;Webster&Mendro,1997;Gray,Jesson,Goldstein,Hedger,&Rabash, 1995;Harker&Nash,1996;Meyer,1996;Bryk,Thum,Easton,&Luppescu,1998)to cope with nested accountability data,although a closer comparison of actual implementations,which would aid broader conceptual and technical development,is still lacking.Another positive contribution with the advent of multilevel models is that they have helped accountability agencies avoid the misleading picture conveyed by computing score aggregates such as the school or district means and focus on student level change instead(Meyer,1996).In discussing school accountability systems today,the term“value-added”is ever conspicuous.Its origins can be traced to notions of goods,value and wealth in political economy (see Saunders(1999)for a general note with a view from the UK).An early use of this term in connection with evaluations of an intervention effect on an educational growth process is found in Bryk and Weisberg(1976).Its modern usage mostly conveys the idea of“relative progress.”A slightly more apolitical interpretation preferred by some statisticians recognizes that in whatever form it takes,value-added analyses center around a model for“adjusted comparison”(Goldstein &Spiegelhalter,1996).In educational accountability,this notion of differential effectiveness of a teacher or a school is most often equated with the output from a residual gains analysis based on successive student assessments.Prominent examples here in the US include the Dallas system(Webster&Mendro, 1997;Meyer,1996)and,overseas in the UK,a similar strategy is employed by Fitz-Gibbon (1997).From this author’s perspective on the measurement of change outlined above,the superior alternative for defining what value-added is employs a mixed-effects repeated measurement design (of which a growth model is a special case).Examples include the research of Sanders and Horn (1994)on the Tennessee Value-Added Assessment System,or TVAAS,and the analyses by Bryk et al.(1998)using longitudinal student assessments from the the Chicago Public Elementary Schools1.It is also important to note that the models employed in TVAAS and at Chicago estimates the true gains based on individual test scores and do not begin with the“raw gain score.”Such an approach to the study of gains makes better use of the available information and allows the analyst to isolate the variance-covariance structure of the tests.The work to be reported in this article extends the mixed-effects or hierarchical linear model for growth modelling in several ways.We develop a procedure for measuring how much is1That the different,yet equivalent,labels(hierarchical modeling,mixed-models)from the statistics literature have invaded popular educational research writing may have caused the general practitioner to misplace the critical subtleties in any particular application.Stone(1999),for example,rightly concludes that the mixed-model analysis associated with the TVAAS may be more precise and less vulnerable to manipulations,contrasting it with the sequence of hierarchical linear regression analyses used in Dallas,TX.These otherwise essentially equivalent modeling frameworks should not be mistaken as“competing”however.The principal distinction to make in any application, in our view,is how we define the“student gain”in the model.Which is,returning to Figure1,do we estimate gains as(Y i2−Y i1),or do we employ the residual gain estimate(Y i2−E(Y i2|Y i1))?gained,and at what precision,by students in a pre-test and post-test situation against a target score on the post-test.We define our productivity index,M j,for teacher or classroom j as the ratio of estimated gains to an estimated standard that is the distance between an estimate of the pre-test score and the target ing language,mathematics,and reading scores on the SAT 9for1999and2000from75public elementary classrooms(grades3,4,5,and6in2000),we employ a Bayesian implementation of a multivariate mixed model for repeated test scores from individual students who in turn are nested within teachers.The strength of the approach lies in a straightforward estimation of the productivity ing the simulated sampling distribution of the posterior mean of the productivity index,we introduce a fuller depiction of progress in the productivity curve,or productivity profile,by calculating the probability that the index exceeds set proportions of the standard.The basic model employed in this study thus contributes three essential components for sound accountability decisions.First,it estimates correlated measurement errors when using multiple measures.In doing so,we take full advantage of the informational redundancy in the measures.Second,it estimates initial status and value-added gains stly,it presents new procedures for representing the uncertainty in individual productivity estimates in the form of a productivity profile.Choice of Metric Matters for Measuring ChangeBroadly speaking,there are two necessary requirements for measuring change.First,the notion of“change”makes little sense if the construct itself has changed.Presuming that the construct is qualitatively constant,measuring change in a construct refers then to detecting changes in degree or in quantity.Thus,and second,measuring change in quantity is impossible if the measurement instrument,or the scale,itself changes in unknown ways.Referring to measuring change in quantity,Seltzer,Frank,and Bryk(1994)show that the choice of metric matters in conveying the results from student assessments.More general points concerning scales that are commonly employed in reporting educational assessment results are found in Glass and Hopkins(1984).For most testing systems,the national percentile rank(NPR)is frequently employed for comparing students.This is a norm-referenced score scale constructed by comparing the student raw score with the average raw score of students on the same test in a national norming sample. When a third grade student scores27on the NPR,we understand that he/she has scored higher than27%of the students in the norming group for third grade.It makes good sense for comparing the relative standing of students from the norm group.Its utility beyond such comparisons is however limited.Although its values range from1to99from test to test and from grade to grade,it is important to recognize that it is(norm)group dependent.A raw score in the third grade for example may be higher or lower on a grade2or a grade5NPR scale. Furthermore,the NPR is a rank and so is not amenable to arithmetic manipulations,such as taking an average,or for interpolation.Each of these two facts about the NPR metric makes it a poor candidate for measuring change in learning.Another popular candidate for comparing amounts of learning is the normal curve equivalent(NCE).It is simply the normal deviate of the corresponding percentile score, standardized to a mean of50and a standard deviation of21.06so that its scale values are now amendable to arithmetic manipulations for scores referring to the same norm.On this scale,two equal differences in third grade reading NCE’s measure the same amount of relative progress in third grade reading achievement.The comparison is even valid when comparing one third grade reading NCE from one year with another third grade reading NCE for a second year.However, just like its parent,the NPR,it is still norm dependent,and as such a given NCE score does not indicate the same quantity,say,from one grade to the next grade,where a zero difference implies “normal growth.”With regard to this“group-based standard score”Hoover(1984b,p.12),Jaeger (1993,pp.95–97)characterized its seeming advantage as“dubious.”It is therefore also unsuited for measuring learning change from one grade to the next(see,for example,Slavin and Madden (1991)).Hills(1984)provided helpful hints as to the meaning and proper use of the NCE.The third candidate we must mention here is the grade equivalent(GE)score.A GE of4.5 is the average score of fourth graders in thefifth month of the school year.It is an improvement over the percentile rank in the sense that within a norm group its values tell the user how he/she is performing at any time during the school year when compared to students in the same norm group.With some notion of time built into its norming,it seems to supply a ready basis for conveying development.But measuring change requires a stronger scale.Recall that the GE values are just labels for norm groups,and so the GE remains a norm-referenced score.For example,a fourth grader who receives a GE of5.3on a fourth grade test is performing like the averagefifth grade student after three months in grade5on the same fourth grade assessment; not that this fourth grader is performing like the averagefifth grader after three months infifth grade on thefifth grade test.The reader may wish to consult an extended discussion of the limits of the GE by Coleman and Karweit(1972),Eissenberg and Rudner(1988),Schulz and Nicewander(1997).See Hoover(1984b,1984a)and Burket(1984)for a revealing exchange on the value of the GE for measuring educational development.Like the NPR and the NCE,we conclude that the GE is again unsuited for measuring learning gains.Finally,the scale score is perhaps the least used for popular communication about student performance although it is designed for the sole purpose of directly measuring amounts of learning or ability.It is a content-referenced scale.The scale score has the necessary psychometric qualities for comparing differential performance on an attribute.Scale scores are placed on an interval scale,and more importantly for most testing systems,scores have been equated vertically from one grade to another and from one year to the next.A scale score of520for reading means the same level of attainment of the reading content from grade to grade.Furthermore,the scaling procedure can produce an estimate of precision(its standard error of measurement,or SEM,a measure of precision)from each student’s responses to his/her test.This precision information should be better utilized if we are to seriously weigh each individual pieces of information for accountability purposes.For an example of an accountability model that uses precision weightedscale scores,see Bryk et al.(1998).What is hard to comprehend is the common failure to realize that the NPR,and hence the NCE that is derived from it,is simply a percentile ranking of scale scores for a specific test form.Thum(2003)argued that accountability modeling should be performed on a metric appropriate for measuring growth(such as the scale score)and that the reporting of the results can return to a metric perhaps better suited for communicating other aspects of the score distribution(such as the NPR).In sum,it bears repeating that the scale score is not just the preferred alternative for the study of change;of the various choices discussed above,it is the only option.2Arizona TAP DataAn illustration of our approach employs data from a set of Arizona elementary schools.It should be noted that,in time,the performance-based accountability system will employ a wider set of outcomes than student standardized test scores.For more information regarding its goals and architecture,the reader should consult Schacter(2001).Table1lists the variables to be employed along with their definitions.Additional variables, especially those that would permit the tracking of students more closely as they move from teacher to teacher will be critical for refining our definition of teacher effectiveness.The data is limited to the Standard Achievement Tests(SAT)9test scores for1999and2000on three subjects,language,mathematics and reading.A total of1,276students who attended grades3,4, 5,and6at the4participating schools provided a maximum of6scores each.In all,observations total3,598.It should be noted that data collection is underway to add additional test scores from 1998and now2001for these students.Also slated for later inclusion are additional schools matched on location and demographics in order to bolster our evaluation of this accountability instrument.More contextual information will be added in order to better place this particular sample among the relevant student population in Arizona.Sample statistics for the75participating teachers are given in Table2.We see that LEP students are concentrated in School1,with classrooms that may serve as much as80%of such students.This school also has a larger proportion of Hispanic students in comparison.Overall, students in School1appear ill-prepared,as judged by the higher average in the proportion of students who do not score above the30th percentile in any of the tested subjects(language, mathematics,and reading).School3also has a larger proportion of Hispanic students but on the whole is comparable to the other two schools that serve more advantaged stly,the pattern of correlations for these classroom composition variables should be examined with caution.The variables are highly skewed and often reflect practices of administrative assignments,2The goal of measuring learning growth with an eye towards understanding what it is that a child has learned over time motivates the choice of tests and scale requirements.Hence,we have argued for a criterion-referenced test aligned with curricular objectives and implementation that is reported on vertically scaled metric.See,however,Yen (1986)for a review of the difficulties inherent in choosing a scale for measuring educational growth,including the scale score.There is unfortunately no real substitute for these requirements in principle in order to construct a sound accountability system.Furthermore,we can also expect to devote significant resources to maintaining the validity of any measurement system in practice.Table1:Student Outcomes,Covariates and Teacher FactorsOutcomes,1999&2000LANGUAGE Language(Form SA/TA)Scale ScoreMATHEMATICS Mathematics Total Scale ScoreREADING Reading Total Scale ScoreStudent Covariates(dummy variables)FEMALE gender,“1”for female and“0”for malesAF-AM ethnicity,“1”for African American and“0”otherwiseHISPANIC ethnicity,“1”for Hispanic and“0”otherwiseLEP Limited English Proficiency status,“1”for LEP and“0”otherwiseGIFTED Gifted status,“1”if designated as“gifted”and“0”otherwiseSPECED Special Education status,“1”for Special Ed.and“0”otherwiseLOWNPR99Low Prior Attainment if not a Special Education student,“1”if the maximum National Percentile Rank(NPR)for1999SAT9Langauge,Mathematics,or Reading is less than30,“0”otherwiseTeacher/Classroom FactorstAF-AM Proportion of students who are African AmericanstHISPANIC Proportion of students who are HispanicstLEP Proportion of students who are designated as Limited English Proficiency status tGIFTED Proportion of students who are designated as“gifted”tSPECED Proportion of students who are designated as Special EducationtLOWNPR99Proportion of students who are LowNPR99some of which may be unique to one or two schools.Not reported in the table is the distribution of class sizes.Classrooms hover at about17 students on average and all75classrooms but one have under30students.Of the75teachers,10 have fewer than10students in their classrooms.While we employ their data in our accountability models,we do not provide productivity estimates for these teachers,mainly because their estimates would not be sufficiently reliable.We employ similar variables at the student level(see Table3).As before,the entries represent values for the participating program schools only and so they should be judged with caution until we receive more information about these students in relation to Arizona’s student populations.The data are however adequate for illustrating the productivity measures to be developed next.Adequate Yearly ProgressAmong the numerous attempts to define adequate yearly progress,there has always been room for local standards and on-site inputs.In consultation with participating teachers in our sample schools,it seems reasonable to expect that,at a minimum,students maintain their pre-test rank on their post-test score when they show“adequate progress”.We therefore consider what we will call a“norm-adjusted pre-test score,”or NAPS,although it should be clear that our generalTable2:Teachers(N=75):Summary StatisticsSchool1234N15173310Grade35893439935308364071Means and Standard DeviationsFactors mean(s.d.)mean(s.d.)mean(s.d.)mean(s.d.)tLEP0.417(0.269)0.045(0.078)0.067(0.115)0.019(0.033)tSPECED0.132(0.256)0.115(0.315)0.138(0.312)0.060(0.037)tGIFTED0.088(0.068)0.141(0.325)0.082(0.091)0.076(0.073)tAF-AM0.013(0.028)0.078(0.077)0.101(0.101)0.038(0.038)tHISPANIC0.461(0.210)0.160(0.100)0.452(0.198)0.047(0.058)tLowNPR990.329(0.142)0.164(0.133)0.146(0.124)0.112(0.087)CorrelationsFactors tLEP tSPECED tGIFTED tAF-AM tHISPANIC tSPECED0.11296tGIFTED-0.03961-0.19044tAF-AM-0.262020.18682-0.15583tHISPANIC0.486870.19067-0.13493-0.07846tLOWNPR990.28489-0.38414-0.189230.009180.15528approach to measuring progress towards a goal does not depend on our choosing this quantity3.Another motivation for this choice can be better explained by referring the reader to Figure2which plots the relationships between the scale score and the percentile rank for grades 3,4,and5.Note that although the same scale score measures the same global attainment,it has a different rank score for each grade(higher for higher grades,as it should).Suppose that a student scores“O”on the third grade test.His percentile rank is30for the third grade but only about20on the fourth grade scale.Now suppose the student scores“C”on the fourth grade test. The usual estimate of his/her gain is measured by the distance between point“O”and point“C”, or“CO.”However,in terms of percentile rank,on the fourth grade scale,a naive reading gives an impression that the gain is between20%and37%,when the percentile rank for the same pre-test is actually30%on the third grade scale.The seeming“discrepancy”in the percentile rank for the same scale score“O”when we move from the third to the fourth grade scale suggests that perhaps an alternative to the raw scale score might be constructed by substituting score“B”for “O.”“B”,the scale score value on the fourth grade scale sharing the same percentile rank on the third grade scale,is then the NAPS score.Given the relationship between percentile ranks and scale scores across grades,this decision 3In an extended report,analyses based on the NAPS will be compared with results using the original pre-test. Preliminary results suggest lower gains on average for the raw scale score,as expected,with minor differences in the effects of covariate.。
统计学术语中英文对照
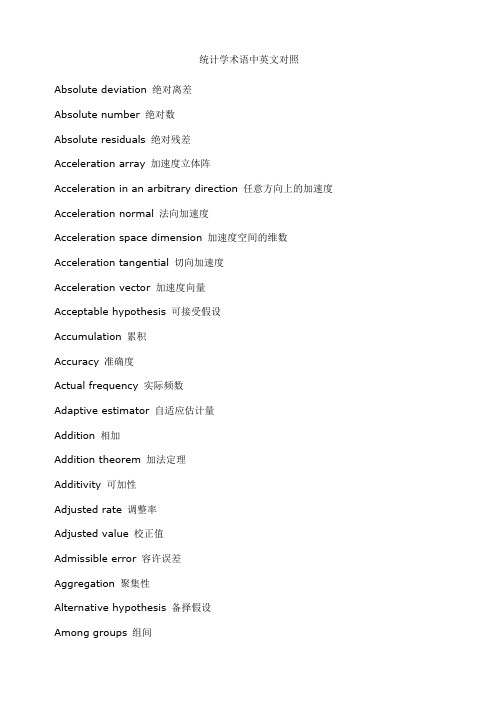
统计学术语中英文对照Absolute deviation 绝对离差Absolute number 绝对数Absolute residuals 绝对残差Acceleration array 加速度立体阵Acceleration in an arbitrary direction 任意方向上的加速度Acceleration normal 法向加速度Acceleration space dimension 加速度空间的维数Acceleration tangential 切向加速度Acceleration vector 加速度向量Acceptable hypothesis 可接受假设Accumulation 累积Accuracy 准确度Actual frequency 实际频数Adaptive estimator 自适应估计量Addition 相加Addition theorem 加法定理Additivity 可加性Adjusted rate 调整率Adjusted value 校正值Admissible error 容许误差Aggregation 聚集性Alternative hypothesis 备择假设Among groups 组间Amounts 总量Analysis of correlation 相关分析Analysis of covariance 协方差分析Analysis of regression 回归分析Analysis of time series 时间序列分析Analysis of variance 方差分析Angular transformation 角转换ANOVA (analysis of variance)方差分析ANOVA Models 方差分析模型Arcing 弧/弧旋Arcsine transformation 反正弦变换Area under the curve 曲线面积AREG 评估从一个时间点到下一个时间点回归相关时的误差ARIMA 季节和非季节性单变量模型的极大似然估计Arithmetic grid paper 算术格纸Arithmetic mean 算术平均数Arrhenius relation 艾恩尼斯关系Assessing fit 拟合的评估Associative laws 结合律Asymmetric distribution 非对称分布Asymptotic bias 渐近偏倚Asymptotic efficiency 渐近效率Asymptotic variance 渐近方差Attributable risk 归因危险度Attribute data 属性资料Attribution 属性Autocorrelation 自相关Autocorrelation of residuals 残差的自相关Average 平均数Average confidence interval length 平均置信区间长度Average growth rate 平均增长率Bar chart 条形图Bar graph 条形图Base period 基期Bayes' theorem Bayes定理Bell-shaped curve 钟形曲线Bernoulli distribution 伯努力分布Best-trim estimator 最好切尾估计量Bias 偏性Binary logistic regression 二元逻辑斯蒂回归Binomial distribution 二项分布Bisquare 双平方Bivariate Correlate 二变量相关Bivariate normal distribution 双变量正态分布Bivariate normal population 双变量正态总体Biweight interval 双权区间Biweight M-estimator 双权M估计量Block 区组/配伍组BMDP(Biomedical computer programs) BMDP统计软件包Boxplots 箱线图/箱尾图Breakdown bound 崩溃界/崩溃点Canonical correlation 典型相关Caption 纵标目Case-control study 病例对照研究Categorical variable 分类变量Catenary 悬链线Cauchy distribution 柯西分布Cause-and-effect relationship 因果关系Cell 单元Censoring 终检Center of symmetry 对称中心Centering and scaling 中心化和定标Central tendency 集中趋势Central value 中心值CHAID -χ2 Automatic Interaction Detector 卡方自动交互检测Chance 机遇Chance error 随机误差Chance variable 随机变量Characteristic equation 特征方程Characteristic root 特征根Characteristic vector 特征向量Chebshev criterion of fit 拟合的切比雪夫准则Chernoff faces 切尔诺夫脸谱图Chi-square test 卡方检验/χ2检验Choleskey decomposition 乔洛斯基分解Circle chart 圆图Class interval 组距Class mid-value 组中值Class upper limit 组上限Classified variable 分类变量Cluster analysis 聚类分析Cluster sampling 整群抽样Code 代码Coded data 编码数据Coding 编码Coefficient of contingency 列联系数Coefficient of determination 决定系数Coefficient of multiple correlation 多重相关系数Coefficient of partial correlation 偏相关系数Coefficient of production-moment correlation 积差相关系数Coefficient of rank correlation 等级相关系数Coefficient of regression 回归系数Coefficient of skewness 偏度系数Coefficient of variation 变异系数Cohort study 队列研究Column 列Column effect 列效应Column factor 列因素Combination pool 合并Combinative table 组合表Common factor 共性因子Common regression coefficient 公共回归系数Common value 共同值Common variance 公共方差Common variation 公共变异Communality variance 共性方差Comparability 可比性Comparison of bathes 批比较Comparison value 比较值Compartment model 分部模型Compassion 伸缩Complement of an event 补事件Complete association 完全正相关Complete dissociation 完全不相关Complete statistics 完备统计量Completely randomized design 完全随机化设计Composite event 联合事件Composite events 复合事件Concavity 凹性Conditional expectation 条件期望Conditional likelihood 条件似然Conditional probability 条件概率Conditionally linear 依条件线性Confidence interval 置信区间Confidence limit 置信限Confidence lower limit 置信下限Confidence upper limit 置信上限Confirmatory Factor Analysis 验证性因子分析Confirmatory research 证实性实验研究Confounding factor 混杂因素Conjoint 联合分析Consistency 相合性Consistency check 一致性检验Consistent asymptotically normal estimate 相合渐近正态估计Consistent estimate 相合估计Constrained nonlinear regression 受约束非线性回归Constraint 约束Contaminated distribution 污染分布Contaminated Gausssian 污染高斯分布Contaminated normal distribution 污染正态分布Contamination 污染Contamination model 污染模型Contingency table 列联表Contour 边界线Contribution rate 贡献率Control 对照Controlled experiments 对照实验Conventional depth 常规深度Convolution 卷积Corrected factor 校正因子Corrected mean 校正均值Correction coefficient 校正系数Correctness 正确性Correlation coefficient 相关系数Correlation index 相关指数Correspondence 对应Counting 计数Counts 计数/频数Covariance 协方差Covariant 共变Cox Regression Cox回归Criteria for fitting 拟合准则Criteria of least squares 最小二乘准则Critical ratio 临界比Critical region 拒绝域Critical value 临界值Cross-over design 交叉设计Cross-section analysis 横断面分析Cross-section survey 横断面调查Crosstabs 交叉表Cross-tabulation table 复合表Cube root 立方根Cumulative distribution function 分布函数Cumulative probability 累计概率Curvature 曲率/弯曲Curvature 曲率Curve fit 曲线拟和Curve fitting 曲线拟合Curvilinear regression 曲线回归Curvilinear relation 曲线关系Cut-and-try method 尝试法Cycle 周期Cyclist 周期性D test D检验Data acquisition 资料收集Data bank 数据库Data capacity 数据容量Data deficiencies 数据缺乏Data handling 数据处理Data manipulation 数据处理Data processing 数据处理Data reduction 数据缩减Data set 数据集Data sources 数据来源Data transformation 数据变换Data validity 数据有效性Data-in 数据输入Data-out 数据输出Dead time 停滞期Degree of freedom 自由度Degree of precision 精密度Degree of reliability 可靠性程度Degression 递减Density function 密度函数Density of data points 数据点的密度Dependent variable 应变量/依变量/因变量Dependent variable 因变量Depth 深度Derivative matrix 导数矩阵Derivative-free methods 无导数方法Design 设计Determinacy 确定性Determinant 行列式Determinant 决定因素Deviation 离差Deviation from average 离均差Diagnostic plot 诊断图Dichotomous variable 二分变量Differential equation 微分方程Direct standardization 直接标准化法Discrete variable 离散型变量DISCRIMINANT 判断Discriminant analysis 判别分析Discriminant coefficient 判别系数Discriminant function 判别值Dispersion 散布/分散度Disproportional 不成比例的Disproportionate sub-class numbers 不成比例次级组含量Distribution free 分布无关性/免分布Distribution shape 分布形状Distribution-free method 任意分布法Distributive laws 分配律Disturbance 随机扰动项Dose response curve 剂量反应曲线Double blind method 双盲法Double blind trial 双盲试验Double exponential distribution 双指数分布Double logarithmic 双对数Downward rank 降秩Dual-space plot 对偶空间图DUD 无导数方法Duncan's new multiple range method 新复极差法/Duncan新法Effect 实验效应Eigenvalue 特征值Eigenvector 特征向量Ellipse 椭圆Empirical distribution 经验分布Empirical probability 经验概率单位Enumeration data 计数资料Equal sun-class number 相等次级组含量Equally likely 等可能Equivariance 同变性Error 误差/错误Error of estimate 估计误差Error type I 第一类错误Error type II 第二类错误Estimand 被估量Estimated error mean squares 估计误差均方Estimated error sum of squares 估计误差平方和Euclidean distance 欧式距离Event 事件Event 事件Exceptional data point 异常数据点Expectation plane 期望平面Expectation surface 期望曲面Expected values 期望值Experiment 实验Experimental sampling 试验抽样Experimental unit 试验单位Explanatory variable 说明变量Exploratory data analysis 探索性数据分析Explore Summarize 探索-摘要Exponential curve 指数曲线Exponential growth 指数式增长EXSMOOTH 指数平滑方法Extended fit 扩充拟合Extra parameter 附加参数Extrapolation 外推法Extreme observation 末端观测值Extremes 极端值/极值F distribution F分布F test F检验Factor 因素/因子Factor analysis 因子分析Factor Analysis 因子分析Factor score 因子得分Factorial 阶乘Factorial design 析因试验设计False negative 假阴性False negative error 假阴性错误Family of distributions 分布族Family of estimators 估计量族Fanning 扇面Fatality rate 病死率Field investigation 现场调查Field survey 现场调查Finite population 有限总体Finite-sample 有限样本First derivative 一阶导数First principal component 第一主成分First quartile 第一四分位数Fisher information 费雪信息量Fitted value 拟合值Fitting a curve 曲线拟合Fixed base 定基Fluctuation 随机起伏Forecast 预测Four fold table 四格表Fourth 四分点Fraction blow 左侧比率Fractional error 相对误差Frequency 频率Frequency polygon 频数多边图Frontier point 界限点Function relationship 泛函关系Gamma distribution 伽玛分布Gauss increment 高斯增量Gaussian distribution 高斯分布/正态分布Gauss-Newton increment 高斯-牛顿增量General census 全面普查GENLOG (Generalized liner models) 广义线性模型Geometric mean 几何平均数Gini's mean difference 基尼均差GLM (General liner models) 通用线性模型Goodness of fit 拟和优度/配合度Gradient of determinant 行列式的梯度Graeco-Latin square 希腊拉丁方Grand mean 总均值Gross errors 重大错误Gross-error sensitivity 大错敏感度Group averages 分组平均Grouped data 分组资料Guessed mean 假定平均数Half-life 半衰期Hampel M-estimators 汉佩尔M估计量Happenstance 偶然事件Harmonic mean 调和均数Hazard function 风险均数Hazard rate 风险率Heading 标目Heavy-tailed distribution 重尾分布Hessian array 海森立体阵Heterogeneity 不同质Heterogeneity of variance 方差不齐Hierarchical classification 组内分组Hierarchical clustering method 系统聚类法High-leverage point 高杠杆率点HILOGLINEAR 多维列联表的层次对数线性模型Hinge 折叶点Histogram 直方图Historical cohort study 历史性队列研究Holes 空洞HOMALS 多重响应分析Homogeneity of variance 方差齐性Homogeneity test 齐性检验Huber M-estimators 休伯M估计量Hyperbola 双曲线Hypothesis testing 假设检验Hypothetical universe 假设总体Impossible event 不可能事件Independence 独立性Independent variable 自变量Index 指标/指数Indirect standardization 间接标准化法Individual 个体Inference band 推断带Infinite population 无限总体Infinitely great 无穷大Infinitely small 无穷小Influence curve 影响曲线Information capacity 信息容量Initial condition 初始条件Initial estimate 初始估计值Initial level 最初水平Interaction 交互作用Interaction terms 交互作用项Intercept 截距Interpolation 内插法Interquartile range 四分位距Interval estimation 区间估计Intervals of equal probability 等概率区间Intrinsic curvature 固有曲率Invariance 不变性Inverse matrix 逆矩阵Inverse probability 逆概率Inverse sine transformation 反正弦变换Iteration 迭代Jacobian determinant 雅可比行列式Joint distribution function 分布函数Joint probability 联合概率Joint probability distribution 联合概率分布K means method 逐步聚类法Kaplan-Meier 评估事件的时间长度Kaplan-Merier chart Kaplan-Merier图Kendall's rank correlation Kendall等级相关Kinetic 动力学Kolmogorov-Smirnove test 柯尔莫哥洛夫-斯米尔诺夫检验Kruskal and Wallis test Kruskal及Wallis检验/多样本的秩和检验/H检验Kurtosis 峰度Lack of fit 失拟Ladder of powers 幂阶梯Lag 滞后Large sample 大样本Large sample test 大样本检验Latin square 拉丁方Latin square design 拉丁方设计Leakage 泄漏Least favorable configuration 最不利构形Least favorable distribution 最不利分布Least significant difference 最小显着差法Least square method 最小二乘法Least-absolute-residuals estimates 最小绝对残差估计Least-absolute-residuals fit 最小绝对残差拟合Least-absolute-residuals line 最小绝对残差线Legend 图例L-estimator L估计量L-estimator of location 位置L估计量L-estimator of scale 尺度L估计量Level 水平Life expectance 预期期望寿命Life table 寿命表Life table method 生命表法Light-tailed distribution 轻尾分布Likelihood function 似然函数Likelihood ratio 似然比line graph 线图Linear correlation 直线相关Linear equation 线性方程Linear programming 线性规划Linear regression 直线回归Linear Regression 线性回归Linear trend 线性趋势Loading 载荷Location and scale equivariance 位置尺度同变性Location equivariance 位置同变性Location invariance 位置不变性Location scale family 位置尺度族Log rank test 时序检验Logarithmic curve 对数曲线Logarithmic normal distribution 对数正态分布Logarithmic scale 对数尺度Logarithmic transformation 对数变换Logic check 逻辑检查Logistic distribution 逻辑斯特分布Logit transformation Logit转换LOGLINEAR 多维列联表通用模型Lognormal distribution 对数正态分布Lost function 损失函数Low correlation 低度相关Lower limit 下限Lowest-attained variance 最小可达方差LSD 最小显着差法的简称Lurking variable 潜在变量Main effect 主效应Major heading 主辞标目Marginal density function 边缘密度函数Marginal probability 边缘概率Marginal probability distribution 边缘概率分布Matched data 配对资料Matched distribution 匹配过分布Matching of distribution 分布的匹配Matching of transformation 变换的匹配Mathematical expectation 数学期望Mathematical model 数学模型Maximum L-estimator 极大极小L 估计量Maximum likelihood method 最大似然法Mean 均数Mean squares between groups 组间均方Mean squares within group 组内均方Means (Compare means) 均值-均值比较Median 中位数Median effective dose 半数效量Median lethal dose 半数致死量Median polish 中位数平滑Median test 中位数检验Minimal sufficient statistic 最小充分统计量Minimum distance estimation 最小距离估计Minimum effective dose 最小有效量Minimum lethal dose 最小致死量Minimum variance estimator 最小方差估计量MINITAB 统计软件包Minor heading 宾词标目Missing data 缺失值Model specification 模型的确定Modeling Statistics 模型统计Models for outliers 离群值模型Modifying the model 模型的修正Modulus of continuity 连续性模Morbidity 发病率Most favorable configuration 最有利构形Multidimensional Scaling (ASCAL) 多维尺度/多维标度Multinomial Logistic Regression 多项逻辑斯蒂回归Multiple comparison 多重比较Multiple correlation 复相关Multiple covariance 多元协方差Multiple linear regression 多元线性回归Multiple response 多重选项Multiple solutions 多解Multiplication theorem 乘法定理Multiresponse 多元响应Multi-stage sampling 多阶段抽样Multivariate T distribution 多元T分布Mutual exclusive 互不相容Mutual independence 互相独立Natural boundary 自然边界Natural dead 自然死亡Natural zero 自然零Negative correlation 负相关Negative linear correlation 负线性相关Negatively skewed 负偏Newman-Keuls method q检验NK method q检验No statistical significance 无统计意义Nominal variable 名义变量Nonconstancy of variability 变异的非定常性Nonlinear regression 非线性相关Nonparametric statistics 非参数统计Nonparametric test 非参数检验Nonparametric tests 非参数检验Normal deviate 正态离差Normal distribution 正态分布Normal equation 正规方程组Normal ranges 正常范围Normal value 正常值Nuisance parameter 多余参数/讨厌参数Null hypothesis 无效假设Numerical variable 数值变量Objective function 目标函数Observation unit 观察单位Observed value 观察值One sided test 单侧检验One-way analysis of variance 单因素方差分析Oneway ANOVA 单因素方差分析Open sequential trial 开放型序贯设计Optrim 优切尾Optrim efficiency 优切尾效率Order statistics 顺序统计量Ordered categories 有序分类Ordinal logistic regression 序数逻辑斯蒂回归Ordinal variable 有序变量Orthogonal basis 正交基Orthogonal design 正交试验设计Orthogonality conditions 正交条件ORTHOPLAN 正交设计Outlier cutoffs 离群值截断点Outliers 极端值OVERALS 多组变量的非线性正规相关Overshoot 迭代过度Paired design 配对设计Paired sample 配对样本Pairwise slopes 成对斜率Parabola 抛物线Parallel tests 平行试验Parameter 参数Parametric statistics 参数统计Parametric test 参数检验Partial correlation 偏相关Partial regression 偏回归Partial sorting 偏排序Partials residuals 偏残差Pattern 模式Pearson curves 皮尔逊曲线Peeling 退层Percent bar graph 百分条形图Percentage 百分比Percentile 百分位数Percentile curves 百分位曲线Periodicity 周期性Permutation 排列P-estimator P估计量Pie graph 饼图Pitman estimator 皮特曼估计量Pivot 枢轴量Planar 平坦Planar assumption 平面的假设PLANCARDS 生成试验的计划卡Point estimation 点估计Poisson distribution 泊松分布Polishing 平滑Polled standard deviation 合并标准差Polled variance 合并方差Polygon 多边图Polynomial 多项式Polynomial curve 多项式曲线Population 总体Population attributable risk 人群归因危险度Positive correlation 正相关Positively skewed 正偏Posterior distribution 后验分布Power of a test 检验效能Precision 精密度Predicted value 预测值Preliminary analysis 预备性分析Principal component analysis 主成分分析Prior distribution 先验分布Prior probability 先验概率Probabilistic model 概率模型probability 概率Probability density 概率密度Product moment 乘积矩/协方差Profile trace 截面迹图Proportion 比/构成比Proportion allocation in stratified random sampling 按比例分层随机抽样Proportionate 成比例Proportionate sub-class numbers 成比例次级组含量Prospective study 前瞻性调查Proximities 亲近性Pseudo F test 近似F检验Pseudo model 近似模型Pseudosigma 伪标准差Purposive sampling 有目的抽样QR decomposition QR分解Quadratic approximation 二次近似Qualitative classification 属性分类Qualitative method 定性方法Quantile-quantile plot 分位数-分位数图/Q-Q图Quantitative analysis 定量分析Quartile 四分位数Quick Cluster 快速聚类Radix sort 基数排序Random allocation 随机化分组Random blocks design 随机区组设计Random event 随机事件Randomization 随机化Range 极差/全距Rank correlation 等级相关Rank sum test 秩和检验Rank test 秩检验Ranked data 等级资料Rate 比率Ratio 比例Raw data 原始资料Raw residual 原始残差Rayleigh's test 雷氏检验Rayleigh's Z 雷氏Z值Reciprocal 倒数Reciprocal transformation 倒数变换Recording 记录Redescending estimators 回降估计量Reducing dimensions 降维Re-expression 重新表达Reference set 标准组Region of acceptance 接受域Regression coefficient 回归系数Regression sum of square 回归平方和Rejection point 拒绝点Relative dispersion 相对离散度Relative number 相对数Reliability 可靠性Reparametrization 重新设置参数Replication 重复Report Summaries 报告摘要Residual sum of square 剩余平方和Resistance 耐抗性Resistant line 耐抗线Resistant technique 耐抗技术R-estimator of location 位置R估计量R-estimator of scale 尺度R估计量Retrospective study 回顾性调查Ridge trace 岭迹Ridit analysis Ridit分析Rotation 旋转Rounding 舍入Row 行Row effects 行效应Row factor 行因素RXC table RXC表Sample 样本Sample regression coefficient 样本回归系数Sample size 样本量Sample standard deviation 样本标准差Sampling error 抽样误差SAS(Statistical analysis system ) SAS统计软件包Scale 尺度/量表Scatter diagram 散点图Schematic plot 示意图/简图Score test 计分检验Screening 筛检SEASON 季节分析Second derivative 二阶导数Second principal component 第二主成分SEM (Structural equation modeling) 结构化方程模型Semi-logarithmic graph 半对数图Semi-logarithmic paper 半对数格纸Sensitivity curve 敏感度曲线Sequential analysis 贯序分析Sequential data set 顺序数据集Sequential design 贯序设计Sequential method 贯序法Sequential test 贯序检验法Serial tests 系列试验Short-cut method 简捷法Sigmoid curve S形曲线Sign function 正负号函数Sign test 符号检验Signed rank 符号秩Significance test 显着性检验Significant figure 有效数字Simple cluster sampling 简单整群抽样Simple correlation 简单相关Simple random sampling 简单随机抽样Simple regression 简单回归simple table 简单表Sine estimator 正弦估计量Single-valued estimate 单值估计Singular matrix 奇异矩阵Skewed distribution 偏斜分布Skewness 偏度Slash distribution 斜线分布Slope 斜率Smirnov test 斯米尔诺夫检验Source of variation 变异来源Spearman rank correlation 斯皮尔曼等级相关Specific factor 特殊因子Specific factor variance 特殊因子方差Spectra 频谱Spherical distribution 球型正态分布Spread 展布SPSS(Statistical package for the social science) SPSS统计软件包Spurious correlation 假性相关Square root transformation 平方根变换Stabilizing variance 稳定方差Standard deviation 标准差Standard error 标准误Standard error of difference 差别的标准误Standard error of estimate 标准估计误差Standard error of rate 率的标准误Standard normal distribution 标准正态分布Standardization 标准化Starting value 起始值Statistic 统计量Statistical control 统计控制Statistical graph 统计图Statistical inference 统计推断Statistical table 统计表Steepest descent 最速下降法Stem and leaf display 茎叶图Step factor 步长因子Stepwise regression 逐步回归Storage 存Strata 层(复数)Stratified sampling 分层抽样Stratified sampling 分层抽样Strength 强度Stringency 严密性Structural relationship 结构关系Studentized residual 学生化残差/t化残差Sub-class numbers 次级组含量Subdividing 分割Sufficient statistic 充分统计量Sum of products 积和Sum of squares 离差平方和Sum of squares about regression 回归平方和Sum of squares between groups 组间平方和Sum of squares of partial regression 偏回归平方和Sure event 必然事件Survey 调查Survival 生存分析Survival rate 生存率Suspended root gram 悬吊根图Symmetry 对称Systematic error 系统误差Systematic sampling 系统抽样Tags 标签Tail area 尾部面积Tail length 尾长Tail weight 尾重Tangent line 切线Target distribution 目标分布Taylor series 泰勒级数Tendency of dispersion 离散趋势Testing of hypotheses 假设检验Theoretical frequency 理论频数Time series 时间序列Tolerance interval 容忍区间Tolerance lower limit 容忍下限Tolerance upper limit 容忍上限Torsion 扰率Total sum of square 总平方和Total variation 总变异Transformation 转换Treatment 处理Trend 趋势Trend of percentage 百分比趋势Trial 试验Trial and error method 试错法Tuning constant 细调常数Two sided test 双向检验Two-stage least squares 二阶最小平方Two-stage sampling 二阶段抽样Two-tailed test 双侧检验Two-way analysis of variance 双因素方差分析Two-way table 双向表Type I error 一类错误/α错误Type II error 二类错误/β错误UMVU 方差一致最小无偏估计简称Unbiased estimate 无偏估计Unconstrained nonlinear regression 无约束非线性回归Unequal subclass number 不等次级组含量Ungrouped data 不分组资料Uniform coordinate 均匀坐标Uniform distribution 均匀分布Uniformly minimum variance unbiased estimate 方差一致最小无偏估计Unit 单元Unordered categories 无序分类Upper limit 上限Upward rank 升秩Vague concept 模糊概念Validity 有效性VARCOMP (Variance component estimation) 方差元素估计Variability 变异性Variable 变量Variance 方差Variation 变异Varimax orthogonal rotation 方差最大正交旋转Volume of distribution 容积W test W检验Weibull distribution 威布尔分布Weight 权数Weighted Chi-square test 加权卡方检验/Cochran检验Weighted linear regression method 加权直线回归Weighted mean 加权平均数Weighted mean square 加权平均方差Weighted sum of square 加权平方和Weighting coefficient 权重系数Weighting method 加权法W-estimation W估计量W-estimation of location 位置W估计量Width 宽度Wilcoxon paired test 威斯康星配对法/配对符号秩和检验Wild point 野点/狂点Wild value 野值/狂值Winsorized mean 缩尾均值Withdraw 失访Youden's index 尤登指数Z test Z检验Zero correlation 零相关Z-transformation Z变换。
反应扩散模型在图灵斑图中的应用及数值模拟

反应扩散模型在图灵斑图中的应用及数值模拟张荣培;王震;王语;韩子健【摘要】反应扩散方程模型常被用于描述生物学中斑图的形成.从反应扩散模型出发,理论推导得到Gierer-Meinhardt模型的斑图形成机理,解释了非线性常微分方程系统的稳定常数平衡态在加入扩散项后会发生失稳并产生图灵斑图的过程.通过计算该模型,得到图灵斑图产生的参数条件.数值方法中采用一类有效的高精度数值格式,即在空间离散条件下采用Chebyshev谱配置方法,在时间离散条件下采用紧致隐积分因子方法.该方法结合了谱方法和紧致隐积分因子方法的优点,具有精度高、稳定性好、存储量小等优点.数值模拟表明,在其他条件一定的情况下,系统控制参数κ 取不同值对于斑图的产生具有重要的影响,数值结果验证了理论结果.%Turing proposed a model for the development of patterns found in nature in 1952. Turing instability is known as diffusion-driven instability, which states that a stable spatially homogeneous equilibrium may lose its stability dueto the unequal spatial diffusion coefficients. The Gierer–Mainhardt modelis an activator and inhibitor system to model the generating mechanism of biological patterns. The reaction-diffusion system is often used to describe the pattern formation model arising in biology. In this paper, the mechanism of the pattern formation of the Gierer-Meinhardt model is deduced from the reactive diffusion model. It is explained that the steady equilibrium state of the nonlinear ordinary differential equation system will be unstable after adding of the diffusion term and produce the Turing pattern. The parameters of the Turing pattern are obtained by calculating the model. There are a variety of numerical methods including finitedifference method and finite element method. Compared with the finite difference method and finite element method, which have low order precision, the spectral method can achieve the convergence of the exponential order with only a small number of nodes and the discretization of the suitable orthogonal polynomials. In the present work, an efficient high-precision numerical scheme is used in the numerical simulation of the reaction-diffusion equations. In spatial discretization, we construct Chebyshev differentiation matrices based on the Chebyshev points and use these matrices to differentiate the second derivative in the reaction-diffusion equation. After the spatial discretization, we obtain the nonlinear ordinary differential equations. Since the spectral differential matrix obtained by the spectral collocation method is full and cannot use the fast solution of algebraic linear equations, we choose the compact implicit integration factor method to solve the nonlinear ordinary differential equations. By introducing a compact representation for the spectral differential matrix, the compact implicit integration factor method uses matrix exponential operations sequentially in every spatial direction. As a result, exponential matrices which are calculated and stored have small sizes, as those in the one-dimensional problem. This method decouples the exact evaluation of the linear part from the implicit treatment of the nonlinear reaction terms. We only solve a local nonlinear system at each spatial grid point. This method combines with the advantages of the spectral method and the compact implicit integration factor method, i.e., high precision, good stability, and small storage and soon. Numerical simulations show that it can have a great influence on the generation of patterns that the system control parameters take different values under otherwise identical conditions. The numerical results verify the theoretical results.【期刊名称】《物理学报》【年(卷),期】2018(067)005【总页数】10页(P50-59)【关键词】反应扩散方程;Gierer-Meinhardt模型;图灵斑图;Chebyshev谱方法【作者】张荣培;王震;王语;韩子健【作者单位】沈阳师范大学数学与系统科学学院,沈阳 110034;山东科技大学数学与系统科学学院,青岛 266590;沈阳师范大学数学与系统科学学院,沈阳 110034;沈阳师范大学数学与系统科学学院,沈阳 110034【正文语种】中文1 引言斑图是在空间或时间上具有某种规律性的非均匀宏观结构,普遍存在于自然界.1952年,著名的英国数学家图灵把他的目光转向生物学领域,用一个反应扩散系统成功地说明了某些生物体表面图纹产生的原理[1].图灵从数学角度表明,在反应扩散系统中,稳定状态会在某些条件下失稳,并自发产生空间定态图纹,此斑图通常称为图灵斑图. 经过多年的研究,各界学者利用反应扩散系统预测得到了更多的图灵斑图,在理论和实验方面取得了许多重要成果.他们证实了化学系统中图灵斑图的形成[2],讨论自催化反应中的动力学行为,探讨此类耦合反应扩散体系中影响图灵斑图的因素[3].给出Gray-Scott模型、Brusselator模型等系统扩散引起不稳定的数学机理[4],并描述了Gierer-Meinhardt,Lengyel-Epstein等模型的某些动力学行为(性质)[5,6].最近几年,图灵斑图在实验方面取得一系列最新的进展,Copie等[7]运用实验在一个双稳态被动非线性共振器中探讨了图灵调制和法拉第参数不稳定性的相互作用;Tompkins等[8]利用微流体化学室证实图灵理论体系,并观测到第七种时空模式;Lacitignola[9]研究了图灵不稳定现象的发生条件,论述了具体形态的电化学反应扩散模型在一个球面上的图案形成的特性;Gaskins等[10]在二氧化氯碘丙二酸反应实验中,通过添加卤化钠盐溶液得到新的图灵斑图.在这些系统中存在两种化学反应物质,它们不仅能相互作用,而且还能进行独自扩散.事实上,图灵斑图的产生对应的是一个非线性反应动力学过程与一种特殊扩散过程的耦合.这个特殊的扩散过程由于两种因子的扩散速度不同会发生失稳,这就是图灵斑图产生的机理.在数学上,图灵斑图可以用无量纲化的反应扩散方程组描述[11],即式中u和v是系统变量,分别代表参与化学反应的两种物质的浓度;c和d是扩散系数,t是时间变量,f(u,v)和g(u,v)表示反应项.设Ω为RN中带有光滑边界的有界区域,Ω=[0,a]×[0,b],边界为∂Ω,边界条件为齐次Neumann边界条件,即其中n表示边界上单位外法向.由于(1)式为耦合的非线性反应扩散方程,很难得到其精确解.近年来,许多学者用有限差分方法、有限元方法、谱方法等[12−14]多种数值方法求解(1)式,这些方法各有特点.相比于有限元方法和有限差分方法的低阶精度,谱方法[14]仅用少量的节点,采用Legendre,Chebyshev等适合的正交多项式离散即可达到指数阶收敛的谱精度.图灵斑图在空间上的结构具有一定的规律,且解比较光滑,因此采用谱方法离散是可行的.常用的谱配置方法主要有Fourier配置法[15],Chebyshev配置法[16],Hermite配置法等[17].由于本文考虑的(1)式边界条件为齐次Neumann边界条件,因此采用Chebyshev配置方法求解(1)式.对(1)式进行空间离散后,得到的是刚性的非线性常微分方程组(ODEs).显式时间离散方法虽可以用迭代的方法求解,但其对时间步长有严格的约束;隐式方法虽然可以允许大的时间步长,但是对于阶数非常大的非线性方程组的求解问题十分复杂,这对于全隐式方法来说是一个巨大的挑战.由于谱配置法所得到的谱微分矩阵是满的,显然利用追赶法等代数线性方程组的快速解法是不合适的,因此交替方向隐式方法在这里并不适用.本文采用紧致隐积分因子(compact implicit integration factor,cIIF)方法求解ODEs.2006年Nie等[18]以隐积分因子(IIF)方法为基础发展了cIIF方法.传统的隐积分因子方法在求解高维问题时,离散矩阵的指数运算的存储量和运算量非常大,导致运算速度缓慢.紧致隐积分因子方法[19]通过引入离散矩阵的紧致表达式并在各个方向进行矩阵的指数运算,使得中央处理器(CPU)的存储大大降低,计算速度也得到了显著提高.本文内容安排如下:第2节对反应扩散方程组进行线性分析,通过特征值解释图灵斑图的数学机理,然后以Gierer-Meinhardt模型为例分析系统处于稳定状态和不稳定状态时各参数需要满足的条件,进而探索斑图形成需要满足的条件;第3节研究数值方法,在空间离散条件下采用Chebyshev谱方法,时间离散条件下采用紧致隐积分因子方法,用MATLAB进行编程求解;第4节给出大量数值实验并对理论分析结果进行验证.2 图灵斑图的形成2.1 斑图形成的数学机理首先考虑(1)式没有扩散项,假设存在惟一的均匀定态解(u0,v0),即常数u0,v0满足令U=u−u0,V=v−v0,并在(u0,v0)处线性化后得到如下系统:式中c11=fu(u0,v0), c12=fv(u0,v0),c21=gu(u0,v0),c22=gv(u0,v0).均匀定态解(u0,v0)在没有扩散时是稳定的,这等价于相应的特征值问题的矩阵的特征值实部是负数.考虑加入扩散项后的反应扩散方程组((1)式).如果此时产生斑图,即(u0,v0)是不稳定的,要求特征值有正实部.所谓不稳定,体现为两种反应物的扩散速度不同,从而引起失稳.对(1)式作线性化处理,研究特征值正实部引起的线性不稳定性,进而推导出原方程的不稳定性.对均匀定态解(u0,v0)作一个微扰,可得线性微扰方程为求解如下方程可得相应的特征值:式中λ为特征值.只要(5)式中的特征值有正实部,则(u0,v0)对于(1)式是不稳定的.考虑到齐次Neumann边界条件,得到(5)式所对应的特征值为具体推导过程见附录A.2.2 Gierer-Meinhardt模型生物的发育过程是复杂的,其中重要的是形态形成阶段,与之对应的是生物体内器官的形成.由于该阶段的重要性,渐渐形成一个新的领域——形态学,主要研究导致细胞分化和定位因素的浓度对组织器官的影响.Gierer-Meinhardt模型是由Gierer和Meinhardt在研究激活物和抑制剂两种不同物质的产生和扩散时建立的[20],之后Gierer和Meinhardt利用数值方法导出一维和二维空间区域中上述系统产生多样斑图的条件.Gierer-Meinhardt模型被广泛应用于形态形成过程中一些基本现象的研究,最近的一些工作可以参见文献[21—23].以Gierer-Meinhardt模型为例,结合上述理论分析,计算产生斑图时需要满足的条件.取(1)式中其中系数κ,η,ε为系统的控制参数,固定η=0.1,ε=0.04.由此得到线性化系统(3)式中的系数为易得该系统的特征值为λ1= −1.2984,λ2=−7.7016,此时系统是稳定的.加入扩散项后,原方程组对应的特征问题为相应的特征方程为为使(8)式含有正实部的特征值,需要考虑两种情况.第一种情况是两个特征值异号,则应满足图1 特征值的实部Re(λ)随参数的变化(a)κ=0.0128;(b)κ=0.0152;(c)κ=0.008Fig.1.Real part Re(λ)of eigenvalues varying with parameters:(a)κ=0.0128;(b)κ=0.0152;(c)κ=0.008.经过化简可以得到此时应满足得0.0093248.第二种情况是两个特征值都是正的,应满足此时κ无解.由于反应扩散方程组联系于解析半群,所以线性化后的正实部特征值引起的不稳定性可以推导出原方程组的不稳定性.故当κ>κ0=0.0093248时,系统处于不稳定状态,因而系统能够产生斑图.特征值的实部Re(λ)在参数κ取不同值时的变化如图1所示.从图1可以看出,当κ = 0.0128>κ0和κ=0.0152>κ0时,特征值的实部会出现正值,此时系统不稳定;当κ=0.008<κ0时,特征值的实部始终为负,系统最后会达到稳定状态.第3节将用数值算例验证该结论.3 数值方法3.1 Chebyshev谱配置法将求解区域[−1,1]2离散为Gauss-Lobatto网格,即其中Nx和Ny是正整数.对于一般的求解区域Ω=[a,b]×[c,d],可以采用公式将区域转化为[−1,1]2.在网格Th中将u(x,y)数值解定义为矩阵形式,U∈R(Nx−1)×(Ny−1),式中ui,j表示u在网格点(xi,xj)的数值解.引入Chebyshev一阶微分矩阵和二阶微分矩阵(具体推导过程见附录B).则u(x,y)关于x的二阶偏导数在配置点的值,可以用矩阵乘积的形式近似为矩阵Ax是在Chebyshev二阶微分矩阵基础上考虑Neumann边界条件得到的,其中同样地,对于y的二阶偏导数,有UAy,其中矩阵Ay定义同Ax.借助谱微分矩阵,可将方程中的Laplace算子离散成矩阵乘积的形式,即将Chebyshev谱配置方法应用于反应扩散方程,得到其半离散形式为式中3.2 紧致隐积分因子法将对空间离散后得到的非线性常微分方程组((12)式)采用紧致隐积分因子方法进行时间离散.定义时间步长为τ=Δt,第n层时间步为tn=nτ,n=0,1,2,···. 在(12)式两端同时左乘指数矩阵e−Axt,右乘指数矩阵e−Ayt.为描述方便,取(10)式c=1,d=1,可将(12)式中第一个等式写为将时间离散为0=t0<t1<···,将(13)式在一个时间步长内关于时间积分,并用梯形公式近似可得二阶紧致隐积分因子格式为进一步化简得在非线性方程组(13)式中,右端第一项可以通过矩阵乘积得到,右端第二项采用Picard迭代方法求解:同理处理(12)式中第二个等式可得该方法中矩阵eAxΔt和eAyΔt的阶数分别为Nx×Nx和Ny×Ny.在空间网格剖分量很大时,该方法可以降低存储量和运算量,使计算速度更快.4 数值算例对于前述Gierer-Meinhardt模型,取Ω =(−1,1)× (−1,1),η=0.1,c=0.04,κ 是不固定的参数.设其中图2 取κ=0.0128时Gierer-Meinhardt模型形成的斑图(a)t=20;(b)t=80;(c)t=170;(d)t=270;(e)t=320;(f)t=340;(g)t=500;(h)t=600;(i)t= 900Fig.2.Turing patterns in Gierer-Meinhardt model whenκ=0.0128:(a)t=20;(b)t=80;(c)t=170;(d)t=270;(e)t=320;(f)t=340;(g)t=500;(h)t =600;(i)t=900.4.1 数值算例I取κ=0.0128,N=100,h=2/100=0.02,τ=0.1h,t取图2所示各值时,得到对应的图像.由图2可知,随着时间的推移,初始扰动不断增强扩大,最终形成清晰的斑图.4.2 数值算例II取κ=0.0152,t取图3所示各值,其他参数与算例I相同,可得到t取不同值时对应的图像.由图3可知,随着时间的推移,初始扰动不断增强扩大,最终形成清晰的斑图. 图3 取κ=0.0152时Gierer-Meinhardt模型形成的斑图(a)t=30;(b)t=80;(c)t=90;(d)t=140;(e)t=160;(f)t=290;(g)t=520;(h)t=620;(i)t=9 90Fig.3.Turing patterns in Gierer-Meinhardt model whenκ=0.0152:(a)t=30;(b)t=80;(c)t=90;(d)t=140;(e)t=160;(f)t=290;(g)t=520;(h)t= 620;(i)t=990.4.3 数值算例III取κ=0.008,其他取值与算例II相同,t取不同值时对应的图像如图4所示.由图4可知,随着时间的推移,系统达到稳定状态,反应扩散模型不能形成斑图.由数值模拟结果来看,其他条件一定的情况下,κ取不同值对于产生斑图有重要的影响.数值模拟结果与理论结果一致.此外,我们也对周期性边界条件的Gierer-Meinhardt模型采用Fourier谱方法进行数值求解,结果显示周期边界条件对斑图的形状几乎没有影响.5 结论介绍了图灵斑图形成的数学机理,并结合Gierer-Meinhardt模型,分析系统不稳定状态的各系数需要满足的条件,即产生斑图的条件.运用紧致隐积分因子方法大大减少了存储和CPU运算时间,该方法对于大时间数值模拟是一个高效、高精度的数值方法.数值算例模拟了斑图形成的过程,验证了理论分析结果.这些结论还可应用于求解带有分数阶的反应扩散方程组.图4 取κ=0.008时Gierer-Meinhardt模型形成的斑图(a)t=30;(b)t=80;(c)t=90;(d)t=140;(e)t=160;(f)t=220;(g)t=290;(h)t=270;(i)t=9 90Fig.4.Turing patterns in Gierer-Meinhardt model whenκ=0.008:(a)t=30;(b)t=80;(c)t=90;(d)t=140;(e)t=160;(f)t=220;(g)t=290;(h)t=2 70;(i)t=990.附录A 图灵斑图的形成机理首先在区域Ω⊂RN(N=1,2)内考虑带有齐次Neumann边界条件的Laplace算子的特征值问题.一维情况下,特征值问题为式中a∈R+.特征值问题可表示为µ2−λ=0,解得只有λ< 0时可解得特征值λk= −(kπ/a)2,且特征值所对应的特征函数为在二维情况下,特征值问题为式中a,b∈R+,应采用分离变量法求解特征值. 设u=X(x)Y(y),代入方程得设解得故特征值为λk,l=且特征值所对应的特征函数为考虑方程组的特征值问题,令代入原方程组可得设则方程组可化为当方程组(A3)有非零解,满足此时方程组所对应的特征值为附录B 谱微分矩阵定义在[−1,1]上的标准k阶Chebyshev多项式Tk(x)为 Tk(x)=cos(k arccosx),k=0,1,2,···. 令x=cosz,则有Tk=coskz,满足如下递推关系:Tk(x)在[−1,1]上的N+1个Gauss-Lobatto点值为零:设N阶多项式uN(x)∈PN在上述配置点xj满足uN(xj)=u(xj),则有式中hj(x)为N阶Lagrange基函数.用配置法求解未知量在网格点处的值,需要表示配置点处的导数值.对(B3)式求p阶导数,得式中系数从而可得一阶谱微分矩阵其中这里二阶谱微分矩阵可以由一阶谱微分矩阵平方得到,即参考文献[1]Turing A M 1952 Philos.Trans.R.Soc.Lond.B 2 37[2]Li X Z,Bai Z G,Li Y,Zhao K,He Y F 2013 Acta Phys.Sin.62 220503(inChinese)[李新政,白占国,李燕,赵昆,贺亚峰2013物理学报62 220503][3]Zhang L,Liu S Y 2007 Appl.Math.Mec.28 1102(in Chinese)[张丽,刘三阳2007应用数学和力学28 1102][4]Li B,Wang M X 2008 Appl.Math.Mec.29 749(in Chinese)[李波,王明新2008应用数学和力学29 749][5]Hu W Y,Shao Y Z 2014 Acta Phys.Sin.63 238202(in Chinese)[胡文勇,邵元智2014物理学报 63 238202][6]Peng R Wang M 2007 Sci.China A 50 377[7]Copie F,Conforti M,Kudlinski A,Mussot A,Trillo S 2016 Phys.Rev.Lett.116 143901[8]Tompkins N,Li N,Girabawe C,Heymann M,Ermentrout G B,Epstein IR,Fraden S 2014 A 111 4397[9]Lacitignola D,Bozzini B,Frittelli M,Sgura I 2017 Commun.Nonlinear Sci.Numer.Simul.48 484[10]Gaskins D K,Pruc E E,Epstein I R,Dolnik M 2016 Phys.Rev.Lett.117 056001[11]Zhang R P,Yu X J,Zhu J,Loula A 2014 Appl.Math.Model.38 1612[12]Zhang R P,Zhu J,Loula A,Yu X J 2016 put.Appl.Math.302 312[13]Bai Z G,Dong L F,Li Y H,Fan W L 2011 Acta Phys.Sin.60 118201(in Chinese)[白占国,董丽芳,李永辉,范伟丽2011物理学报60 118201][14]Zhang R,Zhu J,Yu X,Li M,Loula A F D 2017 put.310 194[15]Lv Z Q,Zhang L M,Wang Y S 2014 Chin.Phys.B 23 120203[16]Wang H 2010 mun.181 325[17]Hoz F D L,Vadillo F 2013 put.Phys.14 1001[18]Nie Q,Zhang Y T,Zhao R 2006 put.Phys.214 521[19]Nie Q,Wan F Y M,Zhang Y T,Liu X F 2008 put.Phys.227 5238[20]Gierer A,Meinhardt H 1972 Kybernetik 12 30[21]Ward M J,Wei J 2003 J.Nonlinear Sci.13 209[22]Wei J,Winter M 2004 J.Math.Pures Appl.83 433[23]Li H X 2015 J.Northeast Normal University 3 26(in Chinese)[李海侠2015东北师大学报3 26]。
偏微分方程求解方法及其比较

偏微分方程求解方法及其比较中图分类号:o24 文献标识码:a 文章编号:1007-0745(2008)10-00摘要:近些年来,无限维动力系统得到了很大的发展.随着对它研究的深入和计算能力的迅速提高,使得与之相关的数值研究越来越被人们关注.谱方法作为一种数值求解偏微分方程的方法,它具有无穷阶收敛性.因此,谱方法也就引起人们更多的关注.关键词:谱方法;偏微分;收敛;逼近;1偏微分方程及其谱方法的介绍偏微分方程主要借助于未知函数及其导数来刻画客观世界的物理量的一般变化规律。
理论上,对偏微分方程解法的研究已经有很长的历史了。
最初的研究工作主要集中在物理,力学,几何学等方面的具体问题,其经典代表是波动方程,热传导方程和位势方程(调和方程)。
通过对这些问题的研究,形成了至今仍然使用的有效方法,例如,分离变量法,fourier变换法等。
早期的偏微分方程研究主要集中在理论上,而在实际操作中其研究方法和研究结果都难以得到广泛的应用。
求解的主要方法为:有限差分法,有限元法,谱方法。
谱方法起源于ritz-galerkin方法,它是以正交多项式(三角多项式,切比雪夫多项式,勒让得多项式等)作为基函数的galerkin 方法、tau方法或配置法,它们分别称为谱方法、tau方法或拟谱方法(配点法),通称为谱方法。
谱方法是以正交函数或固有函数为近似函数的计算方法。
从函数近似角度看.谱方法可分为fourier 方法.chebyshev或legendre方法。
前者适用于周期性问题,后两者适用于非周期性问题。
而这些方法的基础就是建立空间基函数。
下面介绍几种正交多项式各种节点的取值方法及权重。
而chebyshev多项式是令时jacobi多项式的特殊形式,另外legendre多项式是令时jacobi多项式的特殊形式。
2 几种典型的谱方法谱方法是以正交函数或固有函数为近似函数的计算方法。
谱近似可以分为函数近似和方程近似两种近似方式。
从函数近似角度看.谱方法可分为fourier方法.chebyshev或legendre方法。
数据挖掘专业词汇中英对照
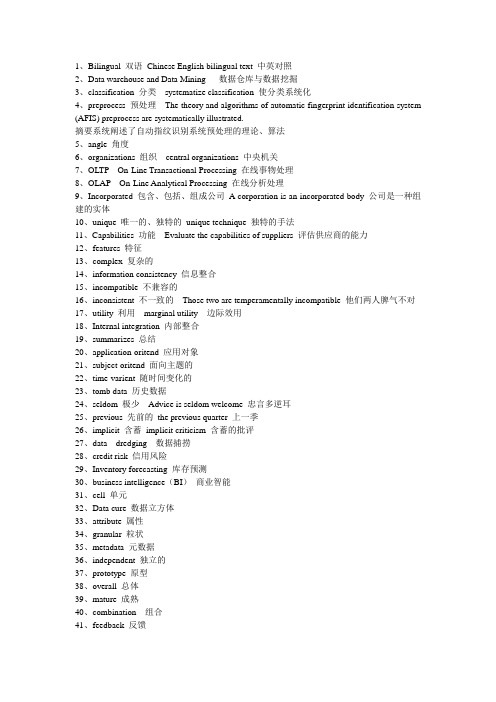
1、Bilingual 双语Chinese English bilingual text 中英对照2、Data warehouse and Data Mining 数据仓库与数据挖掘3、classification 分类systematize classification 使分类系统化4、preprocess 预处理The theory and algorithms of automatic fingerprint identification system (AFIS) preprocess are systematically illustrated.摘要系统阐述了自动指纹识别系统预处理的理论、算法5、angle 角度6、organizations 组织central organizations 中央机关7、OLTP On-Line Transactional Processing 在线事物处理8、OLAP On-Line Analytical Processing 在线分析处理9、Incorporated 包含、包括、组成公司A corporation is an incorporated body 公司是一种组建的实体10、unique 唯一的、独特的unique technique 独特的手法11、Capabilities 功能Evaluate the capabilities of suppliers 评估供应商的能力12、features 特征13、complex 复杂的14、information consistency 信息整合15、incompatible 不兼容的16、inconsistent 不一致的Those two are temperamentally incompatible 他们两人脾气不对17、utility 利用marginal utility 边际效用18、Internal integration 内部整合19、summarizes 总结20、application-oritend 应用对象21、subject-oritend 面向主题的22、time-varient 随时间变化的23、tomb data 历史数据24、seldom 极少Advice is seldom welcome 忠言多逆耳25、previous 先前的the previous quarter 上一季26、implicit 含蓄implicit criticism 含蓄的批评27、data dredging 数据捕捞28、credit risk 信用风险29、Inventory forecasting 库存预测30、business intelligence(BI)商业智能31、cell 单元32、Data cure 数据立方体33、attribute 属性34、granular 粒状35、metadata 元数据36、independent 独立的37、prototype 原型38、overall 总体39、mature 成熟40、combination 组合41、feedback 反馈42、approach 态度43、scope 范围44、specific 特定的45、data mart 数据集市46、dependent 从属的47、motivate 刺激、激励Motivate and withstand higher working pressure个性积极,愿意承受压力.敢于克服困难48、extensive 广泛49、transaction 交易50、suit 诉讼suit pending 案件正在审理中51、isolate 孤立We decided to isolate the patients.我们决定隔离病人52、consolidation 合并So our Party really does need consolidation 所以,我们党确实存在一个整顿的问题53、throughput 吞吐量Design of a Web Site Throughput Analysis SystemWeb网站流量分析系统设计收藏指正54、Knowledge Discovery(KDD)55、non-trivial(有价值的)--Extraction interesting (non-trivial(有价值的), implicit(固有的), previously unknown and potentially useful) patterns or knowledge from huge amounts of data.56、archeology 考古57、alternative 替代58、Statistics 统计、统计学population statistics 人口统计59、feature 特点A facial feature 面貌特征60、concise 简洁a remarkable concise report 一份非常简洁扼要的报告61、issue 发行issue price 发行价格62、heterogeneous (异类的)--Constructed by integrating multiple, heterogeneous (异类的)data sources63、multiple 多种Multiple attachments多实习64、consistent(一贯)、encode(编码)ensure consistency in naming conventions,encoding structures, attribute measures, etc.确保一致性在命名约定,编码结构,属性措施,等等。
NAG Toolbox for Matlab 函数手册
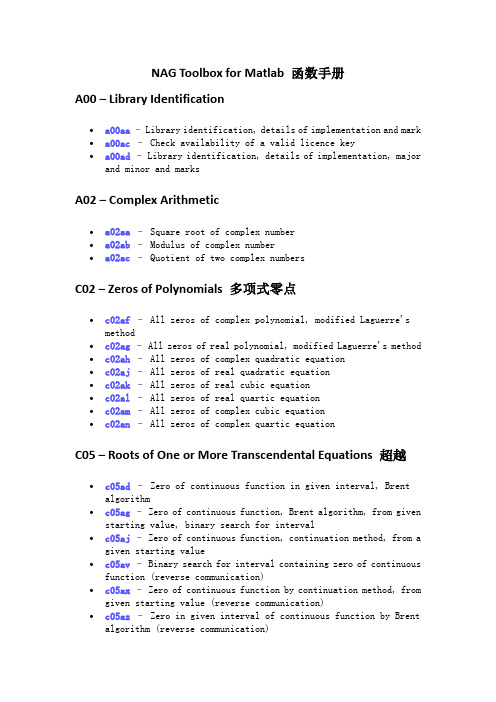
NAG Toolbox for Matlab 函数手册A00 – Library Identification∙a00aa–Library identification, details of implementation and mark ∙a00ac– Check availability of a valid licence key∙a00ad–Library identification, details of implementation, majorand minor and marksA02 – Complex Arithmetic∙a02aa– Square root of complex number∙a02ab– Modulus of complex number∙a02ac– Quotient of two complex numbersC02 – Zeros of Polynomials 多项式零点∙c02af– All zeros of complex polynomial, modified Laguerre's method∙c02ag– All zeros of real polynomial, modified Laguerre's method ∙c02ah– All zeros of complex quadratic equation∙c02aj– All zeros of real quadratic equation∙c02ak– All zeros of real cubic equation∙c02al– All zeros of real quartic equation∙c02am– All zeros of complex cubic equation∙c02an– All zeros of complex quartic equationC05 – Roots of One or More Transcendental Equations 超越∙c05ad– Zero of continuous function in given interval, Brentalgorithm∙c05ag– Zero of continuous function, Brent algorithm, from given starting value, binary search for interval∙c05aj– Zero of continuous function, continuation method, from a given starting value∙c05av– Binary search for interval containing zero of continuous function (reverse communication)∙c05ax– Zero of continuous function by continuation method, from given starting value (reverse communication)∙c05az– Zero in given interval of continuous function by Brent algorithm (reverse communication)∙c05ba– Real values of Lambert's W function, W(x)∙c05nb– Solution of system of nonlinear equations using function values only (easy-to-use)∙c05nc– Solution of system of nonlinear equations using function values only (comprehensive)∙c05nd– Solution of system of nonlinear equations using function values only (reverse communication)∙c05pb– Solution of system of nonlinear equations using first derivatives (easy-to-use)∙c05pc– Solution of system of nonlinear equations using first derivatives (comprehensive)∙c05pd– Solution of system of nonlinear equations using first derivatives (reverse communication)∙c05za– Check user's function for calculating first derivatives C06 – Summation of Series 数列∙c06ba– Acceleration of convergence of sequence, Shanks' transformation and epsilon algorithm∙c06db– Sum of a Chebyshev series∙c06ea– Single one-dimensional real discrete Fourier transform, no extra workspace∙c06eb– Single one-dimensional Hermitian discrete Fourier transform, no extra workspace∙c06ec–Single one-dimensional complex discrete Fourier transform, no extra workspace∙c06ek– Circular convolution or correlation of two real vectors, no extra workspace∙c06fa– Single one-dimensional real discrete Fourier transform, extra workspace for greater speed∙c06fb– Single one-dimensional Hermitian discrete Fourier transform, extra workspace for greater speed∙c06fc–Single one-dimensional complex discrete Fourier transform, extra workspace for greater speed∙c06ff– One-dimensional complex discrete Fourier transform of multi-dimensional data∙c06fj– Multi-dimensional complex discrete Fourier transform of multi-dimensional data∙c06fk– Circular convolution or correlation of two real vectors, extra workspace for greater speed∙c06fp–Multiple one-dimensional real discrete Fourier transforms ∙c06fq– Multiple one-dimensional Hermitian discrete Fourier transforms∙c06fr– Multiple one-dimensional complex discrete Fourier transforms∙c06fu– Two-dimensional complex discrete Fourier transform∙c06fx– Three-dimensional complex discrete Fourier transform ∙c06gb– Complex conjugate of Hermitian sequence∙c06gc– Complex conjugate of complex sequence∙c06gq– Complex conjugate of multiple Hermitian sequences∙c06gs– Convert Hermitian sequences to general complex sequences ∙c06ha– Discrete sine transform∙c06hb– Discrete cosine transform∙c06hc– Discrete quarter-wave sine transform∙c06hd– Discrete quarter-wave cosine transform∙c06la– Inverse Laplace transform, Crump's method∙c06lb– Inverse Laplace transform, modified Weeks' method∙c06lc– Evaluate inverse Laplace transform as computed by c06lb ∙c06pa–Single one-dimensional real and Hermitian complex discrete Fourier transform, using complex data format for Hermitiansequences∙c06pc–Single one-dimensional complex discrete Fourier transform, complex data format∙c06pf– One-dimensional complex discrete Fourier transform of multi-dimensional data (using complex data type)∙c06pj– Multi-dimensional complex discrete Fourier transform of multi-dimensional data (using complex data type)∙c06pk–Circular convolution or correlation of two complex vectors ∙c06pp– Multiple one-dimensional real and Hermitian complex discrete Fourier transforms, using complex data format forHermitian sequences∙c06pq– Multiple one-dimensional real and Hermitian complex discrete Fourier transforms, using complex data format forHermitian sequences∙c06pr– Multiple one-dimensional complex discrete Fourier transforms using complex data format∙c06ps– Multiple one-dimensional complex discrete Fourier transforms using complex data format and sequences stored ascolumns∙c06pu– Two-dimensional complex discrete Fourier transform, complex data format∙c06px– Three-dimensional complex discrete Fourier transform, complex data format∙c06ra– Discrete sine transform (easy-to-use)∙c06rb– Discrete cosine transform (easy-to-use)∙c06rc– Discrete quarter-wave sine transform (easy-to-use)∙c06rd– Discrete quarter-wave cosine transform (easy-to-use)C09 – Wavelet Transforms 小波变换∙c09aa– Wavelet filter query∙c09ca– one-dimensional discrete wavelet transform∙c09cb– one-dimensional inverse discrete wavelet transform ∙c09cc– one-dimensional multi-level discrete wavelet transform ∙c09cd– one-dimensional inverse multi-level discrete wavelet transformD01 – Quadrature 定积分∙d01ah– One-dimensional quadrature, adaptive, finite interval, strategy due to Patterson, suitable for well-behaved integrands ∙d01aj– One-dimensional quadrature, adaptive, finite interval, strategy due to Piessens and de Doncker, allowing for badly behaved integrands(被积函数)∙d01ak– One-dimensional quadrature, adaptive, finite interval, method suitable for oscillating functions∙d01al– One-dimensional quadrature, adaptive, finite interval, allowing for singularities at user-specified break-points ∙d01am– One-dimensional quadrature, adaptive, infinite or semi-infinite interval∙d01an– One-dimensional quadrature, adaptive, finite interval, weight function cos(( omega x)) or sin(( omega x)) ∙d01ap– One-dimensional quadrature, adaptive, finite interval, weight function with end-point singularities ofalgebraico-logarithmic type∙d01aq– One-dimensional quadrature, adaptive, finite interval, weight function 1/(x-c), Cauchy principal value (Hilberttransform)∙d01ar–One-dimensional quadrature, non-adaptive, finite interval with provision for indefinite integrals∙d01as– One-dimensional quadrature, adaptive, semi-infinite interval, weight function cos(( omega x)) or sin(( omega x)) ∙d01at– One-dimensional quadrature, adaptive, finite interval, variant of d01aj efficient on vector machines∙d01au– One-dimensional quadrature, adaptive, finite interval, variant of d01ak efficient on vector machines∙d01ba– One-dimensional Gaussian quadrature∙d01bb–Pre-computed weights and abscissae for Gaussian quadrature rules, restricted choice of rule∙d01bc– Calculation of weights and abscissae for Gaussian quadrature rules, general choice of rule∙d01bd–One-dimensional quadrature, non-adaptive, finite interval ∙d01da– Two-dimensional quadrature, finite region∙d01ea– Multi-dimensional adaptive quadrature overhyper-rectangle, multiple integrands∙d01fb– Multi-dimensional Gaussian quadrature overhyper-rectangle∙d01fc– Multi-dimensional adaptive quadrature overhyper-rectangle∙d01fd– Multi-dimensional quadrature, Sag–Szekeres method, general product region or n-sphere∙d01ga– One-dimensional quadrature, integration of function defined by data values, Gill–Miller method∙d01gb–Multi-dimensional quadrature over hyper-rectangle, Monte Carlo method∙d01gc– Multi-dimensional quadrature, general product region, number-theoretic method∙d01gd– Multi-dimensional quadrature, general product region, number-theoretic method, variant of d01gc efficient on vectormachines∙d01gy– Korobov optimal coefficients for use in d01gc or d01gd, when number of points is prime∙d01gz– Korobov optimal coefficients for use in d01gc or d01gd, when number of points is product of two primes∙d01ja– Multi-dimensional quadrature over an n-sphere, allowing for badly behaved integrands∙d01pa– Multi-dimensional quadrature over an n-simplexD02 –Ordinary Differential EquationsIntegrators for Stiff Ordinary Differential Systems 常微分∙d02ag– ODEs, boundary value problem, shooting and matching technique, allowing interior matching point, general parameters to be determined∙d02bg–ODEs, IVP, Runge–Kutta–Merson method, until a component attains given value (simple driver)∙d02bh–ODEs, IVP, Runge–Kutta–Merson method, until function of solution is zero (simple driver)∙d02bj–ODEs, IVP, Runge–Kutta method, until function of solution is zero, integration over range with intermediate output (simple driver)∙d02cj–ODEs, IVP, Adams method, until function of solution is zero, intermediate output (simple driver)∙d02ej– ODEs, stiff IVP, BDF method, until function of solution is zero, intermediate output (simple driver)∙d02ga–ODEs, boundary value problem, finite difference technique with deferred correction, simple nonlinear problem∙d02gb–ODEs, boundary value problem, finite difference technique with deferred correction, general linear problem∙d02ha– ODEs, boundary value problem, shooting and matching, boundary values to be determined∙d02hb– ODEs, boundary value problem, shooting and matching, general parameters to be determined∙d02ja– ODEs, boundary value problem, collocation and least-squares, single nth-order linear equation∙d02jb– ODEs, boundary value problem, collocation and least-squares, system of first-order linear equations∙d02ka– Second-order Sturm–Liouville problem, regular system, finite range, eigenvalue only∙d02kd– Second-order Sturm–Liouville problem, regular/singular system, finite/infinite range, eigenvalue only, user-specified break-points∙d02ke– Second-order Sturm–Liouville problem, regular/singular system, finite/infinite range, eigenvalue and eigenfunction,user-specified break-points∙d02la– Second-order ODEs, IVP, Runge–Kutta–Nystrom method ∙d02lx– Second-order ODEs, IVP, setup for d02la∙d02ly– Second-order ODEs, IVP, diagnostics for d02la∙d02lz– Second-order ODEs, IVP, interpolation for d02la∙d02mc– Implicit ODE/DAEs, IVP, DASSL method continuation for d02ne∙d02mv– ODEs, IVP, DASSL method, setup for D02M–N functions ∙d02mw– Implicit ODE/DAEs, IVP, setup for d02ne∙d02mz– ODEs, IVP, interpolation for D02M–N functions, natural interpolant∙d02nb– Explicit ODEs, stiff IVP, full Jacobian (comprehensive) ∙d02nc–Explicit ODEs, stiff IVP, banded Jacobian (comprehensive) ∙d02nd–Explicit ODEs, stiff IVP, sparse Jacobian (comprehensive) ∙d02ne– Implicit ODE/DAEs, IVP, DASSL method integrator∙d02ng– Implicit/algebraic ODEs, stiff IVP, full Jacobian (comprehensive)∙d02nh– Implicit/algebraic ODEs, stiff IVP, banded Jacobian (comprehensive)∙d02nj– Implicit/algebraic ODEs, stiff IVP, sparse Jacobian (comprehensive)∙d02nm– Explicit ODEs, stiff IVP (reverse communication, comprehensive)∙d02nn–Implicit/algebraic ODEs, stiff IVP (reverse communication, comprehensive)∙d02np– Implicit ODE/DAEs, IVP linear algebra setup routine for d02ne∙d02nr–ODEs, IVP, for use with D02M–N functions, sparse Jacobian, enquiry function∙d02ns–ODEs, IVP, for use with D02M–N functions, full Jacobian, linear algebra set up∙d02nt–ODEs, IVP, for use with D02M–N functions, banded Jacobian, linear algebra set up∙d02nu–ODEs, IVP, for use with D02M–N functions, sparse Jacobian, linear algebra set up∙d02nv– ODEs, IVP, BDF method, setup for D02M–N functions∙d02nw– ODEs, IVP, Blend method, setup for D02M–N functions ∙d02nx– ODEs, IVP, sparse Jacobian, linear algebra diagnostics, for use with D02M–N functions∙d02ny– ODEs, IVP, integrator diagnostics, for use with D02M–N functions∙d02nz–ODEs, IVP, setup for continuation calls to integrator, for use with D02M–N functions∙d02pc– ODEs, IVP, Runge–Kutta method, integration over range with output∙d02pd–ODEs, IVP, Runge–Kutta method, integration over one step ∙d02pv– ODEs, IVP, setup for d02pc and d02pd∙d02pw– ODEs, IVP, resets end of range for d02pd∙d02px– ODEs, IVP, interpolation for d02pd∙d02py– ODEs, IVP, integration diagnostics for d02pc and d02pd ∙d02pz–ODEs, IVP, error assessment diagnostics for d02pc and d02pd ∙d02qf– ODEs, IVP, Adams method with root-finding (forward communication, comprehensive)∙d02qg– ODEs, IVP, Adams method with root-finding (reverse communication, comprehensive)∙d02qw– ODEs, IVP, setup for d02qf and d02qg∙d02qx– ODEs, IVP, diagnostics for d02qf and d02qg∙d02qy– ODEs, IVP, root-finding diagnostics for d02qf and d02qg ∙d02qz– ODEs, IVP, interpolation for d02qf or d02qg∙d02ra– ODEs, general nonlinear boundary value problem, finite difference technique with deferred correction, continuationfacility∙d02sa– ODEs, boundary value problem, shooting and matching technique, subject to extra algebraic equations, generalparameters to be determined∙d02tg–nth-order linear ODEs, boundary value problem, collocation and least-squares∙d02tk– ODEs, general nonlinear boundary value problem, collocation technique∙d02tv–ODEs, general nonlinear boundary value problem, setup for d02tk∙d02tx– ODEs, general nonlinear boundary value problem, continuation facility for d02tk∙d02ty– ODEs, general nonlinear boundary value problem, interpolation for d02tk∙d02tz– ODEs, general nonlinear boundary value problem, diagnostics for d02tk∙d02xj– ODEs, IVP, interpolation for D02M–N functions, natural interpolant∙d02xk– ODEs, IVP, interpolation for D02M–N functions, C_1 interpolant∙d02za– ODEs, IVP, weighted norm of local error estimate for D02M–N functionsD03 – Partial Differential Equations 偏微分∙d03ea– Elliptic PDE, Laplace's equation, two-dimensional arbitrary domain∙d03eb– Elliptic PDE, solution of finite difference equations by SIP, five-point two-dimensional molecule, iterate to convergence ∙d03ec– Elliptic PDE, solution of finite difference equations by SIP for seven-point three-dimensional molecule, iterate toconvergence∙d03ed– Elliptic PDE, solution of finite difference equations bya multigrid technique∙d03ee– Discretize a second-order elliptic PDE on a rectangle ∙d03fa– Elliptic PDE, Helmholtz equation, three-dimensional Cartesian co-ordinates∙d03ma– Triangulation of plane region∙d03nc–Finite difference solution of the Black–Scholes equations ∙d03nd– Analytic solution of the Black–Scholes equations∙d03ne– Compute average values for d03nd∙d03pc–General system of parabolic PDEs, method of lines, finite differences, one space variable∙d03pd– General system of parabolic PDEs, method of lines, Chebyshev C^0 collocation, one space variable∙d03pe–General system of first-order PDEs, method of lines, Keller box discretisation, one space variable∙d03pf– General system of convection-diffusion PDEs with source terms in conservative form, method of lines, upwind scheme using numerical flux function based on Riemann solver, one space variable ∙d03ph–General system of parabolic PDEs, coupled DAEs, method of lines, finite differences, one space variable∙d03pj–General system of parabolic PDEs, coupled DAEs, method of lines, Chebyshev C^0 collocation, one space variable∙d03pk– General system of first-order PDEs, coupled DAEs, method of lines, Keller box discretisation, one space variable∙d03pl– General system of convection-diffusion PDEs with source terms in conservative form, coupled DAEs, method of lines, upwind scheme using numerical flux function based on Riemann solver, one space variable∙d03pp–General system of parabolic PDEs, coupled DAEs, method of lines, finite differences, remeshing, one space variable∙d03pr– General system of first-order PDEs, coupled DAEs, method of lines, Keller box discretisation, remeshing, one space variable ∙d03ps– General system of convection-diffusion PDEs with source terms in conservative form, coupled DAEs, method of lines, upwind scheme using numerical flux function based on Riemann solver, remeshing, one space variable∙d03pu– Roe's approximate Riemann solver for Euler equations in conservative form, for use with d03pf, d03pl and d03ps∙d03pv–Osher's approximate Riemann solver for Euler equations in conservative form, for use with d03pf, d03pl and d03ps∙d03pw– Modified HLL Riemann solver for Euler equations in conservative form, for use with d03pf, d03pl and d03ps∙d03px– Exact Riemann Solver for Euler equations in conservative form, for use with d03pf, d03pl and d03ps∙d03py– PDEs, spatial interpolation with d03pd or d03pj∙d03pz– PDEs, spatial interpolation with d03pc, d03pe, d03pf, d03ph, d03pk, d03pl, d03pp, d03pr or d03ps∙d03ra– General system of second-order PDEs, method of lines, finite differences, remeshing, two space variables, rectangular region∙d03rb– General system of second-order PDEs, method of lines, finite differences, remeshing, two space variables, rectilinear region∙d03ry– Check initial grid data in d03rb∙d03rz– Extract grid data from d03rb∙d03ua– Elliptic PDE, solution of finite difference equations by SIP, five-point two-dimensional molecule, one iteration∙d03ub– Elliptic PDE, solution of finite difference equations by SIP, seven-point three-dimensional molecule, one iterationD04 – Numerical Differentiation 数值微分∙d04aa– Numerical differentiation, derivatives up to order 14, function of one real variableD05 – Integral Equations 积分∙d05aa– Linear non-singular Fredholm integral equation, second kind, split kernel∙d05ab– Linear non-singular Fredholm integral equation, second kind, smooth kernel∙d05ba– Nonlinear Volterra convolution equation, second kind ∙d05bd– Nonlinear convolution Volterra–Abel equation, second kind, weakly singular∙d05be–Nonlinear convolution Volterra–Abel equation, first kind, weakly singular∙d05bw– Generate weights for use in solving Volterra equations ∙d05by– Generate weights for use in solving weakly singular Abel-type equationsD06 – Mesh Generation∙d06aa– Generates a two-dimensional mesh using a simple incremental method∙d06ab–Generates a two-dimensional mesh using a Delaunay–Voronoi process∙d06ac–Generates a two-dimensional mesh using an Advancing-front method∙d06ba– Generates a boundary mesh∙d06ca– Uses a barycentering technique to smooth a given mesh ∙d06cb– Generates a sparsity pattern of a Finite Element matrix associated with a given mesh∙d06cc– Renumbers a given mesh using Gibbs method∙d06da– Generates a mesh resulting from an affine transformation of a given mesh∙d06db– Joins together two given adjacent (possibly overlapping) meshesE01 – Interpolation∙e01aa–Interpolated values, Aitken's technique, unequally spaced data, one variable∙e01ab– Interpolated values, Everett's formula, equally spaced data, one variable∙e01ae–Interpolating functions, polynomial interpolant, data may include derivative values, one variable∙e01ba– Interpolating functions, cubic spline interpolant, one variable∙e01be– Interpolating functions, monotonicity-preserving, piecewise cubic Hermite, one variable∙e01bf– Interpolated values, interpolant computed by e01be, function only, one variable∙e01bg– Interpolated values, interpolant computed by e01be, function and first derivative, one variable∙e01bh– Interpolated values, interpolant computed by e01be, definite integral, one variable∙e01da– Interpolating functions, fitting bicubic spline, data on rectangular grid∙e01ra– Interpolating functions, rational interpolant, one variable∙e01rb– Interpolated values, evaluate rational interpolant computed by e01ra, one variable∙e01sa– Interpolating functions, method of Renka and Cline, two variables∙e01sb– Interpolated values, evaluate interpolant computed by e01sa, two variables∙e01sg– Interpolating functions, modified Shepard's method, two variables∙e01sh– Interpolated values, evaluate interpolant computed by e01sg, function and first derivatives, two variables ∙e01tg–Interpolating functions, modified Shepard's method, three variables∙e01th– Interpolated values, evaluate interpolant computed by e01tg, function and first derivatives, three variablesE02 – Curve and Surface Fitting∙e02ac– Minimax curve fit by polynomials∙e02ad– Least-squares curve fit, by polynomials, arbitrary data points∙e02ae– Evaluation of fitted polynomial in one variable from Chebyshev series form (simplified parameter list)∙e02af– Least-squares polynomial fit, special data points (including interpolation)∙e02ag– Least-squares polynomial fit, values and derivatives may be constrained, arbitrary data points∙e02ah– Derivative of fitted polynomial in Chebyshev series form ∙e02aj– Integral of fitted polynomial in Chebyshev series form ∙e02ak– Evaluation of fitted polynomial in one variable from Chebyshev series form∙e02ba– Least-squares curve cubic spline fit (including interpolation)∙e02bb– Evaluation of fitted cubic spline, function only∙e02bc– Evaluation of fitted cubic spline, function and derivatives∙e02bd– Evaluation of fitted cubic spline, definite integral ∙e02be– Least-squares cubic spline curve fit, automatic knot placement∙e02ca– Least-squares surface fit by polynomials, data on lines ∙e02cb– Evaluation of fitted polynomial in two variables∙e02da– Least-squares surface fit, bicubic splines∙e02dc– Least-squares surface fit by bicubic splines with automatic knot placement, data on rectangular grid ∙e02dd– Least-squares surface fit by bicubic splines with automatic knot placement, scattered data∙e02de–Evaluation of fitted bicubic spline at a vector of points ∙e02df– Evaluation of fitted bicubic spline at a mesh of points ∙e02ga– L_1-approximation by general linear function∙e02gb– L_1-approximation by general linear function subject to linear inequality constraints∙e02gc– L_ infinity -approximation by general linear function ∙e02ra–Padé approximants∙e02rb–Evaluation of fitted rational function as computed by e02ra ∙e02za–Sort two-dimensional data into panels for fitting bicubic splinesE04 – Minimizing or Maximizing a Function∙e04ab– Minimum, function of one variable using function values only∙e04bb–Minimum, function of one variable, using first derivative ∙e04cb– Unconstrained minimization using simplex algorithm, function of several variables using function values only ∙e04cc– Unconstrained minimum, simplex algorithm, function of several variables using function values only (comprehensive)∙e04dg–Unconstrained minimum, preconditioned conjugate gradient algorithm, function of several variables using first derivatives (comprehensive)∙e04dk– Supply optional parameter values to e04dg∙e04fc– Unconstrained minimum of a sum of squares, combined Gauss–Newton and modified Newton algorithm using function values only (comprehensive)∙e04fy– Unconstrained minimum of a sum of squares, combined Gauss–Newton and modified Newton algorithm using function values only (easy-to-use)∙e04gb– Unconstrained minimum of a sum of squares, combined Gauss–Newton and quasi-Newton algorithm using first derivatives (comprehensive)∙e04gd– Unconstrained minimum of a sum of squares, combined Gauss–Newton and modified Newton algorithm using firstderivatives (comprehensive)∙e04gy– Unconstrained minimum of a sum of squares, combined Gauss–Newton and quasi-Newton algorithm, using first derivatives (easy-to-use)∙e04gz– Unconstrained minimum of a sum of squares, combined Gauss–Newton and modified Newton algorithm using firstderivatives (easy-to-use)∙e04hc– Check user's function for calculating first derivatives of function∙e04hd– Check user's function for calculating second derivatives of function∙e04he– Unconstrained minimum of a sum of squares, combined Gauss–Newton and modified Newton algorithm, using secondderivatives (comprehensive)∙e04hy– Unconstrained minimum of a sum of squares, combined Gauss–Newton and modified Newton algorithm, using secondderivatives (easy-to-use)∙e04jy– Minimum, function of several variables, quasi-Newton algorithm, simple bounds, using function values only (easy-to-use) ∙e04kd– Minimum, function of several variables, modified Newton algorithm, simple bounds, using first derivatives (comprehensive) ∙e04ky– Minimum, function of several variables, quasi-Newton algorithm, simple bounds, using first derivatives (easy-to-use) ∙e04kz– Minimum, function of several variables, modified Newton algorithm, simple bounds, using first derivatives (easy-to-use) ∙e04lb– Minimum, function of several variables, modified Newton algorithm, simple bounds, using first and second derivatives(comprehensive)∙e04ly– Minimum, function of several variables, modified Newton algorithm, simple bounds, using first and second derivatives(easy-to-use)∙e04mf– LP problem (dense)∙e04mh– Supply optional parameter values to e04mf∙e04nc– Convex QP problem or linearly-constrained linear least-squares problem (dense)∙e04ne– Supply optional parameter values to e04nc∙e04nf– QP problem (dense)∙e04nh– Supply optional parameter values to e04nf∙e04nk– LP or QP problem (sparse)∙e04nm– Supply optional parameter values to e04nk∙e04np– Initialization function for e04nq∙e04nq– LP or QP problem (suitable for sparse problems)∙e04ns– Set a single option for e04nq from a character string ∙e04nt– Set a single option for e04nq from an integer argument ∙e04nu– Set a single option for e04nq from a real argument∙e04nx– Get the setting of an integer valued option of e04nq ∙e04ny– Get the setting of a real valued option of e04nq∙e04uc– Minimum, function of several variables, sequential QP method, nonlinear constraints, using function values andoptionally first derivatives (forward communication,comprehensive)∙e04ue– Supply optional parameter values to e04uc or e04uf∙e04uf– Minimum, function of several variables, sequential QP method, nonlinear constraints, using function values andoptionally first derivatives (reverse communication,comprehensive)∙e04ug– NLP problem (sparse)∙e04uj– Supply optional parameter values to e04ug∙e04ur– Supply optional parameter values to e04us∙e04us– Minimum of a sum of squares, nonlinear constraints, sequential QP method, using function values and optionally first derivatives (comprehensive)∙e04vg– Initialization function for e04vh∙e04vh– General sparse nonlinear optimizer∙e04vj– Determine the pattern of nonzeros in the Jacobian matrix for e04vh∙e04vl– Set a single option for e04vh from a character string ∙e04vm– Set a single option for e04vh from an integer argument ∙e04vn– Set a single option for e04vh from a real argument∙e04vr– Get the setting of an integer valued option of e04vh ∙e04vs– Get the setting of a real valued option of e04vh∙e04wb– Initialization function for e04dg, e04mf, e04nc, e04nf, e04uf, e04ug, e04us∙e04wc– Initialization function for e04wd∙e04wd– Solves the nonlinear programming (NP) problem∙e04wf– Set a single option for e04wd from a character string ∙e04wg– Set a single option for e04wd from an integer argument ∙e04wh– Set a single option for e04wd from a real argument ∙e04wk– Get the setting of an integer valued option of e04wd ∙e04wl– Get the setting of a real valued option of e04wd∙e04xa– Estimate (using numerical differentiation) gradient and/or Hessian of a function∙e04ya– Check user's function for calculating Jacobian of first derivatives∙e04yb–Check user's function for calculating Hessian of a sum of squares∙e04yc– Covariance matrix for nonlinear least-squares problem (unconstrained)∙e04zc– Check user's functions for calculating first derivatives of function and constraintsE05 – Global Optimization of a Function∙e05ja– Initialization function for e05jb∙e05jb– Global optimization by multi-level coordinate search, simple bounds, using function values only∙e05jd–Set a single optional parameter for e05jb from a character string∙e05je– Set a single optional parameter for e05jb from an "ON"/"OFF"-valued character argument∙e05jf–Set a single optional parameter for e05jb from an integer argument∙e05jg– Set a single optional parameter for e05jb from a real argument∙e05jh–Determine whether an optional parameter for e05jb has been set by you or not∙e05jj–Get the setting of an "ON"/"OFF"-valued character optional parameter of e05jb∙e05jk– Get the setting of an integer-valued optional parameter of e05jb∙e05jl– Get the setting of a real-valued optional parameter of e05jbF – Linear Algebra。
极坐标系下弹性问题的重心插值配点法

极坐标系下弹性问题的重心插值配点法李树忱;王兆清;袁超【摘要】针对岩土工程中的孔洞及曲梁问题,提出一种在极坐标系下求解二维弹性问题的重心插值配点法.该方法分别在r和θ方向分别布置m和n个节点,生成求解区域上的节点.以一维重心Lagrange插值的张量积插值形式近似二维弹性问题的位移函数,代入位移表达的平衡方程和边界条件,平衡方程和边界条件分别在所有的计算节点和边界节点上精确成立,得到极坐标下弹性力学平衡方程和边界条件的离散代数表达式.利用一维重心Lagrange插值微分矩阵,将离散的平衡方程和边界条件表达为矩阵形式.利用置换法施加边界条件,求得在计算节点处的位移,进而通过微分矩阵直接求得计算节点处的应力.数值算例表明:极坐标下重心插值配点法具有计算格式简单、程序实施容易和计算精度高的特点.【期刊名称】《中南大学学报(自然科学版)》【年(卷),期】2013(044)005【总页数】10页(P2031-2040)【关键词】弹性问题;极坐标系;重心Lagrange插值;微分矩阵;重心插值配点法;无网格方法【作者】李树忱;王兆清;袁超【作者单位】山东大学岩土与结构工程研究中心,山东济南,250061;山东建筑大学工程力学研究所,山东济南,250101;山东大学岩土与结构工程研究中心,山东济南,250061【正文语种】中文【中图分类】TU443弹性力学是研究岩石变形、断裂破坏问题的重要基础,对于复杂的岩石弹性力学问题一般很难得到问题的解析解,数值计算方法成为一种重要的研究手段。
研究弹性力学问题的基本数值方法有有限元方法和有限差分法。
有限元方法是一种基于低阶多项式插值的Galerkin方法,其计算精度依赖于单元大小和积分点数量的选取;有限差分法采用差分算子近似微分算子,其计算精度依赖于差分步长的大小。
近年来兴起的各种类型无网格方法,在岩石力学领域得到广泛关注[1-3]。
能够采用较少的计算代价,得到问题的高精度数值解,是数值分析领域研究者努力寻求的目标。
Global Optimization for Estimating a BRDF with Multiple Specular Lobes
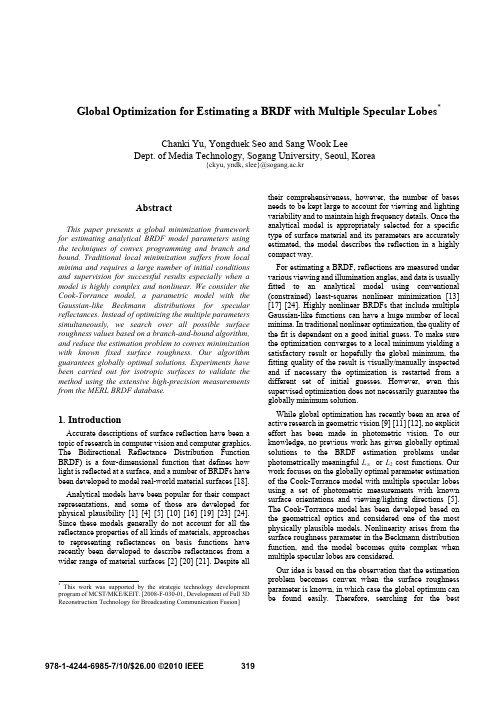
Abstract
This paper presents a global minimization framework for estimating analytical BRDF model parameters using the techniques of convex programming and branch and bound. Traditional local minimization suffers from local minima and requires a large number of initial conditions and supervision for successful results especially when a model is highly complex and nonlinear. We consider the Cook-Torrance model, a parametric model with the Gaussian-like Beckmann distributions for specular reflectances. Instead of optimizing the multiple parameters simultaneously, we search over all possible surface roughness values based on a branch-and-bound algorithm, and reduce the estimation problem to convex minimization with known fixed surface roughness. Our algorithm guarantees globally optimal solutions. Experiments have been carried out for isotropic surfaces to validate the method using the extensive high-precision measurements from the MERL BRDF database.*
Chebyshev配置点法解Volterra型积分微分方程
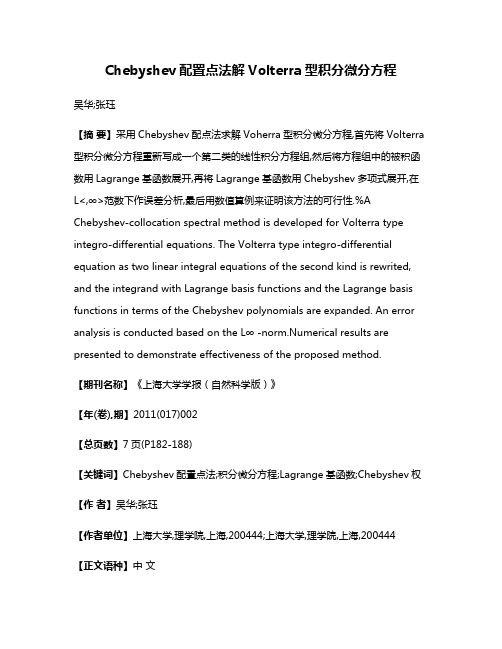
Chebyshev配置点法解Volterra型积分微分方程吴华;张珏【摘要】采用Chebyshev配点法求解Voherra型积分微分方程,首先将Volterra 型积分微分方程重新写成一个第二类的线性积分方程组,然后将方程组中的被积函数用Lagrange基函数展开,再将Lagrange基函数用Chebyshev多项式展开,在L<,∞>范数下作误差分析,最后用数值算例来证明该方法的可行性.%A Chebyshev-collocation spectral method is developed for Volterra type integro-differential equations. The Volterra type integro-differential equation as two linear integral equations of the second kind is rewrited, and the integrand with Lagrange basis functions and the Lagrange basis functions in terms of the Chebyshev polynomials are expanded. An error analysis is conducted based on the L∞ -norm.Numerical results are presented to demonstrate effectiveness of the proposed method.【期刊名称】《上海大学学报(自然科学版)》【年(卷),期】2011(017)002【总页数】7页(P182-188)【关键词】Chebyshev配置点法;积分微分方程;Lagrange基函数;Chebyshev权【作者】吴华;张珏【作者单位】上海大学,理学院,上海,200444;上海大学,理学院,上海,200444【正文语种】中文【中图分类】O175.6由于 Volterra型积分微分方程带有记忆性质,与传统的常微分方程和偏微分方程相比,有着本质区别,因此,该方程的数值求解也更为困难.如何快速高效地求解这类方程,一直受到许多学者的关注.由于当解充分光滑时,用谱方法求出的近似解具有很高的精度,所以谱方法经常被运用到近似计算中.因此,本研究采用 Chebyshev配点法解下述 Volterra型积分微分方程:式中,函数 f(t),g(t)和核函数 R(t,s)都是充分光滑的.为了分析方便,将式 (1)和 (2)转换为定义在[-1,1]上的等价问题.通过变量替换,将式(1)和(2)转化为Jiang等[1]把给定的积分微分方程转换成第二类积分方程的方程组,因此,解积分微分方程的主要工作就转变成求解这个积分方程组.Atkinson[2]提出了多种求解第二类积分方程的数值方法,并且作了误差分析.Tang等[3]提出了用 Legendre配点法求解Volterra型积分方程的方法.Chen等[4-5]分别用Chebyshev配点法和Jacobi配点法求解了带有奇异核的第二类 Volterra型积分方程.Elnagar等[6]用Chebyshev谱方法求解了非线性 Volterra-Hammerstein积分方程.Tian[7]使用谱元法求解Volterra型积分方程,并将谱元法与梯形公式和Simpson公式作了比较,得到谱元法具有更高的收敛阶.Tang等[8]提出了谱处理技术在提高数值解的精度上的应用.另外,其他研究者还提出了获得带有奇异核 Volterra型积分方程和积分微分方程的高阶收敛性质的方法[9-11].本研究主要是利用 Chebyshev配点法求出Volterra型积分微分方程 (3)和 (4)的数值解.虽然Legendre点在计算中具有很高的稳定性,但是Legendre多项式的权是隐式的,不便于计算.然而,Chebyshev点和权能够直接使用,计算方便.而且Chebyshev多项式能够利用快速傅里叶变换 (fast Fourier transform,FFT),因此,Chebyshev谱方法更常用于工程计算中.一般情况下,Chebyshev谱方法多用于计算带有奇异核的积分方程,而不带奇异核的积分方程经常用 Legendre谱方法来计算.本研究采用 Chebyshev谱方法计算不带奇异核的积分方程,并对该方法作了严格的误差分析,用数值算例证明了其收敛阶.本研究中,C代表一个与N无关的正常数,但是依赖于所给方程 a(x),b(x),K(x,s)的边界.类似文献 [1]中的方法,将式 (3)和 (4)写为一种新的形式.记z=u′,则式 (3)等价于一个关于 z的第二类线性 Volterra型积分方程,即出,此方法能达到预期的谱收敛精度.之后,可用 Gauss积分公式来求解上面的方程组中的积分部分.【相关文献】[1] JIANG Y J.On spectral methods for Volterra type integro-differentialequations[J].JComput App lMath,2009,230(2):333-340.[2] ATKINSON K E. The numerical solution of intgral equations of the second kind [M]. Cambridge:Cambridge University Press,1997.[3] TANG T,XU X,CHENG J.On spectral methods for Volterra type integral equations and the convergence analysis[J].J ComputMath,2009,26:825-837.[4] CHEN Y P,TANG T.Convergence analysis for the Cheb yshev collocation methods to Volterra integral equations with aweakly singular kernel[J].SIAM JNumerAnal,2009,233:938-950.[5] CHEN Y P,TANG T.Convergence analysisof the Jacobi spectral-collocation methods for Volterra integral equations with a weakly singular kernel[J].Math Comput,2010,79:147-167.[6] ELNAGAR GN,KAZEM IM.Chebyshev spectral solution of nonlinear Volterra-Hammerstein integral equations[J].J Comput App Math,1996,76:147-158.[7] TIAN H C. Spectral methods for volterrra integral equations[D].Burnaby:Simon Fraser University,1995.[8] TANG T,XU X.Accuracy enhancement using spectral postprocessing for differential equations and integral equations[J].Commun Comput Phys,2009,5:779-792.[9] BRUNNER H.Polynomial spline collocation methods for Volterra integro-differential equationswith weakly singular kernels[J].IMA JNumer Anal,1986,6(2):221-139.[10] DIOGO T,MCKEE S,TANG T.Collocation methods for second-kind Volterra integral equations with weakly singular kernels[C]∥Proceedingsof The Royal Society of Edinburgh.1994,124A:199-210.[11] TANG T. Superconvergence of numerical solutions to weakly singular Volterra integro-differential equations[J].Numer Math,1992,61:373-382.。
科学研究项目中期进展报告
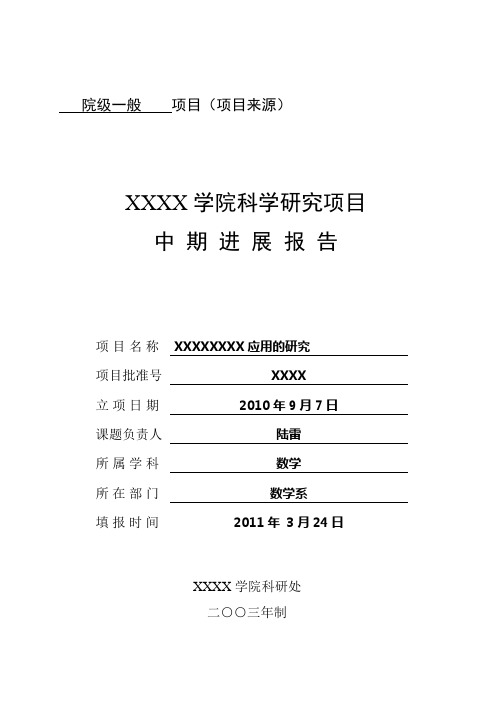
签章: 年 月
8.院学术委意见
签章: 年 月
2、已经对三种基本谱方法:Galerkin法,Tau法和Collocation法或pseudo-spectral法进行了深入的研究以及应用:
1)使用pseudo-spectral法(即伪谱法)对一类二维Navier-Stokes方程解的研究;
2)使用pseudo-spectral法(即伪谱法)对一类线性常微分方程和Burgers方程的应用;
5.当年经费使用情况和下年度经费安排
当年
下年度
收集材料费(打印费),网络使用费用,调研费(交通费、住宿费),课题组成员参加相关会议等已经使用近一千元。
材料收集费用,网络使用费用,调研费,发表论文版面费等约一千元。
6.建议及其它
建议学校可以提供更多的网上资源,特别是外文资料。
项目负责人: (签字) 年 月
2.项目未按原计划完成部分及原因
(1)未按原计划完成部分
在对预期的FourierGalerkin法、Chebyshev Collocation进行研究时,发现这两种现有的研究方法出现漏洞,如果不附加另外的初始条件以及边界条件可能对现有的研究方法无法继续进行,故在此问题的研究上还需要深入考虑。
(2)原因
在前期的考虑中不够细致,对预期的结果的研究可能出现偏差。
3.根据国内外学科发展作了必要调整和变动的项目内容
(1)所作的必要调整:
对预期的4种方法中的FourierGalerkin法、Chebyshev Collocation法作另外考虑,考查对此两类方法附加一定的初始和边界条件来进行考虑。
(2)变动的项目内容:
目前还不需要对项目的基本内容作实质性变动。
统计学术语中英文对照

统计学术语中英文对照Absolute deviation 绝对离差Absolute number 绝对数Absolute residuals 绝对残差Acceleration array 加速度立体阵Acceleration in an arbitrary direction 任意方向上的加速度Acceleration normal 法向加速度Acceleration space dimension 加速度空间的维数Acceleration tangential 切向加速度Acceleration vector 加速度向量Acceptable hypothesis 可接受假设Accumulation 累积Accuracy 准确度Actual frequency 实际频数Adaptive estimator 自适应估计量Addition 相加Addition theorem 加法定理Additivity 可加性Adjusted rate 调整率Adjusted value 校正值Admissible error 容许误差Aggregation 聚集性Alternative hypothesis 备择假设Among groups 组间Amounts 总量Analysis of correlation 相关分析Analysis of covariance 协方差分析Analysis of regression 回归分析Analysis of time series 时间序列分析Analysis of variance 方差分析Angular transformation 角转换ANOVA (analysis of variance)方差分析ANOVA Models 方差分析模型Arcing 弧/弧旋Arcsine transformation 反正弦变换Area under the curve 曲线面积AREG 评估从一个时间点到下一个时间点回归相关时的误差ARIMA 季节和非季节性单变量模型的极大似然估计Arithmetic grid paper 算术格纸Arithmetic mean 算术平均数Arrhenius relation 艾恩尼斯关系Assessing fit 拟合的评估Associative laws 结合律—Asymmetric distribution 非对称分布Asymptotic bias 渐近偏倚Asymptotic efficiency 渐近效率Asymptotic variance 渐近方差Attributable risk 归因危险度Attribute data 属性资料Attribution 属性Autocorrelation 自相关Autocorrelation of residuals 残差的自相关Average 平均数Average confidence interval length 平均置信区间长度Average growth rate 平均增长率Bar chart 条形图Bar graph 条形图Base period 基期Bayes' theorem Bayes定理Bell-shaped curve 钟形曲线Bernoulli distribution 伯努力分布Best-trim estimator 最好切尾估计量Bias 偏性Binary logistic regression 二元逻辑斯蒂回归Binomial distribution 二项分布Bisquare 双平方Bivariate Correlate 二变量相关Bivariate normal distribution 双变量正态分布Bivariate normal population 双变量正态总体Biweight interval 双权区间Biweight M-estimator 双权M估计量Block 区组/配伍组BMDP(Biomedical computer programs) BMDP统计软件包Boxplots 箱线图/箱尾图Breakdown bound 崩溃界/崩溃点Canonical correlation 典型相关Caption 纵标目Case-control study 病例对照研究Categorical variable 分类变量Catenary 悬链线Cauchy distribution 柯西分布Cause-and-effect relationship 因果关系Cell 单元Censoring 终检Center of symmetry 对称中心—Centering and scaling 中心化和定标Central tendency 集中趋势Central value 中心值CHAID -χ2 Automatic Interaction Detector 卡方自动交互检测Chance 机遇Chance error 随机误差Chance variable 随机变量Characteristic equation 特征方程Characteristic root 特征根Characteristic vector 特征向量Chebshev criterion of fit 拟合的切比雪夫准则Chernoff faces 切尔诺夫脸谱图Chi-square test 卡方检验/χ2检验Choleskey decomposition 乔洛斯基分解Circle chart 圆图Class interval 组距Class mid-value 组中值Class upper limit 组上限Classified variable 分类变量Cluster analysis 聚类分析Cluster sampling 整群抽样Code 代码Coded data 编码数据Coding 编码Coefficient of contingency 列联系数Coefficient of determination 决定系数Coefficient of multiple correlation 多重相关系数Coefficient of partial correlation 偏相关系数Coefficient of production-moment correlation 积差相关系数Coefficient of rank correlation 等级相关系数Coefficient of regression 回归系数Coefficient of skewness 偏度系数Coefficient of variation 变异系数Cohort study 队列研究Column 列Column effect 列效应Column factor 列因素Combination pool 合并Combinative table 组合表Common factor 共性因子Common regression coefficient 公共回归系数Common value 共同值Common variance 公共方差Common variation 公共变异—Communality variance 共性方差Comparability 可比性Comparison of bathes 批比较Comparison value 比较值Compartment model 分部模型Compassion 伸缩Complement of an event 补事件Complete association 完全正相关Complete dissociation 完全不相关Complete statistics 完备统计量Completely randomized design 完全随机化设计Composite event 联合事件Composite events 复合事件Concavity 凹性Conditional expectation 条件期望Conditional likelihood 条件似然Conditional probability 条件概率Conditionally linear 依条件线性Confidence interval 置信区间Confidence limit 置信限Confidence lower limit 置信下限Confidence upper limit 置信上限Confirmatory Factor Analysis 验证性因子分析Confirmatory research 证实性实验研究Confounding factor 混杂因素Conjoint 联合分析Consistency 相合性Consistency check 一致性检验Consistent asymptotically normal estimate 相合渐近正态估计Consistent estimate 相合估计Constrained nonlinear regression 受约束非线性回归Constraint 约束Contaminated distribution 污染分布Contaminated Gausssian 污染高斯分布Contaminated normal distribution 污染正态分布Contamination 污染Contamination model 污染模型Contingency table 列联表Contour 边界线Contribution rate 贡献率Control 对照Controlled experiments 对照实验Conventional depth 常规深度Convolution 卷积—Corrected factor 校正因子Corrected mean 校正均值Correction coefficient 校正系数Correctness 正确性Correlation coefficient 相关系数Correlation index 相关指数Correspondence 对应Counting 计数Counts 计数/频数Covariance 协方差Covariant 共变Cox Regression Cox回归Criteria for fitting 拟合准则Criteria of least squares 最小二乘准则Critical ratio 临界比Critical region 拒绝域Critical value 临界值Cross-over design 交叉设计Cross-section analysis 横断面分析Cross-section survey 横断面调查Crosstabs 交叉表Cross-tabulation table 复合表Cube root 立方根Cumulative distribution function 分布函数Cumulative probability 累计概率Curvature 曲率/弯曲Curvature 曲率Curve fit 曲线拟和Curve fitting 曲线拟合Curvilinear regression 曲线回归Curvilinear relation 曲线关系Cut-and-try method 尝试法Cycle 周期Cyclist 周期性D test D检验Data acquisition 资料收集Data bank 数据库Data capacity 数据容量Data deficiencies 数据缺乏Data handling 数据处理Data manipulation 数据处理Data processing 数据处理Data reduction 数据缩减—Data set 数据集Data sources 数据来源Data transformation 数据变换Data validity 数据有效性Data-in 数据输入Data-out 数据输出Dead time 停滞期Degree of freedom 自由度Degree of precision 精密度Degree of reliability 可靠性程度Degression 递减Density function 密度函数Density of data points 数据点的密度Dependent variable 应变量/依变量/因变量Dependent variable 因变量Depth 深度Derivative matrix 导数矩阵Derivative-free methods 无导数方法Design 设计Determinacy 确定性Determinant 行列式Determinant 决定因素Deviation 离差Deviation from average 离均差Diagnostic plot 诊断图Dichotomous variable 二分变量Differential equation 微分方程Direct standardization 直接标准化法Discrete variable 离散型变量DISCRIMINANT 判断Discriminant analysis 判别分析Discriminant coefficient 判别系数Discriminant function 判别值Dispersion 散布/分散度Disproportional 不成比例的Disproportionate sub-class numbers 不成比例次级组含量Distribution free 分布无关性/免分布Distribution shape 分布形状Distribution-free method 任意分布法Distributive laws 分配律Disturbance 随机扰动项Dose response curve 剂量反应曲线Double blind method 双盲法Double blind trial 双盲试验—Double exponential distribution 双指数分布Double logarithmic 双对数Downward rank 降秩Dual-space plot 对偶空间图DUD 无导数方法Duncan's new multiple range method 新复极差法/Duncan新法Effect 实验效应Eigenvalue 特征值Eigenvector 特征向量Ellipse 椭圆Empirical distribution 经验分布Empirical probability 经验概率单位Enumeration data 计数资料Equal sun-class number 相等次级组含量Equally likely 等可能Equivariance 同变性Error 误差/错误Error of estimate 估计误差Error type I 第一类错误Error type II 第二类错误Estimand 被估量Estimated error mean squares 估计误差均方Estimated error sum of squares 估计误差平方和Euclidean distance 欧式距离Event 事件Event 事件Exceptional data point 异常数据点Expectation plane 期望平面Expectation surface 期望曲面Expected values 期望值Experiment 实验Experimental sampling 试验抽样Experimental unit 试验单位Explanatory variable 说明变量Exploratory data analysis 探索性数据分析Explore Summarize 探索-摘要Exponential curve 指数曲线Exponential growth 指数式增长EXSMOOTH 指数平滑方法Extended fit 扩充拟合Extra parameter 附加参数Extrapolation 外推法Extreme observation 末端观测值—Extremes 极端值/极值F distribution F分布F test F检验Factor 因素/因子Factor analysis 因子分析Factor Analysis 因子分析Factor score 因子得分Factorial 阶乘Factorial design 析因试验设计False negative 假阴性False negative error 假阴性错误Family of distributions 分布族Family of estimators 估计量族Fanning 扇面Fatality rate 病死率Field investigation 现场调查Field survey 现场调查Finite population 有限总体Finite-sample 有限样本First derivative 一阶导数First principal component 第一主成分First quartile 第一四分位数Fisher information 费雪信息量Fitted value 拟合值Fitting a curve 曲线拟合Fixed base 定基Fluctuation 随机起伏Forecast 预测Four fold table 四格表Fourth 四分点Fraction blow 左侧比率Fractional error 相对误差Frequency 频率Frequency polygon 频数多边图Frontier point 界限点Function relationship 泛函关系Gamma distribution 伽玛分布Gauss increment 高斯增量Gaussian distribution 高斯分布/正态分布Gauss-Newton increment 高斯-牛顿增量General census 全面普查GENLOG (Generalized liner models) 广义线性模型—Geometric mean 几何平均数Gini's mean difference 基尼均差GLM (General liner models) 通用线性模型Goodness of fit 拟和优度/配合度Gradient of determinant 行列式的梯度Graeco-Latin square 希腊拉丁方Grand mean 总均值Gross errors 重大错误Gross-error sensitivity 大错敏感度Group averages 分组平均Grouped data 分组资料Guessed mean 假定平均数Half-life 半衰期Hampel M-estimators 汉佩尔M估计量Happenstance 偶然事件Harmonic mean 调和均数Hazard function 风险均数Hazard rate 风险率Heading 标目Heavy-tailed distribution 重尾分布Hessian array 海森立体阵Heterogeneity 不同质Heterogeneity of variance 方差不齐Hierarchical classification 组内分组Hierarchical clustering method 系统聚类法High-leverage point 高杠杆率点HILOGLINEAR 多维列联表的层次对数线性模型Hinge 折叶点Histogram 直方图Historical cohort study 历史性队列研究Holes 空洞HOMALS 多重响应分析Homogeneity of variance 方差齐性Homogeneity test 齐性检验Huber M-estimators 休伯M估计量Hyperbola 双曲线Hypothesis testing 假设检验Hypothetical universe 假设总体Impossible event 不可能事件Independence 独立性Independent variable 自变量Index 指标/指数—Indirect standardization 间接标准化法Individual 个体Inference band 推断带Infinite population 无限总体Infinitely great 无穷大Infinitely small 无穷小Influence curve 影响曲线Information capacity 信息容量Initial condition 初始条件Initial estimate 初始估计值Initial level 最初水平Interaction 交互作用Interaction terms 交互作用项Intercept 截距Interpolation 内插法Interquartile range 四分位距Interval estimation 区间估计Intervals of equal probability 等概率区间Intrinsic curvature 固有曲率Invariance 不变性Inverse matrix 逆矩阵Inverse probability 逆概率Inverse sine transformation 反正弦变换Iteration 迭代Jacobian determinant 雅可比行列式Joint distribution function 分布函数Joint probability 联合概率Joint probability distribution 联合概率分布K means method 逐步聚类法Kaplan-Meier 评估事件的时间长度Kaplan-Merier chart Kaplan-Merier图Kendall's rank correlation Kendall等级相关Kinetic 动力学Kolmogorov-Smirnove test 柯尔莫哥洛夫-斯米尔诺夫检验Kruskal and Wallis test Kruskal及Wallis检验/多样本的秩和检验/H检验Kurtosis 峰度Lack of fit 失拟Ladder of powers 幂阶梯Lag 滞后Large sample 大样本Large sample test 大样本检验—Latin square 拉丁方Latin square design 拉丁方设计Leakage 泄漏Least favorable configuration 最不利构形Least favorable distribution 最不利分布Least significant difference 最小显著差法Least square method 最小二乘法Least-absolute-residuals estimates 最小绝对残差估计Least-absolute-residuals fit 最小绝对残差拟合Least-absolute-residuals line 最小绝对残差线Legend 图例L-estimator L估计量L-estimator of location 位置L估计量L-estimator of scale 尺度L估计量Level 水平Life expectance 预期期望寿命Life table 寿命表Life table method 生命表法Light-tailed distribution 轻尾分布Likelihood function 似然函数Likelihood ratio 似然比line graph 线图Linear correlation 直线相关Linear equation 线性方程Linear programming 线性规划Linear regression 直线回归Linear Regression 线性回归Linear trend 线性趋势Loading 载荷Location and scale equivariance 位置尺度同变性Location equivariance 位置同变性Location invariance 位置不变性Location scale family 位置尺度族Log rank test 时序检验Logarithmic curve 对数曲线Logarithmic normal distribution 对数正态分布Logarithmic scale 对数尺度Logarithmic transformation 对数变换Logic check 逻辑检查Logistic distribution 逻辑斯特分布Logit transformation Logit转换LOGLINEAR 多维列联表通用模型Lognormal distribution 对数正态分布Lost function 损失函数—Low correlation 低度相关Lower limit 下限Lowest-attained variance 最小可达方差LSD 最小显著差法的简称Lurking variable 潜在变量Main effect 主效应Major heading 主辞标目Marginal density function 边缘密度函数Marginal probability 边缘概率Marginal probability distribution 边缘概率分布Matched data 配对资料Matched distribution 匹配过分布Matching of distribution 分布的匹配Matching of transformation 变换的匹配Mathematical expectation 数学期望Mathematical model 数学模型Maximum L-estimator 极大极小L 估计量Maximum likelihood method 最大似然法Mean 均数Mean squares between groups 组间均方Mean squares within group 组内均方Means (Compare means) 均值-均值比较Median 中位数Median effective dose 半数效量Median lethal dose 半数致死量Median polish 中位数平滑Median test 中位数检验Minimal sufficient statistic 最小充分统计量Minimum distance estimation 最小距离估计Minimum effective dose 最小有效量Minimum lethal dose 最小致死量Minimum variance estimator 最小方差估计量MINITAB 统计软件包Minor heading 宾词标目Missing data 缺失值Model specification 模型的确定Modeling Statistics 模型统计Models for outliers 离群值模型Modifying the model 模型的修正Modulus of continuity 连续性模Morbidity 发病率Most favorable configuration 最有利构形Multidimensional Scaling (ASCAL) 多维尺度/多维标度—Multinomial Logistic Regression 多项逻辑斯蒂回归Multiple comparison 多重比较Multiple correlation 复相关Multiple covariance 多元协方差Multiple linear regression 多元线性回归Multiple response 多重选项Multiple solutions 多解Multiplication theorem 乘法定理Multiresponse 多元响应Multi-stage sampling 多阶段抽样Multivariate T distribution 多元T分布Mutual exclusive 互不相容Mutual independence 互相独立Natural boundary 自然边界Natural dead 自然死亡Natural zero 自然零Negative correlation 负相关Negative linear correlation 负线性相关Negatively skewed 负偏Newman-Keuls method q检验NK method q检验No statistical significance 无统计意义Nominal variable 名义变量Nonconstancy of variability 变异的非定常性Nonlinear regression 非线性相关Nonparametric statistics 非参数统计Nonparametric test 非参数检验Nonparametric tests 非参数检验Normal deviate 正态离差Normal distribution 正态分布Normal equation 正规方程组Normal ranges 正常范围Normal value 正常值Nuisance parameter 多余参数/讨厌参数Null hypothesis 无效假设Numerical variable 数值变量Objective function 目标函数Observation unit 观察单位Observed value 观察值One sided test 单侧检验One-way analysis of variance 单因素方差分析Oneway ANOVA 单因素方差分析—Open sequential trial 开放型序贯设计Optrim 优切尾Optrim efficiency 优切尾效率Order statistics 顺序统计量Ordered categories 有序分类Ordinal logistic regression 序数逻辑斯蒂回归Ordinal variable 有序变量Orthogonal basis 正交基Orthogonal design 正交试验设计Orthogonality conditions 正交条件ORTHOPLAN 正交设计Outlier cutoffs 离群值截断点Outliers 极端值OVERALS 多组变量的非线性正规相关Overshoot 迭代过度Paired design 配对设计Paired sample 配对样本Pairwise slopes 成对斜率Parabola 抛物线Parallel tests 平行试验Parameter 参数Parametric statistics 参数统计Parametric test 参数检验Partial correlation 偏相关Partial regression 偏回归Partial sorting 偏排序Partials residuals 偏残差Pattern 模式Pearson curves 皮尔逊曲线Peeling 退层Percent bar graph 百分条形图Percentage 百分比Percentile 百分位数Percentile curves 百分位曲线Periodicity 周期性Permutation 排列P-estimator P估计量Pie graph 饼图Pitman estimator 皮特曼估计量Pivot 枢轴量Planar 平坦Planar assumption 平面的假设PLANCARDS 生成试验的计划卡—Point estimation 点估计Poisson distribution 泊松分布Polishing 平滑Polled standard deviation 合并标准差Polled variance 合并方差Polygon 多边图Polynomial 多项式Polynomial curve 多项式曲线Population 总体Population attributable risk 人群归因危险度Positive correlation 正相关Positively skewed 正偏Posterior distribution 后验分布Power of a test 检验效能Precision 精密度Predicted value 预测值Preliminary analysis 预备性分析Principal component analysis 主成分分析Prior distribution 先验分布Prior probability 先验概率Probabilistic model 概率模型probability 概率Probability density 概率密度Product moment 乘积矩/协方差Profile trace 截面迹图Proportion 比/构成比Proportion allocation in stratified random sampling 按比例分层随机抽样Proportionate 成比例Proportionate sub-class numbers 成比例次级组含量Prospective study 前瞻性调查Proximities 亲近性Pseudo F test 近似F检验Pseudo model 近似模型Pseudosigma 伪标准差Purposive sampling 有目的抽样QR decomposition QR分解Quadratic approximation 二次近似Qualitative classification 属性分类Qualitative method 定性方法Quantile-quantile plot 分位数-分位数图/Q-Q图Quantitative analysis 定量分析Quartile 四分位数Quick Cluster 快速聚类—Radix sort 基数排序Random allocation 随机化分组Random blocks design 随机区组设计Random event 随机事件Randomization 随机化Range 极差/全距Rank correlation 等级相关Rank sum test 秩和检验Rank test 秩检验Ranked data 等级资料Rate 比率Ratio 比例Raw data 原始资料Raw residual 原始残差Rayleigh's test 雷氏检验Rayleigh's Z 雷氏Z值Reciprocal 倒数Reciprocal transformation 倒数变换Recording 记录Redescending estimators 回降估计量Reducing dimensions 降维Re-expression 重新表达Reference set 标准组Region of acceptance 接受域Regression coefficient 回归系数Regression sum of square 回归平方和Rejection point 拒绝点Relative dispersion 相对离散度Relative number 相对数Reliability 可靠性Reparametrization 重新设置参数Replication 重复Report Summaries 报告摘要Residual sum of square 剩余平方和Resistance 耐抗性Resistant line 耐抗线Resistant technique 耐抗技术R-estimator of location 位置R估计量R-estimator of scale 尺度R估计量Retrospective study 回顾性调查Ridge trace 岭迹Ridit analysis Ridit分析Rotation 旋转—Rounding 舍入Row 行Row effects 行效应Row factor 行因素RXC table RXC表Sample 样本Sample regression coefficient 样本回归系数Sample size 样本量Sample standard deviation 样本标准差Sampling error 抽样误差SAS(Statistical analysis system ) SAS统计软件包Scale 尺度/量表Scatter diagram 散点图Schematic plot 示意图/简图Score test 计分检验Screening 筛检SEASON 季节分析Second derivative 二阶导数Second principal component 第二主成分SEM (Structural equation modeling) 结构化方程模型Semi-logarithmic graph 半对数图Semi-logarithmic paper 半对数格纸Sensitivity curve 敏感度曲线Sequential analysis 贯序分析Sequential data set 顺序数据集Sequential design 贯序设计Sequential method 贯序法Sequential test 贯序检验法Serial tests 系列试验Short-cut method 简捷法Sigmoid curve S形曲线Sign function 正负号函数Sign test 符号检验Signed rank 符号秩Significance test 显著性检验Significant figure 有效数字Simple cluster sampling 简单整群抽样Simple correlation 简单相关Simple random sampling 简单随机抽样Simple regression 简单回归simple table 简单表Sine estimator 正弦估计量Single-valued estimate 单值估计—Singular matrix 奇异矩阵Skewed distribution 偏斜分布Skewness 偏度Slash distribution 斜线分布Slope 斜率Smirnov test 斯米尔诺夫检验Source of variation 变异来源Spearman rank correlation 斯皮尔曼等级相关Specific factor 特殊因子Specific factor variance 特殊因子方差Spectra 频谱Spherical distribution 球型正态分布Spread 展布SPSS(Statistical package for the social science) SPSS统计软件包Spurious correlation 假性相关Square root transformation 平方根变换Stabilizing variance 稳定方差Standard deviation 标准差Standard error 标准误Standard error of difference 差别的标准误Standard error of estimate 标准估计误差Standard error of rate 率的标准误Standard normal distribution 标准正态分布Standardization 标准化Starting value 起始值Statistic 统计量Statistical control 统计控制Statistical graph 统计图Statistical inference 统计推断Statistical table 统计表Steepest descent 最速下降法Stem and leaf display 茎叶图Step factor 步长因子Stepwise regression 逐步回归Storage 存Strata 层(复数)Stratified sampling 分层抽样Stratified sampling 分层抽样Strength 强度Stringency 严密性Structural relationship 结构关系Studentized residual 学生化残差/t化残差Sub-class numbers 次级组含量Subdividing 分割—Sufficient statistic 充分统计量Sum of products 积和Sum of squares 离差平方和Sum of squares about regression 回归平方和Sum of squares between groups 组间平方和Sum of squares of partial regression 偏回归平方和Sure event 必然事件Survey 调查Survival 生存分析Survival rate 生存率Suspended root gram 悬吊根图Symmetry 对称Systematic error 系统误差Systematic sampling 系统抽样Tags 标签Tail area 尾部面积Tail length 尾长Tail weight 尾重Tangent line 切线Target distribution 目标分布Taylor series 泰勒级数Tendency of dispersion 离散趋势Testing of hypotheses 假设检验Theoretical frequency 理论频数Time series 时间序列Tolerance interval 容忍区间Tolerance lower limit 容忍下限Tolerance upper limit 容忍上限Torsion 扰率Total sum of square 总平方和Total variation 总变异Transformation 转换Treatment 处理Trend 趋势Trend of percentage 百分比趋势Trial 试验Trial and error method 试错法Tuning constant 细调常数Two sided test 双向检验Two-stage least squares 二阶最小平方Two-stage sampling 二阶段抽样Two-tailed test 双侧检验Two-way analysis of variance 双因素方差分析—Two-way table 双向表Type I error 一类错误/α错误Type II error 二类错误/β错误UMVU 方差一致最小无偏估计简称Unbiased estimate 无偏估计Unconstrained nonlinear regression 无约束非线性回归Unequal subclass number 不等次级组含量Ungrouped data 不分组资料Uniform coordinate 均匀坐标Uniform distribution 均匀分布Uniformly minimum variance unbiased estimate 方差一致最小无偏估计Unit 单元Unordered categories 无序分类Upper limit 上限Upward rank 升秩Vague concept 模糊概念Validity 有效性VARCOMP (Variance component estimation) 方差元素估计Variability 变异性Variable 变量Variance 方差Variation 变异Varimax orthogonal rotation 方差最大正交旋转Volume of distribution 容积W test W检验Weibull distribution 威布尔分布Weight 权数Weighted Chi-square test 加权卡方检验/Cochran检验Weighted linear regression method 加权直线回归Weighted mean 加权平均数Weighted mean square 加权平均方差Weighted sum of square 加权平方和Weighting coefficient 权重系数Weighting method 加权法W-estimation W估计量W-estimation of location 位置W估计量Width 宽度Wilcoxon paired test 威斯康星配对法/配对符号秩和检验Wild point 野点/狂点Wild value 野值/狂值Winsorized mean 缩尾均值—Withdraw 失访Youden's index 尤登指数Z test Z检验Zero correlation 零相关Z-transformation Z变换欢迎下载21。
- 1、下载文档前请自行甄别文档内容的完整性,平台不提供额外的编辑、内容补充、找答案等附加服务。
- 2、"仅部分预览"的文档,不可在线预览部分如存在完整性等问题,可反馈申请退款(可完整预览的文档不适用该条件!)。
- 3、如文档侵犯您的权益,请联系客服反馈,我们会尽快为您处理(人工客服工作时间:9:00-18:30)。
A multi-interval Chebyshev collocation method for efficienthigh-accuracy RF circuit simulationBaolin Y angCadence Design Systems,Inc.,SanJose,CA95134byang@Joel PhillipsCadence Berkeley Laboratories,SanJose,CA95134jrp@ABSTRACTMost RF circuit analysis tools use either shooting-Newton or har-monic balance methods.Neither can efficiently achieve high ac-curacy on strongly nonlinear circuits possessing waveforms with rapid transitions.We present a multi-interval-Chebyshev(MIC) method that discretizes the circuit equations by dividing the simu-lation domain into a set of intervals whose size is adaptively chosen and using Chebyshev polynomials to represent the solution in each interval.The MIC method has excellent stability properties,is as effective at solving nonlinear problems as shooting techniques,can achieve high resolution on a wide variety of circuits,and in con-junction with an appropriate preconditioner can be combined with matrix-implicit Krylov-subspace solvers to analyze large circuits with moderate computational cost.1.INTRODUCTIONThe exploding demand for high performance wireless products has increased the need for efficient and accurate simulation techniques for RF integrated circuits.RF circuit simulation is difficult because RF circuits typically contain signals with multiple-timescale prop-erties,as usually the data and carrier signals in a system are sepa-rated in frequency by several orders of magnitude.Special-purpose RF simulators exploit the sparsity of the spectrum in order to make the computations tractable[1].For example,instead of performing a long transient analysis of a circuit driven by a periodic source, we may seek tofind the steady-state directly.In this paper we consider the central problem of periodic-steady-state computation. The periodic-steady-state problem is of interest in its own right, but is also important to study because it forms the basis of more advanced analyses,such as cyclostationary noise analysis,multi-frequency distortion analysis,or envelope simulation.The method presented in this paper can be advantageously applied to all the typical RF simulation problems.Two numerical methods commonly used in steady-state computa-tion are the shooting-Newton method,based on low-orderfinite To appear at37th Design Automation Conference,June2000.Not to be redistributed.difference discretizations such as the second-order Gear method, and the harmonic balance method,based on high-order spectral discretizations[1,2].The advantage of the low-order polynomial-based methods is that they can select time-points based on localized error estimates and as a result can easily handle sharp transitions in circuit waveforms.The harmonic balance method,on the other hand,has the advantage of attaining spectral accuracy for smooth waveforms.Recent developments in matrix-free Krylov-subspace algorithms[5,6]have made these methods even more popular as they can be used to analyze circuits with thousands of devices. High precision computations are often necessary in RF circuit sim-ulation.For example,accurately computing the noisefigure of a highly nonlinear RF circuit often requires accurate determination of the periodic operating point up to many multiples of the funda-mental frequency,as noise may be translated from very high fre-quencies by the mixing action of the time-varying circuit elements to appear at the output.High accuracy is easy to achieve for smooth waveforms using spec-tral approximation in the harmonic balance method.However,non-linear circuits often produce waveforms that have sharp-transition regions.The waveforms may only be in the case of a pulse, which means that the waveforms are continuous but the deriva-tives of the waveforms are not continuous at some points.In this case the spectral accuracy of the harmonic balance method is lost and acceptable accuracy is obtained only at the cost of including very many harmonics and/or timepoints in the simulation.To help handle sharp transitions,Nastov and White introduced the time-mapped harmonic balance(TMHB)method[4].In the TMHB method,a time-map function is used to map the solution in the original time space to a space where the solution is more smooth. This method is an improvement over traditional harmonic balance, but it is still restricted,since,theoretically speaking,a point in the original time space is still a point in the mapped time space,and so one still cannot achieve spectral accuracy.Basically it can only put more points around the points to localize the oscillations inevitably associated with using a spectral method to approximate functions.And,as with all methods that utilize global basis functions,for reasons of numerical stability there are also strong restrictions on how rapidly the timestep can be changed locally.The low-order multi-step methods,such as the Gear methods,typ-ically used in the shooting method offer excellentflexibility in lo-cally adapting the timesteps.For low to moderate accuracy,these methods are preferred for problems that are highly nonlinear and/or contain sharp transitions.However,low-order multi-step methodsPermission to make digital/hardcopy of all or part of this work for personal or classroom use is granted without fee provided that copies are not made or distributed for profit or commercial advantage, the copyright notice, the title of the publication and its date appear, and notice is given that copying is by permission of ACM, Inc. To copy otherwise, to republish, to post on servers or to redistribute to lists, requires prior specific permission and/or a fee.DAC 2000, Los Angeles, California(c) 2000 ACM 1-58113-188-7/00/0006..$5.00are not efficient at high precision because veryfine discretizations are needed,resulting in loss of speed and increased memory re-quirements.In addition,the multistep discretizations used in theshooting method must be causal,and so have stability problems as-sociated with using one-side approximations,i.e.,performing diff-entiation using backward differences.It is generally accepted thatmultistep methods of order greater then three or four are not suf-ficiently stable,and in practice even the third order methods haverelatively stringent stability restrictions on how rapidly timestepsmay be varied.As a result,multi-step method of order higher than are not widely used in circuit simulation.Often,because of the backward-looking nature of the approximation,it is necessary todecrease the order after sharp-transition points or points,often tofirst order,resulting in veryfine grids in those regions.We seek a method that has none of the above disadvantages.Be-cause of inevitable discontinuities in the solution waveforms,resid-uals,or device equations,we perform the discretization by break-ing the simulation interval,of length,into timestep intervals of length,.Within each interval,Chebyshev poly-nomials are used to represent the solution waveform.Chebyshev polynomials have excellent approximation properties,in particular showing spectral convergence as the order is increased.We will show that this method has several desirable properties that lead to an excellent tradeoff between efficiency and accuracy,and further-more can be easily combined with matrix-implicit Krylov-subspace techniques to allow analysis of large circuits.In a sense we are able to exploit the best characteristics of the shooting-Newton and harmonic-balance methods,with the drawbacks of neither.2.CIRCUIT EQUATION DISCRETIZATION Circuit behavior is usually described by a set of nonlinear dif-ferential algebraic equations(DAEs)that can be written as(1)where represents contribution of the dynamic elements such as inductors and capacitors,is the vector representing static elements such as resistors,is the vector of inputs,and the vector contains state variables such as node voltages.The periodic steady-state solution is the solution of Eq.(1)that also satisfies the two-point boundary condition for some period.We now contrast three ways of discretizing the time-derivative op-erator:linear multistep methods,harmonic balance methods,and Chebyshev methods.As representative of linear multistep meth-ods we consider the Gear methods.Given the values,,at the time points:,to perform the discretization at ,we seek an interpolating polynomial of degree based on.The time derivative is approx-imated by evaluating the derivative of the interpolating polynomial at.Thus the time-derivative of the approximate solution is required to match the differential equation at the point.We call such a point a collocation point.The Gear methods are implicit in only“one-point-at-a-time”.They are called one-stage methods.At each timepoint,for a method of order,the solution vector at only points is involved,and if previous timepoints are known,a nonlinear system of size must be solved tofind the solution at the next timepoint.Each step of a shooting method involves the factorization of the circuit Jacobian matrix.Because this matrix is usually very sparse,it can be factored with fewfill-ins,in nearly time.The order of polynomial approximation is usuallyfixed at a low value,and increased accuracy is obtained by locally changing the timesteps based on estimates of the truncation errors induced by the order-polynomial approximation.Note that higher-order Gear methods can not be used at points after or sharp-transition points because approximation of the differential operator using an-order Gear method involves preceding points.Higher-order approximation across points or sharp-transition points leads to oscillation of the solution and makes the solution even less accurate than afirst-order solution.A similar problem occurs if the timestep ratios are changed too rapidly.For contrast,consider a particular(time-domain)construction of the harmonic balance method,known as Fourier-collocation.We seek to form the solution by an interpolating trigonometric polynomial of degree:(2)where denotes an-point interpolation operator.By comput-ing the derivative of the trigonometric polynomial(3)at the Fourier collocation points,, and enforcing the differential equation at these points we have spec-ified the numerical method.We may solve for the Fourier coeffi-cients directly,or express the Fourier coefficients in terms of the values at the Fourier collocation points as(4)The differentiation procedure may be performed efficiently to high order by noting that in the frequency domain,the differentiation op-erator becomes a multiplication operator,so by using the the FFT to convert the to,performing the differentiation,then in-verse transforming,we may accomplish the time-derivative oper-ation in operations.Alternatively,we can use this prescription to obtain a time-domain differentiation matrix such that approximates the value of at the collocation points, where vector is a vector of the values of at the collocation points.Then the derivatives can be obtained directly by a matrix-vector multiplication,requiring operations.Note that, in practice,using the FFT does not provide much advantage over using the matrix explicitly unless the order of approximationis fairly large.Accuracy in the harmonic balance method is usually achieved by increased the order of approximation.For a given set of colloca-tion points,the maximal possible order of approximation is used. With waveforms,this has the very desirable effect of achieving spectral convergence,meaning that as the spacing between the col-location points decreases,then asymptotically the error decreases faster than any polynomial approximation(in particular,faster than the Gear methods).However,note that though the expansion in Fourier series conveniently means automatically enforcing the pe-riodic boundary condition,it also means that the basis functions, sines and cosines,must cover the entire domain.Unlike the Gearmethods,which couple only local points,every point in the so-lution interval is coupled to every other through each basis function.The spectral convergence of the Fourier series is very desirable for smooth waveforms and nearly linear circuits,but un-fortunately,a single discontinuous point can destroy the spectral convergence properties.Because of the global nature of the basis functions,spectral convergence is very difficult to recover once in-terrupted.Most semiconductor device models possess some degree of discontinuity in the derivatives,and so true spectral convergence is rarely observed in practice.An alternative class of basis functions that also possess good ap-proximation properties,including the possibility of spectral conver-gence property,are the Chebyshev polynomials.However,Cheby-shev polynomials have the advantage that they can be applied when the simulation domain has been decomposed into multiple intervals in order to resolve complex or singular behavior,as has been done for electromagnetic wave problems in complex geometries[9]. Here we give the basics of the Chebyshev collocation method.Given the values,,at the Chebyshev Gauss-Lobatto collocation points,,we seek an interpolating polynomial of degree:(5) and compute the derivative of the polynomial as(6)The coefficients,,are computed from the coefficients by us-ing the backward recurrence relation[10](7)We can also obtain a derivative matrix by noting that the inter-polating polynomial can be expressed in terms of a Lagrange inter-polation polynomial.The entries of the Chebyshev differen-tiation matrix are computed by taking the analytical derivative of and evaluating it at the collocation points for,i.e.,.The entries of the matrix are(8)where when and otherwise.It is pointed out in[8]that calculating the matrix directly in this form results in unnecessarily large numerical error,and a formulation that yields more accuracy is given.The key to the multi-interval Chebyshev(MIC)approach is to break the simulation domain into multiple intervals,.In each interval the Cheby-shev collocation method is used to discretize the differential equa-tion.Continuity of the solution is enforced at the boundaries of any two intervals.For thefirst and last interval,this has the effect of enforcing the periodic boundary condition.The reason this de-composition is useful is that waveforms in circuit simulation typ-ically have regions of different properties.There are smooth re-gions where high-order methods can be used and there are sharp-transition regions where interval boundaries must be placed.Our particular combination of high-order Chebyshev discretizations in multiple time intervals provides several important advantages. First,as in harmonic balance,high accuracy is achievable because in each interval all the desirable approximation properties of Cheby-shev polynomials can be exploited.Chebyshev polynomials are usually close to the optimal polynomial approximant of a given order,and spectral convergence can be achieved if order-based(-type)refinement is used in each interval.Because of the rapid con-vergence,once a proper set of intervals is chosen high accuracy can be obtained at little marginal cost.Conversely,there will be few intervals in regions where the solution is smooth,because the intervals there can be quite large.Second,as in the lower-orderfinite difference schemes,the timestep intervals can be adaptively chosen to resolve nonlinear or abrupt transition behavior.The impact of nonlinear behavior can some-times be difficult to diagnose.Solution waveforms,such as volt-ages,may be quite smooth,but residuals,such as currents,will be much more difficult to represent with a Fourier series if the circuitequations are very nonlinear.In the MIC method,if the intervals are chosen such that discontinuities occur only at the interval bound-aries,then the discontinuities can be handled efficiently.Around a transition or strongly nonlinear region,more intervals can be placed in a denser timestep spacing,and possibly the order of the methodlowered.Third,the method has excellent numerical stability properties.It can be shown that our scheme is a member of a particular class of implicit Runge-Kutta(IRK)methods,the collocation-IRK meth-ods[3].Higher-order implicit Runge-Kutta methods are multi-stagemethods,that is,they are implicit in more than one point at a time. Because of the multiple implicit states,and unlike multi-step meth-ods such as the Gear methods,IRK methods can be constructed that are A-stable even at high order.By A-stable,we mean a scheme that is numerically stable for systems with poles located anywhere in the left-half-plane,including the entire imaginary axis.The par-ticular schemes we use in this paper are not A-stable,but we have been able to prove that the schemes of all orders used here are what is called-stable,meaning that if is a pole of the system be-ing integrated,then the numerical scheme is stable with timestep for all in the left-half-plane,except possibly within an an-gle of the imaginary axis.For our methods is usually less thanone degree,and the stability region only excludes a small semi-circle near the imaginary axis,which can be avoided by appropriate choice of timesteps.In addition,because we choose the Gauss-Lobatto-Chebyshev points for collocation,implying we always place a collocation point at the forward interval boundary,our particular construction gives methods that are L-or stiffly stable,just as the Gear methods are. Therefore the method is suitable for solving differential-algebraic equations as initial value problems.This property allows us to use shooting techniques to solve boundary value problems or construct preconditioners.In fact,in a certain sense that is important in practical applications,the MIC approach is more stable than the Gear methods.Since unlike the Gear methods the MIC scheme retains excellent stability properties even when the timesteps arerapidly varied,higher-order schemes can be used near sharp tran-sition points.Finally,the discretization scheme is robust.Unlike harmonic balance schemes,where sufficiently sharp transitions can induce oscillations in the global basis that are next to impossible to eliminate,in the worst possible case the discretization in a single MIC interval degenerates to the backward-Euler scheme which is robust and well-tested.In practice we have never found a need to drop below the second order scheme.3.EFFICIENT IMPLEMENTATIONFor simplicity of presentation,let us discuss Chebyshev-collocation methods for the case of two intervals:and .The number of collocation points in is and the numberof collocation points in is.The solution in is represented by and the solution in is represented by.The continuity condition is en-forced at the internal interval boundary,and the periodicity con-dition relates the two interval endpoints.Assume thatis the differentiation matrix in and is the differentiation matrix in ,Eq.(1)is discretized into the following equation:(9) where is the identity matrix.Newton’s method is used to solve this nonlinear equation.By defining the matrices(10) at each collocation point we can write the the Jacobian matrix for the Newton iteration asNote that and.At each Newton iteration step,we must solve a linear system(11) where is the residual of the previous solution.This step is prob-lematic because in each interval the discretization of the differentia-tion operator is global.That is,the approximation of at every collocation point involves at all the collocation points. Thus the differentiation matrix,like the Fourier collocation differ-entiation matrix,is a full matrix(on each interval).The resulting larger,less-sparse equations systems lead to difficulties in large-scale circuit simulation and is the reason IRK methods are notwidely used to date.Ironically,it is the sheer scale of RF simu-lation problems that makes IRK methods more suitable in the RFcontext.Matrix-implicit Krylov subspace methods,such as GM-RES or QMR,are now commonly used to simultaneously solvethe linear system of equations(Eq.11)resulting from discretiza-tion of the circuit equations over a fundamental period.We nowshow that it is possible to easily construct a preconditioner that ismore effective than those typically used in harmonic balance andcomparable to the preconditioners used for shooting methods,thusassuring rapid convergence of the iterative solvers.For example,ona similar test(the DC-DC converter),with a comparable approach to preconditioner construction,our method requires two orders ofmagnitude fewer GMRES iterations than reported in[4].In each interval we use a“local”preconditioning approach thatis motivated by techniques used for large-scale harmonic balanceanalysis.But because we can decompose into multiple in-tervals,we are able to devise a preconditioner that is much moreefficient than the corresponding harmonic balance preconditioner.In this way,our“global”preconditioning approach is similar tothat used in[5]for shooting methods,where a preconditioner isobtained forfinite difference equations by dropping the top-rightcorner entries in the left-hand-side matrix of the linear system.In harmonic balance,it is common to construct a preconditioner by assuming the capacitance and conductance matrices respec-tively can be well represented by(piecewise)-constant approxima-tions.We adopt both these techniques,for example using a single as an approximation of all the capacitance matrices in interval and as an approximation of all the capacitance matrices in interval,the conductance matrices being treated similarly. When this is done,note that the two large blocks of the precondi-tioning matrix corresponding to the two intervals are only coupled by the subdiagonal block.Hence it suffices to discuss how to in-vert the top diagonal block.To accomplish this,consider forming the Schur decomposition of the relevant portion of the Chebyshev differentiation matrix such that(12) where is a Schur matrix and is a unitary matrix.has2-by-2blocks on the diagonal and all other subdiagonal entries are zeros.By applying this decomposition to the top block of,we can reduce it to an almost upper block-triangular structure with2N-by-2N blocks on the diagonal.These2N-by-2N blocks on the diagonal can be further transformed into a N-by-N complex matrix that has the same sparsity structure as a single-timepoint circuit Jacobian as generated in a transient analysis.It can be factored efficiently,with fewfill-ins,just as the Jacobian matrix in a low-order method. The advantage of this preconditioner over harmonic balance pre-conditioners is that we can approximate the capacitance and con-ductance matrices with averages separately in each interval at lit-tle computational cost,in contrast to using global averages which make the preconditioner very inefficient in simulations of nonlinear circuits.Because the intervals are chosen to resolve the nonlinear behavior,we can expect the cost of applying the preconditioner to be relatively independent of the degree of nonlinearity of the cir-cuit.If the circuit is very nonlinear,there will be very many in-tervals,yet precisely for this reason the preconditioner will still be a good approximation to the original matrix,and in fact will be nearly as cheap to apply as the shooting-method preconditioner.In a practical implementation it is not advantageous to attempt to solve the MIC equations directly.Instead,we use a low-order shooting-Newton method to obtain an initial approximation to the solution waveform.For steady-state problems,the shooting-Newton method has a good global convergence rate in PSS simulations and can rapidly reach the neighborhood of the steady state.From the result of the low-order solution method,we decompose the time interval into a number of subintervals.The objec-tive of thisfirst decomposition is to avoid sharp transitions and points in each interval.Next we determine the order of accuracy desired in each interval.At this point,if a interval contains many time points of the low-order shooting method,the solution in this interval must be relatively smooth and we will choose a relatively high order in this interval.If a interval contains only a few time points,it means that we are in a sharp-transition region and we can choose low-order IRK methods in the interval.Once we have dis-cretized the circuit equations in all the intervals and the boundaries are connected with continuity conditions and the periodicity condi-tions then we can proceed with the Newton iteration.Once an initial solution is obtained,we use an adaptive refinement scheme to split the intervals in any additional sharp-transition re-gions that may appear and increase the order of approximation in other regions where accuracy is not satisfactory.In this stage, we use both h-type(interval decomposition)and p-type(order in-crease)refinement to achieve the required accuracy in the most ef-ficient way.Error control can be performed by examining the trailing Cheby-shev coefficients as indicators of potential numerical truncation er-ror.Our initial experiments have been conducted using the follow-ing simple error criteria,(13)where is the order of the Chebyshev method used in interval, and are the th and th Chebyshev expansion coefficients,normalized by the local maximum in all intervals,is the length of the interval,is the time period,and is the desired level of accuracy.This takes into account both h-type refinement, ,and the p-type refinement,,which is proven to be effective in numerical experiments.If the error criteria is not satisfied in a given interval,then we check the absolute value of and.If,we increase the order of the method in the interval,as this indicates that the order of Chebyshev coefficients is decreasing,so it is reasonable to seek spectral convergence.Otherwise,the solution is likely non-smooth, and we decompose the interval into two new intervals at the middle point.4.NUMERICAL RESULTSIn this Section we show the spectral accuracy of the multidomain Chebyshev collocation method in periodic steady state computa-tions.Efficiency of using h-type(domain decomposition)and p-type(polynomial order increase)refinement in resolving waveforms with sharp transitions is shown.Our method’s ability to resolve sharp transitions with high accuracy is verified.We also demon-strate the efficiency of the preconditioning technique in the solu-tion of the linear system using the matrix-free Krylov-subspace ap-proach.In thefirst example,we show the spectral accuracy of the multido-Figure1:Spectral accuracy of multidomain Chebyshev collo-Figure2:Comparison of h-type,p-type,and hp-type refine-main Chebyshev collocation methods by computing the periodicsteady-state solution of a crystalfilter.It can be seen in Fig.1that our method can achieve machine precision using only time points(we use intervals and16th order in each interval).Spectralconvergence is evident in the exponential decay of the error.In the second example,we show the efficiency of using both h-typeand p-type refinement in resolving solutions with sharp transitions.We introduce nonlinearity into the previousfilter example by plac-ing mixer before it.We compare three refinement strategies:h-type refinement only,p-type refinement only,and hp-type(h-typeand p-type)refinement.In Fig.2,one can notice that both p-typerefinement and hp-type refinement can achieve spectral accuracy.In the hp-type refinement,we use Chebyshev polynomials with or-der varying from to in the intervals and we achieve the same level of accuracy that is achieved using p-type refinement wherethe order as high as in used in two intervals.Note that for thesame total number of time steps,the hp-type refinement is moreefficient.We can clearly see from Fig.2that both hp-type refine-ment and p-type refinement is superior to h-type refinement of a second order method.The advantages of using high-order methods are clear from this example.In the last example,we calculate the periodic steady-state of a verynonlinear DC-DC converter similar to a circuit analyzed in[4].Thefinal mesh of the proposed approach is obtained through three steps of hp-refinement.By using both h-type and p-type refinement,the。