exponential
exponential和linear式子 -回复
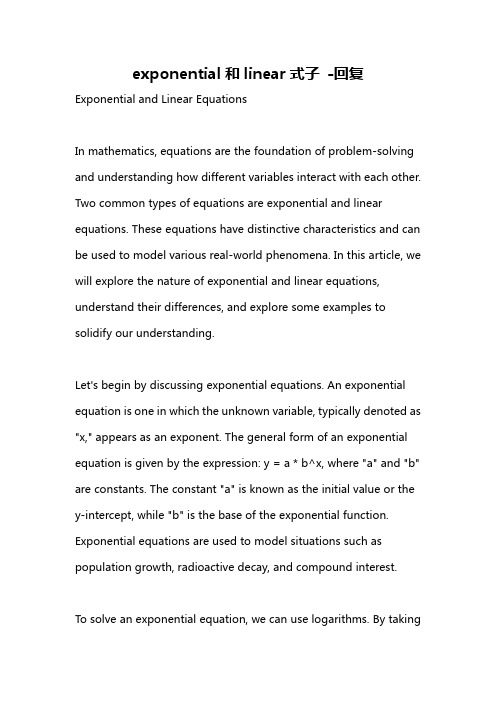
exponential和linear式子-回复Exponential and Linear EquationsIn mathematics, equations are the foundation of problem-solving and understanding how different variables interact with each other. Two common types of equations are exponential and linear equations. These equations have distinctive characteristics and can be used to model various real-world phenomena. In this article, we will explore the nature of exponential and linear equations, understand their differences, and explore some examples to solidify our understanding.Let's begin by discussing exponential equations. An exponential equation is one in which the unknown variable, typically denoted as "x," appears as an exponent. The general form of an exponential equation is given by the expression: y = a * b^x, where "a" and "b" are constants. The constant "a" is known as the initial value or the y-intercept, while "b" is the base of the exponential function. Exponential equations are used to model situations such as population growth, radioactive decay, and compound interest.To solve an exponential equation, we can use logarithms. By takingthe logarithm of both sides of the equation, we can isolate the variable and find its value. For example, consider the equation 2^x = 64. Taking the logarithm (base 2) of both sides gives us x * log (2) = log (64). Simplifying this further, we find x = log (64) / log (2), which is approximately 6.On the other hand, linear equations are equations in which the unknown variable, "x," appears with a power of one. The general form of a linear equation is: y = mx + b, where "m" is the slope or rate of change, and "b" is the y-intercept. Linear equations represent straight lines on a graph and are widely used to represent relationships in various fields such as physics, economics, and engineering.Solving linear equations involves finding the value of the unknown variable that satisfies the equation. We can use various methods such as substitution, elimination, or graphing to solve linear equations. For instance, consider the equation 3x + 2y = 10. By rearranging the equation, we can solve for "x" or "y" in terms of the other variable. Let's solve for "y": 2y = 10 - 3x. Dividing both sides by 2 gives us y = (10 - 3x) / 2, which represents the equation of a line.Now that we have understood the general forms and solving techniques for exponential and linear equations, let's explore some practical examples. Suppose we have an exponential equation that models the growth of a bacterial population in a laboratory. The equation is given by P = 1000 * (1.5)^t, where "P" represents the population size in thousands, and "t" represents the time in hours. To find the population after 5 hours, we substitute t = 5 into the equation: P = 1000 * (1.5)^5. Evaluating this, we find that the population after 5 hours is approximately 2812.5.On the other hand, let's consider a linear equation that represents the cost of a taxi ride. The equation is given by C = 3x + 10, where "C" represents the cost in dollars and "x" represents the distance in miles. If we want to find the cost of a 7-mile taxi ride, we substitute x = 7 into the equation: C = 3 * 7 + 10. Simplifying this, we find that the cost of the 7-mile taxi ride is 31.In summary, exponential and linear equations are essential tools in mathematics that model relationships between variables. Exponential equations involve an unknown variable as an exponent,while linear equations have the variable to the power of one. By understanding the general forms and solving techniques for these equations, we can apply them to model various situations in the real world. Whether it is modeling population growth or calculating taxi fares, exponential and linear equations prove to be valuable in practical problem-solving.。
指数平滑移动平均线【Exponential】

据于上述现象,许多投资者于是用移动平均线来作为买卖股票的主要依据,意思是:当月线指标(即5天均线、10天均线和20天均线)形成多头排列时,他们通常会买进。反之,当月线指标形成空头排列时,他们通常会卖出。但是,由于市场主力经常会进行反技术的操作,故意令股价连连破位,从而打穿上述均线,这样做会令很多人的筹码因此而脱手。
研判标准
1. EXPMA指标由EXPMA1(白线)和EXPMA2(黄线)组成,当白线由下往上穿越黄线时,股价随后通常会不断上升,那么这两根线形成金叉之日便是买入良机。
2. 当一只个股的股价远离白线后,该股的股价随后很快便会回落,然后再沿着白线上移,可见白线是一大支撑点。
3. 同理,当白线由上往下击穿黄线时,股价往往已经发生转势,日后将会以下跌为主,则这两根线的交叉之日便是卖出时机。
Байду номын сангаас市场意义
1. 该指标一般为中短线选股指标,比较符合以中短线为主的投资者,据此信号买入者均有获利机会,但对中线投资者来说,其参考意义似乎更大,主要是因为该指标稳定性大,波动性小。
2. 若白线和黄线始终保持距离地上行,则说明该股后市将继续看好,每次股价回落至白线附近,只要不击穿黄线,则这种回落现象便是良好的买入时机。[1]
指数平滑移动平均线【Exponential】
译指数平滑移动平均线,乃为因应移动平均线被视为落后指标的缺失而发展出来的,为解决一旦价格已脱离均线差值扩大,而平均线未能立即反应,EXPMA可以减少类似缺点。
[管理工具-决策预测]指数平滑法(ExponentialSmoothing,ES)
![[管理工具-决策预测]指数平滑法(ExponentialSmoothing,ES)](https://img.taocdn.com/s3/m/bfb05d71f4335a8102d276a20029bd64783e62e7.png)
指数平滑法(Exponential Smoothing,ES)什么是指数平滑法指数平滑法是布朗(Robert G..Brown)所提出,布朗(Robert G..Brown)认为时间序列的态势具有稳定性或规则性,所以时间序列可被合理地顺势推延;他认为最近的过去态势,在某种程度上会持续到最近的未来,所以将较大的权数放在最近的资料。
指数平滑法是生产预测中常用的一种方法。
也用于中短期经济发展趋势预测,所有预测方法中,指数平滑是用得最多的一种。
简单的全期平均法是对时间数列的过去数据一个不漏地全部加以同等利用;移动平均法则不考虑较远期的数据,并在加权移动平均法中给予近期资料更大的权重;而指数平滑法则兼容了全期平均和移动平均所长,不舍弃过去的数据,但是仅给予逐渐减弱的影响程度,即随着数据的远离,赋予逐渐收敛为零的权数。
也就是说指数平滑法是在移动平均法基础上发展起来的一种时间序列分析预测法,它是通过计算指数平滑值,配合一定的时间序列预测模型对现象的未来进行预测。
其原理是任一期的指数平滑值都是本期实际观察值与前一期指数平滑值的加权平均。
指数平滑法的基本公式指数平滑法的基本公式是:式中,∙S t--时间t的平滑值;∙y t--时间t的实际值;∙S t− 1--时间t-1的平滑值;∙a--平滑常数,其取值范围为[0,1];由该公式可知:1.S t是y t和S t− 1的加权算数平均数,随着a取值的大小变化,决定y t和S t− 1对S t的影响程度,当a 取1时,S t = y t;当a取0时,S t = S t− 1。
2.S t具有逐期追溯性质,可探源至S t−t + 1为止,包括全部数据。
其过程中,平滑常数以指数形式递减,故称之为指数平滑法。
指数平滑常数取值至关重要。
平滑常数决定了平滑水平以及对预测值与实际结果之间差异的响应速度。
平滑常数a越接近于1,远期实际值对本期平滑值影响程度的下降越迅速;平滑常数a越接近于 0,远期实际值对本期平滑值影响程度的下降越缓慢。
f.elu函数
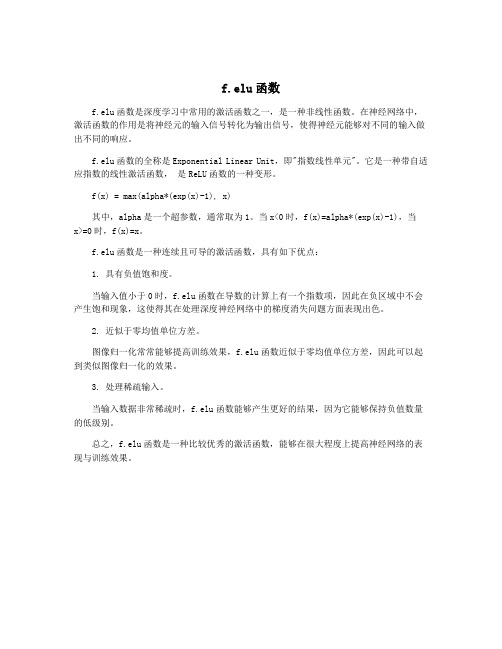
f.elu函数
f.elu函数是深度学习中常用的激活函数之一,是一种非线性函数。
在神经网络中,激活函数的作用是将神经元的输入信号转化为输出信号,使得神经元能够对不同的输入做出不同的响应。
f.elu函数的全称是Exponential Linear Unit,即"指数线性单元"。
它是一种带自适应指数的线性激活函数,是ReLU函数的一种变形。
f(x) = max(alpha*(exp(x)-1), x)
其中,alpha是一个超参数,通常取为1。
当x<0时,f(x)=alpha*(exp(x)-1),当x>=0时,f(x)=x。
f.elu函数是一种连续且可导的激活函数,具有如下优点:
1. 具有负值饱和度。
当输入值小于0时,f.elu函数在导数的计算上有一个指数项,因此在负区域中不会产生饱和现象,这使得其在处理深度神经网络中的梯度消失问题方面表现出色。
2. 近似于零均值单位方差。
图像归一化常常能够提高训练效果,f.elu函数近似于零均值单位方差,因此可以起到类似图像归一化的效果。
3. 处理稀疏输入。
当输入数据非常稀疏时,f.elu函数能够产生更好的结果,因为它能够保持负值数量的低级别。
总之,f.elu函数是一种比较优秀的激活函数,能够在很大程度上提高神经网络的表现与训练效果。
exp翻译中文什么意思

exp翻译中文什么意思exp,它在数学里就是指数函数的意思,英语是exponential。
下面是店铺给大家整理的exp翻译中文什么意思,供大家参阅!exp翻译中文什么意思EXP指的是指数函数exp,高等数学里以自然常数e为底的指数函数,它又是航模名词,全称Exponential(指数曲线)。
在医药说明中,EXP是指使用期限,即Expiry date(Exp date) 。
除此之外,EXP(Expedition) 是世界著名项目管理软件供应商美国Primavera公司的主要产品之一,是国际规范的施工管理和合同及建设信息管理软件。
exp还指行业软件的高级专家版,在灵活性和功能上比专业版(pro)更加强大,也更加复杂。
exp相关信息在游戏里是经验值,experience的缩写由LIB工具从DEF文件生成的输出文件,其中包含了函数和数据项目的输出信息,LINK工具将使用EXP文件来创建动态链接库。
Oracle数据库的命令,用来导出数据EXP.EXE是一个木马程序。
这个木马运行攻击者访问你的计算机,窃取密码和个人数据。
这个进程的安全等级是建议立即进行删除。
exp在Oracle里面的意思介绍导入(IMP)/导出(EXP)是ORACLE幸存的最古老的两个操作系统命令行工具,Exp/Imp是一个好的转储工具,特别是在小型数据库的转储,表空间的迁移,表的抽取,检测逻辑和物理冲突等中有不小的功劳。
它作为小型数据库的物理备份后的一个逻辑辅助备份,也是不错的手段。
对于越来越大的数据库,特别是TB级数据库和越来越多数据仓库的出现,EXP/IMP越来越力不从心了,这个时候,数据库的备份都转向了RMAN和第三方工具。
UNIX如何使exp的帮助以不同的字符集显示:setnls_lang=simplified chinese_china.zhs16gbk,通过设置环境变量,可以让exp的帮助以中文显示,如果setnls_lang=American_america.字符集,那么帮助就是英文的了参数USERID 用户名/口令如:USERID=duanl/duanlFULL 导出整个数据库 (N)BUFFER 数据缓冲区的大小OWNER 所有者用户名列表,你希望导出哪个用户的对象,就用owner=usernameFILE 输出文件 (EXPDAT.DMP)TABLES 表名列表,指定导出的table名称,如:TABLES=table1,table2COMPRESS 导入一个extent (Y)RECORDLENGTH IO 记录的长度GRANTS 导出权限 (Y)INCTYPE 增量导出类型INDEXES 导出索引 (Y)RECORD 跟踪增量导出 (Y)ROWS 导出数据行 (Y)PARFILE 参数文件名,如果你exp的参数很多,可以存成参数文件.CONSTRAINTS 导出约束 (Y)CONSISTENT 交叉表一致性LOG 屏幕输出的日志文件STATISTICS 分析对象 (ESTIMATE)DIRECT 直接路径 (N)TRIGGERS 导出触发器 (Y)FEEDBACK 显示每 x 行 (0) 的进度FILESIZE 各转储文件的最大尺寸QUERY 选定导出表子集的子句下列关键字仅用于可传输的表空间TRANSPORT_TABLESPACE 导出可传输的表空间元数据 (N) TABLESPACES 将传输的表空间列表程序代码IMP的所有参数(括号中为参数的默认值):USERID 用户名/口令FULL 导入整个文件 (N)BUFFER 数据缓冲区大小FROMUSER 所有人用户名列表FILE 输入文件 (EXPDAT.DMP)TOUSER 用户名列表SHOW 只列出文件内容 (N)TABLES 表名列表IGNORE 忽略创建错误 (N)RECORDLENGTH IO 记录的长度GRANTS导入权限 (Y)INCTYPE 增量导入类型INDEXES 导入索引 (Y)COMMIT 提交数组插入 (N)ROWS 导入数据行 (Y)PARFILE 参数文件名LOG 屏幕输出的日志文件CONSTRAINTS 导入限制 (Y)DESTROY覆盖表空间数据文件 (N)INDEXFILE 将表/索引信息写入指定的文件SKIP_UNUSABLE_INDEXES 跳过不可用索引的维护 (N) ANALYZE执行转储文件中的 ANALYZE 语句 (Y) FEEDBACK 显示每 x 行 (0) 的进度TOID_NOVALIDATE 跳过指定类型 id 的校验FILESIZE 各转储文件的最大尺寸RECALCULATE_STATISTICS 重新计算统计值 (N)下列关键字仅用于可传输的表空间TRANSPORT_TABLESPACE 导入可传输的表空间元数据 (N)TABLESPACES 将要传输到数据库的表空间DATAFILES 将要传输到数据库的数据文件TTS_OWNERS 拥有可传输表空间集中数据的用户关于增量参数的说明:exp/imp的增量并不是真正意义上的增量,所以最好不要使用。
什么是ema(exponential moving average)是什么意思,什么叫,定

什么是EMA(Exponential Moving Average)是什么意思,什么叫,定...什么是EMA(Exponential Moving Average)EMA(Exponential Moving Average),指数平均数指标。
也叫EXPMA指标,它也是一种趋向类指标,指数平均数指标是以指数式递减加权的移动平均。
各数值的加权是随时间而指数式递减,越近期的数据加权越重,但较旧的数据也给予一定的加权。
指数平均数指标的原理与MACD指标、DMA指标相比,EXPMA指标由于其计算公式中着重考虑了价格当天(当期)行情的权重,因此指标自身的计算公式决定了作为一类趋势分析指标,它在使用中克服了MACD指标信号对于价格走势的滞后性。
同时也在一定程度中消除了DMA指标在某些时候对于价格走势所产生的信号提前性,是一个非常有效的分析指标。
EXPMA指标的计算公式EXPMA=(当日或当期收盘价-上一日或上期EXPMA)/N+上一日或上期EXPMA其中,首次上期EXPMA值为上一期收盘价,N为天数。
实际上,从EXPMA指标的构造原理和它的使用原则来看,这一指标更接近于均线指标,而且由于EXPMA指标通过对参数进行有效地设定,可以发挥出比均线指标更为直观和有用的信息。
在技术分析软件中,EXPMA指标由三条线构成,价格K线、短期EXPMA线(以白色线条或其他稍浅色的线条表示)、长期EXPMA线(以黄色线条或其他稍深色的线条表示),EXPMA指标的坐标图上,纵坐标代表价格运行的价位,横坐标代表价格运行的时间,这一点也和均线指标保持了一致。
EXPMA指标应用原则1、在多头趋势中,价格K线、短天期天数线、长天期天数线按以上顺序从高到低排列,是为多头特征;在空头趋势中,长天期天数线、短天期天数线、价格K线按以上顺序从高到低排列,是为空头特征。
2、当短天期天数线向下而上穿越长天期天数线时是一个值得注意的买入信号;此时短天期天数线对价格走势将起到助涨的作用,当短天期天数线向上而下穿越长天期天数线时是一个值得注意的卖出信号,此时长天期天数对价格走势将起到助跌的作用。
exp在物理化学中的意思

exp在物理化学中的意思
EXP在物理化学中代表“指数”的意思,它是高等数学中以自然常数e为底的指数函数,全称Exponential(指数曲线)。
在物理化学中,指数函数通常用于描述随自变量变化的快慢程度。
例如,EXP{F(X)}是e的F(X)次方,其中F(X)是一个关于自变量X的函数。
通过比较不同函数的EXP值,可以了解这些函数随自变量变化的快慢程度。
此外,在化学中,EXP也可能代表摩尔质量的单位,即克/摩尔。
摩尔质量是一个物理学单位,是指单位物质的量的物质所具有的质量,用符号M表示。
对于某一化合物来说,它的摩尔质量是固定不变的。
exp()的用法
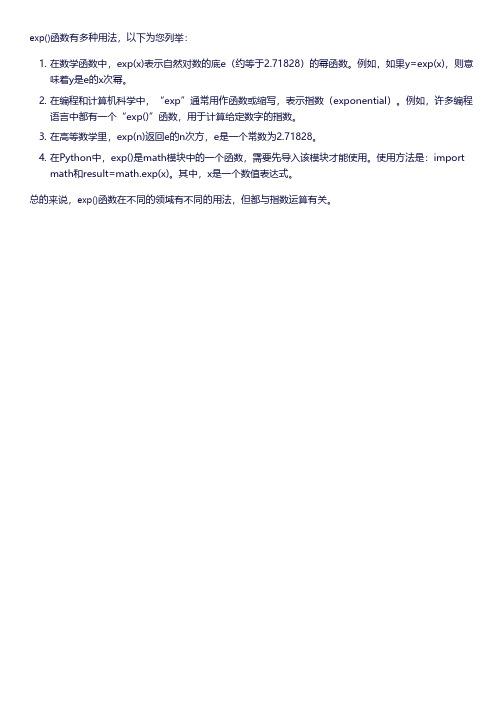
exp()函数有多种用法,以下为您列举:
1. 在数学函数中,exp(x)表示自然对数的底e(约等于
2.71828)的幂函数。
例如,如果y=exp(x),则意
味着y是e的x次幂。
2. 在编程和计算机科学中,“exp”通常用作函数或缩写,表示指数(exponential)。
例如,许多编程
语言中都有一个“exp()”函数,用于计算给定数字的指数。
3. 在高等数学里,exp(n)返回e的n次方,e是一个常数为2.71828。
4. 在Python中,exp()是math模块中的一个函数,需要先导入该模块才能使用。
使用方法是:import
math和result=math.exp(x)。
其中,x是一个数值表达式。
总的来说,exp()函数在不同的领域有不同的用法,但都与指数运算有关。
exponential regression 算法
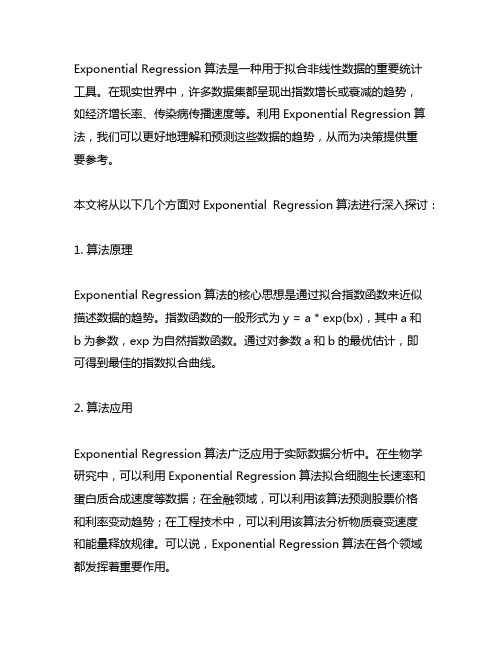
Exponential Regression算法是一种用于拟合非线性数据的重要统计工具。
在现实世界中,许多数据集都呈现出指数增长或衰减的趋势,如经济增长率、传染病传播速度等。
利用Exponential Regression算法,我们可以更好地理解和预测这些数据的趋势,从而为决策提供重要参考。
本文将从以下几个方面对Exponential Regression算法进行深入探讨:1. 算法原理Exponential Regression算法的核心思想是通过拟合指数函数来近似描述数据的趋势。
指数函数的一般形式为y = a * exp(bx),其中a和b为参数,exp为自然指数函数。
通过对参数a和b的最优估计,即可得到最佳的指数拟合曲线。
2. 算法应用Exponential Regression算法广泛应用于实际数据分析中。
在生物学研究中,可以利用Exponential Regression算法拟合细胞生长速率和蛋白质合成速度等数据;在金融领域,可以利用该算法预测股票价格和利率变动趋势;在工程技术中,可以利用该算法分析物质衰变速度和能量释放规律。
可以说,Exponential Regression算法在各个领域都发挥着重要作用。
3. 算法实现在实际应用中,Exponential Regression算法的实现涉及参数估计、误差分析等多个环节。
常用的实现方法有最小二乘法、梯度下降法等。
为了增强算法的稳健性和鲁棒性,还需要考虑异常值处理、数据归一化等技术手段。
在大规模数据集下,算法的计算效率也是一个需要重点关注的问题。
4. 算法评估对于Exponential Regression算法的效果评估,通常采用残差分析、R平方值等指标来衡量拟合效果。
另外,由于指数函数本身的非线性特性,算法的适用范围和局限性也需要认真考虑。
在某些情况下,可能需要借助其他非线性回归模型或数据转换技术来处理。
在总结部分,我们将对Exponential Regression算法的优势和不足进行分析,并展望其在未来的发展方向。
exp是什么意思的缩写_exp是什么意思
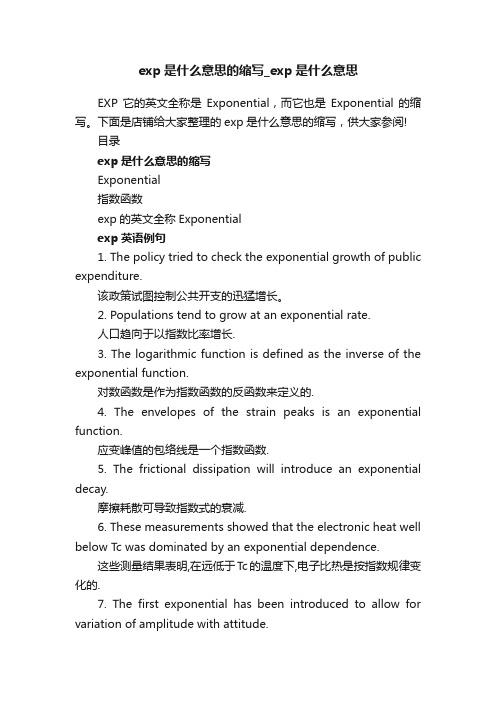
exp是什么意思的缩写_exp是什么意思EXP它的英文全称是Exponential,而它也是Exponential的缩写。
下面是店铺给大家整理的exp是什么意思的缩写,供大家参阅!目录exp是什么意思的缩写Exponential指数函数exp的英文全称Exponentialexp英语例句1. The policy tried to check the exponential growth of public expenditure.该政策试图控制公共开支的迅猛增长。
2. Populations tend to grow at an exponential rate.人口趋向于以指数比率增长.3. The logarithmic function is defined as the inverse of the exponential function.对数函数是作为指数函数的反函数来定义的.4. The envelopes of the strain peaks is an exponential function.应变峰值的包络线是一个指数函数.5. The frictional dissipation will introduce an exponential decay.摩擦耗散可导致指数式的衰减.6. These measurements showed that the electronic heat well below Tc was dominated by an exponential dependence.这些测量结果表明,在远低于T c的温度下,电子比热是按指数规律变化的.7. The first exponential has been introduced to allow for variation of amplitude with attitude.前面的指数是为了考虑波动振幅随高度变化而引入的.8. These exponential or logarithmic relationship, that characterize harmonious growth with changing proportions, are termed " allometric ".作为比例有变化的协调增长这些指数或对数关系被标为“ 开度量” 关系.9. An exponential function is one in which the variable appears in an exponent.指数函数是指数中出现变量的函数.10. Im writing a book about trigonometric and exponential functions.我在写一本关于三角和指数功能的书.11. Relationships between embryo weight and incubation time were exponential regression.乌骨鸡胚胎的重量与孵化时间的关系呈指数式回归.12. The confidence level and uncertainty limit of even exponential of uniform R.均匀随机变数偶指数函数之均值不确定范围之信心准位.13. The Fisher - type statistic is very effective for testing outlier in exponential sample.在指数样本异常值的检验中,Fisher型统计量是十分有效的.14. For many years, processing power has increased at an exponential rate.多年以来, 处理能力以几何级数增长率增加了.15. The hydrolysis rate constant increases at a exponential function with temperature increase.温度升高,水解速率常数呈指数函数关系递增.Exp的双语例句1. May I know what kind of EXP material you have?我可以知道您有什么样的EXP材料吗 ?2. EXP are divided equally among all characters participating in battle.EXP将会平均分配给参加战斗的所有角色.3. The fast and accurate evaluation of transcendental functions ( e . g . exp, log, sin, tan ) is very important in the field of scientific computing.科学计算中的许多领域都需要快速而精确地计算超越函数,即exp 、log 、 sin 、 tan 等此类函数.4. It also discusses two relatively advance application software : schedule management software P 3 and contract software EXP.介绍了目前比较先进的两种项目管理应用软件,P3进度管理软件和EXP合同管理软件.5. The regulations of EXP expression under ethylene, ASA, 1 - MCP, LTC treatments also studied in the experiment.枇杷果实为材料,研究采后果实贮藏过程中EXP基因表达模式, 以及乙烯、 ASA 、 1-MCP和LTC处理对其质地变化的调控和对EXP基因表达的影响.6. Exp . 3 : same as Exp . 1 with new question.实验 3: 与实验1相同,但探究新问题.7. Rien ne vaut l'exp é rience de la douleur pour apprendre à aimer.没有比学习爱更痛苦的经历了.8. Exp : The SOM object model also relies on this two table model.SOM对象模型也依靠这种双表格模型.9. Then he exp 1 ained how he pull the cut together with Stitches.斯通大夫说完后就开始解释他将如何缝伤口.10. True Ca and P digestibility values are 23.41±1.83 % and41.44±6.59 %, respectively . Exp.豆粕钙的真消化率为23.41±1.83%, 磷的真消化率为41.44±6.59%.11. Exp : In addition to acting , you're also a passionate photographer?除了演戏, 你也是一个很热诚的摄影师?12. Now my exp is over 1200, but I never think that is enough.现在虽然我的经验值超过1200了, 但我仍不满足.13. Chinese Imp & Exp Company that register in PuDong Shanghai.汽车和工程机械的零部件进出口公司,注册于浦东新区.14. Still, its a cool single player exp, and the price is right.尽管如此, 刀剑还是款很酷的单机游戏, 价位也比较合理.15. You receive EXP after the battle is won even when KO'd.战斗胜利之后,即使是在KO状态的角色也可以得到经验.。
exponential moving standardize

exponential moving standardize
指数移动标准化(exponentialmovingstandardize)是一种重要的技术分析工具,用于衡量金融市场中的价格趋势与波动性。
它主要通过计算价格的指数移动平均值和标准差来描绘市场的走势和波动
情况。
指数移动平均值是一种平滑的价格计算方法,它对最近的价格数据给予更高的权重,这样可以更准确地反映市场的趋势。
而指数移动标准差则是基于指数移动平均值计算的,它用于衡量价格的波动性。
在使用指数移动标准化进行技术分析时,通常会结合其他技术分析指标一起使用,如RSI、MACD等。
通过多重指标综合分析,可以更全面地了解市场的走势和趋势。
指数移动标准化的使用具有一定的局限性,它只能反映市场价格的趋势和波动情况,而无法预测市场的未来走势。
因此,在进行交易决策时,还需结合市场基本面和其他因素进行综合分析,以减少风险并获得更好的投资回报。
总之,指数移动标准化是一种非常有用的技术分析工具,它可以帮助投资者更准确地了解市场的走势和趋势,从而作出更明智的交易决策。
但是,投资者也需要注意其局限性并结合其他因素进行综合分析。
- 1 -。
指数对数转换

指数转换(exponential transformation)和对数转换(logarithmic transformation)是数学中常用的函数转换方法,用于改变数据的分布或调整数据的尺度。
它们在统计分析和数据处理中经常被应用。
指数转换是将数据通过指数函数进行转换。
常见的指数转换包括平方、立方、开方等。
通过指数转换,可以调整数据的分布形态,使其更接近于正态分布,同时也可以改变数据的尺度。
例如,假设有一组数据集合X = {1, 2, 3, 4, 5},我们可以对其进行平方转换,得到新的数据集合Y = {1, 4, 9, 16, 25}。
通过平方转换,原始数据集中较小的值得到了放大,而较大的值得到了缩小,从而改变了数据的分布。
对数转换是将数据通过对数函数进行转换。
常见的对数转换有自然对数(以 e 为底的对数)和常用对数(以10 为底的对数)。
对数转换可以将数据中较大的值压缩,同时放大较小的值,从而改变数据的分布形态和尺度。
例如,假设有一组正整数数据集合X = {1, 10, 100, 1000},我们可以对其进行常用对数转换,得到新的数据集合Y = {0, 1, 2, 3}。
通过对数转换,原始数据集中较大的值得到了缩小,而较小的值得到了放大,从而改变了数据的尺度。
指数转换和对数转换在统计分析中常用于处理偏态分布的数据、缩小数据范围、线性化关系等。
它们可以使数据更加符合统计模型的假设,提高模型的拟合效果,或者改善数据的可视化效果。
- 1、下载文档前请自行甄别文档内容的完整性,平台不提供额外的编辑、内容补充、找答案等附加服务。
- 2、"仅部分预览"的文档,不可在线预览部分如存在完整性等问题,可反馈申请退款(可完整预览的文档不适用该条件!)。
- 3、如文档侵犯您的权益,请联系客服反馈,我们会尽快为您处理(人工客服工作时间:9:00-18:30)。
All points in Θ1−α have a higher density than any point outside the regions. Often requires iterative solution: Find points such that p(θ|Y ) > h Find probability of that set Adjust h until reach desired coverage may not have symmetric tail areas multimodal posterior may not have an interval
L(β) ∝ β n exp(−β yi )
One Parameter Models – p. 7/2
Gamma Distribution
Z ∼ Gamma(a, b) with mean a/b and variance a/b2 with density ba a−1 f (z) = exp(−zb) z Γ(a) z>0
f (y|β) = β exp(−yβ) L(β; y1 , . . . , yn ) =
i
y>0
β exp (−yi β) yi β)
i
(Likelihood)
= β n exp (−
Look at plot of L(β) for
yi = 336.
One Parameter Models – p. 4/2
Distribution of β
Identify normalizing constant to make this a density for β or recognize the form of the distribution
p(β|y1 , . . . , yn ) = cβ n exp(−β yi )
> qgamma(.025, 10, 336) [1] 0.01427199 > qgamma(.975, 10, 336) [1] 0.05084763
One Parameter Models – p. 15/2
Examples of Conjugate Families
Data Distributions Prior/Posterior Exponential Gamma Gamma Poisson Beta Binomial Normal Normal (known variance) Normal (unknown mean/variance) Normal-Gamma Always available in exponential families
P yi )
(1) (2) (3) (4)
yi )
“Uniform” is a limiting case with a = 1 and b → 0 “default” prior is p(β) ∝ 1/β – a “Gamma(0,0)”
One Parameter Models – p. 12/2
One Parameter Models – p. 10/2
Uniform Prior
“Uniform” prior p(β) = 1 in exponential example is not a proper distribution; although the posterior distribution is a proper distribution. “Formal Bayes” posterior distribution obtained as a limit of a proper Bayes procedure. Be very careful with improper prior distributions, they may not lead to proper posterior distributions!
One Parameter Models – p. 8/2
Examples of Gamma Distributions
0.20
a = 1, r = 1/5 a = 2, r = 1/5 a = 2, r = 1/2
Density
0.05
0.10
0.15
0
2
4 x
6
8
10
One Parameter Models – p. 9/2
One Parameter Models
September 22, 2010
Reading: Hoff Chapter 3
One Parameter Models – p. 1/2
Highest Posterior Density Regions
Find
Θ1−α = {θ : p(θ | Y ) ≥ hα } such that P (θ ∈ Θ1−α | Y ) = 1−α
In this parameterization b is a rate parameter.
rgamma(n, shape=a, scale=s) # mean = shape*scale rgamma(n, shape=a, rate=r) # mean = shape/rate
Mode is at (a − 1)/b if a > 1 and at 0 if a ≤ 1
One Parameter Models – p. 16/2
Exponential Family
One parameter exponential family is expressed as
p(y | θ) = h(y)c(θ) exp(θt(y))
where θ is the natural parameter of the exponential family and t(y) is the sufficient statistic Likelihood: L(θ) ∝ c(θ)n exp(θ n
Likelihood function
l.exp = function(theta, y) { n = length(y) sumy = sum(y) l = thetaˆn*exp(-sumy*theta) return(l) } Vectorized: can be used to evaluate L(θ) at multiple values ot θ rather than using a loop beta = seq(.00001, .25, length=1000) l.exp(beta, y)
where
∞
c = 1/
0
β n exp(−β
yi )dβ yi )
Looks like a Gamma(a = n + 1, b =
normalized likelihood has same shape has likelihood same form that would be obtained using Bayes Theorem with a “uniform” prior
One Parameter Models – p. 11/2
Gamma Prior/Posterior Distributions
Prior and Posterior distributions are in the same family
β ∼ G(a, b) ba a−1 −βb n −β P yi p(β | Y ) ∝ β e β e Γ(a) ∝ β a+n−1 e−β(b+ β | Y ∼ G(a + n, b +
One Parameter Models – p. 5/2
Plot of Likelihood
Exponential Likelihood
2.5e−20 L(β) 0.0e+00 0.00 5.0e−21 1.0e−20 1.5e−20 2.0e−20
0.05
0.10 β
0.15
0.20
0.25
One Parameter Models – p. 6/2
One Parameter Models – p. 3/2
Exponential Example: Rate β
The time between accidents modeled with an exponential distribution with a rate of β accidents per day. n = 10 observations: 1.5 15.0 60.3 30.5 2.8 56.4 27.0 6.4 110.7 25.4
Posterior Quantities
posterior distribution G(10, 336)
ˆ posterior mode β = 0.0268
posterior mean E(β|Y ) = 10/336 = 0.0298 Interval Estimates – Probability Intervals or Credible Regions Highest Posterior Density region [0.012, 0.049] Equal Tail area [0.014, 0.051] After observing the data, we believe there is a 95% chance of [0.01, 0.05] accidents per day (or 1 to 5 accidents per every 100 days)
One Parameter Models – p. 13/2
Highest Posterior Density Region
95% Highest Posterior Density [0.012, 0.05]