外文翻译---在遥感和地理信息系统的规模度量
地理信息系统与遥感技术在测绘中的融合与应用研究
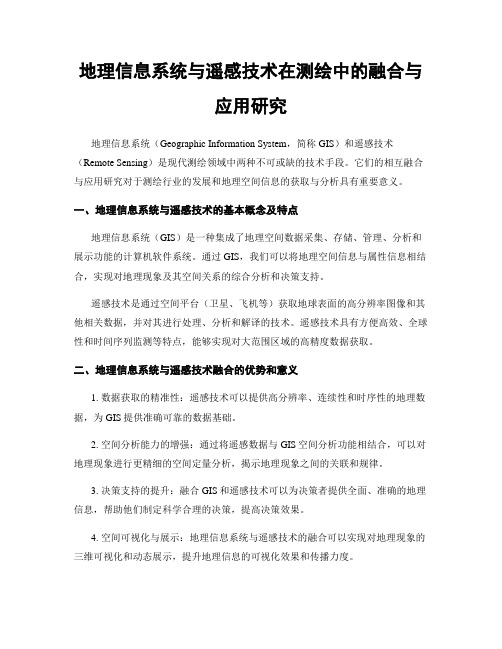
地理信息系统与遥感技术在测绘中的融合与应用研究地理信息系统(Geographic Information System,简称GIS)和遥感技术(Remote Sensing)是现代测绘领域中两种不可或缺的技术手段。
它们的相互融合与应用研究对于测绘行业的发展和地理空间信息的获取与分析具有重要意义。
一、地理信息系统与遥感技术的基本概念及特点地理信息系统(GIS)是一种集成了地理空间数据采集、存储、管理、分析和展示功能的计算机软件系统。
通过GIS,我们可以将地理空间信息与属性信息相结合,实现对地理现象及其空间关系的综合分析和决策支持。
遥感技术是通过空间平台(卫星、飞机等)获取地球表面的高分辨率图像和其他相关数据,并对其进行处理、分析和解译的技术。
遥感技术具有方便高效、全球性和时间序列监测等特点,能够实现对大范围区域的高精度数据获取。
二、地理信息系统与遥感技术融合的优势和意义1. 数据获取的精准性:遥感技术可以提供高分辨率、连续性和时序性的地理数据,为GIS提供准确可靠的数据基础。
2. 空间分析能力的增强:通过将遥感数据与GIS空间分析功能相结合,可以对地理现象进行更精细的空间定量分析,揭示地理现象之间的关联和规律。
3. 决策支持的提升:融合GIS和遥感技术可以为决策者提供全面、准确的地理信息,帮助他们制定科学合理的决策,提高决策效果。
4. 空间可视化与展示:地理信息系统与遥感技术的融合可以实现对地理现象的三维可视化和动态展示,提升地理信息的可视化效果和传播力度。
三、地理信息系统与遥感技术融合的应用案例1. 土地利用与覆盖变化监测:通过遥感技术获取地表覆盖信息,并利用GIS进行土地利用与覆盖变化的监测和分析,帮助决策者及时了解土地利用变化情况,制定适应性政策。
2. 自然灾害监测与应急响应:利用遥感技术实时获取自然灾害现场的图像与数据,结合GIS进行空间分析,及时评估灾害损失并提供决策支持,加强灾害应急响应能力。
毕业论文外文翻译--在遥感和地理信息系统的规模度量(适用于毕业论文外文翻译+中英文对照)
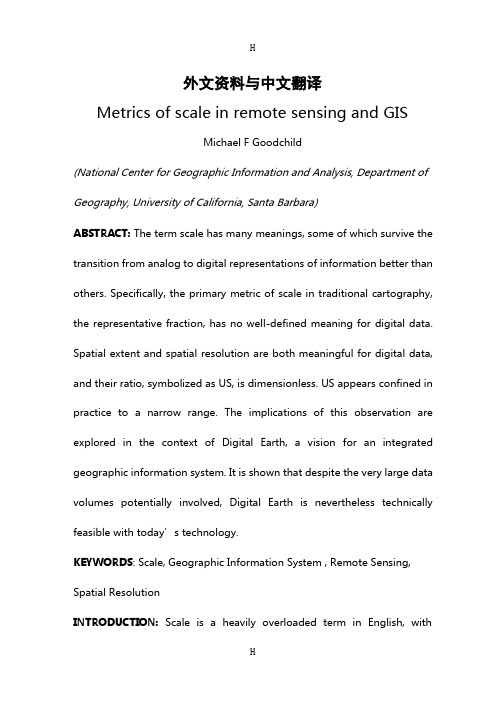
外文资料与中文翻译Metrics of scale in remote sensing and GISMichael F Goodchild(National Center for Geographic Information and Analysis, Department of Geography, University of California, Santa Barbara)ABSTRACT: The term scale has many meanings, some of which survive the transition from analog to digital representations of information better than others. Specifically, the primary metric of scale in traditional cartography, the representative fraction, has no well-defined meaning for digital data. Spatial extent and spatial resolution are both meaningful for digital data, and their ratio, symbolized as US, is dimensionless. US appears confined in practice to a narrow range. The implications of this observation are explored in the context of Digital Earth, a vision for an integrated geographic information system. It is shown that despite the very large data volumes potentially involved, Digital Earth is nevertheless technically feasible with today’s technology.KEYWORDS: Scale, Geographic Information System , Remote Sensing, Spatial ResolutionINTRODUCTION: Scale is a heavily overloaded term in English, withabundant definitions attributable to many different and often independent roots, such that meaning is strongly dependent on context. Its meanings in “the scales of justice” or “scales over ones eyes” have little connection to each other, or to its meaning in a discussion of remote sensing and GIS. But meaning is often ambiguous even in that latter context. For example, scale to a cartographermost likely relates to the representative fraction, or the scaling ratio between the real world and a map representation on a flat, two-dimensional surface such as paper, whereas scale to an environmental scientist likely relates either to spatial resolution (the representati on’s level of spatial detail) or to spatial extent (the representation’s spatial coverage). As a result, a simple phrase like “large scale” can send quite the wrong message when communities and disciplines interact - to a cartographer it implies fine detail, whereas to an environmental scientist it implies coarse detail. A computer scientist might say that in this respect the two disciplines were not interoperable.In this paper I examine the current meanings of scale, with particular reference to the digital world, and the metrics associated with each meaning. The concern throughout is with spatial meanings, although temporal and spectral meanings are also important. I suggest that certain metrics survive the transition to digital technology better than others.The main purpose of this paper is to propose a dimensionless ratio of two such metrics that appears to have interesting and useful properties. I show how this ratio is relevant to a specific vision for the future of geographic information technologies termed Digital Earth. Finally, I discusshow scale might be defined in ways that are accessible to a much wider range of users than cartographers and environmental scientists.FOUR MEANINGS OF SCALE LEVEL OF SPATIAL DETAIL REPRESENTATIVE FRACTIONA paper map is an analog representation of geographic variation, rather than a digital representation. All features on the Earth’s surface are scaled using an approximately uniform ratio known as the representative fraction (it is impossible to use a perfectly uniform ratio because of the curvature of the Earth’s surface). The power of the representative fraction stems from the many different properties that are related to it in mapping practice. First, paper maps impose an effective limit on the positional accuracy of features, because of instability in the material used to make maps, limited ability to control the location of the pen as the map is drawn, and many other practical considerations. Because positional accuracy on the map is limited, effective positional accuracy on the ground is determined by the representative fraction. A typical (and comparatively generous) map accuracy standard is 0.5 mm, and thus positional accuracy is 0.5 mm divided by the representative fraction (eg, 12.5 m for a map at 1:25,000). Second, practical limits on the widths of lines and the sizes of symbols create a similar link between spatial resolution and representative fraction: it is difficult to show features much less than 0.5 mm across withadequate clarity. Finally, representative fraction serves as a surrogate for the features depicted on maps, in part because of this limit to spatial resolution, and in part because of the formal specifications adopted by mapping agencies, that are in turn related to spatial resolution. In summary, representative fraction characterizes many important properties of paper maps.In the digital world these multiple associations are not necessarily linked. Features can be represented as points or lines, so the physical limitations to the minimum sizes of symbols that are characteristic of paper maps no longer apply. For example, a database may contain some features associated with 1:25,000 map specifications, but not all; and may include representations of features smaller than 12.5 m on the ground. Positional accuracy is also no longer necessarily tied to representative fraction, since points can be located to any precision, up to the limits imposed by internal representations of numbers (eg,single precision is limited to roughly 7 significant digits, double precision to 15). Thus the three properties that were conveniently summarized by representative fraction - positional accuracy, spatial resolution, and feature content - are now potentially independent.Unfortunately this has led to a complex system of conventions in an effort to preserve representative fraction as a universal defining characteristic of digital databases. When such databases are created directly from paper maps, by digitizing or scanning, it is possible for all three properties to remain correlated. But in other cases the representative fraction cited for a digital database is the one implied by its positional accuracy (eg, a database has representative fraction 1: 12,000 because its positional accuracy is 6 m); and in other cases it is the feature content or spatial resolution that defines the conventional representative fraction (eg, a database has representative fraction 1:12,000 because features at least 6 m across are included). Moreover, these conventions are typically not understood by novice users - the general public, or children - who may consequently be very confused by the use of a fraction to characterize spatial data, despite its familiarity to specialists.SPATIAL EXTENTThe term scale is often used to refer to the extent or scope of a study or project, and spatial extent is an obvious metric. It can be defined in area measure, but for the purposes of this discussion a length measure is preferred, and the symbolL will be used. For a square project area it can be set to the width of the area, but for rectangular or oddly shaped project areas the square root of area provides a convenient metric. Spatial extent defines the total amount of information relevant to a project, which rises with the square of a length measure.PROCESS SCALEThe term process refers here to a computational model or representation of a landscape-modifying process, such as erosion or runoff. From a computational perspective,a process is a transformation that takes a landscape from its existing state to some new state, and in this sense processes are a subset of the entire range of transformations that can be applied to spatial data.Define a process as a mapping b (x ,2t )=f ( a (x ,1t )) where a is a vector of input fields, b is a vector of output fields, f is a function, t is time, 2t is later in time than 1t , and x denotes location. Processes vary according to how they modify the spatial characteristics of their inputs, and these are best expressed in terms of contributions to the spatial spectrum. For example, some processes determine b(x, ,2t ) based only on the inputs at the same location a(x, 1t ), and thus have minimal effect on spatial spectra.Other processes produce outputs that are smoother than their inputs, through processes of averaging or convolution,and thus act as low-pass filters. Less commonly, processes produce outputs that are more rugged than their inputs, by sharpening rather than smoothing gradients, and thus act as high-pass filters.The scale of a process can be defined by examining the effects of spectral components on outputs. If some wavelength s exists such that components with wavelengths shorter than s have negligible influence on outputs, then the process is said to have a scale of s. It follows that if s is less than the spatial resolution S of the input data, the process will not be accurately modeled.While these conclusions have been expressed in terms of spectra, it is also possible to interpret them in terms of variograms and correlograms. A low-pass filter reduces variance over short distances, relative to variance over long distances. Thus the short-distance part of the variogram is lowered, and the short-distance part of the correlogram is increased. Similarly a high-pass filter increases variance over short distances relative to variance over long distances.L/S RATIOWhile scaling ratios make sense for analog representations, the representative fraction is clearly problematic for digital representations.But spatial resolution and spatial extent both appear to be meaningful in both analog and digital contexts, despite the problems with spatial resolution for vector data. Both Sand L have dimensions of length, so their ratio is dimensionless. Dimensionless ratios often play a fundamental role in science (eg, theReynolds number in hydrodynamics), so it is possible that L/S might play a fundamental role in geographic information science. In this section I examine some instances of the L/S ratio, and possible interpretations that provide support for this speculation.- Today’s computing industry seems to have settled on a screen standard of order 1 megapel, or 1 million picture elements. The first PCs had much coarser resolutions (eg, the CGA standard of the early 198Os), but improvements in display technology led to a series of more and more detailed standards. Today, however, there is little evidence of pressure to improve resolution further, and the industry seems to be content with an L/S ratio of order 103. Similar ratios characterize the current digital camera industry, although professional systems can be found with ratios as high as 4,000.- Remote sensing instruments use a range of spatial resolutions, from the 1 m of IKONOS to the 1 km of AVHRR. Because a complete coverage of the Earth’s surface at 1 m requires on the order of 1015 pixels, data are commonly handled in more manageable tiles, or approximately rectangular arrays of cells. For years, Landsat TM imagery has been tiled in arrays of approximately 3,000 cells x 3,000 cells, for anL/S ratio of 3,000.- The value of S for a paper map is determined by the technology of map-making, and techniques of symbolization, and a value of 0.5 mm is not atypical. A map sheet 1 m across thus achieves an L/S ratio of 2,000.- Finally, the human eye’s S can be defined as the size of a retinal cell, and the typical eye has order 108 retinal cells, implying an L/S ratio of 10,000. Interestingly, then, the screen resolution that users find generally satisfactory corresponds approximately to the parameters of the human visual system; it is somewhat larger, but the computer screen typically fills only a part of the visual field.These examples suggest that L/S ratios of between 103 and 104 are found across a wide range of technologies and settings, including the human eye. Two alternative explanations immediately suggest themselves: the narrow range may be the result of technological and economic constraints, and thus may expand as technology advances and becomes cheaper; or it may be due to cognitive constraints, and thus is likely to persist despite technological change.This tension between technological, economic, and cognitive constraints is well illustrated by the case of paper maps, which evolved under what from today’s perspective were severe technological and economic constraints. For example, there are limits to the stability of paper and to the kinds of markings that can be made by hand-held pens. The costs of printing drop dramatically with the number of copies printed, because of strong economies of scale in the printing process, so maps must satisfy many users to be economically feasible. Goodchild [2000]has elaborated on these arguments. At the same time, maps serve cognitive purposes, and must be designed to convey information as effectively as possible. Any aspect of map design and production can thus be given two alternative interpretations: one, that it results from technological and economic constraints, and the other, that it results from the satisfaction of cognitive objectives. If the former is true, then changes in technology may lead to changes in design and production; but if the latter is true, changes in technology may have no impact.The persistent narrow range of L/S from paper maps to digital databases to the human eye suggests an interesting speculation: That cognitive, not technological or economic objectives, confine L/S to thisrange. From this perspective,L/S ratios of more than 104 have no additional cognitive value, while L/S ratios of less than 103 are perceived as too coarse for most purposes. If this speculation is true, it leads to some useful and general conclusions about the design of geographic information handling systems. In the next section I illustrate this by examining the concept of Digital Earth. For simplicity, the discussion centers on the log to base 10 of the L/S ratio, denoted by log L/S, and the speculation that its effective range is between 3 and 4.This speculation also suggests a simple explanation for the fact that scale is used to refer both to L and to S in environmental science, without hopelessly confusing the listener. At first sight it seems counter~ntuitive that the same term should be used for two independent properties. But if the value of log L/S is effectively fixed, then spatial resolution and extent are strongly correlated: a coarse spatial resolution implies a large extent, and a detailed spatial resolution implies a small extent. If so, then the same term is able to satisfy both needs.THE VISION OF DIGITAL EARTHThe term Digital Earth was coined in 1992 by U.S. Vice President Al Gore [Gore, 19921, but it was in a speech written for delivery in 1998 thatGore fully elaborated the concept (www.d~~Pl9980131 .html): “Imagine, for example, a young child going to a Digital Earth exhibit at a local museum. After donning a headmounted display, she sees Earth as it appears from space. Using a data glove, she zooms in, using higher and higherlevels of resolution, to see continents, then regions, countries, cities, and finally individual houses, trees, and other natural and man-made objects. Having found an area of the planet she is interested in exploring, she takes the equivalent of a ‘magic carpet ride’ through a 3- D visualization of the terrain.”This vision of Digital Earth (DE) is a sophisticated graphics system, linked to a comprehensive database containing representations of many classes of phenomena. It implies specialized hardware in the form of an immersive environment (a head-mounted display), with software capable of rendering the Earth’s surface at high speed, and from any perspective. Its spatial resolution ranges down to 1 m or finer. On the face of it, then, the vision suggests data requirements and bandwidths that are well beyond today’s capabilities. If each pixel of a 1 m resolution representation of the Earth’s surface was allocated an average of 1 byte then a total of 1 Pb of storage would be required; storage of multiple themes could push this total much higher. In order to zoom smoothly down to 1 m it would be necessary to store the data in a consistent data structure that could be accessed at many levels of resolution. Many data types are not obviously renderable (eg, health, demographic, andeconomic data), suggesting a need for extensive research on visual representation.The bandwidth requirements of the vision are perhaps the most daunting problem. To send 1 Pb of data at 1 Mb per second would take roughly a human life time, and over 12,000 years at 56 Kbps. Such requirements dwarf those of speech and even full-motion video. But these calculations assume that the DE user would want to see the entire Earth at Im resolution. The previ ous analysis of log L/S suggested that for cognitive (and possibly technological and economic) reasons user requirements rarely stray outside the range of 3 to 4, whereas a full Earth at 1 m resolution implies a log L/S of approximately 7. A log L/S of 3 suggests that a user interested in the entire Earth would be satisfied with 10 km resolution; a user interested in California might expect 1 km resolution; and a user interested in Santa Barbara County might expect 100 m resolution. Moreover, these resolutions need apply only to the center of the current field of view.On this basis the bandwidth requirements of DE become much more manageable. Assuming an average of 1 byte per pixel, a megapel image requires order 107 bps ifrefreshed once per second. Every one-unit reduction in log L/S results in two orders of magnitude reduction in bandwidth requirements. Thus a Tl connection seems sufficient to support DE, based on reasonable expectations about compression, and reasonable refresh rates. On this basis DE appears to be feasible with today’s communication technology. CONCLUDING COMMENTSI have argued that scale has many meanings, only some of which are well defined for digital data, and therefore useful in the digital world in which we increasingly find ourselves. The practice of establishing conventions which allow the measures of an earlier technology - the paper map - to survive in the digital world is appropriate for specialists, but is likely to make it impossible for non-specialists to identify their needs. Instead, I suggest that two measures, identified here as the large measure L and the small measure S, be used to characterize the scale properties of geographic data.The vector-based representations do not suggest simple bases for defining 5, because their spatial resolutions are either variable or arbitrary. On the other hand spatialvariat;on in S makes good sense in many situations. In social applications, itappears that the processes that characterize human behavior are capable of operating at different scales, depending on whether people act in the intensive pedestrian-oriented spaces of the inner city or the extensive car-oriented spaces of the suburbs. In environmental applications, variation in apparent spatial resolution may be a logical sampling response to a phenomenonthat is known to have more rapid variation in some areas than others; from a geostatistical perspective this might suggest a non-stationary variogram or correlogram (for examples of non-statjonary geostatistical analysis see Atkinson [2001]). This may be one factor in the spatial distribution of weather observation networks (though others, such as uneven accessibility, and uneven need for information are also clearly important).The primary purpose of this paper has been to offer a speculation on the significance of the dimensionless ratio L/S. The ratio is the major determinant of data volume, and consequently processing speed, in digital systems. It also has cognitive significance because it can be defined for the human visual system. I suggest that there are few reasons in practice why log L/S should fall outside the range 3 - 4, and that this provides an important basis for designing systems for handling geographic data. Digital Earth was introduced as one such system. A constrained ratio also implies that L and S are strongly correlated in practice, as suggested by the common use of the same term scale to refer to both.ACKNOWLEDGMENTThe Alexandria Digital Library and its Alexandria Digital Earth Prototype, the source of much of the inspiration for this paper, are supported by the U.S. National Science Foundation.REFERENCESAtkinson, P.M., 2001. Geographical information science: Geocomputation and nonstationarity. Progress in Physical Geography 25(l): 111-122. Goodchild, M F 2000 Communicating geographic information in a digital age. Annals of the Association of American Geographers 90(2): 344-355.Goodchild, M.F. & J. Proctor, 1997. Scale in a digital geographic world.Geographical and Environmental Modelling l(1): 5-23.Gore, A., 1992. Earth in the Balance: Ecology and the Human Spirit.Houghton Mifflin, Boston, 407~~.Lam, N-S & D. Quattrochi, 1992. On the issues of scale, resolution, and fractal analysis in the mapping sciences. Professional Geographer 44(l): 88-98.Quattrochi D.A & M.F. Goodchild (Eds), 1997. Scale in Remote Sensing and GIS. Lewis Publishers, Boca Raton, 406~~.中文翻译:在遥感和地理信息系统的规模度量迈克尔·F古德柴尔德(美国国家地理信息和分析中心,加州大学圣巴巴拉分校地理系)摘要:长期的规模有多种含义,其中一些生存了从模拟到数字表示的信息比别人更好的过渡。
地理信息系统与遥感技术

地理信息系统与遥感技术地理信息系统(Geographic Information System,简称GIS)和遥感技术(Remote Sensing)是现代地理科学中的两个重要分支,它们在地理研究、资源管理、环境保护等领域发挥着重要作用。
本文将介绍地理信息系统和遥感技术的基本概念、应用领域以及未来发展趋势。
一、地理信息系统地理信息系统是一种将地理空间数据与属性数据相结合的信息处理系统。
它通过采集、存储、管理、分析和展示地理数据,帮助人们更好地理解和利用地理信息。
地理信息系统由硬件、软件、数据和人员组成,其中最核心的是地理信息系统软件,它提供了数据输入、编辑、查询、分析和输出等功能。
地理信息系统的应用非常广泛。
在城市规划中,地理信息系统可以帮助规划师分析土地利用、交通流量、人口分布等数据,为城市规划提供科学依据。
在环境保护中,地理信息系统可以监测和分析空气质量、水质状况、土壤侵蚀等环境指标,为环境管理和保护提供支持。
在农业生产中,地理信息系统可以帮助农民进行土壤肥力评估、农作物生长监测等工作,提高农业生产效益。
未来,地理信息系统将继续发展壮大。
随着卫星遥感技术的不断进步,地理信息系统将能够获取更高分辨率、更全面的地理数据,为各个领域的决策提供更准确的支持。
同时,地理信息系统与人工智能、大数据等新兴技术的结合也将带来更多的创新应用。
二、遥感技术遥感技术是利用航空器、卫星等远距离感知设备获取地球表面信息的一种技术。
遥感技术可以获取地表的光谱、热红外、雷达等多种信息,通过对这些信息的处理和分析,可以获取地表的地形、植被、土壤、水体等特征。
遥感技术的应用非常广泛。
在地质勘探中,遥感技术可以帮助寻找矿产资源、识别地质构造等。
在灾害监测中,遥感技术可以实时监测地震、火山喷发、洪水等自然灾害,提供及时的预警和救援信息。
在农业生产中,遥感技术可以监测农作物的生长情况、土壤湿度等指标,为农民提供农业管理的科学依据。
遥感及其相关术语中英文对照
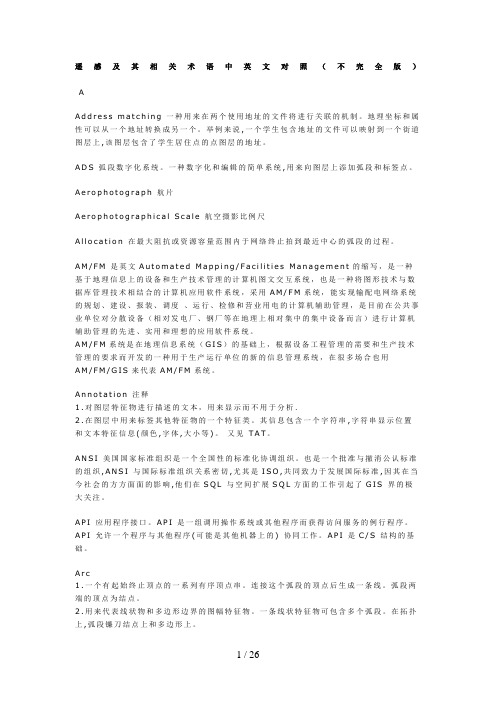
遥感及其相关术语中英文对照(不完全版)AA d d r e s s m a t c h i n g一种用来在两个使用地址的文件将进行关联的机制。
地理坐标和属性可以从一个地址转换成另一个。
举例来说,一个学生包含地址的文件可以映射到一个街道图层上,该图层包含了学生居住点的点图层的地址。
A D S弧段数字化系统。
一种数字化和编辑的简单系统,用来向图层上添加弧段和标签点。
A e r o p h o t o g r a p h航片A e r o p h o t o g r a p h i c a l S c a l e航空摄影比例尺A l l o c a t i o n在最大阻抗或资源容量范围内于网络终止拍到最近中心的弧段的过程。
A M/F M是英文A u t o m a t e d M a p p i n g/F a c i l i t i e s M a n a g e m e n t的缩写,是一种基于地理信息上的设备和生产技术管理的计算机图文交互系统,也是一种将图形技术与数据库管理技术相结合的计算机应用软件系统,采用A M/F M系统,能实现输配电网络系统的规划、建设、报装、调度、运行、检修和营业用电的计算机辅助管理,是目前在公共事业单位对分散设备(相对发电厂、钢厂等在地理上相对集中的集中设备而言)进行计算机辅助管理的先进、实用和理想的应用软件系统。
A M/F M系统是在地理信息系统(G I S)的基础上,根据设备工程管理的需要和生产技术管理的要求而开发的一种用于生产运行单位的新的信息管理系统,在很多场合也用A M/F M/G I S来代表A M/F M系统。
A n n o t a t i o n注释1.对图层特征物进行描述的文本,用来显示而不用于分析.2.在图层中用来标签其他特征物的一个特征类。
其信息包含一个字符串,字符串显示位置和文本特征信息(颜色,字体,大小等)。
又见T A T。
A N S I美国国家标准组织是一个全国性的标准化协调组织。
地理信息系统(GIS)与遥感(RS)的区别与联系

地理信息系统(GIS)与遥感(RS)的区别与联系内容提要:本文介绍了地理信息系统(GIS)与遥感(RS)的区别与联系。
关键词:GIS、RS、区别、联系引言:地理信息系统作为一种新的科学已经逐渐被人们重视起来。
人们发现在短短的几十年中,它所带来的经济价值十分可观。
它涉及的领域很多,在不久的将来各行各业也许都要利用这一专业的的强大功能。
它在遥感中的初步应用已经让我们吃惊,希望随着时代的发展,它会给我们的遥感产业带来伟大的改革。
一、地理信息系统(GIS)的概念地理信息系统(GIS)或称为地学信息系统、资源与环境信息系统。
它是在计算机软硬件、软件系统的支持下,对整个或部分地球表层(包括大气层)空间中的有关地理分布数据进行采集、储存、管理、运算、分析、显示和描述以及辅助决策的技术系统。
地理信息系统处理、管理的对象是多种地理空间实体数据及其关系,包括空间定位数据、图形数据、遥感图像数据、数据数据等,用于分析和处理在一定地理区域内分布的各种现象和过程,解决复杂的规划、决策和管理问题。
二、遥感(RS)的概念遥感的科学定义就是从远处采集信息,即不直接接触物体,从远处通过探测仪器接收来自目标地物的电磁波信息,经过对信息的处理,识别地物。
而广义遥感是泛指一切无接触的远距离探测。
三、GIS与R S的联系和区别地理信息系统和遥感是两个相互独立发展起来的技术领域,但它们存在着密切的关系,一方面,遥感信息是地理信息系统中重要的信息源;另一方面,遥感调查中需要利用地理信息系统中的辅助数据(包括各种地图、地面实测数据、统计资料等)来改善遥感数据的分类精度和制图精度。
GIS 是地理学、测量学、地图学、遥感等与计算机科学相结合发展起来的一门新的边缘学科。
在这些相关学科、技术中,测量和遥感主要从数据源的角度为GIS 服务,而地理学和地图学是GIS 应用所关注的主要领域。
早期的GIS 系统主要以地图制图为目标,地理分析功能极为简单,更接近一个机助地图制图系统。
地理信息系统与遥感应用
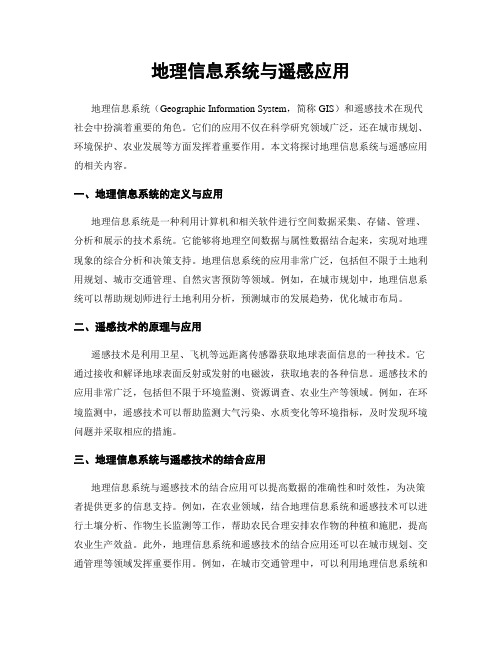
地理信息系统与遥感应用地理信息系统(Geographic Information System,简称GIS)和遥感技术在现代社会中扮演着重要的角色。
它们的应用不仅在科学研究领域广泛,还在城市规划、环境保护、农业发展等方面发挥着重要作用。
本文将探讨地理信息系统与遥感应用的相关内容。
一、地理信息系统的定义与应用地理信息系统是一种利用计算机和相关软件进行空间数据采集、存储、管理、分析和展示的技术系统。
它能够将地理空间数据与属性数据结合起来,实现对地理现象的综合分析和决策支持。
地理信息系统的应用非常广泛,包括但不限于土地利用规划、城市交通管理、自然灾害预防等领域。
例如,在城市规划中,地理信息系统可以帮助规划师进行土地利用分析,预测城市的发展趋势,优化城市布局。
二、遥感技术的原理与应用遥感技术是利用卫星、飞机等远距离传感器获取地球表面信息的一种技术。
它通过接收和解译地球表面反射或发射的电磁波,获取地表的各种信息。
遥感技术的应用非常广泛,包括但不限于环境监测、资源调查、农业生产等领域。
例如,在环境监测中,遥感技术可以帮助监测大气污染、水质变化等环境指标,及时发现环境问题并采取相应的措施。
三、地理信息系统与遥感技术的结合应用地理信息系统与遥感技术的结合应用可以提高数据的准确性和时效性,为决策者提供更多的信息支持。
例如,在农业领域,结合地理信息系统和遥感技术可以进行土壤分析、作物生长监测等工作,帮助农民合理安排农作物的种植和施肥,提高农业生产效益。
此外,地理信息系统和遥感技术的结合应用还可以在城市规划、交通管理等领域发挥重要作用。
例如,在城市交通管理中,可以利用地理信息系统和遥感技术进行交通流量监测、交通拥堵分析等工作,帮助交通部门优化交通路线和减少交通拥堵。
总结:地理信息系统和遥感技术是现代社会中不可或缺的工具。
它们的应用不仅在科学研究领域广泛,还在城市规划、环境保护、农业发展等方面发挥着重要作用。
通过地理信息系统和遥感技术的结合应用,我们可以更好地了解和管理地球上的各种资源和环境,为社会的可持续发展提供有力支持。
地理信息系统与遥感技术

地理信息系统与遥感技术地理信息系统(Geographic Information System,简称GIS)和遥感技术在现代社会中起着重要的作用。
GIS是数字化地图、空间数据库和地理信息分析技术的综合体系,而遥感技术是通过航空或卫星等手段获取地球表面的图像和数据。
本文将探讨地理信息系统与遥感技术的基本概念、运作原理以及应用领域。
一、地理信息系统(GIS)的概念与原理地理信息系统是一个将地理学、计算机科学和信息技术相结合的综合学科。
它以地理空间数据为基础,通过地理信息采集、存储、管理、处理和分析,为用户提供决策支持和空间分析的能力。
GIS的基本原理包括数据输入、数据管理、空间分析和结果展示等。
1. 数据输入:GIS通过地理信息采集设备,如GPS(全球定位系统)、RS(遥感)、测绘仪器等,采集地理空间数据并进行输入。
这些数据包括地图、卫星影像、地形、气象、人口等,形成丰富的空间数据库。
2. 数据管理:GIS能够对输入的地理信息进行分类、整理、存储,形成完整的地理数据库。
这些数据库包含多种格式数据,如矢量数据(点、线、面)、栅格数据(像素)、属性数据(属性表),并通过索引和关系建立相应的数据结构。
3. 空间分析:GIS具备空间分析功能,可以对地理信息进行定位、测量、叠加、缓冲区分析等。
通过空间分析,可以揭示地理现象的内在联系和规律,为决策提供科学依据。
4. 结果展示:GIS可以将分析结果以地图、图表等形式展示,并通过交互式操作和模型构建,使用户更直观地理解地理信息。
二、遥感技术的概念与原理遥感技术是通过航空、卫星等手段获取地球表面的图像和数据,以推断出地球表面的相关信息。
遥感技术可以分为主动遥感和被动遥感。
1. 主动遥感:主动遥感是指通过发射信号,利用信号的反射或散射进行地物探测和数据获取的一种技术。
典型的主动遥感技术是雷达遥感,利用雷达信号的反射来获取地面特征,如地形、水文、植被等。
2. 被动遥感:被动遥感是指通过接收地物辐射的能量进行观测和数据获取的技术。
地理信息系统与卫星遥感数据解读

地理信息系统与卫星遥感数据解读地理信息系统(Geographic Information System,简称GIS)和卫星遥感数据解读是现代地理学领域中的重要研究方向。
GIS是一种将地理空间数据与属性数据相结合的技术,可以用来分析、管理和展示地理信息。
而卫星遥感数据则是通过卫星对地球表面进行观测和记录,获取大范围、高分辨率的地理数据。
地理信息系统与卫星遥感数据结合,可以为地理学研究提供更全面、准确的数据支持。
首先,GIS的基本概念和原理是理解地理信息系统与卫星遥感数据解读的基础。
GIS是一种基于计算机技术的地理信息处理工具,它将地理空间数据和属性数据整合在一起,通过空间分析、数据挖掘等方法,揭示地理现象的内在规律。
卫星遥感数据则是通过卫星对地球表面进行观测,获取地表的光谱、热红外、雷达等数据,可以提供大范围、多时相的地理信息。
GIS通过对卫星遥感数据的解读和分析,可以实现对地表特征、土地利用、环境变化等方面的研究。
其次,GIS与卫星遥感数据的应用范围广泛。
在自然地理学领域,GIS与卫星遥感数据可以用于研究地貌、气候、水文等自然要素的分布和变化规律。
通过对卫星遥感数据的解读,可以获取地表高程、植被覆盖、土壤类型等信息,进而分析地形起伏、植被生长状况以及土地利用的合理性。
在人文地理学领域,GIS与卫星遥感数据可以用于研究城市规划、交通网络、人口分布等人文要素的空间分布和变化趋势。
通过对卫星遥感数据的解读,可以获取城市建筑物分布、道路网络状况、人口密度等信息,进而分析城市发展的趋势和问题。
此外,GIS与卫星遥感数据的结合也在环境保护和资源管理方面发挥着重要作用。
通过对卫星遥感数据的解读和分析,可以监测森林覆盖变化、湖泊水质状况、农田灌溉效果等环境指标,为环境保护和资源管理提供科学依据。
例如,在森林资源管理中,GIS可以结合卫星遥感数据,实现对森林面积、树种组成、砍伐情况等信息的动态监测和分析,为森林保护和可持续利用提供决策支持。
遥感技术与地理信息系统
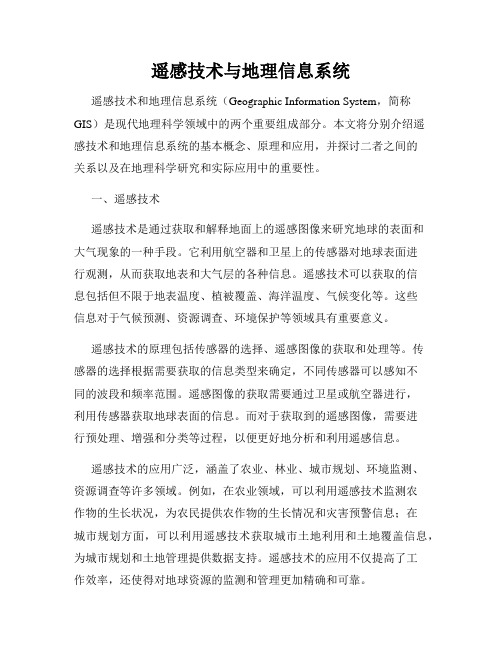
遥感技术与地理信息系统遥感技术和地理信息系统(Geographic Information System,简称GIS)是现代地理科学领域中的两个重要组成部分。
本文将分别介绍遥感技术和地理信息系统的基本概念、原理和应用,并探讨二者之间的关系以及在地理科学研究和实际应用中的重要性。
一、遥感技术遥感技术是通过获取和解释地面上的遥感图像来研究地球的表面和大气现象的一种手段。
它利用航空器和卫星上的传感器对地球表面进行观测,从而获取地表和大气层的各种信息。
遥感技术可以获取的信息包括但不限于地表温度、植被覆盖、海洋温度、气候变化等。
这些信息对于气候预测、资源调查、环境保护等领域具有重要意义。
遥感技术的原理包括传感器的选择、遥感图像的获取和处理等。
传感器的选择根据需要获取的信息类型来确定,不同传感器可以感知不同的波段和频率范围。
遥感图像的获取需要通过卫星或航空器进行,利用传感器获取地球表面的信息。
而对于获取到的遥感图像,需要进行预处理、增强和分类等过程,以便更好地分析和利用遥感信息。
遥感技术的应用广泛,涵盖了农业、林业、城市规划、环境监测、资源调查等许多领域。
例如,在农业领域,可以利用遥感技术监测农作物的生长状况,为农民提供农作物的生长情况和灾害预警信息;在城市规划方面,可以利用遥感技术获取城市土地利用和土地覆盖信息,为城市规划和土地管理提供数据支持。
遥感技术的应用不仅提高了工作效率,还使得对地球资源的监测和管理更加精确和可靠。
二、地理信息系统地理信息系统是一种集成了地理数据收集、存储、管理、分析和可视化等功能的计算机系统。
它通过对地理数据的处理和分析,可以生成各种地图、图表和报告,用以支持地理科学研究和实际应用。
地理信息系统包括硬件、软件、数据和人员等多个组成部分。
地理信息系统的核心是数据管理和空间分析。
数据管理涉及地理数据的采集、存储和整理,使其成为一个可靠和易于访问的数据库。
空间分析则是对地理数据进行统计和分析,通过模型和算法,揭示地理现象之间的关系和规律。
遥感数据与地理信息系统相结合的应用

遥感数据与地理信息系统相结合的应用近年来,随着科技的快速发展,遥感技术及地理信息系统(Geographic Information System,简称GIS)已经成为了现代地理学的重要组成部分。
遥感数据指的是利用卫星、飞机等遥感设备采集到的地球表面的各种信息,而GIS则是一种将地理数据整合、存储、分析和展示的技术体系。
这两者相结合,为各个领域的科学研究、资源管理以及决策支持提供了强有力的工具。
本文将探讨遥感数据与GIS相结合的应用,并重点展示了它在环境保护、城市规划以及农业发展等方面的具体应用案例。
首先,遥感数据与GIS的结合在环境保护方面具有独特的优势。
通过遥感技术,我们可以获取到大范围的空间数据,包括陆地、海洋以及大气等多个层面的信息。
这些信息包括地形、植被类型、水质、空气质量等等。
通过将这些遥感数据整合到GIS系统中,我们可以使用空间分析功能对环境问题进行定位和评估。
例如,在自然保护区的管理中,我们可以利用遥感数据和GIS对植被覆盖、栖息地分布等进行监测和分析,以便科学地进行资源保护和规划。
此外,在气候变化研究中,遥感数据与GIS的结合也可以帮助我们精确地对全球气候变化进行监测和模拟,为制定应对措施提供科学依据。
进一步,遥感数据与GIS的结合在城市规划方面也发挥了重要作用。
随着城市化进程的加速,城市规划变得更加复杂。
然而,通过引入遥感数据和GIS技术,我们能够更好地了解城市的空间分布、土地利用情况和交通流动状况等关键信息。
通过对这些数据进行分析和模拟,可以为城市规划师提供科学的决策支持。
例如,利用卫星遥感数据和GIS技术,我们可以对城市的土地利用进行分类和评估,以便合理规划和利用城市土地资源。
此外,通过GIS系统中的交通网络分析功能,我们可以评估交通状况并优化交通设计,提高城市交通效率和减少交通拥堵。
最后,遥感数据与GIS的结合在农业发展方面也具有广阔的应用前景。
农业是人类社会的基础产业,而科学的农业发展需要充分了解农田和农作物的状况。
地理中的地理信息系统与遥感
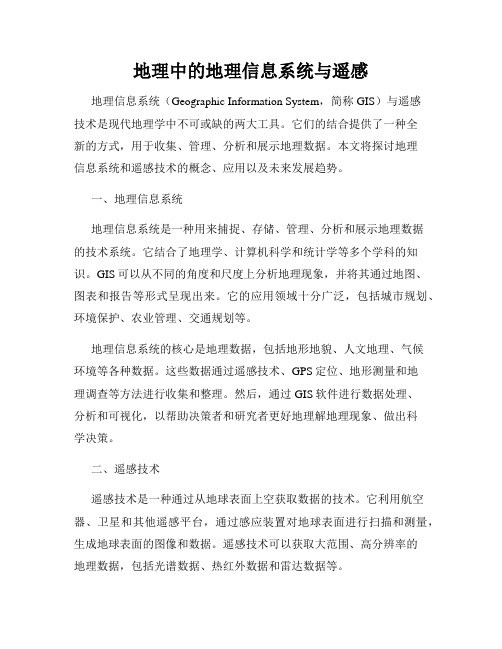
地理中的地理信息系统与遥感地理信息系统(Geographic Information System,简称GIS)与遥感技术是现代地理学中不可或缺的两大工具。
它们的结合提供了一种全新的方式,用于收集、管理、分析和展示地理数据。
本文将探讨地理信息系统和遥感技术的概念、应用以及未来发展趋势。
一、地理信息系统地理信息系统是一种用来捕捉、存储、管理、分析和展示地理数据的技术系统。
它结合了地理学、计算机科学和统计学等多个学科的知识。
GIS可以从不同的角度和尺度上分析地理现象,并将其通过地图、图表和报告等形式呈现出来。
它的应用领域十分广泛,包括城市规划、环境保护、农业管理、交通规划等。
地理信息系统的核心是地理数据,包括地形地貌、人文地理、气候环境等各种数据。
这些数据通过遥感技术、GPS定位、地形测量和地理调查等方法进行收集和整理。
然后,通过GIS软件进行数据处理、分析和可视化,以帮助决策者和研究者更好地理解地理现象、做出科学决策。
二、遥感技术遥感技术是一种通过从地球表面上空获取数据的技术。
它利用航空器、卫星和其他遥感平台,通过感应装置对地球表面进行扫描和测量,生成地球表面的图像和数据。
遥感技术可以获取大范围、高分辨率的地理数据,包括光谱数据、热红外数据和雷达数据等。
遥感技术在地理学中的应用非常广泛。
它可以用来监测和预测自然灾害,如地震、洪水和火灾。
通过获取地表温度和植被指数等数据,遥感技术还可以用于农业、林业和生态环境保护等领域。
此外,遥感技术还可以用来制作地图、进行城市规划、资源勘探等。
三、地理信息系统与遥感的结合地理信息系统和遥感技术的结合可以大大提高地理数据的处理和分析能力。
通过将遥感数据与地理信息系统相结合,可以实现对地理现象的全面观测和分析。
例如,通过遥感技术获取的卫星影像可以与地理信息系统中的地理数据进行融合,实现对城市发展和土地利用的监测和评估。
此外,地理信息系统和遥感技术还可以用于环境保护和资源管理。
地理信息系统与遥感技术高中地理知识前沿领域
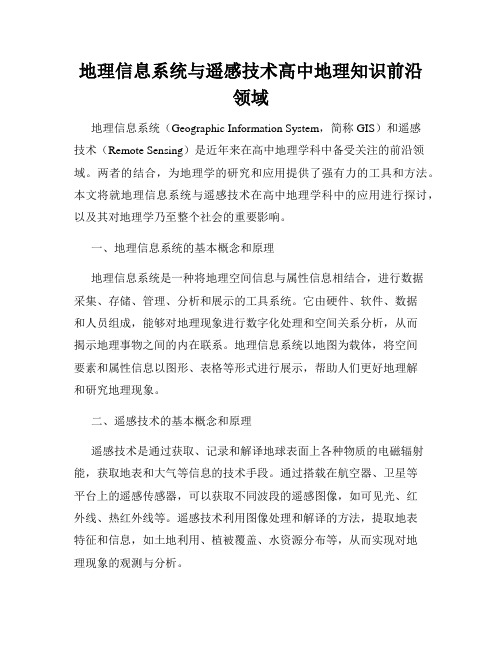
地理信息系统与遥感技术高中地理知识前沿领域地理信息系统(Geographic Information System,简称GIS)和遥感技术(Remote Sensing)是近年来在高中地理学科中备受关注的前沿领域。
两者的结合,为地理学的研究和应用提供了强有力的工具和方法。
本文将就地理信息系统与遥感技术在高中地理学科中的应用进行探讨,以及其对地理学乃至整个社会的重要影响。
一、地理信息系统的基本概念和原理地理信息系统是一种将地理空间信息与属性信息相结合,进行数据采集、存储、管理、分析和展示的工具系统。
它由硬件、软件、数据和人员组成,能够对地理现象进行数字化处理和空间关系分析,从而揭示地理事物之间的内在联系。
地理信息系统以地图为载体,将空间要素和属性信息以图形、表格等形式进行展示,帮助人们更好地理解和研究地理现象。
二、遥感技术的基本概念和原理遥感技术是通过获取、记录和解译地球表面上各种物质的电磁辐射能,获取地表和大气等信息的技术手段。
通过搭载在航空器、卫星等平台上的遥感传感器,可以获取不同波段的遥感图像,如可见光、红外线、热红外线等。
遥感技术利用图像处理和解译的方法,提取地表特征和信息,如土地利用、植被覆盖、水资源分布等,从而实现对地理现象的观测与分析。
三、地理信息系统与遥感技术的应用(一)资源与环境管理地理信息系统和遥感技术在资源与环境管理方面具有广泛的应用。
通过遥感图像的获取和分析,可以实现对土地利用、植被覆盖、水资源状况等的监测与分析,为资源的可持续利用和环境保护提供科学依据。
(二)城市规划与管理在城市规划与管理中,地理信息系统和遥感技术能够提供详细的地理信息和空间数据,帮助规划师更好地对城市进行布局和设计。
通过地理信息系统的分析和模拟,可以实现对城市交通、管网、绿化等方面的优化与管理,从而为城市的可持续发展提供支持。
(三)灾害监测与预警地理信息系统和遥感技术在灾害监测与预警中发挥着重要作用。
通过对遥感图像的分析,可以实时监测和预警自然灾害,如地震、洪水、森林火灾等。
地理信息系统与遥感技术的应用与价值

地理信息系统与遥感技术的应用与价值地理信息系统(Geographic Information System, GIS)是一种将地理空间数据与属性数据进行整合和分析的计算机技术系统。
而遥感技术(Remote Sensing)则是通过获取卫星、航空等遥感数据,对地球表面物体进行观测和测量。
这两项技术的应用与价值在现代社会中日益凸显,不仅在环境保护、城市规划、农业生产等领域发挥重要作用,还为科学研究、资源管理、灾害监测和应急响应等方面提供了支持。
一、地理信息系统的应用与价值地理信息系统广泛应用于城市规划、土地利用、交通运输、环境保护等领域,对于合理规划城市空间,提高土地利用效率具有重要意义。
例如,在城市规划中,利用GIS技术可以对城市现状进行数据采集和分析,为城市规划决策提供科学依据。
在土地利用方面,GIS技术可以对土地资源进行空间分析,评估土地潜力和优化利用。
此外,GIS在交通运输领域的应用也非常重要。
通过收集和分析交通流量、交通网络数据等,可以对交通状况进行实时监测和优化调整,提高交通效率,减少拥堵问题。
同时,在交通规划中,GIS技术可以考虑城市空间特征和道路条件,进行交通路网规划和交通设施布局。
在环境保护方面,GIS技术可以帮助监测和评估环境质量,预测环境风险,为环境决策提供科学依据。
通过将大量环境数据与地理空间信息进行整合,GIS可以准确地分析环境问题的空间分布和相关因素,及时发现环境异常情况并采取相应的措施。
二、遥感技术的应用与价值遥感技术可以获取地表物体的图像和数据,无需直接接触物体,具有全球性、动态性和高精度性等特点,广泛应用于地质勘探、农业生产、资源管理等方面。
在地质勘探领域,遥感技术可以通过获取地下矿产资源的地表特征和地球物理参数,辅助勘探人员找到潜在的矿产资源。
利用遥感技术,可以减少勘探成本和勘探风险,提高勘探效率。
农业生产是遥感技术的重要应用领域之一。
通过获取农作物的遥感数据,可以对农作物的生长状态、病虫害情况、土壤水分分布等进行监测和评估,提供精准的农作物管理措施。
地理信息系统与遥感技术
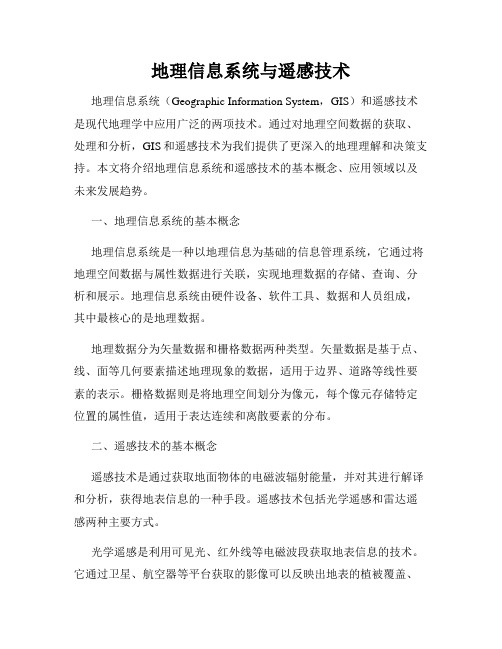
地理信息系统与遥感技术地理信息系统(Geographic Information System,GIS)和遥感技术是现代地理学中应用广泛的两项技术。
通过对地理空间数据的获取、处理和分析,GIS和遥感技术为我们提供了更深入的地理理解和决策支持。
本文将介绍地理信息系统和遥感技术的基本概念、应用领域以及未来发展趋势。
一、地理信息系统的基本概念地理信息系统是一种以地理信息为基础的信息管理系统,它通过将地理空间数据与属性数据进行关联,实现地理数据的存储、查询、分析和展示。
地理信息系统由硬件设备、软件工具、数据和人员组成,其中最核心的是地理数据。
地理数据分为矢量数据和栅格数据两种类型。
矢量数据是基于点、线、面等几何要素描述地理现象的数据,适用于边界、道路等线性要素的表示。
栅格数据则是将地理空间划分为像元,每个像元存储特定位置的属性值,适用于表达连续和离散要素的分布。
二、遥感技术的基本概念遥感技术是通过获取地面物体的电磁波辐射能量,并对其进行解译和分析,获得地表信息的一种手段。
遥感技术包括光学遥感和雷达遥感两种主要方式。
光学遥感是利用可见光、红外线等电磁波段获取地表信息的技术。
它通过卫星、航空器等平台获取的影像可以反映出地表的植被覆盖、城市扩张、水体变化等情况。
雷达遥感则是利用雷达的微波辐射进行探测,可以在夜晚和云层遮挡的情况下获取地表信息。
三、地理信息系统与遥感技术的应用领域地理信息系统与遥感技术在许多领域中都有广泛的应用。
首先,在环境保护中,GIS和遥感技术可以监测森林覆盖率、湿地退化等环境变化情况,为制定保护政策提供科学依据。
其次,在城市规划中,GIS可以分析土地利用状况、人口分布等信息,为城市设计和规划提供决策支持。
另外,农业、水资源管理、交通规划等领域也都离不开地理信息系统和遥感技术的支持。
GIS在农业领域可以帮助农民优化农田管理、监测病虫害等;在水资源管理中,GIS可以进行水质分析、水文模拟等工作;在交通规划中,GIS可以进行道路网络分析、交通流量模拟等方面的研究。
地理中的地理信息系统与遥感技术
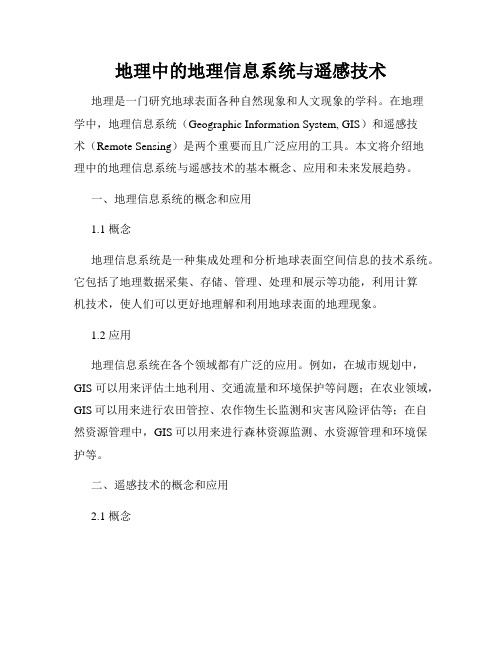
地理中的地理信息系统与遥感技术地理是一门研究地球表面各种自然现象和人文现象的学科。
在地理学中,地理信息系统(Geographic Information System, GIS)和遥感技术(Remote Sensing)是两个重要而且广泛应用的工具。
本文将介绍地理中的地理信息系统与遥感技术的基本概念、应用和未来发展趋势。
一、地理信息系统的概念和应用1.1 概念地理信息系统是一种集成处理和分析地球表面空间信息的技术系统。
它包括了地理数据采集、存储、管理、处理和展示等功能,利用计算机技术,使人们可以更好地理解和利用地球表面的地理现象。
1.2 应用地理信息系统在各个领域都有广泛的应用。
例如,在城市规划中,GIS可以用来评估土地利用、交通流量和环境保护等问题;在农业领域,GIS可以用来进行农田管控、农作物生长监测和灾害风险评估等;在自然资源管理中,GIS可以用来进行森林资源监测、水资源管理和环境保护等。
二、遥感技术的概念和应用2.1 概念遥感技术是利用航天器、飞机等远距离传感器获取地球表面信息的技术。
通过接收、记录、解码和分析这些数据,可以获取地表特征、物质组成和变化规律等信息。
2.2 应用遥感技术在环境监测、资源调查、灾害评估等方面有广泛的应用。
例如,在自然灾害监测方面,通过遥感技术可以实时获取地震、洪水和火灾等灾害的影响范围和程度;在环境保护方面,遥感技术可以用来监测森林覆盖率、水质污染和土壤侵蚀等问题;在土地利用规划中,遥感技术可以提供土地利用变化的数据,帮助制定合理的土地利用政策。
三、地理信息系统与遥感技术的结合应用地理信息系统和遥感技术在很多情况下是相辅相成、相互融合的。
通过将遥感数据与地理信息系统相结合,可以更准确地分析地球表面的变化过程和空间关系。
例如,在城市规划中,可以利用遥感技术获取城市扩张的数据,然后结合地理信息系统分析土地利用和交通流量等信息,为城市规划提供科学的依据。
四、地理中的地理信息系统与遥感技术的未来发展趋势地理信息系统与遥感技术的应用前景广阔,未来有望在各个领域继续深入发展。
地理信息系统与遥感技术
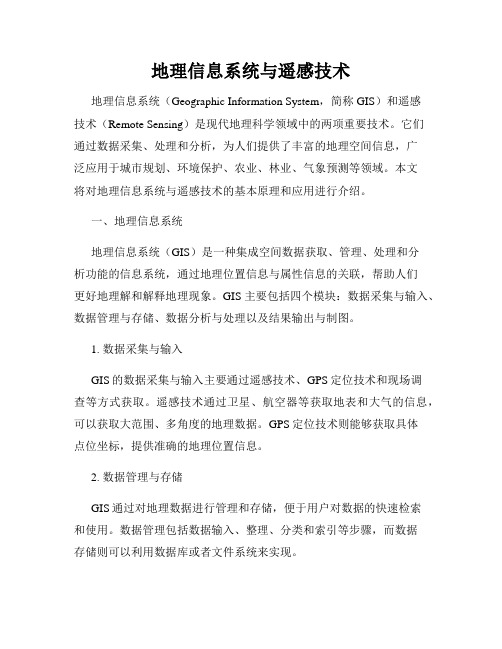
地理信息系统与遥感技术地理信息系统(Geographic Information System,简称GIS)和遥感技术(Remote Sensing)是现代地理科学领域中的两项重要技术。
它们通过数据采集、处理和分析,为人们提供了丰富的地理空间信息,广泛应用于城市规划、环境保护、农业、林业、气象预测等领域。
本文将对地理信息系统与遥感技术的基本原理和应用进行介绍。
一、地理信息系统地理信息系统(GIS)是一种集成空间数据获取、管理、处理和分析功能的信息系统,通过地理位置信息与属性信息的关联,帮助人们更好地理解和解释地理现象。
GIS主要包括四个模块:数据采集与输入、数据管理与存储、数据分析与处理以及结果输出与制图。
1. 数据采集与输入GIS的数据采集与输入主要通过遥感技术、GPS定位技术和现场调查等方式获取。
遥感技术通过卫星、航空器等获取地表和大气的信息,可以获取大范围、多角度的地理数据。
GPS定位技术则能够获取具体点位坐标,提供准确的地理位置信息。
2. 数据管理与存储GIS通过对地理数据进行管理和存储,便于用户对数据的快速检索和使用。
数据管理包括数据输入、整理、分类和索引等步骤,而数据存储则可以利用数据库或者文件系统来实现。
3. 数据分析与处理GIS的数据分析与处理功能是其最重要的特点之一。
通过对地理数据的空间和属性分析,可以揭示地理现象背后的规律和关联。
常见的数据分析方法包括空间叠置分析、空间关系分析、空间插值等。
4. 结果输出与制图GIS可以根据分析结果生成符号化的地图、图表等形式,直观地展示地理现象和分析结果。
这不仅有助于对地理问题的理解,也为决策提供了可视化的参考依据。
二、遥感技术遥感技术是指通过传感器获取地面物体信息,通过信号传输和处理,得到地理信息的一种手段。
遥感技术既包括使用航空器和卫星获取空间信息的遥感技术,也包括使用无人机和手持仪器进行地面遥感的技术。
1. 遥感数据的获取遥感数据的获取主要通过航空器和卫星来实现。
地理信息系统中英文对照外文翻译文献

中英文对照外文翻译(文档含英文原文和中文翻译)A Survey on Spatio-Temporal Data WarehousingAbstractGeographic Information Systems (GIS) have been extensively used in various application domains, ranging from economical, ecological and demographic analysis,to city and route planning. Nowadays, organizations need sophisticated GIS-based Decision Support System (DSS) to analyze their data with respect to geographic information, represented not only as attribute data, but also in maps. Thus, vendors are increasingly integrating their products, leading to the concept of SOLAP (Spatial OLAP). Also, in the last years, and motivated by the explosive growth in the use of PDA devices, the field of moving object data has been receiving attention from the GIS community. However, not much has been done in providing moving object databases with OLAP functionality. In the first part of this paper we survey theSOLAP literature. We then move to Spatio-Temporal OLAP, in particular addressing the problem of trajectory analysis. We finally provide an in-depth comparative analysis between two proposals introduced in the context of the GeoPKDD EU project: the Hermes-MDC system,and Piet, a proposal for SOLAP and moving objects,developed at the University of Buenos Aires, Argentina.Keywords: GIS, OLAP, Data Warehousing, MovingObjects, Trajectories, AggregationINTRODUCTIONGeographic Information Systems (GIS) have been extensively used in various application domains, ranging from economical, ecological and demographic analysis, to city and route planning (Rigaux, Scholl, & V oisard, 2001; Worboys, 1995). Spatial information in a GIS is typically stored in different so-called thematic layers (also called themes). Information in themes can be stored in data structures according to different data models, the most usual ones being the raster model and the vector model. In a thematic layer, spatial data is annotated with classical relational attribute information, of (in general) numeric or string type. While spatial data is stored in data structures suitable for these kinds of data, associated attributes are usually stored in conventional relational databases. Spatial data in the different thematic layers of a GIS system can be mapped univocally to each other using a common frame of reference, like a coordinate system.These layers can be overlapped or overlayed to obtain an integrated spatial view.On the other hand, OLAP (On Line Analytical Processing) (Kimball,1996; Kimball & Ross, 2002) comprises a set of tools and algorithms that allow efficiently querying multidimensional databases, containing large amounts of data, usually called Data Warehouses. In OLAP, data is organized as a set of dimensions and fact tables. In the multidimensional model, data can be perceived as a data cube, where each cell contains a measure or set of (probably aggregated) measures of interest. As we discuss later, OLAP dimensions are further organized in hierarchies that favor the data aggregation process (Cabibbo & Torlone, 1997). Several techniques and algorithms have been developed for query processing, most of them involving some kind of aggregate precomputation (Harinarayan, Rajaraman, & Ullman, 1996).The need for OLAP in GISDifferent data models have been proposed for representing objects in a GIS. ESRI () first introduced the Coverage data model to bind geometric objects to non-spatial attributes that describe them. Later, they extended this model with object-oriented support, in a way that behavior can be defined for geographic features (Zeiler,1999). The idea of the Coverage data model is also supported by the Reference Model proposed by the Open Geospatial Consortium (). Thus, in spite of the model of choice,there is always the underlying idea of binding geometric objects to objects or attributes stored in (mostly) object-relational databases (Stonebraker & Moore, 1996). In addition, query tools in commercial GIS allow users to overlap several thematic layers in order to locate objects of interest within an area, like schools or fire stations.For this, they use indexing structures based on R-trees (Gutman, 1984).GIS query support sometimes includes aggregation of geographic measures, for example, distances or areas (e.g., representing different geological zones). However, these aggregations are not the only ones that are required, as we discuss below.Nowadays, organizations need sophisticated GIS-based Decision Support System (DSS) to analyze their data with respect to geographic information, represented not only as attribute data, but also in maps, probably in different thematic layers. In this sense, OLAP and GIS vendors are increasingly integrating their products (see, for instance,Microstrategy and MapInfo integration in /, and /). In this sense, aggregate queries are central to DSSs. Classical aggregate OLAP queries (like “total sales of cars in California”), and aggregation combined with complex queries involving geometric components (“total sales in all villages crossed by the Mississippi river and within a radius of 100 km around New Orleans”) must be efficiently supported. Moreover, navigation of the results using typical OLAP operations like roll-up or drill-down is also required. These operations are not supported by commercial GIS in a straightforward way. One of the reasons is that the GIS data models discussed above were developed with “transactional” queries in mind. Thus, the databases storing nonspatial attributes or objects are designed to support those (nonaggregate) kinds of queries. Decision support systems need a different data model, where non-spatial data, probably consolidated from different sectors in an organization, is stored in a data warehouse. Here,numerical data are stored in fact tables built along several dimensions.For instance, if we are interested in the sales of certain products in stores in a given region, we may consider the sales amounts in a fact table over the three dimensions Store, Time and Product. In order to guarantee summarizability (Lenz & Shoshani, 1997), dimensions are organized into aggregation hierarchies. For example, stores can aggregate over cities which in turn can aggregate into regions and countries. Each of these aggregation levels can also hold descriptive attributes like city population, the area of a region, etc. To fulfill the requirements of integrated GIS-DSS, warehouse data must be linked to geographic data. For instance, a polygon representing a region must be associated to the region identifier in the warehouse. Besides, system integration in commercial GIS is not an easy task. In the current commercial applications, the GIS and OLAP worlds are integrated in an ad-hoc fashion, probably in a different way (and using different data models) each time an implementation is required, even when a data warehouse is available for non-spatial data.An Introductory Example. We present now a real-world example for illustrating some issues in the spatial warehousing problematic. We selected four layers with geographic and geological features obtained from the National Atlas Website (). Theselayers contain the following information: states, cities, and rivers in North America, and volcanoes in the northern hemisphere (published by the Global V olcanism Program - GVP). Figure 1 shows a detail of the layers containing cities and rivers in North America, displayed using the graphic interface of the Piet implementation we discuss later in the paper. Note the density of the points representing cities (particularly in the eastern region). Rivers are represented as polylines. Figure 2 shows a portion of two overlayed layerscontaining states (represented as polygons) and volcanoes in the northern hemisphere.There is also non-spatial information stored in a conventional data warehouse. In this data warehouse, dimension tables contain customer,stores and product information, and a fact table contains stores sales across time. Also, numerical and textual information on the geographic components exist (e.g., population, area), stored as usual as attributes of the GIS layers.In the scenario above, conventional GIS and organizational data can be integrated for decision support analysis. Sales information could be analyzed in the light of geographical features, conveniently displayed in maps. This analysis could benefit from the integration of both worlds in a single framework. Even though this integration could be possible with existing technologies, ad-hoc solutions are expensive because,besides requiring lots of complex coding, they are hardly portable. To make things more difficult, ad-hoc solutions require data exchange between GIS and OLAP applications to be performed. This implies that the output of a GIS query must be probably exported as members in dimensions of a data cube, and merged for further analysis. For example, suppose that a business analyst is interested in studying the sales of nautical goods in stores located in cities crossed by rivers. She could first query the GIS, to obtain the cities of interest. She probably has stored sales in a data cube containing a dimension Store or Geography with city as a dimension level. She would need to“manually” select the cities of interest (i.e., the ones returned by the GIS query) in the cube, to be able to go on with the analysis (in the best case, an ad-hoc customized middleware could help her). Of course, she must repeat this for each query involving a (geographic) dimension inthe data cube.Figure 1. Two overlayed layers containing cities and rivers in North America.On the contrary, GIS/Data warehousing integration can provide a more natural solution. The second part of this survey is devoted to spatio-temporal datawarehousing and OLAP. Moving objects databases (MOD) have been receiving increasing attention from the database community in recent years, mainly due to the wide variety of applications that technology allows nowadays. Trajectories of moving objects like cars or pedestrians, can be reconstructed by means of samples describing the locations of these objects at certain points in time. Although thereFigure 2. Two overlayed layers containing states in North America and volcanoes in thenorthern hemisphere.exist many proposals for modeling and querying moving objects, only a small part of them address the problem of aggregation of moving objects data in a GIS (Geographic Information Systems) scenario. Many interesting applications arise, involving moving objects aggregation, mainly regarding traffic analysis, truck fleet behavior analysis, commuter traffic in a city, passenger traffic in an airport, or shopping behavior in a mall. Building trajectory data warehouses that can integrate with a GIS is an open problem that is starting to attract database researchers. Finally, the MOD setting is appropriate for data mining tasks, and we also comment on this in the paper. In this paper, we first provide a brief background on GIS, data warehousing and OLAP, and a review of the state-of-the-art in spatial OLAP. After this, we move on to study spatio-temporal data warehousing, OLAP and mining. We then provide a detailed analysis of the Piet framework, aimed at integrating GIS, OLAP and moving object data, and conclude with a comparison between this proposal, and the Hermes data cartrridge and trajectory datawarehouse developed in the context of the GeoPKDD project (Information about the GoePKDD project can be found at http://www.geopkdd.eu).A SHORT BACKGROUNDGISIn general, information in a GIS application is divided over several thematic layers. The information in each layer consists of purely spatial data on the one hand, that is combined with classical alpha-numeric attribute data on the other hand (usually stored in a relational database). Two main data models are used for the representation of the spatial part of the information within one layer, the vector model and the raster model. The choice of model typically depends on the data source from which the information is imported into the GIS.The Vector Model. The vector model is used the most in current GIS (Kuper & Scholl, 2000). In the vector model, infinite sets of points in space are represented as finite geometric structures, or geometries, like, for example, points, polylines and polygons. More concretely, vector data within a layer consists in a finite number of tuples of the form (geometry, attributes) where a geometry can be a point, a polyline or a polygon. There are several possible data structures to actually store these geometries (Worboys, 1995).The Raster Model. In the raster model, the space is sampled into pixels or cells, each one having an associated attribute or set of attributes. Usually, these cells form a uniform grid in the plane. For each cell or pixel, the sample value of some function is computed and associated to the cell as an attribute value, e.g., a numeric value or a color. In general, information represented in the raster model is organized intozones, where the cells of a zone have the same value for some attribute(s). The raster model has very efficient indexing structures and it is very well-suited to model continuous change but its disadvantages include its size and the cost of computing the zones.Spatial information in the different thematic layers in a GIS is often joined or overlayed. Queries requiring map overlay are more difficult to compute in the vector model than in the raster model. On the other hand, the vector model offers a concise representation of the data, independent on the resolution. For a uniform treatment of different layers given in the vector or the raster model, in this paper we treat the raster model as a special case of the vector model. Indeed, conceptually, each cell is, and each pixel can be regarded as, a small polygon; also, the attribute value associated to the cell or pixel can be regarded as an attribute in the vector model.Data Warehousing and OLAPThe importance of data analysis has increased significantly in recent years as organizations in all sectors are required to improve their decision-making processes in order to maintain their competitive advantage. We said before that OLAP (On Line Analytical Processing) (Kimball, 1996; Kimball & Ross, 2002) comprises a set of tools and algorithms that allow efficiently querying databases that contain large amounts of data. These databases, usually designed for read-only access (in general, updating isperformed off-line), are denoted data warehouses. Data warehouses are exploited in different ways. OLAP is one of them. OLAP systems are based on a multidimensional model, which allows a better understanding of data for analysis purposes and provides better performance for complex analytical queries. The multidimensional model allows viewing data in an n-dimensional space, usually called a data cube (Kimball & Ross,2002). In this cube, each cell contains a measure or set of (probably aggregated) measures of interest. This factual data can be analyzed along dimensions of interest, usually organized in hierarchies (Cabibbo & Torlone, 1997). Three typical ways of OLAP tools implementation exist: MOLAP (standing for multidimensional OLAP), where data is stored in proprietary multidimensional structures, ROLAP (relational OLAP), where data is stored in (object) relational databases, and HOLAP (standing for hybrid OLAP, which provides both solutions. In a ROLAP environment, data is organized as a set of dimension tables and fact tables, and we assume this organization in the remainder of the paper.There are a number of OLAP operations that allow exploiting the dimensions and their hierarchies, thus providing an interactive data analysis environment. Warehouse databases are optimized for OLAP operations which, typically, imply data aggregation or de-aggregation along a dimension, called roll-up and drill-down, respectively. Other operations involve selecting parts of a cube (slice and dice) and reorienting the multidimensional view of data (pivoting). In addition to the basic operations described above, OLAP tools provide a great variety of mathematical, statistical, and financial operators for computing ratios, variances, ranks,etc.It is an accepted fact that data warehouse (conceptual) design is still an open issue in the field (Rizzi & Golfarelli, 2000). Most of the data models either provide a graphical representation based on the Entity- Relationship (E/R) model or UML notations, or they just provide some formal definitions without user-oriented graphical support. Recently, Malinowsky and Zimányi (2006) propose the MultiDim model. This model is based on the E/R model and provides an intuitive graphical notation. Also recently, Vaisman (Vaisman, 2006a, 2006b) introduced a methodology for requirement elicitation in Decision Support Systems, arguing that methodologies used for OLTP systems are not appropriate for OLAP systems.Temporal Data WarehousesThe relational data model as proposed by Codd (1970), is not wellsuited for handling spatial and/or temporal data. Data evolution over time must be treated in this model, in the same way as ordinary data. This is not enough for applications that require past, present, and/or future data values to be dealt with by the database. In real life such applications abound. Therefore, in the last decades, much research has been done in the field of temporal databases. Snodgrass (1995) describes the design of the TSQL2 Temporal Query Language, an upward compatible extension of SQL-92. The book, written as a result of a Dagstuhl seminar organized in June 1997 by Etzion, Jajodia, andSripada (1998), contains comprehensive bibliography, glossaries for both temporal database and time granularity concepts, and summaries of work around 1998. The same author (Snodgrass, 1999), in other work, discusses practical research issues on temporal database design and implementation.Regarding temporal data warehousing and OLAP, Mendelzon and Vaisman (2000, 2003) proposed a model, denoted TOLAP, and developed a prototype and a datalog-like query language, based on a (temporal) star schema. Vaisman, Izquierdo, and Ktenas (2006) also present a Web-based implementation of this model, along with a query language, called TOLAP-QL. Eder, Koncilia, and Morzy (2002) also propose a data model for temporal OLAP supporting structural changes. Although these efforts, little attention has been devoted to the problem of conceptual and logical modeling for temporal data warehouses. SPATIAL DATA WAREHOUSING AND OLAPSpatial database systems have been studied for a long time (Buchmann, Günther, Smith, & Wang, 1990; Paredaens, Van Den Bussche, & Gucht, 1994). Rigaux et al. (2001) survey various techniques, such as spatial data models, algorithms, and indexing methods, developed to address specific features of spatial data that are not adequately handled by mainstream DBMS technology.Although some authors have pointed out the benefits of combining GIS and OLAP, not much work has been done in this field. Vega López,Snodgrass, and Moon (2005) present a comprehensive survey on spatiotemporal aggregation that includes a section on spatial aggregation. Also, Bédard, Rivest, and Proulx (2007) present a review of the efforts for integrating OLAP and GIS. As we explain later, efficient data aggregation is crucial for a system with GIS-OLAP capabilities.Conceptual Modeling and SOLAPRivest, Bédard, and Marchand (2001) introduced the concept of SOLAP (standing for Spatial OLAP), a paradigm aimed at being able to explore spatial data by drilling on maps, in a way analogous to what is performed in OLAP with tables and charts. They describe the desirable features and operators a SOLAP system should have.Although they do not present a formal model for this, SOLAP concepts and operators have been implemented in a commercial tool called JMAP, developed by the Centre for Research in Geomatics and KHEOPS, see /en/jmap/solap.jsp. Stefanovic, Han, and Koperski (2000) and Bédard, Merret, and Han (2001), classify spatial dimension hierarchies according to their spatial references in: (a) non-geometric;(b) geometric to non-geometric; and (c) fully geometric. Dimensions of type (a) can be treated as any descriptive dimension (Rivest et al., 2001). In dimensions of types (b) and (c), a geometry is associated to members of the hierarchies. Malinowski and Zimányi (2004) extend this classification to consider that even in the absence of several related spatial levels, a dimension can be considered spatial. Here, a dimension level is spatial if it is represented as a spatial data type (e.g., point, region), allowing them to link spatial levels through topological relationships (e.g., contains, overlaps). Thus, a spatial dimension is a dimension that contains at least one spatial hierarchy. A critical point inspatial dimension modeling is the problem of multiple-dependencies, meaning that an element in one level can be related to more than one element in a level above it in the hierarchy. Jensen, Kligys, Pedersen, and Timko (2004)address this issue, and propose a multidimensional data model for mobile services, i.e., services that deliver content to users, depending on their location.This model supports different kinds of dimension hierarchies, most remarkably multiple hierarchies in the same dimension, i.e., multiple aggregation paths. Full and partial containment hierarchies are also supported. However, the model does not consider the geometry, limiting the set of queries that can be addressed. This means that spatial dimensions are standard dimensions referring to some geographical element (like cities or roads).Malinowski and Zimányi (2006) also propose a model supporting multiple aggregation paths. Pourabbas (2003) introduces a conceptual model that uses binding attributes to bridge the gap between spatial databases and a data cube. The approach relies on the assumption that all the cells in the cube contain a value, which is not the usual case in practice, as the author expresses. Also, the approach requires modifying the structure of the spatial data to support the model. No implementation is presented.Shekhar, Lu, Tan, Chawla, & Vatsavai (2001) introduced MapCube, a visualization tool for spatial data cubes. MapCube is an operator that, given a so-called base map, cartographic preferences and an aggregation hierarchy, produces an album of maps that can be navigated via roll-up and drill-down operations.Spatial Measures. Measures are characterized in two ways in the literature, namely: (a) measures representing a geometry, which can be aggregated along the dimensions; (b) a numerical value, using a topological or metric operator. Most proposals support option (a), either as a set of coordinates (Bédard et al., 2001; Rivest et al., 2001; Malinowski & Zimányi, 2004; Bimonte, Tchounikine, & Miquel, 2005), or a set of pointers to geometric objects (Stefanovic et al., 2000). Bimonte et al. (Bimonte et al., 2005) define measures as complex objects (a measure is thus an object containing several attributes). Malinowski and Zimányi (2004) follow a similar approach, but defining measures as attributes of an n-ary fact relationship between dimensions.Damiani and Spaccapietra (2006) propose MuSD, a model allowing defining spatial measures at different granularities. Here, a spatial measure can represent the location of a fact at multiple levels of (spatial) granularity. Also, an algebra of SOLAP operators is proposed.Spatial AggregationIn light of the discussion above, it should be clear that aggregation is a crucial issue in spatial OLAP. Moreover, there is not yet a consensus about a complete set of aggregate operators for spatial OLAP. We now discuss the classic approaches to spatial aggregation. Han et al. (1998) use OLAP techniques for materializing selected spatial objects, and proposed a so-called Spatial Data Cube, and the set of operations that can be performed on this data cube. The model only supports aggregation of spatial objects.Pedersen and Tryfona (2001) propose the pre-aggregation of spatial facts. First, they pre-process these facts, computing their disjoint parts in order to be able to aggregate them later. This pre-aggregation works if the spatial properties of the objects are distributive over some aggregate function. Again, the spatial measures are geometric objects.Given that this proposal ignores the geometries, queries like “total population of cities crossed by a river” are not supported. The paper does not address forms other than polygons, although the authors claim that other more complex forms are supported by the method, and the authors do not report experimental results.With a different approach, Rao, Zhang, Yu, Li, and Chen (2003), and Zhang, Li, Rao, Yu, Chen, and Liu (2003) combine OLAP and GIS for querying so-called spatial data warehouses, using R-trees for accessing data in fact tables. The data warehouse is then exploited in the usualOLAP way. Thus, they take advantage of OLAP hierarchies for locating information in the R-tree which indexes the fact table.Although the measures here are not only spatial objects, the proposal also ignores the geometric part of the model, limiting the scope of the queries that can be addressed. It is assumed that some fact table, containing the identifiers of spatial objects exists. Finally, these objects happen to be points, which is quite unrealistic in a GIS environment, where different types of objects appear in the different layers. Some interesting techniques have been recently introduced to address the data aggregation problem. These techniques are based on the combined use of (R-tree-based) indexes, materialization (or preaggregation) of aggregate measures, and computational geometry algorithms.Papadias, Tao, Kalnis, and Zhang (2002) introduce the Aggregation Rtree (aR-tree), combining indexing with pre-aggregation. The aR-tree is an R-tree that annotates each MBR (Minimal Bounding Rectangle) with the value of the aggregate function for all the objects that are enclosed by it. They extend this proposal in order to handle historic information (see the section on moving object data below), denoting this extension aRB-tree (Papadias, Tao, Zhang, Mamoulis, Shen, and & Sun, 2002). The approach basically consists in two kinds of indexes: a host index, which is an R-tree with the summarized information, and a B-tree containing time-varying aggregate data. In the most general case, each region has a B-tree associated, with the historical information of the measures of interest in the region. This is a very efficient solution for some kinds of queries, for example, window aggregate queries (i.e., for the computation of the aggregate measure of the regions which intersect a spatio-temporal window). In addition, the method is very effective when a query is posed over a query region whose intersection with the objects in a map must be computed on-thefly,and these objects are totally enclosed in the query region. However, problems may appear when leaf entries partially overlap the query window. In this case, the result must be estimated, or the actual results computed using the base tables. In fact, Tao, Kollios, Considine, Li,and Papadias (2004), show that the aRB-tree can suffer from the distinct counting problem, if the object remains in the same region for several timestamps.时空数据仓库的调查摘要地理信息系统已被广泛应用于不同的应用领域,包括经济,生态和人口统计分析,城市和路线规划。
了解地球上的地理信息系统与遥感技术
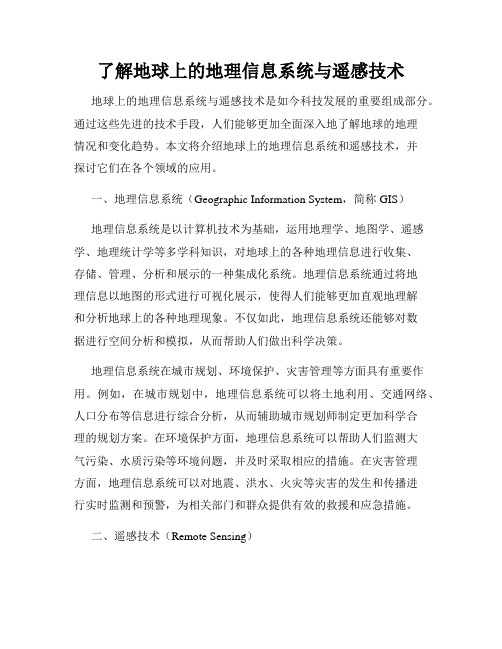
了解地球上的地理信息系统与遥感技术地球上的地理信息系统与遥感技术是如今科技发展的重要组成部分。
通过这些先进的技术手段,人们能够更加全面深入地了解地球的地理情况和变化趋势。
本文将介绍地球上的地理信息系统和遥感技术,并探讨它们在各个领域的应用。
一、地理信息系统(Geographic Information System,简称GIS)地理信息系统是以计算机技术为基础,运用地理学、地图学、遥感学、地理统计学等多学科知识,对地球上的各种地理信息进行收集、存储、管理、分析和展示的一种集成化系统。
地理信息系统通过将地理信息以地图的形式进行可视化展示,使得人们能够更加直观地理解和分析地球上的各种地理现象。
不仅如此,地理信息系统还能够对数据进行空间分析和模拟,从而帮助人们做出科学决策。
地理信息系统在城市规划、环境保护、灾害管理等方面具有重要作用。
例如,在城市规划中,地理信息系统可以将土地利用、交通网络、人口分布等信息进行综合分析,从而辅助城市规划师制定更加科学合理的规划方案。
在环境保护方面,地理信息系统可以帮助人们监测大气污染、水质污染等环境问题,并及时采取相应的措施。
在灾害管理方面,地理信息系统可以对地震、洪水、火灾等灾害的发生和传播进行实时监测和预警,为相关部门和群众提供有效的救援和应急措施。
二、遥感技术(Remote Sensing)遥感技术是利用卫星、飞机等远距离的传感装置对地球进行观测和测量,以获得地球表面的各种信息的一种技术。
遥感技术通过获取地球表面的高分辨率图像和数据,为人们提供了全球尺度和长时间序列的地理信息。
遥感技术可以捕捉到地球表面的各种地理信息,如地貌、植被分布、气候变化等,并追踪这些信息的变化和演变。
遥感技术在农业、林业、气象、水利等领域有着广泛的应用。
在农业方面,遥感技术可以通过监测农田土壤的湿度、植被的状况等信息,为农民提供精准的农业管理和作物种植建议。
在林业方面,遥感技术可以用于森林资源的监测和评估,帮助保护森林生态环境和实现可持续经营。
地理信息系统和遥感
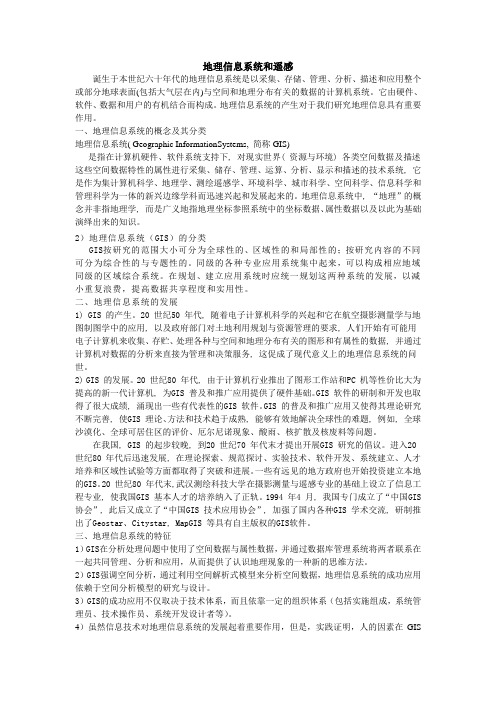
地理信息系统和遥感诞生于本世纪六十年代的地理信息系统是以采集、存储、管理、分析、描述和应用整个或部分地球表面(包括大气层在内)与空间和地理分布有关的数据的计算机系统。
它由硬件、软件、数据和用户的有机结合而构成。
地理信息系统的产生对于我们研究地理信息具有重要作用。
一、地理信息系统的概念及其分类地理信息系统( Geographic InformationSystems, 简称GIS)是指在计算机硬件、软件系统支持下, 对现实世界( 资源与环境) 各类空间数据及描述这些空间数据特性的属性进行采集、储存、管理、运算、分析、显示和描述的技术系统, 它是作为集计算机科学、地理学、测绘遥感学、环境科学、城市科学、空间科学、信息科学和管理科学为一体的新兴边缘学科而迅速兴起和发展起来的。
地理信息系统中, “地理”的概念并非指地理学, 而是广义地指地理坐标参照系统中的坐标数据、属性数据以及以此为基础演绎出来的知识。
2)地理信息系统(GIS)的分类GIS按研究的范围大小可分为全球性的、区域性的和局部性的;按研究内容的不同可分为综合性的与专题性的。
同级的各种专业应用系统集中起来,可以构成相应地域同级的区域综合系统。
在规划、建立应用系统时应统一规划这两种系统的发展,以减小重复浪费,提高数据共享程度和实用性。
二、地理信息系统的发展1) GIS 的产生。
20 世纪50 年代, 随着电子计算机科学的兴起和它在航空摄影测量学与地图制图学中的应用, 以及政府部门对土地利用规划与资源管理的要求, 人们开始有可能用电子计算机来收集、存贮、处理各种与空间和地理分布有关的图形和有属性的数据, 并通过计算机对数据的分析来直接为管理和决策服务, 这促成了现代意义上的地理信息系统的问世。
2) GIS 的发展。
20 世纪80 年代, 由于计算机行业推出了图形工作站和PC 机等性价比大为提高的新一代计算机, 为GIS 普及和推广应用提供了硬件基础。
- 1、下载文档前请自行甄别文档内容的完整性,平台不提供额外的编辑、内容补充、找答案等附加服务。
- 2、"仅部分预览"的文档,不可在线预览部分如存在完整性等问题,可反馈申请退款(可完整预览的文档不适用该条件!)。
- 3、如文档侵犯您的权益,请联系客服反馈,我们会尽快为您处理(人工客服工作时间:9:00-18:30)。
外文资料与中文翻译Metrics of scale in remote sensing and GISMichael F Goodchild(National Center for Geographic Information and Analysis, Department of Geography, University of California, Santa Barbara)ABSTRACT: The term scale has many meanings, some of which survive the transition from analog to digital representations of information better than others. Specifically, the primary metric of scale in traditional cartography, the representative fraction, has no well-defined meaning for digital data. Spatial extent and spatial resolution are both meaningful for digital data, and their ratio, symbolized as US, is dimensionless. US appears confined in practice to a narrow range. The implications of this observation are explored in the context of Digital Earth, a vision for an integrated geographic information system. It is shown that despite the very large data volumes potentially involved, Digital Earth is nevertheless technically feasible with today‟s technology. KEYWORDS: Scale, Geographic Information System , Remote Sensing, Spatial ResolutionINTRODUCTION: Scale is a heavily overloaded term in English, with abundant definitions attributable to many different and often independent roots, such that meaning is strongly dependent on context. Its meanings in “the scales of justice” or “scales over ones eyes” have little connection to each other, or to its meaning in a discussion of remote sensing and GIS. But meaning is often ambiguous even in that latter context. For example, scale to a cartographer most likely relates to the representative fraction, or the scaling ratio between the real world and a map representation on a flat, two-dimensional surface such as paper, whereas scale to an environmental scientist likely relates either tospatial resolution (the representatio n‟s level of spatial detail) or to spatial extent (the representation‟s spatial coverage). As a result, a simple phrase like “large scale” can send quite the wrong message when communities and disciplines interact - to a cartographer it implies fine detail, whereas to an environmental scientist it implies coarse detail. A computer scientist might say that in this respect the two disciplines were not interoperable.In this paper I examine the current meanings of scale, with particular reference to the digital world, and the metrics associated with each meaning. The concern throughout is with spatial meanings, although temporal and spectral meanings are also important. I suggest that certain metrics survive the transition to digital technology better than others.The main purpose of this paper is to propose a dimensionless ratio of two such metrics that appears to have interesting and useful properties. I show how this ratio is relevant to a specific vision for the future of geographic information technologies termed Digital Earth. Finally, I discuss how scale might be defined in ways that are accessible to a much wider range of users than cartographers and environmental scientists.FOUR MEANINGS OF SCALE LEVEL OF SPATIAL DETAIL REPRESENTATIVE FRACTIONA paper map is an analog representation of geographic variation, rather than a digital representation. All features on the Earth‟s surface are scaled using an approximately uniform ratio known as the representative fraction (it is impossible to use a perfectly unif orm ratio because of the curvature of the Earth‟s surface). The power of the representative fraction stems from the many different properties that are related to it in mapping practice. First, paper maps impose an effective limit on the positional accuracy of features, because of instability in the material used to make maps, limited ability to control the location of the pen as the map is drawn, and many other practicalconsiderations. Because positional accuracy on the map is limited, effective positional accuracy on the ground is determined by the representative fraction. A typical (and comparatively generous) map accuracy standard is 0.5 mm, and thus positional accuracy is 0.5 mm divided by the representative fraction (eg, 12.5 m for a map at 1:25,000). Second, practical limits on the widths of lines and the sizes of symbols create a similar link between spatial resolution and representative fraction: it is difficult to show features much less than 0.5 mm across with adequate clarity. Finally, representative fraction serves as a surrogate for the features depicted on maps, in part because of this limit to spatial resolution, and in part because of the formal specifications adopted by mapping agencies, that are in turn related to spatial resolution. In summary, representative fraction characterizes many important properties of paper maps.In the digital world these multiple associations are not necessarily linked. Features can be represented as points or lines, so the physical limitations to the minimum sizes of symbols that are characteristic of paper maps no longer apply. For example, a database may contain some features associated with 1:25,000 map specifications, but not all; and may include representations of features smaller than 12.5 m on the ground. Positional accuracy is also no longer necessarily tied to representative fraction, since points can be located to any precision, up to the limits imposed by internal representations of numbers (eg, single precision is limited to roughly 7 significant digits, double precision to 15). Thus the three properties that were conveniently summarized by representative fraction - positional accuracy, spatial resolution, and feature content - are now potentially independent.Unfortunately this has led to a complex system of conventions in an effort to preserve representative fraction as a universal defining characteristic of digital databases. When such databases are created directly from paper maps, by digitizing or scanning, itis possible for all three properties to remain correlated. But in other cases the representative fraction cited for a digital database is the one implied by its positional accuracy (eg, a database has representative fraction 1: 12,000 because its positional accuracy is 6 m); and in other cases it is the feature content or spatial resolution that defines the conventional representative fraction (eg, a database has representative fraction 1:12,000 because features at least 6 m across are included). Moreover, these conventions are typically not understood by novice users - the general public, or children - who may consequently be very confused by the use of a fraction to characterize spatial data, despite its familiarity to specialists.SPATIAL EXTENTThe term scale is often used to refer to the extent or scope of a study or project, and spatial extent is an obvious metric. It can be defined in area measure, but for the purposes of this discussion a length measure is preferred, and the symbol L will be used. For a square project area it can be set to the width of the area, but for rectangular or oddly shaped project areas the square root of area provides a convenient metric. Spatial extent defines the total amount of information relevant to a project, which rises with the square of a length measure.PROCESS SCALEThe term process refers here to a computational model or representation of a landscape-modifying process, such as erosion or runoff. From a computational perspective,a process is a transformation that takes a landscape from its existing state to some new state, and in this sense processes are a subset of the entire range of transformations that can be applied to spatial data.Define a process as a mapping b (x ,2t )=f ( a (x ,1t )) where a is a vector of input fields, b is a vector of output fields, f is a function, t is time, 2t is later in time thant, and x denotes location. Processes vary according to how they modify the spatial 1characteristics of their inputs, and these are best expressed in terms of contributions tot) based only on the the spatial spectrum. For example, some processes determine b(x, ,2t), and thus have minimal effect on spatial spectra. inputs at the same location a(x,1Other processes produce outputs that are smoother than their inputs, through processes of averaging or convolution, and thus act as low-pass filters. Less commonly, processes produce outputs that are more rugged than their inputs, by sharpening rather than smoothing gradients, and thus act as high-pass filters.The scale of a process can be defined by examining the effects of spectral components on outputs. If some wavelength s exists such that components with wavelengths shorter than s have negligible influence on outputs, then the process is said to have a scale of s. It follows that if s is less than the spatial resolution S of the input data, the process will not be accurately modeled.While these conclusions have been expressed in terms of spectra, it is also possible to interpret them in terms of variograms and correlograms. A low-pass filter reduces variance over short distances, relative to variance over long distances. Thus the short-distance part of the variogram is lowered, and the short-distance part of the correlogram is increased. Similarly a high-pass filter increases variance over short distances relative to variance over long distances.L/S RATIOWhile scaling ratios make sense for analog representations, the representative fraction is clearly problematic for digital representations. But spatial resolution and spatial extent both appear to be meaningful in both analog and digital contexts, despite the problems with spatial resolution for vector data. Both Sand L have dimensions oflength, so their ratio is dimensionless. Dimensionless ratios often play a fundamental role in science (eg, the Reynolds number in hydrodynamics), so it is possible that L/S might play a fundamental role in geographic information science. In this section I examine some instances of the L/S ratio, and possible interpretations that provide support for this speculation.- Today‟s computing industry seems to have settled on a screen standard of order 1 megapel, or 1 million picture elements. The first PCs had much coarser resolutions (eg, the CGA standard of the early 198Os), but improvements in display technology led to a series of more and more detailed standards. Today, however, there is little evidence of pressure to improve resolution further, and the industry seems to be content with an L/S ratio of order 103. Similar ratios characterize the current digital camera industry, although professional systems can be found with ratios as high as 4,000.- Remote sensing instruments use a range of spatial resolutions, from the 1 m of IKONOS to the 1 km of AVHRR. Because a complete coverage of the Earth‟s surface at 1 m requires on the order of 1015 pixels, data are commonly handled in more manageable tiles, or approximately rectangular arrays of cells. For years, Landsat TM imagery has been tiled in arrays of approximately 3,000 cells x 3,000 cells, for an L/S ratio of 3,000.- The value of S for a paper map is determined by the technology of map-making, and techniques of symbolization, and a value of 0.5 mm is not atypical. A map sheet 1 m across thus achieves an L/S ratio of 2,000.- Finally, the human eye‟s S can be defined as the size of a retinal cell, and the typical eye has order 108 retinal cells, implying an L/S ratio of 10,000. Interestingly, then, the screen resolution that users find generally satisfactory corresponds approximately to the parameters of the human visual system; it is somewhat larger, but the computer screentypically fills only a part of the visual field.These examples suggest that L/S ratios of between 103 and 104 are found across a wide range of technologies and settings, including the human eye. Two alternative explanations immediately suggest themselves: the narrow range may be the result of technological and economic constraints, and thus may expand as technology advances and becomes cheaper; or it may be due to cognitive constraints, and thus is likely to persist despite technological change.This tension between technological, economic, and cognitive constraints is well illustrated by the case of paper maps, which evolved under what from today‟s perspective were severe technological and economic constraints. For example, there are limits to the stability of paper and to the kinds of markings that can be made by hand-held pens. The costs of printing drop dramatically with the number of copies printed, because of strong economies of scale in the printing process, so maps must satisfy many users to be economically feasible. Goodchild [2000]has elaborated on these arguments. At the same time, maps serve cognitive purposes, and must be designed to convey information as effectively as possible. Any aspect of map design and production can thus be given two alternative interpretations: one, that it results from technological and economic constraints, and the other, that it results from the satisfaction of cognitive objectives. If the former is true, then changes in technologymay lead to changes in design and production; but if the latter is true, changes in technology may have no impact.The persistent narrow range of L/S from paper maps to digital databases to the human eye suggests an interesting speculation: That cognitive, not technological or economic objectives, confine L/S to this range. From this perspective, L/S ratios of more than 104 have no additional cognitive value, while L/S ratios of less than 103 areperceived as too coarse for most purposes. If this speculation is true, it leads to some useful and general conclusions about the design of geographic information handling systems. In the next section I illustrate this by examining the concept of Digital Earth. For simplicity, the discussion centers on the log to base 10 of the L/S ratio, denoted by log L/S, and the speculation that its effective range is between 3 and 4.This speculation also suggests a simple explanation for the fact that scale is used to refer both to L and to S in environmental science, without hopelessly confusing the listener. At first sight it seems counter~ntuitive that the same term should be used for two independent properties. But if the value of log L/S is effectively fixed, then spatial resolution and extent are strongly correlated: a coarse spatial resolution implies a large extent, and a detailed spatial resolution implies a small extent. If so, then the same term is able to satisfy both needs.THE VISION OF DIGITAL EARTHThe term Digital Earth was coined in 1992 by U.S. Vice President Al Gore [Gore, 19921, but it was in a speech written for delivery in 1998 that Gore fully elaborated the concept (www.d~~Pl9980131 .html): “Imagine, for example, a young child going to a Digital Earth exhibit at a local museum. After donning a headmounted display, she sees Earth as it appears from space. Using a data glove, she zooms in, using higher and higher levels of resolution, to see continents, then regions, countries, cities, and finally individual houses, trees, and other natural and man-made objects. Having found an area of the planet she is interested in exploring, she takes the equivalent of a …magic carpet ride‟ through a 3- D visualization of the terrain.”This vision of Digital Earth (DE) is a sophisticated graphics system, linked to a comprehensive database containing representations of many classes of phenomena. It implies specialized hardware in the form of an immersive environment (a head-mounteddisplay), with software capable of rendering the Earth‟s surface at high speed, and from any perspective. Its spatial resolution ranges down to 1 m or finer. On the face of it, then, the vision suggests data requirements and bandwidths that are well beyond today‟s capabilities. If each pixel of a 1 m resolution representation of the Earth‟s surface was allocated an average of 1 byte then a total of 1 Pb of storage would be required; storage of multiple themes could push this total much higher. In order to zoom smoothly down to 1 m it would be necessary to store the data in a consistent data structure that could be accessed at many levels of resolution. Many data types are not obviously renderable (eg, health, demographic, and economic data), suggesting a need for extensive research on visual representation.The bandwidth requirements of the vision are perhaps the most daunting problem. To send 1 Pb of data at 1 Mb per second would take roughly a human life time, and over 12,000 years at 56 Kbps. Such requirements dwarf those of speech and even full-motion video. But these calculations assume that the DE user would want to see the entire Earth at Im resolution. The previ ous analysis of log L/S suggested that for cognitive (and possibly technological and economic) reasons user requirements rarely stray outside the range of 3 to 4, whereas a full Earth at 1 m resolution implies a log L/S of approximately 7. A log L/S of 3 suggests that a user interested in the entire Earth would be satisfied with 10 km resolution; a user interested in California might expect 1 km resolution; and a user interested in Santa Barbara County might expect 100 m resolution. Moreover, these resolutions need apply only to the center of the current field of view.On this basis the bandwidth requirements of DE become much more manageable. Assuming an average of 1 byte per pixel, a megapel image requires order 107 bps if refreshed once per second. Every one-unit reduction in log L/S results in two orders of magnitude reduction in bandwidth requirements. Thus a Tl connection seems sufficientto support DE, based on reasonable expectations about compression, and reasonable refresh rates. On this basis DE appears to be feasible with today‟s communication technology.CONCLUDING COMMENTSI have argued that scale has many meanings, only some of which are well defined for digital data, and therefore useful in the digital world in which we increasingly find ourselves. The practice of establishing conventions which allow the measures of an earlier technology - the paper map - to survive in the digital world is appropriate for specialists, but is likely to make it impossible for non-specialists to identify their needs. Instead, I suggest that two measures, identified here as the large measure L and the small measure S, be used to characterize the scale properties of geographic data.The vector-based representations do not suggest simple bases for defining 5, because their spatial resolutions are either variable or arbitrary. On the other hand spatial variat;on in S makes good sense in many situations. In social applications, it appears that the processes that characterize human behavior are capable of operating at different scales, depending on whether people act in the intensive pedestrian-oriented spaces of the inner city or the extensive car-oriented spaces of the suburbs. In environmental applications, variation in apparent spatial resolution may be a logical sampling response to a phenomenon that is known to have more rapid variation in some areas than others; from a geostatistical perspective this might suggest a non-stationary variogram or correlogram (for examples of non-statjonary geostatistical analysis see Atkinson [2001]). This may be one factor in the spatial distribution of weather observation networks (though others, such as uneven accessibility, and uneven need for information are also clearly important).The primary purpose of this paper has been to offer a speculation on the significance of the dimensionless ratio L/S. The ratio is the major determinant of datavolume, and consequently processing speed, in digital systems. It also has cognitive significance because it can be defined for the human visual system. I suggest that there are few reasons in practice why log L/S should fall outside the range 3 - 4, and that this provides an important basis for designing systems for handling geographic data. Digital Earth was introduced as one such system. A constrained ratio also implies that L and S are strongly correlated in practice, as suggested by the common use of the same term scale to refer to both.ACKNOWLEDGMENTThe Alexandria Digital Library and its Alexandria Digital Earth Prototype, the source of much of the inspiration for this paper, are supported by the U.S. National Science Foundation.REFERENCESAtkinson, P.M., 2001. Geographical information science: Geocomputation and nonstationarity. Progress in Physical Geography 25(l): 111-122.Goodchild, M F 2000 Communicating geographic information in a digital age. Annals of the Association of American Geographers 90(2): 344-355.Goodchild, M.F. & J. Proctor, 1997. Scale in a digital geographic world. Geographical and Environmental Modelling l(1): 5-23.Gore, A., 1992. Earth in the Balance: Ecology and the Human Spirit. Houghton Mifflin, Boston, 407~~.Lam, N-S & D. Quattrochi, 1992. On the issues of scale, resolution, and fractal analysis in the mapping sciences. Professional Geographer 44(l): 88-98.Quattrochi D.A & M.F. Goodchild (Eds), 1997. Scale in Remote Sensing and GIS.Lewis Publishers, Boca Raton, 406~~.中文翻译:在遥感和地理信息系统的规模度量迈克尔·F古德柴尔德(美国国家地理信息和分析中心,加州大学圣巴巴拉分校地理系)摘要:长期的规模有多种含义,其中一些生存了从模拟到数字表示的信息比别人更好的过渡。