2015数学建模竞赛优秀论文
2015年高教杯大学生数学建模竞赛省奖论文

5
图 2 出租车空载率与出租车需求数量之间的关系图 汽车空载率是通过在人流量较为密集的区域选取几个观测点, 对单位时间通 过道路的空载出租车数量占总通过出租车数量的比值,计算公式为: N (5.3) K 1 100% N 根据我国城市出租车管理协会对国内外一些城市的调查统计得出, 当出租车 的空载率在 30%左右时,城市出租车客运需求和供应几乎达到供求匹配状态,此 时出租车资源能够得到合理地利用,出行行人可以在较合理的时间内打上车,从 而达到整体的一个供求平衡的目的。 5.2 模型求解 本文采用问卷的形式对乘客能够接受最长等待时间进行调查, 调查对象是城 市街道中随机遇到的人群。 芜湖市民打车可接受等车时间调查 调查姓名_______________调查时间__________________调查________________
2
§3 模型的假设
1.出租车不会拒载、搭顺风车等情况 2.城市出行人数不受节假日、天气等不可抗拒因素影响 3.出租车均烧汽油 4.出租车驾驶员的支出只有交给出租车公司的份子钱和油耗 5.出租车每公里的油耗相同 6.每位乘客每次乘出租车行驶距离不超过 30 公里 7.忽略出租车每月维修、维护、保险等费用 8.汽车的油价认定为 6 元每升
1. 请问,您乘坐出租车等候的时间一般是 A.5 分钟以内 B.5—10 分钟
? D. 15—20 分钟
C. 10—15 分钟
2. 请问,您可以接受的等车时间__________?
最后,需要了解您部分个人情况,仅供资料分析,希望可以得到您的理解和配合。 Q1.记录被访者性别: 男 女 Q2.请问你的年龄______________ Q3.请问你的工作性质______________
§2 问题的分析
2015数学建模竞赛优秀论文
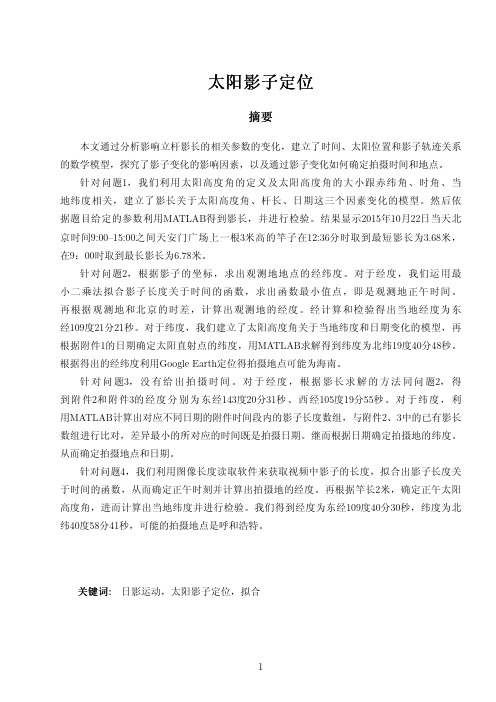
图 2 太阳高度角
由三角形性质,显然,
OB
tan θ =
(1)
OA
即得,
OB H
L = OA =
=
(2)
tan θ tan θ
根据参考文献[1],太阳高度角θ的计算公式为:
sin θ = sin φ sin δ + cos φ cos δ cos σ
(3)
其中,φ为观测地地理纬度,δ为赤纬角,σ为时角。 参考文献[2]:所谓日面中心的时角,即从观测点天球子午圈沿天赤道量至太阳所在时圈的
图 1 夏半年日影运动
由于太阳和地球最短距离为1.471 × 108km,所以太阳光接近地球表面时可以近似看成 是平行光。参考文献[1],太阳高度角是指太阳光的入射方向和地平面之间的夹角,专业上 讲太阳高度角是指某地太阳光线与通过该地与地心相连的地表切线的夹角。如图(2)所 示,OB为竿长,OA为影长,θ即为太阳高度角。
4. 模型的建立
4.1. 问题一模型的建立
4.1.1. 立杆影长随参数变化的模型的建立 为了探求不同时间、不同经纬度下立杆影长的变化规律,我们建立以立杆为参考系的数
学模型。一年四季中除去春分、夏至、秋分、冬至以外,太阳相对于地球都不是严格由正东 向正西方向运动,因此立杆的影子变化不仅在于长度的改变,方向也在改变。同一天,随着 时间的推移,立杆的影子顶点应当是一个弧状轨迹。如图(1),为夏半年日影运动静态模 拟图。图中白色虚线表示影子顶点运动的部分轨迹。
太阳影子定位
摘要
本文通过分析影响立杆影长的相关参数的变化,建立了时间、太阳位置和影子轨迹关系 的数学模型,探究了影子变化的影响因素,以及通过影子变化如何确定拍摄时间和地点。
针 对 问 题1, 我 们 利 用 太 阳 高 度 角 的 定 义 及 太 阳 高 度 角 的 大 小 跟 赤 纬 角 、 时 角 、 当 地纬度相关,建立了影长关于太阳高度角、杆长、日期这三个因素变化的模型。然后依 据题目给定的参数利用MATLAB得到影长,并进行检验。结果显示2015年10月22日当天北 京时间9:00–15:00之间天安门广场上一根3米高的竿子在12:36分时取到最短影长为3.68米, 在9:00时取到最长影长为6.78米。
东三省数学建模比赛C题一等奖
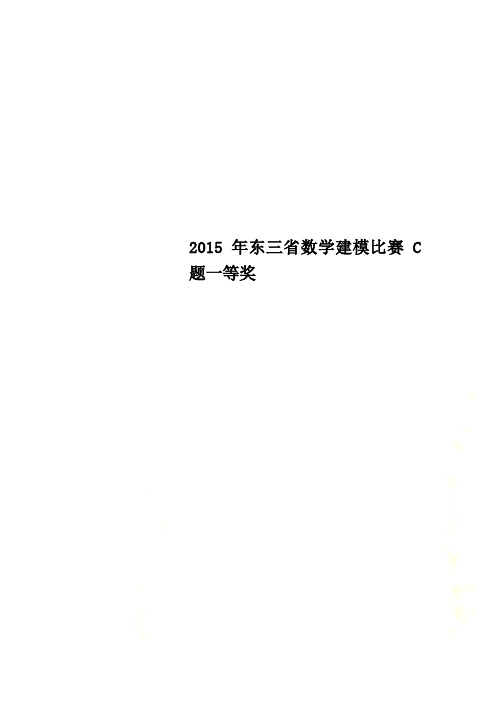
2015年东三省数学建模比赛C 题一等奖2015年东北三省数学建模一等奖论文福田红树林自然保护区湿地生态系统模型框架的构建及应用实例研究摘要目前福田红树林自然保护区湿地生态系统的健康状况日益脆弱,迫切需要建立一个完整的保护管理数字化支撑平台。
问题一,我们建立了集动态监测和健康评估及预警于一身的模型框架,该模型框架的建立基于BP-神经网络的PSR 模型,然后将EWE模型里的鱼类捕捞这一环节去掉,改为在自然条件下的水生动物数量变化,利用改进后EWE模型分析生态系统里的各级生物能量流动、物质循环等生态系统内部功能,并确立一个稳态指标。
利用各营养级之间的能量流动关系,对生态系统内的物种依存、竞争关系进行动态描述。
用层次分析法对影响生态系统的外界因素进行综合识别并建立三层权重指标体系得出相应权重指标,以单项指标的加权平均法来获取综合评价指数进行评价等级的确定,模型会自动反馈,并根据权重大小逐级查找问题来源,并根据问题来源找到合理的解决办法。
从而构建整个生态系统压力,状态,响应为一体化的监督管理系统。
问题二,基于以上模型框架,我们利用3s技术对该湿地生态系统相关数据进行监测分析评估预警,设计监测方案。
问题三,选取水污染作为迫切解决的问题,对目前相关数据代入建立好的模型框架进行分析,确定评价等级,对其未来发展趋势进行预测,给出了合理的建议。
关键词:PSR模型;改进EWE模型;层次分析法;3s技术;一、问题重述深圳福田红树林湿地自然保护区是我国唯一处于城市腹地的国家级自然保护区,保护区对调节当地环境具有极其重要的功能;但是在深圳经济高速发展的 30多年时间里,福田红树林湿地生态系统受城市扩张和环境污染影响较为严重,湿地生态系统的生态健康更加脆,为此,保护区的监测管理尤为重要。
目前的生态健康评价主要采用基于抽样监测数据和专家经验的静态方法,仅仅围绕主要生物因子开展调查而没有覆盖到噪声、大气等环境因子,而且监测点信息的时间、空间离散度较大(时间间隔较长、测点密度过于稀疏),致使难以完全满足福田红树林自然保护区科学管理的实际需要。
2015年全国大学生数学建模竞赛B题国一优秀论文
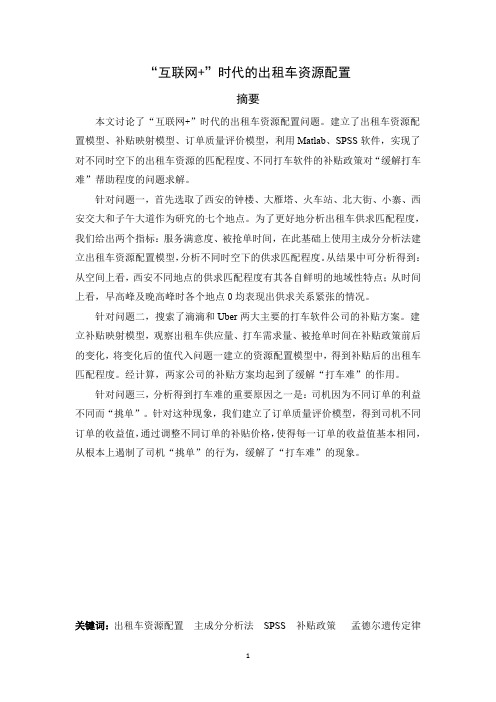
2.1 概论 目前城市“打车难”的社会问题导致越来越多的打车软件出现在市场上。以
此为背景,我们需要首先分析影响出租车资源的“供求匹配”程度的因素,进而 分析现已出台的补贴政策是否能够通过调整“供求匹配”程度进而缓解“打车难” 的现象,并在最后提出了我们自己关于补贴方案的想法。 2.2 问题一分析
0.70
0.53
0.66
0.68
0.40
0.86
0.71
0.71
0.84
0.82
0.88
0.91
0.66
0.68
0.84
0.79
6
2.被抢单时间 t 被抢单时间 t 表示客户使用打车软件下单后被司机接单的时间,可在一定程 度上反映打车难易程度。在滴滴快的打车智能出行平台上,基于需要研究的三个
时间段,采集西安的被抢单时间 t,制作表格如下:
火车站 121.23 142.45 219.44 161.04 210.23 231.67 278.93 240.28 198.67 245.92 221.38 221.99
北大街 67.23 107.52 98.23 90.99 72.92 82.98 187.23 114.38 63.95 145.23 98.25 102.48
小寨 62.19 78.31 103.20 81.23 136.25 178.27 162.73 159.08 83.82 103.27 121.93 103.01
西安交大 子午大道
47.21
43.98
82.34
64.53
102.34 65.92
77.30
58.14
121.94 67.74
167.42 93.03
优秀的数学建模论文范文(通用8篇)
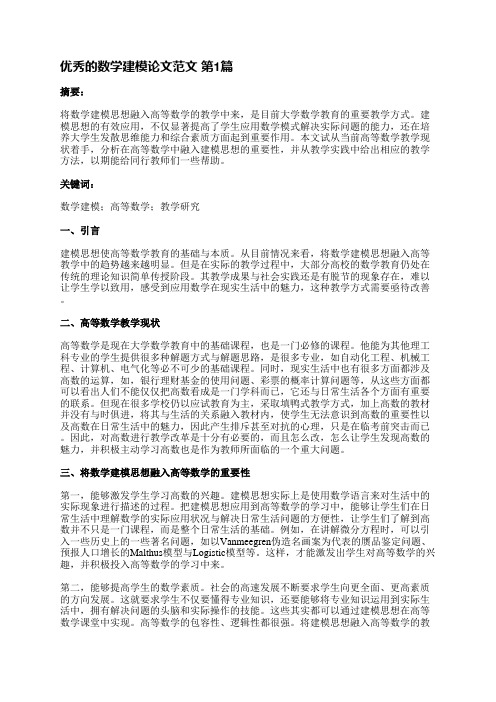
优秀的数学建模论文范文第1篇摘要:将数学建模思想融入高等数学的教学中来,是目前大学数学教育的重要教学方式。
建模思想的有效应用,不仅显著提高了学生应用数学模式解决实际问题的能力,还在培养大学生发散思维能力和综合素质方面起到重要作用。
本文试从当前高等数学教学现状着手,分析在高等数学中融入建模思想的重要性,并从教学实践中给出相应的教学方法,以期能给同行教师们一些帮助。
关键词:数学建模;高等数学;教学研究一、引言建模思想使高等数学教育的基础与本质。
从目前情况来看,将数学建模思想融入高等教学中的趋势越来越明显。
但是在实际的教学过程中,大部分高校的数学教育仍处在传统的理论知识简单传授阶段。
其教学成果与社会实践还是有脱节的现象存在,难以让学生学以致用,感受到应用数学在现实生活中的魅力,这种教学方式需要亟待改善。
二、高等数学教学现状高等数学是现在大学数学教育中的基础课程,也是一门必修的课程。
他能为其他理工科专业的学生提供很多种解题方式与解题思路,是很多专业,如自动化工程、机械工程、计算机、电气化等必不可少的基础课程。
同时,现实生活中也有很多方面都涉及高数的运算,如,银行理财基金的使用问题、彩票的概率计算问题等,从这些方面都可以看出人们不能仅仅把高数看成是一门学科而已,它还与日常生活各个方面有重要的联系。
但现在很多学校仍以应试教育为主,采取填鸭式教学方式,加上高数的教材并没有与时俱进,将其与生活的关系融入教材内,使学生无法意识到高数的重要性以及高数在日常生活中的魅力,因此产生排斥甚至对抗的心理,只是在临考前突击而已。
因此,对高数进行教学改革是十分有必要的,而且怎么改,怎么让学生发现高数的魅力,并积极主动学习高数也是作为教师所面临的一个重大问题。
三、将数学建模思想融入高等数学的重要性第一,能够激发学生学习高数的兴趣。
建模思想实际上是使用数学语言来对生活中的实际现象进行描述的过程。
把建模思想应用到高等数学的学习中,能够让学生们在日常生活中理解数学的实际应用状况与解决日常生活问题的方便性,让学生们了解到高数并不只是一门课程,而是整个日常生活的基础。
2015 高教社杯全国大学生数学建模竞赛 C 题论文

(7)
式中, 为太阳赤纬; 为按(3)式计算黄赤交角。上式可写为:
sin sin *sin s
(8 )
太阳相对于观测点的位置
本研究采用观测点的地心天顶距 来表示太阳在某时刻相对于某一点的位
置,设观测点的经纬度 、 ,则可由下式计算某时的地心天顶距:
模型二的建立与求解
已知确定月亮位置的太阳参数表示如下:
h 279.69668 36000.76892T 0.00030T 2
(12) (13)
2327'8.261'' 46.845'' T 0.0059'' T 2 0.00183'' T 3
根据布朗在 1919 年给出计算月亮位置的天文参数 s , p , N ,其中 s 为月 亮的平黄经,其角速度为每小时 0.5490165 , p 为月亮在近地点的平黄经,其 角速度为每小时 0.0046418 , N 为月亮升交点的平黄经,其角速度为每小时 0.0000020 。其计算公式如下:
2015 高教社杯全国大学生数学建摘 要
“月上柳梢头,人约黄昏后”里面所提到的约会时间,就是月出与黄昏后同 时出现的时间段。本文通过建立数学模型,并以北京为例,计算了北京的各参数 值,与现实数据作比较来验证模型,再判断什么条件下会出现“月上柳梢头,人 约黄昏后”这一现象,从而给出黄昏后的定义以及发生这一情景的条件。然后根 据条件,利用 Excel 表格对哈尔滨、上海、广州、昆明、成都、和乌鲁木齐这六 个城市的经纬度、日落时间、月出时间、以及日落月出时间差等进行了计算和统 计,通过分析比较,从而判断出各个城市地区能否出现“月上柳梢头,人约黄昏 后”这一现象。 关键词:地心天顶距,日落,月出,月亮高度,黄昏后
2015年全国大学生数学建模竞赛国家一等奖论文D题众筹筑屋规划方案设计模型
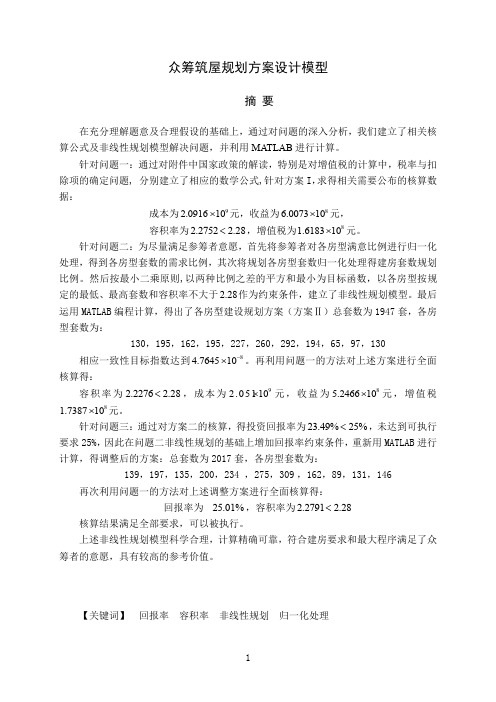
其中: 其他分摊到普通宅的比为普通宅的占地总面积与普通宅和非普通住宅的总面 积和的比值,得到普通宅分摊比可表达为:
LS P
N Ai Dij N Ai Dij N Ai Dij N
i 1 i i 4 i 3 i 1 8 i 11 11
3
A D11
Z ep Ni Ai Dij lS p Ni Ai Dij Z z p
i 1 i 9 3 10
非普通宅增值额 Z epf =非普通宅总售价 Z sjpf +其他宅分摊普通宅总售价 Z sjfpf -非普 通宅可扣除金额 Z zpf 即
Z epf Ni Ai Dij lS pf Ni Ai Dij N11 A11D11 Z zpf
1.7387 108 元。
针对问题三:通过对方案二的核算,得投资回报率为 23.49% 25% ,未达到可执行 要求 25%, 因此在问题二非线性规划的基础上增加回报率约束条件, 重新用 MATLAB 进行 计算,得调整后的方案:总套数为 2017 套,各房型套数为: 139,197,135,200,234 ,275,309 ,162,89,131,146 再次利用问题一的方法对上述调整方案进行全面核算得: 回报率为 25.01% ,容积率为 2.2791 2.28 核算结果满足全部要求,可以被执行。 上述非线性规划模型科学合理,计算精确可靠,符合建房要求和最大程序满足了众 筹者的意愿,具有较高的参考价值。
【关键词】 回报率
容积率 非线性规划
归一化处理
1
一、模型背景与问题的重述
1.1 模型的背景 众筹筑屋是互联网时代一种新型的房地产经营模式,由于其建筑设计阶段用大幅低 于市场价的优惠吸引用户参与众筹。 用户通过众筹筑屋平台对建筑方案提出自己的意见 并参与优化设计。因此,正确、及时的核算建房实际成本与收益、容积率和增值税等信 息尤为重要。从而不仅为众筹者提供满意的住房条件,而且还能为开发商提供科学的决 策依据一。 1.2 问题重述 在建房规划设计中,需考虑诸多因素,如容积率、开发成本、税率、预期收益等。 根据国家相关政策,不同房型的容积率、开发成本、开发费用等在核算上要求均不同, 结合国家相关条例政策和本题具体要求,建立数学模型分析研究解决下面的问题: 问题一 根据方案 I 相关数据计算成本与收益、容积率和增值税等信息。然后对其 建立模型对方案 I 进行全面的核算,帮助其公布相关信息。 问题二 通过对参筹者进行抽样调查,得到了参筹者对 11 种房型购买意愿的比例。 为了尽量满足参筹者的购买意愿,请你重新设计建设规划方案(称为方案Ⅱ),并对方 案 II 进行核算。 问题三 一般对于开发商而言, 只有投资回报率达到 25%以上的众筹项目才会被成功 执行。根据问题二所给出的众筹筑屋方案Ⅱ能否被成功执行,需要通过建立相应模型进 行具体分析说明。
2015数学建模竞赛优秀论文

关键词: 最小二乘拟合 搜索算法 数据拟合 SketchUp 软件 Matlab 编程
1
一、 问题重述
如何确定视频的拍摄地点和拍摄日期是视频数据分析的重要方面,太阳影子定位技 术就是通过分析视频中物体的太阳影子变化,确定视频拍摄的地点和日期的一种方法。 1.建立影子长度变化的数学模型,分析影子长度关于各个参数的变化规律,并应用 你们建立的模型画出 2015 年 10 月 22 日北京时间 9:00-15:00 之间天安门广场 (北纬 39 度 54 分 26 秒,东经 116 度 23 分 29 秒)3 米高的直杆的太阳影子长度的变化曲线。 2.根据某固定直杆在水平地面上的太阳影子顶点坐标数据,建立数学模型确定直杆 所处的地点。 将你们的模型应用于附件 1 的影子顶点坐标数据, 给出若干个可能的地点。 3. 根据某固定直杆在水平地面上的太阳影子顶点坐标数据,建立数学模型确定直 杆所处的地点和日期。将你们的模型分别应用于附件 2 和附件 3 的影子顶点坐标数据, 给出若干个可能的地点与日期。 4.附件 4 为一根直杆在太阳下的影子变化的视频,并且已通过某种方式估计出直 杆的高度为 2 米。请建立确定视频拍摄地点的数学模型,并应用你们的模型给出若干个 可能的拍摄地点。 如果拍摄日期未知,你能否根据视频确定出拍摄地点与日期?
关于太阳影子定位问题的研究
摘要
本文将从建立影子长度变化模型、根据影子顶点坐标数据建立确定直杆所处地点的 模型、直杆所处的地点和日期的模型以及根据影子的变化视频建立确定视频拍摄地点的 模型来研究太阳影子定位问题。 针对问题一,建立影子长度的数学模型,经查阅相关资料【1】可知,影长与太阳高度 角及物体垂直高度有关,而太阳高度角与时角、赤纬角、纬度等有关。先确定太阳高度 角的表达式,再得到影长变化模型。由影子长度变化模型可知:影长与物体垂直高度成 正比,与太阳高度角的正切成反比。根据模型编写 Matlab 程序求解得到所求直杆的影 长变化曲线(见文中图一),其影长最小值为 2.5213 米,北京时间为 11:46;最大值为 5.0980 米,北京时间为 8:56。由于该模型采用的赤纬角的计算公式未考虑年份的影响, 所以本文进一步改进了该模型。 经查阅资料【2】得到更精确的计算赤纬角的公式, 对改进 后的模型求解,由得到的直杆影子变化曲线(见文中图二)知:影长随时间的变化趋势 不变,最小值为 3.2282 米,北京时间为 11:46;最大值为 6.4280 米,北京时间为 8:56。 针对问题二,根据固定直杆影子顶点坐标,建立直杆地点数学模型。先根据坐标数 据求出对应的影长, 再根据模型一中的函数逆推得到影长与经纬度的函数。 通过 Matlab 的搜索算法不断拟合不同经纬度的影长变化曲线,将其与坐标系中影长变化曲线进行误 差分析,选取误差最小的经纬度采用谷歌地图查询地点,得到直杆可能的所在地。将附 件 1 的数据进行上述处理,得到直杆所处的地点最可能为海南三亚(109.5250°E, 18.2795°N),其它可能地点为海南海口(110.3207°E,20.0496°N)和广东玉林 (110.7015°E,22.7865°N)。 针对问题三,根据固定直杆的影子顶点坐标数据,建立确定直杆所处地点和日期的 模型。在问题二所建模型的基础上视时间为变量,将附件 2 的数据代入 Matlab 的搜索 算法求得直杆所在地点的经纬度最可能在新疆 (75.8361°E, 39.4293°N) , 时间为 2015 年 1 月 1 日。 附件 3 中直杆所处地点经纬度最可能在山西 (34.2616°E,109.7023°N) , 时间为 2015 年 1 月 20 日。 针对问题四,根据所给视频,建立确定拍摄地点的数学模型。本文通过 KMPlayer 软件截取视频中连续变化每间隔一分钟的图片,再根据 SketchUp 软件对截取的 41 张图 片建立坐标并求得杆长与影长之比,根据杆长可求得影长,将拟合出的影长作为已知条 件,根据问题二所建模型的方法可以求出拍摄地点最可能在黑龙江佳木斯市 (134.8724°E,48.4232°N)。若拍摄时间未知,可根据问题三所建模型的方法求解, 得拍摄地点为黑龙江佳木斯(134.7823°E,47.4232°N),时间为 2015 年 7 月 12 日。
太阳影子定位-2015年全国数学建模比赛a题全国二等奖论文
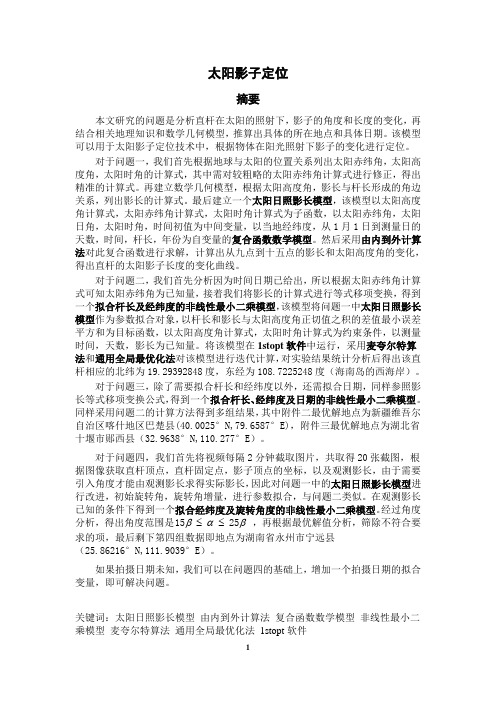
太阳影子定位摘要本文研究的问题是分析直杆在太阳的照射下,影子的角度和长度的变化,再结合相关地理知识和数学几何模型,推算出具体的所在地点和具体日期。
该模型可以用于太阳影子定位技术中,根据物体在阳光照射下影子的变化进行定位。
对于问题一,我们首先根据地球与太阳的位置关系列出太阳赤纬角,太阳高度角,太阳时角的计算式,其中需对较粗略的太阳赤纬角计算式进行修正,得出精准的计算式。
再建立数学几何模型,根据太阳高度角,影长与杆长形成的角边关系,列出影长的计算式。
最后建立一个太阳日照影长模型,该模型以太阳高度角计算式,太阳赤纬角计算式,太阳时角计算式为子函数,以太阳赤纬角,太阳日角,太阳时角,时间初值为中间变量,以当地经纬度,从1月1日到测量日的天数,时间,杆长,年份为自变量的复合函数数学模型。
然后采用由内到外计算法对此复合函数进行求解,计算出从九点到十五点的影长和太阳高度角的变化,得出直杆的太阳影子长度的变化曲线。
对于问题二,我们首先分析因为时间日期已给出,所以根据太阳赤纬角计算式可知太阳赤纬角为已知量,接着我们将影长的计算式进行等式移项变换,得到一个拟合杆长及经纬度的非线性最小二乘模型,该模型将问题一中太阳日照影长模型作为参数拟合对象,以杆长和影长与太阳高度角正切值之积的差值最小误差平方和为目标函数,以太阳高度角计算式,太阳时角计算式为约束条件,以测量时间,天数,影长为已知量。
将该模型在1stopt 软件中运行,采用麦夸尔特算法和通用全局最优化法对该模型进行迭代计算,对实验结果统计分析后得出该直杆相应的北纬为19.29392848度,东经为108.7225248度(海南岛的西海岸)。
对于问题三,除了需要拟合杆长和经纬度以外,还需拟合日期,同样参照影长等式移项变换公式,得到一个拟合杆长、经纬度及日期的非线性最小二乘模型。
同样采用问题二的计算方法得到多组结果,其中附件二最优解地点为新疆维吾尔自治区喀什地区巴楚县(40.0025°N,79.6587°E),附件三最优解地点为湖北省十堰市郧西县(32.9638°N,110.277°E )。
数模优秀论文

数模优秀论文篇一:2015年全国大学生数学建模比赛A题一等奖论文太阳影子定位问题摘要目前,如何确定视频的拍摄地点和拍摄日期是计算机视觉的热点研究问题,是视频数据分析的重要方面,有重要的研究意义。
本文通过建立数学模型,给出了通过分析视频中物体的太阳影子变化,确定视频拍摄的地点和日期的方法。
对于问题一,建立空间三维直角坐标系和球面坐标系对直杆投影和地球进行数学抽象,引入地方时、北京时间、太阳赤纬、杆长、太阳高度角等五个参数,建立了太阳光下物体影子的长度变化综合模型。
求解过程中,利用问题所给的数据,得到太阳赤纬等变量,将太阳赤纬等参量代入模型,求得了北京地区的9:00至15:00的影子长度变化曲线,当12:09时,影子长度最短;并分析出影长随这些参数的变化规律,利用控制变量法思想,总结了五个参数与影子长度的关系。
最后进行模型检验,将该模型运用于东京、西藏两地,得到了这两座城市的影长变化规律曲线,发现变化规律符合实际两地实际情况。
对于问题二,为了消除不同直角坐标系带来的影响,将实际坐标转换为二次曲线的极坐标,建立了极坐标下基于多层优化搜索算法的空间匹配优化模型。
求解时,先将未知点的直角坐标系的点转换为极坐标,然后了多层优化搜索算法,通过多次不同精度的搜索,最后得出实际观测点的经纬度为东经E115?北纬N25?。
同时对模型进行验证,实地测量了现居住地的某个时间段的值,通过模型二来求解出现居住地的经纬度,分析了误差产生的原因:大气层的折射和拟合误差。
对于问题三,将极坐标转换后的基本模型转换为优化模型,建立了基于遗传算法的时空匹配优化模型。
将目标函数作为个体的适应度函数,将经度纬度及日期作为待求解变量,用遗传算法进行求解,得到可能的经度纬度及其日期:北纬20度,东经114度,5月21日;北纬20度,东经114度,7月24日;东经94.5度,北纬33.8度,6月19日。
最后,将遗传算法与多层优化搜索算法进行对比分析,得出遗传算法的求解效率和求解精度均优于多层次搜索算法。
2015年数学建模全国一等奖论文
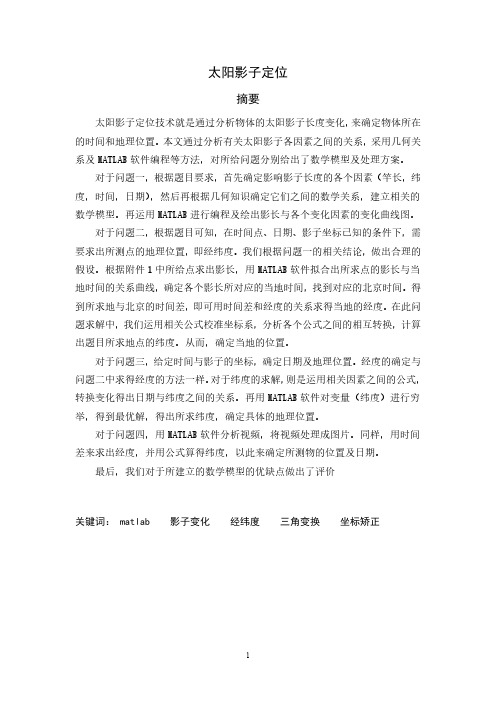
t (ts t 0) (tt 12)
其中 ts 为时间,t 为时差,t0 为最低点时间,t 北为对应的北京时间。 计算出时差 t 。 (3) 经度的求解 已知两地经度相差 1 度,时间相差 4 分钟,所以可列出:
(11)
北
ts
4
(12)
其中 γ 为当地经度,γ 北为北京经度,ts 为时差。 通过公式(12)解得经度 γ (4)纬度的求解 太阳方位角就是太阳在方位上的角度,它和坐标有以下关系:
7
7
6.5
6
影长 L
5.5
5
4.5
4
3.5 9
10
11
12 时间 t
13
14
15
图5
北京 10 月 22 日影长变化
5.2
问题二:
5.2.1 模型的准备
模型建立之前,我们分析数据得到所给影子顶点坐标并非以标准的东西南北 方向坐标系下的坐标, 所以我们必须进行矫正,把坐标系修正成正南正北的坐标 系。而后确定时差来确定经度,进而得到纬度。
(8)
(9)
获得新的坐标(x1,y1) 。 注:矫正坐标系以东西方向为 x 轴,南北方向为 y 轴。
图6
8
(2) 时差的求解 通过所给坐标在 matlab 中进行拟合,得到一条影长 L 关于时间 ts 的抛物线 方程: L=ats2-bts+c 其中 L 为影长,t 为时间。 解出最低点坐标 t0,利用北京时间 12:00 时影子最短,利用比例关系 (10)
5.1.3 模型的求解
模型中及为影长 L 和时间 t,纬度φ,以及日期 n 的函数关系。当其中两个 自变量确定后,就可建立影长 L 和另外一个自变量的模型。 (1) 影长 L 和时间 t 的模型 给定日期 n 和纬度φ, 模型就变成了影长 L 和时间 t 的一元函数, 应用 Matlab 即可得到影长的变化曲线。 在此,我们验证了赤道上 1 月 1 日的影长变化(如图 2) 由图可以看出,当 1 月 1 日时,9:00 到 15:00 的曲线为开口向上的抛物线, 在早上 9:00 时,由于太阳直射南半球,所以影子长,到了当地正午 12:00 时影子 最短,下午又开始增长,符合实际,模型基本成立。
2015年全国大学生数学建模大赛国家二等奖论文
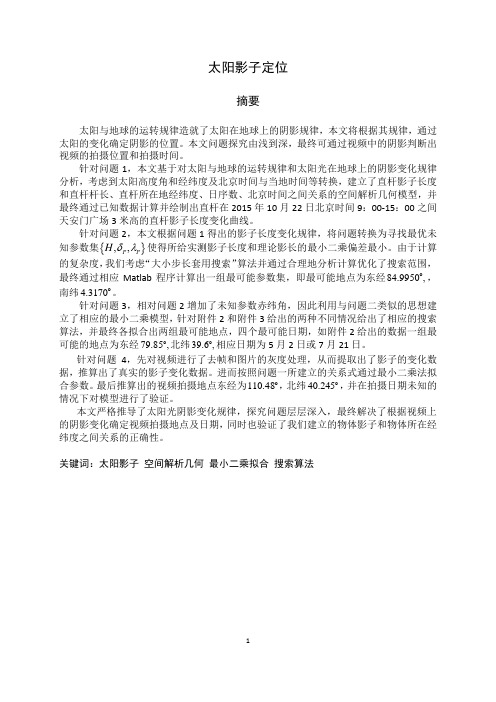
太阳影子定位摘要太阳与地球的运转规律造就了太阳在地球上的阴影规律,本文将根据其规律,通过太阳的变化确定阴影的位置。
本文问题探究由浅到深,最终可通过视频中的阴影判断出视频的拍摄位置和拍摄时间。
针对问题1,本文基于对太阳与地球的运转规律和太阳光在地球上的阴影变化规律分析,考虑到太阳高度角和经纬度及北京时间与当地时间等转换,建立了直杆影子长度和直杆杆长、直杆所在地经纬度、日序数、北京时间之间关系的空间解析几何模型,并最终通过已知数据计算并绘制出直杆在2015年10月22日北京时间9:00-15:00之间天安门广场3米高的直杆影子长度变化曲线。
针对问题2,本文根据问题1得出的影子长度变化规律,将问题转换为寻找最优未知参数集{},,P P H δλ使得所给实测影子长度和理论影长的最小二乘偏差最小。
由于计算的复杂度,我们考虑“大小步长套用搜索”算法并通过合理地分析计算优化了搜索范围,最终通过相应Matlab 程序计算出一组最可能参数集,即最可能地点为东经84.9950, ,南纬4.3170 。
针对问题3,相对问题2增加了未知参数赤纬角,因此利用与问题二类似的思想建立了相应的最小二乘模型,针对附件2和附件3给出的两种不同情况给出了相应的搜索算法,并最终各拟合出两组最可能地点,四个最可能日期,如附件2给出的数据一组最可能的地点为东经79.85, 北纬39.6, 相应日期为5月2日或7月21日。
针对问题4,先对视频进行了去帧和图片的灰度处理,从而提取出了影子的变化数据,推算出了真实的影子变化数据。
进而按照问题一所建立的关系式通过最小二乘法拟合参数。
最后推算出的视频拍摄地点东经为110.48 ,北纬40.245 ,并在拍摄日期未知的情况下对模型进行了验证。
本文严格推导了太阳光阴影变化规律,探究问题层层深入,最终解决了根据视频上的阴影变化确定视频拍摄地点及日期,同时也验证了我们建立的物体影子和物体所在经纬度之间关系的正确性。
2015年江西省研究生数学建模竞赛优秀论文
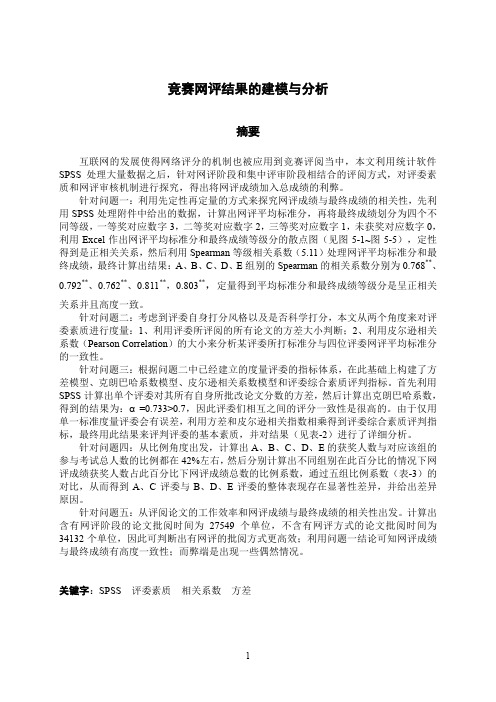
摘要
互联网的发展使得网络评分的机制也被应用到竞赛评阅当中,本文利用统计软件 SPSS 处理大量数据之后,针对网评阶段和集中评审阶段相结合的评阅方式,对评委素 质和网评审核机制进行探究,得出将网评成绩加入总成绩的利弊。 针对问题一:利用先定性再定量的方式来探究网评成绩与最终成绩的相关性,先利 用 SPSS 处理附件中给出的数据,计算出网评平均标准分,再将最终成绩划分为四个不 同等级,一等奖对应数字 3,二等奖对应数字 2,三等奖对应数字 1,未获奖对应数字 0, 利用 Excel 作出网评平均标准分和最终成绩等级分的散点图(见图 5-1~图 5-5),定性 得到是正相关关系,然后利用 Spearman 等级相关系数(5.11)处理网评平均标准分和最 终成绩,最终计算出结果:A、B、C、D、E 组别的 Spearman 的相关系数分别为 0.768**、 0.792**、0.762**、0.811**,0.803**,定量得到平均标准分和最终成绩等级分是呈正相关 关系并且高度一致。 针对问题二:考虑到评委自身打分风格以及是否科学打分,本文从两个角度来对评 委素质进行度量:1、利用评委所评阅的所有论文的方差大小判断;2、利用皮尔逊相关 系数(Pearson Correlation)的大小来分析某评委所打标准分与四位评委网评平均标准分 的一致性。 针对问题三:根据问题二中已经建立的度量评委的指标体系,在此基础上构建了方 差模型、克朗巴哈系数模型、皮尔逊相关系数模型和评委综合素质评判指标。首先利用 SPSS 计算出单个评委对其所有自身所批改论文分数的方差,然后计算出克朗巴哈系数, 得到的结果为:α =0.733>0.7,因此评委们相互之间的评分一致性是很高的。由于仅用 单一标准度量评委会有误差, 利用方差和皮尔逊相关指数相乘得到评委综合素质评判指 标,最终用此结果来评判评委的基本素质,并对结果(见表-2)进行了详细分析。 针对问题四:从比例角度出发,计算出 A、B、C、D、E 的获奖人数与对应该组的 参与考试总人数的比例都在 42%左右, 然后分别计算出不同组别在此百分比的情况下网 评成绩获奖人数占此百分比下网评成绩总数的比例系数,通过五组比例系数(表-3)的 对比,从而得到 A、C 评委与 B、D、E 评委的整体表现存在显著性差异,并给出差异 原因。 针对问题五:从评阅论文的工作效率和网评成绩与最终成绩的相关性出发。计算出 含有网评阶段的论文批阅时间为 27549 个单位,不含有网评方式的论文批阅时间为 34132 个单位,因此可判断出有网评的批阅方式更高效;利用问题一结论可知网评成绩 与最终成绩有高度一致性;而弊端是出现一些偶然情况。
2015数学建模优秀论文
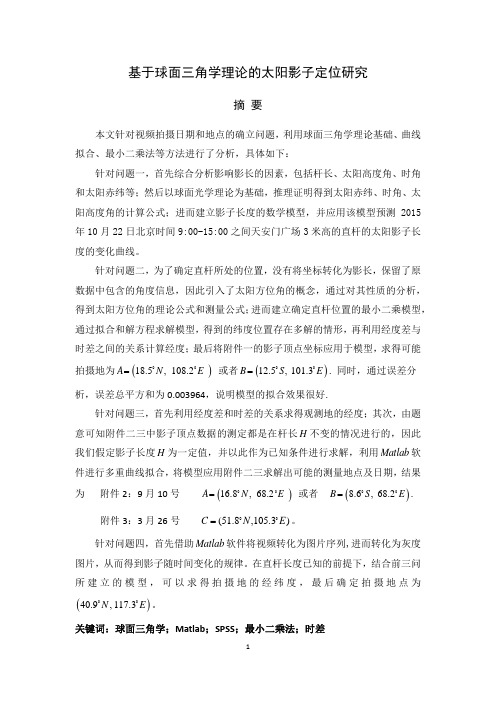
cos(90 ) cos(90 ) cos(90 ) sin(90 ) sin(90 ) sin(90 t ) ,
即
sin sin sin cos cos cos t .
(5.3)
于是得到了太阳高度角 的表达式
arcsin sin sin cos cos cos t
6
(5.4)
其中 t 表示太阳时角,由于自转时间为 24 小时,因此每小时旋转 15 度,从 而
t (tt 12) 15 .
(3)影子长度的数学模型
Hale Waihona Puke (5.5)接下来,继续考虑影子长度的计算方法. 注意到直杆是垂直于地面的,因此 直杆、影子以及太阳光束构成直角三角形,其中影子和光束之间的夹角恰好是太 阳的高度角 ,于是得到影子长度的数学模型
L H tan H tan arcsin sin sin cos cos cos(tt 12) 15 (5.6)
模型(5.6)反映了影子长度关于各参数的变化规律.例如,固定 , 值,由余弦 函数的偶函数特征,影子长度 L 关于 12 点对称,并且在 12 点达到最小值;若固 定 , tt ,则当 从 0 度向 90 度变化时,注意到 sin 的增加率大于 cos 减少率, 因此影子长度逐渐增加,也就是说,地理位置越偏向北方,影子长度越大. 由于各地都以北京时间为准,存在地方时差,因此为了更好地描述不同地点 影子长度的变化规律,我们将模型(5.6)进一步优化,得到如下模型:
tt M F
太阳时角 观测地经度 具体时刻 观测点经度的度值 观测时刻的分值
N
2015数模美赛S奖论文
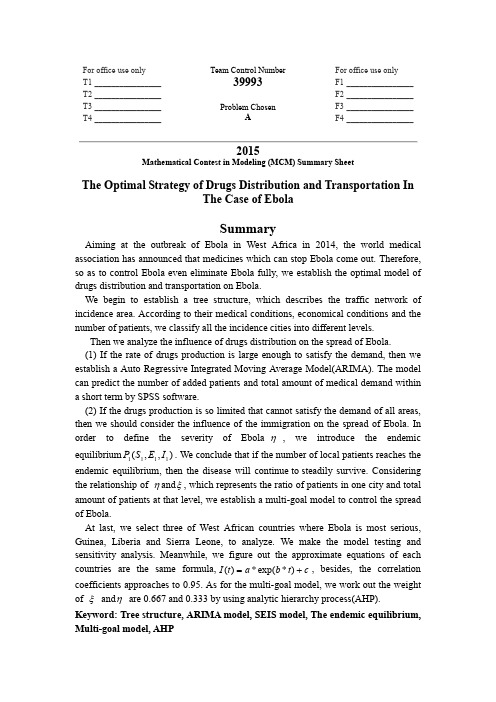
For office use onlyT1 ________________T2 ________________T3 ________________T4 ________________ Team Control Number 39993 Problem Chosen A For office use only F1 ________________ F2 ________________ F3 ________________ F4 ________________ 2015Mathematical Contest in Modeling (MCM) Summary SheetThe Optimal Strategy of Drugs Distribution and Transportation In The Case of EbolaSummaryAiming at the outbreak of Ebola in West Africa in 2014, the world medical association has announced that medicines which can stop Ebola come out. Therefore, so as to control Ebola even eliminate Ebola fully, we establish the optimal model of drugs distribution and transportation on Ebola.We begin to establish a tree structure, which describes the traffic network of incidence area. According to their medical conditions, economical conditions and the number of patients, we classify all the incidence cities into different levels.Then we analyze the influence of drugs distribution on the spread of Ebola.(1) If the rate of drugs production is large enough to satisfy the demand, then we establish a Auto Regressive Integrated Moving Average Model(ARIMA). The model can predict the number of added patients and total amount of medical demand within a short term by SPSS software.(2) If the drugs production is so limited that cannot satisfy the demand of all areas, then we should consider the influence of the immigration on the spread of Ebola. In order to define the severity of Ebola η, we introduce the endemic equilibrium ),,(1111I E S P . We conclude that if the number of local patients reaches the endemic equilibrium, then the disease will continue to steadily survive. Considering the relationship of ηand ξ, which represents the ratio of patients in one city and total amount of patients at that level, we establish a multi-goal model to control the spread of Ebola.At last, we select three of West African countries where Ebola is most serious, Guinea, Liberia and Sierra Leone, to analyze. We make the model testing and sensitivity analysis. Meanwhile, we figure out the approximate equations of each countries are the same formula,c t b a t I +=)*exp(*)(, besides, the correlation coefficients approaches to 0.95. As for the multi-goal model, we work out the weight of ξ and η are 0.667 and 0.333 by using analytic hierarchy process(AHP).Keyword: Tree structure, ARIMA model, SEIS model, The endemic equilibrium, Multi-goal model, AHPContents1. Introduction (1)1.1 Background (1)1.2 Question Retelling (1)2. Model Assumptions (2)2.1 Assumptions of Tree Structure (2)2.2 Assumptions of ARIMA Model (2)2.3 Assumptions of SEIS Model (2)2.4 Assumptions of Multi-goal Model (2)3. Explanation (3)3.1 Explanation of Symbols (3)3.2 Extra Explanation (4)4. The Models (4)4.1The Tree Structure Chart (5)4.2 The Time Series Analysis (7)4.2.1 Auto Regressive Integrated Moving Average Model (7)4.2.2 The Judgment of Models (9)4.3 Establishing SEIS Models (9)4.3.1 The Feature of Ebola (9)4.3.2 The SEIS Model (10)4.3.3 Analyzing The Severity of Ebola (11)4.4 The Multi-goal Programming Model (12)4.4.1 The Analytic Hierarchy Process (AHP) (13)4.4.2 Testing Consistency (14)4.4.3 The Multi-goal Programming Model Equation (15)5. Conclusion And Sensitivity Analysis (17)5.1 The Predicted Result of ARIMA(p,d,q) Model. (17)5.2 The Error Analysis of ARIMA (p,d,q) Model Above (19)5.3 The Stability Analysis of Tree Structure (20)7. Strengths And Weaknesses (21)8. A Non-technical Letter (22)9. References (23)1. Introduction1.1 BackgroundEbola, previously known as Ebola hemorrhagic fever, is a rare and deadly disease caused by infection with one of the Ebola virus strains. Ebola can cause disease in humans and nonhuman primates (monkeys, gorillas, and chimpanzees).Ebola is caused by infection with a virus of the family Filoviridae, genus Ebolavirus. There are five identified Ebola virus species, four of which are known to cause disease in humans: Ebola virus, Sudan virus, Taï Forest virus and Bundibugyo virus. The fifth, Reston virus, has caused disease in nonhuman primates, but not in humans.Ebola viruses are found in several African countries. Ebola was first discovered in 1976 near the Ebola River in what is now the Democratic Republic of the Congo. Since then, outbreaks have appeared sporadically in Africa.1.2 Question RetellingIn recent years, Ebola has attracted many peoples’ eyes. Furthermore, the world medical association has announced that their new medication could stop Ebola and cure patients whose disease is not advanced. Thus, it is necessary that we take measure to optimize the eradication of Ebola.Since we need consider the spread of the disease, the quantity of the medicine needed, possible feasible delivery systems, locations of delivery, and speed of manufacturing of the vaccine or drug and so on, our task is to design an appropriate mathematical model to work out these problems.After that, we will prepare a non-technical letter for the world medical association to use in their announcement.2.Model Assumptions2.1 Assumptions of Tree Structure(1)The capitals are large enough to accept a large number of drugs transportation.(2)The adjacent level in the tree structure can transport medications by railway.(3)The distance between the two adjacent levels cities are the same.2.2 Assumptions of ARIMA Model(1)The short term external factors such as temperature and climate are constant.(2)There is no infective immigrating.(3)The Ebola virus did not mutate, and the infection rate or mortality did not change.2.3 Assumptions of SEIS Model(1)The natural birth rate and the natural death rate keep unchanged, and the totalpopulation is unchanged.(2)Immigration rate u, has nothing to do with the city variation and keeps constant.(3)The patients are always moving along the tree structure from bottom to top.(4)The immigrated people caused by the lack of medicines are only patients.2.4 Assumptions of Multi-goal Model(1)Drugs distribution is only related to the severity of Ebola ηand the ratio ofpatients in one city and total amount of patients in that level ξ.(2)ξis prior to η.3. Explanation3.1 Explanation of Symbols●{})(t I:A non-stationary time series related to Guinea total cases.●t I: The time series’ present value.●P: The auto regressive orders.●q: The moving average orders.●{}tε: A zero-mean white noise series.●μ: The constant term.●ω: The coefficient of the white noise series.●φ: The autoregressive coefficient.●S(t): The number of the susceptible at the certain time t.●E(t): The number of the exposed at a certain time t.●I(t): The number of the exposed at a certain time t.●N: The total number of a population.●β: Mortality due to illness, namely, the radio of deaths due to illness in a unittime and the total infective suffering from Ebola.●λ: Effective contact rate, namely, the ratio of the susceptible who become theexposed through contacting the infective and the total susceptible.●θ: The radio of the exposed that become the infective and the total exposed.●v: Drug production speed, namely, the number of the produced drugs in aunit time.●m: The average number of drugs needed to cure a patient.●γ: Recovery rate, namely, the radio of the infective who become thesusceptible through drug treatment and the total infective.●u: The immigration rate.●R0: T he basic reproduction number.●P1: The endemic equilibrium.●k: The number of total children cites of their parent city.●i b: The number of the total infective in the i-th children city.●i a: The number of the infective that drugs in the i-th children city can meet.●α: The number of the infective who immigrate from children cities to theirparent city, we can easily find ∑=-⋅=kii i a bu1) (α.●η: The severity of Ebola.●ξ: T he ratio of the number of the infective in the city and the number of thetotal infective in the same level city.●A: The comparison matrix.●M: The weight vector of the matrix A.●maxλ: The maximum eigenvalue.●CI: The consistency index.●RI: The random consistency index.●CR: The consistency ratio index.●d: The difference frequency.3.2 Extra Explanation• Susceptible The susceptible are those who are potentially infected individuals and have no immunity to this disease.• Exposed The exposed are those who are infected and become infectious but do not show symptoms.• Infective The infective are those who show the corresponding symptoms and are infectious.• Recovered The recovered are those who recovered from the disease.4.The ModelsOur goal is devising a most reasonable mode of drug transport to control Ebola. Referring to the data, we begin to select three of West African countries where Ebolais most serious to analyze. Moreover, we classify all the infected cities in the three countries into four levels, according to their medical conditions, economical conditions and the number of the infective. We establish a tree structure chart. (see 4.1 The Tree Structure Chart)If the drug production speed is large enough to satisfy the demand, then we will predict the possible number of new patients within a short time and calculate the needed drugs. Then we can distribute the drugs in advance. Doing these, we can stop Ebola within a short time. In order to calculate the possible number of new patients within a short time, we establish a time-series analysis model and using Auto Regressive Integrated Moving Average Model (ARIMA for short) and SPASS to simulate the number.(see 4.2 The Time Series Analysis)If the drug production is limited, the speed cannot satisfy the current demand, and then we establish a multi-goal programming model to work out the optimal allocation. We select two factors, the portion of the number of the patients in the city ξand the severity of Ebola η, and calculate their respective weight. When calculating the value of η, we establish a SEIS model. We work out the endemic equilibrium and get the number of patients I1 at the endemic equilibrium. Through comparing the ratio of the number of existing patients I(t)and I1, I(t)/I1, we definite the value of η. The larger I(t)/I1 is, the largerηis, namely, the more serious Ebola is.(see 4.3 Establishing SEIS Models) Last, we use the multi-goal formulas to calculate the number of needed drugs in each level cities.(see 4.4 The Multi-goal Programming Model)4.1The Tree Structure ChartAccording to the statistics of Ebola incidence (see fig.4-1-1), we select three of West African countries where Ebola is most serious to analyze. They are Guinea, Liberia and Sierra Leone. We collect all the infected cities in the three countries into four levels, according to their medical conditions, economical conditions and the number of the infective. The capitals are the first-level cities; the cities slightly smaller than their respective capital are the second-level cities. And like that, the cities slightly smaller than their respective second-level cities are the third-level cities and the cities slightly smaller than their respective third-level cities are the forth-level cities.FIGURE 4-1-1. The distribution of the infected cities in Guinea, Liberia and Sierra LeoneFIGURE 4-1-2. A tree structure chart of four level cities in GuineaIn order to count the ratio of the distributed drugs more conveniently, we construct a tree structure chart (see fig.4-1-2). In order to achieve the goal of transporting the drugs most fast, we distribute the drugs to the first-level cities, then the first-levelcities distribute the drugs to their second-level cities, then the second-level citiesdistribute the drugs to their third-level cities, and last the third-level cities distribute the drugs to their forth-level cities. Notably, across-level distribution is not allowed. The mode of drugs transportation of the first-level cities is air transportation, the others are railway transportation.The number of distributed drugs of each city is proportional to the ratio of the number of the infective in the city and the number of the total infective in the same level city. Notably, the distributed drugs in each city equal to the sum of the native needed drugs in the city and the total needed drugs in its children cities.4.2 The Time Series AnalysisFIGURE 4-2-1. A line chart of total cases and death cases weekly in Ebola main outbreak area from November, 2014 to February, 2015Combined with the above development relationship between Ebola cases and time (see fig.4-2-1), we establish a time-series analysis model. Studying the model, we can forecast the future Ebola cases at a certain time t. We can analyze how to carry out the optimal strategy to transport the drugs so as to control Ebola.4.2.1 Auto Regressive Integrated Moving Average ModelReferring to the statistics (see table 4-2-1), we build up a time series {})(I t related to Guinea total cases. Time series is classified into the stationary time series and the non-stationary time series. The mean and the variance is constant in stationary timeseries, but having an obvious trend or periodicity in non-stationary time series.[1] Since Guinea’s Ebola total cases show a rising trend, we can conclude that our time series {})(I t is a non-stationary time series. Date Guinea total case (total deaths) Liberia total case(total deaths)Sierra Leone total case (total deaths) 2014.11.11 1919(1166)6878(2812) 5586(1187) 2014.11.21 2047(1214)7082(2963) 6190(1267) 2014.11.30 2164(1327)7635(3145) 7312(1583) 2014.12.7 2292(1428)7719(3177) 7897(1768) 2014.12.20 2571(1586)7830(3376) 8939(2256) 2014.12.28 2767(1790)8018(3423) 9446(2758) 2014.1.10 2799(1807)8278(3515) 10094(3049) 2014.1.20 2873(1880)8524(3636) 10400(3159) 2015.2.1 2975(1944)8668(3710) 10707(3274) Total22407(14142) 70632(29757) 76571(20301) TABLE 4-2-1. Total cases and death cases statistics weekly in West African Ebola main outbreak area from November, 2014 to February, 2015Auto Regressive Integrated Moving Average Model (ARIMA for short) is an important prediction tool suitable for non-stationary time series.[2] So we collect ARIMA(p,d,q) to predict and modify our non-stationary time series {})(I t . The time series’ present value is denoted as t I .Not only does t I relate to the time series’ past value, but a certain dependency also exists between t I and the disturbance which the time series generated in the past time when entering the system. So we use the linear combination of the series’ past value and the linear combination of white noise perturbation term to express t I . t I is as follows:q t q t t p t p t t I I -----⋅⋅⋅--++⋅⋅⋅++=εωεωεφφμ1111I ⑴Where the auto regressive orders is denoted as p , the moving average orders isdenoted as q , the zero-mean white noise series is denoted as {}t ε, the constant term is denoted as μ, the autoregressive coefficient is denoted as φ, the coefficient of the white noise series is denoted as ω.Because {}n ,321,⋅⋅⋅=,,,t I t of d-th order difference is stationary and t I meets the eq.2, the time series’ present value t I belongs to ARIMA . A fter centralizing t I , the operator form is as follows:⎪⎩⎪⎨⎧<∀=E ≠===E =∇t s I t s E Var B I B t s s t E t t tt d ,0(,0)(,)(,0)()()()2εεεσεεεωϕ ⑵ Where 1≤B , )(B ϕ and )(B θ are relatively prime, 0≠q p θϕ.4.2.2 The Judgment of ModelsFirst, we introduce AIC(An Information Criterion). The definition of AIC principle is as follows: The minimum information principle. It is applicable to the problem whose sample data is pretty little. We usually use AIC to judge the development process of forecasting target is closest to which model.)2(2log )(2+++-=q p d n AIC σWhere the sample number is denoted as n , the fitting residual square is denoted as σ.We collect the model whose parameters are least and AIC is minimum as theoptimal model. We collect the model’s order which can make AIC minimum as the optimal order. The fitting model is closer to theoretical distribution when the value of AIC is smaller.4.3 Establishing SEIS Models 4.3.1 The Feature of EbolaIn natural state, the number of a population is constant, natural birth rate and natural death rate keep a balance. Ebola is characterized by quick onset. Its incubation period is usually 2~21 days. Besides, the infective will die in 3~5 days since becoming ill and they do not have immunity after cured. So when Ebola outbreaks, it will have no impact on the natural birth rate and natural death rate of the population ina short time. So we neglect the natural birth rate and the natural death rate, we only consider mortality due to illness. Moreover, Ebola is basically transmitted through body fluids, so we conclude that the transmission mode of Ebola is horizontal transmission. Referring the data, we sort out a table (see table 4-3-1).[3]Age Gender Data Package Cases All ages(total) Female 2015-02-04 9458All ages(total) Male 2015-02-04 91500~14 Both sexes 2015-02-04 361815~44 Both sexes 2015-02-04 1009545+ Both sexes 2015-02-04 4226 TABLE 4-3-1. West African Ebola epidemic cases statistics in a year about age and gender We conclude that Ebola infection rate is irrelevant with gender or age.We know the world medical association has announced that their new medication could stop Ebola and cure patients whose disease is not advanced, therefore, we need deliver the drugs to each Ebola outbreak city.4.3.2 The SEIS ModelWhen the delivered drugs cannot meet the city node’s patients, the infective in the secondary city will immigrate higher level city because of its better medical condition and economical strength. The immigration rate is denoted as u, u is a fixed constant. The corresponding SEIS model isFIGURE 4-3-1. A SEIS model of one mode describes the quantitative change relations among the susceptible S(t), the exposed E(t) and the infective I(t).The flow chart describes the quantitative changing relationships among thesusceptible S(t), the exposed E(t) and the infective I(t). For the susceptible compartment S(t), we can see only one arrow inflows, which shows the number of people in the compartment increases. Since the recovery rate is denoted as γand the infective is denoted as I(t), I γ represents the number of the recovered. Effective contact rate is denoted as λ, so SI λ represents the number of the susceptible S(t) who become the exposed E(t) through effective contacting the infective I(t). Consequently, we can obtain the equationI -I =S dtdsλγ. Similarly, analyzing the remaining compartments, the exposed E(t) and the infective I(t), we can get the following equations.⎪⎪⎪⎩⎪⎪⎪⎨⎧I -I -E +=IE -I =-I =βγθαθλλγdt d S dt dESI dt dS⑶ In eq.3, the radio of the exposed who become the infective and the total exposed is denoted as θ, E θrepresents the number of the exposed who become the infective. And since mortality due to illness is denoted as β, I βrepresents the number of deaths due to illness in the infective compartment I(t).[4]4.3.3 Analyzing the Severity of EbolaIn order to analyze the severity of Ebola, we introduce the basic reproduction number R 0. R 0 represent the number of the second generation of infected cases which a patient generated when he mixed himself inside the susceptible population in his effective infectious period.If 10>R , then the disease is contagious and endemic equilibrium exists. When the disease isn’t controlled before P 1(S 1,E 1,I 1), then the disease will continue to steadily survive. Whereas if 10<R , the disease will gradually disappear.It is obvious that the number of Ebola patients is increasing, so we only consider the situation of 10>R . Now we will calculate our endemic equilibrium P 1(S 1,E 1,I 1).According to the equation(3), we devise the equations (4).⎪⎪⎪⎩⎪⎪⎪⎨⎧∆I -I -+E =∆E -I =∆I -I =),,(),,(),,(I E S T dt dII E S W S dt dEI E S Q S dt dSγβαθθλλγ ⑷ The equations (4) are the functions of Q(S,E,I), W(S,E,I), T(S,E,I) related to S,E,I.Calculating the equations ⎪⎩⎪⎨⎧===0),,(0),,(0),,(111111111I E S T I E S W I E S Q ⑸equals to calculating ⎪⎩⎪⎨⎧=I -I -+E =E -I =I -I 000111111111γβαθθλλγS S ⑹We can work out βαθγλγ=I I =E =111,,S . Now that we have worked out our endemic equilibrium P 1(S 1,E 1,I 1), we begin to analyze the severity of Ebola in a certain area. The severity is denoted as η. The larger the value of η is, the more serious Ebola is. We design a table (see table 4-3-2) to distinguish the severity.I(t)/I 10~30% 30%~70%70%~100%η135Table 4-3-2. Our division of the severity according to the ratio of I(t) and I 14.4 The Multi-goal Programming ModelWe should consider the drug production speed and economical factors when wedistribute the drugs.If the medical supply is adequate enough to satisfy the current demand, then we establish the time series model for short-term forecast of the infected area . We predict the possible number of the new infective within a short time and calculate the needed drugs within a short time. Then we can distribute the drugs in advance. Our thought is to nip in the bud. The measure necessarily decreases the number of the newinfective in the future, and succeed in stopping Ebola.Whereas if the medical supply is limited, the speed cannot satisfy the current demand, then we establish the multi-goal programming model to work out the optimal allocation . We draw up the principles of drug allocation. One is the ratio of the number of the infective in the city and the number of the total infective in the same level city, the ratio is denoted as ξ. Notably, the number of the infective in the city equals to the sum of the number of the native patients in the city and the number of the total patients in its children cities. The other is the severity of Ebola, the severity is denoted as η.4.4.1 The Analytic Hierarchy Process (AHP)First, we use the Analytic Hierarchy Process (AHP for short) to calculate the weights of the two principles.[5] The hierarchical structure diagram isFIGURE 4-4-1. The hierarchical structure diagram about the drug distributionIn the hierarchical structure diagram, the first level is the target, the second is thefactors determining the target.[6]We compare the influence degree of the two factors ξ and η on the target factor, the drug distribution. We use j i a ,to represent the ratio of the influence degree ofξand η on the target factor. Meanwhile, we measure j i a ,according to the 1~9 scale. The matrix is())2,1(1,1,0,,,,,22,====>=⨯j i a a a a a A i i ji i j j i j i ⑺ The scale value isTABLE 4-4-1. Scale valueAccording to the scale value (see table 4-4-1), we establish our comparison matrixA. It is as follows12121Gηξηξ⑻ Through normalizing the matrix’s column vector, we can obtain the weight vector.The weight vector is denoted as M .M333.0667.0666.0334.1333.0333.0667.0667.012121ca =⎪⎪⎭⎫ ⎝⎛−−−−−−−−→−⎪⎪⎭⎫⎝⎛−−−−−−−→−⎪⎪⎭⎫ ⎝⎛−−−−−−−−→−⎪⎪⎪⎭⎫ ⎝⎛=vectorvolumn the g normalizin row each of sum lculating vector volumn the g normalizin A ⎪⎪⎭⎫ ⎝⎛=⎪⎪⎭⎫ ⎝⎛⎪⎪⎪⎭⎫ ⎝⎛=665.0333.1333.0667.012121AM ⑼ We can work out the maximum eigenvalue. It is denoted as max λ.998.1)333.0665.0667.0333.1(21max =+=λ ⑽4.4.2 Testing ConsistencyTypically, the actual judgment matrix obtained is not necessarily consistent, that is, it is not necessarily meet the transitivity and the consistency. Actually, we shouldpromise our matrix is roughly consistent, that is, the degree of inconsistency should be in the allowable range. Consistency index:1max --=n nCI λ. In our model , n=2.Random consistency index: RI . RI is usually defined by the actual experience. It is as follows: TABLE 4-4-2. Random consistency indexConsistency ratio index: RICICR =. When 1.0<CR , we can regard the judgment matrix is consistent. For judging the matrix A ,We have worked out the maximum eigenvalue of the matrix A,max λ (see eq.10).998.1max =λBecause the matrix A is a second order matrix (n=2), the consistency index 0=CIConsequently, the consistency ratio index CR meets 1.0<CR . We can conclude the matrix A is consistent, so we can use its feature vector to substitute its weight. So the weight of ξis 0.667, the weight of ηis 0.333.4.4.3 The Multi-goal Programming Model EquationThen we discuss the multi-goal programming model of different level cities respectively.We set the forth-level cities as an example. We can get444333.0667.0F i i i ηξ+=The meaning of the equation is as follows:The total number of drugs equals to the product of the number of the infective in the city and the proportional coefficient of the infective that can get drugs.Where 4F i represents the multi-goal coefficient of the i-th forth level city. 4i ξ represents the ratio of the number of the infective in the i-th forth-level city and then 1 2 34567891011RI0.58 0.90 1.12 1.24 1.32 1.41 1.45 1.49 1.51number of the total infective in the total forth-lever city. 4i η represents the severity of Ebola in the i-th forth level city. We also get∑=⋅⋅=ni ii iiFF m t I H 14444)(The meaning of the equation is as follows:The total number of drugs equals to the sum of the product of the total number of the city, the proportional coefficient of the infective who can get drugs and the number of needed drugs of each patient.Where n represents the number of a certain level cities. 4)(i t I represents the number of the infective in the i-th forth-level city. 4i H represents the number of the distributed drugs in the i-th forth-level city. m represents the number of needed drugs of each patient.To sum up, the forth-level cities is444333.0667.0F i i i ηξ+= ⑾∑=⋅⋅=ni i i ii F F m t I H 14444)( ⑿Because drugs are only transported from the father city to its children cities, so when we calculate each level’s multi-goal factors, we must add up all the multi-goal factors of its total children cities, which is denoted as∑=ni iF14. Similarly, when wecalculate 3)(i t I , we must add up the number of the infective in its children cites. According to the forth-level cities, we can work out the equations of the third-level and the second-level cities. The third-level cities is∑=++=ni i iiiF 14333333.0667.0F ηξ ⒀∑=⋅⋅=ni i i ii F F m t I H 13333)( ⒁Thesecond-levelcitiesis∑=++=ni i iiiF 13222333.0667.0F ηξ ⒂∑=⋅⋅=n i iii i FF m t I H 12222)( ⒃We set the total number of existing drugs as Y. It is easy to know thatY H i =1 ⒄Besides, the total number of drugs in the i-th c-th-level city is1ic i c i H YH K ⋅= ⒅5. Conclusion and Sensitivity Analysis5.1 The Predicted Result of ARIMA Model.(1) In the time series model, we have found Ebola cases always keep a rising trend in a year, so we can conclude that Ebola cases are independent of the season. That is, the changing relationship between the number of patients and time does not exist seasonal difference. In ARIMA, we take 0,0,0===sq sd sp .(2) We calculate the difference of a non-stationary time series until the series becomes a stationary series, the difference frequency is denoted as d .FIGURE 5-1-1. The difference situation of the prepared time series of Guinea。
2015数学建模竞赛B题优秀论文
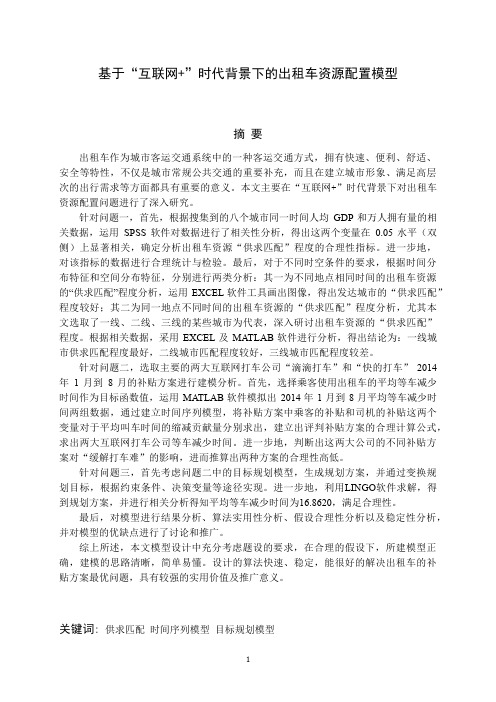
万人拥有量
人均 GDP
由上表1,可得出不同地区的出租车万人拥有量与该地区的人均 GDP的相伴概率值:
Sig 0.05,
即这两个变量在0.05水平(双侧)上显著相关。故GDP是影响出租车“供求匹配”程度 的一个合理性指标。 除此之外,车辆满载率是通过在客流集散较为集中的地点选取几个长期观测点, 公式为:车辆满载率=载客车数(辆)/总通过车数(辆)×100%;里程利用率是一般以一 辆车为单位,公式为:里程利用率=营业里程(公里)/行驶里程(公里)×100%[1]。此指 标反映车辆载客效率,若比例高,说明车辆行驶中载客率比例高,空驶率比较低,乘 客等待时间增加,对于要车的乘客来说供求关系比例紧张;若比例低,说明车辆空驶 率比例高,乘客打车方便,但司机的经济效益下降。 5.1.2 模型的准备 为了衡量指标对出租车的“供求匹配”程度的影响,本文采用出租车万人拥有量 以及 GDP 作为衡量出租车的“供求匹配”程度。 从时间分布上,出租车出行时间分布包括载客时间随时间轴的变化、载客里程随 时间轴的变化以及空驶时间随时间轴的变化,出租车出行在不同时间段上的分布,反映 了城市居民的生活节奏和交通需求在时间上的分布;从空间分布上,出租车的出行空 间分布反映了居民出行空间的流动规律及城市交通的主要流向 , 不同出行目的, 有不 同的空间分布规律[2]。 现采用北京市 24 小时车辆数数据(附件三),通过 MATLAB 软件编程实现,得到 北京市一天出租车需求分布图,如图 1:
4
150 北京市一天出租车需求图 北京市 0 0
5
10 时间t
15
20
25
图 1
北京市郊区一天出租车需求分布图
图 1 表示北京市郊区一天中出租车分布量与需求量,从图中可以得出一天中出租 车的需求量最大的时候就是上下班高峰的时候,出租车的需求量明显增多。而由于一 天二十四小时的出租车分布量与需求量的变化不是固定的。郊区的出租车分布量少, 在一天中大部分时间都小于其需求量,即该地出租车资源“供应匹配”程度明显较低。 5.1.3 模型的求解 为满足在不同时空的条件下,本文分别在不同地点相同时间、不同时间相同地点 下研究供求匹配程度[3]。 (1)不同地点相同时间的出租车“供求匹配”程度分析 首先本文对于不同地点相同时间的出租车“供求匹配”程度进行分析。分别选取 经济发展情况不同的八个城市,分别为:北京、南京、成都、大连、宁波、济南、深 圳、杭州,各城市的人均 GDP 和万人拥有量运用 Excel 进行分析如下图 2:
2015年五一数学建模联赛C题获奖论文
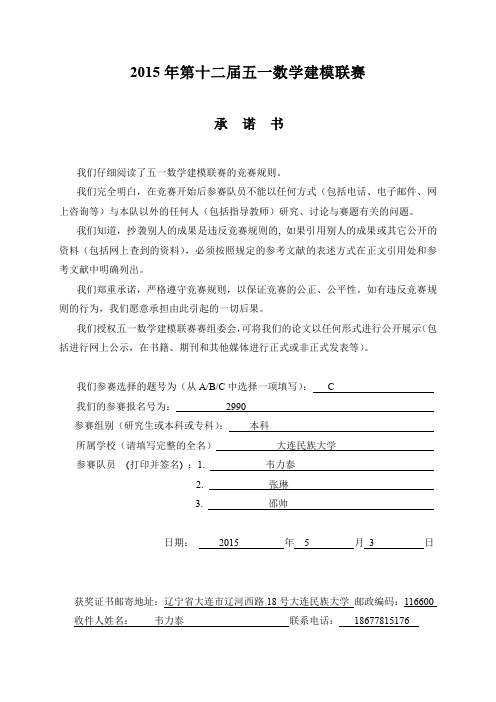
1/5
D3
4
4
1
1/2
D4
6
5
2
1
注:CR=CI/RI=0.0219/0.0244<0.1 通过一致性检验
权重 0.0704 0.1048 0.3122 0.5125
通过建立各系统内部评价指标的判断矩阵,我们从每个系统中筛选出两个权重最大 的指标作为我们评价模型的指标,筛选结果如表:
人口系统 A
表 2-6 生态文明建设评价指标
1.2 问题的提出 为了更好地反映我国的生态文明建设,本文依次提出以下问题: 1)请通过查阅相关文献,了解我国生态文明建设的评价指标和评价模型,列举现有
的生态文明建设的评价指标。 2)对现有生态文明建设的评价指标进行分析,选择其中几个重要的、可行的评价指
标,结合经济发展的情况,建立评价我国生态文明建设状况的数学模型。 3)由于我国地理位置和经济条件的差异,各省(市)生态文明建设水平各有高低,
1
2.3 问题三: 问题三要求利用最新的数据,选取最具有代表性的十个省(市),根据前面建立的
数学模型对这十个省(市)生态文明建设的程度进行评价。我们分别选择了北京市、上 海市、辽宁省、内蒙古自治区、浙江省、陕西省、四川省、云南省、广东省、西藏自治 区等十个省份进行的分析。在本问中,我们利用的是问题二的第二小问(2)中建立的模 型对各个省市的综合指数进行分析的。通过对各个省综合指数的互相比较并与我国综合 指数进行对比,最终这十个省(市)生态文明建设的程度的评价。
我们参赛选择的题号为(从 A/B/C 中选择一项填写): C
我们的参赛报名号为:
2990
参赛组别(研究生或本科或专科): 本科
所属学校(请填写完整的全名)
- 1、下载文档前请自行甄别文档内容的完整性,平台不提供额外的编辑、内容补充、找答案等附加服务。
- 2、"仅部分预览"的文档,不可在线预览部分如存在完整性等问题,可反馈申请退款(可完整预览的文档不适用该条件!)。
- 3、如文档侵犯您的权益,请联系客服反馈,我们会尽快为您处理(人工客服工作时间:9:00-18:30)。
问题三分析:问题三在问题二的基础上又加深了,经度计算仍然和问题2相同。纬度计 算上将日期看成n即可,将n代入计算,得到含n的代数式表示纬度。再由循环求解法将得到 的影子坐标与附件2、3的坐标进行比对,找出可能值。
图 1 夏半年日影运动
由于太阳和地球最短距离为1.471 × 108km,所以太阳光接近地球表面时可以近似看成 是平行光。参考文献[1],太阳高度角是指太阳光的入射方向和地平面之间的夹角,专业上 讲太阳高度角是指某地太阳光线与通过该地与地心相连的地表切线的夹角。如图(2)所 示,OB为竿长,OA为影长,θ即为太阳高度角。
针 对 问 题3, 没 有 给 出 拍 摄 时 间 。 对 于 经 度 , 根 据 影 长 求 解 的 方 法 同 问 题2, 得 到 附 件2和 附 件3的 经 度 分 别 为 东 经143度20分31秒 、 西 经105度19分55秒 。 对 于 纬 度 , 利 用MATLAB计算出对应不同日期的附件时间段内的影子长度数组,与附件2、3中的已有影长 数组进行比对,差异最小的所对应的时间既是拍摄日期。继而根据日期确定拍摄地的纬度。 从而确定拍摄地点和日期。
1
1. 问题重述
太阳影子定位技术是通过分析视频中物体的影子变化,从而确定视频的拍摄时间和地 点。影子变化的根本原因在于地球的公转和自转,已知太阳光是沿直线传播,地球公转的运 动轨迹和地球赤道有一个夹角,因此不同地区的太阳直射点不同。在同一时刻,不同地区的 物体影子变化也是不一样的。而确定拍摄地点和拍摄日期是视频数据分析的重要方面,在实 际生活中应用广泛。因此,建立一个太阳影子定位模型对于解决实际问题是非常必要的。
角距离。在地球上,同一时刻,对同一经度的人来说,太阳对应的时角是相同的。从天文学 上来说,时间可分为平太阳时和真太阳时。平太阳时就是平时参考的国家地区报时时间,如 北京时间,其每天的时间间隔是相等的。真太阳时是以当地太阳位于正南向的瞬时为正午。 由于太阳与地球之间的距离和相对位置随时间在变化,以及地球赤道与其绕太阳运行的轨道 的处平面的不一致,因而真太阳时与钟表指示的时间(平太阳时)之间总会有所差异,它们 的差值即为时差。最大时差可达16min。一年中只有4次时差为零。计算太阳位置时应采用真 太阳时。单位时间地球自转的角度定义为时角,规定正午时角为0,上午时角为负值,下午 时角为正值。地球自转一周360度,对应的时间为24小时,即每小时相应的时角为15度。在 中国地区,经常采用的是北京时间,不是当地时间(真太阳时)。中国地域广阔,东西时差 最大可达到4h,在进行日照分析时,应当采用当地时间。
2
问题四分析:要获取视频内数据,可以利用警视通图像处理系统处理视频,对视频内任 意点定位成坐标,但技术有限本次研究我们采用autoACD 技术进行获取数据。得到数据后 求解方法类似于问题2、3。
3. 问题假设与符号说明
3.1. 问题假设
假设 1:假设太阳光接近地面时近似看成平行光; 假设 2:假设影长随着时间变化规律类似于二次曲线; 假设 3:假设空气折射率对太阳高度角和方位角的影响较小可以忽略; 假设的合理性分析: 对于假设1,由于地球和太阳相距很远,所以把太阳光看作是平行光对结果的影响不 大; 对于假设2,影长随时间先减后增,所以拟合成二次曲线具有科学性。
3.2. 符号说明
• L . . . . . . . . . . . . . . . . . . . . . . . . . . . . . . . . . . . . . . . . . . . . . . . . . . . . . . . . . . . . . . . . . . . . . . . . . . . . 立杆影长 • H . . . . . . . . . . . . . . . . . . . . . . . . . . . . . . . . . . . . . . . . . . . . . . . . . . . . . . . . . . . . . . . . . . . . . . . . . . . . . . . . . 竿长 • θ . . . . . . . . . . . . . . . . . . . . . . . . . . . . . . . . . . . . . . . . . . . . . . . . . . . . . . . . . . . . . . . . . . . . . . . . . . . 太阳高度角 • φ . . . . . . . . . . . . . . . . . . . . . . . . . . . . . . . . . . . . . . . . . . . . . . . . . . . . . . . . . . . . . . . . . . . . . . 观测地地理纬度 • ψ . . . . . . . . . . . . . . . . . . . . . . . . . . . . . . . . . . . . . . . . . . . . . . . . . . . . . . . . . . . . . . . . . . . . . .观测地地理经度 • δ . . . . . . . . . . . . . . . . . . . . . . . . . . . . . . . . . . . . . . . . . . . . . . . . . . . . . . . . . . . . . . . . . . . . . . . . . . . . . . . 赤纬角 • η . . . . . . . . . . . . . . . . . . . . . . . . . . . . . . . . . . . . . . . . . . . . . . . . . . . . . . . . . . . . . . . . . . . . . . . . . . . . . . . 方位角 • σ . . . . . . . . . . . . . . . . . . . . . . . . . . . . . . . . . . . . . . . . . . . . . . . . . . . . . . . . . . . . . . . . . . . . . . . . . . . . . . . . . 时角 • N . . . . . . . . . . . . . . . . . . . . . . . . . . . . . . . . . . . . . . . . . . . . . . . . . . . . . . . . . . . . . . . . . . . . . . . . . . . . . . . . . 积日 • β . . . . . . . . . . . . . . . . . . . . . . . . . . . . . . . . . . . . . . . . . . . . . . . . . . . . . . . . . . . . . . . . . . . . . . . . . . 太阳方位角 • t. . . . . . . . . . . . . . . . . . . . . . . . . . . . . . . . . . . . . . . . . . . . . . . . . . . . . . . . . . . . . . . . . . . . . . . . . . . . . . . .太阳时
针对问题4,我们利用图像长度读取软件来获取视频中影子的长度,拟合出影子长度关 于时间的函数,从而确定正午时刻并计算出拍摄地的经度。再根据竿长2米,确定正午太阳 高度角,进而计算出当地纬度并进行检验。我们得到经度为东经109度40分30秒,纬度为北 纬40度58分41秒,可能的拍摄地点是呼和浩特。
关键词: 日影运动,太阳影子定位,拟合
3
• φ0 . . . . . . . . . . . . . . . . . . . . . . . . . . . . . . . . . . . . . . . . . . . . . . . . . . . . . . . . . . . . . . . . . . . . . 太阳直射点纬度
问题1:给定某个日期、天安门广场的经纬度和北京时间的某个时间段,要求建立影子 长度变化模型,分析影子长度和各个参数的变化规律,并且应用此模型来画出3米高的直杆 的太阳影子长度的变化曲线。
问题2:要求根据某固定直杆在水平地面上的太阳影子顶点坐标,建立数学模型确定直 杆所处的地点。并将所建立的模型应用于附件1的影子顶点坐标数据,给出若干可能的地 点。
针 对 问 题2, 根 据 影 子 的 坐 标 , 求 出 观 测 地 地 点 的 经 纬 度 。 对 于 经 度 , 我 们 运 用 最 小二乘法拟合影子长度关于时间的函数,求出函数最小值点,即是观测地正午时间。 再根据观测地和北京的时差,计算出观测地的经度。经计算和检验得出当地经度为东 经109度21分21秒。对于纬度,我们建立了太阳高度角关于当地纬度和日期变化的模型,再 根据附件1的日期确定太阳直射点的纬度,用MATLAB求解得到纬度为北纬19度40分48秒。 根据得出的经纬度利用Google Earth定位得拍摄地点可能为海南。
2. 问题分析
问题一分析:题目要求我们探究影响影子长度变化的参数,并研究其变化规律。首先应 该从“立杆成影”的原理出发讨论影子形成的原因,显然由于地球的自转和公转造成影子的 变化。所以影响影子的主要参数应该是时间,立杆位置,立杆长度,从而建立影长与经纬 度,时间日期,竿长之间的线性关系,判断变化规律。
太阳影子定位
摘要
本文通过分析影响立杆影长的相关参数的变化,建立了时间、太阳位置和影子轨迹关系 的数学模型,探究了影子变化的影响因素,以及通过影子变化如何确定拍摄时间和地点。