埃博拉病毒的根除数学建模论文
美赛 数学建模 埃博拉
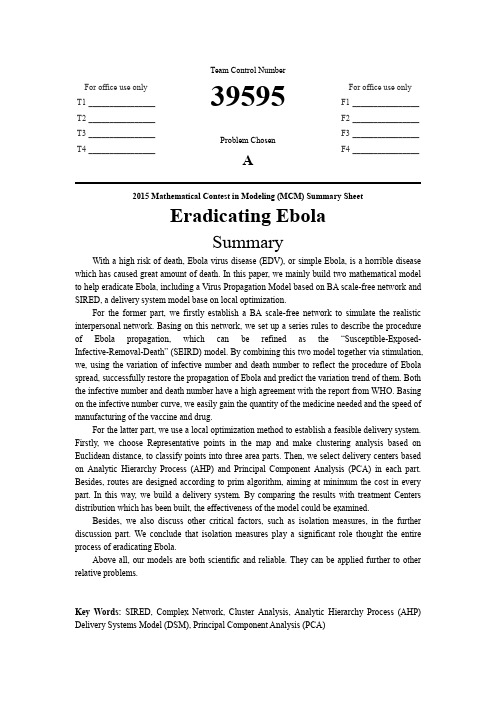
For office use only T1________________ T2________________ T3________________ T4________________Team Control Number39595Problem ChosenAFor office use onlyF1________________F2________________F3________________F4________________2015 Mathematical Contest in Modeling (MCM) Summary SheetEradicating EbolaSummaryWith a high risk of death, Ebola virus disease (EDV), or simple Ebola, is a horrible disease which has caused great amount of death. In this paper, we mainly build two mathematical model to help eradicate Ebola, including a Virus Propagation Model based on BA scale-free network and SIRED, a delivery system model base on local optimization.For the former part, we firstly establish a BA scale-free network to simulate the realistic interpersonal network. Basing on this network, we set up a series rules to describe the procedure of Ebola propagation, which can be refined as the “Susceptible-Exposed- Infective-Removal-Death” (SEIRD) model. By combining this two model toget her via stimulation, we, using the variation of infective number and death number to reflect the procedure of Ebola spread, successfully restore the propagation of Ebola and predict the variation trend of them. Both the infective number and death number have a high agreement with the report from WHO. Basing on the infective number curve, we easily gain the quantity of the medicine needed and the speed of manufacturing of the vaccine and drug.For the latter part, we use a local optimization method to establish a feasible delivery system. Firstly, we choose Representative points in the map and make clustering analysis based on Euclidean distance, to classify points into three area parts. Then, we select delivery centers based on Analytic Hierarchy Process (AHP) and Principal Component Analysis (PCA) in each part. Besides, routes are designed according to prim algorithm, aiming at minimum the cost in every part. In this way, we build a delivery system. By comparing the results with treatment Centers distribution which has been built, the effectiveness of the model could be examined.Besides, we also discuss other critical factors, such as isolation measures, in the further discussion part. We conclude that isolation measures play a significant role thought the entire process of eradicating Ebola.Above all, our models are both scientific and reliable. They can be applied further to other relative problems.Key Words:SIRED, Complex Network, Cluster Analysis, Analytic Hierarchy Process (AHP) Delivery Systems Model (DSM), Principal Component Analysis (PCA)Table of Content1.Introduction (1)1.1.Background (1)1.2.Restatement of the Problem (1)2.Assumptions and Notions (1)2.1.Assumptions and Justifications (1)2.2.Notions (2)3.The Virus Propagation Model Based on Complex Networks and SEIRD Model (3)3.1.Model Overview (3)plex Network Model (3)3.2.1.Small-World Network Model (3)3.2.2.BA Scale-Free Network Model (4)3.3.SIR-Based SEIRD Model (5)3.3.1.SIR Model (5)3.3.2.SEIRD Model (6)3.4.The Study of Infection Rate, Recovery Rate and Death Rate Based on the LeastSquare Method (6)3.4.1.The Relevant Calculation about Infection Rate (6)3.4.2.The Relevant Calculation about the Recovery Rate (7)3.4.3.The Relevant Calculation of Death Rate (7)3.5.The Simulation of the Transmission of Ebola Virus (8)3.5.1.The Simulation of Complex Network Model (9)3.5.2.The Simulation of Virus Transmission (10)3.6.Results and Result Analysis (11)3.6.1. A Complex Network Simulation Results Model (11)3.6.2.The Spread of the Virus the Simulation Results (12)4.Delivery Systems Model(DSM) Based on Local Optimization (13)4.1.Model Overview (13)4.2.Cluster Division Based on Cluster Analysis (14)4.3.Delivery Centers and Routes Planning Based on AHP and PCA (17)4.3.1The Three-hierarchy Structure (18)4.3.2Analytic Hierarchy Process and Principal Component Analysis for DSM .. 194.3.3Obtain the Centers (21)4.3.4Obtain the Routes (22)4.4.Results and Analysis (23)5.Other Critical Factors for Eradicating Ebola (24)5.1The Effect of the Time to Isolate Ebola on Fighting against Ebola (24)5.2The Effect of Timely Medical Treatment to Isolate Ebola on Fighting against Ebola (25)6.Results and results analysis (26)6.1.The virus propagation model based on complex networks (26)6.1.1.The contrast and analysis concerning the results of simulation and thereality (26)6.1.2.Forecast for the future (28)6.2.Delivery Systems Model Based on Local Optimization (28)7.Strengths and Weaknesses (29)7.1.Strengths (29)7.2.Weaknesses (29)8.Conclusion (30)9.Reference (30)10.Appendix (1)1.Introduction1.1.BackgroundWith a high risk of death, Ebola virus disease (EDV), or simple Ebola, is a disease of humans and other primates. Since its first outbreak in March 2014, over 8000 people have lost their lives. And till 3 February 2015, 22,495 suspected cases and 8,981 deaths had been reported. [1] However, this disease spreads only by direct contact with the bold or body fluids of a person who has developed symptoms of the disease. Following infection, patients will typically remain asymptomatic for a period of 2-21 days. During this time, tests for the virus will be negative, and patients are not infectious, posing no public health risk.[2] And recently, the world medicine association has announced that their new medication could stop Ebola and cure patients whose disease is not advanced. Thus, a feasible delivery system is in great demand and measures to eradicating Ebola should be taken immediately.1.2.Restatement of the ProblemWith the background mentioned above, we are required to build a model to help eradicate Ebola, which can be decomposed as:●Build a model, which can estimate the suspects number, exposed number,infect number, death number and recover numbers, to describe the spreadprocedure of the Ebola from its very beginning to the future.●Build an optimized model to help establish a possible and feasible deliverysystem including selecting delivery location and delivery system networkdesign.●Estimate of the quantity of the needed medicine and manufacturing speed ofvaccine or drug, based on the results of our models.●Discuss other critical factors which help eradicate Ebola.2.Assumptions and Notions2.1.Assumptions and JustificationsTo simplify the problem, we make the following basic assumptions, each of which is properly justified.●Assume that there is no people flow between countries after outbreak ofdisease in the country.After the outbreak, countries usually will ban thecontact between locals and foreigners to minimize the incoming of the virus.●Assuming that virus infection rate and fatality rate will not change bythe change of regions.Virus infection rate and fatality rate are largelydetermined by the nature of the virus itself. The different between differentregions just have a little effect and it will be ignored.●Assume that there are only rail, road and aircraft for transportation. Inthe West Africa, waterage is rare. Rail, and road are for nearby transportationwhile aircraft is for faraway.2.2. NotionsAll the variables used in this paper are listed in Table 2.1 and Table 2.2.Table 2.1 Symbols for Virus Propagation Model(VPM)SymbolDefinition Units βInfection Rate for the Susceptible in SIR Model or SEIRD Model unitless γRecovery Rate for the Infective in SIR Model unitless rRateRecovery Rate for the Infective in SEIRD Model unitless dRateDeath Rate for the Infective in the SIR Model or SEIRD Model unitless ∆N iNew Patients on a Daily Basis person N i−1The Total Number of Patients in the Previous Day person nThe Average Degree of Each Node in the Network unitless tTime S ∆D iThe New Death Toll on a Daily Basis person D i−1The Total Number of Patients in the Previous Day person moThe Initial Number of Nodes in the BA Scale-Free Network node mThe Number of Added Sides from One New Node in the BA Scale-Free Network side ∏iThe Probability for the Connection between New Nodes and the Existing Node I in the BA Scale-Free Network unitless NThe Total Number of Nodes in the BA Scale-Free Network node k iThe Degree of Node I in the BA Scale-Free Network unitless eThe Number of Sides in the BA Scale-Free Network side n SThe Number of the Susceptible in the SEIRD Model person n EThe Number of the Exposed in the SEIRD Model person n IThe Number of the Infective in the SEIRD Model person n RThe Number of the Removal in the SEIRD Model person n DThe Number of the Dead in the SEIRD Model person RThe Probability of Virus Propagation from the Recovered unitless RandomE The Number of the Exposed Who Have Reached the Exposed Time Limit Ranging from 2 to 21 Days at the Current Momentperson n E(一天)t The Number of the Exposed Who Have Reached the Final Day of the ExposedTime Limit yet not quarantine personTable 2.2 Symbols for Delivery Systems Model(DSM)SymbolDefinition Units Athe judging matrix unitless a ijThe element of judging matrix unitless λmaxthe greatest eigenvalue of matrix A unitless CI the indicator of consistency check unitlessCR the consistency ratio unitlessRI the random consistency index unitlessCW the weight vector for criteria level unitlessAW the weight vector for alternatives level unitlessY the evaluation grade unitlessV A set of points unitlessV i,V j the point of V unitlessE A set of edges unitlessDis ij The real distance of i and j unitless Arrive ij Judging for whether there is an side between i and j unitless3.The Virus Propagation Model Based on Complex Networks and SEIRD Model3.1.Model OverviewWe aim to build a Susceptible-Exposed-Infective-Removal-Death (SEIRD) virus propagation model which is based on Susceptible-Infective-Removal (SIR) model. The aimed model is featured by complex networks, which exhibit two statistical characteristics, including the Small-World Effect and the Scale-Free Effect. These characteristics could produce relatively real person-to-person and region-to-region networks. Through the statistics of the existing patients and deaths, we will try to find the relationship among the infection rate, the recovery rate and the death rate with the change of time. Then, with the help of SEIRD model, these statistics would be used to simulate the current situation concerning the number of the susceptible, the exposed, the infective, the recovered and the dead and conduct the prediction of the future.plex Network ModelResearches have shown that the person-to-person networks in real life exhibit the Small-World Effect and Scale-Free Effect. Here we will introduce the Small-World Network Model by Watts and Strogatz, and the Scale-Free Network Model by Barabdsi and Albert [3].3.2.1.Small-World Network ModelSince random network and regular network could neither properly present some important characteristics of real network, Watts and Strogatz proposed a new network model between the random network and regular network in 1998, namely WS Small-World Network Model, the construction algorithm of which is as follows.Start from a regular network: consider a regular network which contains N nodes, and these nodes form a ring. Each node is linked with its adjacent nodes, the number of which is K/Z on both left side and right side. Also, K is an even number.Randomized re-connection: the probability P will witness a random re connection with each side in the network. In other words, an endpoint of a certain side will remain unchanged and the other endpoint would be the node in the random selection.There are two rules. The first is two different nodes will at most have one side. The second is every node cannot have a side which is connected with this node [4].Randomized re-connection in construction algorithm of the WS Small-World Model may damage connectivity of network, so Newman and Watts improved this model in 1999. The new one is called NW Small-World Model, the construction algorithm of which is as follows.Start from a regular network: consider a regular network which contains N nodes, and these nodes form a ring. Each node is linked with its adjacent nodes, the number of which is K/Z on both left side and right side. Also, K is an even number.Randomized addition of sides: the probability P will witness the random selection of two nodes and the subsequent addition of a side between these two nodes. There are two rules. The first is two different nodes will at most have one side. The second is every node cannot have a side which is connected with this node[4].The network constructed by the two models are shown in Fig 3.1.Fig 3. 1 WS Small-World network and NW Small-World network3.2.2.BA Scale-Free Network ModelIn October, 1999, Barabdsi and Albert published article in Science called "Emergence of Scaling in Random Networks" [5], which proposed an important discovery that the distribution function of connectivity for many complex networks exhibit a form of power laws. Since no obvious length characteristics of connectivity could be seen among nodes in these networks, so they are called scale-free networks.As for the cause of power laws distribution, Barabasi and Albert believe that many previous network models did not take into account two important characteristics of actual networks: the consistent expanding of network and the nature of new nodes’ prior connection in the network. These characteristics will not only make node degrees which are relatively larger increase much faster, but also produce more new nodes, thus node degrees will become even larger. Then we could see the Matthew Effect. [4]Based on the Scale-Free Network, Barabasi and Albert proposed a scale-free network model, called BA Model, the construction algorithm of which is as followsi.The expanding of network: start from a network which has Mo nodes, thenintroduce a new node after each time interval and connect this node with mnodes. The prerequisite is m≤m0.ii.Prior connection: the probability between a new node and an existing node iis ∏i, the node degree of i is k i, and the node degree of j is k j. These threefactors should satisfy the following equation.∏i=k i/∑k jj(3-1) After t steps,this algorithm could lead to a network featured by m0+t nodes and m×t sides.The network of BA Model is shown in Fig 3.2.Fig 3.2 BA Scale-Free Network3.3.SIR-Based SEIRD Model3.3.1.SIR ModelSIR is the most classic model in the epidemic models, in which S represents susceptible, I represents infective and R represents removal. Specifically, the susceptible are those who are not infected, yet vulnerable to be infected after contact with the confirmed patients. The infective are those who have got the disease and could pass it to the susceptible. As for the removal, it refers to those who are quarantined or immune to a certain disease after they have recovered.In the disease propagation, SIR Model is built with the infection rate as β, the recovery rate as γ, which is shown in Fig 3.3:Fig 3.3 SIR propagation modelThis model is suitable epidemics which have the following features: no latency, only propagated by the patients, difficult to cause death, patients are immune to this disease after recovery once and for all. As for the Ebola virus, this model is insufficient to present the propagation process. Therefore, we propose the SEIRD model based on the SIR model and overcome the defects of the SIR model, thus making the SEIRD model more suitable for the research of Ebola virus.3.3.2.SEIRD ModelThe characteristics of Ebola virus are shown in Table 3.1:Table 3.1 The characteristics of Ebola virus characteristicsDetailsLatency Exposed period ranging from 2 to 21days with no infectivity during this stage[2]Retention After the recovery, there is still a certain chance of propagation[6]Immunity Recovery is accompanied with lifelong immunityTherefore, it is needed to add E (the exposed) and D (the dead) in the SIR model.E represents those who have been infected, have no symptoms, and not contagious. But within 2-21 days, the exposed will become contagious. D represents those who are dead and not contagious.From Table 3.1, we know that Ebola virus has the feature of retention, because even when they are in the state of removal, it is still possible these recovered will be infectious.In the process of disease propagation, SEIRD has witnessed the infection rate as β and the recovery rate as rRate and dRate,which is shown as follows.Fig 3.4SEIRD propagation model3.4.The Study of Infection Rate, Recovery Rate and Death RateBased on the Least Square MethodAs for the calculation of infection rate, recovery rate and death rate, we could make use of the least square method to match the daily confirmed patients and the dead toll, thus getting the function about the relationship with the passage of time.And we choose the relevant data from Guinea since it is in severely hit by the Ebola outbreak in West Africa. The variance regarding the total number of patients and the total death toll could be seen in Appendix 11.1.3.4.1.The Relevant Calculation about Infection RateThe infection rate refers to the probability that the susceptible are in contact (here it refers to the contact with body fluids) with un-isolated patients and infected with the virus. For each infective patient, the number of side is the node degree, namely the number whom he or she could infect. Therefore, the infection rate could be calculatedin this way: the number of new patients each day divides the possible number of whom each confirmed patient could infect. The number of new patients is ∆N i. The total number of patients in the previous day is N i−1. The average node degree in the network is n. The equation is as follows.β=∆N i/(N i−1×n)(3-2) The β could be calculated based on the total number o f patients in Guinea (see Appendix 11.1). Then with time data and the method of least square method, we could do data fitting and calculate the time-dependent equation. The fitting image is listed in Fig 3.5.Fig 3.5 The fitting result image of β with the passage of timeThe result of fitting curve is:β=0.0367×t−0.3189/n(3-3) And n is the average degree of person-to-person network in the process of simulation.3.4.2.The Relevant Calculation about the Recovery RateThe recovery rate refers to the probability of recovery for those who have been infected. Since at present, few instances of recovery from Ebola disease could be witness in the world (probability is almost close to zero), so the rRate here is set to be 0.001.3.4.3.The Relevant Calculation of Death RateThe death rate refers to the probability that patients become dead in process of treatment. And the death rate is calculated in the following way: the total number of new deaths every day divides the total number of patients in the previous day. The total number of new deaths in a new day is ∆D i. The total number of patients in the previous day is D i−1. The equation isdRate=∆D i/D i−1(3-4) The dRate could be calculated based on the total number of dead patients and the total number of patients in Guinea (see Appendix 11.1). Then with time data and the method of least square method, we could do data fitting and calculate the time-dependent equation. The fitting image is listed in Fig 3.6.Fig 3.6 The fitting result image of dRate with the passage of time The result of fitting curve is:dRate=(−6.119e−07)×t2 −0.0001562×t + 0.01558(3-5) 3.5.The Simulation of the Transmission of Ebola VirusThe simulation for Ebola will mainly be divided into two aspects, namely the simulation of complex network model and that of virus spread. The related flow chart will be shown in Fig 3.7.Fig 3.7 Flow chat of Stimulation of Ebola Virus Transmission3.5.1.The Simulation of Complex Network ModelFrom the previous introduction about complex network model, BA Scale-Free Network Model has displayed the Matthew Effect, which means the stronger would be much stronger and the weaker would be much weaker. In social networks, this effect is also widely seen. Take one person who just joins in a group for an example, he would normally contact with those who have the largest circle of friends. Therefore, those who get the least friends can hardly know more new friends. This finally leadsto a phenomenon that the person who is most acquainted will have more and more friends and vice versa.Based on this, the BA Scale-Free Network Model is apparently superior to that of Small-World Model. As a result of that,we would use the former to simulate the interpersonal network.According to the rules of BA network model, we should start from a network which has Mo nodes, then introduce a new node after each time interval and connect this node with m nodes. The prerequisite is m≤m0.During the connection process, the probability between a new node and an existing node i is ∏i, the node degree of i k i, and the node degree of j is k j. These three factors should satisfy the following equation.∏i=k i/∑k jj(3-6) Specifically, when the existing nodes have larger node degrees, it would be much more easier for the new ones to connect with the existing ones.After t steps, there would be a BA Scale-Free Network Model. The number of its nodes is expressed as N and the number of its sides is expressed as e:N=m0+t(3-7)e=m×t(3-8) The population of Sierra Leone now is 6.1 million and we would use this datum to produce its interpersonal network. For more details, please refer to Appendix 11.2.3.5.2.The Simulation of Virus TransmissionIn the transmission process, we assume the infection rate is β, the recovery rate is rRate, and the death rate is dRate. Based on the fitting results we previously get, we can simulate the virus transmission situation as time goes.Here comes the details.In the first place, there would be one patient who initiates the epidemic. Every single day, the virus would transmit to others among the main network and the probability of one-time propagation is β. Also, the patients would have rRate of recovery and dRate of death. Meanwhile, if the patient has been infected for 30 days, he or she would die anyway. The exposed would be in a latent period, during which they are not infectious and asymptomatic. In 2 to 21 days, these exposed ones would become infectious.n S、n E、n I、n R、n D represent the 5 different numbers of people in the SEIRD Model. t means time step (or a day),R represents the probability that those who have recovered patients would infect others. RandomE denotes the number of exposed patients who have reached the period of 2 to 21 days at the current moment.Here is the formula showing the changes in the numbers of those five types of people.n S t+1=n S t−n I t×n×β−n R t×n×β×R(3-9) n E t+1=n E t+n I t×n×β+n R t×n×β×R−RandomE(3-10) n I t+1=n I t+RandomE−n I t×rRate−n I t×dRate(3-11)n R t+1=n R t+n I t×rRate(3-12)n D t+1=n D t+n I t×dRate(3-13) After that, when the transmission has reached a certain scale (20 days after the transmission), the international organizations would adopt the measure of quarantine towards infective patients to avoid further contagion. As for the exposed patients, since they could not be quarantined immediately, so they have one day to infect others and in the next day, they would be quarantined at once.Finally, for those who have recovered, there is still a certain chance that they will propagate the disease within their networks.n Ed t on behalf of the moments lurk in reaching the last day with infectious but has not yet been isolated number. The process of five types of personnel number change:It represents the number of the exposed who have reached their last day of latency, begin to be contagious and have not yet been quarantined at the current moment. During this process, the formula exhibiting changes for these five categories of people could be listed as follows.n S t+1=n S t−n Ed t×n×β−n R t×n×β×R(3-14) n E t+1=n E t+n Ed t×n×β+n R t×n×β×R−RandomE(3-15)n I t+1=n I t+RandomE−n I t×rRate−n I t×dRate(3-16)n R t+1=n R t+n I t×rRate(3-17)n D t+1=n D t+n I t×dRate(3-18)3.6.Results and Result Analysis3.6.1. A Complex Network Simulation Results ModelPersonnel network is illustrated as Fig 3.8. Because of the population is too large so it is difficult to figure out. We use a red point to represent 10000 persons.Fig 3.8 Personnel relation network diagramThe probability distribution of nodes in a network of degrees is illustrated as Fig 3.9.The same node degrees set in 2-4, in it with degree of 4most.On behalf of each person every day in the network average fluid contact with 2-4 people.Fig 3.9 The probability of the node degree distribution map network3.6.2.The Spread of the Virus the Simulation ResultsThe number of every kind of the curveof the change over time in SEIRD model is illustrated in Fig 3.10. Due to the large population, the graph is local amplification. It is unable to find the number of susceptible people in the picture. For the rest of the curve, black represents the exposed, red represents the sufferer and pink for the removed.Fig 3.10 The number of SEIR model with the change of time4.Delivery Systems Model(DSM) Based on Local Optimization4.1.Model OverviewOptimized distributing is the most significant problem while building Delivery Systems, and it is a NP (nondeterministic polynomial) problem. In order to studied the problem, Li Zong-yong, Li Yue and Wang Zhi-xue organized an optimized distributing algorithm based on genetic algorithm in 2006[7]. In addition, Liu Hai-yan, Li Zong-ping, Ye Huai-zhen[8]discussed logistics distribution center allocation problem based on optimization method. With the help of current literature, we build a Delivery Systems Model (DSM) for drug and vaccine delivery, based on Local Optimization.In this topic, in order to establish the feasible delivery systems for WesternAfrica, we take Sierra Leone as an example. There is 14 Districts in Sierra Leone.In this model, we choose points based on Sierra Leone politics. Representative point in every District is selected. The points are located by longitude and latitude. We use Euclidean distance-based clustering analysis to process the data, so that the point set will be classified into three sub-set. Every sub-set is a part. Then, one point in every sub-set will be selected as a delivery center based on Analytic Hierarchy Process (AHP) and Principal Component Analysis (PCA). Besides, we will design the routes based on Prim algorithm, aiming at minimum the cost in every sub-set. In this way, we will build a delivery system.In addition, we will compare the results with Treatment Centers distribution which has been built, to analyze the model.4.2. Cluster Division Based on Cluster AnalysisThere are 14 districts in Sierra Leone. In every district, we choose the center position as a point. In this model, the first step is to cluster. Cluster Analysis is based on similarity. In this model, the similarity could be measured by geography distance. There is different method for cluster.Suppose there are n variable, and the objects are x and y1212(,,,),(,,,),n n x x x x y y y y ==By Euclidean distance, the distance can be calculated by (4-1)(,)d x y =(4-1)By Cosine Similarity distance, the distance can be calculated by (4-2)2(,)ni ix yd x y =∑(4-2)In this model, we use Euclidean distance. We cluster 14 districts into three Parts. First of all, we need to know about the distances between districts. The 14 Districts of Sierra Leone are located by longitude and latitude. Establish a right angle coordinate system. Set the longitude as the abscissa and latitude as ordinate. Set the Greenwich meridian and the Equator as 0 degree. The West and the South are regarded as negative while the East and the North are regarded as positive. In addition, the data related to the latitude and longitude would be converted to standard decimal form. For example, point (1330',830'W N ︒︒) is located as (-13.5, 8.5).According to World Health Organization (WHO )[9]statistics data, the basic data of Sierra Leone’ Districts are obtained, which is shown in Table 4.1 .。
优化抑制埃博拉病毒传播模型

优化抑制埃博拉病毒传播模型高树润;屈恒恒;冯立超【摘要】埃博拉病毒将给世界带来毁灭性的灾难,虽然根除埃博拉病毒协会研制出了埃博拉病毒疫苗,但是该药物并不够先进.为此,建立优化图论PK-PD模型,将时间和药效联系在一起.对于配送节点的最优路径问题,建立自组织映射(SOM)模型,运用MATLAB求解得最优配送路线.根据传染病传播的特点,构建微分方程模型.研究表明,要防止疾病的传播,需减少R0.其中,R0为判断埃博拉病毒流行与否的阙值.为防止疾病传播,需使阙值小于1,方式有加强治疗从而缩短染病期、或减小埃博拉病毒的传染率、或减少易感人群、或提高传染人群治愈率.其中,最有效的方式是提高传染人群治愈率.在传染病传播的过程中,存在模糊、不确定因素影响病毒传播,可利用模糊控制的方法建立模糊控制模型,此模型不但对埃博拉病毒进行了有效的控制,而且对政府采取相关控制措施也起到一定指导作用.【期刊名称】《河北联合大学学报(自然科学版)》【年(卷),期】2016(038)003【总页数】8页(P58-65)【关键词】埃博拉病毒;抑制;优化模型;模糊控制模型【作者】高树润;屈恒恒;冯立超【作者单位】华北理工大学信息工程学院,河北唐山063009;华北理工大学数学建模创新实验室,河北唐山063009;华北理工大学数学建模创新实验室,河北唐山063009;华北理工大学电气工程学院,河北唐山063009;华北理工大学数学建模创新实验室,河北唐山063009;华北理工大学理学院,河北唐山063009【正文语种】中文【中图分类】R512.8埃博拉(Ebola virus)病毒,是一种能引起人类和灵长类动物产生埃博拉出血热的烈性传染病病毒,是目前已知的毒性最大的病毒性疾病,病死率高达,世界卫生组织将其列为对人类危害最严重的病毒之一。
埃博拉病毒被列为生物安全第4级(Biosafety Level)病毒,也同时被视为是生物恐怖主义的工具之一。
埃博拉病毒传播分析与数学建模word精品

****大学数学建模竞赛承诺书我们仔细阅读了****大学数学建模竞赛的参赛规则与竞赛纪律。
我们完全明白,在竞赛开始后参赛队员不能以任何方式(包括电话、电子邮件、网上咨询等)与队外的任何人研究、讨论与赛题有关的问题。
我们知道,抄袭别人的成果是违反竞赛纪律的,如果引用别人的成果或其他公开的资料(包括网上查到的资料),必须按照规定的参考文献的表述方式在正文引用处和参考文献中明确列出。
我们郑重承诺,严格遵守参赛规则和竞赛纪律,以保证竞赛的公正、公平性。
如有违反竞赛纪律的行为,我们将受到严肃处理。
我们授权****大学数学建模竞赛组委会,可将们的论文以任何形式进行公开展示(包括进行网上公示,在书籍、期刊和其他媒体进行正式或非正式发表等)<日期:2015年05月04日埃博拉病毒传播分析摘要本文的研究对象为1976年在苏丹南部和刚果的埃博拉河地区发现的埃博拉病毒。
埃博拉病毒是一种生物安全等级为4级,并且能引起人类和灵长类动物产生埃博拉出血热的烈性传染病病毒,其主要是通过病人的血液、唾液、汗水和分泌物等途径传播。
其病毒的潜伏期通常只有5天至10天,感染后2〜5天出现高热,6〜9天死亡。
面对其强大的传染力和对人类健康的巨大威胁,本文通过数学建模的方法了解埃博拉病毒的传播规律,并分析隔离措施的严格执行和药物治疗效果的提高等措施对控制疫情的作用。
本文中,首先我们根据已给的信息及相关假设数据,通过对已知条件和所给表格书记的分析,我们大致明白了猩猩从潜伏到发病再到死亡或自愈的过程,因此我们采用了excel拟合曲线,分析其发病、潜伏、自愈、死亡和隔离的相应的变化曲线,估计参数,再根据其建立数学模型,并用MATLA求解方程组,调试参数,从而得到我们需要的结果。
其次通过对已经得到的数据和曲线图的分析,可以得出人类通过严格的药物控制过后,对其发病和潜伏的影响,从而能够达到对疫情的控制的作用,并且对埃博拉病毒未来发展趋势有了更深刻的了解,以为更好的控制埃博拉病毒做出贡献。
A题 埃博拉病毒的根除最终

A题埃博拉病毒的根除摘要为了根除埃博拉病毒,根据埃博拉病毒的传播率、感染者人数的预测、药物的合理分配和隔离人数的比重,本文运用随机微分方程、产销平衡和最优控制三种算法分别建立了SIR模型、线性规划模型和最优隔离控制模型。
这三个模型分别解决了埃博拉病毒的传播规律及感染者人数的预测问题、药物的运输问题和以隔离控制为决定性作用因素的优化问题。
针对模型一:首先通过Excel线性拟合分析埃博拉病毒的传播,得到结论:在没有使用药物治疗时,受埃博拉病毒感染的确诊病例及死亡的人数急剧增长。
然后,建立了SIR模型来预测使用药物后的变化趋势,利用Matlab画出()()I t S t的比例曲线,发现病人比例减少。
,针对模型二:假设几内亚、利比里亚、塞拉利昂为需求地,美国、中国、日本、俄罗斯、法国以及瑞士为药物生产地。
利用产销平衡原理,建立了时间优化模型,通过Lingo求得运输到各地用时最短的调运方案为:瑞士运往几内亚耗时4.7小时,美国、日本、俄国运往利比里亚共耗时15.8小时,中国、俄国、法国、瑞士运往塞拉利昂共耗时13小时。
针对模型三:本模型基于SIR模型,利用极值原理给出了最优控制的设计方案,把易感染者、染病者、治愈者、隔离者以及总人口数作为初始值代入目标函数,存在一个最优控制因素,再将其对应的状态解代入协态方程,得到最优控制因素,即隔离的确切最优解。
关键字: 埃博拉病毒;SIR;线性规划;产销平衡;Matlab;Lingo一、 问题重述根除埃博拉病毒世界医学协会已经宣布他们的新药物能阻止埃博拉病毒并可治愈那些得非晚期疾病的患者。
建立一个可行的,明智的,有用的模型,模型不仅要考虑疾病的蔓延、药物的需求量、可能可行的输送系统、输送的位置、疫苗或药物的生产速度,也要考虑你的团队认为有必要作为模型的一部分来优化根除埃博拉病毒,或者至少解决目前压力的其他重要因素。
除了你比赛论文中的建模方法,你还要为世界医学协会在他们的公告中准备使用一封1-2页的非技术性的信。
埃博拉病毒传播分析与数学建模

****大学数学建模竞赛承诺书我们仔细阅读了****大学数学建模竞赛的参赛规则与竞赛纪律。
我们完全明白,在竞赛开始后参赛队员不能以任何方式(包括电话、电子邮件、网上咨询等)与队外的任何人研究、讨论与赛题有关的问题。
我们知道,抄袭别人的成果是违反竞赛纪律的, 如果引用别人的成果或其他公开的资料(包括网上查到的资料),必须按照规定的参考文献的表述方式在正文引用处和参考文献中明确列出。
我们郑重承诺,严格遵守参赛规则和竞赛纪律,以保证竞赛的公正、公平性。
如有违反竞赛纪律的行为,我们将受到严肃处理。
我们授权****大学数学建模竞赛组委会,可将们的论文以任何形式进行公开展示(包括进行网上公示,在书籍、期刊和其他媒体进行正式或非正式发表等)。
参赛的题目(从A/B中选择一项填写) B参赛队员姓名学号院系电话日期:2015年05 月04日埃博拉病毒传播分析摘要本文的研究对象为1976年在苏丹南部和刚果的埃博拉河地区发现的埃博拉病毒。
埃博拉病毒是一种生物安全等级为4级,并且能引起人类和灵长类动物产生埃博拉出血热的烈性传染病病毒,其主要是通过病人的血液、唾液、汗水和分泌物等途径传播。
其病毒的潜伏期通常只有5天至10天,感染后2~5天出现高热,6~9天死亡。
面对其强大的传染力和对人类健康的巨大威胁,本文通过数学建模的方法了解埃博拉病毒的传播规律,并分析隔离措施的严格执行和药物治疗效果的提高等措施对控制疫情的作用。
本文中,首先我们根据已给的信息及相关假设数据,通过对已知条件和所给表格书记的分析,我们大致明白了猩猩从潜伏到发病再到死亡或自愈的过程,因此我们采用了excel拟合曲线,分析其发病、潜伏、自愈、死亡和隔离的相应的变化曲线,估计参数,再根据其建立数学模型,并用MATLAB求解方程组,调试参数,从而得到我们需要的结果。
其次通过对已经得到的数据和曲线图的分析,可以得出人类通过严格的药物控制过后,对其发病和潜伏的影响,从而能够达到对疫情的控制的作用,并且对埃博拉病毒未来发展趋势有了更深刻的了解,以为更好的控制埃博拉病毒做出贡献。
sars数学建模获奖论文
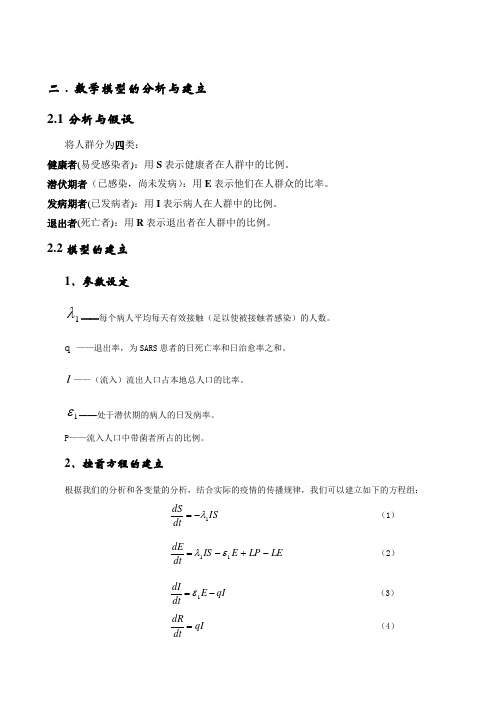
二.数学模型的分析与建立2.1分析与假设将人群分为四类:健康者(易受感染者):用S 表示健康者在人群中的比例。
潜伏期者(已感染,尚未发病):用E 表示他们在人群众的比率。
发病期者(已发病者):用I 表示病人在人群中的比例。
退出者(死亡者):用R 表示退出者在人群中的比例。
2.2模型的建立1.参数设定1λ——每个病人平均每天有效接触(足以使被接触者感染)的人数。
q ——退出率,为SARS 患者的日死亡率和日治愈率之和。
l ——(流入)流出人口占本地总人口的比率。
1ε——处于潜伏期的病人的日发病率。
P ——流入人口中带菌者所占的比例。
2.控前方程的建立根据我们的分析和各变量的分析,结合实际的疫情的传播规律,我们可以建立如下的方程组:IS dtdS 1λ-= (1) LE LP E IS dtdE -+-=11ελ (2) qI E dtdI -=1ε (3) qI dtdR = (4)0000,,,E R I S (初值)3.参数的确定1)1λ ——根据医学资料和有关数据推导而得。
2) q ——由该城市的医疗水平和已知的统计数据分析,求其统计平均值。
3) l ——由城市的出入人口流动情况(主要由经济发达程度和交通状况决定)。
可查有关资料。
4) 1ε——根据医学研究和调查的有关结果和该城市的疫情发展状况可得。
5) P ——由流入该城市人群的地区分布情况和各其他地区的疫情决定。
II 控后模型的建立1.参数设定λ2——不可控人群(在后面的分析中可得到)在发病后到被隔离前平均每天接触的人的数目。
q ——退出率,为SARS 患者的日死亡率和日治愈率之和。
β——接触病源的人的发病率。
ε——每天由可控人群和不可控人群转化为病人的日转化率。
2.控后方程的建立根据上面我们的各种假设和各变量和参数的实际意义,我们可以建立如下控制后的疾病模型的方程组:(5)()qI G dtdI -+=ωεβ (6) qI dtdR = (7) S dt dS ωβλ2-=()G G G S dt dG βεωβωλ-+=2()βεωωωβωλω-+=G S dtd 2 (9) 00000,,,,ωE R I S (初值)在得到这个模型后,我们对模型和数据进行了进一步的分析,发现这个模型中存在以下的问题:(1) 该模型中,没有充分考虑疑似病例,即“疑似者”和“隔离者”的之间的关系不明确。
根除埃博拉病毒

根除埃博拉病毒发表时间:2019-08-14T11:00:44.707Z 来源:《科技新时代》2019年6期作者:石宝义[导读] 根据每月新发病人数的增长率来决定每月派送药物的数量,可知生产药物的速率。
华北理工大学矿业工程学院,057750摘要首先,建立SIR的控前模型,得到无药物治疗情况下Guinean、Liberia、Sierra Leone这三个国家发病人数的曲线图,可知发病人数成上升趋势。
因SIR模型未考虑潜伏期,故对原模型进行改进,建立SEIR的控前模型,得到了新模型下三个国家发病人数的曲线图,将两种模型得到的曲线图分别与实际数据进行对比,验证了SEIR模型较SIR模型更为精准。
其次,基于SEIR的控后模型,引入干预措施的实施强度q,求出需要药物的总量,得到有药物治疗情况下整个西非地区的每月新发病人数和总发病人数的趋势图。
根据每月新发病人数的增长率来决定每月派送药物的数量,可知生产药物的速率。
最后,建立基于P-中值模型的静态确定型“中间定位”设施选址模型,结合感染区的重要程度和交通枢纽的分布,考虑到塞拉利昂地区病情最严重的地区集中分布在首都Freetown,且有国际机场,方便药品和疫苗传送,满足设置中转站的条件,故在Freetown设置中转站,且此中转站仅提供Freetown周边病情严重地区的需求。
关键词:SIR模型;SEIR模型;P-中值模型一问题背景世界医学协会已经宣布他们的新药物能阻止埃博拉病毒并且可以治愈一些处于非晚期疾病患者。
建立一个现实的,合理的并且有用的模型,该模型不仅考虑了疾病的蔓延,需要药物的量,可能可行的输送系统,输送的位置,疫苗或药物的生产速度,而且也要考虑其他重要的因素,诸如你的团队认为有必要作为模型的一部分来进行优化而使埃博拉病毒根除的一些因素,或者至少考虑当前的状态。
二、模型的构建与求解2.1 控前模型2.1.1 疫区的选取埃博拉病情的感染主要集中在西非地区的塞拉利昂、利比里亚、几内亚3个国家,美国、英国、西班牙和塞内加尔的感染数为1,死亡数为0,即被感染病例尚未死亡。
消除埃博拉

图 1 三个国家每天患病人数的变化趋势 表1
训练 地区 聚类分析 科纳 库 克里 鲁 萨 12 1 10 1 3 1 盖 凯 杜 3 3 7 马 桑 达 3 12 9 达 博 拉 3 9 9 吉西 杜古 3 12 12 丁吉 拉伊 3 5 8 特里 梅莱 3 6 10 博 法 3 12 12
3.2 模型假设:
s 1
2.2 结果分析
由表1可知,训练步长越大,类别越多.我们只需要根据两种类 别的参数将几内亚9个地区划分为3类.故只需采取较短步长即可.
3 模型三 3.1 投递地点
首先,根据西非的交通能力和以前国际援助西非国家的经验, 我们建立了由国际到西非三国的三级药品投递系统:国际---该国 首都----该国各疫区.依据哪里需要在哪投放药品的原则,确定了 各国的药品投放点,经过调查,可以知道从首都到各投放点的药物 运送在一天内都可以完成.
3.3 效益费用法
效益费用法与两个因素有关,即总收益与总费。 该方法有两种 分析评价指标:效益费用比和净效益。 效益费用比顾名思义是总效 益与总费用的比值;净效益即收益减去费用的值。 工程项目经济评 价中使用该方法时,首先要对总效益和总费用进行计算,对其的计 算可根据项目资金流的特点,按工程需要或采用现值或采用年值法 进行计算。 该方法主要应用于公路、 桥梁等公共工程项目的经济分 析方案。
1 模型一 1.1 介绍
了解传染病传播规律是理解传染病流行机理,预测流行趋势, 进行防控决策的基础.我们根据埃博拉出血热传播特点对所收集的 数据进行了拟集到的数据时间间隔不均匀,且所得图形时间轴跨度 较大.所以我们对三个国家的数据进行了多项式拟合,得到了他们 日患病率的曲线图.由图1可以看出,A国家和B国家曲线比较相似. 因为他们爆发时间基本相同且最终累计患病人数相似.
A题埃博拉病毒根除

A题埃博拉病毒的根除摘要为了根除埃博拉病毒,根据埃博拉病毒的传播率、感染者人数的预测、药物的合理分配和隔离人数的比重,本文运用随机微分方程、产销平衡和最优控制三种算法分别建立了SIR模型、线性规划模型和最优隔离控制模型。
这三个模型分别解决了埃博拉病毒的传播规律及感染者人数的预测问题、药物的运输问题和以隔离控制为决定性作用因素的优化问题。
针对模型一:首先通过Excel线性拟合分析埃博拉病毒的传播,得到结论:在没有使用药物治疗时,受埃博拉病毒感染的确诊病例及死亡的人数急剧增长。
然后,建立了SIR模型来预测使用药物后的变化趋势,利用Matlab画出()(),I t S t的比例曲线,发现病人比例减少。
针对模型二:假设几内亚、利比里亚、塞拉利昂为需求地,美国、中国、日本、俄罗斯、法国以及瑞士为药物生产地。
利用产销平衡原理,建立了时间优化模型,通过Lingo求得运输到各地用时最短的调运方案为:瑞士运往几内亚耗时4.7小时,美国、日本、俄国运往利比里亚共耗时15.8小时,中国、俄国、法国、瑞士运往塞拉利昂共耗时13小时。
针对模型三:本模型基于SIR模型,利用极值原理给出了最优控制的设计方案,把易感染者、染病者、治愈者、隔离者以及总人口数作为初始值代入目标函数,存在一个最优控制因素,再将其对应的状态解代入协态方程,得到最优控制因素,即隔离的确切最优解。
关键字: 埃博拉病毒;SIR;线性规划;产销平衡;Matlab;Lingo一、问题重述根除埃博拉病毒世界医学协会已经宣布他们的新药物能阻止埃博拉病毒并可治愈那些得非晚期疾病的患者。
建立一个可行的,明智的,有用的模型,模型不仅要考虑疾病的蔓延、药物的需求量、可能可行的输送系统、输送的位置、疫苗或药物的生产速度,也要考虑你的团队认为有必要作为模型的一部分来优化根除埃博拉病毒,或者至少解决目前压力的其他重要因素。
除了你比赛论文中的建模方法,你还要为世界医学协会在他们的公告中准备使用一封1-2页的非技术性的信。
根除埃博拉病毒
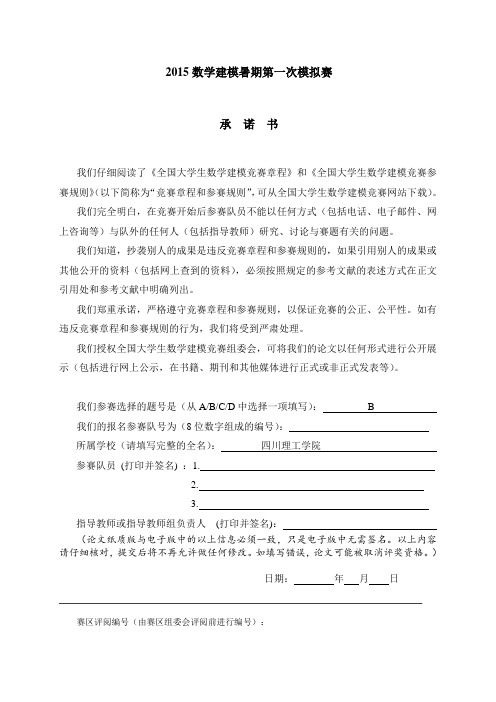
承诺书我们仔细阅读了《全国大学生数学建模竞赛章程》和《全国大学生数学建模竞赛参赛规则》(以下简称为“竞赛章程和参赛规则”,可从全国大学生数学建模竞赛网站下载)。
我们完全明白,在竞赛开始后参赛队员不能以任何方式(包括电话、电子邮件、网上咨询等)与队外的任何人(包括指导教师)研究、讨论与赛题有关的问题。
我们知道,抄袭别人的成果是违反竞赛章程和参赛规则的,如果引用别人的成果或其他公开的资料(包括网上查到的资料),必须按照规定的参考文献的表述方式在正文引用处和参考文献中明确列出。
我们郑重承诺,严格遵守竞赛章程和参赛规则,以保证竞赛的公正、公平性。
如有违反竞赛章程和参赛规则的行为,我们将受到严肃处理。
我们授权全国大学生数学建模竞赛组委会,可将我们的论文以任何形式进行公开展示(包括进行网上公示,在书籍、期刊和其他媒体进行正式或非正式发表等)。
我们参赛选择的题号是(从A/B/C/D中选择一项填写): B我们的报名参赛队号为(8位数字组成的编号):所属学校(请填写完整的全名):四川理工学院参赛队员(打印并签名) :1.2.3.指导教师或指导教师组负责人(打印并签名):(论文纸质版与电子版中的以上信息必须一致,只是电子版中无需签名。
以上内容请仔细核对,提交后将不再允许做任何修改。
如填写错误,论文可能被取消评奖资格。
)日期:年月日赛区评阅编号(由赛区组委会评阅前进行编号):编号专用页赛区评阅编号(由赛区组委会评阅前进行编号):全国统一编号(由赛区组委会送交全国前编号):全国评阅编号(由全国组委会评阅前进行编号):根除埃博拉病毒摘要本文通过分析埃博拉病毒在两个“虚拟种群”中传播的“数据”,建立了病毒传播模型,解决了疫情在猩猩和人类的虚拟种群中的发展情况问题。
首先,根据附录中的数据,用Matlab软件进行数值模拟,画出趋势图,做出数量变化图。
接着基于SIR模型,分析病毒在“虚拟猩猩种群”中的传播,描述人和猩猩疫情的发展,并预测接下来在猩猩中的疫情变化和在这两个群体中的发展情况,最后改变之前模型,做出相轨线,并分析各种疫情控制措施的严格执行和药物(包括防疫药物、检疫药物和治疗药物等)效果的提高等措施对控制疫情的作用。
剖析埃博拉病毒传播模型及规律预测

剖析埃博拉病毒传播模型及规律预测1 模拟真实环境埃博拉病毒的自然宿主虽尚未最后确定,但已有多方证据表明猴子及猩猩等野生非人灵长类动物有埃博拉感染现象。
该病毒的传播途径分为人畜传播、人人传播两种。
2014年,在几内亚、塞拉利昂和利比里亚等国,许多受埃博拉病毒影响的人口都以丛林肉为重要的蛋白质和营养物质来源,与丛林中动物接触频繁。
这为人畜之间的病毒传播创造了条件。
我们现假设两个感染埃博拉病毒的虚拟种群:即某地区内的20万居民和3000只猩猩。
人能以一定的概率接触到所有的猩猩,当接触到有传播能力的猩猩后有一定概率感染病毒,而人发病之后与猩猩的接触可以忽略。
人与猩猩的潜伏期都为2周。
并在出现疫情41周后模拟外界医疗力量的介入,使得人类与猩猩不再发生接触,且隔离治疗人群的治愈率提高到80%。
模拟数据详见附录。
2 建立数学模型2.1 模型假设(1)依据人或猩猩的健康状态,将人或猩猩划分为健康者、埃博拉感染者(也称患病者)、退出者(含自愈者、死亡者);(2)自然封闭条件下,猩猩无自然迁移,故无病源的流入、流出,种群数量不变。
人类数量庞大,在无大规模迁移的情况下,认为人类数目为一定值,保持不变;(3)健康者中不包含退出者;(4)人和猩猩自愈后二度感染的概率均为0,人被治愈后二度感染的几率为0;(5)不存在有效免疫药物可使人对埃博拉病毒产生免疫,同时猩猩对病毒也不免疫;(6)人的传染途径有人传染人、猩猩传染人两条。
两条途径的传染率并不相同,分别假设为传染率C1和传染率C2。
C1猩猩与猩猩之间传染途径只有猩猩传染猩猩一条,假设猩猩之间的传染率为C0;(7)患病人无法传染患病猩猩;(8)41周外界介入后,猩猩与人的传播途径切断,隔离患者的治愈率提高到80%,同时未被隔离的患者治愈率不变。
2.2 符号说明符号说明如表1所示:3 模型的建立与求解3.1 数据处理根据累计死亡个体数,求得每周死亡个体数。
同理,根据累计自愈个体数,求得每周自愈个体数。
两篇Cell论文一网打尽埃博拉病毒、寨卡病毒和登革热病毒的药物靶标
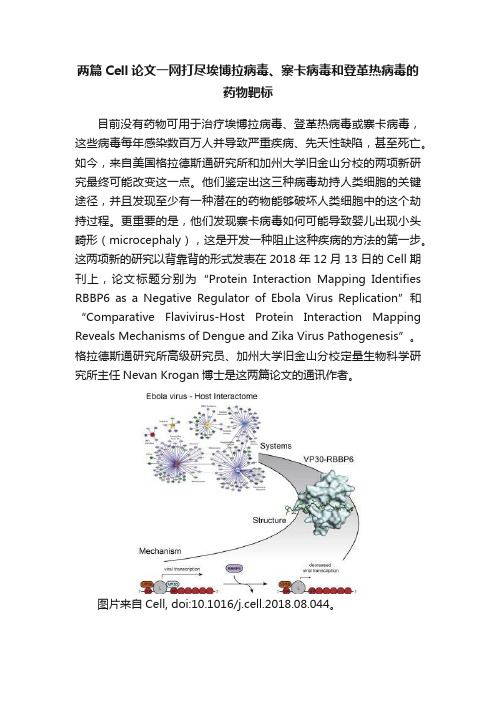
两篇Cell论文一网打尽埃博拉病毒、寨卡病毒和登革热病毒的药物靶标目前没有药物可用于治疗埃博拉病毒、登革热病毒或寨卡病毒,这些病毒每年感染数百万人并导致严重疾病、先天性缺陷,甚至死亡。
如今,来自美国格拉德斯通研究所和加州大学旧金山分校的两项新研究最终可能改变这一点。
他们鉴定出这三种病毒劫持人类细胞的关键途径,并且发现至少有一种潜在的药物能够破坏人类细胞中的这个劫持过程。
更重要的是,他们发现寨卡病毒如何可能导致婴儿出现小头畸形(microcephaly),这是开发一种阻止这种疾病的方法的第一步。
这两项新的研究以背靠背的形式发表在2018年12月13日的Cell期刊上,论文标题分别为“Protein Interaction Mapping Identifies RBBP6 as a Negative Regulator of Ebola Virus Replication”和“Comparative Flavivirus-Host Protein Interaction Mapping Reveals Mechanisms of Dengue and Zika Virus Pathogenesis”。
格拉德斯通研究所高级研究员、加州大学旧金山分校定量生物科学研究所主任Nevan Krogan博士是这两篇论文的通讯作者。
图片来自Cell, doi:10.1016/j.cell.2018.08.044。
在这两篇论文中,这些研究人员采用了一种称为蛋白-蛋白相互作用图谱(protein-protein interaction mapping)的技术来研究这三种病毒。
该方法使用实验室培养皿中的人细胞来构建病毒和人类蛋白之间的每个接触点的图谱。
他们如今正在利用这些综合图谱来靶向这些相互作用并试图清除病毒感染。
Krogan说,“我们在埃博拉病毒、登革热病毒和寨卡病毒中采用了我们的系统性蛋白-蛋白相互作用策略,以便更好地了解这三种非常有问题的病毒如何劫持和感染人类细胞。
埃博拉病毒传染机制与研药策略的数模分析
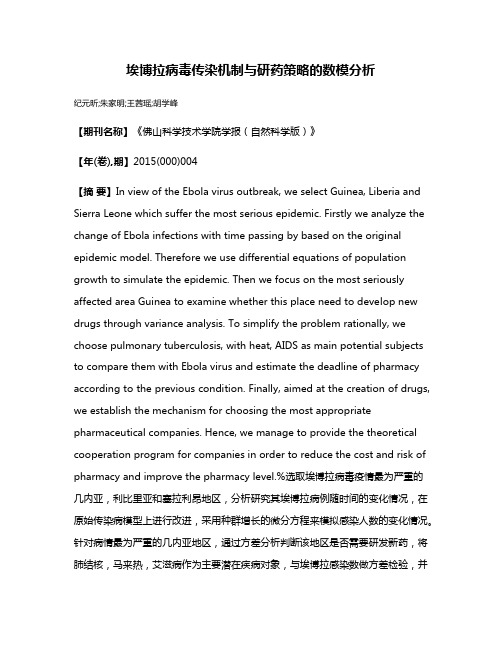
埃博拉病毒传染机制与研药策略的数模分析纪元昕;朱家明;王茜瑶;胡学峰【期刊名称】《佛山科学技术学院学报(自然科学版)》【年(卷),期】2015(000)004【摘要】In view of the Ebola virus outbreak, we select Guinea, Liberia and Sierra Leone which suffer the most serious epidemic. Firstly we analyze the change of Ebola infections with time passing by based on the original epidemic model. Therefore we use differential equations of population growth to simulate the epidemic. Then we focus on the most seriously affected area Guinea to examine whether this place need to develop new drugs through variance analysis. To simplify the problem rationally, we choose pulmonary tuberculosis, with heat, AIDS as main potential subjects to compare them with Ebola virus and estimate the deadline of pharmacy according to the previous condition. Finally, aimed at the creation of drugs, we establish the mechanism for choosing the most appropriate pharmaceutical companies. Hence, we manage to provide the theoretical cooperation program for companies in order to reduce the cost and risk of pharmacy and improve the pharmacy level.%选取埃博拉病毒疫情最为严重的几内亚,利比里亚和塞拉利昂地区,分析研究其埃博拉病例随时间的变化情况,在原始传染病模型上进行改进,采用种群增长的微分方程来模拟感染人数的变化情况。
埃博拉病毒感染数量的一个数学模型

埃博拉病毒感染数量的一个数学模型周后卿;徐幼专【期刊名称】《邵阳学院学报(自然科学版)》【年(卷),期】2014(000)004【摘要】Ebola virus disease (EVD),formerly known as Ebola haemorrhagic fever,is a severe,often fatal illness in humans. EVD outbreaks have a case fatality rate of up to 90%. EVD outbreaks occur primarily in remote villages in Central and West Africa,near tropical rainforests. The virus is transmitted to people from wild animals and spreads in the human population through human-to-human transmission. Severely ill patients require intensive supportive care. No licensed specific treatment or vaccine is available for use in people or animals. In the absence of effective treatment and a human vaccine,raising awareness of the risk factors for Ebola infection and the protective measures being taken are the only two ways to reduce human infection and death. This paper established a mathematical modelof Ebola virus,and made an empirical analysis of epidemic diseases. Furthermore,we made a prediction to the development of epidemic.%埃博拉病毒病(EVD)是严重的、往往致命的人类疾病,病死率高达90%。
治愈埃博拉病毒的优化方案

、
设 计 方 案
1 . 药 物 运 输 模 型 为 了节 约 资 源 和 成 本 , 故 选 择 已 有 的 三 个 医 疗 机 构 为 生产基地 , 以各 城 市 的 省会 为 药 品 中 转 中 心 . 建 立 目标 函数 使 得 每 个 救 助 站 在 所 在 区域 配 送 的 速 度 最 快 , 使 药 物的分 配更为合理. 假 设 每 个 救 治 中心 有 一 架 飞 机 , 第i 架 飞 机 运 送 的货 物 量为 A 设 第 n个 地 点‘ 的 难 度 为 , 使 其 困难 系 数 最 小 . 建立如下模型 :
染后 1 4天 不 治 身 亡 .
【 关键词 】 运输管理 ; 回归分析; 多 目标 规 划 ; 改 进 模 型
引 言
使 用 Ma t l a b软 件 模 拟 出 死 亡 人 数 的 函数 模 型 为 :
d : 一 0. 0 001 1 + 0. 0 2 4 0. 49 一1 2 +1 . 3 x 1 0
一
根据此模 型可以预测 出药 物每 天所 需要 的数 量 , 即 需
要 制 造 的疫 苗 或 药 物 的 速 度 .
所 需 药物 总 量 的 函 数 ( 为 天数 )为 :
Y Y = f一 0 .0 01 9 x +0. 33 x 一1 2 x + 1 . 8 X 1 0 一4. 5× 1 0。1 X m
因素 的 改进 模 型 . - T
2 . 药 物 生 产速 度模 型 假设 治 愈 病 例 不 可 二 次 感 染 , 每 个 埃 博 拉 救 治 中 心 的 生 产 速 率 固 定 即救 治 中心 每 天 的 生 产 药 量 设 为 Ms , 每 位 患
者 每 天 的 用 药 量 为 My , 患者 连续服用 Ⅳ 天 后 可 以痊 愈 , 经 过Ⅳ 后彻底 消除埃 博拉疾 病 , 查询 专业资 料可知 , 一 般 感
埃博拉病毒的根除数学建模论文
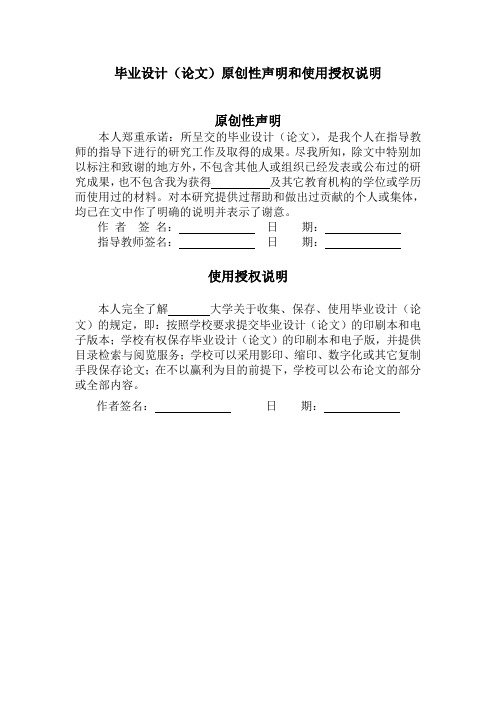
毕业设计(论文)原创性声明和使用授权说明原创性声明本人郑重承诺:所呈交的毕业设计(论文),是我个人在指导教师的指导下进行的研究工作及取得的成果。
尽我所知,除文中特别加以标注和致谢的地方外,不包含其他人或组织已经发表或公布过的研究成果,也不包含我为获得及其它教育机构的学位或学历而使用过的材料。
对本研究提供过帮助和做出过贡献的个人或集体,均已在文中作了明确的说明并表示了谢意。
作者签名:日期:指导教师签名:日期:使用授权说明本人完全了解大学关于收集、保存、使用毕业设计(论文)的规定,即:按照学校要求提交毕业设计(论文)的印刷本和电子版本;学校有权保存毕业设计(论文)的印刷本和电子版,并提供目录检索与阅览服务;学校可以采用影印、缩印、数字化或其它复制手段保存论文;在不以赢利为目的前提下,学校可以公布论文的部分或全部内容。
作者签名:日期:学位论文原创性声明本人郑重声明:所呈交的论文是本人在导师的指导下独立进行研究所取得的研究成果。
除了文中特别加以标注引用的内容外,本论文不包含任何其他个人或集体已经发表或撰写的成果作品。
对本文的研究做出重要贡献的个人和集体,均已在文中以明确方式标明。
本人完全意识到本声明的法律后果由本人承担。
作者签名:日期:年月日学位论文版权使用授权书本学位论文作者完全了解学校有关保留、使用学位论文的规定,同意学校保留并向国家有关部门或机构送交论文的复印件和电子版,允许论文被查阅和借阅。
本人授权大学可以将本学位论文的全部或部分内容编入有关数据库进行检索,可以采用影印、缩印或扫描等复制手段保存和汇编本学位论文。
涉密论文按学校规定处理。
作者签名:日期:年月日导师签名:日期:年月日注意事项1.设计(论文)的内容包括:1)封面(按教务处制定的标准封面格式制作)2)原创性声明3)中文摘要(300字左右)、关键词4)外文摘要、关键词5)目次页(附件不统一编入)6)论文主体部分:引言(或绪论)、正文、结论7)参考文献8)致谢9)附录(对论文支持必要时)2.论文字数要求:理工类设计(论文)正文字数不少于1万字(不包括图纸、程序清单等),文科类论文正文字数不少于1.2万字。
MCM2015

埃博拉病毒治疗的数学模型研究摘要在埃博拉病毒治疗的问题上,我们将从埃博拉病毒的传染速率,治疗疫苗的输送量以及治疗疫苗的输送方式这三个方面上考虑,我们建立三个初等模型来分别代表这三方面来解决在非洲地区上埃博拉病毒的治疗。
第一个模型是埃博拉病毒传播模型——在非洲埃博拉病毒感染地区不变的假设下,运用第二个模型是治疗疫苗的输送量模型——该模型是在模型(1)的基础上,根据非洲各个地区的传播速率,分别计算出病毒感染地区的治疗疫苗需求量以及实际治疗疫苗的输送量。
第二个模型是治疗疫苗的输送方式模型——该模型是在模型(2)的基础上,根据病毒感染地区的治疗疫苗的输送量以及疫苗输送地点的分布,采用关键词目录一、问题重述1.1 问题简介1.2 埃博拉病毒二、问题分析2.1 埃博拉病毒传播问题分析2.2 治疗疫苗的输送量问题分析2.3 治疗疫苗的输送方式问题分析三、模型假设四、模型的建立4.1埃博拉病毒传播模型4.1.1埃博拉病毒传播地区人口埃博拉病毒目前为止主要呈现地方性流行,局限在中非热带雨林和东南非洲热带大草原,但已从开始的苏丹、刚果民主共和国扩展到刚果共和国、中非共和国、利比亚、加蓬、尼日利亚、肯尼亚、科特迪瓦、喀麦隆、津巴布韦、乌干达、埃塞俄比亚以及南非。
非洲以外地区偶有病例报道,均属于输入性或实验室意外感染,未发现有埃博拉出血热流行。
埃博拉病毒仅在个别国家、地区间歇性流行,在时空上有一定的局限性。
模型研究主要集中与西非的Guinea、Sierra Leone和Liberia这三个国家的不同地区。
Guinea、Sierra Leone和Liberia不同地区的人口统计表如下。
表1. Guinea、Sierra Leone和Liberia三个国家的地区人口情况4.1.2埃博拉病毒传播机理分析埃博拉病毒不能通过飞沫传播,故其传染性没有麻疹或流感那么强。
埃博拉病毒感染者只在出现症状后才具有传染性。
与感染者分泌物的直接接触,如唾液,是埃博拉病毒传播的基本途径。
消灭埃博拉病毒美赛二等奖

消灭埃博拉摘要埃博拉出血热是由埃博拉病毒引起的一种急性出血性传染病。
因其极高的致死率而被世界卫生组织列为对人类危害最严重的烈性传染病之一。
由于埃博拉病毒在Guinea, Liberia, Sierra Leone 的感染情况最严重,本文就埃博拉病毒在这三个国家为例,探讨了埃博拉病毒的传播和药物运输系统以及药物生产的需求和速度。
本文引入了经典的Susceptibles Infectives Recovered (SIR )模型来描述埃博拉病毒的传播问题,预测埃博拉病毒随时间变化的传播规律;并对一系列收集数据进行了数值模拟,得到了SIR 模型中日接触率λ和日治愈率μ的时间t 的多项式函数;进一步,根据其拟合的λ和μ,得到感染病人数的时间t 的函数关系式,进行了统计检验,其准确度较高。
关于药品的运输系统问题,选取了America ,England ,Belgium ,Canada 四个国家为生产地,Conakry International Airport (1a ),Nzérékoré Airport (2a ),Lungi International Airport (1b ),Kenema Airport (2b ),Roberts International Airport (1c ), James Spriggs Payne Airport(2c )六个机场为分配地,采用了度量法对六个分配地进行中心仓库选取,将药品运输分为两地足够供应和供应不足两种情况分别进行讨论:制定了抗击埃博拉病毒的药品运输系统,为药品的分发以及药品生产地的选择提供两地足够供应的交通运输网络 两地供应不足的交通运输网络 并且优化运输系统,得到了最优药物运输路线(如下图),使制药产地可以更高效率运输优化交通运输网络根据World Health Organization (WHO)提供的药品需求量的测算公式,得出药品需求量与患病人数之间的函数关系式,从而算得药品生产速度与时间的关系式,给出最优生产速度,求得最短生产时间。
“根除埃博拉”问题研究
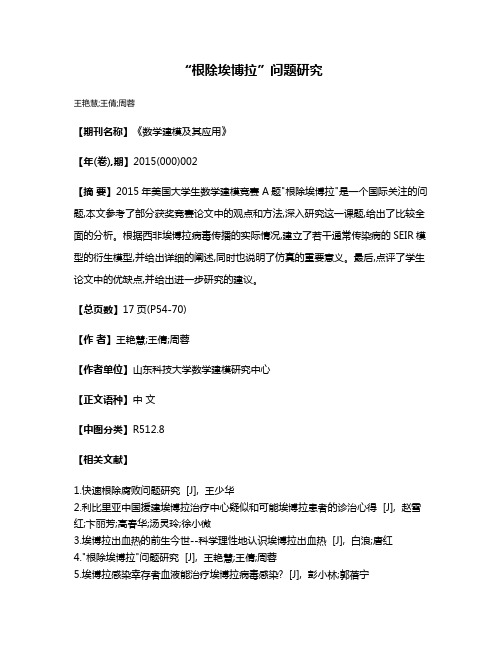
“根除埃博拉”问题研究
王艳慧;王倩;周蓉
【期刊名称】《数学建模及其应用》
【年(卷),期】2015(000)002
【摘要】2015年美国大学生数学建模竞赛A题"根除埃博拉"是一个国际关注的问题,本文参考了部分获奖竞赛论文中的观点和方法,深入研究这一课题,给出了比较全面的分析。
根据西非埃博拉病毒传播的实际情况,建立了若干通常传染病的SEIR模型的衍生模型,并给出详细的阐述,同时也说明了仿真的重要意义。
最后,点评了学生论文中的优缺点,并给出进一步研究的建议。
【总页数】17页(P54-70)
【作者】王艳慧;王倩;周蓉
【作者单位】山东科技大学数学建模研究中心
【正文语种】中文
【中图分类】R512.8
【相关文献】
1.快速根除腐败问题研究 [J], 王少华
2.利比里亚中国援建埃博拉治疗中心疑似和可能埃博拉患者的诊治心得 [J], 赵雪红;卞丽芳;高春华;汤灵玲;徐小微
3.埃博拉出血热的前生今世--科学理性地认识埃博拉出血热 [J], 白浪;唐红
4."根除埃博拉"问题研究 [J], 王艳慧;王倩;周蓉
5.埃博拉感染幸存者血液能治疗埃博拉病毒感染? [J], 彭小林;郭蓓宁
因版权原因,仅展示原文概要,查看原文内容请购买。
- 1、下载文档前请自行甄别文档内容的完整性,平台不提供额外的编辑、内容补充、找答案等附加服务。
- 2、"仅部分预览"的文档,不可在线预览部分如存在完整性等问题,可反馈申请退款(可完整预览的文档不适用该条件!)。
- 3、如文档侵犯您的权益,请联系客服反馈,我们会尽快为您处理(人工客服工作时间:9:00-18:30)。
毕业设计(论文)原创性声明和使用授权说明原创性声明本人郑重承诺:所呈交的毕业设计(论文),是我个人在指导教师的指导下进行的研究工作及取得的成果。
尽我所知,除文中特别加以标注和致谢的地方外,不包含其他人或组织已经发表或公布过的研究成果,也不包含我为获得及其它教育机构的学位或学历而使用过的材料。
对本研究提供过帮助和做出过贡献的个人或集体,均已在文中作了明确的说明并表示了谢意。
作者签名:日期:指导教师签名:日期:使用授权说明本人完全了解大学关于收集、保存、使用毕业设计(论文)的规定,即:按照学校要求提交毕业设计(论文)的印刷本和电子版本;学校有权保存毕业设计(论文)的印刷本和电子版,并提供目录检索与阅览服务;学校可以采用影印、缩印、数字化或其它复制手段保存论文;在不以赢利为目的前提下,学校可以公布论文的部分或全部内容。
作者签名:日期:学位论文原创性声明本人郑重声明:所呈交的论文是本人在导师的指导下独立进行研究所取得的研究成果。
除了文中特别加以标注引用的内容外,本论文不包含任何其他个人或集体已经发表或撰写的成果作品。
对本文的研究做出重要贡献的个人和集体,均已在文中以明确方式标明。
本人完全意识到本声明的法律后果由本人承担。
作者签名:日期:年月日学位论文版权使用授权书本学位论文作者完全了解学校有关保留、使用学位论文的规定,同意学校保留并向国家有关部门或机构送交论文的复印件和电子版,允许论文被查阅和借阅。
本人授权大学可以将本学位论文的全部或部分内容编入有关数据库进行检索,可以采用影印、缩印或扫描等复制手段保存和汇编本学位论文。
涉密论文按学校规定处理。
作者签名:日期:年月日导师签名:日期:年月日注意事项1.设计(论文)的内容包括:1)封面(按教务处制定的标准封面格式制作)2)原创性声明3)中文摘要(300字左右)、关键词4)外文摘要、关键词5)目次页(附件不统一编入)6)论文主体部分:引言(或绪论)、正文、结论7)参考文献8)致谢9)附录(对论文支持必要时)2.论文字数要求:理工类设计(论文)正文字数不少于1万字(不包括图纸、程序清单等),文科类论文正文字数不少于1.2万字。
3.附件包括:任务书、开题报告、外文译文、译文原文(复印件)。
4.文字、图表要求:1)文字通顺,语言流畅,书写字迹工整,打印字体及大小符合要求,无错别字,不准请他人代写2)工程设计类题目的图纸,要求部分用尺规绘制,部分用计算机绘制,所有图纸应符合国家技术标准规范。
图表整洁,布局合理,文字注释必须使用工程字书写,不准用徒手画3)毕业论文须用A4单面打印,论文50页以上的双面打印4)图表应绘制于无格子的页面上5)软件工程类课题应有程序清单,并提供电子文档5.装订顺序1)设计(论文)2)附件:按照任务书、开题报告、外文译文、译文原文(复印件)次序装订教研室(或答辩小组)及教学系意见A题埃博拉病毒的根除摘 要为了根除埃博拉病毒,根据埃博拉病毒的传播率、感染者人数的预测、药物的合理分配和隔离人数的比重,本文运用随机微分方程、产销平衡和最优控制三种算法分别建立了SIR 模型、线性规划模型和最优隔离控制模型。
这三个模型分别解决了埃博拉病毒的传播规律及感染者人数的预测问题、药物的运输问题和以隔离控制为决定性作用因素的优化问题。
针对模型一:首先通过Excel 线性拟合分析埃博拉病毒的传播,得到结论:在没有使用药物治疗时,受埃博拉病毒感染的确诊病例及死亡的人数急剧增长。
然后,建立了SIR 模型来预测使用药物后的变化趋势,利用Matlab 画出()(),I t S t 的比例曲线,发现病人比例减少。
针对模型二:假设几内亚、利比里亚、塞拉利昂为需求地,美国、中国、日本、俄罗斯、法国以及瑞士为药物生产地。
利用产销平衡原理,建立了时间优化模型,通过Lingo 求得运输到各地用时最短的调运方案为:瑞士运往几内亚耗时4.7小时,美国、日本、俄国运往利比里亚共耗时15.8小时,中国、俄国、法国、瑞士运往塞拉利昂共耗时13小时。
针对模型三:本模型基于SIR 模型,利用极值原理给出了最优控制的设计方案,把易感染者、染病者、治愈者、隔离者以及总人口数作为初始值代入目标函数,存在一个最优控制因素,再将其对应的状态解代入协态方程,得到最优控制因素,即隔离的确切最优解。
关键字: 埃博拉病毒;SIR ;线性规划;产销平衡;Matlab ;Lingo一、 问题重述根除埃博拉病毒世界医学协会已经宣布他们的新药物能阻止埃博拉病毒并可治愈那些得非晚期疾病的患者。
建立一个可行的,明智的,有用的模型,模型不仅要考虑疾病的蔓延、药物的需求量、可能可行的输送系统、输送的位置、疫苗或药物的生产速度,也要考虑你的团队认为有必要作为模型的一部分来优化根除埃博拉病毒,或者至少解决目前压力的其他重要因素。
除了你比赛论文中的建模方法,你还要为世界医学协会在他们的公告中准备使用一封1-2页的非技术性的信。
二、问题分析本文关于埃博拉病毒的传播、患病人数的预测、药物的需求量、可行的运输系统、疫苗的预防及药物的治疗、气候、车辆、地形等几个方面展开研究和讨论。
模型一主要解决疾病的传播和患病人口的预测问题。
考虑到埃博拉病毒的传播速度非常快,通过参考以往传染病的有关文章,本文建立了SIR 模型,得到了健康人和患者随时间变化的数量关系方程。
为了求解方程,根据收集来自WHO 的官方数据,得到2014年11月到2015年5月的确诊病例数和死亡人数,从而得到()(),I t S t 的两个图形,进而预测未来的患病人数。
在此基础上,再解决药物需求量的问题。
首先假设埃博拉病毒的传播遵循所建立的模型一,然后将收集到的使用药物治疗后患者人数变化的有关数据进行计算和分析,得到病人数量将会影响药物需求量的结论。
模型二主要解决药物的运输时间及成本问题。
由于几内亚、利比里亚和塞拉利昂这三个国家患病人数最多,所以选择这三个国家作为需求地。
现在具备疫苗或药物生产能力的国家:美国、中国、日本、俄国、法国和瑞士。
本文选择这六个国家作为产地。
本模型只考虑在生产地和需求地之间的药物运输。
首先保证各国所使用的运输机为同款运输机,在运输过程中,速度均为同等速度。
本文将产销平衡模型中的成本替换成运输所用时间,这样成本最低变成时间最短。
然后结合模型一中的患病人口预测结果,再加上每个病人对应药量的比例系数,则计算出任意时刻所需要的药物总量。
在满足各需求地需求量的前提下,本文再利用线性规划模型得到最优调运方案,即时间优化模型。
模型三在模型一、二、的基础上,分析其他可以消灭埃博拉病毒的决定性因素。
首先,本文使用最优隔离控制法,把易感染者、染病者、治愈者、隔离者以及总人口数作为初始值代入目标函数,则会存在一个最优控制因素,再将其对应的状态解代入协态方程,得到最优控制因素,即隔离的确切最优解。
然后,本文分别考虑了气候、运输工具、地形三个因素对埃博拉病毒传播的影响,并得出了相应的结果。
三、基本假设与符号说明2.1基本假设(1)埃博拉病毒能够被生物传播,并且当易感者接触患者时,他们被传染;(2)我们知道的埃博拉病毒有5种,假定每一种埃博拉病毒的传播能力是相同的;(3)每一个人被治愈的可能性是相同的,并且有相同的免疫力;(4)被治愈的人不会再次被传染,当患者被治愈后,他们将对于埃博拉病毒有免疫力;(5)埃博拉病毒的传播遵循所建立的模型一;(6)当实施药物治疗的时候,不计损失;(7)药物的运输对药物的需求有影响;(8)每位感染者的用药量均为一剂量,虽然目前已经研制出应对埃博拉病毒的疫苗或药物,但是不同感染程度的患者所需实际的药剂量数据不易获得;(9)疫苗与药物的生产地,开始培育的时间以及生产速度均相同,且培养药物和疫苗的周期均为t ,但两者的作用对象不同,疫苗作用于健康人群,而药物作用于感染者;(10)各地生产药物的速度相同,每批生产的药量满足前三个国家的需求量;(11)运输问题中各个产地的产量相同,用来运送药物的飞机类型相同,且保持相同速度行进;(12)收集到的数据都是真实可靠的;2.2 符号说明I:患者的数量比例;S :健康人的数量比例;R :康复者和死亡者的数量比例;α:死亡率;λ:感染率;γ:康复率(被隔离的病人治愈率);N :药物运达需求地点时的感染者人数;t ∆:培育一批药物或疫苗的时间;ij c :从i A 到j B 运输药物的成本;ij x :从i A 到j B 的运量;W :环境中的总人口数;J :被隔离患者人数;u :对染病者实施隔离控制的比例;μ:出生率=死亡率;δ:未被隔离染病者死亡率;s :易感者人数;i :染病者人数;r :病愈者人数;q :不易感病者输入比率;η:没有被隔离治疗者治愈率。
四、模型一的建立及求解4.1 模型一的建立埃博拉病毒的传播速度非常快,如果想要克服这个难题,并且使用有效的医疗方法根除埃博拉病毒,必须寻找一种科学且有效的方法来掌握埃博拉病毒的传播规律。
所以,我们建立了SIR 模型来解决这个问题。
根据符号定义,能够得到等式:()()()1I t S t R t ++= ⑴设每个发病者平均每天有效接触的易感者数为()S t λ,()NI t 个发病者平均每天能使()()S t NI t λ个易感者成为病毒潜伏者。
所以有:()()()dS t S t I t dtλ=- ⑵ 单位时间内康复者和死亡者的变化等于发病人群的减少,即()()()dR t I t dtαγ=+ ⑶ 发病人群的变化等于易感人群转入的数量,即()()()()()dI t S t I t I t dtλαγ=-+ ⑷ 记初始时刻的健康者和患者的比例分别为0S 、0R (不妨设00R =)。
根据SIR 模型的准则,得到下列方程组:()()()()00()()()()00dIS t I t I t dt dS S t I t dt I I S Sλαγλ⎧=-+⎪⎪⎪=-⎨⎪=⎪⎪=⎩ ⑸结合⑴式和⑸式,得到()()0R t S t S eλγ-= ⑹通过微积分,结合⑵式、⑹式和⑺式,求解方程组,得到()()000SI S S I S In S γλ=+-+⑺ ⑺式阐述了健康人和患者随时间变化的数量关系。
4.2 模型一的求解根据以上分析,得到了分析患者和健康人比例趋势的方法,接下来,求解模型如下:4.2.1没有药物治疗时确诊病例及死亡人数的变化趋势除了预防措施,没有药物治疗。
换句话说,健康人的数量比例是很小的,从网站http://.who.int/en/收集得到几内亚的有关数据,数据从2014年11月到2015年5月,每半个月统计一次,。
用Excel做线性拟合,得到下图:图1:确证病例的数量趋势图2:死亡人数趋势图1表明确诊病例数量在没有药物治疗的情况下急剧增长,因此,我们得出结论预防措施对患者的数量减少没有效果。