空间插值算法-反距离加权法
ArcGIS中几种空间插值方法
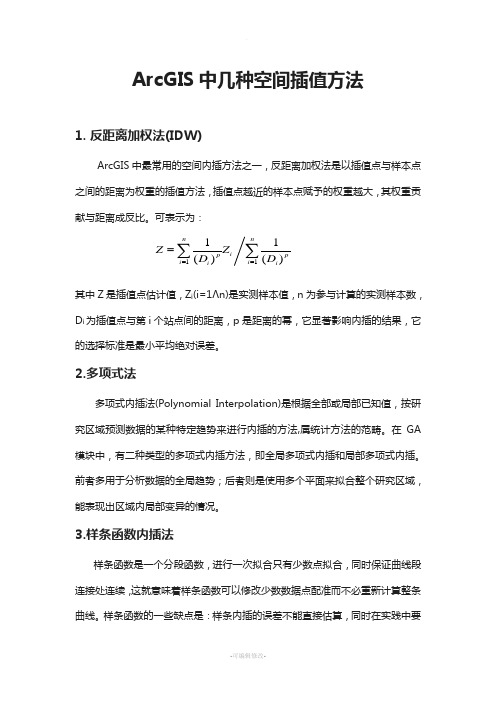
ArcGIS 中几种空间插值方法1. 反距离加权法(IDW)ArcGIS 中最常用的空间内插方法之一,反距离加权法是以插值点与样本点之间的距离为权重的插值方法,插值点越近的样本点赋予的权重越大,其权重贡献与距离成反比。
可表示为:1111()()n nip p i i i i Z Z D D ===∑∑ 其中Z 是插值点估计值,Z i (i=1Λn)是实测样本值,n 为参与计算的实测样本数,D i 为插值点与第i 个站点间的距离,p 是距离的幂,它显著影响内插的结果,它的选择标准是最小平均绝对误差。
2.多项式法多项式内插法(Polynomial Interpolation)是根据全部或局部已知值,按研究区域预测数据的某种特定趋势来进行内插的方法,属统计方法的范畴。
在GA 模块中,有二种类型的多项式内插方法,即全局多项式内插和局部多项式内插。
前者多用于分析数据的全局趋势;后者则是使用多个平面来拟合整个研究区域,能表现出区域内局部变异的情况。
3.样条函数内插法样条函数是一个分段函数,进行一次拟合只有少数点拟合,同时保证曲线段连接处连续,这就意味着样条函数可以修改少数数据点配准而不必重新计算整条曲线。
样条函数的一些缺点是:样条内插的误差不能直接估算,同时在实践中要解决的问题是样条块的定义以及如何在三维空间中将这些“块”拼成复杂曲面,又不引入原始曲面中所没有的异常现象等问题。
4.克里格插值法克里格法是GIS 软件地理统计插值的重要组成部分。
这种方法充分吸收了地理统计的思想,认为任何在空间连续性变化的属性是非常不规则的,不能用简单的平滑数学函数进行模拟,可以用随机表面给予较恰当的描述。
这种连续性变化的空间属性称为“区域性变量”,可以描述象气压、高程及其它连续性变化的描述指标变量。
地理统计方法为空间插值提供了一种优化策略,即在插值过程中根据某种优化准则函数动态的决定变量的数值。
Kriging 插值方法着重于权重系数的确定,从而使内插函数处于最佳状态,即对给定点上的变量值提供最好的线性无偏估计。
ArcGIS中几种空间插值方法
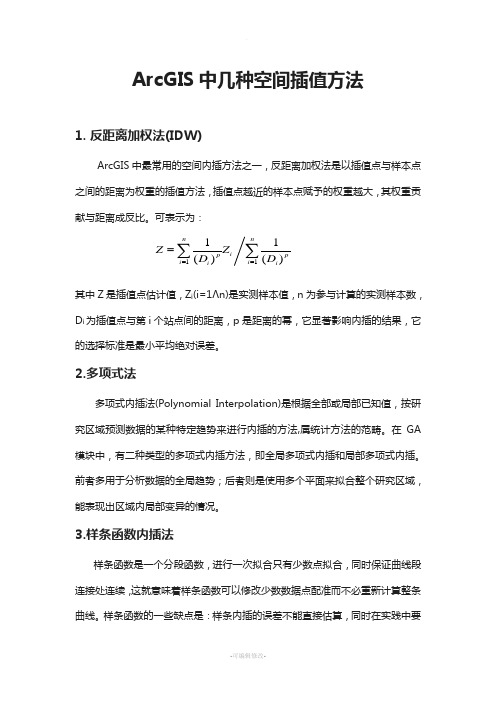
ArcGIS 中几种空间插值方法1. 反距离加权法(IDW)ArcGIS 中最常用的空间内插方法之一,反距离加权法是以插值点与样本点之间的距离为权重的插值方法,插值点越近的样本点赋予的权重越大,其权重贡献与距离成反比。
可表示为:1111()()n nip p i i i i Z Z D D ===∑∑ 其中Z 是插值点估计值,Z i (i=1Λn)是实测样本值,n 为参与计算的实测样本数,D i 为插值点与第i 个站点间的距离,p 是距离的幂,它显著影响内插的结果,它的选择标准是最小平均绝对误差。
2.多项式法多项式内插法(Polynomial Interpolation)是根据全部或局部已知值,按研究区域预测数据的某种特定趋势来进行内插的方法,属统计方法的范畴。
在GA 模块中,有二种类型的多项式内插方法,即全局多项式内插和局部多项式内插。
前者多用于分析数据的全局趋势;后者则是使用多个平面来拟合整个研究区域,能表现出区域内局部变异的情况。
3.样条函数内插法样条函数是一个分段函数,进行一次拟合只有少数点拟合,同时保证曲线段连接处连续,这就意味着样条函数可以修改少数数据点配准而不必重新计算整条曲线。
样条函数的一些缺点是:样条内插的误差不能直接估算,同时在实践中要解决的问题是样条块的定义以及如何在三维空间中将这些“块”拼成复杂曲面,又不引入原始曲面中所没有的异常现象等问题。
4.克里格插值法克里格法是GIS 软件地理统计插值的重要组成部分。
这种方法充分吸收了地理统计的思想,认为任何在空间连续性变化的属性是非常不规则的,不能用简单的平滑数学函数进行模拟,可以用随机表面给予较恰当的描述。
这种连续性变化的空间属性称为“区域性变量”,可以描述象气压、高程及其它连续性变化的描述指标变量。
地理统计方法为空间插值提供了一种优化策略,即在插值过程中根据某种优化准则函数动态的决定变量的数值。
Kriging 插值方法着重于权重系数的确定,从而使内插函数处于最佳状态,即对给定点上的变量值提供最好的线性无偏估计。
反距离加权插值法原理
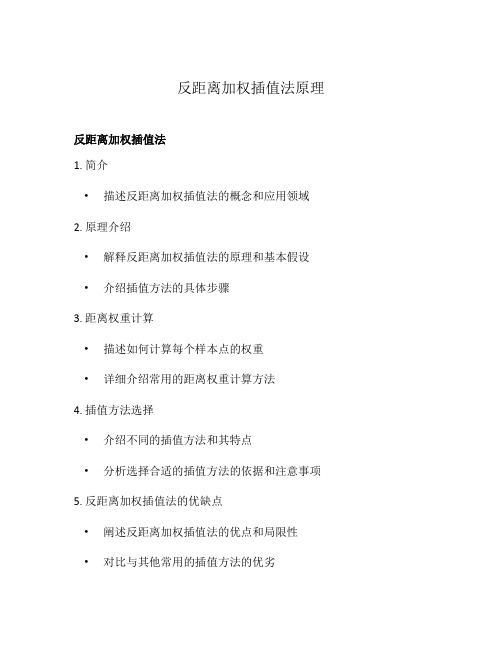
反距离加权插值法原理反距离加权插值法1. 简介•描述反距离加权插值法的概念和应用领域2. 原理介绍•解释反距离加权插值法的原理和基本假设•介绍插值方法的具体步骤3. 距离权重计算•描述如何计算每个样本点的权重•详细介绍常用的距离权重计算方法4. 插值方法选择•介绍不同的插值方法和其特点•分析选择合适的插值方法的依据和注意事项5. 反距离加权插值法的优缺点•阐述反距离加权插值法的优点和局限性•对比与其他常用的插值方法的优劣6. 实例应用•通过一个实际案例介绍反距离加权插值法的应用•详细描述案例中的数据处理流程和结果分析7. 灵敏度分析•描述反距离加权插值法的灵敏度分析方法•指出对结果影响最大的参数并进行分析8. 结论•归纳总结反距离加权插值法的特点和应用场景•提出进一步的研究方向和问题9. 参考文献•列举使用的参考文献及资料来源反距离加权插值法1. 简介反距离加权插值法是一种常用的数据插值方法,广泛应用于地理信息系统、医学影像处理、气象学等领域。
该方法利用距离来评估未知位置的值,根据距离远近进行加权计算,从而估计未知位置的值。
2. 原理介绍反距离加权插值法的基本原理是基于空间上的平面假设,即在原始样本点所在的平面上进行插值计算。
具体步骤如下:1.计算目标点与每个样本点的距离。
2.根据距离计算每个样本点的权重。
3.将权重乘以对应样本点的值,然后进行加权求和。
4.根据加权求和的结果,得到目标点的插值估计值。
3. 距离权重计算距离权重计算是反距离加权插值法的关键步骤,决定了每个样本点在插值计算中的影响力。
常用的距离权重计算方法有以下几种:•反距离权重:将距离的倒数作为权重,距离越近权重越大。
•指数距离权重:使用指数函数对距离进行权重计算,使得距离较远样本点的权重更小。
•克里金权重:根据克里金模型中的半变函数计算权重,对距离进行加权。
4. 插值方法选择在反距离加权插值法中,可以选择不同的插值方法进行计算。
测绘技术中的数据插值方法介绍

测绘技术中的数据插值方法介绍一、引言测绘技术是一门涉及地理空间信息的科学技术,其应用范围广泛,包括地质、地理、工程等领域。
而在测绘过程中,数据的采集和处理是至关重要的一环。
数据插值方法是其中的一个重要环节,它可以将已知点的数据推算到未知点,从而形成连续的地表分布情况。
本文就测绘技术中的数据插值方法进行介绍。
二、经验插值方法1. 反距离加权法反距离加权法是一种简单而常用的插值方法,其基本思想是假设未知点的属性值与其邻近已知点的属性值成正比,且与距离的倒数成正比。
该方法根据已知点到未知点的距离进行插值计算,再根据距离进行加权。
2. 克里金插值法克里金插值法是一种基于地理变量自相关性的插值方法。
该方法认为,地表属性之间的相互影响是通过距离和方向的变化来进行传递的。
克里金插值法可以根据已知点之间的空间关系进行插值计算,并且可以通过调整半方差函数来控制插值结果的平滑程度。
三、基于统计学的插值方法1. 多项式插值法多项式插值法是一种基于统计学原理的插值方法。
它利用已知点的属性值拟合一个多项式函数,并利用该函数来进行插值计算。
多项式插值法可以较好地拟合已知点的属性值,但在插值中容易产生过拟合或欠拟合的问题。
2. 最邻近插值法最邻近插值法是一种简单而直观的插值方法。
它基于已知点与未知点之间的距离,选取与未知点最近的已知点的属性值作为插值结果。
最邻近插值法的优点是计算简单、速度快,但在空间平滑性上存在一定的问题。
四、地统计学插值方法1. 变差函数插值法变差函数插值法是一种基于地表特征的统计学插值方法。
它通过建立变差函数来描述属性字段的空间变异性,并通过该函数来计算未知点的插值结果。
变差函数插值法可以考虑地表属性的空间关联性,从而更准确地估计未知点的属性值。
2. 地统计学Kriging插值法地统计学Kriging插值法是一种常用的高级统计插值方法。
它根据已知点之间的空间关系,建立半方差函数模型,并通过该模型来估计未知点的属性值。
空间插值方法汇总INVERSEDISTANCETOAPOWER(反距离加权插值法...
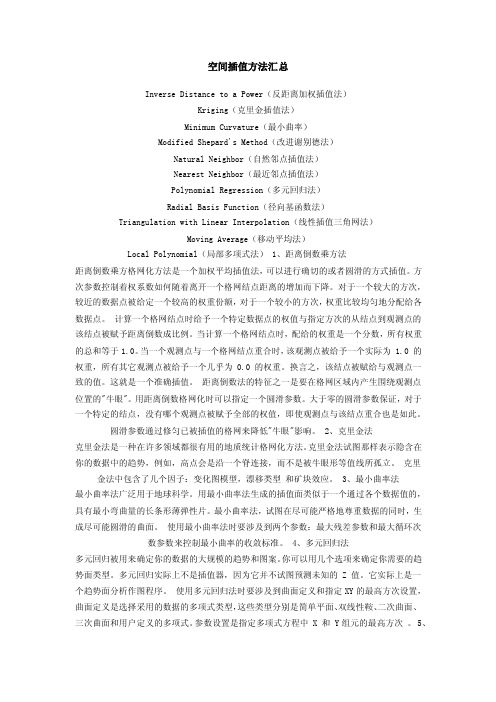
空间插值方法汇总Inverse Distance to a Power(反距离加权插值法)Kriging(克里金插值法)Minimum Curvature(最小曲率)Modified Shepard's Method(改进谢别德法)Natural Neighbor(自然邻点插值法)Nearest Neighbor(最近邻点插值法)Polynomial Regression(多元回归法)Radial Basis Function(径向基函数法)Triangulation with Linear Interpolation(线性插值三角网法)Moving Average(移动平均法)Local Polynomial(局部多项式法) 1、距离倒数乘方法距离倒数乘方格网化方法是一个加权平均插值法,可以进行确切的或者圆滑的方式插值。
方次参数控制着权系数如何随着离开一个格网结点距离的增加而下降。
对于一个较大的方次,较近的数据点被给定一个较高的权重份额,对于一个较小的方次,权重比较均匀地分配给各数据点。
计算一个格网结点时给予一个特定数据点的权值与指定方次的从结点到观测点的该结点被赋予距离倒数成比例。
当计算一个格网结点时,配给的权重是一个分数,所有权重的总和等于1.0。
当一个观测点与一个格网结点重合时,该观测点被给予一个实际为 1.0 的权重,所有其它观测点被给予一个几乎为 0.0 的权重。
换言之,该结点被赋给与观测点一致的值。
这就是一个准确插值。
距离倒数法的特征之一是要在格网区域内产生围绕观测点位置的"牛眼"。
用距离倒数格网化时可以指定一个圆滑参数。
大于零的圆滑参数保证,对于一个特定的结点,没有哪个观测点被赋予全部的权值,即使观测点与该结点重合也是如此。
圆滑参数通过修匀已被插值的格网来降低"牛眼"影响。
2、克里金法克里金法是一种在许多领域都很有用的地质统计格网化方法。
反距离加权插值法和克里金插值法
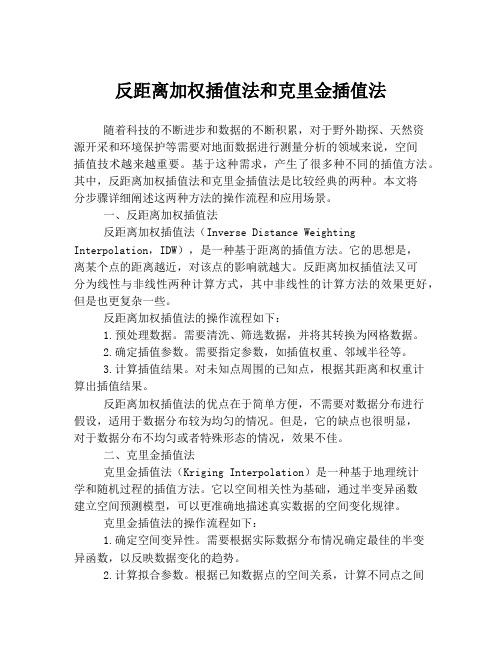
反距离加权插值法和克里金插值法随着科技的不断进步和数据的不断积累,对于野外勘探、天然资源开采和环境保护等需要对地面数据进行测量分析的领域来说,空间插值技术越来越重要。
基于这种需求,产生了很多种不同的插值方法。
其中,反距离加权插值法和克里金插值法是比较经典的两种。
本文将分步骤详细阐述这两种方法的操作流程和应用场景。
一、反距离加权插值法反距离加权插值法(Inverse Distance Weighting Interpolation,IDW),是一种基于距离的插值方法。
它的思想是,离某个点的距离越近,对该点的影响就越大。
反距离加权插值法又可分为线性与非线性两种计算方式,其中非线性的计算方法的效果更好,但是也更复杂一些。
反距离加权插值法的操作流程如下:1.预处理数据。
需要清洗、筛选数据,并将其转换为网格数据。
2.确定插值参数。
需要指定参数,如插值权重、邻域半径等。
3.计算插值结果。
对未知点周围的已知点,根据其距离和权重计算出插值结果。
反距离加权插值法的优点在于简单方便,不需要对数据分布进行假设,适用于数据分布较为均匀的情况。
但是,它的缺点也很明显,对于数据分布不均匀或者特殊形态的情况,效果不佳。
二、克里金插值法克里金插值法(Kriging Interpolation)是一种基于地理统计学和随机过程的插值方法。
它以空间相关性为基础,通过半变异函数建立空间预测模型,可以更准确地描述真实数据的空间变化规律。
克里金插值法的操作流程如下:1.确定空间变异性。
需要根据实际数据分布情况确定最佳的半变异函数,以反映数据变化的趋势。
2.计算拟合参数。
根据已知数据点的空间关系,计算不同点之间的半方差值,拟合统计模型。
3. 插值。
通过拟合的模型,对未知点进行插值计算,得到插值结果。
克里金插值法的优点在于能够精确地反映数据的空间变化状态,适用于各种数据分布情况。
但是,它的计算时间和计算量都比较大,需要大量的计算和处理,具有一定的复杂性。
反距离加权插值法原理(一)

反距离加权插值法原理(一)反距离加权插值法原理介绍反距离加权插值法是一种常用的空间插值方法,用于根据已知离散点数据估计未知位置的值。
该方法利用距离权重来确定每个已知点对未知点的贡献程度,进而进行插值计算。
原理反距离加权插值法的原理可以概括为以下几个步骤:1.计算未知点与所有已知点之间的距离。
2.根据距离计算每个已知点的权重。
一般情况下,离未知点越近的已知点权重越高,距离的增大会导致权重递减。
3.根据已知点的权重和值,通过加权平均计算未知点的估计值。
加权平均的计算公式为:插值公式,其中,w表示权重,z表示已知点的值。
算法步骤根据原理,可以得到反距离加权插值法的具体算法步骤:1.输入已知点坐标和值,以及待插值点的坐标。
2.根据欧氏距离计算待插值点与已知点之间的距离。
3.根据距离计算权重。
常用的权重计算方式为反距离的倒数,即:权重计算公式,其中,d表示待插值点与已知点的距离,p表示距离的指数(调节权重衰减的速度)。
4.根据权重和已知点的值,计算待插值点的估计值。
参数调节反距离加权插值法中,有两个重要的参数可以进行调节,即距离的指数p和权重的归一化。
调节这些参数可以对插值结果产生影响,不同的参数组合可能得到不同的结果。
•距离的指数p:调节权重的衰减速度。
当p较小时,距离较远的已知点对插值点的影响仍较大,而当p较大时,距离较远的已知点对插值点的影响衰减较快。
•权重的归一化:将所有权重除以它们的总和,以确保权重之和为1。
归一化可以消除由于距离和权重的变化而引起的估计值变化。
应用领域反距离加权插值法常被应用于以下领域:•地理信息系统(GIS)中的空间插值。
•气象领域中的气象站点值的插值。
•地质领域中的岩矿样点值的推断。
总结反距离加权插值法是一种常用的空间插值方法,适用于根据已知离散点数据估计未知位置的值。
它利用距离权重来确定每个已知点对未知点的贡献程度,并通过加权平均计算插值值。
参数调节可以影响插值结果,需要根据具体情况选择合适的参数。
ArcGIS中几种空间插值方法
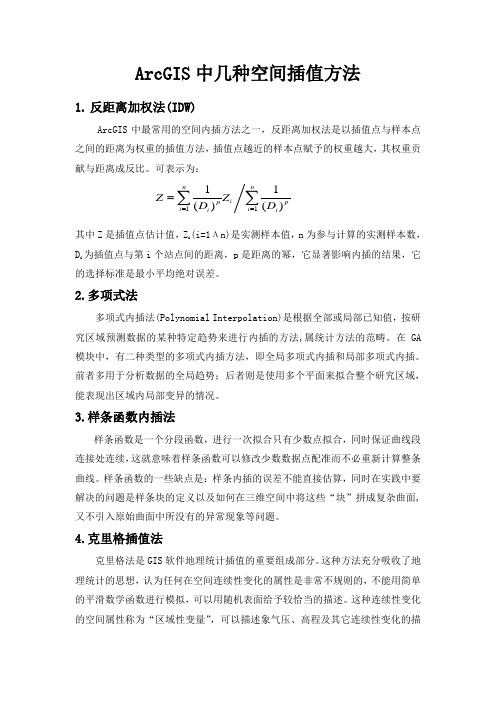
ArcGIS 中几种空间插值方法1. 反距离加权法(IDW)ArcGIS 中最常用的空间内插方法之一,反距离加权法是以插值点与样本点之间的距离为权重的插值方法,插值点越近的样本点赋予的权重越大,其权重贡献与距离成反比。
可表示为:1111()()n nip p i i i i Z Z D D ===∑∑其中Z 是插值点估计值,Z i (i=1Λn)是实测样本值,n 为参与计算的实测样本数,D i 为插值点与第i 个站点间的距离,p 是距离的幂,它显著影响内插的结果,它的选择标准是最小平均绝对误差。
2.多项式法多项式内插法(Polynomial Interpolation)是根据全部或局部已知值,按研究区域预测数据的某种特定趋势来进行内插的方法,属统计方法的范畴。
在GA 模块中,有二种类型的多项式内插方法,即全局多项式内插和局部多项式内插。
前者多用于分析数据的全局趋势;后者则是使用多个平面来拟合整个研究区域,能表现出区域内局部变异的情况。
3.样条函数内插法样条函数是一个分段函数,进行一次拟合只有少数点拟合,同时保证曲线段连接处连续,这就意味着样条函数可以修改少数数据点配准而不必重新计算整条曲线。
样条函数的一些缺点是:样条内插的误差不能直接估算,同时在实践中要解决的问题是样条块的定义以及如何在三维空间中将这些“块”拼成复杂曲面,又不引入原始曲面中所没有的异常现象等问题。
4.克里格插值法克里格法是GIS 软件地理统计插值的重要组成部分。
这种方法充分吸收了地理统计的思想,认为任何在空间连续性变化的属性是非常不规则的,不能用简单的平滑数学函数进行模拟,可以用随机表面给予较恰当的描述。
这种连续性变化的空间属性称为“区域性变量”,可以描述象气压、高程及其它连续性变化的描述指标变量。
地理统计方法为空间插值提供了一种优化策略,即在插值过程中根据某种优化准则函数动态的决定变量的数值。
Kriging 插值方法着重于权重系数的确定,从而使内插函数处于最佳状态,即对给定点上的变量值提供最好的线性无偏估计。
地理空间数据分析中的空间插值技术的使用教程
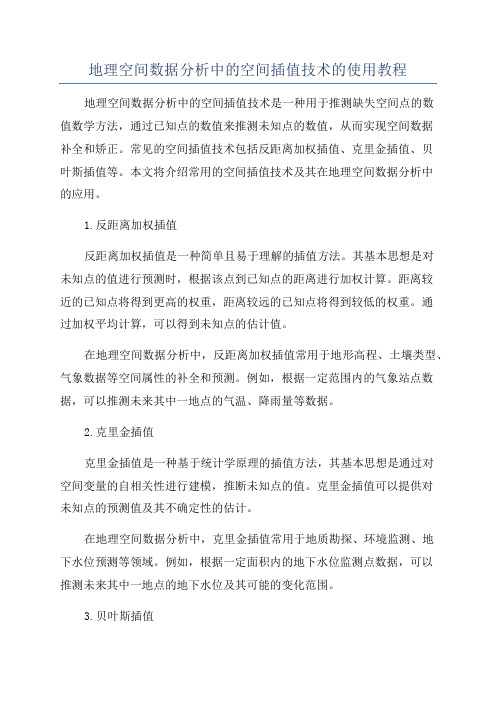
地理空间数据分析中的空间插值技术的使用教程地理空间数据分析中的空间插值技术是一种用于推测缺失空间点的数值数学方法,通过已知点的数值来推测未知点的数值,从而实现空间数据补全和矫正。
常见的空间插值技术包括反距离加权插值、克里金插值、贝叶斯插值等。
本文将介绍常用的空间插值技术及其在地理空间数据分析中的应用。
1.反距离加权插值反距离加权插值是一种简单且易于理解的插值方法。
其基本思想是对未知点的值进行预测时,根据该点到已知点的距离进行加权计算。
距离较近的已知点将得到更高的权重,距离较远的已知点将得到较低的权重。
通过加权平均计算,可以得到未知点的估计值。
在地理空间数据分析中,反距离加权插值常用于地形高程、土壤类型、气象数据等空间属性的补全和预测。
例如,根据一定范围内的气象站点数据,可以推测未来其中一地点的气温、降雨量等数据。
2.克里金插值克里金插值是一种基于统计学原理的插值方法,其基本思想是通过对空间变量的自相关性进行建模,推断未知点的值。
克里金插值可以提供对未知点的预测值及其不确定性的估计。
在地理空间数据分析中,克里金插值常用于地质勘探、环境监测、地下水位预测等领域。
例如,根据一定面积内的地下水位监测点数据,可以推测未来其中一地点的地下水位及其可能的变化范围。
3.贝叶斯插值贝叶斯插值是一种基于贝叶斯统计学原理的插值方法,它通过考虑先验信息和观测数据的后验概率分布,对未知点的值进行推测。
贝叶斯插值可以提供对不确定性的量化估计,并能够进行参数灵敏度分析。
在地理空间数据分析中,贝叶斯插值常用于地震研究、空气质量监测、森林覆盖度估计等领域。
例如,通过考虑不同监测站点的观测数据及其空间关联性,可以对未来其中一地区的地震概率进行推测。
总结来说,地理空间数据分析中的空间插值技术是一种重要的数据处理方法,可以用于补全数据、预测未知点的值、推断空间变量的分布规律等。
不同的插值方法适用于不同的数据类型和空间特征,熟练掌握这些技术可以帮助分析师更好地理解地理空间数据并进行科学的决策。
基于空间位置关系的空间插值反距离权重插值法

基于空间位置关系的空间插值反距离权重插值法
基于空间位置关系的空间插值是指根据样本点之间的空间位置关系来推断未知位置的数据值的一种方法。
其中反距离权重插值法是一种常用的方法。
该方法假设未知位置的数据值与周围的已知点的数据值是成反比例关系的,即离未知位置越近的已知点对未知位置的影响越大。
该方法的基本思路是通过加权平均的方式,计算未知位置与周围已知点的距离的倒数作为权重,由距离较近的已知点对未知位置进行插值。
具体方法如下:
1.确定数据点的分布情况和插值范围;
2.根据所选插值方法计算数据点的权重,反距离加权法计算公式如下:
权重=1/距离^p
其中,距离为未知位置与已知点之间的欧氏距离,p为可调参数,一般取值为2;
3.根据权重值对已知点的属性值进行加权平均,得到未知位置对应的属性值。
反距离权重插值法适用于样本点分布较为密集、局部关系比较显著的情况,但对于距离较远的点影响较小,容易受到噪声和异常值的影响。
因此,在使用该方法进行空间插值时需认真选择插值参数与样本点,避免过拟合和欠拟合。
插值算法(二):反距离加权法IDW

插值算法(⼆):反距离加权法IDW
反距离权重法主要依赖于反距离的幂值,幂参数可基于距输出点的距离来控制已知点对内插值的影响。
幂参数是⼀个正实数,默认值为2。
(⼀般0.5到3的值可获得最合理的结果)。
通过定义更⾼的幂值,可进⼀步强调最近点。
因此,邻近数据将受到更⼤影响,表⾯会变得更加详细(更不平滑)。
随着幂数的增⼤,内插值将逐渐接近最近采样点的值。
指定较⼩的幂值将对距离较远的周围点产⽣更⼤的影响,从⽽导致平⾯更加平滑。
由于反距离权重公式与任何实际的物理过程都不关联,因此⽆法确定特定幂值是否过⼤。
作为常规准则,认为值为30的幂是超⼤幂,因此不建议使⽤。
此外还要牢记⼀点,如果距离或幂值较⼤,则可能⽣成错误结果。
在IDW插值之前,我们可以事先获取⼀个离散点⼦集,⽤于计算插值的权重;
原因1:离散点距离插值点越远,其对插值点的影响⼒越低,甚⾄完全没有影响⼒;
原因2:离散点越少可以加快运算速度;
IDW步骤
IDW插值⽅法假定每个输⼊点都有着局部影响,这种影响随着距离的增加⽽减弱。
步骤:
①计算未知点到所有点的距离;
②计算每个点的权重:权重是距离的倒数的函数。
idw空间插值法

idw空间插值法(实用版)目录1.IDW 插值法的基本概念2.IDW 插值法的原理3.IDW 插值法的应用实例4.IDW 插值法的优缺点正文一、IDW 插值法的基本概念IDW 插值法,全称为 Inverse Distance Weighting,即反距离加权法,是一种基于距离加权的插值方法。
该方法主要应用于空间数据的插值,通过计算空间对象之间的距离,对距离进行加权处理,然后根据加权距离计算各空间对象的插值结果。
二、IDW 插值法的原理IDW 插值法的基本原理是:距离目标点越近的参考点,对目标点的插值结果影响越大。
具体计算方法是,首先计算目标点与所有参考点之间的距离,然后对距离进行反比加权,距离越小,权重越大。
最后,根据加权距离计算目标点的插值结果。
三、IDW 插值法的应用实例IDW 插值法广泛应用于地理信息系统(GIS)、遥感图像处理、环境科学等领域。
例如,在地理信息系统中,IDW 插值法可以用于地形高程的插值、人口密度的估算等;在遥感图像处理中,IDW 插值法可以用于遥感数据的辐射定标、大气校正等;在环境科学中,IDW 插值法可以用于空气质量的预测、地下水位的模拟等。
四、IDW 插值法的优缺点IDW 插值法具有以下优点:1.插值结果较为平滑,能较好地反映空间数据的局部变化特征;2.适用于各种坐标系统,如地理坐标系、投影坐标系等;3.计算方法简单,易于实现。
然而,IDW 插值法也存在一定的缺点:1.对离群点(孤立点)较为敏感,容易受到离群点的影响,导致插值结果偏离真实值;2.当参考点数量较少时,插值结果可能存在较大误差;3.在某些情况下,IDW 插值法可能导致插值结果的局部过平滑,从而影响插值结果的准确性。
综上所述,IDW 插值法是一种常用的空间插值方法,具有一定的优点,但同时也存在一定的局限性。
反距离加权插值法步骤

反距离加权插值法步骤引言反距离加权插值法(Inverse Distance Weighting, IDW)是一种常用的空间插值方法,主要用于根据已知点的属性值推断未知点的属性值。
该方法基于距离的权重,越近的点权重越大,越远的点权重越小。
本文将详细介绍反距离加权插值法的步骤和应用。
二级标题1:反距离加权插值法的原理反距离加权插值法的原理是根据已知点的属性值和距离来推断未知点的属性值。
其基本假设是:在空间上,距离较近的点之间具有较高的相似性,因此可以通过距离的加权来进行插值。
二级标题2:反距离加权插值法的步骤反距离加权插值法的步骤主要包括以下几个部分:三级标题1:确定已知点和未知点首先需要确定已知点和未知点的位置。
已知点是指已经测量或观测到的点,其属性值是已知的;未知点是指需要进行插值的点,其属性值是未知的。
三级标题2:计算距离权重根据已知点和未知点的位置,可以计算出已知点与未知点之间的距离。
常用的距离计算方法有欧氏距离、曼哈顿距离等。
然后,根据距离计算出权重,距离越近的点权重越大,距离越远的点权重越小。
三级标题3:计算插值结果根据已知点的属性值和距离权重,可以计算出未知点的属性值。
一般情况下,使用加权平均的方法进行插值计算,即将已知点的属性值乘以对应的权重,然后求和并除以权重的总和,得到未知点的属性值。
三级标题4:确定权重参数反距离加权插值法中,权重参数的选择对插值结果有重要影响。
一般情况下,可以根据经验或者实际情况来确定权重参数。
常用的权重参数有幂指数、距离阈值等。
二级标题3:反距离加权插值法的应用反距离加权插值法在地理信息系统、地质勘探、气象预测等领域有着广泛的应用。
下面列举几个具体的应用场景:1.地形插值:根据已知地点的高程值,推断未知地点的高程值,用于地形展示和地图制作。
2.污染扩散模拟:根据已知污染源的浓度值,推断未知地点的污染浓度,用于评估污染扩散的影响范围。
3.气象预测:根据已知气象站点的观测数据,推断未知地点的气象数据,用于天气预报和气候研究。
反距离加权插值法公式
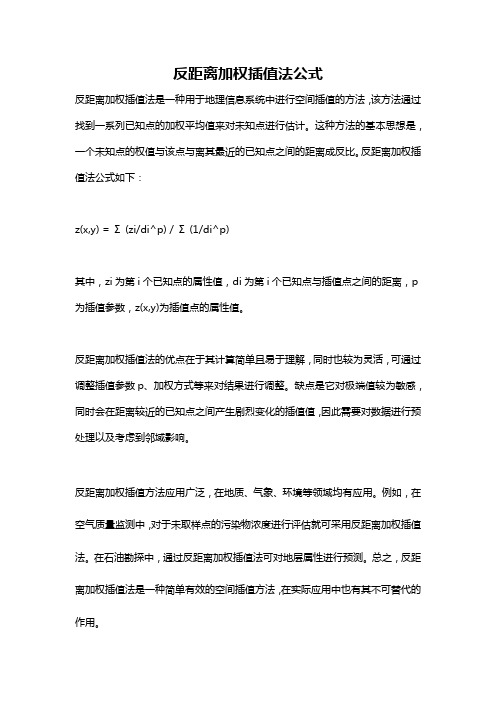
反距离加权插值法公式
反距离加权插值法是一种用于地理信息系统中进行空间插值的方法,该方法通过找到一系列已知点的加权平均值来对未知点进行估计。
这种方法的基本思想是,一个未知点的权值与该点与离其最近的已知点之间的距离成反比。
反距离加权插值法公式如下:
z(x,y) = Σ(zi/di^p) / Σ(1/di^p)
其中,zi为第i个已知点的属性值,di为第i个已知点与插值点之间的距离,p 为插值参数,z(x,y)为插值点的属性值。
反距离加权插值法的优点在于其计算简单且易于理解,同时也较为灵活,可通过调整插值参数p、加权方式等来对结果进行调整。
缺点是它对极端值较为敏感,同时会在距离较近的已知点之间产生剧烈变化的插值值,因此需要对数据进行预处理以及考虑到邻域影响。
反距离加权插值方法应用广泛,在地质、气象、环境等领域均有应用。
例如,在空气质量监测中,对于未取样点的污染物浓度进行评估就可采用反距离加权插值法。
在石油勘探中,通过反距离加权插值法可对地层属性进行预测。
总之,反距离加权插值法是一种简单有效的空间插值方法,在实际应用中也有其不可替代的作用。
idw反距离加权插值法
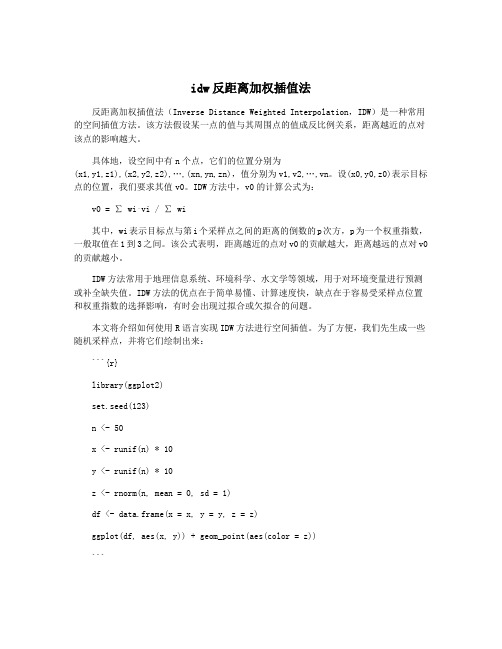
idw反距离加权插值法反距离加权插值法(Inverse Distance Weighted Interpolation,IDW)是一种常用的空间插值方法。
该方法假设某一点的值与其周围点的值成反比例关系,距离越近的点对该点的影响越大。
具体地,设空间中有n个点,它们的位置分别为(x1,y1,z1),(x2,y2,z2),…,(xn,yn,zn),值分别为v1,v2,…,vn。
设(x0,y0,z0)表示目标点的位置,我们要求其值v0。
IDW方法中,v0的计算公式为:v0 = ∑ wi⋅vi / ∑ wi其中,wi表示目标点与第i个采样点之间的距离的倒数的p次方,p为一个权重指数,一般取值在1到3之间。
该公式表明,距离越近的点对v0的贡献越大,距离越远的点对v0的贡献越小。
IDW方法常用于地理信息系统、环境科学、水文学等领域,用于对环境变量进行预测或补全缺失值。
IDW方法的优点在于简单易懂、计算速度快,缺点在于容易受采样点位置和权重指数的选择影响,有时会出现过拟合或欠拟合的问题。
本文将介绍如何使用R语言实现IDW方法进行空间插值。
为了方便,我们先生成一些随机采样点,并将它们绘制出来:```{r}library(ggplot2)set.seed(123)n <- 50x <- runif(n) * 10y <- runif(n) * 10z <- rnorm(n, mean = 0, sd = 1)df <- data.frame(x = x, y = y, z = z)ggplot(df, aes(x, y)) + geom_point(aes(color = z))```这里我们生成了50个二维的随机采样点,其横坐标和纵坐标都在0到10之间,纵轴的值服从均值为0、标准差为1的正态分布。
我们使用ggplot2库将它们绘制出来,其中纵轴的值表示为颜色。
下面是使用IDW方法进行空间插值的代码:```{r}# 定义目标点和权重指数x0 <- 5y0 <- 5p <- 2# 计算距离的倒数的p次方distances <- sqrt((x - x0)^2 + (y - y0)^2)^(-p)distances[is.infinite(distances)] <- 0result```这里我们定义了目标点的位置(x0,y0),以及权重指数p。
空间插值算法-反距离加权法
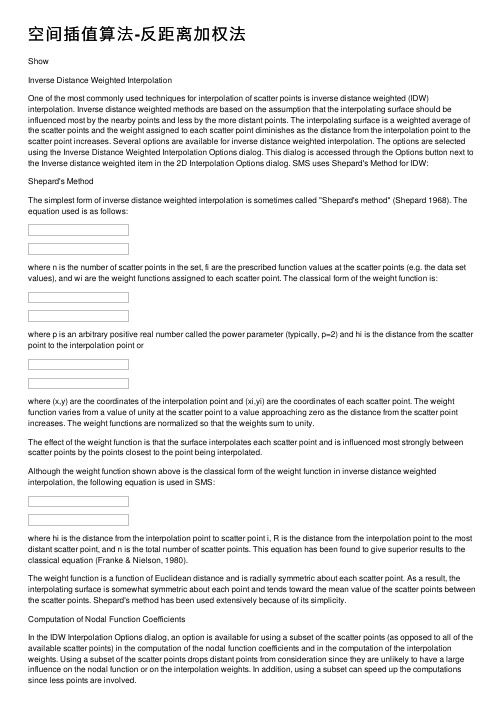
空间插值算法-反距离加权法ShowInverse Distance Weighted InterpolationOne of the most commonly used techniques for interpolation of scatter points is inverse distance weighted (IDW) interpolation. Inverse distance weighted methods are based on the assumption that the interpolating surface should be influenced most by the nearby points and less by the more distant points. The interpolating surface is a weighted average of the scatter points and the weight assigned to each scatter point diminishes as the distance from the interpolation point to the scatter point increases. Several options are available for inverse distance weighted interpolation. The options are selected using the Inverse Distance Weighted Interpolation Options dialog. This dialog is accessed through the Options button next to the Inverse distance weighted item in the 2D Interpolation Options dialog. SMS uses Shepard's Method for IDW:Shepard's MethodThe simplest form of inverse distance weighted interpolation is sometimes called "Shepard's method" (Shepard 1968). The equation used is as follows:where n is the number of scatter points in the set, fi are the prescribed function values at the scatter points (e.g. the data set values), and wi are the weight functions assigned to each scatter point. The classical form of the weight function is:where p is an arbitrary positive real number called the power parameter (typically, p=2) and hi is the distance from the scatter point to the interpolation point orwhere (x,y) are the coordinates of the interpolation point and (xi,yi) are the coordinates of each scatter point. The weight function varies from a value of unity at the scatter point to a value approaching zero as the distance from the scatter point increases. The weight functions are normalized so that the weights sum to unity.The effect of the weight function is that the surface interpolates each scatter point and is influenced most strongly between scatter points by the points closest to the point being interpolated.Although the weight function shown above is the classical form of the weight function in inverse distance weighted interpolation, the following equation is used in SMS:where hi is the distance from the interpolation point to scatter point i, R is the distance from the interpolation point to the most distant scatter point, and n is the total number of scatter points. This equation has been found to give superior results to the classical equation (Franke & Nielson, 1980).The weight function is a function of Euclidean distance and is radially symmetric about each scatter point. As a result, the interpolating surface is somewhat symmetric about each point and tends toward the mean value of the scatter points between the scatter points. Shepard's method has been used extensively because of its simplicity.Computation of Nodal Function CoefficientsIn the IDW Interpolation Options dialog, an option is available for using a subset of the scatter points (as opposed to all of the available scatter points) in the computation of the nodal function coefficients and in the computation of the interpolation weights. Using a subset of the scatter points drops distant points from consideration since they are unlikely to have a large influence on the nodal function or on the interpolation weights. In addition, using a subset can speed up the computations since less points are involved.If the Use subset of points option is chosen, the Subsets button can be used to bring up the Subset Definition dialog. Two options are available for defining which points are included in the subset. In one case, only the nearest N points are used. In the other case, only the nearest N points in each quadrant are used as shown below. This approach may give better results if the scatter points tend to be clustered.The Four Quadrants Surrounding an Interpolation Point.If a subset of the scatter point set is being used for interpolation, a scheme must be used to find the nearest N points. Two methods for finding a subset are provided in the Subset Definition dialog: the global method and the local method.Global MethodWith the global method, each of the scatter points in the set are searched for each interpolation point to determine which N points are nearest the interpolation point. This technique is fast for small scatter point sets but may be slow for large sets. Local MethodWith the local methods, the scatter points are triangulated to form a temporary TIN before the interpolation process begins. To compute the nearest N points, the triangle containing the interpolation point is found and the triangle topology is then used to sweep out from the interpolation point in a systematic fashion until the N nearest points are found. The local scheme is typically much faster than the global scheme for large scatter point sets.Computation of Interpolation WeightsWhen computing the interpolation weights, three options are available for determining which points are included in the subset of points used to compute the weights and perform the interpolation: subset, all points, and enclosing triangle. Subset of PointsIf the Use subset of points option is chosen, the Subset Definition dialog can be used to define a local subset of points.All PointsIf the Use all points option is chosen, a weight is computed for each point and all points are used in the interpolation. Enclosing TriangleThe Use vertices of enclosing triangle method makes the interpolation process a local scheme by taking advantage of TIN topology (Franke & Nielson, 1980). With this technique, the subset of points used for interpolation consists of the three vertices of the triangle containing the interpolation point. The weight function or blending function assigned to each scatter point is a cubic S-shaped function as shown in part a of the figure below. The fact that the slope of the weight function tends to unity at its limits ensures that the slope of the interpolating surface is continuous across triangle boundaries.(a) S-Shaped Weight Function and (b) Delauney Point Group for Point A.The influence of the weight function extends over the limits of the Delauney point group of the scatter point. The Delauney point group is the "natural neighbors" of the scatter point, and the perimeter of the group is made up of the outer edges of the triangles that are connected to the scatter point as shown in part b. The weight function varies from a weight of unity at the scatter point to zero at the perimeter of the group. For every interpolation point in the interior of a triangle there are three nonzero weight functions (the weight functions of the three vertices of the triangle). For a triangle T with vertices i, j, & k, the weights for each vertex are determined as follows:where ||ei|| is the length of the edge opposite vertex i, and bi, bj, bk are the area coordinates of the point (x,y) with respect to triangle T. Area coordinates are coordinates that describe the position of a point within the interior of a triangle relative to the vertices of the triangle. The coordinates are based solely on the geometry of the triangle. Area coordinates are sometimescalled "barycentric coordinates." The relative magnitude of the coordinates corresponds to area ratios as shown below:Barycentric Coordinates for a Point in a Triangle.The XY coordinates of the interior point can be written in terms of the XY coordinates of the vertices using the area coordinates as follows:Solving the above equations for bi, bj, and bk yields:Using the weight functions defined above, the interpolating surface at points inside a triangle is computed as:where wi, wj, and wk are the weight functions and Qi, Qj, and Qk are the nodal functions for the three vertices of the triangle. Related TopicsScatter Interpolation。
反距离加权法

反距离加权插值来源:互联网反距离权(IDW Inverse Distance Weighted)插值法是基于相近相似的原理:即两个物体离得近,它们的性质就越相似,反之,离得越远则相似性越小。
它以插值点与样本点间的距离为权重进行加权平均,离插值点越近的样本点赋予的权重越大。
反距离加权插值法的一般公式如下:其中,为处的预测值;N 为预测计算过程中要使用的预测点周围样点的数量;为预测计算过程中使用的各样点的权重,该值随着样点与预测点之间距离的增加而减少;是在处获得的测量值。
确定权重的计算公式为:其中,P 为指数值;是预测点与各已知样点之间的距离。
样点在预测点值的计算过程中所占权重的大小受参数p 的影响;也就是说,随着采样点与预测值之间距离的增加,标准样点对预测点影响的权重按指数规律减少。
在预测过程中,各样点值对预测点值作用的权重大小是成比例的,这些权重值的总和为1。
在ArcGIS9.0 中利用反距离加权工具进行空间插值的基本步骤为:1. 在ArcMap中加载地统计数据点图层。
2. 单击Geostatistical Analyst模块的下拉箭头点击Geostatistical Wizard命令,弹出图1所示界面;图1 输入数据和方法选择对话框参数说明:(1) Dataset11)Input:选择进行内插的实验数据。
2) Attribute: 选择进行内插的实验数据的属性。
(2)Validation1) Input:选择进行内插的检验数据。
2) Attribute: 选择进行内插的检验数据的属性。
(3) Method:选择数据的内插方法。
为了保证内插的精度,在此选择检验数据。
为了能够将表面精确地描绘出来,需保证训练数据集中有足够的样本。
若训练数据集中数据太少或含有异常值,会造成模型参数错误及输出结果变形。
3. 选择Inverse Distance Weighting ,然后单击Next, 弹出图2所示界面参数设置:(1) Power:即公式中的p参数值。
反距离加权插值法例题
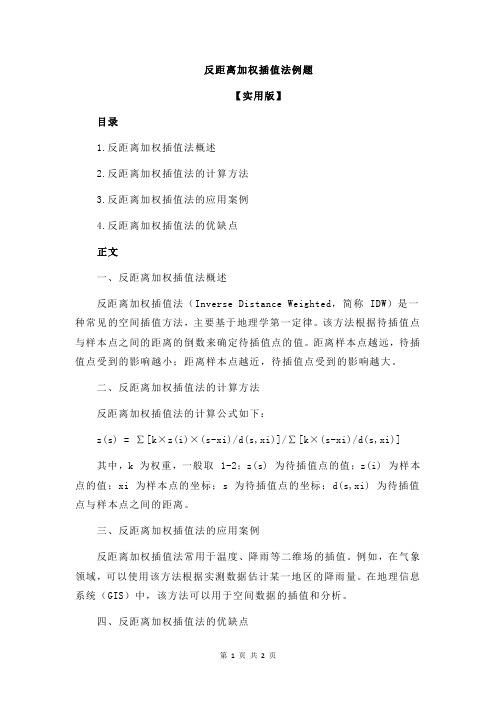
反距离加权插值法例题
【实用版】
目录
1.反距离加权插值法概述
2.反距离加权插值法的计算方法
3.反距离加权插值法的应用案例
4.反距离加权插值法的优缺点
正文
一、反距离加权插值法概述
反距离加权插值法(Inverse Distance Weighted,简称 IDW)是一种常见的空间插值方法,主要基于地理学第一定律。
该方法根据待插值点与样本点之间的距离的倒数来确定待插值点的值。
距离样本点越远,待插值点受到的影响越小;距离样本点越近,待插值点受到的影响越大。
二、反距离加权插值法的计算方法
反距离加权插值法的计算公式如下:
z(s) = ∑[k×z(i)×(s-xi)/d(s,xi)]/∑[k×(s-xi)/d(s,xi)]
其中,k 为权重,一般取 1-2;z(s) 为待插值点的值;z(i) 为样本点的值;xi 为样本点的坐标;s 为待插值点的坐标;d(s,xi) 为待插值点与样本点之间的距离。
三、反距离加权插值法的应用案例
反距离加权插值法常用于温度、降雨等二维场的插值。
例如,在气象领域,可以使用该方法根据实测数据估计某一地区的降雨量。
在地理信息系统(GIS)中,该方法可以用于空间数据的插值和分析。
四、反距离加权插值法的优缺点
优点:
1.计算简单,计算效率较高;
2.适用于不同形状的采样点,具有较强的通用性;
3.能够较好地反映空间数据的局部特征。
反距离加权插值方法研究

倒数距离加权插值,又称“反距离加权平均”或“Shepard 方法”。
设有n 个点,平面坐标为),(i i y x ,垂直高度为i z ,n i ,,2,1 =,倒数距离加权插值的插值函数为⎪⎪⎪⎩⎪⎪⎪⎨⎧===≠=∑∑==时当时当n i y x y x zn i y x y x d d z y x f i i ii i nj p j n j p j j,,2,1,),(),(,,2,1,),(),(1),(11 。
其中,22)()(j j j y y x x d -+-=是),(y x 点到),(j j y x 点的水平距离,n j ,,2,1 =。
p 是一个大于0的常数,称为加权幂指数。
容易看出,z ∑∑===nj p jnj p jjddz 111是 n z z z ,,,21 的加权平均。
),(y x f 是用分段表达式表达的,看起来不连续,实际上,它是处处连续的。
),(lim y x f i i y y x x →→∑∑==→→=n j pjnj p j jy y x x d dz i i 111lim p n p i p i p i p p nn p i i p i i p i i p d d d d d d d z d z d z d z d z i 11111lim1111111110++++++++++++=+-++--→ p n p ip i p i p i p i p p i pn pi n pi p i i i p i p i i p p i d d d d d d d d d d d z d d z z d d z d d z i ++++++++++++=+-++--→ 11111111101lim ),(i i i y x f z == ,所以,),(y x f 在),(i i y x 连续。
加权幂指数p 可以调节插值函数曲面的形状。
p 越大,在节点处函数曲面越平坦;p 越小,在节点处函数曲面越尖锐。
- 1、下载文档前请自行甄别文档内容的完整性,平台不提供额外的编辑、内容补充、找答案等附加服务。
- 2、"仅部分预览"的文档,不可在线预览部分如存在完整性等问题,可反馈申请退款(可完整预览的文档不适用该条件!)。
- 3、如文档侵犯您的权益,请联系客服反馈,我们会尽快为您处理(人工客服工作时间:9:00-18:30)。
ShowInverse Distance Weighted InterpolationOne of the most commonly used techniques for interpolation of scatter points is inverse distance weighted (IDW) interpolation. Inverse distance weighted methods are based on the assumption that the interpolating surface should be influenced most by the nearby points and less by the more distant points. The interpolating surface is a weighted average of the scatter points and the weight assigned to each scatter point diminishes as the distance from the interpolation point to the scatter point increases. Several options are available for inverse distance weighted interpolation. The options are selected using the Inverse Distance Weighted Interpolation Options dialog. This dialog is accessed through the Options button next to the Inverse distance weighted item in the 2D Interpolation Options dialog. SMS uses Shepard's Method for IDW:Shepard's MethodThe simplest form of inverse distance weighted interpolation is sometimes called "Shepard's method" (Shepard 1968). The equation used is as follows:where n is the number of scatter points in the set, fi are the prescribed function values at the scatter points (e.g. the data set values), and wi are the weight functions assigned to each scatter point. The classical form of the weight function is:where p is an arbitrary positive real number called the power parameter (typically, p=2) and hi is the distance from the scatter point to the interpolation point orwhere (x,y) are the coordinates of the interpolation point and (xi,yi) are the coordinates of each scatter point. The weight function varies from a value of unity at the scatter point to a value approaching zero as the distance from the scatter point increases. The weight functions are normalized so that the weights sum to unity.The effect of the weight function is that the surface interpolates each scatter point and is influenced most strongly between scatter points by the points closest to the point being interpolated.Although the weight function shown above is the classical form of the weight function in inverse distance weighted interpolation, the following equation is used in SMS:where hi is the distance from the interpolation point to scatter point i, R is the distance from the interpolation point to the most distant scatter point, and n is the total number of scatter points. This equation has been found to give superior results to the classical equation (Franke & Nielson, 1980).The weight function is a function of Euclidean distance and is radially symmetric about each scatter point. As a result, the interpolating surface is somewhat symmetric about each point and tends toward the mean value of the scatter points between the scatter points. Shepard's method has been used extensively because of its simplicity.Computation of Nodal Function CoefficientsIn the IDW Interpolation Options dialog, an option is available for using a subset of the scatter points (as opposed to all of the available scatter points) in the computation of the nodal function coefficients and in the computation of the interpolation weights. Using a subset of the scatter points drops distant points from consideration since they are unlikely to have a large influence on the nodal function or on the interpolation weights. In addition, using a subset can speed up the computations since less points are involved.If the Use subset of points option is chosen, the Subsets button can be used to bring up the Subset Definition dialog. Two options are available for defining which points are included in the subset. In one case, only the nearest N points are used. In the other case, only the nearest N points in each quadrant are used as shown below. This approach may give better results if the scatter points tend to be clustered.The Four Quadrants Surrounding an Interpolation Point.If a subset of the scatter point set is being used for interpolation, a scheme must be used to find the nearest N points. Two methods for finding a subset are provided in the Subset Definition dialog: the global method and the local method.Global MethodWith the global method, each of the scatter points in the set are searched for each interpolation point to determine which N points are nearest the interpolation point. This technique is fast for small scatter point sets but may be slow for large sets.Local MethodWith the local methods, the scatter points are triangulated to form a temporary TIN before the interpolation process begins. To compute the nearest N points, the triangle containing the interpolation point is found and the triangle topology is then used to sweep out from the interpolation point in a systematic fashion until the N nearest points are found. The local scheme is typically much faster than the global scheme for large scatter point sets.Computation of Interpolation WeightsWhen computing the interpolation weights, three options are available for determining which points are included in the subset of points used to compute the weights and perform the interpolation: subset, all points, and enclosing triangle.Subset of PointsIf the Use subset of points option is chosen, the Subset Definition dialog can be used to define a local subset of points.All PointsIf the Use all points option is chosen, a weight is computed for each point and all points are used in the interpolation.Enclosing TriangleThe Use vertices of enclosing triangle method makes the interpolation process a local scheme by taking advantage of TIN topology (Franke & Nielson, 1980). With this technique, the subset of points used for interpolation consists of the three vertices of the triangle containing the interpolation point. The weight function or blending function assigned to each scatter point is a cubic S-shaped function as shown in part a of the figure below. The fact that the slope of the weight function tends to unity at its limits ensures that the slope of the interpolating surface is continuous across triangle boundaries.(a) S-Shaped Weight Function and (b) Delauney Point Group for Point A.The influence of the weight function extends over the limits of the Delauney point group of the scatter point. The Delauney point group is the "natural neighbors" of the scatter point, and the perimeter of the group is made up of the outer edges of the triangles that are connected to the scatter point as shown in part b. The weight function varies from a weight of unity at the scatter point to zero at the perimeter of the group. For every interpolation point in the interior of a triangle there are three nonzero weight functions (the weight functions of the three vertices of the triangle). For a triangle T with vertices i, j, & k, the weights for each vertex are determined as follows:where ||ei|| is the length of the edge opposite vertex i, and bi, bj, bk are the area coordinates of the point (x,y) with respect to triangle T. Area coordinates are coordinates that describe the position of a point within the interior of a triangle relative to the vertices of the triangle. The coordinates are based solely on the geometry of the triangle. Area coordinates are sometimes called "barycentric coordinates." The relative magnitude of the coordinates corresponds to area ratios as shown below:Barycentric Coordinates for a Point in a Triangle.The XY coordinates of the interior point can be written in terms of the XY coordinates of the vertices using the area coordinates as follows:Solving the above equations for bi, bj, and bk yields:Using the weight functions defined above, the interpolating surface at points inside a triangle is computed as:where wi, wj, and wk are the weight functions and Qi, Qj, and Qk are the nodal functions for the three vertices of the triangle.Related TopicsScatter Interpolation。