数学专业外文翻译--多元函数的极值
多元函数的Taylor公式与极值问题课件

实际应用中的考虑因素
实际问题的背景
在应用极值理论时,需要考虑实际问题的背景和限制条件,如物 理定律、约束条件等。
数据的不确定性
在实际问题中,数据往往存在不确定性,需要考虑这些不确定性 对极值分析的影响。
模型的适用性
在应用极值理论时,需要考虑模型的适用性,确保模型能够准确 地反映实际情况。
07
与望
05
利用Taylor公式求解极
方法概述
定义
Taylor公式是用于近似表达一 个多元函数在某点附近的行 为
的公式。
形式
Taylor公式的一般形式为 f(x)≈f(a)+f'(a)(x−a)+12f''(a) (x−a)2+…+1n!f(n)(a)(x−a)n
+…。
应用
利用Taylor公式,我们可以找 到函数在某点的极值。
06
极求解的注事与 技巧
常见错误分析
忽视函数的定义域
在求解极值问题时,必须先确定函数的定义域,否 则可能导致错误的结论。
对导数的理解不足
导数描述了函数在某一点的切线斜率,若对导数的 理解不准确,可能导致错误的极值点判断。
未考虑多极值点的情况
在某些情况下,函数可能有多个极值点,需要全面 考虑,避免遗漏。
定义
一元函数在某点的Taylor公式是 该函数在该点附近的一个多项式 近似表示。
形式
一元函数的Taylor公式的一般形 式为 f(x) = f(a) + f'(a)(x-a) + f''(a)(x-a)^2/2! + ... + f^(n)(a)(x -a)^n/n! + Rn(x)
数学专业外文翻译--多元函数的极值
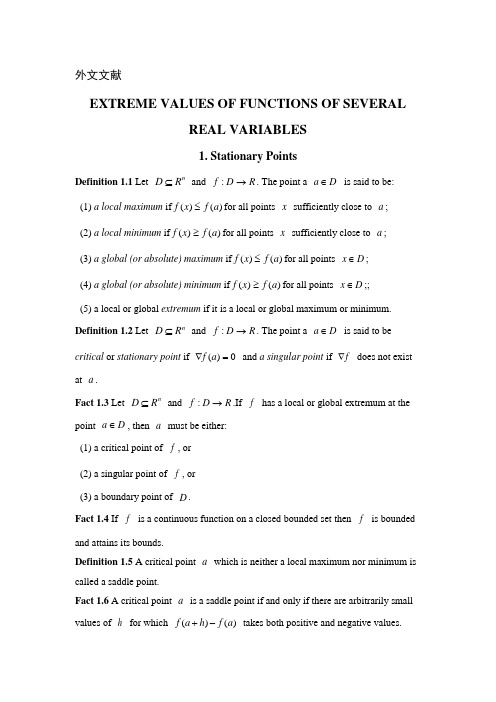
外文文献EXTREME VALUES OF FUNCTIONS OF SEVERALREAL VARIABLES1. Stationary PointsDefinition 1.1 Let n R D ⊆ and R D f →:. The point a D a ∈ is said to be:(1) a local maximum if )()(a f x f ≤for all points x sufficiently close to a ;(2) a local minimum if )()(a f x f ≥for all points x sufficiently close to a ;(3) a global (or absolute) maximum if )()(a f x f ≤for all points D x ∈;(4) a global (or absolute) minimum if )()(a f x f ≥for all points D x ∈;;(5) a local or global extremum if it is a local or global maximum or minimum. Definition 1.2 Let n R D ⊆ and R D f →:. The point a D a ∈ is said to be critical or stationary point if 0)(=∇a f and a singular point if f ∇ does not exist at a .Fact 1.3 Let n R D ⊆ and R D f →:.If f has a local or global extremum at the point D a ∈, then a must be either:(1) a critical point of f , or(2) a singular point of f , or(3) a boundary point of D .Fact 1.4 If f is a continuous function on a closed bounded set then f is bounded and attains its bounds.Definition 1.5 A critical point a which is neither a local maximum nor minimum is called a saddle point.Fact 1.6 A critical point a is a saddle point if and only if there are arbitrarily small values of h for which )()(a f h a f -+ takes both positive and negative values.Definition 1.7 If R R f →2: is a function of two variables such that all second order partial derivatives exist at the point ),(b a , then the Hessian matrix of f at ),(b a is the matrix⎪⎪⎭⎫ ⎝⎛=yy yxxy xx f f f f H where the derivatives are evaluated at ),(b a . If R R f →3: is a function of three variables such that all second order partial derivatives exist at the point ),,(c b a , then the Hessian of f at ),,(c b a is the matrix⎪⎪⎪⎭⎫ ⎝⎛=zz zy zx yz yy yx xz xy xx f f f f f f f f f H where the derivatives are evaluated at ),,(c b a .Definition 1.8 Let A be an n n ⨯ matrix and, for each n r ≤≤1,let r A be the r r ⨯ matrix formed from the first r rows and r columns of A .The determinants det(r A ),n r ≤≤1,are called the leading minors of ATheorem 1.9(The Leading Minor Test). Suppose that R R f →2:is a sufficiently smooth function of two variables with a critical point at ),(b a and H the Hessian of f at ),(b a .If 0)det(≠H , then ),(b a is:(1) a local maximum if 0>det(H 1) = f xx and 0<det(H )=2xy yy xx f f f -;(2) a local minimum if 0<det(H 1) = f xx and 0<det(H )=2xy yy xx f f f -;(3) a saddle point if neither of the above hold.where the partial derivatives are evaluated at ),(b a .Suppose that R R f →3: is a sufficiently smooth function of three variables with a critical point at ),,(c b a and Hessian H at ),,(c b a .If 0)det(≠H , then ),,(c b a is:(1) a local maximum if 0>det(H 1), 0<det(H 2) and 0>det(H 3);(2) a local minimum if 0<det(H 1), 0<det(H 2) and 0>det(H 3);(3) a saddle point if neither of the above hold.where the partial derivatives are evaluated at ),,(c b a .In each case, if det(H )= 0, then ),(b a can be either a local extremum or a saddleExample. Find and classify the stationary points of the following functions:(1) ;1),,(2224+++++=xz z y y x x z y x f(2) ;)1()1(),(422++++=x y x y y x fSolution. (1) 1),,(2224+++++=xz z y y x x z y x f ,so)24),(3z xy x y x f ++=∇(i )2(2y x ++j )2(x z ++kCritical points occur when 0=∇f ,i.e. when(1) z xy x ++=2403(2) y x 202+=(3) x z +=20Using equations (2) and (3) to eliminate y and z from (1), we see that 021433=--x x x or 0)16(2=-x x ,giving 0=x ,66=x and 66-=x .Hence we have three stationary points: )(0,0,0,)(126,121,66-- and )(126,121,66--. Since y x f xx 2122+=,x f xy 2=,1=xz f ,2=yy f ,0=yz f and 2=zz f ,the Hessian matrix is⎪⎪⎪⎭⎫ ⎝⎛+=201022122122x x y x H At )(126,121,66--, ⎪⎪⎪⎪⎭⎫ ⎝⎛=201023/613/66/11H which has leading minors 611>0, 039631123/63/66/11det >=-=⎪⎪⎭⎫ ⎝⎛ And det 042912322>=--=H .By the Leading Minor Test, then, )(126,121,66--is a local minimum. At )(126,121,66--, ⎪⎪⎪⎪⎭⎫ ⎝⎛--=201023/613/66/11H which has leading minors 611>0,039631123/63/66/11det >=-=⎪⎪⎭⎫ ⎝⎛ And det 042912322>=--=H .By the Leading Minor Test, then, )(126,121,66--is also a local minimum. At )(0,0,0, the Hessian is⎪⎪⎪⎭⎫ ⎝⎛=201020100HSince det 2)(-=H , we can apply the leading minor test which tells us that this is a saddle point since the first leading minor is 0. An alternative method is as follows. In this case we consider the value of the expressionhl l k k h h l k h f f D ++++=+++-=22240,0,00,0,0)()(,for arbitrarily small values of h, k and l. But for very small h, k and l , cubic terms and above are negligible in comparison to quadratic and linear terms, sothat hl l k D ++≈22.If h, k and l are all positive, 0>D . However, if 0=k and 0<h and h l <<0,then 0<D .Hence close to )(0,0,0,f both increases and decreases, so )(0,0,0 is a saddle point.(2) 422)1()1(),(++++=x y x y y x f so))1(4)1(2(),(3+++=∇x y x y x f i ))1(2(2+++x y j .Stationary points occur when 0=∇f ,i.e. at )0,1(-.Let us classify this stationary point without considering the Leading Minor Test (in this case the Hessian has determinant 0 at )0,1(- so the test is not applicable). Let.0,10,1422h k h k k h f f D ++=++---=)()(Completing the square we see that .43)2(222h h k D ++=So for any arbitrarily small values of h and k , that are not both 0, 0>D and we see that f has a local maximum at )0,1(-.2. Constrained Extrema and Lagrange MultipliersDefinition 2.1 Let f and g be functions of n variables. An extreme value of f (x )subject to the condition g (x) = 0, is called a constrained extreme value and g (x ) = 0 is called the constraint.Definition 2.2 If R R f n →: is a function of n variables, the Lagrangian function of f subject to the constraint 0),,,(21=n x x x g is the function of n+1 variables),,,,(),,,(),,,,(212121n n n x x x g x x x f x x x L λλ+=where is known as the Lagrange multiplier.The Lagrangian function of f subject to the k constraints0),,,(21=n i x x x g ,k i ≤≤1, is the function with k Lagrange multipliers,i λk i ≤≤1,∑=+=ki n n n x x x g x x x f x x x L 1212121),,,(),,,(),,,,( λλTheorem 2.3 Let R R f →2: and ),(00y x P = be a point on the curve C, withequation g(x,y) = 0, at which f restricted to C has a local extremum.Suppose that both f and g have continuous partial derivatives near to P and that P is not an end point of C and that 0),(00≠∇y x g . Then there is some λ such that ),,(000z y x is a critical point of the Lagrangian Function),(),(),,(y x g y x f y x L λλ+=.Proof. Sketch only. Since P is not an end point and 0≠∇g ,C has a tangent at P with normal g ∇.If f ∇ is not parallel to g ∇at P , then it has non-zero projection along this tangent at P .But then f increases and decreases away from P along C ,so P is not an extremum. Hence f ∇and g ∇are parallel and there is some¸such that g f ∇-=∇λ and the result follows.Example. Find the rectangular box with the largest volume that fits inside the ellipsoid 1222222=++cz b y a x ,given that it sides are parallel to the axes. Solution. Clearly the box will have the greatest volume if each of its corners touch the ellipse. Let one corner of the box be corner (x, y, z) in the positive octant, then the box has corners (±x,±y,±z) and its volume is V= 8xyz .We want to maximize V given that 01222222=-++cz b y a x . (Note that since the constraint surface is bounded a max/min does exist). The Lagrangian is⎪⎪⎭⎫ ⎝⎛-+++=18),,,(222222c z b y a x xyz z y x L λλ and this has critical points when 0=∇L , i.e. when,.280,28022b y zx y L a x yz x L λλ+=∂∂=+=∂∂=⎪⎪⎭⎫ ⎝⎛-++=∂∂=+=∂∂=10,2802222222c z b y a x z L c z xy z L λ (Note that λL will always be the constraint equation.) As we want to maximize V we can assume that 0≠xyz so that 0,,≠z y x .)Hence, eliminating λ, we get,444222zxy c y zx b x yz a -=-=-=λ so that 2222b x a y = and .2222c y b z =But then 222222c z b y a x ==so 2222222231ax c z b y a x =++= or 3a x =,which implies that 3b y = and 3c z = (they are all positive by assumption). So L has only one stationary point ),3,3,3(λc b a (for some value of λ, which we could work out if we wanted to). Since it is the only stationary point it must the required max and the max volume is3383338abc c b a =.中文译文 多元函数的极值1. 稳定点定义1.1 使n R D ⊆并且R D f →:. 对于任意一点D a ∈有以下定义:(1)如果)()(a f x f ≤对于所有x 充分地接近a 时,则)(a f 是一个局部极大值;(2)如果)()(a f x f ≥对于所有x 充分地接近a 时,则)(a f 是一个局部极小值;(3)如果)()(a f x f ≤对于所有点D x ∈成立,则)(a f 是一个全局极大值(或绝对极大值);(4) 如果)()(a f x f ≥对于所有点D x ∈成立,则)(a f 是一个全局极小值(或绝对极小值); (5) 局部极大(小)值统称为局部极值;全局极大(小)值统称为全局极值.定义 1.2 使n R D ⊆并且R D f →:.对于任意一点D a ∈,如果0)(=∇a f ,并且对于任意奇异点a 都不存在f ∇,则称a 是一个关键点或稳定点.结论 1.3 使n R D ⊆并且R D f →:.如果f 有局部极值或全局极值对于一点D a ∈, 则a 一定是:(1)函数f 的一个关键点, 或者(2)函数f 的一个奇异点, 或者(3)定义域D 的一个边界点.结论 1.4 如果函数f 是一个在闭区间上的连续函数,则f 在区间上有边界并且可以取到边界值.定义 1.5 对于任一个关键点a ,当a 既不是局部极大值也不是局部极小值时,a 叫做函数的鞍点.结论 1.6 对于一个关键点a 是鞍点当且仅当h 任意小时,对于函数)()(a f h a f -+取正值和负值.定义 1.7 如果R R f →2: 是二元函数,并且在点),(b a 处所有二阶偏导数都存在,则则根据函数f 在点),(b a 处导数,有f 在点),(b a 处的Hessian 矩阵为:⎪⎪⎭⎫ ⎝⎛=yy yx xy xxf ff f H . 推广:如果R R f →3: 是三元函数,并且在点),,(c b a 处所有二阶偏导数都存在,则根据函数f 在点),,(c b a 处导数,有f 在点),,(c b a 处的Hessian 矩阵为:⎪⎪⎪⎭⎫ ⎝⎛=zz zyzxyz yy yxxz xy xxf f f f f f f f f H . 定义 1.8 矩阵A 是n n ⨯ 阶矩阵,并且对于每一个都有n r ≤≤1,从矩阵A 中选取左上端的r 行和r 列,令其为r r ⨯阶的矩阵r A .则行列式det(r A ),n r ≤≤1,叫做矩阵A 的顺序主子式.定理 1.9 假如R R f →2:是一个充分光滑的二元函数,且在点),(b a 处稳定,其Hessian 矩阵为H .如果0)det(≠H ,则根据偏导数判定),(b a 点是:(1) 一个局部极大值点, 如果0>det(H 1) = f xx 并且0<det(H )=2xy yy xx f f f -; (2) 一个局部极小值点, 如果0<det(H 1) = f xx 并且0<det(H )=2xy yy xx f f f -;(3) 一个鞍点,如果点),(b a 既不是局部极大值点也不是局部极小值点. 假如R R f →3:是一个充分光滑的三元函数,且在点),,(c b a 处稳定,其Hessian 矩阵为H .如果0)det(≠H ,则根据偏导数判定),,(c b a 点是: (1) 一个局部极大值点, 如果当0>det(H 1), 0<det(H 2) 并且 0>det(H 3)时; (2) 一个局部极小值点, 如果当0<det(H 1), 0<det(H 2) 并且 0>det(H 3)时; (3) 一个鞍点,如果点),,(c b a 既不是局部极大值点也不是局部极小值点. 在不同的情况下 ,当det(H )= 0时, 点),(b a 是一个局部极值点,或者是一个鞍点.例. 确定下列函数的稳定点并说明是哪一类点: (1) ;1),,(2224+++++=xz z y y x x z y x f (2) ;)1()1(),(422++++=x y x y y x f 解. (1) 1),,(2224+++++=xz z y y x x z y x f ,so)24),(3z xy x y x f ++=∇(i )2(2y x ++j )2(x z ++k当0=∇f 时有稳定点,也就是说, 当(1) z xy x ++=2403 (2) y x 202+= (3) x z +=20时,将方程(2)和方程(3)带入到方程(1)可以消去变量y 和z, 由此可以得到021433=--x x x 即0)16(2=-x x ,得0=x ,66=x 和66-=x .因此我们可以得到函数的三个稳定点:)(0,0,0,)(126,121,66--和)(126,121,66--. 又因为y x f xx 2122+=,x f xy 2=,1=xz f ,2=yy f ,0=yz f 和2=zz f ,则Hessian 矩阵为⎪⎪⎪⎭⎫⎝⎛+=201022122122x x y x H在点)(126,121,66--处, ⎪⎪⎪⎪⎭⎫⎝⎛=201023/613/66/11H则顺序主子式611>0, 039631123/63/66/11>=-=并且行列式042912322>=--=H .根据主子式判定方法,则点)(126,121,66--是一个局部极小值点.在点)(126,121,66--处, ⎪⎪⎪⎪⎭⎫ ⎝⎛--=201023/613/66/11H则顺序主子式 611>0,039631123/63/66/11>=-=-- 并且行列式042912322>=--=H .根据主子式判定方法,则点)(126,121,66--也是一个极小值点.在点)(0,0,0处,Hessian 矩阵为⎪⎪⎪⎭⎫⎝⎛=201020100H因此det 2)(-=H ,根据主子式判定方法,第一主子式为0,由此我们可以知道该点是一个鞍点. 下面是另一种计算方法,在这种情况下,我们考虑现在下面函数表达式hl l k k h h l k h f f D ++++=+++-=22240,0,00,0,0)()(,的值,对于任意h, k 和l 无限小时. 担当h, k 和l 非常小时, 三次及三次以上方程相对线性二次方程时可忽略不计,则原方程可为hl l k D ++≈22.当h, k 和l 都为正时,0>D .然而, 当0=k 、0<h 和h l <<0,则0<D .因此当接近)(0,0,0时,f 同时增加或者同时减少, 所以 )(0,0,0是一个鞍点. (2) 422)1()1(),(++++=x y x y y x f so))1(4)1(2(),(3+++=∇x y x y x f i ))1(2(2+++x y j .当0=∇f 时有稳定点,也就是说, 当在)0,1(-时.现在我们在不考虑主子式判定方法的情况下为该稳定点进行分类(因为在)0,1(-时Hessian 矩阵的行列式为0,所以该判定方法在此刻无法应用).令.0,10,1422h k h k k h f f D ++=++---=)()(配成完全平方的形式为.43)2(222h h k D ++=所以对h 和k 为任意小时(h 和k 都不为0),有0>D ,因此我们可以确定函数f 在点)0,1(-处有局部极大值.2. 条件极值和Lagrange 乘数法定义 2.1 函数f 和函数g 都是n 元函数.对于限制在条件g (x) = 0下的函数f (x )的极值叫做函数的条件极值,函数g (x ) = 0叫做限制条件.定义 2.2 如果函数R R f n →: 是一个n 元函数, 则对应于函数f 的Lagrange 函数在限制条件0),,,(21=n x x x g 下的函数是一个n +1元函数),,,,(),,,(),,,,(212121n n n x x x g x x x f x x x L λλ+=这就是著名的Lagrange 乘数法.对应于函数f 的Lagrange 函数在k 个限制条件0),,,(21=n i x x x g ,k i ≤≤1时, 带有k 个i λk i ≤≤1,的Lagrange 函数为:∑+=kn n n x x x g x x x f x x x L 212121),,,(),,,(),,,,( λλ定理 2.3 使R R f →2:并且),(00y x P =是曲线C 上的一个点, 有方程 g(x,y) = 0成立,则在限制条件C 上函数f 有局部极值.假设函数f 和函数g 在点P 都有连续的偏导数,点P 不是曲线C 的端点,且0),(00≠∇y x g . 因此存在λ的值使得点),,(000z y x 是Lagrange 函数的关键点),(),(),,(y x g y x f y x L λλ+=.证明.仅仅描述. 因为点P 不是曲线C 的端点,且0≠∇g ,则曲线C 在点P 处的切线与g ∇有关.如果f ∇在点P 处与g ∇平行,则函数在点P 处的切线有非零值.但另一方面函数 f 的值随着P 在C 的运动增加减小,所以点P 不是极值点. 因为f ∇和g ∇平行,所以存在λ使得g f ∇-=∇λ成立.例. 求内接于椭球1222222=++cz b y a x 的体积最大的长方体的体积,长方体的各个面平行于坐标面解:明显地,当长方体的体积最大时,长方体的各个顶点一定在椭球上. 设长方体的一个顶点坐标为(x, y, z) (x>0, y>0, z>0), 则长方体的其他顶点坐标分别为(±x,±y,±z),并且长方体的体积为V= 8xyz.我们要求V 在条件01222222=-++cz b y a x 下的最大值. (注意:因为约束条件是有边界的,故其一定存在极大或者极小值). 其Lagrange 函数为⎪⎪⎭⎫⎝⎛-+++=18),,,(222222c z b y a x xyz z y x L λλ并且存在稳定点当0=∇L 时,也就是说,当,.280,280,280222cz xy z L b yzx y L a x yz x L λλλ+=∂∂=+=∂∂=+=∂∂=⎪⎪⎭⎫ ⎝⎛-++=∂∂=10222222c z b y a x z L 时.(注意:λL 是约束方程.要想求得体积V 的最大值,假设0≠xyz ,则可得0,,≠z y x .)因此, 用其他式子表示λ, 我们可以得到,444222zxyc y zx b x yz a -=-=-=λ 消去λ,有2222b x a y =和.2222c y b z =进而得出 222222cz b y a x ==,因此有2222222231ax c z b y a x =++=或者得出3a x =,同理可得出3by =和3c z = (根据假设可得x, y, z 都是正值).所以函数 L 有且仅有一个稳定点),3,3,3(λc b a (λ为某一计算可得到的常数). 又因为该点是函数L 的唯一稳定点,则该稳定点一定是所要求的最大值点,故其体积的最大值为3383338abcc b a =.。
数学分析 多元函数的极值
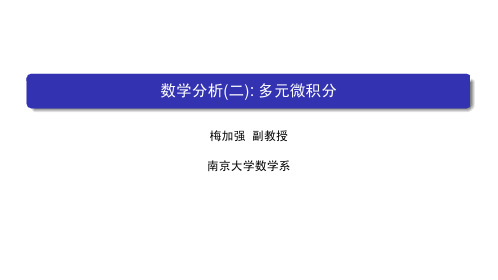
|α|=m+1
Taylor 公式
(Taylor 公式) 设 D ⊂ Rn 为凸域, f ∈ Cm+1(D), x0 ∈ D. 则任给 x ∈ D, 存在 θ ∈ (0, 1) 使得
m
f (x) =
Dαf
(x 0) (x
−
x 0)α
+
Dαf
(x 0
+
θ(x
−
x 0)) (x
−
x 0)α.
α!
α!
k=0 |α|=k
(最小二乘法) 设 (x1, y1), · · · , (xn, yn) 为平面 R2 上 n 个点, 求一条直线 y = ax + b, 使得
n
F (a, b) = (axi + b − yi )2 最小.
i =1
最小二乘法
(最小二乘法)
设 (x1, y1), · · · , (xn, yn) 为平面 R2 上 n 个点, 求一条直线 y = ax + b, 使得
凸函数
设 D ⊂ Rn 为凸域, f : D → R 为多元函数. 如果任给 x = y ∈ D, 均有 f (tx + (1 − t)y ) ≤ tf (x) + (1 − t)f (y ), ∀ t ∈ (0, 1),
则称 f 为 D 中的凸函数. 从定义容易看出, 多元函数为凸函数当且仅当它在每一条直线上的限制是一元 凸函数. 如果 f ∈ C2(D), 则根据之前二阶导数的计算可知, f 为凸函数当且仅当 ∇2f ≥ 0. 如果 f 可微, 则也不难证明 f 为凸函数当且仅当
f (y) ≥ f (x) + ∇f (x) · (y − x), ∀ x, y ∈ D.
8.8多元函数的极值

Lx Ly 0 驻点(0.75,1.25)
L=39.25
(注意此例不能肯定最大值在定义域内部达到)
需要讨论R在边界y = 0和x =0的情况
y=0上 289/8 x=0上 1561/40
乐经良
例 某种金属棒的长度随着温度变化,现 测得一组数据如下表
t (℃) l(mm)
20 1000.36
30 1000.53
i1
的最小值确定f 中的待定常数
最小二乘法
乐经良
设 l a bt ,那么引进
驻点满足
5
Q(a, b) (li (a bti )) 2 i 1
Q
5
a
2 (li
i 1
a bti ) 0
Q
5
b
2 (li
i1
a bti )ti
0
从中可解出a,b的值,从而得到函数 l a bt
Chap8 ―8
多元函数的极值
上海交大乐经良
8.8.1 二元函数的Taylor公式
函数f(x,y)在P0(x0,y0)的邻域有n+1阶连续偏导数 设函数
F (t) f (x0 tx, y0 ty) t [0, 1]
将此函数用Taylor公式展开且取t=1,得到
f
(x0
x, y0
y)
n k 0
二. 极值的必要条件
f(x,y)在P0(x0,y0)处取得极值,且f 可微,则
f x (x0 , y0 ) f y (x0 , y0 ) 0
(满足此式的 点称驻点)
乐经良
注意 驻点未必是极值点 例 考察函数 f (x, y) x在y (0,0)的情况
三. 极值的充分条件
函数在点P0(x0,y0)的邻域内有连续的二阶 偏导数, f x (x0 , y0 ) f y (x0 , y0 ) 0, 记
多元函数的极值与条件极值

多元函数的极值与条件极值在数学中,多元函数是指有多个自变量的函数。
研究多元函数的极值和条件极值可以帮助我们找到函数的最大值和最小值,在各种实际问题中具有广泛的应用。
本文将介绍多元函数的极值和条件极值的概念、判别法以及求解方法,以深入探讨这一重要数学概念。
一、多元函数的极值多元函数的极值指的是函数在定义域内取得的最大值和最小值。
对于具有两个自变量的函数,通常使用偏导数的概念来进行讨论。
偏导数是指将函数对于某一个自变量求导时,将其他自变量看作常数,得到的导数。
考虑一个具有两个自变量的多元函数 f(x, y),其中 x 和 y 是定义域内的变量。
函数 f(x, y) 的极值点可以通过以下步骤确定:1. 求出函数 f(x, y) 的偏导数,即 f 对于 x 的偏导数∂f/∂x 和 f 对于 y 的偏导数∂f/∂y;2. 解方程组∂f/∂x = 0 和∂f/∂y = 0,得到可能的极值点;3. 使用二阶偏导数的判别法判断极值的类型。
当二阶偏导数的行列式D = ∂²f/∂x² * ∂²f/∂y² - (∂²f/∂x∂y)² 大于 0 时,判断该点为极值点,否则不是。
二、多元函数的条件极值条件极值是指多元函数在满足一定条件下取得的极值。
通常在实际问题中,函数的自变量受到一定的限制条件约束。
此时,我们需要使用拉格朗日乘子法来求解条件极值。
假设有一个多元函数 f(x, y) 和一个条件方程 g(x, y) = 0。
使用拉格朗日乘子法求解条件极值的步骤如下:1. 构造拉格朗日函数L(x, y, λ) = f(x, y) + λg(x, y),其中λ 是拉格朗日乘子;2. 求出 L 对于 x、y 和λ 的偏导数∂L/∂x,∂L/∂y 和∂L/∂λ;3. 解方程组∂L/∂x = 0,∂L/∂y = 0 和∂L/∂λ = 0,得到可能的条件极值点;4. 使用二阶偏导数的判别法判断极值的类型。
多元函数的Taylor公式与极值

矩阵为: H f ( x0 )
f11 f21
f12
f
22
f1n
f
2n
设u f (P)在点
f
n1
f
n
2
f
nn
x0
P0 ( x01 , x02 , , x0n ) 的所有二阶偏导数
f11
f12
f1n
f 在点P0的 Hessian
H
f
(P0 )
f21
f
22
f
2n
矩阵为:
f
n1
f
x
, y)2
3 f
2! ,
x py3 p (1 x y)3
( p 0,1,2,3),
4 f 3! , ( p 0,1,2,3,4), x py4 p (1 x y)4
20
x x
y y
f
(0,0)
xfx (0,0)
yf y (0,0)
x
y,
x
y
2
f
(0,0)
x y
由假设, (t) 在 [0,1] 上满足一元函数泰勒公式的条件
于是有 (1) (0) (0) (0)
1!
2!
(n) (0) (n1) ( )
(0
1) .
n!
(n 1)!
(0) f ( x0 , y0 ) , (1) f ( x0 h , y0 k)
利用多元复合函数求导法则可得:
11
(4)在泰勒公式中,如果取 x0 0, y0 0,则 成为n阶麦克劳林(Maclaurin)公式.
f (x, y)
f
(0,0)
x
x
y
多元函数极值问题论文

本科生毕业论文题目:多元函数的极值问题Extremum problem of function of several variables学生:学号:系别:数学与应用数学专业:数学与应用数学指导教师:起止日期:2014 年 5 月 10 日本科毕业论文(设计)诚信声明作者重声明:所呈交的本科毕业论文(设计),是在指导老师的指导下,独立进行研究所取得的成果,成果不存在知识产权争议。
除文中已经注明引用的容外,论文不含任何其他个人或集体已经发表或撰写过的成果。
对论文的研究做出重要贡献的个人和集体均已在文中以明确的方式标明。
本声明的法律结果由作者承担。
本科毕业论文(设计)作者签名:年月日目录多元函数的极值问题 (I)摘要 (I)关键词 (I)Key words (I)1 引言 (1)2 多元函数极值的有关概念 (2)3 多元函数极值的判定方法 (2)3.1无条件极值的判定方法 (2)3.2 条件极值的判定方法 (4)4 无条件极值的求解方法 (5)4.1二元函数极值的求解方法 (5)4.2多元函数极值的求解方法 (6)5 条件极值的求解方法 (8)5.1多元函数极值的求解方法 (8)5.1.1代入消元法 (8)5.1.2拉格朗日乘数法 (8)5.1.3 标准量代换法 (10)5.1.4不等式法 (10)5.1.5 二次方程判别式符号法 (11)5.1.6 梯度法 (12)5.1.7 数形结合法 (13)5.2多元函数条件极值在理论和实际中的应用 (15)5.2.1不等式证明 (15)5.2.2 物理学中光的折射定律证明 (15)5.2.3 生产销售 (16)4.结束语 (18)参考文献 (19)致 (20)多元函数的极值问题摘要在实际问题中,有时会遇到求多元函数的最大值和最小值问题,多元函数的最大值与最小值与极大值、极小值有密切联系.多元函数的极值问题一般涉及到多元函数的极值判定准则、多元函数的极值求法、多元函数极值的应用.尽管有些极值问题用初等的方法可以解决,但是有时显得麻烦,有些极值问题用初等的方法根本无法解决.本文先将一元函数的极值的的判定和求法推广到二元函数,再以二元函数的极值的判定和求法为基础,研究和探讨多元函数的极值的判定和求法;最后来探讨多元函数的极值的应用.关键词函数极值;多元函数;条件极值;拉格朗日乘数法;梯度法Extremum problem of function of several variablesAbstractIn fact, multiple functions of maximum and minimum value are sometimes encountered. But maximum value and minimum value of multivariate function is closely related to the minimum and maximum value. The extreme value of multivariate function generally involves the criterion, methods and application of extreme value of multivariate fun ction. Some extreme value’s questions can be settled with primary methods, which cannot solve all questions. So In this paper the judging and the calculating method of one-variable function is extended into binary function. Then according to the judging and the calculating method of binary function, to research and discuss on the extreme value of multivariate function determination and method; finally to discuss the application of value of multivariate function.Key wordsFunction extreme; multi-function; extreme conditions; Lagrange multiplier method; gradient method.1 引言函数极值问题已广泛地出现于数学、物理、化学等学科中,且它涉及的知识面非常广,所以就要求学生有较高的分析能力和逻辑推理能力,同时也要求学生掌握多种求函数极值的方法,因此对函数极值的研究是非常必要的.多元函数条件极值是多元函数微分学的重要组成部分,它不仅在理论上有重要的应用,而且在其它学科及有关实际问题中有着广泛的应用,于是如何判定与求解多元函数条件极值就成为许多学者研究的问题,虽然以前也有不少学者研究过,但多数还只是理论上的研究,实际利用方面的研究较少.一般实际问题都是一个或者一组多元函数,那么研究清楚这些问题,对我们的工程实际将有莫大的裨益.通过对求解多元函数条件极值问题的研究,从中找到多元函数的判定方法和求出极值的不同方法,在不同的实际应用中对相关问题运用与其相适应的方法,从而在解决问题的过程达到最优化。
§5.7多元函数的Taylor公式与极值

∂m f ∂x ∂y
k
( ∆x )k ( ∆y )m−k m− k
( m = 1,2,⋯n + 1 )
定理 1 设二元函数 f 在点 M 0 ( x0 , y 0 )的某邻域 N ( M 0 )内
有 n + 1阶连续偏导数,则 ∀ M ( x0 + ∆ x , y 0 + ∆ y ) ∈ N ( M 0 ), 有 ∂ ∂ f ( x0 + ∆ x , y 0 + ∆ y ) = f ( x0 , y 0 ) + ∆ x ( + ∆ y ) f ( x0 , y 0 ) ∂x ∂y 1 ∂ ∂ 2 + ( ∆x + ∆ y ) f ( x0 , y 0 ) + ⋯ 2! ∂x ∂y 1 ∂ ∂ n + ( ∆x + ∆ y ) f ( x0 , y 0 ) n! ∂x ∂y 1 ∂ ∂ n +1 + ( ∆x + ∆ y ) f ( x0 + θ∆ x , y 0 + θ∆ y ) ( n + 1)! ∂x ∂y 其中 0 < θ < 1
∂f ∂x = f ( x0 , y0 ) + (∆x, ∆y) ∂f ∂y M0
*
∂2 f 2 1 ∂x + (∆x, ∆y) 2 ∂ f 2 ∂x∂y
∂ f ∂x∂y ∆x 2 ∂ f ∆y ∂y 2 M *
z = f ( x, y) = x 2 + y 2 在 ( 0, ) 取得极值 0, 0 但 ( 0, 0)不是驻点,因为在( 0, 0)处f x , f y不存在。
-多元函数的Taylor公式与极值
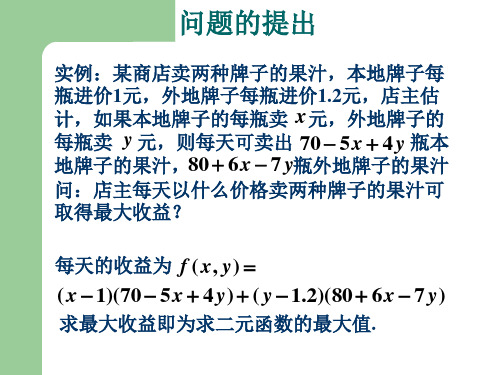
f
y
x
0
②
fz z 0
( x, y,z)0
从方程组②中解出 x , y , z , ,其中(x , y ,z )
即为可能极值点。这种方法叫做拉格朗日乘数法。
方程组②表示了函数 F( x, y,z,) f ( x, y,z) ( x, y,z)
的四个一阶偏导数等于 0:
Fx f x x 0
(2)解方程组
f f
x y
( (
x, x,
y y
)0 )0
,求出一切驻点;
(3)对于每一驻点 ( x , y ) ,求出 A f xx( x , y ) ,
B f xy( x, y ), C f yy( x, y ) 的值;
(4)定出 AC B2 的符号,按定理 7.3 的结论判定 出 f ( x , y ) 是否是极值、是极大值还是极小值。
4x2 1 ,故 x yz 1 。 2
求得驻点 ( 1, 1, 1 ) ,此时相应的距离为 222
d1
1 11 1 2 2 ( )6
6 22 2 3
6;
d2
1 2( 1 )( 1 ) 1 6 4 6 2 22 3
6。
由于驻点只有两个,且最近点与最远点存在,故得
最近点为( 1 , 1 , 1 ) ,最近距离为2 6 ;最远点为
(2) (3)
F 2xy2 yz2xza2 0
(4)
由(1)、(2)、(3)得x y z ,将此代入(4)得
x yz a 。 6
∵在定义域 D{( x, y,z) x0, y0,z0} 内函数只有唯一
的驻点( a , a , a ),而函数V 在 D 内必有最大值, 666
用Mathematica求偏导数与多元函数的极值.docx
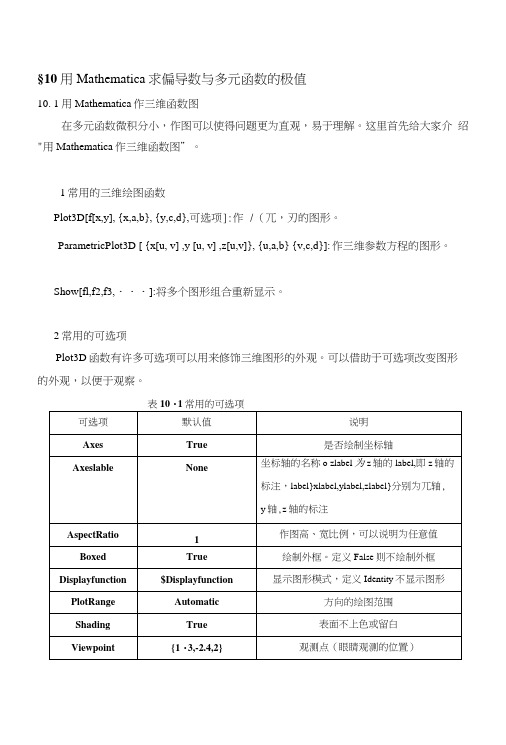
§10用Mathematica求偏导数与多元函数的极值10. 1用Mathematica作三维函数图在多元函数微积分小,作图可以使得问题更为直观,易于理解。
这里首先给大家介绍"用Mathematica作三维函数图”。
1常用的三维绘图函数Plot3D[f[x,y], {x,a,b}, {y,c,d},可选项]:作 /(兀,刃的图形。
ParametricPlot3D [ {x[u, v] ,y [u, v] ,z[u,v]}, {u,a,b} {v,c,d}]: 作三维参数方程的图形。
Show[fl,f2,f3,・・・]:将多个图形组合重新显示。
2常用的可选项Plot3D函数有许多可选项可以用来修饰三维图形的外观。
可以借助于可选项改变图形的外观,以便于观察。
表10・1常用的可选项选择合适的观测点在也有助于观察图形,下面是典型的Viewpoint值: 表10・2典型的VicwPoint值例10.1画出函数Z = sin7x2 + y2图形,并使图形表面不上色。
解In[l]:= Plot3D[Sin[Sqrt[xA2+yTH,{x,0,2Pi},{y,0,2Pi}]Out[l]= -SurfaceGraphics-In[2]:= Show[%,Shading->False]60?226Out[2]= -SurfaceGraphics-例10・2 |Hii岀函数Z二sinxcosy图形,并使调整图形观测点观察图形是否对称。
解In[l]:=Plot3D[Sin[x*y],{x,0,2Pi},{y,0,2Pi},AxesLabel->{“x”,”y”,”z”}]Out[l]= -SurfaceGraphics-In[2]:= Show|%,ViewPoint->{-1,-1,2}]0 0Out[2]= -SurfaceGraphics-例10.3画一单位双曲面。
解首先,写出单位双曲面的参数方程厂x=Cosh[u]*Cos[v]y y=Cosh[u]*Sin[v]< z=uIn[l]:=ParametricPlot3D[{Cosh[uFCo§[v],Cosh[uFSin[v],u},{iiQPi},{v,・Pi,Pi},AxesLabel・>{“x”,”y”,”z”}]Out| 1 ]= -Graphics3D-2 2 2例MU画出函数py話“图形。
多元函数的极值及其应用
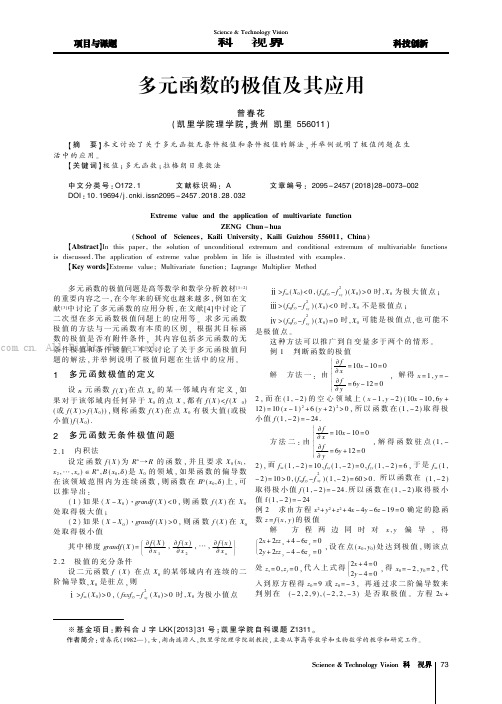
)(-2,2,-3)=
1 36
>0 于
是函数在(-2,2,-3)取得极小值-3.
3 多元函数条件极值问题及其应用
3.1 转化为无条件极值问题 多元函数条件极值问题可以把条件中的某些变量
进行求解袁 再这些代入到目标函数中袁 从而得到无条 件极值问题遥 例如求函数 z=f(x,y)在条件 渍(x,y)=0 下 的可能极值点袁若可由条件 渍(x,y)=0 解出 y=剀(x)袁代 入 z=f(x,y)便化为无条件极值遥
多元函数条件极值问题在很多情形下 将条件极 值化为无条件极值并不容易 需要另一种求条件极值 的专用方法 这就是拉格朗日乘数法 3.2 拉格朗日乘数法
拉格朗日乘数法院要找函数 z =f(x,y)在条件 渍(x, y)=0 下的可能极值点袁可以先构造拉格朗日函数 F(x, y ) = f ( x , y ) + 姿渍 ( x , y ) 袁 其 中 姿 为 拉 格 朗 日 常 数 ?
2 ( y + x ) + 姿xy = 2 袁 xyz = 2 . 得 唯 一 可 能 极 值 点 渊 2 姨 2 , 2
姨2 ,1).根据问题本身的意义及可能极值点的唯一性
可 知 袁 当 水 箱 的 长 尧 宽 各 为 2 姨 2 袁 高 为 1m 时 袁 所 用 的 材料最省遥
揖参考文献铱 咱1暂华东师范大学数学系.数学分析院下册 [M].第 4 版 .北 京 院 高 等 教 育 出 版 社 袁 2014 . 咱2暂同济大学.高等数学院下册[M].第 6 版.北京院高等教育 出 版 社 袁 2007 . 咱3暂 赵 泽 福 . 多 元 函 数 的 应 用 分 析 [J]. 长 春 工 业 大 学 学 报 遥
Science & Technology Vision
多元函数的极值及其应用
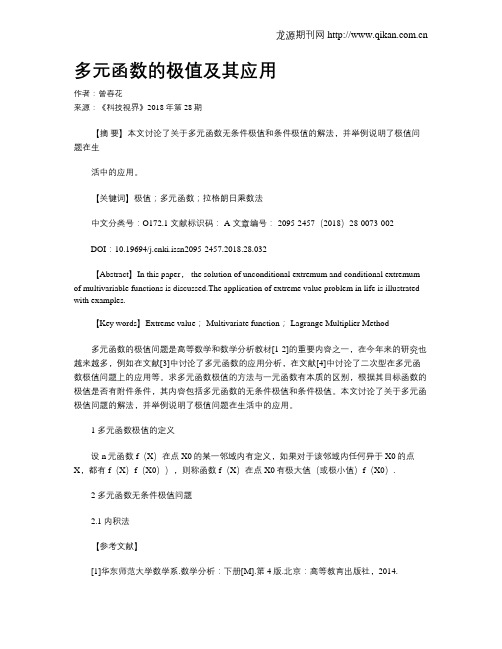
多元函数的极值及其应用作者:曾春花来源:《科技视界》2018年第28期【摘要】本文讨论了关于多元函数无条件极值和条件极值的解法,并举例说明了极值问题在生活中的应用。
【关键词】极值;多元函数;拉格朗日乘数法中文分类号:O172.1 文献标识码: A 文章编号: 2095-2457(2018)28-0073-002DOI:10.19694/ki.issn2095-2457.2018.28.032【Abstract】In this paper, the solution of unconditional extremum and conditional extremum of multivariable functions is discussed.The application of extreme value problem in life is illustrated with examples.【Key words】Extreme value; Multivariate function; Lagrange Multiplier Method多元函数的极值问题是高等数学和数学分析教材[1-2]的重要内容之一,在今年来的研究也越来越多,例如在文献[3]中讨论了多元函数的应用分析,在文献[4]中讨论了二次型在多元函数极值问题上的应用等。
求多元函数极值的方法与一元函数有本质的区别,根据其目标函数的极值是否有附件条件,其内容包括多元函数的无条件极值和条件极值。
本文讨论了关于多元函极值问题的解法,并举例说明了极值问题在生活中的应用。
1 多元函数极值的定义设n元函数f(X)在点X0的某一邻域内有定义,如果对于该邻域内任何异于X0的点X,都有f(X)f(X0)),则称函数f(X)在点X0有极大值(或极小值)f(X0).2 多元函数无条件极值问题2.1 内积法【参考文献】[1]华东师范大学数学系.数学分析:下册[M].第4版.北京:高等教育出版社,2014.[2]同济大学.高等数学:下册[M].第6版.北京:高等教育出版社,2007.[3]赵泽福.多元函数的应用分析[J].长春工业大学学报。
mathematica中二元函数求极值

mathematica中二元函数求极值Title: Calculating Extrema of Bivariate Functions in MathematicaIntroduction:In Mathematica, finding the extrema of bivariate functions can be efficiently accomplished using a combination of builtin functions and techniques. This article aims to provide stepbystep instructions on how to calculate extrema for such functions. We will explore both local and global extrema, discussing the necessary syntax and methodology in Mathematica to obtain accurate results.I. Local Extrema:1. Defining the Bivariate Function:Begin by defining the bivariate function of interest, such as f[x_, y_]:= x^2 + y^2 + 2x + y.2. Finding Critical Points:Utilize the "Grad" function to compute the gradient of the bivariate function, which is necessary for finding critical points.Use the "Solve" or "Reduce" functions to solve for derivatives of x and y to determine the critical points of the function.3. Classifying Critical Points:Find the determinant of the Hessian using the "Det" function.Evaluate the discriminant of the Hessian using the "Simplify" function.Using both determinants, classify the nature of critical points into maximum, minimum, or saddle points.4. Visualizing the Results:Generate a contour plot of the bivariate function using the "ContourPlot" function.Overlay points indicating the critical points obtained in step 2, distinguishing between different types using different colors or shapes.II. Global Extrema:1. Restricting the Domain of the Function:Define the domain over which the function is defined using the "RegionFunction" option in the "Plot3D" function.For example, to analyze the function f[x_, y_]:= x^2 + y^2 + 2x + y only within the range 10 < x < 10 and 10 < y < 10:Plot3D[f[x, y], {x, 10, 10}, {y, 10, 10}, RegionFunction > (10 < 1 < 10 && 10 < 2 < 10 &)]2. Searching for Global Extrema:Utilize the "NMinimize" and "NMaximize" functions to find the global minimum and maximum values by specifying the function and the domain of analysis.For example, to find the global minimum of the function f[x_, y_]:= x^2 + y^2 + 2x + y within the range 10 < x < 10 and 10 < y < 10:NMinimize[{f[x, y], 10 < x < 10 && 10 < y < 10}, {x, y}]3. Visualizing the Results:Generate a 3D plot of the bivariate function using the "Plot3D" function, including the plane representing the global minimum or maximum. Overlay the point(s) representing the global minimum or maximum obtained in step 2.Conclusion:In this article, we have explored the process of finding the extrema of bivariate functions in Mathematica. By following the stepbystep instructions provided, users can confidently calculate both local and global extrema accurately. Understanding and effectively utilizing Mathematica's builtin functions and options such as "Grad," "Solve," "Reduce," "Det," "Simplify," "ContourPlot," "NMinimize," and "NMaximize" enables efficient analysis and visualization of bivariate functions.。
高等数学@9.8 多元函数的极值
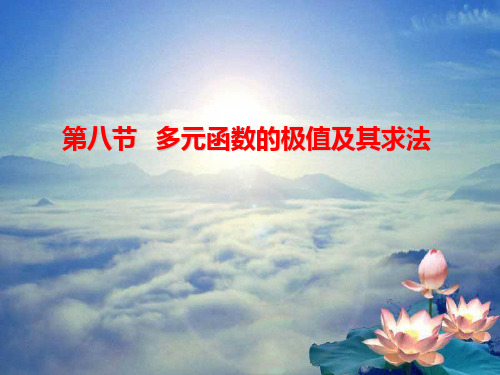
解: 设两边折起 x (cm),折起角为α
断面面积:S 1 (24 2x 24 2x 2x cos )x sin 2
S 24x sin 2x2 sin 1 x2 sin 2 2
Sx S
24sin 4x sin x sin 2 =0 24x cos 2x2 cos x2 cos 2
(1) 求函数z=f(x,y)的极值,其中x,y满足方程φ(x,y)=0。
解 设φ(x,y)=0确定的隐函数为y=y(x),则 dy x
dx y
求驻点: dz f f dy 令 dz 0
dx x y dx
dx
得:
fx
f
y
(
x y
)
0
(1)
设: fy y
在y =0, 0≤ x≤1上: f ( x,0) 3x2 4x
最大f(0,0)=0,最小
f
(
2 3
,0)
4 3
.
在x=1, 0≤y ≤1上: f (1, y) 2 y y2 1 ( y 1)2
最大f(1,1)=0,最小 f(1,0)=-1
驻点函数值: f (1 , 1) 1
故最大值为 umax 63 42 2 6912.
例6 求曲面z=x2+y2与平面x+y-2z=2之间的最短距离
解 设P(x,y,z)为曲面z=x2+y2上任一点
则P到平面x+y-2z=2的距离为:d
1 x y 2z 2. 6
令 F ( x, y, z) ( x y 2z 2)2 (z x2 y2 ),
- 1、下载文档前请自行甄别文档内容的完整性,平台不提供额外的编辑、内容补充、找答案等附加服务。
- 2、"仅部分预览"的文档,不可在线预览部分如存在完整性等问题,可反馈申请退款(可完整预览的文档不适用该条件!)。
- 3、如文档侵犯您的权益,请联系客服反馈,我们会尽快为您处理(人工客服工作时间:9:00-18:30)。
外文文献EXTREME VALUES OF FUNCTIONS OF SEVERALREAL VARIABLES1. Stationary PointsDefinition 1.1 Let n R D ⊆ and R D f →:. The point a D a ∈ is said to be:(1) a local maximum if )()(a f x f ≤for all points x sufficiently close to a ;(2) a local minimum if )()(a f x f ≥for all points x sufficiently close to a ;(3) a global (or absolute) maximum if )()(a f x f ≤for all points D x ∈;(4) a global (or absolute) minimum if )()(a f x f ≥for all points D x ∈;;(5) a local or global extremum if it is a local or global maximum or minimum. Definition 1.2 Let n R D ⊆ and R D f →:. The point a D a ∈ is said to be critical or stationary point if 0)(=∇a f and a singular point if f ∇ does not exist at a .Fact 1.3 Let n R D ⊆ and R D f →:.If f has a local or global extremum at the point D a ∈, then a must be either:(1) a critical point of f , or(2) a singular point of f , or(3) a boundary point of D .Fact 1.4 If f is a continuous function on a closed bounded set then f is bounded and attains its bounds.Definition 1.5 A critical point a which is neither a local maximum nor minimum is called a saddle point.Fact 1.6 A critical point a is a saddle point if and only if there are arbitrarily small values of h for which )()(a f h a f -+ takes both positive and negative values.Definition 1.7 If R R f →2: is a function of two variables such that all second order partial derivatives exist at the point ),(b a , then the Hessian matrix of f at ),(b a is the matrix⎪⎪⎭⎫ ⎝⎛=yy yxxy xx f f f f H where the derivatives are evaluated at ),(b a . If R R f →3: is a function of three variables such that all second order partial derivatives exist at the point ),,(c b a , then the Hessian of f at ),,(c b a is the matrix⎪⎪⎪⎭⎫ ⎝⎛=zz zy zx yz yy yx xz xy xx f f f f f f f f f H where the derivatives are evaluated at ),,(c b a .Definition 1.8 Let A be an n n ⨯ matrix and, for each n r ≤≤1,let r A be the r r ⨯ matrix formed from the first r rows and r columns of A .The determinants det(r A ),n r ≤≤1,are called the leading minors of ATheorem 1.9(The Leading Minor Test). Suppose that R R f →2:is a sufficiently smooth function of two variables with a critical point at ),(b a and H the Hessian of f at ),(b a .If 0)det(≠H , then ),(b a is:(1) a local maximum if 0>det(H 1) = f xx and 0<det(H )=2xy yy xx f f f -;(2) a local minimum if 0<det(H 1) = f xx and 0<det(H )=2xy yy xx f f f -;(3) a saddle point if neither of the above hold.where the partial derivatives are evaluated at ),(b a .Suppose that R R f →3: is a sufficiently smooth function of three variables with a critical point at ),,(c b a and Hessian H at ),,(c b a .If 0)det(≠H , then ),,(c b a is:(1) a local maximum if 0>det(H 1), 0<det(H 2) and 0>det(H 3);(2) a local minimum if 0<det(H 1), 0<det(H 2) and 0>det(H 3);(3) a saddle point if neither of the above hold.where the partial derivatives are evaluated at ),,(c b a .In each case, if det(H )= 0, then ),(b a can be either a local extremum or a saddleExample. Find and classify the stationary points of the following functions:(1) ;1),,(2224+++++=xz z y y x x z y x f(2) ;)1()1(),(422++++=x y x y y x fSolution. (1) 1),,(2224+++++=xz z y y x x z y x f ,so)24),(3z xy x y x f ++=∇(i )2(2y x ++j )2(x z ++kCritical points occur when 0=∇f ,i.e. when(1) z xy x ++=2403(2) y x 202+=(3) x z +=20Using equations (2) and (3) to eliminate y and z from (1), we see that 021433=--x x x or 0)16(2=-x x ,giving 0=x ,66=x and 66-=x .Hence we have three stationary points: )(0,0,0,)(126,121,66-- and )(126,121,66--. Since y x f xx 2122+=,x f xy 2=,1=xz f ,2=yy f ,0=yz f and 2=zz f ,the Hessian matrix is⎪⎪⎪⎭⎫ ⎝⎛+=201022122122x x y x H At )(126,121,66--, ⎪⎪⎪⎪⎭⎫ ⎝⎛=201023/613/66/11H which has leading minors 611>0, 039631123/63/66/11det >=-=⎪⎪⎭⎫ ⎝⎛ And det 042912322>=--=H .By the Leading Minor Test, then, )(126,121,66--is a local minimum. At )(126,121,66--, ⎪⎪⎪⎪⎭⎫ ⎝⎛--=201023/613/66/11H which has leading minors 611>0,039631123/63/66/11det >=-=⎪⎪⎭⎫ ⎝⎛ And det 042912322>=--=H .By the Leading Minor Test, then, )(126,121,66--is also a local minimum. At )(0,0,0, the Hessian is⎪⎪⎪⎭⎫ ⎝⎛=201020100HSince det 2)(-=H , we can apply the leading minor test which tells us that this is a saddle point since the first leading minor is 0. An alternative method is as follows. In this case we consider the value of the expressionhl l k k h h l k h f f D ++++=+++-=22240,0,00,0,0)()(,for arbitrarily small values of h, k and l. But for very small h, k and l , cubic terms and above are negligible in comparison to quadratic and linear terms, sothat hl l k D ++≈22.If h, k and l are all positive, 0>D . However, if 0=k and0<h and h l <<0,then 0<D .Hence close to )(0,0,0,f both increases and decreases, so )(0,0,0 is a saddle point.(2) 422)1()1(),(++++=x y x y y x f so))1(4)1(2(),(3+++=∇x y x y x f i ))1(2(2+++x y j .Stationary points occur when 0=∇f ,i.e. at )0,1(-.Let us classify this stationary point without considering the Leading Minor Test (in this case the Hessian has determinant 0 at )0,1(- so the test is not applicable). Let.0,10,1422h k h k k h f f D ++=++---=)()(Completing the square we see that .43)2(222h h k D ++=So for any arbitrarily small values of h and k , that are not both 0, 0>D and we see that f has a local maximum at )0,1(-.2. Constrained Extrema and Lagrange MultipliersDefinition 2.1 Let f and g be functions of n variables. An extreme value of f (x )subject to the condition g (x) = 0, is called a constrained extreme value and g (x ) = 0 is called the constraint.Definition 2.2 If R R f n →: is a function of n variables, the Lagrangian function of f subject to the constraint 0),,,(21=n x x x g is the function of n+1 variables),,,,(),,,(),,,,(212121n n n x x x g x x x f x x x L λλ+=where is known as the Lagrange multiplier.The Lagrangian function of f subject to the k constraints0),,,(21=n i x x x g ,k i ≤≤1, is the function with k Lagrange multipliers,i λk i ≤≤1,∑=+=ki n n n x x x g x x x f x x x L 1212121),,,(),,,(),,,,( λλTheorem 2.3 Let R R f →2: and ),(00y x P = be a point on the curve C, withequation g(x,y) = 0, at which f restricted to C has a local extremum.Suppose that both f and g have continuous partial derivatives near to P and that P is not an end point of C and that 0),(00≠∇y x g . Then there is some λ such that ),,(000z y x is a critical point of the Lagrangian Function),(),(),,(y x g y x f y x L λλ+=.Proof. Sketch only. Since P is not an end point and 0≠∇g ,C has a tangent at P with normal g ∇.If f ∇ is not parallel to g ∇at P , then it has non-zero projection along this tangent at P .But then f increases and decreases away from P along C ,so P is not an extremum. Hence f ∇and g ∇are parallel and there is some¸such that g f ∇-=∇λ and the result follows.Example. Find the rectangular box with the largest volume that fits inside the ellipsoid 1222222=++cz b y a x ,given that it sides are parallel to the axes. Solution. Clearly the box will have the greatest volume if each of its corners touch the ellipse. Let one corner of the box be corner (x, y, z) in the positive octant, then the box has corners (±x,±y,±z) and its volume is V= 8xyz .We want to maximize V given that 01222222=-++cz b y a x . (Note that since the constraint surface is bounded a max/min does exist). The Lagrangian is⎪⎪⎭⎫ ⎝⎛-+++=18),,,(222222c z b y a x xyz z y x L λλ and this has critical points when 0=∇L , i.e. when,.280,28022b y zx y L a x yz x L λλ+=∂∂=+=∂∂=⎪⎪⎭⎫ ⎝⎛-++=∂∂=+=∂∂=10,2802222222c z b y a x z L c z xy z L λ (Note that λL will always be the constraint equation.) As we want to maximize V we can assume that 0≠xyz so that 0,,≠z y x .)Hence, eliminating λ, we get,444222zxy c y zx b x yz a -=-=-=λ so that 2222b x a y = and .2222c y b z =But then 222222c z b y a x ==so 2222222231ax c z b y a x =++= or 3a x =,which implies that 3b y = and 3c z = (they are all positive by assumption). So L has only one stationary point ),3,3,3(λc b a (for some value of λ, which we could work out if we wanted to). Since it is the only stationary point it must the required max and the max volume is3383338abc c b a =.中文译文 多元函数的极值1. 稳定点定义1.1 使n R D ⊆并且R D f →:. 对于任意一点D a ∈有以下定义:(1)如果)()(a f x f ≤对于所有x 充分地接近a 时,则)(a f 是一个局部极大值;(2)如果)()(a f x f ≥对于所有x 充分地接近a 时,则)(a f 是一个局部极小值;(3)如果)()(a f x f ≤对于所有点D x ∈成立,则)(a f 是一个全局极大值(或绝对极大值);(4) 如果)()(a f x f ≥对于所有点D x ∈成立,则)(a f 是一个全局极小值(或绝对极小值); (5) 局部极大(小)值统称为局部极值;全局极大(小)值统称为全局极值.定义 1.2 使n R D ⊆并且R D f →:.对于任意一点D a ∈,如果0)(=∇a f ,并且对于任意奇异点a 都不存在f ∇,则称a 是一个关键点或稳定点.结论 1.3 使n R D ⊆并且R D f →:.如果f 有局部极值或全局极值对于一点D a ∈, 则a 一定是:(1)函数f 的一个关键点, 或者(2)函数f 的一个奇异点, 或者(3)定义域D 的一个边界点.结论 1.4 如果函数f 是一个在闭区间上的连续函数,则f 在区间上有边界并且可以取到边界值.定义 1.5 对于任一个关键点a ,当a 既不是局部极大值也不是局部极小值时,a 叫做函数的鞍点.结论 1.6 对于一个关键点a 是鞍点当且仅当h 任意小时,对于函数)()(a f h a f -+取正值和负值.定义 1.7 如果R R f →2: 是二元函数,并且在点),(b a 处所有二阶偏导数都存在,则则根据函数f 在点),(b a 处导数,有f 在点),(b a 处的Hessian 矩阵为:⎪⎪⎭⎫ ⎝⎛=yy yx xy xxf ff f H . 推广:如果R R f →3: 是三元函数,并且在点),,(c b a 处所有二阶偏导数都存在,则根据函数f 在点),,(c b a 处导数,有f 在点),,(c b a 处的Hessian 矩阵为:⎪⎪⎪⎭⎫ ⎝⎛=zz zyzxyz yy yxxz xy xxf f f f f f f f f H . 定义 1.8 矩阵A 是n n ⨯ 阶矩阵,并且对于每一个都有n r ≤≤1,从矩阵A 中选取左上端的r 行和r 列,令其为r r ⨯阶的矩阵r A .则行列式det(r A ),n r ≤≤1,叫做矩阵A 的顺序主子式.定理 1.9 假如R R f →2:是一个充分光滑的二元函数,且在点),(b a 处稳定,其Hessian 矩阵为H .如果0)det(≠H ,则根据偏导数判定),(b a 点是:(1) 一个局部极大值点, 如果0>det(H 1) = f xx 并且0<det(H )=2xy yy xx f f f -; (2) 一个局部极小值点, 如果0<det(H 1) = f xx 并且0<det(H )=2xy yy xx f f f -;(3) 一个鞍点,如果点),(b a 既不是局部极大值点也不是局部极小值点. 假如R R f →3:是一个充分光滑的三元函数,且在点),,(c b a 处稳定,其Hessian 矩阵为H .如果0)det(≠H ,则根据偏导数判定),,(c b a 点是: (1) 一个局部极大值点, 如果当0>det(H 1), 0<det(H 2) 并且 0>det(H 3)时; (2) 一个局部极小值点, 如果当0<det(H 1), 0<det(H 2) 并且 0>det(H 3)时; (3) 一个鞍点,如果点),,(c b a 既不是局部极大值点也不是局部极小值点. 在不同的情况下 ,当det(H )= 0时, 点),(b a 是一个局部极值点,或者是一个鞍点.例. 确定下列函数的稳定点并说明是哪一类点: (1) ;1),,(2224+++++=xz z y y x x z y x f (2) ;)1()1(),(422++++=x y x y y x f 解. (1) 1),,(2224+++++=xz z y y x x z y x f ,so)24),(3z xy x y x f ++=∇(i )2(2y x ++j )2(x z ++k当0=∇f 时有稳定点,也就是说, 当(1) z xy x ++=2403 (2) y x 202+= (3) x z +=20时,将方程(2)和方程(3)带入到方程(1)可以消去变量y 和z, 由此可以得到021433=--x x x 即0)16(2=-x x ,得0=x ,66=x 和66-=x .因此我们可以得到函数的三个稳定点:)(0,0,0,)(126,121,66--和)(126,121,66--. 又因为y x f xx 2122+=,x f xy 2=,1=xz f ,2=yy f ,0=yz f 和2=zz f ,则Hessian 矩阵为⎪⎪⎪⎭⎫⎝⎛+=201022122122x x y x H在点)(126,121,66--处, ⎪⎪⎪⎪⎭⎫⎝⎛=201023/613/66/11H则顺序主子式611>0, 039631123/63/66/11>=-=并且行列式042912322>=--=H .根据主子式判定方法,则点)(126,121,66--是一个局部极小值点.在点)(126,121,66--处, ⎪⎪⎪⎪⎭⎫⎝⎛--=201023/613/66/11H则顺序主子式 611>0,039631123/63/66/11>=-=-- 并且行列式042912322>=--=H .根据主子式判定方法,则点)(126,121,66--也是一个极小值点.在点)(0,0,0处,Hessian 矩阵为⎪⎪⎪⎭⎫⎝⎛=201020100H因此det 2)(-=H ,根据主子式判定方法,第一主子式为0,由此我们可以知道该点是一个鞍点. 下面是另一种计算方法,在这种情况下,我们考虑现在下面函数表达式hl l k k h h l k h f f D ++++=+++-=22240,0,00,0,0)()(,的值,对于任意h, k 和l 无限小时. 担当h, k 和l 非常小时, 三次及三次以上方程相对线性二次方程时可忽略不计,则原方程可为hl l k D ++≈22.当h, k 和l 都为正时,0>D .然而, 当0=k 、0<h 和h l <<0,则0<D .因此当接近)(0,0,0时,f 同时增加或者同时减少, 所以 )(0,0,0是一个鞍点. (2) 422)1()1(),(++++=x y x y y x f so))1(4)1(2(),(3+++=∇x y x y x f i ))1(2(2+++x y j .当0=∇f 时有稳定点,也就是说, 当在)0,1(-时.现在我们在不考虑主子式判定方法的情况下为该稳定点进行分类(因为在)0,1(-时Hessian 矩阵的行列式为0,所以该判定方法在此刻无法应用).令.0,10,1422h k h k k h f f D ++=++---=)()(配成完全平方的形式为.43)2(222h h k D ++=所以对h 和k 为任意小时(h 和k 都不为0),有0>D ,因此我们可以确定函数f 在点)0,1(-处有局部极大值.2. 条件极值和Lagrange 乘数法定义 2.1 函数f 和函数g 都是n 元函数.对于限制在条件g (x) = 0下的函数f (x )的极值叫做函数的条件极值,函数g (x ) = 0叫做限制条件.定义 2.2 如果函数R R f n →: 是一个n 元函数, 则对应于函数f 的Lagrange 函数在限制条件0),,,(21=n x x x g 下的函数是一个n +1元函数),,,,(),,,(),,,,(212121n n n x x x g x x x f x x x L λλ+=这就是著名的Lagrange 乘数法.对应于函数f 的Lagrange 函数在k 个限制条件0),,,(21=n i x x x g ,k i ≤≤1时, 带有k 个i λk i ≤≤1,的Lagrange 函数为:∑=+=ki n n n x x x g x x x f x x x L 1212121),,,(),,,(),,,,( λλ定理 2.3 使R R f →2:并且),(00y x P =是曲线C 上的一个点, 有方程 g(x,y) = 0成立,则在限制条件C 上函数f 有局部极值.假设函数f 和函数g 在点P 都有连续的偏导数,点P 不是曲线C 的端点,且0),(00≠∇y x g . 因此存在λ的值使得点),,(000z y x 是Lagrange 函数的关键点),(),(),,(y x g y x f y x L λλ+=.证明.仅仅描述. 因为点P 不是曲线C 的端点,且0≠∇g ,则曲线C 在点P 处的切线与g ∇有关.如果f ∇在点P 处与g ∇平行,则函数在点P 处的切线有非零值.但另一方面函数 f 的值随着P 在C 的运动增加减小,所以点P 不是极值点. 因为f ∇和g ∇平行,所以存在λ使得g f ∇-=∇λ成立.例. 求内接于椭球1222222=++cz b y a x 的体积最大的长方体的体积,长方体的各个面平行于坐标面解:明显地,当长方体的体积最大时,长方体的各个顶点一定在椭球上. 设长方体的一个顶点坐标为(x, y, z) (x>0, y>0, z>0), 则长方体的其他顶点坐标分别为(±x,±y,±z),并且长方体的体积为V= 8xyz.我们要求V 在条件01222222=-++cz b y a x 下的最大值. (注意:因为约束条件是有边界的,故其一定存在极大或者极小值). 其Lagrange 函数为⎪⎪⎭⎫⎝⎛-+++=18),,,(222222c z b y a x xyz z y x L λλ并且存在稳定点当0=∇L 时,也就是说,当,.280,280,280222cz xy z L b y zx y L a x yz x L λλλ+=∂∂=+=∂∂=+=∂∂=⎪⎪⎭⎫ ⎝⎛-++=∂∂=10222222c z b y a x z L 时.(注意:λL 是约束方程.要想求得体积V 的最大值,假设0≠xyz ,则可得0,,≠z y x .)因此, 用其他式子表示λ, 我们可以得到,444222zxyc y zx b x yz a -=-=-=λ 消去λ,有2222b x a y =和.2222c y b z =进而得出 222222cz b y a x ==,因此有2222222231ax c z b y a x =++=或者得出3a x =,同理可得出3by =和3c z = (根据假设可得x, y, z 都是正值).所以函数 L 有且仅有一个稳定点),3,3,3(λc b a (λ为某一计算可得到的常数). 又因为该点是函数L 的唯一稳定点,则该稳定点一定是所要求的最大值点,故其体积的最大值为3383338abcc b a =.。