AI中英文版本对照
AI 中英文对照
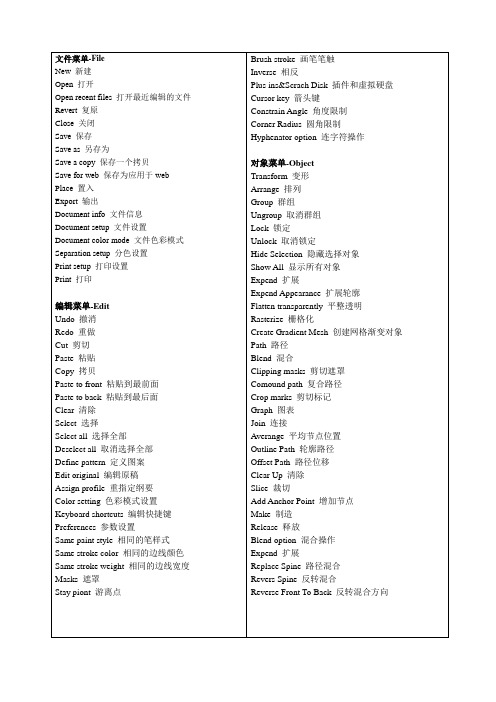
Type 类型 Data 数据 Design 设计 Column 柱形 Marker 标记 Transform Again 再次变形 Move 移动 Scale 缩放 Rotate 旋转 Shear 倾斜 Reflect 镜像 Transform each 单独变形 Reset Bounding 重设调节框 Bring To Front 放在最前层 Bring Forward 放在前一层 Send Backward 放在后一层 Sent to Back 放在最后 Rasterize 光栅化
其他:
Shades 深色
Tins 淡色
Character 字符
Fill 填充
stroke 描边
Opacity 不透明度
transparency 透明度
Sublayer 子图层ቤተ መጻሕፍቲ ባይዱ
Enter/exit isolation mode 进入/退出隔离模式
Make/release clipping mask 创建/释放剪切蒙版
视图菜单-VIEW Outline 路径轮廓视图 Overprint preview 印前视图模式 Pixel preview 象素视图模式 Proof setup 校验设置 Zoom in 放大 Zoom out 缩小 Fit in window 适合窗口 Actual size 实际尺寸 Show/hide edges 显示/隐藏选中路径 Show/hide page tiling 显示/隐藏工作区标志 Show/hide template 显示/隐藏模板 Show/hide rules 显示/隐藏标尺 Show/hide bounding box 显示/隐藏限制框 Show/hide transparency grid 显示/隐藏透明网格 Guides 参考线 Smart guides 实时参考线 Show/hide grid 显示/隐藏网格 Snip to grid 对齐网格
ADOBE ILLUSTRATOR 菜单之中英文对照表
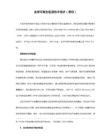
滤镜菜单-FILTER
矢量滤镜
Apply Last Filter - - - - - 应用刚才的滤镜
Last Filter - - - - - - - 刚才的滤镜
Color - - - - - - - - - 色彩
Create - - - - - - - - 创建
Distort - - - - - - - - 扭曲
Copy - - - - - - - - - 拷贝
Paste to front - - - - - - 粘贴到最前面
Paste to back - - - - - - - 清除
Select - - - - - - - - 选择
Select all - - - - - - - 选择全部
Fit headline - - - - - - 适合标题
Greate Outlines - - - - - 创建文字轮廓路径
Find/Change - - - - - - - 查找/替换
Find fond - - - - - - - 选择字体
Check Spelling - - - - - - 拼写检查
Marker - - - - - - - - 标记
Transform Again - - - - - 再次变形
Move - - - - - - - - - 移动
Scale - - - - - - - - - 缩放
Rotate - - - - - - - - 旋转
Shear - - - - - - - - - 倾斜
Export - - - - - - - - 输出
Document info - - - - - - 文件信息
中国百家姓中英文对照

姓“李”会写作“Lee”,姓“吴”会写作“Woo”,怎么会这样?终于找到最准确的解释了。包 括除大陆汉语拼音以外的“香港拼写法”、“台湾拼写法”、以及英文传统发音拼写法。 很多人发现,外界对于中国姓名的拼写于我们不一样,不是汉语拼音,是一种我们看来 新鲜陌生的拼写形式。比如:“李”姓在外界很多时拼写为“Lee”。而“张”姓有的拼写为 “Cheung”,而有的拼写为“Chang”,有人说这是英文对于中国姓氏的标准拼写,也有人说是 威妥玛拼音,其实这些都不正确,或者说都不完善。 首先应该明确一下, 中文姓氏是不存在标准的英文拼写的, 因为中文汉字名不论有没有 内在含义, 一旦转入英文就完全变作拼音名了。 也就是说完全根据中文名的读音来进行拼写。 那么,之所以外界的拼写和我们不同,很大程度上是受到了汉字方言的影响,即:这种情况 普遍出现在没有确立“中国汉语普通话”概念的区域里。 由于我们中国大陆已经确立“普通话”概念,所以大陆范围内无论什么方言,都统统以普 通话读音为汉语规范读音,于是拼音体系也自然以普通话为标准。而相比之下,香港地区的 官方语言是粤语,于是香港地区的拼音体系也自然是粤语音的描述。 例如:姓氏“陈” 普通话读音为:“Chen” 香港粤语读音为:“蚕”,于是根据这个读音,再结合英文字母的搭配发音习惯便拼写为 “Chan”。 再比如:姓氏“刘” 普通话读音为:“Liu” 香港粤语读音贴近于“劳”,于是香港的“刘”姓便拼写为“Lau”。 所以,由此可以得知英文对于中文汉字的语音翻译非常直白,并没有深层追究的成分。 另外, 目前各非英文地区都有一套自定的拼音体系, 确保本地区公民姓名翻译成英文后 依然有法律认证功能,我们中国大陆自然以汉语拼音为默认准绳。而香港;台湾;乃至朝鲜 韩国也都有一套自定的拼音体系, 只是他们都是以本地区语音为拼读发音基础。 所以同一个 汉字在不同区域拼写也不同。 比如:姓氏“金” 中国普通话汉语拼音:“Jin” 中国香港粤语拼音:“Kam” 中国台湾所谓国语通用拼写:“Chin” 中国汉字朝鲜语音拼写:“Kim” 此外还存在拼写为“King”的情况。 由此可见, 同一个字的拼写如此多样, 而之间不能相互取代, 所以并不存在标准的拼写。
欧盟人工智能法案中英文对照全套
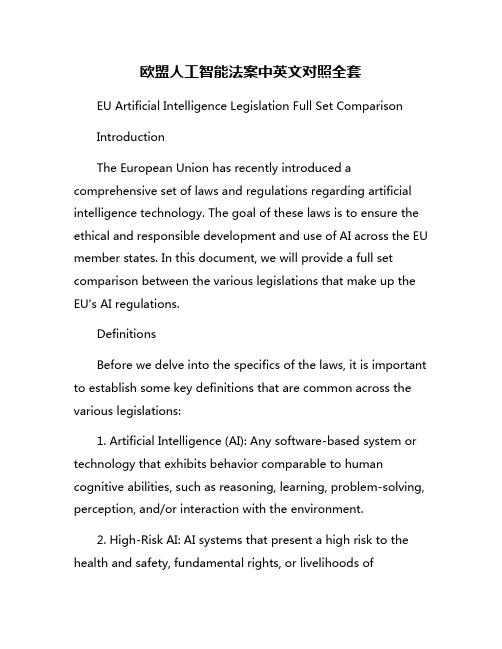
欧盟人工智能法案中英文对照全套EU Artificial Intelligence Legislation Full Set ComparisonIntroductionThe European Union has recently introduced a comprehensive set of laws and regulations regarding artificial intelligence technology. The goal of these laws is to ensure the ethical and responsible development and use of AI across the EU member states. In this document, we will provide a full set comparison between the various legislations that make up the EU's AI regulations.DefinitionsBefore we delve into the specifics of the laws, it is important to establish some key definitions that are common across the various legislations:1. Artificial Intelligence (AI): Any software-based system or technology that exhibits behavior comparable to human cognitive abilities, such as reasoning, learning, problem-solving, perception, and/or interaction with the environment.2. High-Risk AI: AI systems that present a high risk to the health and safety, fundamental rights, or livelihoods ofindividuals. These systems are subject to stricter regulations and oversight.3. Biometric Data: Any information that relates to the physical, physiological, or behavioral characteristics of an individual, which allows for their unique identification.Now let's examine the key components of the EU's AI legislation:1. AI ActThe AI Act is the central piece of legislation that governs AI technology within the EU. It sets out the requirements for the development, deployment, and use of AI systems across various sectors. Some key provisions of the AI Act include:- Transparency: AI systems must be designed and developed in a transparent manner, with clear documentation of their operation and function.- Accountability: Developers and users of AI systems are held accountable for any harm caused by these systems.- Biometric Data: The use of biometric data in AI systems is strictly regulated, with explicit consent required for its collection and processing.2. Data Governance ActThe Data Governance Act complements the AI Act by establishing rules for the sharing and use of data in AI systems. Key provisions of the Data Governance Act include:- Data Sharing: Organizations are required to share data with other entities under certain conditions, to promote collaboration and innovation in AI development.- Data Protection: Data used in AI systems must be protected from unauthorized access or misuse, to ensure the privacy and security of individuals' data.- Data Portability: Individuals have the right to request the transfer of their data between different service providers, to facilitate competition and innovation.3. Data ActThe Data Act focuses specifically on the collection, processing, and use of personal data in AI systems. Key provisions of the Data Act include:- Consent: Individuals must provide explicit consent for the use of their personal data in AI systems, and have the right to withdraw this consent at any time.- Data Minimization: Organizations are required to limit the collection and processing of personal data to what is strictly necessary for the operation of AI systems.- Data Security: Measures must be taken to ensure the security and integrity of personal data in AI systems, to prevent unauthorized access or breaches.ConclusionThe EU's artificial intelligence legislation represents a significant step towards ensuring the ethical and responsible development and use of AI technology within the European Union. By establishing clear rules and standards for the operation of AI systems, these laws aim to protect the rights and well-being of individuals, while promoting innovation and competitiveness in the AI sector. It is crucial for organizations and developers to adhere to these regulations and uphold the principles of transparency, accountability, and data protection in all AI-related activities.。
欧盟人工智能法案中英文对照全套

I. IntroductionThe European Union (EU) has been at the forefront of regulatory innovation in the field of artificial intelligence (AI). The EUArtificial Intelligence Act (AI Act) is a landmark piece of legislation designed to ensure the safe, ethical, and sustainable development and deployment of AI across the EU. This act aims to create a harmonized regulatory framework that protects individuals, fosters innovation, and promotes trust in AI technologies.II. Scope and ObjectivesThe AI Act applies to AI systems deployed in the EU, regardless of where they are developed. It sets out a comprehensive set of rules and standards for AI systems, categorizing them into six risk levels based on their potential impact on human life and safety.The objectives of the AI Act are as follows:1. Ensure high levels of safety and trust in AI systems: The act establishes strict safety and transparency requirements for AI systems, particularly those with a high risk level.2. Promote innovation and competitiveness: The act aims to create alevel playing field for AI developers and businesses in the EU, while also encouraging the development of new AI technologies.3. Protect fundamental rights: The act includes provisions to protect individuals' fundamental rights, such as privacy, freedom of expression, and non-discrimination.4. Enhance consumer protection: The act provides clear guidelines for the marketing and labeling of AI products and services, ensuring that consumers are informed about the AI technologies they use.5. Facilitate cross-border cooperation: The act promotes the exchange of information and best practices among EU member states, fostering a coordinated approach to AI regulation.III. AI Systems and Risk CategoriesThe AI Act categorizes AI systems into six risk levels based on their potential impact on human life and safety:1. High Risk: These AI systems can pose a high risk to human life and safety, including those used in critical infrastructure, transport, healthcare, and law enforcement. Examples include autonomous vehicles, drones, and medical devices.2. Substantial Risk: These AI systems can pose a substantial risk to human life and safety, including those used in employment, education, and credit scoring. Examples include biometric identification systems and predictive maintenance systems.3. Limited Risk: These AI systems have a lower risk to human life and safety, including those used in leisure and entertainment, customer service, and marketing. Examples include chatbots and recommendation systems.4. Low Risk: These AI systems have a very low risk to human life and safety, including those used in administrative tasks, management, and control of non-essential processes. Examples include automated data processing and monitoring systems.5. No Risk: These AI systems have no risk to human life and safety, including those used for administrative purposes and research. Examples include automated data analysis and pattern recognition systems.6. Unspecified Risk: These AI systems fall outside the scope of the AI Act due to their lack of a clear impact on human life and safety. Examples include AI systems used for personal entertainment or hobbies.IV. Key Provisions of the AI ActThe AI Act includes several key provisions to ensure the safe and ethical use of AI:1. Framework for AI Risk Management: The act requires AI developers to conduct a risk assessment and implement appropriate risk management measures for AI systems. This includes identifying potential risks,evaluating their impact, and implementing measures to mitigate those risks.2. Transparency and Explainability: The act requires AI systems to be transparent and explainable, particularly those with a high risk level. This means that users should be able to understand how the AI system works and how it makes decisions.3. Data Quality and Privacy: The act sets out rules for the collection, processing, and storage of data used in AI systems. It also includes provisions to protect individuals' privacy and ensure the security of personal data.4. Human Oversight: The act requires AI systems to be designed and operated in a manner that allows for human oversight, particularly for high-risk AI systems.5. Labeling and Marketing: The act includes provisions for the labeling and marketing of AI products and services, ensuring that consumers are informed about the AI technologies they use.6. Liability: The act establishes rules for determining liability in the event of harm caused by AI systems.V. Implementation and EnforcementThe AI Act will be implemented through a combination of EU regulations and directives. EU institutions, such as the European Commission and the European Parliament, will be responsible for drafting and adopting the necessary regulations. National authorities in each EU member state will be responsible for enforcing the act.VI. ConclusionThe EU Artificial Intelligence Act represents a significant step forward in the regulation of AI technologies. By establishing a comprehensive and harmonized regulatory framework, the AI Act aims to ensure that AIis developed and used in a manner that is safe, ethical, and beneficial to society. As AI continues to evolve, the AI Act will serve as a model for other jurisdictions around the world.---欧盟人工智能法案:全面概述I. 引言欧洲联盟(EU)在人工智能(AI)领域的监管创新一直处于前沿。
AI中英文版本对照
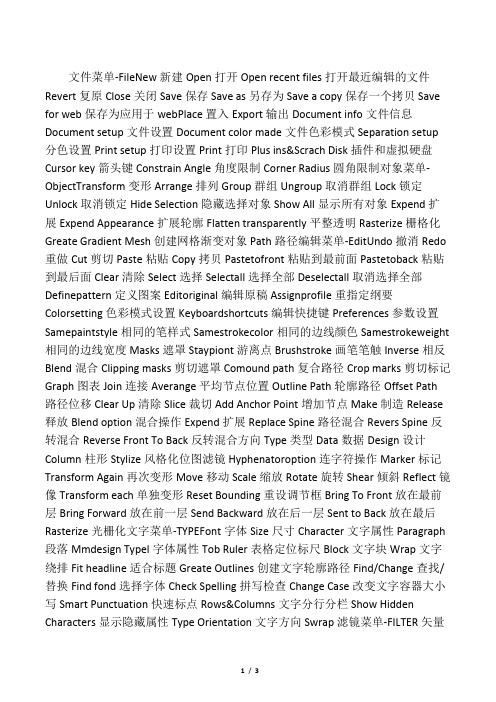
文件菜单-FileNew新建Open打开Open recent files打开最近编辑的文件Revert复原Close关闭Save保存Save as另存为Save a copy保存一个拷贝Save for web保存为应用于webPlace置入Export输出Document info文件信息Document setup文件设置Document color made文件色彩模式Separation setup 分色设置Print setup打印设置Print打印Plus ins&Scrach Disk插件和虚拟硬盘Cursor key箭头键Constrain Angle角度限制Corner Radius圆角限制对象菜单-ObjectTransform变形Arrange排列Group群组Ungroup取消群组Lock锁定Unlock取消锁定Hide Selection隐藏选择对象Show All显示所有对象Expend扩展Expend Appearance扩展轮廓Flatten transparently平整透明Rasterize栅格化Greate Gradient Mesh创建网格渐变对象Path路径编辑菜单-EditUndo撤消Redo 重做Cut剪切Paste粘贴Copy拷贝Pastetofront粘贴到最前面Pastetoback粘贴到最后面Clear清除Select选择Selectall选择全部Deselectall取消选择全部Definepattern定义图案Editoriginal编辑原稿Assignprofile重指定纲要Colorsetting色彩模式设置Keyboardshortcuts编辑快捷键Preferences参数设置Samepaintstyle相同的笔样式Samestrokecolor相同的边线颜色Samestrokeweight 相同的边线宽度Masks遮罩Staypiont游离点Brushstroke画笔笔触Inverse相反Blend混合Clipping masks剪切遮罩Comound path复合路径Crop marks剪切标记Graph图表Join连接Averange平均节点位置Outline Path轮廓路径Offset Path路径位移Clear Up清除Slice裁切Add Anchor Point增加节点Make制造Release 释放Blend option混合操作Expend扩展Replace Spine路径混合Revers Spine反转混合Reverse Front To Back反转混合方向Type类型Data数据Design设计Column柱形Stylize风格化位图滤镜Hyphenatoroption连字符操作Marker标记Transform Again再次变形Move移动Scale缩放Rotate旋转Shear倾斜Reflect镜像Transform each单独变形Reset Bounding重设调节框Bring To Front放在最前层Bring Forward放在前一层Send Backward放在后一层Sent to Back放在最后Rasterize光栅化文字菜单-TYPEFont字体Size尺寸Character文字属性Paragraph 段落Mmdesign Typel字体属性Tob Ruler表格定位标尺Block文字块Wrap文字绕排Fit headline适合标题Greate Outlines创建文字轮廓路径Find/Change查找/替换Find fond选择字体Check Spelling拼写检查Change Case改变文字容器大小写Smart Punctuation快速标点Rows&Columns文字分行分栏Show Hidden Characters显示隐藏属性Type Orientation文字方向Swrap滤镜菜单-FILTER矢量滤镜Apply Last Filter应用刚才的滤镜Last Filter刚才的滤镜Color色彩Create创建Distort扭曲Pen and Ink墨水笔Artistic艺术化Brush Strokes笔刷化Distort扭曲Sketch素描效果Stylize风格化Texture纹理化Adjust Color颜色调整Blend Front to Back混合前后图形的颜色Blend Vertically混合垂直放置图形的颜色Convert to CMYK转换为CMYKConvert to Grayscale转换为灰度Convert to RGB转换为RGBInvert Colors反色Overprint Black黑色压印文字菜单-TYPEFont字体Size 尺寸Character文字属性Paragraph段落Mmdesign Typel字体属性Tob Ruler表格定位标尺Block文字块Wrap文字绕排Fit headline适合标题Greate Outlines创建文字轮廓路径Find/Change查找/替换Find fond选择字体Check Spelling拼写检查Change Case改变文字容器大小写Smart Punctuation快速标点Rows&Columns文字分行分栏Show Hidden Characters显示隐藏属性Type Orientation文字方向Swrap滤镜菜单-FILTER矢量滤镜Apply Last Filter应用刚才的滤镜Last Filter刚才的滤镜Color色彩Create创建Distort扭曲Pen and Ink墨水笔Stylize风格化Distort变形位图滤镜Artistic艺术化Brush Strokes笔刷化Distort扭曲Sketch素描效果Stylize风格化Texture纹理化Adjust Color颜色调整Blend Front to Back混合前后图形的颜色Blend Vertically混合垂直放置图形的颜色Convert to CMYK转换为CMYKConvert to Grayscale转换为灰度Convert to RGB转换为RGBInvert Colors反色Overprint Black黑色压印Show/hide edges显示/隐藏选中路径Show/hide page tiling显示/隐藏工作区标志Show/hide template显示/隐藏模板Show/hide rules显示/隐藏标尺Show/hide bounding box显示/隐藏限制框Show/hide transparency grid显示/隐藏透明网格Guides参考线Smart guides实时参考线Show/hide grid显示/隐藏网格Snip to grid对齐网格Snip to point定点对齐New view新查看方式Edit views编辑查看方式Saturation饱和度Create建立Object Mosaic马赛克效果Trim Mark裁剪标记Distort变形Punk&Bloat尖角和圆角变形Boughen粗糙化Scribble And Tweak潦草和扭曲Twirl涡形旋转ZigZag文字效果Add Arrowhead加箭头Drop shadow加阴影Round corner圆角化Artistic 艺术化Blur模糊化Brush Strokes笔痕Piselate象素化Sharpen锐化Sketch素描效果Stylize风格化Texture纹理化Video视频特效菜单-EFFECTApplylasteffect重复刚才的特效Lasteffect刚才的特效Converttoshape转换形状Distorttransform自由变换Path路径特效Pathfinder路径合并模式Rasterize光栅化Stylize风格化视图菜单-VIEWOutline路径轮廓视图Overprintpreview印前视图模式Pixelpreview象素视图模式Proofsetup校验设置Zoomin放大Zoomout缩小Fitinwindow适合窗口Actualsize实际尺寸。
评估相关术语中英文对照

评估术语表►AAA:美国估价师协会►AACI:(加拿大评估协会)加拿大注册估价师►ABAR:(美国企业估价师协会)商业价值评估审查►ABV: (美国注册会计师协会)商业价值评估资格►AI:美国评估协会►AIC:加拿大评估协会►AICPA: 美国注册会计师协会►AITF:评估问题专责小组►AM: (美国评估师协会) 注册会员►AMC:评估管理公司►APB:(美国资产评估基金会)评估实务委员会►AQB:(美国资产评估基金会)估价师资格委员会►ARM: (美国评估师协会) 评估审查与管理认证►ASA:美国评估师协会/注册高级评估师►ASA BVS:美国评估师协会企业价值评估规范/企业评估准则►ASB:(美国资产评估基金会)评估标准委员会►ASFMRA:美国农场主评估协会►AVA:(美国企业价值评估分析师协会)认证价值分析师►AVM: 自动估价模型►BPO: 经纪人估值意见/经纪人价格意见►BVAL:(美国企业估价师协会)法律诉讼评估师►BVC:美国评估师协会企业估价委员会►CBA: (美国企业估价师协会) 注册企业价值评估师►CBV: 特许企业价值评估师►CFA: (特许金融分析师协会) 特许金融分析师►CFAI: 特许金融分析师协会►CICBV:加拿大特许企业价值评估师协会►CRA:(加拿大评估协会)住宅估价师►CVA:(全国企业价值评估分析师协会)注册价值分析师►FASA:(美国评估师协会)高级会员/荣誉会员►FCBV: (加拿大特许企业价值评估师协会)高级会员►FRICS: 英国皇家特许测量师学会资深会员►IAAO: 国际估税官协会►IACVA: 国际企业价值评估分析师协会/国际注册价值分析师协会►IBA:商业评估师协会/美国企业估价师协会►IEPR:波多黎各评估师协会►IIBV:国际企业价值评估学会►IRWA: 国际通行权协会►IVS: 国际评估准则►IVSC: 国际评估准则理事会►MAI: 美国评估协会会员►MBREA: 马萨诸塞州房地产估价师►MCBA:(美国企业估价师协会)高级注册评估师资格►MRICS:英国皇家特许测量师学会会员►NACVA:美国企业价值评估分析师协会/注册价值分析师全国联盟►NAIFA: 美国独立收费评估师协会►RICS: 英国皇家特许测量师学会►RICS RV:英国皇家特许测量师学会注册估价师►SRA:(美国评估协会)高级住宅估价师►SSVS:(美国注册会计师协会)企业价值评估准则►TAF:美国资产评估基金会/美国评估促进协会►USPAP: 专业估价操作统一标准►VRG:美国财务会计准则委员会美国财务会计准则委员会►VSC BVC:(美国评估师协会)商业评估委员会之下的估价标准委员会。
AI(人工智能)术语中英文对照表
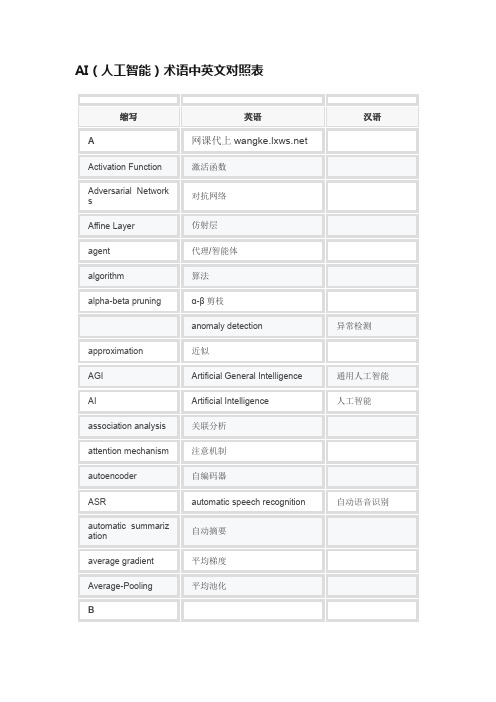
AI(人工智能)术语中英文对照表缩写英语汉语A网课代上Activation Function激活函数Adversarial Networks对抗网络Affine Layer仿射层agent代理/智能体algorithm算法alpha-beta pruningα-β剪枝anomaly detection异常检测approximation近似AGI Artificial General Intelligence通用人工智能AI Artificial Intelligence人工智能association analysis关联分析attention mechanism注意机制autoencoder自编码器ASR automatic speech recognition自动语音识别automatic summarization自动摘要average gradient平均梯度Average-Pooling平均池化BBP backpropagation反向传播BPTT Backpropagation Through Time 通过时间的反向传播BN Batch Normalization分批标准化Bayesian network贝叶斯网络Bias-Variance Dilemma偏差/方差困境Bi-LSTM Bi-directional Long-Short TermMemory双向长短期记忆bias偏置/偏差big data大数据Boltzmann machine玻尔兹曼机CCPU Central Processing Unit中央处理器chunk词块clustering聚类cluster analysis聚类分析co-adapting共适应co-occurrence共现Computation Cost计算成本Computational Linguistics计算语言学computer vision计算机视觉concept drift概念漂移CRF conditional random field条件随机域/场convergence收敛CA conversational agent会话代理convexity凸性CNN convolutional neural network卷积神经网络Cost Function成本函数cross entropy交叉熵DDecision Boundary决策边界Decision Trees决策树DBN Deep Belief Network深度信念网络DCGAN Deep Convolutional GenerativeAdversarial Network深度卷积生成对抗网络DL deep learning深度学习DNN deep neural network深度神经网络Deep Q-Learning深度Q学习DQN Deep Q-Network深度Q网络DNC differentiable neural computer可微分神经计算机dimensionality reduction algorithm降维算法discriminative model判别模型discriminator判别器divergence散度domain adaption领域自适应DropoutDynamic Fusion动态融合EEmbedding嵌入emotional analysis情绪分析End-to-End端到端EM Expectation-Maximization期望最大化Exploding GradientProblem梯度爆炸问题ELM Extreme Learning Machine超限学习机FFAIR Facebook Artificial IntelligenceResearchFacebook人工智能研究所factorization因子分解feature engineering特征工程Featured Learning特征学习Feedforward Neural Networks前馈神经网络Ggame theory博弈论GMM Gaussian Mixture Model高斯混合模型GA Genetic Algorithm遗传算法Generalization泛化GAN Generative Adversarial Networks生成对抗网络Generative Model生成模型Generator生成器Global Optimization全局优化GNMT Google Neural Machine Translation谷歌神经机器翻译Gradient Descent梯度下降graph theory图论GPU graphics processing unit 图形处理单元/图形处理器HHDM hidden dynamic model隐动态模型hidden layer隐藏层HMM Hidden Markov Model隐马尔可夫模型hybrid computing混合计算hyperparameter超参数IICA Independent Component Analysis独立成分分析input输入ICML International Conference for Machine Learning国际机器学习大会language phenomena语言现象latent dirichlet allocation隐含狄利克雷分布JJSD Jensen-Shannon Divergence JS距离KK-Means Clustering K-均值聚类K-NN K-Nearest Neighbours AlgorithmK-最近邻算法Knowledge Representation知识表征KB knowledge base知识库LLatent Dirichlet Allocation隐狄利克雷分布LSA latent semantic analysis潜在语义分析learner学习器Linear Regression线性回归log likelihood对数似然Logistic Regression Logistic回归LSTM Long-Short Term Memory长短期记忆loss损失MMT machine translation机器翻译Max-Pooling最大池化Maximum Likelihood最大似然minimax game最小最大博弈Momentum动量MLP Multilayer Perceptron多层感知器multi-document summarization多文档摘要MLP multi layered perceptron多层感知器multimodal learning多模态学习multiple linear regression多元线性回归NNaive Bayes Classifier朴素贝叶斯分类器named entity recognition命名实体识别Nash equilibrium纳什均衡NLG natural language generation自然语言生成NLP natural language processing自然语言处理NLL Negative Log Likelihood负对数似然NMT Neural Machine Translation神经机器翻译NTM Neural Turing Machine神经图灵机NCE noise-contrastive estimation噪音对比估计non-convex optimization非凸优化non-negative matrix factorization非负矩阵分解Non-Saturating Game非饱和博弈Oobjective function目标函数Off-Policy离策略On-Policy在策略one shot learning一次性学习output输出PParameter参数parse tree解析树part-of-speech tagging词性标注PSO Particle Swarm Optimization粒子群优化算法perceptron感知器polarity detection极性检测pooling池化PPGN Plug and Play Generative Network即插即用生成网络PCA principal component analysis主成分分析Probability Graphical Model概率图模型QQNN Quantized Neural Network量子化神经网络quantum computer量子计算机Quantum Computing量子计算RRBF Radial Basis Function径向基函数Random Forest Algorithm随机森林算法ReLU Rectified Linear Unit 线性修正单元/线性修正函数RNN Recurrent Neural Network循环神经网络recursive neural network递归神经网络RL reinforcement learning强化学习representation表征representation learning表征学习Residual Mapping残差映射Residual Network残差网络RBM Restricted Boltzmann Machine受限玻尔兹曼机Robot机器人Robustness稳健性RE Rule Engine规则引擎Ssaddle point鞍点Self-Driving自动驾驶SOM self organised map自组织映射Semi-Supervised Learning半监督学习sentiment analysis情感分析SLAM simultaneous localization and mapping同步定位与地图构建SVD Singular Value Decomposition奇异值分解Spectral Clustering谱聚类Speech Recognition语音识别SGD stochastic gradient descent随机梯度下降supervised learning监督学习SVM Support Vector Machine支持向量机synset同义词集Tt-SNE T-Distribution Stochastic Neighbour EmbeddingT-分布随机近邻嵌入tensor张量TPU Tensor Processing Units张量处理单元the least square method最小二乘法Threshold阙值Time Step时间步骤tokenization标记化treebank树库transfer learning迁移学习Turing Machine图灵机Uunsupervised learning无监督学习VVanishing Gradient Problem梯度消失问题VC Theory Vapnik–Chervonenkis theory 万普尼克-泽范兰杰斯理论von Neumann architecture 冯·诺伊曼架构/结构WWGAN Wasserstein GANW weight权重word embedding词嵌入WSD word sense disambiguation词义消歧XYZZSL zero-shot learning零次学习zero-data learning零数据学习。
人工智能概论中英文术语对照表
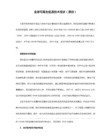
人工智能概论中英文术语对照表动作action专家系统Expert system人工智能语言AI language祖先过滤形策略ancestry-filtered form strategy与节点AND node与或图AND/OR graph与或树AND/OR tree回答语句answer statement人工智能artificial intelligence,AI原子公式atomic formula自动定理证明automatic theorem provingB规则B-rule倒退值backed-up value回溯backtracking盲目搜索,无信息搜索blind search宽度优先搜索breadth-first search子句clause组合爆炸combinatorial explosion冲突解决conflict resolution合取式conjunct合取conjunction合取范式conjunctive normal form连词,连接词connective一致解图consistant solution graph控制策略control strategy费用cost演绎deduction深度优先搜索depth-first search推导表,引导图derivation graph差别difference有向图directed graph析取式disjunct析取disjunction谓词演算辖域domain in predicate calculus论域,文字域domain of discourse搜索算法的效率efficiency of search algorithm空子句empty clause等价equivalence估计费用estimated cost估值函数evaluation function存在量词existential quantifier扩展节点expending node节点的扩展expansion of nodeF规则F-rule事实fact一阶谓词演算first order predicate calculus 博弈game图graph图表示法graph notation图搜索graph search图搜索控制策略graph-search control strategy 启发函数heuristic function启发信息heuristic information启发搜索heuristic search蕴涵,蕴涵式implication推理inference智能intelligence解释器interpreter知识knowledge知识获取knowledge acquisition全局数据库Global database知识库knowledge base知识工程knowledge engineering学习learning启发式搜索Heuristic search线形输入形策略linear-input form strategy文字literal逻辑logic逻辑连词logic connective逻辑推理logic reasoning匹配match模式匹配match pattern母式matrix最一般合一者most general unifierNP完全问题NP-complete problem算符、算子、操作符operator最优解树optimal solution tree有序搜索ordered search谓词predicate谓词演算predicate calculus谓词逻辑predicate logic前缀prefix本原问题primitive problem问题归约problem-reduction问题求解problem solving产生式production产生式规则production rule量词quantifier推理reasoning正向推理forward reasoning逆向推理backward reasoning推理机reasoning machine归约reduction反演refutation反演树refutation tree归结resolution归结原理resolution principle归结反演resolution refutation归结式resolvent可满足性satisfiability模式识别Pattern recognition量词辖域scope of quantifier搜索search, searching搜索算法searching algorithm搜索图searching graph搜索策略searching strategy搜索树searching tree句子sentence解图solution graph解树solution tree可解节点solvable node可解标示过程solvable labeling procedure 状态state状态空间state space代换例substitution instance代换substitution重言式tautology项term定理证明theorem-proving不确定性uncertainty合一unifier最一般合一most general unifier全称量词universal quantifier不可满足集unsatisfiable set不可解标示过程unsolvable-labeling procedure 不可解节点unsolvable node永真式validity合适公式、合式公式well-formed formula (wff)谓词演算公式wffs of predicate calculus人工神经网络artificial neural network遗传算法genetic algorithm机器学习machine learning。
人工智能英汉
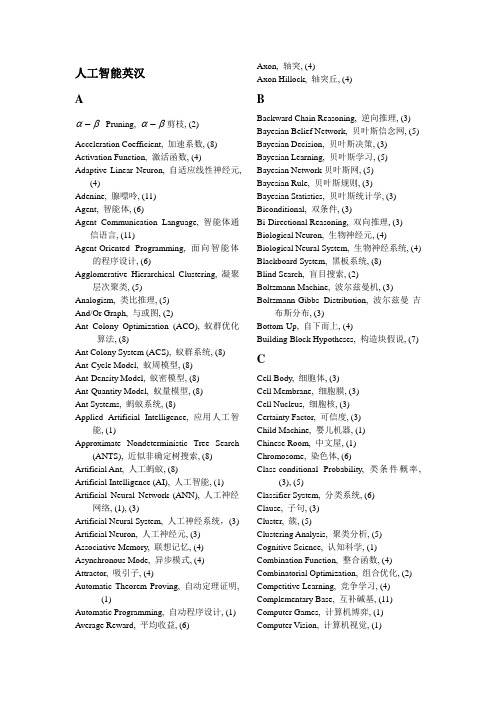
人工智能英汉Aβα-Pruning, βα-剪枝, (2) Acceleration Coefficient, 加速系数, (8) Activation Function, 激活函数, (4) Adaptive Linear Neuron, 自适应线性神经元,(4)Adenine, 腺嘌呤, (11)Agent, 智能体, (6)Agent Communication Language, 智能体通信语言, (11)Agent-Oriented Programming, 面向智能体的程序设计, (6)Agglomerative Hierarchical Clustering, 凝聚层次聚类, (5)Analogism, 类比推理, (5)And/Or Graph, 与或图, (2)Ant Colony Optimization (ACO), 蚁群优化算法, (8)Ant Colony System (ACS), 蚁群系统, (8) Ant-Cycle Model, 蚁周模型, (8)Ant-Density Model, 蚁密模型, (8)Ant-Quantity Model, 蚁量模型, (8)Ant Systems, 蚂蚁系统, (8)Applied Artificial Intelligence, 应用人工智能, (1)Approximate Nondeterministic Tree Search (ANTS), 近似非确定树搜索, (8) Artificial Ant, 人工蚂蚁, (8)Artificial Intelligence (AI), 人工智能, (1) Artificial Neural Network (ANN), 人工神经网络, (1), (3)Artificial Neural System, 人工神经系统,(3) Artificial Neuron, 人工神经元, (3) Associative Memory, 联想记忆, (4) Asynchronous Mode, 异步模式, (4) Attractor, 吸引子, (4)Automatic Theorem Proving, 自动定理证明,(1)Automatic Programming, 自动程序设计, (1) Average Reward, 平均收益, (6) Axon, 轴突, (4)Axon Hillock, 轴突丘, (4)BBackward Chain Reasoning, 逆向推理, (3) Bayesian Belief Network, 贝叶斯信念网, (5) Bayesian Decision, 贝叶斯决策, (3) Bayesian Learning, 贝叶斯学习, (5) Bayesian Network贝叶斯网, (5)Bayesian Rule, 贝叶斯规则, (3)Bayesian Statistics, 贝叶斯统计学, (3) Biconditional, 双条件, (3)Bi-Directional Reasoning, 双向推理, (3) Biological Neuron, 生物神经元, (4) Biological Neural System, 生物神经系统, (4) Blackboard System, 黑板系统, (8)Blind Search, 盲目搜索, (2)Boltzmann Machine, 波尔兹曼机, (3) Boltzmann-Gibbs Distribution, 波尔兹曼-吉布斯分布, (3)Bottom-Up, 自下而上, (4)Building Block Hypotheses, 构造块假说, (7) CCell Body, 细胞体, (3)Cell Membrane, 细胞膜, (3)Cell Nucleus, 细胞核, (3)Certainty Factor, 可信度, (3)Child Machine, 婴儿机器, (1)Chinese Room, 中文屋, (1) Chromosome, 染色体, (6)Class-conditional Probability, 类条件概率,(3), (5)Classifier System, 分类系统, (6)Clause, 子句, (3)Cluster, 簇, (5)Clustering Analysis, 聚类分析, (5) Cognitive Science, 认知科学, (1) Combination Function, 整合函数, (4) Combinatorial Optimization, 组合优化, (2) Competitive Learning, 竞争学习, (4) Complementary Base, 互补碱基, (11) Computer Games, 计算机博弈, (1) Computer Vision, 计算机视觉, (1)Conflict Resolution, 冲突消解, (3) Conjunction, 合取, (3)Conjunctive Normal Form (CNF), 合取范式,(3)Collapse, 坍缩, (11)Connectionism, 连接主义, (3) Connective, 连接词, (3)Content Addressable Memory, 联想记忆, (4) Control Policy, 控制策略, (6)Crossover, 交叉, (7)Cytosine, 胞嘧啶, (11)DData Mining, 数据挖掘, (1)Decision Tree, 决策树, (5) Decoherence, 消相干, (11)Deduction, 演绎, (3)Default Reasoning, 默认推理(缺省推理),(3)Defining Length, 定义长度, (7)Rule (Delta Rule), 德尔塔规则, 18(3) Deliberative Agent, 慎思型智能体, (6) Dempster-Shafer Theory, 证据理论, (3) Dendrites, 树突, (4)Deoxyribonucleic Acid (DNA), 脱氧核糖核酸, (6), (11)Disjunction, 析取, (3)Distributed Artificial Intelligence (DAI), 分布式人工智能, (1)Distributed Expert Systems, 分布式专家系统,(9)Divisive Hierarchical Clustering, 分裂层次聚类, (5)DNA Computer, DNA计算机, (11)DNA Computing, DNA计算, (11) Discounted Cumulative Reward, 累计折扣收益, (6)Domain Expert, 领域专家, (10) Dominance Operation, 显性操作, (7) Double Helix, 双螺旋结构, (11)Dynamical Network, 动态网络, (3)E8-Puzzle Problem, 八数码问题, (2) Eletro-Optical Hybrid Computer, 光电混合机, (11)Elitist strategy for ant systems (EAS), 精化蚂蚁系统, (8)Energy Function, 能量函数, (3) Entailment, 永真蕴含, (3) Entanglement, 纠缠, (11)Entropy, 熵, (5)Equivalence, 等价式, (3)Error Back-Propagation, 误差反向传播, (4) Evaluation Function, 评估函数, (6) Evidence Theory, 证据理论, (3) Evolution, 进化, (7)Evolution Strategies (ES), 进化策略, (7) Evolutionary Algorithms (EA), 进化算法, (7) Evolutionary Computation (EC), 进化计算,(7)Evolutionary Programming (EP), 进化规划,(7)Existential Quantification, 存在量词, (3) Expert System, 专家系统, (1)Expert System Shell, 专家系统外壳, (9) Explanation-Based Learning, 解释学习, (5) Explanation Facility, 解释机构, (9)FFactoring, 因子分解, (11)Feedback Network, 反馈型网络, (4) Feedforward Network, 前馈型网络, (1) Feasible Solution, 可行解, (2)Finite Horizon Reward, 横向有限收益, (6) First-order Logic, 一阶谓词逻辑, (3) Fitness, 适应度, (7)Forward Chain Reasoning, 正向推理, (3) Frame Problem, 框架问题, (1)Framework Theory, 框架理论, (3)Free-Space Optical Interconnect, 自由空间光互连, (11)Fuzziness, 模糊性, (3)Fuzzy Logic, 模糊逻辑, (3)Fuzzy Reasoning, 模糊推理, (3)Fuzzy Relation, 模糊关系, (3)Fuzzy Set, 模糊集, (3)GGame Theory, 博弈论, (8)Gene, 基因, (7)Generation, 代, (6)Genetic Algorithms, 遗传算法, (7)Genetic Programming, 遗传规划(遗传编程),(7)Global Search, 全局搜索, (2)Gradient Descent, 梯度下降, (4)Graph Search, 图搜索, (2)Group Rationality, 群体理性, (8) Guanine, 鸟嘌呤, (11)HHanoi Problem, 梵塔问题, (2)Hebbrian Learning, 赫伯学习, (4)Heuristic Information, 启发式信息, (2) Heuristic Search, 启发式搜索, (2)Hidden Layer, 隐含层, (4)Hierarchical Clustering, 层次聚类, (5) Holographic Memory, 全息存储, (11) Hopfield Network, 霍普菲尔德网络, (4) Hybrid Agent, 混合型智能体, (6)Hype-Cube Framework, 超立方体框架, (8)IImplication, 蕴含, (3)Implicit Parallelism, 隐并行性, (7) Individual, 个体, (6)Individual Rationality, 个体理性, (8) Induction, 归纳, (3)Inductive Learning, 归纳学习, (5) Inference Engine, 推理机, (9)Information Gain, 信息增益, (3)Input Layer, 输入层, (4)Interpolation, 插值, (4)Intelligence, 智能, (1)Intelligent Control, 智能控制, (1) Intelligent Decision Supporting System (IDSS), 智能决策支持系统,(1) Inversion Operation, 倒位操作, (7)JJoint Probability Distribution, 联合概率分布,(5) KK-means, K-均值, (5)K-medoids, K-中心点, (3)Knowledge, 知识, (3)Knowledge Acquisition, 知识获取, (9) Knowledge Base, 知识库, (9)Knowledge Discovery, 知识发现, (1) Knowledge Engineering, 知识工程, (1) Knowledge Engineer, 知识工程师, (9) Knowledge Engineering Language, 知识工程语言, (9)Knowledge Interchange Format (KIF), 知识交换格式, (8)Knowledge Query and ManipulationLanguage (KQML), 知识查询与操纵语言,(8)Knowledge Representation, 知识表示, (3)LLearning, 学习, (3)Learning by Analog, 类比学习, (5) Learning Factor, 学习因子, (8)Learning from Instruction, 指导式学习, (5) Learning Rate, 学习率, (6)Least Mean Squared (LSM), 最小均方误差,(4)Linear Function, 线性函数, (3)List Processing Language (LISP), 表处理语言, (10)Literal, 文字, (3)Local Search, 局部搜索, (2)Logic, 逻辑, (3)Lyapunov Theorem, 李亚普罗夫定理, (4) Lyapunov Function, 李亚普罗夫函数, (4)MMachine Learning, 机器学习, (1), (5) Markov Decision Process (MDP), 马尔科夫决策过程, (6)Markov Chain Model, 马尔科夫链模型, (7) Maximum A Posteriori (MAP), 极大后验概率估计, (5)Maxmin Search, 极大极小搜索, (2)MAX-MIN Ant Systems (MMAS), 最大最小蚂蚁系统, (8)Membership, 隶属度, (3)Membership Function, 隶属函数, (3) Metaheuristic Search, 元启发式搜索, (2) Metagame Theory, 元博弈理论, (8) Mexican Hat Function, 墨西哥草帽函数, (4) Migration Operation, 迁移操作, (7) Minimum Description Length (MDL), 最小描述长度, (5)Minimum Squared Error (MSE), 最小二乘法,(4)Mobile Agent, 移动智能体, (6)Model-based Methods, 基于模型的方法, (6) Model-free Methods, 模型无关方法, (6) Modern Heuristic Search, 现代启发式搜索,(2)Monotonic Reasoning, 单调推理, (3)Most General Unification (MGU), 最一般合一, (3)Multi-Agent Systems, 多智能体系统, (8) Multi-Layer Perceptron, 多层感知器, (4) Mutation, 突变, (6)Myelin Sheath, 髓鞘, (4)(μ+1)-ES, (μ+1) -进化规划, (7)(μ+λ)-ES, (μ+λ) -进化规划, (7) (μ,λ)-ES, (μ,λ) -进化规划, (7)NNaïve Bayesian Classifiers, 朴素贝叶斯分类器, (5)Natural Deduction, 自然演绎推理, (3) Natural Language Processing, 自然语言处理,(1)Negation, 否定, (3)Network Architecture, 网络结构, (6)Neural Cell, 神经细胞, (4)Neural Optimization, 神经优化, (4) Neuron, 神经元, (4)Neuron Computing, 神经计算, (4)Neuron Computation, 神经计算, (4)Neuron Computer, 神经计算机, (4) Niche Operation, 生态操作, (7) Nitrogenous base, 碱基, (11)Non-Linear Dynamical System, 非线性动力系统, (4)Non-Monotonic Reasoning, 非单调推理, (3) Nouvelle Artificial Intelligence, 行为智能,(6)OOccam’s Razor, 奥坎姆剃刀, (5)(1+1)-ES, (1+1) -进化规划, (7)Optical Computation, 光计算, (11)Optical Computing, 光计算, (11)Optical Computer, 光计算机, (11)Optical Fiber, 光纤, (11)Optical Waveguide, 光波导, (11)Optical Interconnect, 光互连, (11) Optimization, 优化, (2)Optimal Solution, 最优解, (2)Orthogonal Sum, 正交和, (3)Output Layer, 输出层, (4)Outer Product, 外积法, 23(4)PPanmictic Recombination, 混杂重组, (7) Particle, 粒子, (8)Particle Swarm, 粒子群, (8)Particle Swarm Optimization (PSO), 粒子群优化算法, (8)Partition Clustering, 划分聚类, (5) Partitioning Around Medoids, K-中心点, (3) Pattern Recognition, 模式识别, (1) Perceptron, 感知器, (4)Pheromone, 信息素, (8)Physical Symbol System Hypothesis, 物理符号系统假设, (1)Plausibility Function, 不可驳斥函数(似然函数), (3)Population, 物种群体, (6)Posterior Probability, 后验概率, (3)Priori Probability, 先验概率, (3), (5) Probability, 随机性, (3)Probabilistic Reasoning, 概率推理, (3) Probability Assignment Function, 概率分配函数, (3)Problem Solving, 问题求解, (2)Problem Reduction, 问题归约, (2)Problem Decomposition, 问题分解, (2) Problem Transformation, 问题变换, (2) Product Rule, 产生式规则, (3)Product System, 产生式系统, (3) Programming in Logic (PROLOG), 逻辑编程, (10)Proposition, 命题, (3)Propositional Logic, 命题逻辑, (3)Pure Optical Computer, 全光计算机, (11)QQ-Function, Q-函数, (6)Q-learning, Q-学习, (6)Quantifier, 量词, (3)Quantum Circuit, 量子电路, (11)Quantum Fourier Transform, 量子傅立叶变换, (11)Quantum Gate, 量子门, (11)Quantum Mechanics, 量子力学, (11) Quantum Parallelism, 量子并行性, (11) Qubit, 量子比特, (11)RRadial Basis Function (RBF), 径向基函数,(4)Rank based ant systems (ASrank), 基于排列的蚂蚁系统, (8)Reactive Agent, 反应型智能体, (6) Recombination, 重组, (6)Recurrent Network, 循环网络, (3) Reinforcement Learning, 强化学习, (3) Resolution, 归结, (3)Resolution Proof, 归结反演, (3) Resolution Strategy, 归结策略, (3) Reasoning, 推理, (3)Reward Function, 奖励函数, (6) Robotics, 机器人学, (1)Rote Learning, 机械式学习, (5)SSchema Theorem, 模板定理, (6) Search, 搜索, (2)Selection, 选择, (7)Self-organizing Maps, 自组织特征映射, (4) Semantic Network, 语义网络, (3)Sexual Differentiation, 性别区分, (7) Shor’s algorithm, 绍尔算法, (11)Sigmoid Function, Sigmoid 函数(S型函数),(4)Signal Function, 信号函数, (3)Situated Artificial Intelligence, 现场式人工智能, (1)Spatial Light Modulator (SLM), 空间光调制器, (11)Speech Act Theory, 言语行为理论, (8) Stable State, 稳定状态, (4)Stability Analysis, 稳定性分析, (4)State Space, 状态空间, (2)State Transfer Function, 状态转移函数,(6)Substitution, 置换, (3)Stochastic Learning, 随机型学习, (4) Strong Artificial Intelligence (AI), 强人工智能, (1)Subsumption Architecture, 包容结构, (6) Superposition, 叠加, (11)Supervised Learning, 监督学习, (4), (5) Swarm Intelligence, 群智能, (8)Symbolic Artificial Intelligence (AI), 符号式人工智能(符号主义), (3) Synapse, 突触, (4)Synaptic Terminals, 突触末梢, (4) Synchronous Mode, 同步模式, (4)TThreshold, 阈值, (4)Threshold Function, 阈值函数, (4) Thymine, 胸腺嘧啶, (11)Topological Structure, 拓扑结构, (4)Top-Down, 自上而下, (4)Transfer Function, 转移函数, (4)Travel Salesman Problem, 旅行商问题, (4) Turing Test, 图灵测试, (1)UUncertain Reasoning, 不确定性推理, (3)Uncertainty, 不确定性, (3)Unification, 合一, (3)Universal Quantification, 全称量词, (4) Unsupervised Learning, 非监督学习, (4), (5)WWeak Artificial Intelligence (Weak AI), 弱人工智能, (1)Weight, 权值, (4)Widrow-Hoff Rule, 维德诺-霍夫规则, (4)。
游戏AI介绍(中英文对照)

1.全文翻译游戏AI介绍在广义上说,大多数游戏都包含一些人工智能(AI)的运用。
例如,开发人员多年来都使用AI来给游戏中无数的人物以生命力,从经典的街机游戏Pac Man的鬼魂到第一人称射击游戏中的机器人,还有很多其他游戏。
各种各样的游戏类型和游戏人物对什么是游戏AI给出了一个相当广泛的解释。
事实上,AI也在其他传统的科学领域得到广泛的运用。
一些开发商认为路径搜索是游戏AI的一部分,有些则认为碰撞检测是游戏AI的一部分。
显然,广义上来说游戏AI是无处不在的。
我们要继续解释什么是游戏AI,包括从简单的追逐和逃避在内的一切运动模式,以及神经网络和遗传算法。
游戏AI属于弱AI的范畴是最适合的,但是,在某个意义上,你可以想象游戏AI应用领域的广泛程度。
在游戏中,我们不一定对给参与游戏的机器方以人水平的智能感兴趣。
也许我们可通过写代码来控制非人类的生物,比如龙、机器人,甚至老鼠。
另外,谁说我们必须赋予机器方智慧呢?不赋予机器方智慧以增加游戏内容的多样性和丰富性。
虽然游戏AI经常被呼吁解决相当复杂的问题,但我们可以利用AI去尝试给机器方以个性模样、不同的性格,或塑造情感和各种性情,如,害怕,焦虑,等等。
游戏AI的定义是相当广泛和灵活的。
凡是能够产生适当水平的智能从而让游戏更加逼真,有挑战性,最重要的是使游戏变得更加有趣的东西,都可以被当做为游戏AI。
正像在游戏中使用真实的物理现象那样,优良的AI增加了游戏的沉浸性,吸引游戏者,使他们一度置身于虚拟世界中。
定性与非定性的AI游戏AI技术一般有两种形式:定性和非定性。
定性定性的行为表现是具有指定和可预见性。
不含有任何的不确定性。
一个简单的追逐算法就是定性行为的一个例子。
您可以在代码中设定机器方角色朝某一目标点沿x 和y坐标轴推进,直到角色的X和Y坐标与目标位置一致。
非定性非定性行为和定性行为正好相反。
这些行为具有一定程度的不确定性并且是不可预测性(不确定性的程度取决于AI方法的应用以及这些方法的执行情况)。
AI中英文对照
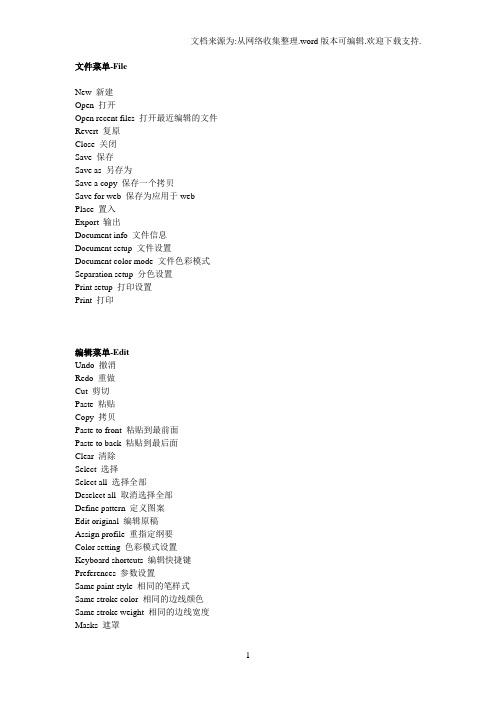
文件菜单-FileNew 新建Open 打开Open recent files 打开最近编辑的文件Revert 复原Close 关闭Save 保存Save as 另存为Save a copy 保存一个拷贝Save for web 保存为应用于web Place 置入Export 输出Document info 文件信息Document setup 文件设置Document color mode 文件色彩模式Separation setup 分色设置Print setup 打印设置Print 打印编辑菜单-EditUndo 撤消Redo 重做Cut 剪切Paste 粘贴Copy 拷贝Paste to front 粘贴到最前面Paste to back 粘贴到最后面Clear 清除Select 选择Select all 选择全部Deselect all 取消选择全部Define pattern 定义图案Edit original 编辑原稿Assign profile 重指定纲要Color setting 色彩模式设置Keyboard shortcuts 编辑快捷键Preferences 参数设置Same paint style 相同的笔样式Same stroke color 相同的边线颜色Same stroke weight 相同的边线宽度Masks 遮罩Stay piont 游离点Brush stroke 画笔笔触Inverse 相反Hyphenator option 连字符操作Plus ins&Scrach Disk 插件和虚拟硬盘Cursor key 箭头键Constrain Angle 角度限制Corner Radius 圆角限制对象菜单-ObjectTransform 变形Arrange 排列Group 群组Ungroup 取消群组Lock 锁定Unlock 取消锁定Hide Selection 隐藏选择对象Show All 显示所有对象Expend 扩展Expend Appearance 扩展轮廓Flatten transparently 平整透明Rasterize 栅格化Create Gradient Mesh 创建网格渐变对象Path 路径Blend 混合Clipping masks 剪切遮罩Comound path 复合路径Crop marks 剪切标记Graph 图表Join 连接Averange 平均节点位置Outline Path 轮廓路径Offset Path 路径位移Clear Up 清除Slice 裁切Add Anchor Point 增加节点Make 制造Release 释放Blend option 混合操作Expend 扩展Replace Spine 路径混合Revers Spine 反转混合Reverse Front To Back 反转混合方向Data 数据Design 设计Column 柱形Marker 标记Transform Again 再次变形Move 移动Scale 缩放Rotate 旋转Shear 倾斜Reflect 镜像Transform each 单独变形Reset Bounding 重设调节框Bring To Front 放在最前层Bring Forward 放在前一层Send Backward 放在后一层Sent to Back 放在最后Rasterize 光栅化文字菜单-TYPEFont 字体Size 尺寸Character 文字属性Paragraph 段落Mmdesign Typel 字体属性Tob Ruler 表格定位标尺Block 文字块Wrap 文字绕排Fit headline 适合标题Greate Outlines 创建文字轮廓路径Find/Change 查找/替换Find fond 选择字体Check Spelling 拼写检查Change Case 改变文字容器大小写Smart Punctuation 快速标点Rows&Columns 文字分行分栏Show Hidden Characters 显示隐藏属性Type Orientation 文字方向滤镜菜单-FILTERApply Last Filter 应用刚才的滤镜Last Filter 刚才的滤镜Create 创建Distort 扭曲Pen and Ink 墨水笔Stylize 风格化位图滤镜Artistic 艺术化Brush Strokes 笔刷化Distort 扭曲Sketch 素描效果Stylize 风格化Texture 纹理化Adjust Color 颜色调整Blend Front to Back 混合前后图形的颜色Blend Vertically 混合垂直放置图形的颜色Convert to CMYK 转换为CMYK Convert to Grayscale 转换为灰度Convert to RGB 转换为RGBInvert Colors 反色Overprint Black 黑色压印Saturation 饱和度Create 建立Object Mosaic 马赛克效果Trim Mark 裁剪标记Distort 变形Punk&Bloat 尖角和圆角变形Boughen 粗糙化Scribble And Tweak 潦草和扭曲Twirl 涡形旋转ZigZag 文字效果Add Arrowhead 加箭头Drop shadow 加阴影Round corner 圆角化Artistic 艺术化Blur 模糊化Brush Strokes 笔痕Distort 变形Piselate 象素化Sharpen 锐化Sketch 素描效果Stylize 风格化Texture 纹理化Video 视频特效菜单-EFFECTApply last effect 重复刚才的特效Last effect 刚才的特效Convert to shape 转换形状Distort transform 自由变换Path 路径特效Pathfinder 路径合并模式Rasterize 光栅化Stylize 风格化视图菜单-VIEWOutline 路径轮廓视图Overprint preview 印前视图模式Pixel preview 象素视图模式Proof setup 校验设置Zoom in 放大Zoom out 缩小Fit in window 适合窗口Actual size 实际尺寸Show/hide edges 显示/隐藏选中路径Show/hide page tiling 显示/隐藏工作区标志Show/hide template 显示/隐藏模板Show/hide rules 显示/隐藏标尺Show/hide bounding box 显示/隐藏限制框Show/hide transparency grid 显示/隐藏透明网格Guides 参考线Smart guides 实时参考线Show/hide grid 显示/隐藏网格Snip to grid 对齐网格。
欧盟人工智能法案中英文对照全套

欧盟人工智能法案中英文对照全套English:The European Union's proposed Artificial Intelligence Act aims to regulate AI systems used in a wide range of applications, focusing on transparency and accountability. The Act classifies AI systems into various categories based on their potential risks, with strict requirements for high-risk systems. These requirements include documentation, data governance, oversight measures, and human oversight. The Act also prohibits certain AI applications such as social scoring and biometric recognition in public spaces unless very strict conditions are met. It introduces a conformity assessment and labeling system for AI providers, emphasizing the importance of ensuring compliance with EU regulations. The Act sets out penalties for non-comp liance, with fines of up to €30 million or 6% of global annual turnover, whichever is higher. Overall, the Act seeks to balance the benefits of AI innovation with the protection of fundamental rights and ensuring a trustworthy AI environment.中文翻译:欧盟提出的人工智能法案旨在规范各种应用中使用的人工智能系统,重点放在透明度和问责制上。
PS-AI中英文对照(打印版)
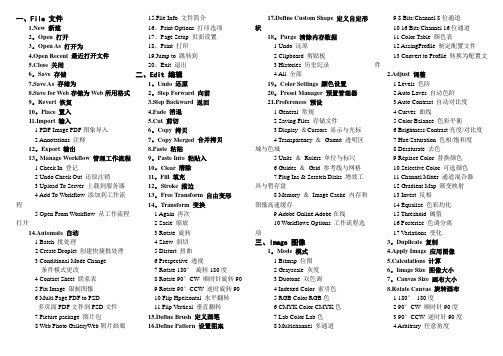
一、File 文件1.New 新建2。
Open 打开3。
Open As 打开为4.Open Recent 最近打开文件5.Close 关闭6。
Save 存储7.Save As 存储为8.Save for Web存储为Web所用格式9。
Revert 恢复10。
Place 置入11.Import 输入1 PDF Image PDF图象导入2 Annotations 注释12。
Export 输出13。
Manage Workflow 管理工作流程1 Check In 登记2 Undo Check Out 还原注销3 Upload To Server 上载到服务器4 Add To Workflow 添加到工作流程5 Open From Workflow 从工作流程打开14.Automate 自动1 Batch 批处理2 Create Droplet 创建快捷批处理3 Conditional Mode Change条件模式更改4 Contact Sheet 联系表5 Fix Image 限制图像6 Multi Page PDF to PSD多页面PDF文件到PSD文件7 Picture package 图片包8 Web Photo GalleryWeb照片画廊15.File Info 文件简介16。
Print Options 打印选项17。
Page Setup 页面设置18。
Print 打印19.Jump to 跳转到20。
Exit 退出二、Edit 编辑1。
Undo 还原2。
Step Forward 向前3.Step Backward 返回4.Fade 消退5.Cut 剪切6。
Copy 拷贝7。
Copy Merged 合并拷贝8.Paste 粘贴9。
Paste Into 粘贴入10。
Clear 清除11。
Fill 填充12。
Stroke 描边13。
Free Transform 自由变形14。
Transform 变换1 Again 再次2 Sacle 缩放3 Rotate 旋转4 Skew 斜切5 Distort 扭曲6 Prespective 透视7 Rotate 180°旋转180度8 Rotate 90°CW 顺时针旋转909 Rotate 90°CCW 逆时旋转9010 Flip Hpeizontal 水平翻转11 Flip Vertical 垂直翻转15.Define Brush 定义画笔16.Define Pattern 设置图案17.Define Custom Shape 定义自定形状18。
AI 中英文对照
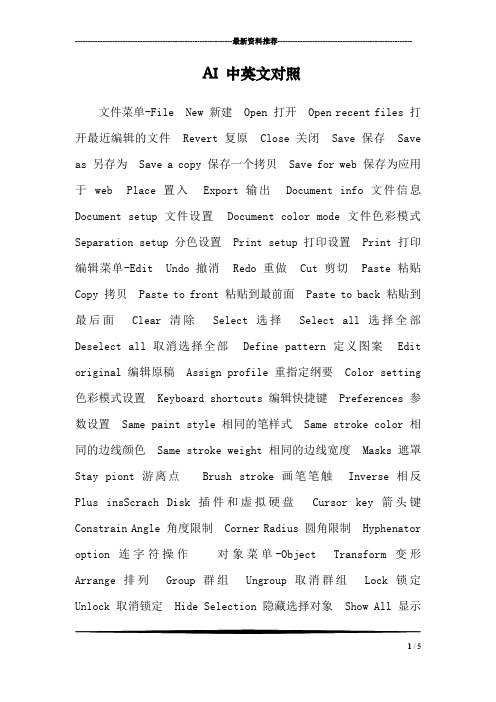
---------------------------------------------------------------最新资料推荐------------------------------------------------------AI 中英文对照文件菜单-File New 新建 Open 打开 Open recent files 打开最近编辑的文件 Revert 复原 Close 关闭 Save 保存 Save as 另存为 Save a copy 保存一个拷贝 Save for web 保存为应用于 web Place 置入 Export 输出 Document info 文件信息Document setup 文件设置 Document color mode 文件色彩模式Separation setup 分色设置 Print setup 打印设置 Print 打印编辑菜单-Edit Undo 撤消 Redo 重做 Cut 剪切 Paste 粘贴Copy 拷贝 Paste to front 粘贴到最前面 Paste to back 粘贴到最后面Clear 清除Select 选择Select all 选择全部Deselect all 取消选择全部 Define pattern 定义图案 Edit original 编辑原稿 Assign profile 重指定纲要 Color setting 色彩模式设置 Keyboard shortcuts 编辑快捷键 Preferences 参数设置 Same paint style 相同的笔样式 Same stroke color 相同的边线颜色 Same stroke weight 相同的边线宽度 Masks 遮罩Stay piont 游离点 Brush stroke 画笔笔触Inverse 相反Plus insScrach Disk 插件和虚拟硬盘Cursor key 箭头键Constrain Angle 角度限制 Corner Radius 圆角限制 Hyphenator option 连字符操作对象菜单-Object Transform 变形Arrange 排列Group 群组Ungroup 取消群组Lock 锁定Unlock 取消锁定 Hide Selection 隐藏选择对象 Show All 显示1 / 5所有对象 Expend 扩展 Expend Appearance 扩展轮廓 Flatten transparently 平整透明 Rasterize 栅格化 Create Gradient Mesh 创建网格渐变对象Path 路径Blend 混合Clipping masks 剪切遮罩 Comound path 复合路径 Crop marks 剪切标记Graph 图表 Join 连接 Averange 平均节点位置 Outline Path 轮廓路径 Offset Path 路径位移 Clear Up 清除 Slice 裁切Add Anchor Point 增加节点 Make 制造 Release 释放 Blend option 混合操作Expend 扩展Replace Spine 路径混合Revers Spine 反转混合 Reverse Front To Back 反转混合方向Type 类型 Data 数据 Design 设计 Column 柱形 Marker 标记Transform Again 再次变形 Move 移动 Scale 缩放 Rotate 旋转Shear 倾斜Reflect 镜像Transform each 单独变形Reset Bounding 重设调节框 Bring To Front 放在最前层 Bring Forward 放在前一层 Send Backward 放在后一层 Sent to Back 放在最后 Rasterize 光栅化文字菜单-TYPE Font 字体 Size 尺寸 Character 文字属性 Paragraph 段落 Mmdesign Typel 字体属性 Tob Ruler 表格定位标尺 Block 文字块 Wrap 文字绕排Fit headline 适合标题Greate Outlines 创建文字轮廓路径Find/Change 查找/替换 Find fond 选择字体 Check Spelling 拼写检查 Change Case 改变文字容器大小写 Smart Punctuation 快速标点 RowsColumns 文字分行分栏 Show Hidden Characters 显示隐藏属性Type Orientation 文字方向滤镜菜单-FILTER---------------------------------------------------------------最新资料推荐------------------------------------------------------ Apply Last Filter 应用刚才的滤镜Last Filter 刚才的滤镜Color 色彩 Create 创建 Distort 扭曲 Pen and Ink 墨水笔Stylize 风格化位图滤镜 Artistic 艺术化 Brush Strokes 笔刷化Distort 扭曲Sketch 素描效果Stylize 风格化Texture 纹理化 Adjust Color 颜色调整 Blend Front to Back 混合前后图形的颜色 Blend Vertically 混合垂直放置图形的颜色Convert to CMYK 转换为 CMYK Convert to Grayscale 转换为灰度Convert to RGB 转换为 RGB Invert Colors 反色 Overprint Black 黑色压印Saturation 饱和度Create 建立Object Mosaic 马赛克效果Trim Mark 裁剪标记Distort 变形PunkBloat 尖角和圆角变形 Boughen 粗糙化 Scribble And Tweak 潦草和扭曲 Twirl 涡形旋转 ZigZag 文字效果 Add Arrowhead 加箭头 Drop shadow 加阴影 Round corner 圆角化 Artistic 艺术化Blur 模糊化Brush Strokes 笔痕Distort 变形Piselate 象素化 Sharpen 锐化 Sketch 素描效果 Stylize 风格化 Texture 纹理化 Video 视频特效菜单-EFFECT Apply last effect 重复刚才的特效 Last effect 刚才的特效 Convert to shape 转换形状 Distort transform 自由变换 Path 路径特效Pathfinder 路径合并模式 Rasterize 光栅化 Stylize 风格化视图菜单-VIEW Outline 路径轮廓视图 Overprint preview 印前视图模式 Pixel preview 象素视图模式 Proof setup 校验设置3 / 5Zoom in 放大 Zoom out 缩小 Fit in window 适合窗口 Actual size 实际尺寸 Show/hide edges 显示/隐藏选中路径 Show/hide page tiling 显示/隐藏工作区标志 Show/hide template 显示/隐藏模板 Show/hide rules 显示/隐藏标尺 Show/hide bounding box 显示/隐藏限制框 Show/hide transparency grid 显示/隐藏透明网格 Guides 参考线 Smart guides 实时参考线 Show/hide grid 显示/隐藏网格 Snip to grid 对齐网格窗口:windows (按 Mac 系统排序) Arrange 排列 Workspace 工作区Browse add-ons 浏览附件Extensions 扩展功能Application frame 应用框架 Application bar 应用栏 Control 控制 Tools 工具 Actions 动作 Align 对齐 Appearance 外观 Artboards 画板 Asset export 项目输出 Attributes 属性 Brushes 画笔 Color guide 颜色参考Color themes 颜色信息CSS properties Document info 文档信息 Flattener preview 拼合预览器 Gradient 渐变 Image trace 图像临摹 Info 信息 Layers 图层 Libraries 库 Links 链接 Magic wand 魔棒 Navigator 导航 Pathfinder 路经查找器 Pattern options 图案选项 Separations preview 分色预览 Stroke 描边 SVG interactivity SVG 交互 Swatches 色板Symbols 符号 Transform 转化 Transparency 透明度 Type 文字Variables 变量其他:Shades 深色 Tins 淡色 Character 字符 Fill 填充stroke 描边 Opacity 不透明度 transparency 透明度---------------------------------------------------------------最新资料推荐------------------------------------------------------ Sublayer 子图层 Enter/exit isolation mode 进入/退出隔离模式Make/release clipping mask 创建/释放剪切蒙版 Merge selected 合并选择 Template 模板5 / 5。
人工智能AI革命外文翻译中英文
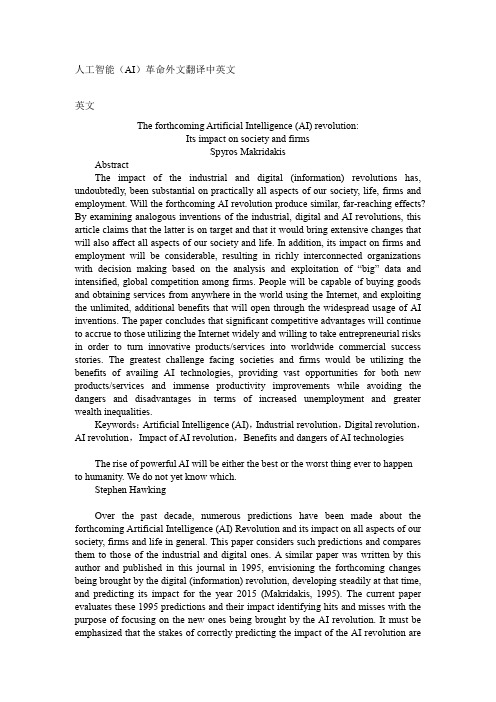
人工智能(AI)革命外文翻译中英文英文The forthcoming Artificial Intelligence (AI) revolution:Its impact on society and firmsSpyros MakridakisAbstractThe impact of the industrial and digital (information) revolutions has, undoubtedly, been substantial on practically all aspects of our society, life, firms and employment. Will the forthcoming AI revolution produce similar, far-reaching effects? By examining analogous inventions of the industrial, digital and AI revolutions, this article claims that the latter is on target and that it would bring extensive changes that will also affect all aspects of our society and life. In addition, its impact on firms and employment will be considerable, resulting in richly interconnected organizations with decision making based on th e analysis and exploitation of “big” data and intensified, global competition among firms. People will be capable of buying goods and obtaining services from anywhere in the world using the Internet, and exploiting the unlimited, additional benefits that will open through the widespread usage of AI inventions. The paper concludes that significant competitive advantages will continue to accrue to those utilizing the Internet widely and willing to take entrepreneurial risks in order to turn innovative products/services into worldwide commercial success stories. The greatest challenge facing societies and firms would be utilizing the benefits of availing AI technologies, providing vast opportunities for both new products/services and immense productivity improvements while avoiding the dangers and disadvantages in terms of increased unemployment and greater wealth inequalities.Keywords:Artificial Intelligence (AI),Industrial revolution,Digital revolution,AI revolution,Impact of AI revolution,Benefits and dangers of AI technologies The rise of powerful AI will be either the best or the worst thing ever to happento humanity. We do not yet know which.Stephen HawkingOver the past decade, numerous predictions have been made about the forthcoming Artificial Intelligence (AI) Revolution and its impact on all aspects of our society, firms and life in general. This paper considers such predictions and compares them to those of the industrial and digital ones. A similar paper was written by this author and published in this journal in 1995, envisioning the forthcoming changes being brought by the digital (information) revolution, developing steadily at that time, and predicting its impact for the year 2015 (Makridakis, 1995). The current paper evaluates these 1995 predictions and their impact identifying hits and misses with the purpose of focusing on the new ones being brought by the AI revolution. It must be emphasized that the stakes of correctly predicting the impact of the AI revolution arefar reaching as intelligent machines may become our “final invention” that may end human supremacy (Barrat, 2013). There is little doubt that AI holds enormous potential as computers and robots will probably achieve, or come close to, human intelligence over the next twenty years becoming a serious competitor to all the jobs currently performed by humans and for the first time raising doubt over the end of human supremacy.This paper is organized into four parts. It first overviews the predictions made in the 1995 paper for the year 2015, identifying successes and failures and concluding that major technological developments (notably the Internet and smartphones) were undervalued while the general trend leading up to them was predicted correctly. Second, it investigates existing and forthcoming technological advances in the field of AI and the ability of computers/machines to acquire real intelligence. Moreover, it summarizes prevailing, major views of how AI may revolutionize practically everything and its impact on the future of humanity. The third section sums up the impact of the AI revolution and describes the four major scenarios being advocated, as well as what could be done to avoid the possible negative consequences of AI technologies. The fourth section discusses how firms will be affected by these technologies that will transform the competitive landscape, how start-up firms are founded and the way success can be achieved. Finally, there is a brief concluding section speculating about the future of AI and its impact on our society, life, firms and employment.1. The 1995 paper: hits and missesThe 1995 paper (Makridakis, 1995) was written at a time when the digital (at that time it was called information) revolution was progressing at a steady rate. The paper predicted that by 2015 “the information revolution should be in full swing” and that “computers/communications” would be in widespread use, whi ch has actually happened, although its two most important inventions (the Internet and smartphones) and their significant influence were not foreseen as such. Moreover, the paper predicted that “a single computer (but not a smartphone) can, in addition to its traditional tasks, also become a terminal capable of being used interactively for the following:” (p. 804–805)• Picture phone and teleconference• Television and videos• Music• Shopping• On line banking and financial services• Reservations• Medic al advice• Access to all types of services• Video games• Other games (e.g., gambling, chess etc.)• News, sports and weather reports• Access to data banksThe above have all materialized and can indeed be accessed by computer,although the extent of their utilization was underestimated as smartphones are now being used widely. For instance, the ease of accessing and downloading scientific articles on one's computer in his/her office or home would have seemed like science fiction back in 1995, when finding such articles required spending many hours in the library (often in its basement for older publications) and making photocopies to keep them for later use. Moreover, having access, from one's smartphone or tablet, to news from anywhere in the world, being able to subscribe to digital services, obtain weather forecasts, purchase games, watch movies, make payments using smartphones and a plethora of other, useful applications was greatly underestimated, while the extensive use of the cloud for storing large amounts of data for free was not predicted at all at that time. Even in 1995 when the implications of Moore's law leading to increasing computer speed and storage while reducing costs were well known, nevertheless, it was hard to imagine that in 2016 there would be 60 trillion web pages, 2.5 billion smartphones, more than 2 billion personal computers and 3.5 billion Google searches a day.The paper correctly predicted “as wireless telecommunications will be possible the above list of capabilities can be accessed from anywhere in the world without the need for regular telephone lines”. What the 1995 paper missed, however, was that in 2015 top smartphones, costing less than €500, would be as powerful as the 1995 supercomputer, allowing access to the Internet and all tasks that were only performed by expensive computers at that time, including an almost unlimited availability of new, powerful apps providing a large array of innovative services that were not imagined twenty years ago. Furthermore, the paper correctly predicted super automation leading to unattended factories stating that “by 2015 there will be little need for people to do repetitive manual or mental tasks”. It also foresaw the decline of large industrial firms, increased global competition and the drop in the percentage of labour force employed in agriculture and manufacturing (more on these predictions in the section The Impact of the AI Revolution on Firms). It missed however the widespread utilization of the Internet (at that time it was a text only service), as well as search engines (notably Google), social networking sites(notably Facebook) and the fundamental changes being brought by the widespread use of Apple's iPhone, Samsung's Galaxy and Google's Android smartphones. It is indeed surprising today to see groups of people in a coffee shop or restaurant using their smartphones instead of speaking to each other and young children as little as three or four years of age playing with phones and tablets. Smartphones and tablets connected to the Internet through Wi-Fi have influenced social interactions to a significant extent, as well as the way we search for information, use maps and GPS for finding locations, and make payments. These technologies were not predicted in the 1995 paper.2. Towards the AI revolutionThe 1995 paper referred to Say, the famous French economist, who wrote in 1828 about the possibility of cars as substitutes for horses:“Nevertheless no machine will ever be able to perform what even the worst horses can - the service of carrying people and goods through the bustle and throng of a great city.” (p. 800)Say could never have dreamed of, in his wildest imagination, self-driving cars, pilotless airplanes, Skype calls, super computers, smartphones or intelligent robots. Technologies that seemed like pure science fiction less than 190 years ago are available today and some like self-driving vehicles will in all likelihood be in widespread use within the next twenty years. The challenge is to realistically predict forthcoming AI technologies without falling into the same short-sighted trap of Say and others, including my 1995 paper, unable to realize the momentous, non-linear advancements of new technologies. There are two observations to be made.First, 190 years is a brief period by historical standards and during this period we went from horses being the major source of transportation to self-driving cars and from the abacus and slide rules to powerful computers in our pockets. Secondly, the length of time between technological inventions and their practical, widespread use is constantly being reduced. For instance, it took more than 200 years from the time Newcomen developed the first workable steam engine in 1707 to when Henry Ford built a reliable and affordable car in 1908. It took more than 90 years between the time electricity was introduced and its extensive use by firms to substantially improve factory productivity. It took twenty years, however, between ENIAC, the first computer, and IBM's 360 system that was mass produced and was affordable by smaller business firms while it took only ten years between 1973 when Dr Martin Cooper made the first mobile call from a handheld device and its public launch by Motorola. The biggest and most rapid progress, however, took place with smartphones which first appeared in 2002 and saw a stellar growth with the release of new versions possessing substantial improvements every one or two years by the likes of Apple, Samsung and several Chinese firms. Smartphones, in addition to their technical features, now incorporate artificial intelligence characteristics that include understanding speech, providing customized advice in spoken language, completing words when writing a text and several other functions requiring embedded AI, provided by a pocket computer smaller in size than a pack of cigarettes.From smart machines to clever computers and to Artificial Intelligence (AI) programs: A thermostat is a simple mechanical device exhibiting some primitive but extremely valuable type of intelligence by keeping temperatures constant at some desired, pre-set level. Computers are also clever as they can be instructed to make extremely complicated decisions taking into account a large number of factors and selection criteria, but like thermostats such decisions are pre-programmed and based on logic, if-then rules and decision trees that produce the exact same results, as long as the input instructions are alike. The major advantage of computers is their lightning speed that allows them to perform billions of instructions per second. AI, on the other hand, goes a step further by not simply applying pre-programmed decisions, but instead exhibiting some learning capabilities.The story of the Watson computer beating Jeopardy's two most successful contestants is more complicated, since retrieving the most appropriate answer out of the 200 million pages of information stored in its memory is not a sign of real intelligence as it relied on its lightning speed to retrieve information in seconds. What is more challenging according to Jennings, one of Jeopardy's previous champions, is“to read clues in a natural language, understand puns and the red herrings, to unpack just the meaning of the clue” (May, 2013). Similarly, it is a sign of intelligence to improve it s performance by “playing 100 games against past winners”. (Best, 2016). Watson went several steps beyond Deep Blue towards AI by being able to understand spoken English and learn from his mistakes (New Yorker, 2016). However, he was still short of AlphaGo that defeated Go Champions in a game that cannot be won simply by using “brute force” as the number of moves in this game is infinite, requiring the program to use learning algorithms that can improve its performance as it plays more and more gamesComputers and real learning: According to its proponents, “the main focus of AI research is in teaching computers to think for themselves and improvise solutions to common problems” (Clark, 2015). But many doubt that computers can learn to think for themselves even though they can display signs of intelligence. David Silver, an AI scientist working at DeepMind, explained that “even though AlphaGo has affectively rediscovered the most subtle concepts of Go, its knowledge is implicit. The computer parse out these concepts –they simply emerge from its statistical comparisons of types of winning board positions at GO” (Chouard, 2016). At the same time Cho Hyeyeon, one of the strongest Go players in Korea commented that “AlphaGo seems like it knows everything!” while others believe that “AlphaGo is likely to start a ‘new revolution’ in the way we play Go”as “it is seeking simply to maximize its probability of reaching winning positions, rather than as human players tend to do –maximize territorial gains” (Chouard, 2016). Does it matter, as Silver said, that AlphaGo's knowledge of the game is implicit as long as it can beat the best players? A more serious issue is whether or not AlphaGo's ability to win games with fixed rules can extend to real life settings where not only the rules are not fixed, but they can change with time, or from one situation to another.From digital computers to AI tools: The Intel Pentium microprocessor, introduced in 1993, incorporated graphics and music capabilities and opened computers up to a large number of affordable applications extending beyond just data processing. Such technologies signalled the beginning of a new era that now includes intelligent personal assistants understanding and answering natural languages, robots able to see and perform an array of intelligent functions, self-driving vehicles and a host of other capabilities which were until then an exclusive human ability. The tech optimists ascertain that in less than 25 years computers went from just manipulating 0 and 1 digits, to utilizing sophisticated neural networkalgorithms that enable vision and the understanding and speaking of natural languages among others. Technology optimists therefore maintain there is little doubt that in the next twenty years, accelerated AI technological progress will lead to a breakthrough, based on deep learning that imitates the way young children learn, rather than the laborious instructions by tailor-made programs aimed for specific applications and based on logic, if-then rules and decision trees (Parloff, 2016).For instance, DeepMind is based on a neural program utilizing deep learning that teaches itself how to play dozens of Atari games, such as Breakout, as well or better than humans, without specific instructions for doing so, but by playing thousands ofgames and improving itself each time. This program, trained in a different way, became the AlphaGo that defeated GO champion Lee Sodol in 2016. Moreover, it will form the core of a new project to learn to play Starcraft, a complicated game based on both long term strategy as well as quick tactical decisions to stay ahead of an opponent, which DeepMind plans to be its next target for advancing deep learning (Kahn, 2016). Deep learning is an area that seems to be at the forefront of research and funding efforts to improve AI, as its successes have sparked a burst of activity in equity funding that reached an all-time high of more than $1 billion with 121 projects for start-ups in the second quarter of 2016, compared to 21 in the equivalent quarter of 2011 (Parloff, 2016).Google had two deep learning projects underway in 2012. Today it is pursuing more than 1000, according to their spokesperson, in all its major product sectors, including search, Android, Gmail, translation, maps, YouTube, and self-driving cars (The Week, 2016). IBM's Watson system used AI, but not deep learning, when it beat the two Jeopardy champions in 2011. Now though, almost all of Watson's 30 component services have been augmented by deep learning. Venture capitalists, who did not even know what deep learning was five years ago, today are wary of start-ups that do not incorporate it into their programs. We are now living in an age when it has become mandatory for people building sophisticated software applications to avoid click through menus by incorporating natural-language processing tapping deep learning (Parloff, 2016).How far can deep learning go? There are no limits according to technology optimists for three reasons. First as progress is available to practically everyone to utilize through Open Source software, researchers will concentrate their efforts on new, more powerful algorithms leading to cumulative learning. Secondly, deep learning algorithms will be capable of remembering what they have learned and apply it in similar, but different situations (Kirkpatrick et al., 2017). Lastly and equally important, in the future intelligent computer programs will be capable of writing new programs themselves, initially perhaps not so sophisticated ones, but improving with time as learning will be incorporated to be part of their abilities. Kurzweil (2005) sees nonbiological intelligence to match the range and subtlety of human intelligence within a quarter of a century and what he calls “Singularity” to occur by 2045, b ringing “the dawning of a new civilization that will enable us to transcend our biological limitations and amplify our creativity. In this new world, there will be no clear distinction between human and machine, real reality and virtual reality”.For some people these predictions are startling, with far-reaching implications should they come true. In the next section, four scenarios associated with the AI revolution are presented and their impact on our societies, life work and firms is discussed.3. The four AI scenariosUntil rather recently, famines, wars and pandemics were common, affecting sizable segments of the population, causing misery and devastation as well as a large number of deaths. The industrial revolution considerably increased the standards of living while the digital one maintained such rise and also shifted employment patterns,resulting in more interesting and comfortable office jobs. The AI revolution is promising even greater improvements in productivity and further expansion in wealth. Today more and more people, at least in developed countries, die from overeating rather than famine, commit suicide instead of being killed by soldiers, terrorists and criminals combined and die from old age rather than infectious disease (Harari, 2016). Table 1 shows the power of each revolution with the industrial one aiming at routine manual tasks, the digital doing so to routine mental ones and AI aiming at substituting, supplementing and/or amplifying practically all tasks performed by humans. The cri tical question is: “what will the role of humans be at a time when computers and robots could perform as well or better andmuch cheaper, practically all tasks that humans do at present?” There are four scenarios attempting to answer this question.The Optimists: Kurzweil and other optimists predict a “science fiction”, utopian future with Genetics, Nanotechnology and Robotics (GNR) revolutionizing everything, allowing humans to harness the speed, memory capacities and knowledge sharing ability of computers and our brain being directly connected to the cloud. Genetics would enable changing our genes to avoid disease and slow down, or even reverse ageing, thus extending our life span considerably and perhaps eventually achieving immortality. Nanotechnology, using 3D printers, would enable us to create virtually any physical product from information and inexpensive materials bringing an unlimited creation of wealth. Finally, robots would be doing all the actual work, leaving humans with the choice of spending their time performing activities of their choice and working, when they want, at jobs that interest them.The Pessimists: In a much quoted article from Wired magazine in 2000, Bill Joy (Joy, 2000) wrote “Our most powerful 21st-century technologies –robotics, genetic engineering, and nanotech –are threatening to make humans an endangered species”. Joy pointed out that as machines become more and more intelligent and as societal problems become more and more complex, people will let machines make all the important decisions for them as these decisions will bring better results than those made by humans. This situation will, eventually, result in machines being in effective control of all important decisions with people dependent on them and afraid to make their own choices. Joy and many other scientists (Cellan-Jones, 2014) and philosophers (Bostrom, 2014) believe that Kurzweil and his supporters vastly underestimate the magnitude of the challenge and the potential dangers which can arise from thinking machines and intelligent robots. They point out that in the utopian world of abundance, where all work will be done by machines and robots, humans may be reduced to second rate status (some saying the equivalent of computer pets) as smarter than them computers and robots will be available in large numbers and people will not be motivated to work, leaving computers/robots to be in charge of making all important decisions. It may not be a bad world, but it will definitely be a different one with people delegated to second rate status.Harari is the newest arrival to the ranks of pessimists. His recent book (Harari, 2016, p. 397) concludes with the following three statements:• “Science is converging to an all-encompassing dogma, which says thatorganisms are algorithm s, and life is data processing”• “Intelligence is decoupling from consciousness”• “Non-conscious but highly intelligent algorithms may soon know us better than we know ourselves”Consequently, he asks three key questions (which are actually answered by the above three statements) with terrifying implications for the future of humanity: • “Are organisms really just algorithms, and is life just data processing?”• “What is more valuable –intelligence or consciousness?”• “What will happen to society, polit ics and daily life when non-conscious but highly intelligent algorithms know us better than we know ourselves?”Harari admits that nobody really knows how technology will evolve or what its impact will be. Instead he discusses the implications of each of his three questions: • If indeed organisms are algorithms then thinking machines utilizing more efficient ones than those by humans will have an advantage. Moreover, if life is just data processing then there is no way to compete with computers that can consult/exploit practically all available information to base their decisions.• The non-conscious algorithms Google search is based on the consultation of millions of possible entries and often surprise us by their correct recommendations. The implications that similar, more advanced algorithms than those utilized by Google search will be developed (bearing in mind Google search is less than twenty years old) in the future and be able to access all available information from complete data bases are far reachi ng and will “provide us with better information than we could expect to find ourselves”.• Humans are proud of their consciousness, but does it matter that self-driving vehicles do not have one, but still make better decisions than human drivers, as can be confirmed by their significantly lower number of traffic accidents?When AI technologies are further advanced and self-driving vehicles are in widespread use, there may come a time that legislation may be passed forbidding or restricting human driving, even though that may still be some time away according to some scientists (Gomes, 2014). Clearly, self-driving vehicles do not exceed speed limits, do not drive under the influence of alcohol or drugs, do not get tired, do not get distracted by talking on the phone or sending SMS or emails and in general make fewer mistakes than human drivers, causing fewer accidents. There are two implications if humans are not allowed to drive. First, there will be a huge labour displacement for the 3.5 million unionized truck drivers in the USA and the 600 thousand ones in the UK (plus the additional number of non-unionized ones) as well as the more than one million taxi and Uber drivers in these two countries. Second, and more importantly, it will take away our freedom of driving, admitting that computers are superior to us. Once such an admission is accepted there will be no limits to letting computers also make a great number of other decisions, like being in charge of nuclear plants, setting public policies or deciding on optimal economic strategies as their biggest advantage is their objectivity and their ability to make fewer mistakes than humans.One can go as far as suggesting letting computers choose Presidents/PrimeMinisters and elected officials using objective criteria rather than having people voting emotionally and believing the unrealistic promises that candidates make. Although such a suggestion will never be accepted, at least not in the near future, it has its merits since people often choose the wrong candidate and later regret their choice after finding out that pre-election promises were not only broken, but they were even reversed. Critics say if computers do eventually become in charge of making all important decisions there will be little left for people to do as they will be demoted to simply observing the decisions made by computers, the same way as being a passenger in a car driven by a computer, not allowed to take control out of the fear of causing an accident. As mentioned before, this could lead to humans eventually becoming computers’ pets.The pragmatists: At present the vast majority of views about the future implications of AI are negative, concerned with its potential dystopian consequences (Elon Musk, the CEO of Tesla, says it is like “summoning the demon” and calls the consequences worse than what nuclear weapons can do). There are fewer optimists and only a couple of pragmatists like Sam Altman and Michio Kaku (Peckham, 2016) who believe that AI technologies can be controlled through “OpenAI” and effective regulation. The ranks of pragmatists also includes John Markoff (Markoff, 2016) who pointed out that the AI field can be distinguished by two categories: The first trying to duplicate human intelligence and the second to augment it by expanding human abilities exploiting the power of computers in order to augment human decision making. Pragmatists mention chess playing where the present world champion is neither a human nor a computer but rather humans using laptop computers (Baraniuk, 2015). Their view is that we could learn to exploit the power of computers to augment our own skills and always stay a step ahead of AI, or at least not be at a disadvantage. The pragmatists also believe that in the worst of cases a chip can be placed in all thinking machines/robots to render them inoperative in case of any danger. By concentrating research efforts on intelligence augmentation, they claim we can avoid or minimize the possible danger of AI while providing the means to stay ahead in the race against thinking machines and smart robots.The doubters: The doubters do not believe that AI is possible and that it will ever become a threat to humanity. Dreyfus (1972), its major proponent, argues that human intelligence and expertise cannot be replicated and captured in formal rules. He believes that AI is a fad promoted by the computer industry. He points out to the many predictions that did not materialize such as those made by Herbert A. Simon in 1958 that “a computer would be the world's chess champion within ten years” and those made in 1965 that “machines will be capable within twenty years, of doing any work a man can do” (Crevier, 1993). Dreyfus claims that Simon's optimism was totally unwarranted as they were based on false assumptions that human intelligence is based on an information processing viewpoint as our mind is nothing like a computer. Although, the doubters’ criticisms may have been valid in the last century, they cannot stand for the new developments in AI. Deep Blue became the world's chess champion in 1997 (missing Simon's forecast by twenty one years) while we are not far today from machines being capable of doing all the work that humans can do (missing。
人工智能(AI)中英文术语对照表
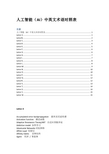
人工智能(AI)中英文术语对照表目录人工智能(AI)中英文术语对照表 (1)Letter A (1)Letter B (2)Letter C (3)Letter D (4)Letter E (5)Letter F (6)Letter G (6)Letter H (7)Letter I (7)Letter K (8)Letter L (8)Letter M (9)Letter N (10)Letter O (10)Letter P (11)Letter Q (12)Letter R (12)Letter S (13)Letter T (14)Letter U (14)Letter V (15)Letter W (15)Letter AAccumulated error backpropagation 累积误差逆传播Activation Function 激活函数Adaptive Resonance Theory/ART 自适应谐振理论Addictive model 加性学习Adversarial Networks 对抗网络Affine Layer 仿射层Affinity matrix 亲和矩阵Agent 代理/ 智能体Algorithm 算法Alpha-beta pruning α-β剪枝Anomaly detection 异常检测Approximation 近似Area Under ROC Curve/AUC Roc 曲线下面积Artificial General Intelligence/AGI 通用人工智能Artificial Intelligence/AI 人工智能Association analysis 关联分析Attention mechanism注意力机制Attribute conditional independence assumption 属性条件独立性假设Attribute space 属性空间Attribute value 属性值Autoencoder 自编码器Automatic speech recognition 自动语音识别Automatic summarization自动摘要Average gradient 平均梯度Average-Pooling 平均池化Action 动作AI language 人工智能语言AND node 与节点AND/OR graph 与或图AND/OR tree 与或树Answer statement 回答语句Artificial intelligence,AI 人工智能Automatic theorem proving自动定理证明Letter BBreak-Event Point/BEP 平衡点Backpropagation Through Time 通过时间的反向传播Backpropagation/BP 反向传播Base learner 基学习器Base learning algorithm 基学习算法Batch Normalization/BN 批量归一化Bayes decision rule 贝叶斯判定准则Bayes Model Averaging/BMA 贝叶斯模型平均Bayes optimal classifier 贝叶斯最优分类器Bayesian decision theory 贝叶斯决策论Bayesian network 贝叶斯网络Between-class scatter matrix 类间散度矩阵Bias 偏置/ 偏差Bias-variance decomposition 偏差-方差分解Bias-Variance Dilemma 偏差–方差困境Bi-directional Long-Short Term Memory/Bi-LSTM 双向长短期记忆Binary classification 二分类Binomial test 二项检验Bi-partition 二分法Boltzmann machine 玻尔兹曼机Bootstrap sampling 自助采样法/可重复采样/有放回采样Bootstrapping 自助法Letter CCalibration 校准Cascade-Correlation 级联相关Categorical attribute 离散属性Class-conditional probability 类条件概率Classification and regression tree/CART 分类与回归树Classifier 分类器Class-imbalance 类别不平衡Closed -form 闭式Cluster 簇/类/集群Cluster analysis 聚类分析Clustering 聚类Clustering ensemble 聚类集成Co-adapting 共适应Coding matrix 编码矩阵COLT 国际学习理论会议Committee-based learning 基于委员会的学习Competitive learning 竞争型学习Component learner 组件学习器Comprehensibility 可解释性Computation Cost 计算成本Computational Linguistics 计算语言学Computer vision 计算机视觉Concept drift 概念漂移Concept Learning System /CLS概念学习系统Conditional entropy 条件熵Conditional mutual information 条件互信息Conditional Probability Table/CPT 条件概率表Conditional random field/CRF 条件随机场Conditional risk 条件风险Confidence 置信度Confusion matrix 混淆矩阵Connection weight 连接权Connectionism 连结主义Consistency 一致性/相合性Contingency table 列联表Continuous attribute 连续属性Convergence收敛Conversational agent 会话智能体Convex quadratic programming 凸二次规划Convexity 凸性Convolutional neural network/CNN 卷积神经网络Co-occurrence 同现Correlation coefficient 相关系数Cosine similarity 余弦相似度Cost curve 成本曲线Cost Function 成本函数Cost matrix 成本矩阵Cost-sensitive 成本敏感Cross entropy 交叉熵Cross validation 交叉验证Crowdsourcing 众包Curse of dimensionality 维数灾难Cut point 截断点Cutting plane algorithm 割平面法Letter DData mining 数据挖掘Data set 数据集Decision Boundary 决策边界Decision stump 决策树桩Decision tree 决策树/判定树Deduction 演绎Deep Belief Network 深度信念网络Deep Convolutional Generative Adversarial Network/DCGAN 深度卷积生成对抗网络Deep learning 深度学习Deep neural network/DNN 深度神经网络Deep Q-Learning 深度Q 学习Deep Q-Network 深度Q 网络Density estimation 密度估计Density-based clustering 密度聚类Differentiable neural computer 可微分神经计算机Dimensionality reduction algorithm 降维算法Directed edge 有向边Disagreement measure 不合度量Discriminative model 判别模型Discriminator 判别器Distance measure 距离度量Distance metric learning 距离度量学习Distribution 分布Divergence 散度Diversity measure 多样性度量/差异性度量Domain adaption 领域自适应Downsampling 下采样D-separation (Directed separation)有向分离Dual problem 对偶问题Dummy node 哑结点Dynamic Fusion 动态融合Dynamic programming 动态规划Letter EEigenvalue decomposition 特征值分解Embedding 嵌入Emotional analysis 情绪分析Empirical conditional entropy 经验条件熵Empirical entropy 经验熵Empirical error 经验误差Empirical risk 经验风险End-to-End 端到端Energy-based model 基于能量的模型Ensemble learning 集成学习Ensemble pruning 集成修剪Error Correcting Output Codes/ECOC 纠错输出码Error rate 错误率Error-ambiguity decomposition 误差-分歧分解Euclidean distance 欧氏距离Evolutionary computation 演化计算Expectation-Maximization 期望最大化Expected loss 期望损失Exploding Gradient Problem 梯度爆炸问题Exponential loss function 指数损失函数Extreme Learning Machine/ELM 超限学习机Letter FExpert system 专家系统Factorization因子分解False negative 假负类False positive 假正类False Positive Rate/FPR 假正例率Feature engineering 特征工程Feature selection特征选择Feature vector 特征向量Featured Learning 特征学习Feedforward Neural Networks/FNN 前馈神经网络Fine-tuning 微调Flipping output 翻转法Fluctuation 震荡Forward stagewise algorithm 前向分步算法Frequentist 频率主义学派Full-rank matrix 满秩矩阵Functional neuron 功能神经元Letter GGain ratio 增益率Game theory 博弈论Gaussian kernel function 高斯核函数Gaussian Mixture Model 高斯混合模型General Problem Solving 通用问题求解Generalization 泛化Generalization error 泛化误差Generalization error bound 泛化误差上界Generalized Lagrange function 广义拉格朗日函数Generalized linear model 广义线性模型Generalized Rayleigh quotient 广义瑞利商Generative Adversarial Networks/GAN 生成对抗网络Generative Model 生成模型Generator 生成器Genetic Algorithm/GA 遗传算法Gibbs sampling 吉布斯采样Gini index 基尼指数Global minimum 全局最小Global Optimization 全局优化Gradient boosting 梯度提升Gradient Descent 梯度下降Graph theory 图论Ground-truth 真相/真实Letter HHard margin 硬间隔Hard voting 硬投票Harmonic mean 调和平均Hesse matrix海塞矩阵Hidden dynamic model 隐动态模型Hidden layer 隐藏层Hidden Markov Model/HMM 隐马尔可夫模型Hierarchical clustering 层次聚类Hilbert space 希尔伯特空间Hinge loss function 合页损失函数Hold-out 留出法Homogeneous 同质Hybrid computing 混合计算Hyperparameter 超参数Hypothesis 假设Hypothesis test 假设验证Letter IICML 国际机器学习会议Improved iterative scaling/IIS 改进的迭代尺度法Incremental learning 增量学习Independent and identically distributed/i.i.d. 独立同分布Independent Component Analysis/ICA 独立成分分析Indicator function 指示函数Individual learner 个体学习器Induction 归纳Inductive bias 归纳偏好Inductive learning 归纳学习Inductive Logic Programming/ILP 归纳逻辑程序设计Information entropy 信息熵Information gain 信息增益Input layer 输入层Insensitive loss 不敏感损失Inter-cluster similarity 簇间相似度International Conference for Machine Learning/ICML 国际机器学习大会Intra-cluster similarity 簇内相似度Intrinsic value 固有值Isometric Mapping/Isomap 等度量映射Isotonic regression 等分回归Iterative Dichotomiser 迭代二分器Letter KKernel method 核方法Kernel trick 核技巧Kernelized Linear Discriminant Analysis/KLDA 核线性判别分析K-fold cross validation k 折交叉验证/k 倍交叉验证K-Means Clustering K –均值聚类K-Nearest Neighbours Algorithm/KNN K近邻算法Knowledge base 知识库Knowledge Representation 知识表征Letter LLabel space 标记空间Lagrange duality 拉格朗日对偶性Lagrange multiplier 拉格朗日乘子Laplace smoothing 拉普拉斯平滑Laplacian correction 拉普拉斯修正Latent Dirichlet Allocation 隐狄利克雷分布Latent semantic analysis 潜在语义分析Latent variable 隐变量Lazy learning 懒惰学习Learner 学习器Learning by analogy 类比学习Learning rate 学习率Learning Vector Quantization/LVQ 学习向量量化Least squares regression tree 最小二乘回归树Leave-One-Out/LOO 留一法linear chain conditional random field 线性链条件随机场Linear Discriminant Analysis/LDA 线性判别分析Linear model 线性模型Linear Regression 线性回归Link function 联系函数Local Markov property 局部马尔可夫性Local minimum 局部最小Log likelihood 对数似然Log odds/logit 对数几率Logistic Regression Logistic 回归Log-likelihood 对数似然Log-linear regression 对数线性回归Long-Short Term Memory/LSTM 长短期记忆Loss function 损失函数Letter MMachine translation/MT 机器翻译Macron-P 宏查准率Macron-R 宏查全率Majority voting 绝对多数投票法Manifold assumption 流形假设Manifold learning 流形学习Margin theory 间隔理论Marginal distribution 边际分布Marginal independence 边际独立性Marginalization 边际化Markov Chain Monte Carlo/MCMC马尔可夫链蒙特卡罗方法Markov Random Field 马尔可夫随机场Maximal clique 最大团Maximum Likelihood Estimation/MLE 极大似然估计/极大似然法Maximum margin 最大间隔Maximum weighted spanning tree 最大带权生成树Max-Pooling 最大池化Mean squared error 均方误差Meta-learner 元学习器Metric learning 度量学习Micro-P 微查准率Micro-R 微查全率Minimal Description Length/MDL 最小描述长度Minimax game 极小极大博弈Misclassification cost 误分类成本Mixture of experts 混合专家Momentum 动量Moral graph 道德图/端正图Multi-class classification 多分类Multi-document summarization 多文档摘要Multi-layer feedforward neural networks 多层前馈神经网络Multilayer Perceptron/MLP 多层感知器Multimodal learning 多模态学习Multiple Dimensional Scaling 多维缩放Multiple linear regression 多元线性回归Multi-response Linear Regression /MLR 多响应线性回归Mutual information 互信息Letter NNaive bayes 朴素贝叶斯Naive Bayes Classifier 朴素贝叶斯分类器Named entity recognition 命名实体识别Nash equilibrium 纳什均衡Natural language generation/NLG 自然语言生成Natural language processing 自然语言处理Negative class 负类Negative correlation 负相关法Negative Log Likelihood 负对数似然Neighbourhood Component Analysis/NCA 近邻成分分析Neural Machine Translation 神经机器翻译Neural Turing Machine 神经图灵机Newton method 牛顿法NIPS 国际神经信息处理系统会议No Free Lunch Theorem/NFL 没有免费的午餐定理Noise-contrastive estimation 噪音对比估计Nominal attribute 列名属性Non-convex optimization 非凸优化Nonlinear model 非线性模型Non-metric distance 非度量距离Non-negative matrix factorization 非负矩阵分解Non-ordinal attribute 无序属性Non-Saturating Game 非饱和博弈Norm 范数Normalization 归一化Nuclear norm 核范数Numerical attribute 数值属性Letter OObjective function 目标函数Oblique decision tree 斜决策树Occam’s razor 奥卡姆剃刀Odds 几率Off-Policy 离策略One shot learning 一次性学习One-Dependent Estimator/ODE 独依赖估计On-Policy 在策略Ordinal attribute 有序属性Out-of-bag estimate 包外估计Output layer 输出层Output smearing 输出调制法Overfitting 过拟合/过配Oversampling 过采样Letter PPaired t-test 成对t 检验Pairwise 成对型Pairwise Markov property成对马尔可夫性Parameter 参数Parameter estimation 参数估计Parameter tuning 调参Parse tree 解析树Particle Swarm Optimization/PSO粒子群优化算法Part-of-speech tagging 词性标注Perceptron 感知机Performance measure 性能度量Plug and Play Generative Network 即插即用生成网络Plurality voting 相对多数投票法Polarity detection 极性检测Polynomial kernel function 多项式核函数Pooling 池化Positive class 正类Positive definite matrix 正定矩阵Post-hoc test 后续检验Post-pruning 后剪枝potential function 势函数Precision 查准率/准确率Prepruning 预剪枝Principal component analysis/PCA 主成分分析Principle of multiple explanations 多释原则Prior 先验Probability Graphical Model 概率图模型Proximal Gradient Descent/PGD 近端梯度下降Pruning 剪枝Pseudo-label伪标记Letter QQuantized Neural Network 量子化神经网络Quantum computer 量子计算机Quantum Computing 量子计算Quasi Newton method 拟牛顿法Letter RRadial Basis Function/RBF 径向基函数Random Forest Algorithm 随机森林算法Random walk 随机漫步Recall 查全率/召回率Receiver Operating Characteristic/ROC 受试者工作特征Rectified Linear Unit/ReLU 线性修正单元Recurrent Neural Network 循环神经网络Recursive neural network 递归神经网络Reference model 参考模型Regression 回归Regularization 正则化Reinforcement learning/RL 强化学习Representation learning 表征学习Representer theorem 表示定理reproducing kernel Hilbert space/RKHS 再生核希尔伯特空间Re-sampling 重采样法Rescaling 再缩放Residual Mapping 残差映射Residual Network 残差网络Restricted Boltzmann Machine/RBM 受限玻尔兹曼机Restricted Isometry Property/RIP 限定等距性Re-weighting 重赋权法Robustness 稳健性/鲁棒性Root node 根结点Rule Engine 规则引擎Rule learning 规则学习Letter SSaddle point 鞍点Sample space 样本空间Sampling 采样Score function 评分函数Self-Driving 自动驾驶Self-Organizing Map/SOM 自组织映射Semi-naive Bayes classifiers 半朴素贝叶斯分类器Semi-Supervised Learning半监督学习semi-Supervised Support Vector Machine 半监督支持向量机Sentiment analysis 情感分析Separating hyperplane 分离超平面Searching algorithm 搜索算法Sigmoid function Sigmoid 函数Similarity measure 相似度度量Simulated annealing 模拟退火Simultaneous localization and mapping同步定位与地图构建Singular Value Decomposition 奇异值分解Slack variables 松弛变量Smoothing 平滑Soft margin 软间隔Soft margin maximization 软间隔最大化Soft voting 软投票Sparse representation 稀疏表征Sparsity 稀疏性Specialization 特化Spectral Clustering 谱聚类Speech Recognition 语音识别Splitting variable 切分变量Squashing function 挤压函数Stability-plasticity dilemma 可塑性-稳定性困境Statistical learning 统计学习Status feature function 状态特征函Stochastic gradient descent 随机梯度下降Stratified sampling 分层采样Structural risk 结构风险Structural risk minimization/SRM 结构风险最小化Subspace 子空间Supervised learning 监督学习/有导师学习support vector expansion 支持向量展式Support Vector Machine/SVM 支持向量机Surrogat loss 替代损失Surrogate function 替代函数Symbolic learning 符号学习Symbolism 符号主义Synset 同义词集Letter TT-Distribution Stochastic Neighbour Embedding/t-SNE T –分布随机近邻嵌入Tensor 张量Tensor Processing Units/TPU 张量处理单元The least square method 最小二乘法Threshold 阈值Threshold logic unit 阈值逻辑单元Threshold-moving 阈值移动Time Step 时间步骤Tokenization 标记化Training error 训练误差Training instance 训练示例/训练例Transductive learning 直推学习Transfer learning 迁移学习Treebank 树库Tria-by-error 试错法True negative 真负类True positive 真正类True Positive Rate/TPR 真正例率Turing Machine 图灵机Twice-learning 二次学习Letter UUnderfitting 欠拟合/欠配Undersampling 欠采样Understandability 可理解性Unequal cost 非均等代价Unit-step function 单位阶跃函数Univariate decision tree 单变量决策树Unsupervised learning 无监督学习/无导师学习Unsupervised layer-wise training 无监督逐层训练Upsampling 上采样Letter VVanishing Gradient Problem 梯度消失问题Variational inference 变分推断VC Theory VC维理论Version space 版本空间Viterbi algorithm 维特比算法Von Neumann architecture 冯·诺伊曼架构Letter WWasserstein GAN/WGAN Wasserstein生成对抗网络Weak learner 弱学习器Weight 权重Weight sharing 权共享Weighted voting 加权投票法Within-class scatter matrix 类内散度矩阵Word embedding 词嵌入Word sense disambiguation 词义消歧。
AI中英文对照使用技巧
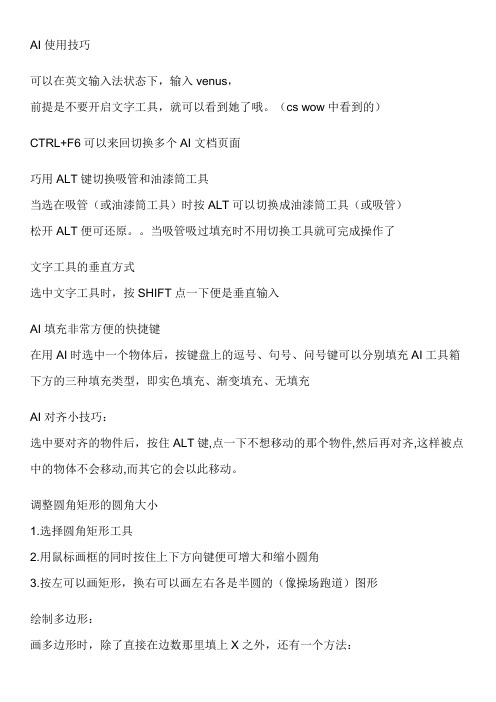
AI使用技巧可以在英文输入法状态下,输入venus,前提是不要开启文字工具,就可以看到她了哦。
(cs wow中看到的)CTRL+F6可以来回切换多个AI文档页面巧用ALT键切换吸管和油漆筒工具当选在吸管(或油漆筒工具)时按ALT可以切换成油漆筒工具(或吸管)松开ALT便可还原。
当吸管吸过填充时不用切换工具就可完成操作了文字工具的垂直方式选中文字工具时,按SHIFT点一下便是垂直输入AI填充非常方便的快捷键在用AI时选中一个物体后,按键盘上的逗号、句号、问号键可以分别填充AI工具箱下方的三种填充类型,即实色填充、渐变填充、无填充AI对齐小技巧:选中要对齐的物件后,按住ALT键,点一下不想移动的那个物件,然后再对齐,这样被点中的物体不会移动,而其它的会以此移动。
调整圆角矩形的圆角大小1.选择圆角矩形工具2.用鼠标画框的同时按住上下方向键便可增大和缩小圆角3.按左可以画矩形,换右可以画左右各是半圆的(像操场跑道)图形绘制多边形:画多边形时,除了直接在边数那里填上X之外,还有一个方法:就是按住多边形工具,且不释放鼠标。
然后按方向键的向上或向下,可以增加或减少边数。
上下键是AI里的一个特殊功能,用处很多的,需要随处留心就会发现,画表格可以增减,圆角可改变大小。
在AI里未转曲线时文字做渐变的方法:在AI 中未转成曲线的文字是无法使用渐变填充的,使用以下方法就可以了,还有其它的方法大家可以告诉我.1.首先打上你要的字2.把字应用一下图形样式里中的默认样式,要记得是图形样式里的第一个样式默认,而不是其它样式3.,然后把字体里的描边再变成无,在这个基础上就可以应用渐变了,还能编辑字体.选择要填充成渐变的文字,然后应用刚才定义的图形样式。
还有一个方法,就是用字符的外观也可以填充渐变,方法是:打上字,然后在字的外观面板上点上面的三角下拉菜单,选择添加新填充,然后在面板上双击新填充,就会出来填充面板,就可以应用渐变了,这种方法比较可靠。
- 1、下载文档前请自行甄别文档内容的完整性,平台不提供额外的编辑、内容补充、找答案等附加服务。
- 2、"仅部分预览"的文档,不可在线预览部分如存在完整性等问题,可反馈申请退款(可完整预览的文档不适用该条件!)。
- 3、如文档侵犯您的权益,请联系客服反馈,我们会尽快为您处理(人工客服工作时间:9:00-18:30)。
Rasterize光栅化
Stylize风格化
视图菜单-VIEW
Outline路径轮廓视图
Overprint preview印前视图模式
Pixel preview象素视图模式
Proof setup校验设置
Zoom in放大
Zoom out缩小
Fit in window适合窗口
Show/hide transparency grid显示/隐藏透明网格
Guides参考线
Smart guides实时参考线
Show/hide grid显示/隐藏网格
Snip to grid对齐网格
Snip to point定点对齐
New view新查看方式
Edit views编辑查看方式
Saturation饱和度
Crop marks 剪切标记
Graph 图表
Join 连接
Averange 平均节点位置
Outline Path 轮廓路径
Offset Path 路径位移
Clear Up 清除
Slice 裁切
Add Anchor Point 增加节点
Make 制造
Release 释放
Blend option 混合操作
Document color made文件色彩模式
Separation setup分色设置
Print setup打印设置
Print打印
编辑菜单-Edit
Undo撤消
Redo重做
Cut剪切
Pasnt粘贴到最前面
Paste to back粘贴到最后面
Clear清除
Select选择
Select all选择全部
Deselect all取消选择全部
Define pattern定义图案
Edit original编辑原稿
Assign profile重指定纲要
Color setting色彩模式设置
Keyboard shortcuts编辑快捷键
Preferences参数设置
矢量滤镜
Apply Last Filter应用刚才的滤镜
Last Filter刚才的滤镜
Color色彩
Create创建
Distort扭曲
Pen and Ink墨水笔
位图滤镜
Artistic艺术化
Brush Strokes笔刷化
Distort扭曲
Sketch素描效果
Stylize风格化
Texture纹理化
Plus ins&Scrach Disk插件和虚拟硬盘
Cursor key箭头键
Constrain Angle角度限制
Corner Radius圆角限制
对象菜单-Object
Transform 变形
Arrange 排列
Group 群组
Ungroup 取消群组
Lock 锁定
Unlock 取消锁定
Hide Selection 隐藏选择对象
Sketch素描效果
Stylize风格化
Texture纹理化
Adjust Color颜色调整
Blend Front to Back混合前后图形的颜色
Blend Vertically混合垂直放置图形的颜色
Convert to CMYK转换为CMYK
Convert to Grayscale转换为灰度
Convert to RGB转换为RGB
Find fond选择字体
Check Spelling拼写检查
Change Case改变文字容器大小写
Smart Punctuation快速标点
Rows&Columns文字分行分栏
Show Hidden Characters显示隐藏属性
Type Orientation文字方向
Swrap
滤镜菜单-FILTER
Piselate象素化
Sharpen锐化
Sketch素描效果
Stylize风格化
Texture纹理化
Video视频
特效菜单-EFFECT
Apply last effect重复刚才的特效
Last effect刚才的特效
Convert to shape转换形状
Distort transform自由变换
Path路径特效
Invert Colors反色
Overprint Black黑色压印
文字菜单-TYPE
Font字体
Size尺寸
Character文字属性
Paragraph段落
Mmdesign Typel字体属性
Tob Ruler表格定位标尺
Block文字块
Wrap文字绕排
Fit headline适合标题
Greate Outlines创建文字轮廓路径
Swrap
滤镜菜单-FILTER
矢量滤镜
Apply Last Filter应用刚才的滤镜
Last Filter刚才的滤镜
Color色彩
Create创建
Distort扭曲
Pen and Ink墨水笔
Stylize风格化
Distort变形
Artistic艺术化
Blur模糊化
Brush Strokes笔痕
Expend 扩展
Replace Spine 路径混合
Revers Spine 反转混合
Reverse Front To Back 反转混合方向
Type 类型
Data 数据
Design 设计
Column 柱形
Stylize风格化
位图滤镜
Artistic艺术化
Brush Strokes笔刷化
Distort扭曲
Find/Change查找/替换
Find fond选择字体
Check Spelling拼写检查
Change Case改变文字容器大小写
Smart Punctuation快速标点
Rows&Columns文字分行分栏
Show Hidden Characters显示隐藏属性
Type Orientation文字方向
Overprint Black黑色压印
Show/hide edges显示/隐藏选中路径
Show/hide page tiling显示/隐藏工作区标志
Show/hide template显示/隐藏模板
Show/hide rules显示/隐藏标尺
Show/hide bounding box显示/隐藏限制框
文件菜单-File
New新建
Open打开
Open recent files打开最近编辑的文件
Revert复原
Close关闭
Save保存
Save as另存为
Save a copy保存一个拷贝
Save for web保存为应用于web
Place置入
Export输出
Document info文件信息
Document setup文件设置
Adjust Color颜色调整
Blend Front to Back混合前后图形的颜色
Blend Vertically混合垂直放置图形的颜色
Convert to CMYK转换为CMYK
Convert to Grayscale转换为灰度
Convert to RGB转换为RGB
Invert Colors反色
Same paint style相同的笔样式
Same stroke color相同的边线颜色
Same stroke weight相同的边线宽度
Masks遮罩
Stay piont游离点
Brush stroke画笔笔触
Inverse相反
Hyphenator option连字符操作
Marker 标记
Transform Again 再次变形
Show All 显示所有对象
Expend 扩展
Expend Appearance 扩展轮廓
Flatten transparently 平整透明
Rasterize 栅格化
Greate Gradient Mesh 创建网格渐变对象
Path 路径
Blend 混合
Clipping masks 剪切遮罩
Comound path 复合路径
Actual size实际尺寸
Move 移动
Scale 缩放
Rotate 旋转
Shear 倾斜
Reflect 镜像
Transform each 单独变形
Reset Bounding 重设调节框
Bring To Front 放在最前层
Bring Forward 放在前一层
Send Backward 放在后一层
Sent to Back 放在最后
Create建立
Object Mosaic马赛克效果
Trim Mark裁剪标记
Distort变形
Punk&Bloat尖角和圆角变形
Boughen粗糙化
Scribble And Tweak潦草和扭曲
Twirl涡形旋转
ZigZag文字效果
Add Arrowhead加箭头
Drop shadow加阴影
Round corner圆角化
Rasterize 光栅化
文字菜单-TYPE
Font字体
Size尺寸
Character文字属性
Paragraph段落
Mmdesign Typel字体属性
Tob Ruler表格定位标尺
Block文字块
Wrap文字绕排
Fit headline适合标题