Bayesiannetwork贝叶斯网络精品PPT课件
《贝叶斯信念网络》PPT课件

•我们可以颠倒因果关系 并且做出诊断。
•例如,已知草地是湿的, 则下过雨的概率可以计 算如下:
22
2021/4/23
23
2021/4/23
现在,假设我们想把喷水器(S)作为草地变 湿的另一个原因,如下图所示。
24
节点W有两个父节 点R和S,因此它的 概率是这两个值上的 条件概率P(W R, S。)
给定已知下过雨,则喷水器导致湿草地的可能性降 低了。已知草地是湿的,下雨和喷水器成为相互依 赖的。
27
2021/4/23
七、贝叶斯信念网络应用实例 : 警报分析(马克威分析系统)
某水文站内装有一个小型的警报系统,与该警报是 否拉响相关的因素有:洪水到来、地震发生,同时 该系统还肩负着安全警报的功能,当水文站发生入 室盗窃时,警报同样也会拉响。
先验概率是由以往的数据分析得到的。根据样 本数据得到更多的信息后,对其重新修正,即 是后验概率。
6
2021/4/23
例:旅客搭乘飞机必须经电子仪器检查是否身上 携带金属物品。
如果携带金属,仪器会发出声音的概率是97%,但 身上无金属物品仪器会发出声音的概率是5%。已 知一般乘客身上带有金属物品的概率是30%,若某 旅客经过仪器检查时发出声音,请问他身上有金 属物品的概率是多少?
判断:X=(女性,年龄介于31~45之间,不具学生 身份,收入中等)会不会办理信用卡。
解:首先根据训练样本计算各属性相对于不同分 类结果的条件概率:
P(办卡)=7/10
P(不办卡)=3/10
P(女性|办卡)=5/7
P(女性|不办卡)=1/3
P(年龄=31~45|办卡)=3/7 P(年龄=31~45|不办卡)=1/3
贝叶斯网络全解课件
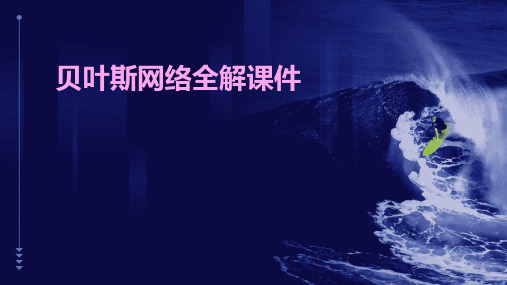
评分函数
定义一个评分函数来评估网络结构的优劣,常用的评分函数包 括BIC(贝叶斯信息准则)和AIC(赤池信息准则)等。
参数学习优化
1 2
参数学习
基于已知的网络结构和数据集,学习网络中各节 点的条件概率分布,使得网络能够最好地拟合数 据集。
最大似然估计
使用最大似然估计方法来估计节点的条件概率分 布,即寻找使得似然函数最大的参数值。
案例三
异常检测:使用贝叶斯网络检测金融市场中的异常交易行为。
06
贝叶斯网络展望
当前研究热点
概率图模型研究
贝叶斯网络作为概率图模型的一种,其研究涉及到对概率图 模型基本理论的研究,包括对概率、图、模型等基本概念的 理解和运用。
深度学习与贝叶斯网络的结合
随着深度学习技术的发展,如何将深度学习技术与贝叶斯网 络相结合,发挥各自的优势,是当前研究的热点问题。
未来发展方向
可解释性机器学习
随着人工智能技术的广泛应用,人们对机器学习模型的可解释性要求越来越高 。贝叶斯网络作为一种概率模型,具有天然的可解释性优势,未来可以在这方 面进行更深入的研究。
大规模贝叶斯网络
随着数据规模的增大,如何构建和处理大规模贝叶斯网络成为未来的一个重要 研究方向。
技术挑战与展望
联合概率
两个或多个事件同时发生的概率。联合概率 的计算公式为 P(A∩B)=P(A|B)⋅P(B)+P(B|A)⋅P(A)。
条件独立性
01
条件独立的概念
在给定某个条件时,两个事件之 间相互独立,即一个事件的发生 不影响另一个事件的发生。
02
条件独立性的应用
03
条件独立性的判断
在贝叶斯网络中,条件独立性用 于简化概率计算,降低模型复杂 度。
AI-05-15-贝叶斯网络-----人工智能课程--浙江大学研究生PPT课件
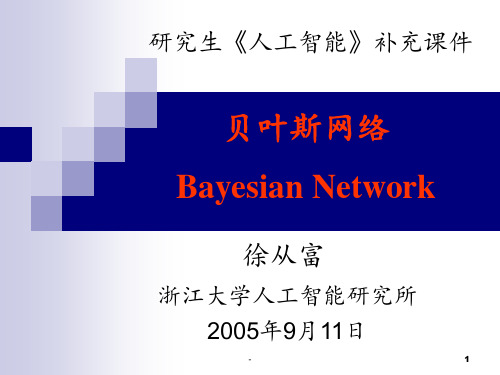
工作压力 大(W)
U P(W)
t 0.90 f 0.05
学校政策 (U)
C P(U) t 0.95 f 0.01
身体状况 差(B)
U P(B) t 0.30 f 0.01
W B P(A)
过劳死 (D)
t t 0.335 t f 0.30
f t 0.05
-
f f 0.00
26
已知:一个事件e = {学校政策U = true, and 工作压力大 = true},
-
28
多连通网络及其CPT: P(C) 0.50 Cloudy
C P(S) t 0.10 f 0.50
Sprinkler
Rain
C P(R) t 0.80 f 0.20
Wet Grass
S R P(W) t t 0.99 t f 0.90 f t 0.90 f f 0.00
-
29
等价的联合树及其CPT:
A. 贝叶斯网络的由来 B. 贝叶斯网络的定义 C. 贝叶斯网络的别名 D. 独立和条件独立 E. 贝叶斯网络示例
-
3
A. 贝叶斯网络的由来
全联合概率计算复杂性十分巨大
朴素贝叶斯太过简单
现实需要一种自然、有效的方式来捕捉 和推理——不确定性知识
变量之间的独立性和条件独立性可大大 减少为了定义全联合概率分布所需的概 率数目
“因果模型”比“诊断模型”需要更少的数 据,且这些数据也更容易得到
-
12
贝叶斯网络中的条件独立关系:
给定父节点,一个节点与它的非后代节点是 条件独立的
给定一个节点的父节点、子节点以及子节点 的父节点——马尔可夫覆盖(Markov blanket), 这个节点和网络中的所有其它节点是条件独 立的
第8章贝叶斯网导论【本科研究生通用机器学习课程精品PPT系列】
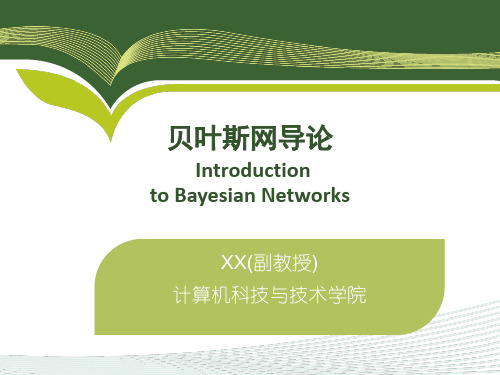
Burglary 独立假设2
独立假设2 Earthquake
Alarm
Alarm
JohnCalls
MaryCalls
1.5解决方案
•合并独立假设1和独立假设2,可得:P(John| Burglary, Earthquake, Alarm)=P(John| Alarm)
合并独立假设1和2
Burglary
P(E e) P( X ) 是 X 的先验分布, P(X | E e) 是 X 的后验分布, P(E e | X ) 称为 X 的似然函数。 P(E e) 是一个归一化常数
后验分布正比于先验分布和似然函数的乘积。
1.3几个重要原理
链规则(chain rule)
利用变量间条件独立性
1.3不确定性推理与联合概率分布
n n 9.1E-1
1.3不确定性推理与联合概率分布
从联合概率分布 P(Burglary,Earthquake, Alarm,John,Mary)出发,先计算边缘分布
P(Burglary, Mary)
P(Burglary, Earthquake, Alarm, John, Mary)
Earthquake, Alarm,John
0.000115
0.61
P(Burglary y, Mary y) P(Burglary n, Mary y) 0.000115 0.000075
1.4存在的问题
直接使用联合分布进行不确定性推理的困难很明显,即它的复杂度
极高。上图中有 5 个二值随机变量,整个联合分布包含25 1 31 个独
n n 2.8E-4 n
n
y
n n 2.9E-5
y
n
人工智能贝叶斯网络.ppt

• Directed Acyclic Graph (DAG)
– Nodes are random variables – Edges indicate causal influences
Burglary
Earthquake
Alarm
JohnCalls
MaryCalls
3
Conditional Probability Tables
– Bayesian Networks: Directed acyclic graphs that indicate causal structure.
– Markov Networks: Undirected graphs that capture general dependencies.
2
Bayesian Networks
JohnCalls
MaryCalls
However, this ignores the prior probability of John calling.
12
Bayes Net Inference
• Example: Given that John calls, what is the probability that there is a Burglary?
7
Independencies in Bayes Nets
• If removing a subset of nodes S from the network renders nodes Xi and Xj disconnected, then Xi and Xj are independent given S, i.e. P(Xi | Xj, S) = P(Xi | S)
贝叶斯信念网络PPT课件
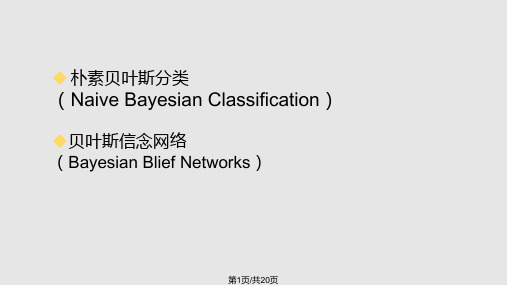
五.朴素贝叶斯分类实例:检测SNS社区中不真实账号 这个问题是这样的,对于SNS社区来说,不真实账号(使用虚
假身份或用户的小号)是一个普遍存在的问题,作为SNS社区的运 营商,希望可以检测出这些不真实账号,从而在一些运营分析报告 中避免这些账号的干扰,亦可以加强对SNS社区的了解与监管。
第5页/共20页
四.朴素贝叶斯分类 1:朴素贝叶斯分类的原理与流程
朴素贝叶斯分类是一种十分简单的分类算法,叫它朴素贝叶斯分类是因 为这种方法的思想真的很朴素,朴素贝叶斯的思想基础是这样的:对于给 出的待分类项(x),求解在此项出现的条件下各个类别(y)出现的概率, 哪个最大,就认为此待分类项属于哪个类别。通俗来说,就好比这么个道 理,你在街上看到一个黑人,我问你你猜这哥们哪里来的,你十有八九猜 非洲。为什么呢?因为黑人中非洲人的比率最高,当然人家也可能是美洲 人或欧洲人,但在没有其它可用信息下,我们会选择条件概率最大的类别, 这就是朴素贝叶斯的思想基础。
朴素贝叶斯分类
(Naive Bayesian Classification)
贝叶斯信念网络 (Bayesian Blief Networks)
第1页/共20页
朴素贝叶斯分类
贝叶斯分类是一类分类算法的总称,这类算法均以贝叶斯定理 为基础,故统称为贝叶斯分类。
这里首先介绍分类问题,对分类问题进行一个正式的定义。然 后,介绍贝叶斯分类算法的基础——贝叶斯定理。最后,通过实例 讨论贝叶斯分类中最简单的一种:朴素贝叶斯分类。
从数学角度来说,分类问题可做如下定义:
其中C叫做类别集合,其中每一个元素是一个类别,而I叫做项集合,其中每一个元素是一个待分 类项,f叫做分类器。分类算法的任务就是构造分类器f。
贝叶斯网络简介PPT课件
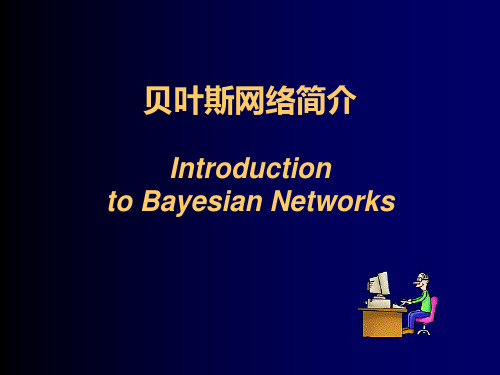
而在贝叶斯网络中,由于存在前述性质,任意随 机变量组合的联合条件概率分布被化简成
其中Parents表示xi的直接前驱节点的联合,概率 值可以从相应条件概率表中查到。
.
6
例子
P(C, S,R,W) = P(C)P(S|C)P(R|S,C)P(W|S,R,C) chain rule
= P(C)P(S|C)P(R|C)P(W|S,R,C) since
= P(C)P(S|C)P(R|C)P.(W|S,R) since
7
贝叶斯网络的构造及训练
1、确定随机变量间的拓扑关系,形成DAG 。这一步通常需要领域专家完成,而想要 建立一个好的拓扑结构,通常需要不断迭 代和改进才可以。
2、训练贝叶斯网络。这一步也就是要完成 条件概率表的构造,如果每个随机变量的 值都是可以直接观察的,方法类似于朴素 贝叶斯分类。但是通常贝叶斯网络的中存 在隐藏变量节点,那么训练方法就是比较 复杂。
4、将收敛结果作为推. 断值。
9
贝叶斯网络应用
医疗诊断,
工业,
金融分析,
计算机(微软Windows,Office),
模式识别:分类,语义理解
军事(目标识别,多目标跟踪,战争身份识别
等),
生态学,
生物信息学(贝叶斯网络在基因连锁分析中应
用),
编码学,
分类聚类,
时序数据和动态模型 .
• 用概率论处理不确定性的主要优点是保 证推理结果的正确性。
.
2
几个重要原理
• 链规则(chain rule)
P ( X 1 , X 2 ,X . n ) . P ( . X 1 ) , P ( X 2 |X 1 ) P ( X .n | . X 1 , . X 2 ,X . n ) ..,
贝叶斯信念网络汇总课件

常用的参数学习方法包括最大似然估计、贝叶斯估计和期望最大化算法等。这些算法可以帮助我们从数据中学习 到最佳的参数设置,使得贝叶斯网络能够最好地拟合概率推理是贝叶斯信念网络的核心,它基于概率理论来描述不 确定性。
02
概率推理的目标是计算给定证据下某个假设的概率,或者计算
06
贝叶斯网络的发展趋势与 未来展望
深度学习与贝叶斯网络的结合
深度学习在特征提取上的 优势
贝叶斯网络在处理复杂、高维数据时,可以 借助深度学习强大的特征提取能力,提高模 型对数据的理解和表达能力。
贝叶斯网络的概率解释能力
贝叶斯网络具有清晰的概率解释,可以为深度学习 模型提供可解释性强的推理框架,帮助理解模型预 测结果。
参数可解释性
通过可视化技术、解释性算法等方法,可以进一步解释贝叶斯网络 中参数的意义和影响,提高模型的可信度和用户接受度。
感谢您的观看
THANKS
联合优化与模型融合
未来研究可以探索深度学习与贝叶斯网络在 结构、参数和优化方法上的联合优化,实现 两者的优势互补。
大数据处理与贝叶斯网络
大数据处理的需求
随着大数据时代的到来,如何高 效处理、分析和挖掘大规模数据 成为关键问题。贝叶斯网络在大 数据处理中具有广阔的应用前景 。
并行计算与分布式
实现
针对大规模数据,可以采用分布 式计算框架,如Hadoop、Spark 等,对贝叶斯网络进行并行化处 理,提高推理和学习的效率。
在贝叶斯网络中,变量间的关系通过 条件独立性来表达。确定条件独立性 有助于简化网络结构,提高推理效率 。
构建有向无环图
根据条件独立性评估结果,可以构建 一个有向无环图来表示贝叶斯网络的 结构。这个图将各个变量连接起来, 反映了它们之间的依赖关系。
BayesNet贝叶斯网络

•
13
Conditional Independence
• • • •
We say that X is conditionally independent of Y given Z if the probability distribution governing X is independent of the value of Y given a value for Z. i.e., (xi,yj,zk) P(X=xi|Y=yj,Z=zk)=P(X=xi|Z=zk) or, P(X|Y,Z)=P(X|Z) This definition can be extended to sets of variables as well: we say that the set of variables X1…Xl is
•
apply. The Naï ve Bayes Classifier uses the conditional independence assumption to defray these costs. However, in many cases, such an assumption is overly restrictive. Bayesian belief networks provide an intermediate approach which allows stating conditional independence assumptions that apply to subsets of the variable.
First guess The money
11
A
Stick or swap?
贝叶斯网络全解共64页
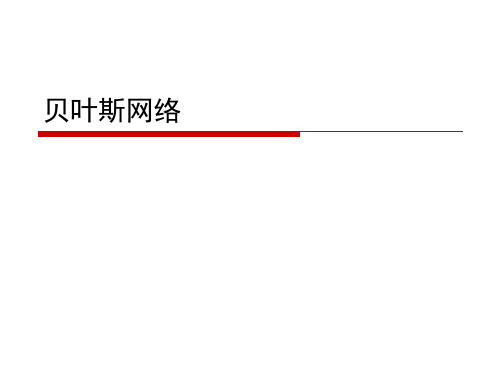
了解马尔科夫链、隐马尔科夫模型的网络拓扑和含 义
9
一个实例
10
后验概率
c1、c2表示左右两个信封。 P(R),P(B)表示摸到红球、黑球的概率。 P(R)=P(R|c1)*P(c1) + P(R|c2)*P(c2):全概率公式 P(c1|R)=P(R|c1)*P(c1)/P(R)
记单词数目为N,即形成词汇表。 将每个样本si向量化:初始化N维向量xi,若
词wj在si中出现,则xij=1,否则,为0。从 而得到1000个N维向量x。 使用:P(c|x)=P(x|c)*P(c) / P(x)
14
分解
P(c|x)=P(x|c)*P(c) / P(x) P(x|c)=P(x1,x2…xN|c)=P(x1|c)*P(x2|c)…P(xN|c) P(x)=P(x1,x2…xN)=P(x1)*P(x2)…P(xN) 带入公式: P(c|x)=P(x|c)*P(cP(c1)=P(c2)=1/2 如果摸到一个红球,那么,这个信封有1美元的概率 是0.6 如果摸到一个黑球,那么,这个信封有1美元的概率 是3/7
11
朴素贝叶斯的假设
一个特征出现的概率,与其他特征(条件)独 立(特征独立性)
其实是:对于给定分类的条件下,特征独立
7
复习:互信息
两个随机变量X,Y的互信息,定义为X,Y 的联合分布和独立分布乘积的相对熵。
I(X,Y)=D(P(X,Y) || P(X)P(Y))
I(X,Y) p(x,y)logp(x,y)
x,y
p(x)p(y)
8
贝叶斯网络
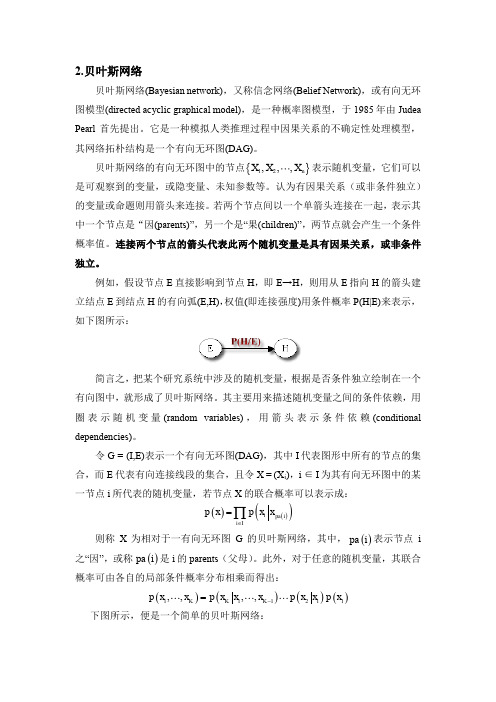
2.贝叶斯网络贝叶斯网络(Bayesian network),又称信念网络(Belief Network),或有向无环图模型(directed acyclic graphical model),是一种概率图模型,于1985年由Judea Pearl 首先提出。
它是一种模拟人类推理过程中因果关系的不确定性处理模型,其网络拓朴结构是一个有向无环图(DAG)。
贝叶斯网络的有向无环图中的节点{}12,,,n X X X 表示随机变量,它们可以是可观察到的变量,或隐变量、未知参数等。
认为有因果关系(或非条件独立)的变量或命题则用箭头来连接。
若两个节点间以一个单箭头连接在一起,表示其中一个节点是“因(parents)”,另一个是“果(children)”,两节点就会产生一个条件概率值。
连接两个节点的箭头代表此两个随机变量是具有因果关系,或非条件独立。
例如,假设节点E 直接影响到节点H ,即E→H ,则用从E 指向H 的箭头建立结点E 到结点H 的有向弧(E,H),权值(即连接强度)用条件概率P(H|E)来表示,如下图所示:简言之,把某个研究系统中涉及的随机变量,根据是否条件独立绘制在一个有向图中,就形成了贝叶斯网络。
其主要用来描述随机变量之间的条件依赖,用圈表示随机变量(random variables),用箭头表示条件依赖(conditional dependencies)。
令G = (I,E)表示一个有向无环图(DAG),其中I 代表图形中所有的节点的集合,而E 代表有向连接线段的集合,且令X = (X i ),i ∈ I 为其有向无环图中的某一节点i 所代表的随机变量,若节点X 的联合概率可以表示成:()()()i pa i i Ip x p x x ∈=∏则称X 为相对于一有向无环图G 的贝叶斯网络,其中,()pa i 表示节点i 之“因”,或称()pa i 是i 的parents (父母)。
此外,对于任意的随机变量,其联合概率可由各自的局部条件概率分布相乘而得出:()()()()111211,,,,K K K p x x p x x x p x x p x -=下图所示,便是一个简单的贝叶斯网络:因为a 导致b ,a 和b 导致c ,所以有:()()()(),,,p a b c p c a b p b a p a =2.1贝叶斯网络的3种结构形式:给定如下图所示的一个贝叶斯网络:(1) x 1, x 2 , …,x 7的联合分布为:()()()()()()()()1234567123412351364745,,,,,,,,,,p x x x x x x x p x p x p x p x x x x p x x x p x x p x x x =(2)x 1和x 2独立(对应head-to-head );(3)x 6和x 7在x 4给定的条件下独立(对应tail-to-tail )根据上图,第(1)点可能很容易理解,但第(2)、(3)点中所述的条件独立是啥意思呢?其实第(2)、(3)点是贝叶斯网络中3种结构形式中的其中二种。
人工智能09贝叶斯网络(PPT57页)
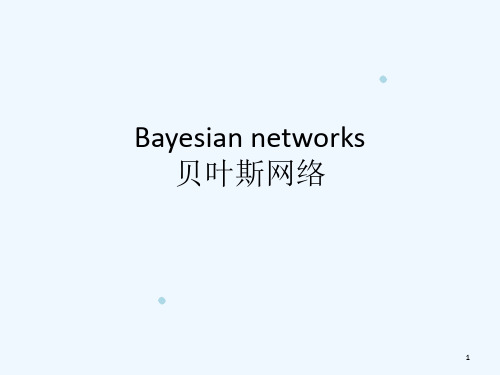
k
18
Global semantics(全局语义)
The full joint distribution is defined as the product of the local conditional distributions: 全联合概率分布可以表示为贝叶斯网络中 的条件概率分布的乘积
19
– Is X independent of Z given Y?
22
Common Cause共同原因
• 另一个基础的形态: two effects of the same cause – Are X and Z independent? – Are X and Z independent given Y?
opportunities.
“某事发生的概率是0.1” 意味着0.1是在无穷 多样本的极限
条件下能够被观察到的比例
但是,在许多情景下不可能进行重复试
验
2
Probability概率
Probability is a rigorous formalism for uncertain knowledge
概率是对不确定知识一种严密的形式化方法
6
什么是图模型?
概率分布的图表示 – 概率论和图论的结合
• Also called 概率图模型 • They augment analysis instead of using pure
algebra(代数)
7
What is a Graph?
• Consists of nodes (also called vertices) and links (also called edges or arcs)
32Βιβλιοθήκη 因果关系?• 当贝叶斯网络反映真正的因果模式时: – Often simpler (nodes have fewer parents) – Often easier to think about – Often easier to elicit from experts(专家)
21.贝叶斯网络

互联网新技术在线教育领航者
13/60
贝叶斯网络
一般而言,贝叶斯网络的有向无环图中的节点表示随 机变量,它们可以是可观察到的变量,或隐变量、未 知参数等。连接两个节点的箭头代表此两个随机变量 是具有因果关系(或非条件独立)。若两个节点间以一 个单箭头连接在一起,表示其中一个节点是“因 (parents)”,另一个是“果(children)”,两节点就会产 生一个条件概率值。
maxPD
|
Ai
PAi
max
PD
|
Ai
max P(Ai | D) max P(D | Ai)
第一个等式:贝叶斯公式;
第二个等式:样本给定,则对于任何Ai,P(D)是常数,仅 为归一化因子;
第三个箭头:若这些结论A1、A2……An的先验概率相等 (或近似),则得到最后一个等式:即第二行的公式。
P(R|c1)=2/4 P(R|c2)=1/3 P(c1)=P(c2)=1/2 如果摸到红球,则该信封有1美元的概率是0.6 如果摸到黑球,则该信封有1美元的概率是3/7
互联网新技术在线教育领航者
11/60
金条问题
现有三个箱子,每个箱子各有两块贵金属。
三个箱子的金银条如下:
互联网新技术在线教育领航者
3/60
复习:相对熵
相对熵,又称互熵,交叉熵,鉴别信息,Kullback 熵,Kullback-Leible散度等
设p(x)、q(x)是X中取值的两个概率分布,则p对q的
相对熵是
D p
||
q
x
pxlog
px qx
Epx
log
贝叶斯网络PPT课件
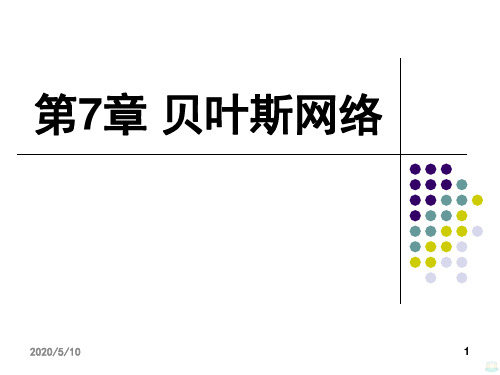
2019/8/21
3.贝叶斯网络学习 贝叶斯网络学习是指由先验的贝叶斯网络得到后验的贝叶斯网络的过程。
先验贝叶斯网络是根据用户的先验知识构造的贝叶斯网络,后验贝叶斯网络 是把先验贝叶斯网络和数据相结合而得到的贝叶斯网络。
贝叶斯网络学习的实质是用现有数据对先验知识的修正。贝叶斯网络能 够持续学习.上次学习得到的后验贝叶斯网络变成下一次学习的先验贝叶斯 网络,每一次学习前用户都可以对先验贝叶斯网络进行调整,使得新的贝叶 斯网络更能体现数据中蕴涵的知识。贝叶斯网络的学习关系如图7.2所示。
(2)具有良好的可理解性和逻辑性,这是神经元网络无法比拟的,神经 元网络从输入层输入影响因素信息,经隐含层处理后传人输出层,是黑匣子 似的预测和评估,而贝叶斯网络是白匣子。
(3)专家知识和试验数据的有效结合相辅相成,忽略次要联系而突出主 要矛盾,可以有效避免过学习。
(4)贝叶斯网络以概率推理为基础,推理结果说服力强,而且相对贝叶 斯方法来说,贝叶斯网络对先验概率的要求大大降低。贝叶斯网络通过实践 积累可以随时进行学习来改进网络结构和参数,提高预测诊断能力,并且基 于网络的概率推理算法,贝叶斯网络接受了新信息后立即更新网络中的概率 信息。
图7.1 基于结点间概率关系的推理
3
2019/8/21
通过长期的观察,或者从别人那里了解,这个中学生的父母知道他 们的女儿参加晚会的概率。通过长时间的数据积累,他们也知道他们的 女儿参加晚会后宿醉的概率。因此,结点party和结点hangover之间有 一条连线。同样,有明显的因果关系或相关关系的结点之间都有一条连 线,并且连线从原因结点出发,指向结果结点。
12
2019/8/21
7.l 引例
先看一个关于概率推理的例子。图7.1中有6个结点:参加晚 会(party,PT)、 宿醉(hangover,HO)、患脑瘤(brain tumor, BT)、头疼(headache,HA)、有酒精味(smell alcohol,SA)和X射 线检查呈阳性(posxray,PX)。可以把图7.1想象成为这样一个场 景:一个中学生回家后,其父母猜测她参加了晚会,并且喝了酒; 第二天这个学生感到头疼,她的父母带她到医院做头部的X光检查 ……
贝叶斯信念网络汇总课件
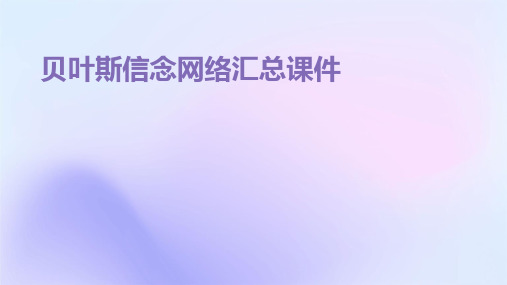
朴素贝叶斯分类器在特征选择上较为简单,通常只考虑特 征是否出现。而贝叶斯信念网络则可以考虑到特征的多个 状态及其概率,提供更丰富的特征信息。
朴素贝叶斯分类器在处理连续型特征时存在困难,而贝叶 斯信念网络则可以很好地处理连续型特征。
与决策树和神经网络的比较
决策树和神经网络是基于数据的全局结构进行分类的 ,而贝叶斯信念网络则基于数据的局部概率分布进行
分类,具有更好的局部细节捕捉能力。
决策树和神经网络通常需要大量的数据才能获得较好 的效果,而贝叶斯信念网络在小样本数据下也能表现
良好。
决策树和神经网络在处理高维数据时容易过拟合,而 贝叶斯信念网络则能够有效地处理高维数据。
与隐马尔可夫模型和卡尔曼滤波器的比较
隐马尔可夫模型和卡尔曼滤波器主要用于时间序列分析,而贝叶斯信念网络则可以用于各种概率分布 的建模。
隐马尔可夫模型和卡尔曼滤波器在处理非线性问题时存在困难,而贝叶斯信念网络则能够很好地处理非 线性问题。
隐马尔可夫模型和卡尔曼滤波器通常用于短期预测,而贝叶斯信念网络则可以用于长期预测和复杂系统 模拟。
可解释性与透明度
随着人工智能技术的广泛应用,模型 的解释性与透明度越来越受到关注。
未来研究可以进一步探索如何提高贝 叶斯信念网络的解释性和透明度,使 其更好地应用于实际问题和决策支持 系统。
贝叶斯信念网络可以通过概率和因果 关系的建模来提供一定的解释性和透 明度。
THANKS。
02
常用的参数学习方法包括最大似 然估计和贝叶斯估计。
最大似然估计基于训练数据估计 参数,使得数据出现的概率最大 。
- 1、下载文档前请自行甄别文档内容的完整性,平台不提供额外的编辑、内容补充、找答案等附加服务。
- 2、"仅部分预览"的文档,不可在线预览部分如存在完整性等问题,可反馈申请退款(可完整预览的文档不适用该条件!)。
- 3、如文档侵犯您的权益,请联系客服反馈,我们会尽快为您处理(人工客服工作时间:9:00-18:30)。
Parameter Learning
• In order to fully specify the Bayesian network and thus fully represent the joint probability distribution, it is necessary to specify for each node X the probability distribution for X conditional upon X's parents
prior possibility P(Y) ( rankings, recent history of their performance)
Introduction
• First half is over • The outcome of the first period may be
treated as a random variable X, the óbserved evidence' that influence your prediction of the final value of Y.
• Prior confidence --------belief • Process--------belief propagation dynamics
causal relationships
statistical dependence between
Bayesian Networks
• DAG: Directed Acyclic Graph • CPT: Conditioanl Probability Tables
• P(Y|X)= PX |YPY Hale Waihona Puke XIntroduction
• A train of consequences • Before the match has started, prior
possibilityP(Y); after observing the evidence X1, posterior probability P(Y|X1); new evidence X2 is acquired, then
d-seperation
d-seperation is a sufficient condition for conditional independence
Markov Blankets
Markov Blanket of a node is the set consisting of its parents, children and spouses
Data
conditional probability tables
Directed Markov Assumption
• Each variable is independent of its nondescendents in the DAG given the values of its parents.
Directed Markov Assumption
Markov Blankets
u1 un
z1
zn
Y1 Yn
feature A is independent of all other features given MB(A)={u1,...un,z1,...zn, Y1,...,Yn}
Markov Blankets
• For any value xi of Xi,
Advantage of BN: X 1,...aX l.ln oa .w.u,ro osne tolryeed sstta iime maan rttiene c gjoad ie nyrtedsl,w aistttc irvriebe a lua yttion lrnlbay n:sfo
small number of parameters
PY|X1,X2PX P 1,X X1 2,|X Y2 P YPX2P |Y X P 2P X1 X |Y 1PY PX2P |Y X P 2 Y |X1
Introduction
• Bayes theorem allows us to transform our prior probabilistic knowledge of an event into a more robust, posterior knowledge of its probability.
d-seperation
• some terminology • head-to-tail at node B • tail-to tail at A
A B C A
B
C
A
C
• head-to-head at B
B
d-seperation
A
B
C D
E
Z is observed: A,B are in Z; C, D or E are not in Z
Hybrid Random Fields Bayes Networks
Jill 5/2/2013
Introduction
• For some mysterious reasons, • Bayes networks are often introduced in the
literature with examples concerning weather forecase: • Is it likely to be sunny on Sunday given the fact that it is raining on Saturday, and that my granny's back hurts?
Introduction
• make prediction on whether your favorite football team will win, lose or draw tonight
• outcome of the match ---random variableY • Y=w, l, d • Rely on your original estimate of a certain