GIS专业英语原文及翻译结果
测绘专业英语原文和部分翻译(1-39)
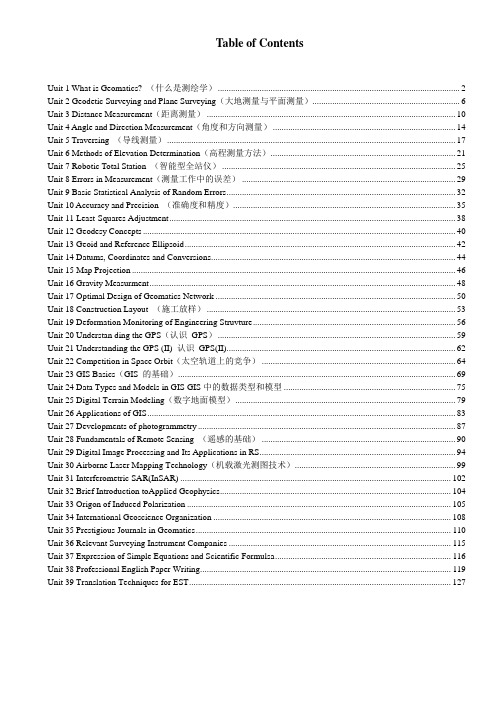
Table of ContentsUuit 1 What is Geomatics? (什么是测绘学) (2)Unit 2 Geodetic Surveying and Plane Surveying(大地测量与平面测量) (6)Unit 3 Distance Measurement(距离测量) (10)Unit 4 Angle and Direction Measurement(角度和方向测量) (14)Unit 5 Traversing (导线测量) (17)Unit 6 Methods of Elevation Determination(高程测量方法) (21)Unit 7 Robotic Total Station (智能型全站仪) (25)Unit 8 Errors in Measurement(测量工作中的误差) (29)Unit 9 Basic Statistical Analysis of Random Errors (32)Unit 10 Accuracy and Precision (准确度和精度) (35)Unit 11 Least-Squares Adjustment (38)Unit 12 Geodesy Concepts (40)Unit 13 Geoid and Reference Ellipsoid (42)Unit 14 Datums, Coordinates and Conversions (44)Unit 15 Map Projection (46)Unit 16 Gravity Measurment (48)Unit 17 Optimal Design of Geomatics Network (50)Unit 18 Construction Layout (施工放样) (53)Unit 19 Deformation Monitoring of Engineering Struvture (56)Unit 20 Understan ding the GPS(认识GPS) (59)Uuit 21 Understanding the GPS (II) 认识GPS(II) (62)Unit 22 Competition in Space Orbit(太空轨道上的竞争) (64)Unit 23 GIS Basics(GIS 的基础) (69)Unit 24 Data Types and Models in GIS GIS中的数据类型和模型 (75)Unit 25 Digital Terrain Modeling(数字地面模型) (79)Unit 26 Applications of GIS (83)Unit 27 Developments of photogrammetry (87)Unit 28 Fundamentals of Remote Sensing (遥感的基础) (90)Unit 29 Digital Image Processing and Its Applications in RS (94)Unit 30 Airborne Laser Mapping Technology(机载激光测图技术) (99)Unit 31 Interferometric SAR(InSAR) (102)Unit 32 Brief Introduction toApplied Geophysics (104)Unit 33 Origon of Induced Polarization (105)Unit 34 International Geoscience Organization (108)Unit 35 Prestigious Journals in Geomatics (110)Unit 36 Relevant Surveying Instrument Companies (115)Unit 37 Expression of Simple Equations and Scientific Formulsa (116)Unit 38 Professional English Paper Writing (119)Unit 39 Translation Techniques for EST (127)Uuit 1 What is Geomatics? (什么是测绘学)Geomatics Defined(测绘学定义)Where does the word Geomatics come from?(Geomatics-测绘或地球空间信息学,这个名词是怎么来的呢?)GEODESY+GEOINFORMATICS=GEOMA TICS or GEO- for earth and –MATICS for mathematical or GEO- for Geoscience and -MATICS for informatics. (大地测量学+地理信息学=GEOMATICS 测绘学或者geo 代表地球,matics 代表数学,或者geo 代表地球科学,matics 代表信息学)It has been said that geomatics is many things to many people.(据说测绘学这个词对不同的人有不同的理解)The term geomatics emerged first in Canada and as an academic discipline; it has been introduced worldwide in a number of institutes of higher education during the past few years, mostly by renaming what was previously called “geodesy” or “surveying”, and by adding a number of computer scienceand/or GIS-oriented courses.(这个术语【term 术语】作为一个学科【academic discipline 学科】第一次形成【emerge】于加拿大;在过去的几年里被全世界的许多高等教育研究机构所熟知,通常是以前的“大地测量学” 或“测量学”在引入了许多计算机科学和GIS 方向【或“基于GIS” 】的课程后重新命名的。
专业英语 gis 遥感 摄影测量
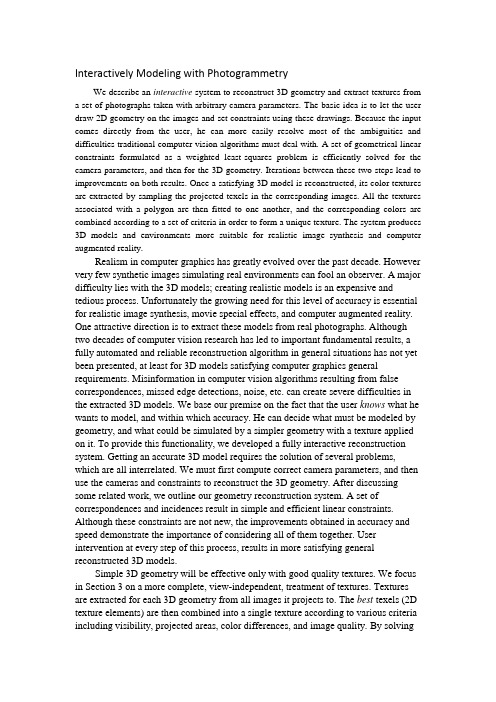
Interactively Modeling with PhotogrammetryWe describe an interactive system to reconstruct 3D geometry and extract textures from a set of photographs taken with arbitrary camera parameters. The basic idea is to let the user draw 2D geometry on the images and set constraints using these drawings. Because the input comes directly from the user, he can more easily resolve most of the ambiguities and difficulties traditional computer vision algorithms must deal with. A set of geometrical linear constraints formulated as a weighted least-squares problem is efficiently solved for the camera parameters, and then for the 3D geometry. Iterations between these two steps lead to improvements on both results. Once a satisfying 3D model is reconstructed, its color textures are extracted by sampling the projected texels in the corresponding images. All the textures associated with a polygon are then fitted to one another, and the corresponding colors are combined according to a set of criteria in order to form a unique texture. The system produces 3D models and environments more suitable for realistic image synthesis and computer augmented reality.Realism in computer graphics has greatly evolved over the past decade. However very few synthetic images simulating real environments can fool an observer. A major difficulty lies with the 3D models; creating realistic models is an expensive and tedious process. Unfortunately the growing need for this level of accuracy is essential for realistic image synthesis, movie special effects, and computer augmented reality. One attractive direction is to extract these models from real photographs. Although two decades of computer vision research has led to important fundamental results, a fully automated and reliable reconstruction algorithm in general situations has not yet been presented, at least for 3D models satisfying computer graphics general requirements. Misinformation in computer vision algorithms resulting from false correspondences, missed edge detections, noise, etc. can create severe difficulties in the extracted 3D models. We base our premise on the fact that the user knows what he wants to model, and within which accuracy. He can decide what must be modeled by geometry, and what could be simulated by a simpler geometry with a texture applied on it. To provide this functionality, we developed a fully interactive reconstruction system. Getting an accurate 3D model requires the solution of several problems, which are all interrelated. We must first compute correct camera parameters, and then use the cameras and constraints to reconstruct the 3D geometry. After discussing some related work, we outline our geometry reconstruction system. A set of correspondences and incidences result in simple and efficient linear constraints. Although these constraints are not new, the improvements obtained in accuracy and speed demonstrate the importance of considering all of them together. User intervention at every step of this process, results in more satisfying general reconstructed 3D models.Simple 3D geometry will be effective only with good quality textures. We focus in Section 3 on a more complete, view-independent, treatment of textures. Textures are extracted for each 3D geometry from all images it projects to. The best texels (2D texture elements) are then combined into a single texture according to various criteria including visibility, projected areas, color differences, and image quality. By solvingaccurately each problem, we will better understand the robustness, stability, and precision of our techniques. It should become easier later on to extend our interactions with more automatic computer vision and image processing techniques in order to alleviate some of the more cumbersome and tedious tasks, while keeping user intervention where required. The results of our system should help us create more precise textured synthetic models from real 3D objects in less time than current 3D modelers, and more robustly than fully automated geometry extraction algorithms. Twenty years of active research on 3D reconstruction from 2D images in computer vision and robotics have left a considerable legacy of important results. The first problem to address concerns camera calibration, i.e. computing camera parameters. This is a difficult and unstable process often improved by the use of specific targets. By putting in correspondence points or lines between images, it becomes possible to calibrate cameras. Similarly with known camera parameters, one can reconstruct a 3D scene up to a scale factor. In these classical approaches, segmentations and correspondences are automatically determined. One typical example of the results obtained by these approaches was recently presented by Sato et al.. A few recent projects such as REALISE, Fac¸ade, PhotoModeler and AIDA propose to integrate more user intervention into the reconstruction process.They are derived from projective geometry, and are applied to the reconstruction of man-made scenes from a set of photographs and correspondences. REALISE integrates user intervention early in the correspondence process, letting the user specify the first correspondences, and then returning to a more classical approach to identify automatically most of the other correspondences. The more stable initial solution greatly helps to reduce the errors of subsequent iterations. Nevertheless the same errors of fully automatic systems can still occur, and the user must then detect and correct the origin of the errors, which is not a simple task as the number of automatic correspondences increases. Fac¸ade develops a series of parameterized block primitives. Each block encodes efficiently and hierarchically several constraints frequently present in architectural design. The user must first place the blocks with a 3D modeler, and then set correspondences between the images and these blocks.Non-linear optimization of an objective function is then used to solve for all these constraints. The system has proven to be quite efficient and provides precise 3D models with little effort. However it requires the user to build with the blocks the model he wants to reconstruct. We believe this might be more difficult when general 3D models cannot be as easily created with these blocks. PhotoModeler is a commercial software for performing photogrammetric measurements on models built from photographs. Once the camera is calibrated, the user has to indicate features and correspondences on the images, and the system computes the 3D scene. The models obtained appear quite good, although it seems to be a lengthy process (they reported a week for a model of 200 3D points) which uses images of very high resolution (around 15 MB each). We also noticed many long thin triangles and gaps in some of their models. The system can apply textures coming from the photographs but does not seem to perform any particular treatment since the shadows, highlights, etc. are still present. No details are provided on the algorithms used. The AIDA system is afully automatic reconstruction system that combines surface reconstruction techniques with object recognition for the generation of 3D models for computer graphics applications. The system possesses a knowledge database of constraints, and selects the constraints to apply to the surface under reconstruction after performing a scene interpretation phase. We believe it might be safer, less cumbersome, and more general to let the user choose which constraints he wants to apply to its 3D primitives rather than letting the system pick some constraints from a knowledge database created specifically for the type of scene to reconstruct.For these reasons, we introduce a system essentially based on user interaction. The user is responsible for (almost) everything, but also has the control on (almost) everything. This should provide a comprehensive tool to improve on the modeling from real 3D objects and on the computer graphics quality of these 3D models, while offering the opportunity to focus on the details important to the designer.System Overview. We have developed an interactive reconstruction system from images. The images define the canvas on which all interaction is based. They can come from any type of cameras (even a virtual synthetic camera) with any settings and position. The user draws points, lines, and polygons on the images which form our basic 2D primitives. The user interactively specifies correspondences between the 2D primitives on different images. He can also assign other constraints between reconstructed 3D primitives simply by clicking on one of their respective 2D primitives. These additional constraints include parallelism, perpendicularity, planarity, and co-planarity. At any time, the user can ask the system to reconstruct all computable cameras and 3D primitives. The reconstructed 3D primitives can be reprojected on the images to estimate the quality of each recovered camera and the 3D primitives. The user then has the choice to iterate a few times to improve on the mathematical solution, or to add new 2D primitives, correspondences, and constraints to refine the 3D models. This process, illustrated in Fig. 1, demonstrates the flexibility and power of our technique. The 3D model is reconstructed incrementally, refined where and when necessary. Each error from the user can also be immediately detected using reprojection. Contrarily to Debevec et al., the user does not create a synthetic model of the geometry he wants to recover, although the reconstructed 3D model can as easily be used to establish new constraints between 2D and reconstructed 3D primitives. Each image thus contains a set of 2D primitives drawn on it, and a camera computed when the set of resolved constraints is sufficient. To bootstrap the reconstruction process, the user assigns a sufficient number of 3D coordinates to 3D primitives via one of their corresponding 2D primitives. For instance, six 3D points in one image allow the computation of the corresponding camera. Once two cameras are computed, all 3D geometry that can be computed by resolving the constraints is reconstructed. With the assigned and the newly computed 3D values, the constraints are resolved again to improve the reconstructed cameras. This process iterates until no more constraints can be resolved, and the 3D geometry and cameras are computed to a satisfactory precision.Typically, a convergence iteration solving the equation systems for computing all the cameras and 3D positions takes between 0.05 and 2 seconds,1 depending on thecomplexity of the scene (50 to 200 3D points) and the constraints used. All our constraints are expressed as linear equations, typically forming an overdetermined set of equations. A least-squares solution to this system is computed by singular value decomposition. We use this solution for the unknown camera parameters, and the unknown 3D coordinates of points and lines. As an exact correspondence is hardly achievable by drawing 2D points on the image plane, we compute the best camera parameters in the least-squares sense. Hartley demonstrate simple conditions under which linear systems of equations used to determine the camera parameters are as precise as their non-linear counterparts. Moreover this technique is simple to implement, efficient, general, always provides a solution, and we observed that it is more robust than the non-linear systems.Additional 3D Constraints.Most man-made scenes exhibit some form of planarity, parallelism, perpendicularity, symmetry, etc. Using correspondences only, reconstructed geometry often does not respect these properties, which can lead to objectionable artifacts in the reconstructed 3D models. It is therefore very important to integrate this type of constraint in a reconstruction system. They are unfortunately difficult to detect automatically, as perspective projection does not preserve them in the image. The user can however very easily indicate each such constraint directly onto the images. They are integrated into our convergence process by adding equations to the system of linear equations used to compute 3D coordinates. Although they are not strictly enforced because they are simply part of a least-squares solution, they often result in more satisfying 3D models especially with respect to the needs of computer graphics models.Coplanarity: A planar polygon with more than three vertices should have all its vertices on the same plane A. For each polygon with 3D vertices Pi, we add a planarity constraint of the form A _ Pi = 0 that will be used during the computation of each Pi. Polygons, points, and lines can also be constrained to lie on the same supporting plane. To compute this plane A = [ a b c d ]T , the user can specify a normal direction N = [ a b c ]T . We compute the best value for d by using the known 3D points. If there is no information about the plane orientation, we compute the best plane in the least-squares sense, that passes through at least three known 3D points of the coplanar primitives, and apply the same constraint.Parallelism: Similarly, several polygons and lines can be parallel to each other, providing additional constraints. For each polygon, we get its orientation Ni from its plane equation, if available. These orientations allow us to calculate an average orientation Navg that will be attributed to all the parallel polygons, even those for which no orientation could be first calculated. A planarity constraint is added to the computation of the polygons 3D points. For parallel lines, we compute their average direction.Perpendicularity: If the normals Nperp to perpendicular polygons are known, we can add other constraints for the computation of Navg. Two perpendicular polygons have perpendicular normals, thus for every polygon orthogonal to a set of parallel polygons, we have Nperp _ Navg = 0. Many other constraints should be exploited. Symmetry couldconstrain characteristics such as lengths or angles. Similarity between models couldspecify two identical elements at different positions. Incidence of points and lines canbe extended to different primitives. These are only a few of the constraints we observein 3D scenes. Each basic constraint described above can be used as a building blockfor more elaborate primitives. A cube for instance becomes a set of planar faces, withperpendicularity and parallelism between its faces and segments, and constrainedlength between its 3D vertices. Rather than letting the user specify all theseconstraints, a new primitive for which all of these are already handled represents amuch more efficient tool for the user. These new primitives can be described in alibrary of primitives organized hierarchically. Debevec et al. shows how thisrepresentation can also reduce significantly the number of constraints to resolve. Wecan also weight the contributions of the constraints depending on their importance inthe current reconstruction. The default weights assigned to each type of constraint canbe edited by the user. The resolution of our equation systems is simply extended to aweighted least-squares.Results of Geometry ReconstructionTo evaluate the precision and the convergence of our iterative process, weconstructed a simple synthetic scene made of seven boxes. Five images of resolution 500 _ 400 where rendered from camera positions indicated by the grey cones in Fig. 3 (left). 2D polygons were manually drawn and put in correspondences within 60 minutes ona 195 MHz R10000 SGI Impact. The 3D coordinates of six points of the central cubeon the floor were entered to bootstrap the system. The three curves in Fig. 3 (right)represent the distance in world coordinates between the real 3D position of threepoints in the scene ((-2,3,0),(0,2,-2),(1,2,-2)) and their reconstructed correspondents.200 iterations without any constraints other than the point correspondences took about5 minutes. We then applied successively the constraints of planarity, coplanarity, andparallelism between all the 3D polygons. Calculating all these constraints typicallyadds a few tenths of a second per iteration depending on the complexity of the 3Dscene. The three curves reach a plateau after a certain number of iterations. This doesnot mean that the 3D model is then perfectly reconstructed, but rather that the solutionis stable and should not change significantly with more iterations. When we introducethe planarity and then the coplanarity constraints for individual polygons, the pointsmove slightly. In this scene where parallelism is preponderant, the addition of this lastconstraint improves significantly the reconstruction for all three points, which isshown by the drop of all three curves after iteration 300. The introduction of a newconstraint can sometimes perturb the whole system, affecting more theless-constrained elements as demonstrated by the sudden spike in curve 2. In mostobserved cases, the system quickly returns to an improved and more stable state. InFig. 3 (center), we reproject in wireframe mode the reconstructed model using thecomputed camera from one of the original images. Distances between the 2D drawnpoints and the reprojected reconstructed points all lie within less than one pixel fromeach other. When 3D constraints are used to improve the model, this distance canreach up to two pixels. The 3D scene then corresponds more to reality but does not fitexactly the drawn primitives when reprojected with the computed projection matrix.The constraints thus compensate for the inaccuracy introduced by the user interaction or by the primitives far from the entered coordinates. Because the user draws 2D primitives at the resolution of the image, getting a maximumof two pixels is considered satisfactory. Sub-pixels accuracy is obtained if these primitives are drawn at sub-pixel precision, but this lengthen the user interaction time.Extracting TextureTextures have been introduced in computer graphics to increase the realism of synthetic surfaces. They encode via a surface parameterization the color for each point on the surface. While the contribution of textures to realism is obvious, it is not always easy to extract a texture from real images. One must correct for perspective foreshortening, surface curvature, hidden portions of the texture, reflections, shading, etc. All these limitations have restricted the type of extracted real textures. However our reconstructed geometry and cameras provide a great context within which we can extract these textures. Most current approaches are based on view-dependent textures. Havaldar et al. use the projection of the 3D primitive in all the images to determine the best source image for the texture. Then we apply to the texture the 2D transformation from the projected polygon in this best image, to the projected polygon in the image from a new viewpoint. Unfortunately, this 2D deformation of the texture is invalid for a perspective projection, and prone to visibility errors. Debevec et al. reprojects each extracted texture for a given primitive as a weighted function based on the viewing angle of the new camera position. The technique provides better results with view-dependent information. However, neglecting the distance factor in the weights can introduce important errors, and aliasing can appear from the use of occlusion maps. Moreover all the textures must be kept in memory as potentially all of them might be reprojected for any new viewpoint. Niem and Broszio identifies the best image for an entire polygon (according to angle and distance criteria), and samples the texture from this image. Because adjacent polygons can have different best images, they then proceed to smooth out the adjacent texels, possibly altering the textures. In our system, a texture is extracted upon user request for a given primitive projecting in a number of images. The texture is the result of recombining the estimated best colors for each point of the surface projected in each image. Even though extracting a single texture is prone to errors as will be discussed later, it is more suitable for general image synthesis applications such as applying it to different primitives, filtering, and use of graphics hardware. For a given 3D point, its projection in one image will most likely be a visible point because the user drew its supporting 3D primitive as a corresponding 2D primitive on the image. However some portion of the 3D primitive might be blocked by another 3D primitive closer to the image plane, thus leading to an incoherent color. A simple test determines the zone with an occlusion risk by intersecting the 2D primitive with all 2D primitives on this image. If there is intersection, we must determine the 3D intersection between the corresponding 2D point on the image (two planes) and the potentially occluding 3D polygon (a third plane). If there is intersection and the depth is smaller than the one of the 3D point, we simply mark this occluded color sample as invalid. We extract acolor for each texel in each image. The final color for this texel must be computed from these colors. The size in pixels of the texel projected in the image is a good indication of the quality of the color extracted for this texel. The larger the projected area of a texel, the more precise the texture should be. Therefore, for all the valid colors of a given texel, we weight its color contribution as a relative function of the projected areas of the texel in all selected images. Ofek et al. stores each pixel in each image into a mipmap pyramidal structure for the texture. Color information is propagated up and down the pyramid, with some indication of certainty according to color variations. The structure fits each texel to the image pixel resolution, thus adapting its processing accordingly. However unless very high resolution textures are required, we believe the extra cost of propagating the information in the pyramid and the inevitable loss of information due to the filtering between levels might not be worth the savings. Our solution is simple, but might require sampling many texels projecting within a small fraction of a pixel area. However all information is kept at the user-specified texel resolution, and as such, we get much flexibility in ways of interpreting and filtering the information. It is also fairly simple to integrate various new criteria to improve on the process of combining the extracted texels. Unfortunately we must be aware that several situations might invalidate any such texture extraction algorithm. Any view-dependent feature that changes the aspect (color) of a 3D point as the camera moves might be a source of errors. These include specular reflections (highlights), mirrors, transparencies, refractions, ignored surface deformations (sharp grooves and peaks), participating media, etc. Without user intervention, a combination of these artifacts can hardly be handled automatically. When one color at one texel is very different than the others for different images, we simply reject its contribution, assuming it was caused by view-dependent features or noise in the image. When all the colors are very different from each other, we simply mark this texel as invalid. We will discuss in the conclusion how these differences could be used to extract such view-dependent information. The color of a pixel at silhouettes and edges of polygons includes not only the texel color, but also background and other geometry colors. Camera registration errors also introduce slight misalignments between the reconstructed 3D primitive and each 2D primitive it should project to. We again simply mark such a texel as invalid. We therefore end out with an extracted texture with some undefined texel colors. Fortunately, these typically represent a small portion of the entire texture. We currently fill in these texels by applying a simple filter, although some filling algorithms have proven to be quite efficient. They should be even more effective for gaps as narrow as those observed so far in our tests.交互式建模与摄影我们描述一个互动系统,以重建三维几何,并从中提取了与任意摄像机拍摄的照片设置纹理参数。
【精品】GIS-毕业设计-文献翻译-中英文-地理信息系统网络应用电力系统克里特岛
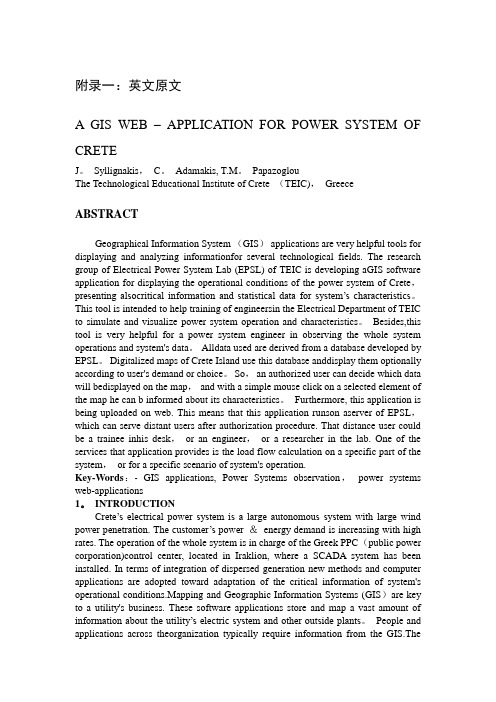
附录一:英文原文A GIS WEB –APPLICATION FOR POWER SYSTEM OF CRETEJ。
Syllignakis,C。
Adamakis, T.M。
PapazoglouThe Technological Educational Institute of Crete (TEIC),Greece ABSTRACTGeographical Information System (GIS)applications are very helpful tools for displaying and analyzing informationfor several technological fields. The research group of Electrical Power System Lab (EPSL) of TEIC is developing aGIS software application for displaying the operational conditions of the power system of Crete,presenting alsocritical information and statistical data for system’s characteristics。
This tool is intended to help training of engineersin the Electrical Department of TEIC to simulate and visualize power system operation and characteristics。
Besides,this tool is very helpful for a power system engineer in observing the whole system operations and system's data。
地理信息系统专业英语
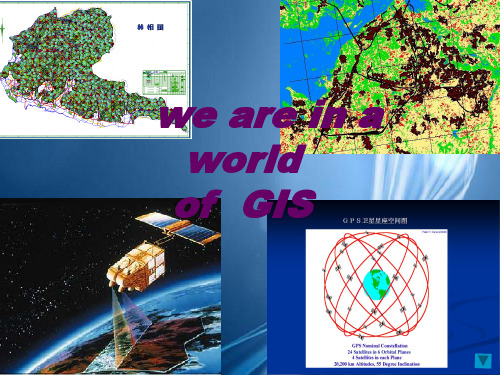
资源配置 (Resource Configuration)
city utilities
disaster relief materials distribution
energy security ……
In this type of application , GIS is to guarantee the most reasonable allocation of resources and to maximize the efficiency.
find the best locate place for a plant
return
On the consumer level
GIS applications combined with Global Positioning System as well as remote sensing technologies enable us
to
***Find the nearest Starbucks ***Get turn-by-turn directions to the closest gas station. ***Find the best locate place
consumer
Resource management
Mainly applied in agriculture and forestry fields, solve the agriculture and forestry resources questions (such as land area, forests and grasslands) classification, statistical ,distribution, etc. Main answering "localization" and "mode" two kinds of problems.
地理信息系统 专业外语 段落翻译
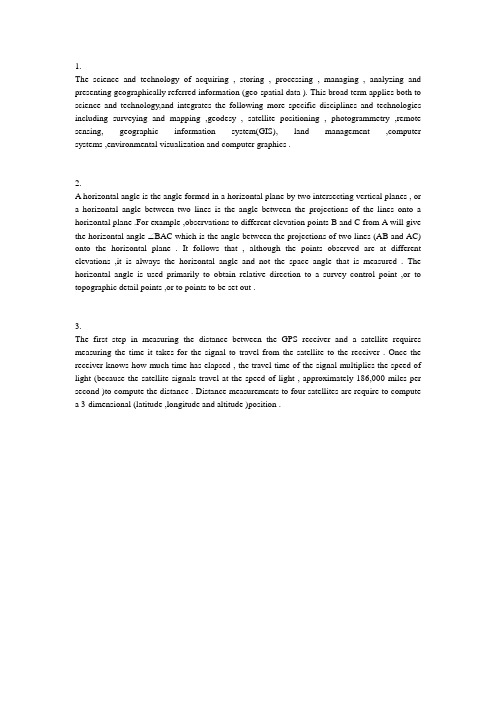
1.The science and technology of acquiring , storing , processing , managing , analyzing and presenting geographically referred information (geo-spatial data ). This broad term applies both to science and technology,and integrates the following more specific disciplines and technologies including surveying and mapping ,geodesy , satellite positioning , photogrammetry ,remote sensing, geographic information system(GIS), land management ,computer systems ,environmental visualization and computer graphics .2.A horizontal angle is the angle formed in a horizontal plane by two intersecting vertical planes , or a horizontal angle between two lines is the angle between the projections of the lines onto a horizontal plane .For example ,observations to different elevation pointsB andC from A will give the horizontal angle BAC which is the angle between the projections of two lines (AB and AC) onto the horizontal plane . It follows that , although the points observed are at different elevations ,it is always the horizontal angle and not the space angle that is measured . The horizontal angle is used primarily to obtain relative direction to a survey control point ,or to topographic detail points ,or to points to be set out .3.The first step in measuring the distance between the GPS receiver and a satellite requires measuring the time it takes for the signal to travel from the satellite to the receiver . Once the receiver knows how much time has elapsed , the travel time of the signal multiplies the speed of light (because the satellite signals travel at the speed of light , approximately 186,000 miles per second )to compute the distance . Distance measurements to four satellites are require to compute a 3-dimensional (latitude ,longitude and altitude )position .。
测绘工程专业英语课文全部翻译
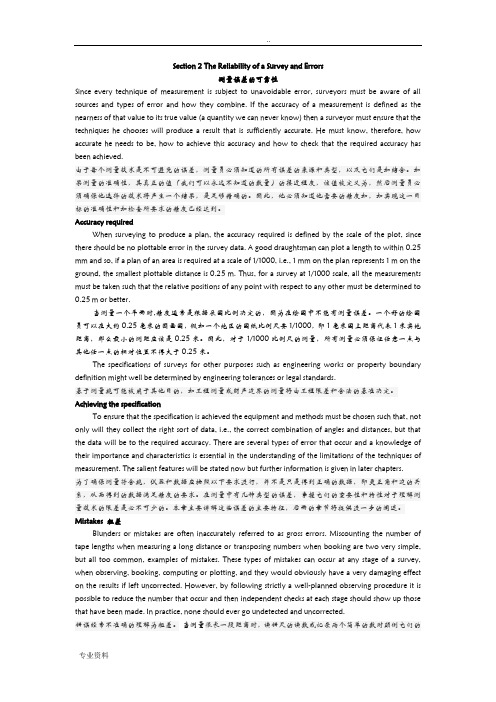
Section 2 The Reliability of a Survey and Errors测量误差的可靠性Since every technique of measurement is subject to unavoidable error, surveyors must be aware of all sources and types of error and how they combine. If the accuracy of a measurement is defined as the nearness of that value to its true value (a quantity we can never know) then a surveyor must ensure that the techniques he chooses will produce a result that is sufficiently accurate. He must know, therefore, how accurate he needs to be, how to achieve this accuracy and how to check that the required accuracy has been achieved.由于每个测量技术是不可避免的误差,测量员必须知道的所有误差的来源和类型,以及它们是如结合。
如果测量的准确性,其真正的值(我们可以永远不知道的数量)的接近程度,该值被定义为,然后测量员必须确保他选择的技术将产生一个结果,是足够精确的。
因此,他必须知道他需要的精度如,如实现这一目标的准确性和如检查所要求的精度已经达到。
Accuracy requiredWhen surveying to produce a plan, the accuracy required is defined by the scale of the plot, since there should be no plottable error in the survey data. A good draughtsman can plot a length to within 0.25 mm and so, if a plan of an area is required at a scale of 1/1000, i.e., 1 mm on the plan represents 1 m on the ground, the smallest plottable distance is 0.25 m. Thus, for a survey at 1/1000 scale, all the measurements must be taken such that the relative positions of any point with respect to any other must be determined to 0.25 m or better.当测量一个平面时,精度通常是根据展图比例决定的,因为在绘图中不能有测量误差。
测绘工程专业英语原文-译文(20-30)单元
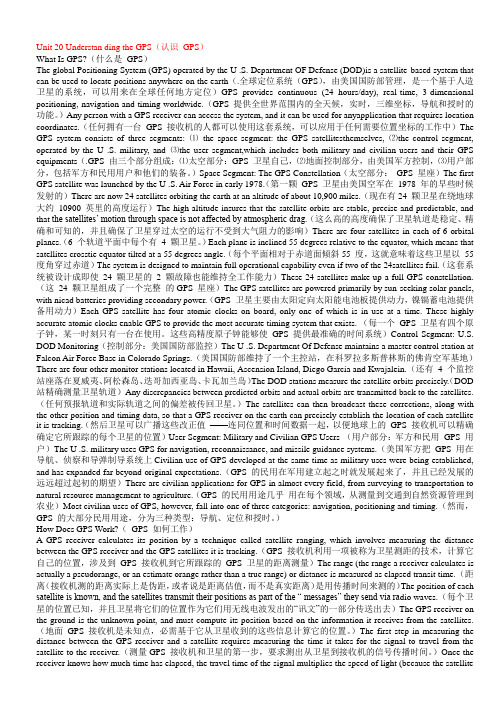
Unit 20 Understan ding the GPS(认识GPS)What Is GPS?(什么是GPS)The global Positioning System (GPS) operated by the U .S. Department OF Defense (DOD)is a satellite-based system that can be used to locate positions anywhere on the earth(.全球定位系统(GPS),由美国国防部管理,是一个基于人造卫星的系统,可以用来在全球任何地方定位)GPS provides continuous (24 hours/day), real-time, 3-dimensional positioning, navigation and timing worldwide.(GPS 提供全世界范围内的全天候,实时,三维坐标,导航和授时的功能。
)Any person with a GPS receiver can access the system, and it can be used for anyapplication that requires location coordinates.(任何拥有一台GPS 接收机的人都可以使用这套系统,可以应用于任何需要位置坐标的工作中)The GPS system consists of three segments: ⑴the space segment: the GPS satellitesthemselves, ⑵the control segment, operated by the U .S. military, and ⑶the user segment,which includes both military and civilian users and their GPS equipments(.GPS 由三个部分组成:⑴太空部分:GPS 卫星自己,⑵地面控制部分,由美国军方控制,⑶用户部分,包括军方和民用用户和他们的装备。
GIS专业英语
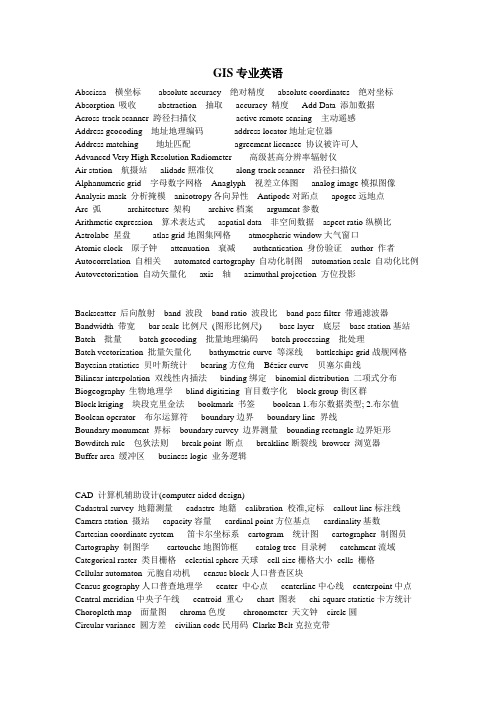
GIS专业英语Abscissa 横坐标absolute accuracy 绝对精度absolute coordinates 绝对坐标Absorption 吸收abstraction 抽取accuracy 精度Add Data 添加数据Across-track scanner 跨径扫描仪active remote sensing 主动遥感Address geocoding 地址地理编码address locator地址定位器Address matching 地址匹配agreement licensee 协议被许可人Advanced Very High Resolution Radiometer 高级甚高分辨率辐射仪Air station 航摄站alidade照准仪along-track scanner 沿径扫描仪Alphanumeric grid 字母数字网格Anaglyph 视差立体图analog image模拟图像Analysis mask 分析掩模anisotropy各向异性Antipode对跖点apogee远地点Arc 弧architecture 架构archive档案argument参数Arithmetic expression 算术表达式aspatial data 非空间数据aspect ratio纵横比Astrolabe 星盘atlas grid地图集网格atmospheric window大气窗口Atomic clock 原子钟attenuation 衰减authentication 身份验证author 作者Autocorrelation 自相关automated cartography 自动化制图automation scale 自动化比例Autovectorization 自动矢量化axis 轴azimuthal projection 方位投影Backscatter 后向散射band 波段band ratio 波段比band-pass filter 带通滤波器Bandwidth 带宽bar scale比例尺(图形比例尺) base layer 底层base station基站Batch 批量batch geocoding 批量地理编码batch processing 批处理Batch vectorization 批量矢量化bathymetric curve 等深线battleships grid战舰网格Bayesian statistics 贝叶斯统计bearing方位角Bézier curve 贝塞尔曲线Bilinear interpolation 双线性内插法binding绑定binomial distribution 二项式分布Biogeography 生物地理学blind digitizing 盲目数字化block group街区群Block kriging 块段克里金法bookmark 书签boolean 1.布尔数据类型; 2.布尔值Boolean operator 布尔运算符boundary边界boundary line 界线Boundary monument 界标boundary survey 边界测量bounding rectangle边界矩形Bowditch rule 包狄法则break point 断点breakline断裂线browser 浏览器Buffer area 缓冲区business logic 业务逻辑CAD 计算机辅助设计(computer-aided design)Cadastral survey 地籍测量cadastre 地籍calibration 校准,定标callout line标注线Camera station 摄站capacity容量cardinal point方位基点cardinality基数Cartesian coordinate system 笛卡尔坐标系cartogram 统计图cartographer 制图员Cartography 制图学cartouche地图饰框catalog tree 目录树catchment流域Categorical raster 类目栅格celestial sphere天球cell size栅格大小cells 栅格Cellular automaton 元胞自动机census block人口普查区块Census geography人口普查地理学center 中心点centerline中心线centerpoint中点Central meridian中央子午线centroid 重心chart 图表chi-square statistic卡方统计Choropleth map 面量图chroma色度chronometer 天文钟circle圆Circular variance 圆方差civilian code民用码Clarke Belt克拉克带Clarke ellipsoid 克拉克椭球Clarke spheroid 克拉克椭球面Clearinghouse(信息或服务)交换中心clinometric map坡度图code-phase GPS 码相位GPS Cognitive map认知图coincident重叠cokriging协同克里金法command 命令Command line 命令行compass north罗经北compass point 罗经点compass rose罗经盘Compass rule罗盘仪法则compression program 压缩程序Computational geometry计算几何学Containment 包含Conformal projection 等角投影,保角投影,正形投影conformality保形性Conic projection 圆锥投影conjoint boundary共同边界constant azimuth恒定方位Content Standard for Digital Geospatial Metadata 数字地理空间元数据的内容标准Continuous raster 连续栅格contour 等高线,等值线contour drawings 等高线图,等值线图Contour interval 等高线间距,等值线间距contour line等高线,等值线Contour tagging 等高线标注,等值线标注contrast ratio 对比度Contrast stretch 对比度扩展convergence angle收敛角conversion转换Convex hull 凸包coordinate geometry坐标几何学coordinate system 坐标系Coordinated universal time 协调世界时correlation相关Corridor analysis走廊分析, 廊道分析county subdivision县级分区Covariance 协方差Coverage 1.覆盖面;2.ESRI图层Cracking 裂化Crandall rule Crandall 法则crop guide 裁切参考线crop marks 裁切标记Cross correlation 交叉相关cross covariance 交叉协方差cross tabulation 交叉表Cross validation 交叉验证Cross variogram交叉变差函数Cubic convolution立方卷积插值法cultural feature人文要素Cultural geography文化地理学curb approach路边通道curve fitting曲线拟合Customizations 自定义cylindrical projection圆柱投影Dangle length悬线长度Dangle tolerance 悬线容差dangling arc 悬弧Dasymetric mapping分区制图(多用于人口数据)data management 数据管理Data table 数据表dataset 数据集datum基准DBMS 数据库管理系统(data-base management system) Dead reckoning 航位推测法Declination 1.偏角;2.磁偏角degree slope坡度Delaunay triangulation 德洛内三角Delimiter 分隔符demography人口统计学Densify 增密densitometer密度计Density slicing 密度分割deploy 部署或安装(硬件、软件等)Depression contour 洼地等高线depth contour等深线Depth curve 深度曲线Descending node 降交点Desire-line analysis期望线分析desktop 桌面Desktop clients 桌面客户端Desktop GIS 桌面GIS destination目标Determinate flow direction确定性流向Deterministic model 确定性模型Detrending 趋势分离developable surface可展表面developer 开发人员Development environment 开发环境Diazo process重氮晒印法difference 差异Differential correction 差分校正Differential Global Positioning System 差分全球定位系统Diffusion 扩散Digital elevation model 数字高程模型Digital Geographic Information Exchange Standard 数字化地理信息交换标准Digital Geographic Information Working Group 数字地理信息工作组Digital image processing 数字图像处理Digital line graph 数字线划图Digital nautical chart 数字海图Digital number 数值Digital orthophoto quadrangle 数字正射影像图Digital orthophoto quarter quadrangle 数字正射影像象限图Digital raster graphic 数字栅格图digital terrain elevation data 数字地形高程数据Digital terrain model 数字地形模型digitizer数字化仪Dijkstra’s algorithm狄捷斯特拉算法dilution of precision精度衰减因子Dimension 尺寸,维,维度directed network flow有向网络流Direction 方向Dirichlet tessellation荻瑞斯莱特镶嵌,荻瑞斯莱特剖分Discovery 发现discrete data离散数据discrete digitizing离散数字化Discrete raster 离散栅格数据Displacement 位移display scale显示比例Display unit显示单位dissemination扩散,传播distance距离Distance decay距离衰减Distance unit距离单位Distortion变形district 地区Dithering 抖动Diurnal arc周日弧docking停靠Doppler shift多普勒位移Doppler-aided GPS 多普勒辅助GPS dot density map点密度图Dot distribution map 点分布图double precision双精度Double-coordinate precision 双坐标精度Douglas-Peucker algorithm 道格拉斯-普克算法downstream下游Drafting 描绘draping叠加,披盖drift漂移drive-time area驾车时间区Drop-down list 下拉列表drum scanner鼓式扫描仪Dual Independent Map Encoding 双重独立坐标地图编码Dynamic zoom 动态缩放Easting 东距eccentricity 偏心率ecliptic 黄道edge边Edgematching 边缘匹配elastic transformation弹性变形Electromagnetic spectrum 电磁光谱electronic atlas电子地图集element 元素Electronic navigational chart 电子航海图Elevation guide 高程指南ellipsoid 椭球体Ellipticity 椭圆率End offset 末端偏移endpoint 端点enterprise GIS 企业级GIS Entity objects 实体对象envelope包络矩形environmental model 环境模型Ephemeris 星历表equal competition area平等竞争区equal-area classification等积分类Equal-area projection 等积投影equal-interval classification等距分类Equatorial plane 赤道面equidistant projection等距投影ESRI Data ESRI 数据Event 事件exponent指数export导出exposure station 摄站expression表达式Extended 扩展extent范围extrapolation 外插法extrude 拉伸extrusion拉伸Face 平面false easting 东移假定值false northing北移假定值feature 要素Federal Geographic Data Committee 美国联邦地理数据委员会field 字段Fill 填充fillet圆角filter过滤器,过滤flow direction流向flow map流向图Focal analysis邻域分析focal functions邻域函数form 地形,形式fractal 分形Framework 框架frequency 频率from-node 起点Full Extent 完整范围Fuzzy boundary 模糊边界Fuzzy classification 模糊分类fuzzy set 模糊集合Fuzzy tolerance 模糊容差Gauss-Krüger projection 高斯-克吕格投影Generalization 概化,(数据库或地图的)综合技术Geocentric coordinate system 地心坐标系geocode地理编码geocoding 地理编码Geocomputation 地理计算geodata 地理数据geodatabase 地理数据库Geodatabase data model 地理数据库数据模型Geodataset 地理数据集Geodesic 测地线Geodetic 测地学geographic coordinate system 地理坐标系Geographic information science 地理信息学Geographic Information System (GIS) 地理信息系统(GIS)Geography 地理学geography level 地理等级Geography Markup Language地理标记语言Geoid 大地水准面geoid-ellipsoid separation大地水准面-地球椭球面分离Geolocation 几何定位geometric coincidence 几何重叠Geometric correction 几何校正Geometric dilution of precision 几何精度衰减因子Geometric network 几何网络Geometric transformation 几何变换Geometry 几何学geomorphology 地貌学Geoprocessing 地理处理Georectification地理校正Georeference 地理参考Georeferencing 地理参考georelational data model 地理相关数据模型Geospatial data 地理空间数据geospatial data clearinghouse 地理空间数据交换中心Geospatial technology 地理空间技术Geospecific model 地学相关模型Geostationary 对地静止geostatistics地理统计学geosynchronous 对地同步Geotypical model 典型地理模型GIS地理信息系统GIScience地理信息学Global Navigation Satellite System 全球卫星导航系统Global Positioning System 全球定位系统GUI GUI (图形用户界面)Global spatial data infrastructure 全球空间数据基础架构Glyph 字形gnomonic projection日晷投影Go to ǿȀ转至ǿȀGPS 全球定位系统Grad 梯度(原英文单词可能有误) gradian 梯度gradient 坡度,斜率graticule 经纬网Gravimeter 重力计gravimetric geodesy 大地重力学gravity model 引力模型Gray scale 灰度great circle 大圆Greenwich mean time格林尼治标准时间Greenwich meridian格林尼治子午线grid 网格grid cell网格单元ground 大地,地面Hachure 晕渲线Hamiltonian circuit汉密尔顿回路Hamiltonian path汉密尔顿路径Height 高度Helmert transformation 线性正形变换hemisphere半球Heuristic 试探算法,试探函数hexadecimal 十六进制High Accuracy Reference Network高精度基准网High Precision Geodetic Network高精度大地基准网Hillshading 坡面阴影,晕渲histogram equalization直方图均衡化Hole 孔洞Horizontal geodetic datum 水平大地基准human geography 人文地理学Hydrography 水文地理学hydrologic cycle水循环hydrology水文学hyperlink 超链接Hypsography 测高学,地势图hypsometric curve等高线hypsometric map地势图Hypsometry 测高法Identify 识别identity link一致性链接illumination照度image coordinate图像坐标Image data 图像数据image division图像除法运算image scale 图像比例尺Image space 图像空间imager成像仪impedance阻抗import 导入IMS IMS (网络地图服务器,Internet Map Server) incident energy入射能量Index 索引index map索引图infrared scanner红外扫描仪Infrastructure 基础设施inset map插图instance 实例instantiation实例化Integer data 整数型数据integration 集成intensity 亮度Interactive vectorization 交互矢量化Interchange format 交换格式Interferogram干涉图intermediate data中间数据International date line 国际日期变更线international meridian国际子午线International Organization for Standardization 国际标准化组织Interpolation内插法interrupted projection分瓣投影intrinsic stationarity 内在稳态Inverse distance weighted interpolation 反距离加权内插法Irregular triangular mesh 不规则三角网Irregular triangular surface model 不规则三角面模型Isanomal 等地平Isarithm 等数线Isobar 等压线isochrone 等时线Isohyet 等雨量线Isolines 等值线isometric line 等容线isopleth 等值线isotherm等温线Isotropy无向性iteration 迭代iterative procedure迭代过程Jaggies 锯齿Jenks’ optimization詹克斯优化joint operations graphic 联合作战地图Junction element 交点元素Kernel 内核key identifier 主标识符kinematic positioning 动态定位Knockout 分离区(信号或通讯的中断) known point 已知点Kohonen map 柯霍南图Kriging 克里金法label标签labeling 标注lag 间隔land cover土地覆盖land information system土地信息系统land use土地利用landform 地形landmark 地标Landsat 陆地卫星landscape ecology景观生态学large scale 大比例尺lattice 点阵面layers 层layout 布局least squares 最小二乘法level 水平leveling 水平测量library 类库license 许可证license agreement 许可协议licensee 被许可人lidar 激光雷达line线line feature线要素line of sight 视线line simplification 线条简化line smoothing 线条平滑linear dimension 线性尺寸linear feature 线性要素linear interpolation 线性内插法linear referencing 线性参考(用于交通GIS) linear unit 线性单位localization 本地化location query 位置查询location-allocation 位置分配location-based services 基于位置的服务logarithm 对数logical network逻辑网络loop traverse 闭合导线loxodrome 恒向线Magnetic bearing 磁方位magnetometer 磁力计majority resampling 多数重新采样Map algebra 地图代数map collar地图边缘map display 地图显示Map document地图文档map element地图元素map extent地图范围Map feature 地图要素map generalization 地图概化,地图综合Map projection 地图投影Map query 地图查询map reading地图阅读map scale 地图比例尺map series地图系列Map service 地图服务map sheet地图map style地图风格map unit 地图单位Mapping 制图mask掩模mass point散点mathematical operator 数学运算符Matrix 矩阵mean center平均中心mean sea level 平均海平面Mean stationarity 平均稳态Measure 测量measure value 测量值Measurement residual 测量残差median中间数median center平均中心Mental map 意境图meridian子午线metadata 元数据Metropolitan statistical area 大都市统计区microdensitometer 测微密度计Micrometer 1.测微计; 2.微米minimum bounding rectangle 最小边界矩形Minimum map unit 最小地图单位minor axis短轴misclosure 闭合差Mitigation 减轻mobile clients 移动客户端Mobile GIS 移动GIS Model 模型Monument 标石morphology 形态学mosaic 镶嵌图mud pit 泥浆池Multichannel receiver 多频道接收器multidimensional data多维数据Multipart feature 多部分要素multipatch feature 带纹理要素Multiplexing channel receiver 多路复用频道接收器multipoint feature 多点要素Multispectral scanner 多光谱扫描仪multivariate analysis 多元分析My Places 我的位置National Spatial Data Infrastructure 美国国家空间数据基础设施Natural breaks classification 自然分类navigation 导航Navstar Navstar (美国国防部全球定位系统联合服务项目)Neighborhood statistics 邻域统计networked 联网node 节点Noncoterminous polygon 非相连多边形nonversioned 非版本normal distribution 正态分布Normal probability distribution 正态概率分布northing 北距Oblate ellipsoid扁椭球体oblate spheroid扁椭球面offset 偏移Oill spill 溢油(原文oill 应为Oil) Online GIS 在线GISOpen Geodata Interoperability Specification 开放地理空间数据互操作规范Open Geospatial Consortium 开放地理空间协会open traverse 不闭合导线OpenGIS Consortium OpenGIS 协会OpenLS OpenLS (OpenGIS所包含的Open Location Service)Operand 运算数operator运算符optical center 光学中心ordinal data序数数据Ordinary kriging 普通克里金法ordinate 纵坐标Ordnance Survey 英国陆地测量局Orientation 方向origin point 原点orthogonal offset 正交偏移Orthographic 正交orthomorphic 正形orthophoto 正射影像Orthophotograph 正射影像orthophotoquad 无等高线正射影像overview map 总览图Orthophotoscope 正射投影仪orthorectification 正射校正outlier 异常值Outline vectorization 轮廓矢量化output data 输出数据Overlay 重叠Overprinting 套印Pan 平移panchromatic sharpening 全色锐化parallax bar 视差尺Parameter 参数parametric curve 参数曲线passive remote sensing 被动遥感Passive sensors 被动传感器Path 路径Pathfinding 路径搜寻peak山峰Percent slope 斜率perigee 近地点persistence 持久性photogeology 摄影地质学Photogrammetry 摄影测量学Photomap 摄影地图photometer光度计Physical geography 自然地理学pit 洼地,山谷placement 放置Planar coordinate system 平面坐标系planar enforcement 平面强化planarize平面化Plane 平面planimetric map 平面图planimetric shift 平面位移Platform 平台Plot 绘图plotter绘图仪plumb line铅垂线point 点point digitizing 点数字化Point event 点事件point feature 点要素point line 点线Point mode digitizing 点模式数字化point size点大小Point-in-polygon overlay 多边形内点重叠polar aspect 极方位坡向Polar flattening 极向扁率polar orbit 极轨道polar radius 极半径Policy and management 政策与管理Polygon overlay 多边形重叠Polyhedron 多面体Polyline 折线position位置postal code 邮政编码precision code 精确码Prime meridian 本初子午线prime vertical 东西圈probability map概率图Profile graph 剖面图projected coordinate system 投影坐标系Projective transformation 射影变换prolate ellipsoid 长椭球体property属性Proximity analysis 邻近分析pseudo node 伪节点pseudo-random number伪随机数Public Land Survey System美国公共土地测量系统pyramid金字塔QQ plot QQ 图quadrangle maps 梯形图幅quadrant象限quadrat analysis样方分析Quadtree 四叉树quantile 分位数quantile classification 分位数分类Quantile scatter chart 分位数散点图quantitative data 数量数据Quantitative geography 数量地理学query 查询Radar altimeter 雷达测高计Radar interferometry 雷达干涉测量Radian 弧度Radiation 辐射radio button 单选按钮radio waves 无线电波radiometer 辐射计Radiometric 辐射测量radius半径random noise随机噪声range范围,距离Range domain 范围域,距离域raster 栅格raster band栅格层raster cell 栅格单元Raster data model栅格数据模型Raster dataset band 栅格数据层Raster model 栅格模型Raster preprocessing 栅格预处理Raster snapping 栅格贴齐Raster tracing 栅格跟踪Rasterization 栅格化ratioing 比值法ray tracing 光线跟踪RDBMS 关系数据库管理系统reclassification 重分类Record 记录Record selector 记录选择器rectangular survey 矩形测量rectification 校正Rectilinear 直线,纵横线redistricting 重新区划reference data 参考数据Reference grid 参考网格Reference level 基准面Reference map 基准图Reference spheroid 参考椭球面Reference system 参考系统Referential integrity 参照完整性Reflectance 反射率reflected back 反射Region 地区,区域regression回归relational join 关系结合Relational operator 关系运算符relationship 关系relative accuracy相对精度Relative bearing 相对方位relative mode 相对模式relative path 相对路径Release of hazardous liquids 有害液体的泄漏relief efforts 救助Relief shading 地貌晕渲remote-sensing imagery 遥感图像Replaced hachuring 替代晕渲法replication 复制Representation 表示法,表现Representative fraction数字比例尺reprojection 重新投影resampling 重采样Residuals 残差resolution merging 分辨率融合restriction 限制Reverse geocoding 反地理编码rhumb line 恒向线ring 圆环ring study圆环分析River addressing 河道寻址rotation 旋转route路线row行R-tree R 树Satellite image 卫星图像satellite imagery 卫星图像saturation饱和度Scalable 可伸缩scale bar 比例尺scale factor 比例系数scale range 比例尺范围Scatter chart 散点图scene 场景,景(卫星图像单位) Schema 架构Seamless pan 无缝平移secant projection 正割投影section 弧段segment线段Self-organizing map 自组织影射图semantics 语义semimajor axis 半长轴Semiminor axis 半短轴semivariogram 半变差函数Sensitivity analysis 敏感度分析Sensor 传感器sequence 序列sequential analysis 顺序分析Serialization 序列化Server GIS 服务器GIS sextant 六分仪shaded relief image 晕渲地貌图Shaded relief map 晕渲地貌图shading 晕渲Shape 形状Shapefile 形状文件(ESRI数据格式) shield盾牌,(地质学)地盾shift位移Shortcuts 快捷方式short-range variation 短程变化signal 信号Signal-to-noise ratio 信噪比signature特征significance level 显著性水平Sill 基台simple kriging 简单克里金法simultaneous conveyance 同时传达Sink 端点,汇点site prospecting 选址分析slope坡度smooth 平滑Snapping tolerance 捕捉容差soil 土壤sonar 声纳soundex 语音编码算法Source 起点,源点source data 源数据space coordinate system 空间坐标系Spaghetti data 无位相数据spaghetti digitizing 无位相数字化spatial analysis 空间分析Spatial cognition 空间认知spatial data 空间数据Spatial Data Transfer Standard空间数据传输标准spatial database空间数据库Spatial join 空间结合spatial modeling 空间建模spatial overlay空间叠加Spatial query 空间查询spatial reference空间参考spatial weights matrix空间权重矩阵Spatialization 空间化spectral resolution 光谱分辨率spectral signature 光谱特征Spectrometer 光谱仪spectrophotometer分光光度计Spectroscopy 光谱学Spectrum 光谱sphere球体spheroid 椭球面,椭球体spider diagram蛛网图Spike 尖峰,异常线spline 样条函数spot 点spurious polygon 伪多边形Standard deviation 标准偏差Standard Generalized Markup Language 标准通用标记语言Standard Industrial Classification codes 标准工业分类代码Star diagram 星形图state状态state plane coordinate system 国家平面坐标系Static positioning 静态定位Stationarity 稳态Stationing 定位参考Statistical surface 统计表面steep 陡峭steradian 球面度Stereocompilation 立体测图Stereogrammatic organization 立体法结构Stereographic projection 球极平面投影Stereometer 体积计Stereomodel 立体模型Stereopair 立体像对Stereoplotter 立体绘图仪stochastic model 随机性模型stream digitizing 流数字化Stream mode digitizing 流模式数字化stream tolerance 流容差streaming 数据流Stretch 拉伸string 线段串,字符串Structure 结构study area 研究区域Surface fitting 曲面拟合surface model 曲面模型surround element 周边元素Survey marker 方位标survey monument方位标survey station测点Symbol 符号Tangent projection 切面投影taskbar 任务栏temporal data 时态数据Temporal GIS 时态GIS territory 地域Tessellation 网格化textbox 文本框Texture 纹理thematic map 专题地图theodolite 经纬仪Thiessen polygons 泰森多边形Thinning 细化third normal form 第三范式three-dimensional shape 三维形状Three-tier configuration 三层结构threshold ring analysis 阈值环分析Tidal datum 潮位基准面tie point 连接点tie survey 连接测量Tissot indicatrix 天梭指示线tolerance 容差toolbar 工具栏,工具条toolbox 工具箱Tools toolbox 工具工具箱topographic contours 地形等高线topography地形学, 地形Topological overlay 拓扑重叠Topology error 拓扑误差toponym 地名tour巡回路线Township 镇区tracing 跟踪tracking data 跟踪数据tract 人口普查区transaction事务Transformation 变换transit rule 过渡法则translation平移,转换Transverse aspect横轴法投影traverse 导线triangulated irregular network 不规则三角网Triangulation 三角测量trilateration 三边测量true bearing 真实方位true curve 真实曲线True north 真北tuple 元组turn impedance转弯阻抗turn-by-turn maps多段显示地图Tutorial 教程uninitialized flow direction 未初始化的流向United States Geological Survey 美国地质勘测局univariate distribution 一元分布Universal kriging 通用克里金法universal polar stereographic 通用极球面投影坐标网Universal Soil Loss Equation 通用土壤流失方程universal time 世界时Universal transverse Mercator 统一横轴墨卡托投影upstream 上游Urban geography 城市地理学Urban Vector Map 城市矢量图Valency 度validation验证variable 变量variance 方差Variance-covariance matrix 方差协方差矩阵 Variogram 变差函数Variography 变差法Vector 矢量vectorization 矢量化verbal scale 言语比例尺Vertex 顶点Vertical axis 纵轴vertical coordinate system 垂直坐标系Vertical exaggeration 垂直夸大Vertical geodetic datum垂直大地基准Vertical photograph 垂直航拍图viewshed 视域visible scale range 可见比例范围Visual center 视觉中心visual hierarchy 视觉层次visualization可视化V oronoi diagram V oronoi 图V oxel 三维像素Warping 变形waterfall model 瀑布模型Watershed 分水岭Wavelength 波长wavelet compression 小波压缩wayfinding 路线搜寻Waypoint 路点Web clients Web 客户端Web-enabled 支持Web Weight 权重Weighted mean center 加权平均中心weighted moving average 加权移动平均Weighted overlay 加权重叠weird polygon 复杂多边形well 水井World 世界Windowing 窗口Wireframe 线框workbook 工作簿,练习册workflow 工作流Zenithal projection 天顶投影zonal analysis 区域分析zonal functions 区域函数zone of interpolation 内插区zoning 分区zoom 缩放。
GIS英文介绍

Geographic Information SystemsGeographic Information Systems integrate computer hardware, software, and trained personnel to link resource data that are geographically referenced (NASA’s define). Computer allows one to access or display data spatially, locate points, lines or areas geographically, associate data with them, and allows retrievals and calculations to be made based upon geographic locations. They store where’s and what’s, coordinates and associated attributes. A GIS staff (trained personnel) is organised to take care of maintaining the system, guiding and controlling input of data, and supporting users during the project period.The U.S. Geological Survey offers a frequently cited definition of GIS: “A computer system capable of capturing, storing, analyzing, and displaying geographically referenced information; that is, data identified according to location. Practitioners also define a GIS as including the procedures, operating personnel, and spatial data that go into the system.”GIS allows any data with a geographic component (city, ZIP Code, country, etc.) to be displayed on a map.Much of the power of GIS lies in its ability to layer information and data. Data that mean little in table format can make a strong visual impact as a map. For instance, a table of rainfall amounts in major Virginia cities may not be as effective as a map of Virginia displaying the cities and their rainfall amounts. GIS takes this idea a step further: it enables sets of data from a database to be displayed together on demand, even in unusual combinations, and therefore can reveal patterns that otherwise would be difficult to see.Historical BackgroundThe predecessors to GIS are unique uses of maps. Historically, maps were used solely for displaying geographic boundaries and features. One famous exception is a map of London created by Dr. John Snow in 1854. He suspected that an outbreak of cholera was tied to the water system. To investigate, he plotted the London water system and locations where people died from cholera on a map. This map revealed a possible relationship between the water system and the disease —an idea contrary to mainstream thinking at the time. The outbreak was eventually traced to acontaminated pump shown on Snow’s map.Another well-known example of a unique use of a map is Charles Joseph Minard’s “Losses of French Army in Napoleon’s Russian Campaign.”This 1869 map represents Napoleon’s army as a bar and plots its locations as it enters and exits Russia over time. The start of the campaign is represented as a wide bar along the Polish-Russian border. The bar narrows as it proceeds into Russia, and by the time it returns to Poland the bar has thinned to the width of a pen stroke—a dramatic representation of the army’s losses.The first technological forerunner of modern GIS was the Canada Geographic Information System, created by the Canadian government to classify uses of land. This system, developed in the 1960s, brought about many technological advances that eventually led to today’s GIS technologies. The most widely used technology today is ESRI’s ArcGIS system. Similar systems are available, such as those by MapInfo.FeaturesGIS gives new visual access to data by layering them with mapping/spatial information. Layering data allows you to adjust their display and conduct analysis ranging from basic to complex. Some typical uses for GIS include the following: ﹒Mapping: creating maps for basic analysis and communication﹒Planning: using GIS as an analytical tool to assist in planning decisions, such as selecting the site for a new store location﹒Research: using GIS as an analytical tool to explore research questions, find new relationships between data, and find new patterns in data﹒Routing: using GIS to delineate efficient route options (for example, shipping companies plotting delivery routes).Recently developed Web-based GIS (the integration of Web technology into GIS) applications make the technology even more powerful. These applications offer several benefits:﹒Inexpensive distribution of electronic maps and mapping data, whether over the Internet or a corporate intranet﹒Interactivity, which permits users to adjust the appearance of maps to meet their needs﹒Electronic map formats, which allow users to use the electronic maps generated in other documents or formatsTypes of GISGeographical variation, in the real worlds is infinitively complex. The closer you look the more detail you see almost without limit. It would take an infinitively large database to capture the real world precisely data must somehow be reduced to a finite and manageable quantity by a process of generalization or abstraction. Geographical variation must be represented in terms of discrete elements or objects. The rules used to convert real geographical variation into discrete objects is the data model. Data model is set of guidelines for the representation of the logical organization of the data in a database.Current GISs differ according the way in which they organize reality through the data model. Each model tends to fit certain types of data and applications better than others. The software available, the training of the key individuals and historical precedent, also influences the data model chosen for a particular project or application. There are two major choices of data model: raster and vector.Raster model divides the entire study area into a regular grid of cells in specific sequence. The conventional sequence is row by row from the top left corner. Each cell contains a single value. It is space-filling since every location in the study area corresponds to a cell in the raster. One set of cells and associated values is called as layer or coverage. There maybe many layers in a database, e.g. soil type, elevation, land use, land cover.Vector model uses discrete line segments or points to identify locations. Discrete objects (boundaries, streams, cities) are formed by connecting line segments. Vector objects do not necessarily fill space, not all locations in space need to be referenced in the area.A raster model tells what occurs everywhere at each place in the area. A vector model tells where everything occurs and gives a location for every object.Conceptually the raster models are the simplest of the available data models.Raster GISConsider a raster by coding each cell with a value that represents the rock type, which appears in the majority of that cell areas, when finished every cell, will have a coded value. There are several methods for creating raster databases. Direct entry of each layer cell by cell is the simplest. Much raster data is already in digital form, as images. Remote sensing generates images in the digital form. The type of values contained in a raster depends on both the reality being coded and the GIS. Each pixel or cell is assumed to have only one value.The data for an area can be visualised as a set of maps of layers. Only one item of information is available for each location within a single layer. Multiple items of information require multiple layers. Typical raster databases contain up a hundred layers. Each layer typically contains hundreds or thousands of cells.Important characteristics of a layer are its resolution, orientation and zone(s). In general resolution can be defined as the minimum linear dimension of the smallest unit geographic space for which data are recorded. The smallest units are known as cells, pixels. High resolution refers to rasters with small cell dimensions. Orientation is the angle between through north and the direction defined by the columns of the raster. Each zone of a map layer is a set of contiguous locations that exhibit the same value. These might be: ownership parcels, political units such as countries or nations, lakes or islands or individual patches of the same soil or vegetation type.Value is the item of information stored in a layer for each pixel or cell. Cells in the same zone have the same value. Location is defined by an ordered pair of coordinates that identify the location of each unit of geographic space in the raster.The vector or object GISVector data model based on vectors. Fundamental primitive is a point. Connecting points with straight lines create objects. Areas are defined by sets of lines. The term polygon is synonymous with area in vector databases because of the use of straight-line connection between points. Very large vector databases have been built for different purposes.Area objects in one class or layer cannot overlap and must exhaust the space of a layer. Every piece of boundary line is a common boundary between two areas. The strech of common boundary between two junctions (nodes) has various names such as edge, chain or arc. Arc is used by several systems and has attributes, which identify the polygons on either side. Arcs are fundamental in vector GIS. There are two ways of storing areas. In polygon storage every polygon is stored as a sequence of coordinates. Although most boundaries are shared between two adjacent areas, all are in out and coded twice, once for each adjacent polygon. This method is used by many automated mapping packages. The second method is arc storage. In this method every arcs is stored as a sequence of coordinates. Areas are built by linking arcs. Only one version of each internal shared boundary is input and stored. Used in most current vector-based GISs.Sampling the worldThe world is infinitely complex. The contents of a spatial database represent a particular view of the world. The used sees the real world through the medium of the database. The measurements and samples contained in the database must present as complete and accurate a view of the world as possible. The contents of the database must be relevant in terms of themes and characteristics captured, the time period covered and the study area.A database consists of digital representation of discrete objects. The features shown on a map, e.g. lakes, benchmarks, contours can be through of as discrete objects. Thus the contents of a map can be captured in a database by turning map features into database objects. Many of the features shown on a map are fictitious and do not exist in the real world. Contours do not really exist, but houses and lakes are real objects. The contents of a spatial database include: digital versions of real objects, e.g. houses, digital versions of artificial map features, e.g. contours and artificial objects created for the purpose of the database, e.g. pixels.Some characte ristics exist everywhere and vary continuously over the earth’s surface e.g. elevation, atmospheric temperature and pressure, natural vegetation or soil type. This kind of variation can be represented in a several ways: by takingmeasurements at sampling points, e.g. weather station; by taking transects; by dividing the area into patches or zones, and assuming the variable is constant, within each zone, e.g. soil mapping; by drawing contours, e.g. topographic mapping.Each of these methods creates discrete objects. The objects in each case are points, lines or areas.Since the 1960s, GIS has quietly transformed decision-making in universities, government, and industry by bringing digital spatial data sets and geographic analysis to desktop computers. Geographic Information Sciences include Geographic Information Systems as well as the disciplines of geography (examining the patterns of the Earth’s people and physical environment), cartography (mapmaking), geodesy (the science of measuring and surveying the Earth), and remote sensing (studying the Earth from space). GIS also provide a technology and method to analyze spatial data, or information about the Earth. The earth’s climate, natural hazards, population, geology, vegetation, soils, land use, and other characteristics can be analyzed in a GIS using computerized maps, aerial photographs, satellite images, databases, and graphs. By analyzing phenomena about the Earth’s hydrosphere, lithosphere, atmosphere, and biosphere, a GIS helps people understand patterns, linkages, and trends about our planet.《地理信息系统》参考译文根据NASA的定义,地理信息系统(GIS)是指由专业人员利用计算机硬件和软件将各种地理资源数据连接的系统。
GIS专业外语
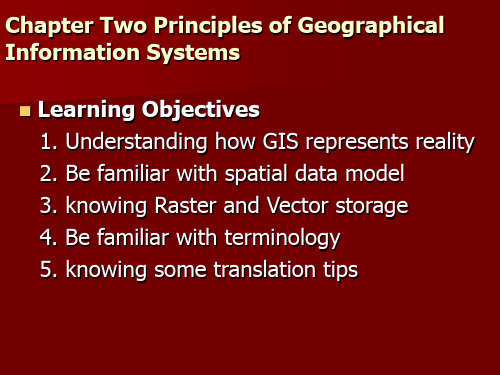
2.2.1 The Raster View of the World A raster-based system displays, locates, and stores graphical data rasterby using a matrix or grid of cells such us squares, triangular, or hexagonal cells, or even irregular triangles or polygons, which are tessellated to form geographical representations. The most-used is the regular grid, as illustrated in the next mostfigure(fig.2.3) A unique reference coordinate represents each pixel either at a corner or the centroid (shown as Fig.2.4). (翻 译)
Notes and Phrases discrete objects 离散对象 raster data 栅格数据 vector data 矢量数据 continuous fields 连续场 spatial data model 空间数据模型 digital terrain models (DTM) 数字地形模型 数字地形 地形模型 digital elevation models (DEM) 数字高程模型 exhaustive enumeration 穷举法 runrun-length encoding 行程长度编码 hierarchical File 层次文件 relational file 关系文件
GIS专业英语第一章翻译
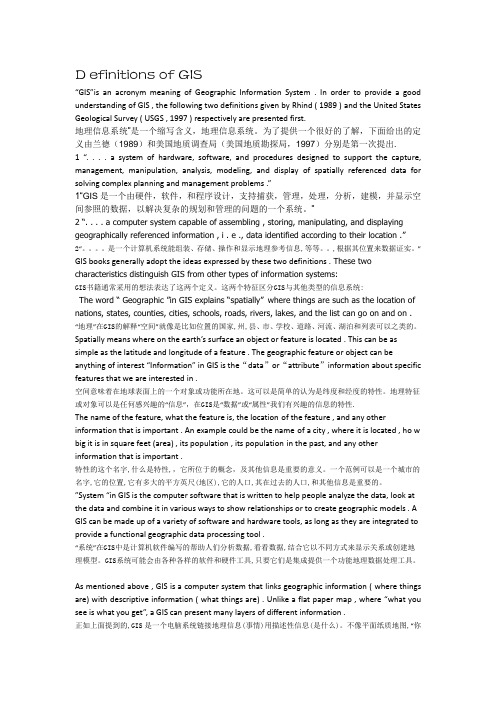
D efinitions of GIS“GIS”is an acronym meaning of Geographic Information System . In order to provide a good understanding of GIS , the following two definitions given by Rhind ( 1989 ) and the United States Geological Survey ( USGS , 1997 ) respectively are presented first.地理信息系统”是一个缩写含义,地理信息系统。
为了提供一个很好的了解,下面给出的定义由兰德(1989)和美国地质调查局(美国地质勘探局,1997)分别是第一次提出.1 “. . . . a system of hardware, software, and procedures designed to support the captu re, management, manipulation, analysis, modeling, and display of spatially referenced data for solving complex planning and manage ment problems .”1“GIS是一个由硬件,软件,和程序设计,支持捕获,管理,处理,分析,建模,并显示空间参照的数据,以解决复杂的规划和管理的问题的一个系统。
”2 “. . . . a computer system capable of assembling , storing, manipulating, and displaying geographically referenced information , i . e ., data identified according to their location .”2“。
GIS专业英语chapter 3 Geo

GIS专业英语chapter 3 Geo-referencing部分翻译Geo-referencing describes the process of locating an entity in “real world” coordinates, establishing a relation between raster or vector images to map projections or coordinate systems. This procedure is thus imperative to data modeling in the field of GIS and other cartographic methods. When data from different sources need to be combined and then used in a GIS application, it becomes essential to have a common referencing system. There are many findings devoted to Geo-referencing. Topics which are usually included in the contents of geo-referencing are:GeodesyMap projectionsCoordinate Systems空间参照描述的过程在“现实世界”的坐标定位一个实体,建立栅格或矢量图像映射关系预测或坐标系统。
这个过程是必须的数据建模领域的GIS和其他制图方法。
当需要将来自不同数据源的数据组合,然后用于GIS应用程序,就必须有一个共同的参照系统。
有许多研究致力于注释。
主题在注释的内容通常包括:大地测量学地图投影坐标系统Geodesy is the science of measuring and monitoring the size and shape of the Earth and the location of points on its surface. The elements of Geodesy are the figure of the Earth, Datum and gravity.The Earth's shape is nearly spherical, with a radius of about 3,963 miles (6,378 km), and it`s surface is very irregular. Mountains and valleys make actually measuring this surface impossible because an infinite amount of data would be needed.大地测量学是科学的测量和监测地球的大小和形状和表面上的点的位置。
gis专业英语
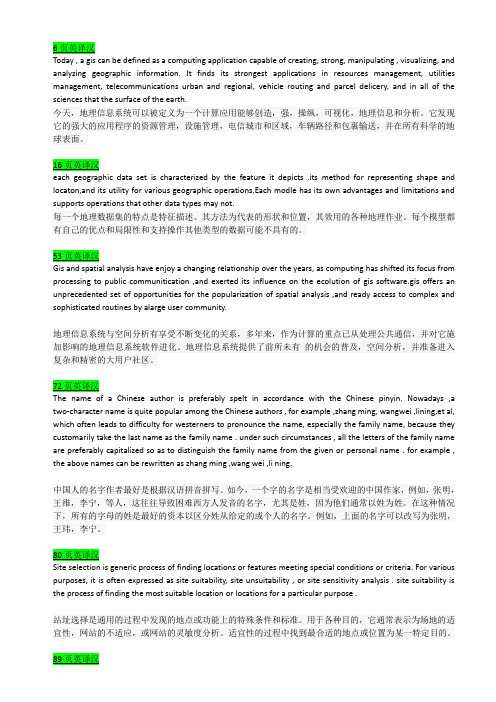
Today , a gis can be defined as a computing application capable of creating, strong, manipulating , visualizing, and analyzing geographic information. It finds its strongest applications in resources management, utilities management, telecommunications urban and regional, vehicle routing and parcel delicery, and in all of the sciences that the surface of the earth.今天,地理信息系统可以被定义为一个计算应用能够创造,强,操纵,可视化,地理信息和分析。
它发现它的强大的应用程序的资源管理,设施管理,电信城市和区域,车辆路径和包裹输送,并在所有科学的地球表面。
each geographic data set is characterized by the feature it depicts .its method for representing shape and locaton,and its utility for various geographic operations.Each modle has its own advantages and limitations and supports operations that other data types may not.每一个地理数据集的特点是特征描述。
其方法为代表的形状和位置,其效用的各种地理作业。
每个模型都有自己的优点和局限性和支持操作其他类型的数据可能不具有的。
Gis and spatial analysis have enjoy a changing relationship over the years, as computing has shifted its focus from processing to public communitication ,and exerted its influence on the ecolution of gis software.gis offers an unprecedented set of opportunities for the popularization of spatial analysis ,and ready access to complex and sophisticated routines by alarge user community.地理信息系统与空间分析有享受不断变化的关系,多年来,作为计算的重点已从处理公共通信,并对它施加影响的地理信息系统软件进化。
资勘专业英语段落翻译

Unit1 Cosmic Beginnings宇宙的起源1.Where and when does the history of the Earth begin? Only in the last few decades could this question be asked with any hope of a scientific answer. 地球的历史上是何时何地开始的?只有在过去的几十年里,这个问题才有了一个比较科学的回答来解释。
Certainly one good point at which to start is the time when the materials that were to become the Earth became separated in space from materials that were to become other members of the solar system. 当然存在一个较好的说法是地球的起源时间是当组成地球的物质在宇宙中开始与太空中组成太阳系其它成员的物质分离的时候.Although the story could well commence here, a great many important questions would remain unanswered.虽然故事很可能开始在这里,许多重要的问题仍悬而未决。
Something needs to be said about the materials that make up the Earth, and this pushes the question of origin to a more remote period. 一些有必要提及的物质构成了地球,这将推动更偏远的起源问题。
Earth's partners in space must also be considered. 地球在太空的合作伙伴也必须加以考虑。
用英语介绍地理信息科学专业的作文
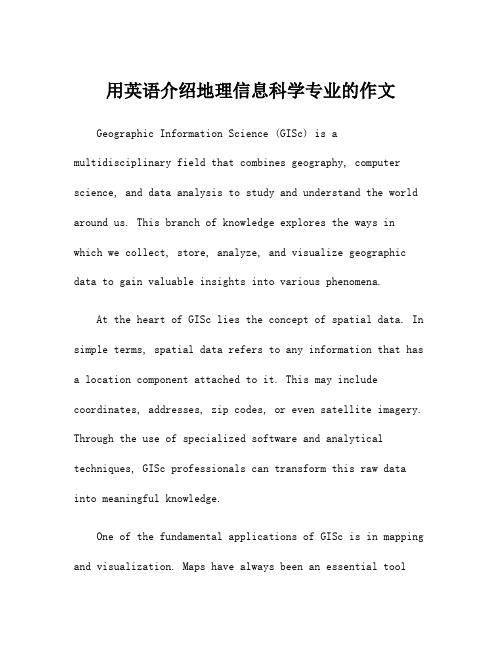
用英语介绍地理信息科学专业的作文Geographic Information Science (GISc) is a multidisciplinary field that combines geography, computer science, and data analysis to study and understand the world around us. This branch of knowledge explores the ways in which we collect, store, analyze, and visualize geographic data to gain valuable insights into various phenomena.At the heart of GISc lies the concept of spatial data. In simple terms, spatial data refers to any information that has a location component attached to it. This may include coordinates, addresses, zip codes, or even satellite imagery. Through the use of specialized software and analytical techniques, GISc professionals can transform this raw data into meaningful knowledge.One of the fundamental applications of GISc is in mapping and visualization. Maps have always been an essential toolfor understanding spatial relationships and patterns. With the advent of GISc, mapping has become more than just lines and colors on paper. It has evolved into a powerful tool for decision-making and problem-solving.GISc provides a unique platform for integrating various data sources. It allows researchers and professionals to combine data from different domains, such as demographics, economics, and environmental science, into a single comprehensive framework. This integration unlocks the potential for uncovering hidden patterns and relationships that can inform policy-making and planning.Moreover, GISc plays a crucial role in addressing real-world challenges. It helps in managing natural resources, predicting and mitigating natural disasters, and planning urban development. By analyzing spatial data, GISc specialists can assess the impact of human activities on theenvironment and develop sustainable solutions for a better future.In recent years, GISc has witnessed groundbreaking technological advancements. The emergence of remote sensing, GPS, and mobile mapping has revolutionized the field. These technologies enable us to collect data at an unprecedented scale and accuracy, opening doors to new discoveries and applications.To excel in the field of GISc, one must possess a rangeof skills. Proficiency in computer programming, data analysis, and spatial modeling is essential. Additionally, a deep understanding of geography, cartography, and datavisualization techniques is crucial for effectively communicating results to diverse audiences.GISc professionals find employment opportunities invarious sectors. Government agencies rely on their expertiseto develop land use policies, manage transportation networks,and assess environmental impacts. Private companies utilize GISc applications for market analysis, site selection, and logistics optimization. Non-profit organizations harness the power of GISc to support conservation efforts, disaster response, and social justice initiatives.In conclusion, Geographic Information Science is a fascinating field that merges geography with cutting-edge technology. It empowers us to unlock the potential of spatial data and gain valuable insights into the world we inhabit. By harnessing the power of GISc, we can make informed decisions and shape a better future for generations to come.。
地理信息专业英语复习资料

翻译:1、GIS is a system of hardware,software aad procedures to facilitate the manipulation,analysis,modeling,representation and display of geo—referencedcomplex problems regarding planning and management of resollrces,翻译:gis是一个由硬件、软件和程序组成的系统,便于管理、处理、分析、模拟、表现并显示地理参照数据,从而解决规划和资源管理的复杂问题。
2、GIS technology,integrates common database operations such as query and statisticalanalysis with the unique visualization and geographical analysis benefits offered by maps.Theseabilities distinguish GIS from other information systems and make it valuable to a wide range ofpublic and private enterprises for explaining events,predicting outcomes,and planning strategies(ESRI).翻译:地理信息系统技术将诸如查询和统计分析的常见的数据库操作和地图特有的可视化功能和地理分析优势集成起来。
这些功能是区分地理信息系统和其他信息系统的关键,并且对于众多的公共和私营企业用于事件解析,结果预测和战略规划十分有价值(ESEI)。
3、Projection is a fundamental component of mapmaking.A projection is a mathematicalmeans of transferring information from the earth’s three—dimensional,curved surface to a twodimensional medium--paper or a computer screen.Mathematically speaking,map projectionsare transformations of geographic coordinates(1atitude,longitude)into the Cartesian(x,y)coordinate space of the map.翻译:投影是地图制作的一个基本要素,同时也是将信息从地球的三维曲面上传递到纸张或电脑屏幕二维介质上的一种数学手段。
地理信息系统中英文对照外文翻译文献

中英文对照外文翻译(文档含英文原文和中文翻译)A Survey on Spatio-Temporal Data WarehousingAbstractGeographic Information Systems (GIS) have been extensively used in various application domains, ranging from economical, ecological and demographic analysis,to city and route planning. Nowadays, organizations need sophisticated GIS-based Decision Support System (DSS) to analyze their data with respect to geographic information, represented not only as attribute data, but also in maps. Thus, vendors are increasingly integrating their products, leading to the concept of SOLAP (Spatial OLAP). Also, in the last years, and motivated by the explosive growth in the use of PDA devices, the field of moving object data has been receiving attention from the GIS community. However, not much has been done in providing moving object databases with OLAP functionality. In the first part of this paper we survey theSOLAP literature. We then move to Spatio-Temporal OLAP, in particular addressing the problem of trajectory analysis. We finally provide an in-depth comparative analysis between two proposals introduced in the context of the GeoPKDD EU project: the Hermes-MDC system,and Piet, a proposal for SOLAP and moving objects,developed at the University of Buenos Aires, Argentina.Keywords: GIS, OLAP, Data Warehousing, MovingObjects, Trajectories, AggregationINTRODUCTIONGeographic Information Systems (GIS) have been extensively used in various application domains, ranging from economical, ecological and demographic analysis, to city and route planning (Rigaux, Scholl, & V oisard, 2001; Worboys, 1995). Spatial information in a GIS is typically stored in different so-called thematic layers (also called themes). Information in themes can be stored in data structures according to different data models, the most usual ones being the raster model and the vector model. In a thematic layer, spatial data is annotated with classical relational attribute information, of (in general) numeric or string type. While spatial data is stored in data structures suitable for these kinds of data, associated attributes are usually stored in conventional relational databases. Spatial data in the different thematic layers of a GIS system can be mapped univocally to each other using a common frame of reference, like a coordinate system.These layers can be overlapped or overlayed to obtain an integrated spatial view.On the other hand, OLAP (On Line Analytical Processing) (Kimball,1996; Kimball & Ross, 2002) comprises a set of tools and algorithms that allow efficiently querying multidimensional databases, containing large amounts of data, usually called Data Warehouses. In OLAP, data is organized as a set of dimensions and fact tables. In the multidimensional model, data can be perceived as a data cube, where each cell contains a measure or set of (probably aggregated) measures of interest. As we discuss later, OLAP dimensions are further organized in hierarchies that favor the data aggregation process (Cabibbo & Torlone, 1997). Several techniques and algorithms have been developed for query processing, most of them involving some kind of aggregate precomputation (Harinarayan, Rajaraman, & Ullman, 1996).The need for OLAP in GISDifferent data models have been proposed for representing objects in a GIS. ESRI () first introduced the Coverage data model to bind geometric objects to non-spatial attributes that describe them. Later, they extended this model with object-oriented support, in a way that behavior can be defined for geographic features (Zeiler,1999). The idea of the Coverage data model is also supported by the Reference Model proposed by the Open Geospatial Consortium (). Thus, in spite of the model of choice,there is always the underlying idea of binding geometric objects to objects or attributes stored in (mostly) object-relational databases (Stonebraker & Moore, 1996). In addition, query tools in commercial GIS allow users to overlap several thematic layers in order to locate objects of interest within an area, like schools or fire stations.For this, they use indexing structures based on R-trees (Gutman, 1984).GIS query support sometimes includes aggregation of geographic measures, for example, distances or areas (e.g., representing different geological zones). However, these aggregations are not the only ones that are required, as we discuss below.Nowadays, organizations need sophisticated GIS-based Decision Support System (DSS) to analyze their data with respect to geographic information, represented not only as attribute data, but also in maps, probably in different thematic layers. In this sense, OLAP and GIS vendors are increasingly integrating their products (see, for instance,Microstrategy and MapInfo integration in /, and /). In this sense, aggregate queries are central to DSSs. Classical aggregate OLAP queries (like “total sales of cars in California”), and aggregation combined with complex queries involving geometric components (“total sales in all villages crossed by the Mississippi river and within a radius of 100 km around New Orleans”) must be efficiently supported. Moreover, navigation of the results using typical OLAP operations like roll-up or drill-down is also required. These operations are not supported by commercial GIS in a straightforward way. One of the reasons is that the GIS data models discussed above were developed with “transactional” queries in mind. Thus, the databases storing nonspatial attributes or objects are designed to support those (nonaggregate) kinds of queries. Decision support systems need a different data model, where non-spatial data, probably consolidated from different sectors in an organization, is stored in a data warehouse. Here,numerical data are stored in fact tables built along several dimensions.For instance, if we are interested in the sales of certain products in stores in a given region, we may consider the sales amounts in a fact table over the three dimensions Store, Time and Product. In order to guarantee summarizability (Lenz & Shoshani, 1997), dimensions are organized into aggregation hierarchies. For example, stores can aggregate over cities which in turn can aggregate into regions and countries. Each of these aggregation levels can also hold descriptive attributes like city population, the area of a region, etc. To fulfill the requirements of integrated GIS-DSS, warehouse data must be linked to geographic data. For instance, a polygon representing a region must be associated to the region identifier in the warehouse. Besides, system integration in commercial GIS is not an easy task. In the current commercial applications, the GIS and OLAP worlds are integrated in an ad-hoc fashion, probably in a different way (and using different data models) each time an implementation is required, even when a data warehouse is available for non-spatial data.An Introductory Example. We present now a real-world example for illustrating some issues in the spatial warehousing problematic. We selected four layers with geographic and geological features obtained from the National Atlas Website (). Theselayers contain the following information: states, cities, and rivers in North America, and volcanoes in the northern hemisphere (published by the Global V olcanism Program - GVP). Figure 1 shows a detail of the layers containing cities and rivers in North America, displayed using the graphic interface of the Piet implementation we discuss later in the paper. Note the density of the points representing cities (particularly in the eastern region). Rivers are represented as polylines. Figure 2 shows a portion of two overlayed layerscontaining states (represented as polygons) and volcanoes in the northern hemisphere.There is also non-spatial information stored in a conventional data warehouse. In this data warehouse, dimension tables contain customer,stores and product information, and a fact table contains stores sales across time. Also, numerical and textual information on the geographic components exist (e.g., population, area), stored as usual as attributes of the GIS layers.In the scenario above, conventional GIS and organizational data can be integrated for decision support analysis. Sales information could be analyzed in the light of geographical features, conveniently displayed in maps. This analysis could benefit from the integration of both worlds in a single framework. Even though this integration could be possible with existing technologies, ad-hoc solutions are expensive because,besides requiring lots of complex coding, they are hardly portable. To make things more difficult, ad-hoc solutions require data exchange between GIS and OLAP applications to be performed. This implies that the output of a GIS query must be probably exported as members in dimensions of a data cube, and merged for further analysis. For example, suppose that a business analyst is interested in studying the sales of nautical goods in stores located in cities crossed by rivers. She could first query the GIS, to obtain the cities of interest. She probably has stored sales in a data cube containing a dimension Store or Geography with city as a dimension level. She would need to“manually” select the cities of interest (i.e., the ones returned by the GIS query) in the cube, to be able to go on with the analysis (in the best case, an ad-hoc customized middleware could help her). Of course, she must repeat this for each query involving a (geographic) dimension inthe data cube.Figure 1. Two overlayed layers containing cities and rivers in North America.On the contrary, GIS/Data warehousing integration can provide a more natural solution. The second part of this survey is devoted to spatio-temporal datawarehousing and OLAP. Moving objects databases (MOD) have been receiving increasing attention from the database community in recent years, mainly due to the wide variety of applications that technology allows nowadays. Trajectories of moving objects like cars or pedestrians, can be reconstructed by means of samples describing the locations of these objects at certain points in time. Although thereFigure 2. Two overlayed layers containing states in North America and volcanoes in thenorthern hemisphere.exist many proposals for modeling and querying moving objects, only a small part of them address the problem of aggregation of moving objects data in a GIS (Geographic Information Systems) scenario. Many interesting applications arise, involving moving objects aggregation, mainly regarding traffic analysis, truck fleet behavior analysis, commuter traffic in a city, passenger traffic in an airport, or shopping behavior in a mall. Building trajectory data warehouses that can integrate with a GIS is an open problem that is starting to attract database researchers. Finally, the MOD setting is appropriate for data mining tasks, and we also comment on this in the paper. In this paper, we first provide a brief background on GIS, data warehousing and OLAP, and a review of the state-of-the-art in spatial OLAP. After this, we move on to study spatio-temporal data warehousing, OLAP and mining. We then provide a detailed analysis of the Piet framework, aimed at integrating GIS, OLAP and moving object data, and conclude with a comparison between this proposal, and the Hermes data cartrridge and trajectory datawarehouse developed in the context of the GeoPKDD project (Information about the GoePKDD project can be found at http://www.geopkdd.eu).A SHORT BACKGROUNDGISIn general, information in a GIS application is divided over several thematic layers. The information in each layer consists of purely spatial data on the one hand, that is combined with classical alpha-numeric attribute data on the other hand (usually stored in a relational database). Two main data models are used for the representation of the spatial part of the information within one layer, the vector model and the raster model. The choice of model typically depends on the data source from which the information is imported into the GIS.The Vector Model. The vector model is used the most in current GIS (Kuper & Scholl, 2000). In the vector model, infinite sets of points in space are represented as finite geometric structures, or geometries, like, for example, points, polylines and polygons. More concretely, vector data within a layer consists in a finite number of tuples of the form (geometry, attributes) where a geometry can be a point, a polyline or a polygon. There are several possible data structures to actually store these geometries (Worboys, 1995).The Raster Model. In the raster model, the space is sampled into pixels or cells, each one having an associated attribute or set of attributes. Usually, these cells form a uniform grid in the plane. For each cell or pixel, the sample value of some function is computed and associated to the cell as an attribute value, e.g., a numeric value or a color. In general, information represented in the raster model is organized intozones, where the cells of a zone have the same value for some attribute(s). The raster model has very efficient indexing structures and it is very well-suited to model continuous change but its disadvantages include its size and the cost of computing the zones.Spatial information in the different thematic layers in a GIS is often joined or overlayed. Queries requiring map overlay are more difficult to compute in the vector model than in the raster model. On the other hand, the vector model offers a concise representation of the data, independent on the resolution. For a uniform treatment of different layers given in the vector or the raster model, in this paper we treat the raster model as a special case of the vector model. Indeed, conceptually, each cell is, and each pixel can be regarded as, a small polygon; also, the attribute value associated to the cell or pixel can be regarded as an attribute in the vector model.Data Warehousing and OLAPThe importance of data analysis has increased significantly in recent years as organizations in all sectors are required to improve their decision-making processes in order to maintain their competitive advantage. We said before that OLAP (On Line Analytical Processing) (Kimball, 1996; Kimball & Ross, 2002) comprises a set of tools and algorithms that allow efficiently querying databases that contain large amounts of data. These databases, usually designed for read-only access (in general, updating isperformed off-line), are denoted data warehouses. Data warehouses are exploited in different ways. OLAP is one of them. OLAP systems are based on a multidimensional model, which allows a better understanding of data for analysis purposes and provides better performance for complex analytical queries. The multidimensional model allows viewing data in an n-dimensional space, usually called a data cube (Kimball & Ross,2002). In this cube, each cell contains a measure or set of (probably aggregated) measures of interest. This factual data can be analyzed along dimensions of interest, usually organized in hierarchies (Cabibbo & Torlone, 1997). Three typical ways of OLAP tools implementation exist: MOLAP (standing for multidimensional OLAP), where data is stored in proprietary multidimensional structures, ROLAP (relational OLAP), where data is stored in (object) relational databases, and HOLAP (standing for hybrid OLAP, which provides both solutions. In a ROLAP environment, data is organized as a set of dimension tables and fact tables, and we assume this organization in the remainder of the paper.There are a number of OLAP operations that allow exploiting the dimensions and their hierarchies, thus providing an interactive data analysis environment. Warehouse databases are optimized for OLAP operations which, typically, imply data aggregation or de-aggregation along a dimension, called roll-up and drill-down, respectively. Other operations involve selecting parts of a cube (slice and dice) and reorienting the multidimensional view of data (pivoting). In addition to the basic operations described above, OLAP tools provide a great variety of mathematical, statistical, and financial operators for computing ratios, variances, ranks,etc.It is an accepted fact that data warehouse (conceptual) design is still an open issue in the field (Rizzi & Golfarelli, 2000). Most of the data models either provide a graphical representation based on the Entity- Relationship (E/R) model or UML notations, or they just provide some formal definitions without user-oriented graphical support. Recently, Malinowsky and Zimányi (2006) propose the MultiDim model. This model is based on the E/R model and provides an intuitive graphical notation. Also recently, Vaisman (Vaisman, 2006a, 2006b) introduced a methodology for requirement elicitation in Decision Support Systems, arguing that methodologies used for OLTP systems are not appropriate for OLAP systems.Temporal Data WarehousesThe relational data model as proposed by Codd (1970), is not wellsuited for handling spatial and/or temporal data. Data evolution over time must be treated in this model, in the same way as ordinary data. This is not enough for applications that require past, present, and/or future data values to be dealt with by the database. In real life such applications abound. Therefore, in the last decades, much research has been done in the field of temporal databases. Snodgrass (1995) describes the design of the TSQL2 Temporal Query Language, an upward compatible extension of SQL-92. The book, written as a result of a Dagstuhl seminar organized in June 1997 by Etzion, Jajodia, andSripada (1998), contains comprehensive bibliography, glossaries for both temporal database and time granularity concepts, and summaries of work around 1998. The same author (Snodgrass, 1999), in other work, discusses practical research issues on temporal database design and implementation.Regarding temporal data warehousing and OLAP, Mendelzon and Vaisman (2000, 2003) proposed a model, denoted TOLAP, and developed a prototype and a datalog-like query language, based on a (temporal) star schema. Vaisman, Izquierdo, and Ktenas (2006) also present a Web-based implementation of this model, along with a query language, called TOLAP-QL. Eder, Koncilia, and Morzy (2002) also propose a data model for temporal OLAP supporting structural changes. Although these efforts, little attention has been devoted to the problem of conceptual and logical modeling for temporal data warehouses. SPATIAL DATA WAREHOUSING AND OLAPSpatial database systems have been studied for a long time (Buchmann, Günther, Smith, & Wang, 1990; Paredaens, Van Den Bussche, & Gucht, 1994). Rigaux et al. (2001) survey various techniques, such as spatial data models, algorithms, and indexing methods, developed to address specific features of spatial data that are not adequately handled by mainstream DBMS technology.Although some authors have pointed out the benefits of combining GIS and OLAP, not much work has been done in this field. Vega López,Snodgrass, and Moon (2005) present a comprehensive survey on spatiotemporal aggregation that includes a section on spatial aggregation. Also, Bédard, Rivest, and Proulx (2007) present a review of the efforts for integrating OLAP and GIS. As we explain later, efficient data aggregation is crucial for a system with GIS-OLAP capabilities.Conceptual Modeling and SOLAPRivest, Bédard, and Marchand (2001) introduced the concept of SOLAP (standing for Spatial OLAP), a paradigm aimed at being able to explore spatial data by drilling on maps, in a way analogous to what is performed in OLAP with tables and charts. They describe the desirable features and operators a SOLAP system should have.Although they do not present a formal model for this, SOLAP concepts and operators have been implemented in a commercial tool called JMAP, developed by the Centre for Research in Geomatics and KHEOPS, see /en/jmap/solap.jsp. Stefanovic, Han, and Koperski (2000) and Bédard, Merret, and Han (2001), classify spatial dimension hierarchies according to their spatial references in: (a) non-geometric;(b) geometric to non-geometric; and (c) fully geometric. Dimensions of type (a) can be treated as any descriptive dimension (Rivest et al., 2001). In dimensions of types (b) and (c), a geometry is associated to members of the hierarchies. Malinowski and Zimányi (2004) extend this classification to consider that even in the absence of several related spatial levels, a dimension can be considered spatial. Here, a dimension level is spatial if it is represented as a spatial data type (e.g., point, region), allowing them to link spatial levels through topological relationships (e.g., contains, overlaps). Thus, a spatial dimension is a dimension that contains at least one spatial hierarchy. A critical point inspatial dimension modeling is the problem of multiple-dependencies, meaning that an element in one level can be related to more than one element in a level above it in the hierarchy. Jensen, Kligys, Pedersen, and Timko (2004)address this issue, and propose a multidimensional data model for mobile services, i.e., services that deliver content to users, depending on their location.This model supports different kinds of dimension hierarchies, most remarkably multiple hierarchies in the same dimension, i.e., multiple aggregation paths. Full and partial containment hierarchies are also supported. However, the model does not consider the geometry, limiting the set of queries that can be addressed. This means that spatial dimensions are standard dimensions referring to some geographical element (like cities or roads).Malinowski and Zimányi (2006) also propose a model supporting multiple aggregation paths. Pourabbas (2003) introduces a conceptual model that uses binding attributes to bridge the gap between spatial databases and a data cube. The approach relies on the assumption that all the cells in the cube contain a value, which is not the usual case in practice, as the author expresses. Also, the approach requires modifying the structure of the spatial data to support the model. No implementation is presented.Shekhar, Lu, Tan, Chawla, & Vatsavai (2001) introduced MapCube, a visualization tool for spatial data cubes. MapCube is an operator that, given a so-called base map, cartographic preferences and an aggregation hierarchy, produces an album of maps that can be navigated via roll-up and drill-down operations.Spatial Measures. Measures are characterized in two ways in the literature, namely: (a) measures representing a geometry, which can be aggregated along the dimensions; (b) a numerical value, using a topological or metric operator. Most proposals support option (a), either as a set of coordinates (Bédard et al., 2001; Rivest et al., 2001; Malinowski & Zimányi, 2004; Bimonte, Tchounikine, & Miquel, 2005), or a set of pointers to geometric objects (Stefanovic et al., 2000). Bimonte et al. (Bimonte et al., 2005) define measures as complex objects (a measure is thus an object containing several attributes). Malinowski and Zimányi (2004) follow a similar approach, but defining measures as attributes of an n-ary fact relationship between dimensions.Damiani and Spaccapietra (2006) propose MuSD, a model allowing defining spatial measures at different granularities. Here, a spatial measure can represent the location of a fact at multiple levels of (spatial) granularity. Also, an algebra of SOLAP operators is proposed.Spatial AggregationIn light of the discussion above, it should be clear that aggregation is a crucial issue in spatial OLAP. Moreover, there is not yet a consensus about a complete set of aggregate operators for spatial OLAP. We now discuss the classic approaches to spatial aggregation. Han et al. (1998) use OLAP techniques for materializing selected spatial objects, and proposed a so-called Spatial Data Cube, and the set of operations that can be performed on this data cube. The model only supports aggregation of spatial objects.Pedersen and Tryfona (2001) propose the pre-aggregation of spatial facts. First, they pre-process these facts, computing their disjoint parts in order to be able to aggregate them later. This pre-aggregation works if the spatial properties of the objects are distributive over some aggregate function. Again, the spatial measures are geometric objects.Given that this proposal ignores the geometries, queries like “total population of cities crossed by a river” are not supported. The paper does not address forms other than polygons, although the authors claim that other more complex forms are supported by the method, and the authors do not report experimental results.With a different approach, Rao, Zhang, Yu, Li, and Chen (2003), and Zhang, Li, Rao, Yu, Chen, and Liu (2003) combine OLAP and GIS for querying so-called spatial data warehouses, using R-trees for accessing data in fact tables. The data warehouse is then exploited in the usualOLAP way. Thus, they take advantage of OLAP hierarchies for locating information in the R-tree which indexes the fact table.Although the measures here are not only spatial objects, the proposal also ignores the geometric part of the model, limiting the scope of the queries that can be addressed. It is assumed that some fact table, containing the identifiers of spatial objects exists. Finally, these objects happen to be points, which is quite unrealistic in a GIS environment, where different types of objects appear in the different layers. Some interesting techniques have been recently introduced to address the data aggregation problem. These techniques are based on the combined use of (R-tree-based) indexes, materialization (or preaggregation) of aggregate measures, and computational geometry algorithms.Papadias, Tao, Kalnis, and Zhang (2002) introduce the Aggregation Rtree (aR-tree), combining indexing with pre-aggregation. The aR-tree is an R-tree that annotates each MBR (Minimal Bounding Rectangle) with the value of the aggregate function for all the objects that are enclosed by it. They extend this proposal in order to handle historic information (see the section on moving object data below), denoting this extension aRB-tree (Papadias, Tao, Zhang, Mamoulis, Shen, and & Sun, 2002). The approach basically consists in two kinds of indexes: a host index, which is an R-tree with the summarized information, and a B-tree containing time-varying aggregate data. In the most general case, each region has a B-tree associated, with the historical information of the measures of interest in the region. This is a very efficient solution for some kinds of queries, for example, window aggregate queries (i.e., for the computation of the aggregate measure of the regions which intersect a spatio-temporal window). In addition, the method is very effective when a query is posed over a query region whose intersection with the objects in a map must be computed on-thefly,and these objects are totally enclosed in the query region. However, problems may appear when leaf entries partially overlap the query window. In this case, the result must be estimated, or the actual results computed using the base tables. In fact, Tao, Kollios, Considine, Li,and Papadias (2004), show that the aRB-tree can suffer from the distinct counting problem, if the object remains in the same region for several timestamps.时空数据仓库的调查摘要地理信息系统已被广泛应用于不同的应用领域,包括经济,生态和人口统计分析,城市和路线规划。
- 1、下载文档前请自行甄别文档内容的完整性,平台不提供额外的编辑、内容补充、找答案等附加服务。
- 2、"仅部分预览"的文档,不可在线预览部分如存在完整性等问题,可反馈申请退款(可完整预览的文档不适用该条件!)。
- 3、如文档侵犯您的权益,请联系客服反馈,我们会尽快为您处理(人工客服工作时间:9:00-18:30)。
Is What You See, What You Get? GeospatialVisualizations Address Scale and UsabilityAashishChaudhary and Jeff BaumesUnlimited geospatial information now is at everyone’s fingertips with the proliferation of GPS-embedded mobile devices and large online geospatial databases. To fully understand these data and make wise decisions, more people are turning to informatics and geospatial visualization, which are used to solve many real-world problems.To effec tively gather information from data, it’s critical to address scalability and intuitive user interactions and visualizations. New geospatial analysis and visualization techniques are being used in fields such as video analysis for national defense, urban planning and hydrology.Why Having Data Isn’t Good Enough AnymorePeople are realizing that data are only useful if they can find the relevant pieces of data to make better decisions. This has broad applicability, from finding a movie to watch to elected officials deciding how much funding to allocate for an aging bridge. Information can easily be obtained, but how can it be sorted, organized, made sense of and acted on? The field of informatics solves this challenge by taking large amounts of data and processing them into meaningful, truthful insights.In informatics, two main challenges arise when computers try to condense information down to meaningful concepts: disorganization and size. Some information is available in neat, organized tables, ready for users to pull out the needed pieces, but most is scattered across and hidden in news articles, blog posts and poorly organized lists.Researchers are feverishly working on new ways to retrieve key ideas and facts from these types of messy data sources. For example, services such as Google News use computers that constantly "read" news articles and posts worldwide, and then automatically rank them by popularity, group them by topic, or organize them based on what the computer thinks is important to viewers. Researchers at places such as the University of California, Irvine, and Sandia National Laboratories are investigating the next approaches to sort through large amounts of documents using powerful supercomputers.The other obstacle is the sheer vo lume of data. It’s difficult to use informatics techniques that only work on data of limited size. Facebook, Google and Twitter have data centers that constantly process huge quantities of information to deliver timely and relevant information and advertisements to each person currently logged on..Figure 1. A collection of videos are displayed without overlap (top). The outline color represents how close each video matches a query. An alternate view (bottom) places thevideos on top of each other in a stack, showing only the strongest match result.Informatics is a key tool, but it’s not enough to simply find these insights that explain the data. Geospatial visualization bridges the gap from computer number-crunching to human understanding. If informatics is compared to finding the paths in a forest, visualization is like creating a visual map of those paths so a person can navigate through the forest with ease.Most people today are familiar with basic geospatial visualizations such as weather maps and Web sites for driving directions. The news media are starting to test more-complex geospatial visualizations such as online interactive maps to help navigate politicians’ stances on issues, exit polls and precinct reports during election times. People are just beginning to see the impact that well-designed geospatial visualizations have on their understanding of the world..Geospatial Visualization in the Real WorldPeople have been looking at data for decades, but the relevant information that accompanies the data has changed in recent years. In late 1999, Esri released a new software suite, ArcGIS, that could use data from various sources. ArcGIS provides an easy-to-use interface for visualizing 2-D and 3-D data in a geospatial context. In 2005, Google Earth launched and made geospatial visualization available to the general public.Geospatial visualization is becoming more significant and will continue to grow as it allows people to look at the totality of the data, not just one aspect. This enables better understanding and comprehension, because it puts the data in context with their surroundings. The following three cases demonstrate geospatial visualization use in real-world scenarios:1. Urban PlanningPlanners use geomodeling and geovisualization tools to explore possible scenarios and communicate their design decisions to team members or the general public. For example, urban planners may look at the presence of underground water and the terrain’s surrounding topology before deciding to build a new suburb. This is relevant for areas around Phoenix, for example, where underground water presence and proximity to a knoll or hill can determine the suitability of a location for construction.Figure 2. Videos from the same location are partially visible, resembling a stack of cards. Each video is outlined by the color representing the degree to which it matches the query.Looking at a 3-D model of a house with its surroundings gives a completely different perspective than just looking at the model of a house by itself. This also can help provide clear solutions to problems, such as changing the elevation of a building’s base to make it stand better.Urban planning is one of the emerging applications of computer-generated simulation. Cities’ rapid growth places a strain on natural resources that sustain growth. Water management, in particular, becomes a critical issue.The East Valley Water Forum is a regional cooperative of water providers east of Phoenix, and it’s designing a water-management plan for the next 100 years. Water resources in this region come from the Colorado River, the Salt River Project, groundwater, and other local and regional water resources. These resources are affected directly and indirectly by local and global factors such as population, weather, topography, etc.To best understand the relationship among water resources and various factors, the Arizona Department of Water Resources analyzes hydrologic data in the region using U.S. Geological Survey MODFLOW software, which simulates the status of underground water resources in the region. For better decision making and effective water management, a comprehensive scientific understanding of the inputs, outputs and uncertainties is needed. These uncertainties include local factors such as drought and urban growth.Looking at numbers or 2-D graphs to understand the complex relationship between input, output and other factors is insufficient in most cases. Integrating geospatial visualizations with MODFLOW simulations, for example, creates visuals that accurately represent the model inputs and outputs in ways that haven’t been previously presented.For such visualizations, two water surfaces are positioned side-by-side—coming from two different simulations—with contour lines drawn on top. In this early prototype, a simple solution—providing a geospatial plane that can be moved vertically—brings the dataset into a geospatial context. This plane includes a multi-resolution map with transparency. Because these water layers are drawn in geospatial coordinates, it matches exactly with the geospatial plane. This enables researchers to quickly see the water supplies of various locations.2. Image and Video AnalysisDefense Advanced Research Projects Agency launched a program, Video and Image Retrieval nd Analysis Tool (VIRAT), for understanding large video collections. The project’s core requirement is to add video-analysis capabilities that perform the following:• Filter and prioritize massive amounts of archived and strea ming video based on events.• Present high-value intelligence content clearly and intuitively to video analysts.• Reduce analyst workload while increasing quality and accuracy of intelligence yield.Visualization is an integral component of the VIRAT system, which uses geospatial metadata and video descriptors to display results retrieved from a database.Analysts may want to look at retrieval result sets from a specific location or during a specific time range. The results are short clips containing the object of interest and its recent trajectory. By embedding these results in a larger spatiotemporal context, analysts can determine whether a retrieved result is important.3. Scientific VisualizationU.S. Army Corps of Engineers’ research organ ization, the Engineer Research and Development Center, is working to extend the functionality of the Computational Model Builder (CMB) environment in the area of simulation models for coastal systems, with an emphasis on the Chesapeake and Delaware bays.The CMB environment consists of a suite of applications that provide the capabilities necessary to define a model (consisting of geometry and attribute information) that’s suitable for hydrological simulation. Their simulations are used to determine the impact that environmental conditions, such as human activities, have on bodies of water.Figure 3. Google Earth was used to display Chesapeake Bay’s relative salt (top) and oxygen (bottom) content (higher concentrations in red).One goal is to visualize simulation data post-processed by CMB tools. Spatiotemporal information, for example, is included in oxygen content and salinity data. Drawing data in geospatial context lets users or analysts see which locations are near certain features, giving the data orientation and scale that can easily be understood. Figure 3 shows the oxygen and salt content of Chesapeake Bay, where red shows higher concentrations and blue shows lower concentrations.Moving ForwardVisualizations that can be understood at all levels will be key in politics, economics, national security, urban planning and countless other fields. As information becomes increasingly complex, it will be harder for computers to extract and display those insights in ways people can understand.More research must be done in new geospatial analysis and visualization capabilities before we drown in our own data. And it’s even more important to educate people in how to use and interpret the wealth of analysis tools already available, extending beyond the basic road map.High schools, colleges and the media should push the envelope with new types of visuals and animations that show data in richer ways. The price of explaining these new views will be repaid when audiences gain deeper insights into the real issues otherwise hidden by simple summaries. Progress isn’t limited by the volume of available information, but by the ability to consume it.翻译:你所看到的,你得到了什么?地理空间可视化的处理规模和可用性作者:AashishChaudhary和包密斯·杰夫无限的空间信息现在就在每个人的指尖,其与扩散的嵌入式GPS移动设备和大型网上地理空间数据库。