西瓜无损检测方法
论文-利用超声检测西瓜的成熟度
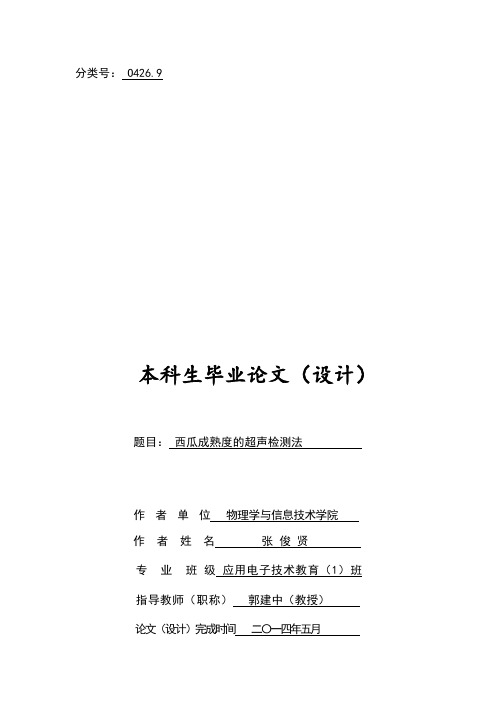
分类号: O426.9本科生毕业论文(设计)题目:西瓜成熟度的超声检测法作者单位物理学与信息技术学院作者姓名张俊贤专业班级应用电子技术教育(1)班指导教师(职称)郭建中(教授)论文(设计)完成时间二〇一四年五月西瓜成熟度的超声检测法张俊贤陕西师范大学物理学与信息技术学院,陕西西安 710062摘要: 超声波法无损检测技术以其操作简单、快速、适应性强、检测精度较高等特点而在农产品品质无损检测中得到了广泛应用。
为达无损检测西瓜成熟度的目的,本研究在自主搭建的超声学检测台上采集透过西瓜的超声学信号,提取超声从脉冲发射点传到接收点过程中声透过率参数(δ)。
从中选取相关系数前30位的声透过率值,借助软件中多元线性回归函数建立多元线性回归方程。
研究不同发射点和信号接收点组合所建模型的预测值和实测值。
试验结果表明,发射点和接收点位于赤道部位能获得较好的测定模型。
利用Matlab软件中的仿真技术,对超声衰减与西瓜硬度进行仿真。
成熟度指数与坚硬度有较为接近的线性关系。
因此,用西瓜成熟度指数来检验西瓜的成熟程度是一种比较有效的无损检测方法。
关键词:西瓜;超声衰减;Matlab仿真;坚硬度1前言随着现代食品工业的发展以及为了满足市场竞争的需求,人们越来越多地把主要精力投向提高产品品质。
因此,建立有效的农产品无损检测方法对促进食品产业健康蓬勃发展以及提高国民生活质量发挥着重要作用。
在农产品加工中,水果的成熟度以及随后的质量评估都非常重要。
要求有一种可靠、快速、非破坏性的检测技术,用来测量水果中与成熟和品质有关的一些物理性质。
西瓜作为世界上的重要水果之一,为人们所喜爱,给人们带来可观的经济效益。
在判断西瓜成熟度方面,人们积累了丰富的经验,比如“计算生长期”,“观察外部形态”,“触、拍、压、闻”等无损检验法,为消费者提供成熟的西瓜起到了关键作用。
但这些方法依赖于个人经验等主观因素,尤其消费者难以掌握。
然而按西瓜成熟度分级销售,对销售者和消费者都是有益的。
西瓜品质检测

2.2 动态特性测试法
▪ 在不同预加载荷、激振功率、成熟程度等条 件下,测定动态试验的弹性模量和相位角。 测试原理是正弦交变应力- 应变动态试验。 作轴向加载时,正弦力由正弦波发生器经过 放大器后,由电动式振荡器产生,一个压电 加速度传感器固定到振荡器轴上,样品夹在 两个圆盘之间,一个圆盘连接到振动器上, 另一个经动态力传感器连接到刚性支撑之间, 以测定静态预载荷,加速度和力传感器的信 号经放大,通过水平和垂直通道输入存储示 波器,通过调节功率放大器改变激振功率 (测定输入激振器的功率获得激振功率)
托盘运动到成熟度测定处,两个定位臂自动动作,使其停止在 预定位置上。三个互成120℃可沿导杆垂直上下移动的听筒状声 波传感器根据果高测定装置测出的果高,自动下移至西瓜赤道 高度后,再水平移动,与西瓜表面接触。此时,和声波传感器, 同步下移的打击装置动作打击西瓜。打击声波被三个传感器检 测,经放大及模式转换后,输入电子计算机进行声波解析,并 根据预定的鉴别基准,判断成熟度和内部空洞,判定结果输出 到成熟度表示装置,控制臂动作压下托盘上相应的表示装置。 然后传感器和打击装置离开西瓜并升起,完成一次测定。该装 置测定一个西瓜只需4s。
西瓜物理ห้องสมุดไป่ตู้质主要包括质量、硬度、 等方面。
判定西瓜物理品质的方法主要有声 波振动法、动态特性测试法
2.1声波振动法
利用打击声波解析测定装置,判定 西瓜的成熟度及内部空洞。
2.1.1 西瓜声波检测装置示意图
2.1.2 基本检测原理
果品的声波特性随内部果肉的性质不同而 变化。西瓜果实受到打击时,产生的声波 的特点与西瓜的内部品质有关。
声波的特点:波形 功率谱峰值频率 对称度 指数衰减率
未熟西瓜
▪ 打击声波为非对称 波 形,波形对称度 为 0.55-0.80,指 数衰减率为0.400.76由快速傅立叶 变换法解析出的功 率谱峰值频率为 164-280Hz
无损检测水果品质的方法及果品仪器清单

无损检测水果品质的方法及果品仪器清单水果品质的主要评定指标通常包括糖度、酸度、成熟度、新鲜度、硬度等多种理化指标。
传统的水果品质评定方法主要是化学分析法,需要耗费相当的化学试剂,测试分析过程烦琐,速度慢,通常用测定少量的样本来代替批次水果的品质,而且测试时必须破坏水果,在水果品质分级中实际意义不大。
近年来兴起的无损检测法,依据水果物理力学、光学、电学、生物学特性,在不破坏水果本身测定了水果的相关理化指标,不需要化学试剂和烦琐的分析过程,方法简单、快捷,测试数据准确性高,有利于实现果品内部品质的在线分级控制,因此有广泛的应用前景。
水果品质的无损检测法在国内外均有相当的研究,有的已得到商业应用。
本文将介绍电特性测试法、动态特性测试法、近红外线测试法、声学特性分析法等几种水果无损检测法的测试原理。
1、果品仪器—电特性测试法水果的生物组织内部存在大量带电粒子形成的生物电场,水果在生长成熟、受损及腐败变质过程中的生物化学反应将伴随物质和能量的转换,导致生物组织内各类化学物质所带电荷量及电荷的空间分布的变化。
生物电场的分布和强度,从宏观上影响水果的电特性。
Thompson 等人研究了300~900 MHz 频段内苹果电特性的变化规律,发现苹果电特性与其成熟度密切相关。
Mclendon 等人发现在0.5~5 kHz频段内梨的介电常数和损耗角正切随频率增长而呈减小趋势。
但随着梨的逐渐成熟,两者均逐渐增大。
Nelson 等人在0.2~20 GHz 频段内对多种水果进行了研究,结果表明所测试的水果的介电常数随频率的增加均稳定减小。
加藤宏郎在10 Hz~13 MHz 频段内对比分析了损坏水果与正常水果在电特性方面的差异,结果表明损坏水果的串、并联等效电阻及阻抗在该频段内低于正常水果,而串联等效电容及损耗因数值则比正常水果大,串联等效电阻与水果的新鲜度或成熟度相关。
水果内部品质与宏观电特性的关系如下:水果电特性的检测方法有切片、突刺、接触和非接触等方法,前二种属于有损检测,后二种将被测水果直接放入平板电极间测定其电特性参数,属于无损检测。
西瓜成熟度音频无损检测技术
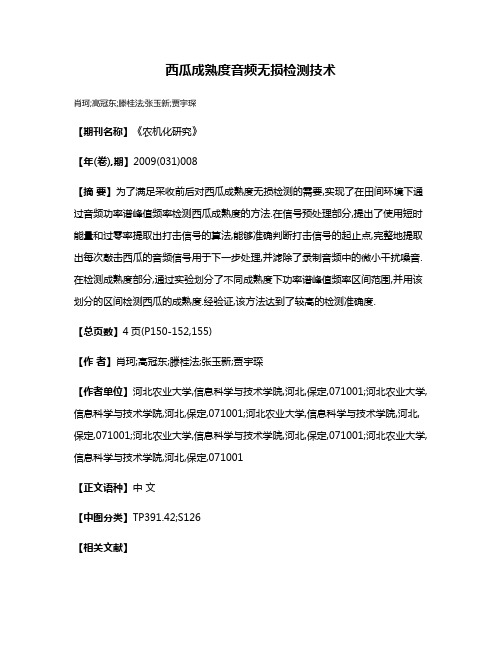
西瓜成熟度音频无损检测技术
肖珂;高冠东;滕桂法;张玉新;贾宇琛
【期刊名称】《农机化研究》
【年(卷),期】2009(031)008
【摘要】为了满足采收前后对西瓜成熟度无损检测的需要,实现了在田间环境下通过音频功率谱峰值频率检测西瓜成熟度的方法.在信号预处理部分,提出了使用短时能量和过零率提取出打击信号的算法,能够准确判断打击信号的起止点,完整地提取出每次敲击西瓜的音频信号用于下一步处理,并滤除了录制音频中的微小干扰噪音.在检测成熟度部分,通过实验划分了不同成熟度下功率谱峰值频率区间范围,并用该划分的区间检测西瓜的成熟度.经验证,该方法达到了较高的检测准确度.
【总页数】4页(P150-152,155)
【作者】肖珂;高冠东;滕桂法;张玉新;贾宇琛
【作者单位】河北农业大学,信息科学与技术学院,河北,保定,071001;河北农业大学,信息科学与技术学院,河北,保定,071001;河北农业大学,信息科学与技术学院,河北,保定,071001;河北农业大学,信息科学与技术学院,河北,保定,071001;河北农业大学,信息科学与技术学院,河北,保定,071001
【正文语种】中文
【中图分类】TP391.42;S126
【相关文献】
1.小型西瓜果实成熟度的无损定性判别 [J], 李永玉;赵洪卫;常冬;韩东海
2.基于音频特性的西瓜成熟度无损检测技术研究 [J], 张玉新;赵洋;陶佳
3.浅谈具有成熟度无损检测功能的8424型西瓜大棚控制系统 [J], 耿同
4.基于MEMS的西瓜成熟度无损振动检测 [J], 涂文俊;张宇;耿涛;李晓;王薪淼
5.西瓜成熟度无损检测技术的研究进展 [J], 黄语燕;朱亨银
因版权原因,仅展示原文概要,查看原文内容请购买。
论文-利用超声检测西瓜的成熟度
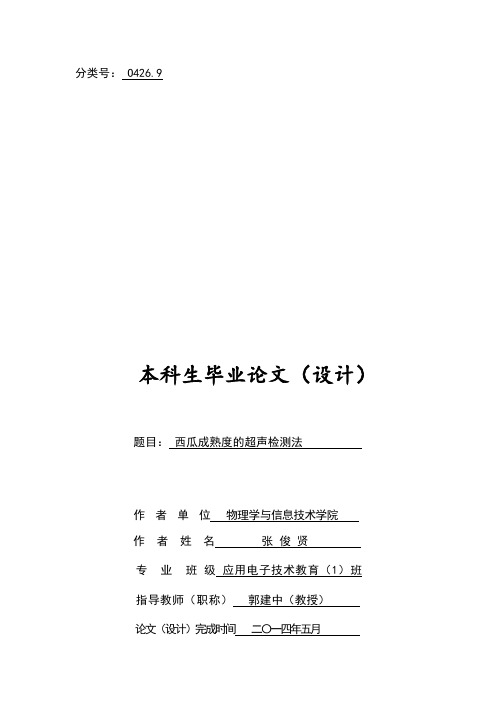
分类号: O426.9本科生毕业论文(设计)题目:西瓜成熟度的超声检测法作者单位物理学与信息技术学院作者姓名张俊贤专业班级应用电子技术教育(1)班指导教师(职称)郭建中(教授)论文(设计)完成时间二〇一四年五月西瓜成熟度的超声检测法张俊贤陕西师范大学物理学与信息技术学院,陕西西安 710062摘要: 超声波法无损检测技术以其操作简单、快速、适应性强、检测精度较高等特点而在农产品品质无损检测中得到了广泛应用。
为达无损检测西瓜成熟度的目的,本研究在自主搭建的超声学检测台上采集透过西瓜的超声学信号,提取超声从脉冲发射点传到接收点过程中声透过率参数(δ)。
从中选取相关系数前30位的声透过率值,借助软件中多元线性回归函数建立多元线性回归方程。
研究不同发射点和信号接收点组合所建模型的预测值和实测值。
试验结果表明,发射点和接收点位于赤道部位能获得较好的测定模型。
利用Matlab软件中的仿真技术,对超声衰减与西瓜硬度进行仿真。
成熟度指数与坚硬度有较为接近的线性关系。
因此,用西瓜成熟度指数来检验西瓜的成熟程度是一种比较有效的无损检测方法。
关键词:西瓜;超声衰减;Matlab仿真;坚硬度1前言随着现代食品工业的发展以及为了满足市场竞争的需求,人们越来越多地把主要精力投向提高产品品质。
因此,建立有效的农产品无损检测方法对促进食品产业健康蓬勃发展以及提高国民生活质量发挥着重要作用。
在农产品加工中,水果的成熟度以及随后的质量评估都非常重要。
要求有一种可靠、快速、非破坏性的检测技术,用来测量水果中与成熟和品质有关的一些物理性质。
西瓜作为世界上的重要水果之一,为人们所喜爱,给人们带来可观的经济效益。
在判断西瓜成熟度方面,人们积累了丰富的经验,比如“计算生长期”,“观察外部形态”,“触、拍、压、闻”等无损检验法,为消费者提供成熟的西瓜起到了关键作用。
但这些方法依赖于个人经验等主观因素,尤其消费者难以掌握。
然而按西瓜成熟度分级销售,对销售者和消费者都是有益的。
基于无损检测技术的西瓜糖度数据库的设计与实现
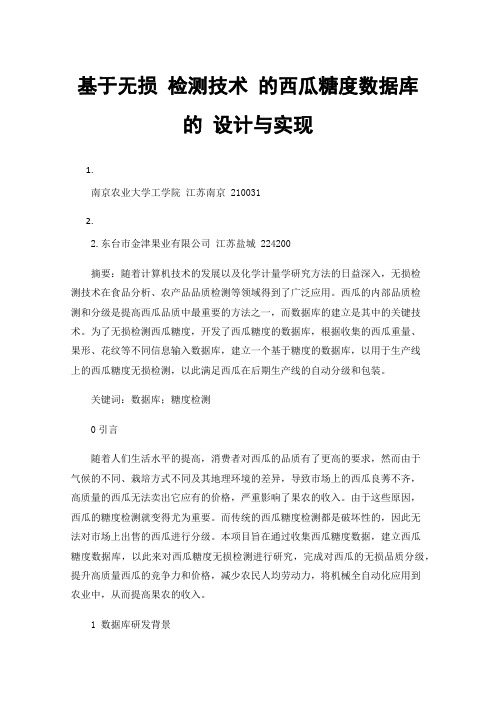
基于无损检测技术的西瓜糖度数据库的设计与实现1.南京农业大学工学院江苏南京 2100312.2.东台市金津果业有限公司江苏盐城 224200摘要:随着计算机技术的发展以及化学计量学研究方法的日益深入,无损检测技术在食品分析、农产品品质检测等领域得到了广泛应用。
西瓜的内部品质检测和分级是提高西瓜品质中最重要的方法之一,而数据库的建立是其中的关键技术。
为了无损检测西瓜糖度,开发了西瓜糖度的数据库,根据收集的西瓜重量、果形、花纹等不同信息输入数据库,建立一个基于糖度的数据库,以用于生产线上的西瓜糖度无损检测,以此满足西瓜在后期生产线的自动分级和包装。
关键词:数据库;糖度检测0引言随着人们生活水平的提高,消费者对西瓜的品质有了更高的要求,然而由于气候的不同、栽培方式不同及其地理环境的差异,导致市场上的西瓜良莠不齐,高质量的西瓜无法卖出它应有的价格,严重影响了果农的收入。
由于这些原因,西瓜的糖度检测就变得尤为重要。
而传统的西瓜糖度检测都是破坏性的,因此无法对市场上出售的西瓜进行分级。
本项目旨在通过收集西瓜糖度数据,建立西瓜糖度数据库,以此来对西瓜糖度无损检测进行研究,完成对西瓜的无损品质分级,提升高质量西瓜的竞争力和价格,减少农民人均劳动力,将机械全自动化应用到农业中,从而提高果农的收入。
1 数据库研发背景九十年代,N Sarkar、Dabenel A 等专家学者便开始使用计算机的图像处理手段和模式识别技术对果品大小、表面损伤等分级进行了研究。
Gutiérrez 等使用移动高光谱成像和不同的机器学习算法在田间条件下对大量葡萄品种进行分类。
日本对水果内部检测技术进行研究是在上世纪八十年代,截止目前,全球最先进的水果分级筛选设备是由日本MAKI公司制造的,该设备可以做到在线同时检测多种指标,可以判断水果内部病变,检测速度可以达到每秒三个及以上。
意大利的SACMI公司具有温湿度补偿功能的西瓜糖度、酸度、成熟度和空心的高精度检测近红外光谱检测分级装备。
基于MEMS的西瓜成熟度无损振动检测

本系统使用 / . 2 m z 来表示西瓜的成熟度 ( 其中 , 代表西瓜 的一 阶 固有频率 H z ,m代表西瓜的质量 ) 。选择这个指数的另外一个原 因 是它与 国外对水果成熟度无损检测所取的指数一致 。 国外研究甜瓜 、 桃、 苹果 、菠萝等水果时也采用 厂 m 作 为成熟度 的物理检验指数 ,以代 替有损伤 的压缩试验的检验方法 。 而且成熟度指数与含糖量有较为线性 的关 系, 因此用西瓜成熟度指数来检验西瓜 的成熟度是一种 比较有效的
通过 多次试验发现 :对于质量一定 的西瓜 ,振动基频越高 ,含糖量
越低 ; 但 西瓜 的含糖量还与其质量有一定 的关系 , 即对于振动基频一定
的西瓜 , 质量越大 , 含糖量也越低 。 西瓜的成熟度指数与质量和频率都
有关系。 成熟西瓜 的固有频率范围 :1 1 0 ~ 1 6 0 H z 。 成熟度指数标 准 p = 7 5 0 0 0 , 小于该指数则西瓜成熟 , 大 于该指数为
、
引言
近年来 , 随着对水果需求 的提高 , 国内外水果 的产量逐年上升 , 人 们对水果 品质也有 了更高的要求 。 研究表明水果在采摘 、 包装 、 储 运及 加工等作业环节 中, 由于不同成熟度的水果相互混杂等原因造成的损失 率高达 3 0 . 4 5 %, 若能根据水果的不同物理特性对水果 品质进行评 定与 分级 , 从 而对过 于成熟和未成熟的水果能及时加工 , 对提高储藏质量 是 非常重要 的, 为此 , 国内外许多科研工作者对水果 的物理特性进行了大
感器 电路 、单片机、按键 、L C D显示器 。系统结构如 图 l 所示 : 8 6 中国电子商务 2 0 1 3 ・ 0 5
试验选用的西瓜 品种为 “ 景欣二号” , 选取数量为 1 5 个 ,图 3为对
小型西瓜果实成熟度的无损定性判别
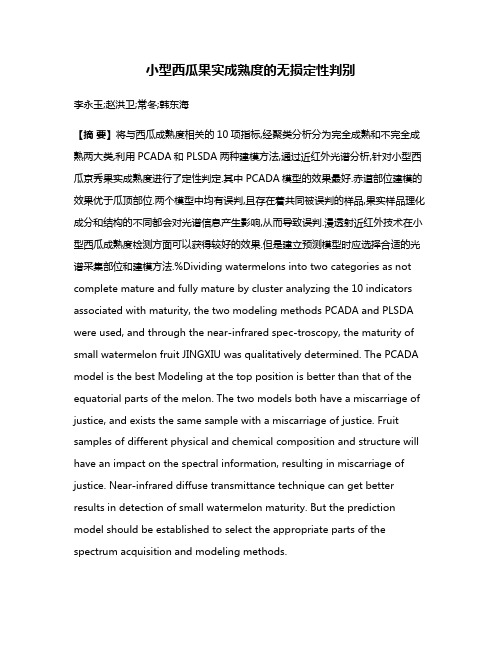
小型西瓜果实成熟度的无损定性判别李永玉;赵洪卫;常冬;韩东海【摘要】将与西瓜成熟度相关的10项指标,经聚类分析分为完全成熟和不完全成熟两大类,利用PCADA和PLSDA两种建模方法,通过近红外光谱分析,针对小型西瓜京秀果实成熟度进行了定性判定.其中PCADA模型的效果最好.赤道部位建模的效果优于瓜顶部位.两个模型中均有误判,且存在着共同被误判的样品,果实样品理化成分和结构的不同都会对光谱信息产生影响,从而导致误判.漫透射近红外技术在小型西瓜成熟度检测方面可以获得较好的效果.但是建立预测模型时应选择合适的光谱采集部位和建模方法.%Dividing watermelons into two categories as not complete mature and fully mature by cluster analyzing the 10 indicators associated with maturity, the two modeling methods PCADA and PLSDA were used, and through the near-infrared spec-troscopy, the maturity of small watermelon fruit JINGXIU was qualitatively determined. The PCADA model is the best Modeling at the top position is better than that of the equatorial parts of the melon. The two models both have a miscarriage of justice, and exists the same sample with a miscarriage of justice. Fruit samples of different physical and chemical composition and structure will have an impact on the spectral information, resulting in miscarriage of justice. Near-infrared diffuse transmittance technique can get better results in detection of small watermelon maturity. But the prediction model should be established to select the appropriate parts of the spectrum acquisition and modeling methods.【期刊名称】《光谱学与光谱分析》【年(卷),期】2012(032)006【总页数】5页(P1526-1530)【关键词】小型西瓜;果实成熟度;近红外光谱;定性判别【作者】李永玉;赵洪卫;常冬;韩东海【作者单位】中国农业大学工学院,北京100083;中国农业大学食品科学与营养工程学院,北京100083;中国农业大学工学院,北京100083;中国农业大学食品科学与营养工程学院,北京100083【正文语种】中文【中图分类】O433.4现有西瓜品质光谱学无损检测多为定量检测。
西瓜无损检测
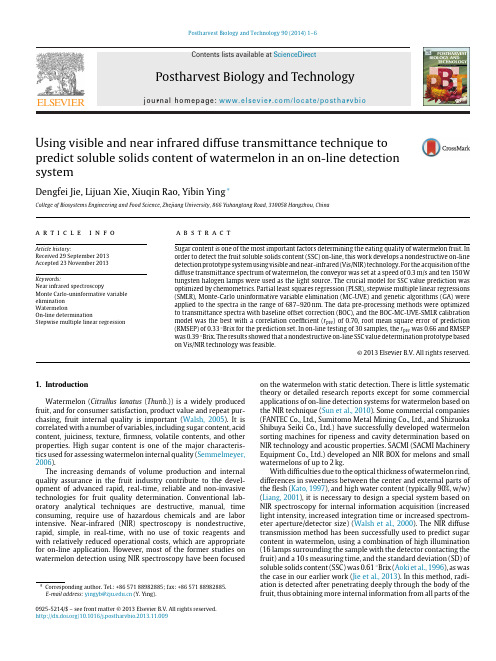
Postharvest Biology and Technology 90(2014)1–6Contents lists available at ScienceDirectPostharvest Biology andTechnologyj o u r n a l h o m e p a g e :w w w.e l s e v i e r.c o m /l o c a t e /p o s t h a r v b ioUsing visible and near infrared diffuse transmittance technique to predict soluble solids content of watermelon in an on-line detection systemDengfei Jie,Lijuan Xie,Xiuqin Rao,Yibin Ying ∗College of Biosystems Engineering and Food Science,Zhejiang University,866Yuhangtang Road,310058Hangzhou,Chinaa r t i c l ei n f oArticle history:Received 29September 2013Accepted 23November 2013Keywords:Near infrared spectroscopyMonte Carlo-uninformative variable elimination WatermelonOn-line determinationStepwise multiple linear regressiona b s t r a c tSugar content is one of the most important factors determining the eating quality of watermelon fruit.In order to detect the fruit soluble solids content (SSC)on-line,this work develops a nondestructive on-line detection prototype system using visible and near-infrared (Vis/NIR)technology.For the acquisition of the diffuse transmittance spectrum of watermelon,the conveyor was set at a speed of 0.3m/s and ten 150W tungsten halogen lamps were used as the light source.The crucial model for SSC value prediction was optimized by chemometrics.Partial least squares regression (PLSR),stepwise multiple linear regressions (SMLR),Monte-Carlo uninformative variable elimination (MC-UVE)and genetic algorithms (GA)were applied to the spectra in the range of 687–920nm.The data pre-processing methods were optimized to transmittance spectra with baseline offset correction (BOC),and the BOC-MC-UVE-SMLR calibration model was the best with a correlation coefficient (r pre )of 0.70,root mean square error of prediction (RMSEP)of 0.33◦Brix for the prediction set.In on-line testing of 30samples,the r pre was 0.66and RMSEP was 0.39◦Brix.The results showed that a nondestructive on-line SSC value determination prototype based on Vis/NIR technology was feasible.©2013Elsevier B.V.All rights reserved.1.IntroductionWatermelon (Citrullus lanatus (Thunb.))is a widely produced fruit,and for consumer satisfaction,product value and repeat pur-chasing,fruit internal quality is important (Walsh,2005).It is correlated with a number of variables,including sugar content,acid content,juiciness,texture,firmness,volatile contents,and other properties.High sugar content is one of the major characteris-tics used for assessing watermelon internal quality (Semmelmeyer,2006).The increasing demands of volume production and internal quality assurance in the fruit industry contribute to the devel-opment of advanced rapid,real-time,reliable and non-invasive technologies for fruit quality determination.Conventional lab-oratory analytical techniques are destructive,manual,time consuming,require use of hazardous chemicals and are labor intensive.Near-infrared (NIR)spectroscopy is nondestructive,rapid,simple,in real-time,with no use of toxic reagents and with relatively reduced operational costs,which are appropriate for on-line application.However,most of the former studies on watermelon detection using NIR spectroscopy have been focused∗Corresponding author.Tel.:+8657188982885;fax:+8657188982885.E-mail address:yingyb@ (Y.Ying).on the watermelon with static detection.There is little systematic theory or detailed research reports except for some commercial applications of on-line detection systems for watermelon based on the NIR technique (Sun et al.,2010).Some commercial companies (FANTEC Co.,Ltd.,Sumitomo Metal Mining Co.,Ltd.,and Shizuoka Shibuya Seiki Co.,Ltd.)have successfully developed watermelon sorting machines for ripeness and cavity determination based on NIR technology and acoustic properties.SACMI (SACMI Machinery Equipment Co.,Ltd.)developed an NIR BOX for melons and small watermelons of up to 2kg.With difficulties due to the optical thickness of watermelon rind,differences in sweetness between the center and external parts of the flesh (Kato,1997),and high water content (typically 90%,w/w)(Liang,2001),it is necessary to design a special system based on NIR spectroscopy for internal information acquisition (increased light intensity,increased integration time or increased spectrom-eter aperture/detector size)(Walsh et al.,2000).The NIR diffuse transmission method has been successfully used to predict sugar content in watermelon,using a combination of high illumination (16lamps surrounding the sample with the detector contacting the fruit)and a 10s measuring time,and the standard deviation (SD)of soluble solids content (SSC)was 0.61◦Brix (Aoki et al.,1996),as was the case in our earlier work (Jie et al.,2013).In this method,radi-ation is detected after penetrating deeply through the body of the fruit,thus obtaining more internal information from all parts of the0925-5214/$–see front matter ©2013Elsevier B.V.All rights reserved./10.1016/j.postharvbio.2013.11.0092D.Jie et al./Postharvest Biology and Technology 90(2014)1–6Fig.1.Schematic of spectra on-line measurement prototype system.fruit and making whole fruit sugar content assessment possible.SSC was chosen as one of the most important internal quality factors for evaluation.The commercial large-scale on-line fruit internal qual-ity detection instruments using NIR spectroscopy have shown that visible and near-infrared spectroscopy (Vis/NIRS)is a promising technique to predict the SSC value of intact watermelons.To approach the on-line detection SSC value of watermelons,we have developed a complete determination system include the hardware and software for SSC prediction.The main objectives of this work were to:(1)develop a prototype detection system that could be used for on-line detection in a nondestructive way based on the Vis/NIRS technique;(2)further test the usefulness of the system for on-line detection of large watermelon SSC values;(3)simplify the on-line predictive model.2.Materials and methods2.1.On-line detection systemThe on-line measurement prototype system for watermelon sugar content detection (Fig.1)used a motor driven conveyor for fruit movement.There was a hole in the center of the tray (the diameter is 300mm)with diameter of 50mm,and these were placed on the conveyor belt.The free tray was designed to block the light leaking through the contact surface between the sam-ples and the tray,concentric grooves were made inside the tray and ring shape silicon pads were put on the tray to accommo-date varying watermelon shape and size (Abebe,2006).In order to eliminate interference from environmental background radiation as far as possible,an illumination box optical device was used for NIR diffuse transmittance measurement.In this box,light sources comprised ten 150W commercial-grade tungsten halogen lamps powered by a full-stabilized DC power supply.An adjustable col-limating lenses (diameter 50mm)embedded optical fiber (Ocean Optics Inc.,USA)connected to a commercial miniature fiber optic spectrometer (Ocean Optics Inc.,USA)was used to diffuse trans-mitted light collection through the center hole of the free tray.When spectral acquisitions were made,each sample was put on a tray and was fed onto the conveyor belt and moved into the illumination box at a speed of 0.3m/s.The spectrometer was set to external trigger mode to collect spectral data.A pair of optoelec-tronic sensors (SIKO SENSOR Co.,Ltd.,Nanjing,China)acted as a switch of the spectrometer.The position of the trigger was decidedby the relative location of the holes on the conveyor belt and in the tray.When the sample came to the given location in the illumina-tion box,the optoelectronic sensor transmitted an electrical signal to the computer,and then the computer controlled the spectrom-eter to spectral collection automatically in the integration time.Reference and dark spectra were measured before sample spectral measurement.The reference was measured with each set of sam-ples.Spectrometer parameters settings,and spectra collection and storage were carried out via software developed by ourselves.2.2.Spectra acquisitionOne hundred samples of Qilin (Cucimus melo )watermelon were harvested from a local farm in Hangzhou,Zhejiang Province,China.All fruit were cleaned and held at room temperature (25◦C)for 24h before spectra acquisition.The characteristic parameters (equator diameter,length and rind thickness)were obtained using a vernier caliper (Shenhan measurements tools Co.,Ltd.,Shanghai,China).After seven samples with different defects were eliminated,93intact watermelons were employed for further analysis,with 62samples randomly chosen as a calibration set and the other 31as the prediction set (a ratio of 2:1).Samples were manually loaded on the free tray.The measure-ment was expressed as percent transmission (%T ).Integration time was optimized to record maximum counts without saturation,since previous work has shown that if the acquired count level was approximately 5%of detector saturation,it would result in a low signal to noise ratio (SNR)and poor calibration performance (Greensill,2000).The integration time of the spectrometer was set to 200ms.We acquired the spectra after the spectrometer and light source warmed up to a stable status.2.3.SSC determinationAfter spectra acquisition,all watermelons were cut into halves from stem end to calyx end and edible portions were removed and cut into pieces for obtaining watermelon juice using a juicer (Liven SCI-TECH CO.,Ltd.,LL-A small cyclone juicer,Beijing,China),the juice was poured into a conical flask with filter paper (medium speed type,30–50m,Shuangquan Brand,China),and clear juice was obtained.A digital refractometer (Atago Co.,Ltd.,PR-201␣Brix-Meter,Tokyo,Japan)with a ◦Brix range of 0–60and ±0.1◦Brix accuracy was used to determine SSC values (◦Brix).The value of SSCD.Jie et al./Postharvest Biology and Technology90(2014)1–63of each sample was obtained by averaging three measurements of the sample.2.4.Data analysisIn NIRS application,it is always necessary to adopt chemomet-rics,which can reduce the numerous spectral data and establish calibration models for quantitative analysis,and furthermore extend the potential use of the NIRS technique.In our research, building an accurate and robust predictive model was crucial for achieving on-line detection as well as acquiring the spectra effec-tively.Specific application of chemometrics includes three facets: (1)spectral data pre-processing;(2)extraction of feature wave-lengths;(3)establishment of regression models.2.5.Spectra pre-processingSpectra pre-processing sometimes can improve the model’s per-formance.Several spectral pre-processing algorithms,including Savitzky–Golay smoothing(SGS),multiplicative scatter correction (MSC),baseline correction and standard normal variate(SNV)were implemented using“Unscrambler V9.7”(CAMO PROCESS AS,Oslo, Norway).The SNV and MSC had the similar function of reducing the physical variability between samples due to scatter and adjusting for baseline shifts between samples(Dhanoa et al.,1994).Baseline offset correction(BOC)is often used to adjust the spectral offset by adjusting the data to the minimum point in the data.2.6.Regression models2.6.1.Partial least square regression(PLSR)PLSR is used for modeling the linear relationship between the variable matrix X(the spectra)and the variable matrix Y(the prop-erties of interest),but PLSR is based on latent variables(LVs).More details about this method can be found in Helland(2004).We used the PLSR algorithm to establish predictive models such as pre-processing-based models until the variables was reduced low enough to establish the MLR model(Walmsley,1997).2.6.2.Feature wavelengths extractionFor on-line detection based on NIRS,variable selection can make the model simpler and so obtain a better interpretation and lower measurement system cost(Andersen and Bro,2010).Many factors could result in noise,such as motor rotation and fan vibration,so eliminating the wavelengths containing irrelevant information can ensure stability of the predictive model.We therefore need to pick out the feature wavelengths that are most sensitive and contain abundant information.2.6.3.Monte Carlo-uninformative variable elimination(MC-UVE) and genetic algorithm(GA)The UVE method usually uses the stability to evaluate the reli-ability of each variable.For the MC-UVE method,the stability values were obtained by the MC method replacing the leave-one-out (LOO)procedure in UVE.MC cross-validation leaves out a major part of the samples so enhancing the impact of validation on mod-eling and increasing the probability of selecting the best model(Xu et al.,2004).MC-UVE can eliminate the variables that have no more information for modeling than noise;the wavelengths selected by GA are less dispersed than with other methods(Leardi,2000).GA is an adaptive search procedure based on the mechanics of natural genetics and natural selection.It combines the advantages of optimization algorithms based on random processes(stochas-tic methods)with those provided by hill-climbing(deterministic) procedures(Bangalore et al.,1996).Our former research has shown that MC-UVE combined with the GA method were effective to select the feature wavelengths(Jie et al.,2013).We implemented the automated wavelength selection procedure in MATLAB7.11(The Math Works,Natick,USA).2.6.4.Stepwise multivariate linear regression(SMLR)SMLR modeling was performed using a TQ Analyst8.0(Thermo Electron Corp.,USA).The stepwise algorithm of SMLR in the TQ Analyst software automatically determines the most appropriate wavelengths.It begins by selecting thefirst wavelength for the sample component.Additional wavelengths that provide comple-mentary information may be used to generate the best prediction (Xie et al.,2009).2.7.Model evaluationThe evaluation indices of predicted accuracy for all developed models are the correlation coefficient of calibration(r cal)and pre-diction(r pre),the root mean square error of calibration(RMSEC) and prediction(RMSEP)(Cozzolino et al.,2007).Generally,a good model should have higher|r cal|and|r pre|values,lower RMSEC and RMSEP values,and a difference between RMSEC and RMSEP.3.Results and discussion3.1.System considerationThe on-line acquisition of a set of stable spectra from moving fruit samples is significant,it depends on many different parame-ters:first is the characteristics of samples such as the fruit physical nature(size,shape,orientation and density)(Fernández-Ahumada, 2008);second is the instrument parameters such as optical path and integration time,and the average distance from the sample to the optics will significantly influence the quality,repeatability and reproducibility of the spectra generated and integration time should be optimized before spectra collection(Berntsson et al., 2001).To complete an on-line fruit internal quality determina-tion system based on Vis/NIR spectroscopy,we need to incorporate hardware according to characteristics of the samples.In order to have enough light intensity to obtain spectra with high SNR,we installed ten lamps in a special accurate lamp holder at two sides of the conveying rollers to illuminate fruit from both sides.For samples with larger volume,angle of incidence ray is an impor-tant parameter for obtaining a higher SNR of diffuse transmittance spectra;the axis lights and detector with respect to the center of the fruit samples at120◦angles need to provide a balanced area illumi-nation to the watermelon samples.The spectrometer in this work was in the range of200–1110nm and the resolution of0.47nm.3.2.Physical parameters and SSCSome physical parameters used to describe the characteristic of the watermelons are listed in Table1.From Table1,we can see that the watermelon rinds had different thickness at different pos-itions.Rind at the navel was the thinnest(7.88mm in calibration set)compared with the rind at the other two positions(11.81mm and12.77mm in calibration set).In order to acquire as much trans-mittance spectra as possible,we chose the navel side to collect spectra.The SSC values of samples were distributed in different ranges.3.3.Characteristics of spectraMany factors can affect SNR during the process of spectral col-lection,especially fruit with large volumes such as watermelon. Thus it is very important to investigate the stability of the spectra acquisition with the equipment used in our study.Fig.2shows the4 D.Jie et al./Postharvest Biology and Technology90(2014)1–6Table1Statistics of physical parameters and SSC values of watermelon samples in calibration and prediction sets,respectively.Index Weight(g)Height(cm)Diameter(cm)Rind thickness(mm)SSC(◦Brix)Equator Navel StalkCalibration setMin384418.861.38.13 5.01 6.137.47Max560022.569.414.9310.8918.119.37 Mean448520.364.911.817.8812.778.52SD376.50.79 1.95 1.58 1.25 2.390.45Prediction setMin378018.561.38.87 5.788.677.47Max541422.870.015.8411.7718.269.37 Mean454420.465.011.418.1813.578.55SD398.10.80 1.91 1.48 1.36 2.310.46Fig.2.NIR transmission spectra of watermelon samples.spectra obtained by the on-line determination system.The smooth and regular curves are satisfactory.We retained the wavelength range from687to920nm for further analysis.Other wavelength ranges were eliminated because of noise and lack of information.As illustrated in Fig.2,the collected transmittance spectra had similar shapes,though the intensity was obviously different.There were obvious valleys of the curve around738and836nm;two peaks of the curve were located around803and726nm.3.4.Spectral pre-processing based PLSR modelingFirst the PLSR model was established using the original spec-tra as the input for testing the importance of the variables.Thefirst three loading spectra of SSC are shown in Fig.3.FromthisFig.3.Thefirst three loading spectra of SSC.we canfind that thefirst loading spectrum developed features at 718,746,803nm,the second loading spectrum showed other fea-tures at710nm,and the third loading spectrum had features at 716,755,798and832nm.Thus the spectral range of687–920nm used for analysis was reasonable.Different pre-processing was then employed on the original spectra.We established the PLSR models using the different inputs(Table2).The BOC pretreatment showed better performance than orig-inal spectra with a higher correlation coefficient(r pre of0.753) and lower root mean square error(RMSEP of0.295◦Brix).The difference between RMSEC and RMSEP of BOC-PLSR model was smaller(0.022◦Brix)than the raw-PLSR model(0.025◦Brix).The other pretreatment applied to the processed spectra was by BOC. The BOC-Savitzky–Golay smoothing-PLSR showed the same result as BOC-PLSR,and other pretreatments based models showed worse performance.Since data reduction could improve the detection time,the following analyses were carried out using the spectra only with BOC pre-processing.3.5.Feature wavelength extractionEither for MLR modeling or for improving the speed of on-line detection,feature wavelength extraction is very important. Wefirstly implemented SMLR using the BOC processed spectra as input;the variables reduced to14,but the performance of the predictive model was very limited with r pre of0.604and RMSEP of0.388◦Brix.The specific wavelengths were as follows:709.18, 711.44,714.46,718.24,720.50,737.84,740.10,755.89,764.14, 776.14,800.07,913.40,916.33and918.54nm.Then we adopted the MC-UVE approach to reduce irrelevant variables.In the MC-UVE process,the stability was used to evaluate the reliability of each variable in order to decide which one should be eliminated.Fig.4shows the obtained stability value of each vari-able for SSC values through the MC-UVE.The dotted line indicates the threshold value.The cut-off determined the number of the stable variables selected to build thefinal model through MC-UVE. The variables,where absolute values of stability were greater than the absolute threshold value,were selected as the input for PLSR modeling.After the MC-UVE processingfinished,80variables were kept and the corresponding percent transmittance values were Table2PLS modeling after using different pre-processing methods.Pretreatment Calibration Predictionr cal RMSEC r pre RMSEP Raw0.7830.2850.7190.310 BOC0.8030.2730.7530.295 BOC+Smoothing0.8030.2730.7530.295 BOC+SNV0.8430.2460.7460.336 BOC+MSC0.8390.2490.7040.345D.Jie et al./Postharvest Biology and Technology90(2014)1–65Fig.4.Stability distributions of variables for prediction of SSC by the MC-UVE method.used as the inputs for the PLSR model to evaluate the ability of this algorithm.As shown in Table3,the BOC-MC-UVE-PLSR displayed better performance,and the r pre and RMSEP were improved.The80variables selected by MC-UVE were employed as the inputs of SMLR and GA.The GA calculation procedure was conducted ten times,and both the MC-UVE-SMLR and MC-UVE-GA algorithmsfinally retained13variables.The details of the selected wavelengths by BOC-MC-UVE-SMLR and BOC-MC-UVE-GA,are respectively:687.99,711.44,714.46,724.28,727.29,779.88, 782.88,785.12,828.38,835.07,853.62,911.92,916.33nm and 724.28,725.03,760.39,779.88,783.62,815.73,851.40,898.68, 904.57,908.98,911.19,915.60,917.07nm.SMLR retained more wavelengths in the700–800nm range and fewer wavelengths over 900nm than GA.In the principle of NIRS,the band assignments for the major water and sugar(OH and CH)vibrations include sec-ond and third overtones of OH stretching at970and740nm,a OH combination band at840nm,the third overtone of a CH stretch at 910and a CH2stretch at930nm(Golic et al.,2003).The spectra used for analysis ignored the wavelengths above920nm without absorption,so the selection of an area around910nm is logically attributed to the third overtone of the CH stretch.Ito et al.(1998) patented an apparatus to non-destructively measure sugar content of a vegetable using three NIR lasers in the range of860–890nm (specifically910–915nm(SSC),860–890nm and920–925nm non-SSC reference bands).Table3summarizes the performance of the different models using the feature wavelengths.Though the BOC-MC-UVE-PLSR had better prediction results with r pre of0.771 and RMSEP0.285◦Brix,there were80variables,which was more than the BOC-MC-UVE-SMLR model(13variables).We therefore chose BOC-MC-UVE-SMLR as the better one on the basis of fewer wavelengths and faster detection speed.BOC-MC-UVE-SMLR was optimal with r pre of0.701and RMSEP of0.328◦Brix.Table3also shows the number of variables employed in each model.The percentage of wavelengths used to develop the BOC-SMLR,BOC-GA-MLR,BOC-MC-VUE-SMLR andTable3Calibration and prediction results for SSC of watermelons based on different wave-lengths extraction methods.Method No.of variables Calibration Predictionr cal RMSEC r pre RMSEP BOC-PLS3140.8030.2730.7530.295 BOC-MC-UVE-PLS800.8030.2730.7710.285 BOC-SMLR140.8410.2460.6040.388 BOC-MC-UVE-SMLR130.7730.2930.7010.328 BOC-MC-UVE-GA-MLR130.7520.3010.6710.333Fig.5.Scatter plot of predicted versus measured SSC obtained by MC-UVE-SMLR. BOC-MC-UVE-GA-MLR models were 4.45%, 4.14%,and 4.14%, respectively.Feature wavelengths selected by the BOC-MC-UVE-SMLR method were considered suitable to establish predictive models for on-line determination of the SSC values of watermel-ons.Fig.5shows the scatter plot of the predicted results and determined SSC values of the BOC-MC-UVE-SMLR model.It depicts the distribution of the predicted and determined values.Most of the determined SSC values were between8.0and9.0◦Brix,and the predicted SSC values were high,most between8.5and9.5◦Brix. The differences in the SSC values were very small,so unlikely to affect the r pre.3.6.On-line testingBased on the MLR model obtained combined with the hard-ware,we made a few adjustments on the software to carry out on-line testing.Thirty samples were placed on the tray and passed by the detector,and the software recorded predictive SSC val-ues automatically.After spectral acquisition,SSC values were determined using the same approaches as the samples used for modeling.The predictive and determined values are shown in Fig.6. Generally,the largest difference between the determined and pre-dicted SSC value was no more than0.7◦Brix.There were afewFig.6.Predicted and determined SSC obtained by the on-line detection system using the model.6 D.Jie et al./Postharvest Biology and Technology90(2014)1–6more samples that had larger predicted values than determined value.The average predicted SSC value was8.86◦Brix,SD was 0.456and the average determined value was8.73◦Brix,SD0.447; both predicted and determined valuesfluctuated in a definitive range.To improve the performance of the model,we still need an extensive multi-disciplinary investigation,looking at wavelength range,wavelength resolution and stability,detector sensitivity, SNR,spectral sensitivity,power and incident angle of illumination, integration time and calibration model performance.4.ConclusionThis study developed the use of a prototype system designed for on-line determination of watermelon SSC values based on Vis/NIR spectroscopy.The results confirmed that an on-line sys-tem at a speed of0.3m/s,200ms integral time could collect stable fruit spectra.Based on spectra obtained,we adopted different approaches for feature wavelength extraction and to establish cal-ibration models for on-line prediction.Thefinal results showed that the system is feasible and the best model used in this sys-tem was the BOC-MC-UVE-SMLR calibration model,of which only 13variables were adopted and the r pre was0.70,and RMSEP was 0.33◦Brix for the prediction set.However,the precision still needs to be improved for watermelon fruit.In on-line testing of30sam-ples,the r pre was0.66and RMSEP0.39◦Brix.Both the parameters of the hardware and the models applied in the system need to be optimized.Future goals will be a more precise study on the acquisition of spectra and developing a high-speed on-line sorting system.AcknowledgmentsThe authors gratefully acknowledge thefinancial support pro-vided by the Project of‘Twelfth Five-Year-Plan’in National Science and Technology for the Rural Area in China(No.2012AA10A504). ReferencesAbebe,A.T.,2006.Total sugar and maturity evaluation of intact watermelon using near infrared spectroscopy.J.Near Infrared Spectrosc.14,67–70.Andersen, C.M.,Bro,R.,2010.Variable selection in regression–a tutorial.J.Chemometr.24,728–737.Aoki,H.,Kouno,Y.,Matumoto,K.,Mizuno,T.,Maeda,H.,1996.Nondestruc-tive determination of sugar content in melon and watermelon using near infrared(NIR)transmittance.In:Vijiaysegaran,S.,Pauziah,M.,Mohamed, M.S.,Ahmad,S.(Eds.),International Conference on Tropical Fruit.MalaysiaAgricultural Research and Development Institute,Kuala Lumpur,Malaysia, pp.207–218.Bangalore,A.S.,Shaffer,R.E.,Small,G.W.,Arnold,M.A.,1996.Genetic algorithm-based method for selecting wavelengths and model size for use with partial least-squares regression:application to near-infrared spectroscopy.Anal.Chem.68,4200–4212.Berntsson,O.,Danielsson,L.G.,Folestad,S.,2001.Characterization of diffuse reflectancefiber probe sampling on moving solids using a Fourier transform near-infrared spectrometer.Anal.Chim.Acta431,125–131.Cozzolino,D.,Kwiatkowski,M.,Waters,E.,Gishen,M.,2007.A feasibility study on the use of visible and short wavelengths in the near-infrared region for the non-destructive measurement of wine composition.Anal.Bioanal.Chem.387, 2289–2295.Dhanoa,M.,Lister,S.,Sanderson,R.,Barnes,R.,1994.The link between multiplicative scatter correction(MSC)and standard normal variate(SNV)transformations of NIR spectra.J.Near Infrared Spectrosc.2,43–47.Fernández-Ahumada,E.,(Ph.D.thesis)2008.Assessment of the potential of near infrared reflectance spectroscopy to be implemented in the feed manufacturing industry for process control.University of Córdoba.Golic,M.,Walsh,K.,Lawson,P.,2003.Short-wavelength near-infrared spectra of sucrose,glucose,and fructose with respect to sugar concentration and temper-ature.Appl.Spectrosc.57,139–145.Greensill,C.V.,(Ph.D.thesis)2000.Non-invasive assessment of fruit quality by near-infrared spectroscopy for fruit grading in an in-line setting.Central Queensland University.Helland,I.,2004.Partial Least Squares Regression,Encyclopedia of Statistical Sci-ences.John Wiley&Sons,Inc.,Hoboken,NJ,USA.Ito,M.,Iida,J.,Terashima,A.,Kishimoto,T.,1998.In:Ito,M.(Ed.),Non-destructive sugar content measuring apparatus.Sumitomo Metal Mining Co.,Ltd.,USA. Jie,D.F.,Xie,L.J.,Fu,X.P.,Rao,X.Q.,Ying,Y.B.,2013.Variable selection for partial least squares analysis of soluble solids content in watermelon using near-infrared diffuse transmission technique.J.Food Eng.118,387–392.Kato,K.,1997.Electrical density sorting and estimation of soluble solids content of watermelon.J.Agric.Eng.Res.67,161–170.Leardi,R.,2000.Application of genetic algorithm-PLS for feature selection in spectral data sets.J.Chemometr.14,643–655.Liang,C.P.,(M.Sc.thesis)2001.Quality evaluation of Honeydew Melon:effects of ethylene treatment.University of Georgia.Semmelmeyer,E.,2006.OECD guidance on objective testing to determine the ripeness of fruit.J.Fruit Ornam.Plant Res.14,101–112.Sun,T.,Huang,K.,Xu,H.R.,Ying,Y.B.,2010.Research advances in nondestructive determination of internal quality in watermelon/melon:a review.J.Food Eng.100,569–577.Walmsley,A.D.,1997.Improved variable selection procedure for multivariate linear regression.Anal.Chim.Acta354,225–232.Walsh,K.B.,mercial adoption of technologies for fruit grading,with emphasis on NIRS.In:Information and Technology for Sustainable Fruit and Vegetable Production(FRUTIC05),Montpellier,France,pp.399–408.Walsh,K.B.,Guthrie,J.A.,Burney,J.W.,2000.Application of commercially available, low-cost,miniaturised NIR spectrometers to the assessment of the sugar content of intact fruit.Aust.J.Plant Physiol.27,1175–1186.Xie,L.J.,Ying,Y.B.,Ying,T.J.,2009.Rapid determination of ethylene content in toma-toes using visible and short-wave near-infrared spectroscopy and wavelength b.97,141–145.Xu,Q.S.,Liang,Y.Z.,Du,Y.P.,2004.Monte Carlo cross-validation for selecting a model and estimating the prediction error in multivariate calibration.J.Chemometr.18,112–120.。
西瓜成熟度无损检验的冲击振动方法

第15卷第3期1999年9月农业工程学报T ransacti ons of the CSA E V o l .15 N o.3Sep t . 1999西瓜成熟度无损检验的冲击振动方法王书茂① 焦群英(中国农业大学)籍俊杰(河北省农业机械化研究所)摘 要:在试验分析了西瓜的力学和物理特性的基础上,提出了利用西瓜的振动频率响应来判断西瓜成熟度的无损检验方法。
研究表明,传统的判断西瓜成熟度的含糖量与西瓜的振动基频有较好的相关关系。
在此基础上,考虑了西瓜质量的影响,提出了用f 2m 2 3做为西瓜的成熟度指数,并初步确定了“京欣”品种西瓜的成熟度指数范围。
研究结果表明,冲击振动方法是一种比较有效的西瓜成熟度无损检验方法,为工程应用在线测量及分机处理奠定了基础。
关键词:西瓜;成熟度;无损检验;冲击;振动响应收稿日期:1998212218①王书茂,副教授,副院长,北京市清华东路17号 中国农业大学(东校区)车辆工程学院,100083西瓜是我国夏季主要水果。
在判断西瓜成熟度方面,人类已经积累了丰富的经验,如“计算生长期”,“观察外部形态”,“触、拍、压、闻”等无损检验法,为消费者提供成熟的西瓜起到了关键作用。
但这些方法依赖于个人经验等主观因素,尤其消费者难以掌握。
因此,如何科学地、客观地无损检验,按西瓜成熟度分级销售,对销售者和消费者都是有益的。
国内外学者已经开始了这方面的研究。
Yam am o to [1]、何东健[2]等人用测量冲击振动产生的声波特性来研究西瓜等水果品质与固有频率的关系,做了有益的探索。
但由于振动产生的声波易受周围噪声环境的污染,只能在实验室内或夜深人静时进行,难以实用推广。
Kato [3]研制了基于密度的西瓜分级样机,他对80个同一品种的西瓜分别进行了果汁含糖量和整体密度的分析。
他认为西瓜成熟后,密度变小,改变量从不成熟度时的01985g c m 3到成熟时的01888g c m 3。
Chen [4]等人研究了甜瓜的基本力学特性,并用有限元方法计算了甜瓜的振动固有频率和振型。
基于声学特性的西瓜品质无损检测试验研究

基于声学特性的西瓜品质无损检测试验研究Nondestructive testing of watermelon quality based on acousticcharacteristics摘要我国对农产品的质量要求越来越严格,我们将采取许多方法来提高西瓜市场的竞争力。
目的都是为了提高西瓜的品质质量,而想要提高质量就要提升并完善对西瓜的栽培技术。
对于西瓜的采收和分级评定都离不开西瓜内部的品质的无损检测技术支持。
本次的研究就是为了用无损检测方法中的超声来对新瓜内部进行检测。
此次采用声穿透法,利用非金属检测仪,采取双探头进行检测。
根据内置超声波感应平台上通过西瓜果肉的超声波信号,声音的传输速率从脉冲传输点传输到接收点。
声速,波形及增益等。
研究西瓜内部不同点之间的声速、波形、检测不同点之间的声程的变化以及随时间果肉内部发生变化是否有关系,以此来对西瓜的进行分级,判定西瓜果肉质量的检测方法。
主要是通过西瓜在不同方向上声程的衰减变化来对西瓜品质进行鉴定。
关键字:无损检测;声穿透法;质量;衰减;北京理工大学珠海学院2016届本科生毕业论文AbstractOur country is more and more strict to the quality of agricultural products, we will take a lot of methods to improve the competitiveness of the watermelon market, the purpose is to improve the quality of watermelon quality, and to improve the quality of watermelon to improve the cultivation technology, The harvest and grading of watermelon can not be separated from the technical support of NDT for the internal quality of watermelon. The purpose of this study is to detect the inside of the new melon with the ultrasonic method of nondestructive testing. This time, the method of sound penetration is adopted, and the non-metal detector is adopted to detect with double probes. According to the ultrasonic signal of watermelon pulp on the built-in ultrasonic induction platform, the transmission rate of sound is transmitted from the pulse transmission point to the receiving point, including sound path, sound speed, waveform and gain, etc. In order to classify watermelon, the speed and wave form of sound between different points, the change of sound path between different points and whether there is a relationship between the changes of pulp with time were studied. By studying the sound velocity waveform between different points inside the watermelon and detecting whether there is a relationship between the change of sound path between different points and the change within the flesh over time, we can classify the watermelon. The main北京理工大学珠海学院2016届本科生毕业论文method to determine the quality of watermelon flesh is to identify the quality of watermelon by the attenuation of sound path in different directions.Key words: nondestructive testing; Sound penetration; Quality; attenuation;目录目录一、前言 (1)二、西瓜无损检测研究现状 (2)三、西瓜无损检测研究目标、研究内容 (3)(一)研究目标 (3)(二)研究内容 (3)四、超声波检测原理 (4)五、实验方案 (5)(一)研究的基本思路和方法、技术路线 (5)(二)实验步骤 (7)(三)实验测得数据 (8)六、实验分析 (11)北京理工大学珠海学院2016届本科生毕业论文七、总结 (24)参考文献 (25)一、前言随着中国人民物质生活水平的逐步提高,人们对产品质量的追求也在提高。
利用振动理论对西瓜成熟度进行无损检测的研究

第14卷 第2期农业工程学报Vol .14 N o .2 1998年 6月T r ansactions of the CSA E J un. 1998 收稿日期:1997-10-09 ¹葛 屯,副教授,镇江市 江苏理工大学振动所41信箱,212013利用振动理论对西瓜成熟度进行无损检测的研究葛 屯¹ 徐 凌 夏 恒(江苏理工大学)摘 要 基于对西瓜结构振动特性的分析,提出了对西瓜进行理论建模的空心球与实心球理论,通过有限元计算与振动模态试验对比,验证了上述理论的合理性,并找出了多边形振型这一区别成熟与未成熟瓜的特征模态。
关键词 西瓜 振动 成熟度 无损检测瓜果的品质检测多年来一直是许多学者关心的问题,而其中利用声振法对瓜果类产品进行成熟度的无损检测无疑是一种颇有价值的有效方法[1]。
近年来,国外许多科研工作者对此进行了大量的研究,Yam am oto 等人(1980,1981)基于瓜果的声学响应研究了无损测量苹果与西瓜内部质量的技术。
Sasao (1985)利用橡胶球敲击西瓜进行了田间实测西瓜声学响应的研究。
A ffeldt 与Abo ott(1989)研究了评价“红富士”苹果品质的快速声传递法。
Armstrong(1990)测量了苹果的第一阶固有频率并利用弹性球模型的纯压缩振动模式预测了苹果的弹性模量。
P.Chen 等人(1992)研究了影响苹果声学响应的因素,并指出声调强度与苹果的第一、二阶固有频率有直接的相干性。
M .L .Sto ne 等人(1996)研究了利用声脉冲阻抗技术确定西瓜成熟度的方法。
目前这方面的研究在我国尚未得到很好的开展,而无论从学术角度还是应用前景来看,利用声振法对瓜果产品进行无损检测的研究均具有重要意义。
为此笔者近年来通过振动试验模态与动力有限元相结合的方法对国产西瓜进行了大量无损检测研究,在此拟就该问题作一探讨。
1 西瓜的成熟度建模及其动态特性的动力有限元分析西瓜的组织结构可分为瓜皮和瓜瓤(忽略瓜籽)两部分组成,经试验及国外同类研究发现,成长初期的西瓜,其瓜皮和瓜瓤的性能参数如密度、杨氏模量、泊松比等均相差不大,可近似看作同一种非线性材料。
基于BMV特征的西瓜成熟度无损检测方法
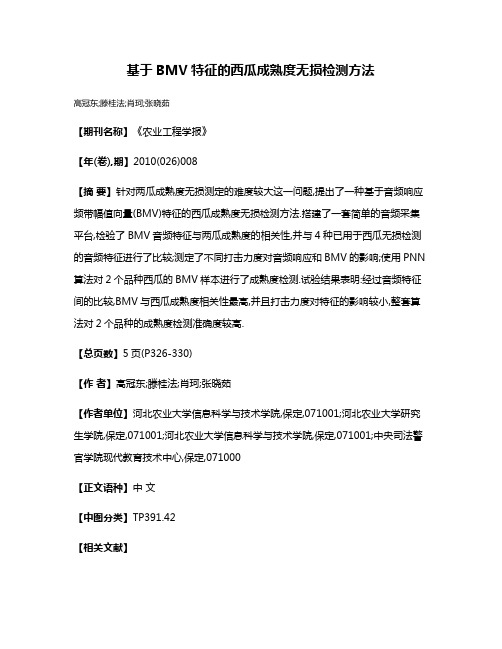
基于BMV特征的西瓜成熟度无损检测方法
高冠东;滕桂法;肖珂;张晓茹
【期刊名称】《农业工程学报》
【年(卷),期】2010(026)008
【摘要】针对两瓜成熟度无损测定的难度较大这一问题,提出了一种基于音频响应频带幅值向量(BMV)特征的西瓜成熟度无损检测方法.搭建了一套简单的音频采集平台,检验了BMV音频特征与两瓜成熟度的相关性,并与4种已用于西瓜无损检测的音频特征进行了比较;测定了不同打击力度对音频响应和BMV的影响;使用PNN 算法对2个品种西瓜的BMV样本进行了成熟度检测.试验结果表明:经过音频特征间的比较,BMV与西瓜成熟度相关性最高,并且打击力度对特征的影响较小,整套算法对2个品种的成熟度检测准确度较高.
【总页数】5页(P326-330)
【作者】高冠东;滕桂法;肖珂;张晓茹
【作者单位】河北农业大学信息科学与技术学院,保定,071001;河北农业大学研究生学院,保定,071001;河北农业大学信息科学与技术学院,保定,071001;中央司法警官学院现代教育技术中心,保定,071000
【正文语种】中文
【中图分类】TP391.42
【相关文献】
1.基于MEMS与单片机的西瓜成熟度无损检测系统的研究 [J], 沈蓉尔;韩玉杰;吴刚
2.基于音频特性的西瓜成熟度无损检测技术研究 [J], 张玉新;赵洋;陶佳
3.基于西瓜声学特性测定其成熟度 [J], 危艳君;饶秀勤;漆兵;林鹏鸟;李江波
4.基于MEMS的西瓜成熟度无损振动检测 [J], 涂文俊;张宇;耿涛;李晓;王薪淼
5.基于音频和近红外光谱融合技术的西瓜成熟度判别 [J], 邹小波;张俊俊;黄晓玮;郑开逸;吴胜斌;石吉勇
因版权原因,仅展示原文概要,查看原文内容请购买。
小型西瓜品质近红外无损检测的光谱信息采集
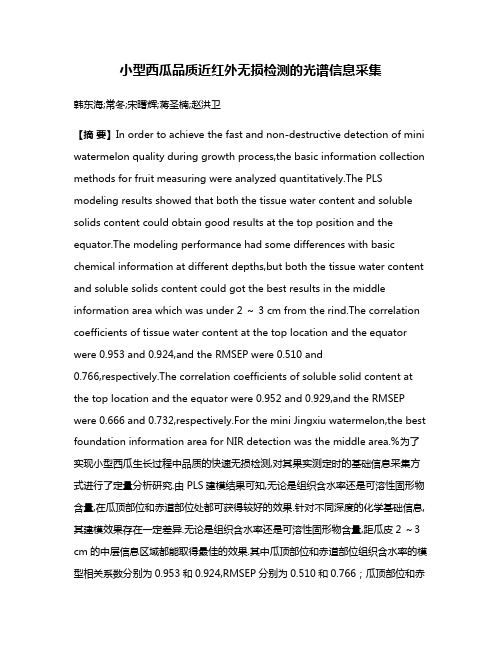
小型西瓜品质近红外无损检测的光谱信息采集韩东海;常冬;宋曙辉;蒋圣楠;赵洪卫【摘要】In order to achieve the fast and non-destructive detection of mini watermelon quality during growth process,the basic information collection methods for fruit measuring were analyzed quantitatively.The PLS modeling results showed that both the tissue water content and soluble solids content could obtain good results at the top position and the equator.The modeling performance had some differences with basic chemical information at different depths,but both the tissue water content and soluble solids content could got the best results in the middle information area which was under 2 ~ 3 cm from the rind.The correlation coefficients of tissue water content at the top location and the equator were 0.953 and 0.924,and the RMSEP were 0.510 and0.766,respectively.The correlation coefficients of soluble solid content at the top location and the equator were 0.952 and 0.929,and the RMSEP were 0.666 and 0.732,respectively.For the mini Jingxiu watermelon,the best foundation information area for NIR detection was the middle area.%为了实现小型西瓜生长过程中品质的快速无损检测,对其果实测定时的基础信息采集方式进行了定量分析研究.由PLS建模结果可知,无论是组织含水率还是可溶性固形物含量,在瓜顶部位和赤道部位处都可获得较好的效果.针对不同深度的化学基础信息,其建模效果存在一定差异.无论是组织含水率还是可溶性固形物含量,距瓜皮2 ~3 cm的中层信息区域都能取得最佳的效果.其中瓜顶部位和赤道部位组织含水率的模型相关系数分别为0.953和0.924,RMSEP分别为0.510和0.766;瓜顶部位和赤道部位可溶性固形物含量的相关系数分别为0.952和0.929,RMSEP分别为0.666和0.732.整体说明小型西瓜果实(品种京秀)近红外检测的最佳基础信息区域为中层信息区.【期刊名称】《农业机械学报》【年(卷),期】2013(044)007【总页数】5页(P174-178)【关键词】小型西瓜;近红外;无损检测;信息采集【作者】韩东海;常冬;宋曙辉;蒋圣楠;赵洪卫【作者单位】中国农业大学食品科学与营养工程学院,北京100083;中粮营养健康研究院食品质量与安全中心,北京100020;北京市农林科学院蔬菜研究中心,北京100097;中国农业大学信息与电气工程学院,北京100083;中国农业大学食品科学与营养工程学院,北京100083【正文语种】中文【中图分类】O657.33;S651引言我国是西瓜生产大国,其栽培面积及产量均居世界之首。
- 1、下载文档前请自行甄别文档内容的完整性,平台不提供额外的编辑、内容补充、找答案等附加服务。
- 2、"仅部分预览"的文档,不可在线预览部分如存在完整性等问题,可反馈申请退款(可完整预览的文档不适用该条件!)。
- 3、如文档侵犯您的权益,请联系客服反馈,我们会尽快为您处理(人工客服工作时间:9:00-18:30)。
原理: 西瓜成熟度的根本是 果蔬糖度、酸度、果 肉软硬程度等内部品 质指标。而有损检测 的最大特点就是要打 开西瓜才能验明正身
方法:糖度计等检测
ห้องสมุดไป่ตู้
无损检测
• NDT (Non-destructive testing),就是利 用声、光、磁和电等特性,在不损害或不 影响被检对象(西瓜)使用性能的前提下, 检测被检对象中是否存在缺陷或不均匀性, 给出缺陷的大小、位置、性质和数量等信 息,进而判定被检对象所处技术状态(品 质等)的所有技术手段的总称。
其他方法
除此之外, 还常利用密度、硬度、强制变形及生物传 感器等技术方法对果蔬进行无损检测与分选。
各种方法的评价与展望
对于各种无损检测方法, 它们各有自己的优缺点。 光学检测中利用红外光照射和红外反射所得的图像进行分析,
来检测西瓜产品是否有损伤, 但只能对西瓜表面的损伤进 行较好的检测; 力学检验中撞击技术则容易对西瓜造成新的损伤; 电学检测 尚在研究; 核磁共振检测、电子鼻检测其实验仪器成本较高; 声学特性则是实现硬度自动检测的有效方法; 机器视觉技术的应用是实现西瓜产品品质自动识别和分级的 最有效的方法。
很多学者就对果蔬产品的声波振动特性进行过深入研究, 他们把坚硬系数f 2m 2ö3 (f 和m 分别各自代表第二共鸣频 率和果蔬的质量) [5][6][7]作为果蔬产品硬度品质的独立指 标。
利用核磁共振技术
果蔬在成熟过程中,水、油和糖的氢质子的迁移率会随其 含量的变化而变化。另外,水、油、糖的浓度和迁移率还 与其他一些因素( 如机械破损、组织衰竭、腐烂、虫害以 及霜冻损害等)有关[8]。 基于以上特点,核磁共振可用于检测西瓜的压伤、虫 害、成熟度等。
期
[3]应义斌,刘燕德.水果内部品质光特性无损检测研究及应用.浙江大学学报.125129 2019.
[4]李静,操庆国.无损检测技术在农产品品质检验中的应用.农业工程学报.10018972.2019.21.032.
[5]於锋.西瓜成熟度的非生物学信息研究及检测仪器开发. [6]王书茂,焦群英.西瓜成熟度无损检验的冲击振动方法.农业工程学报.Vo l. 15
国内可参考应义斌, 刘燕德等(2019) [3]介绍了水果内部品质光学 特性检测原理、检测系统组成以及国内外最新研究进展和应用前 景的论文。
利用果蔬产品的声波振动特性
声学特性检测是利用样品在声波作用下的反射特性、散射 特性、透射特性、吸收特性、衰减系数和声波传播速度及 本身声阻抗、固有频率等的变化与农产品内部组织变化信 息间的关系来反映声波与样品相互作用的基本规律,并据 此做定量分析[4]。
利用机器视觉技术
机器视觉技术于农业中的应用研究, 始于20 世纪70 年代末期, 主要进行的是植物种类的鉴别、农产品品质检测和分级。
随着图像处理技术的迅猛发展和计算机软硬件的日益提高, 机器视觉系统在果蔬品质自动检测和分级领域的应用已得 到了较大的发展, 并促进了新的算法和硬件体系结构的发 展, 以便于将该技术应用于水果和蔬菜内部品质的自动分 选系统。
随着计算机技术、自动化控制技术等的发展,各种检测技术 的综合,西瓜的检测将会越来越简单、快速、智能。
各种检测仪器
糖度计
手持数显糖度计
小植物磁共振成像仪
多功能水果无损检测分析仪 (光电)
但现在市场最多的是基于红外反射的检测仪
参考文献
[1]张立彬.果蔬产品品质无损检测技术的研究进展.农业工程学报.Vo l. 21 No. 4 [2]韩东海,王加华.水果内部品质近红外光谱无损检测研究进展.中国激光.2019年08
利用西瓜的光学特性
由于西瓜的内部成分及外部特性不同, 在不同波长的射线照射下, 会 有不同的吸收或反射特性, 且吸收量与西瓜的组成成分、波长及照射 路径有关。主要有规则反射光法、漫反射光法和透射光法。
其中,近红外光谱在农产品品质检测及分级领域的应用[2]已经 取得很大进展,主要集中于粮食、水果等原料分级、成熟度、 新鲜度、含水量、含糖量等指标的测定。 现状:目前这种方法是无损检测与分拣技术中最实用的和最成 功的技术之一, 具有适应性强、检测灵敏度高、对人体无害、使 用灵活、设备轻巧、成本低和易实现自动化等优点, 目前国外已 逐步进入实际应用阶段。
应用方法
• 利用西瓜的电学特性 • 利用西瓜的光学特性 • 利用西瓜的声波振动
特性 • 利用核磁共振技术 • 利用机器视觉技术 • 利用电子鼻技术 • 利用撞击技术 • 利用X射线 • 利用其他方法
利用西瓜的电学特性
国外:Nelson (1980~1983)、日本学者加藤宏朗(1988) [1]等研究 表明:果蔬的一些介电参数与其内部品质有一定的相关性, 且介电参 数的测量结果与所选择的测试频率有密切关系;
利用电子鼻技术
• 对很多水果和蔬菜来说, 芳香是一种重要的品质属 性。目前, 电鼻子仍然是 测量食品和农产品气味和 芳香的最好的检测器[10]。
• 用来判断水果成熟度的一 种商业芬芳传感器于1990 年在日本就投入市场。
利用撞击技术
一个弹性球体撞击一个刚性表面的反作用力与撞击的速率、 质量、曲率半径、弹性系数和球体的泊松比等有关。研究 者发现水果对刚性表面的撞击基本上能用弹性球体进行模 拟, 水果的硬度对撞击的反作用力有直接的影响。
但目前, 这种方法在国内有关这方面的研究报道比较少, 且由 于此方法本身存在导致水果损伤的可能性缺陷, 必须要求 有较高的传感器系统。
利用X射线
X 射线具有穿透能力、衍射 作用和激发荧光的特性, 其无损检测原理主要是基 于其穿透能力的性质。
由于检测对象内部存在的缺 陷或者异物会引起穿透射 线强度的变化,通过检测 穿透后的射线强度,按照 一定方法转化成图像,并 进行分析以达到无损检测 的目的。
国内:尚处于起步阶段,张立彬进行过苹果、梨的电学特性与新鲜度 的关系的研究,结果表明, 水果的电学特性参数与水果品质密切相关, 为实现水果在线无损品质检测和自动分级奠定了理论基础。
现状:目前, 这方面的技术研究还不 甚成熟, 尚未真正 投入生产应用, 在国内也正处于研究的起步阶段, 因 此想用来检测西瓜成熟度还路漫漫其 修远兮~