Zhang-Yang波动率计算
波动率计算公式
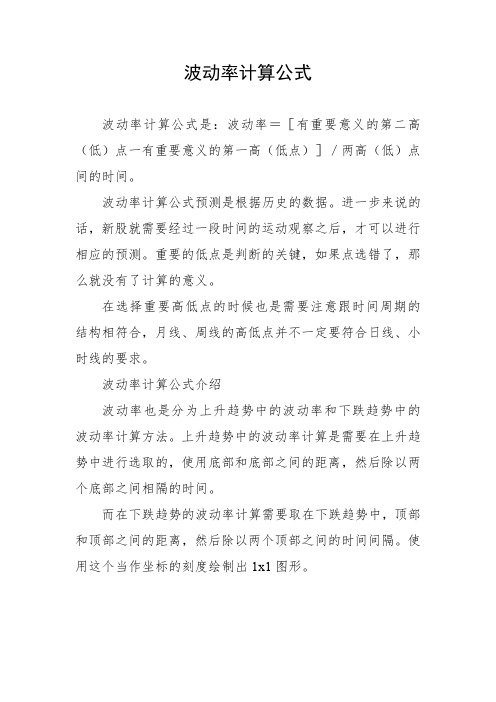
波动率计算公式
波动率计算公式是:波动率=[有重要意义的第二高(低)点一有重要意义的第一高(低点)]/两高(低)点间的时间。
波动率计算公式预测是根据历史的数据。
进一步来说的话,新股就需要经过一段时间的运动观察之后,才可以进行相应的预测。
重要的低点是判断的关键,如果点选错了,那么就没有了计算的意义。
在选择重要高低点的时候也是需要注意跟时间周期的结构相符合,月线、周线的高低点并不一定要符合日线、小时线的要求。
波动率计算公式介绍
波动率也是分为上升趋势中的波动率和下跌趋势中的波动率计算方法。
上升趋势中的波动率计算是需要在上升趋势中进行选取的,使用底部和底部之间的距离,然后除以两个底部之间相隔的时间。
而在下跌趋势的波动率计算需要取在下跌趋势中,顶部和顶部之间的距离,然后除以两个顶部之间的时间间隔。
使用这个当作坐标的刻度绘制出1x1图形。
日波动率计算公式

日波动率计算公式日波动率是用来衡量金融市场价格走势的波动程度的指标,它是投资者对市场风险的一种评估工具。
常见的计算日波动率的方法包括简单波动率、历史波动率和隐含波动率等。
1. 简单波动率(Simple Volatility)简单波动率是通过计算价格序列的标准差来估计市场的波动程度。
简单波动率的计算公式如下:σ = √(1/N * Σ(Pi - Pavg)^2)其中,σ表示简单波动率,N表示价格序列的观测个数,Pi表示第i 个价格,Pavg表示价格序列的平均值。
2. 历史波动率(Historical Volatility)历史波动率是通过计算价格序列的对数收益率的标准差来估计市场的波动程度。
历史波动率的计算公式如下:σ = √(1/N * Σ(Ri - Ravg)^2)其中,σ表示历史波动率,N表示价格序列的观测个数,Ri表示第i 天的对数收益率,Ravg表示对数收益率的平均值。
3. 隐含波动率(Implied Volatility)隐含波动率是通过将期权价格反推出市场对未来波动率的预期值。
隐含波动率的计算需要使用期权定价模型,如Black-Scholes模型。
隐含波动率的计算公式无法简洁地表示,但可以使用数值计算方法进行估计。
日波动率的计算方法选择通常根据具体的情况和需求来决定。
简单波动率容易计算,但忽略了价格序列的非线性和自相关性;历史波动率考虑了价格序列的非线性和自相关性,但对过去的数据敏感;隐含波动率能够反映市场对未来波动率的预期,但需要使用期权定价模型进行计算。
除了以上方法,还有其他一些衍生的波动率计算方法,如加权波动率、平滑波动率和波动率扩散等。
这些方法在具体应用中有各自的特点和适用范围。
需要注意的是,日波动率只是对市场波动程度的估计,并不能预测未来的价格波动。
投资者在实际操作中需要结合其他指标和分析方法进行综合判断,以降低投资风险。
1_波动率的计算
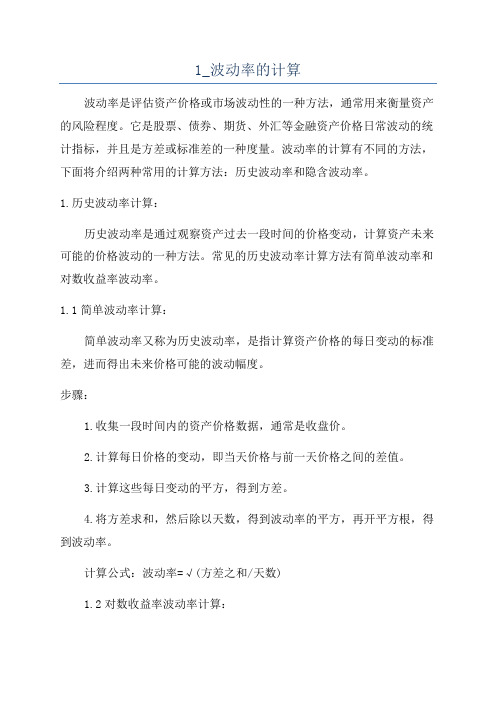
1_波动率的计算波动率是评估资产价格或市场波动性的一种方法,通常用来衡量资产的风险程度。
它是股票、债券、期货、外汇等金融资产价格日常波动的统计指标,并且是方差或标准差的一种度量。
波动率的计算有不同的方法,下面将介绍两种常用的计算方法:历史波动率和隐含波动率。
1.历史波动率计算:历史波动率是通过观察资产过去一段时间的价格变动,计算资产未来可能的价格波动的一种方法。
常见的历史波动率计算方法有简单波动率和对数收益率波动率。
1.1简单波动率计算:简单波动率又称为历史波动率,是指计算资产价格的每日变动的标准差,进而得出未来价格可能的波动幅度。
步骤:1.收集一段时间内的资产价格数据,通常是收盘价。
2.计算每日价格的变动,即当天价格与前一天价格之间的差值。
3.计算这些每日变动的平方,得到方差。
4.将方差求和,然后除以天数,得到波动率的平方,再开平方根,得到波动率。
计算公式:波动率=√(方差之和/天数)1.2对数收益率波动率计算:对数收益率波动率是对资产价格取对数之后计算的波动率,它是用来解决价格波动随时间变化而变动的问题,并更好地符合实际情况。
步骤:1.收集一段时间内的资产价格数据,通常是收盘价。
2.计算对数收益率,即每天收益率的对数,可以使用自然对数或对数收益率公式。
3.计算对数收益率的标准差,并进行年化处理,得到对数收益率波动率。
计算公式:波动率=对数收益率标准差×√天数×√(年度交易天数) 2.隐含波动率计算:隐含波动率是根据期权价格计算的,它反映了市场参与者对未来价格波动的预期。
步骤:1.收集目标资产的期权合约价格。
2.使用期权定价模型(如布莱克-斯科尔斯期权定价模型)来计算隐含波动率。
3.通过对期权价格的归一化,将价格转化为波动率。
隐含波动率是从事期权交易的投资者对未来波动率的预期,因此它反映了市场对资产未来可能波动的看法。
总结:波动率是评估资产价格或市场波动性的一种方法,对于投资者来说是非常重要的风险指标。
收益波动率计算
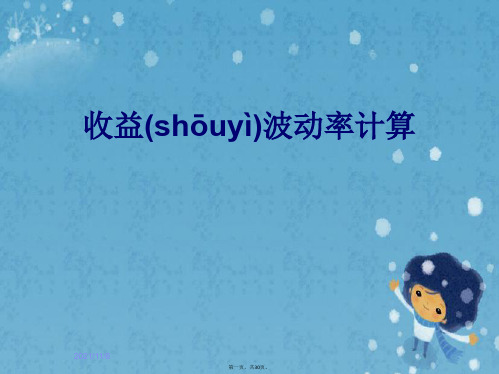
多个股票(gǔpiào)波动率计算
利用简单加权移动平均(SMA)模型计算日波动率。 将日对数收益率5, 10, 20天交叠滚动,输出日波动率 数据集:sma_05;sma_10;sma_20。 上述三个数据集的变量包括(bāokuò):日期、用移动 平均法计算的全部A股股票的日波动率。 计算上述三个数据集日波动率在全样本期的均值、最 大、最小值,输出数据集:sma_051; sma_101; sma_201。 上述三个数据集的变量包括(bāokuò):股票代码、股 票名称、样本数、样本期波动率均值、最大值和最小 值。
proc sort data=b; by Date; data sma_&aa; /*数据集中(jízhōng)保存的是用移动平均法计算的日波动率*/ merge sma_&aa b; by Date; proc means data=b noprint; var z&x; output out=c max=max mean=mean min=min; data c(drop=_type_); set c; stkcd=substr("&x",4,6); lstknm="&y"; data sma_&bb; /*数据集中(jízhōng)保存的是日波动率在全样本期的均值、最大、 最小值*/ set sma_&bb c; %mend a; %include mac; %mend b; %b(05,051,6); %b(10,101,11); %b(20,201,21); run;
第七页,共30页。
GARCH模型(móxíng)
GARCH(Generalized Autoregressive Conditional Heteroskedasticity)模型称广义自回归条件异方差模型, 或称为广义ARCH模型, GARCH模型假定收益的方差服从一个(yī ɡè)可预测的过程, 它依赖于最新的收益,也依赖于先前的方差。
波动率 的加权计算方式
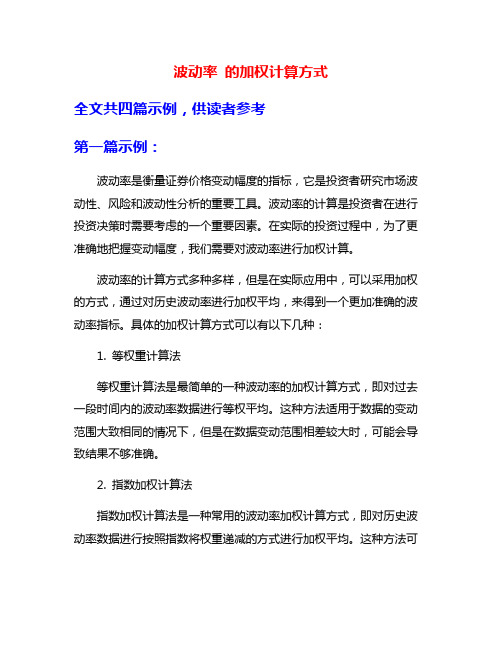
波动率的加权计算方式全文共四篇示例,供读者参考第一篇示例:波动率是衡量证券价格变动幅度的指标,它是投资者研究市场波动性、风险和波动性分析的重要工具。
波动率的计算是投资者在进行投资决策时需要考虑的一个重要因素。
在实际的投资过程中,为了更准确地把握变动幅度,我们需要对波动率进行加权计算。
波动率的计算方式多种多样,但是在实际应用中,可以采用加权的方式,通过对历史波动率进行加权平均,来得到一个更加准确的波动率指标。
具体的加权计算方式可以有以下几种:1. 等权重计算法等权重计算法是最简单的一种波动率的加权计算方式,即对过去一段时间内的波动率数据进行等权平均。
这种方法适用于数据的变动范围大致相同的情况下,但是在数据变动范围相差较大时,可能会导致结果不够准确。
2. 指数加权计算法指数加权计算法是一种常用的波动率加权计算方式,即对历史波动率数据进行按照指数将权重递减的方式进行加权平均。
这种方法可以更好地反映近期的波动情况,更适用于投资者需要更加关注短期波动情况的情况。
在实际应用中,我们可以根据具体的投资需求和市场情况选择适用的加权计算方式来计算波动率。
不同的加权计算方式会影响到波动率的准确度和适用性,投资者需要根据自身的需求和情况来选择合适的计算方法。
波动率的加权计算方式是投资者在进行投资决策时需要考虑的一个重要因素。
通过选择合适的加权计算方式,可以更准确地把握市场的波动情况,对投资决策起到重要的指导作用。
希望以上内容能够帮助投资者更好地理解波动率的加权计算方式及其应用。
第二篇示例:波动率是金融市场中一个重要的指标,用来衡量资产价格的波动程度,是投资者评估风险和收益的重要指标之一。
在金融领域中,波动率可以通过多种方式计算,其中之一就是加权计算方式。
加权计算方式是一种通过给不同数据赋予不同权重来计算波动率的方法。
在实际应用中,通常会将历史数据按照时间顺序进行排序,并给不同的数据赋予不同的权重,然后根据这些权重计算波动率。
波动率的计算金融数学
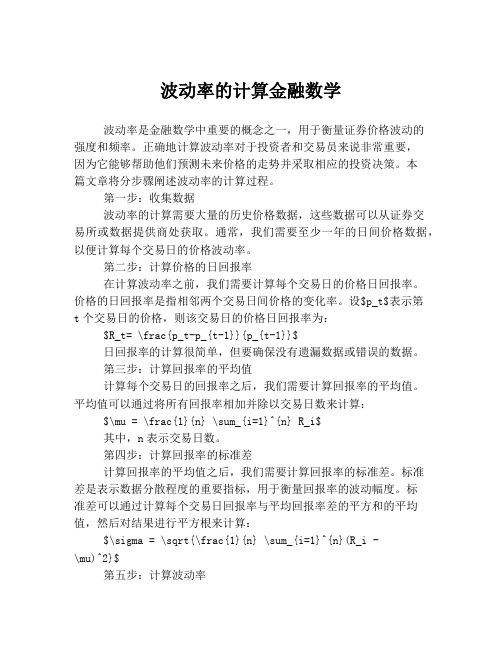
波动率的计算金融数学波动率是金融数学中重要的概念之一,用于衡量证券价格波动的强度和频率。
正确地计算波动率对于投资者和交易员来说非常重要,因为它能够帮助他们预测未来价格的走势并采取相应的投资决策。
本篇文章将分步骤阐述波动率的计算过程。
第一步:收集数据波动率的计算需要大量的历史价格数据,这些数据可以从证券交易所或数据提供商处获取。
通常,我们需要至少一年的日间价格数据,以便计算每个交易日的价格波动率。
第二步:计算价格的日回报率在计算波动率之前,我们需要计算每个交易日的价格日回报率。
价格的日回报率是指相邻两个交易日间价格的变化率。
设$p_t$表示第t个交易日的价格,则该交易日的价格日回报率为:$R_t= \frac{p_t-p_{t-1}}{p_{t-1}}$日回报率的计算很简单,但要确保没有遗漏数据或错误的数据。
第三步:计算回报率的平均值计算每个交易日的回报率之后,我们需要计算回报率的平均值。
平均值可以通过将所有回报率相加并除以交易日数来计算:$\mu = \frac{1}{n} \sum_{i=1}^{n} R_i$其中,n表示交易日数。
第四步:计算回报率的标准差计算回报率的平均值之后,我们需要计算回报率的标准差。
标准差是表示数据分散程度的重要指标,用于衡量回报率的波动幅度。
标准差可以通过计算每个交易日回报率与平均回报率差的平方和的平均值,然后对结果进行平方根来计算:$\sigma = \sqrt{\frac{1}{n} \sum_{i=1}^{n}(R_i -\mu)^2}$第五步:计算波动率现在,我们可以使用标准差来计算波动率。
波动率是标准差的百分比表示,通常用年化百分比来表达。
计算波动率的公式如下:$\text{Volatility} = \sigma \times \sqrt{252}$其中,252表示每年的交易日数。
这个公式意味着,每年的波动率等于每日波动率乘以根号252,因为每年有252个交易日。
波动率计算例子

波动率计算例子股票波动率(也称波幅)是指一支股票在一段时间内价格变动的幅度,是投资者和研究人员研究股价变动趋势、研究证券市场行情水平、考察股市风险等方面的重要指标之一。
股票波动率计算方式有多种,下面以简单算法计算为例来说明计算股票波动率的各个步骤。
首先,我们需要确定一支股票,用A股票作为计算榜样。
假设历史记录的股价数据如下所示:11月3日,该股的价格为14元;11月4日,该股的价格为13元;11月5日,该股的价格为15元;11月6日,该股的价格为16元。
接下来,我们要算出每日收益率,公式为:收益率=(当日股价-昨日股价)/昨日股价。
计算后,11月3日,收益率为0;11月4日,收益率为-1/14;11月5日,收益率为1/13;11月6日,收益率为1/15。
第三步,计算每日收益率的标准差,公式为:标准差=√[(1/n)*sum((x-x̅)(x-x̅)的平方)],其中n为计算的数据的个数,x为每日收益率,x̅为每日收益率的均值。
最后,用相应的公式计算波动率,公式为:波动率=标准差*距今时间长度/年化距今时间长度。
其中,距今时间可以以天数为单位,也可以以月份或周数为单位,比如以计算A股一个月的股价波动率,距今时间长度为4天,而年化距今时间长度为365天。
根据这些数据,我们就可以计算出A股在1个月内的波动率,结果如下:波动率=标准差*4/365=(0.45)*4/365≈0.62%以上就是股票波动率的简单算法的具体操作步骤,它的优点是易于计算,缺点是用一段较短时间内的股价数据来计算股票波动率,得出的结果不太准确,这可能会导致投资者的错误判断。
在实践中,投资者们还可以根据不同的计算方式,来更全面、准确地分析股票波动率。
波动率研究
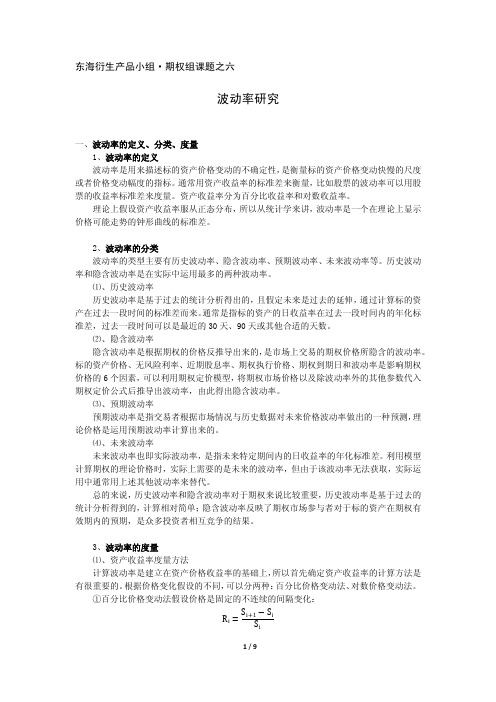
=σ
我们知道,随着样本容量的增大,总体标准差与样本标准差之间的偏差越小,偏差程度 可以表示为: s = b(N)σ 式中:b N =
2 τ (2 ) 就是总体标准差的无偏估计。
通常,为了得到更准确的结果,会使用更多的样本数据进行估计,以尽量减小抽样误差 带来的影响。但是,样本量过多,样本中更可能掺杂与当前市场状态无关的数据。所以一般 有两种方法解决抽样误差的问题, 一是可以通过使用更高频率的估计量, 二是使用没有完全 剔除收盘价以外所有数据的估计量。上述的计算都是基于收盘价-收盘价的关系,为了得到 更好的估计量,需要引入更多的数据信息,从而得到以下四种估计量: ①、Parkinson 估计量: 1 4N ln 2
方差。 λ 越小意味着越早期的波动率对当前波动率的影响越小, 而越近期的波动对当前波动 率的影响越大。这个方法的优点是简单易用,便于理解,缺点是不够灵敏。如果一件事情确 实一个异常事件,比如业绩公告之类的,如果在未来预测期内确定没有类似的事情发生,那 最好将它从数据集中剔除。 该方法另一问题在于它并没有考虑最近的波动率估计量所处的市 场环境,比如波动率的均值回复特性。 2、广义自回归条件异方差(GARCH)模型族 由于这类模型引入了预期回复的长期平均方差水平项, 所以如果当前方差水平处于高位, 我们会期待它在短期内维持高位, 但最终还是会回归到正常水平。 GARCH(1,1)模型表达式如 下: 2 2 σ2 t = γ V + β σ t −1 + α rt −1 式中, V 是长期方差水平项, γ + α + β = 1。 所以 GARCH(1,1)模型表达式一般可表示为: 2 2 σ t =ω +β σ 2 t −1 + α rt −1 所以,V = 1−α
波动率计算方式及应用思考
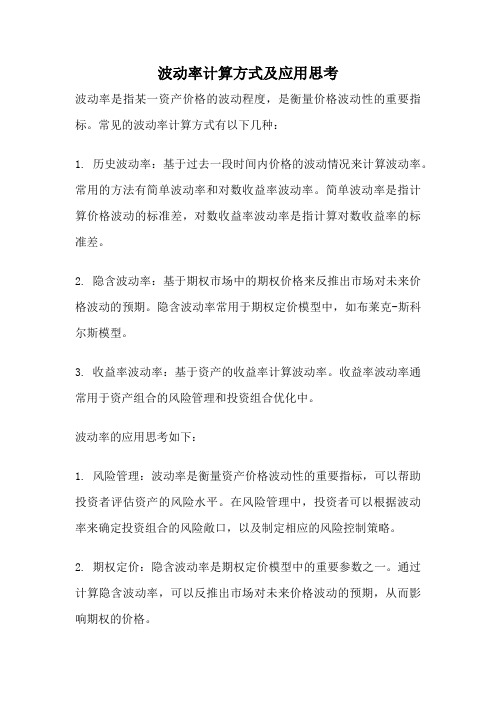
波动率计算方式及应用思考
波动率是指某一资产价格的波动程度,是衡量价格波动性的重要指标。
常见的波动率计算方式有以下几种:
1. 历史波动率:基于过去一段时间内价格的波动情况来计算波动率。
常用的方法有简单波动率和对数收益率波动率。
简单波动率是指计算价格波动的标准差,对数收益率波动率是指计算对数收益率的标准差。
2. 隐含波动率:基于期权市场中的期权价格来反推出市场对未来价格波动的预期。
隐含波动率常用于期权定价模型中,如布莱克-斯科尔斯模型。
3. 收益率波动率:基于资产的收益率计算波动率。
收益率波动率通常用于资产组合的风险管理和投资组合优化中。
波动率的应用思考如下:
1. 风险管理:波动率是衡量资产价格波动性的重要指标,可以帮助投资者评估资产的风险水平。
在风险管理中,投资者可以根据波动率来确定投资组合的风险敞口,以及制定相应的风险控制策略。
2. 期权定价:隐含波动率是期权定价模型中的重要参数之一。
通过计算隐含波动率,可以反推出市场对未来价格波动的预期,从而影响期权的价格。
3. 投资组合优化:波动率可以作为投资组合优化的一个约束条件。
投资者可以根据波动率来平衡投资组合的风险和收益,以实现最优的资产配置。
4. 量化交易:波动率可以作为量化交易策略中的一个信号指标。
通过监测波动率的变化,可以捕捉价格波动的机会,制定相应的交易策略。
波动率是投资领域中常用的风险指标,对于风险管理、期权定价、投资组合优化和量化交易等方面都具有重要的应用价值。
波动率计算的三种方法
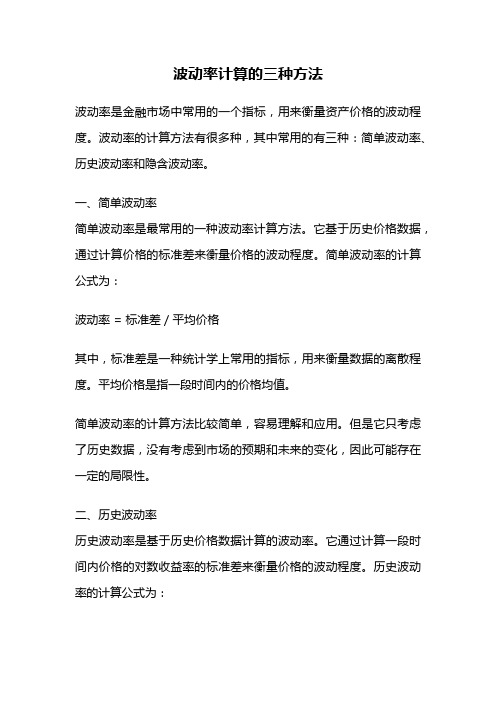
波动率计算的三种方法波动率是金融市场中常用的一个指标,用来衡量资产价格的波动程度。
波动率的计算方法有很多种,其中常用的有三种:简单波动率、历史波动率和隐含波动率。
一、简单波动率简单波动率是最常用的一种波动率计算方法。
它基于历史价格数据,通过计算价格的标准差来衡量价格的波动程度。
简单波动率的计算公式为:波动率 = 标准差 / 平均价格其中,标准差是一种统计学上常用的指标,用来衡量数据的离散程度。
平均价格是指一段时间内的价格均值。
简单波动率的计算方法比较简单,容易理解和应用。
但是它只考虑了历史数据,没有考虑到市场的预期和未来的变化,因此可能存在一定的局限性。
二、历史波动率历史波动率是基于历史价格数据计算的波动率。
它通过计算一段时间内价格的对数收益率的标准差来衡量价格的波动程度。
历史波动率的计算公式为:波动率 = 标准差 / 平均对数收益率其中,对数收益率是指价格的对数变化。
历史波动率考虑了历史数据的波动情况,相对于简单波动率更加准确。
但是它也存在一个问题,就是对历史数据的依赖性较强,可能无法准确反映未来的波动情况。
三、隐含波动率隐含波动率是市场参与者对未来波动率的预期。
它是通过期权的市场价格反推出来的,可以被视为市场对未来波动的一种估计。
隐含波动率的计算方法比较复杂,需要使用期权定价模型来计算。
隐含波动率的计算方法相对于前两种方法更加复杂,但它可以提供更准确的预测。
因为它是市场参与者对未来波动的共识,反映了市场的预期。
波动率是衡量资产价格波动程度的重要指标。
常用的波动率计算方法有三种:简单波动率、历史波动率和隐含波动率。
每种方法都有其优缺点,应根据实际情况选择合适的方法进行计算和分析。
在使用波动率进行投资决策时,还需要考虑其他因素的影响,做出准确的判断和预测。
1_波动率的计算
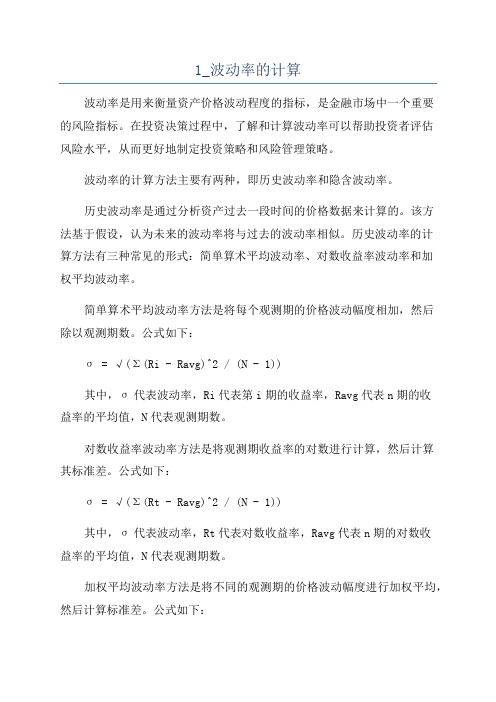
1_波动率的计算波动率是用来衡量资产价格波动程度的指标,是金融市场中一个重要的风险指标。
在投资决策过程中,了解和计算波动率可以帮助投资者评估风险水平,从而更好地制定投资策略和风险管理策略。
波动率的计算方法主要有两种,即历史波动率和隐含波动率。
历史波动率是通过分析资产过去一段时间的价格数据来计算的。
该方法基于假设,认为未来的波动率将与过去的波动率相似。
历史波动率的计算方法有三种常见的形式:简单算术平均波动率、对数收益率波动率和加权平均波动率。
简单算术平均波动率方法是将每个观测期的价格波动幅度相加,然后除以观测期数。
公式如下:σ = √(Σ(Ri - Ravg)^2 / (N - 1))其中,σ代表波动率,Ri代表第i期的收益率,Ravg代表n期的收益率的平均值,N代表观测期数。
对数收益率波动率方法是将观测期收益率的对数进行计算,然后计算其标准差。
公式如下:σ = √(Σ(Rt - Ravg)^2 / (N - 1))其中,σ代表波动率,Rt代表对数收益率,Ravg代表n期的对数收益率的平均值,N代表观测期数。
加权平均波动率方法是将不同的观测期的价格波动幅度进行加权平均,然后计算标准差。
公式如下:σ = √(Σw_i(Ri - Ravg)^2)其中,σ代表波动率,Ri代表第i期的收益率,Ravg代表n期的收益率的加权平均值,wi代表第i期的权重。
除了历史波动率,投资者还可以使用隐含波动率来衡量未来价格的波动。
隐含波动率是反推出来的指标,是根据期权市场上的期权价格推测出来的预期波动率,代表了市场对未来波动率的预期。
使用隐含波动率的方法主要有两种:布莱克-修尔斯公式和季度化波动率。
布莱克-修尔斯公式是根据欧式期权的定价公式反向估算波动率的方法。
该方法假定市场上的期权价格合理,通过反推波动率来与市场价格进行匹配。
通过反复计算,可以得到合理的波动率估计。
季度化波动率方法是将年化的波动率值除以一个合适的季度因子,将波动率转化为季度水平的估计,以便进行更加准确的风险评估。
波动率的计算
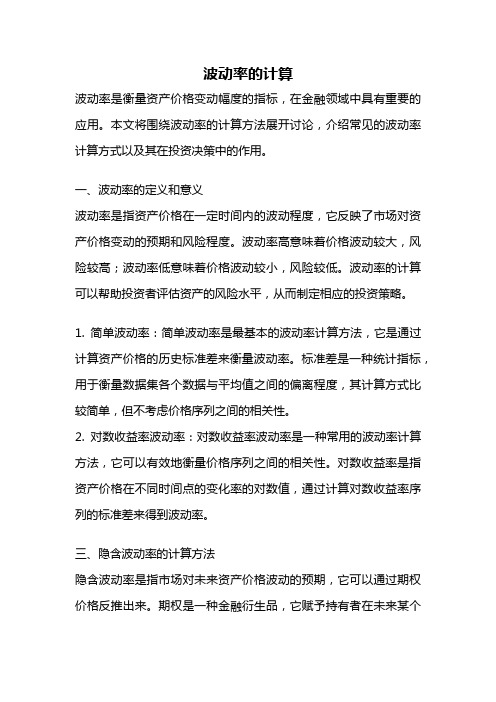
波动率的计算波动率是衡量资产价格变动幅度的指标,在金融领域中具有重要的应用。
本文将围绕波动率的计算方法展开讨论,介绍常见的波动率计算方式以及其在投资决策中的作用。
一、波动率的定义和意义波动率是指资产价格在一定时间内的波动程度,它反映了市场对资产价格变动的预期和风险程度。
波动率高意味着价格波动较大,风险较高;波动率低意味着价格波动较小,风险较低。
波动率的计算可以帮助投资者评估资产的风险水平,从而制定相应的投资策略。
1. 简单波动率:简单波动率是最基本的波动率计算方法,它是通过计算资产价格的历史标准差来衡量波动率。
标准差是一种统计指标,用于衡量数据集各个数据与平均值之间的偏离程度,其计算方式比较简单,但不考虑价格序列之间的相关性。
2. 对数收益率波动率:对数收益率波动率是一种常用的波动率计算方法,它可以有效地衡量价格序列之间的相关性。
对数收益率是指资产价格在不同时间点的变化率的对数值,通过计算对数收益率序列的标准差来得到波动率。
三、隐含波动率的计算方法隐含波动率是指市场对未来资产价格波动的预期,它可以通过期权价格反推出来。
期权是一种金融衍生品,它赋予持有者在未来某个时间点以特定价格买入或卖出资产的权利。
根据期权定价模型,可以通过已知期权价格和其他参数推导出隐含波动率,从而反映市场对未来价格波动的预期。
四、波动率在投资决策中的应用1. 风险管理:波动率是衡量资产风险的重要指标,投资者可以根据波动率的水平来评估资产的风险水平,并制定相应的风险管理策略,如设置止盈止损点位,控制仓位规模等。
2. 选股策略:在股票投资中,波动率可以用来评估股票的波动程度,投资者可以选择波动率较高的股票进行投资,以获取更高的收益机会。
3. 期权交易:隐含波动率是期权定价的重要参数,投资者可以根据隐含波动率的水平来判断期权价格是否偏高或偏低,从而制定相应的期权交易策略。
波动率是衡量资产价格变动幅度的重要指标,通过不同的计算方法可以得到历史波动率和隐含波动率。
资产波动率计算方法(一)
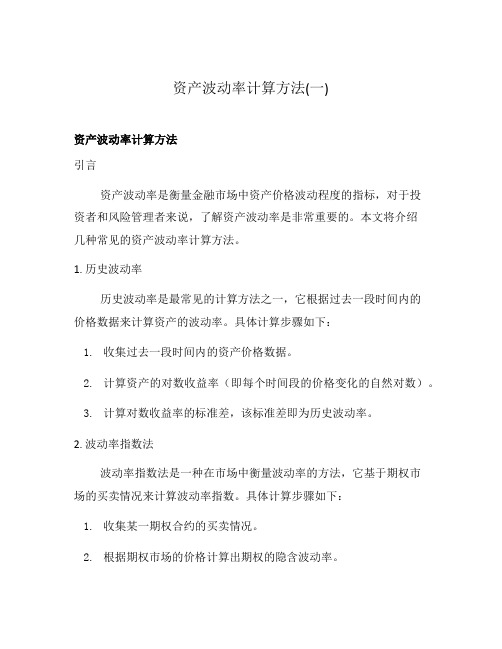
资产波动率计算方法(一)资产波动率计算方法引言资产波动率是衡量金融市场中资产价格波动程度的指标,对于投资者和风险管理者来说,了解资产波动率是非常重要的。
本文将介绍几种常见的资产波动率计算方法。
1. 历史波动率历史波动率是最常见的计算方法之一,它根据过去一段时间内的价格数据来计算资产的波动率。
具体计算步骤如下:1.收集过去一段时间内的资产价格数据。
2.计算资产的对数收益率(即每个时间段的价格变化的自然对数)。
3.计算对数收益率的标准差,该标准差即为历史波动率。
2. 波动率指数法波动率指数法是一种在市场中衡量波动率的方法,它基于期权市场的买卖情况来计算波动率指数。
具体计算步骤如下:1.收集某一期权合约的买卖情况。
2.根据期权市场的价格计算出期权的隐含波动率。
3.将多个期权的隐含波动率加权平均,得到波动率指数。
3. GARCH模型GARCH(Generalized Autoregressive Conditional Heteroskedasticity)模型是一种时间序列分析模型,通过考虑资产价格的自回归和条件异方差性,来计算波动率。
具体计算步骤如下:1.根据历史数据估计资产价格的自回归项和条件异方差项。
2.根据自回归项和条件异方差项预测未来资产价格的波动率。
4. 基于波动率交易的模型基于波动率交易的模型是一种基于资产波动率进行投资决策的方法。
它通过根据波动率预测,选取适当的投资组合来获取超额收益。
具体计算步骤如下:1.收集资产价格和波动率数据。
2.根据波动率数据预测未来的波动率。
3.根据预测的波动率选择适当的投资组合。
结论以上介绍了几种常见的资产波动率计算方法,每种方法都有其适用的场景和优缺点。
选择合适的计算方法需要根据具体的需求和数据情况来进行判断。
希望本文对读者了解资产波动率计算有所帮助。
注意:本文仅供参考,投资决策需谨慎。
5. 隐含波动率法隐含波动率法是一种基于期权市场来计算资产波动率的方法。
【波动率研究】系列之一:波动率分类与特点介绍

【波动率研究】系列之一:波动率分类与特点介绍随着金融产品的创新,波动率成为衍生品定价和风险管理技术中最关键的参数之一。
对于衍生品交易员而言,无论是交易期权、或是交易更加复杂的金融衍生品,他们或多或少都需要对交易产品未来的波动水平进行预估。
一般获得波动率可通过两种角度:一是历史波动率法,二是隐含波动率法。
前一种指基于标的资产历史交易数据获得波动率的方法;后一种指的是通过现在期权价格反推隐含的波动率。
本文就成这两个角度出发,介绍波动率的分类以及其各自计算方法与特点。
一、历史波动率法1.1 不同估计方法的理论介绍A.样本标准差通过计算过去一段时间交易标的的对数收益率的标准差,作为该段时间交易价格的波动率。
其公式如下:简单的说,上面就是统计学中方差的计算公式。
另外由于衍生品定价公式采用的是年化波动率,所以一般计算出来的标准差都需要经过年化处理。
一般年化的方法是乘以相应单位周期一年交易时间的平方根。
由于一年交易日大概252天,从而以日线获得的标准差需要乘以252的平方根,获得年化波动率;如果是以周线获得的标准差需要乘以252/5的平方根,获得年化波动率;如果以月线获得的标准差需要乘以12(=252/21)的平方根,获得年化波动率。
B.极差波动率上面介绍的标准差波动率,作为统计学中标准算法,其没有考虑很多实际情况。
很多学者在其基础上,做出了一些改进。
分别介绍如下:Parkinson(1980)用交易区间内最高价和最低价两个价格数据,利用极差进行估计波动率。
该估计方法的优点在于只需要较少的时间周期就可以收敛于真实波动率。
缺点在于没有考虑隔夜、价格存在漂移的特征,同时未必是无偏统计,是一种经验性的研究分析。
Garman-Klass(1980)用交易区间内最高价、最低价和收盘价三个价格数据进行波动率估计。
该估计方法的优点在于一方面只需要较少的时间周期就可以收敛于真实波动率,另一方面可通过将估计量除以调整因子来纠正存在的偏差,以便得到方差的无偏估计。
历史波动率的计算方法

历史波动率的计算方法步骤1:收集资产价格数据首先,需要收集与所要计算波动率的资产相关的历史价格数据。
这些数据可以从金融数据供应商、证券交易所或相关的网站上获得。
通常,应选择一段时间内的每日收盘价或其他时间间隔的价格。
时间段的长度通常根据具体情况决定,可以是几个月、一年或更长的时间。
步骤2:计算资产的收益率基于收集的价格数据,需要计算资产的收益率。
收益率是指资产价格的变化百分比,通常使用对数收益率来计算。
对数收益率是通过取自然对数(ln)来表示价格变化的百分比。
对数收益率的计算公式如下:r(t) = ln(P(t) / P(t-1))其中,r(t)是时间t的收益率,P(t)是时间t的价格,P(t-1)是时间t-1的价格。
步骤3:计算平均收益率获得资产的收益率之后,需要计算这些收益率的平均值。
平均收益率可以通过计算所有收益率的算术平均值来获得。
步骤4:计算波动率σ = √(Σ(r(t) - r(avg))^2 / (n-1))其中,σ是波动率,r(t)是每个时间点的收益率,r(avg)是收益率的平均值,n是数据的数量。
步骤5:年化波动率根据历史波动率的计算结果,可以将其转化为年化波动率。
这是为了方便对不同时间段的资产进行比较。
年化波动率可以通过将波动率乘以每年的交易日数量的平方根来计算。
通常,一年的交易日数量为252天。
annualized_volatility = σ * √(n)其中,annualized_volatility是年化波动率,σ是波动率,n是数据的数量。
步骤6:数据的优化和调整在计算历史波动率之前,可能需要对数据进行一些优化和调整,以确保数据的准确性和可靠性。
例如,经常出现中断或数据缺失的价格可以通过选择较短的时间段来规避。
此外,还可以通过使用加权移动平均值等价格平滑技术来消除价格的瞬时波动。
总结起来,历史波动率的计算方法包括收集资产价格数据、计算资产的收益率、计算平均收益率、计算标准差、计算年化波动率以及数据的优化和调整。
股票波动率怎么算?股票波动率计算公式是什么
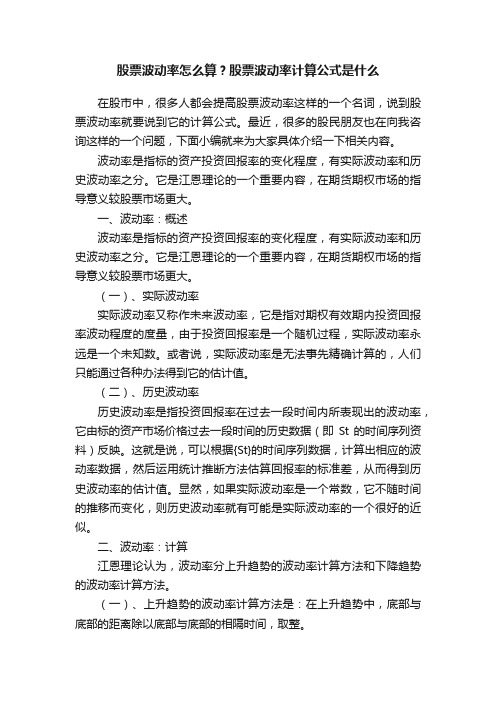
股票波动率怎么算?股票波动率计算公式是什么在股市中,很多人都会提高股票波动率这样的一个名词,说到股票波动率就要说到它的计算公式。
最近,很多的股民朋友也在向我咨询这样的一个问题,下面小编就来为大家具体介绍一下相关内容。
波动率是指标的资产投资回报率的变化程度,有实际波动率和历史波动率之分。
它是江恩理论的一个重要内容,在期货期权市场的指导意义较股票市场更大。
一、波动率:概述波动率是指标的资产投资回报率的变化程度,有实际波动率和历史波动率之分。
它是江恩理论的一个重要内容,在期货期权市场的指导意义较股票市场更大。
(一)、实际波动率实际波动率又称作未来波动率,它是指对期权有效期内投资回报率波动程度的度量,由于投资回报率是一个随机过程,实际波动率永远是一个未知数。
或者说,实际波动率是无法事先精确计算的,人们只能通过各种办法得到它的估计值。
(二)、历史波动率历史波动率是指投资回报率在过去一段时间内所表现出的波动率,它由标的资产市场价格过去一段时间的历史数据(即St的时间序列资料)反映。
这就是说,可以根据{St}的时间序列数据,计算出相应的波动率数据,然后运用统计推断方法估算回报率的标准差,从而得到历史波动率的估计值。
显然,如果实际波动率是一个常数,它不随时间的推移而变化,则历史波动率就有可能是实际波动率的一个很好的近似。
二、波动率:计算江恩理论认为,波动率分上升趋势的波动率计算方法和下降趋势的波动率计算方法。
(一)、上升趋势的波动率计算方法是:在上升趋势中,底部与底部的距离除以底部与底部的相隔时间,取整。
上升波动率=(第二个底部-第一个底部)/两底部的时间距离(二)、下降趋势的波动率计算方法是:在下降趋势中,顶部与顶部的距离除以顶部与顶部的相隔时间,取整。
并用它们作为坐标刻度在纸上绘制。
下降波动率=(第二个顶部-第一个顶部)/两顶部的时间距离。
股票波动率指标计算公式

股票波动率指标计算公式股票波动率是衡量股票价格变动幅度的一个重要指标,也是投资者评估风险和收益的重要依据。
波动率越高,代表股票价格波动越大,风险也相应增加。
为了更好地理解和使用股票波动率指标,下面将介绍波动率指标的计算公式,并探讨其应用和解读方法。
股票波动率指标有多种计算方法,其中最常用的是历史波动率和隐含波动率。
历史波动率是通过分析过去一段时间的股票价格变动数据来计算的,而隐含波动率则是通过期权市场上的期权价格推算出来的。
历史波动率的计算公式如下:1. 首先,计算每天的对数收益率。
对数收益率可以通过计算每天的股票收盘价与前一天的收盘价之间的差异来得到。
公式如下:对数收益率 = ln(当天收盘价 / 前一天收盘价)2. 计算每日的对数收益率的平方,并对这些平方数进行求和。
公式如下:平方和= Σ(每日对数收益率^2)3. 将每日的对数收益率的平方和除以总天数的减一,然后再乘以250(一年的交易日数)来得到年化波动率。
公式如下:历史波动率= √(平方和 / (总天数 - 1)) × √250隐含波动率的计算公式则较为复杂,需要通过期权价格等参数来推算。
简单来说,隐含波动率是指使得市场观察者能够根据期权定价模型得出的理论价格等于市场上实际交易价格的波动率。
隐含波动率的计算一般是由期权定价模型(如Black-Scholes模型)进行的。
股票波动率指标的应用非常广泛。
对于投资者而言,波动率可以用来评估股票的风险水平。
波动率较高的股票通常存在较大的价格波动,风险也相应增加,因此投资者可能需要承担更大的风险。
此外,波动率的计算还可以用于构建投资组合的优化,帮助投资者更好地分配资金。
解读波动率时,一般来说,波动率越高代表股票价格波动范围越大,市场风险也相应增加。
投资者可以根据波动率的大小来调整自己的投资策略,比如在高波动率时减少仓位或谨慎持有,以降低风险。
同时,波动率也可以被视为判断股票市场情绪的一个指标。
上下行波动率因子公式

上下行波动率因子公式
摘要:
1.波动率因子的定义
2.上下行波动率因子公式的推导
3.上下行波动率因子公式的应用
4.总结
正文:
一、波动率因子的定义
波动率因子是金融领域中一个重要的概念,用于衡量资产价格波动的不确定性。
波动率因子可以反映市场对未来风险的预期,因此在投资策略中具有很高的实用价值。
二、上下行波动率因子公式的推导
为了更深入地了解波动率因子,我们首先需要了解上下行波动率因子公式的推导过程。
在此过程中,我们假设有一个资产价格的时间序列{X_t},其对应的对数收益率为{r_t}。
根据定义,波动率因子可以表示为:
波动率因子= E[(r_t - μ)^2] / σ^2
其中,E[·] 表示期望,(r_t - μ) 是收益率的偏差,σ^2 是收益率的方差。
三、上下行波动率因子公式的应用
上下行波动率因子公式在实际应用中有很多优势,例如:
1.可以用于衡量资产价格的波动性,为投资者提供风险管理的依据;
2.可以用于预测未来收益,帮助投资者制定投资策略;
3.可以用于评估投资组合的绩效,为投资者提供有效的决策依据。
四、总结
综上所述,上下行波动率因子公式是金融领域中一个重要的概念,它可以用来衡量资产价格波动的不确定性,并为投资者提供风险管理和投资决策的依据。
如何计算波动率?

如何计算波动率?
在标准的定义中,波动率就是指标的价格的波动程度。
波动率的表现形式也有很多。
常见的分类是将通过现有的历史数据计算出的波动率称为历史波动率(Historical Volatility)或是实际波动率(Realized Volatility),习惯上简称为HV 或RV。
常用的HV计算方法十分简单,即某段时间内标的涨跌幅的标准差。
这里有两个时间参数,其一是时间段的总长,与均线系统中的时间参数概念相同,常用有20日、40日、60日、120日HV;其二就是涨跌幅计算的频率,习惯上采用每日收盘数据,当然也可以根据需要截取更高频的日内数据。
用收盘价对收盘价(Close to Close)计算得到的HV有明显的缺陷,就是无法显示盘中与隔夜跳空的波动。
长上下影线究竟能不能反映行情的实际波动呢?这就见仁见智了。
当然,即使选择忽略这些信息也可以将其作为参考。
可以采用Parkinson方法将日内振幅纳入计算,或加入开盘价计算出隔夜跳空波动,或直接用Garman and Klass 方法将日内振幅与跳空全部纳入计算,当然还有其他更为细致的方法,这里不多做介绍,可以参考《Volatility Trading》(by Euan Sinclair)进一步学习。
IV的计算虽然涉及BS模型,但实际操作也非常简便,只要将标的价格、到期时间、行权价等参数逐一输入模型即可得到IV值。
随着国内期权市场不断完善,能够支持IV计算的工具、软件层出不穷,不一定非要自行计算。
来源:期权投资。
- 1、下载文档前请自行甄别文档内容的完整性,平台不提供额外的编辑、内容补充、找答案等附加服务。
- 2、"仅部分预览"的文档,不可在线预览部分如存在完整性等问题,可反馈申请退款(可完整预览的文档不适用该条件!)。
- 3、如文档侵犯您的权益,请联系客服反馈,我们会尽快为您处理(人工客服工作时间:9:00-18:30)。
2
The price range, defined as the difference between the highest and lowest market prices over a fixed sampling interval, has been known for a long time and recently experienced renewed interest as an estimator of the latent volatility. The information contained in the opening, highest, lowest, and closing prices of an asset is widely used in Japanese candlestick charting techniques and other technical indicators (Nisson, 1991). Early application of range in the field of finance can be traced to Mandelbrot (1971), and the academic work on the range-based volatility estimator started from the early 1980s. Several authors, back to Parkinson (1980), developed from it several volatility measures far more efficient than the classical return-based volatility estimators.
Keywords: CARR, DCC, GARCH, high/low range, realized volatility, multivariate volatility, volatility.
*
Corresponding author. Contact address: Institute of Economics, Academia Sinica, #128, Yen-Jio-Yuan Road, Sec 2, Nankang, Taipei, Taiwan. Telephone: 886-2-27822791 ext 321, fax: 886-2-27853946, email: rchou@.tw. 1
Range Volatility Models and Their Applications in Finance
Ray Yeutien Chou* Institute of Economics, Academia Sinica & Institute of Business Management, National Chiao Tung University
Building on the earlier results of Parkinson (1980), many studies1 show that one can use the price range information to improve volatility estimation. In addition to being significantly more efficient than the squared daily return, Alizadeh, Brandt, and Diebold (2002) also demonstrate that the conditional distribution of the log range is approximately Gaussian, thus greatly facilitating maximum likelihood estimation of stochastic volatility models. Moreover, as pointed by Alizadeh, Brandt, and Diebold (2002), and Brandt and Diebold (2006), the range-based volatility estimator appears robust to microstructure noise such as bid-ask bounce. By adding microstructure noise to the Monte Carlo simulation, Shu and Zhang (2006) also support that the finding of Alizadeh, Brandt, and Diebold (2002), that range estimators are fairly robust toward microstructure effects.
This version: January 6, 2009
Abstract There has been a rapid growth of range volatility due to the demand of empirical finance. This paper contains a review of the important development of range volatility, including various range estimators and range-based volatility models. In addition, other alternative models developed recently, such as range-based multivariate volatility models and realized ranges, are also considered here. At last, this paper provides some relevant financial applications for range volatility.
Hengchih Chou Department of Shipping & Transportation Management, National Taiwan Ocean University
Nathan Liu Institute of Finance, National Chiao Tung University
ቤተ መጻሕፍቲ ባይዱ
1
See Garman and Klass (1980), Beckers(1983), Ball and Torous (1984), Wiggins (1991), Rogers and Satchell (1991), Kunitomo (1992), Yang and Zhang (2000), Alizadeh, Brandt and Diebold (2002), Brandt and Diebold (2006), Brandt and Jones (2006), Chou (2005, 2006), Cheung (2007), Martens and van Dijk (2007), Chou and Wang (2007), Chou, Liu and Wu (2007), and Chou and Liu (2008a,b). 3
I. Introduction With the continual development of new financial instruments, there is a growing demand for theoretical and empirical knowledge of the financial volatility. It is well-known that financial volatility has played such a central role in derivative pricing, asset allocation, and risk management. Following Barndorff-Nielsen and Shephard (2003) or Andersen et al. (2003), financial volatility is a latent factor and hence is not directly observable, however. Financial volatility can only be estimated using its signature on certain known market price process; when the underlying process is more sophisticated or when observed market prices suffer from market microstructure noise effects, the results are less clear.
It is well known that many financial time series exhibit volatility clustering or autocorrelation. In incorporating the characteristics into the dynamic process, the generalized autoregressive conditional heteroskedasticity (GARCH) family of models proposed by Engle (1982) and Bollerslev (1986), and the stochastic volatility (SV) models advocated by Taylor (1986) are two popular and useful alternatives for estimating and modeling time-varying conditional financial volatility. However, as pointed by Alizadeh, Brandt, and Diebold (2002), Brandt and Diebold (2006), Chou (2005) and other authors, both GARCH and SV models are inaccurate and inefficient, because they are based on the closing prices, of the reference period, failing to used the information contents inside the reference. In other words, the path of the price inside the reference period is totally ignored when volatility is estimated by these models. Especially in turbulent days with drops and recoveries of the markets, the traditional close-to-close volatility indicates a low level while the daily price range shows correctly that the volatility is high.