2008数学建模国家一等奖论文(神经网络)
2008B大学学费问题2008数学建模国家一等奖论文

C = Ri [G *Q *(1− b)] + (1.0502x1 −1.1959x2 −1.3108x3 −1.3636x4 − 0.7929x5) * Ri * b ;
由此便可得到综合学费标准 C 的取值范围。然后,我们随机选取了同一区域不 同专业,并根据表达式计算这些专业的学费,结果发现对社会收益大,个人收益 小的专业如地质学的学费范围为:3469.8~3506.3 元之间;对社会收益小,个人 收益大的专业如广告设计的学费范围为:7931.0~8014.5 元之间。与通常高校实 现的一刀切政策有了明显的优点。
获得这两个基本原则,我们从影响普通高校学费能力的众多因素中选取全国
人均 GDP 和学生就读的专业这两个基本因素进行研究,通过建立相关模型确
定这两个因素的权重系数,
2、模型的准备
根据查询国家统计局显示的数据资料[7],我们搜集到从 1995 年到 2004 年间
我国普通高校生均学费和人均 GDP 的值如表 5. 1 所示:
从中国的国情出发,收集诸如近几年来关于我国教育经费方面的及家庭收入 等数据[1-4],并通过分析数据建立数学模型,就几类学校或专业的费用标准进行 定量分析,并从中得出明确、有说服力的结论。
二、 问题分析
(一)我国教育收费的现状
通过国家统计局相关资料检索得到 2000 年到 2005 年我国普通高等学校教育
151828
172775
278253
256375
215440
210796
学费和杂 费 1926109 2824417 3906526 5057307 6476921 7919249
其他教育经费 1677772 2158574 2840985 3220992 3785362 4662641
NOI国家集训队论文分类(至2008)(摘抄自C博客)
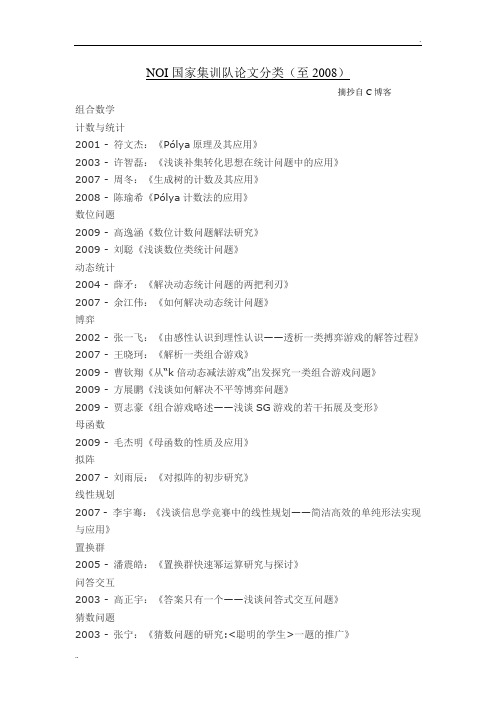
摘抄自C博客组合数学计数与统计2001 - 符文杰:《Pólya原理及其应用》2003 - 许智磊:《浅谈补集转化思想在统计问题中的应用》2007 - 周冬:《生成树的计数及其应用》2008 - 陈瑜希《Pólya计数法的应用》数位问题2009 - 高逸涵《数位计数问题解法研究》2009 - 刘聪《浅谈数位类统计问题》动态统计2004 - 薛矛:《解决动态统计问题的两把利刃》2007 - 余江伟:《如何解决动态统计问题》博弈2002 - 张一飞:《由感性认识到理性认识——透析一类搏弈游戏的解答过程》2007 - 王晓珂:《解析一类组合游戏》2009 - 曹钦翔《从“k倍动态减法游戏”出发探究一类组合游戏问题》2009 - 方展鹏《浅谈如何解决不平等博弈问题》2009 - 贾志豪《组合游戏略述——浅谈SG游戏的若干拓展及变形》母函数2009 - 毛杰明《母函数的性质及应用》拟阵2007 - 刘雨辰:《对拟阵的初步研究》线性规划2007 - 李宇骞:《浅谈信息学竞赛中的线性规划——简洁高效的单纯形法实现与应用》置换群2005 - 潘震皓:《置换群快速幂运算研究与探讨》问答交互2003 - 高正宇:《答案只有一个——浅谈问答式交互问题》猜数问题2003 - 张宁:《猜数问题的研究:<聪明的学生>一题的推广》2006 - 龙凡:《一类猜数问题的研究》数据结构数据结构2005 - 何林:《数据关系的简化》2006 - 朱晨光:《基本数据结构在信息学竞赛中的应用》2007 - 何森:《浅谈数据的合理组织》2008 - 曹钦翔《数据结构的提炼与压缩》结构联合2001 - 高寒蕊:《从圆桌问题谈数据结构的综合运用》2005 - 黄刚:《数据结构的联合》块状链表2005 - 蒋炎岩:《数据结构的联合——块状链表》2008 - 苏煜《对块状链表的一点研究》动态树2006 - 陈首元:《维护森林连通性——动态树》2007 - 袁昕颢:《动态树及其应用》左偏树2005 - 黄源河:《左偏树的特点及其应用》跳表2005 - 魏冉:《让算法的效率“跳起来”!——浅谈“跳跃表”的相关操作及其应用》2009 - 李骥扬《线段跳表——跳表的一个拓展》SBT2007 - 陈启峰:《Size Balance Tree》线段树2004 - 林涛:《线段树的应用》单调队列2006 - 汤泽:《浅析队列在一类单调性问题中的应用》哈希表2005 - 李羽修:《Hash函数的设计优化》2007 - 杨弋:《Hash在信息学竞赛中的一类应用》Splay2004 - 杨思雨:《伸展树的基本操作与应用》图论图论2005 - 任恺:《图论的基本思想及方法》模型建立2004 - 黄源河:《浅谈图论模型的建立与应用》2004 - 肖天:《“分层图思想”及其在信息学竞赛中的应用》网络流2001 - 江鹏:《从一道题目的解法试谈网络流的构造与算法》2002 - 金恺:《浅谈网络流算法的应用》2007 - 胡伯涛:《最小割模型在信息学竞赛中的应用》2007 - 王欣上:《浅谈基于分层思想的网络流算法》2008 - 周冬《两极相通——浅析最大—最小定理在信息学竞赛中的应用》最短路2006 - 余远铭:《最短路算法及其应用》2008 - 吕子鉷《浅谈最短径路问题中的分层思想》2009 - 姜碧野《SPFA算法的优化及应用》欧拉路2007 - 仇荣琦:《欧拉回路性质与应用探究》差分约束系统2006 - 冯威:《数与图的完美结合——浅析差分约束系统》平面图2003 - 刘才良:《平面图在信息学中的应用》2007 - 古楠:《平面嵌入》2-SAT2003 - 伍昱:《由对称性解2-SAT问题》最小生成树2004 - 吴景岳:《最小生成树算法及其应用》2004 - 汪汀:《最小生成树问题的拓展》二分图2005 - 王俊:《浅析二分图匹配在信息学竞赛中的应用》Voronoi图2006 - 王栋:《浅析平面Voronoi图的构造及应用》偶图2002 - 孙方成:《偶图的算法及应用》树树2002 - 周文超:《树结构在程序设计中的运用》2005 - 栗师:《树的乐园——一些与树有关的题目》路径问题2009 - 漆子超《分治算法在树的路径问题中的应用》最近公共祖先2007 - 郭华阳:《RMQ与LCA问题》划分问题2004 - 贝小辉:《浅析树的划分问题》数论欧几里得算法2009 - 金斌《欧几里得算法的应用》同余方程2003 - 姜尚仆:《模线性方程的应用——用数论方法解决整数问题》搜索搜索2001 - 骆骥:《由“汽车问题”浅谈深度搜索的一个方面——搜索对象与策略的重要性》2002 - 王知昆:《搜索顺序的选择》2005 - 汪汀:《参数搜索的应用》启发式2009 - 周而进《浅谈估价函数在信息学竞赛中的应用》优化2003 - 金恺:《探寻深度优先搜索中的优化技巧——从正方形剖分问题谈起》2003 - 刘一鸣:《一类搜索的优化思想——数据有序化》2006 - 黄晓愉:《深度优先搜索问题的优化技巧》背包问题2009 - 徐持衡《浅谈几类背包题》匹配2004 - 楼天城:《匹配算法在搜索问题中的巧用》概率概率2009 - 梅诗珂《信息学竞赛中概率问题求解初探》数学期望2009 - 汤可因《浅析竞赛中一类数学期望问题的解决方法》字符串字符串2003 - 周源:《浅析“最小表示法”思想在字符串循环同构问题中的应用》多串匹配2004 - 朱泽园:《多串匹配算法及其启示》2006 - 王赟:《Trie图的构建、活用与改进》2009 - 董华星《浅析字母树在信息学竞赛中的应用》后缀数组2004 - 许智磊:《后缀数组》2009 - 罗穗骞《后缀数组——处理字符串的有力工具》字符串匹配2003 - 饶向荣:《病毒的DNA———剖析一道字符匹配问题解析过程》2003 - 林希德:《求最大重复子串》动态规划动态规划2001 - 俞玮:《基本动态规划问题的扩展》2006 - 黄劲松:《贪婪的动态规划》2009 - 徐源盛《对一类动态规划问题的研究》状态压缩2008 - 陈丹琦《基于连通性状态压缩的动态规划问题》状态设计2008 - 刘弈《浅谈信息学中状态的合理设计与应用》树形DP2007 - 陈瑜希:《多角度思考创造性思维——运用树型动态规划解题的思路和方法探析》优化2001 - 毛子青:《动态规划算法的优化技巧》2003 - 项荣璟:《充分利用问题性质——例析动态规划的“个性化”优化》2004 - 朱晨光:《优化,再优化!——从《鹰蛋》一题浅析对动态规划算法的优化》2007 - 杨哲:《凸完全单调性的加强与应用》计算几何立体几何2003 - 陆可昱:《长方体体积并》2008 - 高亦陶《从立体几何问题看降低编程复杂度》计算几何思想2004 - 金恺:《极限法——解决几何最优化问题的捷径》2008 - 程芃祺《计算几何中的二分思想》2008 - 顾研《浅谈随机化思想在几何问题中的应用》圆2007 - 高逸涵:《与圆有关的离散化》半平面交2002 - 李澎煦:《半平面交的算法及其应用》2006 - 朱泽园:《半平面交的新算法及其实用价值》矩阵矩阵2008 - 俞华程《矩阵乘法在信息学中的应用》高斯消元2002 - 何江舟:《用高斯消元法解线性方程组》数学方法数学思想2002 - 何林:《猜想及其应用》2003 - 邵烜程:《数学思想助你一臂之力》数学归纳法2009 - 张昆玮《数学归纳法与解题之道》多项式2002 - 张家琳:《多项式乘法》数形结合2004 - 周源:《浅谈数形结合思想在信息学竞赛中的应用》黄金分割2005 - 杨思雨:《美,无处不在——浅谈“黄金分割”和信息学的联系》其他算法遗传算法2002 - 张宁:《遗传算法的特点及其应用》2005 - 钱自强:《关于遗传算法应用的分析与研究》信息论2003 - 侯启明:《信息论在信息学竞赛中的简单应用》染色与构造2002 - 杨旻旻:《构造法——解题的最短路径》2003 - 方奇:《染色法和构造法在棋盘上的应用》一类问题区间2008 - 周小博《浅谈信息学竞赛中的区间问题》序2005 - 龙凡:《序的应用》系2006 - 汪晔:《信息学中的参考系与坐标系》物理问题2008 - 方戈《浅析信息学竞赛中一类与物理有关的问题》编码与译码2008 - 周梦宇《码之道—浅谈信息学竞赛中的编码与译码问题》对策问题2002 - 骆骥:《浅析解“对策问题”的两种思路》优化算法优化2002 - 孙林春:《让我们做得更好——从解法谈程序优化》2004 - 胡伟栋:《减少冗余与算法优化》2005 - 杨弋:《从<小H的小屋>的解法谈算法的优化》2006 - 贾由:《由图论算法浅析算法优化》程序优化2006 - 周以苏:《论反汇编在时间常数优化中的应用》2009 - 骆可强《论程序底层优化的一些方法与技巧》语言C++2004 - 韩文弢:《论C++语言在信息学竞赛中的应用》策略策略2004 - 李锐喆:《细节——不可忽视的要素》2005 - 朱泽园:《回到起点——一种突破性思维》2006 - 陈启峰:《“约制、放宽”方法在解题中的应用》2006 - 李天翼:《从特殊情况考虑》2007 - 陈雪:《问题中的变与不变》2008 - 肖汉骏《例谈信息学竞赛分析中的“深”与“广”》倍增2005 - 朱晨光:《浅析倍增思想在信息学竞赛中的应用》二分2002 - 李睿:《二分法与统计问题》2002 - 许智磊:《二分,再二分!——从Mobiles(IOI2001)一题看多重二分》2005 - 杨俊:《二分策略在信息学竞赛中的应用》调整2006 - 唐文斌:《“调整”思想在信息学中的应用》随机化2007 - 刘家骅:《浅谈随机化在信息学竞赛中的应用》非完美算法2005 - 胡伟栋:《浅析非完美算法在信息学竞赛中的应用》2008 - 任一恒《非完美算法初探》提交答案题2003 - 雷环中:《结果提交类问题》守恒思想2004 - 何林:《信息学中守恒法的应用》极限法2003 - 王知昆:《浅谈用极大化思想解决最大子矩形问题》贪心2008 - 高逸涵《部分贪心思想在信息学竞赛中的应用》压缩法2005 - 周源:《压去冗余缩得精华——浅谈信息学竞赛中的“压缩法”》逆向思维2005 - 唐文斌:《正难则反——浅谈逆向思维在解题中的应用》穷举2004 - 鬲融:《浅谈特殊穷举思想的应用》目标转换2002 - 戴德承:《退一步海阔天空——“目标转化思想”的若干应用》2004 - 栗师:《转化目标在解题中的应用》类比2006 - 周戈林:《浅谈类比思想》分割与合并2006 - 俞鑫:《棋盘中的棋盘——浅谈棋盘的分割思想》2007 - 杨沐:《浅析信息学中的“分”与“合”》平衡思想2008 - 郑暾《平衡规划——浅析一类平衡思想的应用》。
全国大学生数学建模大赛国家一等奖论文A题
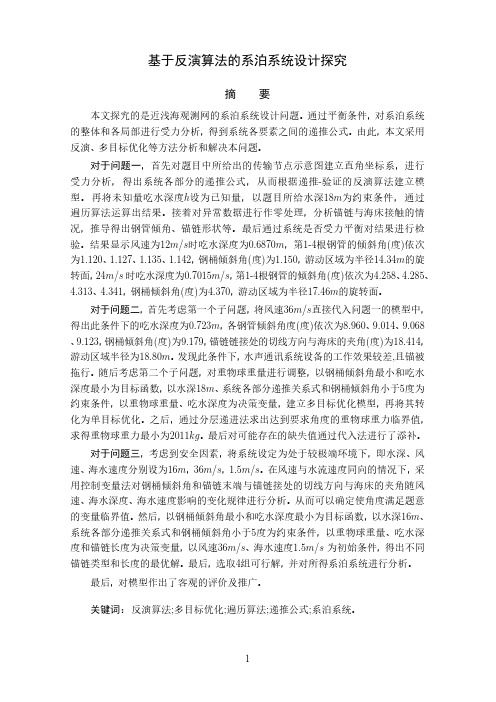
=
− − ( − 1)′
, = 1, 2, · · ·, 210
当逐渐增大,锚链受到的竖直向下方向的合力与支持力之差先逐渐接近于0,
再等于0,直至小于0。当合力小于0时,锚链以海床接触,此时海床提供向上的支持
力,其大小与′ 相等。因此可将小于0 的值都作零处理,故锚链接触海床时,
对于问题二,首先考虑第一个子问题,将风速36/直接代入问题一的模型中,
得出此条件下的吃水深度为0.723,各钢管倾斜角度(度)依次为8.960、9.014、9.068
、9.123,钢桶倾斜角(度)为9.179,锚链链接处的切线方向与海床的夹角(度)为18.414,
游动区域半径为18.80。发现此条件下,水声通讯系统设备的工作效果较差,且锚被
计与应用对海上科学发展有重要意义。
1.2 问题的提出
已知某近浅海传输节点(如图1所示),将浮标视作底面直径2为、高为2、质量
为1000的圆柱体,锚的质量为600,钢管共4节,每节长度为1,直径为50,
每节钢管的质量为10。水声通讯系统安装在一个长为1、外径为30的密封圆
柱形钢桶内,设备和钢桶总质量为100。
Step1: 遍历求解
令吃水深度ℎ的初始值为0.1,以0.0005为单位逐步增加至2。( 浮标高度为2,
完全浸没时吃水深度ℎ则为2 ),记录对应的数据,选取水下物体竖直方向高度和
与海域水深最接近的组别,进一步进行计算,结果如下表所示(具体程序见附录):
表 1: 不同风速的相关结果表
以风速24/的情况为例,绘制游动区域图:
题意的变量临界值。以水深16、系统各部分递推关系式和钢桶与竖直方向夹角小
于5°为约束条件,将多目标优化转化为单目标优化。通过调节决策变量中锚链的型
优秀的数学建模论文范文(通用8篇)
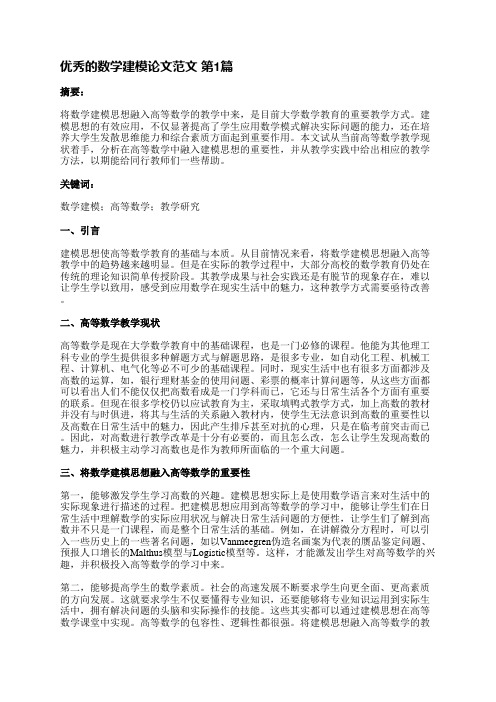
优秀的数学建模论文范文第1篇摘要:将数学建模思想融入高等数学的教学中来,是目前大学数学教育的重要教学方式。
建模思想的有效应用,不仅显著提高了学生应用数学模式解决实际问题的能力,还在培养大学生发散思维能力和综合素质方面起到重要作用。
本文试从当前高等数学教学现状着手,分析在高等数学中融入建模思想的重要性,并从教学实践中给出相应的教学方法,以期能给同行教师们一些帮助。
关键词:数学建模;高等数学;教学研究一、引言建模思想使高等数学教育的基础与本质。
从目前情况来看,将数学建模思想融入高等教学中的趋势越来越明显。
但是在实际的教学过程中,大部分高校的数学教育仍处在传统的理论知识简单传授阶段。
其教学成果与社会实践还是有脱节的现象存在,难以让学生学以致用,感受到应用数学在现实生活中的魅力,这种教学方式需要亟待改善。
二、高等数学教学现状高等数学是现在大学数学教育中的基础课程,也是一门必修的课程。
他能为其他理工科专业的学生提供很多种解题方式与解题思路,是很多专业,如自动化工程、机械工程、计算机、电气化等必不可少的基础课程。
同时,现实生活中也有很多方面都涉及高数的运算,如,银行理财基金的使用问题、彩票的概率计算问题等,从这些方面都可以看出人们不能仅仅把高数看成是一门学科而已,它还与日常生活各个方面有重要的联系。
但现在很多学校仍以应试教育为主,采取填鸭式教学方式,加上高数的教材并没有与时俱进,将其与生活的关系融入教材内,使学生无法意识到高数的重要性以及高数在日常生活中的魅力,因此产生排斥甚至对抗的心理,只是在临考前突击而已。
因此,对高数进行教学改革是十分有必要的,而且怎么改,怎么让学生发现高数的魅力,并积极主动学习高数也是作为教师所面临的一个重大问题。
三、将数学建模思想融入高等数学的重要性第一,能够激发学生学习高数的兴趣。
建模思想实际上是使用数学语言来对生活中的实际现象进行描述的过程。
把建模思想应用到高等数学的学习中,能够让学生们在日常生活中理解数学的实际应用状况与解决日常生活问题的方便性,让学生们了解到高数并不只是一门课程,而是整个日常生活的基础。
数学建模全国一等奖论文系列(27)
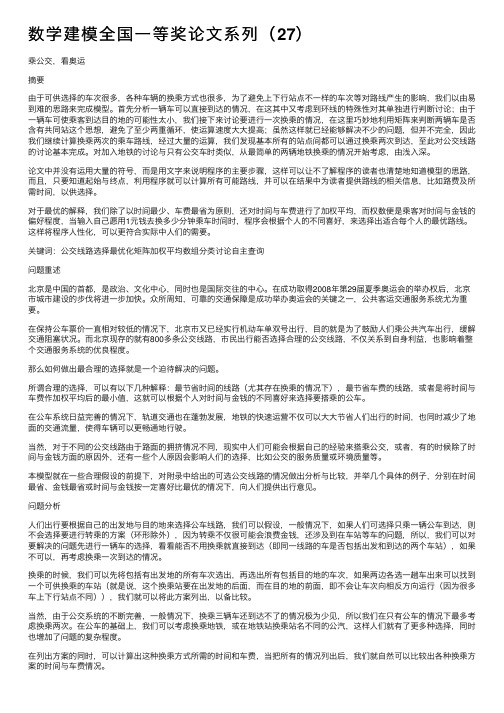
数学建模全国⼀等奖论⽂系列(27)乘公交,看奥运摘要由于可供选择的车次很多,各种车辆的换乘⽅式也很多,为了避免上下⾏站点不⼀样的车次等对路线产⽣的影响,我们以由易到难的思路来完成模型。
⾸先分析⼀辆车可以直接到达的情况,在这其中⼜考虑到环线的特殊性对其单独进⾏判断讨论;由于⼀辆车可使乘客到达⽬的地的可能性太⼩,我们接下来讨论要进⾏⼀次换乘的情况,在这⾥巧妙地利⽤矩阵来判断两辆车是否含有共同站这个思想,避免了⾄少两重循环,使运算速度⼤⼤提⾼;虽然这样就已经能够解决不少的问题,但并不完全,因此我们继续计算换乘两次的乘车路线,经过⼤量的运算,我们发现基本所有的站点间都可以通过换乘两次到达,⾄此对公交线路的讨论基本完成。
对加⼊地铁的讨论与只有公交车时类似,从最简单的两辆地铁换乘的情况开始考虑,由浅⼊深。
论⽂中并没有运⽤⼤量的符号,⽽是⽤⽂字来说明程序的主要步骤,这样可以让不了解程序的读者也清楚地知道模型的思路,⽽且,只要知道起始与终点,利⽤程序就可以计算所有可能路线,并可以在结果中为读者提供路线的相关信息,⽐如路费及所需时间,以供选择。
对于最优的解释,我们除了以时间最少、车费最省为原则,还对时间与车费进⾏了加权平均,⽽权数便是乘客对时间与⾦钱的偏好程度,当输⼊⾃⼰愿⽤1元钱去换多少分钟乘车时间时,程序会根据个⼈的不同喜好,来选择出适合每个⼈的最优路线。
这样将程序⼈性化,可以更符合实际中⼈们的需要。
关键词:公交线路选择最优化矩阵加权平均数组分类讨论⾃主查询问题重述北京是中国的⾸都,是政治、⽂化中⼼,同时也是国际交往的中⼼。
在成功取得2008年第29届夏季奥运会的举办权后,北京市城市建设的步伐将进⼀步加快。
众所周知,可靠的交通保障是成功举办奥运会的关键之⼀,公共客运交通服务系统尤为重要。
在保持公车票价⼀直相对较低的情况下,北京市⼜已经实⾏机动车单双号出⾏,⽬的就是为了⿎励⼈们乘公共汽车出⾏,缓解交通阻塞状况。
数学建模国家一等奖 论文
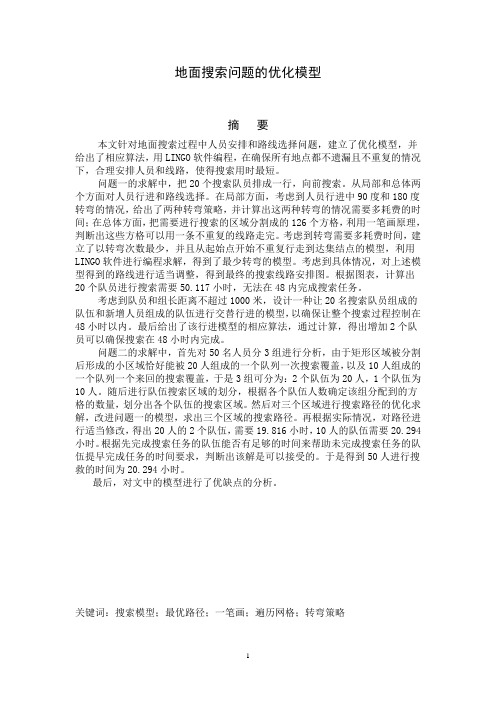
地面搜索问题的优化模型摘要本文针对地面搜索过程中人员安排和路线选择问题,建立了优化模型,并给出了相应算法,用LINGO软件编程,在确保所有地点都不遗漏且不重复的情况下,合理安排人员和线路,使得搜索用时最短。
问题一的求解中,把20个搜索队员排成一行,向前搜索。
从局部和总体两个方面对人员行进和路线选择。
在局部方面,考虑到人员行进中90度和180度转弯的情况,给出了两种转弯策略,并计算出这两种转弯的情况需要多耗费的时间;在总体方面,把需要进行搜索的区域分割成的126个方格,利用一笔画原理,判断出这些方格可以用一条不重复的线路走完。
考虑到转弯需要多耗费时间,建立了以转弯次数最少,并且从起始点开始不重复行走到达集结点的模型,利用LINGO软件进行编程求解,得到了最少转弯的模型。
考虑到具体情况,对上述模型得到的路线进行适当调整,得到最终的搜索线路安排图。
根据图表,计算出20个队员进行搜索需要50.117小时,无法在48内完成搜索任务。
考虑到队员和组长距离不超过1000米,设计一种让20名搜索队员组成的队伍和新增人员组成的队伍进行交替行进的模型,以确保让整个搜索过程控制在48小时以内。
最后给出了该行进模型的相应算法,通过计算,得出增加2个队员可以确保搜索在48小时内完成。
问题二的求解中,首先对50名人员分3组进行分析,由于矩形区域被分割后形成的小区域恰好能被20人组成的一个队列一次搜索覆盖,以及10人组成的一个队列一个来回的搜索覆盖,于是3组可分为:2个队伍为20人,1个队伍为10人。
随后进行队伍搜索区域的划分,根据各个队伍人数确定该组分配到的方格的数量,划分出各个队伍的搜索区域。
然后对三个区域进行搜索路径的优化求解,改进问题一的模型,求出三个区域的搜索路径。
再根据实际情况,对路径进行适当修改,得出20人的2个队伍,需要19.816小时,10人的队伍需要20.294小时。
根据先完成搜索任务的队伍能否有足够的时间来帮助未完成搜索任务的队伍提早完成任务的时间要求,判断出该解是可以接受的。
河南省2008年全国大学生数学建模竞赛获奖名单(本科组)
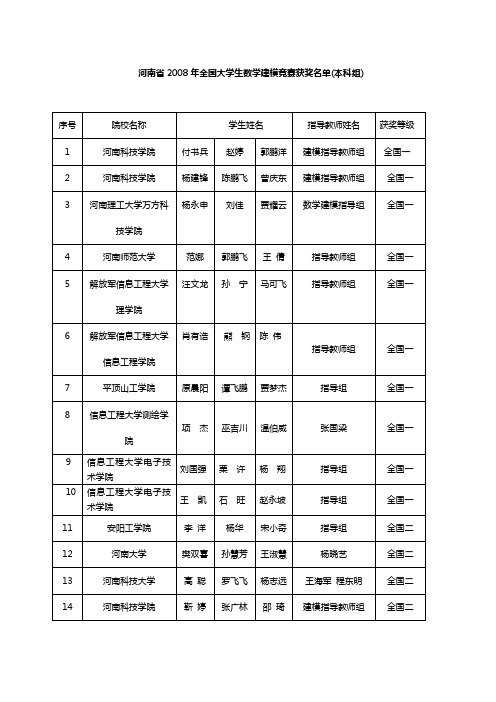
4 5
河南师范大学 解放军信息工程大学 理学院
范娜 汪文龙
郭鹏飞 孙 宁
王 倩 马可飞
指导教师组 指导教师组
全国一 全国一
6
解放军信息工程大学 信息工程学院
肖有诰
熊 钢
陈 伟 指导教师组 全国一
7 8
平顶山工学院 信息工程大学测绘学
原晨阳
谭飞鹏
贾梦杰
指导组
全国一
项 杰 院 9 10 信息工程大学电子技 术学院 信息工程大学电子技 术学院 11 12 13 14 安阳工学院 河南大学 河南科技大学 河南科技学院 刘国强 王 凯
指导教师组
省一等奖
125
解放军信息工程大学 理学院
彭 邵
杨 浩
张 敏
指导教师组
省一等奖
126
解放军信息工程大学 信息工程学院
陈 新
刘晓鹤
何颖 指导教师组 颖 省一等奖
127Βιβλιοθήκη 解放军信息工程大学 信息工程学院
薛凌鸿
刘俞伯
建珍 指导教师组 珍 省一等奖
128
解放军信息工程大学 信息工程学院
张 威
薛 珂
刘长 指导教师组 猛 省一等奖
92
河南理工大学万方科 技学院
袁英
樊马迪
杨文韬
数学建模指导组
省一等奖
93 94 95 96
河南农业大学 河南农业大学 河南农业大学 河南师范大学
杨文娟 李春慧 张海洋 朱颖苗
邹许雷 史媛媛 周 光 闫攀攀
李永超 李倩倩 王甲甲 强志明
数学建模指导组 数学建模指导组 数学建模指导组 指导教师组
省一等奖 省一等奖 省一等奖 省一等奖
2011数学建模A题神经网络优秀论文,带代码
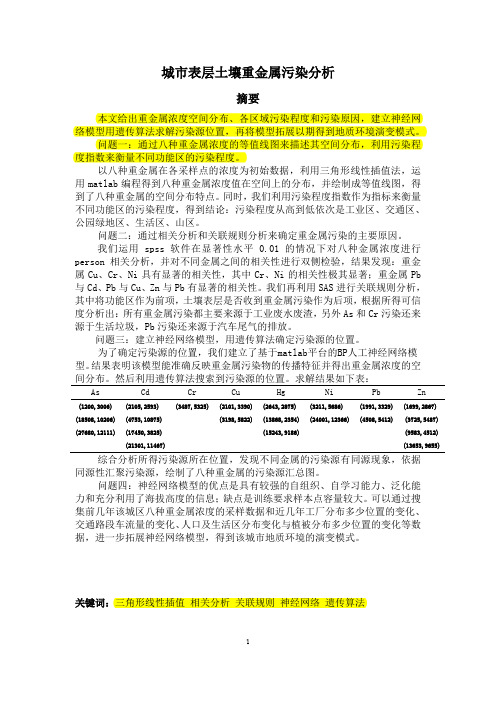
图 1 该城区的地形分布图
首先,我们根据样本点的位置和海拔绘制出该城区的地貌,见图 1。我们运 用 matlab 软件,根据各个网格区域中的重金属含量,用三角形线性插值的方法 得到各种重金属含量在空间上分布的等值线图。
1 图 2-1
2
1 图 2-2
2
图 2-1 给出了 As 在该区域的空间分布:图中可以观察到 As 有两个明显的高 值中心,我们标记为区域 1 和 2。这两个区域都处于工业区分布范围内,并以该 两个区域作为中心向外延伸, 浓度逐渐减少,同时我们注意到在山区的很多区域
Ni
(3211,5686) (24001,12366)
Pb
(1991,3329) (4508,5412)
Zn
(1699,2867) (3725,5487) (9583,4512) (13653,9655)
综合分析所得污染源所在位置,发现不同金属的污染源有同源现象,依据 同源性汇聚污染源,绘制了八种重金属的污染源汇总图。 问题四:神经网络模型的优点是具有较强的自组织、自学习能力、泛化能 力和充分利用了海拔高度的信息;缺点是训练要求样本点容量较大。可以通过搜 集前几年该城区八种重金属浓度的采样数据和近几年工厂分布多少位置的变化、 交通路段车流量的变化、 人口及生活区分布变化与植被分布多少位置的变化等数 据,进一步拓展神经网络模型,得到该城市地质环境的演变模式。
符号
意义
k i j
x ij
xi
表示不同功能区 表示金属的种类 表示不同的样本 表示样本 j 中金属 i 的浓度 表示金属 i 背景值的平均值 表示金属 i 背景值的标准差
表示 x i j 标准化后的值
i
Y ij
i
Ik
08--历年数学建模优秀论文大全

Can We Assess a Health Care System's Performance?参赛队员:董希望(自动化学院),刘琳燕(城环学院)刘福亮(软件学院)指导教师:肖 剑参赛单位:重庆大学参赛时间:2008年2月15∼18日Can We Assess a Health Care System's Performance?1.BackgroundHealth systems consist of all the people and actions whose primary purpose is to improve health. They may be integrated and centrally directed, but often they are not. After centuries as small-scale, largely private or charitable, mostly ineffectual entities, they have grown explosively in this century as knowledge has been gained and applied. They have contributed enormously to better health, but their contribution could be greater still, especially for the poor. Failure to achieve that potential is due more to systemic failings than to technical limitations. It is therefore urgent to assess current performance and to judge how health systems can reach their potential.The World Health Organization (WHO) is a specialized agency of the United Nations (UN) that acts as a coordinating authority on international public health. Established on 7 April 1948, and headquartered in Geneva, Switzerland, the agency inherited the mandate and resources of its predecessor, the Health Organization, which had been an agency of the League of Nations.The WHO's constitution states that its objective "is the attainment by all peoples of the highest possible level of health." Its major task is to combat disease, especially key infectious diseases, and to promote the general health of the people of the world.As well as coordinating international efforts to monitor outbreaks of infectious diseases, such as SARS, malaria, and AIDS, the WHO also sponsors programs to prevent and treat such diseases. The WHO supports the development and distribution of safe and effective vaccines, pharmaceutical diagnostics, and drugs. The WHO also carries out various health-related campaigns — for example, to boost the consumption of fruits and vegetables worldwide and to discourage tobacco use.The annual World Health Report (http://www.who.int/whr/en/index.html) assesses global health factors and World Health Statistics provides health statistics for the countries in the UN. The production and dissemination of health statistics is a major function of the WHO. To many people, these data and the associated analyses are considered unbiased and very valuable to the world community.2. Basic Assumption and Hypotheses1.Assume that in a certain interval such as 5years the main components (metrics) ofthe health care system stays steady, that is to say the metric won’t change continually.2.Assume that all the statistics we get from the database of the WHO is authentic.3.Assume that the ranking of the world's health systems in 2000 made by WHO isscientific and dependable.4.During the data processing if a data little than x we can replace it with x.5.If there existing data missing for some year’s indicator we can value it with thecorresponding value of the near years.3. SymbolsSymbol Definition and Property'Z The matrix before standardizationZ The matrix after standardizationz j The statistic of the j indicator to each countryu The main component to be evaluatedu m The m th main component of the indicatorl ij The load of the original indicatorR1 The correlation matrix of Zc i The contribution rate of the i th main componentS i The summation of the front i main components’ contribution rateQ The integrated score of each countryX The project set ( the Member States)U The attribute set which also means main component seta ij The attribute value of x i in reference to u jA The decision making matrixa i The mean value of the line I in the primitive matrixb i The standard deviation of row I in the primitive matrix4. Problem AnalysisTo determine several important and viable metrics for assessing the performance of a health care system and comparing health care systems in different countries. We have to know what metrics or indicators are there in a health care system, as is shown in the problem we search the web of the WHO and get the database of the indicators. There exists statistics for 50 core indicators on mortality, morbidity, risk factors, service coverage, and health systems, which take on more than one hundred and fifty terms of raw indicators. We must use some data mining technology or method to distill the crucial metrics.Considering the data is promiscuous and inconsistent and not all the countries have the corresponding data to each indicator from the year 1960 to 2006, we first need to choose certain year’s data as our study object. Then to the mass actual statistical data we can’t expect all the indicators are complete so what to do with the incomplete data to make sure that all the indicators or all the data we used below are universal or effective is an inevitable problem. There are 159 raw indicators how could we select the most important ones and combine them scientifically to make them more useful in measuring quality is another basal problem. Then how could we accomplish this goal? The main components analysis method which we could use to devise our first model will help a lot.Furthermore how could we assess a country’s health care system and make some comparisons with the combined metrics? This situation much agrees with the multiple attribute decision problems. So we could solve this problem by ranking all the countries health care systems using this multiple attribute decision method.5. The Establishment of Model5.1 The Primary Data and Indicators ProcessingAccording to the above problem analysis part we know that we could obtain enough raw data for almost 159 indicators from 1960 to 2006. We first choose a year 2004 whose data is much completer than other years as our study object. Then if some of the indicators of certain country in 2004 have no value and the year close to 2004 such as the year of 2005 or 2003 has the corresponding value we treat this close value as the valve of the country in that indicator in 2004.Based on these we select the indicators that 95% of the country has the corresponding data for them from all the 159 raw indicators. By doing this primary selection we make sure that all the indicators or all the data we used below are universal or effective. After the primary selection we get 48 crucial indicators as our primary outcomes (metrics).5.2 Model 1 DesignFollowing the above analysis we utilize the main components analysis method to devise our first model.When it comes to main components analysis the biggest effect to it is the dimension of the data. So in the practical application we first should make standardization to the data.Assume that 'Z is the matrix before standardization Z is the matrix after standardization z j is the statistic of the j indicator to each country; u is the main component to be evaluated, so the objective function could be:11111221221122221122p p p p m m m mp p u l z l z l z u l z l z l z u l z l z l z =++⎧⎪=++⎪⎨⎪⎪=++⎩""""""" (1)Where u 1, u 2,… u m is called the 1st, 2nd, … mth main component of the indicator z 1, z 2, z p ; l ij is the load of the original indicator z j (j=1,2, …,p) in each main component.The detailed process of this solution is as follows:Step1: Evaluate the standardized matrix Z of the matrix'Z The standardization of the 'Z is just replace the (i=1,2, …,p) and the z 'i z ijof the matrix 'Z with z i (i=1,2, …,p) and with z ij respectively, which is shown in table1Step 2: Evaluate the correlation matrix R1 of matrix ZR1 could be evaluated by the following matrix:1112121222121p p p p pp r r r r r r R r r r ⎡⎤⎢⎥⎢=⎢⎢⎥⎢⎥⎣⎦""##"#"⎥⎥ (2) Where r ij (i, j =1, 2,…,p) is the original indicator z i and z j ’s correlation coefficient specially r ij =r ji . r ij could be derived by the following formula:ij r = (3) Step 3: By formula 1 we can compute the characteristic root and characteristic vector of matrix R1 then rank the characteristic values of R1 as expression 110I R λ−= (4)(5)0≥≥≥≥λλλ"p 21Step 4: Evaluate the contribution rate and the accumulative contribution rate according to formula 1and 1of the main components, determine the proper number of the main components.(6) λ∑==="1(1,2,,i i p k k c i λ)pWhere is the contribution rate of the i th main component.i c(7) S i λ∑p λ====∑"11(1,2,,)i k k i p k k Here is the summation of the front i main components’ contribution rate. If thevalue of the accumulative contribution rate reaches to more than 80% we can approbate the effect of the main components.i S Step 5: Compute the load of the main component l ij(y ,z )(,1,2,,)ij i j ij l p i j p ===" (8)Step 6: Sum up the above five steps get our objective function11111221221122221122............p p p p m m m mp u l z l z l z u l z l z l z u l z l z l z =+++⎧⎪=+++⎪⎨⎪⎪=+++⎩"""p Step 7: Evaluate the integrated value of each country and make a ranking of them with the formula 1.112211(m m m ii )u u λλλλ==+++∑"Q u (9) Q is the integrated score of each country.5.3 Model 2 Design5.3.1 The Description of the PrincipleThe method of the multiple attribute decision making based on dispersion maximization is used to solve the multiple attribute decision making problems with the uncertain weight attribute. We can use this method to make ranking and comparison between different projects with multiple attributes.In more details the smaller the difference between certain attribute for all the projects is the less affection it has on the decision making and ranking of the projects. On the contrary the bigger it is the more affection it has on the decision making and ranking. As a result in the view of ranking the bigger of one attribute’s deviation is the bigger weight of this attribute should be given. Especially if there is no deviation for certain attribute to all the projects which means that this attribute will have little affection on the ranking we can value a zero to its weight.5.3.2 Model DevelopmentStep 1.Structure and Normalize the Decision Making Matrix1.1 Structure the Decision Making MatrixAssume that:M={1,2,…,m},N={1,2,…,n} (10)The projects set which also is the set of the Member States in WHO is XX={x 1,x 2,…,x n } (11)The attribute set which means main component set here is UU={u 1,u 2,…,u m } (12) is the attribute value of in reference to so we obtain the decision making matrix ()ij n m A a ×=whose form is shown as table 2 Table2. The form of the decision making matrixu 1u 2… u m x 1a 11a 12… a 1m x 2a 21a 22… a 2m ## # # x na n1a n2… a nm5.4 Model 3 DesignModel 3 is our predictive model, from model 1 we can get the objective function with the data of that year.When it comes to predicating for the convenience of evaluating the main components we can change the main component which is expressed by the standardization indicator z i into the form that expressed by nonstandard indicator z i ’ to predicate the main components.''''''1111122110''''''2211222220''''''11220(13)............p p p p m m m mp p m u l z l z l z l u l z l z l z l u l z l z l z l ⎧=++++⎪=++++⎪⎨⎪⎪=++++⎩"""Here(14)'()/i i i z z a b =−i Substitute into formula 1 can we obtain the formula 2.'i z i a is the mean value of the line i in the primitive matrix; is the standard deviationof row i in the primitive matrix.i bThen utilize the formula 9 to compute the synthetic score and get variability ofthe system.Normalize the Decision Making MatrixThere are many types of attributes such as benefit type, cost type, fixation type, deviate type, interval type, deviate interval type etc. In our model all the attribute could be sorted to two types the benefit type and the cost type approximately. The benefit cost requires the value of the attribute as big as possible; the cost type requires the value of the attribute as small as possible.To eliminate the impact of the different dimensions to the decision making result we should normalize the decision making matrix A whose values could be obtained from the model 1.Assume that I i (i=1, 2) stands for the subscript set of the benefit type and cost type. If the attribute is benefit type we value i in I i as 1. If the attribute is cost type we value i in I i as 2.1min(),,max()min()ij ij i ij ij ij ii a a r i a a N j I −=−∈∈ (15)2max(),,max()min()ij ij i ij ij ij i i a a r i a a N j I −=−∈∈ (16)After this step we get the normalized matrix ()ij n m R r ×= whose form is the samewith the matrix A.Step 2: Calculus the optimization weight vector w11111,n n ji kj i k j m n nij kj j i k r r w r r =====−=−∑∑∑∑∑j M ∈ (17) Where w j is the j th main component’s weight.Step 3: Computer the synthetic attribute z i (w) (i ∈N) of project x i .1(),,mi ij j j z w r w i N j ==∈∑M ∈ (18)Step 4: Make ranking and comparison to the projects (countries) using z i (w)(i ∈N)6. Applying the Model1 and Model 26.1 Applying the Model 1 to the Statistics of the Year 20046.1.1 Data for Model 1 in the Year of 2004We first select the indicators that 95% of the countries own these indicators from all 159 indicators getting 28 indicators which could be seen in appendix Ⅰ. Then weselect the countries that have the data for all these 28 indicators from all 193 Member States getting 163 countries. By doing these we have made good preparation for our model 1’s solution.6.1.2 Solution of the Model 1 for the Year of 2004Based on the above data we solve our model 1 in matlab using the function of zscore to normalize the data, and then we calculate the characteristic roots and characteristic vector. The characteristic roots are shown in the table 3.Table3. Part Valves of Model 1From the table 3 we can see that the front six red colored components’ accumulative contribution rate reaches to 81.5% which means that most of the main components are involved, so these six components are just our combined metrics. We renamed these six combined indicators with A, B, C, D, E, F metrics all of which are constituted by several raw indicators and could reflect certain performance of a health care system.In more detail the metric A is much positively related with life expectancy, per capita total expenditure on health at international dollar rate etc and much negatively related with mortality rate, incidence of tuberculosis (per 100 000 population per year) etc. The visual relationship between the metric and the 28 indicators is shown in figure 1. The x axis is the order of the 28 indicators which maps to corresponding 28 indicators in appendix Ⅰ. The y axis is the affection of each of the 28 indicator on metric A. All the rest five figures follow this instruction so we won’t explain the rest five figures again.Figure1. The affection of the 28 indicators on metric A The metric B is much positively related with expenditure on health, disease detection rate etc and much negatively related with government expenditure on health, alcohol consumption etc.Figure2. The affection of the 28 indicators on metric B The metric C is much positively related with General government expenditure on health as percentage of total expenditure on health, immunized with disease etc and much negatively related with private expenditure on health as percentage of total expenditure on health, population (in thousands) total etc.Figure3. The affection of the 28 indicators on metric C The metric D is much positively related with private expenditure on health as percentage of total expenditure on health, immunized with disease etc and muchnegatively related with General government expenditure on health as percentage of total expenditure on health etc.Figure4. The affection of the 28 indicators on metric D The metric E is much positively related with external resources for health as percentage of total expenditure on health etc and much negatively related with tuberculosis: DOTS case detection rate, probability of dying (per 1 000 population) between 15 and 60 years etc.Figure5. The affection of the 28 indicators on metric E The metric F is much positively related with immunized with disease, out-of-pocket expenditure as percentage of private expenditure on health etc and much negatively related with general government expenditure on health as percentage of total government expenditure, population (in thousands) total etc.Figure6. The affection of the 28 indicators on metric FThe above descriptions show that our metrics is reasonable, moreover all the 28indicarors we selected could be found in 92% of all Member States, which means that our metrics could be easily collected.Furthermore we get the ranking for all the 163 countries that own orbicular and effective data. The front and the back 20 countries in our ranking and their scores calculated by our model 1 are listed as table 4:Table4. Part of our Ranking by Our Model1The whole ranking is shown in appendix Ⅱ.After obtaining the six metrics we treat the still missing value’s indicator as zero then recompute the ranking of the year 2004 with the model 1 and get another ranking for all the Member States which we list in appendix Ⅲ.In conclusion we put forward 28 important indicators from all the 159 indicators furthermore we combine the 28 important indicators getting 6 main components which we renamed as metric A, B, C, D, E and F. Then we assess the health care system with these six metrics and make a ranking of all the 163 countries.6.1.3 Applying model 1 to the Statistics of the Year 2000Using model 1 and the six metrics obtained from 4.11 we assess the health care system of each country in the year of 2000. This time we only utilize the data of this year, which means that we just substitute the data of 2004 with that of 2000.By doing this we get the ranking of this year as table 5 which just show out the front and the back 20 countries too.Table5. The Part Ranking of the Year 2000 by Model 1 (There are 194 Member States in 2000; the score here is just a relative value computed by our model; the whole ranking is shown in appendix Ⅳ)6.2 Applying the Model 2The six metrics obtained from model1 is ordered. Although the six metrics keep the same in the model 2 as what they are in model 1 according to our assumptions, there is no certain order among them in our model 2. The data processing methods for the raw data are the same with what we have described and used before.6.2.1 Applying the Model 2 to the year of 2004With the help of the software matlab we realize the algorithm of dispersion maximization computing the weight of the six metrics, and then we calculate the synthetic score of each of the 163 country that own holonomic statistics after our data mining process. After comparing the synthetic score of these countries we get the ranking of their health system as shown in table 6.Table6. Part of the ranking for the 163Member States(The score here is just a relative value computed by our model; the whole ranking is shown in appendix Ⅴ)6.2.2 Applying the Model 2 to the year of 2000Similar to 4.1.2 we just replace the data of 4.2.1 with the data of the year 2000 then compute the synthetic score of all the 194 Member States. After comparing the different countries we get the ranking as table7.Table7. Part of the ranking for 2000 by model 27. Comparisons7.1 Comparisons between Different RankingsFrom the above solution we obtain 4 different rankings. The precise clues of our models have already showed their validity. Besides we can load a ranking for all the 190 Member states in 2000 from the WHO’s official web which we list in appendix Ⅷ.By making comparisons between our two rankings with the official ranking of the year 2000 we can test the reliability and practicability of our model to a certain extent. The figure7 shows their relationship clearly.Figure7. The corresponding relationship between our rankings and the WHO’s We can see that the dots which stand for parts of the countries in the rankings match quite well with each other in the three polygonal lines. That means the model1 and model 2’s results not only agree with each other but also agree with the official results quite well. So we can conclude that our two models are practical and reasonable.Since the solution for the year of 2000 is dependable, we have reason enough to predicate that our solution for 2004 is authentic as the only difference between 2004 and 2000 is the substituted statistics and the data of 2004 is more holonomic than that of 2000.To make sure that both of our models’ results for the year 2004 are unitive we make a comparison between their rankings. We select some characteristic countries in both rankings and compare those countries rankings as shown in figure 8.Figure8. The comparison between the two rankings for the year 2004From the figure we find that the two rankings match quite well.In conclusion our models are scientific and our results are authentic.7.2 Comparisons between US and FranceIn the 2000’s ranking of WHO France takes the first place, which also could be seen clearly in the appendix. In the year 2004 there is no official ranking so we assess these two countries health care system with our model 1 to see which country has the better health care system then.The table8 shows their score according to our six metrics:Table8. The comparisons between US and France’s health system according to our metricsThe metric A, C, F belongs to benefit type and the rest belong to cost type. Base on this we can see that the health care system of US in 2004 is better than France in metric A, B, D. According to our table 1 we know that the synthetic score of US is better than France.7.3 Comparisons between US and IndiaIn the ranking of WHO the health system of US is better than India. With the help of our mode 1 we consider that India has the poor health care system in 2004, so we make a comparison between them.Table9.The comparisons between US and India’s health system according to our metricsSimilar to 7.2 we can see that the health care system of US is better than India in metric A, B, C, D, F. Also from the table 9 we know that the ranking of US is much better than India.8. Applying the Model 3Based on model 1 and model 2 with the help of the software matlab we realize the algorithm in model 3 and get the predictive function of the synthetic scores as follows:''''1234'7''''67891''''1112131410.0033810.00966920.0105590.00194680.000522790.00052406 3.06100.0782130.00421940.0003620.00873510.00956890.00984890.000392260.0043929Q z z z z z z z z z '5z z z z z z −=−++−−−−×−−−−++−+'5''''1617181920''''2122232425'''2627280.0053440.0257520.00377190.000143460.000182770.000109410.000136790.00534410.056440.00292340.000840420.029650.012447 3.8668''z z z z z z z z z z z z ++−++++−+−−++−z (19)Considering the affection of the weight on the synthetic score we could find that the bigger the absolute value of weight is the bigger the impact is on the synthetic score of the country. On the contrary if the absolute value of weight is small then the variation of the metric won’t produce big changes to the synthetic score. Then we take some indicators of the all 28 indicators as examples to discuss what affection it will has on the health care system if the various changes are occurred.'8z is the formula is the total fertility rate (per woman). It has a negative correlation with the synthetic score. What’s more it has a big affection on the score so this indicator should be as small as possible, which means that the government should take some measures to control the population within a proper range to improve the health care system of the nation.'24z is the total expenditure on health as percentage of gross domestic product. Itis an indicator that positively related with the synthetic score which means that the more it spend on the total expenditure on health as percentage of gross domestic product the better score it has in the system.'17z is the general government expenditure on health as percentage of totalgovernment expenditure. It is an indicator that positively related with the synthetic score which means that the bigger the general government expenditure on health as percentage of total government expenditure is the better score it has in the system'3z is the life expectancy at birth (years) males. It is an indicator that positivelyrelated with the synthetic score which means that the longer the life expectancy at birth (years) males is the better score it has in the system.'z stands for the neonatal mortality rate (per 1 000 live births). It has a negative 11correlation with the synthetic score. That’s to say the smaller the neonatal mortality rate (per 1 000 live births)is the better the health care system will become.9. The Strength and Weakness9.1The StrengthWe obtain the statistics directly from the raw database of the WHO’s official web not from the report of the WHO. We use some data mining technology to draw the available and effective data from thousands terms of data ourselves.We develop three different models to solve all the six parts of the problem, those models are built with precise logic, scientific principle which could solve the problems efficaciously.We don’t solve the problem part by part but solve them in our models’ development and solution process, which keeps the whole paper’s with a good continuity.We compare our result with the practical result, which tests our models’ practicability and validity greatly.Our models could be easily extended to other fields to solve the multiple attribute decision making problems.Our models are independent to the metric (indicators) to a certain extent as the algorithm of our models has the universal applications.9.2 The WeaknessThe raw data we get is the data from the real world, which means that there must be some imperfect data which do have some negative impact on our result.As there are so many indictors that it is hard to select proper metrics to assess the health system properly without some kind of error.Because the limitation of the time and resource it’s inevitable to have some imperfect aspects in our models, analysis and paper.10. References[1] Zeshui Xu, 8/2004, Uncertain Multiple Attribute Decision Making: Methods andApplications, Tsinghua University Press.[2] Qiyuan Jiang, Jinxing Xie, 12/2004, Mathematical Model, Higher Education Press[3] The World Health Report 2000 - Health systems: improving performance.http://www.who.int/whr/2000/en/whr00_en.pdf[4] World Health Organization, http://www.who.int/research/en/s[5] Principal Component Analysis,/jpkc/jldlx/admin/ewebeditor/UploadFile/200783101241734.ppt[6] /wiki/World_Health_Organisation,"World Health Organization"11. AppendixAppendixⅠ: The list of all the 28 indicators and their sequence numberAppendixⅡ: The Ranking of all 163 Countries in 2004 by model 1Appendix Ⅲ: The Ranking of all 194 Countries in 2004 by model 1。
B题:电工杯数学建模竞赛获奖论文

1.预测每次航行各周预订舱位的人数,完善各航次每周实际预订人数非完全 累积表 sheet2。要求至少采用三种预测方法进行预测,并分析结果。
2.预测每次航行各周预订舱位的价格,完善每次航行预订舱位价格表 sheet3。 3.依据附件中表 sheet4 给出的每周预订价格区间以及每周意愿预订人数,预 测出公司每周给出的预订平均价格。 4.依据附件中表 sheet1-sheet4,建立邮轮每次航行的最大预期售票收益模型, 并计算第 8 次航行的预期售票收益。 5.在头等、二等舱位未满的情况下,游客登船后,可进行升舱(即原订二等 舱游客可通过适当的加价升到头等舱,三等舱游客也可通过适当的加价升到头等 舱、二等舱)。建立游客升舱意愿模型,为公司制定升舱方案使其预期售票收益 最大。
3.模型的假设与符号说明
3.1 模型的假设
1.假设邮轮旅游不存在高峰期,邮轮票价、预定人数等保持平稳状态; 2.假设题目表格中给出的平均价格在价格浮动比之内; 3.假设邮轮各个舱位预定平均价格和距离邮轮出发时间的关系保持一致; 4.假设意愿预定人数和实际预定人数的转换只和价格、舱位种类有关。 5.假设游客上船之后升舱没有任何手续费; 6.假设每个舱位中的人数和舱位的价格成反比例关系,并且三种舱位的比例 关系相同;
数学建模国家一等奖优秀论文

承诺书我们仔细阅读了《全国大学生数学建模竞赛章程》和《全国大学生数学建模竞赛参赛规则》(以下简称为“竞赛章程和参赛规则”,可从全国大学生数学建模竞赛网站下载)。
我们完全明白,在竞赛开始后参赛队员不能以任何方式(包括电话、电子邮件、网上咨询等)与队外的任何人(包括指导教师)研究、讨论与赛题有关的问题。
我们知道,抄袭别人的成果是违反竞赛章程和参赛规则的,如果引用别人的成果或其他公开的资料(包括网上查到的资料),必须按照规定的参考文献的表述方式在正文引用处和参考文献中明确列出。
我们郑重承诺,严格遵守竞赛章程和参赛规则,以保证竞赛的公正、公平性。
如有违反竞赛章程和参赛规则的行为,我们将受到严肃处理。
我们授权全国大学生数学建模竞赛组委会,可将我们的论文以任何形式进行公开展示(包括进行网上公示,在书籍、期刊和其他媒体进行正式或非正式发表等)。
我们参赛选择的题号是(从A/B/C/D中选择一项填写): B我们的报名参赛队号为(8位数字组成的编号):所属学校(请填写完整的全名):参赛队员(打印并签名) :1.2.3.指导教师或指导教师组负责人(打印并签名):(论文纸质版与电子版中的以上信息必须一致,只是电子版中无需签名。
以上内容请仔细核对,提交后将不再允许做任何修改。
如填写错误,论文可能被取消评奖资格。
)日期: 2014 年 9 月 15日赛区评阅编号(由赛区组委会评阅前进行编号):编号专用页赛区评阅编号(由赛区组委会评阅前进行编号):全国统一编号(由赛区组委会送交全国前编号):全国评阅编号(由全国组委会评阅前进行编号):创意平板折叠桌摘要目前住宅空间的紧张导致越来越多的折叠家具的出现。
某公司设计制作了一款折叠桌以满足市场需要。
以此折叠桌为背景提出了三个问题,本文运用几何知识、非线性约束优化模型等方法成功解决了这三个问题,得到了折叠桌动态过程的描述方程以及在给定条件下怎样选择最优设计加工参数,并针对任意形状的桌面边缘线等给出了我们的设计。
数学建模获奖论文(优秀范文10篇)11000字
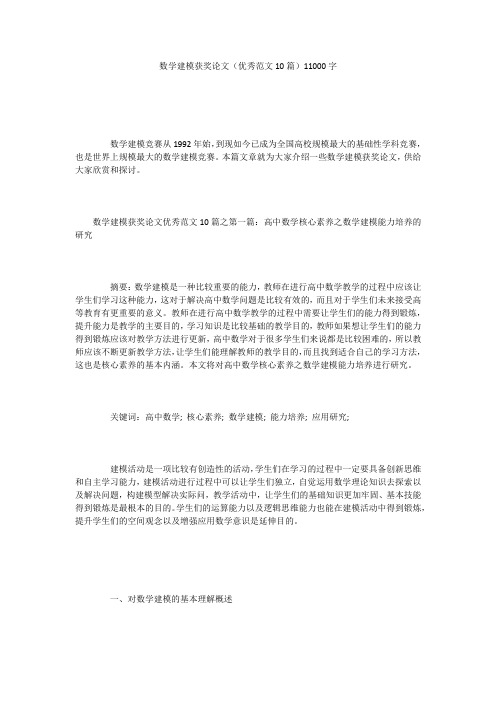
数学建模获奖论文(优秀范文10篇)11000字数学建模竞赛从1992年始,到现如今已成为全国高校规模最大的基础性学科竞赛,也是世界上规模最大的数学建模竞赛。
本篇文章就为大家介绍一些数学建模获奖论文,供给大家欣赏和探讨。
数学建模获奖论文优秀范文10篇之第一篇:高中数学核心素养之数学建模能力培养的研究摘要:数学建模是一种比较重要的能力,教师在进行高中数学教学的过程中应该让学生们学习这种能力,这对于解决高中数学问题是比较有效的,而且对于学生们未来接受高等教育有更重要的意义。
教师在进行高中数学教学的过程中需要让学生们的能力得到锻炼,提升能力是教学的主要目的,学习知识是比较基础的教学目的,教师如果想让学生们的能力得到锻炼应该对教学方法进行更新,高中数学对于很多学生们来说都是比较困难的,所以教师应该不断更新教学方法,让学生们能理解教师的教学目的,而且找到适合自己的学习方法,这也是核心素养的基本内涵。
本文将对高中数学核心素养之数学建模能力培养进行研究。
关键词:高中数学; 核心素养; 数学建模; 能力培养; 应用研究;建模活动是一项比较有创造性的活动,学生们在学习的过程中一定要具备创新思维和自主学习能力,建模活动进行过程中可以让学生们独立,自觉运用数学理论知识去探索以及解决问题,构建模型解决实际问,教学活动中,让学生们的基础知识更加牢固、基本技能得到锻炼是最根本的目的。
学生们的运算能力以及逻辑思维能力也能在建模活动中得到锻炼,提升学生们的空间观念以及增强应用数学意识是延伸目的。
一、对数学建模的基本理解概述高中数学建模最简单的解释就是利用学生们学习过的理论知识来建立数学模型解决遇到的问题。
数学建模的基本过程就是对生活中或者课本中比较抽象问题解决的过程。
通过抽象可以建立刻画出一种较强的数学手段,通过运用数学思维也能观察分析各种事物的基本性质和特点。
学生们可以从复杂的问题中抽离出自己熟悉的模型,然后在利用好数学模型去解决实际问题基本就是事半功倍。
国赛数学建模竞赛优秀论文
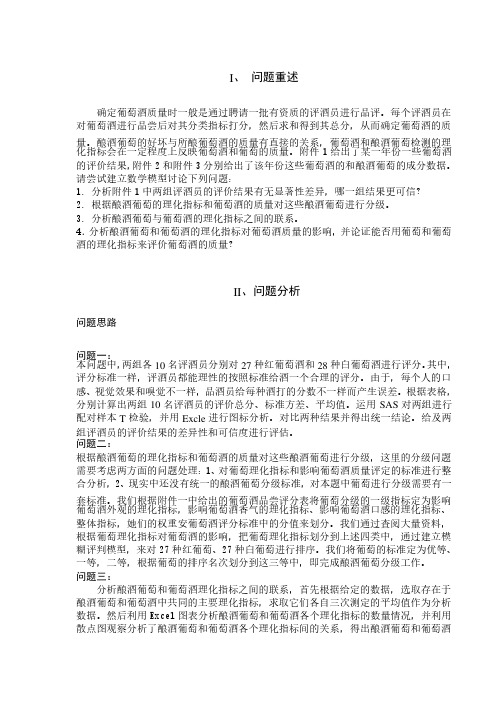
I 、问题重述 确定葡萄酒质量时一般是通过聘请一批有资质的评酒员进行品评。
每个评酒员在对葡萄酒进行品尝后对其分类指标打分,然后求和得到其总分,从而确定葡萄酒的质量。
酿酒葡萄的好坏与所酿葡萄酒的质量有直接的关系,葡萄酒和酿酒葡萄检测的理化指标会在一定程度上反映葡萄酒和葡萄的质量。
附件1给出了某一年份一些葡萄酒的评价结果,附件2和附件3分别给出了该年份这些葡萄酒的和酿酒葡萄的成分数据。
请尝试建立数学模型讨论下列问题:请尝试建立数学模型讨论下列问题: 1. 分析附件1中两组评酒员的评价结果有无显著性差异,哪一组结果更可信?中两组评酒员的评价结果有无显著性差异,哪一组结果更可信? 2. 根据酿酒葡萄的理化指标和葡萄酒的质量对这些酿酒葡萄进行分级。
根据酿酒葡萄的理化指标和葡萄酒的质量对这些酿酒葡萄进行分级。
3. 分析酿酒葡萄与葡萄酒的理化指标之间的联系。
分析酿酒葡萄与葡萄酒的理化指标之间的联系。
4.分析酿酒葡萄和葡萄酒的理化指标对葡萄酒质量的影响,并论证能否用葡萄和葡萄酒的理化指标来评价葡萄酒的质量?酒的理化指标来评价葡萄酒的质量?II 、问题分析问题思路问题一: 本问题中,两组各10名评酒员分别对27种红葡萄酒和28种白葡萄酒进行评分。
其中,评分标准一样,评酒员都能理性的按照标准给酒一个合理的评分。
由于,每个人的口感、视觉效果和嗅觉不一样,品酒员给每种酒打的分数不一样而产生误差。
品酒员给每种酒打的分数不一样而产生误差。
根据表格,根据表格,分别计算出两组10名评酒员的评价总分、标准方差、平均值。
运用SAS 对两组进行配对样本T 检验,并用Excle 进行图标分析。
对比两种结果并得出统一结论。
给及两组评酒员的评价结果的差异性和可信度进行评估。
组评酒员的评价结果的差异性和可信度进行评估。
问题二:根据酿酒葡萄的理化指标和葡萄酒的质量对这些酿酒葡萄进行分级,这里的分级问题需要考虑两方面的问题处理:1、对葡萄理化指标和影响葡萄酒质量评定的标准进行整合分析,2、现实中还没有统一的酿酒葡萄分级标准,现实中还没有统一的酿酒葡萄分级标准,对本题中葡萄进行分级需要有一对本题中葡萄进行分级需要有一套标准。
数学建模优秀论文的范文
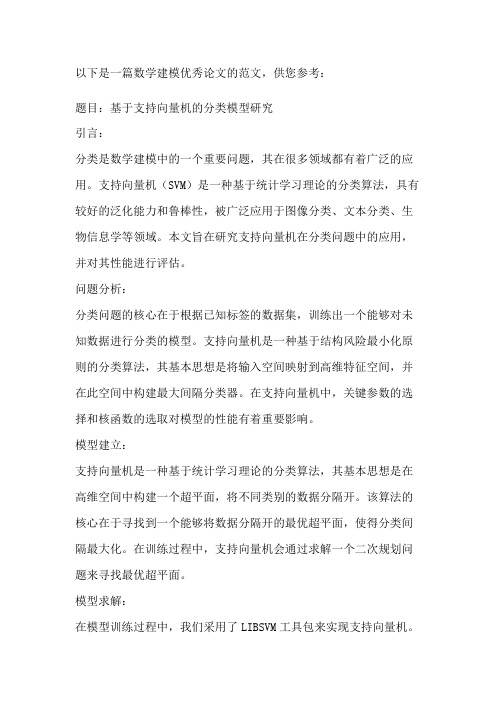
以下是一篇数学建模优秀论文的范文,供您参考:题目:基于支持向量机的分类模型研究引言:分类是数学建模中的一个重要问题,其在很多领域都有着广泛的应用。
支持向量机(SVM)是一种基于统计学习理论的分类算法,具有较好的泛化能力和鲁棒性,被广泛应用于图像分类、文本分类、生物信息学等领域。
本文旨在研究支持向量机在分类问题中的应用,并对其性能进行评估。
问题分析:分类问题的核心在于根据已知标签的数据集,训练出一个能够对未知数据进行分类的模型。
支持向量机是一种基于结构风险最小化原则的分类算法,其基本思想是将输入空间映射到高维特征空间,并在此空间中构建最大间隔分类器。
在支持向量机中,关键参数的选择和核函数的选取对模型的性能有着重要影响。
模型建立:支持向量机是一种基于统计学习理论的分类算法,其基本思想是在高维空间中构建一个超平面,将不同类别的数据分隔开。
该算法的核心在于寻找到一个能够将数据分隔开的最优超平面,使得分类间隔最大化。
在训练过程中,支持向量机会通过求解一个二次规划问题来寻找最优超平面。
模型求解:在模型训练过程中,我们采用了LIBSVM工具包来实现支持向量机。
LIBSVM是一种常用的支持向量机实现工具包,其提供了高效的求解算法和方便的接口。
在实验中,我们采用了交叉验证和网格搜索等方法来选择最优的参数组合,并对其进行评估。
结果分析:在实验中,我们采用了多种数据集来验证支持向量机的性能,包括图像分类、文本分类和生物信息学等领域的数据集。
实验结果表明,支持向量机在多个领域中都取得了较好的分类效果,其准确率、召回率和F1得分等指标均优于其他传统分类算法。
同时,我们还对其进行了误差分析,发现支持向量机具有较好的泛化性能和鲁棒性。
结论与展望:本文研究了支持向量机在分类问题中的应用,并对其性能进行了评估。
实验结果表明,支持向量机在多个领域中都取得了较好的分类效果,其准确率、召回率和F1得分等指标均优于其他传统分类算法。
同时,支持向量机还具有较好的泛化性能和鲁棒性。
高教社杯全国大学生数学建模竞赛获奖论文(精品)
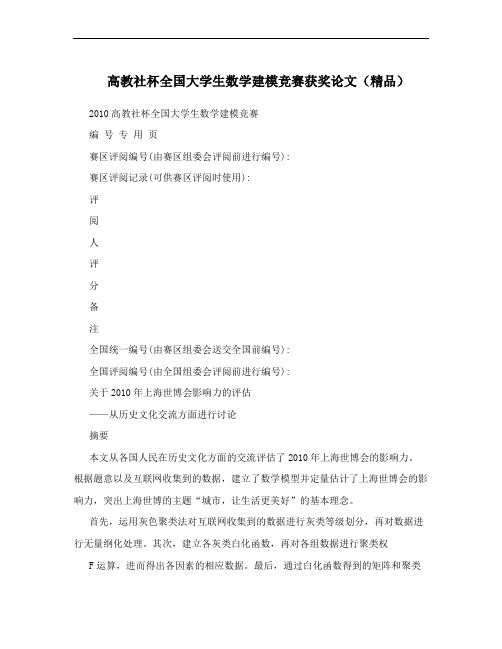
高教社杯全国大学生数学建模竞赛获奖论文(精品)2010高教社杯全国大学生数学建模竞赛编号专用页赛区评阅编号(由赛区组委会评阅前进行编号):赛区评阅记录(可供赛区评阅时使用):评阅人评分备注全国统一编号(由赛区组委会送交全国前编号):全国评阅编号(由全国组委会评阅前进行编号):关于2010年上海世博会影响力的评估——从历史文化交流方面进行讨论摘要本文从各国人民在历史文化方面的交流评估了2010年上海世博会的影响力。
根据题意以及互联网收集到的数据,建立了数学模型并定量估计了上海世博会的影响力,突出上海世博的主题“城市,让生活更美好”的基本理念。
首先,运用灰色聚类法对互联网收集到的数据进行灰类等级划分,再对数据进行无量纲化处理。
其次,建立各灰类白化函数,再对各组数据进行聚类权F运算,进而得出各因素的相应数据。
最后,通过白化函数得到的矩阵和聚类n权运算得到的函数,应用求聚类公式,求得各聚类对象的,,,fd*,LjjLLj,,,jL,1j各灰色聚类系数及结果。
然后应用层次分析法,推导出一种进行加权分析的方法,利用本方法对影响世博会的各个因素进行加权,得出了各个世博城市关于T,通过比较得到上海世博会影影响力的组合权重数据为(0.3634,0.3620,0.2743)响力均高于爱知、汉诺威世博会。
合适的评估体系是本课题的关键。
我们充分利用互联网收集到的数据进行分析及统计,并考虑到方案的可操作性。
通过组合权重数据,得到了三个世博城市关于影响力的权重。
由于此模型不受指数的影响,有很好的灵活性,使得我们可以根据实际情况灵活选取指数,减少模型的工作量,增加模型精度。
关键字:定量估计、层次分析法、灰色聚类法1一、问题重述2010年上海世博会是首次在中国举办的世界博览会。
从1851年伦敦的“万国工业博览会”开始,世博会正日益成为各国人民交流历史文化、展示科技成果、体现合作精神、展望未来发展等的重要舞台。
可以从我们感兴趣的某个侧面,建立数学模型,利用互联网数据,定量评估2010年上海世博会的影响力。
2008年全国数学建模竞赛C题
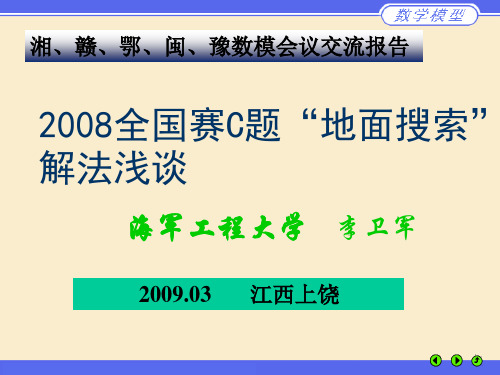
10人组 人组 转向31次 直穿” 转向 次,“直穿”69 格 1号外拐 次,内拐 次;10号外拐 ,内拐 次 号外拐17次 内拐14次 号外拐14,内拐17次 号外拐 号外拐 1号搜索用时: 号搜索用时: 号搜索用时 69 × 400 + 17 × 1287.38 + 14 × 87.38 = 69108.72秒 0. 0.6 = 19.197小时 1号“空走”用时: 号 空走”用时: 1号总用时: 号总用时: 号总用时 380 + 800 + 20 = 1000秒 = 0.278小时 19.475小时 小时 1.2 综上讨论,全部50名队员中 人组的1号最 综上讨论,全部 名队员中10人组的 号最 名队员中 人组的 后到达,总用时: 后到达,总用时:19.475小时 小时
∑x
k =1
4
(k ) ij
=2
(1) ij + 1
x x
(3) ij
(1) ij + 1+ (1 x Nhomakorabea)(1 x
(3) ij
) = 1 (1 ≤ j ≤ 13)
(2) (2) xij xi(4) j + (1 xij )(1 xi(4) j ) = 1 (1 ≤ i ≤ 8) + 1, + 1,
2、问题的分析 、 (1)搜索时间下限的估计 ) 需要搜索的面积为11200米×7200米=8064ⅹ104平方米, 需要搜索的面积为 米 米 ⅹ10 平方米, 一个队员的搜索半径为20米 一个队员的搜索半径为 米,若将上述矩形区域划分成 40ⅹ40的小正方形,共有 的小正方形, 个小正方形, 个队员 个队员, ⅹ 的小正方形 共有50400个小正方形,20个队员, 个小正方形 平均每个队员要搜索2520个小正方形 个小正方形. 平均每个队员要搜索 个小正方形 一个队员搜索一个小正方形的最短用时 “直穿” 40/0.6=66.67秒 直穿” 直穿 秒 “转向” 66.67秒 +“扫角” 转向” 扫角” 转向 秒 扫角 “扫角” 扫角” 扫角 1 20( 2 1)( + 1.2) = 20.71秒 0.6
精选五篇数学建模优秀论文
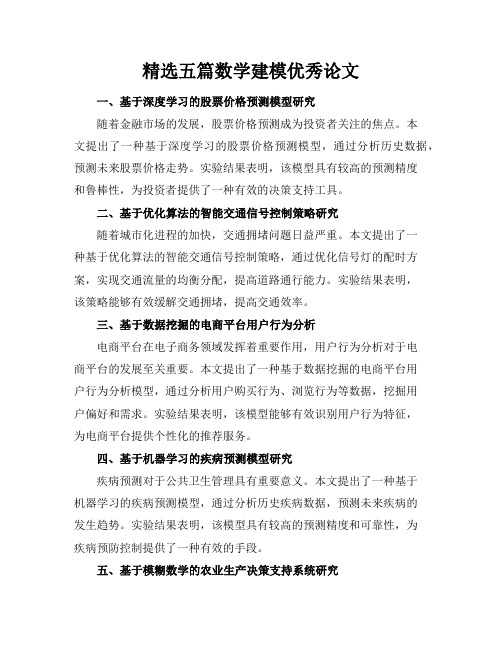
精选五篇数学建模优秀论文一、基于深度学习的股票价格预测模型研究随着金融市场的发展,股票价格预测成为投资者关注的焦点。
本文提出了一种基于深度学习的股票价格预测模型,通过分析历史数据,预测未来股票价格走势。
实验结果表明,该模型具有较高的预测精度和鲁棒性,为投资者提供了一种有效的决策支持工具。
二、基于优化算法的智能交通信号控制策略研究随着城市化进程的加快,交通拥堵问题日益严重。
本文提出了一种基于优化算法的智能交通信号控制策略,通过优化信号灯的配时方案,实现交通流量的均衡分配,提高道路通行能力。
实验结果表明,该策略能够有效缓解交通拥堵,提高交通效率。
三、基于数据挖掘的电商平台用户行为分析电商平台在电子商务领域发挥着重要作用,用户行为分析对于电商平台的发展至关重要。
本文提出了一种基于数据挖掘的电商平台用户行为分析模型,通过分析用户购买行为、浏览行为等数据,挖掘用户偏好和需求。
实验结果表明,该模型能够有效识别用户行为特征,为电商平台提供个性化的推荐服务。
四、基于机器学习的疾病预测模型研究疾病预测对于公共卫生管理具有重要意义。
本文提出了一种基于机器学习的疾病预测模型,通过分析历史疾病数据,预测未来疾病的发生趋势。
实验结果表明,该模型具有较高的预测精度和可靠性,为疾病预防控制提供了一种有效的手段。
五、基于模糊数学的农业生产决策支持系统研究农业生产决策对于提高农业效益和农民收入具有重要意义。
本文提出了一种基于模糊数学的农业生产决策支持系统,通过分析农业环境、市场需求等因素,为农民提供合理的生产决策建议。
实验结果表明,该系统能够有效提高农业生产效益,促进农业可持续发展。
精选五篇数学建模优秀论文一、基于深度学习的股票价格预测模型研究随着金融市场的发展,股票价格预测成为投资者关注的焦点。
本文提出了一种基于深度学习的股票价格预测模型,通过分析历史数据,预测未来股票价格走势。
实验结果表明,该模型具有较高的预测精度和鲁棒性,为投资者提供了一种有效的决策支持工具。
历届数学建模优秀论文

历届数学建模优秀论文引言数学建模是一种将现实问题转化为数学模型,并通过数学方法进行求解和分析的方法。
在数学建模竞赛中,评选出的优秀论文不仅反映了参赛团队的实力,也对数学建模的发展起到了积极的推动作用。
本文将对历届数学建模优秀论文进行回顾和总结,以展示数学建模领域的发展趋势和研究方向。
第一届数学建模优秀论文第一届数学建模竞赛于1995年举办,该届共有来自全国50个高校的120支队伍参赛。
在该届中,以下论文脱颖而出,成为第一届数学建模的优秀论文:1.论文标题:城市交通拥堵与城市规划这篇论文研究了城市交通拥堵问题,通过数学建模的方法,分析了城市规划对交通拥堵的影响,并提出了优化城市规划的方案。
这篇论文不仅展示了数学建模在解决实际问题中的效果,也对城市交通规划提供了有益的参考意见。
2.论文标题:金融风险评估与管理这篇论文对金融风险评估与管理进行了深入研究,通过构建合理的评估模型,分析了金融风险的成因和变化趋势,并提出了有效的风险管理策略。
该论文在金融行业引起了广泛的关注,为金融机构的风险管理提供了有力的支持。
第二届数学建模优秀论文第二届数学建模竞赛于1996年举办,参赛高校增加到100所。
以下是第二届的优秀论文:1.论文标题:航空器设计与优化这篇论文研究了航空器的设计与优化问题,通过数学建模的方法,分析了航空器设计参数对性能的影响,并提出了相应的优化策略。
该论文对航空器设计的理论和实践具有重要意义。
2.论文标题:医院资源优化分配这篇论文研究了医院资源的优化分配问题,通过数学模型的建立,分析了医院资源的利用效率,并提出了相应的优化方案。
该论文在医疗卫生领域引起了广泛的关注,为医院资源的合理配置提供了重要的参考。
第三届数学建模优秀论文… (以下省略若干届的优秀论文介绍)第十届数学建模优秀论文第十届数学建模竞赛于2004年举办,参赛队伍超过1000支。
以下是第十届的优秀论文:1.论文标题:气象预测模型的研究与改进这篇论文对气象预测模型进行了深入研究,通过改进传统的气象预测模型,提高了气象预测的准确度。
2004年A题全国数学建模优秀论文4
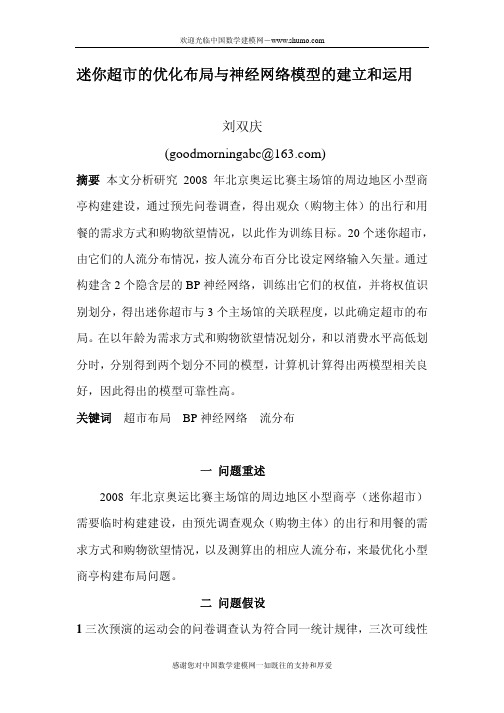
复结果比重较大,则认为该训练得到的权值是该输入和输出的良好隐
射。
因此此类算法可以运用:
1 空间场能量分布,边坡应力分析等。
byage = 0.2027 0.3323 0.4650
感谢您对中国数学建模网一如既往的支持和厚爱
欢迎光临中国数学建模网-
100
orrr
10-2
E
quaed r S-
10-4
mu
S
10-6 0
5
4
aet 3
Rg
Leannri
2
1
0 0
Training for 55 Epochs
图 四 流量相关分析 根据题目说明的,出行均采取最短路径,因此上图中的相关的连线(可 行道路),对于每一个迷你超市来说,并不是所有的都分配到。在计 算相关流量时,亦定义:
ζ =M×N k
其中:ζ 为第 k 超市分配到的百分总和比,M 为三个主场管某场管 k
的总人数,N 为调查观众(购物主体)的出行和用餐的需求方式和购 物欲望情况百分率分布。此题先把所有连接进行表格描述,然后通过 人工选择确定归属。其中: a=4; b=6; c=10; c1=a*(bb(1)+bb(4))+c*bb(1); c2=a*(bb(1)+bb(4)+bb(3))+b*bb(1)+c*bb(1); c3=1*(a*(bb(2)+bb(5)+bb(6)+bb(7)+bb(8)+bb(9))+c*bb(1)); c4=1*(a*(bb(2)+bb(4)+bb(5)+bb(6)+bb(7)+bb(8)+bb(9))+c*bb(1)); 其中a,b,c(注:a, c因为程序关系,位置与实际数据不对应) 为场管总容纳人数,bb(i) 为购物主体的兴趣分布,每一项的右项为人
以下是一篇数学建模优秀论文的范1

以下是一篇数学建模优秀论文的范文,供您参考:论文题目:基于神经网络的图像分类模型设计与实现引言:随着人工智能技术的快速发展,图像分类成为了重要的研究领域之一。
图像分类是将输入的图像分类到不同的类别中,是计算机视觉领域中的基础性问题。
近年来,神经网络在图像分类领域取得了显著的进展。
本文旨在设计并实现一个基于神经网络的图像分类模型,以提高图像分类的准确度和鲁棒性。
问题分析:图像分类是一个多分类问题,需要将输入的图像映射到不同的类别中。
传统的方法通常基于手工特征和分类器,但是这些方法在面对复杂的图像时往往表现出局限性。
神经网络作为一种自适应的学习方法,能够自动地从数据中学习特征表示,从而在图像分类领域取得了良好的效果。
模型建立:本文所设计的模型是基于深度学习的卷积神经网络(CNN)架构。
模型主要由卷积层、池化层和全连接层组成。
卷积层用于从输入图像中提取特征,池化层用于降低特征的维度,全连接层用于将特征映射到不同的类别中。
模型的输入为一张图像,输出为该图像所属的类别。
模型求解:采用随机梯度下降(SGD)算法对模型进行优化。
损失函数选择交叉熵损失函数,以衡量模型预测结果与真实标签之间的差距。
在训练过程中,通过不断调整模型的参数,使得损失函数的值逐渐降低,从而提高模型的分类准确度和鲁棒性。
结果分析:通过对模型的训练和测试结果进行分析,发现所设计的模型在图像分类任务中取得了较高的准确度和鲁棒性。
与传统的图像分类方法相比,所设计的模型能够自动地从数据中学习特征表示,避免了手工特征设计的繁琐过程,并且在面对复杂的图像时具有更好的表现。
结论与展望:本文设计并实现了一个基于神经网络的图像分类模型,经过训练和测试,发现该模型在图像分类任务中具有较高的准确度和鲁棒性。
未来,可以进一步优化模型的架构和参数设置,以更好地适应不同类型的图像分类任务。
此外,还可以将该模型应用于其他相关领域,如目标检测、人脸识别等,以推动相关领域的发展。
- 1、下载文档前请自行甄别文档内容的完整性,平台不提供额外的编辑、内容补充、找答案等附加服务。
- 2、"仅部分预览"的文档,不可在线预览部分如存在完整性等问题,可反馈申请退款(可完整预览的文档不适用该条件!)。
- 3、如文档侵犯您的权益,请联系客服反馈,我们会尽快为您处理(人工客服工作时间:9:00-18:30)。
高等教育学费标准的研究摘要本文从搜集有关普通高等学校学费数据开始,从学生个人支付能力和学校办学利益获得能力两个主要方面出发,分别通过对这两个方面的深入研究从而制定出各自有关高等教育学费的标准,最后再综合考虑这两个主要因素,进一步深入并细化,从而求得最优解。
模块Ⅰ中,我们将焦点锁定在从学生个人支付能力角度制定合理的学费标准。
我们从选取的数据和相关资料出发,发现1996年《高等学校收费管理暂行办法》规定高等学校学费占生均教育培养的成本比例最高不得超过25%,而由数据得到图形可知,从2002年开始学费占教育经费的比例超过了25%,并且生均学费和人均GDP 的比例要远远超过美国的10%到15%。
由此可见,我国的学费的收取过高。
紧接着,我们从个人支付能力角度出发,研究GDP 和学费的关系。
并因此制定了修正参数,由此来获取生均学费的修正指标。
随后,我们分析了高校专业的相关系数,从个人支付能力角度,探讨高校收费与专业的关系,进一步得到了高校收费标准1i i y G R Q =。
在模块Ⅱ中,我们从学校办学利益获得能力出发,利用回归分析对学生应交的学杂费与教育经费总计、国家预算内教育经费、社会团体和公民个人办学经验、社会捐投资和其他费用的关系,发现学杂费与教育经费总计成正相关,与其他几项费用成负相关。
对此产生的数据验证分析符合标准。
然后,再根据专业相关系数来确定学校收取学费的标准。
从而,得到了学校办学利益的收费标准2i i i y y R =。
在模块Ⅲ中,为了获取最优解,我们综合了前面两个模块所制定的收费指标,并分别给予不同权系数,得到最终学费的表达式12i i C ay by =+。
然后,我们从学校收费指标的权系数b 考虑,利用神经网络得到的区域划分,根据不同区域而计算出的权系数b 的范围。
最终得到的表达式[]12345**(1)(1.0502 1.1959 1.3108 1.36360.7929)**b i i C R G Q b x x x x x R =-+----;由此便可得到综合学费标准C 的取值范围。
然后,我们随机选取了同一区域不同专业,并根据表达式计算这些专业的学费,结果发现对社会收益大,个人收益小的专业如地质学的学费范围为:3469.8~3506.3元之间;对社会收益小,个人收益大的专业如广告设计的学费范围为:7931.0~8014.5元之间。
与通常高校实现的一刀切政策有了明显的优点。
最后,我们从本论文研究方向考虑,为优化高校费用标准的制定提出参考意见,如建立反馈制度和特殊生补贴制度的建议。
【关键字】相关系数 回归模型 自组织竞争神经网络一、问题提出高等教育事关高素质人才培养、国家创新能力增强、和谐社会建设的大局,因此受到党和政府及社会各方面的高度重视和广泛关注。
高等教育的一个核心指标是培养质量,不同的学科、专业在设定不同的培养目标后,都需要有相应的经费来保证其质量。
高等教育属于非义务教育,在世界各地其经费都是由政府财政拨款、学校自筹、社会捐赠和学费收入等几部份组成的。
世纪之交和“十五”期间,对我国高等教育制度进行改革与发展,我国高等教育面临着大有作为的重要战略机遇期,也面临着新的挑战。
随着改革的进行,学费问题也面临着严重的矛盾。
学费问题涉及每一个大学生及其家庭,是一个敏感而又复杂的问题:由于中国的经济限制,中国的人均收入并不是太高,特别是一些偏远山区和西北部地区,若学费过高会是许多学生因为无力支付学费而辍学;若学费太低,会导致学校的财力不足以致无法保障教学质量。
因此,学费问题在近年来的各种媒体上都引起了热烈的讨论。
从中国的国情出发,收集诸如近几年来关于我国教育经费方面的及家庭收入等数据[1-4],并通过分析数据建立数学模型,就几类学校或专业的费用标准进行定量分析,并从中得出明确、有说服力的结论。
二、问题分析(一)我国教育收费的现状通过国家统计局相关资料检索得到2000年到2005年我国普通高等学校教育经费统计[5]如表2. 1所示:表2. 1 表2. 1 表2. 1 表2. 1 表2. 1 表2. 1 表2. 1表2. 1 2002~2005年我国普通高校教育经费情况(单位:万元)年份2000 2001 2002 2003 2004 2005 项目合计9133504 11665762 14878590 17543468 21297613 25502371国家财政性教育经5311854 6328004 7521463 8405779 9697909 10908369 费预算内教育经费5044173 6060683 7243459 8074148 9309882 10463734社会团体和公民个65941 181993 331363 603015 1121982 1801315 人办学经费社会捐资和集资办151828 172775 278253 256375 215440 210796 学经费学费和杂费1926109 2824417 3906526 5057307 6476921 7919249 其他教育经费1677772 2158574 2840985 3220992 3785362 4662641学费占教育经费的0.21 0.24 0.26 0.29 0.30 0.31比例以表2. 1所示数据中的年份为横坐标,学费占教育经费的比例为纵坐标,利用MATLAB作图得到图2. 1 各年度学费占教育经费的比例的情况图2. 1 各年度学费占教育经费的比例的情况1996年12月16日颁布的《高等学校收费管理暂行办法》规定在现阶段,高等学校学费占年生均教育培养成本的比例最高不得超过25%。
[6]鉴于用于计算生均培养成本的相关数据的搜集工作难度系数较大,我们借用全国高校总的学费收入和总的教育经费的比值来表示高校学费占年生均教育培养成本的比例。
正如图2. 1 各年度学费占教育经费的比例的情况(二)影响我国普通高校学费标准制定的因素我们若想要具体确定学费标准,首先必须要搞清楚到底有哪些因素会影响学费标准的制定。
影响高等教育学费的因素是很多的,包括政治因素、历史文化传统因素、思想观念因素、国际因素、经济因素等。
前四种因素主观性都比较强,很难量化,因此本文暂不多加考虑。
经济方面的影响因素当然也包括很多,不过本文中主要涉及四种,即各方面的承受能力、高等教育个人收益率、生均培养成本以及地区差异。
(三)我国普通高校学费标准制定的原则至于学费标准制定的原则,研究者已有不少,如王善迈认为教育投资负担的基本依据是收益原则和能力原则,学费制定则应坚持教育成本的一部分原则和多数居民可以承受原则,其实这二者是一致的,如多数居民可以承受原则也就是能力原则。
全国政协常委辜胜阻对此谈得比较全面。
他认为,合理的成本分担机制建设应坚持一下八条原则:第一,成本合理分担原则;第二、承受能力原则;第三,收费标准差别性原则;第四,办学投入多元化原则;第五,办学主体多元化原则;第六,高等学校成本核算管理原则;第七,政府投入到为原则;第八,保障教育公平性原则。
也有很多学者有一些其他的观点,不过绝大多数学者还是认为要以能力支付原则和利益获得这两个最基本的原则来制定学费。
接下来我们就是要根据这两个基本原则来制定我国普通高校学费标准。
所谓能力支付原则,是根据利益获得者的付款能力来确定负担主体及负担程度。
教育成本支出最终来源于国民收入,国民收入通过初次分配和再分配被各社会群体所占有。
从理论上说,谁占有国民收入,谁就应当担负教育成本。
但是由于国民收入在分配上存在着不均等的现象,各群体的付款能力不同,教育成本的负担应该根据付款能力不同确定负担的程度与比例。
所谓利益获得原则,简言之,谁受益谁负担,获益多者多负担。
用于教育的成本支出就其性质而言是一种可获得预期收益的投资。
由于教育具有经济功能,用于教育的成本是可以获得预期的经济和非经济收益的一种投资。
由于教育的公共物品或准公共物品的特性,教育投资可以产生外部效益,不仅受教育者可以获益,全社会都可以从中获益。
因而社会各成员应根据其所获得的利益,分摊教育成本的负担。
三、 模型假设1、 假设收集的数据均真实有效。
2、 假设不考虑第三批本科国家不给予补助;3、 因为《中国统计年鉴》公布有关高等教育数据的滞后性,我们假设选取2005年的数据对本论文不构成影响。
四、 定义与符号说明G —— 人均GDPQ —— 人均GDP 的权重系数i R —— 各专业的权重系数i y —— 各专业学费的收费标准1x —— 国家财政性教育经费2x —— 预算内教育经费3x —— 社会团体和公民个人办学经费4x —— 社会捐款和集资办学经费5x —— 其他教育经费i b —— i x 相对应的系数C —— 学费制定标准1i y —— 学生期望的各专业学费标准y——学校期望的各专业学费标准2iy的权重系数a——1iy的权重系数b——2i五、模型的建立与求解(一)基于学生个人支付能力能力制定的学费标准——模块Ⅰ1、模型的分析首先,就学生家庭的经济承受能力这个角度来看,基于能力支付和利益获得这两个基本原则,我们从影响普通高校学费能力的众多因素中选取全国人均GDP和学生就读的专业这两个基本因素进行研究,通过建立相关模型确定这两个因素的权重系数,2、模型的准备根据查询国家统计局显示的数据资料[7],我们搜集到从1995年到2004年间我国普通高校生均学费和人均GDP的值如错误!未找到引用源。
表2. 1 表5. 1(1)人均GDP的权重系数Q我们根据国内和国外的高校学费占人均GDP的比例各自所占的权重系数,求出我国高校学费占人均GDP的一般比例,从而根据我国的人均GDP算出我国所有普通高校专业的平均学费。
根据错误!未找到引用源。
所列的数据,以各个年份为横坐标,普通高校生均学费和人均GDP的比值为纵坐标作图如图2. 1各年度学费占教育经费的比例的情况错误!未找到引用源。
图5. 1 各年度高校生均学费与人均GDP比值的变化情况很显然,由表2. 1可以看出,我国普通高校生均学费与人均GDP的比值总体上呈不断上升的趋势,1995年~1998年增长幅度较小(范围在0.20~0.30之内),1998年以后出现猛烈增长,两年之内从0.28突增至0.50,之后两年基本稳定,2002到2004年内又有小范围的滑落,但整体上一直处于高水平状态,保持再0.45以上。
1999年6月24日,教育部和国家计委联合宣布,1999年普通高校招生从上年的108万人扩大到156万人,增幅高达44.44﹪,中国高校大规模的扩招从此拉开了帷幕。
然而这个政策的推行却给民众带来了极大的困扰,扩招后学费高得离谱,令人难以接受。
从1995年到2004年,我国高校生年人均学费从一千元上涨到了五千元左右,而人均GDP在1995年至今的十年间增长不过一倍多。