表情识别应用1
人脸识别中的表情识别技术
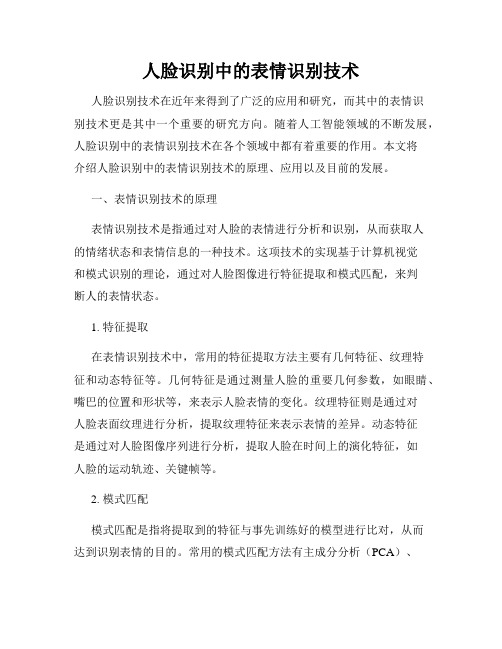
人脸识别中的表情识别技术人脸识别技术在近年来得到了广泛的应用和研究,而其中的表情识别技术更是其中一个重要的研究方向。
随着人工智能领域的不断发展,人脸识别中的表情识别技术在各个领域中都有着重要的作用。
本文将介绍人脸识别中的表情识别技术的原理、应用以及目前的发展。
一、表情识别技术的原理表情识别技术是指通过对人脸的表情进行分析和识别,从而获取人的情绪状态和表情信息的一种技术。
这项技术的实现基于计算机视觉和模式识别的理论,通过对人脸图像进行特征提取和模式匹配,来判断人的表情状态。
1. 特征提取在表情识别技术中,常用的特征提取方法主要有几何特征、纹理特征和动态特征等。
几何特征是通过测量人脸的重要几何参数,如眼睛、嘴巴的位置和形状等,来表示人脸表情的变化。
纹理特征则是通过对人脸表面纹理进行分析,提取纹理特征来表示表情的差异。
动态特征是通过对人脸图像序列进行分析,提取人脸在时间上的演化特征,如人脸的运动轨迹、关键帧等。
2. 模式匹配模式匹配是指将提取到的特征与事先训练好的模型进行比对,从而达到识别表情的目的。
常用的模式匹配方法有主成分分析(PCA)、独立成分分析(ICA)、支持向量机(SVM)等。
通过建立模型和训练样本的匹配关系,可以实现对人脸表情的识别。
二、表情识别技术的应用表情识别技术在人脸识别领域中有着广泛的应用,下面将介绍一些主要的应用场景。
1. 情感分析表情识别技术可以帮助我们判断人的情感状态,对于情感分析具有重要的意义。
比如在社交媒体中,通过分析用户在照片或视频中的表情,可以了解用户对某个事件或产品的态度和情感,从而为商家或企业提供有效的市场调研数据。
2. 人机交互表情识别技术可以应用于人机交互领域,通过对用户面部表情的识别,可以实现更加智能和自然的人机交互方式。
比如在游戏中,人脸表情的识别可以作为控制命令的输入方式,实现更加沉浸式的游戏体验。
3. 安防监控表情识别技术可以应用于安防监控领域,通过对行人或人群中的表情状态进行分析,可以判断是否存在可疑人员或异常行为。
人脸表情识别技术的使用教程与情绪分析应用
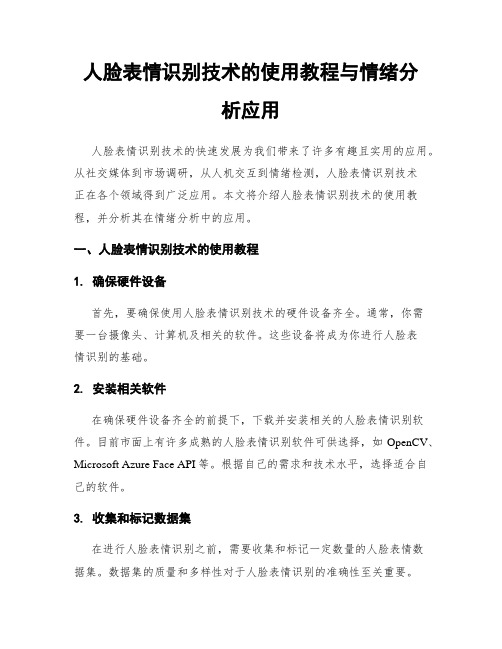
人脸表情识别技术的使用教程与情绪分析应用人脸表情识别技术的快速发展为我们带来了许多有趣且实用的应用。
从社交媒体到市场调研,从人机交互到情绪检测,人脸表情识别技术正在各个领域得到广泛应用。
本文将介绍人脸表情识别技术的使用教程,并分析其在情绪分析中的应用。
一、人脸表情识别技术的使用教程1. 确保硬件设备首先,要确保使用人脸表情识别技术的硬件设备齐全。
通常,你需要一台摄像头、计算机及相关的软件。
这些设备将成为你进行人脸表情识别的基础。
2. 安装相关软件在确保硬件设备齐全的前提下,下载并安装相关的人脸表情识别软件。
目前市面上有许多成熟的人脸表情识别软件可供选择,如OpenCV、Microsoft Azure Face API等。
根据自己的需求和技术水平,选择适合自己的软件。
3. 收集和标记数据集在进行人脸表情识别之前,需要收集和标记一定数量的人脸表情数据集。
数据集的质量和多样性对于人脸表情识别的准确性至关重要。
你可以通过在线数据库、社交媒体或者自己的照片进行数据集的搜集。
同时,要确保数据集中包含不同表情的照片,以提高模型的泛化能力。
4. 训练人脸表情识别模型在准备好数据集之后,你需要使用相关的机器学习算法来训练人脸表情识别模型。
常用的机器学习算法有支持向量机(SVM)、卷积神经网络(CNN)等。
根据选择的软件以及数据集的特点,你可以选择合适的算法进行训练。
5. 测试和优化模型在完成模型训练后,你需要进行模型的测试和优化。
通过测试模型在新数据上的表现,可以评估模型的准确性和鲁棒性。
如果模型表现不佳,你可以调整模型的参数、增加训练数据集的数量或进行其他优化策略。
6. 部署和应用模型当你对模型满意后,可以将其部署到实际应用中。
根据需求,你可以将人脸表情识别技术应用于社交媒体、人机交互、市场调研等领域。
例如,将人脸表情识别技术应用于视频聊天软件,可以实时识别对方的表情,提高交流质量。
二、人脸表情识别技术在情绪分析中的应用人脸表情识别技术在情绪分析中有广泛的应用。
面部表情识别技术在智慧医疗中的应用研究
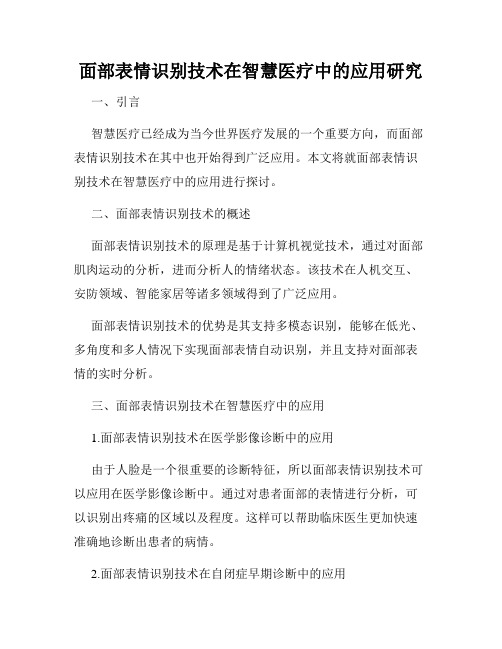
面部表情识别技术在智慧医疗中的应用研究一、引言智慧医疗已经成为当今世界医疗发展的一个重要方向,而面部表情识别技术在其中也开始得到广泛应用。
本文将就面部表情识别技术在智慧医疗中的应用进行探讨。
二、面部表情识别技术的概述面部表情识别技术的原理是基于计算机视觉技术,通过对面部肌肉运动的分析,进而分析人的情绪状态。
该技术在人机交互、安防领域、智能家居等诸多领域得到了广泛应用。
面部表情识别技术的优势是其支持多模态识别,能够在低光、多角度和多人情况下实现面部表情自动识别,并且支持对面部表情的实时分析。
三、面部表情识别技术在智慧医疗中的应用1.面部表情识别技术在医学影像诊断中的应用由于人脸是一个很重要的诊断特征,所以面部表情识别技术可以应用在医学影像诊断中。
通过对患者面部的表情进行分析,可以识别出疼痛的区域以及程度。
这样可以帮助临床医生更加快速准确地诊断出患者的病情。
2.面部表情识别技术在自闭症早期诊断中的应用自闭症是一种神经发育障碍,早期诊断是非常重要的。
而面部表情识别技术可以通过分析婴儿或儿童的面部表情来及早发现自闭症的症状,提高诊断的准确率。
3.面部表情识别技术在疾病预测中的应用面部表情识别技术可以分析患者的面部表情来预测患者的病情。
例如,通过分析患者的面部表情来判断是否患有抑郁症、焦虑症等疾病。
这样可以更早地发现患者的病情,及时进行干预和治疗。
4.面部表情识别技术在药物监测中的应用药物治疗是一种治疗疾病的手段。
然而药物治疗需要进行剂量调整,而面部表情识别技术可以被用于监测患者的情绪状态和病情变化等信息。
这样医生可以根据面部表情的分析结果来对治疗进行个性化的调整,以达到更好的治疗效果。
四、面部表情识别技术的应用前景展望随着智能技术的不断发展,面部表情识别技术也将得到更广泛的应用。
未来,这技术将会被应用于手术机器人的研发、通过分析患者面部表情来改善病房环境、以及通过分析医护人员面部表情来提高医患沟通效果等领域。
《计算机视觉》教学课件 第11章1-人脸检测、识别与表情识别1

• 自行构造人脸数据库和测试集
2024/7/13
6
项目任务
➢基于ResNet进行表情识别
➢使用Kaggle ICML表情数据集
• 包含35,887张48*48大小的表情灰度图片,共计七种类别:愤怒、厌恶、恐惧、高兴、悲伤、
惊讶和中性,并被保存在csv文件中(保存的是像素值)
MTCNN)
• 将人脸区域检测与人脸关键点检测放在一
起
• 这三个级联的网络
• P-Net生成候选框
• R-Net高精度候选框过滤选择
• O-Net生成最终候选框与人脸关键点
• 图像金字塔、非极大抑制
2024/7/13
13
知识链接-MTCNN
• P-Net,R-Net和O-Net的体系结构
• “MP”表示最大池化,“Conv”表示卷积
2024/7/13
18
知识链接-FaceNet
• Batch normalization 批归一化
• 对每层输出进行归一化处理
• 假设一个batch中有m个样本,在某一层的输出分别是 {1 , 2 , … , }, 可能是一维向量,
也可能是二维特征图
2024/7/13
19
知识链接-FaceNet
• 有三张图片参与计算
• 使得提取出来的特征,在相似图片上距离相近,不同图片上距离远
min
anchor
2024/7/13
positive
anchor negative
16
知识链接-FaceNet
2024/7/13
17
知识链接-FaceNet
• Batch normalization 批归一化
人脸表情识别技术的情感分析应用研究
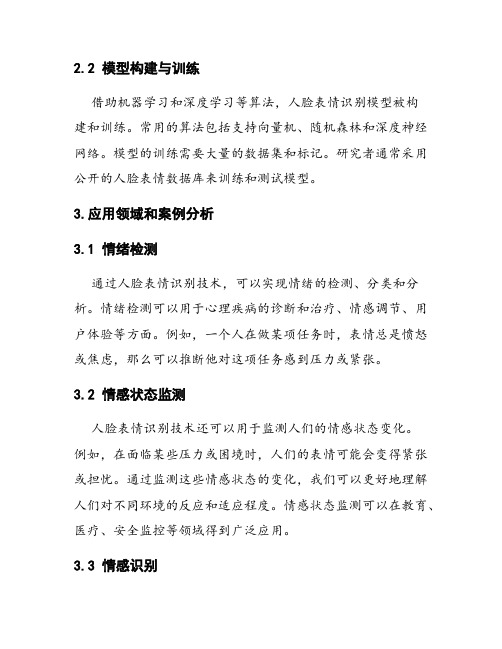
人脸表情识别技术的情感分析应用研究引言:随着科技的迅速发展,人脸表情识别技术成为了一个非常火热的研究领域。
这一技术在情感分析领域具有广泛的应用前景。
情感分析旨在通过分析人的表情来推断他们的情感状态。
本文将探讨人脸表情识别技术的应用和相关研究的进展。
1.人脸表情的情感分析意义人的表情是情感的重要表达方式,通过分析人脸表情,可以获取和推断个体的情感状态。
情感分析可以应用于情绪检测、情感状态监测、情感识别等领域。
2.人脸表情识别技术的方法2.1 特征提取特征提取是人脸表情识别的核心环节。
常用的方法包括图像处理、特征选择和特征提取。
图像处理技术可以去除背景杂乱干扰、调整图像大小和对比度,使图像更适合表情识别。
特征选择和特征提取方法可以帮助提取图像中最有代表性的特征。
2.2 模型构建与训练借助机器学习和深度学习等算法,人脸表情识别模型被构建和训练。
常用的算法包括支持向量机、随机森林和深度神经网络。
模型的训练需要大量的数据集和标记。
研究者通常采用公开的人脸表情数据库来训练和测试模型。
3.应用领域和案例分析3.1 情绪检测通过人脸表情识别技术,可以实现情绪的检测、分类和分析。
情绪检测可以用于心理疾病的诊断和治疗、情感调节、用户体验等方面。
例如,一个人在做某项任务时,表情总是愤怒或焦虑,那么可以推断他对这项任务感到压力或紧张。
3.2 情感状态监测人脸表情识别技术还可以用于监测人们的情感状态变化。
例如,在面临某些压力或困境时,人们的表情可能会变得紧张或担忧。
通过监测这些情感状态的变化,我们可以更好地理解人们对不同环境的反应和适应程度。
情感状态监测可以在教育、医疗、安全监控等领域得到广泛应用。
3.3 情感识别人脸表情识别技术的另一个重要应用领域是情感识别。
通过分析人的表情,可以准确推断他们的情感状态,例如高兴、悲伤、惊喜等。
情感识别可以应用于广告评估、市场调研、用户反馈等方面。
例如,通过分析消费者购物时的表情,可以了解他们对商品的兴趣度和满意度,从而改进产品的设计和销售策略。
人脸表情识别技术的应用教程
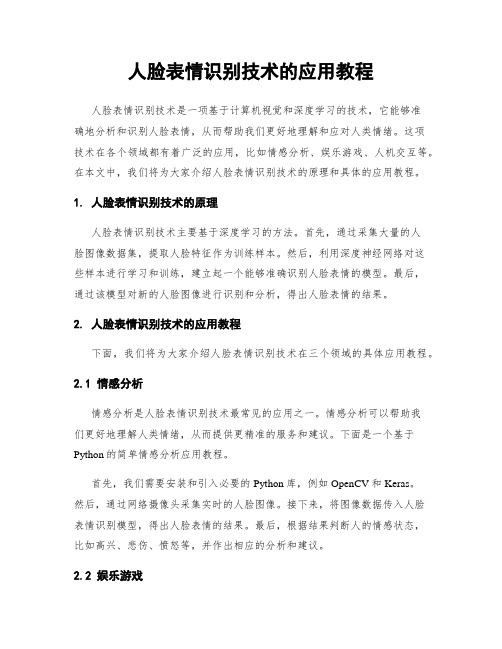
人脸表情识别技术的应用教程人脸表情识别技术是一项基于计算机视觉和深度学习的技术,它能够准确地分析和识别人脸表情,从而帮助我们更好地理解和应对人类情绪。
这项技术在各个领域都有着广泛的应用,比如情感分析、娱乐游戏、人机交互等。
在本文中,我们将为大家介绍人脸表情识别技术的原理和具体的应用教程。
1. 人脸表情识别技术的原理人脸表情识别技术主要基于深度学习的方法。
首先,通过采集大量的人脸图像数据集,提取人脸特征作为训练样本。
然后,利用深度神经网络对这些样本进行学习和训练,建立起一个能够准确识别人脸表情的模型。
最后,通过该模型对新的人脸图像进行识别和分析,得出人脸表情的结果。
2. 人脸表情识别技术的应用教程下面,我们将为大家介绍人脸表情识别技术在三个领域的具体应用教程。
2.1 情感分析情感分析是人脸表情识别技术最常见的应用之一。
情感分析可以帮助我们更好地理解人类情绪,从而提供更精准的服务和建议。
下面是一个基于Python的简单情感分析应用教程。
首先,我们需要安装和引入必要的Python库,例如OpenCV和Keras。
然后,通过网络摄像头采集实时的人脸图像。
接下来,将图像数据传入人脸表情识别模型,得出人脸表情的结果。
最后,根据结果判断人的情感状态,比如高兴、悲伤、愤怒等,并作出相应的分析和建议。
2.2 娱乐游戏人脸表情识别技术在娱乐游戏领域也有着广泛的应用。
通过该技术,我们可以开发出一些能够与人脸表情互动的娱乐游戏,例如拳击游戏、表情匹配等。
下面是一个基于Unity引擎的简单娱乐游戏应用教程。
首先,我们需要创建一个虚拟环境,并引入必要的游戏资源和编程代码。
然后,通过网络摄像头获取实时的人脸图像,并将其传递给人脸表情识别模型进行分析。
接下来,根据分析结果,将特定表情与游戏中的操作相匹配,从而与玩家进行互动。
最后,根据玩家的表情变化,不断更新游戏的状态和难度。
2.3 人机交互人脸表情识别技术还可以应用于人机交互领域,帮助我们更自然和便捷地与计算机进行交互。
面部表情识别技术的使用方法和常见问题解答

面部表情识别技术的使用方法和常见问题解答面部表情识别技术是一种通过人脸图像或视频中的面部表情信息来分析和识别人类情绪状态的技术。
它在计算机视觉和人机交互领域有着广泛的应用。
本文将介绍面部表情识别技术的使用方法,并提供一些常见问题的解答。
一、面部表情识别技术的使用方法1. 数据收集:在使用面部表情识别技术之前,需要收集一定量的数据用于模型训练。
这些数据通常包括人脸图像或视频,以及与之相关的情感标签。
可以通过从现有的面部表情数据集中收集样本,或者自己收集数据来满足训练需求。
2. 数据预处理:在数据收集之后,需要对数据进行预处理操作,以提高面部表情识别模型的准确度。
预处理操作包括对图像或视频进行裁剪、缩放、灰度化等处理。
3. 特征提取:面部表情识别技术的关键在于提取面部表情中蕴含的特征。
常用的特征提取方法包括使用光流法提取面部表情运动信息,利用神经网络模型提取高阶特征等。
4. 模型训练:通过采用监督学习方法,可以使用已标记的数据来训练面部表情识别模型。
常用的模型包括卷积神经网络(CNN)、支持向量机(SVM)等。
在训练过程中,可以使用交叉验证等技术对模型进行优化和验证。
5. 模型测试和应用:在模型训练完毕后,可以使用测试数据来评估模型的准确度和性能。
一旦模型通过测试,就可以将其应用于实际场景中,如情感分析、人机交互等。
二、常见问题解答1. 面部表情识别技术的准确度如何?面部表情识别技术的准确度受多种因素影响,包括数据质量、特征提取方法、模型训练参数等。
通常情况下,准确度可以达到较高水平,但仍存在误识别和漏识别的问题。
不同的应用场景和需求可以采用不同的指标来评估准确度。
2. 面部表情识别技术是否需要摄像头?面部表情识别技术的基础是人脸图像或视频,因此通常需要使用摄像头来采集人脸数据。
但是,在某些场景下,也可以使用已有的图像或视频数据进行识别,无需额外的摄像设备。
3. 面部表情识别技术是否能够识别不同人种、不同年龄段的面部表情?面部表情识别技术在设计和训练时通常考虑到抗干扰性和泛化能力,可以对不同人种和年龄段的面部表情进行识别。
面部表情识别技术在疾病诊断中的应用
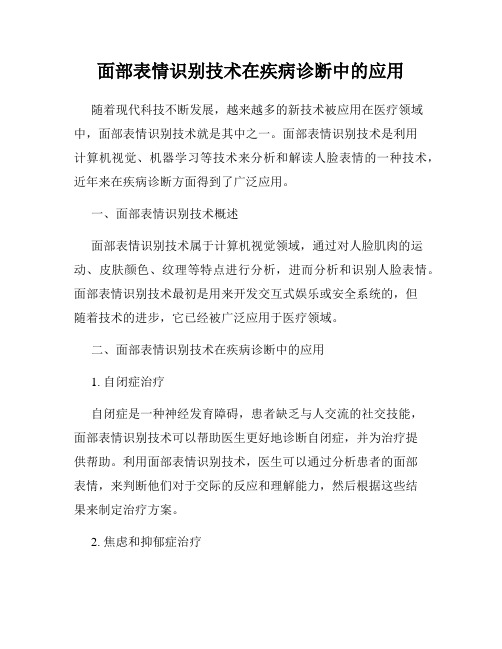
面部表情识别技术在疾病诊断中的应用随着现代科技不断发展,越来越多的新技术被应用在医疗领域中,面部表情识别技术就是其中之一。
面部表情识别技术是利用计算机视觉、机器学习等技术来分析和解读人脸表情的一种技术,近年来在疾病诊断方面得到了广泛应用。
一、面部表情识别技术概述面部表情识别技术属于计算机视觉领域,通过对人脸肌肉的运动、皮肤颜色、纹理等特点进行分析,进而分析和识别人脸表情。
面部表情识别技术最初是用来开发交互式娱乐或安全系统的,但随着技术的进步,它已经被广泛应用于医疗领域。
二、面部表情识别技术在疾病诊断中的应用1. 自闭症治疗自闭症是一种神经发育障碍,患者缺乏与人交流的社交技能,面部表情识别技术可以帮助医生更好地诊断自闭症,并为治疗提供帮助。
利用面部表情识别技术,医生可以通过分析患者的面部表情,来判断他们对于交际的反应和理解能力,然后根据这些结果来制定治疗方案。
2. 焦虑和抑郁症治疗面部表情识别技术也可以被用来诊断焦虑和抑郁症等精神疾病。
人的面部表情对于情绪状态的反应非常敏感,面部表情识别技术可以分析患者的面部表情来评估他们的情绪状态,以便制定准确的治疗方案。
3. 帕金森病治疗帕金森病是一种神经系统疾病,患者缺乏正常的运动协调能力。
利用面部表情识别技术,医生可以分析患者的面部表情来评估他们的运动能力和协调能力,从而更准确地诊断疾病,并制定更好的治疗方案。
4. 认知障碍治疗认知障碍患者缺乏对周围环境的认知和理解能力,面部表情识别技术可以帮助医生更好地评估患者的认知能力和理解能力。
通过对患者面部表情的分析,医生可以更好地理解和评估患者的认知水平,为治疗提供更好的指导。
三、面部表情识别技术的优势与传统的疾病诊断方法相比,面部表情识别技术具有如下优势:1. 非侵入性面部表情识别技术不需要进行任何身体接触,也不会对患者身体造成任何伤害,因此非常适合应用于小儿科、老人科等人群的疾病诊断。
2. 测量准确面部表情识别技术可以测量非常微小的面部运动和表情变化,从而提高诊断的准确率。
面部表情识别技术在智慧教育领域中的应用研究

面部表情识别技术在智慧教育领域中的应用研究一、引言随着人工智能技术的不断发展,越来越多的场景开始应用人工智能技术,其中智慧教育领域也不例外。
面部表情识别技术作为人工智能技术的一种,已经应用于很多领域,在智慧教育领域中的应用也越来越受到重视。
本文将对面部表情识别技术在智慧教育领域中的应用进行研究。
二、面部表情识别技术的概述面部表情识别技术是指通过对人脸图像进行分析,识别人脸的表情状态。
其主要包括人脸检测、特征提取和表情识别三个步骤。
人脸检测是指在图像中找到人脸的位置和大小;特征提取是指从人脸图像中提取出表情所需要的特征;表情识别是指通过对提取的特征进行分析,来识别人脸的表情状态。
三、面部表情识别技术在智慧教育领域中的应用1.智能教学智慧教育的发展趋势是以学生为中心,提高教学效果。
面部表情识别技术可以帮助教师更好地了解学生的学习情况,以及哪些知识点需要重点讲解。
例如,在学生学习时,通过对学生面部表情的识别,可以大致判断出学生的学习状态,包括专注度、理解能力和兴趣。
根据学生的表情状态,教师可以及时调整教学策略,更好地满足学生的需求,提高学习效果。
2.教学评估面部表情识别技术可以帮助教师进行教学评估。
教学评估是衡量教师教学水平的标准之一。
通过对学生在教学过程中的面部表情进行识别,可以大致判断出教学的成效。
例如,如果学生在课堂上的表情状态整体较为平静,那么说明学生对教学内容有了基本理解,成果也比较显著。
如果学生的表情状态整体较为无聊,那么可能说明教学内容不够生动有趣,需要加强教学技巧和方法,以便更好地吸引学生的注意力。
3.在线教育随着网络技术的不断发展,越来越多的教育机构开始采用在线教育的方式进行教学。
在线教育具有灵活、高效的特点,但是在线教育有一定的缺陷,比如难以了解学生的学习状态和表现。
面部表情识别技术可以帮助在线教育机构更好地了解学生的学习状态,以及哪些知识点需要加强。
通过对学生在学习过程中的面部表情进行分析,可以及时调整在线教育内容,满足学生的需求,提高学习效果。
计算机视觉技术在面部表情识别中的应用案例分享
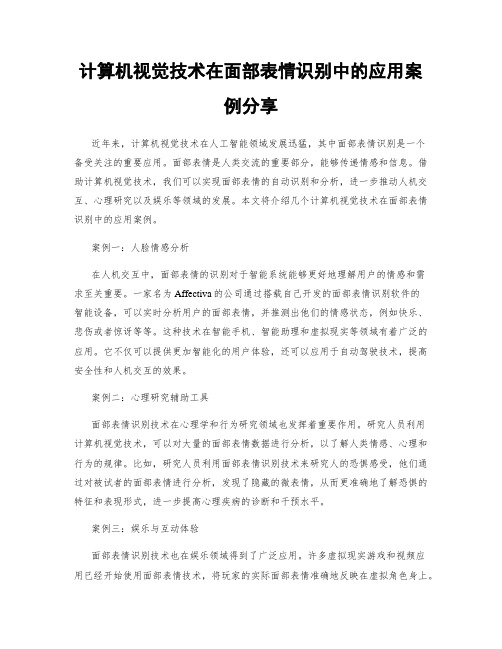
计算机视觉技术在面部表情识别中的应用案例分享近年来,计算机视觉技术在人工智能领域发展迅猛,其中面部表情识别是一个备受关注的重要应用。
面部表情是人类交流的重要部分,能够传递情感和信息。
借助计算机视觉技术,我们可以实现面部表情的自动识别和分析,进一步推动人机交互、心理研究以及娱乐等领域的发展。
本文将介绍几个计算机视觉技术在面部表情识别中的应用案例。
案例一:人脸情感分析在人机交互中,面部表情的识别对于智能系统能够更好地理解用户的情感和需求至关重要。
一家名为Affectiva的公司通过搭载自己开发的面部表情识别软件的智能设备,可以实时分析用户的面部表情,并推测出他们的情感状态,例如快乐、悲伤或者惊讶等等。
这种技术在智能手机、智能助理和虚拟现实等领域有着广泛的应用。
它不仅可以提供更加智能化的用户体验,还可以应用于自动驾驶技术,提高安全性和人机交互的效果。
案例二:心理研究辅助工具面部表情识别技术在心理学和行为研究领域也发挥着重要作用。
研究人员利用计算机视觉技术,可以对大量的面部表情数据进行分析,以了解人类情感、心理和行为的规律。
比如,研究人员利用面部表情识别技术来研究人的恐惧感受,他们通过对被试者的面部表情进行分析,发现了隐藏的微表情,从而更准确地了解恐惧的特征和表现形式,进一步提高心理疾病的诊断和干预水平。
案例三:娱乐与互动体验面部表情识别技术也在娱乐领域得到了广泛应用。
许多虚拟现实游戏和视频应用已经开始使用面部表情技术,将玩家的实际面部表情准确地反映在虚拟角色身上。
这样可以增加游戏的沉浸感,并提供更加真实的互动体验。
此外,一些社交媒体平台也利用面部表情识别技术,通过对用户的面部表情的分类、分析和识别,提供更个性化的内容推荐,增强用户的互动体验。
案例四:犯罪和情报分析面部表情识别技术在犯罪和情报分析中也扮演了重要角色。
通过对监控摄像头中的面部表情进行实时分析,可以帮助警方追踪嫌疑人的行踪和情绪变化。
同时,面部表情的识别也能够辅助犯罪心理学研究,帮助探索犯罪者的动机和行为特征。
人脸表情识别技术的使用教程
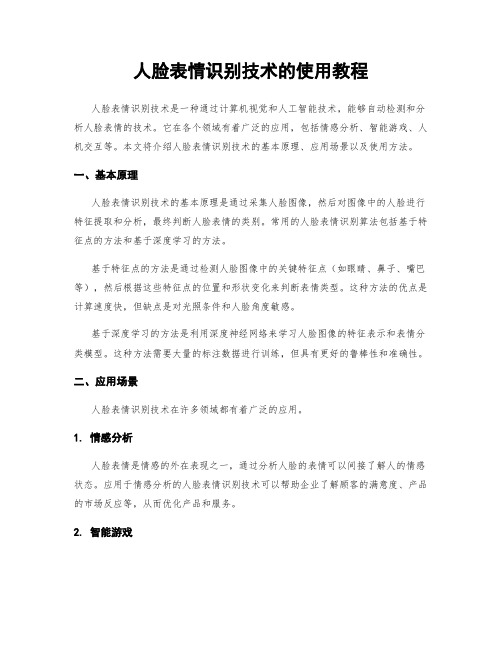
人脸表情识别技术的使用教程人脸表情识别技术是一种通过计算机视觉和人工智能技术,能够自动检测和分析人脸表情的技术。
它在各个领域有着广泛的应用,包括情感分析、智能游戏、人机交互等。
本文将介绍人脸表情识别技术的基本原理、应用场景以及使用方法。
一、基本原理人脸表情识别技术的基本原理是通过采集人脸图像,然后对图像中的人脸进行特征提取和分析,最终判断人脸表情的类别。
常用的人脸表情识别算法包括基于特征点的方法和基于深度学习的方法。
基于特征点的方法是通过检测人脸图像中的关键特征点(如眼睛、鼻子、嘴巴等),然后根据这些特征点的位置和形状变化来判断表情类型。
这种方法的优点是计算速度快,但缺点是对光照条件和人脸角度敏感。
基于深度学习的方法是利用深度神经网络来学习人脸图像的特征表示和表情分类模型。
这种方法需要大量的标注数据进行训练,但具有更好的鲁棒性和准确性。
二、应用场景人脸表情识别技术在许多领域都有着广泛的应用。
1. 情感分析人脸表情是情感的外在表现之一,通过分析人脸的表情可以间接了解人的情感状态。
应用于情感分析的人脸表情识别技术可以帮助企业了解顾客的满意度、产品的市场反应等,从而优化产品和服务。
2. 智能游戏人脸表情识别技术可以被应用于智能游戏中,实现更加真实和互动的游戏体验。
例如,游戏可以根据玩家的表情变化来调整难度、提供不同的游戏道具或者触发特定的剧情。
3. 人机交互人脸表情识别技术可以应用于人机交互系统中,使得系统可以感知用户的情感状态,从而提供更加个性化和自适应的服务。
例如,智能助理可以根据用户的表情来调整回答问题的语气和表达方式,以更好地满足用户的需求。
三、使用方法使用人脸表情识别技术通常需要以下几个步骤:1. 数据采集首先需要采集一些包含不同表情的人脸图像数据,可以使用摄像头或者已有的人脸图像数据集。
确保图像质量良好,表情明显,同时应包含各种不同的表情类型。
2. 特征提取和模型训练采用合适的人脸表情识别算法,根据采集的数据进行特征提取和模型训练。
面部表情识别技术应用
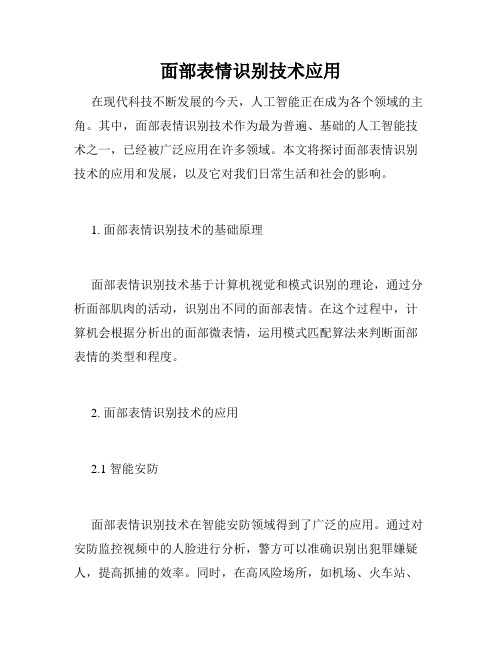
面部表情识别技术应用在现代科技不断发展的今天,人工智能正在成为各个领域的主角。
其中,面部表情识别技术作为最为普遍、基础的人工智能技术之一,已经被广泛应用在许多领域。
本文将探讨面部表情识别技术的应用和发展,以及它对我们日常生活和社会的影响。
1. 面部表情识别技术的基础原理面部表情识别技术基于计算机视觉和模式识别的理论,通过分析面部肌肉的活动,识别出不同的面部表情。
在这个过程中,计算机会根据分析出的面部微表情,运用模式匹配算法来判断面部表情的类型和程度。
2. 面部表情识别技术的应用2.1 智能安防面部表情识别技术在智能安防领域得到了广泛的应用。
通过对安防监控视频中的人脸进行分析,警方可以准确识别出犯罪嫌疑人,提高抓捕的效率。
同时,在高风险场所,如机场、火车站、商场等地方,面部表情识别技术也可以用来识别潜在的恐怖袭击者。
2.2 教育领域在教育领域,面部表情识别技术可以用来评估学生的学习情况和识别出学生的情绪变化。
面部表情识别技术可以分析学生在学习中的面部表情,从而评估其学习兴趣和学习效果,并提供更好的帮助和指导。
2.3 医疗领域面部表情识别技术在医疗领域的应用主要是辅助诊断和疾病预防。
医生可以通过对患者面部表情的分析,判断出患者是否有抑郁症、焦虑症等情绪疾病。
同时,在疾病预防方面,面部表情识别技术也可以用于早期识别自闭症、阿尔茨海默病等疾病。
2.4 娱乐和游戏面部表情识别技术在娱乐和游戏领域中也有广泛的应用。
例如,在电影中,面部表情识别技术可以用来制作更逼真的特效;在游戏中,面部表情识别技术可以使得游戏角色更加具有情感和交互性。
3. 面部表情识别技术的应用前景随着人工智能技术的不断发展,面部表情识别技术的应用前景也越来越广阔。
未来,通过对面部表情识别技术进行更深入的研究和开发,我们可以看到更多的应用场景,例如:3.1 个性化广告通过对消费者的面部表情进行识别,广告公司可以更好地了解消费者的需求和兴趣,提供更适合消费者的个性化广告。
人工智能在表情识别中的各种应用方案
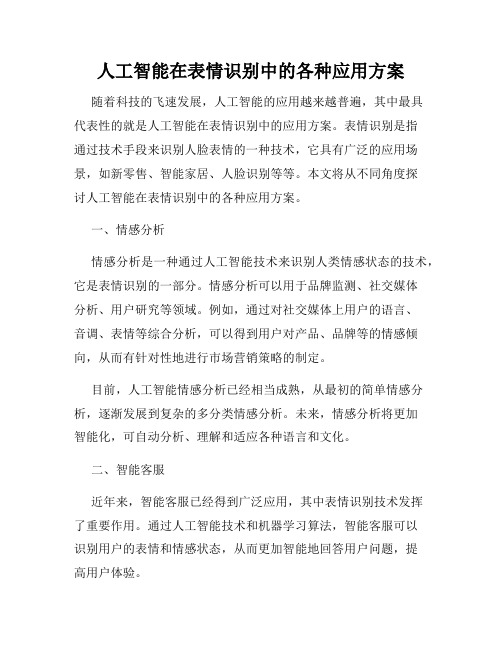
人工智能在表情识别中的各种应用方案随着科技的飞速发展,人工智能的应用越来越普遍,其中最具代表性的就是人工智能在表情识别中的应用方案。
表情识别是指通过技术手段来识别人脸表情的一种技术,它具有广泛的应用场景,如新零售、智能家居、人脸识别等等。
本文将从不同角度探讨人工智能在表情识别中的各种应用方案。
一、情感分析情感分析是一种通过人工智能技术来识别人类情感状态的技术,它是表情识别的一部分。
情感分析可以用于品牌监测、社交媒体分析、用户研究等领域。
例如,通过对社交媒体上用户的语言、音调、表情等综合分析,可以得到用户对产品、品牌等的情感倾向,从而有针对性地进行市场营销策略的制定。
目前,人工智能情感分析已经相当成熟,从最初的简单情感分析,逐渐发展到复杂的多分类情感分析。
未来,情感分析将更加智能化,可自动分析、理解和适应各种语言和文化。
二、智能客服近年来,智能客服已经得到广泛应用,其中表情识别技术发挥了重要作用。
通过人工智能技术和机器学习算法,智能客服可以识别用户的表情和情感状态,从而更加智能地回答用户问题,提高用户体验。
例如,当用户表现出负面情绪时,智能客服可以通过表情识别技术及时识别并主动进行回应。
这不仅可以避免用户流失,还可以向用户传递公司的关心和尽责。
三、身份识别另外,表情识别技术也可以用于身份识别。
通过分析人的面部信息,人工智能可以精确识别不同的用户及其身份信息,从而帮助安防系统进行身份验证和用户识别。
例如,在智能门禁系统中,通过人脸识别技术识别用户的面部信息,结合表情识别技术进行情感分析,可以实现高效精准的身份识别,确保门禁的安全性。
四、人体健康另外,表情识别技术在医疗行业中也有广泛的应用前景。
通过分析人的面部特征和表情信息,可以识别出人的情感状态和心理健康状况,有助于进行心理健康治疗和疾病预防。
例如,通过分析人的面部信息,医疗系统可以判断出病人的心理状态,为医生提供更全面的医疗诊断信息,从而更加精准地进行治疗和疾病预防。
人脸表情识别算法的研究与应用

人脸表情识别算法的研究与应用人脸表情识别算法是计算机视觉领域中的一项重要技术。
随着计算机的发展和智能化程度的提高,人脸表情识别算法的应用范围也越来越广泛,成为大数据、人工智能等领域的热门技术之一。
1. 研究背景随着科技的不断发展,智能设备和智能应用已经深入我们的生活。
人脸识别、虚拟现实和智能交互等技术不断涌现。
而人脸表情识别技术则成为了其中一个重要的组成部分。
人脸表情识别算法可以通过识别人脸表情来还原人类的情感反应,可以应用于情绪计量分析、心理诊断和交互式游戏等领域。
2. 研究意义人脸表情识别技术不仅可以扩展人们对视觉上的认知,更有助于科技的普及和智能化的发展,可以为人类创造出更多丰富的应用体验。
例如,人脸表情识别技术能够实现与智能设备的人机交互,也可以为医学领域提供实时的心理评估,帮助临床医生更快、更准确地定位患者的情绪状态。
在商业领域,人脸表情识别技术还可以分析消费者的情绪状态,为品牌营销和市场推广等提供更为准确的数据支持。
3. 研究现状当前,针对人脸识别技术的研究主要集中在两个方向上:一是快速人脸检测和定位技术,二是人脸表情分类算法。
人脸表情分类算法最初采用传统的机器学习方法进行研究,主要使用分类器和特征选择器来训练分类模型。
然而,随着深度学习模型的引入,诸如卷积神经网络(CNN)等,人脸表情识别技术取得了重大突破。
在此基础上,又有了一些新的变种,如局部细节分析(LBP)和频域分析等。
这些技术的应用,促进了人脸表情识别算法更加智能化和快速化的发展。
4. 应用场景人脸表情识别技术可以应用于许多领域,包括医学、心理学、娱乐、教育、电子商务等。
在医学方面,该技术可以帮助医生识别患者的情绪状态,辅助医学诊断。
在心理学方面,人脸表情识别技术可以通过分析语音、肢体动作和面部表情等,为心理学家提供更为准确的认知心理学数据。
在娱乐领域,这项技术已被广泛应用,例如王者荣耀游戏等,可以通过识别玩家面部表情,提高游戏的互动性和娱乐性。
面部表情识别技术在人机交互中的应用
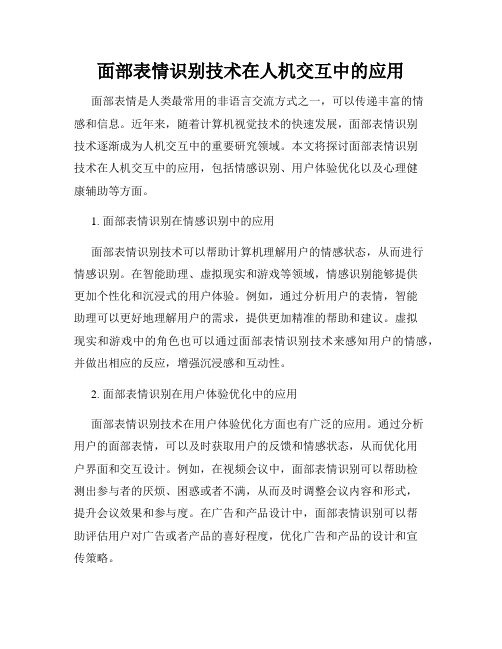
面部表情识别技术在人机交互中的应用面部表情是人类最常用的非语言交流方式之一,可以传递丰富的情感和信息。
近年来,随着计算机视觉技术的快速发展,面部表情识别技术逐渐成为人机交互中的重要研究领域。
本文将探讨面部表情识别技术在人机交互中的应用,包括情感识别、用户体验优化以及心理健康辅助等方面。
1. 面部表情识别在情感识别中的应用面部表情识别技术可以帮助计算机理解用户的情感状态,从而进行情感识别。
在智能助理、虚拟现实和游戏等领域,情感识别能够提供更加个性化和沉浸式的用户体验。
例如,通过分析用户的表情,智能助理可以更好地理解用户的需求,提供更加精准的帮助和建议。
虚拟现实和游戏中的角色也可以通过面部表情识别技术来感知用户的情感,并做出相应的反应,增强沉浸感和互动性。
2. 面部表情识别在用户体验优化中的应用面部表情识别技术在用户体验优化方面也有广泛的应用。
通过分析用户的面部表情,可以及时获取用户的反馈和情感状态,从而优化用户界面和交互设计。
例如,在视频会议中,面部表情识别可以帮助检测出参与者的厌烦、困惑或者不满,从而及时调整会议内容和形式,提升会议效果和参与度。
在广告和产品设计中,面部表情识别可以帮助评估用户对广告或者产品的喜好程度,优化广告和产品的设计和宣传策略。
3. 面部表情识别在心理健康辅助中的应用面部表情识别技术在心理健康辅助方面也有着潜在的应用价值。
面部表情可以反映出个体的情感状态和心理健康水平。
通过分析面部表情,可以进行情绪监测和心理诊断,帮助人们更好地了解自己的心理状态,并及时采取相应的调节和干预措施。
此外,面部表情识别技术还可以应用于心理疾病的识别和治疗辅助。
例如,对于抑郁症患者,面部表情识别可以帮助监测患者的情绪波动,及时发现并干预。
总之,面部表情识别技术在人机交互中具有广阔的应用前景。
它可以帮助计算机理解用户的情感状态,提供个性化的服务和优化用户体验。
在情感识别、用户体验优化以及心理健康辅助等方面,面部表情识别技术都能发挥重要的作用。
AI技术在人脸表情识别中的实际应用

AI技术在人脸表情识别中的实际应用一、引言人脸表情识别已经成为人工智能技术发展中的重要领域之一。
随着深度学习和计算机视觉方面的快速进步,AI技术在人脸表情识别中展现出了巨大的潜力。
本文将探讨AI技术在人脸表情识别中的实际应用。
二、人脸表情识别的挑战人脸表情识别是指通过对人脸图像或视频进行分析和处理,来判断出被拍摄者当前所处的表情状态。
然而,由于面部表情的复杂性和多变性,使得该任务具有一定的挑战性。
首先,面部表情通常受到多种因素的影响,如肌肉运动、眼神以及唇形等。
这些因素相互作用并产生微小而细微的变化,给算法提供了极大的挑战。
其次,人类在观察和理解不同表情时会倾向于依赖上下文信息进行综合判断。
然而,在计算机视觉领域中获取和利用上下文信息则更具挑战性。
三、AI技术在人脸表情识别中的应用1.面部表情分析AI技术中的基于深度学习的模型能够对人脸图像进行高精度的面部表情分析。
通过构建大规模的标注数据集和优化算法,这些模型能够准确地识别出照片或视频中人脸的表情状态,如快乐、悲伤、愤怒等。
2.情绪识别除了分析面部表情外,AI技术还有助于对人体其他特征进行情绪识别。
例如,声音、语调和姿势等传达的信息都可以被捕捉和理解。
基于这些特征,AI系统能够推断并判断出某个人的情感状态,从而帮助人们更好地理解和与他们进行交流。
3.移动应用与虚拟现实在移动应用中,借助AI技术可实现实时的表情检测与跟踪。
这为用户提供了一种全新的互动方式。
同时,在虚拟现实技术领域中,AI技术也可用于增强用户体验。
例如,在游戏中使用摄像头来捕捉玩家的表情,并将其映射到游戏角色身上,使得游戏过程更加真实和沉浸。
4.情绪监控与用户体验改进AI技术在人脸表情识别中的应用还可以进一步扩展到情绪监控和用户体验改进方面。
通过分析用户的表情,企业可以更好地了解他们对产品或服务的态度和反应。
这有助于优化产品设计和提供更个性化的服务,以提高用户满意度。
四、AI技术在人脸表情识别中的挑战尽管AI技术在人脸表情识别中具有广阔的应用前景,但目前仍存在一些挑战需要克服。
人工智能在面部表情识别中的应用
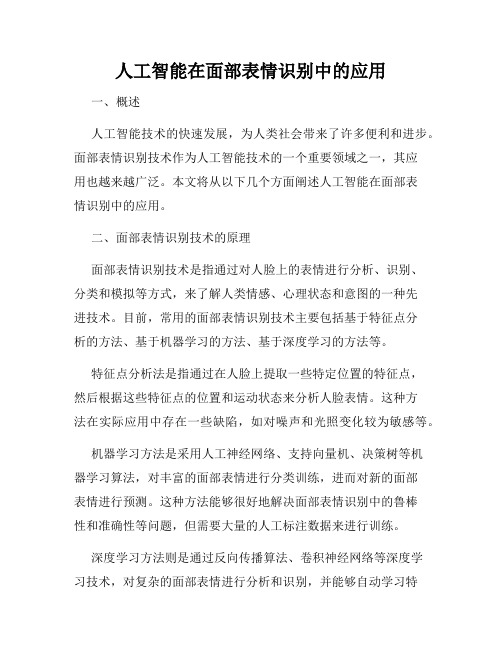
人工智能在面部表情识别中的应用一、概述人工智能技术的快速发展,为人类社会带来了许多便利和进步。
面部表情识别技术作为人工智能技术的一个重要领域之一,其应用也越来越广泛。
本文将从以下几个方面阐述人工智能在面部表情识别中的应用。
二、面部表情识别技术的原理面部表情识别技术是指通过对人脸上的表情进行分析、识别、分类和模拟等方式,来了解人类情感、心理状态和意图的一种先进技术。
目前,常用的面部表情识别技术主要包括基于特征点分析的方法、基于机器学习的方法、基于深度学习的方法等。
特征点分析法是指通过在人脸上提取一些特定位置的特征点,然后根据这些特征点的位置和运动状态来分析人脸表情。
这种方法在实际应用中存在一些缺陷,如对噪声和光照变化较为敏感等。
机器学习方法是采用人工神经网络、支持向量机、决策树等机器学习算法,对丰富的面部表情进行分类训练,进而对新的面部表情进行预测。
这种方法能够很好地解决面部表情识别中的鲁棒性和准确性等问题,但需要大量的人工标注数据来进行训练。
深度学习方法则是通过反向传播算法、卷积神经网络等深度学习技术,对复杂的面部表情进行分析和识别,并能够自动学习特征和模式。
这种方法能够处理大量的数据,并且在识别准确率上能够实现更高的水平。
三、人工智能在面部表情识别中的应用面部表情识别技术可以广泛应用于人脸识别、情感识别、用户体验等领域。
1. 人脸识别面部表情识别技术可以帮助人脸识别系统更准确地识别个体。
通过对面部表情进行分析和比对,可以更准确地识别人脸。
如苹果公司在iPhone X推出的Face ID功能,就是通过3D传感器和AI面部识别技术,实现更安全和更准确的人脸解锁功能。
2. 情感识别面部表情识别技术还可以帮助用户更准确地了解其他人的情感状态,如快乐、悲伤、愤怒等。
这种技术可以在商业应用中得到广泛的应用,如在电商平台上,通过用户的面部表情来了解其购买意愿,从而为用户提供更好的推荐服务。
3. 用户体验面部表情识别技术还可以在用户体验方面得到应用。
人脸表情识别技术在心理健康评估中的应用
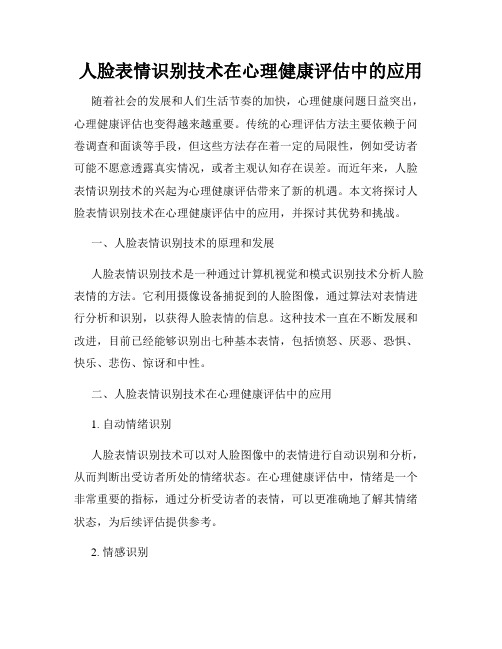
人脸表情识别技术在心理健康评估中的应用随着社会的发展和人们生活节奏的加快,心理健康问题日益突出,心理健康评估也变得越来越重要。
传统的心理评估方法主要依赖于问卷调查和面谈等手段,但这些方法存在着一定的局限性,例如受访者可能不愿意透露真实情况,或者主观认知存在误差。
而近年来,人脸表情识别技术的兴起为心理健康评估带来了新的机遇。
本文将探讨人脸表情识别技术在心理健康评估中的应用,并探讨其优势和挑战。
一、人脸表情识别技术的原理和发展人脸表情识别技术是一种通过计算机视觉和模式识别技术分析人脸表情的方法。
它利用摄像设备捕捉到的人脸图像,通过算法对表情进行分析和识别,以获得人脸表情的信息。
这种技术一直在不断发展和改进,目前已经能够识别出七种基本表情,包括愤怒、厌恶、恐惧、快乐、悲伤、惊讶和中性。
二、人脸表情识别技术在心理健康评估中的应用1. 自动情绪识别人脸表情识别技术可以对人脸图像中的表情进行自动识别和分析,从而判断出受访者所处的情绪状态。
在心理健康评估中,情绪是一个非常重要的指标,通过分析受访者的表情,可以更准确地了解其情绪状态,为后续评估提供参考。
2. 情感识别除了情绪识别外,人脸表情识别技术还可以帮助识别出人脸图像中的其他情感表达,例如焦虑、抑郁等。
这些情感在心理健康评估中也具有重要的意义,通过分析受访者的情感表达,可以更好地理解其心理健康状况。
3. 自动评分传统的心理评估方法需要专业人员进行面谈和分析,可能存在个人主观偏见,评估结果的可靠性受到一定影响。
而人脸表情识别技术可以自动对受访者的表情进行分析和评分,减少了人为因素的干扰,提高了评估结果的客观性和准确性。
三、人脸表情识别技术在心理健康评估中的优势1. 非侵入性人脸表情识别技术不需要任何特殊操作或器械,只需要使用摄像设备捕捉到人脸图像即可。
这种非侵入性的特点使得心理评估过程更加舒适和自然,受访者更容易展示真实的情感和表情。
2. 高效准确人脸表情识别技术可以实现对大量数据的快速分析和处理,大大提高了评估的效率。
人脸表情识别技术在心理学研究中的应用教程分析
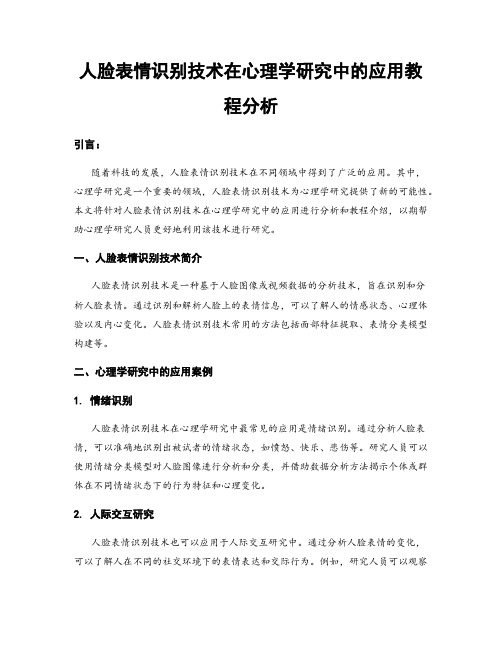
人脸表情识别技术在心理学研究中的应用教程分析引言:随着科技的发展,人脸表情识别技术在不同领域中得到了广泛的应用。
其中,心理学研究是一个重要的领域,人脸表情识别技术为心理学研究提供了新的可能性。
本文将针对人脸表情识别技术在心理学研究中的应用进行分析和教程介绍,以期帮助心理学研究人员更好地利用该技术进行研究。
一、人脸表情识别技术简介人脸表情识别技术是一种基于人脸图像或视频数据的分析技术,旨在识别和分析人脸表情。
通过识别和解析人脸上的表情信息,可以了解人的情感状态、心理体验以及内心变化。
人脸表情识别技术常用的方法包括面部特征提取、表情分类模型构建等。
二、心理学研究中的应用案例1. 情绪识别人脸表情识别技术在心理学研究中最常见的应用是情绪识别。
通过分析人脸表情,可以准确地识别出被试者的情绪状态,如愤怒、快乐、悲伤等。
研究人员可以使用情绪分类模型对人脸图像进行分析和分类,并借助数据分析方法揭示个体或群体在不同情绪状态下的行为特征和心理变化。
2. 人际交互研究人脸表情识别技术也可以应用于人际交互研究中。
通过分析人脸表情的变化,可以了解人在不同的社交环境下的表情表达和交际行为。
例如,研究人员可以观察人脸表情在面对不同情境(如社交互动、竞争等)时的变化,进而探索人际交互的模式和规律。
3. 健康心理学研究人脸表情识别技术在健康心理学研究中也有广泛的应用。
通过分析人脸表情,可以有效地评估个体的精神健康状态,如焦虑、抑郁等。
同时,该技术还可用于研究人脸表情与各种心理障碍的关联,从而为临床治疗提供有力的依据。
三、人脸表情识别技术在心理学研究中的应用教程1. 数据收集和处理在运用人脸表情识别技术进行心理学研究时,首先需要收集和处理相关的人脸图像或视频数据。
可以通过实验室拍摄、采集现有的数据集或借助专门的身份识别设备进行数据采集。
然后,利用图像处理技术进行图像预处理和面部特征提取,以得到准确的人脸表情数据。
2. 表情分类模型构建为了准确识别和分类人脸表情,需要构建表情分类模型。
表情识别技术的应用前景

表情识别技术的应用前景随着信息技术的飞速发展,人工智能、机器学习等技术正在逐渐应用到各行各业。
表情识别技术也是其中之一。
表情识别技术是指通过对人脸表情进行分析,提取出人脸表情中所包含的情感信息,从而达到“看懂”人脸的效果。
表情识别技术的应用前景十分广泛,下面,我们就来一起探讨一下。
一、智能家居智能家居是目前较为流行的一种家居模式,其本质上是一种基于物联网技术和智能家居设备的系统,能够让我们更加便捷、智能地控制家庭中的各项事务。
表情识别技术可以在智能家居中扮演一个重要角色。
举个例子,我们可以安装摄像头和表情识别设备,让其能够识别出家庭成员的表情,从而推断出情绪,从而更好地安排家庭任务,包括洗衣、洗碗、做饭等,从而提高我们的生活品质。
二、智能保安现今智能保安行业也在日益发展。
表情识别技术可以在保安行业中发挥很大的作用。
因为保安人员往往需要通过人脸来判断来客人物的身份,而人脸的识别率并不是很高,经常会出现误判,从而带来安全隐患。
而表情识别技术则可以通过对人脸表情进行分析,直观地了解客人的情绪和动作,从而更好地进行判断,提高保安行业的效率。
同时,表情识别技术也可以用于监控区域内活动的人员,来判断其身份信息,以达到更好的监控效果。
三、医疗领域在医疗领域中,表情识别技术也可以发挥很大的作用。
同样以摄像头为例,我们可以在手术时,通过分析医生面部的表情,来为他们提供更好的辅助服务。
而在患者护理时,也可以通过分析患者面部的表情,来了解患者的感受,更好地调整医疗服务,从而提高治疗效果。
四、游戏行业对于游戏行业来说,表情识别技术也可以用于游戏互动上。
在游戏中我们可以通过分析用户的面部表情,来预判其游戏操作动作,从而更好地进行游戏体验。
而在电子竞技等竞技游戏中,表情识别技术还可以用于判断在玩家和敌人中谁处于更加劣势的情况下,从而给与玩家更好的提示,使得玩家能够更好地进行竞技。
总结:表情识别技术尽管存在一些问题,如如隐私安全、技术先进性不足等,但它的应用前景还是十分广泛的。
- 1、下载文档前请自行甄别文档内容的完整性,平台不提供额外的编辑、内容补充、找答案等附加服务。
- 2、"仅部分预览"的文档,不可在线预览部分如存在完整性等问题,可反馈申请退款(可完整预览的文档不适用该条件!)。
- 3、如文档侵犯您的权益,请联系客服反馈,我们会尽快为您处理(人工客服工作时间:9:00-18:30)。
The Painful Face–Pain Expression Recognition UsingActive Appearance ModelsAhmed Bilal Ashraf∗bilal@Simon Lucey∗slucey@Jeffrey F.Cohn∗†jeffcohn@Tsuhan Chen∗tsuhan@Zara Ambadar†ambadar@Ken Prkachin‡kmprk@unbc.caPatty Solomon§solomon@mcmaster.caBarry-John Theobald¶b.theobald@uea.aABSTRACTPain is typically assessed by patient self-report.Self-reportedpain,however,is difficult to interpret and may be impairedor not even possible,as in young children or the severelyill.Behavioral scientists have identified reliable and validfacial indicators of pain.Until now they required manualmeasurement by highly skilled observers.We developed anapproach that automatically recognizes acute pain.Adultpatients with rotator cuffinjury were video-recorded whilea physiotherapist manipulated their affected and unaffectedshoulder.Skilled observers rated pain expression from thevideo on a5-point Likert-type scale.From these ratings,sequences were categorized as no-pain(rating of0),pain(rating of3,4,or5),and indeterminate(rating of1or2).We explored machine learning approaches for pain-no painclassification.Active Appearance Models(AAM)were usedto decouple shape and appearance parameters from the dig-itized face images.Support vector machines(SVM)wereused with several representations from the ing aleave-one-out procedure,we achieved an equal error rate of19%(hit rate=81%)using canonical appearance and shapefeatures.Thesefindings suggest the feasibility of automaticpain detection from video.e07(a)(b)(c)(d)Figure1:Examples of temporally subsampled sequences.(a)and(c):Pain;(b)and(d):No Painexpressions related to emotion[16,17].While much of this effort has used simulated emotion with little or no head mo-tion,several systems have reported success in facial action recognition in real-world facial behavior,such as people ly-ing or telling the truth,watching movie clips intended to elicit emotion,or engaging in social interaction[3,5,18].In real-world applications and especially in patients experienc-ing acute pain,out-of-plane head motion and rapid changes in head motion and expression are particularly challenging. Extending the approach of[14],we applied machine learn-ing to the task of automatic pain detection in a real-world clinical setting involving patients undergoing assessment for pain.In machine learning,the choice of representation is known to influence recognition performance[10].Both appear-ance and shape representations have been investigated.Ex-amples of appearance based representations are raw pixels and Gaborfilters[1,2].A drawback of appearance based approaches is that they lack shape registration,and thus cannot locate vital expression indicators,such as the eyes, brows,eyelids,and mouth.Shape-based representations,which include Active Shape Models[6]and Active Appearance Models(AAM)[6,15]ad-dress this concern.They decouple shape and appearance and perform well in the task of expression recognition,es-pecially in the context of non-rigid head motion[19].In our previous work[14],we explored various representations derived from AAMs and concluded that this decoupling be-tween shape and appearance was indeed beneficial for action unit recognition.In this paper we extend our work based on AAM representations to pain expression recognition.2.IMAGE DATAImage data were from the UNBC-McMaster Shoulder Pain Expression Archive,which includes video clips for129sub-jects(63male,66female).Each subject was seen in“active”and“passive”conditions.In the active condition,subjects initiated shoulder rotation on their own;in passive,a phys-iotherapist was responsible for the movement.Camera angle for active tests was approximately frontal to start;camera angle for passive tests was approximately70degrees to start. Following each movement,subjects completed a10cm Vi-sual Analog Scale to indicate their level of subjective pain. The scale was presented on paper,with anchors of“no pain”and“worst pain imaginable”.Video of each trial was then rated by an observer with considerable training in the iden-tification of pain expression,using a0(no pain)–5(strong pain)Likert-type scale.Reliability of coding was estab-lished with a second coder,proficient in Facial Action Cod-ing System(F ACS)[11].The Pearson correlation between the observers’ratings on210randomly selected tests was 0.80.Correlation between observer rating and subject self-reported pain on VAS was0.74,for the tests used for recog-nition of pain from no pain.This correlation suggests mod-erate to high concurrent validity for pain intensity.For the current study,we included subjects in the active condition who had one or more pain ratings of both pain(0)and no-pain(3,4,or5).Fifty eight subjects met this initial cri-terion.Of these58,31were excluded for reasons including face out of frame,glasses,facial hair,bad image quality,oc-clusion,and hair in face.Thefinal sample consisted of21 subjects.Videos were captured at a resolution of320x240,out of which the face area spanned an average of approximately140x200(28000)pixels.Sample pain sequences are shown in Figure1.3.AAM SIn this section we briefly describe Active Appearance Mod-els(AAMs).The key dividend of AAMs is that they provide a decoupling between shape and appearance of a face image. Given a pre-defined linear shape model with linear appear-ance variation,AAMs have been demonstrated to produce excellent alignment of the shape model to an unseen im-age containing the object of interest.In general,AAMsfit their shape and appearance components through a gradient descent search,although other optimization methods have been employed with similar results[6].Keyframes within each video sequence were manually labeled,while the re-maining frames were automatically aligned using a gradient-descent AAMfit described in[15].3.1AAM Derived RepresentationsThe shape s of an AAM[6]is described by a2D trian-gulated mesh.In particular,the coordinates of the mesh vertices define the shape s(see row1,column(a),of Fig-ure2for examples of this mesh).These vertex locations correspond to a source appearance image,from which the shape was aligned(see row2,column(a),of Figure2).Since AAMs allow linear shape variation,the shape s can be ex-pressed as a base shape s0plus a linear combination of m shape vectors s i:s=s0+mi=1p i s i(1)where the coefficients p=(p1,...,p m)T are the shape pa-rameters.These shape parameters can typically be divided into similarity parameters p s and object-specific parame-ters p o,such that p T=[p T s,p T o].Similarity parameters are associated with the geometric similarity transform(i.e., translation,rotation and scale).The object-specific parame-ters,are the residual parameters representing geometric vari-ations associated with the actual object shape(e.g.,mouth opening,eyes shutting,etc.).Procrustes alignment[6]is employed to estimate the base shape s0.Once we have estimated the base shape and shape pa-rameters,we can normalize for various variables to achieve different representations as outlined in the following subsec-tions.3.1.1Similarity Normalized Shape,s nAs the name suggests,this representation gives the vertex locations after all similarity variation(translation,rotation and scale)has been removed.The similarity normalized shape s n can be obtained by synthesizing a shape instance of s,using Equation1,that ignores the similarity parameters of p.An example of this similarity normalized mesh can be seen in row1,column(b),of Figure2.3.1.2Similarity Normalized Appearance,a nThis representation contains appearance from which sim-ilarity variation has been removed.Once we have similarity normalized shape s n,as computed in section3.1.1,a sim-ilarity normalized appearance a n can then be synthesized by employing a piece-wise affine warp on each triangle patch appearance in the source image(see row2,column(b),of Figure2)so the appearance contained within s now aligns with the similarity normalized shape s n.3.1.3Shape Normalized Appearance,a0If we can remove all shape variation from an appearance, we’ll get a representation that can be called as shape nor-malized appearance,a0.a0can be synthesized in a similar fashion as a n was computed in section3.1.2,but instead ensuring the appearance contained within s now aligns with the base shape s0.We shall refer to this as the face’s canon-ical appearance(see row2,column(c),of Figure2for an example of this canonical appearance image)a0.3.2FeaturesBased on the AAM derived representations in Section3.1 we define three representations:S-PTS:similarity normalized shape s n representation(see Equation1)of the face and its facial features.Thereare68vertex points in s n for both x−and y−co-ordinates,resulting in a raw136dimensional featurevector.S-APP:similarity normalized appearance a n representa-tion.Due to the number of pixels in a n varying fromimage to image,we apply a mask based on s0so thatthe same number of pixels(approximately27,000)arein a n for each image.C-APP:canonical appearance a0representation where all shape variation has been removed from the sourceappearance except the base shape s0.This results in anapproximately27,000dimensional raw feature vectorbased on the pixel values within s0.The naming convention S-PTS,S-APP,and C-APP will be employed throughout the rest of this paper.4.SVM CLASSIFIERSSupport vector machines(SVMs)have been proven useful in a number of pattern recognition tasks including face and facial action recognition.Because they are binary classifiers, they are well suited to the task of Pain Vs No Pain classifica-tion.SVMs attempt tofind the hyper-plane that maximizes the margin between positive and negative observations for a specified class.A linear SVM classification decision is made for an unlabeled test observation x∗by,w T x∗true≷falseb(2)where w is the vector normal to the separating hyperplane and b is the bias.Both w and b are estimated so that they minimize the structural risk of a train-set,thus avoiding the possibility of overfitting to the training data.Typically,w is not defined explicitly,but through a linear sum of support vectors.As a result SVMs offer additional appeal as they allow for the employment of non-linear combination func-tions through the use of kernel functions,such as the radial basis function(RBF),polynomial and sigmoid kernels.A linear kernel was used in our experiments due to its ability to generalize well to unseen data in many pattern recogni-tion tasks[13].Please refer to[13]for additional information on SVM estimation and kernel selection.Figure 2:Example of AAM Derived Representations (a)Top row :input shape(s),Bottom row :input image,(b)Top row:Similarity Normalized Shape(s n ),Bottom Row:Similarity Normalized Appearance(a n ),(c)Top Row:Base Shape(s 0),Bottom Row:Shape Normalized Appearance(a 0)Frame # 10 Frame # 20 Frame # 29 Frame # 150 Frame # 300(a)0.911.11.21.3(b)Figure 3:Example of Video Sequence Prediction (a)Sample frames from a pain-video sequence with theirframe indices,(b)Scores for individual frames.Points corresponding to the frames shown in (a)are high-lighted.For the example sequence shown,a cumulative score D sequence =T i =1d i =195was computed and compared to the derived equal error threshold of −3(as explained in Section 5.2)to yield an output decision of ‘Pain’5.EXPERIMENTS5.1Pain Model LearningTo ascertain the utility of various AAM representations, different classifiers were trained by using features of Sec-tion3.2in the following combinations:S-PTS:similarity normalized shape s nS-APP:similarity normalized appearance a nC-APP+S-PTS:canonical appearance a0combined with the similarity normalized shape s nTo check for subject generalization,a leave-one-subject-out strategy was employed for cross validation.Thus,there was no overlap of subjects between the training and test-ing set.The number of training frames from all the video sequences was prohibitively large to train an SVM,as the training time complexity for SVM is O(m3),where m is the number of training examples.In order to make the step of model learning practical,while making the best use of training data,each video sequence wasfirst clustered into a preset number of clusters.Standard K-Means clustering algorithm was used,with K set to a value that reduces the training set to a size manageable by SVM.Linear SVM training models were learned by by itera-tively leaving one subject out,which gives rise to N number of models,where N is the number of subjects.Since the ground truth was available at the level of an entire video sequence,all the clusters belonging to a pain containing se-quence were considered as positive(pain)examples,while clusters belonging to‘no pain’sequence were counted as neg-ative(no pain)training examples.5.2Video Sequence PredictionWhile learning was done on clustered video frames,testing was carried out on individual frames.The output for every frame was a score proportional to the distance of test-point from the separating hyperplane.In order to predict a video as a pain sequence or not,the output scores of individual frames were summed together to give a cumulative score for the entire sequence:D sequence=Ti=1d i(3)where d i is the output score for the i th frame and T is the total number of frames in the sequence.Having computed the sequence level cumulative score in Equation3,we seek a decision rule of the form:D sequencepain≷no painT hreshold(4)Using this decision rule,a threshold was set such that false accept rate equaled false reject rate(i.e.,equal error rate point was computed).Output scores for a sample se-quence along with the implementation of decision rule is shown in Figure3.The score values track the pain expres-sion,with a peak response corresponding to frame29shown in Figure3(a).Features Hit Rate0.2899S-APP0.46550.1879Table1:Results of experiments performed in sec-tion 5.Column1indicates the features used for training,Columns2and3represent the correspond-ing Equal-Error and Hit Rates respectively6.RESULTSTable1shows equal-error rates(EER)using each of the representations.It is intuitively satisfying that the results highlight the importance of shape features for pain expres-sion.The best results(EER=0.1879,Hit Rate:0.8121) are for canonical appearance combined with similarity nor-malized shape(C-APP+S-PTS).This result is consistent with our previous work[14],in which we used AAMs for facial action unit recognition.It is surprising,however,that similarity normalized appear-ance features(S-APP)performed at close-to-chance levels despite the fact that this representation can be fully derived from canonical appearance and similarity normalized shape. S-PTS combined with C-APP may add additional dimen-sions that aid infinding an optimal separating hyperplane between two classes.7.DISCUSSIONIn this paper,we have explored various face representa-tions derived from AAMs for recognizing facial expression of pain.We have demonstrated the utility of AAM represen-tations for the task of pain-no pain classification.We have also presented a method to handle large training data when learning SVM models.Some conclusions of our experiments are:•Use of concatenated similarity normalized shape andshape normalized appearance(S-PTS+C-APP)is su-perior to either similarity normalized shape(S-PTS)orsimilarity normalized appearance(S-APP)alone.•Shape features have an important role to play in ex-pression recognition generally,and pain detection par-ticularly.•Automatic pain detection through video appears tobe a feasible task.Thisfinding suggests an oppor-tunity to build interactive systems to detect pain indiverse populations,including infants and speech im-paired adults.Additional applications for system de-velopment include pain-triggered automatic drug dis-pensation and discriminating between feigned and realpain.•Two limitations may be noted.One was the exclu-sion of subjects wearing glasses or having facial hair,which limits generalizability.A second limitation wasthe availability of ground-truth at the level of videorecorded tests instead of the level of individual frames.Not all frames in a pain sequence actually correspondto pain.Providing a frame-level ground-truth couldassist the model-learning step and thus improve recog-nition performance.This limitation can be overcomeeither by expert labeling of frames or by automatic re-finement of ground-truth by exploiting the information embedded in no-pain video sequences.Incorporating temporal information is also expected to positively in-fluence the performance.Based on our initialfindings, further improvements on above lines can pave way to-wards developing automatic pain detection tools for clinical use.8.ACKNOWLEDGMENTSThis research was supported by CIHR grant MOP77799 and NIMH grant MH514359.REFERENCES[1]M.Bartlett,G.Littlewort,inscesk,I.Fasel,andJ.Movellan.Machine learning methods for fullyautomatic recognition of facial expressions and facialactions.IEEE International Conference on Systems,Man and Cybernetics,pages592–597,October2004.[2]M.S.Bartlett,G.Littlewort,M.Frank,inscesk,I.Fasel,and J.Movellan.Recognizing facialexpression:Machine learning and application tospontaneous behavior.IEEE Conference on Computer Vision and Pattern Recognition(CVPR),2:568–573,June2005.[3]M.S.Bartlett,G.Littlewort,M.Frank,inscsek,I.Fasel,and J.Movellan.Fully automatic facial actionrecognition in spontaneous behavior.Proceedings ofthe IEEE International Conference on Automatic Face and Gesture Recognition,pages223–228,2006.[4]J.F.Cohn,Z.Ambadar,and P.Ekman.Observer-based measurement of facial expression with the facial action coding system.In J.A.Coan andJ.B.Allen,editors,The handbook of emotionelicitation and assessment.Oxford University PressSeries in Affective Science,pages203–221.OxfordUniversity Press,New York,NY,2007.[5]J.F.Cohn and K.L.Schmidt.The timing of facialmotion in posed and spontaneous smiles.International Journal of Wavelets,Multiresolution and Information Processing,2:1–12,2004.[6]T.Cootes,G.Edwards,and C.Taylor.Activeappearance models.PAMI,23(6):681–685,2001. [7]R.R.Cornelius.The science of emotion.PrenticeHall,Upper Saddler River,New Jersey,1996.[8]K.D.Craig,K.M.Prkachin,and R.V.E.Grunau.The facial expression of pain.In D.C.Turk andR.M.,editors,Handbook of pain assessment.Guilford, New York,2nd edition,2001.[9]A.C.d.C.Williams,H.T.O.Davies,andY.Chadury.Simple pain rating scales hide complexidiosyncratic meanings.Pain,85:457–463.[10]R.O.Duda,P.E.Hart,and D.G.Stork.PatternClassification.John Wiley and Sons,Inc.,New York, NY,USA,2nd edition,2001.[11]P.Ekman,W.V.Friesen,and J.C.hager.Facialaction coding system:Research workResearch Information,Salt Lake City,UT,2002. [12]T.Hadjistavropoulos and K.D.Craig.Socialinfluences and the communication of pain.In Pain:Psychological perspectives,pages87–112.Erlbaum,New York,2004.[13]C.W.Hsu,C.C.Chang,and C.J.Lin.A practicalguide to support vector classification.TechnicalReport,2005.[14]S.Lucey,A.B.Ashraf,and J.Cohn.Investigatingspontaneous facial action recognition through AAMrepresentations of the face.In K.Kurihara,editor,Face Recognition Book.Pro Literatur Verlag,Mammendorf,Germany,April2007.[15]I.Matthews and S.Baker.Active appearance modelsrevisited.IJCV,60(2):135–164,2004.[16]M.Pantic,A.Pentland,A.Niholt,and T.S.Huang.Human computing and machine understanding ofhuman behavior:A survey.Proceedings of the ACMInternational Conference on Multimodal Interfaces,2006.[17]Y.Tian,J.F.Cohn,and T.Kanade.Facial expressionanalysis.In S.Z.Li and A.K.Jain,editors,Handbook of face recognition,pages247–276.Springer,NewYork,NY,2005.[18]M.F.Valstar,M.Pantic,Z.Ambadar,and J.F.Cohn.Spontaneous vs.posed facial behavior:Automatic analysis of brow actions.Proceedings of the ACM International Conference on MultimodalInterfaces,pages162–170,November2006.[19]J.Xiao,S.Baker,I.Matthews,and T.Kanade.(Inpress).2d vs.3d deformable face models:Representational power,construction,and real-timefitting.International Journal of Computer Vision,Springer Science.。