2017美赛数学建模M奖论文
美国大学生数学建模竞赛优秀论文
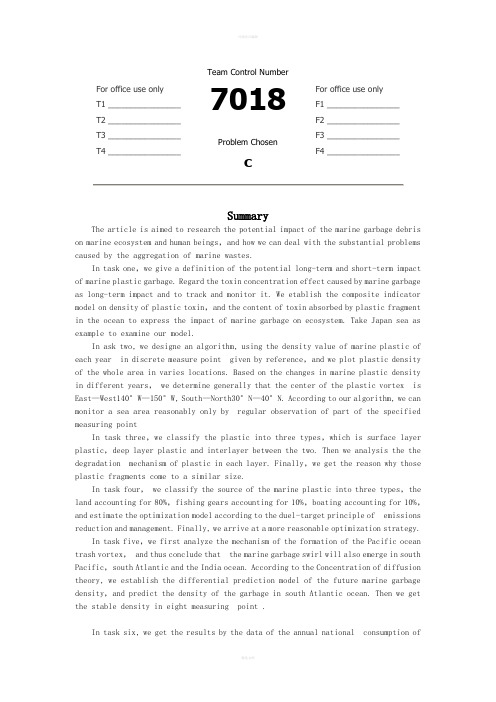
For office use onlyT1________________ T2________________ T3________________ T4________________Team Control Number7018Problem ChosencFor office use onlyF1________________F2________________F3________________F4________________ SummaryThe article is aimed to research the potential impact of the marine garbage debris on marine ecosystem and human beings,and how we can deal with the substantial problems caused by the aggregation of marine wastes.In task one,we give a definition of the potential long-term and short-term impact of marine plastic garbage. Regard the toxin concentration effect caused by marine garbage as long-term impact and to track and monitor it. We etablish the composite indicator model on density of plastic toxin,and the content of toxin absorbed by plastic fragment in the ocean to express the impact of marine garbage on ecosystem. Take Japan sea as example to examine our model.In ask two, we designe an algorithm, using the density value of marine plastic of each year in discrete measure point given by reference,and we plot plastic density of the whole area in varies locations. Based on the changes in marine plastic density in different years, we determine generally that the center of the plastic vortex is East—West140°W—150°W, South—North30°N—40°N. According to our algorithm, we can monitor a sea area reasonably only by regular observation of part of the specified measuring pointIn task three,we classify the plastic into three types,which is surface layer plastic,deep layer plastic and interlayer between the two. Then we analysis the the degradation mechanism of plastic in each layer. Finally,we get the reason why those plastic fragments come to a similar size.In task four, we classify the source of the marine plastic into three types,the land accounting for 80%,fishing gears accounting for 10%,boating accounting for 10%,and estimate the optimization model according to the duel-target principle of emissions reduction and management. Finally, we arrive at a more reasonable optimization strategy.In task five,we first analyze the mechanism of the formation of the Pacific ocean trash vortex, and thus conclude that the marine garbage swirl will also emerge in south Pacific,south Atlantic and the India ocean. According to the Concentration of diffusion theory, we establish the differential prediction model of the future marine garbage density,and predict the density of the garbage in south Atlantic ocean. Then we get the stable density in eight measuring point .In task six, we get the results by the data of the annual national consumption ofpolypropylene plastic packaging and the data fitting method, and predict the environmental benefit generated by the prohibition of polypropylene take-away food packaging in the next decade. By means of this model and our prediction,each nation will reduce releasing 1.31 million tons of plastic garbage in next decade.Finally, we submit a report to expediction leader,summarize our work and make some feasible suggestions to the policy- makers.Task 1:Definition:●Potential short-term effects of the plastic: the hazardeffects will be shown in the short term.●Potential long-term effects of the plastic: thepotential effects, of which hazards are great, willappear after a long time.The short- and long-term effects of the plastic on the ocean environment:In our definition, the short-term and long-term effects of the plastic on the ocean environment are as follows.Short-term effects:1)The plastic is eaten by marine animals or birds.2) Animals are wrapped by plastics, such as fishing nets, which hurt or even kill them.3)Deaden the way of the passing vessels.Long-term effects:1)Enrichment of toxins through the food chain: the waste plastic in the ocean has no natural degradation in theshort-term, which will first be broken down into tinyfragments through the role of light, waves,micro-organisms, while the molecular structure has notchanged. These "plastic sands", easy to be eaten byplankton, fish and other, are Seemingly very similar tomarine life’s food,causing the enrichment and delivery of toxins.2)Accelerate the greenhouse effect: after a long-term accumulation and pollution of plastics, the waterbecame turbid, which will seriously affect the marineplants (such as phytoplankton and algae) inphotosynthesis. A large number of plankton’s deathswould also lower the ability of the ocean to absorbcarbon dioxide, intensifying the greenhouse effect tosome extent.To monitor the impact of plastic rubbish on the marine ecosystem:According to the relevant literature, we know that plastic resin pellets accumulate toxic chemicals , such as PCBs、DDE , and nonylphenols , and may serve as a transport medium and soure of toxins to marine organisms that ingest them[]2. As it is difficult for the plastic garbage in the ocean to complete degradation in the short term, the plastic resin pellets in the water will increase over time and thus absorb more toxins, resulting in the enrichment of toxins and causing serious impact on the marine ecosystem.Therefore, we track the monitoring of the concentration of PCBs, DDE, and nonylphenols containing in the plastic resin pellets in the sea water, as an indicator to compare the extent of pollution in different regions of the sea, thus reflecting the impact of plastic rubbish on ecosystem.To establish pollution index evaluation model: For purposes of comparison, we unify the concentration indexes of PCBs, DDE, and nonylphenols in a comprehensive index.Preparations:1)Data Standardization2)Determination of the index weightBecause Japan has done researches on the contents of PCBs,DDE, and nonylphenols in the plastic resin pellets, we illustrate the survey conducted in Japanese waters by the University of Tokyo between 1997 and 1998.To standardize the concentration indexes of PCBs, DDE,and nonylphenols. We assume Kasai Sesside Park, KeihinCanal, Kugenuma Beach, Shioda Beach in the survey arethe first, second, third, fourth region; PCBs, DDE, andnonylphenols are the first, second, third indicators.Then to establish the standardized model:j j jij ij V V V V V min max min --= (1,2,3,4;1,2,3i j ==)wherej V max is the maximum of the measurement of j indicator in the four regions.j V min is the minimum of the measurement of j indicatorstandardized value of j indicator in i region.According to the literature [2], Japanese observationaldata is shown in Table 1.Table 1. PCBs, DDE, and, nonylphenols Contents in Marine PolypropyleneTable 1 Using the established standardized model to standardize, we have Table 2.In Table 2,the three indicators of Shioda Beach area are all 0, because the contents of PCBs, DDE, and nonylphenols in Polypropylene Plastic Resin Pellets in this area are the least, while 0 only relatively represents the smallest. Similarly, 1 indicates that in some area the value of a indicator is the largest.To determine the index weight of PCBs, DDE, and nonylphenolsWe use Analytic Hierarchy Process (AHP) to determine the weight of the three indicators in the general pollution indicator. AHP is an effective method which transforms semi-qualitative and semi-quantitative problems into quantitative calculation. It uses ideas of analysis and synthesis in decision-making, ideally suited for multi-index comprehensive evaluation.Hierarchy are shown in figure 1.Fig.1 Hierarchy of index factorsThen we determine the weight of each concentrationindicator in the generall pollution indicator, and the process are described as follows:To analyze the role of each concentration indicator, we haveestablished a matrix P to study the relative proportion.⎥⎥⎥⎦⎤⎢⎢⎢⎣⎡=111323123211312P P P P P P P Where mn P represents the relative importance of theconcentration indicators m B and n B . Usually we use 1,2,…,9 and their reciprocals to represent different importance. The greater the number is, the more important it is. Similarly, the relative importance of m B and n B is mn P /1(3,2,1,=n m ).Suppose the maximum eigenvalue of P is m ax λ, then theconsistency index is1max --=n nCI λThe average consistency index is RI , then the consistencyratio isRICI CR = For the matrix P of 3≥n , if 1.0<CR the consistency isthougt to be better, of which eigenvector can be used as the weight vector.We get the comparison matrix accoding to the harmful levelsof PCBs, DDE, and nonylphenols and the requirments ofEPA on the maximum concentration of the three toxins inseawater as follows:⎥⎥⎥⎦⎤⎢⎢⎢⎣⎡=165416131431P We get the maximum eigenvalue of P by MATLAB calculation0012.3max =λand the corresponding eigenvector of it is()2393.02975.09243.0,,=W1.0042.012.1047.0<===RI CI CR Therefore,we determine the degree of inconsistency formatrix P within the permissible range. With the eigenvectors of p as weights vector, we get thefinal weight vector by normalization ()1638.02036.06326.0',,=W . Defining the overall target of pollution for the No i oceanis i Q , among other things the standardized value of threeindicators for the No i ocean is ()321,,i i i i V V V V = and the weightvector is 'W ,Then we form the model for the overall target of marine pollution assessment, (3,2,1=i )By the model above, we obtained the Value of the totalpollution index for four regions in Japanese ocean in Table 3T B W Q '=In Table3, the value of the total pollution index is the hightest that means the concentration of toxins in Polypropylene Plastic Resin Pellets is the hightest, whereas the value of the total pollution index in Shioda Beach is the lowest(we point up 0 is only a relative value that’s not in the name of free of plastics pollution)Getting through the assessment method above, we can monitor the concentration of PCBs, DDE and nonylphenols in the plastic debris for the sake of reflecting the influence to ocean ecosystem.The highter the the concentration of toxins,the bigger influence of the marine organism which lead to the inrichment of food chain is more and more dramatic.Above all, the variation of toxins’ concentration simultaneously reflects the distribution and time-varying of marine litter. We can predict the future development of marine litter by regularly monitoring the content of these substances, to provide data for the sea expedition of the detection of marine litter and reference for government departments to make the policies for ocean governance.Task 2:In the North Pacific, the clockwise flow formed a never-ending maelstrom which rotates the plastic garbage. Over the years, the subtropical eddy current in North Pacific gathered together the garbage from the coast or the fleet, entrapped them in the whirlpool, and brought them to the center under the action of the centripetal force, forming an area of 3.43 million square kilometers (more than one-third of Europe) .As time goes by, the garbage in the whirlpool has the trend of increasing year by year in terms of breadth, density, and distribution. In order to clearly describe the variability of the increases over time and space, according to “Count Densities of Plastic Debris from Ocean Surface Samples North Pacific Gyre 1999—2008”, we analyze the data, exclude them with a great dispersion, and retain them with concentrated distribution, while the longitude values of the garbage locations in sampled regions of years serve as the x-coordinate value of a three-dimensional coordinates, latitude values as the y-coordinate value, the Plastic Count per cubic Meter of water of the position as the z-coordinate value. Further, we establish an irregular grid in the yx plane according to obtained data, and draw a grid line through all the data points. Using the inverse distance squared method with a factor, which can not only estimate the Plastic Count per cubic Meter of water of any position, but also calculate the trends of the Plastic Counts per cubic Meter of water between two original data points, we can obtain the unknown grid points approximately. When the data of all the irregular grid points are known (or approximately known, or obtained from the original data), we can draw the three-dimensional image with the Matlab software, which can fully reflect the variability of the increases in the garbage density over time and space.Preparations:First, to determine the coordinates of each year’s sampled garbage.The distribution range of garbage is about the East - West 120W-170W, South - North 18N-41N shown in the “Count Densities of Plastic Debris from Ocean Surface Samples North Pacific Gyre 1999--2008”, we divide a square in the picture into 100 grids in Figure (1) as follows:According to the position of the grid where the measuring point’s center is, we can identify the latitude and longitude for each point, which respectively serve as the x- and y- coordinate value of the three-dimensional coordinates.To determine the Plastic Count per cubic Meter of water. As the “Plastic Count per cubic Meter of water” provided by “Count Densities of P lastic Debris from Ocean Surface Samples North Pacific Gyre 1999--2008”are 5 density interval, to identify the exact values of the garbage density of one year’s different measuring points, we assume that the density is a random variable which obeys uniform distribution in each interval.Uniform distribution can be described as below:()⎪⎩⎪⎨⎧-=01a b x f ()others b a x ,∈We use the uniform function in Matlab to generatecontinuous uniformly distributed random numbers in each interval, which approximately serve as the exact values of the garbage density andz-coordinate values of the three-dimensional coordinates of the year’s measuring points.Assumptions(1)The data we get is accurate and reasonable.(2)Plastic Count per cubic Meter of waterIn the oceanarea isa continuous change.(3)Density of the plastic in the gyre is a variable by region.Density of the plastic in the gyre and its surrounding area is interdependent , However, this dependence decreases with increasing distance . For our discussion issue, Each data point influences the point of each unknown around and the point of each unknown around is influenced by a given data point. The nearer a given data point from the unknown point, the larger the role.Establishing the modelFor the method described by the previous,we serve the distributions of garbage density in the “Count Pensities of Plastic Debris from Ocean Surface Samples North Pacific Gyre 1999--2008”as coordinates ()z y,, As Table 1:x,Through analysis and comparison, We excluded a number of data which has very large dispersion and retained the data that is under the more concentrated the distribution which, can be seen on Table 2.In this way, this is conducive for us to get more accurate density distribution map.Then we have a segmentation that is according to the arrangement of the composition of X direction and Y direction from small to large by using x co-ordinate value and y co-ordinate value of known data points n, in order to form a non-equidistant Segmentation which has n nodes. For the Segmentation we get above,we only know the density of the plastic known n nodes, therefore, we must find other density of the plastic garbage of n nodes.We only do the sampling survey of garbage density of the north pacificvortex,so only understand logically each known data point has a certain extent effect on the unknown node and the close-known points of density of the plastic garbage has high-impact than distant known point.In this respect,we use the weighted average format, that means using the adverse which with distance squared to express more important effects in close known points. There're two known points Q1 and Q2 in a line ,that is to say we have already known the plastic litter density in Q1 and Q2, then speculate the plastic litter density's affects between Q1、Q2 and the point G which in the connection of Q1 and Q2. It can be shown by a weighted average algorithm22212221111121GQ GQ GQ Z GQ Z Z Q Q G +*+*=in this formula GQ expresses the distance between the pointG and Q.We know that only use a weighted average close to the unknown point can not reflect the trend of the known points, we assume that any two given point of plastic garbage between the changes in the density of plastic impact the plastic garbage density of the unknown point and reflecting the density of plastic garbage changes in linear trend. So in the weighted average formula what is in order to presume an unknown point of plastic garbage density, we introduce the trend items. And because the greater impact at close range point, and thus the density of plastic wastes trends close points stronger. For the one-dimensional case, the calculation formula G Z in the previous example modify in the following format:2212122212212122211111112121Q Q GQ GQ GQ Q Q GQ Z GQ Z GQ Z Z Q Q Q Q G ++++*+*+*=Among them, 21Q Q known as the separation distance of the known point, 21Q Q Z is the density of plastic garbage which is the plastic waste density of 1Q and 2Q for the linear trend of point G . For the two-dimensional area, point G is not on the line 21Q Q , so we make a vertical from the point G and cross the line connect the point 1Q and 2Q , and get point P , the impact of point P to 1Q and 2Q just like one-dimensional, and the one-dimensional closer of G to P , the distant of G to P become farther, the smaller of the impact, so the weighting factor should also reflect the GP in inversely proportional to a certain way, then we adopt following format:221212222122121222211111112121Q Q GQ GP GQ GQ Q Q GQ GP Z GQ Z GQ Z Z P Q Q Q Q G ++++++*+*+*=Taken together, we speculated following roles:(1) Each known point data are influence the density of plastic garbage of each unknown point in the inversely proportional to the square of the distance;(2) the change of density of plastic garbage between any two known points data, for each unknown point are affected, and the influence to each particular point of their plastic garbage diffuse the straight line along the two known particular point; (3) the change of the density of plastic garbage between any two known data points impact a specific unknown points of the density of plastic litter depends on the three distances: a. the vertical distance to a straight line which is a specific point link to a known point;b. the distance between the latest known point to a specific unknown point;c. the separation distance between two known data points.If we mark 1Q ,2Q ,…,N Q as the location of known data points,G as an unknown node, ijG P is the intersection of the connection of i Q ,j Q and the vertical line from G to i Q ,j Q()G Q Q Z j i ,,is the density trend of i Q ,j Q in the of plasticgarbage points and prescribe ()G Q Q Z j i ,,is the testing point i Q ’ s density of plastic garbage ,so there are calculation formula:()()∑∑∑∑==-==++++*=Ni N ij ji i ijGji i ijG N i Nj j i G Q Q GQ GPQ Q GQ GP G Q Q Z Z 11222222111,,Here we plug each year’s observational data in schedule 1 into our model, and draw the three-dimensional images of the spatial distribution of the marine garbage ’s density with Matlab in Figure (2) as follows:199920002002200520062007-2008(1)It’s observed and analyzed that, from 1999 to 2008, the density of plastic garbage is increasing year by year and significantly in the region of East – West 140W-150W, south - north 30N-40N. Therefore, we can make sure that this region is probably the center of the marine litter whirlpool. Gathering process should be such that the dispersed garbage floating in the ocean move with the ocean currents and gradually close to the whirlpool region. At the beginning, the area close to the vortex will have obviously increasable about plastic litter density, because of this centripetal they keeping move to the center of the vortex ,then with the time accumulates ,the garbage density in the center of the vortex become much bigger and bigger , at last it becomes the Pacific rubbish island we have seen today.It can be seen that through our algorithm, as long as the reference to be able to detect the density in an area which has a number of discrete measuring points,Through tracking these density changes ,we Will be able to value out all the waters of the density measurement through our models to determine,This will reduce the workload of the marine expedition team monitoring marine pollution significantly, and also saving costs .Task 3:The degradation mechanism of marine plasticsWe know that light, mechanical force, heat, oxygen, water, microbes, chemicals, etc. can result in the degradation of plastics . In mechanism ,Factors result in the degradation can be summarized as optical ,biological,and chemical。
2017全国研究生数学建模竞赛优秀论文发表(2)

2017全国研究生数学建模竞赛优秀论文发表(2)数学优秀论文篇1浅谈多媒体辅助教学在初中数学中的应用一、多媒体辅助教学的优势初中数学其实主要研究的就是数量关系和空间形式,在教学中若能巧妙的使用多媒体进行辅助,有时可以起到事半功倍的效果,特别是在有关空间形式的教学中,利用多媒体可以更加生动形象的将教学内容展示给学生,帮助学生理解相关的知识,激发学生的数学思维。
除此外多媒体辅助教学大大的提高了教学效率,节省了板书时间,从另一个角度来说则是加大了原本的课程容量,使得学生的知识得到了拓展。
多媒体辅助教学在初中数学中的应用主要有一下几个特点。
1.拓宽学生的视野,培养学生的学习兴趣板书在以往的课堂上都占用了很多一部分的时间,应用多媒体进行辅助教学,教师在授课的过程中通常就不需要进行书写板书,从而节省下来很客观的课堂时间。
有了这些节省下来的时间,再加上多媒体对教学带来的便利,教师可以将这些时间用在补充拓展课外知识之上,课外知识不仅能够拓展学生的视野,更能激发和培养学生的兴趣。
兴趣是最好的老师,实际上,老师的教学对学生起到的更重要的是一种引导作用,学生想要学的更好,更需要的是进行自主的学习和探究。
举个例子,在初中的几何学习中,教师可以通过应用多媒体技术,将一些精美的几何图案向学生进行展示,让学生不仅得到美的享受,也加深对几何知识的理解。
同时基于对美好事物的喜爱,还能激发学生的学习热情,增强学生的学习积极性。
2.突出重点,突破难点初中生的思维正处于从具体思维向抽象思维过度的时期,而数学真好具有形象性和抽象性,这就使得学生在学习数学的时候会遇到一定的困难。
多媒体辅助教学可以再一定程度上解决这一问题。
利用多媒体,教室可以很明确的将教学重点进行突出,对教学的难点进行重点的突破。
举个简单的例子,在初中数学中,学生需要进行评议、旋转等等知识的学习,这些知识的学习要求学生将抽象的知识转化为形象的模型,这对学生的想象能力和思维能力都有很高的要求。
数学建模 美赛获奖论文

________________
F2
________________
F3
________________
F4
________________
2010 Mathematical Contest in Modeling (MCM) Summary Sheet
(Attach a copy of this page to each copy of your solution paper.)
Keywords:simple harmonic motion system , differential equations model , collision system
2017年数学建模优秀论文
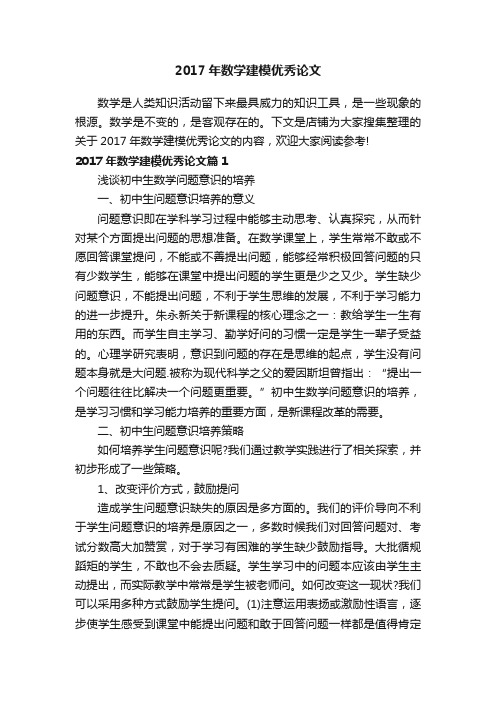
2017年数学建模优秀论文数学是人类知识活动留下来最具威力的知识工具,是一些现象的根源。
数学是不变的,是客观存在的。
下文是店铺为大家搜集整理的关于2017年数学建模优秀论文的内容,欢迎大家阅读参考!2017年数学建模优秀论文篇1浅谈初中生数学问题意识的培养一、初中生问题意识培养的意义问题意识即在学科学习过程中能够主动思考、认真探究,从而针对某个方面提出问题的思想准备。
在数学课堂上,学生常常不敢或不愿回答课堂提问,不能或不善提出问题,能够经常积极回答问题的只有少数学生,能够在课堂中提出问题的学生更是少之又少。
学生缺少问题意识,不能提出问题,不利于学生思维的发展,不利于学习能力的进一步提升。
朱永新关于新课程的核心理念之一:教给学生一生有用的东西。
而学生自主学习、勤学好问的习惯一定是学生一辈子受益的。
心理学研究表明,意识到问题的存在是思维的起点,学生没有问题本身就是大问题.被称为现代科学之父的爱因斯坦曾指出:“提出一个问题往往比解决一个问题更重要。
”初中生数学问题意识的培养,是学习习惯和学习能力培养的重要方面,是新课程改革的需要。
二、初中生问题意识培养策略如何培养学生问题意识呢?我们通过教学实践进行了相关探索,并初步形成了一些策略。
1、改变评价方式,鼓励提问造成学生问题意识缺失的原因是多方面的。
我们的评价导向不利于学生问题意识的培养是原因之一,多数时候我们对回答问题对、考试分数高大加赞赏,对于学习有困难的学生缺少鼓励指导。
大批循规蹈矩的学生,不敢也不会去质疑。
学生学习中的问题本应该由学生主动提出,而实际教学中常常是学生被老师问。
如何改变这一现状?我们可以采用多种方式鼓励学生提问。
(1)注意运用表扬或激励性语言,逐步使学生感受到课堂中能提出问题和敢于回答问题一样都是值得肯定和鼓励的。
(2)把学生课堂提问是否积极作为对学生评价的一个重要方面。
(3)有目的进行一些提问竞赛等活动。
2、夯实学习基础,让学生能问教学实践中我们体会到学生能否提出问题与学生学习基础有密切关系,学习基础较好的学生更容易提出问题。
2017年全国数学建模论文
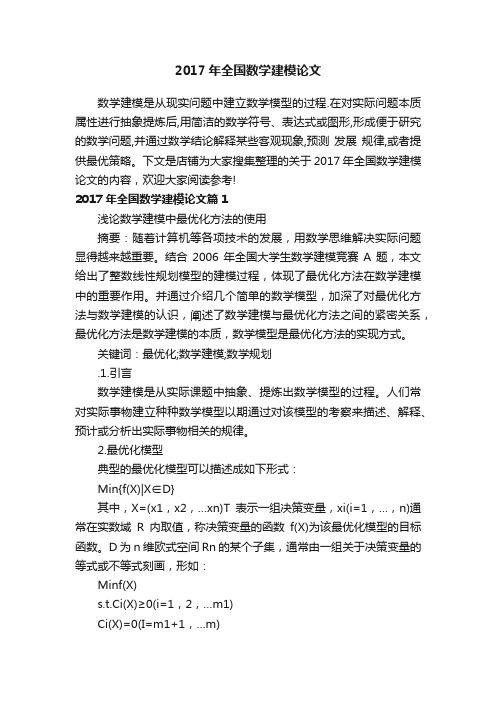
2017年全国数学建模论文数学建模是从现实问题中建立数学模型的过程.在对实际问题本质属性进行抽象提炼后,用简洁的数学符号、表达式或图形,形成便于研究的数学问题,并通过数学结论解释某些客观现象,预测发展规律,或者提供最优策略。
下文是店铺为大家搜集整理的关于2017年全国数学建模论文的内容,欢迎大家阅读参考!2017年全国数学建模论文篇1浅论数学建模中最优化方法的使用摘要:随着计算机等各项技术的发展,用数学思维解决实际问题显得越来越重要。
结合2006年全国大学生数学建模竞赛A题,本文给出了整数线性规划模型的建模过程,体现了最优化方法在数学建模中的重要作用。
并通过介绍几个简单的数学模型,加深了对最优化方法与数学建模的认识,阐述了数学建模与最优化方法之间的紧密关系,最优化方法是数学建模的本质,数学模型是最优化方法的实现方式。
关键词:最优化;数学建模;数学规划.1.引言数学建模是从实际课题中抽象、提炼出数学模型的过程。
人们常对实际事物建立种种数学模型以期通过对该模型的考察来描述、解释、预计或分析出实际事物相关的规律。
2.最优化模型典型的最优化模型可以描述成如下形式:Min{f(X)|X∈D}其中,X=(x1,x2,…xn)T表示一组决策变量,xi(i=1,…,n)通常在实数域R内取值,称决策变量的函数f(X)为该最优化模型的目标函数。
D为n维欧式空间Rn的某个子集,通常由一组关于决策变量的等式或不等式刻画,形如:Minf(X)s.t.Ci(X)≥0(i=1,2, (1)Ci(X)=0(I=m1+1,…m)这时,称模型中关于决策变量的等式或不等式Ci(X)≥0(i=1,2,…m1)、Ci(X)=0(I=m1+1,…m)为约束条件,而称满足全部约束条件的空间Rn中的点X为该模型的可行解,称即由所有可行解构成的集合为该模型的可行域。
称X*∈D为最优化模型Min{f(X)|X∈D}的(全局)最优解,若满足:对?X∈D均有f(X*)≤f(X),这时称X*∈D处的目标函数值f(X*)为最优化模型Min{f(X)|X∈D}的(全局)最优值;称X*∈D为最优化模型Min{f(X)|X∈D}的局部最优解,若存在δ>0,对?X∈D∩{X∈Rn| }均有f(X*)≤f(X)。
2017数学建模优秀论文d题方面的

2017数学建模优秀论文d题方面的数学建模就是学习如何把物理的复杂的世界用适当的数学语言描述出来,进而用数学的手段对模型加以分析,然后再用所得结论回归现实,指导实践。
下文是店铺为大家搜集整理的关于2017数学建模优秀论文的内容,欢迎大家阅读参考!2017数学建模优秀论文篇1浅谈大学生数学建模的意义【摘要】本文重点分析了数学建模对当前数学教育教学改革的现实意义,探讨了数学建模对学生应用数学能力的培养,阐述了计算机在数学建模竞赛中的作用和地位,最后介绍了数学建模对数学教学改革的启示意义。
【关键词】数学建模;综合素质;教学改革长期以来,我国的数学教学中一直普遍存在着重结论而轻过程、重形式而轻内容、重解法而轻应用等弊端,不注重学生数学能力和素质的培养;过分强调对定义、定理、法则、公式等知识的灌输与讲授,不注重这些知识的应用,割断了理论与实际的联系,造成学与用的严重脱节,致使在我们的数学教育体制下培养出来的学生的能力结构都形成了一种严重的病态,主要表现在:数学理论知识掌握得还可以,但应用知识的能力很差,不能学以致用,缺乏创造力和解决实际问题的能力,这些问题使我们的学生在走向工作岗位时上手速度慢,面对新的数学问题时束手无策,不能将所学的知识灵活运用到实际中去。
显然,这种教育体制和理念与现代教育理念是背道而驰的,是必须抛弃的。
开展数学建模教学或数学建模竞赛,能够培养学生各方面的综合能力,提高学生的综合素质,对于当前数学教育教学改革有着极为重要的现实意义。
1 数学建模能够丰富和优化学生的知识结构,开拓学生的视野数学建模所涉及到的许多问题都超出了学生所学的专业,例如“基金的最佳适用”、“会议筹备”、“地震搜索”等许多建模问题,分别属于不同的学科与专业,为了解决这些问题,学生必须查阅和学习与该问题相关的专业书籍和科技资料,了解这些专业的相关知识,从而软化或削弱了目前教育中僵死的专业界限,使学生掌握宽广而扎实的基础知识,使他们不断拓宽分析问题、解决问题的思路,朝着复合型人才和具备全面综合素质人才的方向发展。
数学建模美赛一等奖优秀专业论文
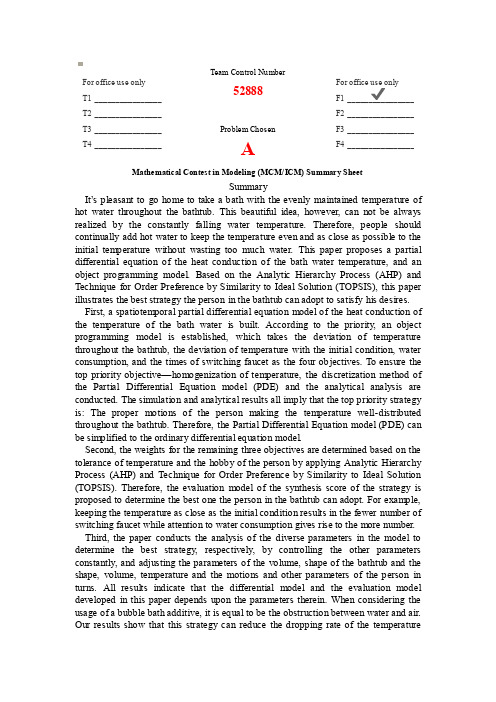
For office use onlyT1________________ T2________________ T3________________ T4________________ Team Control Number52888Problem ChosenAFor office use onlyF1________________F2________________F3________________F4________________Mathematical Contest in Modeling (MCM/ICM) Summary SheetSummaryIt’s pleasant t o go home to take a bath with the evenly maintained temperature of hot water throughout the bathtub. This beautiful idea, however, can not be always realized by the constantly falling water temperature. Therefore, people should continually add hot water to keep the temperature even and as close as possible to the initial temperature without wasting too much water. This paper proposes a partial differential equation of the heat conduction of the bath water temperature, and an object programming model. Based on the Analytic Hierarchy Process (AHP) and Technique for Order Preference by Similarity to Ideal Solution (TOPSIS), this paper illustrates the best strategy the person in the bathtub can adopt to satisfy his desires. First, a spatiotemporal partial differential equation model of the heat conduction of the temperature of the bath water is built. According to the priority, an object programming model is established, which takes the deviation of temperature throughout the bathtub, the deviation of temperature with the initial condition, water consumption, and the times of switching faucet as the four objectives. To ensure the top priority objective—homogenization of temperature, the discretization method of the Partial Differential Equation model (PDE) and the analytical analysis are conducted. The simulation and analytical results all imply that the top priority strategy is: The proper motions of the person making the temperature well-distributed throughout the bathtub. Therefore, the Partial Differential Equation model (PDE) can be simplified to the ordinary differential equation model.Second, the weights for the remaining three objectives are determined based on the tolerance of temperature and the hobby of the person by applying Analytic Hierarchy Process (AHP) and Technique for Order Preference by Similarity to Ideal Solution (TOPSIS). Therefore, the evaluation model of the synthesis score of the strategy is proposed to determine the best one the person in the bathtub can adopt. For example, keeping the temperature as close as the initial condition results in the fewer number of switching faucet while attention to water consumption gives rise to the more number. Third, the paper conducts the analysis of the diverse parameters in the model to determine the best strategy, respectively, by controlling the other parameters constantly, and adjusting the parameters of the volume, shape of the bathtub and the shape, volume, temperature and the motions and other parameters of the person in turns. All results indicate that the differential model and the evaluation model developed in this paper depends upon the parameters therein. When considering the usage of a bubble bath additive, it is equal to be the obstruction between water and air. Our results show that this strategy can reduce the dropping rate of the temperatureeffectively, and require fewer number of switching.The surface area and heat transfer coefficient can be increased because of the motions of the person in the bathtub. Therefore, the deterministic model can be improved as a stochastic one. With the above evaluation model, this paper present the stochastic optimization model to determine the best strategy. Taking the disparity from the initial temperature as the suboptimum objectives, the result of the model reveals that it is very difficult to keep the temperature constant even wasting plentiful hot water in reality.Finally, the paper performs sensitivity analysis of parameters. The result shows that the shape and the volume of the tub, different hobbies of people will influence the strategies significantly. Meanwhile, combine with the conclusion of the paper, we provide a one-page non-technical explanation for users of the bathtub.Fall in love with your bathtubAbstractIt’s pleasant t o go home to take a bath with the evenly maintained temperature of hot water throughout the bathtub. This beautiful idea, however, can not be always realized by the constantly falling water temperature. Therefore, people should continually add hot water to keep the temperature even and as close as possible to the initial temperature without wasting too much water. This paper proposes a partial differential equation of the heat conduction of the bath water temperature, and an object programming model. Based on the Analytic Hierarchy Process (AHP) and Technique for Order Preference by Similarity to Ideal Solution (TOPSIS), this paper illustrates the best strategy the person in the bathtub can adopt to satisfy his desires. First, a spatiotemporal partial differential equation model of the heat conduction of the temperature of the bath water is built. According to the priority, an object programming model is established, which takes the deviation of temperature throughout the bathtub, the deviation of temperature with the initial condition, water consumption, and the times of switching faucet as the four objectives. To ensure the top priority objective—homogenization of temperature, the discretization method of the Partial Differential Equation model (PDE) and the analytical analysis are conducted. The simulation and analytical results all imply that the top priority strategy is: The proper motions of the person making the temperature well-distributed throughout the bathtub. Therefore, the Partial Differential Equation model (PDE) can be simplified to the ordinary differential equation model.Second, the weights for the remaining three objectives are determined based on the tolerance of temperature and the hobby of the person by applying Analytic Hierarchy Process (AHP) and Technique for Order Preference by Similarity to Ideal Solution (TOPSIS). Therefore, the evaluation model of the synthesis score of the strategy is proposed to determine the best one the person in the bathtub can adopt. For example, keeping the temperature as close as the initial condition results in the fewer number of switching faucet while attention to water consumption gives rise to the more number. Third, the paper conducts the analysis of the diverse parameters in the model to determine the best strategy, respectively, by controlling the other parameters constantly, and adjusting the parameters of the volume, shape of the bathtub and the shape, volume, temperature and the motions and other parameters of the person in turns. All results indicate that the differential model and the evaluation model developed in this paper depends upon the parameters therein. When considering the usage of a bubble bath additive, it is equal to be the obstruction between water and air. Our results show that this strategy can reduce the dropping rate of the temperature effectively, and require fewer number of switching.The surface area and heat transfer coefficient can be increased because of the motions of the person in the bathtub. Therefore, the deterministic model can be improved as a stochastic one. With the above evaluation model, this paper present the stochastic optimization model to determine the best strategy. Taking the disparity from the initial temperature as the suboptimum objectives, the result of the model reveals that it is very difficult to keep the temperature constant even wasting plentiful hotwater in reality.Finally, the paper performs sensitivity analysis of parameters. The result shows that the shape and the volume of the tub, different hobbies of people will influence the strategies significantly. Meanwhile, combine with the conclusion of the paper, we provide a one-page non-technical explanation for users of the bathtub.Keywords:Heat conduction equation; Partial Differential Equation model (PDE Model); Objective programming; Strategy; Analytical Hierarchy Process (AHP) Problem StatementA person fills a bathtub with hot water and settles into the bathtub to clean and relax. However, the bathtub is not a spa-style tub with a secondary hearing system, as time goes by, the temperature of water will drop. In that conditions,we need to solve several problems:(1) Develop a spatiotemporal model of the temperature of the bathtub water to determine the best strategy to keep the temperature even throughout the bathtub and as close as possible to the initial temperature without wasting too much water;(2) Determine the extent to which your strategy depends on the shape and volume of the tub, the shape/volume/temperature of the person in the bathtub, and the motions made by the person in the bathtub.(3)The influence of using b ubble to model’s results.(4)Give a one-page non-technical explanation for users that describes your strategyGeneral Assumptions1.Considering the safety factors as far as possible to save water, the upper temperature limit is set to 45 ℃;2.Considering the pleasant of taking a bath, the lower temperature limit is set to 33℃;3.The initial temperature of the bathtub is 40℃.Table 1Model Inputs and SymbolsSymbols Definition UnitT Initial temperature of the Bath water ℃℃T∞Outer circumstance temperatureT Water temperature of the bathtub at the every moment ℃t Time hx X coordinates of an arbitrary point my Y coordinates of an arbitrary point mz Z coordinates of an arbitrary point mαTotal heat transfer coefficient of the system 2()⋅/W m K1SThe surrounding-surface area of the bathtub 2m 2S The above-surface area of water2m 1H Bathtub’s thermal conductivity/W m K ⋅() D The thickness of the bathtub wallm 2H Convection coefficient of water2/W m K ⋅() a Length of the bathtubm b Width of the bathtubm h Height of the bathtubm V The volume of the bathtub water3m c Specific heat capacity of water/()J kg ⋅℃ ρ Density of water3/kg m ()v t Flooding rate of hot water3/m s r TThe temperature of hot water ℃Temperature ModelBasic ModelA spatio-temporal temperature model of the bathtub water is proposed in this paper. It is a four dimensional partial differential equation with the generation and loss of heat. Therefore the model can be described as the Thermal Equation.The three-dimension coordinate system is established on a corner of the bottom of the bathtub as the original point. The length of the tub is set as the positive direction along the x axis, the width is set as the positive direction along the y axis, while the height is set as the positive direction along the z axis, as shown in figure 1.Figure 1. The three-dimension coordinate systemTemperature variation of each point in space includes three aspects: one is the natural heat dissipation of each point in space; the second is the addition of exogenous thermal energy; and the third is the loss of thermal energy . In this way , we build the Partial Differential Equation model as follows:22212222(,,,)(,,,)()f x y z t f x y z t T T T T t x y z c Vαρ-∂∂∂∂=+++∂∂∂∂ (1) Where● t refers to time;● T is the temperature of any point in the space;● 1f is the addition of exogenous thermal energy;● 2f is the loss of thermal energy.According to the requirements of the subject, as well as the preferences of people, the article proposes these following optimization objective functions. A precedence level exists among these objectives, while keeping the temperature even throughout the bathtub must be ensured.Objective 1(.1O ): keep the temperature even throughout the bathtub;22100min (,,,)(,,,)t t V V F t T x y z t dxdydz dt t T x y z t dxdydz dt ⎡⎤⎡⎤⎛⎫=-⎢⎥ ⎪⎢⎥⎢⎥⎣⎦⎝⎭⎣⎦⎰⎰⎰⎰⎰⎰⎰⎰ (2) Objective 2(.2O ): keep the temperature as close as possible to the initial temperature;[]2200min (,,,)tV F T x y z t T dxdydz dt ⎛⎫=- ⎪⎝⎭⎰⎰⎰⎰ (3) Objective 3(.3O ): do not waste too much water;()30min tF v t dt =⋅⎰ (4) Objective 4(.4O ): fewer times of switching.4min F n = (5)Since the .1O is the most crucial, we should give priority to this objective. Therefore, the highest priority strategy is given here, which is homogenization of temperature.Strategy 0 – Homogenization of T emperatureThe following three reasons are provided to prove the importance of this strategy. Reason 1-SimulationIn this case, we use grid algorithm to make discretization of the formula (1), and simulate the distribution of water temperature.(1) Without manual intervention, the distribution of water temperature as shown infigure 2. And the variance of the temperature is 0.4962. 00.20.40.60.8100.51 1.5200.5Length WidthH e i g h t 4242.54343.54444.54545.5Distribution of temperature at the length=1Distribution of temperatureat the width=1Hot water Cool waterFigure 2. Temperature profiles in three-dimension space without manual intervention(2) Adding manual intervention, the distribution of water temperature as shown infigure 3. And the variance of the temperature is 0.005. 00.5100.51 1.5200.5 Length WidthH e i g h t 44.744.7544.844.8544.944.9545Distribution of temperatureat the length=1Distribution of temperature at the width=1Hot water Cool waterFigure 3. Temperature profiles in three-dimension space with manual interventionComparing figure 2 with figure 3, it is significant that the temperature of water will be homogeneous if we add some manual intervention. Therefore, we can assumed that222222()0T T T x y zα∂∂∂++≠∂∂∂ in formula (1). Reason 2-EstimationIf the temperature of any point in the space is different, then222222()0T T T x y zα∂∂∂++≠∂∂∂ Thus, we find two points 1111(,,,)x y z t and 2222(,,,)x y z t with:11112222(,,,)(,,,)T x y z t T x y z t ≠Therefore, the objective function 1F could be estimated as follows:[]2200200001111(,,,)(,,,)(,,,)(,,,)0t t V V t T x y z t dxdydz dt t T x y z t dxdydz dt T x y z t T x y z t ⎡⎤⎡⎤⎛⎫-⎢⎥ ⎪⎢⎥⎢⎥⎣⎦⎝⎭⎣⎦≥->⎰⎰⎰⎰⎰⎰⎰⎰ (6) The formula (6) implies that some motion should be taken to make sure that the temperature can be homogeneous quickly in general and 10F =. So we can assumed that: 222222()0T T T x y zα∂∂∂++≠∂∂∂. Reason 3-Analytical analysisIt is supposed that the temperature varies only on x axis but not on the y-z plane. Then a simplified model is proposed as follows:()()()()()()()2sin 000,0,,00,000t xx x T a T A x l t l T t T l t t T x x l π⎧=+≤≤≤⎪⎪⎪==≤⎨⎪⎪=≤≤⎪⎩ (7)Then we use two ways, Fourier transformation and Laplace transformation, in solving one-dimensional heat equation [Qiming Jin 2012]. Accordingly, we get the solution:()()2222/22,1sin a t l Al x T x t e a l πππ-=- (8) Where ()0,2x ∈, 0t >, ()01|x T f t ==(assumed as a constant), 00|t T T ==.Without general assumptions, we choose three specific value of t , and gain a picture containing distribution change of temperature in one-dimension space at different time.00.20.40.60.811.2 1.4 1.6 1.8200.511.522.533.54Length T e m p e r a t u r e time=3time=5time=8Figure 4. Distribution change of temperature in one-dimension space at different timeT able 2.V ariance of temperature at different timet3 5 8 variance0.4640 0.8821 1.3541It is noticeable in Figure 4 that temperature varies sharply in one-dimensional space. Furthermore, it seems that temperature will vary more sharply in three-dimension space. Thus it is so difficult to keep temperature throughout the bathtub that we have to take some strategies.Based on the above discussion, we simplify the four dimensional partial differential equation to an ordinary differential equation. Thus, we take the first strategy that make some motion to meet the requirement of homogenization of temperature, that is 10F =.ResultsTherefore, in order to meet the objective function, water temperature at any point in the bathtub needs to be same as far as possible. We can resort to some strategies to make the temperature of bathtub water homogenized, which is (,,)x y z ∀∈∀. That is,()(),,,T x y z t T t =Given these conditions, we improve the basic model as temperature does not change with space.112213312()()()()/()p r H S dT H S T T H S T T c v T T c V V dt D μρρ∞⎡⎤=++-+-+--⎢⎥⎣⎦(9) Where● 1μis the intensity of people’s movement ;● 3H is convection between water and people;● 3S is contact area between water and people;● p T is body surface temperature;● 1V is the volume of the bathtub;● 2V is the volume of people.Where the μ refers to the intensity of people ’s movement. It is a constant. However , it is a random variable in reality, which will be taken into consideration in the following.Model T estingWe use the oval-shaped bathtub to test our model. According to the actual situation, we give initial values as follows:0.19λ=,0.03D =,20.54H =,25T ∞=,040T =00.20.40.60.8125303540Time T e m p e r a t u r eFigure 5. Basic modelThe Figure 5 shows that the temperature decreases monotonously with time. And some signs of a slowing down in the rate of decrease are evident in the picture. Reaching about two hours, the water temperature does not change basically and be closely to the room temperature. Obviously , it is in line with the actual situation, indicating the rationality of this model.ConclusionOur model is robust under reasonable conditions, as can be seen from the testing above. In order to keep the temperature even throughout the bathtub, we should take some strategies like stirring constantly while adding hot water to the tub. Most important of all, this is the necessary premise of the following question.Strategy 1 – Fully adapted to the hot water in the tubInfluence of body surface temperatureWe select a set of parameters to simulate two kinds of situation separately.The first situation is that do not involve the factor of human1122()()/H S dT H S T T cV dt D ρ∞⎡⎤=+-⎢⎥⎣⎦(10) The second situation is that involves the factor of human112213312()()()/()p H S dT H S T T H S T T c V V dt D μρ∞⎡⎤=++-+--⎢⎥⎣⎦(11) According to the actual situation, we give specific values as follows, and draw agraph of temperature of two functions.33p T =,040T =204060801001201401601803838.53939.540TimeT e m p e r a t u r eWith body Without bodyFigure 6a. Influence of body surface temperature50010001500200025003000350025303540TimeT e m p e r a t u r eWith body Without bodyCoincident pointFigure 6b. Influence of body surface temperatureThe figure 6 shows the difference between two kinds of situation in the early time (before the coincident point ), while the figure 7 implies that the influence of body surface temperature reduces as time goes by . Combing with the degree of comfort ofbath and the factor of health, we propose the second optimization strategy: Fully adapted to the hot water after getting into the bathtub.Strategy 2 –Adding water intermittentlyInfluence of adding methods of waterThere are two kinds of adding methods of water. One is the continuous; the other is the intermittent. We can use both different methods to add hot water.1122112()()()/()r H S dT H S T T c v T T c V V dt D μρρ∞⎡⎤=++-+--⎢⎥⎣⎦(12) Where r T is the temperature of the hot water.To meet .3O , we calculated the minimum water consumption by changing the flow rate of hot water. And we compared the minimum water consumptions of the continuous with the intermittent to determine which method is better.A . Adding water continuouslyAccording to the actual situation, we give specific values as follows and draw a picture of the change of temperature.040T =, 37d T =, 45r T =5001000150020002500300035003737.53838.53939.54040.5TimeT e m p e r a t u r eadd hot waterFigure 7. Adding water continuouslyIn most cases, people are used to have a bath in an hour. Thus we consumed that deadline of the bath: 3600final t =. Then we can find the best strategy in Figure 5 which is listed in Table 2.T able 3Strategy of adding water continuouslystart t final tt ∆ vr T varianceWater flow 4 min 1 hour56 min537.410m s -⨯45℃31.8410⨯0.2455 3mB . Adding water intermittentlyMaintain the values of 0T ,d T ,r T ,v , we change the form of adding water, and get another graph.5001000150020002500300035003737.53838.53939.540TimeT e m p e r a t u r et1=283(turn on)t3=2107(turn on)t2=1828(turn off)Figure 8. Adding water intermittentlyT able 4.Strategy of adding water intermittently()1t on ()2t off 3()t on vr T varianceWater flow 5 min 30 min35min537.410m s -⨯45℃33.610⨯0.2248 3mConclusionDifferent methods of adding water can influence the variance, water flow and the times of switching. Therefore, we give heights to evaluate comprehensively the methods of adding hot water on the basis of different hobbies of people. Then we build the following model:()()()2213600210213i i n t t i F T t T dtF v t dtF n -=⎧=-⎪⎪⎪=⎨⎪⎪=⎪⎩⎰∑⎰ (13) ()112233min F w F w F w F =++ (14)12123min ..510mini i t s t t t +>⎧⎨≤-≤⎩Evaluation on StrategiesFor example: Given a set of parameters, we choose different values of v and d T , and gain the results as follows.Method 1- AHPStep 1:Establish hierarchy modelFigure 9. Establish hierarchy modelStep 2: Structure judgment matrix153113511133A ⎡⎤⎢⎥⎢⎥=⎢⎥⎢⎥⎢⎥⎣⎦Step 3: Assign weight1w 2w3w 0.650.220.13Method 2-TopsisStep1 :Create an evaluation matrix consisting of m alternatives and n criteria, with the intersection of each alternative and criteria given as ij x we therefore have a matrixStep2:The matrix ij m n x ⨯()is then normalised to form the matrix ij m n R r ⨯=(), using thenormalisation method21r ,1,2,,;1,2,ijij mij i x i n j m x====∑…………,Step3:Calculate the weighted normalised decision matrix()(),1,2,,ij j ij m n m nT t w r i m ⨯⨯===⋅⋅⋅where 1,1,2,,nj j jj w W Wj n ===⋅⋅⋅∑so that11njj w==∑, and j w is the original weight given to the indicator,1,2,,j v j n =⋅⋅⋅.Step 4: Determine the worst alternative ()w A and the best alternative ()b A()(){}{}()(){}{}max 1,2,,,min 1,2,,1,2,,n ,min 1,2,,,max 1,2,,1,2,,n ,w ij ij wjbijij bjA t i m j J t i m j J t j A t i m j J t i m j J tj -+-+==∈=∈====∈=∈==where, {}1,2,,J j n j +==⋅⋅⋅ associated with the criteria having a positive impact, and {}1,2,,J j n j -==⋅⋅⋅associated with the criteria having a negative impact. Step 5: Calculate the L2-distance between the target alternative i and the worst condition w A()21,1,2,,m niw ij wj j d tt i ==-=⋅⋅⋅∑and the distance between the alternative i and the best condition b A()21,1,2,,m nib ij bj j d t t i ==-=⋅⋅⋅∑where iw d and ib d are L2-norm distances from the target alternative i to the worst and best conditions, respectively .Step 6 :Calculate the similarity to the worst condition Step 7 : Rank the alternatives according to ()1,2,,iw s i m =⋅⋅⋅ Step 8 : Assign weight1w2w 3w 0.55 0.170.23ConclusionAHP gives height subjectively while TOPSIS gives height objectively. And the heights are decided by the hobbies of people. However, different people has different hobbies, we choose AHP to solve the following situations.Impact of parametersDifferent customers have their own hobbies. Some customers prefer enjoying in the bath, so the .2O is more important . While other customers prefer saving water, the .3O is more important. Therefore, we can solve the problem on basis of APH . 1. Customers who prefer enjoying: 20.83w =,30.17w =According to the actual situation, we give initial values as follows:13S =,11V =,2 1.4631S =,20.05V =,33p T =,110μ=Ensure other parameters unchanged, then change the values of these parameters including 1S ,1V ,2S ,2V ,d T ,1μ. So we can obtain the optimal strategies under different conditions in Table 4.T able 5.Optimal strategies under different conditions2.Customers who prefer saving: 20.17w =,30.83w =Just as the former, we give the initial values of these parameters including1S ,1V ,2S ,2V ,d T ,1μ, then change these values in turn with other parameters unchanged. So we can obtain the optimal strategies as well in these conditions.T able 6.Optimal strategies under different conditionsInfluence of bubbleUsing the bubble bath additives is equivalent to forming a barrier between the bath water and air, thereby slowing the falling velocity of water temperature. According to the reality, we give the values of some parameters and gain the results as follows:5001000150020002500300035003334353637383940TimeT e m p e r a t u r eWithour bubbleWith bubbleFigure 10. Influence of bubbleT able 7.Strategies (influence of bubble)Situation Dropping rate of temperature (the larger the number, the slower)Disparity to theinitial temperatureWater flow Times of switchingWithout bubble 802 1.4419 0.1477 4 With bubble 34499.85530.01122The Figure 10 and the Table 7 indicates that adding bubble can slow down the dropping rate of temperature effectively . It can decrease the disparity to the initial temperature and times of switching, as well as the water flow.Improved ModelIn reality , human ’s motivation in the bathtub is flexible, which means that the parameter 1μis a changeable measure. Therefore, the parameter can be regarded as a random variable, written as ()[]110,50t random μ=. Meanwhile, the surface of water will come into being ripples when people moves in the tub, which will influence the parameters like 1S and 2S . So, combining with reality , we give the range of values as follows:()[]()[]111222,1.1,1.1S t random S S S t random S S ⎧=⎪⎨=⎪⎩Combined with the above model, the improved model is given here:()[]()[]()[]11221121111222()()()/()10,50,1.1,1.1a H S dT H S T T c v T T c V V dt D t random S t random S S S t random S S μρρμ∞⎧⎡⎤=++-+--⎪⎢⎥⎣⎦⎨⎪===⎩(15)Given the values, we can get simulation diagram:050010001500200025003000350039.954040.0540.140.15TimeT e m p e r a t u r eFigure 11. Improved modelThe figure shows that the variance is small while the water flow is large, especially the variance do not equals to zero. This indicates that keeping the temperature of water is difficult though we regard .2O as the secondary objective.Sensitivity AnalysisSome parameters have a fixed value throughout our work. By varying their values, we can see their impacts.Impact of the shape of the tub0.70.80.91 1.1 1.2 1.3 1.433.23.43.63.84Superficial areaT h e t i m e sFigure 12a. Times of switching0.70.80.91 1.11.21.31.43890390039103920393039403950Superficial areaV a r i a n c eFigure 12b. V ariance of temperature0.70.80.91 1.1 1.2 1.3 1.40.190.1950.20.2050.21Superficial areaW a t e r f l o wFigure 12c. Water flowBy varying the value of some parameters, we can get the relationships between the shape of tub and the times of switching, variance of temperature, and water flow et. It is significant that the three indexes will change as the shape of the tub changes. Therefore the shape of the tub makes an obvious effect on the strategies. It is a sensitive parameter.Impact of the volume of the tub0.70.80.91 1.1 1.2 1.3 1.4 1.533.544.55VolumeT h e t i m e sFigure 13a. Times of switching。
2017全国数学建模优秀论文
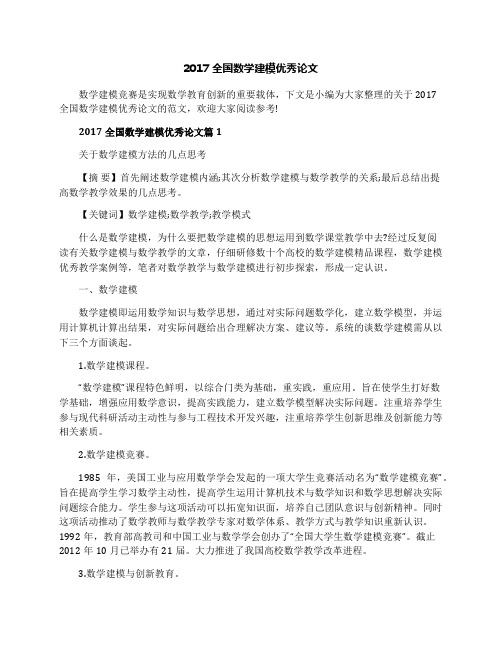
2017全国数学建模优秀论文数学建模竞赛是实现数学教育创新的重要载体,下文是小编为大家整理的关于2017全国数学建模优秀论文的范文,欢迎大家阅读参考!2017全国数学建模优秀论文篇1关于数学建模方法的几点思考【摘要】首先阐述数学建模内涵;其次分析数学建模与数学教学的关系;最后总结出提高数学教学效果的几点思考。
【关键词】数学建模;数学教学;教学模式什么是数学建模,为什么要把数学建模的思想运用到数学课堂教学中去?经过反复阅读有关数学建模与数学教学的文章,仔细研修数十个高校的数学建模精品课程,数学建模优秀教学案例等,笔者对数学教学与数学建模进行初步探索,形成一定认识。
一、数学建模数学建模即运用数学知识与数学思想,通过对实际问题数学化,建立数学模型,并运用计算机计算出结果,对实际问题给出合理解决方案、建议等。
系统的谈数学建模需从以下三个方面谈起。
1.数学建模课程。
“数学建模”课程特色鲜明,以综合门类为基础,重实践,重应用。
旨在使学生打好数学基础,增强应用数学意识,提高实践能力,建立数学模型解决实际问题。
注重培养学生参与现代科研活动主动性与参与工程技术开发兴趣,注重培养学生创新思维及创新能力等相关素质。
2.数学建模竞赛。
1985年,美国工业与应用数学学会发起的一项大学生竞赛活动名为“数学建模竞赛”。
旨在提高学生学习数学主动性,提高学生运用计算机技术与数学知识和数学思想解决实际问题综合能力。
学生参与这项活动可以拓宽知识面,培养自己团队意识与创新精神。
同时这项活动推动了数学教师与数学教学专家对数学体系、教学方式与教学知识重新认识。
1992年,教育部高教司和中国工业与数学学会创办了“全国大学生数学建模竞赛”。
截止2012年10月已举办有21届。
大力推进了我国高校数学教学改革进程。
3.数学建模与创新教育。
创新教育是现代教育思想的灵魂。
数学建模竞赛是实现数学教育创新的重要载体。
如2012年A题,葡萄酒的评价中,要求学生对葡萄酒原料与酿造、储存于葡萄酒色泽、口味等有全面认识;而2012年D题,机器人行走避障问题,要求学生了解对机器人行走特点;2008年B题,乘公交看奥运,要求学生了解公交换乘系统。
2017数学建模获奖论文

2017数学建模获奖论文在我国倡导素质教育的今天,数学建模受到的关注与日俱增。
数学建模已成为国际、国内数学教育中稳定的内容和热点之一。
下文是店铺为大家搜集整理的关于2017数学建模获奖论文的内容,欢迎大家阅读参考!2017数学建模获奖论文篇1浅谈初中生数学建模能力的培养摘要:在中学数学教学中,加强数学建模能力的培养有助于数学应用意识的渗透,培养学生用数学解决实际问题的能力。
关键词:数学建模能力在中学数学教学中,加强数学建模能力的培养有助于数学应用意识的渗透,培养学生用数学解决实际问题的能力。
那么,如何培养初中生数学的建模能力呢?一、初中生数学建模能力培养的意义。
根据数学建模的特点,在初中数学教学中,渗透建模思想,开展建模活动,具有重要意义。
1、促进理论与实践相结合,培养学生应用数学的意识。
数学建模的过程,是实践―理论―实践的过程,是理论与实践的有机结合。
强化数学建模的教学,不仅能使学生更好地掌握数学基础知识,学会数学的思想、方法、语言,也是为了学生树立正确的数学观,增强应用数学的意识,全面认识数学及其与科学、技术、社会的关系,提高分析问题和解决问题的能力。
2、培养学生的能力。
数学建模的教学体现了多方面能力的培养:①翻译能力,能将实际问题用数学语言表达出来,建立数学模型,并能把数学问题的解用一般人所能理解的非数学语言表达出来;②运用数学能力;③交流合作能力;④创造能力。
3、发挥了学生的参与意识,体现了学生的主体性。
根据现代建构主义学习观,知识不能简单地由教师或其他人传授给学生,而只能由学生依据自身已有的知识和经验主动地加以建构。
所以数学建模的教学,符合现代教学理念,必将有助于教学质量的提高。
二、数学建模思想培养的基本原则在课堂设计方面,数学建模教学要遵循下列教学设计原则:1、所有的学习活动都应该与教学的任务或目标挂钩。
也就是说,学习活动应带有明确的目的性,学以致用。
2、把支持学习者发掘问题作为学习活动的刺激物,使学习成为自愿的事,而不是强加给他们学习目标和以通过测试为目的。
2017年美赛论文C题M奖中文国际一等奖
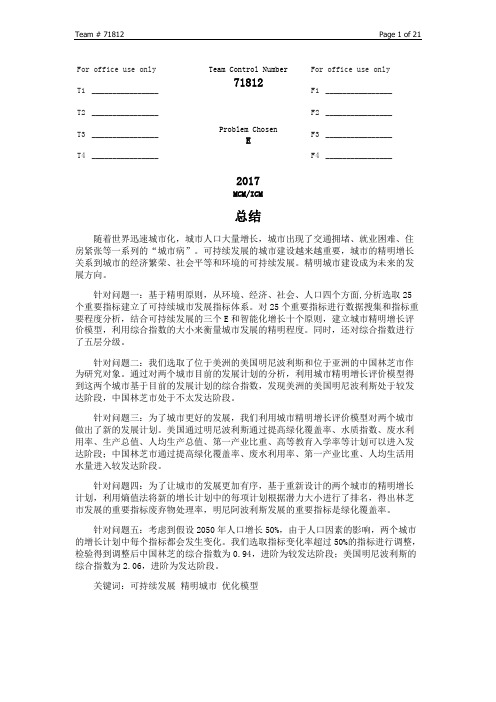
For office use only T1________________ T2________________ T3________________ T4________________ Team Control Number71812Problem ChosenEFor office use onlyF1________________F2________________F3________________F4________________ 2017MCM/ICM总结随着世界迅速城市化,城市人口大量增长,城市出现了交通拥堵、就业困难、住房紧张等一系列的“城市病”。
可持续发展的城市建设越来越重要,城市的精明增长关系到城市的经济繁荣、社会平等和环境的可持续发展。
精明城市建设成为未来的发展方向。
针对问题一:基于精明原则,从环境、经济、社会、人口四个方面,分析选取25个重要指标建立了可持续城市发展指标体系。
对25个重要指标进行数据搜集和指标重要程度分析,结合可持续发展的三个E和智能化增长十个原则,建立城市精明增长评价模型,利用综合指数的大小来衡量城市发展的精明程度。
同时,还对综合指数进行了五层分级。
针对问题二:我们选取了位于美洲的美国明尼波利斯和位于亚洲的中国林芝市作为研究对象。
通过对两个城市目前的发展计划的分析,利用城市精明增长评价模型得到这两个城市基于目前的发展计划的综合指数,发现美洲的美国明尼波利斯处于较发达阶段,中国林芝市处于不太发达阶段。
针对问题三:为了城市更好的发展,我们利用城市精明增长评价模型对两个城市做出了新的发展计划。
美国通过明尼波利斯通过提高绿化覆盖率、水质指数、废水利用率、生产总值、人均生产总值、第一产业比重、高等教育入学率等计划可以进入发达阶段;中国林芝市通过提高绿化覆盖率、废水利用率、第一产业比重、人均生活用水量进入较发达阶段。
针对问题四:为了让城市的发展更加有序,基于重新设计的两个城市的精明增长计划,利用熵值法将新的增长计划中的每项计划根据潜力大小进行了排名,得出林芝市发展的重要指标废弃物处理率,明尼阿波利斯发展的重要指标是绿化覆盖率。
建模美赛获奖范文
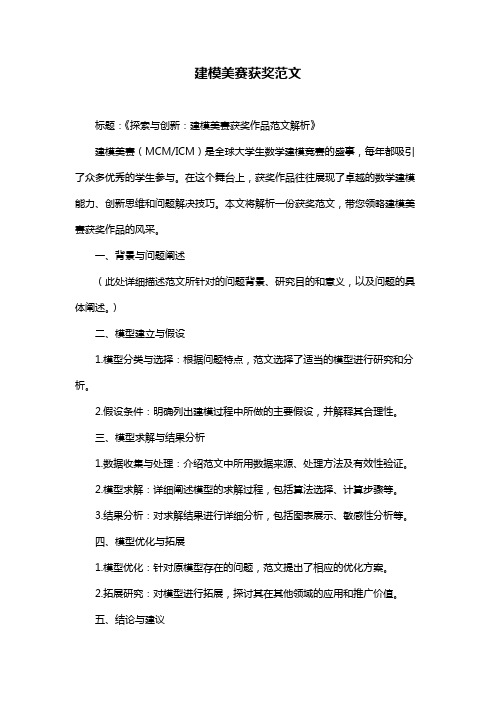
建模美赛获奖范文标题:《探索与创新:建模美赛获奖作品范文解析》建模美赛(MCM/ICM)是全球大学生数学建模竞赛的盛事,每年都吸引了众多优秀的学生参与。
在这个舞台上,获奖作品往往展现了卓越的数学建模能力、创新思维和问题解决技巧。
本文将解析一份获奖范文,带您领略建模美赛获奖作品的风采。
一、背景与问题阐述(此处详细描述范文所针对的问题背景、研究目的和意义,以及问题的具体阐述。
)二、模型建立与假设1.模型分类与选择:根据问题特点,范文选择了适当的模型进行研究和分析。
2.假设条件:明确列出建模过程中所做的主要假设,并解释其合理性。
三、模型求解与结果分析1.数据收集与处理:介绍范文中所用数据来源、处理方法及有效性验证。
2.模型求解:详细阐述模型的求解过程,包括算法选择、计算步骤等。
3.结果分析:对求解结果进行详细分析,包括图表展示、敏感性分析等。
四、模型优化与拓展1.模型优化:针对原模型存在的问题,范文提出了相应的优化方案。
2.拓展研究:对模型进行拓展,探讨其在其他领域的应用和推广价值。
五、结论与建议1.结论总结:概括范文的研究成果,强调其创新点和贡献。
2.实践意义:分析建模结果在实际问题中的应用价值和意义。
3.建议:针对问题解决,提出具体的建议和措施。
六、获奖亮点与启示1.创新思维:范文在模型选择、求解方法等方面展现出创新性。
2.严谨论证:文章结构清晰,逻辑严密,数据充分,论证有力。
3.团队合作:建模美赛强调团队协作,范文体现了成员间的紧密配合和分工合作。
总结:通过分析这份建模美赛获奖范文,我们可以学到如何从问题背景出发,建立合理的模型,进行严谨的求解和分析,以及如何优化和拓展模型。
同时,也要注重创新思维和团队合作,才能在建模美赛中脱颖而出。
2017年全国数学建模大赛获奖优秀论文(2)
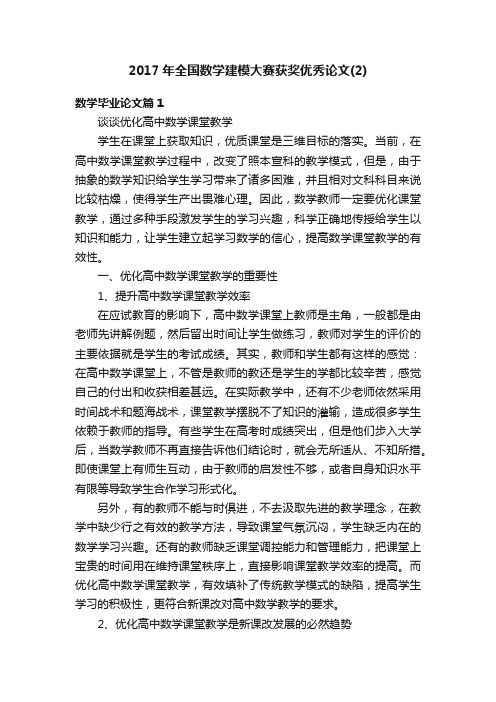
2017年全国数学建模大赛获奖优秀论文(2)数学毕业论文篇1谈谈优化高中数学课堂教学学生在课堂上获取知识,优质课堂是三维目标的落实。
当前,在高中数学课堂教学过程中,改变了照本宣科的教学模式,但是,由于抽象的数学知识给学生学习带来了诸多困难,并且相对文科科目来说比较枯燥,使得学生产出畏难心理。
因此,数学教师一定要优化课堂教学,通过多种手段激发学生的学习兴趣,科学正确地传授给学生以知识和能力,让学生建立起学习数学的信心,提高数学课堂教学的有效性。
一、优化高中数学课堂教学的重要性1、提升高中数学课堂教学效率在应试教育的影响下,高中数学课堂上教师是主角,一般都是由老师先讲解例题,然后留出时间让学生做练习,教师对学生的评价的主要依据就是学生的考试成绩。
其实,教师和学生都有这样的感觉:在高中数学课堂上,不管是教师的教还是学生的学都比较辛苦,感觉自己的付出和收获相差甚远。
在实际教学中,还有不少老师依然采用时间战术和题海战术,课堂教学摆脱不了知识的灌输,造成很多学生依赖于教师的指导。
有些学生在高考时成绩突出,但是他们步入大学后,当数学教师不再直接告诉他们结论时,就会无所适从、不知所措。
即使课堂上有师生互动,由于教师的启发性不够,或者自身知识水平有限等导致学生合作学习形式化。
另外,有的教师不能与时俱进,不去汲取先进的教学理念,在教学中缺少行之有效的教学方法,导致课堂气氛沉闷,学生缺乏内在的数学学习兴趣。
还有的教师缺乏课堂调控能力和管理能力,把课堂上宝贵的时间用在维持课堂秩序上,直接影响课堂教学效率的提高。
而优化高中数学课堂教学,有效填补了传统教学模式的缺陷,提高学生学习的积极性,更符合新课改对高中数学教学的要求。
2、优化高中数学课堂教学是新课改发展的必然趋势优化高中数学课堂教学是新课改的要求,也是构建高效课堂的保障。
高中数学课堂教学并不是一个独立的个体,有着丰富的内涵。
在新课改背景下,需要改革的内容多种多样,除了创新教学内容和教学目标以外,最主要是就是改革课堂教学模式。
2017全国研究生数学建模竞赛优秀论文发表

2017全国研究生数学建模竞赛优秀论文发表现如今,数学建模学科竞赛可以锻炼学生创新能力、团队合作,越来越受到高校的重视。
下文是店铺为大家整理的关于数学建模竞赛优秀论文的范文,希望能对大家有所帮助,欢迎大家阅读参考!数学建模竞赛优秀论文篇1浅谈研究生数学建模的特点与培训策略摘要:数学建模就是用数学语言描述实际现象的一个过程。
是一种数学的思考方法,是运用数学的语言和方法,通过抽象、假设、简化建立能近似刻画并解决实际问题的一种强有力的数学手段。
本文结合教学实践,结合建模的特点,对数学建模的课程的教学改革提出几点建议。
关键词:数学建模;数学语言;教学改革全国研究生数学建模竞赛是针对当前全国在读研究生的竞赛活动,主要是激发研究生对生活实际的创新同时提高研究生的学习兴趣,提高学生对于与数学模型的建立和通过运用计算机对实际问题进行解决的综合能力,拓展学生的知识面,培养大家的团队合作意识和对事物的创新精神,从而使优秀的学生能够在过程中通过实践脱颖而出,迅速地成长起来。
推动研究生教育改革,能够更好地增进学校与学校之间的友谊关系。
从2004年起开始举办以来,我校参加了历次竞赛,均取得了优秀的成绩,这项竞赛在我校研究生中的影响力越来越大,在广大研究生中也打下了扎实基础。
该活动已经成为我校一项重要的课外活动之一,也成为研究生培养阶段的一个重要实践环节。
一、数学建模的概念数学建模是利用数学方法解决实际问题的一种实践方式。
通过抽象、简化、假设、引进变量等途径将实际问题用数学的方式表达出来。
建立数学模型,运用数学方法和先进的计算机技术对实际问题进行解答。
二、研究生数学建模的特点我国的大学生数学建模竞赛是从1992年开始的,分析20多年来的赛题可以发现,这些赛题虽然来自于实际问题,但这些问题经过命题人和全国组委会的研讨和加工后,距离真正的数学问题已经很接近了,需要学生事先做的假设并不是很多。
由于大多数命题人都是数学老师,尽管赛题具有一定的实际背景,但赛题本身所包含的专业知识不是很多,对于本科生而言,读懂赛题需要的时间并不是很多。
2017数学建模优秀论文(2)
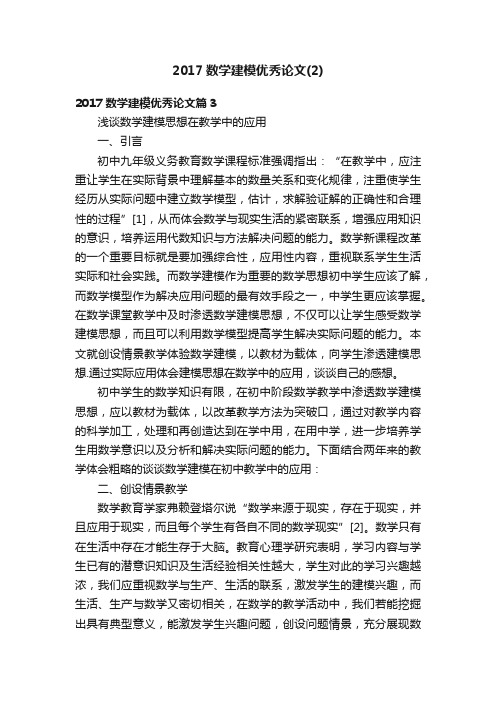
2017数学建模优秀论文(2)2017数学建模优秀论文篇3浅谈数学建模思想在教学中的应用一、引言初中九年级义务教育数学课程标准强调指出:“在教学中,应注重让学生在实际背景中理解基本的数量关系和变化规律,注重使学生经历从实际问题中建立数学模型,估计,求解验证解的正确性和合理性的过程”[1],从而体会数学与现实生活的紧密联系,增强应用知识的意识,培养运用代数知识与方法解决问题的能力。
数学新课程改革的一个重要目标就是要加强综合性,应用性内容,重视联系学生生活实际和社会实践。
而数学建模作为重要的数学思想初中学生应该了解,而数学模型作为解决应用问题的最有效手段之一,中学生更应该掌握。
在数学课堂教学中及时渗透数学建模思想,不仅可以让学生感受数学建模思想,而且可以利用数学模型提高学生解决实际问题的能力。
本文就创设情景教学体验数学建模,以教材为载体,向学生渗透建模思想.通过实际应用体会建模思想在数学中的应用,谈谈自己的感想。
初中学生的数学知识有限,在初中阶段数学教学中渗透数学建模思想,应以教材为载体,以改革教学方法为突破口,通过对教学内容的科学加工,处理和再创造达到在学中用,在用中学,进一步培养学生用数学意识以及分析和解决实际问题的能力。
下面结合两年来的教学体会粗略的谈谈数学建模在初中教学中的应用:二、创设情景教学数学教育学家弗赖登塔尔说“数学来源于现实,存在于现实,并且应用于现实,而且每个学生有各自不同的数学现实”[2]。
数学只有在生活中存在才能生存于大脑。
教育心理学研究表明,学习内容与学生已有的潜意识知识及生活经验相关性越大,学生对此的学习兴趣越浓,我们应重视数学与生产、生活的联系,激发学生的建模兴趣,而生活、生产与数学又密切相关,在数学的教学活动中,我们若能挖掘出具有典型意义,能激发学生兴趣问题,创设问题情景,充分展现数学的应用价值,就能激发学生的求知欲。
三、课内外相结合初中九年级义务教育数学课程标准强调指出:强调数学与生活经验的联系(实践性);强调学生主体化的活动;突出学生的主体性,强调了综合应用(综合应用的含义—不是围绕知识点来进行的,而是综合运用知识来解决问题的)[3]。
美赛论文mcm模版

Number of People Entered Shanghai World Expo ParkAbstract:Key words:Contents1. Introduction (3)1.1 Why does toll way collects toll? (3)1.2 Toll modes (3)1.3 Toll collection methods (3)1.4 Annoyance in toll plazas (3)1.5 The origin of the toll way problem (3)1.6 Queuing theory (4)2. The Description of Problem (5)2.1 How do we approximate the whole course of paying toll? (5)2.2 How do we define the optimal configuration? (5)2.2.1 From the perspective of motorist (5)2.2.2 From the perspective of the toll plaza (6)2.2.3 Compromise (6)2.3 Overall optimization and local optimization (6)2.4 The differences in weights and sizes of vehicles (7)2.5 What if there is no data available? (7)3. Models (7)3.1 Basic Model (7)3.1.1 Symbols and Definitions (7)3.1.2 Assumptions (8)3.1.3 The Foundation of Model (9)3.1.4 Solution and Result (11)3.1.5 Analysis of the Result (11)3.1.6 Strength and Weakness (13)3.2 Improved Model (14)3.2.1 Extra Symbols (14)3.2.2 Additional Assumptions (14)3.2.3 The Foundation of Model (14)3.2.4 Solution and Result (15)3.2.5 Analysis of the Result (18)3.2.6 Strength and Weakness (19)4. Conclusions (19)4.1 Conclusions of the problem (19)4.2 Methods used in our models (19)4.3 Application of our models (19)5. Future Work (19)5.1 Another model (19)5.2 Another layout of toll plaza (23)5.3 The newly- adopted charging methods (23)6.References (23)7.Appendix (23)Programs and codes (24)I. IntroductionIn order to indicate the origin of the toll way problems, the following background is worth mentioning.1.11.21.31.41.51.6II. The Description of the Problem2.1 How do we approximate the whole course of paying toll?●●●●2.2 How do we define the optimal configuration?1) From the perspective of motorist:2) From the perspective of the toll plaza:3) Compromise:2.3 The local optimization and the overall optimization●●●Virtually:2.4 The differences in weights and sizes of vehicles2.5 What if there is no data available?III. Models3.1 Basic Model3.1.1 Terms, Definitions and SymbolsThe signs and definitions are mostly generated from queuing theory.●●●●●3.1.2 Assumptions●●●●●3.1.3 The Foundation of Model1) The utility function●The cost of toll plaza:●The loss of motorist:●The weight of each aspect:●Compromise:2) The integer programmingAccording to queuing theory, we can calculate the statistical properties as follows.3)The overall optimization and the local optimization●The overall optimization:●The local optimization:●The optimal number of tollbooths:3.1.4 Solution and Result1) The solution of the integer programming:2) Results:3.1.5 Analysis of the Result●Local optimization and overall optimization:●Sensitivity: The result is quite sensitive to the change of the threeparameters●Trend:●Comparison:3.1.6 Strength and Weakness●Strength: In despite of this, the model has proved that . Moreover, wehave drawn some useful conclusions about . T he model is fit for, such as●Weakness: This model just applies to . As we have stated, . That’sjust what we should do in the improved model.3.2 Improved Model3.2.1 Extra SymbolsSigns and definitions indicated above are still valid. Here are some extra signs and definitions.●●●●3.2.2 Additional Assumptions●●●Assumptions concerning the anterior process are the same as the Basic Model.3.2.3 The Foundation of Model1) How do we determine the optimal number?As we have concluded from the Basic Model,3.2.4 Solution and Result1) Simulation algorithmBased on the analysis above, we design our simulation arithmetic as follows.●Step1:●Step2:●Step3:●Step4:●Step5:●Step6:●Step7:●Step8:●Step9:2) Flow chartThe figure below is the flow chart of the simulation.3) Solution3.2.5 Analysis of the Result3.2.6 Strength and Weakness●Strength: The Improved Model aims to make up for the neglect of .The result seems to declare that this model is more reasonable than the Basic Model and much more effective than the existing design.●Weakness: . Thus the model is still an approximate on a large scale. Thishas doomed to limit the applications of it.IV. Conclusions4.1 Conclusions of the problem●●●4.2 Methods used in our models●●●4.3 Applications of our models●●●V. Future Work5.1 Another model5.1.1The limitations of queuing theory 5.1.25.1.35.1.41)●●●●2)●●●3)●●●4)5.2 Another layout of toll plaza5.3 The newly- adopted charging methodsVI. References[1][2][3][4]VII. Appendix。
美赛论文
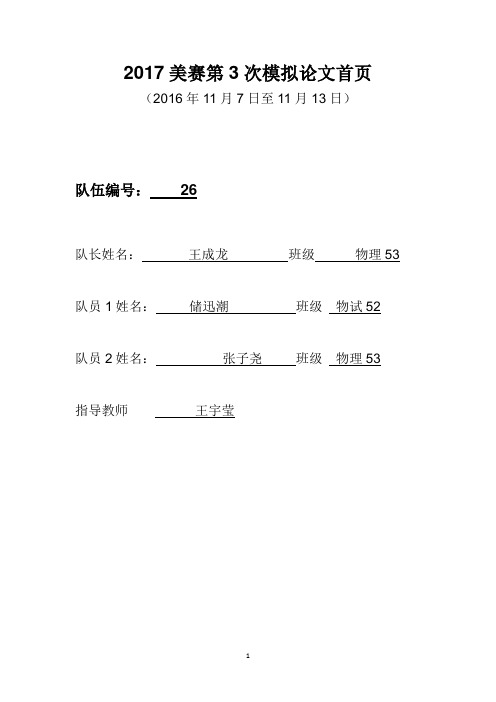
2017美赛第3次模拟论文首页(2016年11月7日至11月13日)队伍编号:26队长姓名:王成龙班级物理53 队员1姓名:储迅潮班级物试52队员2姓名:张子尧班级物理53指导教师王宇莹For office use onlyT1________________ T2________________ T3________________ T4________________ Team Control Number26Problem ChosenFor office use onlyF1________________F2________________F3________________F4________________2017 Mathematical Contest in Modeling (MCM或ICM) Summary Sheet(Attach a copy of this page to your solution paper.)A Fair System of School Education EvaluationSummaryOur goal is a model that can evaluate the performance of the teachers as there exist many non-teaching factors affecting the qualities of teachers. We construct models to analysis the relation between non-teaching factors and the final cases, calculate the qualities of teachers and exam the validity of our models respectivelyOur Control Variable model focuses on the relation between each non-teaching factors and the final scores. We carefully assign each factors reasonably, and examine the trend between each independent variables and the final scores by averaging the teaching-factors and removing other non-teaching factors.After determine the related variable in non-teaching factors by doing analysis qualitatively, a quantitative formula is essential for getting a accurate value to each teachers. By using Linear Regression model and Principle Component Estimate model, we can easily get a equation consisting of non-teaching factors, teaching factors and the final scores. And it is fair and logical to set teaching factors as the qualities of teachers which can remove the non-teaching factors effectively. Since then, a fair evaluation system is preliminary completed.“The improvement of teachers”will not be ignored as well. By controlling the variables, we get the relation between teaching time and final scores for different professional titles. By average the date in each time, we build parameters as “average addition”. Comparing the addition with average addition is also a measure to evaluate teachers, and we add it into the system above.We also do sensitivity analysis for our models and choose the student numbers and final scores to examine, and it shows that our models are not sensitive at all with the 15% of the disturbance.Additionally we divide the score into 4 levels. By doing Sequencing Logistics Analysis, we make this system more useful and by examining 40 cases, we confirm the validity of the former models.Contents1.Introduction1.1background and question1.2analysis of the queation2.Assumption and Justifiction3.Notations4.Model overviwe5.The Fair Evaluation System Model5.1The Control Variable Model5.2The Basic Linear Regression Model5.3The Principle Component Estimate Model6.Results: A fair system of school education evaluation6.1Confirm the Correction between Variables and Final Scores6.1.1The Relation between Class Size and Final Scores6.1.2 The Relation between Other Variables and Final Scores6.2Setting a Fair System of School Education Evaluation6.2.1 A Basic model reacting the Teaching Quality6.2.2 A Refined model majoring in Teachers Process7.Sensitivity Analysis8.Further Discussions9.Strengths and Weaknesses9.1Strengths9.2weaknessReference1. IntroductionTaking the scores of student intentions is a usual measure to evaluate the qualities of the teachers nowadays. While some non-teaching factors, like the student number of class, the title and gender of the teachers and some others will affect the final scores in reality. For example, a teacher works in a similar level for two class, the number of students in class A is 10 and in class B is 100. It is evident that he will get a higher score in class A than B, for the students in the latter can solve their questions facilely through communicating with teacher directly. For this reason, setting a new evaluation system which will get rid of the non-teaching factors to calculate the quality of teachers is obliged.1.1 Background of this questionWith the development of the education, most of universities focus on setting a fair system to evaluate teachers. We also find some papers of this topic such as <The exploration and analysis of the education evaluation in our country> and <The build of the teacher evaluation basing on the modern education idea>. We can get the idea that a fair education which consists many factors is not easy to get and for this reason, these papers only give us some misty measure, which do not contribute to the quantitative calculation.1.2 Analysis of the questionAs there exist some non-teaching factors affecting the final scores, we are supported to analysis the relationship among the non-teaching factors, teaching factors and the final scores,that is to say our first step is to confirm the non-teaching factors which have been given.After fulfill this work, we will build a function described by non-teaching factors, teaching factors and the final scores as()()()nonteacherF=factors-+finalscoresGTteachingfactorsIt is illogical to take all factors into consideration to evaluate a teacher since different teachers should face complex non-teaching factors, and it is unfair to compare a teacher who leads a bad class to another who leads a wally one. Combining the function above with the reality, we select the teaching factors as the criterion to evaluate the quality of teachers, and we can get the new function clearly as()()()teachersquailtyG-=T-factorsteachingnonFscoresfinalAnother factor should be focused as “the improvement of the teachers ”. Assume that teacher will improve his final scores with time going on, compare his improvement with the average value in the same title, and it can be a measure to evaluate a teacher.2. Assumption and JustificationTo simplify the problem, we take the following basic assumption, each of which is properly justified.·Every data is referable while there exist some cases can be removed. In order to make a liner regression model, we must delete individual cases to simulate the model better.·Non-teaching factors only include the variables given in the data form. Surveys only contain these terms, so we will only investigate the relationship between these terms and the final scores.3. NotationsAll the variables and constants used in this paper are listed in Table 14. Model OverviewFor the question “determine the non-teaching factors ”, as the final scores consist of both non-teaching factors and teaching factors while the latter one has not beendetermined, we are required to use Control Variable model analysis to average the teaching factors and then remove this variable in order to do qualitative analysis for the first step.Further, we ought to do quantitative calculations for the teaching factors(teaching quality) so that we can get a scores as the qualities of the teacher by using Linear Regression model and Principle Component Estimate model.Meanwhile, we select “the improvement of the teacher”as an independent significant term to evaluate the teachers By using Control Variable model, we can obtain the average improvement in one year for each titles, and then compare the one’s improvement with the average value, we can easily evaluate him.5. The Fair Evaluation System ModelWe will start with the idea of the basic model. Next we present the Control Variable model, Linear Regression model and Principle Component Estimate model, then explain the algorithm. Finally, we introduce our additional work in the refined model.5.1 The Control Variable ModelThe final scores consist of two parameters as non-teaching factors and teaching factors. When an important parameter teaching factors is not given in the form, we are required to adopt Variable Control for independent variables, so that we can divide the data into groups and average the teaching factors for each group. Further, since the indeterminate parameter teaching factors can be removed, we are capable of comparing only one variable in one group. Duplicate this work in the following procedures and plot the statistical data, then we will obtain the relation between the variables and the final score as shown in Figure 5.1Figure 5.1 The Flow Chart of Variable Control5.2 The Basic Linear Regression ModelSetting the final scores as a variable Y influenced by non-teaching variables X 1, X 2, X 3, X 4... and teaching variable Z , and here is a linear equation:,...3322110b Z a X a X a X a a Y n +++++=here,a n are indeterminate parameters; b is a mean value whose variance is c >0. Assumes that b~N(0,c), and this equation is a linear regression model. For the first step, we should estimate a n . And we can get that⎪⎪⎩⎪⎪⎨⎧+++++=+++++=+++++=------n p n p n n n p p p p b x a x a x a a y b x a x a x a a y b x a x a x a a y 1,12211021,212222110211,1112211101............Here b 1, b 2 ... b n are independent and obey the N(0,c) distribution. Set,...1..................1...1,...1,32311,222211,1121121⎥⎥⎥⎥⎥⎦⎤⎢⎢⎢⎢⎢⎣⎡=⎥⎥⎥⎥⎦⎤⎢⎢⎢⎢⎣⎡=---p n p p n x x x x x x x x x X y y y Y ....,...21121⎥⎥⎥⎥⎦⎤⎢⎢⎢⎢⎣⎡=⎥⎥⎥⎥⎥⎦⎤⎢⎢⎢⎢⎢⎣⎡=-n p c c c c b b b b and the equation can be simplified as :c Xb Y+=5.3 The Principle Component Estimate ModelIn order to get over the multiple collinearity of Least Square (LS ), we are supported to adopt the principle component estimate model to solve the question.Using principle component estimate model is to transform the basic independent variables into the new ones which names Principle Component (PC), and select part of the significant PC as the new independent variables, and estimate the parameters of this model by using LS. Finally we change back to the basic model and obtain the parameters.Image that there are non-teaching variable x 1,x 2,...,x p-1 and the final scores x p , they value x i1, x i2,... ,x ip in the i th experiment, set them as a matrix⎪⎪⎪⎪⎪⎭⎫⎝⎛=np n n p p x x x x x x x x x X (21)2222111211Take the Linear Regression model above, assume that X is standardized, then we can get∑===ni iY n Y b 101and for each linear combination of independent variable∑==+++=pj j p p c x c x c x c z 1222111,...Regard z as a new variable, and the value of z in i th experiment is),...,2,1(...2211n i x c x c x c z ip p i i =+++=Since X has been standardized, we can obtain that011111111====∑∑∑∑∑=====ni ijp j j nn i pj ijj nni inxc xc zzHere ()Tp c c c w ,...,,11=, then()()()()Xw Xw z z zTnni inni in11211212M ==-=∑∑==*The new variable z can be removed if *2M is not large; on the contrary, it will affect a lot.The eigenvalue of X X T ,...21P λλλ≥≥≥and their normalized orthogonal feature vectors are .,...,,p 21ηηηWhen 1η=w ,()()n Xw Xw M T /*2=will be the maximum while the new variable z is(),,...,2,1k i x z i T i ==ηWhen we come back the Linear Regression Model and transform p x into the principal component p z , then()⎪⎪⎪⎪⎪⎭⎫⎝⎛==np n n p p p z z zz z z z z z X Z ΛM M M ΛΛΛ21222211121121,,,ηηη here ()p 21,...,,ηηη=Q and XQ Z =, draw in a new parameter b Q T =α, soc Z b ZQ b Y T +++=α0Block the Q and α⎪⎪⎭⎫⎝⎛==2121),,(αααQ Q QThen the Principle Component Estimate of α is()T 0,ˆˆ1αα= And the Principle Component Estimate of β is11121ˆ0ˆ),(ˆααβQ Q Q =⎪⎪⎭⎫⎝⎛=6. Results: A fair system of school education evaluationTo set a fair system of school education, first we should do is to confirm the related variables. So we ought to divide this problem into two pieces.6.1 Confirm the Correction between Variables and Final ScoresFor the first step we digitized the variables with reality in Table, for the reason we can deal the question with models above as shown in Table 6.1Table 6.1 Variable AssignmentHere is a reasonable order:·For the title, professor > associate professor > assistance professor.·For the year and season, we set 2012 as the 1st year, spring as 0 and autumn as 0.5. ·For the gender, we value male as 1, female as 2, and this term is casual.·For the types of students, under postgraduate<undergraduate<MBA.·For the size of the class, we set 30 students, 60 students as the size boundary.After fulfilling the basic work, we started to analysis the relation between the final scores and the non-teaching factors with the Variable Control Model.6.1.1 The Relation between Class Size and Final ScoresOur survey towards the class size gives us the average result by controlling different variables, and then we plot the data into a bar chart in Figure 6.1Figure 6.1 All Class SizeBecause the relation is not apparent in the figure above, we choose to average the same size in each group, and here is the line chart which appears the trend as shown in Figure 6.2Figure 6.2 Average ScoreIn this period, we can get the negative correlation between the class size and final scores. With regard to this result, we have obtained the relation between them logically at the beginning, and it also validates the rationality of this model.6.1.2 The Relation between Other Variables and Final ScoresDoing the same work to other variables basing on the same principle and method, and here are the line charts in Figure 6.3, 6.4, 6.5, 6.6Figure 6.3 the relation between the student types and the final scoresFigure 6.4 the relation between the teaching time and the final scoresIt shows that teachers will develop their teaching skill with time going by, and will get a higher scores (or exception).Figure 6.5 the relation between the teachers title and the final scoresIt shows that teachers with high professional title have more experience to teach students and will get a high scores (or exception).Figure 6.6 the relation between the genders and the final scoresIt shows that the gender is not related to the final scores.Conclusion:In summary, only the factor gender is not related to the final scores, here is the correction between each factors and final scores in Table 2Title Gender Student Teaching Time Class Size Final Score positive no relate negative positive negative6.2 Setting a Fair System of School Education Evaluation6.2.1 A Basic model reacting the Teaching QualityAfter confirming the variables and for the sake of giving a quantitative calculation to the teaching factors (teachers quality ), we choose the Principle Component Estimate model to calculate.Doing factor analysis to reduce the dimensions, we can obtain the Contribution of each variables and Degree component matrix in Table 6.3 and 6.4Table 6.3 Variance Can be ExplainedTable 6.4 Component MatrixUsing 11121ˆ0ˆ),(ˆααβQ Q Q =⎪⎪⎭⎫ ⎝⎛=, we can finally get the equation of the teaching quality asConclusion:)(022.0)(37.0)(33.0-)(07.0)(91.0)(time teaching size class type student Z title Z scores fianl Z Quality Teacher P +-+= After getting the P(Teacher Quality) of each teachers, we can sort ascending the scores and then school can carry out the policy of awards and penalties.Here is a 3-dimenation bar graph which consists the final scores, teacher qualities and the account with some cases, and it works well as shown inFigure 6.10 The Example of the model6.2.2 A Refined model majoring in Teachers ProcessEvaluate a teacher not only adhere his teaching quality in one year, but we must pay attention to the process of the teachers, that is to say, we can make an inspirational evaluation system.By adopting Variable Control Model , we only change two variables as teachers title and teaching time. And the corresponding liner charts are given in Figure 6.7~6.9Figure 6.7 The Scores of V.Pr.Figure 6.8 The Scores of TA.Figure 6.9 The Scores of Pr.Here V.Pr. means associate professor; Pr. means professor; TA means assistant teacher; average addition stands for the average slope of two phases.Conclusion:Title Associate professor Teacher assistant Professor Average condition 0.03556 0.099403 0.08965That is to say if one teacher do not improve his scores in one years beyond the average additions belonging to his title, then he will be published; on the contrary he will be awarded.7. Sensitivity AnalysisSometimes input of our model may be hard to obtain or there might be some uncertainty in our inputs. Both these kinds of deviation might influence the result of our model. To test the robustness of our model, we implement a sensitivity analysis. We test our model in scores form students and the number of students. Actually,for the 1st question we select the non-teaching factors by control the variables and then confirm the factors by observing the trend between the independent variable and the final scores qualitatively, hence the conclusion will not change being disturbed; for the 2st question, as the equation is a liner regression equation, the result will only change in a slight range which can be ignored. For this reason, our model does not demonstrate a chaotic behavior, show a good sensitivity.Here are two scatter diagrams which has 15% of the turbulence for two variables as Figure 7.1 and 7.2Figure 7.1 The Sensitivity Analysis of Scroces From StudentsFigure 7.2 The Sensitivity Analysis of The Number of StudentsConclusion:We can conclude that our model will not be disturbed in a slight range.8. Further DiscussionsConsider that school will not concentrate on the detailed scores of teaching quality meanwhile in order to make an inspirational evaluation system, we select 5%, 25%, 50%, 20% of the teachers and sort their teaching quality as well as final scores out . We select 300 cases to do Sequencing Logistics Analysis, and using the rest cases to check up. Doing hypothesis as following Table 8.1Level Explain Probability4 excellentπ13 good jobπ22 acceptedπ31 limitedπ4Table 8.1 Variable AssignmentAnd here is the linear regression modelCompare with the traditional Logistic Model, 321211ππππππ+++、、make a Logit change, that is to say it will influence the Cumulative Probability of order value level as shown in Table 8.2Estimation Sig. [Quality = 1.00] -39.658 .000 [Q u ality = 2.00]-12.201 .000 [Quality = 3.00] 4.927 .050 [Title=1.00] -7.351 .000 [Title=2.00] -3.917 .000 [Title=3.00] 0a . [students=1.00] -19.682 .000 [students=2.00] -9.018 .000 [students=3.00] 0a . [classes=1.00] -24.349 .000 [classes=2.00] -12.431 .000 [classes=3.00] 0a . [Score=1.00] 10.223 .000 [Score=2.00] 6.434 .009 [Score=3.00] 2.072 .394 [Score=4.00]0a.(Sig.<0.05,and this model is reasonable)Table 8.2 Relevant Parameter()()()()()()3)(Scroce *2.0722)(Scroce *6.4341)(Scroce *10.2232)(classes *12.431-1)(classes *24.349-2Students *9.018-1Students *19.682-2)(Title *3.917-1)(Title *7.351927.41log3)(Scroce *2.0722)(Scroce *6.4341)(Scroce *10.2232)(classes *12.431-1)(classes *24.349-2Students *9.018-1Students *19.682-2)(Title *3.917-1)(Title *7.351207.121log3)(Scroce *2.0722)(Scroce *6.4341)(Scroce *10.2232)(classes *12.431-1)(classes *24.349-2Students *9.018-1Students *19.682-2)(Title *3.917-1)(Title *7.351658.391log321321212111=+=+=======-=---++=+=+=+======--=--+=+=+=+======--=-ππππππππππππObey the formula following, check out the 40 rest cases, and 38 cases have been predicted successfully.Here are the ten cases of the successful ones as shown in Table 8.3P1 P2 P3 P4 Quality of teacher0.33 0.35 0.20 0.56 4 0.33 0.35 0.20 0.56 4 0.33 0.35 0.20 0.56 4 0.33 0.33 0.74 0.03 3 0.33 0.33 0.74 0.03 3 0.33 0.69 0.25 0.06 2 0.33 0.69 0.25 0.06 2 0.33 0.69 0.25 0.06 2 0.68 0.39 0.33 0.33 1 0.680.390.330.331Conclusion:It shows that our model is reasonable and right9. Strengths and Weaknesses9.1 StrengthsOur models have some merits as follow:• When we Analysis control variable test , our model use the method of average to the rest of the independent variable tension to ensure the independence of the results.• Use of contingency table analysis, the paper analyzes the interactio n between various factors and the main effect of the size, for the following principal component analysis provides a realistic basis.• We use ordinal logistic model to test the results of quantitative analysis of the income, which achieve the actual effect of hypothesis test.• After studying a large number of social investigation, combined with existing data, this paper researches the teaching actual situation, and found some practical problems. For this, we established the student-led multi-index evaluation system. The system not only eliminate the influence which are relatively big external factors in the fair and justice, but also establish evaluating progress in the incentive effect.9.2 WeaknessesOur model also have some disadvantages.• When we use factors of family composition analysis, paper miss the theory of discrete variables treated as continuous variable. But SPSS has identified the nominal amount in data process,so it don’t influence the results.• Because we only have teac hing evaluation results form students, the evalua tion system of guidance is in the face of students,and it can not fully reflect t he overall level of teachers.• Our method of qualitative analysis in external factors is single, and we have’t related hypothesis testing on the conclusion.References·[1] Jun Shao(2003) Mathematical Statistics. Springer Press·[2] Fred C. Pampel(2015) Logistic Regression Introduction SAGE Publications Inc.·[3] Scott Menard(2016) Applied Logistic Regression Analyse(the second edition) SAGE Publications Inc.·[4]Yu Shi Yaowu Li(2005) Probability Theory and Applied Mathmatical Statistics Xi’an Jiaotong University Press·[5] Shengke Chen Rong Liu(2010) SPSS Satistical Analysis from Entry to Master(the third edition) Tsinghua University Press·[6] Alan Agresti(2012) Categorical Data Analysis Chongqing University Press。
历年美赛数学建模优秀论文大全
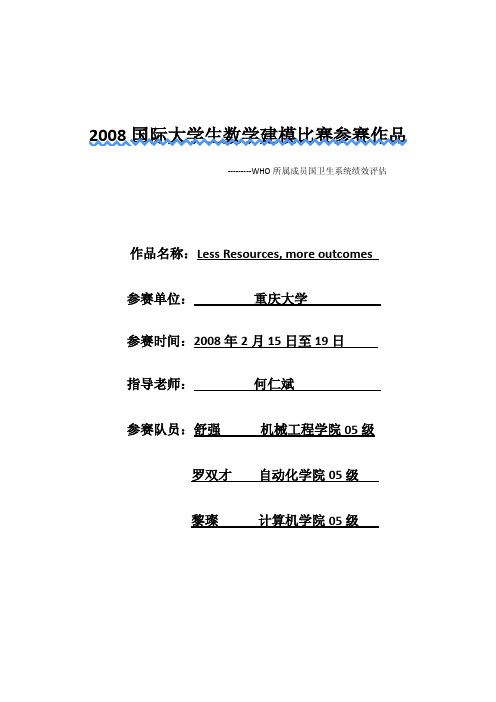
2008国际大学生数学建模比赛参赛作品---------WHO所属成员国卫生系统绩效评估作品名称:Less Resources, more outcomes参赛单位:重庆大学参赛时间:2008年2月15日至19日指导老师:何仁斌参赛队员:舒强机械工程学院05级罗双才自动化学院05级黎璨计算机学院05级ContentLess Resources, More Outcomes (4)1. Summary (4)2. Introduction (5)3. Key Terminology (5)4. Choosing output metrics for measuring health care system (5)4.1 Goals of Health Care System (6)4.2 Characteristics of a good health care system (6)4.3 Output metrics for measuring health care system (6)5. Determining the weight of the metrics and data processing (8)5.1 Weights from statistical data (8)5.2 Data processing (9)6. Input and Output of Health Care System (9)6.1 Aspects of Input (10)6.2 Aspects of Output (11)7. Evaluation System I : Absolute Effectiveness of HCS (11)7.1Background (11)7.2Assumptions (11)7.3Two approaches for evaluation (11)1. Approach A : Weighted Average Evaluation Based Model (11)2. Approach B: Fuzzy Comprehensive Evaluation Based Model [19][20] (12)7.4 Applying the Evaluation of Absolute Effectiveness Method (14)8. Evaluation system II: Relative Effectiveness of HCS (16)8.1 Only output doesn’t work (16)8.2 Assumptions (16)8.3 Constructing the Model (16)8.4 Applying the Evaluation of Relative Effectiveness Method (17)9. EAE VS ERE: which is better? (17)9.1 USA VS Norway (18)9.2 USA VS Pakistan (18)10. Less Resources, more outcomes (19)10.1Multiple Logistic Regression Model (19)10.1.1 Output as function of Input (19)10.1.2Assumptions (19)10.1.3Constructing the model (19)10.1.4. Estimation of parameters (20)10.1.5How the six metrics influence the outcomes? (20)10.2 Taking USA into consideration (22)10.2.1Assumptions (22)10.2.2 Allocation Coefficient (22)10.3 Scenario 1: Less expenditure to achieve the same goal (24)10.3.1 Objective function: (24)10.3.2 Constraints (25)10.3.3 Optimization model 1 (25)10.3.4 Solutions of the model (25)10.4. Scenario2: More outcomes with the same expenditure (26)10.4.1Objective function (26)10.4.2Constraints (26)10.4.3 Optimization model 2 (26)10.4.4Solutions to the model (27)15. Strengths and Weaknesses (27)Strengths (27)Weaknesses (27)16. References (28)Less Resources, More Outcomes1. SummaryIn this paper, we regard the health care system (HCS) as a system with input and output, representing total expenditure on health and its goal attainment respectively. Our goal is to minimize the total expenditure on health to archive the same or maximize the attainment under given expenditure.First, five output metrics and six input metrics are specified. Output metrics are overall level of health, distribution of health in the population,etc. Input metrics are physician density per 1000 population, private prepaid plans as % private expenditure on health, etc.Second, to evaluate the effectiveness of HCS, two evaluation systems are employed in this paper:●Evaluation of Absolute Effectiveness(EAE)This evaluation system only deals with the output of HCS,and we define Absolute Total Score (ATS) to quantify the effectiveness. During the evaluation process, weighted average sum of the five output metrics is defined as ATS, and the fuzzy theory is also employed to help assess HCS.●Evaluation of Relative Effectiveness(ERE)This evaluation system deals with the output as well as its input, and also we define Relative Total Score (RTS) to quantify the effectiveness. The measurement to ATS is units of output produced by unit of input.Applying the two kinds of evaluation system to evaluate HCS of 34 countries (USA included), we can find some countries which rank in a higher position in EAE get a relatively lower rank in ERE, such as Norway and USA, indicating that their HCS should have been able to archive more under their current resources .Therefore, taking USA into consideration, we try to explore how the input influences the output and archive the goal: less input, more output. Then three models are constructed to our goal:●Multiple Logistic RegressionWe model the output as function of input by the logistic equation. In more detains, we model ATS (output) as the function of total expenditure on health system. By curve fitting, we estimate the parameters in logistic equation, and statistical test presents us a satisfactory result.●Linear Optimization Model on minimizing the total expenditure on healthWe try to minimize the total expenditure and at the same time archive the same, that is to get a ATS of 0.8116. We employ software to solve the model, and by the analysis of the results. We cut it to 2023.2 billion dollars, compared to the original data 2109.8 billion dollars.●Linear Optimization Model on maximizing the attainment. We try to maximize the attainment (absolute total score) under the same total expenditure in2007.And we optimize the ATS to 0.8823, compared to the original data 0.8116.Finally, we discuss strengths and weaknesses of our models and make necessary recommendations to the policy-makers。
2017年全国大学生数学建模竞赛优秀论文
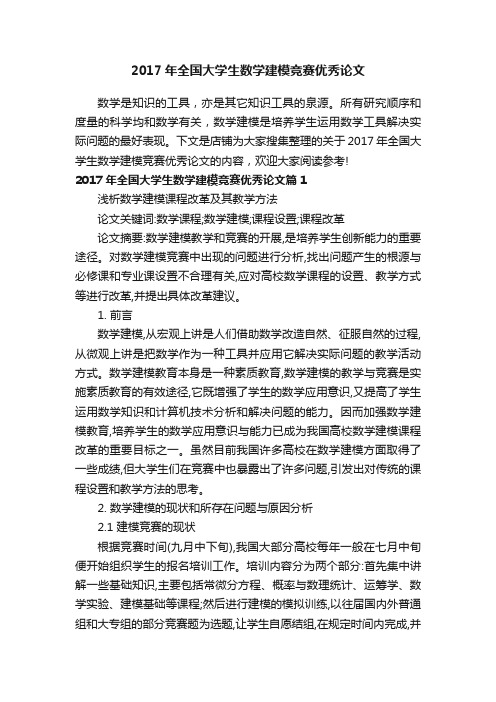
2017年全国大学生数学建模竞赛优秀论文数学是知识的工具,亦是其它知识工具的泉源。
所有研究顺序和度量的科学均和数学有关,数学建模是培养学生运用数学工具解决实际问题的最好表现。
下文是店铺为大家搜集整理的关于2017年全国大学生数学建模竞赛优秀论文的内容,欢迎大家阅读参考!2017年全国大学生数学建模竞赛优秀论文篇1浅析数学建模课程改革及其教学方法论文关键词:数学课程;数学建模;课程设置;课程改革论文摘要:数学建模教学和竞赛的开展,是培养学生创新能力的重要途径。
对数学建模竞赛中出现的问题进行分析,找出问题产生的根源与必修课和专业课设置不合理有关,应对高校数学课程的设置、教学方式等进行改革,并提出具体改革建议。
1. 前言数学建模,从宏观上讲是人们借助数学改造自然、征服自然的过程,从微观上讲是把数学作为一种工具并应用它解决实际问题的教学活动方式。
数学建模教育本身是一种素质教育,数学建模的教学与竞赛是实施素质教育的有效途径,它既增强了学生的数学应用意识,又提高了学生运用数学知识和计算机技术分析和解决问题的能力。
因而加强数学建模教育,培养学生的数学应用意识与能力已成为我国高校数学建模课程改革的重要目标之一。
虽然目前我国许多高校在数学建模方面取得了一些成绩,但大学生们在竞赛中也暴露出了许多问题,引发出对传统的课程设置和教学方法的思考。
2. 数学建模的现状和所存在问题与原因分析2.1 建模竞赛的现状根据竞赛时间(九月中下旬),我国大部分高校每年一般在七月中旬便开始组织学生的报名培训工作。
培训内容分为两个部分:首先集中讲解一些基础知识,主要包括常微分方程、概率与数理统计、运筹学、数学实验、建模基础等课程;然后进行建模的模拟训练,以往届国内外普通组和大专组的部分竞赛题为选题,让学生自愿结组,在规定时间内完成,并自愿为同学讲解各自的解题思路和方法。
参赛学生首先要参加培训,他们一般是先关注校园网上的通知,再到各院系自愿报名而组成,经培训后选拔出参赛队员。
- 1、下载文档前请自行甄别文档内容的完整性,平台不提供额外的编辑、内容补充、找答案等附加服务。
- 2、"仅部分预览"的文档,不可在线预览部分如存在完整性等问题,可反馈申请退款(可完整预览的文档不适用该条件!)。
- 3、如文档侵犯您的权益,请联系客服反馈,我们会尽快为您处理(人工客服工作时间:9:00-18:30)。
For office use onlyT1________________ T2________________ T3________________ T4________________ Team Control Number70028Problem ChosenBFor office use onlyF1________________F2________________F3________________F4________________2017MCM/ICMSummary Sheet(Your team's summary should be included as the first page of your electronic submission.)Type a summary of your results on this page. Do not include the name of your school, advisor, or team members on this page.SummaryThe performance of highway toll plaza directly affects the capacity of the highway, so the design of road toll plaza is imperative.In this paper, we conduct performance analysis for a specific toll plaza in New Jersey, USA, including accident prevention, throughput and cost. First of all, we usegrey model to predict the future output of the toll plaza, and compared with the realdata, the average value of the residual value is 0.429. Then we can draw a conclusionthat the throughput performance of the toll plaza is secondary. Next, we use queuingtheory to get the service index of the toll plaza in the light and heavy traffic, and thecellular automaton model is used to consider the changing circumstances of servicelevel, uses regression model to establish a function relation between traffic accidentand four factors. Then, we find that the rate of change has the greatest influence onit and the pavement performance has the least influence . In terms of cost, weconsider the toll plaza land and road construction. And the cost of road constructionis divided into the labor cost and material cost.Next, according to the influence of road geometry on the traffic performance of Toll Plaza, we select the transition curve trajectory model to improve the toll plazatransition, which can also have an improvement on the size and shape of the toll plazaand merge mode.Finally, we do a series of performance studies for our improved toll plaza. First of all, the improvement in the square flow and car flow under the condition of servicelevel are determined respectively through simulation .Next, we draw a conclusion thatthe service performance of the toll plaza is not obvious in small car flow, but there is amarked increase in large flow. Then, due to the fact that the unmanned vehicle coulddeal with a variety of road conditions, it undoubtedly expands our improved optionalscheme. Eventually, we obtain the throughput of toll before and after the improvementunder the different proportion of mixed charge mode and find that the improvedthroughput in the toll plaza has been increased on the performance.contents1 Introduction: (1)1.1 Problem background: (1)1.2 Steps: (1)1.3 Our work: (1)2 Assumptions (2)3 Nomenclature (2)4 Throughput analysis of grey forecasting model (3)5 error analysis (4)6 Service level of toll station (5)7 Vehicle lane changing rules based on Cellular Automata (6)8 Security analysis based on multivariate statistical regression mode (8)8.1 Study on the rate of change of Toll Plaza (8)8.2 Study on the longitudinal slope of entrance section of Toll Plaza (9)8.3 Research on service level of toll station (10)8.4 Study on pavement performance of toll station (10)9 Safety performance evaluation model of toll station (11)10 Cost analysis model of toll station (11)11 Analysis of the influence of lane geometry parameters on its capacity (12)11.1 Determination of lane changing rate (12)11.2 Influence of geometric parameters on the flow of the car lane (14)11.3 Energy consumption analysis based on cellular automata model (15)Definition of energy consumption: (16)Numerical simulation and analysis of the results: (17)Influence of curvature radius on energy consumption (17)Influence of arc length on energy consumption (18)12 The effect of traffic flow on service performance based on improved queuing theory 1913 The influence of unmanned vehicles on the improved model of Toll Plaza .. 2114 The influence of charging method on improving model of Toll Plaza (21)15 Strengths and Weaknesses (22)15.1 Strengths: (22)15.2 Weaknesses: (22)15.3 Future Model Development: (22)Comprehensive improvement strategy of tollplaza1Introduction:1.1Problem background:Highway toll and toll plaza is to ensure traffic safety and unimpeded, however because of lack of unified design specification, toll station and its square construction exists many problems. Such as: low value because of the technical indicators to make square construction scale too small and cause the toll plaza opened only few years as the traffic bottleneck, and use the high value on the one hand, because of the technical indicators and make the toll station construction scale is too large, waste a lot of money and resources. Due to incorrect linear indicators, or too short, the gradual square square length is insufficient, square road centerline offset, etc., it is too difficult to use after the completion of the square.so establishing the toll gates and the toll plaza design norms, as soon as possible, has the very vital significance in standardizing the construction of the toll station, ensuring the smooth general characteristic of toll plaza and traffic safety, improving the charging efficiency and management level, reducing the land acquisition and controlling investment and so on .1.2Steps:·A performance analysis of any particular toll plaza design that may already be implemented through the following three factors: accident prevention, throughput and cost .·Determine if there are better solutions (shape, size, and merging pattern) than any in common use.·Consider the performance of your solution in light and heavy traffic.·Consider the situation where more autonomous (self-driving) vehicles are added and how the solution is affected by the proportions of conventional (human-staffed) tollbooths, exact-change (automated) tollbooths, and electronic toll collection booths (such as electronic toll collection via a transponder in the vehicle)1.3Our work:·Based on the available data ,we make a performance analysis of any particulartoll plaza design that may already be implemented .·According to the problem from the performance analysis ,we make out a better solutions (shape, size, and merging pattern) than any in common use.·Determine the performance of the solution in light and heavy traffic ,how the solution change as more autonomous (self-driving) vehicles are added to the traffic mix and how the solution is affected by the proportions of conventional (human-staffed) tollbooths, exact-change (automated) tollbooths, and electronic toll collection booths.2AssumptionsTo simplify the problem and make it convenient for us to simulate real-life conditions, we make the following basic assumptions.1. Each section of roads is one-way traffic2.Vehicles in the retention period of toll station can be neglected3.In any hour of the vehicle arrival rate is proportional to the length of time4.The probability of any vehicle arrival in one hour of time is not affected by the previous history .5. The vehicles arrive in line with the Poisson distribution, namely the headway is negative exponential distribution3Nomenclatureε(0)(t)the residual errorq(t)the relative errorc the variance ratioP the small error probabilityr the curvature of the bend radiusu the static friction coefficientl the gradual change ratiok the number of serving drivewayρ/k traffic intensityw mean time to stay at a toll stationd automotive braking distancef the tire and road surface friction coefficientY the number of traffic accidents in toll stations per year∆W Width of the gradualα1curve angle R 1the radius of convex curve points pdelay probability e(n,t) energy consumption of the first n vehicles from time t to t+14 Throughput analysis of grey forecasting modelFigure 4-0-1Schematic diagram of New Jersey toll plazaFirst of all, we chose a toll plaza on the New Jersey in the United States for a specific performance analysis of toll plaza, and it includes the accident prevention, throughput, and cost.In view of the throughput of the toll plaza, we choose the grey forecasting model GM(1,1) , to predict the throughput of the toll plaza. Due to the problem of uncertainty, so we take the grey prediction model to deal with it.Suppose x (0)(1),x (0)(2)…,x (0)(M )In order to overcome the irregular , we use accumulation processx (1)(t )=∑x (0)(i)M i<1 Such a relatively smooth new series approximation can be described by the following differential equation:dx (1)dt +ax (1)=μ Its an albino form discrete solution of differential equation is: x ̂(1)(i +1)=.x (1)−u a /e ;ai +u aThe type of the parameter a、u be determined by the least squares fitting method is as follows:(1)(2)(3)A ̂=0a u 1=(B T B);1B T Y N Among them the matrix is:B =[ −12,x (1)(1)+x (1)(2)-1−12,x (1)(2)+x (1)(3)-1⋯⋯−12,x (1)(m −1)+x (1)(m )-1] Y N =(x (0)(2),x (0)(3),⋯,x (0)(m ))TSo the original data fitting sequence is:x ̂(0)(1)=x (0)(1)x ̂(0)(i +1)=x (1)(i +1)−x (1)(i )Table 4-0-1 Traffic flow prediction table5 error analysisIn equation (11), and regulations, the original data of reducing value and its residual error and relative error between observed value is as follows{ε(0)(t )=x (0)(t )−x′(0)(t )q (t )=ε(0)(t )x (0)(t )×100%The following inspection of the accuracy: x(0)=1M ∑x (0)(t )M t<0 ε(0)=1M;1∑(ε(0)(t )−ε0M t<2)2Second, calculate the variance ratio c =s 2s 1and small error probability P =2|ε(0)(t )−ε(0)|<0.6745s 13(4)(5)(6) (7) (8) (9) (10) (11)Figure 5-0-2comparison chart of grey prediction modelWe use m、p、v max to represent quality of the vehicle, random delayprobability and maximum speed respectively, g represents the local acceleration of gravity, r represents curvature of the bend radius and u represents the static friction coefficient . With the road statistical analysis carried out on the real value and the error of predicted value, we obtain the following res ults:It shows that the GM(1,1)model prediction results have a better response to .reflect the actual situation.6 Service level of toll stationThe direct feeling of the driver to the traffic environment of the toll station is from the queue length of the toll lane, and the length of the queue depends on the service level of the toll station V/C. In this regard, we use the queuing theory model of multichannel Queuing service, in which the vehicle arrival time is in a Poisson distribution, which is the negative exponential distribution; Suppose m is random arrival rate ,c i is output rate,k is the number of serving driveway, ρ=m c .There is the probability of having no vehicle in the queuing theoryρ(0)=1,∑1n!k−1n=0p n :1k!ρk k k−ρ- Average number of vehicles in queueing theory:n =ρ+p n ρ(0)k!k n−k (1;ρk )2 (12)(13)queue length: q =n −ρ=p n ρ(0)k!k n−k (1;ρk )2 Average number of waiting vehicles per lanea =q kAverage waiting time in queue systems:d =n m =q m +1c Average waiting time in queue:W =q mMean tardinessDeceleration time of vehicle entering toll stationt 1=v 03.6a 1Mean time to stay at a toll stationw =E ,S -+W qVehicle acceleration time of leaving toll stationt 2=v 03.6a 2 In this equation, v 0 is the normal traffic flow (km/h); a 1 、a 2 are deceleration of the vehicle (m/s 2); W q is average queue time (s); E ,S - is expected service time (s);7 Vehicle lane changing rules based on CellularAutomataWe apply the previous cellular automata model, which is now extended to multi Lane case. The main difference between multi lane and single lane is to consider the model of lane changing. In this paper, we take 4 lanes as an example.In reality, it may be possible to change lanes when the driver is found to be close to the exit and the front of the adjacent lane is empty. If you want to change lanes ,you should consider the vehicle behind the adjacent lane. When the distance (14)(15) (16) (17) (18) (19) (20)to the rear of the adjacent lane reaches to a certain length, you can change the road. Lane change scenarios can be shown in figure (), when the c car on the 1 Lane is blocked by the c 1 car, while the c 2 and c 3 cars on the 2 lanes are relatively large. in order to maintain the speed, c car will change to the road lane 2.Figure 7-0-3Schematic diagram of lane changingWhether or not the driver chooses the lane change is mainly decided by the d 0,d n,otℎer 、d n ,back three indicators, through the previous research, this paper thinks that the lane changing rule is:When d n,back >v maxC n ={1−C n d n <min{v n +1,v max } d n,otℎer >d n ,d n,back >v max c n Otℎer circumstancesWhen d n,back ≤v max ,C n ={1−C n d n <min{v n +1,v max } d n,otℎer >d nv max −θ(−∆x )α>1+min{d n,otℎer +1,v max }−min *V n +1,v max +c n Otℎer circumstancesAmong them, C n is the n car in the lane , C n =0 or 1,d n 、d n,otℎer andd n,back are the distance between the first n vehicle and the front vehicle, the distance from the adjacent lane and the distance from the vehicle in the adjacent lane, respectively. d safe is safety lane change model.d n,back −v max , ∆x <0, v max −θ(−∆x )α is the distance between the vehicle and the vehicle in the adjacent lane after correction by the value function, 1+min{d n,otℎer +1,v max }−min *V n +1,v max + is Limit Lane distance. The parameters α and θchange according to the psychological status of driver. If α>1, the greater α is, the more careful the driver is. If θ>1, the greater θis, the more careful the driver is. When α=1,θ=1,that ’s Lane changing model.(21) (22)In order to discuss the αandθ, we use Cellular automata simulation. In a two lane road with a length of7.5km, adopting the open boundary condition, each lane is composed of 1000cells with a length of7.5km, the maximum speed of vehicle v max=5. The random slowing down rate was 0.2.8Security analysis based on multivariate statistical regression modeAimed at the prevention of the accident, we use multiple linear regression to establish a function between the number of traffic accidents and the following four factors: toll square gradient, service level, Toll plaza entrance section of the longitudinal slope, the Pavement performance of Toll station .Figure 8-1Cause analysis of accident8.1Study on the rate of change of Toll PlazaFan in and fan out area of toll plaza are designed to make the gradual vehicles more natural smoothly in and out of the toll plaza. In order to drive vehicle easily , there has a requirement on its gentle gradient change. Otherwise the driver could produce driving deviation, which may cause improper operation and endangers safety.The relationship is as follow:(23)l=b,LAccording to the experience, the vehicles with straight into another lane deviation than at around 0.9m s⁄, drivers usually have no move feeling and uncomfortable feeling.Figure 8-1 The relationship between Accident number and Toll plaza ramp rateFigure 8-1 shows the relation curve between highway toll plaza ramp rate and traffic accident, the figure demonstrates that as the toll plaza ramp rate increases, the traffic accidents will increase, whereas the security of the toll plaza will decrease.Through the data regression analysis, we get the related models between toll plaza ramp rate and the number of traffic accidentsY =1.423e .0064xIn this equation, Y is the forecasted numbers of traffic accident corresponding to the toll plaza ramp rate , x is the toll plaza ramp rate of toll plaza.The correlation coefficient in the model R 2=0.8621, it shows that description model of correlation is higher, From the model ,we can learn that the occurrence of traffic accident frequency is proportional to the toll plaza ramp rate. Gradient length is insufficient, so it can't meet to slow down and change lanes entering the toll plaza vehicle safety requirements, resulting in the occurrence of traffic accidents .8.2 Study on the longitudinal slope of entrance section ofToll PlazaHighway toll entrance section of the longitudinal slope design without fully considering the characteristics of vehicles entering the toll plaza, a long downhill or turn downhill and so on bad road alignment, those will affect the normal operation of the pilot and make the vehicles entering the toll plaza slowdown not sufficient, longitudinal safe driving distance not enough and driving direction can't adjust to the charge lane ,which will causetraffic accidents. This will lead to serious losses. (24)Figure 8-0-4 entrance section of the longitudinal slope and accident numberThrough regression analysis, we get the relevant model between the toll plaza entrance section of longitudinal wave and traffic accidentsY =2.6254e 0.638xIn this equation, Y is the forecasted numbers of traffic accident corresponding to the toll plaza ramp rate , x is the longitudinal wave of t oll plaza’s entry section .The correlation coefficient in the model R 2=0.9219,it shows the correlation of this model is relatively high. But we can learn that toll station ‘s traffic accident and its entrance section of longitudinal wave have a positive correlation from figure model representation ,.The greater the slope, the lower charge war security.8.3 Research on service level of toll stationBased on the previous research of service performance of toll station, we take V C as the measure of service level and Cite previous results. 8.4 Study on pavement performance of toll stationAccording to the vehicle dynamics, the vehicle's braking distance can be expressed as follows:d =u 257.9(f:I) In this equation, d is automotive braking distance , u is the speed at the beginning of the automobile brake, f is the tire and road surface friction coefficient, Iis road longitudinal slope(25)9 Safety performance evaluation model of toll stationBased on the above analysis, the evaluation model of descriptive can be written as the equation form, using multiple linear regression model .Y is the number of traffic accidents in toll stations every year , x 1=1l ,x 2=V C ,x 3=i,则Y =β0+β1x 1+β2x 2+β3x 3N is sample size , Y i (i =1,2,…,N ) represent the Y value of sample i , x i 1,x i 2,…x i n (i =1,2…,N) represent the value of each variable insample I, respectively.令Y =[Y 1Y 2⋮Y n], X =[11⋮1x 11x 21⋮x n 1⋯⋯⋮⋯x 1n x 2n ⋮x n n ] β=[β0β1⋮βn ] Y =Xβ,making maximum likelihood estimate of each variable coefficient β1,β2,…βn , it can get a normal equations:X T Xβ=X T YSo you can get the following regression equationY =−4.4012−9.947511l +10.098V C +11.25i 10 Cost analysis model of toll stationWe selected the American New Jersey a toll plaza to make cost analysisFirstly, according to relevant data, we learn that New Jersey’s average price is (26) (27)(28)(29)(30)$3500 per mu,And the toll plaza which we analyzed occupies about 5 mu, therefore, the land price of the toll plaza is about $17500;Second, the road construction costs include labor and material cost, and the local construction industry ’s average monthly salary is $3000, we use it to calculate labor, this occupies the largest in the road construction costs; As for material cost, we calculate by the current prices in the United States, is about $40 per cubic meter, then according to the size of the toll plaza, it will cost about $45000.In conclusion, the cost of toll plaza spend mainly on the labor cost of highway construction, the material cost also accordingly account for part of it.11Analysis of the influence of lane geometry parameters on its capacity11.1Determination of lane changing rateAccording to the analysis of vehicle trajectory and running state of vehicle , vehicle trajectory in the middle of the gradual path is similar to vehicle lane changing trajectory, and considering the factors when the driver turns, we select the easement curve trajectory model to design the gradual change section of toll plaza. And in the middle of the two convex type curve , we join a long for L straight section , it is shown in the figure belowFigure 11-0-5Toll plaza improvementsAccording to characteristics of convex curve geometric elements, we can use the following formula to calculate the first period of convex curve of easement curve tangent length T1:T1=(R1+p1)tanα1+q1(31)2In this equation, R 1 is the radius of the first section of convex curve points , ρ1 is Within shift, q 1 is tangent increment, α1 is curve angle, and α1=2β1, β1 is easement curve angleSuppose the first and second convex curve gradient width are ∆W 1 and ∆W 2 respectively, the width of one side with the gradient is ∆W .Depending on the figure with the easement curve in orbit, there are: ∆W 1=T 1∙sin α1∆W 2=T 2∙sin α2∆W =∆W 1+∆W 2+Lsinα1∆W =0(R 1+p 1)(1−cos L S1R 1 )+q 11∙sin L S1R 1 +0(R 2+p 2)(1−cos L S2R 2)+q 21∙sin Ls2R 2 +Lsinα1 L S1 and L S2 are the length of easement curve of two convex curve respectivelyL is radial tangent of two convex curve, so α1=α2,then it Can be introduced as follows:L S1R 1 =L S2R 2 Associate (38) and (39),we can get the length of easement curve of two - Section convex curve L S1 and L S2, then the transition section longitudinal distance L y can use the following formula to calculate:L y =[(R 1+p 1)tan L S12R 1 +q 1+(R 2+p 2)tan L S22R 2 +q 2](1+cos L S1R 1 )+Lcosα1 Suppose the ramp rate of transition period is K ,then we can adopt the following equation:K =∆WL y From this equation , we can learn that the driving radius and the straight line segment L have a great influence on the length and the gradient of the gradient. The greater the radius, the longer the straight line, the longer the length of the gradient, the smaller the rate of change(32) (33) (34) (35) (36)(37)(38)11.2 I nfluence of geometric parameters on the flow of thecar laneAssuming C 0 and C 1=dC dl represent respectively bend and itsgradient , l represents the length of the curve itself , we can get C (l )=C 0+C 1lso ,the bend of the direction Angle isφ(l )=φ0+∫C(τ)l 0dτ=φ0+C 0l +12C 1l 2 The bend of the longitudinal distance x(l) and transverse distance y(l) are{x (l )=x 0+∫cosφ(τ)dτl 0y (l )=y 0+∫sinφ(τ)dτl 0 Assuming sinφ≈φ,cosφ≈1,and when x 0(l )=0,x (l )=l , then the bend of transverse distance y(x) and direction angle φ(x) can be expressed{φ(x )=φ0+C 0x +12C 1x 2y (x )=y 0+φl +12C 0x 2+16C 1l 3 Using the ideas of analytical mechanics, assuming that the longitudinal velocity along the x axis for x ′, along the y axis transverse speed for y ′ , along the z axis of horizontal pendulum angular velocity as the bits of ψ′, then from The Lagrange's equations we can get{ d dt .ðE T ðẋ/−ψðE T ðẏ=F Q 1d dt .ðE T ðẏ/+ψðE T ðẋ=F Q 2d dt .ðE T ðψ/+ẋ ðE T ðẏ−y ðE T ðẋ=F Q 3 Defining the system kinetic energy E T =12m (ẋ+ẏ)+12I z ψ2In the formula, m,I z respectively represent Vehicle quality and Rotary inertia take the derivative of (46),we can get{ d dt .ðE T ðẋ/−ψðE T ðẏ=d dt(mẋ)−ψ (mẏ)d dt .ðE T ðẏ/+ψðE T ðẋ=d dt (mẏ)−ψ (mẋ)d dt .ðE T ðψ/+ẋ ðE T ðẏ−y ðE T ðẋ=d dt (I z ψ)−x (mẏ)−y (mẋ) (39)(40)(41)(42)(43)Delimiting generalized force: {F Q 1=∑F xF Q 2=∑F y F Q 3=∑M zIn summary we can get the Vehicle longitudinal coupling model.We mainly consider the lateral situation∑F y =F yr +F xf +F xf cosδ If the vehicle driving in the bend is only disturbed by small disturbance near the equilibrium state, the front wheel angle is small enough , so cosδ≈1,sinδ≈δ ∑F y =−(C f +C r )y ẋ−(aC f −bC f )ψẋ+(F xf +C f )δ We put the formula () and formula () into ()y =−d 2ẏẋ−.ẋ+kd 3ẋ/ψ−(F xf :C f m )δ In the formula d 2=C f :C r m ,d 3=aC f ;bC rI z ,k =I z mThen, the resultant force ∑M z along the vertical direction is∑M z =aF xf sinδ+aF xf cosδ−bF yrWhen sinφ≈φ,cosφ≈1,then∑F y =−(a 2C f +b 2C r )ψẋ−(aC f −bC f )ẏẋ+a(F xf +C f )δψ=−d 4ψẋ−−d 3y ẋ+a I z (F xf :C f m )δ In the formula, d 4=(a 2C f :b 2C r )I z 11.3 E nergy consumption analysis based on cellularautomata modelConsidering the influence of different shapes on traffic performance is mainly reflected in the curve, we mainly study the influence of the curve on the whole problem. On the road segment, Lane set of sections containing only one plane curve, the curve is provided with the deceleration section of L , the road will be regarded as the length of the L 1D discrete lattice chain, each lattice point at each moment or is empty or occupied for a car.m 、p and v max represent the quality of the vehicle, the (44) (45)(46)(47) (48) (49) (50)(51)stochastic delay probability and maximum speed ,respectively, g is the local acceleration of gravity, r and u represent the static friction coefficient of curvature radius and static coefficient of friction between wheel and road, respectively. The vertical direction of the vehicle is subjected to a pair of balance forces, and the influence of tangential friction on the vehicle is mainly reflected in the change of the speed, Therefore , the centripetal force required for the safety of the vehicle is provided by the normal static friction force,v safe is maximum speed of safetyturning, then mv safe2r =μmg,⁄v safe =√μgr .In each step of t →t +1 , all vehicles are in accordance with the following rules of the evolution of the speed and location of the synchronization update :Determine the vehicle delay probability p :When the vehicle is in the buffer section , if v >v safe,take the probability of delay p =p 1 (larger), in other cases, take p =p 2 (smaller),Acceleration process: v n (t)→min (v n (t )+1,v max );deterministic deceleration process: v n (t)→min (v n (t ),gap n (t))Stochastic deceleration process with probability p :v n (t)→max (v n (t )−1,0) deceleration process :When the vehicle is in the corner of the road, and the speed v (t )>v safe , in order to turn the corner ,it must be slowed down :v n (t)→min (v n (t ),v safe )location update process: x n (t )→x n (t )+v n (t)Among them, v n (t) and x n (t ) are the speed and position of the first n vehicle at time t respectively , x n:1(t ) is the position of the first n +1 vehicle at time t . gap n (t )=x n:1(t )−x n (t )−1is the spacing between the first n car and the foregoing vehicle which is close to it.Definition of energy consumptionSuppose the mass of vehicle is m , when it slows down, its kinetic energy is reduced, we define the kinetic energy reduction for energy consumption, e(n,t) represents that energy consumption of the first n vehicles from time t to t+1 .e (n,t )={m,v 2(n,,t );v 2(n,,t:1)-2v (n,t )>v (n,t +1);0,v (n,t )≤v (n,t +1)The average energy consumption per vehicle per unit time:E d =1T 1N ∑∑e(n,t) N n<1t0:T;1t<t0 N is the total number of vehicles on the driveway, t 0 is relaxation time. For(52) (53)the energy consumption of the vehicle, if it is because the speed of t moment is greater than the Vehicle-to-vehicle distance v(n,t)>gap n(t), the vehicle decelerates, thatis defined as the interaction energy, denoted by E di; If it is because of the random deceleration caused, defined as the random deceleration energy consumption, denotedby E dr;if it is because the car speed In the corner v(n,t)>v safe, there is deceleration for the sake of driving safely, defined as safe energy consumption, denoted by E ds.Then total energy consumption is:E d=E di+E dr+E ds(54)Numerical simulation and analysis of the resultsTo simplify the problem, assuming that the length of actual road is 7.5km, Divided into 1000lattices, equivalent to the actual length of each grid correspondsto 7.5m, Delay probability p1=0.8,p2=0.25,Quality unit is defined 1. Entering probability changes from 0~1.0.The state of each vehicle is represented by its own speed v, v∈,0,v max-We let v max=5cell he actual speed is135km/h.We take8×104time steps every run .Influence of curvature radius on energy consumptionThe arc length s, the friction coefficient μand the radius of curvature of r are carried out numerical simulation. parameters are as follows: s=30m,μ=0.5,r=10、50、100、200、300m.According to v max=5cell/s,the maximum speed of the vehicle v max=37.5m/s. Results show that when r=300m, the safetyspeed v safe=√μgr=38.73m/s,v safe>v max, the bottleneck of the curve disappears and the speed limit is lost. The change of the probability in_p of therandom energy consumption(E di、E dr、E ds、E d)is shown in the figure.。