第四章计量经济学答案范文
【VIP专享】计量经济学第四章练习题及参考解答

(2) 3.060 1.657ln() 1.057ln()
(0.337) (0.092) (0.215)0.992 0.991 F 1275.093
GDP CPI R =-+-===进口居民消费价格指数的回归系数的符号不能进行合理的经济意义解释可能数据中有多重共线性。
计算相关系数:
22ln Y 4.09071.2186ln () t= (-10.6458) (34.6222)
0.9828 0.9820 1198.698
GDP R R F =-+===ln Y 5.4424 2.6637ln (PI)C =-+
从修正的可决系数和F统计量可以看出,全部变量对数线性多元回归整体对样本拟合很好,著。
可是其中的lnX3、lnX4、lnX6对lnY影响不显著,而且lnX2、lnX5
可以看出lnx1与lnx2、lnx3、lnx4、lnx5、lnx6之间高度相关,许多相关系数高于作为解释变量,很可能会出现严重多重共线性问题。
在本章开始的“引子”提出的“农业的发展反而会减少财政收入吗?
表4.13 1978-2007
财政收入(亿元)CS农业增加值(亿元)NZ工业增加值(亿元)GZ建筑业增加值
1132.31027.51607
1146.41270.21769.7
1159.91371.61996.5
1175.81559.52048.4
(1)根据样本数据得到各解释变量的样本相关系数矩阵如下:样本相关系数矩阵
解释变量之间相关系数较高,特别是农业增加值、工业增加值、建筑业增加值、最终消费之间,相关系数都在这显然与第三章对模型的无多重共线性假定不符合。
(完整word版)计量经济学第四章习题详解

第四章习题4.1 没有进行t检验,并且调整的可决系数也没有写出来,也就是没有考虑自由度的影响,会使结果存在误差.4.3200224430.3120332。
7 330.6200334195。
6135822.8 334。
6200446435.8159878.3 l347.7200554273.7183084.8 353.9200663376.9211923。
5 359。
2200773284。
6249529。
9 376.5200879526.5314045.4 398.7200968618。
4340902。
8 395。
9201094699.3401512.8 408。
92011113161.4472881.6 431.0一研究的目的和要求我们知道,商品进口额与很多因素有关,了解其变化对进出口产品有很大帮助。
为了探究和预测商品进口额的变化,需要定量地分析影响商品进口额变化的主要因素。
二、模型的设定及其估计经分析,商品进口额可能与国内生产总值、居民消费价格指数有关。
为此,考虑国内生产总值GDP、居民消费价格指数CPI为主要因素。
各影响变量与商品进口额呈正相关。
为此,设定如下形式的计量经济模型:=+ln+lnCP式中,亿元);lnGDP为国内生产总值(亿元);lnCPI为居民消费价格指数(以1985年为100)。
各解释变量前的回归系数预期都大于零。
为估计模型,根据上表的数据,利用EViews软件,生成Y、lnGDP、lnCPI等数据,采用OLS方法估计模型参数,得到的回归结果如下图所示:模型方程为:lnY=-3。
111486+1。
338533lnGDP-0.421791lnCPI(0。
463010)(0。
088610)(0。
233295)t= (—6。
720126) (15。
10582)(—1。
807975)=0.988051 =0.987055 F=992。
2582该模型=0.988051,=0。
987055,可决系数很高,F检验值为992.2582,明显显著。
计量经济学庞皓第二版第四章答案

4.1(1) 存在3322ˆˆˆˆβγβα==且。
因为()()()()()()()23223223232322ˆ∑∑∑∑∑∑∑--=ii i i i i ii i i i x x x x x x x y x x y β 当32X X 与之间的相关系数为零时,离差形式的032=∑i i x x 有()()()()222223222322ˆˆαβ===∑∑∑∑∑∑i i ii i i i i x x y x x x x y 同理有:33ˆˆβγ= (2)会的。
(3) 存在()()()()3322ˆvar ˆvar ˆvar ˆvar γβαβ==且。
因为()()∑-=22322221ˆvar r x i σβ当023=r 时,()()()22222232222ˆvar 1ˆvar ασσβ==-=∑∑i i x r x 同理,有()()33ˆvar ˆvar γβ=4.3(1)参数估计结果如下: 093.1275F 991.0 992.0(-4.923) (17.967) (-9.069) ln 0571.1ln 6567.10601.3ˆln 22===-+-=R R CPI GDP Y t(2)数据中有多重共线性,居民消费价格指数的回归系数的符号不能进行合理的经济意义解释,且其简单相关系数呈现正向变动。
(3)分别拟合的回归模型如下:983.0(34.622)(-10.646) ln 187.1745.3ˆln 2=+-=R GDP Y867.0(11.681) (-4.341) PI ln 6638.24424.5ˆln 2=+-=R C Y931.0(16.814) (-1.958) ln 246.2438.1ˆln 2=+-=R CPI P DG单方程拟合效果都很好,回归系数显著,判定系数较高,GDP 和CPI 对进口的显著的单一影响,在这两个变量同时引入模型时影响方向发生了改变;GDP 对CPI 进行回归分析,回归系数显著,判定系数较高,说明GDP 和CPI 有很强的线性关系,这正是原模型多重共线性的原因。
计量经济学课后答案第四、五章(内容参考)
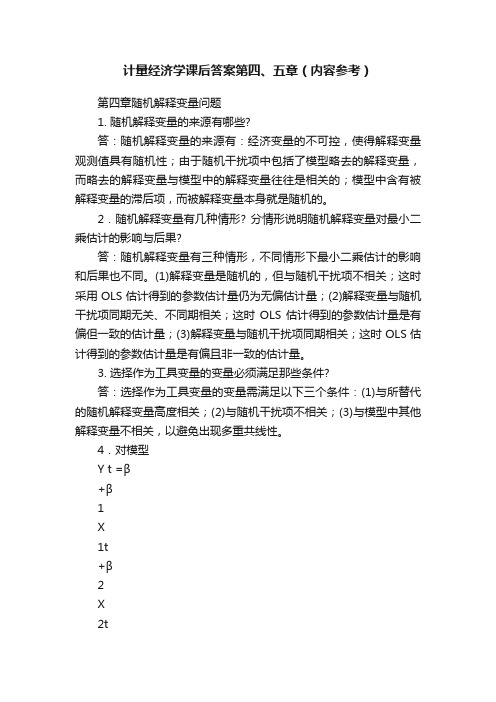
计量经济学课后答案第四、五章(内容参考)第四章随机解释变量问题1. 随机解释变量的来源有哪些?答:随机解释变量的来源有:经济变量的不可控,使得解释变量观测值具有随机性;由于随机干扰项中包括了模型略去的解释变量,而略去的解释变量与模型中的解释变量往往是相关的;模型中含有被解释变量的滞后项,而被解释变量本身就是随机的。
2.随机解释变量有几种情形? 分情形说明随机解释变量对最小二乘估计的影响与后果?答:随机解释变量有三种情形,不同情形下最小二乘估计的影响和后果也不同。
(1)解释变量是随机的,但与随机干扰项不相关;这时采用OLS估计得到的参数估计量仍为无偏估计量;(2)解释变量与随机干扰项同期无关、不同期相关;这时OLS估计得到的参数估计量是有偏但一致的估计量;(3)解释变量与随机干扰项同期相关;这时OLS估计得到的参数估计量是有偏且非一致的估计量。
3. 选择作为工具变量的变量必须满足那些条件?答:选择作为工具变量的变量需满足以下三个条件:(1)与所替代的随机解释变量高度相关;(2)与随机干扰项不相关;(3)与模型中其他解释变量不相关,以避免出现多重共线性。
4.对模型Y t =β+β1X1t+β2X2t+β3Yt-1+μt假设Yt-1与μt相关。
为了消除该相关性,采用工具变量法:先求Y t关于X1t与 X2t回归,得到Yt,再做如下回归:Y t =β+β1X1t+β2X2t+β3Y t?1-+μt试问:这一方法能否消除原模型中Yt的相关性? 为什么?解答:能消除。
在基本假设下,X1t,X2t与μt应是不相关的,由此知,由X1t 与X2t估计出的Yt应与μt不相关。
5.对于一元回归模型Y t =β+β1Xt*+μt假设解释变量Xt *的实测值Xt与之有偏误:Xt= Xt*+et,其中et是具有零均值、无序列相关,且与Xt不相关的随机变量。
试问:(1) 能否将X t= X t*+e t代入原模型,使之变换成Y t=β0+β1X t+νt后进行估计? 其中,νt为变换后模型的随机干扰项。
计量经济学第四章作业参考答案
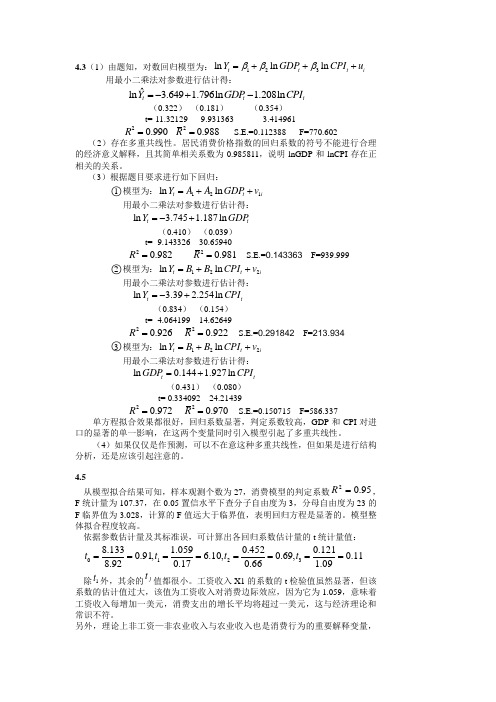
4.3(1)由题知,对数回归模型为:123ln ln ln t t t i Y G D P C PI u βββ=+++ 用最小二乘法对参数进行估计得:ˆl n 3.6491.796l n 1.208l nt tt Y G D P C P I =-+- (0.322) (0.181) (0.354)t=-11.32129 9.931363 -3.41496120.990R = 20.988R = S.E.=0.112388 F=770.602(2)存在多重共线性。
居民消费价格指数的回归系数的符号不能进行合理的经济意义解释,且其简单相关系数为0.985811,说明lnGDP 和lnCPI 存在正相关的关系。
(3)根据题目要求进行如下回归: ○1模型为:121ln ln t t i Y A A G D P v =++ 用最小二乘法对参数进行估计得: l n 3.7451.187l nt t Y G D P =-+ (0.410) (0.039) t= -9.143326 30.65940 20.982R = 20.981R = S.E.=0.143363 F=939.999 ○2模型为:122ln ln t t i Y B B C PI v =++用最小二乘法对参数进行估计得: l n 3.392.254l n t t Y CPI =-+(0.834) (0.154) t= -4.064199 14.62649 20.926R = 20.922R = S.E.=0.291842 F=213.934○3模型为:122ln ln tt i Y B B C PI v =++用最小二乘法对参数进行估计得:l n 0.1441.927l n t t GDP CPI =+ (0.431) (0.080)t= 0.334092 24.2143920.972R = 20.970R = S.E.=0.150715 F=586.337单方程拟合效果都很好,回归系数显著,判定系数较高,GDP 和CPI 对进口的显著的单一影响,在这两个变量同时引入模型引起了多重共线性。
计量经济学第4章课后答案

17CHAPTER 4SOLUTIONS TO PROBLEMS4.2 (i) and (iii) generally cause the t statistics not to have a t distribution under H 0.Homoskedasticity is one of the CLM assumptions. An important omitted variable violates Assumption MLR.3. The CLM assumptions contain no mention of the sample correlations among independent variables, except to rule out the case where the correlation is one.4.3 (i) While the standard error on hrsemp has not changed, the magnitude of the coefficient has increased by half. The t statistic on hrsemp has gone from about –1.47 to –2.21, so now the coefficient is statistically less than zero at the 5% level. (From Table G.2 the 5% critical value with 40 df is –1.684. The 1% critical value is –2.423, so the p -value is between .01 and .05.)(ii) If we add and subtract 2βlog(employ ) from the right-hand-side and collect terms, we havelog(scrap ) = 0β + 1βhrsemp + [2βlog(sales) – 2βlog(employ )] + [2βlog(employ ) + 3βlog(employ )] + u = 0β + 1βhrsemp + 2βlog(sales /employ ) + (2β + 3β)log(employ ) + u ,where the second equality follows from the fact that log(sales /employ ) = log(sales ) – log(employ ). Defining 3θ ≡ 2β + 3β gives the result.(iii) No. We are interested in the coefficient on log(employ ), which has a t statistic of .2, which is very small. Therefore, we conclude that the size of the firm, as measured by employees, does not matter, once we control for training and sales per employee (in a logarithmic functional form).(iv) The null hypothesis in the model from part (ii) is H 0:2β = –1. The t statistic is [–.951 – (–1)]/.37 = (1 – .951)/.37 ≈ .132; this is very small, and we fail to reject whether we specify a one- or two-sided alternative.4.4 (i) In columns (2) and (3), the coefficient on profmarg is actually negative, although its t statistic is only about –1. It appears that, once firm sales and market value have been controlled for, profit margin has no effect on CEO salary.(ii) We use column (3), which controls for the most factors affecting salary. The t statistic on log(mktval ) is about 2.05, which is just significant at the 5% level against a two-sided alternative.18(We can use the standard normal critical value, 1.96.) So log(mktval ) is statistically significant. Because the coefficient is an elasticity, a ceteris paribus 10% increase in market value is predicted to increase salary by 1%. This is not a huge effect, but it is not negligible, either.(iii) These variables are individually significant at low significance levels, with t ceoten ≈ 3.11 and t comten ≈ –2.79. Other factors fixed, another year as CEO with the company increases salary by about 1.71%. On the other hand, another year with the company, but not as CEO, lowers salary by about .92%. This second finding at first seems surprising, but could be related to the “superstar” effect: firms that hire CEOs from outside the company often go after a small pool of highly regarded candidates, and salaries of these people are bid up. More non-CEO years with a company makes it less likely the person was hired as an outside superstar.4.7 (i) .412 ± 1.96(.094), or about .228 to .596.(ii) No, because the value .4 is well inside the 95% CI.(iii) Yes, because 1 is well outside the 95% CI.4.8 (i) With df = 706 – 4 = 702, we use the standard normal critical value (df = ∞ in Table G.2), which is 1.96 for a two-tailed test at the 5% level. Now t educ = −11.13/5.88 ≈ −1.89, so |t educ | = 1.89 < 1.96, and we fail to reject H 0: educ β = 0 at the 5% level. Also, t age ≈ 1.52, so age is also statistically insignificant at the 5% level.(ii) We need to compute the R -squared form of the F statistic for joint significance. But F = [(.113 − .103)/(1 − .113)](702/2) ≈ 3.96. The 5% critical value in the F 2,702 distribution can be obtained from Table G.3b with denominator df = ∞: cv = 3.00. Therefore, educ and age are jointly significant at the 5% level (3.96 > 3.00). In fact, the p -value is about .019, and so educ and age are jointly significant at the 2% level.(iii) Not really. These variables are jointly significant, but including them only changes the coefficient on totwrk from –.151 to –.148.(iv) The standard t and F statistics that we used assume homoskedasticity, in addition to the other CLM assumptions. If there is heteroskedasticity in the equation, the tests are no longer valid.4.11 (i) Holding profmarg fixed, n rdintensΔ = .321 Δlog(sales ) = (.321/100)[100log()sales ⋅Δ] ≈ .00321(%Δsales ). Therefore, if %Δsales = 10, n rdintens Δ ≈ .032, or only about 3/100 of a percentage point. For such a large percentage increase in sales,this seems like a practically small effect.(ii) H 0:1β = 0 versus H 1:1β > 0, where 1β is the population slope on log(sales ). The t statistic is .321/.216 ≈ 1.486. The 5% critical value for a one-tailed test, with df = 32 – 3 = 29, is obtained from Table G.2 as 1.699; so we cannot reject H 0 at the 5% level. But the 10% criticalvalue is 1.311; since the t statistic is above this value, we reject H0 in favor of H1 at the 10% level.(iii) Not really. Its t statistic is only 1.087, which is well below even the 10% critical value for a one-tailed test.1920SOLUTIONS TO COMPUTER EXERCISESC4.1 (i) Holding other factors fixed,111log()(/100)[100log()](/100)(%),voteA expendA expendA expendA βββΔ=Δ=⋅Δ≈Δwhere we use the fact that 100log()expendA ⋅Δ ≈ %expendA Δ. So 1β/100 is the (ceteris paribus) percentage point change in voteA when expendA increases by one percent.(ii) The null hypothesis is H 0: 2β = –1β, which means a z% increase in expenditure by A and a z% increase in expenditure by B leaves voteA unchanged. We can equivalently write H 0: 1β + 2β = 0.(iii) The estimated equation (with standard errors in parentheses below estimates) isn voteA = 45.08 + 6.083 log(expendA ) – 6.615 log(expendB ) + .152 prtystrA(3.93) (0.382) (0.379) (.062) n = 173, R 2 = .793.The coefficient on log(expendA ) is very significant (t statistic ≈ 15.92), as is the coefficient on log(expendB ) (t statistic ≈ –17.45). The estimates imply that a 10% ceteris paribus increase in spending by candidate A increases the predicted share of the vote going to A by about .61percentage points. [Recall that, holding other factors fixed, n voteAΔ≈(6.083/100)%ΔexpendA ).] Similarly, a 10% ceteris paribus increase in spending by B reduces n voteAby about .66 percentage points. These effects certainly cannot be ignored.While the coefficients on log(expendA ) and log(expendB ) are of similar magnitudes (andopposite in sign, as we expect), we do not have the standard error of 1ˆβ + 2ˆβ, which is what we would need to test the hypothesis from part (ii).(iv) Write 1θ = 1β +2β, or 1β = 1θ– 2β. Plugging this into the original equation, and rearranging, givesn voteA = 0β + 1θlog(expendA ) + 2β[log(expendB ) – log(expendA )] +3βprtystrA + u ,When we estimate this equation we obtain 1θ≈ –.532 and se( 1θ)≈ .533. The t statistic for the hypothesis in part (ii) is –.532/.533 ≈ –1. Therefore, we fail to reject H 0: 2β = –1β.21C4.3 (i) The estimated model isn log()price = 11.67 + .000379 sqrft + .0289 bdrms (0.10) (.000043) (.0296)n = 88, R 2 = .588.Therefore, 1ˆθ= 150(.000379) + .0289 = .0858, which means that an additional 150 square foot bedroom increases the predicted price by about 8.6%.(ii) 2β= 1θ – 1501β, and solog(price ) = 0β+ 1βsqrft + (1θ – 1501β)bdrms + u= 0β+ 1β(sqrft – 150 bdrms ) + 1θbdrms + u .(iii) From part (ii), we run the regressionlog(price ) on (sqrft – 150 bdrms ), bdrms ,and obtain the standard error on bdrms . We already know that 1ˆθ= .0858; now we also getse(1ˆθ) = .0268. The 95% confidence interval reported by my software package is .0326 to .1390(or about 3.3% to 13.9%).C4.5 (i) If we drop rbisyr the estimated equation becomesn log()salary = 11.02 + .0677 years + .0158 gamesyr (0.27) (.0121) (.0016)+ .0014 bavg + .0359 hrunsyr (.0011) (.0072)n = 353, R 2= .625.Now hrunsyr is very statistically significant (t statistic ≈ 4.99), and its coefficient has increased by about two and one-half times.(ii) The equation with runsyr , fldperc , and sbasesyr added is22n log()salary = 10.41 + .0700 years + .0079 gamesyr(2.00) (.0120) (.0027)+ .00053 bavg + .0232 hrunsyr (.00110) (.0086)+ .0174 runsyr + .0010 fldperc – .0064 sbasesyr (.0051) (.0020) (.0052) n = 353, R 2 = .639.Of the three additional independent variables, only runsyr is statistically significant (t statistic = .0174/.0051 ≈ 3.41). The estimate implies that one more run per year, other factors fixed,increases predicted salary by about 1.74%, a substantial increase. The stolen bases variable even has the “wrong” sign with a t statistic of about –1.23, while fldperc has a t statistic of only .5. Most major league baseball players are pretty good fielders; in fact, the smallest fldperc is 800 (which means .800). With relatively little variation in fldperc , it is perhaps not surprising that its effect is hard to estimate.(iii) From their t statistics, bavg , fldperc , and sbasesyr are individually insignificant. The F statistic for their joint significance (with 3 and 345 df ) is about .69 with p -value ≈ .56. Therefore, these variables are jointly very insignificant.C4.7 (i) The minimum value is 0, the maximum is 99, and the average is about 56.16. (ii) When phsrank is added to (4.26), we get the following:n log() wage = 1.459 − .0093 jc + .0755 totcoll + .0049 exper + .00030 phsrank (0.024) (.0070) (.0026) (.0002) (.00024)n = 6,763, R 2 = .223So phsrank has a t statistic equal to only 1.25; it is not statistically significant. If we increase phsrank by 10, log(wage ) is predicted to increase by (.0003)10 = .003. This implies a .3% increase in wage , which seems a modest increase given a 10 percentage point increase in phsrank . (However, the sample standard deviation of phsrank is about 24.)(iii) Adding phsrank makes the t statistic on jc even smaller in absolute value, about 1.33, but the coefficient magnitude is similar to (4.26). Therefore, the base point remains unchanged: the return to a junior college is estimated to be somewhat smaller, but the difference is not significant and standard significant levels.(iv) The variable id is just a worker identification number, which should be randomly assigned (at least roughly). Therefore, id should not be correlated with any variable in the regression equation. It should be insignificant when added to (4.17) or (4.26). In fact, its t statistic is about .54.23C4.9 (i) The results from the OLS regression, with standard errors in parentheses, aren log() psoda =−1.46 + .073 prpblck + .137 log(income ) + .380 prppov (0.29) (.031) (.027) (.133)n = 401, R 2 = .087The p -value for testing H 0: 10β= against the two-sided alternative is about .018, so that we reject H 0 at the 5% level but not at the 1% level.(ii) The correlation is about −.84, indicating a strong degree of multicollinearity. Yet eachcoefficient is very statistically significant: the t statistic for log()ˆincome β is about 5.1 and that forˆprppovβ is about 2.86 (two-sided p -value = .004).(iii) The OLS regression results when log(hseval ) is added aren log() psoda =−.84 + .098 prpblck − .053 log(income ) (.29) (.029) (.038) + .052 prppov + .121 log(hseval ) (.134) (.018)n = 401, R 2 = .184The coefficient on log(hseval ) is an elasticity: a one percent increase in housing value, holding the other variables fixed, increases the predicted price by about .12 percent. The two-sided p -value is zero to three decimal places.(iv) Adding log(hseval ) makes log(income ) and prppov individually insignificant (at even the 15% significance level against a two-sided alternative for log(income ), and prppov is does not have a t statistic even close to one in absolute value). Nevertheless, they are jointly significant at the 5% level because the outcome of the F 2,396 statistic is about 3.52 with p -value = .030. All of the control variables – log(income ), prppov , and log(hseval ) – are highly correlated, so it is not surprising that some are individually insignificant.(v) Because the regression in (iii) contains the most controls, log(hseval ) is individually significant, and log(income ) and prppov are jointly significant, (iii) seems the most reliable. It holds fixed three measure of income and affluence. Therefore, a reasonable estimate is that if the proportion of blacks increases by .10, psoda is estimated to increase by 1%, other factors held fixed.。
计量经济学第四章练习题及参考解答

第四章练习题及参考解答4.1 假设在模型i i i i u X X Y +++=33221βββ中,32X X 与之间的相关系数为零,于是有人建议你进行如下回归:ii i i i i u X Y u X Y 23311221++=++=γγαα(1)是否存在3322ˆˆˆˆβγβα==且?为什么? (2)111ˆˆˆβαγ会等于或或两者的某个线性组合吗? (3)是否有()()()()3322ˆvar ˆvar ˆvar ˆvar γβαβ==且?练习题4.1参考解答:(1) 存在3322ˆˆˆˆβγβα==且。
因为()()()()()()()23223223232322ˆ∑∑∑∑∑∑∑--=iiiii iii iii x x x x x x x y x x y β当32X X 与之间的相关系数为零时,离差形式的032=∑i ix x有()()()()222223222322ˆˆαβ===∑∑∑∑∑∑iiiiiiii xx y x x x x y 同理有:33ˆˆβγ= (2) 111ˆˆˆβαγ会等于或的某个线性组合 因为 12233ˆˆˆY X X βββ=--,且122ˆˆY X αα=-,133ˆˆY X γγ=- 由于3322ˆˆˆˆβγβα==且,则 11222222ˆˆˆˆˆY Y X Y X X αααββ-=-=-= 11333333ˆˆˆˆˆY Y X Y X X γγγββ-=-=-= 则 1112233231123ˆˆˆˆˆˆˆY Y Y X X Y X X Y X X αγβββαγ--=--=--=+- (3) 存在()()()()3322ˆvar ˆvar ˆvar ˆvar γβαβ==且。
因为()()∑-=22322221ˆvar r x iσβ当023=r 时,()()()22222232222ˆvar 1ˆvar ασσβ==-=∑∑iixr x 同理,有()()33ˆvar ˆvar γβ=4.2在决定一个回归模型的“最优”解释变量集时人们常用逐步回归的方法。
《计量经济学》第四章精选题及答案

第四章:多重共线性二、简答题1、导致多重共线性的原因有哪些?2、多重共线性为什么会使得模型的预测功能失效?3、如何利用辅回归模型来检验多重共线性?4、判断以下说法正确、错误,还是不确定?并简要陈述你的理由。
(1)尽管存在完全的多重共线性,OLS 估计量还是最优线性无偏估计量(BLUE )。
(2)在高度多重共线性的情况下,要评价一个或者多个偏回归系数的个别显著性是不可能的。
(3)如果某一辅回归显示出较高的2i R 值,则必然会存在高度的多重共线性。
(4)变量之间的相关系数较高是存在多重共线性的充分必要条件。
(5)如果回归的目的仅仅是为了预测,则变量之间存在多重共线性是无害的。
5、考虑下面的一组数据:12233i i i Y X X βββ=++来对以上数据进行拟合回归。
(1) 我们能得到这3个估计量吗?并说明理由。
(2) 如果不能,那么我们能否估计得到这些参数的线性组合?可以的话,写出必要的计算过程。
6、考虑以下模型:231234i i i i i Y X X X ββββμ=++++由于2X 和3X 是X 的函数,那么它们之间存在多重共线性。
这种说法对吗?为什么? 7、在涉及时间序列数据的回归分析中,如果回归模型不仅含有解释变量的当前值,同时还含有它们的滞后值,我们把这类模型称为分布滞后模型(distributed-lag model )。
我们考虑以下模型:12313233i t t t t t Y X X X X βββββμ---=+++++其中Y ——消费,X ——收入,t ——时间。
该模型表示当期的消费是其现期的收入及其滞后三期的收入的线性函数。
(1) 在这一类模型中是否会存在多重共线性?为什么? (2) 如果存在多重共线性的话,应该如何解决这个问题? 8、设想在模型12233i i i iY X X βββμ=+++中,2X 和3X 之间的相关系数23r 为零。
如果我们做如下的回归:1221i i i Y X ααμ=++ 1332i i i Y X γγμ=++(1)会不会存在22ˆˆαβ=且33ˆˆγβ=?为什么? (2)1ˆβ会等于1ˆα或1ˆγ或两者的某个线性组合吗? (3)会不会有22ˆˆvar()var()βα=且33ˆˆvar()var()γβ=? 9、通过一些简单的计量软件(比如EViews 、SPSS ),我们可以得到各变量之间的相关矩阵:2323232311 1k k k k r r r r R r r ⎛⎫ ⎪ ⎪= ⎪ ⎪ ⎪⎝⎭。
《计量经济学》第3章、第4章课后题答案
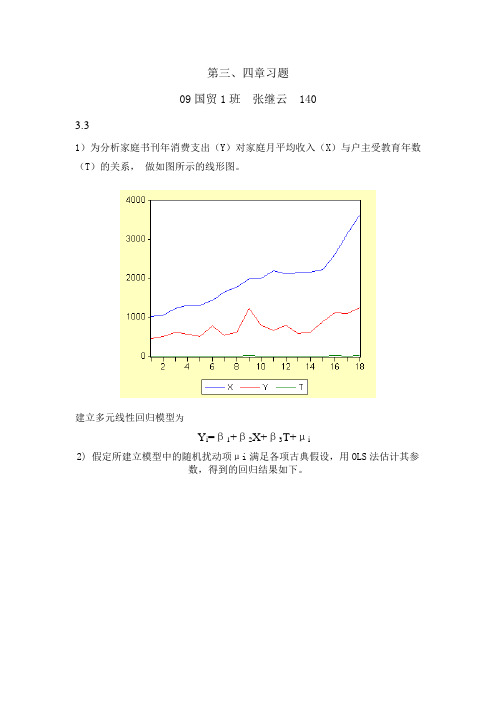
第三、四章习题09国贸1班张继云 1403.31)为分析家庭书刊年消费支出(Y)对家庭月平均收入(X)与户主受教育年数(T)的关系,做如图所示的线形图。
建立多元线性回归模型为Y i=β1+β2X+β3T+μi2) 假定所建立模型中的随机扰动项μi满足各项古典假设,用OLS法估计其参数,得到的回归结果如下。
可用规范形式将参数估计和检验结果写为Y = -50.01638+0.086450X+52.37031T(49.46026)(0.029363)(5.202167)t=(-1.011244)(2.944186)(10.06702)R2=0.951235 F=146.2974 n=183)对回归系数β3的t检验:针对H0:β3=0和H1:β3≠0,由回归结果中还可以看出,估计的回归系数β3的标准误差和t值分别为:SE(β3)= 5.202167, t(β3)= 10.6702。
当α=0.05时,查t分布表得自由度n-3=18-3=15的临界值t0.025(15)=2.131。
因为t(β1)= 10.6702> t0.025(16)=2.131,所以应该拒绝H0:β2=0。
这表明户主受教育年数对家庭书刊年消费支出有显著性影响。
4)所估计的模型的经济意义是当户主受教育年数保持不变时,家庭月平均收入每增加一元时将导致家庭书刊年消费支出增加0.086450元。
而当家庭月平均收入保持不变时,户主受教育年数每增加一年时将导致家庭书刊年消费支出增加52.37031元。
此模型可用于预测将来的家庭书刊年消费支出。
4.31)假定所建立模型中的随机扰动项μi满足各项古典假设,用OLS法估计其参数,得到的回归结果如下。
可用规范形式将参数估计和检验结果写为LnY t = -3.060638+1.056682lnGDP t-1.656536lnCPI t(0.337331)(0.092174) (0.214570)t = (-9.073096) (17.97182) (-4.924656)R2=0.992222 F=1275.739 n=232)数据中有多重共线性,居民消费价格指数的回归系数的符号不能进行合理的经济意义解释,且其简单相关系数呈现正向变动。
计量经济学练习题答案第四章
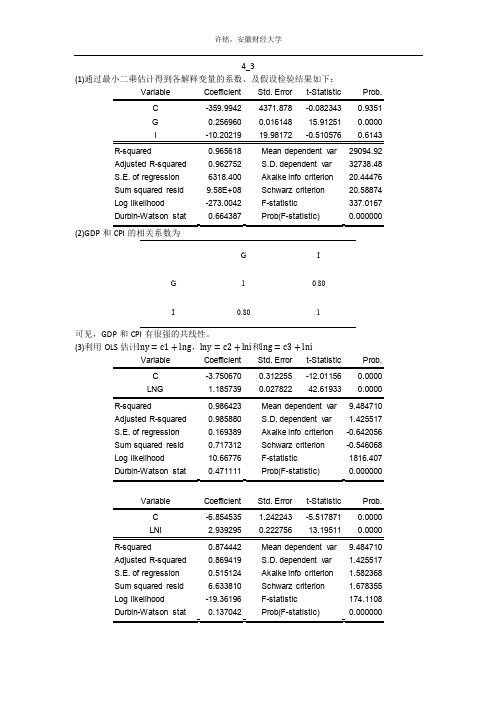
4_3(1)通过最小二乘估计得到各解释变量的系数、及假设检验结果如下:C -359.9942 4371.878 -0.082343 0.9351G 0.256960 0.016148 15.91251 0.0000R-squared 0.965618 Mean dependent var 29094.92Adjusted R-squared 0.962752 S.D. dependent var 32738.48S.E. of regression 6318.400 Akaike info criterion 20.44476Sum squared resid 9.58E+08 Schwarz criterion 20.58874Log likelihood -273.0042 F-statistic 337.0167Durbin-Watson stat 0.664387 Prob(F-statistic) 0.000000(2)GDP和CPI的相关系数为G IG 1 0.80I 0.80 1可见,GDP和CPI有很强的共线性。
(3)利用OLS估计lny=c1+lng,lny=c2+lni和lng=c3+lniC -3.750670 0.312255 -12.01156 0.0000R-squared 0.986423 Mean dependent var 9.484710Adjusted R-squared 0.985880 S.D. dependent var 1.425517S.E. of regression 0.169389 Akaike info criterion -0.642056Sum squared resid 0.717312 Schwarz criterion -0.546068Log likelihood 10.66776 F-statistic 1816.407Durbin-Watson stat 0.471111 Prob(F-statistic) 0.000000Variable Coefficient Std. Error t-Statistic Prob.C -6.854535 1.242243 -5.517871 0.0000LNI 2.939295 0.222756 13.19511 0.0000R-squared 0.874442 Mean dependent var 9.484710Adjusted R-squared 0.869419 S.D. dependent var 1.425517S.E. of regression 0.515124 Akaike info criterion 1.582368Sum squared resid 6.633810 Schwarz criterion 1.678355Log likelihood -19.36196 F-statistic 174.1108C -2.796381 0.882798 -3.167634 0.0040LNI 2.511022 0.158302 15.86227 0.0000 R-squared 0.909621 Mean dependent var 11.16214Adjusted R-squared 0.906005 S.D. dependent var 1.194029S.E. of regression 0.366072 Akaike info criterion 0.899213Sum squared resid 3.350216 Schwarz criterion 0.995201Log likelihood -10.13938 F-statistic 251.6117Durbin-Watson stat 0.099623 Prob(F-statistic) 0.000000 Lny、lng和lni的相关系数如下:LNG LNI LNYLNG 1 0.953740317415 0.993188514728LNI 0.953740317415 1 0.935115922429LNY 0.993188514728 0.935115922429 14_4利用OLS估计参数,结果如下:C -221.8540 130.6532 -1.698038 0.1030CZZC 0.090114 0.044367 2.031129 0.0540GDP -0.025334 0.005069 -4.998036 0.0000R-squared 0.999857 Mean dependent var 22572.56Adjusted R-squared 0.999838 S.D. dependent var 27739.49S.E. of regression 353.0540 Akaike info criterion 14.70707Sum squared resid 2866884. Schwarz criterion 14.89905Log likelihood -194.5455 F-statistic 53493.93Durbin-Watson stat 1.458128 Prob(F-statistic) 0.000000 为计税依据所以GDP越大,税收也会越多。
计量经济学第四章课后习题
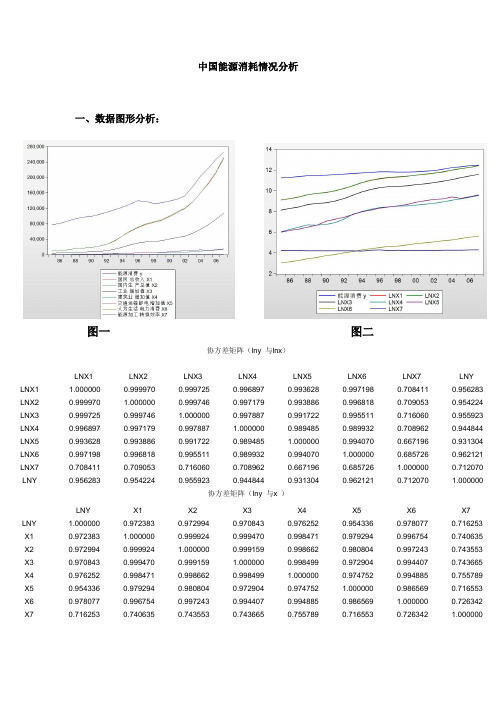
中国能源消耗情况分析一、数据图形分析:图一 图二协方差矩阵(lny 与lnx )LNX1 LNX2 LNX3 LNX4 LNX5 LNX6 LNX7 LNY LNX1 1.000000 0.999970 0.999725 0.996897 0.993628 0.997198 0.708411 0.956283 LNX2 0.999970 1.000000 0.999746 0.997179 0.993886 0.996818 0.709053 0.954224 LNX3 0.999725 0.999746 1.000000 0.997887 0.991722 0.995511 0.716060 0.955923 LNX4 0.996897 0.997179 0.997887 1.000000 0.989485 0.989932 0.708962 0.944844 LNX5 0.993628 0.993886 0.991722 0.989485 1.000000 0.994070 0.667196 0.931304 LNX6 0.997198 0.996818 0.995511 0.989932 0.994070 1.000000 0.685726 0.962121 LNX7 0.708411 0.709053 0.716060 0.708962 0.667196 0.685726 1.000000 0.712070 LNY0.9562830.9542240.9559230.9448440.9313040.9621210.7120701.000000协方差矩阵(lny 与x )LNY X1 X2 X3 X4 X5 X6 X7 LNY 1.000000 0.972383 0.972994 0.970843 0.976252 0.954336 0.978077 0.716253 X1 0.972383 1.000000 0.999924 0.999470 0.998471 0.979294 0.996754 0.740635 X2 0.972994 0.999924 1.000000 0.999159 0.998662 0.980804 0.997243 0.743553 X3 0.970843 0.999470 0.999159 1.000000 0.998499 0.972904 0.994407 0.743665 X4 0.976252 0.998471 0.998662 0.998499 1.000000 0.974752 0.994885 0.755789 X5 0.954336 0.979294 0.980804 0.972904 0.974752 1.000000 0.986569 0.716553 X6 0.978077 0.996754 0.997243 0.994407 0.994885 0.986569 1.000000 0.726342 X70.716253 0.7406350.7435530.7436650.7557890.7165530.7263421.000000分析:将录入Eviews的数据Y,X1,X2,X3, X4,X5,X6,X7进行分析,通过每组数据随时间变化趋势可以发现,这八组数据都是逐年增长的,但增长速率有所变动。
中级计量经济学-第四章-习题以及解答思路(EViews)

中级计量经济学-第四章-习题以及解答思路(EViews)第4章习题一表1给出了1965~1970年美国制造业利润和销售额的季度数据。
假定利润不仅与销售额有关,而且和季度因素有关。
要求对下列二种情况分别估计利润模型:(1)如果认为季度影响使利润平均值发生变异,应如何引入虚拟变量?(2)如果认为季度影响使利润对销售额的变化率发生变异,如何引入虚拟变量?表1Quarterly 65-70Quick- Equation EstimationY c x @seas(1) @seas(2) @seas(3)Dependent Variable: YMethod: Least SquaresDate: 11/26/14 Time: 18:38Sample: 1965Q1 1970Q4Included observations: 24Variable Coefficient Std. Error t-Statistic Prob.C6868.0151892.766 3.6285590.0018 X0.0382650.011483 3.3322520.0035 @SEAS(1)-182.1690654.3568-0.2783940.7837 @SEAS(2)1140.294630.6806 1.8080380.0865 @SEAS(3)-400.3371636.1128-0.6293490.5366R-squared0.525596Mean dependentvar12838.54Adjusted R-squared0.425721S.D. dependentvar1433.284S.E. of regression1086.160Akaike infocriterion17.00174Sum squared resid22415107Schwarz criterion17.24716 Log likelihood-199.0208F-statistic 5.262563Durbin-Watson stat0.388380Prob(F-statistic)0.005024T和P在5%情况下都不通过,第二季度相对还好一点假设第二季度显著,结果的经济含义是什么?Y c x @seas(2) @seas(3) @seas(4)Dependent Variable: YMethod: Least SquaresDate: 11/26/14 Time: 18:47Sample: 1965Q1 1970Q4Included observations: 24Variable Coefficient Std. Error t-Statistic Prob.C6685.8461711.618 3.9061550.0009 X0.0382650.0114833.3322520.0035 @SEAS(2)1322.463638.4258 2.0714440.0522 @SEAS(3)-218.1681632.1991-0.3450940.7338@SEAS(4)182.1690654.35680.2783940.7837R-squared0.525596Mean dependentvar12838.54Adjusted R-squared0.425721S.D. dependentvar1433.284S.E. of regression1086.160Akaike infocriterion17.00174Sum squared resid22415107Schwarz criterion17.24716 Log likelihood-199.0208F-statistic 5.262563Durbin-Watson stat0.388380Prob(F-statistic)0.005024第二季度依旧显著影响四种都试一下(去掉一个季节),选一个最显著的124Dependent Variable: YMethod: Least SquaresDate: 11/26/14 Time: 18:51Sample: 1965Q1 1970Q4Included observations: 24Variable Coefficient Std. Error t-Statistic Prob.C6467.6781789.178 3.6148880.0018 X0.0382650.011483 3.3322520.0035 @SEAS(1)218.1681632.19910.3450940.7338 @SEAS(2)1540.632628.3419 2.4519000.0241 @SEAS(4)400.3371636.11280.6293490.5366R-squared0.525596Mean dependentvar12838.54Adjusted R-squared0.425721S.D. dependentvar1433.284S.E. of regression1086.160Akaike infocriterion17.00174Sum squared resid22415107Schwarz criterion17.24716 Log likelihood-199.0208F-statistic 5.262563Durbin-Watson stat0.388380Prob(F-statistic)0.005024134Dependent Variable: Y Method: Least SquaresDate: 11/26/14 Time: 18:52 Sample: 1965Q1 1970Q4 Included observations: 24Variable Coefficient Std. Error t-Statistic Prob.C8008.3091827.543 4.3820090.0003 X0.0382650.011483 3.3322520.0035 @SEAS(1)-1322.463638.4258-2.0714440.0522 @SEAS(3)-1540.632628.3419-2.4519000.0241 @SEAS(4)-1140.294630.6806-1.8080380.0865R-squared0.525596Mean dependentvar12838.54Adjusted R-squared0.425721S.D. dependentvar1433.284S.E. of regression1086.160Akaike infocriterion17.00174Sum squared resid22415107Schwarz criterion17.24716 Log likelihood-199.0208F-statistic 5.262563Durbin-Watson stat0.388380Prob(F-statistic)0.005024(2)Y=c+βx+α1D1X+α2D2X+α3D3XD1=1(第一季度)0(其他)Y c x @seas(1)*x @seas(2)*x @seas(3)*xDependent Variable: Y Method: Least SquaresDate: 11/26/14 Time: 19:00 Sample: 1965Q1 1970Q4 Included observations: 24Variable Coefficient Std. Error t-Statistic Prob.C6965.8521753.642 3.9722200.0008 X0.0373630.011139 3.3542150.0033 @SEAS(1)*X-0.0008930.004259-0.2095880.8362 @SEAS(2)*X0.0077120.003962 1.9465020.0665 @SEAS(3)*X-0.0022910.004041-0.5669850.5774R-squared0.528942Mean dependentvar12838.54Adjusted R-squared0.429771S.D. dependentvar1433.284S.E. of regression1082.323Akaike infocriterion16.99466Sum squared resid22257030Schwarz criterion17.24009 Log likelihood-198.9359F-statistic 5.333675Durbin-Watson stat0.418713Prob(F-statistic)0.004722Dependent Variable: Y Method: Least SquaresDate: 11/26/14 Time: 19:10 Sample: 1965Q1 1970Q4 Included observations: 24Variable Coefficient Std. Error t-Statistic Prob.C8008.3091827.543 4.3820090.0003 X0.0382650.011483 3.3322520.0035 @SEAS(1)-1322.463638.4258-2.0714440.0522 @SEAS(3)-1540.632628.3419-2.4519000.0241 @SEAS(4)-1140.294630.6806-1.8080380.0865R-squared0.525596Mean dependentvar12838.54Adjusted R-squared0.425721S.D. dependent 1433.284varS.E. of regression1086.160Akaike infocriterion17.00174Sum squared resid22415107Schwarz criterion17.24716 Log likelihood-199.0208F-statistic 5.262563Durbin-Watson stat0.388380Prob(F-statistic)0.005024Dependent Variable: Y Method: Least SquaresDate: 11/26/14 Time: 19:11 Sample: 1965Q1 1970Q4 Included observations: 24Variable Coefficient Std. Error t-Statistic Prob.C6965.8521753.642 3.9722200.0008 X0.0350720.011790 2.9746750.0078 @SEAS(1)*X0.0013980.0042410.3297360.7452 @SEAS(2)*X0.0100030.004068 2.4588230.0237 @SEAS(4)*X0.0022910.0040410.5669850.5774R-squared0.528942Mean dependentvar12838.54Adjusted R-squared0.429771S.D. dependentvar1433.284S.E. of regression1082.323Akaike infocriterion16.99466Sum squared resid22257030Schwarz criterion17.24009 Log likelihood-198.9359F-statistic 5.333675Durbin-Watson stat0.418713Prob(F-statistic)0.004722Dependent Variable: Y Method: Least SquaresDate: 11/26/14 Time: 19:11 Sample: 1965Q1 1970Q4 Included observations: 24Variable Coefficient Std. Error t-Statistic Prob.C6965.8521753.642 3.9722200.0008 X0.0364710.012353 2.9524150.0082 @SEAS(2)*X0.0086040.004237 2.0305390.0565 @SEAS(3)*X-0.0013980.004241-0.3297360.7452@SEAS(4)*X0.0008930.0042590.2095880.8362R-squared0.528942Mean dependentvar12838.54Adjusted R-squared0.429771S.D. dependent 1433.284varS.E. of regression1082.323Akaike infocriterion16.99466Sum squared resid22257030Schwarz criterion17.24009 Log likelihood-198.9359F-statistic 5.333675Durbin-Watson stat0.418713Prob(F-statistic)0.004722。
第四章练习题及参考解答(第四版)计量经济学

第四章练习题及参考解答4.1 假设在模型i i i i u X X Y +++=33221βββ中,32X X 与之间的相关系数为零,有人建议你分别进行如下回归:1221i i i Y X u αα=++ 1332i i i Y X u γγ=++(1) 是否存在3322ˆˆˆˆβγβα==且?为什么? (2) 1ˆβ会等于1ˆα或1ˆγ或者两者的某个线性组合吗? (3) 是否有()()22ˆˆVar Var βα=且()()33ˆˆVar Var βγ=?【练习题4.1参考解答】(1) 存在2233ˆˆˆˆαβγβ==且 。
因为 ()()()()()()()22332322222323ˆi iii ii iiii iy x x y x x xx x x x β-=-∑∑∑∑∑∑∑当23X X 与 之间的相关系数为零时,离差形式的230i ixx =∑有 ()()()()223222222223ˆˆi i i i i iiiy x x y x xx x βα===∑∑∑∑∑∑ 同理有: 33ˆˆγβ= (2)会的。
(3) 存在 ()()()()2233ˆˆˆˆvar var var var βαβγ==且 因为 ()()2222223ˆvar 1ix r σβ=-∑当 230r = 时, ()()()22222222223ˆˆvar var 1iix x r σσβα===-∑∑ 同理,有 ()()33ˆˆvar var βγ=4.2 表4.4给出了1995—2016年中国商品进口额Y 、国内生产总值GDP 、居民消费价格指数CPI 的数据。
表4.4 中国商品进口额、国内生产总值、居民消费价格指数资料来源:《中国统计年鉴2017》考虑建立模型: i t t t u CPI GDP Y ++=ln ln ln 321βββ+ (1)利用表中数据估计此模型的参数。
(2)你认为数据中有多重共线性吗?(3)进行以下回归:121ln ln t t i Y A A GDP v =++ 122ln ln t t i Y B B CPI v =++ 123ln ln t t i GDP C C CPI v =++ 根据这些回归你能对多重共线性的性质有什么认识?(4)假设经检验数据有多重共线性,但模型中32ˆˆββ和在5%水平上显著,并且F 检验也显著,你对此模型的应用有何建议?【练习题4.2参考解答】建立模型: i t t t u CPI GDP Y ++=ln ln ln 321βββ+ (1)利用表中数据估计此模型的参数。
计量经济学第四章习题详解

第四章习题没有进行t检验,并且调整的可决系数也没有写出来,也就是没有考虑自由度的影响,会使结果存在误差。
一研究的目的和要求我们知道,商品进口额与很多因素有关,了解其变化对进出口产品有很大帮助。
为了探究和预测商品进口额的变化,需要定量地分析影响商品进口额变化的主要因素。
二、模型的设定及其估计经分析,商品进口额可能与国内生产总值、居民消费价格指数有关。
为此,考虑国内生产总值GDP 居民消费价格指数CPI为主要因素。
各影响变量与商品进口额呈正相关。
为此,设定如下形式的计量经济模型:I ri¥仁B 1 + 021 n GOPt + D 釘nCpI ttJS'piftriCKnivana 朋eLNGDH 晩ri0 世 L 帥squares Dale MM 5/17 Trl^ : 21.09 盟mo 怙]£852011 induded obsefvauons: 2?Coeffrdent SW Error l-3t3tis(ic Frplj.CLNCFI-2.796331 0.062793 -3.157634OOO+O2.511022 0.155302lS&6i£70 05 JOR*iquared Adjusted 冏GD I 3-L. ofregrssaion Surnsquundr 卵 id L M likBiihcod F-st 前血O'. 3 0 5021 Mean dftp tn dent vaf 111G214 P 与S 啊的相爲数很高,证明存在多劇性。
3.3&C215 -1C.1cO33 25ll.e-117 Schwaizcrilcriort Hsnnan Ouinn alter. Du 『biiTWat$Qn $t 日tO.OOT5 & DepentlsntVariatle: LNCA hlethed: Least Sajares DdlK. 04/15^17 Time. 2110' Sdrritifr 19352011 IriClLdeO oas 加汕 27YRUKEllP CnsiriciPnrtsvt ErrorT^siat qtir PrebC 1.515402C.256313 5.912301 O.flODDLHC CPcm 遊 71&.S65S7do 越DR-squarftd0.90^21Meandependerttfar5.550900 Adju&ted R'SQLiarsd O.9DM0S O.D. depindenl 畑 3+53513 £.E. □ri'eur«ision 0 13M4: AkaiKe ink BL 曰ion-1.030394Sumsqjafecifesirt 01R3317M 備gIMf)-3 &4&A07 1 CiQ ・曲niXKl 阳HR 加sfvOLi 门 n cnT=r J OOfB 宓 F-stststc351.5117 □ Ljrtnir>-waisor Q 114553PrcitxF-gtatisttaO.ODCWOO式中,Y 伪第t 年中国商品进U 额(亿元);In GDP 为第t 坏国内生产总值(亿元);InCPI 为居民消费价格指 数(以1985年为100)。
计量经济学答案部分Word版
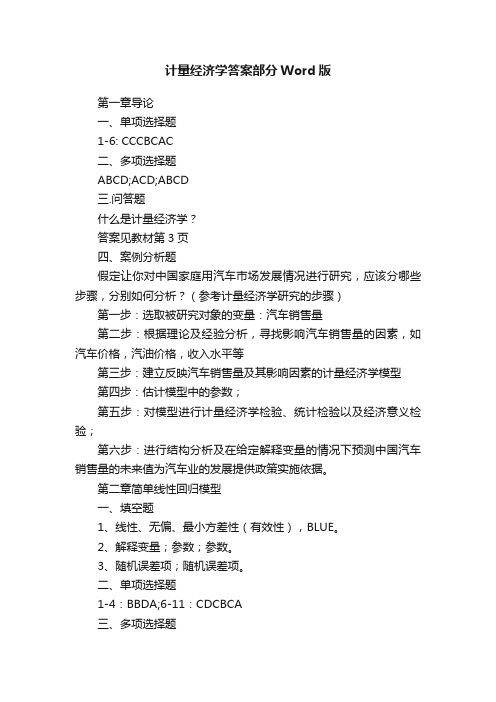
计量经济学答案部分Word版第一章导论一、单项选择题1-6: CCCBCAC二、多项选择题ABCD;ACD;ABCD三.问答题什么是计量经济学?答案见教材第3页四、案例分析题假定让你对中国家庭用汽车市场发展情况进行研究,应该分哪些步骤,分别如何分析?(参考计量经济学研究的步骤)第一步:选取被研究对象的变量:汽车销售量第二步:根据理论及经验分析,寻找影响汽车销售量的因素,如汽车价格,汽油价格,收入水平等第三步:建立反映汽车销售量及其影响因素的计量经济学模型第四步:估计模型中的参数;第五步:对模型进行计量经济学检验、统计检验以及经济意义检验;第六步:进行结构分析及在给定解释变量的情况下预测中国汽车销售量的未来值为汽车业的发展提供政策实施依据。
第二章简单线性回归模型一、填空题1、线性、无偏、最小方差性(有效性),BLUE。
2、解释变量;参数;参数。
3、随机误差项;随机误差项。
二、单项选择题1-4:BBDA;6-11:CDCBCA三、多项选择题1.ABC;2.ABC;3.BC;4.ABE;5.AD;6.BC四、判断正误:1. 错;2. 错;3. 对;4.错;5. 错;6. 对;7. 对;8.错五、简答题:1.为什么模型中要引入随机扰动项?答:模型是对经济问题的一种数学模型,在模型中,被解释变量是研究的对象,解释变量是其确定的解释因素,但由于实际问题的错综复杂,影响被解释变量的因素中,除了包括在模型中的解释变量以外,还有其他一些因素未能包括在模型中,但却影响被解释变量,我们把这类变量统一用随机误差项表示。
随机误差项包含的因素有:第一,未知影响因素的代表;第二,无法取得数据的已知因素的代表;第三,众多细小影响因素的综合代表;第四,模型的设定误差;第五,变量的观测误差;第六,经济现象的内在随机性。
由此可见,随机误差项有十分丰富的内容,在计量经济研究中起着重要的作用,一定程度上,随机误差项的性质决定着计量经济方法的选择和使用。
计量经济学庞皓第二版第四章习题答案(2020年10月整理).pdf
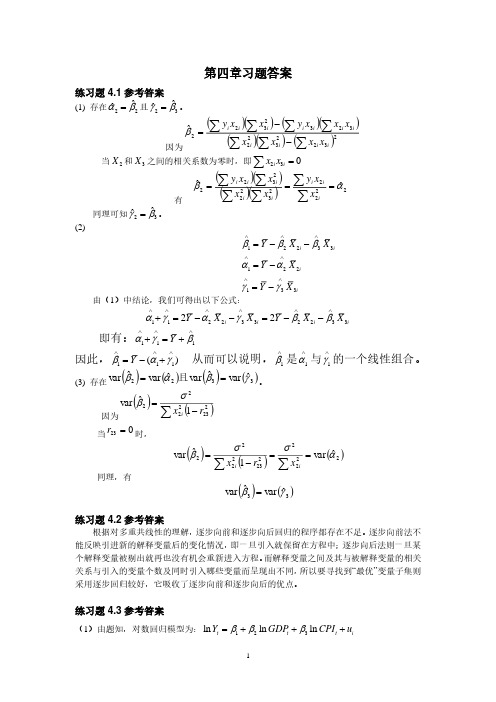
即有:
1
+
1
=Y
+
1
因此,
1
=Y
− (1+ 1)
从而可以说明,
1
是
1
与
1
的一个线性组合。
( ) ( ) (3) 存在 var ˆ2 = var(ˆ2 )且 var ˆ3 = var(ˆ3 )。
( ) var ˆ2 =
( ) 因为
2
x
2 2i
1−
r223
当 r23 = 0 时,
同理,有
( ) ( ) var ˆ2 =
( )( ) ˆ2 = ( )( ) 有
yi x2i x22i
x32i = x32i
yi x2i x22i
= ˆ 2
同理可知 ˆ2 = ˆ3 。
(2)
1 = Y − 2 X 2i − 3 X 3i
1 = Y − 2 X 2i
1 = Y − 3 X 3i
由(1)中结论,我们可得出以下公式:
1+ 1 = 2Y − 2 X 2i − 3 X 3i = 2Y − 2 X 2i − 3 X 3i
计值过大,该值为工资收入对消费边际效应,因为它为 1.059,意味着工资收入每增加一美 元,消费支出的增长平均将超过一美元,这与经济理论和常识不符。
另外,理论上非工资—非农业收入与农业收入也是消费行为的重要解释变量,但两者的
t 检验都没有通过。这些迹象表明,模型中存在严重的多重共线性,不同收入部分之间的相
0.1168 0.8057 0.4140 0.8547
4
X3-X3(-1) X2-X2(-1) X1-X1(-1)
C
0.116616 -10.92141 11.01442 2470.655
计量经济学课后习题答案

第一章1.计量经济学是一门什么样的学科?答:计量经济学的英文单词是Econometrics,本意是“经济计量”,研究经济问题的计量方法,因此有时也译为“经济计量学”。
将Econometrics译为“计量经济学”是为了强调它是现代经济学的一门分支学科,不仅要研究经济问题的计量方法,还要研究经济问题发展变化的数量规律。
可以认为,计量经济学是以经济理论为指导,以经济数据为依据,以数学、统计方法为手段,通过建立、估计、检验经济模型,揭示客观经济活动中存在的随机因果关系的一门应用经济学的分支学科。
2.计量经济学与经济理论、数学、统计学的联系和区别是什么?答:计量经济学是经济理论、数学、统计学的结合,是经济学、数学、统计学的交叉学科(或边缘学科)。
计量经济学与经济学、数学、统计学的联系主要是计量经济学对这些学科的应用。
计量经济学对经济学的应用主要体现在以下几个方面:第一,计量经济学模型的选择和确定,包括对变量和经济模型的选择,需要经济学理论提供依据和思路;第二,计量经济分析中对经济模型的修改和调整,如改变函数形式、增减变量等,需要有经济理论的指导和把握;第三,计量经济分析结果的解读和应用也需要经济理论提供基础、背景和思路。
计量经济学对统计学的应用,至少有两个重要方面:一是计量经济分析所采用的数据的收集与处理、参数的估计等,需要使用统计学的方法和技术来完成;一是参数估计值、模型的预测结果的可靠性,需要使用统计方法加以分析、判断。
计量经济学对数学的应用也是多方面的,首先,对非线性函数进行线性转化的方法和技巧,是数学在计量经济学中的应用;其次,任何的参数估计归根结底都是数学运算,较复杂的参数估计方法,或者较复杂的模型的参数估计,更需要相当的数学知识和数学运算能力,另外,在计量经济理论和方法的研究方面,需要用到许多的数学知识和原理。
计量经济学与经济学、数学、统计学的区别也很明显,经济学、数学、统计学中的任何一门学科,都不能替代计量经济学,这三门学科简单地合起来,也不能替代计量经济学。
- 1、下载文档前请自行甄别文档内容的完整性,平台不提供额外的编辑、内容补充、找答案等附加服务。
- 2、"仅部分预览"的文档,不可在线预览部分如存在完整性等问题,可反馈申请退款(可完整预览的文档不适用该条件!)。
- 3、如文档侵犯您的权益,请联系客服反馈,我们会尽快为您处理(人工客服工作时间:9:00-18:30)。
第四章一元线性回归第一部分学习目的和要求本章主要介绍一元线性回归模型、回归系数的确定和回归方程的有效性检验方法。
回归方程的有效性检验方法包括方差分析法、t检验方法和相关性系数检验方法。
本章还介绍了如何应用线性模型来建立预测和控制。
需要掌握和理解以下问题:1 一元线性回归模型2 最小二乘方法3 一元线性回归的假设条件4 方差分析方法5 t检验方法6 相关系数检验方法7 参数的区间估计8 应用线性回归方程控制与预测9 线性回归方程的经济解释第二部分练习题一、术语解释1 解释变量2 被解释变量3 线性回归模型4 最小二乘法5 方差分析6 参数估计7 控制8 预测二、填空ξ,目的在于使模型更1 在经济计量模型中引入反映()因素影响的随机扰动项t符合()活动。
2 在经济计量模型中引入随机扰动项的理由可以归纳为如下几条:(1)因为人的行为的()、社会环境与自然环境的()决定了经济变量本身的();(2)建立模型时其他被省略的经济因素的影响都归入了()中;(3)在模型估计时,()与归并误差也归入随机扰动项中;(4)由于我们认识的不足,错误的设定了()与()之间的数学形式,例如将非线性的函数形式设定为线性的函数形式,由此产生的误差也包含在随机扰动项中了。
3 ()是因变量离差平方和,它度量因变量的总变动。
就因变量总变动的变异来源看,它由两部分因素所组成。
一个是自变量,另一个是除自变量以外的其他因素。
()是拟合值的离散程度的度量。
它是由自变量的变化引起的因变量的变化,或称自变量对因变量变化的贡献。
()是度量实际值与拟合值之间的差异,它是由自变量以外的其他因素所致,它又叫残差或剩余。
4 回归方程中的回归系数是自变量对因变量的()。
某自变量回归系数β的意义,指的是该自变量变化一个单位引起因变量平均变化( )个单位。
5 模型线性的含义,就变量而言,指的是回归模型中变量的( );就参数而言,指的是回归模型中的参数的( );通常线性回归模型的线性含义是就( )而言的。
6 样本观察值与回归方程理论值之间的偏差,称为( ),我们用残差估计线性模型中的( )。
三、简答题1 在线性回归方程中,“线性”二字如何理解?2 用最小二乘法求线性回归方程系数的意义是什么?3 一元线性回归方程的基本假设条件是什么?4 方差分析方法把数据总的平方和分解成为两部分的意义是什么?5 试叙述t 检验法与相关系数检验法之间的联系。
6 应用线性回归方程控制和预测的思想。
7 线性回归方程无效的原因是什么?8 回归分析中的随机误差项i ε有什么作用?它与残差项t e 有何区别?9 判断如下模型,哪些是线性模型,哪些不是。
以及它们经过怎样的变化能够变成线性模型? 模型 描述性名称121.i i i a Y X ββε⎛⎫=++⎪⎝⎭倒数 12.ln i i i b Y X ββε=++ 半对数 12.ln i i i c Y X ββε=++ 反半对数 12.ln ln ln i i i c Y X ββε=++ 对数或双对数121.ln i i i c Y X ββε⎛⎫=-+ ⎪⎝⎭对数倒数10 如下模型是线性回归模型吗?并说出原因。
12.i i X i a Y e ββε++=121.1i ii X b Y e ββε++=+121.ln i i i c Y X ββε⎛⎫=++ ⎪⎝⎭()2211.(0.5)i X i i d Y eβββε--=+-+312.i i i e Y X ββε=++四 计算题1 给定如下表第一列的假设,说明第二列中的假定是与之等效的。
关于经典模型的假设共四个等级)、GMAT分数以及每年学费的数据。
a. 用双变量回归模型分析GPA是否对ASP有影响?b.用合适的回归模型分析GMAT分数是否与ASP有关系?c.每年的学费与ASP有关吗?你是如何知道的?如果两变量之间正相关,是否意味着进到最高费用的商业学校是有利的。
d.你同意高学费的商业学校意味着高质量的MBA成绩吗?为什么?3 你的朋友将不同年度的债券价格作为该年利率(在相等的风险水平下)的函数,估计出的简单方程如下:ˆ101.40 4.78i iY X =- 其中:ˆiY =第i 年美国政府债券价格(每100美元债券) i X =第i 年联邦资金利率(按百分比)请回答以下问题:(1) 解释两个所估系数的意义。
所估的符号与你所期望的符号一样吗?(2) 为何方程左边的变量是ˆiY 而不是Y ? (3) 你朋友在估计的方程中是否遗漏了随机误差项?(4) 此方程的经济意义是什么?对此模型你有何评论?(提示:联邦资金利率是一种适用于银行间隔夜持有款项的利率)(1)试建立Y 与X 之间的样本回归方程。
(2)预测收入为6000元这类家庭的平均消费支出(显著性水平0.05α=) (3)以95%的概率预测某个收入为6000元的家庭的消费支出。
(1) 试建立样本回归方程,并在5%的水平下进行显著性检验。
(2) 求简单相关系数。
(3) 如果0X =200千克,以90%的概率对()0E Y 和0Y 进行预测。
6 下表给出了1977-1991年期间美国的黄金价格、消费者指数和纽约股票交易所指数数据。
a. 在同一散布图中描绘黄金价格,CPI 和NYSE 指数。
b. 一种投资,如果它的价格和(或)回报率至少赶得上通货膨胀,就被认为是(对通货膨胀)保值(能抵御通货膨胀)的。
为检验这一假设:投资是保值的,假定a 中的散点图表明拟合以下模型是最适宜的:1212t i i t i iCPI NYSE CPI ββεββε=++=++黄金价格指数a. 将当年美元和不变(即1992年)美元数据对时间描图。
b. 用Y 表示GDP ,X 表示时间(按年历从1代表1959,2代表1960开始,直至39代表1997)。
看以下模型是否适合GDP 数据:12t t t Y X ββε=++ 试用当年美元和不变美元两种数据分别估计此模型。
c. 你会怎样解释2β?d. 如果用当年美元估计2β和不变美元GDP 估计的有所不同,你会怎样解释这个差距?e. 从你计算的结果,你能对样本时期美国通货膨胀的性质得出什么评论?第三部分 参考答案 一、术语解释1 解释变量:也称自变量,是在模型中对被解释变量起解释作用的变量。
如模型t t t y x αβε=++中的t x 。
2 被解释变量:也称因变量,在模型中假设其变动由解释变量引起,对解释变量起依存关系。
如模型t t t y x αβε=++中的t y 。
3 线性回归模型:是相对于模型的参数而言的,即对于其参数是一次的。
对于变量而言,模型可以是线性的,也可能不是线性的。
4 最小二乘法:普通最小二乘法归功于德国数学家高斯,在一定假设下,最小二乘法有一系列非常令人向往的统计性质,是回归中较常用的一种方法。
如,对模型t t ty x αβε=++(t t y x αβ=+)而言,通过使得统计量()2tt Q y y =-∑最小而求得参数α、β。
5 方差分析:通过分析总离差平方和()22TtS y y =-∑与回归平方和()22RtS y y =-∑、剩余平方和()22EttS y y =-∑的数值,及相互之间的数量关系,来分析变量之间的关系和回归模型。
6 参数估计:选定模型,根据解释变量和被解释变量的数据,使用一定的估计方法得出模型中的未知参数,称为参数估计。
7 控制:是预测的反问题,即要求观察值在某个区间()''12,y y 取值时,解释变量t x 应控制在什么范围。
8 预测:根据回归模型和已估计出的参数,在给定解释变量t x 时,预测被解释变量t y 的取值或取值范围。
二、填空题1 不确定性;客观经济。
2 随机性;随机性;随机性;随机扰动项;测量误差;被解释变量;解释变量。
3 总离差平方和;回归平方和;残差平方和。
4 净影响;β。
5 指数是一次;指数是一次;参数。
6 残差;随机扰动项。
三、简答题1答:在线性回归方程中,“线性”二字指的是方程对参数而言是线性的的,即参数的次数为一次。
对于变量而言,模型可以是线性的,也可能不是线性的。
2答:最小二乘法归功于德国数学家高斯,在一定假设下,最小二乘法有一系列非常令人向往的统计性质,是回归中较常用的一种方法。
如,对模型t t t y x αβε=++(t t y x αβ=+)而言,通过使得统计量()2ttQ y y =-∑最小而求得参数α、β。
最小二乘法使得回归的参差平方和尽可能的小,即总体上来说,t y 与t y 的偏差最小。
在满足一定的条件下,最小二乘法具有最优线性无偏估计量的性质(BLUE )。
3答:假设1,随即误差项t ε的均值为0,方差为2σ,且服从正态分布。
即()20,tN εσ假设2,随即误差项123,,,n εεεε之间是两两不相关的。
即()cov ,0i j εε=由于正态分布的随即变量不相关与独立是等价的,因此改假设实际上表示各随机变量误差项相互独立。
假设3,随机误差项与解释变量X 之间不相关,即()cov ,01,2,t t x t n ε==实际上,如果X 是可观察或可控制变量,则它就不是随机变量,因此改条件一定成立。
4答:方差分析通过分析总离差平方和()22TtS y y =-∑与回归平方和()22RtS y y =-∑、剩余平方和()22EttS y y =-∑的数值,及相互之间的数量关系,来分析变量之间的关系和回归模型。
通过方差分析,我们可以判断线性回归方程的好坏。
我们可以知道总离差平方和()22Tt S y y =-∑的构成情况,回归平方和()22Rt S y y =-∑反映由于x 与y 之间的线性关系而引起的回归值的离散程度,而剩余平方和()22EttS y y =-∑则反映了除x 与y 之间的线性关系以外引起数据y 波动的因素,这种波动性实际上是由于观测误差等随机因素引起的。
这样,我们就通过平方和的分解把引起数据y 波动的两种原因在数值上分开了。
5答:t 检验的思路,若线性假设符合实际,那么b 不应该为零。
否则,若b=0,那么y 就不依赖于x 了。
因此,我们需要检验假设:01:0:0H b H b =≠,通过计算t 统计量b bt L -=,及相应的临界值得出拒绝域。
当假设0:0H b =被拒绝时,我们认为线性回归效果是显著的;反之,则认为线性回归效果不显著。
相关系数检验法主要是通过由数据观测值计算出的样本相关系数xy L r =系数xy ρ的估计值,通过xy r 的大小来判断x 与y 之间线性关系的密切程度。
因此,我们需要检验假设:01:0:0H H ρρ=≠,通过计算检验统计量r t =,及相应的临界值得出拒绝域。
对于一元线性回归方程而言,有v a xyb r =,所以对于一元线性回归方程而言,t 检验中的假设0:0H b =等价于相关系数中的假设0:0H ρ=。