第6章 计算机辅助工艺设计
计算机辅助工艺规程设计(教案)
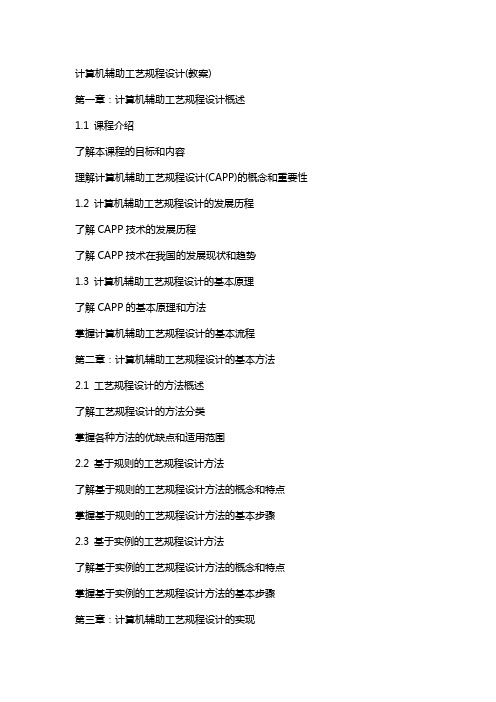
计算机辅助工艺规程设计(教案)第一章:计算机辅助工艺规程设计概述1.1 课程介绍了解本课程的目标和内容理解计算机辅助工艺规程设计(CAPP)的概念和重要性1.2 计算机辅助工艺规程设计的发展历程了解CAPP技术的发展历程了解CAPP技术在我国的发展现状和趋势1.3 计算机辅助工艺规程设计的基本原理了解CAPP的基本原理和方法掌握计算机辅助工艺规程设计的基本流程第二章:计算机辅助工艺规程设计的基本方法2.1 工艺规程设计的方法概述了解工艺规程设计的方法分类掌握各种方法的优缺点和适用范围2.2 基于规则的工艺规程设计方法了解基于规则的工艺规程设计方法的概念和特点掌握基于规则的工艺规程设计方法的基本步骤2.3 基于实例的工艺规程设计方法了解基于实例的工艺规程设计方法的概念和特点掌握基于实例的工艺规程设计方法的基本步骤第三章:计算机辅助工艺规程设计的实现3.1 计算机辅助工艺规程设计系统的组成了解计算机辅助工艺规程设计系统的组成和功能掌握各种组成模块的作用和相互关系3.2 计算机辅助工艺规程设计的关键技术了解计算机辅助工艺规程设计的关键技术掌握各种技术的方法和实现步骤3.3 计算机辅助工艺规程设计系统的开发与实现了解计算机辅助工艺规程设计系统的开发方法和流程掌握计算机辅助工艺规程设计系统的实现技术和工具第四章:计算机辅助工艺规程设计应用实例4.1 计算机辅助工艺规程设计在机械加工中的应用了解计算机辅助工艺规程设计在机械加工中的应用实例掌握各种应用实例的方法和特点4.2 计算机辅助工艺规程设计在汽车制造中的应用了解计算机辅助工艺规程设计在汽车制造中的应用实例掌握各种应用实例的方法和特点4.3 计算机辅助工艺规程设计在其他领域中的应用了解计算机辅助工艺规程设计在其他领域中的应用实例掌握各种应用实例的方法和特点第五章:计算机辅助工艺规程设计的未来发展趋势5.1 计算机辅助工艺规程设计技术的发展趋势了解计算机辅助工艺规程设计技术的发展趋势掌握各种发展趋势的方法和特点5.2 计算机辅助工艺规程设计在制造业中的应用前景了解计算机辅助工艺规程设计在制造业中的应用前景掌握各种应用前景的方法和特点5.3 计算机辅助工艺规程设计面临的挑战和解决方案了解计算机辅助工艺规程设计面临的挑战掌握各种解决方案的方法和特点第六章:计算机辅助工艺规程设计系统的用户界面设计6.1 用户界面设计原则了解用户界面设计的基本原则掌握如何设计直观、易用的用户界面6.2 用户界面设计方法学习用户界面设计的方法和技巧了解当前流行的用户界面设计工具6.3 用户界面设计实例分析典型的计算机辅助工艺规程设计系统用户界面学习如何根据用户需求设计有效的用户界面第七章:计算机辅助工艺规程设计系统的数据管理7.1 数据管理概述了解数据管理在计算机辅助工艺规程设计系统中的重要性掌握数据管理的基本概念和方法7.2 数据库技术学习数据库的基本原理和常用数据库管理系统掌握如何在计算机辅助工艺规程设计系统中使用数据库7.3 数据管理实例分析典型的计算机辅助工艺规程设计系统数据管理方案学习如何有效地管理工艺规程设计过程中的数据第八章:计算机辅助工艺规程设计系统的软件工程实现8.1 软件工程基本概念了解软件工程的的目标和基本原则掌握软件生命周期的基本阶段8.2 计算机辅助工艺规程设计系统的软件设计学习软件设计的方法和技巧了解计算机辅助工艺规程设计系统软件设计的关键环节8.3 软件工程实例分析典型的计算机辅助工艺规程设计系统软件工程实践学习如何运用软件工程方法实现计算机辅助工艺规程设计系统第九章:计算机辅助工艺规程设计系统的测试与维护9.1 系统测试概述了解系统测试的目标和基本原则掌握系统测试的方法和技巧9.2 系统测试实例分析典型的计算机辅助工艺规程设计系统测试方案学习如何进行系统测试以确保系统质量9.3 系统维护概述了解系统维护的目标和基本原则掌握系统维护的方法和技巧9.4 系统维护实例分析典型的计算机辅助工艺规程设计系统维护实践学习如何进行系统维护以保证系统长期稳定运行第十章:计算机辅助工艺规程设计的未来发展趋势10.1 技术发展趋势了解计算机辅助工艺规程设计技术的发展趋势掌握各种发展趋势的方法和特点10.2 应用领域拓展了解计算机辅助工艺规程设计应用领域的拓展掌握各种应用领域的特点和需求10.3 面临的挑战与对策了解计算机辅助工艺规程设计面临的挑战学习如何应对这些挑战,推动计算机辅助工艺规程设计技术的发展重点解析本文教案主要介绍了计算机辅助工艺规程设计(CAPP)的基本概念、方法、实现以及应用实例和发展趋势。
微电子第六章集成电路计算机辅助设计
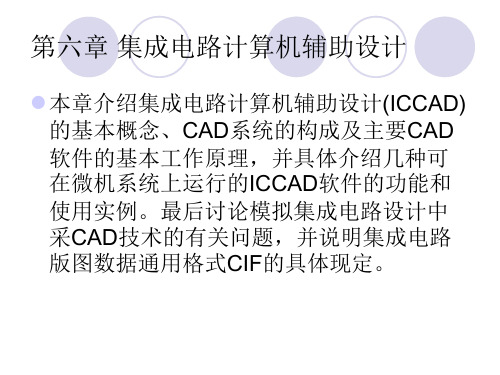
(1)版图生成
对数字电路,目前已有不少版图白动设计软件。但是对模拟集成电路, 基本还要依靠手工设计,即调用版图设计软件中的版图绘制模块,由 设计人员以人机交互方式完成版图的绘制。
(2)版图校验
为了保证生成的版图“正确无误”,一般需要进行下述3方面校验工作。 (a)设计规则校验(DRC:Design Rules check):检查版图几何尺寸是否
6.1.1 计算机辅助设计(CAD)和设计自动 化(DA)
1 设计自动化[DA]
如果计算机能根据集成电路的设计指标要求, 自动完成电路设计和版图设计任务,就称之为设 计自动化(Design Automation)。
2 计算机辅助设计[CAD]
目前在集成电路的设计领域内,只对个别的情况, 例如采用可编程逻辑阵列 (PLA)结构实现的集成 电路,做到了设计自动化。大部分集成电路设计 中,要由 “人”为主导,同时需借助于计算机帮 助入工迅速而准确地完成设计任务。
6.1.2 CAD技术的优点
(a)减轻人工劳动,缩短设计周期:在集成电路版图 设计中要绘制、修改版图并要处理大量数据。
(b)保证设计的正确性:用手工方法绘版图和统计坐 标数据时,在几十万甚至几百万个矩形图形和坐 标数据中山现个别错误几乎是不可避免的。
(c)提高设计质量、节省设计费用:采用CAD技术可 以不必经过投片,而在线路设计阶段可对不同方 案进行计算机模拟分析,选取出较好的方案,并 进而对择优选用的电路进行灵敏度分桥、容差分 析和中心值优化设计,在提高设计质量的同时又 节省了研制费用。
6.2.2 OrCAD/Capture CIS软件
1. Capture CIS软件的构成
6.2.2 OrCAD/Capture CIS软件
第6章 计算机辅助工艺规程设计

齿轮零件的LJBM-1编码
序号 编码 编码 描述 的零 件信 息
1 3 2 0 3 2 4 5 0 2 6 1 7 5 8 0 9 0
10 11 12 13 14 15
4
6
5
6
3
3
齿 圆 外 无 轮 柱 部 外 类 外 表 部 齿 面 功 轮 形 能 状 要 为 素 双 向 台 阶
内 部 表 面 为 单 向 台 阶 通 孔
• 零件信息的描述与输入是CAPP开发中的 第一个,也是最重要的问题。 • 零件信息输入的方法: – CAD图形数据库中直接提取; – 人机交互输入。
设计过程
• 派生式(变异式)系统的设计过程:
选择编 码系统 工艺的 表达
零件 分组
样件 设计
标准工 艺制定 软件 设计
建立数 据库
零件组的划分
• 对于工艺相似、结构形状相似和尺寸相似的一些零 件划分为一个零件加工组,简称零件组; • 工艺和结构相似的零件采用相似的加工方法,尺寸 相似的可以使用同类型和规格的机床和工艺装备。 • 一个零件组一般包含了若干个相似零件,每个相似 零件组用一个样件来代表,该样件的制造方法为标 准工艺规程,全组只能有一个标准工艺规程。
零件出 现频数
20~ 25% 标准件
70%
5~10% 零件复杂程度
相似件
复杂件
机械产品中不同复杂程度零件分布规律
GT的客观基础
机械零件之间存在相似性 这种相似性主要表现在三个方面: 结构(形状、尺寸、精度…) 材料(材质、毛坯、热处理…) 工艺(加工方法、加工设备…) 一次相似性 二次相似性
机的零件属于相似件,构成实施成组技术的 客观基础。
内 部 功 能 要 素 为 环 槽
计算机辅助工艺设计
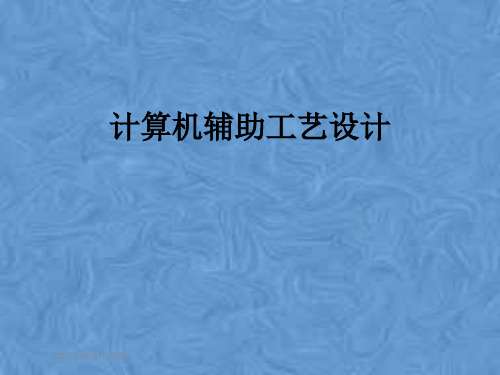
五、CAPP的基本类型
1. 检索式CAPP系统 2. 派生式CAPP系统 3. 创成式CAPP系统 4. 半创成式CAPP系统 5. 广义CAPP系统 6. 智能型CAPP系统
一 计算机辅助工艺设计概念
五、CAPP的基本类型
1. 检索式CAPP系统
2.
适用范围:大批量生产模式,工件种类少,零件变化不大且相似程度 高。
及其 推理。 (8)了解网络化CAPP系统的组成。
一 计算机辅助工艺设计概念
第一节
计算机辅助工艺设计概念
一 计算机辅助工艺设计概念
一、计算机辅助工艺设计的 概念
概念:
计算机辅助工艺设计(Computer Aided Process Planning, CAPP)是利用计算机技术辅助工艺师完成零件 从毛坯到成品的设计和制造过程,是将产品的设计信息转 换为制造信息的一种技术。是通过向计算机输入被加工零 件的几何信息(形状、尺寸等)和工艺信息(材料、热处 理、批量等),由计算机自动输出零件的工艺路线和工序 内容的过程。
一 计算机辅助工艺设计概念
三、CAPP发展背景
传统的工艺设计存在的问题
1. 劳动强度大,效率低 2. 设计周期长,不能适应市场瞬息多变的需求 3. 依赖于工艺师的水平 4. 标准化和最优化差,经验的继承性困难。
一 计算机辅助工艺设计概念
三、CAPP发展背景
工艺在生产制造中的重要地位
市场需求
CAD
一 计算机辅助工艺设计概念
五、CAPP的基本类型
4. 半创成式CAPP系统(综合型CAPP系统)
适用范围:广泛,以单件、中小批量生产企业为主。 工作原理:是派生式和创成式CAPP的综合,采用派生的方法
第六章计算机辅助工艺设计
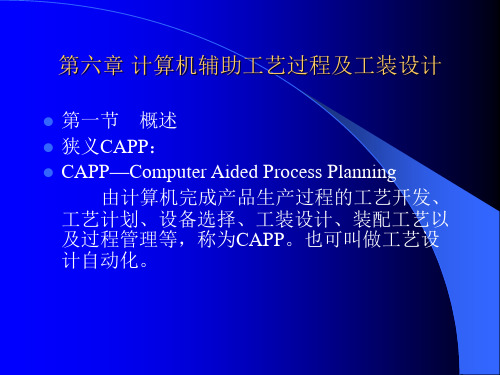
第二节 CAPP系统的工作模式
CAPP的工作过程
第二节 CAPP系统的工作模式
零件信息描述的基本方法:
零件信息描述
零件分类编码描述法 形状特征描述法 语言描述法 知识表示描述法
CAD-CAPP
第二节 CAPP系统的工作模式
零件分类编码描述法:基于成组技术原理, 采用编码系统
特点:简单易行,适用于检索式与派生式 CAPP系统。
编制标准工艺文件存储于数据库,然后,对按 待加工零件的类别检索工艺。
⑵创成法:计算机根据知识库存储的知识, 对待加工零件进行分析,通过推理、决策,确 定加工工艺。
第二节 CAPP系统的工作模式
CAPP系统应具有的基本功能 ⑴检索标准工艺文件 ⑵选择加工方法 ⑶安排工艺路线 ⑷选择机床、刀具、夹具、量具 ⑸选择切削用量 ⑹计算切削参数、加工时间、加工费用 ⑺确定工序尺寸和公差、选择毛坯 ⑻绘制工序图 ⑼给出刀具运动轨迹,自动进行NC编程 ⑽加工过程模拟
缺点:对零件信息的描述过粗,对零件的 具体形状、尺寸、精度描述不是十 分清楚。
第二节 CAPP系统的工作模式
形状特征描述法:将组成零件的各个特征 按一定顺序逐个地输入到计算机中去。
特点:特征与加工方法对应,方便加工方 法确定; 包含了尺寸、公差、粗糙度以及热 处理。
缺点:这种方法比较繁琐、费时。
第二节 CAPP系统的工作模式
第一节 概述
国内主要CAPP软件: 1. 机械加工工艺手册(软件版) 2. 金叶CAPP 3. 开目 CAPP 4. 天河工艺设计(TH-CAPP)
第二节 CAPP系统的工作模式
Capp系统的工作原理 到目前为止,国内外发表的CAPP系统分两大
类,其工作原理为派生法(检索法)和创成法。 ⑴派生法:根据成组技术将工件分类,按类
计算机辅助工艺规程设计(教案)
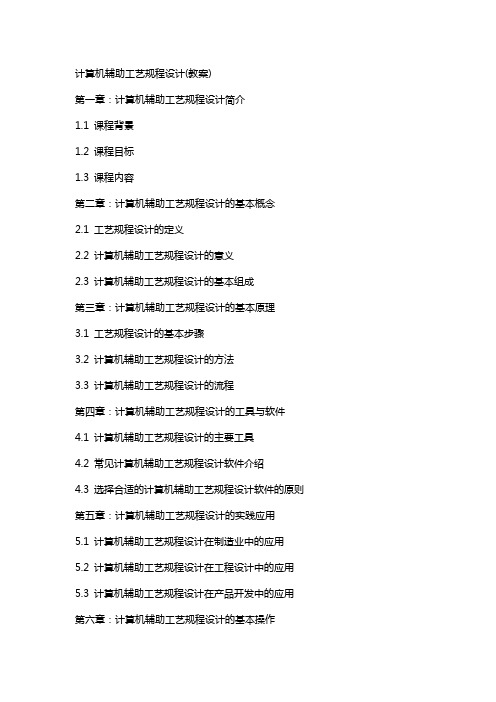
计算机辅助工艺规程设计(教案)第一章:计算机辅助工艺规程设计简介1.1 课程背景1.2 课程目标1.3 课程内容第二章:计算机辅助工艺规程设计的基本概念2.1 工艺规程设计的定义2.2 计算机辅助工艺规程设计的意义2.3 计算机辅助工艺规程设计的基本组成第三章:计算机辅助工艺规程设计的基本原理3.1 工艺规程设计的基本步骤3.2 计算机辅助工艺规程设计的方法3.3 计算机辅助工艺规程设计的流程第四章:计算机辅助工艺规程设计的工具与软件4.1 计算机辅助工艺规程设计的主要工具4.2 常见计算机辅助工艺规程设计软件介绍4.3 选择合适的计算机辅助工艺规程设计软件的原则第五章:计算机辅助工艺规程设计的实践应用5.1 计算机辅助工艺规程设计在制造业中的应用5.2 计算机辅助工艺规程设计在工程设计中的应用5.3 计算机辅助工艺规程设计在产品开发中的应用第六章:计算机辅助工艺规程设计的基本操作6.1 工艺规程设计数据的输入与编辑6.2 工艺规程设计参数的设置与调整6.3 工艺规程设计结果的输出与展示第七章:计算机辅助工艺规程设计的高级应用7.1 复杂工艺规程设计的处理方法7.2 计算机辅助工艺规程设计在协同工作中的应用7.3 计算机辅助工艺规程设计在智能制造中的应用第八章:计算机辅助工艺规程设计的优化与改进8.1 计算机辅助工艺规程设计的效果评价8.2 工艺规程设计中的问题诊断与解决8.3 计算机辅助工艺规程设计的持续优化方法第九章:计算机辅助工艺规程设计的行业案例分析9.1 制造业案例分析9.2 工程设计行业案例分析9.3 产品开发行业案例分析第十章:计算机辅助工艺规程设计的未来发展10.1 计算机辅助工艺规程设计的发展趋势10.2 计算机辅助工艺规程设计在新技术应用中的潜力10.3 计算机辅助工艺规程设计在产业发展中的作用与挑战重点和难点解析一、计算机辅助工艺规程设计的基本概念补充和说明:计算机辅助工艺规程设计通过对生产过程的详细规划和优化,实现生产效率的提升和成本的降低。
第6章计算机辅助工艺规程设计
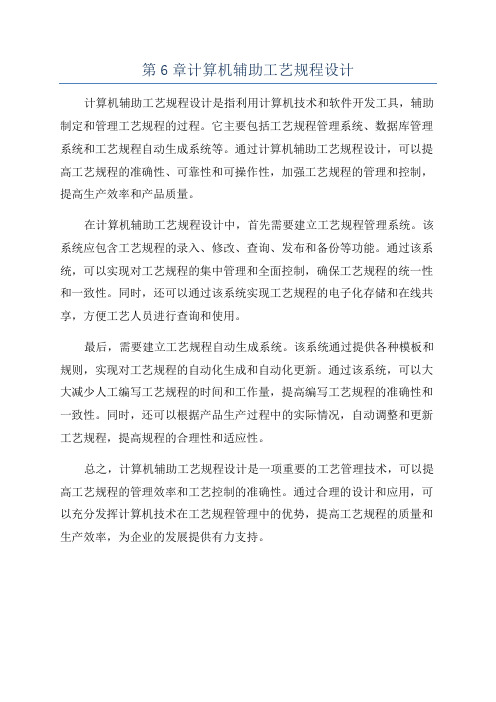
第6章计算机辅助工艺规程设计
计算机辅助工艺规程设计是指利用计算机技术和软件开发工具,辅助制定和管理工艺规程的过程。
它主要包括工艺规程管理系统、数据库管理系统和工艺规程自动生成系统等。
通过计算机辅助工艺规程设计,可以提高工艺规程的准确性、可靠性和可操作性,加强工艺规程的管理和控制,提高生产效率和产品质量。
在计算机辅助工艺规程设计中,首先需要建立工艺规程管理系统。
该系统应包含工艺规程的录入、修改、查询、发布和备份等功能。
通过该系统,可以实现对工艺规程的集中管理和全面控制,确保工艺规程的统一性和一致性。
同时,还可以通过该系统实现工艺规程的电子化存储和在线共享,方便工艺人员进行查询和使用。
最后,需要建立工艺规程自动生成系统。
该系统通过提供各种模板和规则,实现对工艺规程的自动化生成和自动化更新。
通过该系统,可以大大减少人工编写工艺规程的时间和工作量,提高编写工艺规程的准确性和一致性。
同时,还可以根据产品生产过程中的实际情况,自动调整和更新工艺规程,提高规程的合理性和适应性。
总之,计算机辅助工艺规程设计是一项重要的工艺管理技术,可以提高工艺规程的管理效率和工艺控制的准确性。
通过合理的设计和应用,可以充分发挥计算机技术在工艺规程管理中的优势,提高工艺规程的质量和生产效率,为企业的发展提供有力支持。
CADCAM技术_第06章计算机辅助工艺过程设计
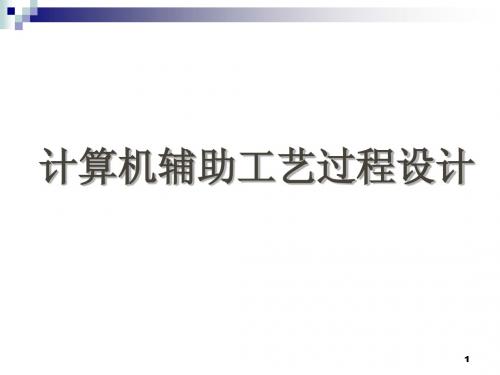
CAPP发展现状
1)CAPP系统零件信息的描述与输入 2)CAPP系统的通用性 3)系统的柔性 4)工艺数据库与知识库的建造 5)探索有效的工艺决策方法和系统结构 6)工序尺寸的自动确定和工序图自动生成
25
CAPP应用环境千差万别 系统应同时具有基于 典型工艺或实例的检索式 设计功能,基于实例的派 生式设计功能与基于知识 的创成式设计等功能满足 不同用户 系统不但能生成面向 普通机床的工艺文件,还 应能生成面向数控加工环 境的工艺文件,输出各种 格式的工艺文件
向CAM提供零件加工所需的设备、 工装、切削参数、装夹参数及反映 零件切削过程的刀具轨迹文件; 接收CAM反馈的工艺修改意见 CAD CAM 工装CAD 向工装CAD提供 工艺过程文件和 工装设计任务书
CAPP 向MIS(管理信息 系统)提供各种工 艺过程文件和夹具、 MIS MAS CAQ 刀具…信息; 接 受MIS反馈的工作 向MAS(制造自动化系统)提供各种过 报告和工艺修改意 程文件和夹具、刀具等信息; 见 接受MAS反馈的工作报告和工艺修改意见
工步 设计 确定 工艺 尺寸 选择 切削 用量
确定工序中的各 工步内容和次序 工时 成本 计算
10
CAPP的主要内容
产品信息建模
信息建模
制造资源建模 工艺数据建模 加工方法选择
工艺决策
切削参数计算 加工顺序安排
……
工艺设计数据 管理与输出
数据浏览与查询
数据输出
11
CAPP系统工作过程与步骤
12
CAPP应用意义:
例如,根据零件的长度和最大直径选取车床;根据零件上待加 工孔的最大直径选取钻床„
工序设计 在工序设计时,首先需要提取当前工序的加工表 面要素,然后再对它们按照一定的工艺决策逻辑进行整理排 序,最后调出标准工序模块进行工序设计,得到详细的工序 内容。
第六章 CAPP讲稿
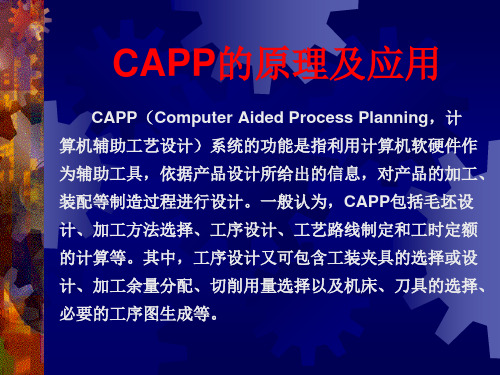
(1) 零件分类编码系统
要揭示与利用零件的相似性,零件的分类编码则必不可少。零件编 码系统是由代表零件的设计和(或)制造特征符号所组成。这些符号代 码可以是数字,也可以是字母,或者两者的组合。在一般情况下,大多 数分类编码系统只使用数字,在成组技术实际应用中,有三种基本编码 结构。
在树式结构中,码位之间是隶属关系,即除第一码位内的特征码外, 其他各码位的确切含义都要根据前一码位来确定(如图6.1a)。由树式 代码组成的层次结构具有相对密实性,能以有限个位数传递大量有关零 件信息。
CAPP系统就其工作原理主要可以分为派生式、创成式、综合式和交 互式四大类。
(1)派生式(Variant)CAPP系统是利用成组技术原理将零件
按几何形状和工艺相似性分类、归组,每一零件组有一个典型样件, 并为典型样件设计出相应的标准样件工艺,存入工艺文件库中。当需 设计一个零件的工艺时,输入零件的成组编码,计算机按此编码检索 出相应的零件组的标准样件工艺,并可根据零件的具体结构及工艺要 求由计算机自动编辑修改或人工编辑修改,从而得到所需的工艺规程。 派生式CAPP系统的基础是成组技术,它依赖于事先归纳整理出来的典 型工艺。
(2)创成式(Generative)CAPP系统由系统中的工艺决策
逻辑与算法根据输入的详细零件特征信息对零件的加工工艺进行一系列 的决策,从无到有自动地生成零件的工艺规程。创成式CAPP系统人工 干预少,自动化程度高,易于保证工艺的一致性和实现优化。但由于零 件结构的多样性、复杂性以及工艺决策逻辑随环境变化等因素的影响, 应用面较宽的全面创成系统还不多见。但创成式CAPP系统代表着 CAPP的发展方向,目前人们较多的是将人工智能技术如专家系统、人 工神经网络、遗传基因算法等引入到CAPP系统的开发中,这为创成式 CAPP系统的研究与开发增添了新的活力。
计算机辅助工艺设计
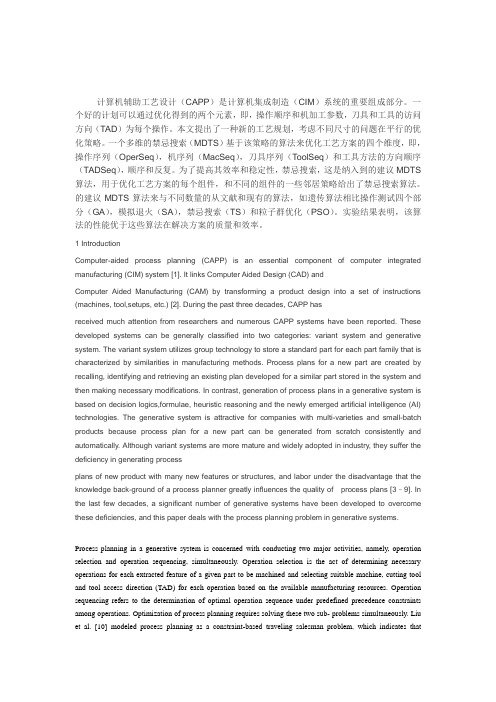
计算机辅助工艺设计(CAPP)是计算机集成制造(CIM)系统的重要组成部分。
一个好的计划可以通过优化得到的两个元素,即,操作顺序和机加工参数,刀具和工具的访问方向(TAD)为每个操作。
本文提出了一种新的工艺规划,考虑不同尺寸的问题在平行的优化策略。
一个多维的禁忌搜索(MDTS)基于该策略的算法来优化工艺方案的四个维度,即,操作序列(OperSeq),机序列(MacSeq),刀具序列(ToolSeq)和工具方法的方向顺序(TADSeq),顺序和反复。
为了提高其效率和稳定性,禁忌搜索,这是纳入到的建议MDTS 算法,用于优化工艺方案的每个组件,和不同的组件的一些邻居策略给出了禁忌搜索算法。
的建议MDTS算法来与不同数量的从文献和现有的算法,如遗传算法相比操作测试四个部分(GA),模拟退火(SA),禁忌搜索(TS)和粒子群优化(PSO)。
实验结果表明,该算法的性能优于这些算法在解决方案的质量和效率。
1 IntroductionComputer-aided process planning (CAPP) is an essential component of computer integrated manufacturing (CIM) system [1]. It links Computer Aided Design (CAD) andComputer Aided Manufacturing (CAM) by transforming a product design into a set of instructions (machines, tool,setups, etc.) [2]. During the past three decades, CAPP hasreceived much attention from researchers and numerous CAPP systems have been reported. These developed systems can be generally classified into two categories: variant system and generative system. The variant system utilizes group technology to store a standard part for each part family that is characterized by similarities in manufacturing methods. Process plans for a new part are created by recalling, identifying and retrieving an existing plan developed for a similar part stored in the system and then making necessary modifications. In contrast, generation of process plans in a generative system is based on decision logics,formulae, heuristic reasoning and the newly emerged artificial intelligence (AI) technologies. The generative system is attractive for companies with multi-varieties and small-batch products because process plan for a new part can be generated from scratch consistently and automatically. Although variant systems are more mature and widely adopted in industry, they suffer the deficiency in generating processplans of new product with many new features or structures, and labor under the disadvantage that the knowledge back-ground of a process planner greatly influences the quality of process plans [3–9]. In the last few decades, a significant number of generative systems have been developed to overcome these deficiencies, and this paper deals with the process planning problem in generative systems.Process planning in a generative system is concerned with conducting two major activities, namely, operation selection and operation sequencing, simultaneously. Operation selection is the act of determining necessary operations for each extracted feature of a given part to be machined and selecting suitable machine, cutting tool and tool access direction (TAD) for each operation based on the available manufacturing resources. Operation sequencing refers to the determination of optimal operation sequence under predefined precedence constraints among operations. Optimization of process planning requires solving these two sub- problems simultaneously. Liu et al. [10] modeled process planning as a constraint-based traveling salesman problem, which indicates thatoptimization of process planning is combinatorially intractable and efficient optimization strategy as well as search algorithm is necessary. The existing literature witnessed emerging applications of optimizationalgorithms to process planning problems in recent years. However, little efforts were made on the improvement of optimization strategy for process planning problem. In this paper, a novel optimization strategy is proposed to address the process planning problem and tabu search (TS) is utilized as the search algorithm to obtain optimal or near-optimal solutions in reasonable computational time. The arrangement of this paper is as follows. Section 2 reviews the existing researches on process planning problem. Section 3 formulates the process planning problem anddifferentiates the optimization strategy proposed in this paper from the existing approaches. The multi-dimensional tabu search algorithm is explained in detail in Section 4 and experiments are presented in Section 5. Conclusions are drawn in Section 6.2 Related workThe process planning problem has been extensively studied in recent years and numerous approaches, including heuristic and metaheuristic algorithms, have been proposed to obtain optimal or near-optimal solutions. Lee et al.[11] suggested optimal and heuristic branch and fathoming algorithms that could give optimal and near-optimal solutionsrespectively. Lee et al. [12] presented three iterative algorithms to solve the operation selection and operation sequencing iteratively until optimal and near-optimal solutions were obtained. In recent years, many metaheuristic algorithms have been applied to process planning problemdue to their superiority in solving combinatorial optimization problems. These algorithms can be categorized as genetic algorithm (GA) [13–21], simulated annealing (SA) [22, 2, 23], tabu search (TS) [24, 25], particle swarm optimization (PSO) [26] and ant colony optimization (ACO) [10].Zhang et al. [13] studied the process planning problem in job shop manufacturing environment and presented a CAPP model that considers various decision making activities simultaneously. GA was selected to obtain near-optimal process plans through specially designed genetic operators. Qiao et al. [14] used GA to optimize the operation sequencefor prismatic parts and four types of process planning rules were considered, namely, precedence rules, clustering rules, adjacent order rules and optimization rules. Alam et al. [16] reported a GA application in a real CAPP system named IMOLD_CAPP for the manufacture of injection moulds. Ding et al. [17] provided an optimization strategy for process planning based on multiple objectives and GA was incorporated with neural network and analytical hierarchical process to facilitate the definition of a globally optimized fitness function. Li et al. [18] studied the process planning problem in distributed manufacturing environments and GA was applied to generate optimal or near-optimal process plans. Hua et al. [20] proposed a GA-based synthesis approach for machining scheme selection and operation sequencing optimization. Salehi and Tavakkoli-Moghaddam [21] divided the process planning into preliminary planning and secondary and detailed planning. The aim of preliminary planning was to generate several feasible operation sequences using GA. Then in the detailed planning stage, the optimized operation sequence and the optimized selection of the machine, tool, and TAD for each operation were obtained using GA again.Ma et al. [2] described an approach that modeled the constraints of process planning problems in a concurrent manner and SA was developed to search for the optimal solution. Li et al. [22] developed a hybrid GA and SA approach to solve the process planning problem with constraints. The GA was carried out in the first stage to generate some good process plans. After that, the SA algorithm was employed to search for alternative optimal or near-optimal process plans based on a few plans selected from the process plans obtained in the first stage. Ma et al. [23] presented a CAPP system based on GA and SA. The developed system provided flexible optimizationcriteria that could satisfy the various needs from different job-shops and/or job-batches. Lee et al. [24] proposed 6 local search heuristics based on SA and TS to obtain good solutions for practically-sized process planning problems within a reasonable amount of computational time. Li et al. [25] developed and embedded a hybrid constraint-handling method in the TS-based optimization algorithm to conduct the search efficiently in a large-size constraint-based space. In their optimization model, costs of the utilized machines and cutting tools, machine changes, tool changes, setups and departure from good manufacturing practices (penalty function) are the optimization evaluation criteria. Guo et al. [26] implemented a PSO approach for optimization of operation sequencing in process planning. Initial process plans were formed and encoded into particles of the PSO algorithm which ‘flew’intelligently in the search space to achieve the best solution. New operators were developed to help PSO explore the search space comprehensively and avoid being trapped into local optima. Most recently, Liu et al.[10] proposed an ACO approach for the optimization of process planning. Firstly, the process planning problem is mapped to a weighted graph and is converted to a constraint-based travelling salesman problem. Then, the mathematical model for process planning problem is constructed by considering the machining constraints and goal of optimization. The ACO algorithm was employed to solve the mathematical model. Results of process planning are feasible process plans that are defined by a sequence of operations and various machining parameters (only machine, tool and TAD are considered in this paper) for each operation. In other words, a process plan has different dimensions. For the process planning problem studied in this paper, process plans have operation dimension and various parameter dimensions (machine dimension, tool dimension and TAD dimension). Operation dimension refers to the operation sequence by which a product is manufactured. And each parameter dimension refers to the parameter sequence selected for each operation. An examination of the existing literature listed above reveals that the algorithms developed for process planning follow the same optimization strategy: an operation together with its machining parameters (machine, tool and TAD) is treated as the basic element to be manipulated by various algorithms which aim at obtaining optimal operation sequence as well as optimal machining parameters for each operation. This optimization strategy is simple and straightforward to encode a process plan to a solution in an algorithm. However, it suffers from the following drawbacks: firstly, since operation and its machining parameters are integrated as a basic unit, algorithms could only search in the solution space of operation dimension. In other words, problem-specific operators must be designed to explore the solution space of machining parameters dimensions of each operation; secondly, there generally exist several machining parameters for each operation, the efficiency of algorithms that are designed for optimizing operation’s machining parameters deteriorates greatly when the number of parameters increases. Different form the existing optimization strategy that operates on the operation dimension of a process plan, this paper proposes a novel strategy that manipulates on operation dimension as well as machining parameters dimensions. Under this optimization strategy, TS is adopted as an underlying search algorithm that is responsible for search in each dimension of the process planning problem. The optimization strategy is further explained in Section 3 using an illustrative example and TS is elaborated in Section 4.3 Process planning problem3.1 Problem definition and illustration using an example part Process planning is concerned with solving two interrelated problems, namely, operation selection and operation sequencing, simultaneously. Operation selection refers to the determination of necessary operations for each manufacturing feature and the selection of machine, tool and TAD for each operation, while operation sequencing is the act of determining an optimal operations sequence subject to predefined precedence constraints among machine features. Process planning is NP-hard due to the numerous possibilities of operation sequence, alternative machining resources for each operation and the existence of complex precedence constraintsamong operations. A prismatic part taken from Zhang et al. [13] is used here to illustrate the process planning problem. This part consists of 5 machining features that could be mapped into 5 operations. The example part is shown in Figure 1 and the available machining resources are given in Table 1.Table 1 shows that there are 5 operations to be conducted for the prismatic part and there exist alternative machining resources for each operation. For example, operation 5 (milling) has one alternative machine (M1), two alternative tools (T2, T6) and two alternative TADs (+z, +y). In addition, the precedence constraints generally exist amongoperations of a process plan. They mainly come from geometric and manufacturing interactions between features as well as technological requirements to produce every feature with the best possible accuracy [13]. These interactions and technological requirements could be summarized as fixture interactions, tool interactions, datum interactions, thin-wall interactions, feature priorities, material-removal interactions and fixed order of machining operations [22]. The precedence constraints for the example part are given as follows: F1 must be drilled before F2, F2 must be machined before F4 and F4 must be drilled before F3. A feasible operation sequence (1, 5, 2, 4, 3) is shown in T able 2. In Table 2, each element in position i of the first row represents the i th operation of the final process plan and each element in the same position of the rest three rows indicates the selected machine, tool and TAD for the i th operation respectively.3.2 The proposed optimization strategyThe process plan shown in Table 2 is used in this section to further describe the proposed optimization strategy. Itcan be seen from Table 2 that a process plan is determined by different dimensions, namely, operation dimension and parameter dimensions (machine dimension, tool dimension and TAD dimension). In traditional optimization strategy developed for process planning, the three parameter dimensions are incorporated into the operation dimension to facilitate optimization of operation dimension. The existing optimization strategy encodes the process plan in Table 2 into a solution in metaheuristic algorithms as [(1, 2, 1, y), (5, 1, 6, +y), (2, 1, 2, +z), (4, 2, 3, z), (3, 1, 4, +y)]. Thefirst element of this encoding scheme represents an operation of the process plan and the rest elements indicate machining parameters of each operation. The existing optimization strategy tries to obtain optimal solutions in the operation dimension, that is, optimal operation sequence. Parameter dimensions are optimized to adapt to operation dimension. The optimization strategy proposed in this paper treats each dimension equally and optimizes different dimensions respectively. The encoded solution of the process plan in Table 2 under our new optimization strategy is [(1, 3,5, 4, 2), (2, 1, 1, 2, 1), (1, 2, 4, 3, 6), (y, +z, +y, z, +y)]. The encoding scheme will be fully explained in the following sections. It is easy to find the differences between the two encoded solutions using different optimization strategies. The optimization strategy considers the four dimensions of process planning equally. We name the four dimensions as operation sequence (OperSeq), machine sequence (MacSeq), tool sequence (ToolSeq) and TAD sequence (TADSeq). The optimization strategy proposed in this paper is based on the separate and iterative optimization of these four dimensions. In other words, in each iteration, the four dimensions are optimized to local optima sequentially and when one dimension is under optimization, theother three dimensions remain unchanged. These four Table 2 A feasible process plan of the example part Operation 1 5 2 4 3Machine 2 1 1 2 1Tool 1 6 2 3 4TAD y +y +z z +ydimensions construct a complete solution of the process planning problem at hand and each dimension cooperates with other dimensions to evaluate its fitness. 3.3 Optimization objectives In this paper, the total weighted cost (TWC) is employed to calculate the fitness of a process plan. The TWC consists of machine cost (MC), tool cost (TC), machine change cost (MCC), tool change cost (TCC) and setup change cost (SCC). Definitions of these costs are provided in Li, et al. [22] and therefore omitted here. The fitness function is computed by [22]1 2 3 4 5TWC w MC w TC w MCC w TCC w SCC, where ( 1, 2, ,5) iw i are the weights for different costs.3.4 Constraints-handling algorithmDue to the existence of various precedence constraints among operations, operation sequence that is generated randomly or manipulated by some search operators may be infeasible. This paper adopts the constraints-handling algorithm proposed by Tseng [27] to adjust infeasible solutions into feasible ones. This algorithm firstly constructs a binary-tree structure based on the precedence relationships among operations. And the feasible solution is obtained by inorderly traversing the binary tree. Notations used in the algorithm are [27]: P, operation sequence; g h, operation inthe hth position of P; r, root node point; l, leaf node point. Details of the constraints adjusting algorithm are described as follow:Step 1. Set h = 2.Step 2. Set gl’s corresponding operation at root nodepoint R.Step 3. Set gh’s corresponding operation at leaf node point l, and decide the precedence relationship of r and l.(1) If pr,l= 1, operation l should be machined before operation r.(a) If r’s left child node point is not empty, then set r’s leftnode point at the new root node point r and repeat Step 3.(b) If r’s left child node point is empty, then insert l at r’sleft node point. Set h = h +1 and go to Step 4.(2) If pr,l= 0, there is no precedence constraints betweenoperations r and l.(a) If r’s right node child node point is not empty, thenset r’s right node point at the new root node point r and goto Step 3.(b) If r’s right child node point is empty, then insert l atr’s right node point. Set h = h +1, and got to Step 4.Step 4. If h = m, go to Step 5; otherwise go to Step 2.Step 5. List feasible solutions according to the inordertraversal rank and stop the algorithm.4 The proposed multi-dimensional tabu search(MDTS) algorithm4.1 The rationaleIn recent years, metaheuristic algorithms have been extensively studied to solve various combinatorial optimization problems. Due to their combinatorial intractability, process planning has attracted researchers to employ metaheuristic algorithms to obtain optimal or near-optimal solutions with reasonable computational cost. As previous section indicates, solution to the process planning problem consists offour dimensions, namely, OperSeq, MacSeq, ToolSeq and TADSeq. Traditional optimization strategy mainly operates on the OperSeq and thus lacks efficiency in exploring Mac-Seq, ToolSeq and TADSeq. However, study of Moriarty and Miikkulainen [28] indicates that several parallel searches for different dimensions of the solution are more efficient than a single search for the entire solution. Metaheuristic algorithms designed based on this hypothesis include symbiotic evolutioanry algorithm (SEA) [29] and endosymbiotic evoluitoanry algorithm (EEA) [30] and have been sucessfully applied to address some scheduling problems including mixed model assembly balancing and sequencing [31], integrated process planning and scheduling [29], process planning [32] and mixed-model U-lines balancing and sequencing [30]. In both SEA and EEA, a separate population is maitained throughout the optimization process for each part of the solution to the problem at hand and additional efforts must be made to design specific cooperations among populations. In addition, maintenance of separate population for each solution component requires more computational time. Based on the above analysis, this paper proposes a multi-dimensional tabu search (MDTS) algorithm to address the process planning problem. The advantage of the proposed MDTS is twofold. First, a single individual is maintained for each dimension of the solution, which could greatly shorten the compuotational time; second, tabu search is utilized to optimize each of the four components, which ensures the efficiency and effectiveness of the proposed MDTS algorithm. Details of the proposedMDTS algorithm are described in the following sections. 4.2 The overall procedure of the MDTS algorithm In the proposed MDTS algorithm, we use OperSeq, MacSeq, ToolSeq and TADSeq to represent the individualmaintained for each component of the solution to the process planning problem. Initially, each individual is generated randomly and assigned to a fitness value. Note that in the problem considered here, the fitness of each individual equals the TWC that is calculated by combining the four individuals to form an entire solution. The overall procedure of the MDTS algorithm is depicted by Figure 2. Procedures of the proposed MDTS algorithm could also be depicted using the following pseudo-code:1) If the termination criterion is not met, repeat the following steps:2) Apply TS to optimize OperSeq and keep MacSeq, ToolSeq and TADSeq unchanged.3) Apply TS to optimize MacSeq and keep OperSeq, ToolSeq and TADSeq unchanged.4) Apply TS to optimize ToolSeq and keep OperSeq, MacSeq and TADSeq unchanged.5) Apply TS to optimize TADSeq and keep OperSeq, MacSeq and ToolSeq unchanged.Note that Figure 2 describes a single iteration of the MDTS algorithm and each oval in Figure 2 indicates one or more steps of tabu search. To be specific, at each iteration one or more steps of tabu search are executed to move one solution dimension (OperSeq, MacSeq, ToolSeq and TAD-Seq) to its neighborhoods. While one dimension progresses, it cooperates with other dimensions to calculate and update its fitness. For example, when the fitness of OperSeq is to be calculated, MacSeq, ToolSeq and TADSeq are selected to cooperate with OperSeq to construct a complete solution.Note that while one dimension is under optimization, the other three dimensions remain unchanged. This process is carried out sequentially for all the solution components. When all the solution components are updated, a cycle completes. The cycle is repeated until the stopping criterion is satisfied. The workflow of the proposed MDTS algorithm is given below and shown in Figure 3.Step 1. Initialize a process plan by randomly generating its OperSeq, MacSeq, ToolSeq and TADSeq. Set the maxi-mum iteration number MaxIter = N and the current iteration CurrIter = 0.Step 2. Initialize a separate tabu list for OperSeq, MacSeq, ToolSeq and TADSeq respectively.Step 3. Conduct the following steps sequentially:a) OperSeq′= TabuSearch (OperSeq, NumOper).b) MacSeq′= TabuSearch (MacSeq, NumMac).c) ToolSeq′= TabuSearch (ToolSeq, NumTool).d) TADSeq′= TabuSearch (TADSeq, NumTAD).e) CurrIter = CurrIter+1.Step 4. If CurrIter>MaxIter, then stop. Otherwise, go toStep 2.In the procedure described above, the function of TabuSearch (parameter 1, parameter 2) is the tabu search algorithm that is used to optimize the four dimensions of process planning problem. TabuSearch (abbreviation for tabu search) receives two parameters, namely, parameter 1 for the individual to be optimized and parameter 2 for the number of steps to be conducted and returns the optimized individual. Note that, tabu search could be replaced by other efficient heuristic algorithms to construct new algorithms. Details of tabu search are elaborated in the next subsection.4.3 The tabu search algorithm The proposed MDTS algorithm employs tabu search to explore the search space of OperSeq, MacSeq, ToolSeq andTADSeq sequentially and iteratively. The tabu search implemented in the proposed MDTS algorithm takes the forms: output=TabuSearch (parameter 1, parameter 2); parameter 1 represents the individual to be optimized; parameter 2 is the number of steps that tabu search is executed. The word “Output”in Figure 4 represents the optimized individual for parameter 1. Details of the tabu search algorithm are described as fol-lows and in Figure 4. Step 1. Assign parameter 1 to the current solution (Curr- Sol). Take parameter 2 as the maximal number of steps (MaxIter) that tabu search will be executed. Let iter = 0. Step 2. Evaluate currentsolution’s fitness by combining it with the other three individuals to form an entire process plan. Assign the current solution to elite solution (EliteSol).Step 3. Generate Nsnumber of neighborhood solutions of the current solution. Evaluate their corresponding fitness by combining each of them with the other three individuals. Choose the best neighborhood solution as the candidatesolution (CandSol). Step 4. If the candidate solution CandSol is better than the elite solution EliteSol, replace the current CurrSol and elite solution EliteSol with the candidate solution CandSol. Add CandSol into the tabu list. Then go to Step 6; otherwise go to Step 5Step 5. If the candidate solution CandSol already exists in the current individual’s tabu list, choose the best neighborhood solution that is not in the tabu list as the candidate solution. Add the candidate solution CandSol to the tabu list and use it to replace the current solution.Step 6. Let iter = iter + 1. If iter>parameter 2, return the current solution CurrSol as the Output. Otherwise, go to Step 3.4.4 Encoding schemeThe process plan given in Table 2 is used here to elaborate the encoding scheme. The encoded process plan is shown in Table 3. The first row in Table 3 indicates the operation number of the process plan. Each column represents an operation with its corresponding machine, tool and TAD. Note that OperSeq indicates the position of an operation in a process plan. For example, operations 1–5 locate in the 1, 3, 5, 4, 2 of the process plan given inTable 2, so the OperSeq is made up of (1, 3, 5, 4, 2). MacSeq, ToolSeq and TADSeq store the information of the operation that the operation number indicates.4.5 Neighborhood structures4.5.1 Neighborhood structure of OperSeqThe neighborhood structure of OperSeq includes Insert and Swap proposed by Li et al. [22]. Insert is to pick one element in the OperSeq randomly and insert it into another position. Swap means to exchange the position of two randomly selected elements of OperSeq. These two neighborhood structures are depicted in Figure 5.4.5.2 Neighborhood structure of MacSeq, ToolSeq andTADSeqThe same neighborhood structure named “single pointmutation”is applied to MacSeq, ToolSeq and TADSeq toobtain neighborhood solutions. It involves selecting a random element from MacSeq, ToolSeq and TADSeq and replaces it with another candidate value. The neighborhood structure of MacSeq, ToolSeq and TADSeq is shown in Figure 6.5 ExperimentsIn this section, the performance and search capability of the proposed MDTS are validated using four parts taken from literature. Parameters of the proposed MDTS algorithm include size of tabu list (Ns), number of steps for OperSeq,MacSeq, ToolSeq and TADSeq (NOper , Nmac, Ntool and N TAD)and maximal number of iteration (MaxIter). Preliminary experiments showed that the following setting provides good results: Ns= 20, Noper= 5, Nmac= Ntool=NTAD= 10 and MaxIter = 200.5.1 Experiment 1A prismatic part (Part 1) taken from Li et al. [22] is firstly used to test the proposed algorithm. Part 1 consists of 14 manufacturing features and 20 operations. Computational experiments are conducted under the following two conditions to demonstrate the advantages of the proposed MDTS to the existing approaches in literature including GA, SA, TS and PSO.1) All machines and tools are available, and w1-w5 in the equation given in Section 3.3 are set as 1.2) All machines and tools are available, and w2=w5=0,w1=w3=w4=1.Under conditions (1) and (2) described in Table 4, 50independent trials were conducted to evaluate the proposed MDTS lgorithm’s performance for Part 1. Table 4 lists the computational results of MDTS, GA, SA, TS on Part 1 under two different conditions. Comparison of MDTS with PSO under condition (1) is also presented. For each algorithm under each condition, 50 trials were conducted to obtain the mean objective value, maximum objective value and minimum objective value. From the observations of Table 4 under conditions (1) and (2), our proposed algorithm has obtained lower mean, maximum and minimum costs. Figure 7 illustrates the optimization process of the proposed MDTS and GA, SA, TS. It can be seen from the figure that GA is prone to local optima in the early stage, SA performs better than GA and TS is superior to both GA and SA. The proposed MDTS outperformed GA, SA and TS both in computational time and efficiency.5.2 Experiment 2The second part used by Li et al. [25] consists of 14 features and 14 operations. Two conditions are considered for studies on this part. 1) All machines and tools are available, and w1w5 in theequation given in Section 3.3 are set as 1.2) All machines and tools are available, and w2=w5=0,w1=w3=w4=1.Table 5 lists the computational results in terms of mean objective value, maximum objective value and minimum objective value of 50 runs. The results of MDTS are based on 50 independent runs with randomly generated initial plan. Results of GA, SA and TS are obtained from Li et al. [25]. It can be seen from Table 5 that results obtained by the proposed MDTS with that obtained previously by Li et al. [25] for the lowest machining cost under conditions (1) and (2) are the same; but the mean and maximum machining costs under both conditions obtained by our proposed MDTS outperform GA, SA and TS. Figure 7 Comparison of MDTS with GA, SA and TS. 5.3 Experiment 3 The third part presented by Ma et al. [2] consists of 9 features and 13 operations. The following two conditions are considered for studies on Part 3.1) All machines and tools are available;2) M2 is down.Computational results of MDTS and SA under the two conditions are listed in Table 6. Table 6 shows that the proposed MDTS algorithm can find better solutions in both conditions.5.4 Experiment 4。
推荐-6计算机辅助工艺设计 精品
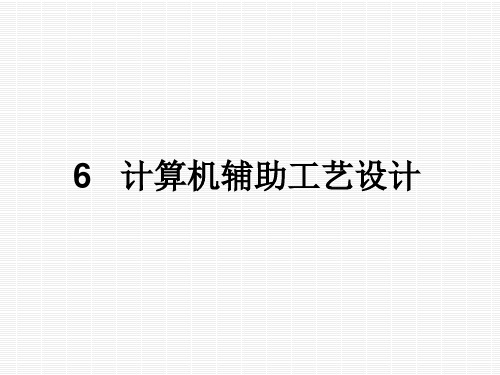
6.1 计算机辅助工艺规程设计 6.1.1 CAPP的基本概念
零件分类编码:
是建立在成组技术(Group Technology ,简称GT)基础上的 一种方法。利用成组技术编码来描述一个零件,其实质是用 数字描述零件的几何形状、尺寸大小、材料和工艺特征,也 就是零件特征数字化。
成组技术:将企业生产的多种产品、部件和零件,按照一定
4
6.1 计算机辅助工艺规程设计 6.1.1 CAPP的基本概念
2.传统工艺设计方法的缺点
‧经验积累 ‧手工方式:查资料、手册 来进行工艺计算、绘
工序图、填写工艺卡片及表格。 ‧不足之处:
人工编制,劳动强度大,效率低; 设计周期长; 经验性很强; 工艺设计优化、标准化很差。
5
6.1 计算机辅助工艺规程设计 6.1.1 CAPP的基本概念 3.现代制造的特点
条件及参数等;
3
6.1 计算机辅助工艺规程设计 6.1.1 CAPP的基本概念
b.工艺规程设计主要内容有:
‧选择加工方法、机床和工装设备。 ‧安排合理的加工工序; ‧选择定位基准、确定各工序的加工余量和毛坯,计算工艺尺
寸和公差; ‧选用合理的切削用量; ‧计算工时定额和加工成本; ‧编写各类工艺文件等
实际上,这也是目前CAPP系统难以做到通用的主要原因。
9
6.1 计算机辅助工艺规程设计 6.1.1 CAPP的基本概念
5. CAPP的主要功能
(1) 自动选择毛坯和材料; (2) 加工方法、加工设备、加工用量的选择; (3) 工序编排与工艺安排的决策; (4) 工艺尺寸换算,工时定额计算及NC编程等。 (5) 确定重要工序的质量检测项目和检测方法等。
的相似性准则分类成组,并以这种分组为基础组织生产的全 过程,从而实现产品设计、制造和生产管理的合理化及高效 益。
第6章 CAPP应用
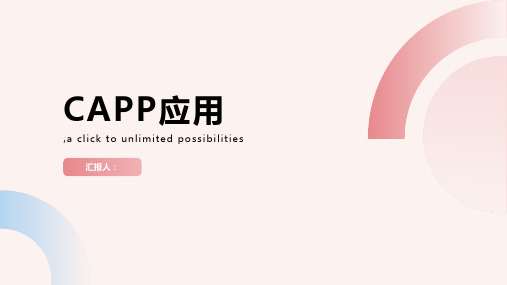
数据安全问题:CAPP涉及大量制 造数据,如何保证数据安全和隐私 是一个重要问题
添加标题
添加标题
添加标题
添加标题
集成难度高:CAPP需要与CAD、 CAM等其他制造系统进行集成,实 现数据共享和流程优化
人才短缺:具备CAPP技能和经验 的人才相对较少,需要加强人才培 养和引进
CAPP的发展趋势和未来展 望
第六章
CAPP的发展趋势
CAPP与CAD、CAM的集成 CAPP系统的智能化 CAPP系统的网络化 CAPP系统的标准化
CAPP的未来展望
智能化发展:利用人工智能技术提高CAPP的自动化程度和智能化水平
云化发展:将CAPP系统迁移到云端,实现更加灵活、高效、可靠的应用 定制化发展:根据不同行业和企业的需求,提供更加定制化的CAPP解决 方案 集成化发展:与其他制造信息系统进行集成,实现信息共享和流程优化
CAPP技术将促 进企业数字化转 型,提高企业核 心竞争力
感谢您的观看
汇报人:
工艺优化
工艺流程设计:根据产品特性和生产需求,优化工艺流程,提高生产效率 工艺参数优化:通过对工艺参数的调整和优化,提高产品质量和稳定性 工艺资源管理:对工艺资源进行合理配置和调度,确保生产过程的顺利进行 工艺知识库建设:积累和整理工艺知识,为工艺设计和优化提供支持
CAPP的应用场景
第四章
总结与展望
第七章
对CAPP的总结
CAPP的定义、 发展历程和应用 领域
CAPP的核心技 术和关键功能
CAPP在生产制 造中的应用和优 势
CAPP的未来发 展趋势和挑战
对CAPP的展望
CAPP技术将不 断进步,提高生 产效率和产品质 量
CAPP系统将更 加智能化,实现 自动化和智能化 融合
计算机辅助工艺规程
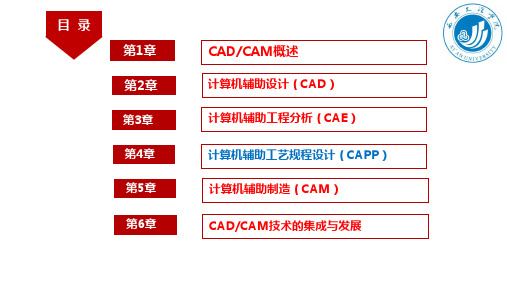
4.1.2 CAPP 的发展历程
1965年 1969年
20世纪60 年代末
20世纪 70年代
20世纪 80年代
2003年
Nibe首先提出了 CAPP的概念
挪威开发出世界上第一 套具有实用价值的 Auto-Pros系统
美国CAM-I公司推出 APP系统
建造BOM
截止1989 年末,国 际上已开 发出近 200个 CAPP系统
04 计算机辅助工艺规程设计(CAPP)
4.1.3 CAPP 的基本模式和原理
1. 派生式(Variant)CAPP系统
2. 创成式(Generative)CAPP系统
3. 综合式CAPP系统
4. CAPP专家系统
• 利用零件的相似性,相似的零 件有相似的工艺过程;一个新 零件的工艺规程是通过检索相 似零件的工艺规程并加以筛选 或编辑而成。由此得到“派生” 这个名称。派生式工艺过程设 计系统也称为检索式、修订式 或变异式工艺过程设计系统。
• 实现CAD/CAPP/CAM系统的集成化 • 实现基于企业信息的集成化 • CAPP与上游CAD系统实现产品信息的双向交流和传送,与下游生产调度系统、质
量控制系统等不同的企业生产管理信息系统建立起内在联系
通用化:满足不同产品企业类型、不同生产规 模、不同企业部门的工艺设计和工艺管理的基 本需求
• 建立通用CAPP系统的基本结构 • 建立通用CAPP系统的基本工作流程 • 建立通用CAPP系统的标准的用户界面
• 1)系统原理简单,易于实现,继 承和应用了企业成熟的传统工艺, 而且发展较早,在应用方面有一定 优势。
• 2)派生式CAPP计算机存储的是一 些标准工艺过程和标准工序,而不 是工艺决策逻辑。
计算机辅助工艺规划

工序图的生成与绘制
工序图的生成与绘制是CAPP系统研制和开发的一个 关键性技术难题。是一种动态的过程。
当工件形状、尺寸改变时,相应的绘图信息参数也要 改变。
工序图实际上是结合零件图形信息和工艺信息过程。 从功能上看,工序图生成可分为信息转换、图形生成、
– 对零件组内各零件的工艺要仔细分析、概括、 总结,要考虑各种因素并征求有关人员意见。
– 典型工艺要包含详细的工序内容。
– 以数据库的形式存放典型工艺,以便存储、检 索、编辑、输出。
3. 工艺规程的生成和编辑
1. 对设计零件按所选分类编码系统进行编码, 完成对零件的描述;
2. 输入零件信息; 3. 检索并判断新零件属于的零件族; 4. 调出典型工艺,包括工艺路线和工序内容; 5. 对典型工艺进行修改编辑,生成新零件的
工艺规程设计是工厂工艺部门的一项经 常性工作,是连接产品设计和制造的中 间环节
传统工艺规程设计是实践经验丰富的工 艺工程师担任
计算机辅助工艺过程设计,可以取代大 量的人工劳动
CAPP上于CAD相接,下于CAM相接。 形成一种新的现代设计与制造技术。
6.2.1计算机辅助工艺过程设计的 意义
(1)代替工艺设计人员的手工劳动
– 零件信息包括两方面:几何信息和工艺信息 – 按组成结构分:分类法、型面法、形体法等(表6-27) – 从描述结构分:编码描述、语言描述、数学描述。
零件信息输入
– 人机交互式信息输入 – 从CAD系统中提取零件信息。这要求统一的数据交换
标准和数据管理规定。
6.3.2 派生式CAPP系统
利用成组技术中的零件相似性原理进行设计,通过 编辑、修改相似零件的典型工艺,派生出新零件的 工艺过程。也称变异法、样件法
第六章 计算机辅助工艺设计与先进制造技术
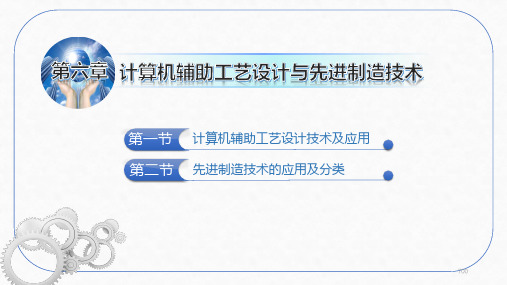
2.CAPP 的基本原理 计算机辅助工艺设计系统就是按以上人工设计工艺规程的四个阶段进行工 艺规程设计的。如图所示为 CAPP 系统进行工艺规程设计的流程图。从图可 以清楚地看出:第一,将零件的特征信息以代码或数据的形式输入计算机, 并建立起零件信息的数据库;第二,把工艺人员编制工艺的经验、工艺知识 和逻辑思想以工艺决策规则的形式输入计算机,建立起工艺决策规则库(工 艺知识库);第三,把制造资源、工艺参数以适当的形式输入计算机,建立 起制造资源和工艺参数库;第四,通过程序设计充分利用计算机的计算、逻 辑分析、判断、存储以及查询、编辑等功能来自动生成工艺规程。这就是 CAPP 的基本原理。
708
4.工序决策模块 工序决策模块的主要任务是生成工序卡,计算工序尺寸,生成工序图。 5.工步决策模块 工步决策模块的主要任务是对工步内容进行设计,确定切削用量,提供形 成 NC 加工控制指令所需的刀位文件。 6.NC 加工指令生成模块 NC 加工指令生成模块依据工步决策模块所提供的刀位文件,调用 NC 代 码库中适用于具体机床的 NC 指令代码系统,产生 NC 加工控制指令。
705
二、计算机辅助工艺设计系统的体系结构
计算机辅助工艺设计(CAPP)系统的体系结构,视其工作原理、功能要 求、产品对象、规模大小不同而有较大的差异。根据 CAD/CAPP/CAM 集成 的要求,CAPP 系统由控制模块、零件信息输入模块、工艺规程设计模块、工 序决策模块、工步决策模块、NC 加工指令生成模块、工艺文件管理和输出模 块、加工过程动态仿真、工艺数据库和知识库等基本模块组成。如图所示为 计算机辅助工艺设计系统的体系结构。
704
由于计算机集成制造系统(CIMS)的出现,计算机辅助工艺设计(CAPP) 上与计算机辅助设计(computer aided design,简称 CAD)相接,下与计算机 辅助制造(computer aided manufacturing,简称 CAM)相连,是连接设计与制 造之间的桥梁,产品设计信息只能通过工艺设计才能生成制造信息,产品设 计只能通过工艺设计才能与制造过程实现功能和信息的集成。由此可见 CAPP 在实现生产自动化中的重要地位。
第六章计算机辅助工艺规程设计
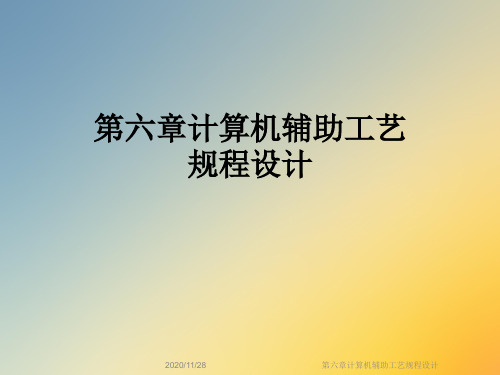
6.2.3 派生法CAPP系统实例
1. TOJICAP系统:该系统用于生成回转体零件 的工艺规程。工艺规程内容详细完整,系统 采用BASIC语言编程。
2. WLCAPP系统:WLCAPP系统是北京理工大 学为航天部某卫星制造厂开发的派生CAPP系 统,采用FOXBASE为主要开发工具,运行环 境为各类微机。整个系统有计算机辅助编码 子系统和计算机辅助工艺设计子系统组成, 通过分级下拉式菜单驱动各模块的运行。
派生法CAPP系统的工作原理:是根据成组 技术中相似性原理,如果零件的结构形状相似, 则它们的工艺规程也有相似性。
第六章计算机辅助工艺规程设计
6.2.2派生法CAPP系统的研制过程
1.零件编码 :首先对已有的零件进行编码。 编码方法有手工编码和计算机辅助编码两种 方法。 手工编码是编码人员根据分类系统的编 码法则,对照零件图用人工方式逐一编出各 码位的代码。 计算机辅助编码以人机对话方式进行, 把零件的信息输入给计算机,计算机软件进 行逻辑判断并自动编出零件的代码,并在终 端显示器上显示或打印输出。
第六章计算机辅助工艺规程设计
创成法CAPP系统的工作原理
目前技术上存在的困难 ¡ 零件图样上的各种信息要完全难确地描述还有在
困难,持别是对复杂零件的三维模型的建立也还 没有完全解决。 ¡ 工艺知识是一种经验型知识,如何建立完善的工 艺决策模型,使计算机能够识别、处理还有待进 一步解决。 ¡ 工艺过程的优化理论还不完善,没有严格的理论 和数学模型。
标准工艺规程是由各种加工工序组成的,一个工 序又可以分为多个工步,所以工步是标准工艺规程中 最基本的组成要素。如车外圆、钻孔、铣平面、磨外 圆、滚齿、拉花键等。
第六章计算机辅助工艺规程设计
计算机辅助工艺设计
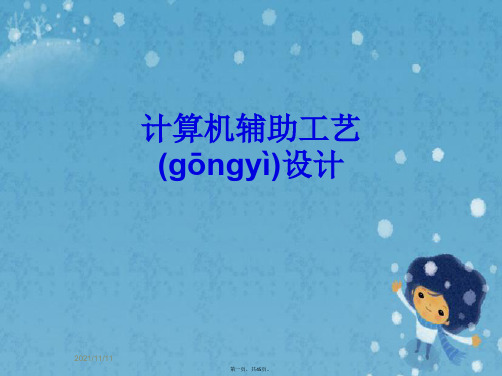
6.1 计算机辅助工艺规程(guīchéng)设 计6.1.1 CAPP的基本概念
假如计算机读取的零件信息中部分信息超出了计算机能识别处理的 范围,即找不到零件对应的零件族,或不能用预先确定的逻辑生 成工艺时,则计算机将无能为力,只能报错。
所以,计算机只能按CAPP软件规定的方式生成工艺过程,而不能 创造新的工艺方法和新的加工参数。一旦新的工艺方法或新的加 工参数出现(chūxiàn),就必须修改CAPP系统中的某些部分,使 之适应新的加工制造环境。
出来; 提高企业工艺设计的规范化、标准化水平,促进工艺设
计水平的提高; 能有效的积累和继承工艺设计人员的经验,提高企业工
艺设计的继承性。
第十六页,共45页。
6.1 计算机辅助工艺(gōngyì)规程设计 6.1.1 CAPP的基本概念
7.CAPP的作用与意义 为现代制造系统集成提供技术桥梁 与计算机辅助设计(shèjì)(CAD)系统之间的信息交
第二十三页,共45页。
6.1 计算机辅助工艺(gōngyì)规程设 计6.1.2 CAPP系统(xìtǒng)的分 类6.1.2.2 创成式CAPP系统 (Generative)
创成式CAPP系统与派生式系统不同,它的生成并不是通过修改 或编辑相似零件组的工艺规程实现的,而是靠系统中的决策逻 辑生成一个新零件的工艺规程。
艺尺寸和公差; ‧选用合理的切削用量; ‧计算(jì suàn)工时定额和加工成本; ‧编写各类工艺文件等
第四页,共45页。
6.1 计算机辅助工艺规程(guīchéng)设计
6.1.1 CAPP的基本概念
2.传统工艺设计方法的缺点 ‧经验积累 ‧手工方式:查资料、手册 来进行(jìnxíng)工艺计算、绘
- 1、下载文档前请自行甄别文档内容的完整性,平台不提供额外的编辑、内容补充、找答案等附加服务。
- 2、"仅部分预览"的文档,不可在线预览部分如存在完整性等问题,可反馈申请退款(可完整预览的文档不适用该条件!)。
- 3、如文档侵犯您的权益,请联系客服反馈,我们会尽快为您处理(人工客服工作时间:9:00-18:30)。
§6.2 CAPP系统的工作模式
常用的分类编码系统
Opitz系统是一个十进制九位代码的混合结构分 类编码系统。它由德国亚琛(Aachen)工业大学 H.Opitz教授提出的,在成组技术领域是最著名的 分类编码系统。 KK-3系统是日本通产省机械设计研究所提出的 草案,是十进制21位代码的混合结构系统。
§6.1 概述
零件信息的描述与输入
零件信息的描述和输入是CAPP系统的重要组成部分。 零件信息常用的输入方式主要有人机交互输入和从CAD 造型系统提供的模型中直接获取两种方法。 零件信息主要包括零件的几何信息和工艺信息,还有生 产管理信息。
§6.1 概述
零件信息的描述方法
(1)零件分类编码描述法 是基于成组技术原理,方法简单,适用于变异式CAPP系统,无 法完整的描述零件的具体形状、尺寸、精度等信息,当码位很长时 编码效率低。又出现了柔性编码法。 (2)形状特征描述法 任何零件都由一个或若干个形状特征组成,如圆柱面、圆锥面、 螺纹、槽等,按一定顺序输入计算机。 根据零件特征,以具有明显的工程语义的实体来描述零件。在 许多CAPP系统中得到应用。 (3)直接从CAD系统获得零件信息 将CAPP与CAD系统直接相连,使CAPP所需的各种信息直接来源于 CAD系统。
§6.1 概述
CAPP发展趋势
早期的CAPP系统,以工艺卡片的检索为主(变异式 CAPP系统)。 创成式CAPP系统,半创成式CAPP系统。 80年代中期开始CAPP专家系统的研究工作。
CAPP的应用范围:工艺设计、工序设计、工装设计等。 CAPP的发展趋势:集成化、通用化、智能化。
§6.1 概述
开目CAPP
§6.2 CAPP系统的工作模式
变异式CAPP系统
工作原理 变异式CAPP系统利用成组技术原理,将零 件按几何形状及工艺相似性分类、归族,每一 族有一个典型样件。根据此样件建立典型工艺 文件,即典型工艺规程,存入工艺文件库中。 当需设计一个新的零件工艺规程时,按照其成 组编码,确定其所属零件族,由计算机检索出 相应零件族的典型工艺规程,再根据零件的具 体要求,对典型工艺进行修改,最后得到所需 要的工艺规程。
§6.2 CAPP系统的工作模式
成组技术的优点: 1)有利于零件设计的标准化,减少设计工作的重复性。 利用成组技术的相似性原理,在对零件进行分类成组的基础上, 可以将零件族存储起来。在设计时,检索出相似的零件族,经过编辑 修改而形成新的零件,这样不仅可以大大减少设计工作量,还可以最 大限度地利用已有的设计信息。 2)有利于工艺设计的标准化。 在对零件进行分类成组时,是以工艺相似性为特征进行分类。 3)有利于降低成本、简化生产计划、缩短生产周期。 成组技术可以使零件图的种类减少、结构相似性增加,零件工艺 规程数量减少,机床调整准备时间缩短,工艺装备种类减少、机床利 用率显著提高。统计表明,采用成组技术后,零件设计工作量减少50%, 零件图纸减少10%,车间面积减少20%,加工设备数量减少60%,原材料 费用降低40%,生产准备时间减少70%。
§6.1 概述
CAPP与先进制造系统的其他子系统的联系 MIS
CAD
CAPP
CAM
MAS
工装CAD
CAQ
CAPP与其他子系统的信息流
§6.1 概述
为现代制造系统集成提供技术桥梁 CAPP系统接受计算机辅助设计(CAD)系统的产品几 何拓扑、材料信息以及精度、表面粗糙度等工艺信息;向 CAD系统反馈产品的结构工艺性评价信息。 向计算机辅助制造系统(CAM)提供零件加工所需的 设备、工装、切削参数、加工过程以及反映零件切削过程 的刀具轨迹文件;同时接收CAM反馈的工艺修改意见。
§6.1 概述
向工装CAD提供工艺过程文件和工装设计任务书。 向管理信息系统(MIS)提供工艺过程、设备、工装、 材料定额等信息 。同时,接收MIS的信息。 向制造自动化系统(MAS)提供各种加工工艺过程文件、 所需的工艺装备以及NC加工指令等信息。同时,接收MAS 的信息。 向质量保证系统(CAQ)提供工序、设备、工装、检测 等工艺数据,以生成质量控制计划和质量检测规程。同时 接收CAQ反馈的数据。
成组技术的基本原理是对相似的零件进行识别和分 制造中充分利用这些相似特征,以获得所期望的技术经济 效益。 零件的相似性有两类:设计性质方面的相似性和制造 方面的相似性。它的应用不仅仅局限于机械加工领域,也 可用于产品零件设计、工艺设计、工厂设计、市场预测、 劳动量测定、生产管理等各个领域,是一项贯穿整个生产 过程的综合性技术。
第六章
计算机辅助工艺设计
§6 计算机辅助工艺设计 本章要点
• • •
概述 CAPP系统的工作模式 基于知识的CAPP系统
§6.1 概述
CAPP的由来
长期以来,工艺过程设计工作都是采用手工方式逐件 进行零件工艺设计,工艺设计的质量在很大程度上取决于 工艺人员的个人主观因素。所以,工艺设计工作既富于极 大的灵活性,又缺乏慎密的科学性,带有很强的个人经验 色彩。 传统的工艺设计方法是逐件地设计企业自制基本零件 的工艺,这样设计得到的零件工艺称为单独工艺。传统的 设计设计方法带来了两个主要问题: 1)工艺设计工作的重复性 2)同类零件的工艺多样性
§6.1 概述
20世纪80年代中期,创成式CAPP系统的研究转向具有人 工智能的专家系统方面,这是CAPP未来的发展趋势。系统可 以模仿工艺专家的逻辑思维方式,利用专家的知识对非确定 性的工艺过程设计进行逻辑决策。目前,各国学者正致力于 专家系统的实用化和工具化。 目前,CAPP系统主要应用于零件的机械加工方面,但 已逐渐向其它工艺领域扩展,如热处理、锻造、焊接、冲压 和装配等,应用前景是广阔的。
§6.1 概述
CAPP系统的结构组成
人机交互界面
零件信息 获取
工艺决策
工艺文件 管理/输出
零件 信息库
工艺 数据库
工艺 文件库
§6.1 概述
CAPP系统的分类
CAPP系统目前常用的可分为变异式、创成式和综合 式三大类。 变异式(也叫派生式、修订型):理论基础比较成熟, 应用也较成熟,目前已开发的大部分的CAPP系统都属于 这种类型。 创成式:利用创成原理开发应用系统困难较大,理论不 太完善。在已开发的大部分应用系统只是部分应用了创 成法原理。 综合式:也称半创成式CAPP系统,综合派生式CAPP和创 成式CAPP的方法和原理。
§6.2 CAPP系统的工作模式
变异式CAPP系统的主要功能模块
1、零件信息检索 2、零件信息输入 3、零件成组编码 4、典型工艺搜索模块 5、工艺编辑模块 6、工艺设计过程管理 7、工艺文件输出 8、CAPP相关工具
§6.2 CAPP系统的工作模式
变异式CAPP系统的特点和应用
是以成组技术为理论基础,理论上比较成熟; 变异式CAPP系统问世较早,应用范围较广,有较好的实 用性。 主要适用于结构比较简单的零件,在回转类零件中应用 尤为普遍。 与常规手工工艺设计方法类似,集成和应用了企业较成 熟的传统工艺,但系统的柔性较差。 对于复杂零件和相似性较差的零件,由于难以用编码描 述,难以形成零件族,不适宜采用变异式CAPP系统。
§6.2 CAPP系统的工作模式
零件分类编码 零件分类编码是指利用成组技术正确抽 出零件图纸中为制定工艺过程所必需的必要 信息。由代表设计或制造的特征符号组成。 符号代码可以是数字,也可以是字母,或两 者都有。
§6.2 CAPP系统的工作模式
编码结构 三种基本编码结构: (1)层次结构 后级符号的意义取决于前级符号的值。是一种树状结 构,也称单码结构。 (2)链式结构 码位上每个数字代表的含义是固定的,与其它各位的 码值无关,亦称多码结构。 (3)混合结构 层次结构和链式结构的综合应用。
§6.1 概述
国内CAPP研发与应用简介
国内多数采用变异式CAPP原理,目前国内尚没有 较成熟的、实用的创成式CAPP 系统供企业使用。 国内的CAPP软件: 1、机械加工工艺手册(软件版) 2、金叶CAPP 3、开目CAPP 4、天河工艺设计(TH-CAPP)
§6.1 概述
开目CAPP
§6.1 概述
§6.2 CAPP系统的工作模式
零件族的分类 怎样将大量的零件分类成组形成零件族,是成组技术要解 决的关键问题之一,通常有三种方法: 1)直接观察法 是通过人工方式来识别零件进行分类成组的。这种方法 往往需要有实际经验的工程技术人员来完成,和人员的知识水 平关系密切,分类粗糙、不准确。 2)分类编码法 是通过分类编码系统对零件进行分类。这种方法首先将 待分类的零件进行编码,利用编码所反映的零件特征来确定零 件的相似性,从而将零件进行分组。 3)生产流程分析法 是通过对现有零件的加工工艺流程进行分析,把具有相 似或相同加工工序和加工工艺流程的零件聚合在一起构成零件 族(工艺族)。
§6.1 概述
CAPP系统
工艺卡
图纸
要解决上述两个主要问题的有效途径就是利用计算机来进 行工艺过程设计工作,即计算机辅助工艺过程设计CAPP。CAPP 就是利用计算机来完成工艺设计工作中的部分或全部工作,包 括拟定工艺路线、选择机床及工夹量具、计算切削用量、确定 工时定额等。 CAPP能迅速编制出完整而详尽的工艺文件,可以使工艺人 员从繁重的重复性工作中解脱出来,同时可很好地保证工艺的 一致性。
§6.1 概述
CAPP技术现状
CAPP从上世纪60年代末期研制,到目前已研制 了很多CAPP系统,其中有不少的系统投入了实际使 用中。但目前世界各国的CAPP系统还主要用于回转 类零件、棱柱类零件和板块类零件的工艺设计,适 用于非回转类零件的CAPP系统很少,且多用于单件 小批量生产类型。
§6.1 概述
JLBM-1系统是原机械工业部开发的,一个十 进制15位代码的混合结构分类编码系统。结合了 Opitz系统和KK-3系统。
§6.2 CAPP系统的工作模式