神经网络简介abstract( 英文的)
neural information processing systems介绍
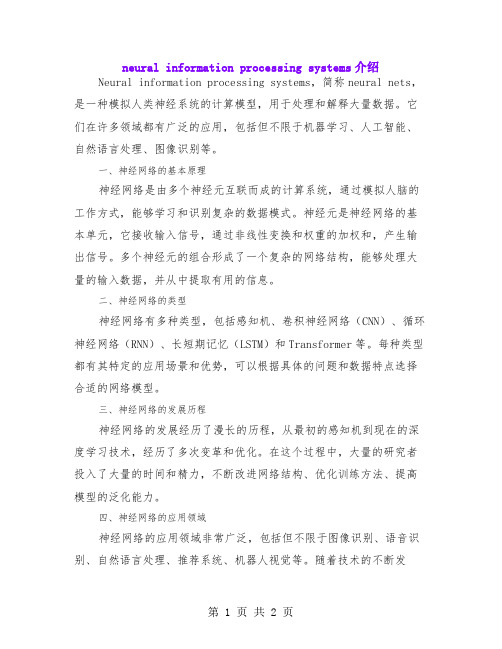
neural information processing systems介绍Neural information processing systems,简称neural nets,是一种模拟人类神经系统的计算模型,用于处理和解释大量数据。
它们在许多领域都有广泛的应用,包括但不限于机器学习、人工智能、自然语言处理、图像识别等。
一、神经网络的基本原理神经网络是由多个神经元互联而成的计算系统,通过模拟人脑的工作方式,能够学习和识别复杂的数据模式。
神经元是神经网络的基本单元,它接收输入信号,通过非线性变换和权重的加权和,产生输出信号。
多个神经元的组合形成了一个复杂的网络结构,能够处理大量的输入数据,并从中提取有用的信息。
二、神经网络的类型神经网络有多种类型,包括感知机、卷积神经网络(CNN)、循环神经网络(RNN)、长短期记忆(LSTM)和Transformer等。
每种类型都有其特定的应用场景和优势,可以根据具体的问题和数据特点选择合适的网络模型。
三、神经网络的发展历程神经网络的发展经历了漫长的历程,从最初的感知机到现在的深度学习技术,经历了多次变革和优化。
在这个过程中,大量的研究者投入了大量的时间和精力,不断改进网络结构、优化训练方法、提高模型的泛化能力。
四、神经网络的应用领域神经网络的应用领域非常广泛,包括但不限于图像识别、语音识别、自然语言处理、推荐系统、机器人视觉等。
随着技术的不断发展,神经网络的应用场景也在不断扩展,为许多领域带来了革命性的变革。
五、神经网络的未来发展未来神经网络的发展将面临许多挑战和机遇。
随着数据量的不断增加和计算能力的提升,神经网络将更加深入到各个领域的应用中。
同时,如何提高模型的泛化能力、降低计算复杂度、解决过拟合问题等也是未来研究的重要方向。
此外,神经网络的算法和理论也需要不断完善和深化,为未来的应用提供更加坚实的基础。
六、结论神经信息处理系统是一种强大的计算模型,具有广泛的应用领域和巨大的发展潜力。
外文翻译---人工神经网络
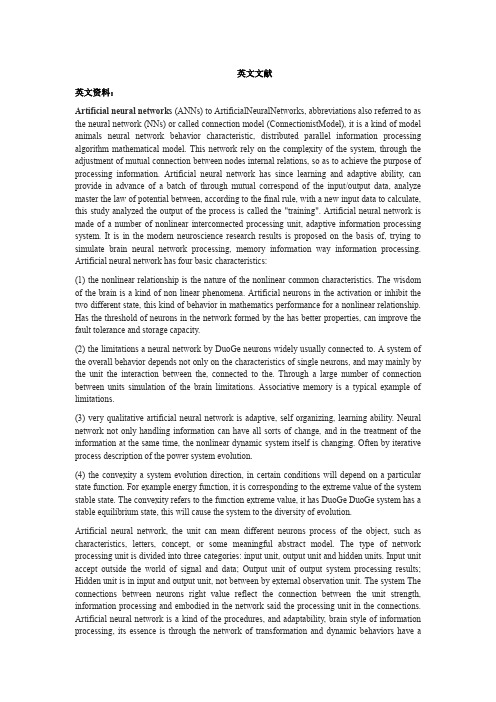
英文文献英文资料:Artificial neural networks (ANNs) to ArtificialNeuralNetworks, abbreviations also referred to as the neural network (NNs) or called connection model (ConnectionistModel), it is a kind of model animals neural network behavior characteristic, distributed parallel information processing algorithm mathematical model. This network rely on the complexity of the system, through the adjustment of mutual connection between nodes internal relations, so as to achieve the purpose of processing information. Artificial neural network has since learning and adaptive ability, can provide in advance of a batch of through mutual correspond of the input/output data, analyze master the law of potential between, according to the final rule, with a new input data to calculate, this study analyzed the output of the process is called the "training". Artificial neural network is made of a number of nonlinear interconnected processing unit, adaptive information processing system. It is in the modern neuroscience research results is proposed on the basis of, trying to simulate brain neural network processing, memory information way information processing. Artificial neural network has four basic characteristics:(1) the nonlinear relationship is the nature of the nonlinear common characteristics. The wisdom of the brain is a kind of non-linear phenomena. Artificial neurons in the activation or inhibit the two different state, this kind of behavior in mathematics performance for a nonlinear relationship. Has the threshold of neurons in the network formed by the has better properties, can improve the fault tolerance and storage capacity.(2) the limitations a neural network by DuoGe neurons widely usually connected to. A system of the overall behavior depends not only on the characteristics of single neurons, and may mainly by the unit the interaction between the, connected to the. Through a large number of connection between units simulation of the brain limitations. Associative memory is a typical example of limitations.(3) very qualitative artificial neural network is adaptive, self-organizing, learning ability. Neural network not only handling information can have all sorts of change, and in the treatment of the information at the same time, the nonlinear dynamic system itself is changing. Often by iterative process description of the power system evolution.(4) the convexity a system evolution direction, in certain conditions will depend on a particular state function. For example energy function, it is corresponding to the extreme value of the system stable state. The convexity refers to the function extreme value, it has DuoGe DuoGe system has a stable equilibrium state, this will cause the system to the diversity of evolution.Artificial neural network, the unit can mean different neurons process of the object, such as characteristics, letters, concept, or some meaningful abstract model. The type of network processing unit is divided into three categories: input unit, output unit and hidden units. Input unit accept outside the world of signal and data; Output unit of output system processing results; Hidden unit is in input and output unit, not between by external observation unit. The system The connections between neurons right value reflect the connection between the unit strength, information processing and embodied in the network said the processing unit in the connections. Artificial neural network is a kind of the procedures, and adaptability, brain style of information processing, its essence is through the network of transformation and dynamic behaviors have akind of parallel distributed information processing function, and in different levels and imitate people cranial nerve system level of information processing function. It is involved in neuroscience, thinking science, artificial intelligence, computer science, etc DuoGe field cross discipline.Artificial neural network is used the parallel distributed system, with the traditional artificial intelligence and information processing technology completely different mechanism, overcome traditional based on logic of the symbols of the artificial intelligence in the processing of intuition and unstructured information of defects, with the adaptive, self-organization and real-time characteristic of the study.Development historyIn 1943, psychologists W.S.M cCulloch and mathematical logic W.P home its established the neural network and the math model, called MP model. They put forward by MP model of the neuron network structure and formal mathematical description method, and prove the individual neurons can perform the logic function, so as to create artificial neural network research era. In 1949, the psychologist put forward the idea of synaptic contact strength variable. In the s, the artificial neural network to further development, a more perfect neural network model was put forward, including perceptron and adaptive linear elements etc. M.M insky, analyzed carefully to Perceptron as a representative of the neural network system function and limitations in 1969 after the publication of the book "Perceptron, and points out that the sensor can't solve problems high order predicate. Their arguments greatly influenced the research into the neural network, and at that time serial computer and the achievement of the artificial intelligence, covering up development new computer and new ways of artificial intelligence and the necessity and urgency, make artificial neural network of research at a low. During this time, some of the artificial neural network of the researchers remains committed to this study, presented to meet resonance theory (ART nets), self-organizing mapping, cognitive machine network, but the neural network theory study mathematics. The research for neural network of research and development has laid a foundation. In 1982, the California institute of J.J.H physicists opfield Hopfield neural grid model proposed, and introduces "calculation energy" concept, gives the network stability judgment. In 1984, he again put forward the continuous time Hopfield neural network model for the neural computers, the study of the pioneering work, creating a neural network for associative memory and optimization calculation, the new way of a powerful impetus to the research into the neural network, in 1985, and scholars have proposed a wave ears, the study boltzmann model using statistical thermodynamics simulated annealing technology, guaranteed that the whole system tends to the stability of the points. In 1986 the cognitive microstructure study, puts forward the parallel distributed processing theory. Artificial neural network of research by each developed country, the congress of the United States to the attention of the resolution will be on jan. 5, 1990 started ten years as the decade of the brain, the international research organization called on its members will the decade of the brain into global behavior. In Japan's "real world computing (springboks claiming)" project, artificial intelligence research into an important component.Network modelArtificial neural network model of the main consideration network connection topological structure, the characteristics, the learning rule neurons. At present, nearly 40 kinds of neural network model, with back propagation network, sensor, self-organizing mapping, the Hopfieldnetwork.the computer, wave boltzmann machine, adapt to the ear resonance theory. According to the topology of the connection, the neural network model can be divided into:(1) prior to the network before each neuron accept input and output level to the next level, the network without feedback, can use a loop to no graph. This network realization from the input space to the output signal of the space transformation, it information processing power comes from simple nonlinear function of DuoCi compound. The network structure is simple, easy to realize. Against the network is a kind of typical prior to the network.(2) the feedback network between neurons in the network has feedback, can use a no to complete the graph. This neural network information processing is state of transformations, can use the dynamics system theory processing. The stability of the system with associative memory function has close relationship. The Hopfield network.the computer, wave ear boltzmann machine all belong to this type.Learning typeNeural network learning is an important content, it is through the adaptability of the realization of learning. According to the change of environment, adjust to weights, improve the behavior of the system. The proposed by the Hebb Hebb learning rules for neural network learning algorithm to lay the foundation. Hebb rules say that learning process finally happened between neurons in the synapse, the contact strength synapses parts with before and after the activity and synaptic neuron changes. Based on this, people put forward various learning rules and algorithm, in order to adapt to the needs of different network model. Effective learning algorithm, and makes the godThe network can through the weights between adjustment, the structure of the objective world, said the formation of inner characteristics of information processing method, information storage and processing reflected in the network connection. According to the learning environment is different, the study method of the neural network can be divided into learning supervision and unsupervised learning. In the supervision and study, will the training sample data added to the network input, and the corresponding expected output and network output, in comparison to get error signal control value connection strength adjustment, the DuoCi after training to a certain convergence weights. While the sample conditions change, the study can modify weights to adapt to the new environment. Use of neural network learning supervision model is the network, the sensor etc. The learning supervision, in a given sample, in the environment of the network directly, learning and working stages become one. At this time, the change of the rules of learning to obey the weights between evolution equation of. Unsupervised learning the most simple example is Hebb learning rules. Competition rules is a learning more complex than learning supervision example, it is according to established clustering on weights adjustment. Self-organizing mapping, adapt to the resonance theory is the network and competitive learning about the typical model.Analysis methodStudy of the neural network nonlinear dynamic properties, mainly USES the dynamics system theory and nonlinear programming theory and statistical theory to analysis of the evolution process of the neural network and the nature of the attractor, explore the synergy of neural network behavior and collective computing functions, understand neural information processing mechanism. In order to discuss the neural network and fuzzy comprehensive deal of information may, the concept of chaos theory and method will play a role. The chaos is a rather difficult toprecise definition of the math concepts. In general, "chaos" it is to point to by the dynamic system of equations describe deterministic performance of the uncertain behavior, or call it sure the randomness. "Authenticity" because it by the intrinsic reason and not outside noise or interference produced, and "random" refers to the irregular, unpredictable behavior, can only use statistics method description. Chaotic dynamics of the main features of the system is the state of the sensitive dependence on the initial conditions, the chaos reflected its inherent randomness. Chaos theory is to point to describe the nonlinear dynamic behavior with chaos theory, the system of basic concept, methods, it dynamics system complex behavior understanding for his own with the outside world and for material, energy and information exchange process of the internal structure of behavior, not foreign and accidental behavior, chaos is a stationary. Chaotic dynamics system of stationary including: still, stable quantity, the periodicity, with sex and chaos of accurate solution... Chaos rail line is overall stability and local unstable combination of results, call it strange attractor.A strange attractor has the following features: (1) some strange attractor is a attractor, but it is not a fixed point, also not periodic solution; (2) strange attractor is indivisible, and that is not divided into two and two or more to attract children. (3) it to the initial value is very sensitive, different initial value can lead to very different behavior.superiorityThe artificial neural network of characteristics and advantages, mainly in three aspects: first, self-learning. For example, only to realize image recognition that the many different image model and the corresponding should be the result of identification input artificial neural network, the network will through the self-learning function, slowly to learn to distinguish similar images. The self-learning function for the forecast has special meaning. The prospect of artificial neural network computer will provide mankind economic forecasts, market forecast, benefit forecast, the application outlook is very great. The second, with lenovo storage function. With the artificial neural network of feedback network can implement this association. Third, with high-speed looking for the optimal solution ability. Looking for a complex problem of the optimal solution, often require a lot of calculation, the use of a problem in some of the design of feedback type and artificial neural network, use the computer high-speed operation ability, may soon find the optimal solution.Research directionThe research into the neural network can be divided into the theory research and application of the two aspects of research. Theory study can be divided into the following two categories:1, neural physiological and cognitive science research on human thinking and intelligent mechanism.2, by using the neural basis theory of research results, with mathematical method to explore more functional perfect, performance more superior neural network model, the thorough research network algorithm and performance, such as: stability and convergence, fault tolerance, robustness, etc.; The development of new network mathematical theory, such as: neural network dynamics, nonlinear neural field, etc.Application study can be divided into the following two categories:1, neural network software simulation and hardware realization of research.2, the neural network in various applications in the field of research. These areas include: pattern recognition, signal processing, knowledge engineering, expert system, optimize the combination, robot control, etc. Along with the neural network theory itself and related theory, related to the development of technology, the application of neural network will further.Development trend and research hot spotArtificial neural network characteristic of nonlinear adaptive information processing power, overcome traditional artificial intelligence method for intuitive, such as mode, speech recognition, unstructured information processing of the defects in the nerve of expert system, pattern recognition and intelligent control, combinatorial optimization, and forecast areas to be successful application. Artificial neural network and other traditional method unifies, will promote the artificial intelligence and information processing technology development. In recent years, the artificial neural network is on the path of human cognitive simulation further development, and fuzzy system, genetic algorithm, evolution mechanism combined to form a computational intelligence, artificial intelligence is an important direction in practical application, will be developed. Information geometry will used in artificial neural network of research, to the study of the theory of the artificial neural network opens a new way. The development of the study neural computers soon, existing product to enter the market. With electronics neural computers for the development of artificial neural network to provide good conditions.Neural network in many fields has got a very good application, but the need to research is a lot. Among them, are distributed storage, parallel processing, since learning, the organization and nonlinear mapping the advantages of neural network and other technology and the integration of it follows that the hybrid method and hybrid systems, has become a hotspot. Since the other way have their respective advantages, so will the neural network with other method, and the combination of strong points, and then can get better application effect. At present this in a neural network and fuzzy logic, expert system, genetic algorithm, wavelet analysis, chaos, the rough set theory, fractal theory, theory of evidence and grey system and fusion.汉语翻译人工神经网络(ArtificialNeuralNetworks,简写为ANNs)也简称为神经网络(NNs)或称作连接模型(ConnectionistModel),它是一种模范动物神经网络行为特征,进行分布式并行信息处理的算法数学模型。
神经网络基础精选
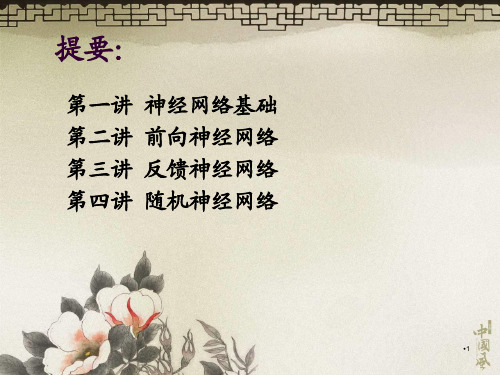
第一讲 神经网络基础
突触:突触是神经元的树突末梢连接另一神经元的突触 后膜 (postsynaptic membrane)的部分。它是神经元之 间相联系并进行信息传送的结构,是神经元之间连接的 接口。两个神经元的细胞质并不直接连通,两者彼此联 系是通过突触这种结构接口的。
膜电位:神经元细胞膜内外之间存在电位差,称为膜电 位。膜外为正,膜内为负。膜电压接受神经其它神经元 的输入后,电位上升或下降。当传入冲动的时空整合结 果,使膜电位上升,而且当超过叫做动作电位的阈值时, 细胞进入兴奋状态,产生神经冲动,由轴突输出,这个 过程称为兴奋。
•9
第一讲 神经网络基础
2 突触传递信息动作原理
膜电位(mv)
兴奋期, 大于动作阈值
动 作
绝对不应期:不响应任何刺激 阈
值
相对不应期:很难相应
t (ms)
根据突触传递信息的动作过 -55
程可以分为两种类型:兴奋型 -70
12
3
和抑制型。神经冲动使得细胞 膜电压升高超过动作电压进入
1ms 1ms 3ms
•5
树突
细胞体
细胞核 轴突
轴突末梢
图1-1a 神经元的解剖
•6
图1-1b 神经元的解剖
•7
第一讲 神经网络基础
细胞体:细胞体是由很多分子形成的综合体,内部含有 一个细胞核、核糖体、原生质网状结构等,它是神经元 活动的能量供应地,在这里进行新陈代谢等各种生化过 程。包括细胞核,细胞膜和细胞质。
n
Ii W ijXj为 第 i个 神 经 元 的 净 输 入
j1
•12
第一讲 神经网络基础
四 人工神经元与生物神经元区别 (1)模型传递的是模拟信号,生物输入输出均
神经网络的综述
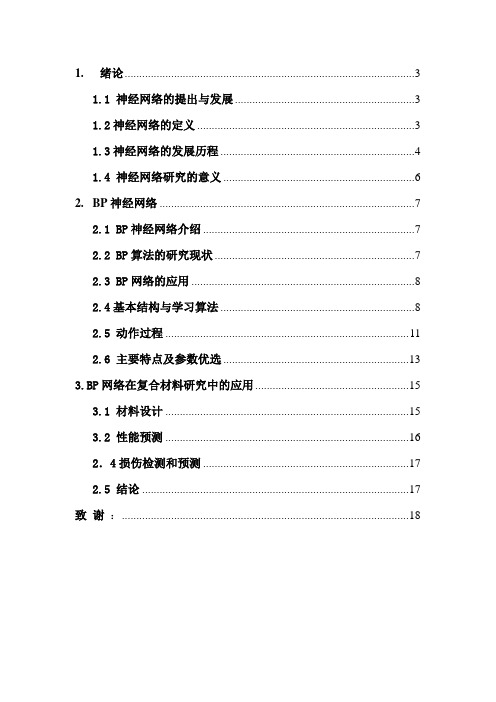
1.绪论 (3)1.1 神经网络的提出与发展 (3)1.2神经网络的定义 (3)1.3神经网络的发展历程 (4)1.4 神经网络研究的意义 (6)2.BP神经网络 (7)2.1 BP神经网络介绍 (7)2.2 BP算法的研究现状 (7)2.3 BP网络的应用 (8)2.4基本结构与学习算法 (8)2.5 动作过程 (11)2.6 主要特点及参数优选 (13)3.BP网络在复合材料研究中的应用 (15)3.1 材料设计 (15)3.2 性能预测 (16)2.4损伤检测和预测 (17)2.5 结论 (17)致谢: (18)BP神经网络综述摘要:本文阐述了人工神经网络和神经网络控制的基本概念特点以及两者之间的关系,讨论了人工神经网络的两个主要研究方向神经网络的VC 维计算和神经网络的数据挖掘,着重介绍了人工神经网络的工作原理和神经网络控制技术的应用首先介绍了神经网络的发展历程,随后对BP神经网络的学习方法分为了导师知识学习训练和模式识别决策,并重点分析了导师知识学习训练的网络结构和学习算法,最后介绍了BP神经网络在性能预测中的应用。
关键词:人工神经网络;神经网络控制;应用;维;数据挖掘Abstract:It expounds the basic concepts, characteristics of the artificial neural network and neural network control and the relationship between them.It discusses two aspects: the Vapnik-Chervonenkis dimension calculation and the data mining in neural nets.And the basic principle of artificial neural networks and applications of neural network control technology are emphatically introduced. Key words:Artificial Neural Networks; Neural Network Control;this paper introduces the developing process of neural networks, and then it divides the learning methods of BP neural network into a inst ructor knowledge learning training and pattern recognition decisions, and focus on analysis of the network structure and learning algorith m of knowledge and learning mentors training .And finally it introduc es the applications of BP neural network in performance prediction.Application;Vapnik-Chervonenkis Mimension;Data Mining1.绪1.1 神经网络的提出与发展系统的复杂性与所要求的精确性之间存在尖锐的矛盾。
神经网络介绍

神经网络简介神经网络简介:人工神经网络是以工程技术手段来模拟人脑神经元网络的结构和特征的系统。
利用人工神经网络可以构成各种不同拓扑结构的神经网络,他是生物神经网络的一种模拟和近似。
神经网络的主要连接形式主要有前馈型和反馈型神经网络。
常用的前馈型有感知器神经网络、BP 神经网络,常用的反馈型有Hopfield 网络。
这里介绍BP (Back Propagation )神经网络,即误差反向传播算法。
原理:BP (Back Propagation )网络是一种按误差逆传播算法训练的多层前馈网络,是目前应用最广泛的神经网络模型之一。
BP 神经网络模型拓扑结构包括输入层(input )、隐层(hide layer)和输出层(output layer),其中隐层可以是一层也可以是多层。
图:三层神经网络结构图(一个隐层)任何从输入到输出的连续映射函数都可以用一个三层的非线性网络实现 BP 算法由数据流的前向计算(正向传播)和误差信号的反向传播两个过程构成。
正向传播时,传播方向为输入层→隐层→输出层,每层神经元的状态只影响下一层神经元。
若在输出层得不到期望的输出,则转向误差信号的反向传播流程。
通过这两个过程的交替进行,在权向量空间执行误差函数梯度下降策略,动态迭代搜索一组权向量,使网络误差函数达到最小值,从而完成信息提取和记忆过程。
单个神经元的计算:设12,...ni x x x 分别代表来自神经元1,2...ni 的输入;12,...i i ini w w w 则分别表示神经元1,2...ni 与下一层第j 个神经元的连接强度,即权值;j b 为阈值;()f ∙为传递函数;j y 为第j 个神经元的输出。
若记001,j j x w b ==,于是节点j 的净输入j S 可表示为:0*nij ij i i S w x ==∑;净输入j S 通过激活函数()f ∙后,便得到第j 个神经元的输出:0()(*),nij j ij i i y f S f w x ===∑激活函数:激活函数()f ∙是单调上升可微函数,除输出层激活函数外,其他层激活函数必须是有界函数,必有一最大值。
神经网络的研究和应用(英文文献)
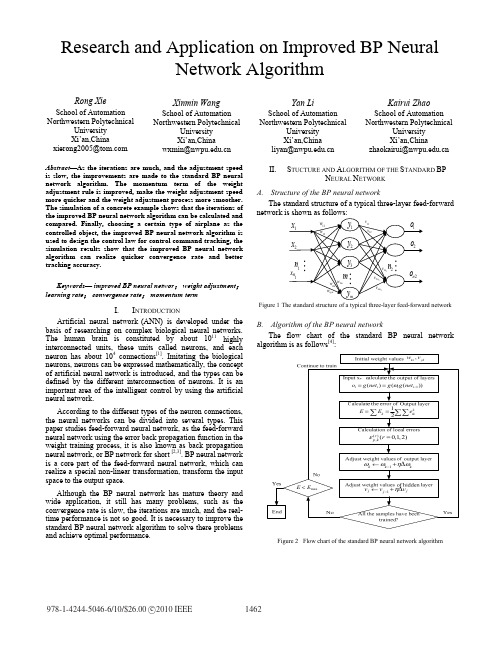
network is shown as follows:
x1
w11 w12
y1
v11 v12
o1
x2
y2
o2
# xn1n1
y3
m# w2m m
wn1m
ym
#n v1n2 2
v3n2
on2
vmn2
Figure 1 The standard structure of a typical three-layer feed-forward network
1462
III. IMPROVEMENT OF THE STANDARD BP NEURAL NETWORK ALGORITHM
The convergence rate of the standard BP algorithm is slow, and the iterations of the standard BP algorithm are much, they all have negative influences on the rapidity of the control system. In this paper, improvement has been made to the learning rate of the standard BP algorithm to accelerate the training speed of the neural network.
From formula (1), the learning rate η influences the
weight adjustment value ǻW(n), and then influences the convergence rate of the network. If the learning rate η is too
神经网络(NeuralNetwork)
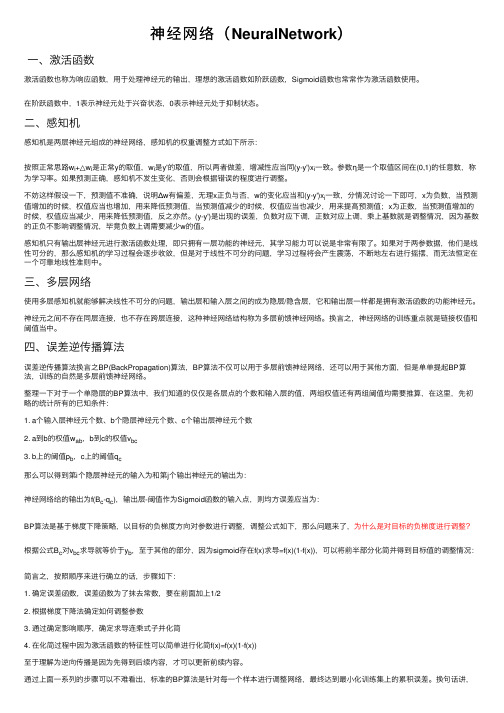
神经⽹络(NeuralNetwork)⼀、激活函数激活函数也称为响应函数,⽤于处理神经元的输出,理想的激活函数如阶跃函数,Sigmoid函数也常常作为激活函数使⽤。
在阶跃函数中,1表⽰神经元处于兴奋状态,0表⽰神经元处于抑制状态。
⼆、感知机感知机是两层神经元组成的神经⽹络,感知机的权重调整⽅式如下所⽰:按照正常思路w i+△w i是正常y的取值,w i是y'的取值,所以两者做差,增减性应当同(y-y')x i⼀致。
参数η是⼀个取值区间在(0,1)的任意数,称为学习率。
如果预测正确,感知机不发⽣变化,否则会根据错误的程度进⾏调整。
不妨这样假设⼀下,预测值不准确,说明Δw有偏差,⽆理x正负与否,w的变化应当和(y-y')x i⼀致,分情况讨论⼀下即可,x为负数,当预测值增加的时候,权值应当也增加,⽤来降低预测值,当预测值减少的时候,权值应当也减少,⽤来提⾼预测值;x为正数,当预测值增加的时候,权值应当减少,⽤来降低预测值,反之亦然。
(y-y')是出现的误差,负数对应下调,正数对应上调,乘上基数就是调整情况,因为基数的正负不影响调整情况,毕竟负数上调需要减少w的值。
感知机只有输出层神经元进⾏激活函数处理,即只拥有⼀层功能的神经元,其学习能⼒可以说是⾮常有限了。
如果对于两参数据,他们是线性可分的,那么感知机的学习过程会逐步收敛,但是对于线性不可分的问题,学习过程将会产⽣震荡,不断地左右进⾏摇摆,⽽⽆法恒定在⼀个可靠地线性准则中。
三、多层⽹络使⽤多层感知机就能够解决线性不可分的问题,输出层和输⼊层之间的成为隐层/隐含层,它和输出层⼀样都是拥有激活函数的功能神经元。
神经元之间不存在同层连接,也不存在跨层连接,这种神经⽹络结构称为多层前馈神经⽹络。
换⾔之,神经⽹络的训练重点就是链接权值和阈值当中。
四、误差逆传播算法误差逆传播算法换⾔之BP(BackPropagation)算法,BP算法不仅可以⽤于多层前馈神经⽹络,还可以⽤于其他⽅⾯,但是单单提起BP算法,训练的⾃然是多层前馈神经⽹络。
机器学习与人工智能领域中常用的英语词汇
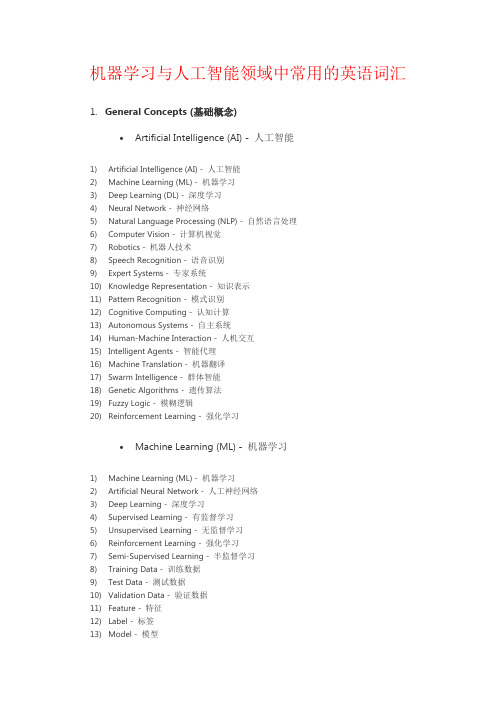
机器学习与人工智能领域中常用的英语词汇1.General Concepts (基础概念)•Artificial Intelligence (AI) - 人工智能1)Artificial Intelligence (AI) - 人工智能2)Machine Learning (ML) - 机器学习3)Deep Learning (DL) - 深度学习4)Neural Network - 神经网络5)Natural Language Processing (NLP) - 自然语言处理6)Computer Vision - 计算机视觉7)Robotics - 机器人技术8)Speech Recognition - 语音识别9)Expert Systems - 专家系统10)Knowledge Representation - 知识表示11)Pattern Recognition - 模式识别12)Cognitive Computing - 认知计算13)Autonomous Systems - 自主系统14)Human-Machine Interaction - 人机交互15)Intelligent Agents - 智能代理16)Machine Translation - 机器翻译17)Swarm Intelligence - 群体智能18)Genetic Algorithms - 遗传算法19)Fuzzy Logic - 模糊逻辑20)Reinforcement Learning - 强化学习•Machine Learning (ML) - 机器学习1)Machine Learning (ML) - 机器学习2)Artificial Neural Network - 人工神经网络3)Deep Learning - 深度学习4)Supervised Learning - 有监督学习5)Unsupervised Learning - 无监督学习6)Reinforcement Learning - 强化学习7)Semi-Supervised Learning - 半监督学习8)Training Data - 训练数据9)Test Data - 测试数据10)Validation Data - 验证数据11)Feature - 特征12)Label - 标签13)Model - 模型14)Algorithm - 算法15)Regression - 回归16)Classification - 分类17)Clustering - 聚类18)Dimensionality Reduction - 降维19)Overfitting - 过拟合20)Underfitting - 欠拟合•Deep Learning (DL) - 深度学习1)Deep Learning - 深度学习2)Neural Network - 神经网络3)Artificial Neural Network (ANN) - 人工神经网络4)Convolutional Neural Network (CNN) - 卷积神经网络5)Recurrent Neural Network (RNN) - 循环神经网络6)Long Short-Term Memory (LSTM) - 长短期记忆网络7)Gated Recurrent Unit (GRU) - 门控循环单元8)Autoencoder - 自编码器9)Generative Adversarial Network (GAN) - 生成对抗网络10)Transfer Learning - 迁移学习11)Pre-trained Model - 预训练模型12)Fine-tuning - 微调13)Feature Extraction - 特征提取14)Activation Function - 激活函数15)Loss Function - 损失函数16)Gradient Descent - 梯度下降17)Backpropagation - 反向传播18)Epoch - 训练周期19)Batch Size - 批量大小20)Dropout - 丢弃法•Neural Network - 神经网络1)Neural Network - 神经网络2)Artificial Neural Network (ANN) - 人工神经网络3)Deep Neural Network (DNN) - 深度神经网络4)Convolutional Neural Network (CNN) - 卷积神经网络5)Recurrent Neural Network (RNN) - 循环神经网络6)Long Short-Term Memory (LSTM) - 长短期记忆网络7)Gated Recurrent Unit (GRU) - 门控循环单元8)Feedforward Neural Network - 前馈神经网络9)Multi-layer Perceptron (MLP) - 多层感知器10)Radial Basis Function Network (RBFN) - 径向基函数网络11)Hopfield Network - 霍普菲尔德网络12)Boltzmann Machine - 玻尔兹曼机13)Autoencoder - 自编码器14)Spiking Neural Network (SNN) - 脉冲神经网络15)Self-organizing Map (SOM) - 自组织映射16)Restricted Boltzmann Machine (RBM) - 受限玻尔兹曼机17)Hebbian Learning - 海比安学习18)Competitive Learning - 竞争学习19)Neuroevolutionary - 神经进化20)Neuron - 神经元•Algorithm - 算法1)Algorithm - 算法2)Supervised Learning Algorithm - 有监督学习算法3)Unsupervised Learning Algorithm - 无监督学习算法4)Reinforcement Learning Algorithm - 强化学习算法5)Classification Algorithm - 分类算法6)Regression Algorithm - 回归算法7)Clustering Algorithm - 聚类算法8)Dimensionality Reduction Algorithm - 降维算法9)Decision Tree Algorithm - 决策树算法10)Random Forest Algorithm - 随机森林算法11)Support Vector Machine (SVM) Algorithm - 支持向量机算法12)K-Nearest Neighbors (KNN) Algorithm - K近邻算法13)Naive Bayes Algorithm - 朴素贝叶斯算法14)Gradient Descent Algorithm - 梯度下降算法15)Genetic Algorithm - 遗传算法16)Neural Network Algorithm - 神经网络算法17)Deep Learning Algorithm - 深度学习算法18)Ensemble Learning Algorithm - 集成学习算法19)Reinforcement Learning Algorithm - 强化学习算法20)Metaheuristic Algorithm - 元启发式算法•Model - 模型1)Model - 模型2)Machine Learning Model - 机器学习模型3)Artificial Intelligence Model - 人工智能模型4)Predictive Model - 预测模型5)Classification Model - 分类模型6)Regression Model - 回归模型7)Generative Model - 生成模型8)Discriminative Model - 判别模型9)Probabilistic Model - 概率模型10)Statistical Model - 统计模型11)Neural Network Model - 神经网络模型12)Deep Learning Model - 深度学习模型13)Ensemble Model - 集成模型14)Reinforcement Learning Model - 强化学习模型15)Support Vector Machine (SVM) Model - 支持向量机模型16)Decision Tree Model - 决策树模型17)Random Forest Model - 随机森林模型18)Naive Bayes Model - 朴素贝叶斯模型19)Autoencoder Model - 自编码器模型20)Convolutional Neural Network (CNN) Model - 卷积神经网络模型•Dataset - 数据集1)Dataset - 数据集2)Training Dataset - 训练数据集3)Test Dataset - 测试数据集4)Validation Dataset - 验证数据集5)Balanced Dataset - 平衡数据集6)Imbalanced Dataset - 不平衡数据集7)Synthetic Dataset - 合成数据集8)Benchmark Dataset - 基准数据集9)Open Dataset - 开放数据集10)Labeled Dataset - 标记数据集11)Unlabeled Dataset - 未标记数据集12)Semi-Supervised Dataset - 半监督数据集13)Multiclass Dataset - 多分类数据集14)Feature Set - 特征集15)Data Augmentation - 数据增强16)Data Preprocessing - 数据预处理17)Missing Data - 缺失数据18)Outlier Detection - 异常值检测19)Data Imputation - 数据插补20)Metadata - 元数据•Training - 训练1)Training - 训练2)Training Data - 训练数据3)Training Phase - 训练阶段4)Training Set - 训练集5)Training Examples - 训练样本6)Training Instance - 训练实例7)Training Algorithm - 训练算法8)Training Model - 训练模型9)Training Process - 训练过程10)Training Loss - 训练损失11)Training Epoch - 训练周期12)Training Batch - 训练批次13)Online Training - 在线训练14)Offline Training - 离线训练15)Continuous Training - 连续训练16)Transfer Learning - 迁移学习17)Fine-Tuning - 微调18)Curriculum Learning - 课程学习19)Self-Supervised Learning - 自监督学习20)Active Learning - 主动学习•Testing - 测试1)Testing - 测试2)Test Data - 测试数据3)Test Set - 测试集4)Test Examples - 测试样本5)Test Instance - 测试实例6)Test Phase - 测试阶段7)Test Accuracy - 测试准确率8)Test Loss - 测试损失9)Test Error - 测试错误10)Test Metrics - 测试指标11)Test Suite - 测试套件12)Test Case - 测试用例13)Test Coverage - 测试覆盖率14)Cross-Validation - 交叉验证15)Holdout Validation - 留出验证16)K-Fold Cross-Validation - K折交叉验证17)Stratified Cross-Validation - 分层交叉验证18)Test Driven Development (TDD) - 测试驱动开发19)A/B Testing - A/B 测试20)Model Evaluation - 模型评估•Validation - 验证1)Validation - 验证2)Validation Data - 验证数据3)Validation Set - 验证集4)Validation Examples - 验证样本5)Validation Instance - 验证实例6)Validation Phase - 验证阶段7)Validation Accuracy - 验证准确率8)Validation Loss - 验证损失9)Validation Error - 验证错误10)Validation Metrics - 验证指标11)Cross-Validation - 交叉验证12)Holdout Validation - 留出验证13)K-Fold Cross-Validation - K折交叉验证14)Stratified Cross-Validation - 分层交叉验证15)Leave-One-Out Cross-Validation - 留一法交叉验证16)Validation Curve - 验证曲线17)Hyperparameter Validation - 超参数验证18)Model Validation - 模型验证19)Early Stopping - 提前停止20)Validation Strategy - 验证策略•Supervised Learning - 有监督学习1)Supervised Learning - 有监督学习2)Label - 标签3)Feature - 特征4)Target - 目标5)Training Labels - 训练标签6)Training Features - 训练特征7)Training Targets - 训练目标8)Training Examples - 训练样本9)Training Instance - 训练实例10)Regression - 回归11)Classification - 分类12)Predictor - 预测器13)Regression Model - 回归模型14)Classifier - 分类器15)Decision Tree - 决策树16)Support Vector Machine (SVM) - 支持向量机17)Neural Network - 神经网络18)Feature Engineering - 特征工程19)Model Evaluation - 模型评估20)Overfitting - 过拟合21)Underfitting - 欠拟合22)Bias-Variance Tradeoff - 偏差-方差权衡•Unsupervised Learning - 无监督学习1)Unsupervised Learning - 无监督学习2)Clustering - 聚类3)Dimensionality Reduction - 降维4)Anomaly Detection - 异常检测5)Association Rule Learning - 关联规则学习6)Feature Extraction - 特征提取7)Feature Selection - 特征选择8)K-Means - K均值9)Hierarchical Clustering - 层次聚类10)Density-Based Clustering - 基于密度的聚类11)Principal Component Analysis (PCA) - 主成分分析12)Independent Component Analysis (ICA) - 独立成分分析13)T-distributed Stochastic Neighbor Embedding (t-SNE) - t分布随机邻居嵌入14)Gaussian Mixture Model (GMM) - 高斯混合模型15)Self-Organizing Maps (SOM) - 自组织映射16)Autoencoder - 自动编码器17)Latent Variable - 潜变量18)Data Preprocessing - 数据预处理19)Outlier Detection - 异常值检测20)Clustering Algorithm - 聚类算法•Reinforcement Learning - 强化学习1)Reinforcement Learning - 强化学习2)Agent - 代理3)Environment - 环境4)State - 状态5)Action - 动作6)Reward - 奖励7)Policy - 策略8)Value Function - 值函数9)Q-Learning - Q学习10)Deep Q-Network (DQN) - 深度Q网络11)Policy Gradient - 策略梯度12)Actor-Critic - 演员-评论家13)Exploration - 探索14)Exploitation - 开发15)Temporal Difference (TD) - 时间差分16)Markov Decision Process (MDP) - 马尔可夫决策过程17)State-Action-Reward-State-Action (SARSA) - 状态-动作-奖励-状态-动作18)Policy Iteration - 策略迭代19)Value Iteration - 值迭代20)Monte Carlo Methods - 蒙特卡洛方法•Semi-Supervised Learning - 半监督学习1)Semi-Supervised Learning - 半监督学习2)Labeled Data - 有标签数据3)Unlabeled Data - 无标签数据4)Label Propagation - 标签传播5)Self-Training - 自训练6)Co-Training - 协同训练7)Transudative Learning - 传导学习8)Inductive Learning - 归纳学习9)Manifold Regularization - 流形正则化10)Graph-based Methods - 基于图的方法11)Cluster Assumption - 聚类假设12)Low-Density Separation - 低密度分离13)Semi-Supervised Support Vector Machines (S3VM) - 半监督支持向量机14)Expectation-Maximization (EM) - 期望最大化15)Co-EM - 协同期望最大化16)Entropy-Regularized EM - 熵正则化EM17)Mean Teacher - 平均教师18)Virtual Adversarial Training - 虚拟对抗训练19)Tri-training - 三重训练20)Mix Match - 混合匹配•Feature - 特征1)Feature - 特征2)Feature Engineering - 特征工程3)Feature Extraction - 特征提取4)Feature Selection - 特征选择5)Input Features - 输入特征6)Output Features - 输出特征7)Feature Vector - 特征向量8)Feature Space - 特征空间9)Feature Representation - 特征表示10)Feature Transformation - 特征转换11)Feature Importance - 特征重要性12)Feature Scaling - 特征缩放13)Feature Normalization - 特征归一化14)Feature Encoding - 特征编码15)Feature Fusion - 特征融合16)Feature Dimensionality Reduction - 特征维度减少17)Continuous Feature - 连续特征18)Categorical Feature - 分类特征19)Nominal Feature - 名义特征20)Ordinal Feature - 有序特征•Label - 标签1)Label - 标签2)Labeling - 标注3)Ground Truth - 地面真值4)Class Label - 类别标签5)Target Variable - 目标变量6)Labeling Scheme - 标注方案7)Multi-class Labeling - 多类别标注8)Binary Labeling - 二分类标注9)Label Noise - 标签噪声10)Labeling Error - 标注错误11)Label Propagation - 标签传播12)Unlabeled Data - 无标签数据13)Labeled Data - 有标签数据14)Semi-supervised Learning - 半监督学习15)Active Learning - 主动学习16)Weakly Supervised Learning - 弱监督学习17)Noisy Label Learning - 噪声标签学习18)Self-training - 自训练19)Crowdsourcing Labeling - 众包标注20)Label Smoothing - 标签平滑化•Prediction - 预测1)Prediction - 预测2)Forecasting - 预测3)Regression - 回归4)Classification - 分类5)Time Series Prediction - 时间序列预测6)Forecast Accuracy - 预测准确性7)Predictive Modeling - 预测建模8)Predictive Analytics - 预测分析9)Forecasting Method - 预测方法10)Predictive Performance - 预测性能11)Predictive Power - 预测能力12)Prediction Error - 预测误差13)Prediction Interval - 预测区间14)Prediction Model - 预测模型15)Predictive Uncertainty - 预测不确定性16)Forecast Horizon - 预测时间跨度17)Predictive Maintenance - 预测性维护18)Predictive Policing - 预测式警务19)Predictive Healthcare - 预测性医疗20)Predictive Maintenance - 预测性维护•Classification - 分类1)Classification - 分类2)Classifier - 分类器3)Class - 类别4)Classify - 对数据进行分类5)Class Label - 类别标签6)Binary Classification - 二元分类7)Multiclass Classification - 多类分类8)Class Probability - 类别概率9)Decision Boundary - 决策边界10)Decision Tree - 决策树11)Support Vector Machine (SVM) - 支持向量机12)K-Nearest Neighbors (KNN) - K最近邻算法13)Naive Bayes - 朴素贝叶斯14)Logistic Regression - 逻辑回归15)Random Forest - 随机森林16)Neural Network - 神经网络17)SoftMax Function - SoftMax函数18)One-vs-All (One-vs-Rest) - 一对多(一对剩余)19)Ensemble Learning - 集成学习20)Confusion Matrix - 混淆矩阵•Regression - 回归1)Regression Analysis - 回归分析2)Linear Regression - 线性回归3)Multiple Regression - 多元回归4)Polynomial Regression - 多项式回归5)Logistic Regression - 逻辑回归6)Ridge Regression - 岭回归7)Lasso Regression - Lasso回归8)Elastic Net Regression - 弹性网络回归9)Regression Coefficients - 回归系数10)Residuals - 残差11)Ordinary Least Squares (OLS) - 普通最小二乘法12)Ridge Regression Coefficient - 岭回归系数13)Lasso Regression Coefficient - Lasso回归系数14)Elastic Net Regression Coefficient - 弹性网络回归系数15)Regression Line - 回归线16)Prediction Error - 预测误差17)Regression Model - 回归模型18)Nonlinear Regression - 非线性回归19)Generalized Linear Models (GLM) - 广义线性模型20)Coefficient of Determination (R-squared) - 决定系数21)F-test - F检验22)Homoscedasticity - 同方差性23)Heteroscedasticity - 异方差性24)Autocorrelation - 自相关25)Multicollinearity - 多重共线性26)Outliers - 异常值27)Cross-validation - 交叉验证28)Feature Selection - 特征选择29)Feature Engineering - 特征工程30)Regularization - 正则化2.Neural Networks and Deep Learning (神经网络与深度学习)•Convolutional Neural Network (CNN) - 卷积神经网络1)Convolutional Neural Network (CNN) - 卷积神经网络2)Convolution Layer - 卷积层3)Feature Map - 特征图4)Convolution Operation - 卷积操作5)Stride - 步幅6)Padding - 填充7)Pooling Layer - 池化层8)Max Pooling - 最大池化9)Average Pooling - 平均池化10)Fully Connected Layer - 全连接层11)Activation Function - 激活函数12)Rectified Linear Unit (ReLU) - 线性修正单元13)Dropout - 随机失活14)Batch Normalization - 批量归一化15)Transfer Learning - 迁移学习16)Fine-Tuning - 微调17)Image Classification - 图像分类18)Object Detection - 物体检测19)Semantic Segmentation - 语义分割20)Instance Segmentation - 实例分割21)Generative Adversarial Network (GAN) - 生成对抗网络22)Image Generation - 图像生成23)Style Transfer - 风格迁移24)Convolutional Autoencoder - 卷积自编码器25)Recurrent Neural Network (RNN) - 循环神经网络•Recurrent Neural Network (RNN) - 循环神经网络1)Recurrent Neural Network (RNN) - 循环神经网络2)Long Short-Term Memory (LSTM) - 长短期记忆网络3)Gated Recurrent Unit (GRU) - 门控循环单元4)Sequence Modeling - 序列建模5)Time Series Prediction - 时间序列预测6)Natural Language Processing (NLP) - 自然语言处理7)Text Generation - 文本生成8)Sentiment Analysis - 情感分析9)Named Entity Recognition (NER) - 命名实体识别10)Part-of-Speech Tagging (POS Tagging) - 词性标注11)Sequence-to-Sequence (Seq2Seq) - 序列到序列12)Attention Mechanism - 注意力机制13)Encoder-Decoder Architecture - 编码器-解码器架构14)Bidirectional RNN - 双向循环神经网络15)Teacher Forcing - 强制教师法16)Backpropagation Through Time (BPTT) - 通过时间的反向传播17)Vanishing Gradient Problem - 梯度消失问题18)Exploding Gradient Problem - 梯度爆炸问题19)Language Modeling - 语言建模20)Speech Recognition - 语音识别•Long Short-Term Memory (LSTM) - 长短期记忆网络1)Long Short-Term Memory (LSTM) - 长短期记忆网络2)Cell State - 细胞状态3)Hidden State - 隐藏状态4)Forget Gate - 遗忘门5)Input Gate - 输入门6)Output Gate - 输出门7)Peephole Connections - 窥视孔连接8)Gated Recurrent Unit (GRU) - 门控循环单元9)Vanishing Gradient Problem - 梯度消失问题10)Exploding Gradient Problem - 梯度爆炸问题11)Sequence Modeling - 序列建模12)Time Series Prediction - 时间序列预测13)Natural Language Processing (NLP) - 自然语言处理14)Text Generation - 文本生成15)Sentiment Analysis - 情感分析16)Named Entity Recognition (NER) - 命名实体识别17)Part-of-Speech Tagging (POS Tagging) - 词性标注18)Attention Mechanism - 注意力机制19)Encoder-Decoder Architecture - 编码器-解码器架构20)Bidirectional LSTM - 双向长短期记忆网络•Attention Mechanism - 注意力机制1)Attention Mechanism - 注意力机制2)Self-Attention - 自注意力3)Multi-Head Attention - 多头注意力4)Transformer - 变换器5)Query - 查询6)Key - 键7)Value - 值8)Query-Value Attention - 查询-值注意力9)Dot-Product Attention - 点积注意力10)Scaled Dot-Product Attention - 缩放点积注意力11)Additive Attention - 加性注意力12)Context Vector - 上下文向量13)Attention Score - 注意力分数14)SoftMax Function - SoftMax函数15)Attention Weight - 注意力权重16)Global Attention - 全局注意力17)Local Attention - 局部注意力18)Positional Encoding - 位置编码19)Encoder-Decoder Attention - 编码器-解码器注意力20)Cross-Modal Attention - 跨模态注意力•Generative Adversarial Network (GAN) - 生成对抗网络1)Generative Adversarial Network (GAN) - 生成对抗网络2)Generator - 生成器3)Discriminator - 判别器4)Adversarial Training - 对抗训练5)Minimax Game - 极小极大博弈6)Nash Equilibrium - 纳什均衡7)Mode Collapse - 模式崩溃8)Training Stability - 训练稳定性9)Loss Function - 损失函数10)Discriminative Loss - 判别损失11)Generative Loss - 生成损失12)Wasserstein GAN (WGAN) - Wasserstein GAN(WGAN)13)Deep Convolutional GAN (DCGAN) - 深度卷积生成对抗网络(DCGAN)14)Conditional GAN (c GAN) - 条件生成对抗网络(c GAN)15)Style GAN - 风格生成对抗网络16)Cycle GAN - 循环生成对抗网络17)Progressive Growing GAN (PGGAN) - 渐进式增长生成对抗网络(PGGAN)18)Self-Attention GAN (SAGAN) - 自注意力生成对抗网络(SAGAN)19)Big GAN - 大规模生成对抗网络20)Adversarial Examples - 对抗样本•Encoder-Decoder - 编码器-解码器1)Encoder-Decoder Architecture - 编码器-解码器架构2)Encoder - 编码器3)Decoder - 解码器4)Sequence-to-Sequence Model (Seq2Seq) - 序列到序列模型5)State Vector - 状态向量6)Context Vector - 上下文向量7)Hidden State - 隐藏状态8)Attention Mechanism - 注意力机制9)Teacher Forcing - 强制教师法10)Beam Search - 束搜索11)Recurrent Neural Network (RNN) - 循环神经网络12)Long Short-Term Memory (LSTM) - 长短期记忆网络13)Gated Recurrent Unit (GRU) - 门控循环单元14)Bidirectional Encoder - 双向编码器15)Greedy Decoding - 贪婪解码16)Masking - 遮盖17)Dropout - 随机失活18)Embedding Layer - 嵌入层19)Cross-Entropy Loss - 交叉熵损失20)Tokenization - 令牌化•Transfer Learning - 迁移学习1)Transfer Learning - 迁移学习2)Source Domain - 源领域3)Target Domain - 目标领域4)Fine-Tuning - 微调5)Domain Adaptation - 领域自适应6)Pre-Trained Model - 预训练模型7)Feature Extraction - 特征提取8)Knowledge Transfer - 知识迁移9)Unsupervised Domain Adaptation - 无监督领域自适应10)Semi-Supervised Domain Adaptation - 半监督领域自适应11)Multi-Task Learning - 多任务学习12)Data Augmentation - 数据增强13)Task Transfer - 任务迁移14)Model Agnostic Meta-Learning (MAML) - 与模型无关的元学习(MAML)15)One-Shot Learning - 单样本学习16)Zero-Shot Learning - 零样本学习17)Few-Shot Learning - 少样本学习18)Knowledge Distillation - 知识蒸馏19)Representation Learning - 表征学习20)Adversarial Transfer Learning - 对抗迁移学习•Pre-trained Models - 预训练模型1)Pre-trained Model - 预训练模型2)Transfer Learning - 迁移学习3)Fine-Tuning - 微调4)Knowledge Transfer - 知识迁移5)Domain Adaptation - 领域自适应6)Feature Extraction - 特征提取7)Representation Learning - 表征学习8)Language Model - 语言模型9)Bidirectional Encoder Representations from Transformers (BERT) - 双向编码器结构转换器10)Generative Pre-trained Transformer (GPT) - 生成式预训练转换器11)Transformer-based Models - 基于转换器的模型12)Masked Language Model (MLM) - 掩蔽语言模型13)Cloze Task - 填空任务14)Tokenization - 令牌化15)Word Embeddings - 词嵌入16)Sentence Embeddings - 句子嵌入17)Contextual Embeddings - 上下文嵌入18)Self-Supervised Learning - 自监督学习19)Large-Scale Pre-trained Models - 大规模预训练模型•Loss Function - 损失函数1)Loss Function - 损失函数2)Mean Squared Error (MSE) - 均方误差3)Mean Absolute Error (MAE) - 平均绝对误差4)Cross-Entropy Loss - 交叉熵损失5)Binary Cross-Entropy Loss - 二元交叉熵损失6)Categorical Cross-Entropy Loss - 分类交叉熵损失7)Hinge Loss - 合页损失8)Huber Loss - Huber损失9)Wasserstein Distance - Wasserstein距离10)Triplet Loss - 三元组损失11)Contrastive Loss - 对比损失12)Dice Loss - Dice损失13)Focal Loss - 焦点损失14)GAN Loss - GAN损失15)Adversarial Loss - 对抗损失16)L1 Loss - L1损失17)L2 Loss - L2损失18)Huber Loss - Huber损失19)Quantile Loss - 分位数损失•Activation Function - 激活函数1)Activation Function - 激活函数2)Sigmoid Function - Sigmoid函数3)Hyperbolic Tangent Function (Tanh) - 双曲正切函数4)Rectified Linear Unit (Re LU) - 矩形线性单元5)Parametric Re LU (P Re LU) - 参数化Re LU6)Exponential Linear Unit (ELU) - 指数线性单元7)Swish Function - Swish函数8)Softplus Function - Soft plus函数9)Softmax Function - SoftMax函数10)Hard Tanh Function - 硬双曲正切函数11)Softsign Function - Softsign函数12)GELU (Gaussian Error Linear Unit) - GELU(高斯误差线性单元)13)Mish Function - Mish函数14)CELU (Continuous Exponential Linear Unit) - CELU(连续指数线性单元)15)Bent Identity Function - 弯曲恒等函数16)Gaussian Error Linear Units (GELUs) - 高斯误差线性单元17)Adaptive Piecewise Linear (APL) - 自适应分段线性函数18)Radial Basis Function (RBF) - 径向基函数•Backpropagation - 反向传播1)Backpropagation - 反向传播2)Gradient Descent - 梯度下降3)Partial Derivative - 偏导数4)Chain Rule - 链式法则5)Forward Pass - 前向传播6)Backward Pass - 反向传播7)Computational Graph - 计算图8)Neural Network - 神经网络9)Loss Function - 损失函数10)Gradient Calculation - 梯度计算11)Weight Update - 权重更新12)Activation Function - 激活函数13)Optimizer - 优化器14)Learning Rate - 学习率15)Mini-Batch Gradient Descent - 小批量梯度下降16)Stochastic Gradient Descent (SGD) - 随机梯度下降17)Batch Gradient Descent - 批量梯度下降18)Momentum - 动量19)Adam Optimizer - Adam优化器20)Learning Rate Decay - 学习率衰减•Gradient Descent - 梯度下降1)Gradient Descent - 梯度下降2)Stochastic Gradient Descent (SGD) - 随机梯度下降3)Mini-Batch Gradient Descent - 小批量梯度下降4)Batch Gradient Descent - 批量梯度下降5)Learning Rate - 学习率6)Momentum - 动量7)Adaptive Moment Estimation (Adam) - 自适应矩估计8)RMSprop - 均方根传播9)Learning Rate Schedule - 学习率调度10)Convergence - 收敛11)Divergence - 发散12)Adagrad - 自适应学习速率方法13)Adadelta - 自适应增量学习率方法14)Adamax - 自适应矩估计的扩展版本15)Nadam - Nesterov Accelerated Adaptive Moment Estimation16)Learning Rate Decay - 学习率衰减17)Step Size - 步长18)Conjugate Gradient Descent - 共轭梯度下降19)Line Search - 线搜索20)Newton's Method - 牛顿法•Learning Rate - 学习率1)Learning Rate - 学习率2)Adaptive Learning Rate - 自适应学习率3)Learning Rate Decay - 学习率衰减4)Initial Learning Rate - 初始学习率5)Step Size - 步长6)Momentum - 动量7)Exponential Decay - 指数衰减8)Annealing - 退火9)Cyclical Learning Rate - 循环学习率10)Learning Rate Schedule - 学习率调度11)Warm-up - 预热12)Learning Rate Policy - 学习率策略13)Learning Rate Annealing - 学习率退火14)Cosine Annealing - 余弦退火15)Gradient Clipping - 梯度裁剪16)Adapting Learning Rate - 适应学习率17)Learning Rate Multiplier - 学习率倍增器18)Learning Rate Reduction - 学习率降低19)Learning Rate Update - 学习率更新20)Scheduled Learning Rate - 定期学习率•Batch Size - 批量大小1)Batch Size - 批量大小2)Mini-Batch - 小批量3)Batch Gradient Descent - 批量梯度下降4)Stochastic Gradient Descent (SGD) - 随机梯度下降5)Mini-Batch Gradient Descent - 小批量梯度下降6)Online Learning - 在线学习7)Full-Batch - 全批量8)Data Batch - 数据批次9)Training Batch - 训练批次10)Batch Normalization - 批量归一化11)Batch-wise Optimization - 批量优化12)Batch Processing - 批量处理13)Batch Sampling - 批量采样14)Adaptive Batch Size - 自适应批量大小15)Batch Splitting - 批量分割16)Dynamic Batch Size - 动态批量大小17)Fixed Batch Size - 固定批量大小18)Batch-wise Inference - 批量推理19)Batch-wise Training - 批量训练20)Batch Shuffling - 批量洗牌•Epoch - 训练周期1)Training Epoch - 训练周期2)Epoch Size - 周期大小3)Early Stopping - 提前停止4)Validation Set - 验证集5)Training Set - 训练集6)Test Set - 测试集7)Overfitting - 过拟合8)Underfitting - 欠拟合9)Model Evaluation - 模型评估10)Model Selection - 模型选择11)Hyperparameter Tuning - 超参数调优12)Cross-Validation - 交叉验证13)K-fold Cross-Validation - K折交叉验证14)Stratified Cross-Validation - 分层交叉验证15)Leave-One-Out Cross-Validation (LOOCV) - 留一法交叉验证16)Grid Search - 网格搜索17)Random Search - 随机搜索18)Model Complexity - 模型复杂度19)Learning Curve - 学习曲线20)Convergence - 收敛3.Machine Learning Techniques and Algorithms (机器学习技术与算法)•Decision Tree - 决策树1)Decision Tree - 决策树2)Node - 节点3)Root Node - 根节点4)Leaf Node - 叶节点5)Internal Node - 内部节点6)Splitting Criterion - 分裂准则7)Gini Impurity - 基尼不纯度8)Entropy - 熵9)Information Gain - 信息增益10)Gain Ratio - 增益率11)Pruning - 剪枝12)Recursive Partitioning - 递归分割13)CART (Classification and Regression Trees) - 分类回归树14)ID3 (Iterative Dichotomiser 3) - 迭代二叉树315)C4.5 (successor of ID3) - C4.5(ID3的后继者)16)C5.0 (successor of C4.5) - C5.0(C4.5的后继者)17)Split Point - 分裂点18)Decision Boundary - 决策边界19)Pruned Tree - 剪枝后的树20)Decision Tree Ensemble - 决策树集成•Random Forest - 随机森林1)Random Forest - 随机森林2)Ensemble Learning - 集成学习3)Bootstrap Sampling - 自助采样4)Bagging (Bootstrap Aggregating) - 装袋法5)Out-of-Bag (OOB) Error - 袋外误差6)Feature Subset - 特征子集7)Decision Tree - 决策树8)Base Estimator - 基础估计器9)Tree Depth - 树深度10)Randomization - 随机化11)Majority Voting - 多数投票12)Feature Importance - 特征重要性13)OOB Score - 袋外得分14)Forest Size - 森林大小15)Max Features - 最大特征数16)Min Samples Split - 最小分裂样本数17)Min Samples Leaf - 最小叶节点样本数18)Gini Impurity - 基尼不纯度19)Entropy - 熵20)Variable Importance - 变量重要性•Support Vector Machine (SVM) - 支持向量机1)Support Vector Machine (SVM) - 支持向量机2)Hyperplane - 超平面3)Kernel Trick - 核技巧4)Kernel Function - 核函数5)Margin - 间隔6)Support Vectors - 支持向量7)Decision Boundary - 决策边界8)Maximum Margin Classifier - 最大间隔分类器9)Soft Margin Classifier - 软间隔分类器10) C Parameter - C参数11)Radial Basis Function (RBF) Kernel - 径向基函数核12)Polynomial Kernel - 多项式核13)Linear Kernel - 线性核14)Quadratic Kernel - 二次核15)Gaussian Kernel - 高斯核16)Regularization - 正则化17)Dual Problem - 对偶问题18)Primal Problem - 原始问题19)Kernelized SVM - 核化支持向量机20)Multiclass SVM - 多类支持向量机•K-Nearest Neighbors (KNN) - K-最近邻1)K-Nearest Neighbors (KNN) - K-最近邻2)Nearest Neighbor - 最近邻3)Distance Metric - 距离度量4)Euclidean Distance - 欧氏距离5)Manhattan Distance - 曼哈顿距离6)Minkowski Distance - 闵可夫斯基距离7)Cosine Similarity - 余弦相似度8)K Value - K值9)Majority Voting - 多数投票10)Weighted KNN - 加权KNN11)Radius Neighbors - 半径邻居12)Ball Tree - 球树13)KD Tree - KD树14)Locality-Sensitive Hashing (LSH) - 局部敏感哈希15)Curse of Dimensionality - 维度灾难16)Class Label - 类标签17)Training Set - 训练集18)Test Set - 测试集19)Validation Set - 验证集20)Cross-Validation - 交叉验证•Naive Bayes - 朴素贝叶斯1)Naive Bayes - 朴素贝叶斯2)Bayes' Theorem - 贝叶斯定理3)Prior Probability - 先验概率4)Posterior Probability - 后验概率5)Likelihood - 似然6)Class Conditional Probability - 类条件概率7)Feature Independence Assumption - 特征独立假设8)Multinomial Naive Bayes - 多项式朴素贝叶斯9)Gaussian Naive Bayes - 高斯朴素贝叶斯10)Bernoulli Naive Bayes - 伯努利朴素贝叶斯11)Laplace Smoothing - 拉普拉斯平滑12)Add-One Smoothing - 加一平滑13)Maximum A Posteriori (MAP) - 最大后验概率14)Maximum Likelihood Estimation (MLE) - 最大似然估计15)Classification - 分类16)Feature Vectors - 特征向量17)Training Set - 训练集18)Test Set - 测试集19)Class Label - 类标签20)Confusion Matrix - 混淆矩阵•Clustering - 聚类1)Clustering - 聚类2)Centroid - 质心3)Cluster Analysis - 聚类分析4)Partitioning Clustering - 划分式聚类5)Hierarchical Clustering - 层次聚类6)Density-Based Clustering - 基于密度的聚类7)K-Means Clustering - K均值聚类8)K-Medoids Clustering - K中心点聚类9)DBSCAN (Density-Based Spatial Clustering of Applications with Noise) - 基于密度的空间聚类算法10)Agglomerative Clustering - 聚合式聚类11)Dendrogram - 系统树图12)Silhouette Score - 轮廓系数13)Elbow Method - 肘部法则14)Clustering Validation - 聚类验证15)Intra-cluster Distance - 类内距离16)Inter-cluster Distance - 类间距离17)Cluster Cohesion - 类内连贯性18)Cluster Separation - 类间分离度19)Cluster Assignment - 聚类分配20)Cluster Label - 聚类标签•K-Means - K-均值1)K-Means - K-均值2)Centroid - 质心3)Cluster - 聚类4)Cluster Center - 聚类中心5)Cluster Assignment - 聚类分配6)Cluster Analysis - 聚类分析7)K Value - K值8)Elbow Method - 肘部法则9)Inertia - 惯性10)Silhouette Score - 轮廓系数11)Convergence - 收敛12)Initialization - 初始化13)Euclidean Distance - 欧氏距离14)Manhattan Distance - 曼哈顿距离15)Distance Metric - 距离度量16)Cluster Radius - 聚类半径17)Within-Cluster Variation - 类内变异18)Cluster Quality - 聚类质量19)Clustering Algorithm - 聚类算法20)Clustering Validation - 聚类验证•Dimensionality Reduction - 降维1)Dimensionality Reduction - 降维2)Feature Extraction - 特征提取3)Feature Selection - 特征选择4)Principal Component Analysis (PCA) - 主成分分析5)Singular Value Decomposition (SVD) - 奇异值分解6)Linear Discriminant Analysis (LDA) - 线性判别分析7)t-Distributed Stochastic Neighbor Embedding (t-SNE) - t-分布随机邻域嵌入8)Autoencoder - 自编码器9)Manifold Learning - 流形学习10)Locally Linear Embedding (LLE) - 局部线性嵌入11)Isomap - 等度量映射12)Uniform Manifold Approximation and Projection (UMAP) - 均匀流形逼近与投影13)Kernel PCA - 核主成分分析14)Non-negative Matrix Factorization (NMF) - 非负矩阵分解15)Independent Component Analysis (ICA) - 独立成分分析16)Variational Autoencoder (VAE) - 变分自编码器17)Sparse Coding - 稀疏编码18)Random Projection - 随机投影19)Neighborhood Preserving Embedding (NPE) - 保持邻域结构的嵌入20)Curvilinear Component Analysis (CCA) - 曲线成分分析•Principal Component Analysis (PCA) - 主成分分析1)Principal Component Analysis (PCA) - 主成分分析2)Eigenvector - 特征向量3)Eigenvalue - 特征值4)Covariance Matrix - 协方差矩阵。
abstract的单词
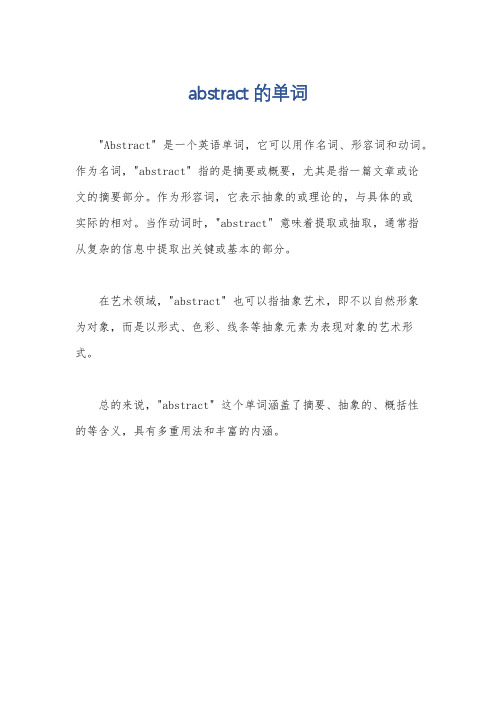
abstract的单词
"Abstract" 是一个英语单词,它可以用作名词、形容词和动词。
作为名词,"abstract" 指的是摘要或概要,尤其是指一篇文章或论
文的摘要部分。
作为形容词,它表示抽象的或理论的,与具体的或
实际的相对。
当作动词时,"abstract" 意味着提取或抽取,通常指
从复杂的信息中提取出关键或基本的部分。
在艺术领域,"abstract" 也可以指抽象艺术,即不以自然形象
为对象,而是以形式、色彩、线条等抽象元素为表现对象的艺术形式。
总的来说,"abstract" 这个单词涵盖了摘要、抽象的、概括性
的等含义,具有多重用法和丰富的内涵。
卷积神经网络机器学习外文文献翻译中英文2020
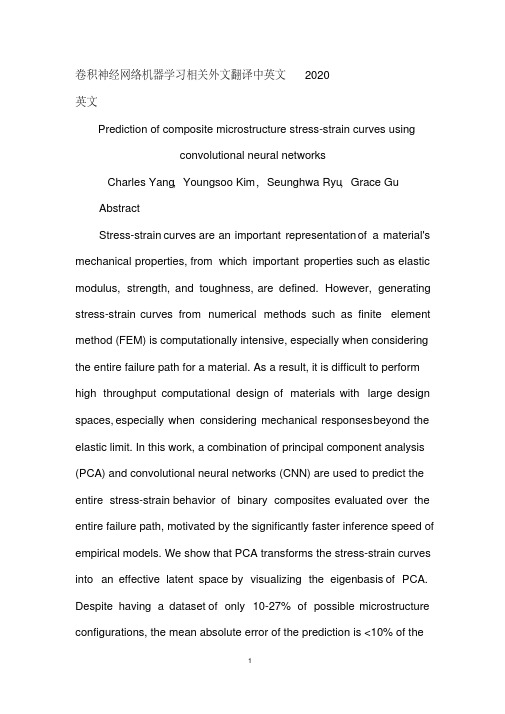
卷积神经网络机器学习相关外文翻译中英文2020英文Prediction of composite microstructure stress-strain curves usingconvolutional neural networksCharles Yang,Youngsoo Kim,Seunghwa Ryu,Grace GuAbstractStress-strain curves are an important representation of a material's mechanical properties, from which important properties such as elastic modulus, strength, and toughness, are defined. However, generating stress-strain curves from numerical methods such as finite element method (FEM) is computationally intensive, especially when considering the entire failure path for a material. As a result, it is difficult to perform high throughput computational design of materials with large design spaces, especially when considering mechanical responses beyond the elastic limit. In this work, a combination of principal component analysis (PCA) and convolutional neural networks (CNN) are used to predict the entire stress-strain behavior of binary composites evaluated over the entire failure path, motivated by the significantly faster inference speed of empirical models. We show that PCA transforms the stress-strain curves into an effective latent space by visualizing the eigenbasis of PCA. Despite having a dataset of only 10-27% of possible microstructure configurations, the mean absolute error of the prediction is <10% of therange of values in the dataset, when measuring model performance based on derived material descriptors, such as modulus, strength, and toughness. Our study demonstrates the potential to use machine learning to accelerate material design, characterization, and optimization.Keywords:Machine learning,Convolutional neural networks,Mechanical properties,Microstructure,Computational mechanics IntroductionUnderstanding the relationship between structure and property for materials is a seminal problem in material science, with significant applications for designing next-generation materials. A primary motivating example is designing composite microstructures for load-bearing applications, as composites offer advantageously high specific strength and specific toughness. Recent advancements in additive manufacturing have facilitated the fabrication of complex composite structures, and as a result, a variety of complex designs have been fabricated and tested via 3D-printing methods. While more advanced manufacturing techniques are opening up unprecedented opportunities for advanced materials and novel functionalities, identifying microstructures with desirable properties is a difficult optimization problem.One method of identifying optimal composite designs is by constructing analytical theories. For conventional particulate/fiber-reinforced composites, a variety of homogenizationtheories have been developed to predict the mechanical properties of composites as a function of volume fraction, aspect ratio, and orientation distribution of reinforcements. Because many natural composites, synthesized via self-assembly processes, have relatively periodic and regular structures, their mechanical properties can be predicted if the load transfer mechanism of a representative unit cell and the role of the self-similar hierarchical structure are understood. However, the applicability of analytical theories is limited in quantitatively predicting composite properties beyond the elastic limit in the presence of defects, because such theories rely on the concept of representative volume element (RVE), a statistical representation of material properties, whereas the strength and failure is determined by the weakest defect in the entire sample domain. Numerical modeling based on finite element methods (FEM) can complement analytical methods for predicting inelastic properties such as strength and toughness modulus (referred to as toughness, hereafter) which can only be obtained from full stress-strain curves.However, numerical schemes capable of modeling the initiation and propagation of the curvilinear cracks, such as the crack phase field model, are computationally expensive and time-consuming because a very fine mesh is required to accommodate highly concentrated stress field near crack tip and the rapid variation of damage parameter near diffusive cracksurface. Meanwhile, analytical models require significant human effort and domain expertise and fail to generalize to similar domain problems.In order to identify high-performing composites in the midst of large design spaces within realistic time-frames, we need models that can rapidly describe the mechanical properties of complex systems and be generalized easily to analogous systems. Machine learning offers the benefit of extremely fast inference times and requires only training data to learn relationships between inputs and outputs e.g., composite microstructures and their mechanical properties. Machine learning has already been applied to speed up the optimization of several different physical systems, including graphene kirigami cuts, fine-tuning spin qubit parameters, and probe microscopy tuning. Such models do not require significant human intervention or knowledge, learn relationships efficiently relative to the input design space, and can be generalized to different systems.In this paper, we utilize a combination of principal component analysis (PCA) and convolutional neural networks (CNN) to predict the entire stress-strain c urve of composite failures beyond the elastic limit. Stress-strain curves are chosen as the model's target because t hey are difficult to predict given their high dimensionality. In addition, stress-strain curves are used to derive important material descriptors such as modulus, strength, and toughness. In this sense, predicting stress-straincurves is a more general description of composites properties than any combination of scaler material descriptors. A dataset of 100,000 different composite microstructures and their corresponding stress-strain curves are used to train and evaluate model performance. Due to the high dimensionality of the stress-strain dataset, several dimensionality reduction methods are used, including PCA, featuring a blend of domain understanding and traditional machine learning, to simplify the problem without loss of generality for the model.We will first describe our modeling methodology and the parameters of our finite-element method (FEM) used to generate data. Visualizations of the learned PCA latent space are then presented, a long with model performance results.CNN implementation and trainingA convolutional neural network was trained to predict this lower dimensional representation of the stress vector. The input to the CNN was a binary matrix representing the composite design, with 0's corresponding to soft blocks and 1's corresponding to stiff blocks. PCA was implemented with the open-source Python package scikit-learn, using the default hyperparameters. CNN was implemented using Keras with a TensorFlow backend. The batch size for all experiments was set to 16 and the number of epochs to 30; the Adam optimizer was used to update the CNN weights during backpropagation.A train/test split ratio of 95:5 is used –we justify using a smaller ratio than the standard 80:20 because of a relatively large dataset. With a ratio of 95:5 and a dataset with 100,000 instances, the test set size still has enough data points, roughly several thousands, for its results to generalize. Each column of the target PCA-representation was normalized to have a mean of 0 and a standard deviation of 1 to prevent instable training.Finite element method data generationFEM was used to generate training data for the CNN model. Although initially obtained training data is compute-intensive, it takes much less time to train the CNN model and even less time to make high-throughput inferences over thousands of new, randomly generated composites. The crack phase field solver was based on the hybrid formulation for the quasi-static fracture of elastic solids and implementedin the commercial FEM software ABAQUS with a user-element subroutine (UEL).Visualizing PCAIn order to better understand the role PCA plays in effectively capturing the information contained in stress-strain curves, the principal component representation of stress-strain curves is plotted in 3 dimensions. Specifically, we take the first three principal components, which have a cumulative explained variance ~85%, and plot stress-strain curves in that basis and provide several different angles from which toview the 3D plot. Each point represents a stress-strain curve in the PCA latent space and is colored based on the associated modulus value. it seems that the PCA is able to spread out the curves in the latent space based on modulus values, which suggests that this is a useful latent space for CNN to make predictions in.CNN model design and performanceOur CNN was a fully convolutional neural network i.e. the only dense layer was the output layer. All convolution layers used 16 filters with a stride of 1, with a LeakyReLU activation followed by BatchNormalization. The first 3 Conv blocks did not have 2D MaxPooling, followed by 9 conv blocks which did have a 2D MaxPooling layer, placed after the BatchNormalization layer. A GlobalAveragePooling was used to reduce the dimensionality of the output tensor from the sequential convolution blocks and the final output layer was a Dense layer with 15 nodes, where each node corresponded to a principal component. In total, our model had 26,319 trainable weights.Our architecture was motivated by the recent development and convergence onto fully-convolutional architectures for traditional computer vision applications, where convolutions are empirically observed to be more efficient and stable for learning as opposed to dense layers. In addition, in our previous work, we had shown that CNN's werea capable architecture for learning to predict mechanical properties of 2Dcomposites [30]. The convolution operation is an intuitively good fit forpredicting crack propagation because it is a local operation, allowing it toimplicitly featurize and learn the local spatial effects of crack propagation.After applying PCA transformation to reduce the dimensionality ofthe target variable, CNN is used to predict the PCA representation of thestress-strain curve of a given binary composite design. After training theCNN on a training set, its ability to generalize to composite designs it hasnot seen is evaluated by comparing its predictions on an unseen test set.However, a natural question that emerges i s how to evaluate a model's performance at predicting stress-strain curves in a real-world engineeringcontext. While simple scaler metrics such as mean squared error (MSE)and mean absolute error (MAE) generalize easily to vector targets, it isnot clear how to interpret these aggregate summaries of performance. It isdifficult to use such metrics to ask questions such as “Is this modeand “On average, how poorly will aenough to use in the real world” given prediction be incorrect relative to some given specification”. Although being able to predict stress-strain curves is an importantapplication of FEM and a highly desirable property for any machinelearning model to learn, it does not easily lend itself to interpretation. Specifically, there is no simple quantitative way to define whether two-world units.stress-s train curves are “close” or “similar” with real Given that stress-strain curves are oftentimes intermediary representations of a composite property that are used to derive more meaningful descriptors such as modulus, strength, and toughness, we decided to evaluate the model in an analogous fashion. The CNN prediction in the PCA latent space representation is transformed back to a stress-strain curve using PCA, and used to derive the predicted modulus, strength, and toughness of the composite. The predicted material descriptors are then compared with the actual material descriptors. In this way, MSE and MAE now have clearly interpretable units and meanings. The average performance of the model with respect to the error between the actual and predicted material descriptor values derived from stress-strain curves are presented in Table. The MAE for material descriptors provides an easily interpretable metric of model performance and can easily be used in any design specification to provide confidence estimates of a model prediction. When comparing the mean absolute error (MAE) to the range of values taken on by the distribution of material descriptors, we can see that the MAE is relatively small compared to the range. The MAE compared to the range is <10% for all material descriptors. Relatively tight confidence intervals on the error indicate that this model architecture is stable, the model performance is not heavily dependent on initialization, and that our results are robust to differenttrain-test splits of the data.Future workFuture work includes combining empirical models with optimization algorithms, such as gradient-based methods, to identify composite designs that yield complementary mechanical properties. The ability of a trained empirical model to make high-throughput predictions over designs it has never seen before allows for large parameter space optimization that would be computationally infeasible for FEM. In addition, we plan to explore different visualizations of empirical models-box” of such models. Applying machine in an effort to “open up the blacklearning to finite-element methods is a rapidly growing field with the potential to discover novel next-generation materials tailored for a variety of applications. We also note that the proposed method can be readily applied to predict other physical properties represented in a similar vectorized format, such as electron/phonon density of states, and sound/light absorption spectrum.ConclusionIn conclusion, we applied PCA and CNN to rapidly and accurately predict the stress-strain curves of composites beyond the elastic limit. In doing so, several novel methodological approaches were developed, including using the derived material descriptors from the stress-strain curves as interpretable metrics for model performance and dimensionalityreduction techniques to stress-strain curves. This method has the potential to enable composite design with respect to mechanical response beyond the elastic limit, which was previously computationally infeasible, and can generalize easily to related problems outside of microstructural design for enhancing mechanical properties.中文基于卷积神经网络的复合材料微结构应力-应变曲线预测查尔斯,吉姆,瑞恩,格瑞斯摘要应力-应变曲线是材料机械性能的重要代表,从中可以定义重要的性能,例如弹性模量,强度和韧性。
Hopfield神经网络综述

Hopfield神经⽹络综述题⽬: Hopfield神经⽹络综述⼀、概述:1.什么是⼈⼯神经⽹络(Artificial Neural Network,ANN)⼈⼯神经⽹络是⼀个并⾏和分布式的信息处理⽹络结构,该⽹络结构⼀般由许多个神经元组成,每个神经元有⼀个单⼀的输出,它可以连接到很多其他的神经元,其输⼊有多个连接通路,每个连接通路对应⼀个连接权系数。
⼈⼯神经⽹络系统是以⼯程技术⼿段来模拟⼈脑神经元(包括细胞体,树突,轴突)⽹络的结构与特征的系统。
利⽤⼈⼯神经元可以构成各种不同拓扑结构的神经⽹络,它是⽣物神经⽹络的⼀种模拟和近似。
主要从两个⽅⾯进⾏模拟:⼀是结构和实现机理;⼆是从功能上加以模拟。
根据神经⽹络的主要连接型式⽽⾔,⽬前已有数⼗种不同的神经⽹络模型,其中前馈型⽹络和反馈型⽹络是两种典型的结构模型。
1)反馈神经⽹络(Recurrent Network)反馈神经⽹络,⼜称⾃联想记忆⽹络,其⽬的是为了设计⼀个⽹络,储存⼀组平衡点,使得当给⽹络⼀组初始值时,⽹络通过⾃⾏运⾏⽽最终收敛到这个设计的平衡点上。
反馈神经⽹络是⼀种将输出经过⼀步时移再接⼊到输⼊层的神经⽹络系统。
反馈⽹络能够表现出⾮线性动⼒学系统的动态特性。
它所具有的主要特性为以下两点:(1).⽹络系统具有若⼲个稳定状态。
当⽹络从某⼀初始状态开始运动,⽹络系统总可以收敛到某⼀个稳定的平衡状态;(2).系统稳定的平衡状态可以通过设计⽹络的权值⽽被存储到⽹络中。
反馈⽹络是⼀种动态⽹络,它需要⼯作⼀段时间才能达到稳定。
该⽹络主要⽤于联想记忆和优化计算。
在这种⽹络中,每个神经元同时将⾃⾝的输出信号作为输⼊信号反馈给其他神经元,它需要⼯作⼀段时间才能达到稳定。
2.Hopfield神经⽹络Hopfield⽹络是神经⽹络发展历史上的⼀个重要的⾥程碑。
由美国加州理⼯学院物理学家J.J.Hopfield 教授于1982年提出,是⼀种单层反馈神经⽹络。
Hopfield神经⽹络是反馈⽹络中最简单且应⽤⼴泛的模型,它具有联想记忆的功能。
神经网络简介
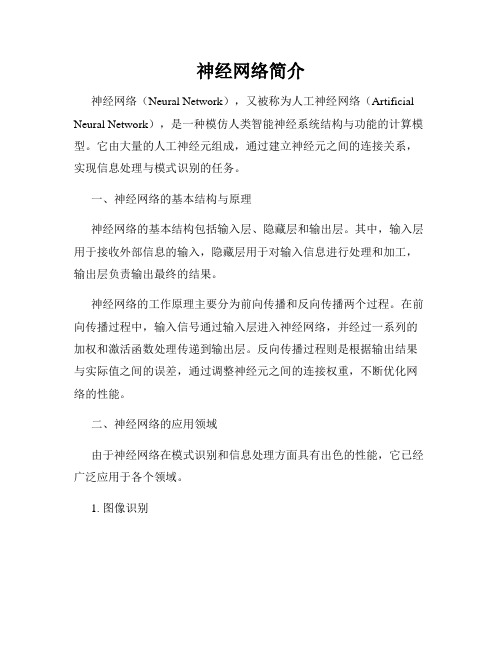
神经网络简介神经网络(Neural Network),又被称为人工神经网络(Artificial Neural Network),是一种模仿人类智能神经系统结构与功能的计算模型。
它由大量的人工神经元组成,通过建立神经元之间的连接关系,实现信息处理与模式识别的任务。
一、神经网络的基本结构与原理神经网络的基本结构包括输入层、隐藏层和输出层。
其中,输入层用于接收外部信息的输入,隐藏层用于对输入信息进行处理和加工,输出层负责输出最终的结果。
神经网络的工作原理主要分为前向传播和反向传播两个过程。
在前向传播过程中,输入信号通过输入层进入神经网络,并经过一系列的加权和激活函数处理传递到输出层。
反向传播过程则是根据输出结果与实际值之间的误差,通过调整神经元之间的连接权重,不断优化网络的性能。
二、神经网络的应用领域由于神经网络在模式识别和信息处理方面具有出色的性能,它已经广泛应用于各个领域。
1. 图像识别神经网络在图像识别领域有着非常广泛的应用。
通过对图像进行训练,神经网络可以学习到图像中的特征,并能够准确地判断图像中的物体种类或者进行人脸识别等任务。
2. 自然语言处理在自然语言处理领域,神经网络可以用于文本分类、情感分析、机器翻译等任务。
通过对大量语料的学习,神经网络可以识别文本中的语义和情感信息。
3. 金融预测与风险评估神经网络在金融领域有着广泛的应用。
它可以通过对历史数据的学习和分析,预测股票价格走势、评估风险等,并帮助投资者做出更科学的决策。
4. 医学诊断神经网络在医学领域的应用主要体现在医学图像分析和诊断方面。
通过对医学影像进行处理和分析,神经网络可以辅助医生进行疾病的诊断和治疗。
5. 机器人控制在机器人领域,神经网络可以用于机器人的感知与控制。
通过将传感器数据输入到神经网络中,机器人可以通过学习和训练来感知环境并做出相应的反应和决策。
三、神经网络的优缺点虽然神经网络在多个领域中都有着广泛的应用,但它也存在一些优缺点。
神经网络基本介绍PPT课件
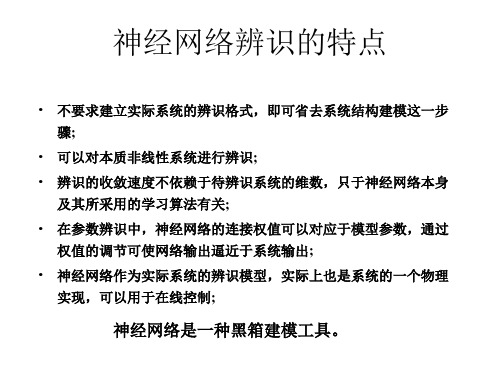
神经系统的基本构造是神经元(神经细胞 ),它是处理人体内各部分之间相互信息传 递的基本单元。
每个神经元都由一个细胞体,一个连接 其他神经元的轴突和一些向外伸出的其它 较短分支—树突组成。
轴突功能是将本神经元的输出信号(兴奋 )传递给别的神经元,其末端的许多神经末 梢使得兴奋可以同时传送给多个神经元。
将神经网络与专家系统、模糊逻辑、遗传算法 等相结合,可设计新型智能控制系统。
(4) 优化计算 在常规的控制系统中,常遇到求解约束
优化问题,神经网络为这类问题的解决提供 了有效的途径。
常规模型结构的情况下,估计模型的参数。 ② 利用神经网络的线性、非线性特性,可建立线
性、非线性系统的静态、动态、逆动态及预测 模型,实现非线性系统的建模。
(2) 神经网络控制器 神经网络作为实时控制系统的控制器,对不
确定、不确知系统及扰动进行有效的控制,使控 制系统达到所要求的动态、静态特性。 (3) 神经网络与其他算法相结合
4 新连接机制时期(1986-现在) 神经网络从理论走向应用领域,出现
了神经网络芯片和神经计算机。 神经网络主要应用领域有:模式识别
与图象处理(语音、指纹、故障检测和 图象压缩等)、控制与优化、系统辨识 、预测与管理(市场预测、风险分析) 、通信等。
神经网络原理 神经生理学和神经解剖学的研究表 明,人脑极其复杂,由一千多亿个神经 元交织在一起的网状结构构成,其中大 脑 皮 层 约 140 亿 个 神 经 元 , 小 脑 皮 层 约 1000亿个神经元。 人脑能完成智能、思维等高级活动 ,为了能利用数学模型来模拟人脑的活 动,导致了神经网络的研究。
(2) 学习与遗忘:由于神经元结构的可塑 性,突触的传递作用可增强和减弱,因 此神经元具有学习与遗忘的功能。 决定神经网络模型性能三大要素为:
神经网络辨识英文资料
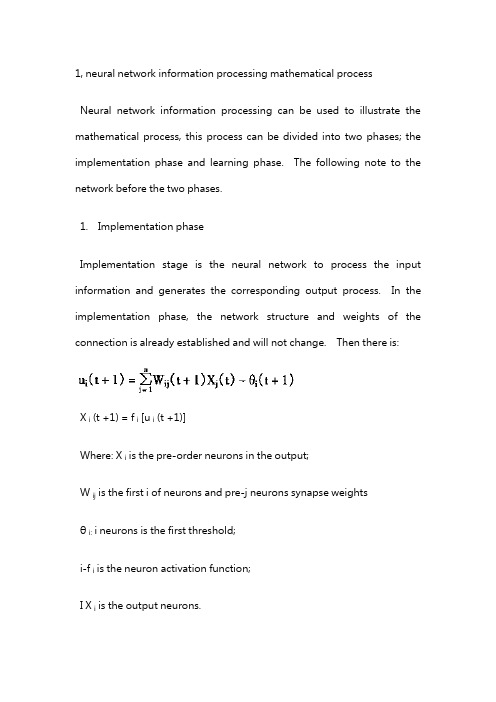
1, neural network information processing mathematical processNeural network information processing can be used to illustrate the mathematical process, this process can be divided into two phases; the implementation phase and learning phase. The following note to the network before the two phases.1. Implementation phaseImplementation stage is the neural network to process the input information and generates the corresponding output process. In the implementation phase, the network structure and weights of the connection is already established and will not change. Then there is:X i (t +1) = f i [u i (t +1)]Where: X i is the pre-order neurons in the output;W ij is the first i of neurons and pre-j neurons synapse weightsθ i: i neurons is the first threshold;i-f i is the neuron activation function;I X i is the output neurons.2. Learning phaseNeural network learning phase is from the sound stage; this time, the learning network according to certain rule changes synaptic weights W ij,in order to enable end fixed measure function E is minimized. General access:E = (T i, X i) (1-9)Where, T i is the teacher signal;X i is the neuron output.Learning formula can be expressed as the following mathematical expression:Where: Ψ is a nonlinear function;η ij is the weight rate of change;n is the number of iterations during learning.For the gradient learning algorithm, you can use the following specific formula:Neural networks of information processing in general need to learn and implementation phases and combined to achieve a reasonable process. Neural network learning is to obtain information on the adaptability of information, or information of the characteristics; and neural network implementation process of information is characteristic of information retrieval or classification process.Learning and neural network implementation is indispensable to the two treatment and function. Neural network behavior and the role of various effective are two key processes by which to achieve.Through the study phase, can be a pair neural network training mode is particularly sensitive information, or have some characteristics of dynamic systems. Through the implementation phase, you can use neural networks to identify the information model or feature.In intelligent control, using neural network as controller, then the neural network learning is to learn the characteristics of controlled object, so that neural network can adapt to the input-output relationship between the controlled object; Thus, in implementation, neural network will be able to learn the knowledge of an object to achieve just the right control.Second, back-propagation BP modelNeural network learning is one of the most important and most impressive features. In neural network development process, learning algorithm has a very important position. At present, people put forward neural network model and learning algorithm are appropriate. So, sometimes people do not go to pray on the model and algorithm are strict definition or distinction. Some models can have a variety of algorithms. However, some algorithms may be used for a variety of models. However, sometimes also known as the model algorithm.Since the 40's Hebb learning rule has been proposed, people have proposed a variety of learning algorithms. Among them, in 1986, proposed by Rumelhart and other back-propagation method, that is, BP (error BackPropagation) method most widely affected. Even today, BP control algorithm is still the most important application of the most effective algorithm.1.2.1 Neural network learning mechanisms and institutionsIn the neural network, the model provided on the external environment to learn the training samples, and to store this model is called sensor; ability to adapt to external environment, can automatically extract the external environmental characteristics, is called cognitive device .Neural Networks in the study, generally divided into a study of two teachers and not teachers. Sensor signal by a teacher to learn, and cognitive devicesare used to learn without teacher signals. Such as BP neural network in the main network, Hopfield network, ART network and Kohonen network; BP network and Hopfield network is necessary for teachers to learn the signal can be; and ART network and Kohonen network signals do not need teachers to learn. The so-called teacher signal, that is, learning in neural network model of sample provided by an external signal.First, the learning structure of sensorPerceptron learning is the most typical neural network learning.At present, the control application is a multilayer feedforward network, which is a sensor model, learning algorithm is BP method, it is a supervised learning algorithm.A teacher of the learning system can be expressed in Figure 1-7. This learning system is divided into three parts: input Ministry of Training Department of the Ministry and output.Input received from outside the Department of input samples X, conducted by the Training Department to adjust the network weights W, and then the Department of the output from the output. Zai this process, the desired output signal can be used as teacher signal input, by the teacher signal and the actual output Jinxingbijiao, produce the Wucha right to Kongzhixiugai系数W.Learning organization structure can be expressed as shown in Figure 1-8.In the figure, X l, X 2, ..., X n, is the input sample signals, W 1, W 2, ..., W n are weights. Input sample signal X i can take discrete values "0" or "1." Input sample signa ls weights role in the u produces the output ΣW i X i, that is:u = ΣW i X i = W 1 X 1 + W 2 X 2 + ... + W n X nThen the desired output signal Y (t) and u compare the resulting error signal e. Body weight that is adjusted according to the error e to the power factor of the learning system be modified, modify the direction of the error e should be made smaller, and constantly go on, so that the error e is zero, then the actual output value of u and the desired output value Y ( t) exactly the same, then the end of the learning process.Neural network learning generally require repeated training, error tends gradually to zero, and finally reaches zero. Then the output will be consistent with expectations. neural network learning is the consumption of a certain period, some of the learning process to be repeated many times, even up to 10 000 secondary. The reason is that neural network weights W have a lot of weight W 1, W 2 ,---- W n; that is, more than one parameter to modify the system. Adjusting the system parameters must be time-consuming consumption. At present, the neural network to improve the learning speed and reduce thenumber of repeat learn the importance of research topic is real-time control of the key issues.Second, Perceptron learning algorithmSensor is a single-layer neural network computing unit, from the linear elements and the threshold component composition. Sensor shown in Figure 1-9.Figure 1-9 Sensor structureThe mathematical model of sensor:Where: f [.] Is a step function, and thereθ is the threshold.The greatest effect sensor is able to enter the sample classificationThat is, when the sensor output to 1, the input samples as A; output is -1, the input sample as B class. From the sensor can see the classification boundaries are:Only two components in the input sample X1, X2, then a classification boundary conditions:ThatW 1 X 1 + W 2 X 2-θ = 0 (1-17)Can also be written asThen the classification as shown in solid 1-10.Perceptron learning algorithm aims to find appropriate weights w = (w1.w2, ..., Wn), the system for a particular sample x = (xt, x2, ..., xn) Bear generate expectations d. When x is classified as category A, the expected value of d = 1; X to B class, d =- 1. To facilitate the description perceptron learningalgorithm, the threshold θ and w in the human factor, while the corresponding increase in the sample x is also a component of x n +1.So that:W n +1 =- θ, X n +1 = 1 (1-19)The sensor output can be expressed as:Perceptron learning algorithm as follows:1. Set initial value of the weights wOn the weights w = (W 1. W 2, ..., W n, W n +1) of the various components of the zero set of a small random value, but W n +1 =-G. And recorded as W l (0), W 2 (0), ..., W n (0), while there Wn +1 (0) =- θ. Where W i (t) as the time from i-tEnter the weight coefficient, i = 1,2, ..., n. W n +1 (t) for the time t when the threshold.2. Enter the same as the X = (X 1, X 2, ..., X n +1) and its expected output d. Desired output value d in samples of different classes are not the same time value. If x is A class, then take d = 1, if x is B, then take -1. The desired output signal d that is, the teacher.3. Calculate the actual output value of Y4. According to the actual output error e requeste = d-Y (t) (1-21)5. With error e to modify the weightsi = 1,2, ..., n, n +1 (1-22)Where, η is called the weight change rate, 0 <η ≤ 1In equation (1-22) in, η the value can not be too much. If a value too large will affect the w i (t) stability; the value can not be too small, too small will make W i (t) the process of deriving the convergence rate is too slow.When the actual output and expected the same d are:W i (t +1) = W i (t)6. Go to point 2, has been implementing to all the samples were stable. From the above equation (1-14) known, sensor is actually a classifier, it is this classification and the corresponding binary logic. Therefore, the sensor can be used to implement logic functions. Sensor to achieve the following logic function on the situation of some description.Example: Using sensors to achieve the logic function X 1 VX 2 of the true value:To X1VX2 = 1 for the A class to X1VX2 = 0 for the B category, there are equationsThat is:From (1-24) are:W 1≥θ, W 2≥θSo that W 1 = 1, W 2 = 2Have: θ ≤ 1Take θ = 0.5There are: X1 + X2-0.5 = 0, the classification shown in Figure 1-11.Figure 1-11 Logic Function X 1 VX 2 classification1.2.2 Gradient Neural Network LearningDevice from the flu, such as the learning algorithm known, the purpose of study is on changes in the network weights, so that the network model for the input samples can be correctly classified. When the study ended, that is when the neural network correctly classified, the weight coefficient is clearly reflected in similar samples of the input common mode characteristics. In other words, weight is stored in the input mode. As the power factor is theexisting decentralized, so there is a natural neural network distributed storage features.Sensor in front of the transfer function is a step function, so it can be used as a classifier. The previous section about the Perceptron learning algorithm because of its transfer function is simple and limitations.Perceptron learning algorithm is quite simple, and when the function to ensure convergence are linearly separable. But it is also problematic: that function is not linearly separable, then seek no results; Also, can not be extended to the general feed-forward network.In order to overcome the problems, so people put forward an alternative algorithm - gradient algorithm (that is, LMS method).In order to achieve gradient algorithm, so the neurons can be differential excitation function to function, such as Sigmoid function, Asymmetric Sigmoid function f (X) = 1 / (1 + e-x), Symmetric Sigmoid function f (X) = (1-e-x) / (1 + e-x); instead of type (1-13) of the step function.For a given sample set X i (i = 1,2,, n), gradient method seeks to find weights W *, so f [W *. X i] and the desired output Yi as close as possible.Set error e using the following formula, said:Where, Y i = f 〔W *· X i] is the corresponding sample X i s i real-time output I-Y i is the corresponding sample X i of the desired output.For the smallest error e, can first obtain the gradient of e:Of which:So that U k = W. X k, there are:That is:Finally, the negative gradient direction changes according to the weight coefficient W, amend the rules:Can also be written as:In the last type (1-30), type (1-31) in, μ is the weight change rate, the situation is different depending on different values, usually take between 0-1 decimal. Obviously, the gradient method than the original perceptron learning algorithm into a big step. The key lies in two things:1. Neuron transfer function using a continuous s-type function, rather than the step function;2. Changes on the weight coefficient used to control the error of gradient, rather than to control the error. dynamic characteristics can be better, that enhance its convergence process.But the gradient method for the actual study, the feeling is still too slow; Therefore, this algorithm is still not ideal.1.2.3 BP algorithm back-propagation learningBack-propagation algorithm, also known as BP. Because of this algorithm is essentially a mathematical model of neural network, so, sometimes referred to as BP model.BP algorithm is to solve the multilayer feedforward neural network weights optimization of their argument; Therefore, BP algorithm is also usually impliesthat the topology of neural network is a multilayer no feedback to the network. . Sometimes also called non-feedback neural networks using the BP model.Here, not too hard to distinguish between arguments and the relevant algorithms and models of both similarities and differences. Perceptron learning algorithm is a single-layer network learning algorithm. In the multi-layer network. It can only change the final weights. Therefore, the perceptron learning algorithm can not be used for multi-layer neural network learning. In 1986, Rumelhart proposed back propagation learning algorithm, that is, BP (backpropagation) algorithm. This algorithm can be in each layer, to amend the Weights and therefore suitable for multi-network learning. BP algorithm is the most widely used learning algorithm of neural network is one of the most useful in the control of the learning algorithm.1, BP algorithm theoryBP algorithm is used for feed-forward multi-layer network learning algorithm It contains input and output layer and input and output layers in the middle layer. The middle layer has single or multi-layer, because they have no direct contact with the outside world, it is also known as the hidden layer. In the hidden layer neurons, also known as hidden units. Although the hidden layer and the outside world are not connected. However, their status will affect therelationship between input and output. It is also said to change the hidden layer weights, you can change the multi-layer neural network performance.M with a layer of neural network and the input layer plus a sample of X; set the first layer of i k input neurons is expressed as the sum of U i k, the output X i k; k-1 layer from the first j months neuron to i-k layer neurons coefficient W ij the weight each neuron excitation function f, then the relationship between various variables related to mathematics can be expressed as the following:X i k = f (U i k)Back-propagation algorithm is divided into two parts, namely, forward propagation and back propagation. The work of these two processes are summarized below.1. Forward propagationInput samples from the input layer after layer of a layer of hidden units for processing, after the adoption of all the hidden layer, then transmitted to the output layer; in the process of layer processing, the state of neurons in each layer under a layer of nerve only element of state influence. In the output layer to the current output and expected output compare, if the current output is not equal to expected output, then enter the back-propagation process.2. Back-propagationReverse propagation, the error signal being transmitted by the original return path back, and each hidden layer neuron weights all be modified to look towards the smallest error signal.Second, BP algorithm is a mathematical expressionBP algorithm is essentially the problem to obtain the minimum error function. This algorithm uses linear programming in the steepest descent method, according to the negative gradient of error function changes the direction of weights.To illustrate the BP algorithm, first define the error function e. Get the desired output and the square of the difference between actual output and the error function, there are:Where: Y i is the expected output units; it is here used as teacher signals;X i m is the actual output; because the first m layer is output layer.As the BP algorithm by error function e of the negative gradient direction changes the weight coefficient, it changes the weight coefficient W ij the amount Aw ij, and eWhere: η is learning rate, that step.Clearly, according to the principles of BP algorithm, seeking ae / aW ij the most critical. The following requirements ae / aW ij; haveAsWhere: η is learning rate, that step, and generally the number between 0-1. Can see from above, d i k the actual algorithm is still significant given the end of the formula, the following requirements d i k formula.To facilitate derivation, taking f is continuous. And generally the non-linear continuous function, such as Sigmoid function. When taking a non-symmetrical Sigmoid function f, are:Have: f '(U i k) = f' (U i k) (1-f (U i k))= X i k (1-X i k) (1-45)Consider equation (1-43) in the partial differential ae / aX i k, there are two cases to be considered:If k = m, is the output layer, then there is Y i is the expected output, it is constant. From (1-34) haveThus d i m = X i m (1-X i m) (X i m-Y i)2. If k <m, then the layer is hidden layer. Then it should be considered on the floor effect, it has:From (1-41), the known include:From (1-33), the known are:Can see from the above process: multi-layer network training method is to add a sample of the input layer, and spread under the former rules:X i k = f (U i k)Keep one level to the output layer transfer, the final output in the output layer can be X i m.The Xim and compare the expected output Yi. If the two ranges, the resulting error signal eNumber of samples by repeated training, while gradually reducing the error on the right direction factor is corrected to achieve the eventual elimination of error. From the above formula can also be aware that if the network layer is higher, the use of a considerable amount of computation, slow convergence speed.To speed up the convergence rate, generally considered the last of the weight coefficient, and to amend it as the basis of this one, a modified formula:W here: η is the learning rate that step, η = 0.1-0.4 or soɑ constant for the correction weights, taking around 0.7-0.9.In the above formula (1-53) also known as the generalized Delta rule. For there is no hidden layer neural network, it is desirableWhere:, Y i is the desired output;X j is the actual output of output layer;X i for the input layer of input.This is obviously a very simple case, equation (1-55), also known as a simple Delta rule.In practice, only the generalized Delta rule type (1-53) or type (1-54) makes sense. Simple Delta rule type (1-55) only useful on the theoretical derivation. 3, BP algorithm stepsIn the back-propagation algorithm is applied to feed-forward multi-layer network, with the number of Sigmoid as excited face when the network can use the following steps recursively weights W ij strike. Note that for each floor there are n neurons, when, that is, i = 1,2, ..., n; j = 1,2, ..., n. For the first i-k layer neurons, there are n-weights W i1, W i2, ..., W in, another to take over - a W in +1for that threshold θ i; and the input sample X When taking x = (X 1, X 2, ..., X n, 1).Algorithm implementation steps are as follows:1. On the initial set weights W ij.On the weights W ij layers a smaller non-zero set of random numbers, but W i, n +1 =- θ.2. Enter a sample X = (x l, x 2, ..., x n, 1), and the corresponding desired output Y = (Y 1, Y 2, ..., Y n).3. Calculate the output levelsI-level for the first k output neurons X i k, are:X i k = f (U i k)4. Demand levels of learning error d i kFor the output layer has k = m, thered i m = X i m (1-X i m) (X i m-Y i)For the other layers, there5. Correction weights Wij and threshold θ Using equation (1-53) when:Using equation (1-54) when:Of which:6. When the weights obtained after the various levels, can determine whether a given quality indicators to meet the requirements. If you meet the requirements, then the algorithm end; If you do not meet the requirements, then return to (3) implementation.This learning process, for any given sample X p = (X p1, X p2, ... X pn, 1) and the desired output Y p = (Y p1, Y p2, ..., Y pn) have implemented until All input and output to meet the requirement.。
人工神经网络知识概述
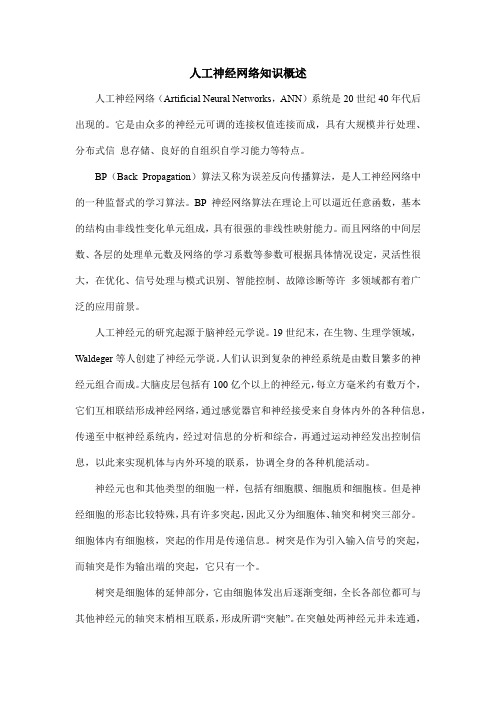
人工神经网络知识概述人工神经网络(Artificial Neural Networks,ANN)系统是20世纪40年代后出现的。
它是由众多的神经元可调的连接权值连接而成,具有大规模并行处理、分布式信息存储、良好的自组织自学习能力等特点。
BP(Back Propagation)算法又称为误差反向传播算法,是人工神经网络中的一种监督式的学习算法。
BP 神经网络算法在理论上可以逼近任意函数,基本的结构由非线性变化单元组成,具有很强的非线性映射能力。
而且网络的中间层数、各层的处理单元数及网络的学习系数等参数可根据具体情况设定,灵活性很大,在优化、信号处理与模式识别、智能控制、故障诊断等许多领域都有着广泛的应用前景。
人工神经元的研究起源于脑神经元学说。
19世纪末,在生物、生理学领域,Waldeger等人创建了神经元学说。
人们认识到复杂的神经系统是由数目繁多的神经元组合而成。
大脑皮层包括有100亿个以上的神经元,每立方毫米约有数万个,它们互相联结形成神经网络,通过感觉器官和神经接受来自身体内外的各种信息,传递至中枢神经系统内,经过对信息的分析和综合,再通过运动神经发出控制信息,以此来实现机体与内外环境的联系,协调全身的各种机能活动。
神经元也和其他类型的细胞一样,包括有细胞膜、细胞质和细胞核。
但是神经细胞的形态比较特殊,具有许多突起,因此又分为细胞体、轴突和树突三部分。
细胞体内有细胞核,突起的作用是传递信息。
树突是作为引入输入信号的突起,而轴突是作为输出端的突起,它只有一个。
树突是细胞体的延伸部分,它由细胞体发出后逐渐变细,全长各部位都可与其他神经元的轴突末梢相互联系,形成所谓“突触”。
在突触处两神经元并未连通,它只是发生信息传递功能的结合部,联系界面之间间隙约为(15~50)×10米。
突触可分为兴奋性与抑制性两种类型,它相应于神经元之间耦合的极性。
每个神经元的突触数目正常,最高可达10个。
各神经元之间的连接强度和极性有所不同,并且都可调整、基于这一特性,人脑具有存储信息的功能。
1.神经网络
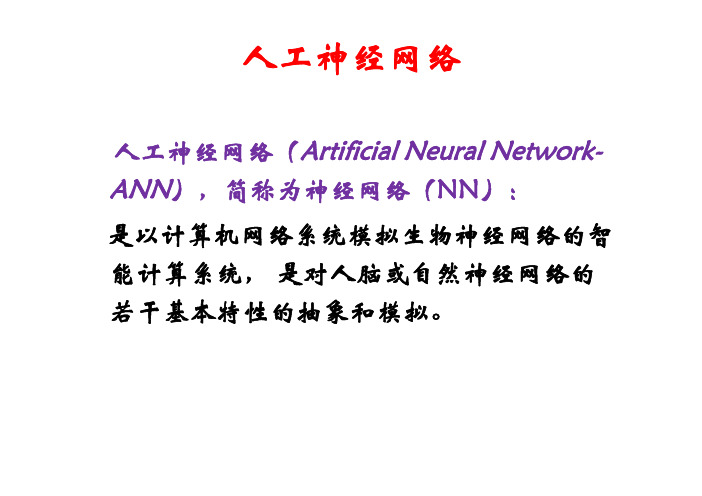
人工神经网络人工神经网络(Artificial Neural Network-ANN),简称为神经网络(NN):是以计算机网络系统模拟生物神经网络的智能计算系统,是对人脑或自然神经网络的若干基本特性的抽象和模拟。
生物神经系统1生物神经元●树突:接受刺激并将兴奋传入细胞体;每个神经元可以有多个;●轴突:把细胞体的输出信号导向其他神经元;每个神经元只有一个;●突触:是一个神经细胞的轴突和另一个神经细胞树突的结合点。
神经元的排列和突触的强度确立了神经网络的功能。
神经元主要由细胞体、树突、轴突和突触组成。
每个神经元约与104-105个神经元通过突触联接。
突触A B生物神经元1.1 生物神经网生物神经网络的六个基本特征:1)神经元及其联接;2)神经元之间的联接强度决定信号传递的强弱;3)神经元之间的联接强度是可以随训练改变的;4)信号可以是刺激作用的,也可以是抑制作用的;5)一个神经元接受的信号的累积效果决定该神经元的状态;6)每个神经元可以有一个“阈值”。
2019/6/107生物神经元人工神经元抽象1+n i i i v w x b==∑()y f v =1.2 人工神经网阈值M-P模型●w称为权重(weight),一个input(输入)都与一个权重w相联系;如果权重为正,就会有激发作用;权重为负,则会有抑制作用.●圆的‘核’是一个函数,确定各类输入的总效果,它把所有经过权重调整后的输入全部加起来,形成单个的激励值。
1n i i i v w x b==+∑()y f v =●阈值/偏置:决定神经元能否被激活,即是否产生输出。
●激活函数/传递函数/转移函数:神经元的信息处理特性,对所获得的输入的变换。
()y f v=1,0()0,0x f x x ≥⎧=⎨<⎩1n i i i v w x b ==+∑1()n i i i f y w x b ==+∑单层感知器☐感知器的模式识别超平面(分类边界)是:1Ni i i w x b =+=∑11220w x w x b ++=当N维数是2是,分类的超平面是一条直线☐感知器实质是一个分类器。
神经网络简介
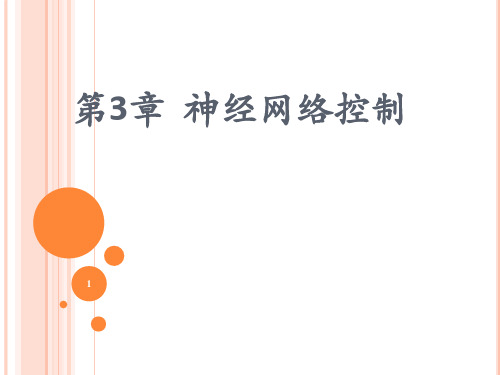
4
3.1.2 人工神经元模型
人工神经元是利用物理器件对生物神经元的一种模拟 与简化。它是神经网络的基本处理单元。如图所示为 一种简化的人工神经元结构。它是一个多输入、单输 出的非线性元件。
5
6
其输入、输出关系可描述为
n
Ii wijxj i j1
yi f(Ii)
其中,xj(j1,2,,是n)从其他神经元传来的输入信号; w表ij
号),计算
n
s
wi xpi
i1
计算感知器实际输出 ypf(s){ 11
调整连接权
选取另外一组样本,重复上述2)~4)的过程,直 到权值对一切样本均稳定不变为止,学习过程结束。
16
3.2.2 BP网络
误差反向传播神经网络,简称BP网络(Back Propagation),是一种单向传播的多层前向网络。在模 式识别、图像处理、系统辨识、函数拟合、优化计算、 最优预测和自适应控制等领域有着较为广泛的应用。如 图是BP网络的示意图。
下面要介绍的多层前馈网的神经元变换函数采用S型函 数,因此输出量是0到1之间的连续量,它可以实现从 输入到输出的任意的非线性映射。
17
18
误差反向传播的BP算法简称BP算法,其基本思 想是最小二乘算法。它采用梯度搜索技术,以 期使网络的实际输出值与期望输出值的误差均 方值为最小。
BP算法的学习过程由正向传播和反向传播组成。 在正向传播过程中,输入信息从输入层经隐含 层逐层处理,并传向输出层,每层神经元(节 点)的状态只影响下一层神经元的状态。如果 在输出层不能得到期望的输出,则转人反向传 播,将误差信号沿原来的连接通路返回,通过 修改各层神经元的权值,使误差信号最小。
26
神经网络训练的具体步骤如下
神经网络的基本知识点总结
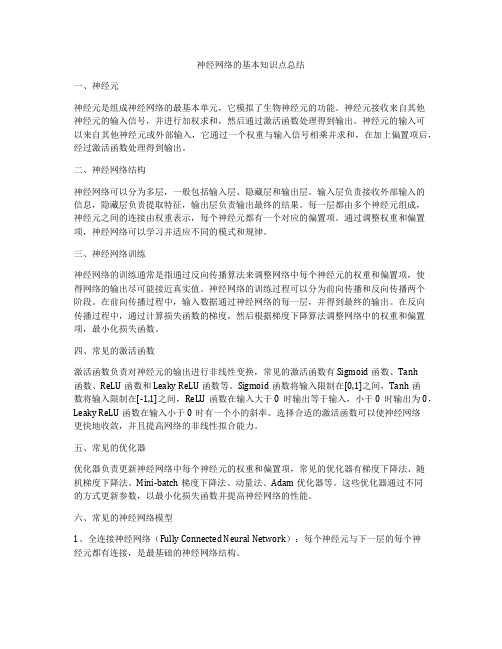
神经网络的基本知识点总结一、神经元神经元是组成神经网络的最基本单元,它模拟了生物神经元的功能。
神经元接收来自其他神经元的输入信号,并进行加权求和,然后通过激活函数处理得到输出。
神经元的输入可以来自其他神经元或外部输入,它通过一个权重与输入信号相乘并求和,在加上偏置项后,经过激活函数处理得到输出。
二、神经网络结构神经网络可以分为多层,一般包括输入层、隐藏层和输出层。
输入层负责接收外部输入的信息,隐藏层负责提取特征,输出层负责输出最终的结果。
每一层都由多个神经元组成,神经元之间的连接由权重表示,每个神经元都有一个对应的偏置项。
通过调整权重和偏置项,神经网络可以学习并适应不同的模式和规律。
三、神经网络训练神经网络的训练通常是指通过反向传播算法来调整网络中每个神经元的权重和偏置项,使得网络的输出尽可能接近真实值。
神经网络的训练过程可以分为前向传播和反向传播两个阶段。
在前向传播过程中,输入数据通过神经网络的每一层,并得到最终的输出。
在反向传播过程中,通过计算损失函数的梯度,然后根据梯度下降算法调整网络中的权重和偏置项,最小化损失函数。
四、常见的激活函数激活函数负责对神经元的输出进行非线性变换,常见的激活函数有Sigmoid函数、Tanh函数、ReLU函数和Leaky ReLU函数等。
Sigmoid函数将输入限制在[0,1]之间,Tanh函数将输入限制在[-1,1]之间,ReLU函数在输入大于0时输出等于输入,小于0时输出为0,Leaky ReLU函数在输入小于0时有一个小的斜率。
选择合适的激活函数可以使神经网络更快地收敛,并且提高网络的非线性拟合能力。
五、常见的优化器优化器负责更新神经网络中每个神经元的权重和偏置项,常见的优化器有梯度下降法、随机梯度下降法、Mini-batch梯度下降法、动量法、Adam优化器等。
这些优化器通过不同的方式更新参数,以最小化损失函数并提高神经网络的性能。
六、常见的神经网络模型1、全连接神经网络(Fully Connected Neural Network):每个神经元与下一层的每个神经元都有连接,是最基础的神经网络结构。
神经网络在金融行业中的应用研究

神经网络在金融行业中的应用研究Abstract神经网络(Neural Network,简称NN)是一种模拟生物神经网络信息处理的数学模型,近年来在金融行业中得到了广泛的应用。
本文将介绍神经网络在金融领域上的应用现状,着重探讨了神经网络在金融市场预测和金融风险管理方面的应用。
通过对神经网络在这两个方面的应用案例、优点和缺点的分析可以看出,神经网络技术不断的改进,为金融行业带来了更多的好处和优势,同时它所存在的问题和要求的技术门槛也在不断提高。
因此,未来神经网络在金融行业的应用前景依然广阔,但同时也需要不断迭代和完善。
Introduction神经网络是一种计算机算法,其算法模拟人类大脑神经元之间的相互作用处理信息的过程,它采用分布式并行处理模型,具有神经元存储信息和学习自适应性的功能,并且能够快速地学习和处理海量数据。
这种算法最早由Arthur Samuel 创造,后来由 Rosenblatt 在1962年发明的感知机(Perceptron)发展而来。
神经网络在金融领域的应用可追溯到20世纪90年代初期,随着技术的发展和数据的积累,神经网络在金融领域的应用越来越广泛,包括市场预测、风险管理、信用评估和投资组合的优化等多个方面。
神经网络在金融预测中的应用神经网络在金融预测中被广泛应用,包括股票价格预测、外汇汇率预测、大宗商品价格预测等。
相较于传统的统计模型,神经网络能够处理非线性关系,具有更好的预测精度。
神经网络技术可以应用在基本面分析和技术分析上,通过学习历史数据,挖掘市场内部隐藏的规律和未来发展趋势。
此外,神经网络模型的预测结果具有稳定性和鲁棒性,能够处理金融市场波动带来的影响。
以股票价格预测为例,神经网络应用于股票预测的基本思路是将历史数据输入神经网络模型,通过学习寻找相应的规律,将历史价格和其他因素组成的向量同预测价格的向量相互映射。
该方法的优点是能够捕捉股票市场中的非线性因素的影响,解决传统方法所不能解决的问题,并在严重的市场震荡期间表现出更好的承受能力。
- 1、下载文档前请自行甄别文档内容的完整性,平台不提供额外的编辑、内容补充、找答案等附加服务。
- 2、"仅部分预览"的文档,不可在线预览部分如存在完整性等问题,可反馈申请退款(可完整预览的文档不适用该条件!)。
- 3、如文档侵犯您的权益,请联系客服反馈,我们会尽快为您处理(人工客服工作时间:9:00-18:30)。
Abstract:
Artificial Neural Network is a math model which is applied to process information of the structure which is similar to Brain synaptic connection in a distributed and parallel way. Artificial Neural Network is a computing model, and it contains of many neurons and the connection of the neurons. Every neuron represents a special output function which is called activation function. The connection of neurons represents a weighted value of the connection’s signal.
Neuron is a basic and essential part of Artificial Neural Network, and it includes the sum of weighted value, single-input single-output (SISO) system and nonlinear function mapping. The element of neuron can represent different thing, such as feature, alphabet, conception and some meaningful abstract pattern. In the network, the style of neuron’s element divided into three categories: input element, output element and hidden element. The input element accepts the signal and data of outer world; the output element processes result output for system; the hidden element cannot find by outer world, it between input element and output element. The weighted value represents the strength of connection between neurons.
Artificial Neural Network adopted the mechanisms that completely different from traditional artificial intelligence and information processing technology. It conquers the flaw of traditional artificial intelligence in Intuitive handling and unstructured information processing aspect. It is adaptive, self-organized and learning timely, and widely used in schematic identification signal processing.。