美赛论文优秀模版
美赛数学建模比赛论文模板

The Keep-Right-Except-To-Pass RuleSummaryAs for the first question, it provides a traffic rule of keep right except to pass, requiring us to verify its effectiveness. Firstly, we define one kind of traffic rule different from the rule of the keep right in order to solve the problem clearly; then, we build a Cellular automaton model and a Nasch model by collecting massive data; next, we make full use of the numerical simulation according to several influence factors of traffic flow; At last, by lots of analysis of graph we obtain, we indicate a conclusion as follow: when vehicle density is lower than 0.15, the rule of lane speed control is more effective in terms of the factor of safe in the light traffic; when vehicle density is greater than 0.15, so the rule of keep right except passing is more effective In the heavy traffic.As for the second question, it requires us to testify that whether the conclusion we obtain in the first question is the same apply to the keep left rule. First of all, we build a stochastic multi-lane traffic model; from the view of the vehicle flow stress, we propose that the probability of moving to the right is 0.7and to the left otherwise by making full use of the Bernoulli process from the view of the ping-pong effect, the conclusion is that the choice of the changing lane is random. On the whole, the fundamental reason is the formation of the driving habit, so the conclusion is effective under the rule of keep left.As for the third question, it requires us to demonstrate the effectiveness of the result advised in the first question under the intelligent vehicle control system. Firstly, taking the speed limits into consideration, we build a microscopic traffic simulator model for traffic simulation purposes. Then, we implement a METANET model for prediction state with the use of the MPC traffic controller. Afterwards, we certify that the dynamic speed control measure can improve the traffic flow .Lastly neglecting the safe factor, combining the rule of keep right with the rule of dynamical speed control is the best solution to accelerate the traffic flow overall.Key words:Cellular automaton model Bernoulli process Microscopic traffic simulator model The MPC traffic controlContentContent (2)1. Introduction (3)2. Analysis of the problem (3)3. Assumption (3)4. Symbol Definition (3)5. Models (4)5.1 Building of the Cellular automaton model (4)5.1.1 Verify the effectiveness of the keep right except to pass rule (4)5.1.2 Numerical simulation results and discussion (5)5.1.3 Conclusion (8)5.2 The solving of second question (8)5.2.1 The building of the stochastic multi-lane traffic model (9)5.2.2 Conclusion (9)5.3 Taking the an intelligent vehicle system into a account (9)5.3.1 Introduction of the Intelligent Vehicle Highway Systems (9)5.3.2 Control problem (9)5.3.3 Results and analysis (9)5.3.4 The comprehensive analysis of the result (10)6. Improvement of the model (11)6.1 strength and weakness (11)6.1.1 Strength (11)6.1.2 Weakness (11)6.2 Improvement of the model (11)7. Reference (13)1. IntroductionAs is known to all, it’s essential for us to drive automobiles, thus the driving rules is crucial important. In many countries like USA, China, drivers obey the rules which called “The Keep-Right-Except-To-Pass (that is, when driving automobiles, the rule requires drivers to drive in the right-most unless theyare passing another vehicle)”.2. Analysis of the problemFor the first question, we decide to use the Cellular automaton to build models,then analyze the performance of this rule in light and heavy traffic. Firstly,we mainly use the vehicle density to distinguish the light and heavy traffic; secondly, we consider the traffic flow and safe as the represent variable which denotes the light or heavy traffic; thirdly, we build and analyze a Cellular automaton model; finally, we judge the rule through two different driving rules,and then draw conclusions.3. AssumptionIn order to streamline our model we have made several key assumptions●The highway of double row three lanes that we study can representmulti-lane freeways.●The data that we refer to has certain representativeness and descriptive●Operation condition of the highway not be influenced by blizzard oraccidental factors●Ignore the driver's own abnormal factors, such as drunk driving andfatigue driving●The operation form of highway intelligent system that our analysis canreflect intelligent system●In the intelligent vehicle system, the result of the sampling data hashigh accuracy.4. Symbol Definitioni The number of vehiclest The time5. ModelsBy analyzing the problem, we decided to propose a solution with building a cellular automaton model.5.1 Building of the Cellular automaton modelThanks to its simple rules and convenience for computer simulation, cellular automaton model has been widely used in the study of traffic flow in recent years. Let )(t x i be the position of vehicle i at time t , )(t v i be the speed of vehicle i at time t , p be the random slowing down probability, and R be the proportion of trucks and buses, the distance between vehicle i and the front vehicle at time t is:1)()(1--=-t x t x gap i i i , if the front vehicle is a small vehicle.3)()(1--=-t x t x gap i i i , if the front vehicle is a truck or bus.5.1.1 Verify the effectiveness of the keep right except to pass ruleIn addition, according to the keep right except to pass rule, we define a new rule called: Control rules based on lane speed. The concrete explanation of the new rule as follow:There is no special passing lane under this rule. The speed of the first lane (the far left lane) is 120–100km/h (including 100 km/h);the speed of the second lane (the middle lane) is 100–80km8/h (including80km/h);the speed of the third lane (the far right lane) is below 80km/ h. The speeds of lanes decrease from left to right.● Lane changing rules based lane speed controlIf vehicle on the high-speed lane meets control v v <, ),1)(min()(max v t v t gap i f i +≥, safe b i gap t gap ≥)(, the vehicle will turn into the adjacent right lane, and the speed of the vehicle after lane changing remains unchanged, where control v is the minimum speed of the corresponding lane.● The application of the Nasch model evolutionLet d P be the lane changing probability (taking into account the actual situation that some drivers like driving in a certain lane, and will not takethe initiative to change lanes), )(t gap f i indicates the distance between the vehicle and the nearest front vehicle, )(t gap b i indicates the distance between the vehicle and the nearest following vehicle. In this article, we assume that the minimum safe distance gap safe of lane changing equals to the maximum speed of the following vehicle in the adjacent lanes.Lane changing rules based on keeping right except to passIn general, traffic flow going through a passing zone (Fig. 5.1.1) involves three processes: the diverging process (one traffic flow diverging into two flows), interacting process (interacting between the two flows), and merging process (the two flows merging into one) [4].Fig.5.1.1 Control plan of overtaking process(1) If vehicle on the first lane (passing lane) meets ),1)(min()(max v t v t gap i f i +≥ and safe b i gap t gap ≥)(, the vehicle will turn into the second lane, the speed of the vehicle after lane changing remains unchanged.5.1.2 Numerical simulation results and discussionIn order to facilitate the subsequent discussions, we define the space occupation rate as L N N p truck CAR ⨯⨯+=3/)3(, where CAR N indicates the number ofsmall vehicles on the driveway,truck N indicates the number of trucks and buses on the driveway, and L indicates the total length of the road. The vehicle flow volume Q is the number of vehicles passing a fixed point per unit time,T N Q T /=, where T N is the number of vehicles observed in time duration T .The average speed ∑∑⨯=T it i a v T N V 11)/1(, t i v is the speed of vehicle i at time t . Take overtaking ratio f p as the evaluation indicator of the safety of traffic flow, which is the ratio of the total number of overtaking and the number of vehicles observed. After 20,000 evolution steps, and averaging the last 2000 steps based on time, we have obtained the following experimental results. In order to eliminate the effect of randomicity, we take the systemic average of 20 samples [5].Overtaking ratio of different control rule conditionsBecause different control conditions of road will produce different overtaking ratio, so we first observe relationships among vehicle density, proportion of large vehicles and overtaking ratio under different control conditions.(a) Based on passing lane control (b) Based on speed control Fig.5.1.3Fig.5.1.3 Relationships among vehicle density, proportion of large vehicles and overtaking ratio under different control conditions.It can be seen from Fig. 5.1.3:(1) when the vehicle density is less than 0.05, the overtaking ratio will continue to rise with the increase of vehicle density; when the vehicle density is larger than 0.05, the overtaking ratio will decrease with the increase of vehicle density; when density is greater than 0.12, due to the crowding, it willbecome difficult to overtake, so the overtaking ratio is almost 0.(2) when the proportion of large vehicles is less than 0.5, the overtaking ratio will rise with the increase of large vehicles; when the proportion of large vehicles is about 0.5, the overtaking ratio will reach its peak value; when the proportion of large vehicles is larger than 0.5, the overtaking ratio will decrease with the increase of large vehicles, especially under lane-based control condition s the decline is very clear.● Concrete impact of under different control rules on overtaking ratioFig.5.1.4Fig.5.1.4 Relationships among vehicle density, proportion of large vehicles and overtaking ratio under different control conditions. (Figures in left-hand indicate the passing lane control, figures in right-hand indicate the speed control. 1f P is the overtaking ratio of small vehicles over large vehicles, 2f P is the overtaking ratio of small vehicles over small vehicles, 3f P is the overtaking ratio of large vehicles over small vehicles, 4f P is the overtaking ratio of large vehicles over large vehicles.). It can be seen from Fig. 5.1.4:(1) The overtaking ratio of small vehicles over large vehicles under passing lane control is much higher than that under speed control condition, which is because, under passing lane control condition, high-speed small vehicles have to surpass low-speed large vehicles by the passing lane, while under speed control condition, small vehicles are designed to travel on the high-speed lane, there is no low- speed vehicle in front, thus there is no need to overtake. ● Impact of different control rules on vehicle speedFig. 5.1.5 Relationships among vehicle density, proportion of large vehicles and average speed under different control conditions. (Figures in left-hand indicates passing lane control, figures in right-hand indicates speed control.a X is the average speed of all the vehicles, 1a X is the average speed of all the small vehicles, 2a X is the average speed of all the buses and trucks.).It can be seen from Fig. 5.1.5:(1) The average speed will reduce with the increase of vehicle density and proportion of large vehicles.(2) When vehicle density is less than 0.15,a X ,1a X and 2a X are almost the same under both control conditions.Effect of different control conditions on traffic flowFig.5.1.6Fig. 5.1.6 Relationships among vehicle density, proportion of large vehicles and traffic flow under different control conditions. (Figure a1 indicates passing lane control, figure a2 indicates speed control, and figure b indicates the traffic flow difference between the two conditions.It can be seen from Fig. 5.1.6:(1) When vehicle density is lower than 0.15 and the proportion of large vehicles is from 0.4 to 1, the traffic flow of the two control conditions are basically the same.(2) Except that, the traffic flow under passing lane control condition is slightly larger than that of speed control condition.5.1.3 ConclusionIn this paper, we have established three-lane model of different control conditions, studied the overtaking ratio, speed and traffic flow under different control conditions, vehicle density and proportion of large vehicles.5.2 The solving of second question5.2.1 The building of the stochastic multi-lane traffic model5.2.2 ConclusionOn one hand, from the analysis of the model, in the case the stress is positive, we also consider the jam situation while making the decision. More specifically, if a driver is in a jam situation, applying ))(,2(x P B R results with a tendency of moving to the right lane for this driver. However in reality, drivers tend to find an emptier lane in a jam situation. For this reason, we apply a Bernoulli process )7.0,2(B where the probability of moving to the right is 0.7and to the left otherwise, and the conclusion is under the rule of keep left except to pass, So, the fundamental reason is the formation of the driving habit.5.3 Taking the an intelligent vehicle system into a accountFor the third question, if vehicle transportation on the same roadway was fully under the control of an intelligent system, we make some improvements for the solution proposed by us to perfect the performance of the freeway by lots of analysis.5.3.1 Introduction of the Intelligent Vehicle Highway SystemsWe will use the microscopic traffic simulator model for traffic simulation purposes. The MPC traffic controller that is implemented in the Matlab needs a traffic model to predict the states when the speed limits are applied in Fig.5.3.1. We implement a METANET model for prediction purpose[14].5.3.2 Control problemAs a constraint, the dynamic speed limits are given a maximum and minimum allowed value. The upper bound for the speed limits is 120 km/h, and the lower bound value is 40 km/h. For the calculation of the optimal control values, all speed limits are constrained to this range. When the optimal values are found, they are rounded to a multiplicity of 10 km/h, since this is more clear for human drivers, and also technically feasible without large investments.5.3.3 Results and analysisWhen the density is high, it is more difficult to control the traffic, since the mean speed might already be below the control speed. Therefore, simulations are done using densities at which the shock wave can dissolve without using control, and at densities where the shock wave remains. For each scenario, five simulations for three different cases are done, each with a duration of one hour. The results of the simulations are reported in Table 5.1, 5.2, 5.3.●Enforced speed limits●Intelligent speed adaptationFor the ISA scenario, the desired free-flow speed is about 100% of the speed limit. The desired free-flow speed is modeled as a Gaussian distribution, with a mean value of 100% of the speed limit, and a standard deviation of 5% of the speed limit. Based on this percentage, the influence of the dynamic speed limits is expected to be good[19].5.3.4 The comprehensive analysis of the resultFrom the analysis above, we indicate that adopting the intelligent speed control system can effectively decrease the travel times under the control of an intelligent system, in other words, the measures of dynamic speed control can improve the traffic flow.Evidently, under the intelligent speed control system, the effect of the dynamic speed control measure is better than that under the lane speed control mentioned in the first problem. Because of the application of the intelligent speed control system, it can provide the optimal speed limit in time. In addition, it can guarantee the safe condition with all kinds of detection device and the sensor under the intelligent speed system.On the whole, taking all the analysis from the first problem to the end into a account, when it is in light traffic, we can neglect the factor of safe with the help of the intelligent speed control system.Thus, under the state of the light traffic, we propose a new conclusion different from that in the first problem: the rule of keep right except to pass is more effective than that of lane speed control.And when it is in the heavy traffic, for sparing no effort to improve the operation efficiency of the freeway, we combine the dynamical speed control measure with the rule of keep right except to pass, drawing a conclusion that the application of the dynamical speed control can improve the performance of the freeway.What we should highlight is that we can make some different speed limit as for different section of road or different size of vehicle with the application of the Intelligent Vehicle Highway Systems.In fact, that how the freeway traffic operate is extremely complex, thereby,with the application of the Intelligent Vehicle Highway Systems, by adjusting our solution originally, we make it still effective to freeway traffic.6. Improvement of the model6.1 strength and weakness6.1.1 Strength●it is easy for computer simulating and can be modified flexibly to consideractual traffic conditions ,moreover a large number of images make the model more visual.●The result is effectively achieved all of the goals we set initially, meantimethe conclusion is more persuasive because of we used the Bernoulli equation.●We can get more accurate result as we apply Matlab.6.1.2 Weakness●The relationship between traffic flow and safety is not comprehensivelyanalysis.●Due to there are many traffic factors, we are only studied some of the factors,thus our model need further improved.6.2 Improvement of the modelWhile we compare models under two kinds of traffic rules, thereby we come to the efficiency of driving on the right to improve traffic flow in some circumstance. Due to the rules of comparing is too less, the conclusion is inadequate. In order to improve the accuracy, We further put forward a kinds of traffic rules: speed limit on different type of cars.The possibility of happening traffic accident for some vehicles is larger, and it also brings hidden safe troubles. So we need to consider separately about different or specific vehicle types from the angle of the speed limiting in order to reduce the occurrence of traffic accidents, the highway speed limit signs is in Fig.6.1.Fig .6.1Advantages of the improving model are that it is useful to improve the running condition safety of specific type of vehicle while considering the difference of different types of vehicles. However, we found that the rules may be reduce the road traffic flow through the analysis. In the implementation it should be at the 85V speed of each model as the main reference basis. In recent years, the85V of some researchers for the typical countries from Table 6.1[ 21]:Author Country ModelOttesen and Krammes2000 AmericaLC DC L DC V C ⨯---=01.0012.057.144.10285Andueza2000Venezuela ].[308.9486.7)/894()/2795(25.9885curve horizontal L DC Ra R V T++--=].[tan 819.27)/3032(69.10085gent L R V T +-= Jessen2001America][00239.0614.0279.080.86185LSD ADT G V V P --+=][00212.0432.010.7285NLSD ADT V V P -+=Donnell2001 America22)2(8500724.040.10140.04.78T L G R V --+=22)3(85008369.048.10176.01.75T L G R V --+=22)4(8500810.069.10176.05.74T L G R V --+=22)5(8500934.008.21.83T L G V --=BucchiA.BiasuzziK. And SimoneA.2005Italy DCV 124.0164.6685-= DCE V 4.046.3366.5585--=2855.035.1119.0745.65DC E DC V ---=FitzpatrickAmericaKV 98.17507.11185-= Meanwhile, there are other vehicles driving rules such as speed limit in adverseweather conditions. This rule can improve the safety factor of the vehicle to some extent. At the same time, it limits the speed at the different levels.7. Reference[1] M. Rickert, K. Nagel, M. Schreckenberg, A. Latour, Two lane trafficsimulations using cellular automata, Physica A 231 (1996) 534–550.[20] J.T. Fokkema, Lakshmi Dhevi, Tamil Nadu Traffi c Management and Control inIntelligent Vehicle Highway Systems,18(2009).[21] Yang Li, New Variable Speed Control Approach for Freeway. (2011) 1-66。
美赛论文(最终版)

For office use onlyT1________________ T2________________ T3________________ T4________________Team Control Number 46639Problem ChosenCFor office use onlyF1________________F2________________F3________________F4________________2016 MCM/ICM Summary SheetAn Optimal Investment Strategy ModelSummaryWe develop an optimal investment strategy model that appears to hold promise for providing insight into not only how to sort the schools according to investment priority, but also identify optimal investment amount of a specific school. This model considers a large number of parameters thought to be important to investment in the given College Scorecard Data Set.In order to develop the required model, two sub-models are constructed as follows: 1.For Analytic Hierarchy Process (AHP) Model, we identify the prioritizedcandidate list of schools by synthesizing the elements which have an influence on investment. First we define the specific value of any two elements’ effect on investment. And then the weight of each element’s influence on investment can be identified. Ultimately, we take the relevant parameters into the calculated weight, and then we get any school’s recommended value of investment.2.For Return On Investment M odel, it’s constructed on the basis of AHP Model.Let us suppose that all the investment is used to help the students to pay tuition fee.Then we can see optimal investment as that we help more students to the universities of higher return rate. However, because of dropout rate, there will be an optimization investment amount in each university. Therefore, we can change the problem into a nonlinear programming problem. We identify the optimal investment amount by maximizing return-on-investment.Specific attention is given to the stability and error analysis of our model. The influence of the model is discussed when several fundamental parameters vary. We attempt to use our model to prioritize the schools and identify investment amount of the candidate schools, and then an optimal investment strategy is generated. Ultimately, to demonstrate how our model works, we apply it to the given College Scorecard Data Set. For various situations, we propose an optimal solution. And we also analyze the strengths and weaknesses of our model. We believe that we can make our model more precise if more information are provided.Contents1.Introduction 21.1Restatement of the Problem (2)1.2Our Approach (2)2.Assumptions 23.Notations 34.The Optimal Investment Model 44.1Analytic Hierarchy Process Model (4)4.1.1Constructing the Hierarchy (4)4.1.2Constructing the Judgement Matrix (5)4.1.3Hierarchical Ranking (7)4.2Return On Investment Model (8)4.2.1Overview of the investment strategy (8)4.2.2Analysis of net income and investment cost (9)4.2.3Calculate Return On Investment (11)4.2.4Maximize the Total Net Income (11)5.Test the Model125.1Error Analysis (12)5.2Stability Analysis (13)6.Results136.1Results of Analytic Hierarchy Process (13)6.2Results of Return On Investment Model (14)7.Strengths and Weaknesses157.1Strengths (15)7.2Weaknesses (16)References16 Appendix A Letter to the Chief Financial Officer, Mr. Alpha Chiang.171.Introduction1.1Restatement of the ProblemIn order to help improve educational performance of undergraduates attending colleges and universities in the US, the Goodgrant Foundation intends to donate a total of $100,000,000 to an appropriate group of schools per year, for five years, starting July 2016. We are to develop a model to determine an optimal investment strategy that identifies the school, the investment amount per school, the return on that investment, and the time duration that the organization’s money should be provided to have the highest likelihood of producing a strong positive effect on student performance. Considering that they don’t want to duplicate the investments and focus of other large grant organizations, we interpret optimal investment as a strategy that maximizes the ROI on the premise that we help more students attend better colleges. So the problems to be solved are as follows:1.How to prioritize the schools by optimization level.2.How to measure ROI of a school.3.How to measure investment amount of a specific school.1.2Our ApproachWe offer a model of optimal investment which takes a great many factors in the College Scorecard Data Set into account. To begin with, we make a 1 to N optimized and prioritized candidate list of school we are recommending for investment by the AHP model. For the sake that we invest more students to better school, several factors are considered in the AHP model, such as SAT score, ACT score, etc. And then, we set investment amount of each university in the order of the list according to the standard of maximized ROI. The implement details of the model will be described in section 4.2.AssumptionsWe make the following basic assumptions in order to simplify the problem. And each of our assumptions is justified.1.Investment amount is mainly used for tuition and fees. Considering that theincome of an undergraduate is usually much higher than a high school students, we believe that it’s necessary to help more poor students have a chance to go to college.2.Bank rates will not change during the investment period. The variation ofthe bank rates have a little influence on the income we consider. So we make this assumption just to simplify the model.3.The employment rates and dropout rates will not change, and they aredifferent for different schools4.For return on investment, we only consider monetary income, regardlessof the intangible income.3.NotationsWe use a list of symbols for simplification of expression.4.The Optimal Investment ModelIn this section, we first prioritize schools by the AHP model (Section 4.1), and then calculate ROI value of the schools (Section 4.2). Ultimately, we identify investment amount of every candidate schools according to ROI (Section 4.3).4.1Analytic Hierarchy Process ModelIn order to prioritize schools, we must consider each necessary factor in the College Scorecard Data Set. For each factor, we calculate its weight value. And then, we can identify the investment necessity of each school. So, the model can be developed in 3 steps as follows:4.1.1Constructing the HierarchyWe consider 19 elements to measure priority of candidate schools, which can be seen in Fig 1. The hierarchy could be diagrammed as follows:Fig.1AHP for the investment decisionThe goal is red, the criteria are green and the alternatives are blue. All the alternatives are shown below the lowest level of each criterion. Later in the process, each alternatives will be rated with respect to the criterion directly above it.As they build their hierarchy, we should investigate the values or measurements of the different elements that make it up. If there are published fiscal policy, for example, or school policy, they should be gathered as part of the process. This information will be needed later, when the criteria and alternatives are evaluated.Note that the structure of the investment hierarchy might be different for other foundations. It would definitely be different for a foundation who doesn't care how much his score is, knows he will never dropout, and is intensely interested in math, history, and the numerous aspects of study[1].4.1.2Constructing the Judgement MatrixHierarchy reflects the relationship among elements to consider, but elements in the Criteria Layer don’t always weigh equal during aim measure. In deciders’ mind, each element accounts for a particular proportion.To incorporate their judgments about the various elements in the hierarchy, decision makers compare the elements “two by two”. The fundamental scale for pairwise comparison are shown in Fig 2.Fig 2Right now, let's see which items are compared. Our example will begin with the six criteria in the second row of the hierarchy in Fig 1, though we could begin elsewhere if we want. The criteria will be compared as to how important they are to the decisionmakers, with respect to the goal. Each pair of items in this row will be compared.Fig 3 Investment Judgement MatrixIn the next row, there is a group of 19 alternatives under the criterion. In the subgroup, each pair of alternatives will be compared regarding their importance with respect to the criterion. (As always, their importance is judged by the decision makers.) In the subgroup, there is only one pair of alternatives. They are compared as to how important they are with respect to the criterion.Things change a bit when we get to the alternatives row. Here, the factor in each group of alternatives are compared pair-by-pair with respect to the covering criterion of the group, which is the node directly above them in the hierarchy. What we are doing here is evaluating the models under consideration with respect to score, then with respect to Income, then expenditure, dropout rate, debt and graduation rate.The foundation can evaluate alternatives against their covering criteria in any order they choose. In this case, they choose the order of decreasing priority of the covering criteria.Fig 4 Score Judgement MatrixFig 5 Expenditure Judgement MatrixFig 6 Income Judgement MatrixFig 7 Dropout Judgement MatrixFig 8 Debt Judgement MatrixFig 9 Graduation Matrix4.1.3 Hierarchical RankingWhen the pairwise comparisons are as numerous as those in our example, specialized AHP software can help in making them quickly and efficiently. We will assume that the foundation has access to such software, and that it allows the opinions of various foundations to be combined into an overall opinion for the group.The AHP software uses mathematical calculations to convert these judgments to priorities for each of the six criteria. The details of the calculations are beyond the scope of this article, but are readily available elsewhere[2][3][4][5]. The software also calculates a consistency ratio that expresses the internal consistency of the judgments that have been entered. In this case the judgments showed acceptable consistency, and the software used the foundation’s inputs to assign these new priorities to the criteria:Fig 10.AHP hierarchy for the foundation investing decision.In the end, the AHP software arranges and totals the global priorities for each of the alternatives. Their grand total is 1.000, which is identical to the priority of the goal. Each alternative has a global priority corresponding to its "fit" to all the foundation's judgments about all those aspects of factor. Here is a summary of the global priorities of the alternatives:Fig 114.2 ROI Model4.2.1 Overview of the investment strategyConsider a foundation making investment on a set of N geographically dispersed colleges and university in the United States, D = {1, 2, 3……N }. Then we can select top N schools from the candidate list which has been sorted through analytic hierarchy process. The total investment amount is M per year which is donated by the Goodgrant Foundation. The investment amount is j m for each school j D ∈, satisfying the following balance constraint:j j D mM ∈=∑ (1)W e can’t invest too much or too little money to one school because we want to help more students go to college, and the student should have more choices. Then the investment amount for each school must have a lower limit lu and upper limit bu as follows:j lu m bu ≤≤ (2)The tuition and fees is j p , and the time duration is {1,2,3,4}j t ∈. To simplify ourmodel, we assume that our investment amount is only used for freshmen every year. Because a freshmen oriented investment can get more benefits compared with others. For each school j D ∈, the number of the undergraduate students who will be invested is j n , which can be calculated by the following formula :,jj j j m n j D p t =∈⨯ (3)Figure12The foundation can use the ROI model to identify j m and j t so that it canmaximize the total net income. Figure1 has shown the overview of our investment model. We will then illustrate the principle and solution of this model by a kind of nonlinear programming method.4.2.2 Analysis of net income and investment costIn our return on investment model, we first focus on analysis of net income and investment cost. Obviously, the future earnings of undergraduate students are not only due to the investment itself. There are many meaning factors such as the effort, the money from their parents, the training from their companies. In order to simplify the model, we assume that the investment cost is the most important element and we don’t consider other possible influence factors. Then we can conclude that the total cost of the investment is j m for each school j D ∈.Figure 13For a single student, the meaning of the investment benefits is the expected earnings in the future. Assuming that the student is not going to college or university after graduating from high school and is directly going to work. Then his wage base is 0b as a high school graduate. If he works as a college graduate, then his wage base is 0a . Then we can give the future proceeds of life which is represented symbolically by T and we use r to represent the bank rates which will change over time. We assume that the bank rates will not change during the investment period. Here, we use bank rates in 2016 to represent the r . The future proceeds of life of a single undergraduate student will be different due to individual differences such as age, physical condition environment, etc. If we consider these differences, the calculation process will be complicated. For simplicity’s sake, we uniform the future proceeds of life T for 20 years. Then we will give two economics formulas to calculate the total expected income in the next T years for graduates and high school graduates:40(1)Tk k a u r +==+∑(4) 40(1)T kk b h r +==+∑(5) The total expected income of a graduate is u , and the total expected income of a highschool graduate is h .Then, we continue to analyze the net income. The net income can be calculated by the following formula:os NetIncome TotalIncome C t =- (6) For each school j D ∈, the net income is j P , the total income is j Q , and the cost is j m . Then we will get the following equation through formula (6):j j j P Q m =- (7)Therefore, the key of the problem is how to calculate j Q . In order to calculate j Q, weneed to estimate the number of future employment j ne . The total number of the invested is j n , which has been calculated above. Considering the dropout rates j α and the employment rates j β for each school j , we can calculate the number of future employment j ne through the following formula:(4)(1)jt j j j j n e n βα-=⨯⨯- (8)That way, we can calculate j Q by the following formula:()j j Q ne u h =⨯- (9)Finally, we take Eq. (2) (3) (4) (7) (8) into Eq. (6), and we will obtain Eq. (9) as follows:4(4)00400(1)()(1)(1)j TT t j j j j j k kk k j jm a b P m p t r r βα+-+===⨯⨯-⨯--⨯++∑∑ (10) We next reformulate the above equation of j P for concise presentation:(4)(1)j t j jj j j jc m P m t λα-⨯⨯=⨯-- (11)where jj j p βλ= and 400400(1)(1)TT k kk k a b c r r ++===-++∑∑ .4.2.3 Calculate Return On InvestmentROI is short of return on investment which can be determined by net income andinvestment cost [7]. It conveys the meaning of the financial assessment. For each schoolj D ∈ , the net income is j P , and the investment cost equals to j m . Then the j ROIcan be calculated by the following formula:100%j j jP ROI m =⨯ (12)We substitute Eq. (10) into Eq. (11), and we will get a new formula as follows:(4)((1)1)100%j t j j j jc ROI t λα-⨯=⨯--⨯ (13)4.2.4 Maximize the Total Net IncomeGiven the net income of each school, we formulate the portfolio problem that maximize the total net income, S=Max(4)((1))j t j jj j j j Dj Djc m P m t λα-∈∈⨯⨯=⨯--∑∑ (14)S. T.jj DmM ∈=∑,{1,2,3,4}t = ,j lu m bu ≤≤ ,Considering the constraint jj DmM ∈=∑, we can further simplify the model,S is equivalent to S’=Max(4)((1))j t j jj j j Dj Djc m P t λα-∈∈⨯⨯=⨯-∑∑ (15)S. T.jj DmM ∈=∑,{1,2,3,4t = ,j l u m b u ≤≤. By solving the nonlinear programming problem S’, we can get the sameanswer as problem S.5. Testing the Model 5.1 Error AnalysisSince the advent of analytic hierarchy process, people pay more attention to it due to the specific applicability, convenience, practicability and systematization of the method. Analytic hierarchy process has not reached the ideal situation whether in theory or application level because the results depend largely on the preference and subjective judgment. In this part, we will analyze the human error problem in analytic hierarchy process.Human error is mainly caused by human factors. The human error mainly reflects on the structure of the judgment matrix. The causes of the error are the following points:1. The number of times that human judge the factors’ importance is excessive.2. The calibration method is not perfect.Then we will give some methods to reduce errors:1. Reduce times of human judgment. One person repeatedly gave the samejudgment between two factors. Or many persons gave the same judgment between two factors one time. Finally, we take the average as result.2. Break the original calibration method. If we have defined the ranking vector111121(,...)n a a a a =between the factor 1A with others. Then we can get all theother ranking vector. For example : 12122111(,1...)na a a a a =.5.2 Stability AnalysisIt is necessary to analyze the stability of ranking result [6], because the strong subjectivefactors. If the ranking result changed a little while the judgment changed a lot, we can conclude that the method is effective and the result is acceptable, and vice versa. We assume that the weight of other factors will change if the weight of one factor changed from i ξ to i η:[8](1)(,1,2...,)(1)i j j i i j n i j ηξηξ-⨯==≠- (16)And it is simple to verify the equation:11nii η==∑ (17)And the new ranking vector ω will be:A ωη=⨯ (18)By this method, the Relative importance between other factors remain the same while one of the factor has changed.6. Results6.1 Results of Analytic Hierarchy ProcessWe can ranking colleges through the analytic hierarchy process, and we can get the top N = 20 schools as follows6.2 Results of Return On Investment ModelBased on the results above, we next use ROI model to distribute investment amountj m and time duration j t for each school j D ∈ by solving the following problem:Max (4)((1))j t j jj j j Dj Djc m P t λα-∈∈⨯⨯=⨯-∑∑S. T.jj DmM ∈=∑,{1,2,3,4t = , j l u m b u≤≤ . In order to solve the problem above, we collected the data from different sources. Inthe end, we solve the model with Lingo software. The program code is as follows:model: sets:roi/1..20/:a,b,p,m,t;endsets data:a = 0.9642 0.9250 0.9484 0.9422 0.9402 0.9498 0.90490.9263 0.9769 0.9553 0.9351 0.9123 0.9410 0.98610.9790 0.9640 0.8644 0.9598 0.9659 0.9720;b = 0.8024 0.7339 0.8737 0.8308 0.8681 0.7998 0.74920.6050 0.8342 0.8217 0.8940 0.8873 0.8495 0.87520.8333 0.8604 0.8176 0.8916 0.7527 0.8659;p = 3.3484 3.7971 3.3070 3.3386 3.3371 3.4956 3.22204.0306 2.8544 3.1503 3.2986 3.3087 3.3419 2.78452.9597 2.92713.3742 2.7801 2.5667 2.8058;c = 49.5528;enddatamax=@sum(roi(I):m(I)/t(I)/p(I)*((1-b(I))^4)*c*(1-a(I)+0.05)^(4-t(I)));@for(roi:@gin(t));@for(roi(I):@bnd(1,t(I),4));@for(roi(I):@bnd(0,m(I),100));@sum(roi(I):m(I))=1000;ENDFinally, we can get the investment amount and time duration distribution as follows:7.Strengths and Weaknesses7.1Strengths1.Fixing the bank rates during the investment period may run out, but it will haveonly marginal influences.2.For return on investment, we only consider monetary income, regardless of the3.intangible income. But the quantization of these intangible income is very importantand difficult. It needs to do too much complicated technical analysis and to quantify 4.too many variables. Considering that the investment persists for a short time, thiskind of random error is acceptable.5.Due to our investment which is freshmen oriented, other students may feel unfair.It is likely to produce adverse reaction to our investment strategy.6.The cost estimation is not impeccable. We only consider the investment amount andignore other non-monetary investment.5. AHP needs higher requirements for personnel quality.7.2Weaknesses1.Our investment strategy is distinct and clear, and it is convenient to implement.2.Our model not only identifies the investment amount for each school, but alsoidentifies the time duration that the organization’s money should be provide d.3.Data processing is convenient, because the most data we use is constant, average ormedian.4.Data sources are reliable. Our investment strategy is based on some meaningful anddefendable subset of two data sets.5.AHP is more simple, effective and universal.References[1] Saaty, Thomas L. (2008). Decision Making for Leaders: The Analytic Hierarchy Process for Decisions in a Complex World. Pittsburgh, Pennsylvania: RWS Publications. ISBN 0-9620317-8-X.[2] Bhushan, Navneet, Kanwal Rai (January 2004). Strategic Decision Making: Applying the Analytic Hierarchy Process. London: Springer-Verlag. ISBN 1-8523375-6-7.[3] Saaty, Thomas L. (2001). Fundamentals of Decision Making and Priority Theory. Pittsburgh, Pennsylvania: RWS Publications. ISBN 0-9620317-6-3.[4] Trick, Michael A. (1996-11-23). "Analytic Hierarchy Process". Class Notes. Carnegie Mellon University Tepper School of Business. Retrieved 2008-03-02.[5] Meixner, Oliver; Reiner Haas (2002). Computergestützte Entscheidungs-findung: Expert Choice und AHP – innovative Werkzeuge zur Lösung komplexer Probleme (in German). Frankfurt/Wien: Redline Wirtschaft bei Ueberreuter. ISBN 3-8323-0909-8.[6] Hazelkorn, E. The Impact of League Tables and Ranking System on Higher Education Decision Making [J]. Higher Education Management and Policy, 2007, 19(2), 87-110.[7] Leslie: Trainer Assessment: A Guide to Measuring the Performance of Trainers and Facilitors, Second Edition, Gower Publishing Limited, 2002.[8] Aguaron J, Moreno-Jimenea J M. Local stability intervals in the analytic hierarchy process. European Journal of Operational Research. 2000Letter to the Chief Financial Officer, Mr. Alpha Chiang. February 1th, 2016.I am writing this letter to introduce our optimal investment strategy. Before I describe our model, I want to discuss our proposed concept of a return-on-investment (ROI). And then I will describe the optimal investment model by construct two sub-model, namely AHP model and ROI model. Finally, the major results of the model simulation will be showed up to you.Considering that the Goodgrant Foundation aims to help improve educational performance of undergraduates attending colleges and universities in the US, we interpret return-on-investment as the increased income of undergraduates. Because the income of an undergraduate is generally much higher than a high school graduate, we suggest all the investment be used to pay for the tuition and fees. In that case, if we take both the income of undergraduates’ income and dropout rate into account, we can get the return-in-investment value.Our model begins with the production of an optimized and prioritized candidate list of schools you are recommending for investment. This sorted list of school is constructed through the use of specification that you would be fully qualified to provided, such as the score of school, the income of graduate student, the dropout rate, etc. With this information, a precise investment list of schools will be produced for donation select.Furthermore, we developed the second sub-model, ROI model, which identifies the investment amount of each school per year. If we invest more money in a school, more students will have a chance to go to college. However, there is an optimal investment amount of specific school because of the existence of dropout. So, we can identify every candidate school’s in vestment amount by solve a nonlinear programming problem. Ultimately, the result of the model simulation show that Washington University, New York University and Boston College are three schools that worth investing most. And detailed simulation can be seen in our MCM Contest article.We hope that this model is sufficient in meeting your needs in any further donation and future philanthropic educational investments within the United States.。
美赛论文模版
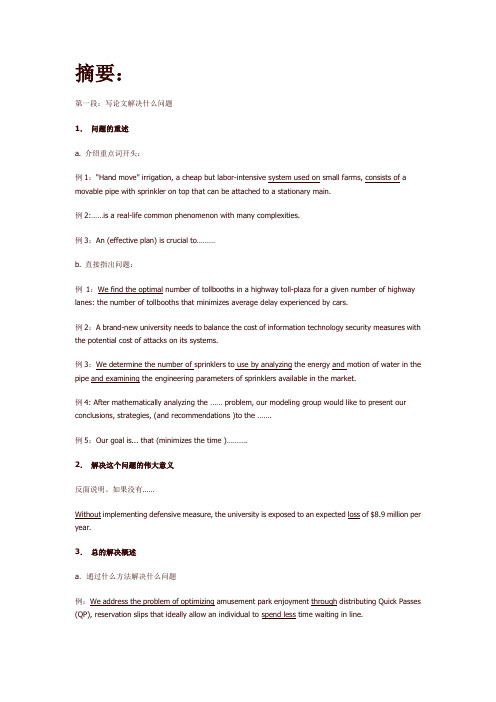
摘要:第一段:写论文解决什么问题1.问题的重述a. 介绍重点词开头:例1:“Hand move” irrigation, a cheap but labor-intensive system used on small farms, consists of a movable pipe with sprinkler on top that can be attached to a stationary main.例2:……is a real-life common phenomenon with many complexi t ies.例3:An (effective plan) is crucial to………b. 直接指出问题:例1:We find the optimal number of tollbooths in a highway toll-plaza for a given number of highway lanes: the number of tollbooths that minimizes average delay experienced by cars.例2:A brand-new university needs to balance the cost of information technology security measures wi t h the potential cost of attacks on its systems.例3:We determine the number of sprinklers to use by analyzing the energy and motion of water in the pipe and examining the engineering parameters of sprinklers available in the market.例4: After mathematically analyzing the …… problem, our modeling group would like to present our conclusions, strategies, (and recommendations )to the …….例5:Our goal is... that (mini mizes the time )……….2.解决这个问题的伟大意义反面说明。
美赛写作材料模板及参赛经验分享
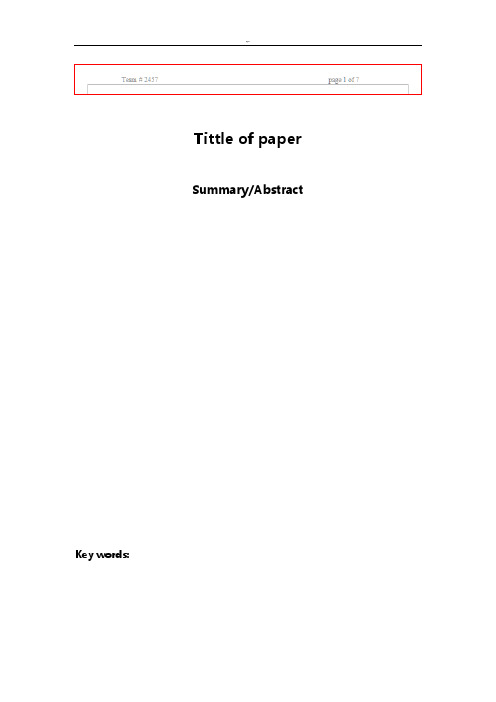
Tittle of paperSummary/Abstract Key words:I.Introduction(引言)Organ transplantation is a preferable treatment for the most serious forms of end-stage diseases. In recent years, advances in medical science and technology have made solid organ transplantation an increasingly successful and common medical procedure, a literal ''second chance at life". Not only does it offer the best hope for complete rehabilitation, but it has also proved to be the most cost-effective of all treatment options, including dialysis. Consequently, more and more people are benefiting from organ transplants and their survival rates are steadily improving. The surgical techniques involved have been mastered for half a century and are now considered as routine. The two main sources of kidneys for transplantation are deceased-donor kidneys and live-donations from family and friends. However, unfortunately, there is a considerable shortage of donor organs, compared to demands. As a matter of fact, efficient matching and allocation of organs donated has become an exigent problem.The United Network for Organ Sharing (UNOS), as the operator of the Organ Procurement and Transplantation Network (OPTN), is responsible for transplant organ distribution in the United States. UNOS oversees the allocation of many different types of transplants, including liver, kidney, pancreas, heart, lung, and cornea.Focusing on kidney transplantation, based on UNOS Kidney Allocation Model, we develop a mathematical model for US transplant networks. First, incomingorgans are matched with waiting candidates by medical institutions considering the factors as ABO blood compatibility, the degree of recipient major HLA mismatch in order to obtain a matching degree applied on the allocation part. After that, from the patients’perspective, on the basis of linear regression, priority weight is defined by pondering age, disease severity, time on waiting, PRA level, and region. Applying this mechanism of ranking, we realize MWBM (Maximum Weight Bipartite-graph Matching) and SMGS (Stable Matching based on Gale-Shapley algorithm). MWBM focuses on the optimal assignment of donors following the algorithm of bipartite-graph maximum weight matching; SMGS emphasizes the process of exchanges in order to obtain the stable exchanges between donors and candidates on the waiting list.II.The Description of Problem(问题重述)III.Basic Assumptions●The level of mismatch is only relative to the number of antigens.●The data and information are accurately registered according to the medicalmeasures●The data and information are refreshed in time according to the status of thepatients●No differences in the quality of the donor kidneys●The quality of the donor kidney is constantIV.Definitions and Notations●Kidney transplantation: A kidney transplant is a surgical procedure to implant ahealthy kidney into a patient with kidney failure.●Prioritization●MD: Matching Degree●PW: Prioritization weight●MWSM: Maximum Weight Bipartite Matching●SMGS: Stable Matching based on Gale-Shapley algorithm或V.ModelsThrough the investigation of US transplantation network, we draw a general picture of the mechanism. With reference to some resources available on the website of UNOS, a flow chart (Figure 1) is developed showing the procedure of the network.Currently, the initial waiting list is composed of patients who are waiting for a kidney or combined kidney-pancreas transplant. For the first time, the patients arerequested to show the correct and scientific information to the US kidney transplant network which is needed for donor-recipient matching, the ranking of patients on the waiting list, and determining the outcome of those transplanted. The patients’waiting lists are composed of initial patients, historical patients and unsuccessful recipient after transplantation. Historical patients refer to registered patients whose status have changed and have an influence on the procedure. A patient is taken off the waiting list when a graft is offered and accepted by that patient or the patient is dead while waiting for a transplant. Unsuccessful recipients refer to the patients who have a bad result of transplantation calling for transplantation again, as it is so-called relistFigure 1. A schematic depicting the steps occurring in the transplantation networks......Table 1.Survival rate involving HLA mismatchVI.Conclusions.Our model for the optimal allocation of the donor organs is established by three modules, procurement of MD and PW, optimal assignment by MWBM model and Stable Matching of Gale-Shapley algorithm. The model has offered a convincing procedure of the allocation with the ……VII.Strengths and weaknesses(模型优缺点)Strengths●……Weaknesses●VIII.References注意文献的积累,不要等到文章写完再去重新寻找文献。
美赛论文LaTeX模板
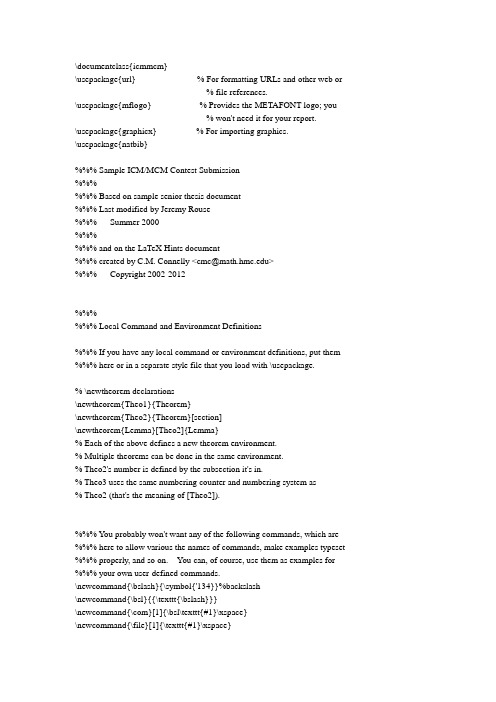
\documentclass{icmmcm}\usepackage{url} % For formatting URLs and other web or% file references.\usepackage{mflogo} % Provides the METAFONT logo; you% won't need it for your report.\usepackage{graphicx} % For importing graphics.\usepackage{natbib}%%% Sample ICM/MCM Contest Submission%%%%%% Based on sample senior thesis document%%% Last modified by Jeremy Rouse%%% Summer 2000%%%%%% and on the LaTeX Hints document%%% created by C.M. Connelly <cmc@>%%% Copyright 2002-2012%%% ---------------%%% Local Command and Environment Definitions%%% If you have any local command or environment definitions, put them %%% here or in a separate style file that you load with \usepackage.% \newtheorem declarations\newtheorem{Theo1}{Theorem}\newtheorem{Theo2}{Theorem}[section]\newtheorem{Lemma}[Theo2]{Lemma}% Each of the above defines a new theorem environment.% Multiple theorems can be done in the same environment.% Theo2's number is defined by the subsection it's in.% Theo3 uses the same numbering counter and numbering system as% Theo2 (that's the meaning of [Theo2]).%%% Y ou probably won't want any of the following commands, which are %%% here to allow various the names of commands, make examples typeset %%% properly, and so on. Y ou can, of course, use them as examples for %%% your own user-defined commands.\newcommand{\bslash}{\symbol{'134}}%backslash\newcommand{\bsl}{{\texttt{\bslash}}}\newcommand{\com}[1]{\bsl\texttt{#1}\xspace}\newcommand{\file}[1]{\texttt{#1}\xspace}\newcommand{\pdftex}{PDF\tex}\newcommand{\pdflatex}{PDF\latex}\newcommand{\acronym}[1]{\textsc{#1}\xspace}\newcommand{\key}[1]{\textsf{\emph{#1}}\xspace}\newcommand{\class}[1]{\textsf{#1}\xspace}\newcommand{\package}[1]{\textsf{#1}\xspace}\newcommand{\env}[1]{\texttt{#1}\xspace}\newcommand{\prog}[1]{\texttt{#1}\xspace}\newcommand{\command}[1]{\texttt{\bsl{}#1}\xspace}\newcommand{\ctt}{\texttt{comp.text.tex}\xspace}\newcommand{\tex}{\TeX\xspace}\newcommand{\latex}{\LaTeX\xspace}%%% Note that the \xspace command comes from the xspace package. It %%% allows you type a command that inserts text without having to %%% worry about how you ``end'' that command.%%%%%% Without \xspace, you would need to end a command with a backslash %%% followed by a space or with an empty set of braces if you followed %%% the command with a space. For example,%%%%%% \foo is a very important algorithm.%%%%%% might produce%%%%%% The foobarbaz algorithmis a very important algorithm.%%%%%% whereas with the \xspace command, the same code would produce %%%%%% The foobarbaz algorithm is a very important algorithm.%%%%%% If you need to butt a command that produces text against a letter %%% of some sort -- say, to pluralize it -- you need to tell TeX%%% where your command name ends so that it expands the correct %%% macro. So you might do%%%%%% \bar{}s are very busy creatures.%%% TeX has an amazingly good hyphenation algorithm, but sometimes it %%% gets confused and needs some help.%%%%%% For words that only occur once or twice, you can insert hints%%% directly into your text, as in%%%%%% our data\-base system is one of the most complex ever devised %%%%%% For words that you use a lot, and that seem to keep ending up at %%% the end of a line, however, inserting the hints each time gets to %%% be a drag. Y ou can use the \hyphenation command to globally tell %%% TeX where to hyphenate words it can't figure out on its own.\hyphenation{white-space}%%% End Local Command and Environment Definitions%%% ---------------%%% ---------------%%% Title Block\title{\latex Hints for ICM/MCM Contest Reports}%%% Which contest are you taking part in? (Just one!)\contest{ICM/MCM}%%% The question you answered. (Again, just the one.)\question{Report Sample}%%% Y our Contest Team Control Number\team{21247}%%% A normal document would specify the author's name (and possibly %%% their affiliation or other information) in an \author command. %%% Because the ICM/MCM Contest rules specify that the names of the %%% team members, their advisor, and their institution should not%%% appear anywhere in the report, do *not* define an \author command.%%% Defining the \date command is optional. If you leave it blank, %%% your document will include the date that the file is typeset, in %%% the form ``Month dd, yyyy''.% \date{}%%% End Title Block%%% ---------------\begin{document}%%% ---------------%%% Summary\begin{summary}This document is meant to give you a quick introduction to \TeX\ and \LaTeX. It covers a lot of material, but still barely manages to scratch the surface. It should provide you with some inspiration and, I hope, with some useful code you can copy, modify, and use in your report.Y ou should use the \file{blank-template.tex} file as a basis foryour report rather than this file. Be sure to change its name to something sensible (maybe your team control number), and to set the values of the \com{title}, \com{question}, and \com{team} commands to appropriate values.Good luck!\hfill{}-- Claire\end{summary}%%% End Summary%%% ---------------%%% ---------------%%% Print Title Block, Contents, et al.\maketitle\tableofcontents%%% Uncomment the following lines if you have figures or tables in %%% your report:\listoffigures\listoftables%%% End Print Title Block, Contents, et al.%%% ---------------\section{Introduction: What Is \latex?}%\label{sec:introduction}\latex is a tool that allows you to concentrate on your writing while taking advantage of the \tex typesetting system to producehigh-quality typeset documents.\latex's benefits include\begin{enumerate}\item Standardized document classes\item Structural frameworks for organizing documents\item Automatic numbering and cross-referencing of structural elements \item ``Floating'' figures and tables\item High-level programming interface for accessing \tex'stypesetting capabilities\item Access to \latex extensions through loading ``packages''\end{enumerate}\section{Structured Writing}%\label{sec:structured-writing}Like HTML,\footnote{HyperText Markup Language} \latex is a markup language rather than a \acronym{Wysiwyg}{}\footnote{What Y ou See Is What Y ou Get.} system. Y ou write plain text files that use special \key{commands} and \key{environments} that govern the appearance and function of parts of your text in your final typeset document.\subsection{Document Classes}%\label{sec:document-classes}The general appearance of your document is determined by your choice of \key{document class}. Document classes also load \latex packagesto provide additional functionality.\latex provides a number of basic classes, including \class{article},\class{letter}, \class{report}, and \class{book}. There are also alarge number of other document classes available, including\class{amsart} and \class{amsbook}, created by the American Mathematical Society and providing some additional mathematically useful structures and commands; \class{foils}, \class{prosper}, and\class{seminar}, which allow you to create ``slides'' for presentations; the math department's \class{thesis} class, forformatting senior theses; and many journal- or company-specific classes that format your document to match the ``house style'' of a particular periodical or publisher.\subsection{Packages}%\label{sec:packages}%\label{sec:ctan}\latex packages, or \key{style files}, define additional commands and environments, or change the way that previously defined commands and environments work. By loading packages, you can change the fonts used in your document, write your document in a non-English language with a non-\acronym{Ascii} font encoding, include graphics, format program listings, add custom headers and footers to your document, and much more.A typical \tex installation includes hundreds of style files, andhundreds more are available from the Comprehensive \tex Archive Network (CTAN), at \url{/}.\subsection{Structural Commands}%\label{sec:structural-commands}\begin{table}\centering\begin{tabular}{ll}\topruleCommand & Notes \\ \midrule\com{part} & \class{book} \& \class{report} only \\\com{chapter} &\class{book} \& \class{report} only \\\com{section} \\\com{subsection} \\\com{subsubsection} \\\com{paragraph} \\\com{subparagraph} \\\bottomrule\end{tabular}\caption[Structural commands in \latex]{Structural commands in \latex.}% \label{tab:structural-commands}\latex provides a set of structural commands for defining sections ofyour document, as shown in Table~\ref{tab:structural-commands}.Note that the argument to structural commands are moving arguments (see Section~\ref{sec:fragile-commands}) because they can be reused in the table of contents or in page headers or footers. Structural commands can take an optional argument in which you specify nonfragile commands or a shorter version of the actual section title that fits.Y ou'll generally know when you need to provide an optional argument by \TeX's behavior.\subsection{Labels and References}%\label{sec:labels-and-references}Sections are numbered automatically by \latex during typesetting. Ifyou change your mind and decide that a subsection should be promotedto a section, or moved to the end of your document, the sections willbe renumbered so that the numbers are consistent.Sections can also be \command{label}{}ed with a tag such as\begin{quote}\begin{verbatim}\section{Our Complicated Equations}%\label{sec:complicated-eqs}\end{verbatim}\end{quote}and referred to with a \command{ref} or \command{pageref} command, as in\begin{quote}\begin{verbatim}In Section~\ref{sec:complicated-eqs}, we pointed out...\end{verbatim}\end{quote}or\begin{quote}\begin{verbatim}On page~\pageref{fig:gordian-knot}, we illustrated...\end{verbatim}\end{quote}\latex substitutes the correct section number when typesetting yourThe same commands can be used with numbered environments such as\env{equation}, \env{theorem}, and so forth.Use \emph{meaningful} labels---labeling a section as \texttt{sec12}may seem useful, but it will be confusing if you end up moving it to a different place in the document and its number changes to Section~34.It's also easier to remember what reference you want if you use a meaningful name.Y ou may also want to impose some additional organization through the use of \emph{namespaces}, as I've done in this document. Rather than give different types of objects undistinguished labels, I precedesection labels with \texttt{sec:}, equations with \texttt{eq:},figures with \texttt{fig:}, tables with \texttt{tab:}, and so on.Emacs with Aux\tex and Ref\tex gives you easy access to these labels,as do many other editors with \tex-specific features. It's mucheasier to find the particular label you're looking for if you havesome additional information to help you. Adding the prefixes also reminds you of what text should precede the \com{ref} command.\subsection{Commands}\latex uses commands for changes that are very limited in scope (a few words) or are unlimited in scope (the rest of a document). For example, the commands\begin{quote}\begin{verbatim}\textbf{bold}\emph{italic (emphasized)}\textsf{sans serif}\end{verbatim}\end{quote}produce the following output in a typeset document:\begin{quote}\textbf{bold} \emph{italic (emphasized)} \textsf{sans serif}\end{quote}These are ``commands with arguments''---the command itself starts witha backslash (\bsl), and its \key{argument} appears inside braces{\verb+{ }+). Some commands may also have \key{optional arguments},which are typed inside brackets (\verb+[ ]+).There are also commands that take no arguments, such as\command{noindent}, \command{raggedright}, and \command{pagebreak}.Y ou can define your own commands, as discussed inSection~\ref{sec:customization}.\subsection{Environments}%\label{sec:environments}\latex provides a number of \key{environments} that affect the appearance of text, and are generally used for more structurally significant purposes. For example, the commands listed above are typeset inside a \env{verbatim} environment typed inside a \env{quote} environment. Their results were typeset inside a \env{quote} environment.Environments use special commands to start and close---\command{begin} and \command{end}, followed by the name of the environment in braces, as in\begin{quote}\begin{verbatim}\begin{quote}``This is disgusting---I can't eat this. That arugala is sobitter\ldots{} It's like my algebra teacher on bread.''\flushright -- Julia Roberts in \emph{Full Frontal}\end{quote}\end{verbatim}\end{quote}producing\begin{quote}``This is disgusting---I can't eat this. That arugala is sobitter\ldots{} It's like my algebra teacher on bread.''\flushright -- Julia Roberts in \emph{Full Frontal}\end{quote}Some environments may take additional arguments in braces (required)or brackets (optional).Note that the order in which environments nest is extremely important.If you type an environment inside another environment, the inner environment must be \command{end}{}ed \emph{before} the secondenvironment is closed. It's also vitally important that you have an\command{end} line for each \command{begin} line, or \latex will complain.\subsubsection{The \env{document} Environment and the Preamble}%\label{sec:document-environment}The most important environment is the \env{document} environment, which encloses the \key{body} of your document. The code before the \command{begin}\verb+{document}+ line is called the \key{preamble}, and includes the all-powerful \command{documentclass} command, which loads a particular document class (seeSection~\ref{sec:document-classes}); optional \command{usepackage} commands, which load in additional \latex packages (seeSection~\ref{sec:packages}); and other setup commands, such asuser-defined commands and environments, counter settings, and so forth.I generally also include the commands defining the title, author, anddate in my preambles, but other people include them just after\command{begin}\verb+{document}+, before the \command{maketitle} command, which creates the title block of your document.\subsubsection{Math Environments}%\label{sec:math-environments}One of the major hallmarks of \tex is its ability to typesetmathematical equations.The two primary ways of doing so are with the use of \key{inline} and\key{display math environments}. These environments are used so often that there are shorthands provided for typing them. Inline math environments, such as $a^2 + b^2 = c^2$, can be typed as\begin{quote}\begin{verbatim}\begin{math}a^{2} + b^{2} = c^{2}\end{math}\end{verbatim}\end{quote}or\begin{quote}\begin{verbatim}$a^{2} + b^{2} = c^{2}$.\end{verbatim}\end{quote}Display math environments set your equation apart from your running text. They're generally used for more complicated expressions, such as\[f(x) = \int \left( \frac{x^2 + x^3}{1} \right)dx\]which can be typed as\begin{quote}\begin{verbatim}\begin{displaymath}f(x) = \int \left( \frac{x^2 + x^3}{1} \right)dx\end{displaymath}\end{verbatim}\end{quote}or\begin{quote}\begin{verbatim}\[f(x) = \int \left( \frac{x^2 + x^3}{1} \right)dx\]\end{verbatim}\end{quote}Generally, you'll want to use the \verb+$+ %$ <- fool font-lock-modedelimited form for inline math, and the \com{[} \com{]} form for display math environments. [Besides being easy to type, these forms are \key{robust}, which means that they can be used in \key{moving arguments}, elements that \tex may need to typeset in more than one place (such as a table of contents) or adjust (such as footnotes).]\paragraph{The \env{equation} Environment}%\label{sec:equation-environment}Y ou'll probably want to use the \env{equation} environment for any formula you plan to refer to. \latex not only typesets the contentsof an \env{equation} environment in display mode, it also numbers it, as in\begin{equation}\label{eq:myequation}f(x) = \int \left( \frac{x^2 + x^3}{1} \right)dx\end{equation}written as\begin{quote}\begin{verbatim}\begin{equation}\label{eq:myequation}f(x) = \int \left( \frac{x^2 + x^3}{1} \right)dx\end{equation}\end{verbatim}\end{quote}Note that you can refer to this formula asEquation~\ref{eq:myequation} with\begin{verbatim}\ref{eq:myequation}.\end{verbatim}\subsection{Fonts}%\label{sec:fonts}Generally you'll want to let \latex handle the fonts for you---Knuth's Computer Modern fonts are used by default, and include a wide range of variations that can cover most any use you can think of.If you want to get fancy (and portable; seeSection~\ref{sec:fuzzy-fonts}), you can use Type~1 PostScript fonts, such as Times, Palatino, Utopia, and so forth. These font sets are accessible with packages with names like \package{times},\package{palatino}, and \package{utopia}. There are others, aswell---a command such as \com{locate psnfss | grep sty} will find mostof them.Y ou can also get fonts from CTAN (see Section~\ref{sec:ctan}), both bitmap and Type 1. There's even support for TrueType fonts in some\TeX\ systems.\subsubsection{Font Commands}%\label{sec:font-commands}Most of your concern about fonts is probably related to what you're writing. Y ou might want some \emph{emphasized} or \textbf{bold} textto stress a point or highlight a key term. Filenames might be set in\texttt{typewriter text} (although you should consider using the\package{url} package to help you out---by default, text set intypewriter text isn't hyphenated, which can lead to some unattractiveline breaks).Y ou can also set text in \textsf{sans serif} or \textsc{small caps}.Table~\ref{tab:font-commands} shows you some of the most commonly used font commands provided by \latex.\begin{table}[htbp]\centering\begin{tabular}{ll}\topruleCommand & Result\\\midrule\com{emph} & \emph{emphasized text}\\\com{textsf} & \textsf{sans-serif text}\\\com{texttt} & \texttt{typewriter text}\\\com{textbf} & \textbf{bold text}\\\com{textsc} & \textsc{small caps text}\\\com{textsl} & \textsl{slanted text}\\\com{textit} & \textit{italic text}\\\bottomrule\end{tabular}\caption[Commonly used font commands]{Commonly used font commands.} \label{tab:font-commands}\end{table}I recommend that you use \com{emph} in preference to \com{textit}, anduse \com{textbf} sparingly. \com{emph} is a smarter command than\com{textit}---it switches back to the roman font when necessary. For example, \emph{She loved \emph{Scooby Doo}.} versus \textit{He loved\textit{Titanic}.}For complicated font changes, or for special font usages that you'retyping a lot, creating a macro (Section~\ref{sec:customization}) isthe way to go. I often just write, tossing in custom commands as Igo, and waiting to define them until just before I compile thedocument.\subsection{Customization}%\label{sec:customization}The main advantage of using commands and environments is that they allow you to organize your writing. A useful side-effect is that youcan change your mind about the way an element is typeset, and change all the appearances of that element in document by editing one pieceof code. For example, in this document the names of environments have been set in ``typewriter text'', using a command I created called\command{env}, which is defined as\begin{quote}\begin{verbatim}\newcommand{\env}[1]{\texttt{#1}\xspace}\end{verbatim}\end{quote}All I have to do to make the names of all the environments in the document appear in sans-serif type instead is to change that one lineto\begin{quote}\begin{verbatim}\newcommand{\env}[1]{\textsf{#1}\xspace}\end{verbatim}\end{quote}Y ou can do the same with almost anything you can conceptualize---key terms, people's names (especially names of people fromnon-English-speaking countries), files, functions, and so on.\section{Mathematical Notation}%\label{sec:mathematical-notation}As we saw in Section~\ref{sec:math-environments}, math is typed into one of several kinds of math environments. Choose your environment based on the context and importance of the content. Any formula you plan to refer to should be typed in an \env{equation} environment (ora similar environment that supports labels).Y ou should punctuate your mathematics as if the formulae were normal parts of English sentences. Reading them aloud is often a useful method for ensuring that you have all the commas in the right places. Where appropriate, you should also follow a displayed formula at the end of a sentence with a period.\subsection{Sums and Products}%\label{sec:sums-n-products}It's easy to typeset sums and products. For example,\begin{equation}f(n) = \sqrt[n]{\sum_{k=1}^{n} {n \choose k} f \left( n - k \right)},~\prod_{n=2}^{\infty} \frac{n^{3}-1}{n^{3}+1} = \frac{2}{3}.\end{equation}%%% The ~ in the equation puts a nonbreaking space (equivalent to an%%% interword space in text mode) between the two halves of the equation. %%%%%% Also, note that the use of the \choose command here causes the%%% amsmath package to issue the warning%%%%%% Package amsmath Warning: Foreign command \atopwithdelims; %%% (amsmath) \frac or \genfrac should be used instead %%% (amsmath) on input line 557.%%%%%% amsmath would prefer the use of the \binom command it supplies.\subsection{Matrices}%\label{sec:matrices}It's a little more difficult to create matrices, but not too bad:%%% In LaTeX, & is the alignment tab, and separates columns. \\ is the end of %%% line marker, and separates rows. The ccc denotes that there are three %%% columns. The array environment and the tabular environment are %%% more or less identical, so what goes here also applies to a table.%%%\begin{equation}\left[ \begin{array}{ccc}2 & 1 & 2\\1 & 0 & 2\\2 & 1 & 1\end{array} \right]\left[ \begin{array}{ccc}-2 & 1 & 2\\3 & -2 & -2\\1 & 0 & -1\end{array} \right] =\left[ \begin{array}{ccc}1 & 0 & 0\\0 & 1 & 0\\0 & 0 & 1\end{array} \right].\end{equation}\subsection{Symbols}%\label{sec:symbols}\LaTeX provides an enormous number of symbols. Additional packages (loaded with \com{usepackage}) may provide additional symbols and fonts.For example, $\mathbb{N}$, $\mathbb{Z}$, $\mathbb{Q}$, $\mathbb{R}$, and $\mathbb{C}$ require you to load the \package{amsfonts} package (which is automatically loaded by the \texttt{icmmcm} class). These symbols are generated by \com{mathbb}, which only works in math mode.Subscripts and superscripts are easy---\verb!$a_n$! produces $a_n$,and \verb!$x^2$! produces $x^2$. Ordinal numbers, such as$3^{\textrm{rd}}$, $n^{\textrm{th}}$, and so forth,\footnote{Somefonts may include their own ordinals that can be accessed withspecial commands.} can be produced with code like\verb!$3^{\textrm{rd}}$!, \verb!$n^{\textrm{th}}$!.Equation~\ref{eq:superscript} shows a formula with a superscript.\begin{equation}\label{eq:superscript}\int_{0}^{\pi} \, \cos^{2n+1} x \, {\rm d} x = 0 \qquad\forall \, n \in \mathbb{N}.\end{equation}Notice that \com{cos} produces a nice roman ``$\cos$'' within math mode. There are similar commands for common functions like \com{log}, \com{exp}, and so forth. More can be defined with the\com{DeclareMathOperator} command provided by the \package{amsmath} package.Y ou can stack symbols over other symbols. In math formulas,\begin{equation}m\ddot{x} + \gamma\dot{x} + kx = 0,\end{equation}or to produce diacritical accents, as in\begin{quote}Paul Erd\H{o}s s'est reveill\'{e} t\^{o}t pour enseigner lefran\c{c}ais \`{a} son fr\`{e}re et sa s\oe{}ur.\end{quote}\LaTeX{} has lots of Greek letters and ellipses too, some of which areshown in Figure~\ref{fig:greek-symbols}.\begin{figure}\begin{center}\begin{equation}\sqrt{\left[\begin{array}{cccccc}\alpha & \beta & \gamma & \delta & \epsilon & \zeta \\\eta & \theta & \iota & \kappa & \lambda & \mu \\\nu & \xi & o & \rho & \pi & \sigma \\\tau & \upsilon & \phi & \chi & \psi & \omega \\\Gamma & \Delta & \Theta & \Lambda & \Xi & \Pi \\\Sigma & \Upsilon & \Phi & \Psi & \Omega & \varphi\\\cdots & \ldots & \vdots & \ddots & : & \cdot\end{array}\right ] }.\end{equation}\end{center}\caption[Greek letters and some symbols]{Greek letters and some symbols.}% \label{fig:greek-symbols}\end{figure}See \cite{gratzer-mil}, pp.~455--474, or \cite{kopka-daly-guide},pp.~123--127, for lists of the symbols available. Intext, you mightsee some of these symbols used as\begin{quote}The Strong Induction Principle asserts that if a statement holds forthe integers $1$,~$2$,\dots,~$n$, and if whenever it holds for $n =1$, \dots,~$k$ then it also holds for $n = k+1$, then the statementholds for the integers $1$,~$2$,~$3$, $\ldots\,$ Using thisPrinciple, it can be shown that $1+2+\cdots+n = n(n+1)/2$ for allpositive integers~$n$.\end{quote}Notice that in the lists of integers, the ellipsis was made using the\com{ldots} command, and that the periods were nicely spaced betweenthe commas. In the sum, the dots were made with \com{cdots} and were centered on the line. The \package{amsmath} package provides a``smart'' \com{dots} command that can generally get things right basedon the context.So, with \com{dots} alone, the previous examples come out as\begin{quote}$1$,~$2$,~\dots,~$n$\\$n = 1$, \dots,~$k$\\$1$,~$2$,~$3$, $\dots\,$\\$1+2+\dots+n = n(n+1)/2$\end{quote}The general $n \times n$ matrix can be typeset as follows:\begin{equation}\left[\begin{array}{cccc}a_{11} & a_{12} & \ldots & a_{1n}\\a_{21} & a_{22} & \ldots & a_{2n}\\\vdots & \vdots & \ddots & \vdots\\a_{n1} & a_{n2} & \ldots & a_{nn}\\\end{array}\right].\end{equation}A fine point: lists of numbers that you're using in a mathematicalsense (as opposed to dates, numbers of objects, etc.) should be typedin math mode. For example, $341$, $541$, $561$, and $641$. The same numbers without math mode are 341, 541, 561, and 641. Depending on the fonts and packages that you're using, you may notice a little bitmore space around the first set than the second. With some packages, numbers intext may be set using old-style figures by default, as in\oldstylenums{341}, \oldstylenums{541}, \oldstylenums{561}, and\oldstylenums{641}. %%% But without the \oldstylenums commands!\subsection{More Math}In Fourier analysis, we talk about the $z$-domain.If $a$ is an even number, then\[ a + \phi(a) < \frac{3 a}{2}, \]and\[ \sigma(a) > \frac{2^{\alpha+1}-1}{2^{\alpha}} \, a \geq \frac{3a}{2}, \]where $\alpha$ is the greatest power of 2 that divides $a$, $\phi(a)$is the number of integers less than $a$ and relatively primeto $a$, and $\sigma(a)$ is the sum of the divisors of $a$ (including$1$ and $a$).。
美赛论文优秀模版

For office use onlyT1________________ T2________________ T3________________ T4________________ Team Control Number11111Problem ChosenABCDFor office use onlyF1________________F2________________F3________________F4________________ 2015Mathematical Contest in Modeling (MCM/ICM) Summary Sheet In order to evaluate the performance of a coach, we describe metrics in five aspects: historical record, game gold content, playoff performance, honors and contribution to the sports. Moreover, each aspect is subdivided into several secondary metrics. Take playoff performance as example, we collect postseason result (Sweet Sixteen, Final Four, etc.) per year from NCAA official website, Wikimedia and so on.First, ****grade.To eval*** , in turn, are John Wooden, Mike Krzyzewski, Adolph Rupp, Dean Smith and Bob Knight.Time line horizon does make a difference. According to turning points in NCAA history, we divide the previous century into six periods with different time weights which lead to the change of ranking.We conduct sensitivity analysis on FSE to find best membership function and calculation rule. Sensitivity analysis on aggregation weight is also performed. It proves AM performs better than single model. As a creative use, top 3 presidents (U.S.) are picked out: Abraham Lincoln, George Washington, Franklin D. Roosevelt.At last, the strength and weakness of our mode are discussed, non-technical explanation is presented and the future work is pointed as well.Key words: Ebola virus disease; Epidemiology; West Africa; ******ContentsI. Introduction (2)1.1 (2)1.2 (2)1.3 (2)1.4 (2)1.5 (2)1.6 (2)II. The Description of the Problem (2)2.1 How do we approximate the whole course of paying toll? (2)2.2 How do we define the optimal configuration? (2)2.3 The local optimization and the overall optimization (3)2.4 The differences in weights and sizes of vehicles (3)2.5 What if there is no data available? (3)III. Models (3)3.1 Basic Model (3)3.1.1 Terms, Definitions and Symbols (3)3.1.2 Assumptions (3)3.1.3 The Foundation of Model (4)3.1.4 Solution and Result (4)3.1.5 Analysis of the Result (4)3.1.6 Strength and Weakness (4)3.2 Improved Model (4)3.2.1 Extra Symbols (4)3.2.2 Additional Assumptions (5)3.2.3 The Foundation of Model (5)3.2.4 Solution and Result (5)3.2.5 Analysis of the Result (5)3.2.6 Strength and Weakness (6)IV. Conclusions (6)4.1 Conclusions of the problem (6)4.2 Methods used in our models (6)4.3 Applications of our models (6)V. Future Work (6)5.1 Another model (6)5.1.1 The limitations of queuing theory (6)5.1.2 (6)5.1.3 (7)5.1.4 (7)5.2 Another layout of toll plaza (7)5.3 The newly- adopted charging methods (7)VI. References (7)VII. Appendix (8)I. IntroductionIn order to indicate the origin of the toll way problems, the following background is worth mentioning.1.11.21.31.41.51.6II. The Description of the Problem2.1 How d o we approximate the whole course of paying toll?●●●●1) From the perspective of motorist:2) From the perspective of the toll plaza:3) Compromise:2.3 The l ocal optimization and the overall optimization●●●Virtually:2.4 The differences in weights and sizes of vehicl es2.5 What if there is no data availabl e?III. Models3.1 Basic Model3.1.1 Terms, Definitions and SymbolsThe signs and definitions are mostly generated from queuing theory.●●●●●3.1.2 Assumptions●●●●3.1.3 The Foundation of Model1) The utility function●The cost of toll plaza:●The loss of motorist:●The weight of each aspect:●Compromise:2) The integer programmingAccording to queuing theory, we can calculate the statistical properties as follows.3)The overall optimization and the local optimization●The overall optimization:●The local optimization:●The optimal number of tollbooths:3.1.4 Solution and Result1) The solution of the integer programming:2) Results:3.1.5 Analysis of the Result●Local optimization and overall optimization:●Sensitivity: The result is quite sensitive to the change of the three parameters●Trend:●Comparison:3.1.6 Strength and Weakness●Strength: In despite of this, the model has proved that . Moreover, we have drawnsome useful conclusions about . T he model is fit for, such as●Weakness: This model just applies to . As we have stated, .That’s just whatwe should do in the improved model.3.2 Improved Model3.2.1 Extra Symbols●●●●3.2.2 Additional Assumptions●●●Assumptions concerning the anterior process are the same as the Basic Model.3.2.3 The Foundation of Model1) How do we determine the optimal number?As we have concluded from the Basic Model,3.2.4 Solution and Result1) Simulation algorithmBased on the analysis above, we design our simulation arithmetic as follows.●Step1:●Step2:●Step3:●Step4:●Step5:●Step6:●Step7:●Step8:●Step9:2) Flow chartThe figure below is the flow chart of the simulation.3) Solution3.2.5 Analysis of the Result3.2.6 Strength and Weakness●Strength: The Improved Model aims to make up for the neglect of . The resultseems to declare that this model is more reasonable than the Basic Model and much more effective than the existing design.●Weakness: . Thus the model is still an approximate on a large scale. This hasdoomed to limit the applications of it.IV. Conclusions4.1 Conclusions of the probl em●●●4.2 Methods used in our mod els●●●4.3 Applications of our mod els●●●V. Future Work5.1 Another model5.1.1 The limitations of queuing theory5.1.25.1.41)●●●●2)●●●3)●●●4)5.2 Another layout of toll plaza5.3 The newly- ad opted charging methodsVI. References[1][2][4]VII. Appendix。
美赛数学建模比赛论文实用模板
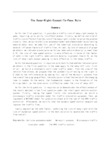
The Keep-Right-Except-To-Pass RuleSummaryAs for the first question, it provides a traffic rule of keep right except to pass, requiring us to verify its effectiveness. Firstly, we define one kind of traffic rule different from the rule of the keep right in order to solve the problem clearly; then, we build a Cellular automaton model and a Nasch model by collecting massive data; next, we make full use of the numerical simulation according to several influence factors of traffic flow; At last, by lots of analysis of graph we obtain, we indicate a conclusion as follow: when vehicle density is lower than 0.15, the rule of lane speed control is more effective in terms of the factor of safe in the light traffic; when vehicle density is greater than 0.15, so the rule of keep right except passing is more effective In the heavy traffic.As for the second question, it requires us to testify that whether the conclusion we obtain in the first question is the same apply to the keep left rule. First of all, we build a stochastic multi-lane traffic model; from the view of the vehicle flow stress, we propose that the probability of moving to the right is 0.7and to the left otherwise by making full use of the Bernoulli process from the view of the ping-pong effect, the conclusion is that the choice of the changing lane is random. On the whole, the fundamental reason is the formation of the driving habit, so the conclusion is effective under the rule of keep left.As for the third question, it requires us to demonstrate the effectiveness of the result advised in the first question under the intelligent vehicle control system. Firstly, taking the speed limits into consideration, we build a microscopic traffic simulator model for traffic simulation purposes. Then, we implement a METANET model for prediction state with the use of the MPC traffic controller. Afterwards, we certify that the dynamic speed control measure can improve the traffic flow .Lastly neglecting the safe factor, combining the rule of keep right with the rule of dynamical speed control is the best solution to accelerate the traffic flow overall.Key words:Cellular automaton model Bernoulli process Microscopic traffic simulator model The MPC traffic controlContentContent (2)1. Introduction (3)2. Analysis of the problem (3)3. Assumption (3)4. Symbol Definition (3)5. Models (4)5.1 Building of the Cellular automaton model (4)5.1.1 Verify the effectiveness of the keep right except to pass rule (4)5.1.2 Numerical simulation results and discussion (5)5.1.3 Conclusion (8)5.2 The solving of second question (8)5.2.1 The building of the stochastic multi-lane traffic model (9)5.2.2 Conclusion (9)5.3 Taking the an intelligent vehicle system into a account (9)5.3.1 Introduction of the Intelligent Vehicle Highway Systems (9)5.3.2 Control problem (9)5.3.3 Results and analysis (9)5.3.4 The comprehensive analysis of the result (10)6. Improvement of the model (11)6.1 strength and weakness (11)6.1.1 Strength (11)6.1.2 Weakness (11)6.2 Improvement of the model (11)7. Reference (13)1. IntroductionAs is known to all, it’s essential for us to drive automobiles, thus the driving rules is crucial important. In many countries like USA, China, drivers obey the rules which called “The Keep-Right-Except-To-Pass (that is, when driving automobiles, the rule requires drivers to drive in the right-most unless theyare passing another vehicle)”.2. Analysis of the problemFor the first question, we decide to use the Cellular automaton to build models,then analyze the performance of this rule in light and heavy traffic. Firstly,we mainly use the vehicle density to distinguish the light and heavy traffic; secondly, we consider the traffic flow and safe as the represent variable which denotes the light or heavy traffic; thirdly, we build and analyze a Cellular automaton model; finally, we judge the rule through two different driving rules,and then draw conclusions.3. AssumptionIn order to streamline our model we have made several key assumptions●The highway of double row three lanes that we study can representmulti-lane freeways.●The data that we refer to has certain representativeness and descriptive●Operation condition of the highway not be influenced by blizzard oraccidental factors●Ignore the driver's own abnormal factors, such as drunk driving andfatigue driving●The operation form of highway intelligent system that our analysis canreflect intelligent system●In the intelligent vehicle system, the result of the sampling data hashigh accuracy.4. Symbol Definitioni The number of vehiclest The time5. ModelsBy analyzing the problem, we decided to propose a solution with building a cellular automaton model.5.1 Building of the Cellular automaton modelThanks to its simple rules and convenience for computer simulation, cellular automaton model has been widely used in the study of traffic flow in recent years. Let )(t x i be the position of vehicle i at time t , )(t v i be the speed of vehicle i at time t , p be the random slowing down probability, and R be the proportion of trucks and buses, the distance between vehicle i and the front vehicle at time t is:1)()(1--=-t x t x gap i i i , if the front vehicle is a small vehicle.3)()(1--=-t x t x gap i i i , if the front vehicle is a truck or bus.5.1.1 Verify the effectiveness of the keep right except to pass ruleIn addition, according to the keep right except to pass rule, we define a new rule called: Control rules based on lane speed. The concrete explanation of the new rule as follow:There is no special passing lane under this rule. The speed of the first lane (the far left lane) is 120–100km/h (including 100 km/h);the speed of the second lane (the middle lane) is 100–80km8/h (including80km/h);the speed of the third lane (the far right lane) is below 80km/ h. The speeds of lanes decrease from left to right.● Lane changing rules based lane speed controlIf vehicle on the high-speed lane meets control v v <, ),1)(min()(max v t v t gap i f i +≥, safe b i gap t gap ≥)(, the vehicle will turn into the adjacent right lane, and the speed of the vehicle after lane changing remains unchanged, where control v is the minimum speed of the corresponding lane.● The application of the Nasch model evolutionLet d P be the lane changing probability (taking into account the actual situation that some drivers like driving in a certain lane, and will not takethe initiative to change lanes), )(t gap f i indicates the distance between the vehicle and the nearest front vehicle, )(t gap b i indicates the distance between the vehicle and the nearest following vehicle. In this article, we assume that the minimum safe distance gap safe of lane changing equals to the maximum speed of the following vehicle in the adjacent lanes.Lane changing rules based on keeping right except to passIn general, traffic flow going through a passing zone (Fig. 5.1.1) involves three processes: the diverging process (one traffic flow diverging into two flows), interacting process (interacting between the two flows), and merging process (the two flows merging into one) [4].Fig.5.1.1 Control plan of overtaking process(1) If vehicle on the first lane (passing lane) meets ),1)(min()(max v t v t gap i f i +≥ and safe b i gap t gap ≥)(, the vehicle will turn into the second lane, the speed of the vehicle after lane changing remains unchanged.5.1.2 Numerical simulation results and discussionIn order to facilitate the subsequent discussions, we define the space occupation rate as L N N p truck CAR ⨯⨯+=3/)3(, where CAR N indicates the number ofsmall vehicles on the driveway,truck N indicates the number of trucks and buses on the driveway, and L indicates the total length of the road. The vehicle flow volume Q is the number of vehicles passing a fixed point per unit time,T N Q T /=, where T N is the number of vehicles observed in time duration T .The average speed ∑∑⨯=T it i a v T N V 11)/1(, t i v is the speed of vehicle i at time t . Take overtaking ratio f p as the evaluation indicator of the safety of traffic flow, which is the ratio of the total number of overtaking and the number of vehicles observed. After 20,000 evolution steps, and averaging the last 2000 steps based on time, we have obtained the following experimental results. In order to eliminate the effect of randomicity, we take the systemic average of 20 samples [5].Overtaking ratio of different control rule conditionsBecause different control conditions of road will produce different overtaking ratio, so we first observe relationships among vehicle density, proportion of large vehicles and overtaking ratio under different control conditions.(a) Based on passing lane control (b) Based on speed control Fig.5.1.3Fig.5.1.3 Relationships among vehicle density, proportion of large vehicles and overtaking ratio under different control conditions.It can be seen from Fig. 5.1.3:(1) when the vehicle density is less than 0.05, the overtaking ratio will continue to rise with the increase of vehicle density; when the vehicle density is larger than 0.05, the overtaking ratio will decrease with the increase of vehicle density; when density is greater than 0.12, due to the crowding, it willbecome difficult to overtake, so the overtaking ratio is almost 0.(2) when the proportion of large vehicles is less than 0.5, the overtaking ratio will rise with the increase of large vehicles; when the proportion of large vehicles is about 0.5, the overtaking ratio will reach its peak value; when the proportion of large vehicles is larger than 0.5, the overtaking ratio will decrease with the increase of large vehicles, especially under lane-based control condition s the decline is very clear.● Concrete impact of under different control rules on overtaking ratioFig.5.1.4Fig.5.1.4 Relationships among vehicle density, proportion of large vehicles and overtaking ratio under different control conditions. (Figures in left-hand indicate the passing lane control, figures in right-hand indicate the speed control. 1f P is the overtaking ratio of small vehicles over large vehicles, 2f P is the overtaking ratio of small vehicles over small vehicles, 3f P is the overtaking ratio of large vehicles over small vehicles, 4f P is the overtaking ratio of large vehicles over large vehicles.). It can be seen from Fig. 5.1.4:(1) The overtaking ratio of small vehicles over large vehicles under passing lane control is much higher than that under speed control condition, which is because, under passing lane control condition, high-speed small vehicles have to surpass low-speed large vehicles by the passing lane, while under speed control condition, small vehicles are designed to travel on the high-speed lane, there is no low- speed vehicle in front, thus there is no need to overtake.● Impact of different control rules on vehicle speedFig. 5.1.5 Relationships among vehicle density, proportion of large vehicles and average speed under different control conditions. (Figures in left-hand indicates passing lane control, figures in right-hand indicates speed control.a X is the average speed of all the vehicles, 1a X is the average speed of all the small vehicles, 2a X is the average speed of all the buses and trucks.).It can be seen from Fig. 5.1.5:(1) The average speed will reduce with the increase of vehicle density and proportion of large vehicles.(2) When vehicle density is less than 0.15,a X ,1a X and 2a X are almost the same under both control conditions.Effect of different control conditions on traffic flowFig.5.1.6Fig. 5.1.6 Relationships among vehicle density, proportion of large vehicles and traffic flow under different control conditions. (Figure a1 indicates passing lane control, figure a2 indicates speed control, and figure b indicates the traffic flow difference between the two conditions.It can be seen from Fig. 5.1.6:(1) When vehicle density is lower than 0.15 and the proportion of large vehicles is from 0.4 to 1, the traffic flow of the two control conditions are basically the same.(2) Except that, the traffic flow under passing lane control condition is slightly larger than that of speed control condition.5.1.3 ConclusionIn this paper, we have established three-lane model of different control conditions, studied the overtaking ratio, speed and traffic flow under different control conditions, vehicle density and proportion of large vehicles.5.2 The solving of second question5.2.1 The building of the stochastic multi-lane traffic model5.2.2 ConclusionOn one hand, from the analysis of the model, in the case the stress is positive, we also consider the jam situation while making the decision. More specifically, if a driver is in a jam situation, applying ))(,2(x P B R results with a tendency of moving to the right lane for this driver. However in reality, drivers tend to find an emptier lane in a jam situation. For this reason, we apply a Bernoulli process )7.0,2(B where the probability of moving to the right is 0.7and to the left otherwise, and the conclusion is under the rule of keep left except to pass, So, the fundamental reason is the formation of the driving habit.5.3 Taking the an intelligent vehicle system into a accountFor the third question, if vehicle transportation on the same roadway was fully under the control of an intelligent system, we make some improvements for the solution proposed by us to perfect the performance of the freeway by lots of analysis.5.3.1 Introduction of the Intelligent Vehicle Highway SystemsWe will use the microscopic traffic simulator model for traffic simulation purposes. The MPC traffic controller that is implemented in the Matlab needs a traffic model to predict the states when the speed limits are applied in Fig.5.3.1. We implement a METANET model for prediction purpose[14].5.3.2 Control problemAs a constraint, the dynamic speed limits are given a maximum and minimum allowed value. The upper bound for the speed limits is 120 km/h, and the lower bound value is 40 km/h. For the calculation of the optimal control values, all speed limits are constrained to this range. When the optimal values are found, they are rounded to a multiplicity of 10 km/h, since this is more clear for human drivers, and also technically feasible without large investments.5.3.3 Results and analysisWhen the density is high, it is more difficult to control the traffic, since the mean speed might already be below the control speed. Therefore, simulations are done using densities at which the shock wave can dissolve without using control, and at densities where the shock wave remains. For each scenario, five simulations for three different cases are done, each with a duration of one hour. The results of the simulations are reported in Table 5.1, 5.2, 5.3. Table.5.1 measured results for the unenforced speed limit scenariodem q case#1 #2 #3 #4 #5 TTS:mean(std ) TPN 4700no shock 494.7452.1435.9414.8428.3445.21(6.9%) 5:4wave 3 5 8 8 0 14700nocontrolled520.42517.48536.13475.98539.58517.92(4.9%)6:364700 controlled 513.45488.43521.35479.75-486.5500.75(4.0%)6:244700 no shockwave493.9472.6492.78521.1489.43493.96(3.5%)6:034700 uncontrolled635.1584.92643.72571.85588.63604.84(5.3%)7:244700 controlled 575.3654.12589.77572.15586.46597.84(6.4%)7:19●Enforced speed limits●Intelligent speed adaptationFor the ISA scenario, the desired free-flow speed is about 100% of the speed limit. The desired free-flow speed is modeled as a Gaussian distribution, with a mean value of 100% of the speed limit, and a standard deviation of 5% of the speed limit. Based on this percentage, the influence of the dynamic speed limits is expected to be good[19].5.3.4 The comprehensive analysis of the resultFrom the analysis above, we indicate that adopting the intelligent speed control system can effectively decrease the travel times under the control of an intelligent system, in other words, the measures of dynamic speed control can improve the traffic flow.Evidently, under the intelligent speed control system, the effect of the dynamic speed control measure is better than that under the lane speed control mentioned in the first problem. Because of the application of the intelligent speed control system, it can provide the optimal speed limit in time. In addition, it can guarantee the safe condition with all kinds of detection device and the sensor under the intelligent speed system.On the whole, taking all the analysis from the first problem to the end into a account, when it is in light traffic, we can neglect the factor of safe with the help of the intelligent speed control system.Thus, under the state of the light traffic, we propose a new conclusion different from that in the first problem: the rule of keep right except to pass is more effective than that of lane speed control.And when it is in the heavy traffic, for sparing no effort to improve the operation efficiency of the freeway, we combine the dynamical speed control measure with the rule of keep right except to pass, drawing a conclusion that the application of the dynamical speed control can improve the performance ofthe freeway.What we should highlight is that we can make some different speed limit as for different section of road or different size of vehicle with the application of the Intelligent Vehicle Highway Systems.In fact, that how the freeway traffic operate is extremely complex, thereby, with the application of the Intelligent Vehicle Highway Systems, by adjusting our solution originally, we make it still effective to freeway traffic.6. Improvement of the model6.1 strength and weakness6.1.1 Strength●it is easy for computer simulating and can be modified flexibly to consideractual traffic conditions ,moreover a large number of images make the model more visual.●The result is effectively achieved all of the goals we set initially, meantimethe conclusion is more persuasive because of we used the Bernoulli equation.●We can get more accurate result as we apply Matlab.6.1.2 Weakness●The relationship between traffic flow and safety is not comprehensivelyanalysis.●Due to there are many traffic factors, we are only studied some of the factors,thus our model need further improved.6.2 Improvement of the modelWhile we compare models under two kinds of traffic rules, thereby we come to the efficiency of driving on the right to improve traffic flow in some circumstance. Due to the rules of comparing is too less, the conclusion is inadequate. In order to improve the accuracy, We further put forward a kinds of traffic rules: speed limit on different type of cars.The possibility of happening traffic accident for some vehicles is larger, and it also brings hidden safe troubles. So we need to consider separately about different or specific vehicle types from the angle of the speed limiting in order to reduce the occurrence of traffic accidents, the highway speed limit signs is in Fig.6.1.Fig .6.1Advantages of the improving model are that it is useful to improve the running condition safety of specific type of vehicle while considering the difference of different types of vehicles. However, we found that the rules may be reduce the road traffic flow through the analysis. In the implementation it should be at the 85V speed of each model as the main reference basis. In recent years, the 85V of some researchers for the typical countries from Table 6.1[ 21]: Table 6.1 Operating speed prediction modeAuthorCountry Model Ottesen andKrammes2000America LC DC L DC V C ⨯---=01.0012.057.144.10285Andueza2000Venezuel a ].[308.9486.7)/894()/2795(25.9885curve horizontal L DC Ra R V T ++--= ].[tan 819.27)/3032(69.10085gent L R V T +-= Jessen2001 America ][00239.0614.0279.080.86185LSD ADT G V V P --+=][00212.0432.010.7285NLSD ADT V V P -+=Donnell2001 America 22)2(8500724.040.10140.04.78T L G R V --+=22)3(85008369.048.10176.01.75T L G R V --+= 22)4(8500810.069.10176.05.74T L G R V --+=22)5(8500934.008.21.83T L G V --=BucchiA.BiasuzziK.And SimoneA.2005Italy DC V 124.0164.6685-= DC E V 4.046.3366.5585--= 2855.035.1119.0745.65DC E DC V ---= Fitzpatrick America KV 98.17507.11185-= Meanwhile, there are other vehicles driving rules such as speed limit in adverseweather conditions. This rule can improve the safety factor of the vehicle to some extent. At the same time, it limits the speed at the different levels.7. Reference[1] M. Rickert, K. Nagel, M. Schreckenberg, A. Latour, Two lane traffi csimulations using cellular automata, Physica A 231 (1996) 534–550.[20] J.T. Fokkema, Lakshmi Dhevi, Tamil Nadu Traffi c Management and Control inIntelligent Vehicle Highway Systems,18(2009).[21] Yang Li, New Variable Speed Control Approach for Freeway. (2011) 1-66。
美赛论文模板(超实用)

TitileSummaryDuring cell division, mitotic spindles are assembled by microtubule-based motor proteins1, 2. The bipolar organization of spindles is essential for proper segregation of chromosomes, and requires plus-end-directed homotetrameric motor proteins of the widely conserved kinesin-5 (BimC) family3. Hypotheses for bipolar spindle formation include the 'push−pull mitotic muscle' model, in which kinesin-5 and opposing motor proteins act between overlapping microtubules2, 4, 5. However, the precise roles of kinesin-5 during this process are unknown. Here we show that the vertebrate kinesin-5 Eg5 drives the sliding of microtubules depending on their relative orientation. We found in controlled in vitro assays that Eg5 has the remarkable capability of simultaneously moving at 20 nm s-1 towards the plus-ends of each of the two microtubules it crosslinks. For anti-parallel microtubules, this results in relative sliding at 40 nm s-1, comparable to spindle pole separation rates in vivo6. Furthermore, we found that Eg5 can tether microtubule plus-ends, suggesting an additional microtubule-binding mode for Eg5. Our results demonstrate how members of the kinesin-5 family are likely to function in mitosis, pushing apart interpolar microtubules as well as recruiting microtubules into bundles that are subsequently polarized by relative sliding. We anticipate our assay to be a starting point for more sophisticated in vitro models of mitotic spindles. For example, the individual and combined action of multiple mitotic motors could be tested, including minus-end-directed motors opposing Eg5 motility. Furthermore, Eg5 inhibition is a major target of anti-cancer drug development, and a well-defined and quantitative assay for motor function will be relevant for such developmentsContentTitile (1)Summary (1)1Introduction (1)1.1Restatement of the Problem (1)1.2Background (1)1.1.1Common Solving Technique (1)1.1.2Previous Works (1)1.3Example (1)2Analysis of the Problem (1)2.1Outline of the Approach (1)2.2Basic Assumptions (2)2.3Definitions and Key Terms (2)3Calculating and Simplifying the Model (2)4The Model Results (3)5Validating the Model (3)6Strengths and Weaknesses (3)6.1Strengths (3)6.2Weaknesses (3)7Food for Thought (3)8Conclusion (3)References (4)Appendices (4)Appendix A Source Code (4)Appendix B (4)1Introduction1.1Restatement of the Problem …1.2Background…1.1.1Common Solving Technique…1.1.2Previous Works…1.3Example…2Analysis of the Problem …2.1Outline of the Approach…2.2Basic Assumptions●●●●●2.3Definitions and Key Terms●●●●Table 1.…Symbol Meaning Unit3Calculating and Simplifying the Model …4The Model Results……5Validating the Model…6Strengths and Weaknesses6.1S trengths●●●●6.2W eaknesses●●●●7Food for Thought…8Conclusion….References…AppendicesAppendix A Source CodeHere are the simulation programmes we used in our model as follow. Input matlab source:……….Appendix B…….Input C++ source:…………..…………..。
美赛数学建模比赛论文资料材料模板
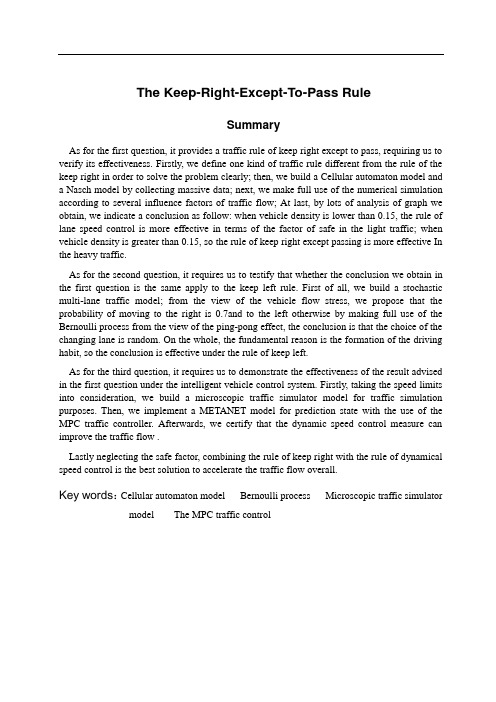
The Keep-Right-Except-To-Pass RuleSummaryAs for the first question, it provides a traffic rule of keep right except to pass, requiring us to verify its effectiveness. Firstly, we define one kind of traffic rule different from the rule of the keep right in order to solve the problem clearly; then, we build a Cellular automaton model and a Nasch model by collecting massive data; next, we make full use of the numerical simulation according to several influence factors of traffic flow; At last, by lots of analysis of graph we obtain, we indicate a conclusion as follow: when vehicle density is lower than 0.15, the rule of lane speed control is more effective in terms of the factor of safe in the light traffic; when vehicle density is greater than 0.15, so the rule of keep right except passing is more effective In the heavy traffic.As for the second question, it requires us to testify that whether the conclusion we obtain in the first question is the same apply to the keep left rule. First of all, we build a stochastic multi-lane traffic model; from the view of the vehicle flow stress, we propose that the probability of moving to the right is 0.7and to the left otherwise by making full use of the Bernoulli process from the view of the ping-pong effect, the conclusion is that the choice of the changing lane is random. On the whole, the fundamental reason is the formation of the driving habit, so the conclusion is effective under the rule of keep left.As for the third question, it requires us to demonstrate the effectiveness of the result advised in the first question under the intelligent vehicle control system. Firstly, taking the speed limits into consideration, we build a microscopic traffic simulator model for traffic simulation purposes. Then, we implement a METANET model for prediction state with the use of the MPC traffic controller. Afterwards, we certify that the dynamic speed control measure can improve the traffic flow .Lastly neglecting the safe factor, combining the rule of keep right with the rule of dynamical speed control is the best solution to accelerate the traffic flow overall.Key words:Cellular automaton model Bernoulli process Microscopic traffic simulator model The MPC traffic controlContentContent (2)1. Introduction (3)2. Analysis of the problem (3)3. Assumption (3)4. Symbol Definition (3)5. Models (3)5.1 Building of the Cellular automaton model (3)5.1.1 Verify the effectiveness of the keep right except to pass rule (4)5.1.2 Numerical simulation results and discussion (5)5.1.3 Conclusion (8)5.2 The solving of second question (8)5.2.1 The building of the stochastic multi-lane traffic model (8)5.2.2 Conclusion (8)5.3 Taking the an intelligent vehicle system into a account (8)5.3.1 Introduction of the Intelligent Vehicle Highway Systems (9)5.3.2 Control problem (9)5.3.3 Results and analysis (9)5.3.4 The comprehensive analysis of the result (9)6. Improvement of the model (10)6.1 strength and weakness (10)6.1.1 Strength (10)6.1.2 Weakness (10)6.2 Improvement of the model (10)7. Reference (12)1. IntroductionAs is known to all, it ’s essential for us to drive automobiles, thus the driving rules is crucial important. In many countries like USA, China, drivers obey the rules which called “The Keep-Right-Except-To-Pass (that is, when driving automobiles, the rule requires drivers to drive in the right-most unless they are passing another vehicle)”.2. Analysis of the problemFor the first question, we decide to use the Cellular automaton to build models, then analyze the performance of this rule in light and heavy traffic. Firstly, we mainly use the vehicle density to distinguish the light and heavy traffic; secondly, we consider the traffic flow and safe as the represent variable which denotes the light or heavy traffic; thirdly, we build and analyze a Cellular automaton model; finally, we judge the rule through two different driving rules, and then draw conclusions.3. AssumptionIn order to streamline our model we have made several key assumptions● The highway of double row three lanes that we study can representmulti-lane freeways.● The data that we refer to has certain representativeness and descriptive● Operation condition of the highway not be influenced by blizzard or accidental factors ● Ignore the driver's own abnormal factors, such as drunk driving and fatigue driving ● The operation form of highway intelligent system that our analysis can reflectintelligent system● In the intelligent vehicle system, the result of the sampling data has high accuracy.4. Symbol Definitioni The number of vehiclest The time5. ModelsBy analyzing the problem, we decided to propose a solution with building a cellular automaton model.5.1 Building of the Cellular automaton modelThanks to its simple rules and convenience for computer simulation, cellular automaton model has been widely used in the study of traffic flow in recent years.Let )(t x i be the position of vehicle i at time t , )(t v i be the speed of vehicle i at time t ,p be the random slowing down probability, and R be the proportion of trucks and buses, the distance between vehicle i and the front vehicle at time t is:1)()(1--=-t x t x gap i i i , if the front vehicle is a small vehicle.3)()(1--=-t x t x gap i i i , if the front vehicle is a truck or bus.5.1.1 Verify the effectiveness of the keep right except to pass ruleIn addition, according to the keep right except to pass rule, we define a new rule called: Control rules based on lane speed. The concrete explanation of the new rule as follow:There is no special passing lane under this rule. The speed of the first lane (the far left lane) is 120–100km/h (including 100 km/h);the speed of the second lane (the middle lane) is 100–80km8/h (including80km/h);the speed of the third lane (the far right lane) is below 80km/ h. The speeds of lanes decrease from left to right.● Lane changing rules based lane speed controlIf vehicle on the high-speed lane meets control v v <, ),1)(min()(max v t v t gap i f i +≥, safe b i gap t gap ≥)(, the vehicle will turn into the adjacent right lane, and the speed of the vehicle after lane changing remains unchanged, where control v is the minimum speed of the corresponding lane.● The application of the Nasch model evolutionLet d P be the lane changing probability (taking into account the actual situation that some drivers like driving in a certain lane, and will not take the initiative to change lanes), )(t gap f i indicates the distance between the vehicle and the nearest front vehicle, )(t gap b i indicates the distance between the vehicle and the nearest following vehicle. In this article, we assume that the minimum safe distance gap safe of lane changing equals to the maximum speed of the following vehicle in the adjacent lanes.● Lane changing rules based on keeping right except to passIn general, traffic flow going through a passing zone (Fig. 5.1.1) involves three processes: the diverging process (one traffic flow diverging into two flows), interacting process (interacting between the two flows), and merging process (the two flows merging into one)[4].Fig.5.1.1 Control plan of overtaking process(1) If vehicle on the first lane (passing lane) meets ),1)(min()(max v t v t gap i f i +≥ and safe b i gap t gap ≥)(, the vehicle will turn into the second lane, the speed of the vehicle after lane changing remains unchanged.5.1.2 Numerical simulation results and discussionIn order to facilitate the subsequent discussions, we define the space occupation rate as L N N p truck CAR ⨯⨯+=3/)3(, where CAR N indicates the number of small vehicles on the driveway,truck N indicates the number of trucks and buses on the driveway, and L indicates the total length of the road. The vehicle flow volume Q is the number of vehicles passing a fixed point per unit time,T N Q T /=, where T N is the number of vehicles observed in time duration T .The average speed ∑∑⨯=T it i a v T N V 11)/1(, t i v is the speed of vehicle i at time t . Take overtaking ratio f p as the evaluation indicator of the safety of traffic flow, which is the ratio of the total number of overtaking and the number of vehicles observed. After 20,000 evolution steps, and averaging the last 2000 steps based on time, we have obtained the following experimental results. In order to eliminate the effect of randomicity, we take the systemic average of 20 samples [5].Overtaking ratio of different control rule conditionsBecause different control conditions of road will produce different overtaking ratio, so we first observe relationships among vehicle density, proportion of large vehicles and overtaking ratio under different control conditions.(a) Based on passing lane control (b) Based on speed controlFig.5.1.3Fig.5.1.3Relationships among vehicle density, proportion of large vehicles and overtaking ratio under different control conditions.It can be seen from Fig. 5.1.3:(1) when the vehicle density is less than 0.05, the overtaking ratio will continue to rise with the increase of vehicle density; when the vehicle density is larger than 0.05, the overtaking ratio will decrease with the increase of vehicle density; when density is greater than 0.12, due to the crowding, it will become difficult to overtake, so the overtaking ratio is almost 0.(2) when the proportion of large vehicles is less than 0.5, the overtaking ratio will rise with the increase of large vehicles; when the proportion of large vehicles is about 0.5, the overtaking ratio will reach its peak value; when the proportion of large vehicles is larger than 0.5, the overtaking ratio will decrease with the increase of large vehicles, especially under lane-based control condition s the decline is very clear.Concrete impact of under different control rules on overtaking ratioFig.5.1.4Fig.5.1.4 Relationships among vehicle density, proportion of large vehicles and overtaking ratio under different control conditions. (Figures in left-hand indicate the passing lane control, figures in right-hand indicate thespeed control. 1f P is the overtaking ratio of small vehicles over large vehicles, 2f P is the overtaking ratio ofsmall vehicles over small vehicles, 3f P is the overtaking ratio of large vehicles over small vehicles, 4f P is the overtaking ratio of large vehicles over large vehicles.).It can be seen from Fig. 5.1.4:(1) The overtaking ratio of small vehicles over large vehicles under passing lane control is much higher than that under speed control condition, which is because, under passing lane control condition, high-speed small vehicles have to surpass low-speed large vehicles by the passing lane, while under speed control condition, small vehicles are designed to travel on the high-speed lane, there is no low- speed vehicle in front, thus there is no need to overtake. ● Impact of different control rules on vehicle speedFig. 5.1.5 Relationships among vehicle density, proportion of large vehicles and average speed under different control conditions. (Figures in left-hand indicates passing lane control, figures in right-hand indicates speed control. a X is the average speed of all the vehicles, 1a X is the average speed of all the small vehicles, 2a X is the average speed of all the buses and trucks.).It can be seen from Fig. 5.1.5:(1) The average speed will reduce with the increase of vehicle density and proportion of large vehicles.(2) When vehicle density is less than 0.15,a X ,1a X and 2a X are almost the same under both control conditions.● Effect of different control conditions on traffic flowFig.5.1.6Fig. 5.1.6Relationships among vehicle density, proportion of large vehicles and traffic flow under different control conditions. (Figure a1 indicates passing lane control, figure a2 indicates speed control, and figure b indicates the traffic flow difference between the two conditions.It can be seen from Fig. 5.1.6:(1) When vehicle density is lower than 0.15 and the proportion of large vehicles is from 0.4 to 1, the traffic flow of the two control conditions are basically the same.(2) Except that, the traffic flow under passing lane control condition is slightly larger than that of speed control condition.5.1.3 ConclusionIn this paper, we have established three-lane model of different control conditions, studied the overtaking ratio, speed and traffic flow under different control conditions, vehicle density and proportion of large vehicles.5.2 The solving of second question5.2.1 The building of the stochastic multi-lane traffic model5.2.2 ConclusionOn one hand, from the analysis of the model, in the case the stress is positive, we also consider the jam situation while making the decision. More specifically, if a driver is in a jam BP(situation, applying ))results with a tendency of moving to the right lane for this,2(xRdriver. However in reality, drivers tend to find an emptier lane in a jam situation. For this reason, we apply a Bernoulli process )7.0,2(B where the probability of moving to the right is 0.7and to the left otherwise, and the conclusion is under the rule of keep left except to pass, So, the fundamental reason is the formation of the driving habit.5.3 Taking the an intelligent vehicle system into a accountFor the third question, if vehicle transportation on the same roadway was fully under the control of an intelligent system, we make some improvements for the solution proposed by usto perfect the performance of the freeway by lots of analysis.5.3.1 Introduction of the Intelligent Vehicle Highway SystemsWe will use the microscopic traffic simulator model for traffic simulation purposes. The MPC traffic controller that is implemented in the Matlab needs a traffic model to predict the states when the speed limits are applied in Fig.5.3.1. We implement a METANET model for prediction purpose[14].5.3.2 Control problemAs a constraint, the dynamic speed limits are given a maximum and minimum allowed value. The upper bound for the speed limits is 120 km/h, and the lower bound value is 40 km/h. For the calculation of the optimal control values, all speed limits are constrained to this range. When the optimal values are found, they are rounded to a multiplicity of 10 km/h, since this is more clear for human drivers, and also technically feasible without large investments.5.3.3 Results and analysisWhen the density is high, it is more difficult to control the traffic, since the mean speed might already be below the control speed. Therefore, simulations are done using densities at which the shock wave can dissolve without using control, and at densities where the shock wave remains. For each scenario, five simulations for three different cases are done, each with a duration of one hour. The results of the simulations are reported in Table5.1, 5.2, 5.3.●Enforced speed limits●Intelligent speed adaptationFor the ISA scenario, the desired free-flow speed is about 100% of the speed limit. The desired free-flow speed is modeled as a Gaussian distribution, with a mean value of 100% of the speed limit, and a standard deviation of 5% of the speed limit. Based on this percentage, the influence of the dynamic speed limits is expected to be good[19].5.3.4 The comprehensive analysis of the resultFrom the analysis above, we indicate that adopting the intelligent speed control system can effectively decrease the travel times under the control of an intelligent system, in other words, the measures of dynamic speed control can improve the traffic flow.Evidently, under the intelligent speed control system, the effect of the dynamic speed control measure is better than that under the lane speed control mentioned in the first problem. Becauseof the application of the intelligent speed control system, it can provide the optimal speed limit in time. In addition, it can guarantee the safe condition with all kinds of detection device and the sensor under the intelligent speed system.On the whole, taking all the analysis from the first problem to the end into a account, when it is in light traffic, we can neglect the factor of safe with the help of the intelligent speed control system.Thus, under the state of the light traffic, we propose a new conclusion different from that in the first problem: the rule of keep right except to pass is more effective than that of lane speed control.And when it is in the heavy traffic, for sparing no effort to improve the operation efficiency of the freeway, we combine the dynamical speed control measure with the rule of keep right except to pass, drawing a conclusion that the application of the dynamical speed control can improve the performance of the freeway.What we should highlight is that we can make some different speed limit as for different section of road or different size of vehicle with the application of the Intelligent Vehicle Highway Systems.In fact, that how the freeway traffic operate is extremely complex, thereby, with the application of the Intelligent Vehicle Highway Systems, by adjusting our solution originally, we make it still effective to freeway traffic.6. Improvement of the model6.1 strength and weakness6.1.1 Strength●it is easy for computer simulating and can be modified flexibly to consider actual trafficconditions ,moreover a large number of images make the model more visual.●The result is effectively achieved all of the goals we set initially, meantime the conclusion ismore persuasive because of we used the Bernoulli equation.●We can get more accurate result as we apply Matlab.6.1.2 Weakness●The relationship between traffic flow and safety is not comprehensively analysis.●Due to there are many traffic factors, we are only studied some of the factors, thus ourmodel need further improved.6.2 Improvement of the modelWhile we compare models under two kinds of traffic rules, thereby we come to the efficiency of driving on the right to improve traffic flow in some circumstance. Due to the rules of comparing is too less, the conclusion is inadequate. In order to improve the accuracy, Wefurther put forward a kinds of traffic rules: speed limit on different type of cars.The possibility of happening traffic accident for some vehicles is larger, and it also brings hidden safe troubles. So we need to consider separately about different or specific vehicle types from the angle of the speed limiting in order to reduce the occurrence of traffic accidents, the highway speed limit signs is in Fig.6.1.Fig.6.1Advantages of the improving model are that it is useful to improve the running condition safety of specific type of vehicle while considering the difference of different types of vehicles. However, we found that the rules may be reduce the road traffic flow through the analysis. In the implementation it should be at the85V speed of each model as the main reference basis. Inrecent years, the85V of some researchers for the typical countries from Table 6.1[ 21]:Author Country ModelOttesen andKrammes2000America LCDCLDCVC⨯---=01.0012.057.144.10285Andueza2000 Venezuela].[308.9486.7)/894()/2795(25.9885curvehorizontalLDCRaRVT++--=].[tan819.27)/3032(69.10085gentLRVT+-=Jessen2001 America][00239.0614.0279.080.86185LSDADTGVVP--+=][00212.0432.010.7285NLSDADTVVP-+=Donnell2001 America22)2(8500724.040.10140.04.78TLGRV--+=22)3(85008369.048.10176.01.75TLGRV--+=22)4(8500810.069.10176.05.74TLGRV--+=22)5(8500934.008.21.83TLGV--=BucchiA.BiasuzziK.And SimoneA.2005 ItalyDCV124.0164.6685-=DCEV4.046.3366.5585--=Meanwhile, there are other vehicles driving rules such as speed limit in adverse weather conditions. This rule can improve the safety factor of the vehicle to some extent. At the same time, it limits the speed at the different levels.7. Reference[1] M. Rickert, K. Nagel, M. Schreckenberg, A. Latour, Two lane traffic simulations usingcellular automata, Physica A 231 (1996) 534–550.[20] J.T. Fokkema, Lakshmi Dhevi, Tamil Nadu Traffic Management and Control inIntelligent Vehicle Highway Systems,18(2009).[21] Yang Li, New Variable Speed Control Approach for Freeway. (2011) 1-66。
美赛论文模板
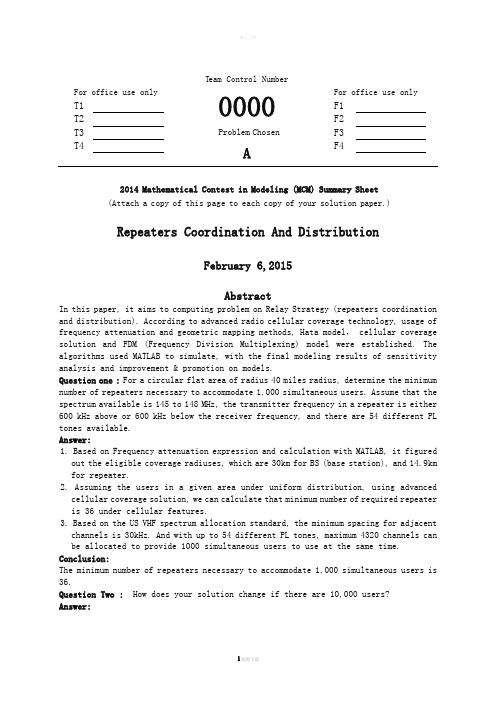
T eam Control NumberFor office use only0000For office use onlyT1 F1T2 F2T3 Problem Chosen F3T4 A F42014 Mathematical Contest in Modeling (MCM) Summary Sheet(Attach a copy of this page to each copy of your solution paper.)Repeaters Coordination And DistributionFebruary 6,2015AbstractIn this paper, it aims to computing problem on Relay Strategy (repeaters coordination and distribution). According to advanced radio cellular coverage technology, usage of frequency attenuation and geometric mapping methods, Hata model, cellular coverage solution and FDM (Frequency Division Multiplexing) model were established. The algorithms used MATLAB to simulate, with the final modeling results of sensitivity analysis and improvement & promotion on models.Question one : For a circular flat area of radius 40 miles radius, determine the minimum number of repeaters necessary to accommodate 1,000 simultaneous users. Assume that the spectrum available is 145 to 148 MHz, the transmitter frequency in a repeater is either 600 kHz above or 600 kHz below the receiver frequency, and there are 54 different PL tones available.Answer:1. Based on Frequency attenuation expression and calculation with MATLAB, it figuredout the eligible coverage radiuses, which are 30km for BS (base station), and 14.9km for repeater.2. Assuming the users in a given area under uniform distribution, using advancedcellular coverage solution, we can calculate that minimum number of required repeater is 36 under cellular features.3. Based on the US VHF spectrum allocation standard, the minimum spacing for adjacentchannels is 30kHz. And with up to 54 different PL tones, maximum 4320 channels can be allocated to provide 1000 simultaneous users to use at the same time. Conclusion:The minimum number of repeaters necessary to accommodate 1,000 simultaneous users is 36.Question Two : How does your solution change if there are 10,000 users?Answer:1. Since the given spectrum is in a fixed range, even if 54 different PL tones can not be allocated enough channels for 10,000 simultaneous users. So the number of repeaters will be increased, meanwhile, the given area will be divided into different parts.2. On the assumption that uniform distribution of the population in the given area, it will be divided into 3 sub-regions equally by analyzing the binding domain, frequency spectrum and PL tones three independent factors. And then the number of repeaters within each sub-region will be classified discussion.3. The FDM (Frequency Division Multiplexing) model is established here to improve channel efficiency to accommodate up to 10,000 simultaneous users Conclusion:The minimum number of repeaters necessary to accommodate 10,000 simultaneous users is 126.Question Three : Discuss the case where there might be defects in line-of-sight propagation caused by mountainous areas. Answer:Basically, under the same condition for question 1&2, the mountainous area will be analyzed as following:1. The function for relationship between radio attenuation x caused by obstacles and the eligible coverage radius d for repeater is 2249.354371.4110x d -=, which is to analyze the impact on the number of repeaters under full signal coverage. 2. For the mountain barrier, based on the different situation of mountains, the addition of repeaters on the suitable location will be discussed to achieve full coverage. This paper describes model established by using of cellular coverage technology and frequency attenuation expression, to achieve simple, fast, accurate algorithm. And also illustrated the effect takes the entire article. In the end, the sensitivity analysis and error calculation are applied for modeling, making the model practically.Key words: Cellular Coverage technology, frequency attenuation expression, channel allocation, MatlabRepeaters coordination and distributionContent1 Restatement of the Problem (1)1.1 Introduction (1)1.2 The Problem (1)2 Simplifying Assumption (1)3 Phrase explain (1)4 Model (2)4.1 Model I (2)4.1.1 Analysis of the Problem (2)4.1.2 Model Design (2)5 Sensitivity analysis (2)6 Model extension (2)7 Evaluating our model (2)7.1 The strengths of model (2)7.2 The weaknesses of model (2)References (3)1 Restatement of the Problem1.1 IntroductionThe VHF radio spectrum involves line-of-sight transmission and reception. This limitation can be overcome by “repeaters,” which pick up weak signals, amplify them, and retransmit them on a different frequency. Thus, using a repeater, low-power users (such as mobile stations) can communicate with one another in situations where direct user-to-user contact would not be possible. However, repeaters can interfere with one another unless they are far enough apart or transmit on sufficiently separated frequencies.1.2 The ProblemYour job is to:◆Design a scheme that determines the minimum number of repeaters necessaryto accommodate 1,000 simultaneous users in a circular flat area of radius40 miles radius.And assume that the spectrum available is 145 to 148 MHz,the transmitter frequency in a repeater is either 600 kHz above or 600 kHz below the receiver frequency, and there are 54 different PL tones available.◆Change your scheme to accommodate 1,0000 simultaneous users base on yourmodel.◆Discuss the case where there might be defects in line-of-sight propagationcaused by mountainous areas.2 Simplifying Assumption3 Phrase explain4 Model4.1 Model I4.1.1 Analysis of the Problem4.1.2 Model Design5 Sensitivity analysisSymbol◆N: the number of total repeaters in the circle area ◆Q: the number of the users in the circle area◆k: the number of the red circle in figure 2最前面最好有一个Symbol List6 Model extension7 Evaluating our model7.1 The strengths of model7.2 The weaknesses of modelReferences参考文献不要引用非常差的期刊的论文,要引用比较厉害的英文期刊,证明你有足够的阅读文献量。
美赛(mcm)论文LaTeX模板
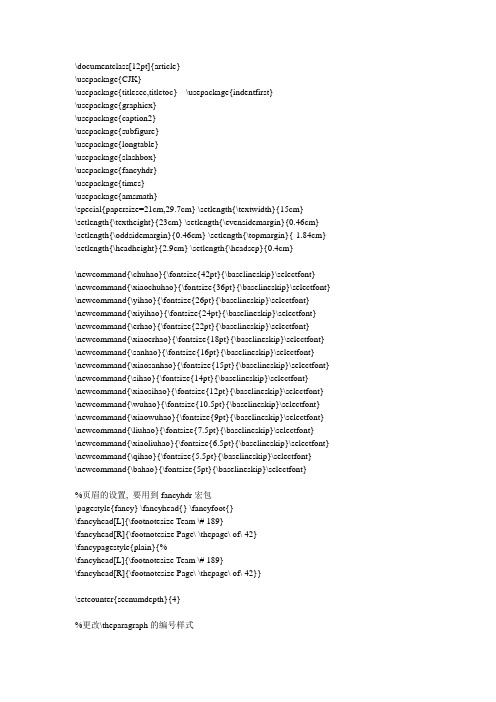
\documentclass[12pt]{article}\usepackage{CJK}\usepackage{titlesec,titletoc} \usepackage{indentfirst}\usepackage{graphicx}\usepackage{caption2}\usepackage{subfigure}\usepackage{longtable}\usepackage{slashbox}\usepackage{fancyhdr}\usepackage{times}\usepackage{amsmath}\special{papersize=21cm,29.7cm} \setlength{\textwidth}{15cm}\setlength{\textheight}{23cm} \setlength{\evensidemargin}{0.46cm}\setlength{\oddsidemargin}{0.46cm} \setlength{\topmargin}{-1.84cm} \setlength{\headheight}{2.9cm} \setlength{\headsep}{0.4cm}\newcommand{\chuhao}{\fontsize{42pt}{\baselineskip}\selectfont}\newcommand{\xiaochuhao}{\fontsize{36pt}{\baselineskip}\selectfont} \newcommand{\yihao}{\fontsize{26pt}{\baselineskip}\selectfont}\newcommand{\xiyihao}{\fontsize{24pt}{\baselineskip}\selectfont}\newcommand{\erhao}{\fontsize{22pt}{\baselineskip}\selectfont}\newcommand{\xiaoerhao}{\fontsize{18pt}{\baselineskip}\selectfont} \newcommand{\sanhao}{\fontsize{16pt}{\baselineskip}\selectfont}\newcommand{\xiaosanhao}{\fontsize{15pt}{\baselineskip}\selectfont} \newcommand{\sihao}{\fontsize{14pt}{\baselineskip}\selectfont}\newcommand{\xiaosihao}{\fontsize{12pt}{\baselineskip}\selectfont} \newcommand{\wuhao}{\fontsize{10.5pt}{\baselineskip}\selectfont} \newcommand{\xiaowuhao}{\fontsize{9pt}{\baselineskip}\selectfont} \newcommand{\liuhao}{\fontsize{7.5pt}{\baselineskip}\selectfont}\newcommand{\xiaoliuhao}{\fontsize{6.5pt}{\baselineskip}\selectfont} \newcommand{\qihao}{\fontsize{5.5pt}{\baselineskip}\selectfont}\newcommand{\bahao}{\fontsize{5pt}{\baselineskip}\selectfont}%页眉的设置, 要用到fancyhdr宏包\pagestyle{fancy} \fancyhead{} \fancyfoot{}\fancyhead[L]{\footnotesize Team \# 189}\fancyhead[R]{\footnotesize Page\ \thepage\ of\ 42}\fancypagestyle{plain}{%\fancyhead[L]{\footnotesize Team \# 189}\fancyhead[R]{\footnotesize Page\ \thepage\ of\ 42}}\setcounter{secnumdepth}{4}%更改\theparagraph的编号样式\makeatletter\renewcommand{\theparagraph}{\@arabic\c@paragraph}\makeatother%章节格式的设置\titleformat{\section}{\erhao\bf}{}{0em}{}[]\titleformat{\subsection}{\xiaoerhao\bf}{}{0em}{}[]\titleformat{\subsubsection}{\sanhao\bf}{}{0em}{}[]\titleformat{\paragraph}[hang]{\vspace*{0.5ex}\sihao\bf}{\hspace*{1em}\theparagraph)}{0.5em }{}[\vspace*{-0.5ex}]%更改插图的标题\renewcommand{\figurename}{\wuhao\bf\sf Figure}\renewcommand{\captionlabeldelim}{\ }%更改表格的标题\renewcommand{\tablename}{\wuhao\bf\sf Table}%更改图形或表格与其标题的间距\setlength{\abovecaptionskip}{10pt}\setlength{\belowcaptionskip}{10pt}%定义产生不浮动图形和表格的标题的命令\figcaption和\tabcaption\makeatletter\newcommand\figcaption{\def\@captype{figure}\caption}\newcommand\tabcaption{\def\@captype{table}\caption}\makeatother%自定义的可以调整粗细的水平线命令, 用于绘制表格, 调用格式\myhline{0.5mm}.\makeatletter\def\myhline#1{%\noalign{\ifnum0=`}\fi\hrule \@height #1 \futurelet\reserved@a\@xhline}\makeatother%第一层列表序号为带圈的阿拉伯数字\renewcommand{\labelenumi}{\textcircled{\arabic{enumi}}}%更改脚注设置\renewcommand{\thefootnote}{\fnsymbol{footnote}}\begin{document}\begin{CJK*}{GBK}{song}\CJKtilde\title{\bf\yihao Aviation Baggage Screening\\{\&} Flight Schedule}\author{}\date{}\maketitle\section{Introduction}Following the terrorist attacks on September 11, 2001, there isintense interest in improving the security screening process forairline passengers and their baggage. Airlines and airports areconsidered high-threat targets for terrorism, so aviation securityis crucial to the safety of the air-travelling public. Bombs andexplosives have been known to be introduced to aircraft by holdbaggage and cargo, carried on by passengers, and hidden withinaircraft supplies.At present To Screen or Not to Screen, that is a Hobson's choice.US Current laws mandate 100{\%} screening of all checked bags at the 429passenger airports throughout the nation by explosive detection systems(EDS) by the end of the Dec 31 2003. However, because the manufacturers arenot able to produce the expected number of EDS required to meet the federalmandate, so it is significant to determine the correct number of devicesdeploy at each airport, and to take advantage of them effectively.The Transportation Security Administration (TSA) needs a complicatedanalysis on how to allocate limited device and how to best use them.Our paper contains the mathematical models to determine the number of EDSsand flight schedules for all airports in Midwest Region. We also discuss theETD devices as the additional security measures and the future developmentof the security systems.\section{Assumption and Hypothesis}\begin{itemize}\item The passengers who will get on the same airplane will arrive uniformly, namely the distribution is flat.\item The detection systems, both EDS and ETD, operate all the time during peak hour, except downtime.\item The airline checks the passengers randomly, according to its claim.\item The passengers, who are just landing and leave out, do not have to be checked through EDS or ETD.\item According to the literature, the aircraft loads approximately equal among the sets of departing flight during the peak hour.\item The landing flight did not affect the departure of the plane.\item Once a passenger arrives, he can go to EDS to be checked, except he has to wait in line.\item Once passengers finish screening, they can broad on the plane in no time.\item During peak hours, a set of flights departs at the same time every the same minutes.\item All the runways are used as much as possible during peak hours.\item The maximum number of the baggage is two, which a passenger can carry on plane. []\item The detection machine examines the bags at the same speed.\item EDS cannot make mistakes that it detect a normal object as an explosive.\end{itemize}\section{Variable and Definition}\begin{longtable}{p{100pt}p{280pt}}\caption{Variables}\\ %第一页表头的标题\endfirsthead %第一页的标题结束\caption{(continued)}\\ %第二页的标题\endhead %第二页的标题结束\hline\hline\textbf{Symbol}&\textbf{Description}\\\hline$n_{ij}$&The airplane number of the $i^{\mathrm{th}}$ type in the $j^{\mathrm{th}}$ flight set\\\hline${NP}_i\:(i=1,2,\ldots)$&The number of passengers on each airplanes of the same type.\\\hline$\xi_{ij}\:(i,j = 1,2,\cdots)$&The number of baggage on each airplane of the $j^{\mathrm{th}}$ flights\\\hline$a$&The maximal number of airplanes type\\\hline$B_j^{set}$&The total baggage number of each set of flight\\\hline${NF}_i$&Number of airplanes of each type\\\hline$\bar{\rho}$&The mean value of passengers' baggage coming per minute in every flight set\\ \hline$N_{set}$&The number of flight sets\\\hline$B_{total}$&The total number of checked baggage during the peak hour\\\hline$H_{peak}$&The length of the peak hour\\\hline$T_{set}$&The time length during which each flight set's passengers wait to be checked\\\hline$\Delta t$&The time interval between two consecutive flight set\\\hline$N_{EDS}$&The number of all the EDSs\\\hline$N_{shadow}$&The number of flight sets whose passengers will be mixed up before being checked\\\hline$v_{EDS}$&The number of baggage checking by one EDS per minute\\\hline$\rho_j$&The number of passengers' baggage coming per minute in one flight set\\\hline$N_{runway}$&The number of an airport's runway\\\hline\\*[-2.2ex]${\bar{B}}^{set}$&The mean value of checked baggage number of every flight set\\\hline$M$&The security cost\\\hline\hline\label{tab1}\end{longtable}\subsubsection{Definition:}\begin{description}\item[Flight set] A group of flights take off at the same time\item[The length of peak hour] The time between the first set of flight and the last set\end{description}\section{Basic Model}During a peak hour, many planes and many passengers would departfrom airports. Therefore, It is difficult to arrange for thepassengers to enter airports. If there are not enough EDSs forpassengers' baggage to check, it will take too long time for themto enter. That would result in the delay of airplanes. On thecontrary, if there are too many EDSs, it will be a waste. It isour task to find a suitable number of EDSs for airport. In orderto reach this objective, we use the linear programming method tosolve it.\subsection{Base analysis}The airplanes are occupied at least partly. The passengers'baggage would be checked by EDSs before they get on the airplanes.We have assumed that every passenger carry two baggages. Thisassumption would simplify the problem. According to the data fromthe problem sheet, we can obtain the useful information thatairlines claim 20{\%} of the passengers do not check any luggage,20{\%} check one bag, and the remaining passengers check two bags.Therefore, we can gain the total number of passengers' baggagethat should be carried on one plane: $\xi_{ij}$. Moreover, we canget the equation that calculate $\xi_{ij}$:\[\xi_{ij}={NP}_i\times 20\%+{NP}_i\times 60\%\times 2\]We define the matrix below as airplane baggage number matrix:\[\overset{\rightharpoonup}{\xi}_j=\left[\xi_{1j}\quad\xi_{2j}\quad\cdots\quad\xi_{ij}\quad\cdots\ right]\]We define the matrix below as flight schedule matrix:\[\left[\begin{array}{llcl}n_{11}&n_{12}&\cdots&n_{1N_{set}}\\n_{21}&n_{22}&\cdots&n_{2N_{set}}\\\multicolumn{4}{c}\dotfill\\n_{a1}&n_{a2}&\cdots&n_{aN_{set}}\end{array}\right]\]In this matrix, $n_{ij}$ is the airplane number of the$i^{\mathrm{th}}$ type in the $j^{\mathrm{th}}$ flight set whichwill take off. Apparently, this value is an integer.We define the matrix below as flight set baggage number matrix:\[\left[B_1^{set}\quad B_2^{set}\quad\cdots\quad B_j^{set}\quad\cdots\quad B_a^{set}\right] \]It is clear that they meet the relation below:\begin{equation}\begin{array}{cl}&\left[\xi_{1j}\quad\xi_{2j}\quad\cdots\quad\xi_{ij}\quad\cdots\right]\cdot\left[\begin{array}{llcl}n_{11}&n_{12}&\cdots&n_{1N_{set}}\\n_{21}&n_{22}&\cdots&n_{2N_{set}}\\\multicolumn{4}{c}\dotfill\\n_{a1}&n_{a2}&\cdots&n_{aN_{set}}\end{array}\right]\\=&\left[B_1^{set}\quad B_2^{set}\quad\cdots\quad B_j^{set}\quad\cdots\quadB_a^{set}\right]\end{array}\label{Flight:baggage}\end{equation}Then, we know:\[B_j^{set}=\sum\limits_{i=1}^a\xi_{ij}\times n_{ij}\]There are some constraints to the equation (\ref{Flight:baggage}).First, for each set of flight, the total number of airplanesshould be less than the number of runways. Second, the totalairplane number of the same type listed in the equation(\ref{Flight:baggage}) from every set of flight should be equal tothe actual airplane number of the same type during the peak hour.We can express them like these:\[\sum\limits_{i=1}^a n_{ij}\le N_{runway}\quad\quad\sum\limits_{j=1}^b n_{ij}={NF}_i \]We should resolve the number of flight sets. According to our assumptions,during the peak hour, the airlines should make the best use of the runways.Then get the number of flight sets approximately based on the number of allthe airplanes during the peak hour and that of the runways. We use anequation below to express this relation:\begin{equation}N_{set}=\left\lceil\frac{\sum\limits_{j=1}^{N_{set}}\sum\limits_{i=1}^an_{ij}}{N_{runway}}\right\rceil\label{sets:number}\end{equation}The checked baggage numbers of each flight set are equal to eachother according to our assumption. We make it based on literature.It can also simplify our model. We define $\bar{B}^{set}$ as themean value of checked baggage number of every flight set.Moreover, We define $\bar{\rho}$ as the mean value of checkedbaggage number of every flight set per minute:\[\bar{B}^{set}=\frac{B_{total}}{N_{set}}\]\[\bar{\rho}=\frac{\bar{B}^{set}}{T_{set}}=\frac{B_{total}}{T_{set}N_{set}}=\frac{B_{total}\Delta t}{T_{set}H_{peak}}\]The course of passengers' arrival and entering airport isimportant for us to decide the number of EDSs and to make the flights schedule. Therefore, we should analyze this process carefully. Passengers will arrive between forty-five minutes andtwo hours prior to the departure time, and the passengers who will get on the same airplane will arrive uniformly. Then we can getthe flow density of all checked baggage at any time during passengers' entering. This value is the sum of numbers of passengers' checked baggage coming per minute. To calculate this value, firstly, we should obtain flow density of each flight set's checked baggage. We define $\rho_j $, namely the number of checked baggage per minute of one flight set:\[\rho_j=\frac{B_j^{set}}{T_{set}}\]Secondly, we draw graphic to help us to understand. We use rectangle to express the time length for all the passengers of one flight set to come and check bags. In the graphic, the black partis the period for them to come. During the white part, no passengers for this flight set come. According to the problem sheet, the former is 75 minute, and the latter is 45 minute. The length of rectangle is 120 minute. $T_{set}$ is the period during which all passengers of one flight set wait to be checked. Sincewe have assumed that each time interval between two consecutive flight set is same value, we define $\Delta t$ as it. Observe the section that value we want to solve is $\sum\limits_j\rho_j$. Moreover, we can get another important equation from the graphic below:\begin{equation}N_{set}=\frac{H_{peak}}{\Delta t}\label{PeakHour}\end{equation}\begin{figure}[hbtp]\centering\includegraphics[width=298.2pt,totalheight=141.6pt]{fig01.eps} \caption{}\label{fig1}\end{figure}Each EDS has certain capacity. If the number of EDSs is $N_{EDS}$and one EDS can check certain number of baggage per minute (Thatis checking velocity, marked by $v_{EDS}$), the total checkingcapacity is $N_{EDS}\cdot\frac{v_{EDS}}{60}$. $v_{EDS}$ is between160 and 210.Now we can easily decide in what condition the passengers can be checkedwithout delay:\[\sum\limits_j\rho_j\le v_{EDS}\]The passengers have to queue before being checked:$\sum\limits_j\rho_j>v_{EDS}$Well then, how can we decide how many $\rho_j$? It depends on howmany flight sets whose passengers will be mixed up before beingchecked. We note it as $N_{shadow} $. Return to the Figure\ref{fig1}, we can know:\[N_{shadow}=\left\lfloor\frac{T_{set}}{\Delta t}\right\rfloor\]\begin{figure}%[htbp]\centering\includegraphics[width=240pt,totalheight=131.4pt]{fig02.eps}\caption{}\label{fig2}\end{figure}From Figure \ref{fig1} and Figure \ref{fig2}, we can get theresult as follows:\begin{enumerate}\item If $N_{shadow}\le N_{set}$, namely $H_{peak}>T_{set}$, then $\sum\limits_{j=1}^{N_{shadow}}\rho _j\le N_{EDS}\frac{v_{EDS}}{60}$\renewcommand{\theequation}{\arabic{equation}a}That is:\begin{equation}N_{EDS}\ge\frac{60}{v_{EDS}}\sum\limits_{j=1}^{N_{shadow}}\rho_j\approx\frac{60}{v_{ EDS}}N_{shadow}\bar{\rho}=\frac{60B_{total}\Deltat}{v_{EDS}T_{set}H_{peak}}N_{shadow}\label{EDS:number:a}\end{equation}\item If $N_{shadow}>N_{set}$, namely $H_{peak}\le T_{set}$, then $\sum\limits_{j=1}^{N_{set}}\rho_j\le N_{EDS}\frac{v_{EDS}}{60}$\setcounter{equation}{3}\renewcommand{\theequation}{\arabic{equation}b}That is:\begin{equation}N_{EDS}\ge\frac{60}{v_{EDS}}\sum\limits_{j=1}^{N_{set}}\rho_j\approx\frac{60}{v_{EDS} }N_{set}\bar{\rho}=\frac{60B_{total}\Delta t}{v_{EDS}T_{set}H_{peak}}N_{set}\label{EDS:number:b}\end{equation}\end{enumerate}\subsection{The number of EDSs}Then we begin to resolve the number of EDSs assisted by the linearprogramming method.EDS is operational about 92{\%} of the time. That is to say, whenever it isduring a peak hour, there are some EDSs stopping working. Then the workingefficiency of all the EDSs is less than the level we have expected.Therefore, the airline has to add more EDSs to do the work, which can bedone with less EDSs without downtime.We use binomial distribution to solve this problem. $N$ is the number ofactual EDSs with downtime and $k$ is the number of EDSs without downtime. Ifprobability is $P$, we can get the equation below:\[\left(\begin{array}{c}N\\k\end{array}\right)\cdot98\%^k\cdot(1-98\%)^{N-k}=P\]We can obtain $N$ when we give $P$ a certain value. In this paper,$P$ is 95{\%}. The $N_{EDS}$ is the actual number we obtainthrough the equation above.Now we have assumed that passengers can be checked unless be delayed by thepeople before him once he arrives at airport. Apparently, if the time lengthbetween two sets of flight is short, the density of passengers will begreat. It will bring great stress to security check and may even make somepassengers miss their flight. To resolve this question, the airline has toinstall more EDSs to meet the demand. However, this measure will cost muchmore money. Consequently, we have to set a suitable time interval betweentwo set of flight.Based on the base analysis above. We can use the equation(\ref{sets:number}) to decide the number of flight sets $N_{set}$assuming we know the number of runways of a certain airport. Thenbased on the equation (\ref{PeakHour}), we can decide the peakhour length $H_{peak}$ when we assume a time interval between two consecutive flight sets. Then we use \textcircled{1} and\textcircled{2} to decide which to choose between equation(\ref{EDS:number:a}) and equation (\ref{EDS:number:b}). In consequence, we can obtain the minimum of EDSs number.If we choose different numbers of runways and the time intervalsbetween two flight sets, we can get different EDSs numbers. Inthis paper that followed, we gain a table of some value of$N_{runway}$ and $\Delta t$ with the corresponding EDSs numbers. Moreover, we draw some figure to reflect their relation.For a certain airport, its number of runway is known. Givencertain time interval ($\Delta t$), we can get the length of thepeak hour ($H_{peak}$). When the $N_{runway}$ is few enough,perhaps $H_{peak}$ is too long to be adopted. However, for acertain airline, they can decide the time interval of their ownpeak hour. In this given time interval, they could find theminimum of $N_{runway}$ through the Figure \ref{fig3}. We draw a sketch map to describe our steps.\begin{figure}[hbtp]\centering\includegraphics[width=352.8pt,totalheight=214.2pt]{fig03.eps}\caption{}\label{fig3}\end{figure}\subsection{The Flight Schedule }According to the base analysis, we can know that the flightschedule matrix and $\Delta t$ is one form of flight timetable. In``The number of EDSs'', we can get suitable $\Delta t$. Then weshould resolve the flight schedule matrix.Because we have assumed that the checked baggage numbers of each flight set are equal to each other. It can be described as follows:\[\left\{\begin{array}{l}\rho_j\approx\bar{\rho}\\B_j^{set}\approx\bar{B}^{set}\end{array}\right.\begin{array}{*{20}c}\hfill&{j=1,2,\cdots,N_{set}}\hfill\end{array}\]The flight schedule matrix subject to this group:\[\left\{\begin{array}{ll}\sum\limits_{j=1}^{N_{set}}n_{ij}={NF}_i&i=1,2,\cdots\\\sum\limits_{i=1}^a n_{ij}\le N_{runway}&j=1,2,\cdots,N_{set}\\n_{ij}\ge0,&\mathrm{and}\:n_{ij}\:\mathrm{is}\:\mathrm{a}\:\mathrm{Integer}\end{array}\right.\]In order to make the best use of runway, we should make$\sum\limits_{i=1}^a n_{ij}$ as great as we can unless it exceed$N_{runway}$.Then we can see that how to resolve the flight schedule matrix is a problemof divide among a group of integers. This group is all the numbers of eachflight passengers' baggage in one flight set. We program for this problemusing MA TLAB and we get at least one solution in the end. However, thematrix elements we have obtained are not integer, we have to adjust them tobe integers manually.\subsection{Results and Interpretation for Airport A and B}The number of passengers in a certain flight (${NP}_i$), the timelength of security checking ($T_{set}$), the checking velocity ofEDS ($v_{EDS}$), and the number of baggage carried by onepassenger are random.\subsubsection{Data Assumption:}\begin{itemize}\item $T_{set}$ is 110 minutes, which is reasonable for airline.\item To simplify the problem, we assume that every passenger carry 2 baggage. If some of thepassengers carry one baggage, the solution based on 2 baggages per passenger meets therequirement.\item The number of runways in airport A and airport B is 5.\end{itemize}\subsubsection{Airport A:}Once the number of runway and the number of the flights aredecided, the flight schedule matrix is decided, too. We producethis matrix using MATLAB. This matrix companied by $\Delta t$ isthe flight schedule for airport A. $\Delta t$ will be calculatedin (\ref{Flight:baggage}), (\ref{sets:number}) and(\ref{PeakHour}).We calculate $N_{EDS}$ and make the flight timetable in threeconditions. The three conditions and the solution are listed asfollowed:\paragraph{Every flight are fully occupied}The checking speed of EDS is 160 bags/hour.\begin{table}[htbp]\centering\caption{}\begin{tabular}{*{11}c}\myhline{0.4mm}$\mathbf{\Deltat(\min)}$&\textbf{2}&\textbf{4}&\textbf{6}&\textbf{8}&\textbf{10}&\textbf{12}&\textbf{14} &\textbf{16}&\textbf{18}&\textbf{20}\\\myhline{0.4mm}$N_{EDS}(\ge)$&31&31&31&31&31&29&24&22&20&17\\\hline$H_{peak}(\min)$&20&40&60&80&100&120&140&160&180&200\\\myhline{0.4mm}\end{tabular}\label{tab2}\end{table}We assume that the suitable value of $H_{peak}$ is 120 minutes.Then the suitable value of $\Delta t$ is about 12 minutes, and$N_{EDS}$ is 29 judged from Figure \ref{fig4}. Certainly, we canwork $\Delta t$ and $N_{EDS}$ out through equation.\begin{figure}[htbp]\centering\includegraphics[width=294.6pt,totalheight=253.2pt]{fig04.eps}\caption{}\label{fig4}\end{figure}\paragraph{Every flight is occupied by the minimal number of passengers onstatistics in the long run.}The checking speed of EDS is 210 bags/hour.\begin{table}[htbp]\centering\caption{}\begin{tabular}{*{11}c}\myhline{0.4mm}$\mathbf{\Deltat(\min)}$&\textbf{2}&\textbf{4}&\textbf{6}&\textbf{8}&\textbf{10}&\textbf{12}&\textbf{14} &\textbf{16}&\textbf{18}&\textbf{20}\\\myhline{0.4mm}$N_{EDS}(\ge)$&15&15&15&15&15&14&13&12&10&7\\\hline$H_{peak}(\min)$&20&40&60&80&100&120&140&160&180&200\\\myhline{0.4mm}\end{tabular}\label{tab3}\end{table}We assume that the suitable value of $H_{peak}$ is 120 minutes.Then the suitable value of $\Delta t$ is about 12 minutes, and$N_{EDS}$ is 14 judging from Figure \ref{fig5}. Certainly, we canwork $\Delta t$ and $N_{EDS}$ out through equation.\begin{figure}[htbp]\centering\includegraphics[width=294.6pt,totalheight=253.2pt]{fig05.eps}\caption{}\label{fig5}\end{figure}\paragraph{${NP}_i$ and $v_{EDS}$ are random value produced by MATLAB.}\begin{table}[htbp]\centering\caption{}\begin{tabular}{*{11}c}\myhline{0.4mm}$\mathbf{\Deltat(\min)}$&\textbf{2}&\textbf{4}&\textbf{6}&\textbf{8}&\textbf{10}&\textbf{12}&\textbf{14} &\textbf{16}&\textbf{18}&\textbf{20}\\\myhline{0.4mm}$N_{EDS}(\ge)$&15&22&21&21&15&17&21&16&13&14\\\hline$H_{peak}(\min)$&20&40&60&80&100&120&140&160&180&200\\\myhline{0.4mm}\end{tabular}\label{tab4}\end{table}We assume that the suitable value of $H_{peak}$ is 120 minutes.Then the suitable value of $\Delta t$ is about 12 minutes, and$N_{EDS}$ is 17 judging from Figure \ref{fig6}. Certainly, we canwork $\Delta t$ and $N_{EDS}$ out through equation.\begin{figure}[htbp]\centering\includegraphics[width=294.6pt,totalheight=249.6pt]{fig06.eps}\caption{}\label{fig6}\end{figure}\subsubsection{Interpretation:}By analyzing the results above, we can conclude that when$N_{EDS}$ is 29, and $\Delta t$ is 12, the flight schedule willmeet requirement at any time. The flight schedule is:\\[\intextsep]\begin{minipage}{\textwidth}\centering\tabcaption{}\begin{tabular}{c|*{8}c|c|c}\myhline{0.4mm}\backslashbox{\textbf{Set}}{\textbf{Type}}&\textbf{1}&\textbf{2}&\textbf{3}&\textbf{4}&\te xtbf{5}&\textbf{6}&\textbf{7}&\textbf{8}&\textbf{Numbers of Bags}&\textbf{Numbers of Flights}\\\myhline{0.4mm}1&2&0&0&0&2&1&0&0&766&5\\\hline2&2&0&2&0&2&0&0&0&732&4\\\hline3&0&1&1&1&2&0&0&0&762&4\\\hline4&0&1&0&0&2&1&0&0&735&4\\\hline5&0&1&0&0&2&1&0&0&735&5\\\hline6&2&0&0&0&1&0&0&1&785&5\\\hline7&2&0&0&0&2&0&1&0&795&5\\\hline8&0&1&0&0&2&1&0&0&735&4\\\hline9&2&0&0&0&2&1&0&0&766&5\\\hline10&0&0&0&2&2&0&0&0&758&5\\\hlineTotal&10&4&3&3&19&5&1&1&7569&46\\\myhline{0.4mm}\end{tabular}\label{tab5}\end{minipage}\\[\intextsep]We have produced random value for ${NP}_i$ and $v_{EDS}$. On thiscondition, the number of EDSs is 17, which is less than 29 that wedecide for the airport A. That is to say our solution can meet thereal requirement.\subsubsection{Airport B:}\paragraph{The passenger load is 100{\%}}The checking speed of EDS is 160 bags/hour.\begin{table}[htbp]\centering\caption{}\begin{tabular}{*{11}c}\myhline{0.4mm}$\mathbf{\Deltat(\min)}$&\textbf{2}&\textbf{4}&\textbf{6}&\textbf{8}&\textbf{10}&\textbf{12}&\textbf{14} &\textbf{16}&\textbf{18}&\textbf{20}\\\myhline{0.4mm}$N_{EDS}(\ge)$&33&33&33&33&33&30&27&23&21&19\\\hline$H_{peak}(\min)$&20&40&60&80&100&120&140&160&180&200\\\myhline{0.4mm}\end{tabular}\label{tab6}\end{table}We assume that the suitable value of $H_{peak}$ is 120 minutes.Then the suitable value of $\Delta t$ is about 12 minutes, and。
美赛论某文LaTeX实用模板

%% 本论文的排版主要参考了LaTeX2e插图指南(王磊), LaTeX2e用户手册, media的中文学位%% 论文宏包(CDT), happaytex的ORmain1.tex等文件以及ChinaTeX, CTeX论坛上的诸多贴子. %%% 本论文采用了Miktex2.2的方式在ChinaTeX.iso系统下得到了实现,其编译方式为%% latex(得到DVI文件)+dvips(得到PS文件)+ps2pdf(可得PDF文件).%%\documentclass[12pt]{article}%需要的一些宏包\usepackage{CJK} % 中文输入环境宏包\usepackage{titlesec,titletoc} % 配合命令在后面, 章节标题设置\usepackage{indentfirst} % 使首段首行缩进\usepackage{graphicx} % 插图宏包\usepackage{caption2} % 可以更改插图, 表格的标题样式\usepackage{subfigure} % 产生并列的子图或子表, 命令\subfigure, \subtable\usepackage{longtable} % 如果表格太长, 超过了一页时, 就可以试试longtable 宏包所定义的longtable 环境\usepackage{slashbox} % 在表格中绘制斜线\usepackage{fancyhdr} % 更改页眉的宏包, 并可在页眉插入图片\usepackage{times} % Times Roman + Helvetica + Courier\usepackage{amsmath} % 数学符号宏包AMS-LaTeX, 如下面的\overset需要此宏包%页面的设置\special{papersize=21cm,29.7cm} \setlength{\textwidth}{15cm}\setlength{\textheight}{23cm} \setlength{\evensidemargin}{0.46cm}\setlength{\oddsidemargin}{0.46cm} \setlength{\topmargin}{-1.84cm}\setlength{\headheight}{2.9cm} \setlength{\headsep}{0.4cm}%字号设置\newcommand{\chuhao}{\fontsize{42pt}{\baselineskip}\selectfont}\newcommand{\xiaochuhao}{\fontsize{36pt}{\baselineskip}\selectfont}\newcommand{\yihao}{\fontsize{26pt}{\baselineskip}\selectfont}\newcommand{\xiyihao}{\fontsize{24pt}{\baselineskip}\selectfont}\newcommand{\erhao}{\fontsize{22pt}{\baselineskip}\selectfont}\newcommand{\xiaoerhao}{\fontsize{18pt}{\baselineskip}\selectfont}\newcommand{\sanhao}{\fontsize{16pt}{\baselineskip}\selectfont}\newcommand{\xiaosanhao}{\fontsize{15pt}{\baselineskip}\selectfont}\newcommand{\sihao}{\fontsize{14pt}{\baselineskip}\selectfont}\newcommand{\xiaosihao}{\fontsize{12pt}{\baselineskip}\selectfont}\newcommand{\wuhao}{\fontsize{10.5pt}{\baselineskip}\selectfont} \newcommand{\xiaowuhao}{\fontsize{9pt}{\baselineskip}\selectfont} \newcommand{\liuhao}{\fontsize{7.5pt}{\baselineskip}\selectfont}\newcommand{\xiaoliuhao}{\fontsize{6.5pt}{\baselineskip}\selectfont} \newcommand{\qihao}{\fontsize{5.5pt}{\baselineskip}\selectfont}\newcommand{\bahao}{\fontsize{5pt}{\baselineskip}\selectfont}%页眉的设置, 要用到fancyhdr宏包\pagestyle{fancy} \fancyhead{} \fancyfoot{}\fancyhead[L]{\footnotesize Team \# 189}\fancyhead[R]{\footnotesize Page\ \thepage\ of\ 42}\fancypagestyle{plain}{%\fancyhead[L]{\footnotesize Team \# 189}\fancyhead[R]{\footnotesize Page\ \thepage\ of\ 42}}\setcounter{secnumdepth}{4}%更改\theparagraph的编号样式\makeatletter\renewcommand{\theparagraph}{\@arabic\c@paragraph}\makeatother%章节格式的设置\titleformat{\section}{\erhao\bf}{}{0em}{}[]\titleformat{\subsection}{\xiaoerhao\bf}{}{0em}{}[]\titleformat{\subsubsection}{\sanhao\bf}{}{0em}{}[]\titleformat{\paragraph}[hang]{\vspace*{0.5ex}\sihao\bf}{\hspace*{1em}\theparagr aph)}{0.5em}{}[\vspace*{-0.5ex}]%更改插图的标题\renewcommand{\figurename}{\wuhao\bf\sf Figure}\renewcommand{\captionlabeldelim}{\ }%更改表格的标题\renewcommand{\tablename}{\wuhao\bf\sf Table}%更改图形或表格与其标题的间距\setlength{\abovecaptionskip}{10pt}\setlength{\belowcaptionskip}{10pt}%定义产生不浮动图形和表格的标题的命令\figcaption和\tabcaption\makeatletter\newcommand\figcaption{\def\@captype{figure}\caption}\newcommand\tabcaption{\def\@captype{table}\caption}\makeatother%自定义的可以调整粗细的水平线命令, 用于绘制表格, 调用格式\myhline{0.5mm}. \makeatletter\def\myhline#1{%\noalign{\ifnum0=`}\fi\hrule \@height #1 \futurelet\reserved@a\@xhline}\makeatother%第一层列表序号为带圈的阿拉伯数字\renewcommand{\labelenumi}{\textcircled{\arabic{enumi}}}%更改脚注设置\renewcommand{\thefootnote}{\fnsymbol{footnote}}\begin{document}\begin{CJK*}{GBK}{song}\CJKtilde\title{\bf\yihao Aviation Baggage Screening\\{\&} Flight Schedule}\author{}\date{}\maketitle\section{Introduction}Following the terrorist attacks on September 11, 2001, there isintense interest in improving the security screening process forairline passengers and their baggage. Airlines and airports areconsidered high-threat targets for terrorism, so aviation securityis crucial to the safety of the air-travelling public. Bombs andexplosives have been known to be introduced to aircraft by holdbaggage and cargo, carried on by passengers, and hidden withinaircraft supplies.At present To Screen or Not to Screen, that is a Hobson's choice.US Current laws mandate 100{\%} screening of all checked bags at the 429 passenger airports throughout the nation by explosive detection systems (EDS) by the end of the Dec 31 2003. However, because the manufacturers are not able to produce the expected number of EDS required to meet the federal mandate, so it is significant to determine the correct number of devices deploy at each airport, and to take advantage of them effectively.The Transportation Security Administration (TSA) needs a complicated analysis on how to allocate limited device and how to best use them.Our paper contains the mathematical models to determine the number of EDSs and flight schedules for all airports in Midwest Region. We also discuss theETD devices as the additional security measures and the future developmentof the security systems.\section{Assumption and Hypothesis}\begin{itemize}\item The passengers who will get on the same airplane will arrive uniformly, namely the distribution is flat.\item The detection systems, both EDS and ETD, operate all the time during peak hour, except downtime.\item The airline checks the passengers randomly, according to its claim.\item The passengers, who are just landing and leave out, do not have to be checked through EDS or ETD.\item According to the literature, the aircraft loads approximately equal among the sets of departing flight during the peak hour.\item The landing flight did not affect the departure of the plane.\item Once a passenger arrives, he can go to EDS to be checked, except he has to wait in line.\item Once passengers finish screening, they can broad on the plane in no time.\item During peak hours, a set of flights departs at the same time every the same minutes.\item All the runways are used as much as possible during peak hours.\item The maximum number of the baggage is two, which a passenger can carry on plane. []\item The detection machine examines the bags at the same speed.\item EDS cannot make mistakes that it detect a normal object as an explosive. \end{itemize}\section{Variable and Definition}\begin{longtable}{p{100pt}p{280pt}}\caption{Variables}\\ %第一页表头的标题\endfirsthead %第一页的标题结束\caption{(continued)}\\ %第二页的标题\endhead %第二页的标题结束\hline\hline\textbf{Symbol}&\textbf{Description}\\\hline$n_{ij}$&The airplane number of the $i^{\mathrm{th}}$ type in the $j^{\mathrm{th}}$ flight set\\\hline${NP}_i\:(i=1,2,\ldots)$&The number of passengers on each airplanes of the same\hline$\xi_{ij}\:(i,j = 1,2,\cdots)$&The number of baggage on each airplane of the $j^{\mathrm{th}}$ flights\\\hline$a$&The maximal number of airplanes type\\\hline$B_j^{set}$&The total baggage number of each set of flight\\\hline${NF}_i$&Number of airplanes of each type\\\hline$\bar{\rho}$&The mean value of passengers' baggage coming per minute in every flight set\\\hline$N_{set}$&The number of flight sets\\\hline$B_{total}$&The total number of checked baggage during the peak hour\\\hline$H_{peak}$&The length of the peak hour\\\hline$T_{set}$&The time length during which each flight set's passengers wait to be checked\\$\Delta t$&The time interval between two consecutive flight set\\\hline$N_{EDS}$&The number of all the EDSs\\\hline$N_{shadow}$&The number of flight sets whose passengers will be mixed up before being checked\\\hline$v_{EDS}$&The number of baggage checking by one EDS per minute\\\hline$\rho_j$&The number of passengers' baggage coming per minute in one flight set\\\hline$N_{runway}$&The number of an airport's runway\\\hline\\*[-2.2ex]${\bar{B}}^{set}$&The mean value of checked baggage number of every flight set\\\hline$M$&The security cost\\\hline\hline\label{tab1}\end{longtable}\subsubsection{Definition:}\begin{description}\item[Flight set] A group of flights take off at the same time\item[The length of peak hour] The time between the first set of flight and the last set\end{description}\section{Basic Model}During a peak hour, many planes and many passengers would departfrom airports. Therefore, It is difficult to arrange for thepassengers to enter airports. If there are not enough EDSs forpassengers' baggage to check, it will take too long time for themto enter. That would result in the delay of airplanes. On thecontrary, if there are too many EDSs, it will be a waste. It isour task to find a suitable number of EDSs for airport. In orderto reach this objective, we use the linear programming method tosolve it.\subsection{Base analysis}The airplanes are occupied at least partly. The passengers'baggage would be checked by EDSs before they get on the airplanes.We have assumed that every passenger carry two baggages. Thisassumption would simplify the problem. According to the data fromthe problem sheet, we can obtain the useful information thatairlines claim 20{\%} of the passengers do not check any luggage,20{\%} check one bag, and the remaining passengers check two bags. Therefore, we can gain the total number of passengers' baggagethat should be carried on one plane: $\xi_{ij}$. Moreover, we canget the equation that calculate $\xi_{ij}$:\[\xi_{ij}={NP}_i\times 20\%+{NP}_i\times 60\%\times 2\]We define the matrix below as airplane baggage number matrix:\[\overset{\rightharpoonup}{\xi}_j=\left[\xi_{1j}\quad\xi_{2j}\quad\cdots\quad\xi_{ij}\ quad\cdots\right]\]We define the matrix below as flight schedule matrix:\[\left[\begin{array}{llcl}n_{21}&n_{22}&\cdots&n_{2N_{set}}\\\multicolumn{4}{c}\dotfill\\n_{a1}&n_{a2}&\cdots&n_{aN_{set}}\end{array}\right]\]In this matrix, $n_{ij}$ is the airplane number of the$i^{\mathrm{th}}$ type in the $j^{\mathrm{th}}$ flight set whichwill take off. Apparently, this value is an integer.We define the matrix below as flight set baggage number matrix:\[\left[B_1^{set}\quad B_2^{set}\quad\cdots\quad B_j^{set}\quad\cdots\quad B_a^{set}\right]\]It is clear that they meet the relation below:\begin{equation}\begin{array}{cl}&\left[\xi_{1j}\quad\xi_{2j}\quad\cdots\quad\xi_{ij}\quad\cdots\right]\cdot\left[\begin{array}{llcl}n_{21}&n_{22}&\cdots&n_{2N_{set}}\\\multicolumn{4}{c}\dotfill\\n_{a1}&n_{a2}&\cdots&n_{aN_{set}}\end{array}\right]\\=&\left[B_1^{set}\quad B_2^{set}\quad\cdots\quad B_j^{set}\quad\cdots\quad B_a^{set}\right]\end{array}\label{Flight:baggage}\end{equation}Then, we know:\[B_j^{set}=\sum\limits_{i=1}^a\xi_{ij}\times n_{ij}\]There are some constraints to the equation (\ref{Flight:baggage}).First, for each set of flight, the total number of airplanesshould be less than the number of runways. Second, the totalairplane number of the same type listed in the equation(\ref{Flight:baggage}) from every set of flight should be equal tothe actual airplane number of the same type during the peak hour.We can express them like these:\[\sum\limits_{i=1}^a n_{ij}\le N_{runway}\quad\quad\sum\limits_{j=1}^b n_{ij}={NF}_i\]We should resolve the number of flight sets. According to our assumptions, during the peak hour, the airlines should make the best use of the runways.Then get the number of flight sets approximately based on the number of allthe airplanes during the peak hour and that of the runways. We use anequation below to express this relation:\begin{equation}N_{set}=\left\lceil\frac{\sum\limits_{j=1}^{N_{set}}\sum\limits_{i=1}^an_{ij}}{N_{runway}}\right\rceil\label{sets:number}\end{equation}The checked baggage numbers of each flight set are equal to eachother according to our assumption. We make it based on literature.It can also simplify our model. We define $\bar{B}^{set}$ as themean value of checked baggage number of every flight set.Moreover, We define $\bar{\rho}$ as the mean value of checkedbaggage number of every flight set per minute:\[\bar{B}^{set}=\frac{B_{total}}{N_{set}}\]\[\bar{\rho}=\frac{\bar{B}^{set}}{T_{set}}=\frac{B_{total}}{T_{set}N_{set}}=\frac{B_{tota l}\Delta t}{T_{set}H_{peak}}\]The course of passengers' arrival and entering airport isimportant for us to decide the number of EDSs and to make theflights schedule. Therefore, we should analyze this processcarefully. Passengers will arrive between forty-five minutes andtwo hours prior to the departure time, and the passengers who willget on the same airplane will arrive uniformly. Then we can getthe flow density of all checked baggage at any time duringpassengers' entering. This value is the sum of numbers ofpassengers' checked baggage coming per minute. T o calculate thisvalue, firstly, we should obtain flow density of each flight set'schecked baggage. We define $\rho_j $, namely the number of checked baggage per minute of one flight set:\[\rho_j=\frac{B_j^{set}}{T_{set}}\]Secondly, we draw graphic to help us to understand. We use rectangle to express the time length for all the passengers of one flight set to come and check bags. In the graphic, the black partis the period for them to come. During the white part, no passengers for this flight set come. According to the problem sheet, the former is 75 minute, and the latter is 45 minute. The length of rectangle is 120 minute. $T_{set}$ is the period during which all passengers of one flight set wait to be checked. Sincewe have assumed that each time interval between two consecutive flight set is same value, we define $\Delta t$ as it. Observe the section that value we want to solve is $\sum\limits_j\rho_j$. Moreover, we can get another important equation from the graphic below:\begin{equation}N_{set}=\frac{H_{peak}}{\Delta t}\label{PeakHour}\end{equation}\begin{figure}[hbtp]\centering\includegraphics[width=298.2pt,totalheight=141.6pt]{fig01.eps}\caption{}\label{fig1}\end{figure}Each EDS has certain capacity. If the number of EDSs is $N_{EDS}$and one EDS can check certain number of baggage per minute (Thatis checking velocity, marked by $v_{EDS}$), the total checkingcapacity is $N_{EDS}\cdot\frac{v_{EDS}}{60}$. $v_{EDS}$ is between160 and 210.Now we can easily decide in what condition the passengers can be checked without delay:\[\sum\limits_j\rho_j\le v_{EDS}\]The passengers have to queue before being checked:$\sum\limits_j\rho_j>v_{EDS}$Well then, how can we decide how many $\rho_j$? It depends on howmany flight sets whose passengers will be mixed up before beingchecked. We note it as $N_{shadow} $. Return to the Figure\ref{fig1}, we can know:\[N_{shadow}=\left\lfloor\frac{T_{set}}{\Delta t}\right\rfloor\]\begin{figure}%[htbp]\centering\includegraphics[width=240pt,totalheight=131.4pt]{fig02.eps}\caption{}\label{fig2}\end{figure}From Figure \ref{fig1} and Figure \ref{fig2}, we can get theresult as follows:\begin{enumerate}\item If $N_{shadow}\le N_{set}$, namely $H_{peak}>T_{set}$, then $\sum\limits_{j=1}^{N_{shadow}}\rho _j\le N_{EDS}\frac{v_{EDS}}{60}$\renewcommand{\theequation}{\arabic{equation}a}That is:\begin{equation}N_{EDS}\ge\frac{60}{v_{EDS}}\sum\limits_{j=1}^{N_{shadow}}\rho_j\approx\frac{60} {v_{EDS}}N_{shadow}\bar{\rho}=\frac{60B_{total}\Deltat}{v_{EDS}T_{set}H_{peak}}N_{shadow}\label{EDS:number:a}\end{equation}\item If $N_{shadow}>N_{set}$, namely $H_{peak}\le T_{set}$, then $\sum\limits_{j=1}^{N_{set}}\rho_j\le N_{EDS}\frac{v_{EDS}}{60}$\setcounter{equation}{3}\renewcommand{\theequation}{\arabic{equation}b} That is:\begin{equation}N_{EDS}\ge\frac{60}{v_{EDS}}\sum\limits_{j=1}^{N_{set}}\rho_j\approx\frac{60}{v_{E DS}}N_{set}\bar{\rho}=\frac{60B_{total}\Delta t}{v_{EDS}T_{set}H_{peak}}N_{set} \label{EDS:number:b}\end{equation}\end{enumerate}\subsection{The number of EDSs}Then we begin to resolve the number of EDSs assisted by the linearprogramming method.EDS is operational about 92{\%} of the time. That is to say, whenever it is during a peak hour, there are some EDSs stopping working. Then the working efficiency of all the EDSs is less than the level we have expected.Therefore, the airline has to add more EDSs to do the work, which can bedone with less EDSs without downtime.We use binomial distribution to solve this problem. $N$ is the number of actual EDSs with downtime and $k$ is the number of EDSs without downtime. If probability is $P$, we can get the equation below:\[\left(\begin{array}{c}N\\k\end{array}\right)\cdot98\%^k\cdot(1-98\%)^{N-k}=P \]We can obtain $N$ when we give $P$ a certain value. In this paper,$P$ is 95{\%}. The $N_{EDS}$ is the actual number we obtainthrough the equation above.Now we have assumed that passengers can be checked unless be delayed by the people before him once he arrives at airport. Apparently, if the time length between two sets of flight is short, the density of passengers will begreat. It will bring great stress to security check and may even make some passengers miss their flight. To resolve this question, the airline has to install more EDSs to meet the demand. However, this measure will cost much more money. Consequently, we have to set a suitable time interval between two set of flight.Based on the base analysis above. We can use the equation(\ref{sets:number}) to decide the number of flight sets $N_{set}$ assuming we know the number of runways of a certain airport. Thenbased on the equation (\ref{PeakHour}), we can decide the peakhour length $H_{peak}$ when we assume a time interval between two consecutive flight sets. Then we use \textcircled{1} and\textcircled{2} to decide which to choose between equation(\ref{EDS:number:a}) and equation (\ref{EDS:number:b}). In consequence, we can obtain the minimum of EDSs number.If we choose different numbers of runways and the time intervalsbetween two flight sets, we can get different EDSs numbers. Inthis paper that followed, we gain a table of some value of$N_{runway}$ and $\Delta t$ with the corresponding EDSs numbers. Moreover, we draw some figure to reflect their relation.For a certain airport, its number of runway is known. Givencertain time interval ($\Delta t$), we can get the length of thepeak hour ($H_{peak}$). When the $N_{runway}$ is few enough,perhaps $H_{peak}$ is too long to be adopted. However, for acertain airline, they can decide the time interval of their ownpeak hour. In this given time interval, they could find theminimum of $N_{runway}$ through the Figure \ref{fig3}. We draw asketch map to describe our steps.\begin{figure}[hbtp]\centering\includegraphics[width=352.8pt,totalheight=214.2pt]{fig03.eps}\caption{}\label{fig3}\end{figure}\subsection{The Flight Schedule }According to the base analysis, we can know that the flightschedule matrix and $\Delta t$ is one form of flight timetable. In``The number of EDSs'', we can get suitable $\Delta t$. Then weshould resolve the flight schedule matrix.Because we have assumed that the checked baggage numbers of each flight setare equal to each other. It can be described as follows:\[\left\{\begin{array}{l}\rho_j\approx\bar{\rho}\\B_j^{set}\approx\bar{B}^{set}\end{array}\right.\begin{array}{*{20}c}\hfill&{j=1,2,\cdots,N_{set}}\hfill\end{array}\]The flight schedule matrix subject to this group:\[\left\{\begin{array}{ll}\sum\limits_{j=1}^{N_{set}}n_{ij}={NF}_i&i=1,2,\cdots\\\sum\limits_{i=1}^a n_{ij}\le N_{runway}&j=1,2,\cdots,N_{set}\\n_{ij}\ge0,&\mathrm{and}\:n_{ij}\:\mathrm{is}\:\mathrm{a}\:\mathrm{Integer} \end{array}\right.\]In order to make the best use of runway, we should make$\sum\limits_{i=1}^a n_{ij}$ as great as we can unless it exceed$N_{runway}$.Then we can see that how to resolve the flight schedule matrix is a problem of divide among a group of integers. This group is all the numbers of each flight passengers' baggage in one flight set. We program for this problem using MATLAB and we get at least one solution in the end. However, the matrix elements we have obtained are not integer, we have to adjust them to be integers manually.\subsection{Results and Interpretation for Airport A and B}The number of passengers in a certain flight (${NP}_i$), the timelength of security checking ($T_{set}$), the checking velocity ofEDS ($v_{EDS}$), and the number of baggage carried by onepassenger are random.\subsubsection{Data Assumption:}\begin{itemize}\item $T_{set}$ is 110 minutes, which is reasonable for airline.\item To simplify the problem, we assume that every passenger carry 2 baggage. If some of thepassengers carry one baggage, the solution based on 2 baggages per passenger meets therequirement.\item The number of runways in airport A and airport B is 5.\end{itemize}\subsubsection{Airport A:}Once the number of runway and the number of the flights aredecided, the flight schedule matrix is decided, too. We producethis matrix using MATLAB. This matrix companied by $\Delta t$ isthe flight schedule for airport A. $\Delta t$ will be calculatedin (\ref{Flight:baggage}), (\ref{sets:number}) and(\ref{PeakHour}).We calculate $N_{EDS}$ and make the flight timetable in threeconditions. The three conditions and the solution are listed asfollowed:\paragraph{Every flight are fully occupied}The checking speed of EDS is 160 bags/hour.\begin{table}[htbp]\centering\caption{}\begin{tabular}{*{11}c}\myhline{0.4mm}$\mathbf{\Deltat(\min)}$&\textbf{2}&\textbf{4}&\textbf{6}&\textbf{8}&\textbf{10}&\textbf{12}&\t extbf{14}&\textbf{16}&\textbf{18}&\textbf{20}\\\myhline{0.4mm}$N_{EDS}(\ge)$&31&31&31&31&31&29&24&22&20&17\\\hline$H_{peak}(\min)$&20&40&60&80&100&120&140&160&180&200\\\myhline{0.4mm}\end{tabular}\label{tab2}\end{table}We assume that the suitable value of $H_{peak}$ is 120 minutes.Then the suitable value of $\Delta t$ is about 12 minutes, and$N_{EDS}$ is 29 judged from Figure \ref{fig4}. Certainly, we canwork $\Delta t$ and $N_{EDS}$ out through equation.\begin{figure}[htbp]\centering\includegraphics[width=294.6pt,totalheight=253.2pt]{fig04.eps}\caption{}\label{fig4}\end{figure}\paragraph{Every flight is occupied by the minimal number of passengers on statistics in the long run.}The checking speed of EDS is 210 bags/hour.\begin{table}[htbp]\centering\caption{}\begin{tabular}{*{11}c}\myhline{0.4mm}$\mathbf{\Deltat(\min)}$&\textbf{2}&\textbf{4}&\textbf{6}&\textbf{8}&\textbf{10}&\textbf{12}&\t extbf{14}&\textbf{16}&\textbf{18}&\textbf{20}\\\myhline{0.4mm}$N_{EDS}(\ge)$&15&15&15&15&15&14&13&12&10&7\\\hline$H_{peak}(\min)$&20&40&60&80&100&120&140&160&180&200\\ \myhline{0.4mm}\end{tabular}\label{tab3}\end{table}We assume that the suitable value of $H_{peak}$ is 120 minutes.Then the suitable value of $\Delta t$ is about 12 minutes, and$N_{EDS}$ is 14 judging from Figure \ref{fig5}. Certainly, we canwork $\Delta t$ and $N_{EDS}$ out through equation.\begin{figure}[htbp]\centering\includegraphics[width=294.6pt,totalheight=253.2pt]{fig05.eps}\caption{}\label{fig5}\end{figure}\paragraph{${NP}_i$ and $v_{EDS}$ are random value produced by MATLAB.}\begin{table}[htbp]\centering\caption{}\begin{tabular}{*{11}c}\myhline{0.4mm}$\mathbf{\Deltat(\min)}$&\textbf{2}&\textbf{4}&\textbf{6}&\textbf{8}&\textbf{10}&\textbf{12}&\t extbf{14}&\textbf{16}&\textbf{18}&\textbf{20}\\\myhline{0.4mm}$N_{EDS}(\ge)$&15&22&21&21&15&17&21&16&13&14\\\hline$H_{peak}(\min)$&20&40&60&80&100&120&140&160&180&200\\\myhline{0.4mm}\end{tabular}\label{tab4}\end{table}We assume that the suitable value of $H_{peak}$ is 120 minutes.Then the suitable value of $\Delta t$ is about 12 minutes, and$N_{EDS}$ is 17 judging from Figure \ref{fig6}. Certainly, we canwork $\Delta t$ and $N_{EDS}$ out through equation.\begin{figure}[htbp]\centering\includegraphics[width=294.6pt,totalheight=249.6pt]{fig06.eps}\caption{}\label{fig6}\end{figure}\subsubsection{Interpretation:}By analyzing the results above, we can conclude that when$N_{EDS}$ is 29, and $\Delta t$ is 12, the flight schedule willmeet requirement at any time. The flight schedule is:\\[\intextsep]\begin{minipage}{\textwidth}\centering\tabcaption{}\begin{tabular}{c|*{8}c|c|c}\myhline{0.4mm}\backslashbox{\textbf{Set}}{\textbf{Type}}&\textbf{1}&\textbf{2}&\textbf{3}&\textb f{4}&\textbf{5}&\textbf{6}&\textbf{7}&\textbf{8}&\textbf{Numbers of Bags}&\textbf{Numbers of Flights}\\\myhline{0.4mm}1&2&0&0&0&2&1&0&0&766&5\\\hline2&2&0&2&0&2&0&0&0&732&4\\\hline3&0&1&1&1&2&0&0&0&762&4\\\hline4&0&1&0&0&2&1&0&0&735&4\\\hline5&0&1&0&0&2&1&0&0&735&5\\\hline6&2&0&0&0&1&0&0&1&785&5\\\hline7&2&0&0&0&2&0&1&0&795&5\\\hline8&0&1&0&0&2&1&0&0&735&4\\\hline9&2&0&0&0&2&1&0&0&766&5\\\hline10&0&0&0&2&2&0&0&0&758&5\\\hlineTotal&10&4&3&3&19&5&1&1&7569&46\\ \myhline{0.4mm}\end{tabular}\label{tab5}\end{minipage}\\[\intextsep]We have produced random value for ${NP}_i$ and $v_{EDS}$. On this condition, the number of EDSs is 17, which is less than 29 that wedecide for the airport A. That is to say our solution can meet thereal requirement.\subsubsection{Airport B:}\paragraph{The passenger load is 100{\%}}The checking speed of EDS is 160 bags/hour.\begin{table}[htbp]\centering\caption{}\begin{tabular}{*{11}c}\myhline{0.4mm}$\mathbf{\Deltat(\min)}$&\textbf{2}&\textbf{4}&\textbf{6}&\textbf{8}&\textbf{10}&\textbf{12}&\t extbf{14}&\textbf{16}&\textbf{18}&\textbf{20}\\\myhline{0.4mm}$N_{EDS}(\ge)$&33&33&33&33&33&30&27&23&21&19\\\hline。
数模美赛论文模板
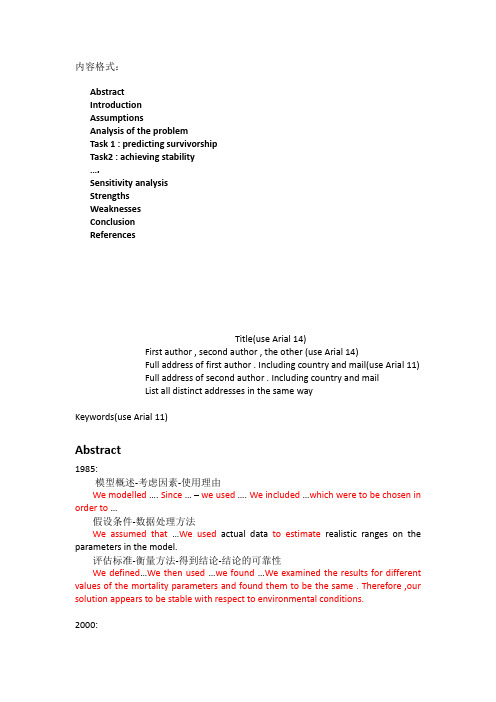
内容格式:AbstractIntroductionAssumptionsAnalysis of the problemTask 1 : predicting survivorshipTask2 : achieving stability….Sensitivity analysisStrengthsWeaknessesConclusionReferencesTitle(use Arial 14)First author , second author , the other (use Arial 14)Full address of first author . Including country and mail(use Arial 11)Full address of second author . Including country and mailList all distinct addresses in the same wayKeywords(use Arial 11)Abstract1985:模型概述-考虑因素-使用理由We modelled …. Since … – we used …. We included …which were to be chosen in order to …假设条件-数据处理方法We assumed that…We used actual data to estimate realistic ranges on the parameters in the model.评估标准-衡量方法-得到结论-结论的可靠性We defined…We then used …we found …We examined the results for different values of the mortality parameters and found them to be the same . Therefore ,our solution appears to be stable with respect to environmental conditions.2000:背景设置-模型引入…in order to determine …, we develop models using…解决问题-模型解决(层次排比)For the solution of …, we develop… model based … to … . For the solution of … , we employ … What is more , a self-adaptive traffic light is employed to … according to …模型检验-模型修正By comparison of … simulation results , the models are evaluated . …is formulated to judge which solution is effective .2002:The task is to … we begin by constructing a model of … base on … Using this model we can … Using… , we model … through … and obtain … we compare the performance of our model in … simulations show that …2001:We examine the … . Such evacuations been required due to … . in order to … , we begin with an analysis of … . For a more realistic estimate , including the effects of … , we formulate models of … . The model we construct is based on … .This model leads to a … and it further show that … what’ more , it agrees with …2004:(修正递进式模型摘要模板)The purpose of this paper is to propose … . We propose that … . To build a solid foundation , we define and test a simple model for … . We then develop … system , but we find it would be far from optimal in practice . We then propose that the best model is one that adapts to … . We implement … . We simulate the … with … and we find this system quickly converges to a nearly optimal solution subject to our constraints . It is , however , sensitive to some parameters . We discuss the effects of these findings on the expected effectiveness of the system in a real environment . We conclude that … is a good solution .问题分析句式:… is a real-life common phenomenon with many complexities .For a better view of … , we …To give a clear expression, we will introduce the method presented by …模型引入句式:In this paper , we present … model to simulate efficient methods for …We also apply … methods to solve …We address the problem of …. through ….We formulate the problem as …We formulate a … model to account for …Base on … , we establish a model ….We build a model to determine …We modify the model to reflect …To provide a more complete account for … , ... model has been employed .In this paper , in order to … , we design a … model based on …We propose a solution that …We have come up with an … for … andStrong evidence of ... , and powerful models have been created to estimate ...模型推进:… will scale up to an effective model for …模型求解:We employ … , one based on … and the other … , whose results agree closely.To combat this , we impose …结合数据:Using data from … , we determine …To ground this model in reality , we incorporate extensive demographic data ….We use data assembled by …Using a wide scale regression , we found that …We extrapolate from longevity data and explore the long-term behavior of …The base we developed was based off real-life data that gathered by …We estimate these characteristic numbers for a representative sample of …We fit the modified model to data , we conclude that …By statistical processing to results of …By analyzing the … on the basis of historic data in the same way mentioned in …结果给出句式:Results of this computation are presented , and ….We elicit that a conclusion …We conclude with a series of recommendations for …Given a … deviation in the value of the parameter , we calculate the percentage change in the value that the system converges to …As we can find out , in the situation of …. , …. is subject to the logistic regression .We conclude through analyzing that ….it is apparent that …Through comparing the … , we conclude that …According to the laws of … , we draw a conclusion of …From the formula , we know that …Thus we arrive at the conclusion :We elicit that a conclusion …As a consequence , we can get …模型可行性:Our suggested solution , which is easy to ….Since our model is based on … it can be applied to …Importantly , we use some practical data to test our model and analysis its stability , we simulate this model and receive a well effect .Therefore , we trust this model as an accurate testing ground for …Our algorithm is broad enough to accommodate various …定理可靠说明:That is the theoretical basis for … in many application areas .模型简化With further simplification, utilizing … we can reach …承上启下:In addition to the model , we also discuss …Because the movement of … operates by the same laws and equations as the movement of … , we can ….Based on the above discussion, considering …., let’s …误差句式:… does not deviate more than … from the target value .Theoretically , error due to … should not play a tremendous role under our model .Up to this point , we have made many approximations , not all of which are justified theoretically , but the results of algorithm are quite reasonable .This is a naïve approach which may mot ensure the …. , however if we … , the error is negligible .Context:方程给出:We derive the equation expressing the … as a function of… we haveA simple formula determined by the … is given by the equation :… can be written as the following system of equations :In particular , the … is defined in terms of … ,It is convenient to rewrite equations into the vector form:The expression of … can be expanded as …Equation (1) is reduced to ..Substitute the values into equation (1) ,we get …So its expression can be derived from equation (1) with small changes .Our results are summarized in the formula for …Plugging (1) into the equation for (2) , we obtain …Therefore , from (1)(2)(3) , we have the junction that …We use the following initial conditions to determine …When computing , we suppose … ,so this suppose doesn’t … ,then by … , we can get :According to equation (1)(2) , we can eliminate … ,then we can acquire :By connecting equation (6)(7) , we get the conclusion that …Then we can acquire … through the following equation :Its solution is the following …, in which … is …客观条件引入:A commonly accepted fact is that …A lot of research has been done to explain and find …In our model , we prefer to follow the conclusion used by …Here , we cite the model constructed by …There is one paper from … ,which concluded that …We get performance of … by citing the experimental results of …Here, we will introduce some terms used to address the problem, and we …方法给出句式:We construct … intelligent algorithms , a conservative approach , and an enthusiastic system to ..We formulate a simplified differential equation governing …This equation will be based on …The above considerations lead us to formulate the … asGiven this , it is easy to determine …To analyze the accuracy of our model , and determine a reasonable value for …We will evaluate the performance of our … by …We tabulate the … as a function of …Analysis for the … can be carried out exactly in the same fashion as …The main goal in attempting to model … is to determine … and … should be considered .The first requirement that the model reproduce is to determine if the model is reasonable .We begin our analysis of the phenomenon of congestion with the question of …In modeling this behavior , we begin with …In laying down the mathematics of this model , we begin with …When … is not taken into account ,it is …We give the criterion that …We fix A and examine the change of B with respect to CAttention has been draw to determine …To reveal the trend of … in a long horizonTo deal with the problem, we need figure out …图表句式:We can graph … with …,and such a plot is shown in …According to the above data , we can see that … . This phenomenon shows that … . Hence, we can safely arrive the conclusion that …Table 7 reports the general statistics under …文献句式:There is rather substantial literature on models for evaluating regional health condition, and most models fall into one of two categories: microscopic and macroscopic. Nevertheless, to get a more accurate understanding, we need to conduct quantitative modeling in our call for a better evaluation and predictive model.假设句式:In order to present our solution , we have made numerous simplifications to the given problem . we made several assumptions regarding either the problem domain itself , or …For the sake of simplicity , we will generally assume in our discussions that …… are assumed to have …We assume … are …Due to the symmetry of the image we can assume that …We came up with a few different hypotheses that …For simplicity we consider …We will use the following symbols and definitions in the description of the model:To get the general picture of the … , we rely on the assumption that …Hence we are bale to …This assumption makes sense , because we expect …Assumption … is valid , since if it was not the case , the … would …Make the following assumptions to approximate and simplify the problem …As a sweet spot , there is no doubt that … ,if not , …For the purpose of reaching a conclusion conveniently , we assume …We adopt a set of assumptions as follows …模型验证:To test our model , we developed a … simulator based on … , and the simulator was written in … and can be executed on several platforms .To demonstrate how our model works , we apply it to …跨段内容:For a further discussion of this model , please see Appendix A .The underlying idea is fairly simple内容引导:What we are really interested in is …With this consideration in mind , we now …Our goal is to … , one that would …We must restate the problem mathematically by narrowing our focus and defining our goals in order to obtain a good model .Given this idea , it is clear that we cannot compromise the …This immediately leads to useful conclusions . For example , …Given these assumptions , the following results can be quickly derived …We will pursue this goal …We turn our attention to …We restrict our attention to …In addition to the model , we also discuss policies for …In our paper , we take … factors into consideration to …… is not as simple a task as it might seem , because …For the … , we only take … into account .As the next step , we will introduce our advanced method to …Under the premise of this , …On the basis of previous analyses , we find the …效果评价:This is a good indication that our simulations are producing reasonable results .The results of our simulations ,shown in … indicate that the performance of …This is no surprise the distinguishing features of …This would appear very encouraging indeed , were it not …We therefore regard this model as reasonable .Here very little congestion occurs …As we will see when we apply our model to … ,this model works well . It should be noted ,though that this is not the only way to define the location of generator points , but it is a very good firs approach .//数据缺乏的理论化模型Of course , only theoretical explanations are not completely convincing . But mass of data relevant to our calculation can be obtained through experiments . Owing to our limit conditions , we only quote some experimental results from other literatures to assist and analyze our derived theoretical conclusion .变量指定句式:Let … denote … , and … to be …The … is … , where … is , W stands for , and … stands for bucks .For better description we assign …Note for brief description of the model , we will denote …解释句式:In fact , this assumption is reasonable not only because … but also that …In other word , …The key feature of this algorithm is …It is important to note that … can no longer be ignored when considering …If … , we can gain more insight into the nature of …The reason we care about … is that … ,the problem is determining …, if … so we ..Our approach weighs heavily on …To show that … are negligible , we vary …This is likely due to …修正:We modify … according to …We modify the model to reflect … and generalize the model to …影响:Because the effect of … and omit the resistance of …As the effect of …The model also incorporates the …At other impact points , the impact may …... has been implicated as the major component of ...图表用语:However , from this figure it is clear that the …From this figure one can see that …,one also notices that …This graph can be compared to the results of the symbolic model to see how well the model agrees with our simulated …The two plots at above which show … .According to …, there exists the fact that …According to …, it is no doubt that…拟合近似,模拟:Given the above assumptions , we may approximate …In simulations over a suitably long time period , we find …For each run of the simulation we fire enough blobs so that results are …An appropriate estimate for the … can be obtain as follows .In assessing the accuracy of a mathematical model , …We now propose a way to extend our model using a computer simulation of …It is obvious , however , that this is a highly subjective value that must be determined through experiment for each … that our model is applied to .This model leads to a computer simulation of …主要因素:Analyzing what parameters have the greatest effect on the simulation results indicates the things that we should focus on in order to produce a more effective evacuation scheme .Sensitivity analysisWe use the sensitivity analysis to defend our model . The sensitivity of a model is a measure of how sensitive the result changes at small changes of parameters . A good model is corresponding to low sensitiveness .Considering the parameters used in our solutions , here we provide following discussion .The above models we have built is based on … ,which has well solved … . To the final issue , we have take … into account in order to …Strength:On the whole , we have hound our model to be quite natural and easy to apply . Here , we list some of the advantages of our approach to the problem .The most distinct advantage of our model is that …Our methods can incorporate various scenarios : ….This model is simple enough for … to understand .Our method is robust (健壮), so that other variables or situations can be easily introduced .Our model … and is not overly sensitive to small changes in …Additionally , we avoided harsh assumptions that would constrain the possible …Most of the assumptions we did make could be accounted for as well in a more general model .We use random events to simulate the chaos of real world .The key insight to our model is that ….Our model also takes into account the …The model allows … which is more close to reality .The model is able to handle a range of …Besides rigorous theoretical derivation , we also citing the research results of numerous experts and scholars to test the result of our method .Weakness:Of course , there are many ways to attack a problem such as this one , In this section , we discuss some of the drawbacks of our approach to the problem , and some things that could have been done to deal with these issues .Some special data can’t be found , and it makes that we have to do some proper assumption before the solution of our models . A more abundant data resource can guarantee a better result in our model .The model responds slowly to dramatic changes in …The method does not allow … , which might be possible with a more radicalmodel .However , … constraints arise when pursuing this methodology .Additionally , this method would have required … , which would …However , the algorithms do have unique strengths and weaknesses when …Our model does take into account the complicated effects that …Factors considered in our method is relatively unitary , we only take the important factor into consideration .Lacking brief numerical calculation in our method , though we display rigorous theoretical derivation .。
美赛数学建模优秀论文
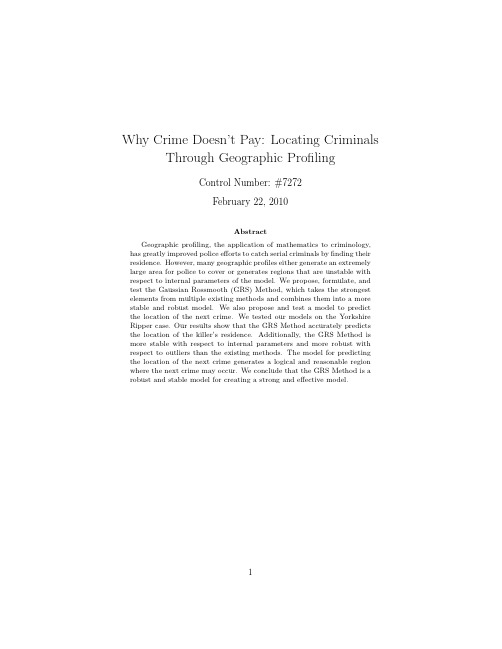
Why Crime Doesn’t Pay:Locating Criminals Through Geographic ProfilingControl Number:#7272February22,2010AbstractGeographic profiling,the application of mathematics to criminology, has greatly improved police efforts to catch serial criminals byfinding their residence.However,many geographic profiles either generate an extremely large area for police to cover or generates regions that are unstable with respect to internal parameters of the model.We propose,formulate,and test the Gaussian Rossmooth(GRS)Method,which takes the strongest elements from multiple existing methods and combines them into a more stable and robust model.We also propose and test a model to predict the location of the next crime.We tested our models on the Yorkshire Ripper case.Our results show that the GRS Method accurately predicts the location of the killer’s residence.Additionally,the GRS Method is more stable with respect to internal parameters and more robust with respect to outliers than the existing methods.The model for predicting the location of the next crime generates a logical and reasonable region where the next crime may occur.We conclude that the GRS Method is a robust and stable model for creating a strong and effective model.1Control number:#72722Contents1Introduction4 2Plan of Attack4 3Definitions4 4Existing Methods54.1Great Circle Method (5)4.2Centrography (6)4.3Rossmo’s Formula (8)5Assumptions8 6Gaussian Rossmooth106.1Properties of a Good Model (10)6.2Outline of Our Model (11)6.3Our Method (11)6.3.1Rossmooth Method (11)6.3.2Gaussian Rossmooth Method (14)7Gaussian Rossmooth in Action157.1Four Corners:A Simple Test Case (15)7.2Yorkshire Ripper:A Real-World Application of the GRS Method167.3Sensitivity Analysis of Gaussian Rossmooth (17)7.4Self-Consistency of Gaussian Rossmooth (19)8Predicting the Next Crime208.1Matrix Method (20)8.2Boundary Method (21)9Boundary Method in Action21 10Limitations22 11Executive Summary2311.1Outline of Our Model (23)11.2Running the Model (23)11.3Interpreting the Results (24)11.4Limitations (24)12Conclusions25 Appendices25 A Stability Analysis Images252Control number:#72723List of Figures1The effect of outliers upon centrography.The current spatial mean is at the red diamond.If the two outliers in the lower leftcorner were removed,then the center of mass would be locatedat the yellow triangle (6)2Crimes scenes that are located very close together can yield illog-ical results for the spatial mean.In this image,the spatial meanis located at the same point as one of the crime scenes at(1,1)..7 3The summand in Rossmo’s formula(2B=6).Note that the function is essentially0at all points except for the scene of thecrime and at the buffer zone and is undefined at those points..9 4The summand in smoothed Rossmo’s formula(2B=6,φ=0.5, and EPSILON=0.5).Note that there is now a region aroundthe buffer zone where the value of the function no longer changesvery rapidly (13)5The Four Corners Test Case.Note that the highest hot spot is located at the center of the grid,just as the mathematics indicates.15 6Crimes and residences of the Yorkshire Ripper.There are two residences as the Ripper moved in the middle of the case.Someof the crime locations are assaults and others are murders (16)7GRS output for the Yorkshire Ripper case(B=2.846).Black dots indicate the two residences of the killer (17)8GRS method run on Yorkshire Ripper data(B=2).Note that the major difference between this model and Figure7is that thehot zones in thisfigure are smaller than in the original run (18)9GRS method run on Yorkshire Ripper data(B=4).Note that the major difference between this model and Figure7is that thehot zones in thisfigure are larger than in the original run (19)10The boundary region generated by our Boundary Method.Note that boundary region covers many of the crimes committed bythe Sutcliffe (22)11GRS Method onfirst eleven murders in the Yorkshire Ripper Case25 12GRS Method onfirst twelve murders in the Yorkshire Ripper Case263Control number:#727241IntroductionCatching serial criminals is a daunting problem for law enforcement officers around the world.On the one hand,a limited amount of data is available to the police in terms of crimes scenes and witnesses.However,acquiring more data equates to waiting for another crime to be committed,which is an unacceptable trade-off.In this paper,we present a robust and stable geographic profile to predict the residence of the criminal and the possible locations of the next crime.Our model draws elements from multiple existing models and synthesizes them into a unified model that makes better use of certain empirical facts of criminology.2Plan of AttackOur objective is to create a geographic profiling model that accurately describes the residence of the criminal and predicts possible locations for the next attack. In order to generate useful results,our model must incorporate two different schemes and must also describe possible locations of the next crime.Addi-tionally,we must include assumptions and limitations of the model in order to ensure that it is used for maximum effectiveness.To achieve this objective,we will proceed as follows:1.Define Terms-This ensures that the reader understands what we aretalking about and helps explain some of the assumptions and limitations of the model.2.Explain Existing Models-This allows us to see how others have at-tacked the problem.Additionally,it provides a logical starting point for our model.3.Describe Properties of a Good Model-This clarifies our objectiveand will generate a sketelon for our model.With this underlying framework,we will present our model,test it with existing data,and compare it against other models.3DefinitionsThe following terms will be used throughout the paper:1.Spatial Mean-Given a set of points,S,the spatial mean is the pointthat represents the middle of the data set.2.Standard Distance-The standard distance is the analog of standarddeviation for the spatial mean.4Control number:#727253.Marauder-A serial criminal whose crimes are situated around his or herplace of residence.4.Distance Decay-An empirical phenomenon where criminal don’t traveltoo far to commit their crimes.5.Buffer Area-A region around the criminal’s residence or workplacewhere he or she does not commit crimes.[1]There is some dispute as to whether this region exists.[2]In our model,we assume that the buffer area exists and we measure it in the same spatial unit used to describe the relative locations of other crime scenes.6.Manhattan Distance-Given points a=(x1,y1)and b=(x2,y2),theManhattan distance from a to b is|x1−x2|+|y1−y2|.This is also known as the1−norm.7.Nearest Neighbor Distance-Given a set of points S,the nearestneighbor distance for a point x∈S ismin|x−s|s∈S−{x}Any norm can be chosen.8.Hot Zone-A region where a predictive model states that a criminal mightbe.Hot zones have much higher predictive scores than other regions of the map.9.Cold Zone-A region where a predictive model scores exceptionally low. 4Existing MethodsCurrently there are several existing methods for interpolating the position of a criminal given the location of the crimes.4.1Great Circle MethodIn the great circle method,the distances between crimes are computed and the two most distant crimes are chosen.Then,a great circle is drawn so that both of the points are on the great circle.The midpoint of this great circle is then the assumed location of the criminal’s residence and the area bounded by the great circle is where the criminal operates.This model is computationally inexpensive and easy to understand.[3]Moreover,it is easy to use and requires very little training in order to master the technique.[2]However,it has certain drawbacks.For example,the area given by this method is often very large and other studies have shown that a smaller area suffices.[4]Additionally,a few outliers can generate an even larger search area,thereby further slowing the police effort.5Control number:#727264.2CentrographyIn centrography ,crimes are assigned x and y coordinates and the “center of mass”is computed as follows:x center =n i =1x i ny center =n i =1y i nIntuitively,centrography finds the mean x −coordinate and the mean y -coordinate and associates this pair with the criminal’s residence (this is calledthe spatial mean ).However,this method has several flaws.First,it can be unstablewith respect to outliers.Consider the following set of points (shown in Figure 1:Figure 1:The effect of outliers upon centrography.The current spatial mean is at the red diamond.If the two outliers in the lower left corner were removed,then the center of mass would be located at the yellow triangle.Though several of the crime scenes (blue points)in this example are located in a pair of upper clusters,the spatial mean (red point)is reasonably far away from the clusters.If the two outliers are removed,then the spatial mean (yellow point)is located closer to the two clusters.A similar method uses the median of the points.The median is not so strongly affected by outliers and hence is a more stable measure of the middle.[3]6Control number:#72727 Alternatively,we can circumvent the stability problem by incorporating the 2-D analog of standard deviation called the standard distance:σSD=d center,iNwhere N is the number of crimes committed and d center,i is the distance from the spatial center to the i th crime.By incorporating the standard distance,we get an idea of how“close together”the data is.If the standard distance is small,then the kills are close together. However,if the standard distance is large,then the kills are far apart. Unfortunately,this leads to another problem.Consider the following data set (shown in Figure2):Figure2:Crimes scenes that are located very close together can yield illogical results for the spatial mean.In this image,the spatial mean is located at the same point as one of the crime scenes at(1,1).In this example,the kills(blue)are closely clustered together,which means that the centrography model will yield a center of mass that is in the middle of these crimes(in this case,the spatial mean is located at the same point as one of the crimes).This is a somewhat paradoxical result as research in criminology suggests that there is a buffer area around a serial criminal’s place of residence where he or she avoids the commission of crimes.[3,1]That is,the potential kill area is an annulus.This leads to Rossmo’s formula[1],another mathematical model that predicts the location of a criminal.7Control number:#727284.3Rossmo’s FormulaRossmo’s formula divides the map of a crime scene into grid with i rows and j columns.Then,the probability that the criminal is located in the box at row i and column j isP i,j=kTc=1φ(|x i−x c|+|y j−y c|)f+(1−φ)(B g−f)(2B−|x i−x c|−|y j−y c|)gwhere f=g=1.2,k is a scaling constant(so that P is a probability function), T is the total number of crimes,φputs more weight on one metric than the other,and B is the radius of the buffer zone(and is suggested to be one-half the mean of the nearest neighbor distance between crimes).[1]Rossmo’s formula incorporates two important ideas:1.Criminals won’t travel too far to commit their crimes.This is known asdistance decay.2.There is a buffer area around the criminal’s residence where the crimesare less likely to be committed.However,Rossmo’s formula has two drawbacks.If for any crime scene x c,y c,the equality2B=|x i−x c|+|y j−y c|,is satisfied,then the term(1−φ)(B g−f)(2B−|x i−x c|−|y j−y c|)gis undefined,as the denominator is0.Additionally,if the region associated withij is the same region as the crime scene,thenφi c j c is unde-fined by the same reasoning.Figure3illustrates this:This“delta function-like”behavior is disconcerting as it essentially states that the criminal either lives right next to the crime scene or on the boundary defined by Rossmo.Hence,the B-value becomes exceptionally important and needs its own heuristic to ensure its accuracy.A non-optimal choice of B can result in highly unstable search zones that vary when B is altered slightly.5AssumptionsOur model is an expansion and adjustment of two existing models,centrography and Rossmo’s formula,which have their own underlying assumptions.In order to create an effective model,we will make the following assumptions:1.The buffer area exists-This is a necessary assumption and is the basisfor one of the mathematical components of our model.2.More than5crimes have occurred-This assumption is importantas it ensures that we have enough data to make an accurate model.Ad-ditionally,Rossmo’s model stipulates that5crimes have occurred[1].8Control number:#72729Figure3:The summand in Rossmo’s formula(2B=6).Note that the function is essentially0at all points except for the scene of the crime and at the buffer zone and is undefined at those points3.The criminal only resides in one location-By this,we mean thatthough the criminal may change residence,he or she will not move toa completely different area and commit crimes there.Empirically,thisassumption holds,with a few exceptions such as David Berkowitz[1].The importance of this assumption is it allows us to adapt Rossmo’s formula and the centrography model.Both of these models implicitly assume that the criminal resides in only one general location and is not nomadic.4.The criminal is a marauder-This assumption is implicitly made byRossmo’s model as his spatial partition method only considers a small rectangular region that contains all of the crimes.With these assumptions,we present our model,the Gaussian Rossmooth method.9Control number:#7272106Gaussian Rossmooth6.1Properties of a Good ModelMuch of the literature regarding criminology and geographic profiling contains criticism of existing models for catching criminals.[1,2]From these criticisms, we develop the following criteria for creating a good model:1.Gives an accurate prediction for the location of the criminal-This is vital as the objective of this model is to locate the serial criminal.Obviously,the model cannot give a definite location of the criminal,but it should at least give law enforcement officials a good idea where to look.2.Provides a good estimate of the location of the next crime-Thisobjective is slightly harder than thefirst one,as the criminal can choose the location of the next crime.Nonetheless,our model should generate a region where law enforcement can work to prevent the next crime.3.Robust with respect to outliers-Outliers can severely skew predic-tions such as the one from the centrography model.A good model will be able to identify outliers and prevent them from adversely affecting the computation.4.Consitent within a given data set-That is,if we eliminate data pointsfrom the set,they do not cause the estimation of the criminal’s location to change excessively.Additionally,we note that if there are,for example, eight murders by one serial killer,then our model should give a similar prediction of the killer’s residence when it considers thefirstfive,first six,first seven,and all eight murders.5.Easy to compute-We want a model that does not entail excessivecomputation time.Hence,law enforcement will be able to get their infor-mation more quickly and proceed with the case.6.Takes into account empirical trends-There is a vast amount ofempirical data regarding serial criminals and how they operate.A good model will incorporate this data in order to minimize the necessary search area.7.Tolerates changes in internal parameters-When we tested Rossmo’sformula,we found that it was not very tolerant to changes of the internal parameters.For example,varying B resulted in substantial changes in the search area.Our model should be stable with respect to its parameters, meaning that a small change in any parameter should result in a small change in the search area.10Control number:#7272116.2Outline of Our ModelWe know that centrography and Rossmo’s method can both yield valuable re-sults.When we used the mean and the median to calculate the centroid of a string of murders in Yorkshire,England,we found that both the median-based and mean-based centroid were located very close to the home of the criminal. Additionally,Rossmo’s method is famous for having predicted the home of a criminal in Louisiana.In our approach to this problem,we adapt these methods to preserve their strengths while mitigating their weaknesses.1.Smoothen Rossmo’s formula-While the theory behind Rossmo’s for-mula is well documented,its implementation isflawed in that his formula reaches asymptotes when the distance away from a crime scene is0(i.e.point(x i,y j)is a crime scene),or when a point is exactly2B away froma crime scene.We must smoothen Rossmo’s formula so that idea of abuffer area is mantained,but the asymptotic behavior is removed and the tolerance for error is increased.2.Incorporate the spatial mean-Using the existing crime scenes,we willcompute the spatial mean.Then,we will insert a Gaussian distribution centered at that point on the map.Hence,areas near the spatial mean are more likely to come up as hot zones while areas further away from the spatial mean are less likely to be viewed as hot zones.This ensures that the intuitive idea of centrography is incorporated in the model and also provides a general area to search.Moreover,it mitigates the effect of outliers by giving a probability boost to regions close to the center of mass,meaning that outliers are unlikely to show up as hot zones.3.Place more weight on thefirst crime-Research indicates that crimi-nals tend to commit theirfirst crime closer to their home than their latter ones.[5]By placing more weight on thefirst crime,we can create a model that more effectively utilizes criminal psychology and statistics.6.3Our Method6.3.1Rossmooth MethodFirst,we eliminated the scaling constant k in Rossmo’s equation.As such,the function is no longer a probability function but shows the relative likelihood of the criminal living in a certain sector.In order to eliminate the various spikes in Rossmo’s method,we altered the distance decay function.11Control number:#727212We wanted a distance decay function that:1.Preserved the distance decay effect.Mathematically,this meant that thefunction decreased to0as the distance tended to infinity.2.Had an interval around the buffer area where the function values wereclose to each other.Therefore,the criminal could ostensibly live in a small region around the buffer zone,which would increase the tolerance of the B-value.We examined various distance decay functions[1,3]and found that the func-tions resembled f(x)=Ce−m(x−x0)2.Hence,we replaced the second term in Rossmo’s function with term of the form(1−φ)×Ce−k(x−x0)2.Our modified equation was:E i,j=Tc=1φ(|x i−x c|+|y j−y c|)f+(1−φ)×Ce−(2B−(|x i−x c|+|y j−y c|))2However,this maintained the problematic region around any crime scene.In order to eliminate this problem,we set an EPSILON so that any point within EPSILON(defined to be0.5spatial units)of a crime scene would have a weighting of a constant cap.This prevented the function from reaching an asymptote as it did in Rossmo’s model.The cap was defined asCAP=φEPSILON fThe C in our modified Rossmo’s function was also set to this cap.This way,the two maximums of our modified Rossmo’s function would be equal and would be located at the crime scene and the buffer zone.12Control number:#727213This function yielded the following curve (shown in in Figure4),which fit both of our criteria:Figure 4:The summand in smoothed Rossmo’s formula (2B =6,φ=0.5,and EPSILON =0.5).Note that there is now a region around the buffer zone where the value of the function no longer changes very rapidly.At this point,we noted that E ij had served its purpose and could be replaced in order to create a more intuitive idea of how the function works.Hence,we replaced E i,j with the following sum:Tc =1[D 1(c )+D 2(c )]where:D 1(c )=min φ(|x i −x c |+|y j −y c |),φEPSILON D 2(c )=(1−φ)×Ce −(2B −(|x i −x c |+|y j −y c |))2For equal weighting on both D 1(c )and D 2(c ),we set φto 0.5.13Control number:#7272146.3.2Gaussian Rossmooth MethodNow,in order to incorporate the inuitive method,we used centrography to locate the center of mass.Then,we generated a Gaussian function centered at this point.The Gaussian was given by:G=Ae −@(x−x center)22σ2x+(y−y center)22σ2y1Awhere A is the amplitude of the peak of the Gaussian.We determined that the optimal A was equal to2times the cap defined in our modified Rossmo’s equation.(A=2φEPSILON f)To deal with empirical evidence that thefirst crime was usually the closest to the criminal’s residence,we doubled the weighting on thefirst crime.However, the weighting can be represented by a constant,W.Hence,ourfinal Gaussian Rosmooth function was:GRS(x i,y j)=G+W(D1(1)+D2(1))+Tc=2[D1(c)+D2(c)]14Control number:#7272157Gaussian Rossmooth in Action7.1Four Corners:A Simple Test CaseIn order to test our Gaussain Rossmooth(GRS)method,we tried it against a very simple test case.We placed crimes on the four corners of a square.Then, we hypothesized that the model would predict the criminal to live in the center of the grid,with a slightly higher hot zone targeted toward the location of the first crime.Figure5shows our results,whichfits our hypothesis.Figure5:The Four Corners Test Case.Note that the highest hot spot is located at the center of the grid,just as the mathematics indicates.15Control number:#727216 7.2Yorkshire Ripper:A Real-World Application of theGRS MethodAfter the model passed a simple test case,we entered the data from the Yorkshire Ripper case.The Yorkshire Ripper(a.k.a.Peter Sutcliffe)committed a string of13murders and several assaults around Northern England.Figure6shows the crimes of the Yorkshire Ripper and the locations of his residence[1]:Figure6:Crimes and residences of the Yorkshire Ripper.There are two res-idences as the Ripper moved in the middle of the case.Some of the crime locations are assaults and others are murders.16Control number:#727217 When our full model ran on the murder locations,our data yielded the image show in Figure7:Figure7:GRS output for the Yorkshire Ripper case(B=2.846).Black dots indicate the two residences of the killer.In this image,hot zones are in red,orange,or yellow while cold zones are in black and blue.Note that the Ripper’s two residences are located in the vicinity of our hot zones,which shows that our model is at least somewhat accurate. Additionally,regions far away from the center of mass are also blue and black, regardless of whether a kill happened there or not.7.3Sensitivity Analysis of Gaussian RossmoothThe GRS method was exceptionally stable with respect to the parameter B. When we ran Rossmo’s model,we found that slight variations in B could create drastic variations in the given distribution.On many occassions,a change of 1spatial unit in B caused Rossmo’s method to destroy high value regions and replace them with mid-level value or low value regions(i.e.,the region would completely dissapper).By contrast,our GRS method scaled the hot zones.17Control number:#727218 Figures8and9show runs of the Yorkshire Ripper case with B-values of2and 4respectively.The black dots again correspond to the residence of the criminal. The original run(Figure7)had a B-value of2.846.The original B-value was obtained by using Rossmo’s nearest neighbor distance metric.Note that when B is varied,the size of the hot zone varies,but the shape of the hot zone does not.Additionally,note that when a B-value gets further away from the value obtained by the nearest neighbor distance metric,the accuracy of the model decreases slightly,but the overall search areas are still quite accurate.Figure8:GRS method run on Yorkshire Ripper data(B=2).Note that the major difference between this model and Figure7is that the hot zones in this figure are smaller than in the original run.18Control number:#727219Figure9:GRS method run on Yorkshire Ripper data(B=4).Note that the major difference between this model and Figure7is that the hot zones in this figure are larger than in the original run.7.4Self-Consistency of Gaussian RossmoothIn order to test the self-consistency of the GRS method,we ran the model on thefirst N kills from the Yorkshire Ripper data,where N ranged from6to 13,inclusive.The self-consistency of the GRS method was adversely affected by the center of mass correction,but as the case number approached11,the model stabilized.This phenomenon can also be attributed to the fact that the Yorkshire Ripper’s crimes were more separated than those of most marauders.A selection of these images can be viewed in the appendix.19Control number:#7272208Predicting the Next CrimeThe GRS method generates a set of possible locations for the criminal’s resi-dence.We will now present two possible methods for predicting the location of the criminal’s next attack.One method is computationally expensive,but more rigorous while the other method is computationally inexpensive,but more intuitive.8.1Matrix MethodGiven the parameters of the GRS method,the region analyzed will be a square with side length n spatial units.Then,the output from the GRS method can be interpreted as an n×n matrix.Hence,for any two runs,we can take the norm of their matrix difference and compare how similar the runs were.With this in mind,we generate the following method.For every point on the grid:1.Add crime to this point on the grid.2.Run the GRS method with the new set of crime points.pare the matrix generated with these points to the original matrix bysubtracting the components of the original matrix from the components of the new matrix.4.Take a matrix norm of this difference matrix.5.Remove the crime from this point on the grid.As a lower matrix norm indicates a matrix similar to our original run,we seek the points so that the matrix norm is minimized.There are several matrix norms to choose from.We chose the Frobenius norm because it takes into account all points on the difference matrix.[6]TheFrobenius norm is:||A||F=mi=1nj=1|a ij|2However,the Matrix Method has one serious drawback:it is exceptionally expensive to compute.Given an n×n matrix of points and c crimes,the GRS method runs in O(cn2).As the Matrix method runs the GRS method at each of n2points,we see that the Matrix Method runs in O(cn4).With the Yorkshire Ripper case,c=13and n=151.Accordingly,it requires a fairly long time to predict the location of the next crime.Hence,we present an alternative solution that is more intuitive and efficient.20Control number:#7272218.2Boundary MethodThe Boundary Method searches the GRS output for the highest point.Then,it computes the average distance,r,from this point to the crime scenes.In order to generate a resonable search area,it discards all outliers(i.e.,points that were several times further away from the high point than the rest of the crimes scenes.)Then,it draws annuli of outer radius r(in the1-norm sense)around all points above a certain cutoffvalue,defined to be60%of the maximum value. This value was chosen as it was a high enough percentage value to contain all of the hot zones.The beauty of this method is that essentially it uses the same algorithm as the GRS.We take all points on the hot zone and set them to“crime scenes.”Recall that our GRS formula was:GRS(x i,y j)=G+W(D1(1)+D2(1))+Tc=2[(D1(c)+D2(c))]In our boundary model,we only take the terms that involve D2(c).However, let D 2(c)be a modified D2(c)defined as follows:D 2(c)=(1−φ)×Ce−(r−(|x i−x c|+|y j−y c|))2Then,the boundary model is:BS(x i,y j)=Tc=1D 2(c)9Boundary Method in ActionThis model generates an outer boundary for the criminal’s next crime.However, our model does notfill in the region within the inner boundary of the annulus. This region should still be searched as the criminal may commit crimes here. Figure10shows the boundary generated by analyzing the Yorkshire Ripper case.21。
美赛写作模板及参赛经验分享
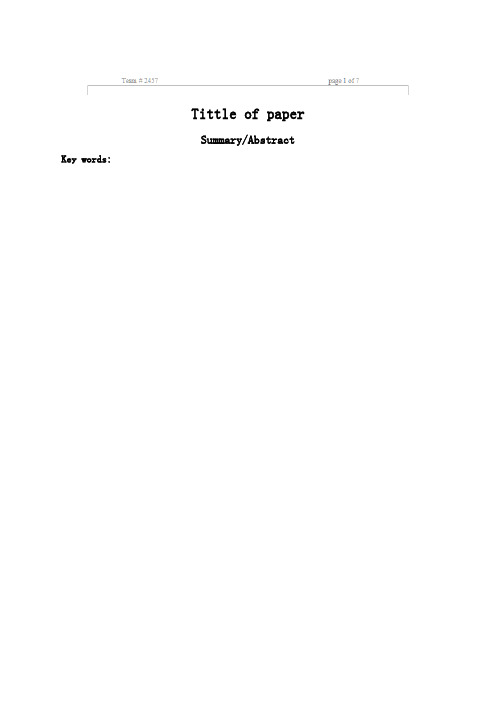
Tittle of paperSummary/Abstract Key words:I.Introduction(引言)Organ transplantation is a preferable treatment for the most serious forms of end-stage diseases. In recent years, advances in medical science and technology have made solid organ transplantation an increasingly successful and common medical procedure, a literal ''second chance at life". Not only does it offer the best hope for complete rehabilitation, but it has also proved to be the most cost-effective of all treatment options, including dialysis. Consequently, more and more people are benefiting from organ transplants and their survival rates are steadily improving. The surgical techniques involved have been mastered for half a century and are now considered as routine. The two main sources of kidneys for transplantation are deceased-donor kidneys and live-donations from family and friends. However, unfortunately, there is a considerable shortage of donor organs, compared to demands. As a matter of fact, efficient matching and allocation of organs donated has become an exigent problem.The United Network for Organ Sharing (UNOS), as the operator of the Organ Procurement and Transplantation Network (OPTN), is responsible for transplant organ distribution in the United States. UNOS oversees the allocation of many different types of transplants,including liver, kidney, pancreas, heart, lung, and cornea.Focusing on kidney transplantation, based on UNOS Kidney Allocation Model, we develop a mathematical model for US transplant networks. First, incoming organs are matched with waiting candidates by medical institutions considering the factors as ABO blood compatibility, the degree of recipient major HLA mismatch in order to obtain a matching degree applied on the allocation part. After that, from the patients’perspective, on the basis of linear regression, priority weight is defined by pondering age, disease severity, time on waiting, PRA level, and region. Applying this mechanism of ranking, we realize MWBM (Maximum Weight Bipartite-graph Matching) and SMGS (Stable Matching based on Gale-Shapley algorithm). MWBM focuses on the optimal assignment of donors following the algorithm of bipartite-graph maximum weight matching; SMGS emphasizes the process of exchanges in order to obtain the stable exchanges between donors and candidates on the waiting list.II.T he Description of Problem(问题重述)III.Basic Assumptions●The level of mismatch is only relative to the number of antigens.●The data and information are accurately registered according tothe medical measures●The data and information are refreshed in time according to thestatus of the patients●No differences in the quality of the donor kidneys●The quality of the donor kidney is constantIV.D efinitions and Notations●Kidney transplantation: A kidney transplant is a surgical procedure to implant a healthykidney into a patient with kidney failure.●Prioritization●MD: Matching Degree●PW: Prioritization weight●MWSM: Maximum Weight Bipartite Matching●SMGS: Stable Matching based on Gale-Shapley algorithm或V.ModelsThrough the investigation of US transplantation network, we draw a general picture of the mechanism. With reference to some resources available on the website of UNOS, a flow chart (Figure 1) is developed showing the procedure of the network.Currently, the initial waiting list is composed of patients whoare waiting for a kidney or combined kidney-pancreas transplant. For the first time, the patients are requested to show the correct and scientific information to the US kidney transplant network which is needed for donor-recipient matching, the ranking of patients on the waiting list, and determining the outcome of those transplanted. The patients’waiting lists are composed of initial patients, historical patients and unsuccessful recipient after transplantation. Historical patients refer to registered patients whose status have changed and have an influence on the procedure.A patient is taken off the waiting list when a graft is offered and accepted by that patient or the patient is dead while waiting for a transplant. Unsuccessful recipients refer to the patients who have a bad result of transplantation calling for transplantation again, as it is so-called relistFigure 1. A schematic depicting the steps occurring in thetransplantation networks......Table 1.Survival rate involving HLA mismatchVI.C onclusions.Our model for the optimal allocation of the donor organs is established by three modules, procurement of MD and PW, optimal assignment by MWBM model and Stable Matching of Gale-Shapley algorithm. The model has offered a convincing procedure of the allocation with the ……VII.Strengths and weaknesses(模型优缺点)Strengths……WeaknessesVIII.References注意文献的积累,不要等到文章写完再去重新寻找文献。
数学建模美赛三等奖论文
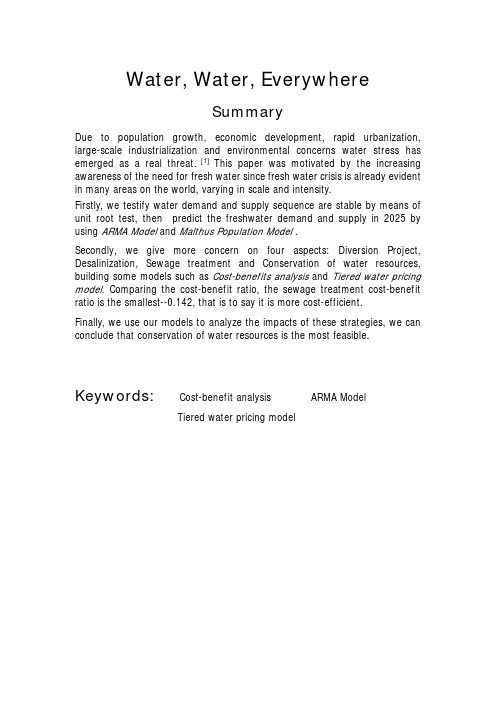
Water, Water, EverywhereSummaryDue to population growth, economic development, rapid urbanization, large-scale industrialization and environmental concerns water stress has emerged as a real threat. [1]This paper was motivated by the increasing awareness of the need for fresh water since fresh water crisis is already evident in many areas on the world, varying in scale and intensity.Firstly, we testify water demand and supply sequence are stable by means of unit root test, then predict the freshwater demand and supply in 2025 by using ARMA Model and Malthus Population Model .Secondly, we give more concern on four aspects: Diversion Project, Desalinization, Sewage treatment and Conservation of water resources, building some models such as Cost-benefits analysis and Tiered water pricing model. Comparing the cost-benefit ratio, the sewage treatment cost-benefitratio is the smallest--0.142, that is to say it is more cost-efficient.Finally, we use our models to analyze the impacts of these strategies, we can conclude that conservation of water resources is the most feasible.Keywords:Cost-benefit analysis ARMA ModelTiered water pricing modelA Letter to a governmental leadershipFebruary 4, 2013Dear Sir,During the four days working, our team spares no effort using cost and benefits analysis determine water strategy for 2013 about how to use water efficiently to meet the need in 2025. Now, we outline our conclusion to you.z Diversion ProjectThe South-North Water Transfer Project is a multi-decade infrastructure project solved the unbalance of water resource. The cost is 6.2yuan/3m, and it will much higher while the distance is more than 40 kilometers.z DesalinizationDesalinization utilizes the enormous seawater and provides freshwater in a cheaper price. However, interior regions with water scarcity can hardly benefit from it as most desalinization manufacturers located on eastern coastal areas. The cost of production is 5.446 yuan/t, but the transport costs less the cost-efficient competitiveness. The cost can be decreased by using more advanced technology.z Sewage treatmentSewage treatment can relief the environmental impact of water pollution by removing contaminants from water, the cost of Sewage treatment is 0.5yuan/t. z Conservation of water resourcesConservation makes sure of the source of rational use of water. There are several approaches on water resources conservation, the main problem is the lack of supervision. The benefit-cost ratio is between 0.95 and 3.23, and it has a high return-investment ratio.z Each of the above water strategy has its own advantages and disadvantages, we should consider the aspects of economic, physical, environmental, geographical, and technique factors overall, then choose the optimal strategy for different area.Yours sincerely,COMAP #23052ContentI Introduction (2)II Assumptions (3)III Models (3)3.1 The prediction of freshwater shortage in 2025 (3)3.1.1 The prediction of freshwater demand (3)3.1.1.1 The description of basic model (3)3.1.1.2 Model building (4)3.1.1.3 Model prediction (5)3.1.2 The prediction of freshwater supply (7)3.1.2.1 Model building (7)3.1.2.2 Model prediction (8)3.1.3. Conclusion (9)3.2Water strategy (9)3.2.1 Diversion Project (9)3.2.2 Desalinization (14)3.2.3 Sewage Treatment (16)3.2.4 Conservation of water resources (19)3.2.4.1 Agricultural water saving (20)3.2.4.2 Life water saving (21)IV The influence of our strategy (25)4.1 The influence of Water Diversion Project (25)4.2 The influence of desalination (25)4.3 The influence of sewage treatment (26)4.4 Water-saving society construction (26)V References (27)VI Appendix (28)I IntroductionAccording to relevant data shows that 99 percent of all water on earth is unusable, which is located in oceans, glaciers, atmospheric water and other saline water. And even of the remaining fraction of 1 percent, much of that is not available for our uses. For a detailed explanation, the following bar charts show the distribution of Earth's water: The left-side bar shows where the water on Earth exists; about 97 percent of all water is in the oceans. The middle bar shows the distribution of that 3 percent of all Earth's water that is fresh water. The majority, about 69 percent, is locked up in glaciers and icecaps, mainly in Greenland and Antarctica.[2] Except for the deep groundwater which is difficult to extract, what can be really used in our daily life is just 0.26 percent of all water on earth.Figure 1 The distribution of Earth's waterFreshwater is an important natural resource necessary for the survival of all ecosystems. There is a variety of unexpected consequence due to the lack of freshwater: 6,000 children die every day from diseases associated with unsafe water and poor sanitation and hygiene; Unsafe water and sanitation leads to 80% of all the diseases in the developing world;[3]Species which live in freshwater may be extinct, thus, breaking the food chain balance severely; The development of economic slow down in no small measure.It is with these thoughts in mind, many people think freshwater is very important than ever before.So, how to use freshwater efficiently? What is the best water strategy? Readmore and you will find more.II AssumptionsIn order to streamline our model we have made several key assumptions :1. We chose China as the object study.2. The water consumption of the whole nation could be approximate regardedas the demand of water .3. The Precipitation is in accordance with the supply of water .4. No considering about sea level rising because of global warmingIII Models3.1 The prediction of freshwater shortage in 2025How much freshwater should our strategy supply? Firstly, our work is to predict the gap between freshwater demand and supply in 2025. We obtain thefreshwater consumption data from China Statistical Yearbook. 3.1.1 The prediction of freshwater demandWe forecast the per capita demand for freshwater by building the ARMA Model .3.1.1.1 The description of basic modelThe notation ARMA(p, q) refers to the model with p autoregressive termsand q moving-average terms. This model contains the AR(p) and MA(q) models,mathematical formula is:qt q t t t p t p t t t y y y y −−−−−−−−−−+++=εθεθεθεφφφ......22112211 (1) AR(p) modelt p t p t t t y y y y εφφφ+++=−−−...2211 (2) MA(q) model q t q t t t t y −−−−−−−=εθεθεθε....2211 (3)),.....,2,1(p i i =φ ,),.....,2,1(q j j =θare undetermined coefficients of themodel, t ε is error term, t y is a stationary time series.3.1.1.2 Model buildingAll steps achieved by using EviewsStep1: ADF test stability of sequenceNull hypothesis:1:0=ρH , 1:1≠ρH , ρis unit root.Table 1 Null Hypothesis: Y has a unit root Exogenous: Constant Lag Length: 3 (Automatic based on SIC, MAXLAG=3) t-Statistic Prob. Augmented Dickey-Fuller test statistic -5.3783580.0040 Test critical values: 1% level-4.582648 5% level -3.32096910% level -2.801384We know Prob=0.0040 that we can reject the null hypothesis, and thenydoesn’t have a unit root, in other words, is stationary series. Step 2: Building the ARMA ModelThen we try to make sure of p and q by using the stationary series y .Table 2Date: 02/02/13 Time: 11:08Sample(adjusted): 2001 2011Included observations: 11 after adjusting endpointsConvergence achieved after 12 iterationsBackcast: 1998 2000Variable Coefficie nt Std. Error t-StatisticProb.AR(1) 1.0105040.005813173.8325 0.0000MA(3) 0.9454040.03650725.89639 0.0000R-squared 0.831422 Mean dependent varAdjustedR-squared 0.812692 S.D. dependent varS.E. of regression 5.085256 Akaike info criterionSo, we can get the final model, is:310.9454041.010504−−+=t t t d y y ε (4)3.1.1.3 Model predictionStep 1: The prediction of per capita freshwater demandWe use model (4) to predict the per capita demand of freshwater in the year2025, the result as Figure3.Figure 2 sequence diagram of dynamic predictionFrom the diagram, we can see the per capita freshwater demand is raising.The detailed data as Table3: Table 3 2010 2011 2012 2013 2014 2015 2016 2017 483.3584 488.4357 493.5662 498.7507503.9896509.2836514.6332 520.03892018 2019 2020 2021 2022 2023 2024 2025 525.5015 531.0214 536.5993 542.2358547.9315553.6871559.503 565.3801(cu.m/person)Through the above efforts, we get the 2025 per capita freshwater demand is565.3801 cu.mStep 2: The prediction of the whole freshwater demandThe relationship among d Q ,t N ,daverage Q is: daverage t d Q N Q ×= (5)d Q is the whole demand of freshwater, t N is the total population ,daverage Q is per capita of freshwater demand.Then we etimate the total population by the Malthus Population Model . rt e N t N 0)(=[4] (6))(t N is the population at time t,0N is the population at time 0,r is net relative growth rate of the populationrt e N N 2011)2025(= (7)By calculating, we get:(billion)42.11.347)2025(1500479.0≈=×e N (8)At last,we could get the whole demand of freshwater while the time is 2025.38.5652.14)2025(×=×=daverage d Q N Q ()cu.m million 100 8028.396= (9)3.1.2 The prediction of freshwater supplySimilarily,we predict freshwater supply using the ARMA Model. 3.1.2.1 Model buildingStep1: ADF test stability of sequenceNull hypothesis:1:0=ρH , 1:1≠ρH , ρis unit root. Table 4 Null Hypothesis: D(Y) has a unit root Exogenous: Constant Lag Length: 2 (Automatic based on SIC, MAXLAG=3)t-Statistic Prob. Augmented Dickey-Fuller test statistic-9.433708 0.0002 Test critical values: 1% level -4.803492 5% level -3.40331310% level -2.841819From the table, we find that first difference of supply data is smooth, we canreject the null hypothesis, that is ()y D is a smooth series.Step 2: Building the ARMA ModelWe use the smooth series ()y D to make sure the number of order.Table 5Date: 02/02/13 Time: 14:16Sample(adjusted): 2002 2010 Backcast: 1999 2001Variable CoefficientStd. Error t-Statistic Prob. AR(1) 0.6351030.158269 4.012804 0.0051 MA(3) -0.9923370.069186-14.34306 0.0000 R-squared 0.812690 Mean dependent var 50.51111Adjusted R-squared 0.785931 S.D. dependent var 119.1793S.E. of regression 55.14139 Akaike info criterion 11.05081Sum squared resid 21284.01 Schwarz criterion11.09464 Log likelihood -47.72864 Durbin-Watson stat 2.895553Then ,we get the final model is:)0.992337D(-)0.635103D()(31−−=t t t s y y D ε (10) 3.1.2.2 Model predictionWe use the effective model to predict freshwater supply in short-term until theyear 2025.Figure 3 sequence diagram of dynamic predictionFrom the diagram, we can see the supply remains unchanged basically .T The detailed data as Table6: Table 6 2010 2011 2012 2013 2014 2015 2016 2017 5630.203 5630.594 5630.843 5631.0015631.1025631.1655631.206 5631.2322018 2019 2020 2021 2022 2023 2024 2025 5631.248 5631.258 5631.265 5631.2695631.2725631.2735631.275 5631.275(100 million cu.m)According to the above data,we gain the supply of freshwater 2025, is5631.275(100 million cu.m)3.1.3. ConclusionFrom the above result,we find a serious issue:Table 7Year Demand offreshwater Supply of freshwater Net demand Unit2025 8028.396 5631.275 2397.121(100 million cu.m)In the year 2025, China will face the serious situation of freshwater shortage, the gap will reach 2397.121(100 million cu.m), therefore, in order to avoid this, we need to determine a series strategy to utilize freshwater efficiently.3.2Water strategy3.2.1 Diversion ProjectOn one hand, in view of Figure4, we can get information: Southeast coast is of the maximum precipitation, followed by the northern region, the western least.Figure 4 Precipitation Allocation Map of Major CitiesOn the other hand, in view of Figure 5, we can get information: The northern region and the southern coastal areas have the most water consumption, the western use less.Figure 5 Water Use MapDetailed data see to attached Table8 and Table9.South-to-North Water Diversion ProjectThe South–North Water Transfer Project is a multi-decade infrastructure project of China to better utilize water resources. This is because heavily industrialized Northern China has a much lower rainfall and its rivers are running dry. The project includes a Eastern, a Central and a Western route.Figure 6 The route of South-to-North Water Diversion ProjectHere, we take Western Route Project (WRP) as a representative, analysis the cost and benefits. As the strategic project to solve the problem of poorer water Northwest and North China, WRP will divert water from the upper reach of Yangtze River into Yellow Rive.Cost and benefits analysisThe direct quantitative economic benefits include urban water supply economic benefits, ecological environment water supply economic benefits, and the Yellow River mainstream hydroelectric economic benefits.[5]Urban water supply economic benefits:(1) Calculation MethodIn view of the water shadow price is difficult to determine, the equivalent engineering is not easy to choose, and the lack of water loss index is unpredictable, combined with the stage job characteristics, we select the method of sharing coefficient to calculate the urban water supply economic benefits.(2) Calculation ParametersThe Water consumption quota of per ten thousand yuan industrial output value is based on status quota, the predicted water consumption quota of per ten thousand yuan output value according to reach in 2 0 2 0 is :Lanzhou tom/ ten thousand yuan, gantry to Sanmenxia HeKouZhen river section for 26 3m/ ten thousand yuan. After a comprehensive analysis, set the reach for 20 3industrial water supply benefit allocation coefficient values 2.0 %.(3) Calculation ResultsAccording to (1) and (2), get table 10:Table 10water supply 3.2 billion 3.mproject benefits 20 billion yuan.8yuan /3maverage economic benefit 70z Ecological environment water supply economic benefits:(1) Calculation methodTake Forestry and animal husbandry as the representative, calculate whoseirrigation Economic benefits, and consider the allocation function of water supply. Analyse forestry benefits in reference with the increased wood savings, Animal husbandry in reference with the increased output of animals which were feeded by the incresed irrigation pasture (represented by sheep), both Forestry and animal husbandry account for half of the Ecological environment water supply.(2) Calculation parameters Set the water consumption quotas of Forestry irrigation unified as 233750hm m , the water supply sharing coefficient of Xiang irrigation as 0.60. In the calculation of forestry benefit, the increase of accumulated timber amount is ()a hm m ⋅235.22, timber price is 3300m yuan ; in the calculation of animal husbandry benefit , the increased stocking rates of unit pasture area is 25.22hm , taken a standard sheep price as yuan 260.(3) Calculation ResultsAccording to (1) and (2), the ecological environment water supply economic benefits is 714.1 billion, in which, The Yellow River replenishment economic benefits is 008.1billion yuan.z Hydroelectric economic benefits.(1) Diversion increased energy indicators:The increased electricity indicators is 306.9billion h kw ⋅, capacity enlargement the scale of 241 ten thousand kw .(2) Calculation methodTake the Optimal equivalent alternative engineering cost method, chosen fire electricity as an alternative project which can meet the power requirements of grid electricity equally. The sum of alternative engineering required annualinvestment translation and the annual running costs is increased annual power generating efficiency of the Yellow River cascade hydropower stations. (3) Calculation parametersThe power plant construction investment of kw $450, duration of five years, the investment proportion were 10%, 25%, 35%, 25%, 5%. Both the economic life of mechanical and electrical equipment and the metal structures equipment are taken as 20 years, considering the update ratio as 80% of the original investment. Standard coal price is taken as 160 dollars, standard coal consumption is taken as ()h kw g ⋅350. The fixed run rates take 4.5%, thesocial discount rate is 12%, the hydropower economic useful life of 5 years.(4) Calculation ResultsBy analysis and calculation, the first phase of water regulation produce the hydropower economic benefit is 3.087 billion.z Total economic benefits:Preliminary cost estimates of the project diversionOn the basis of economic nature classification, the total cost includes themachinery depreciation charges, wages and welfare costs, repair costs, thecost of materials,water district maintenance fees, management fees, water fees, interest expense and other . Analysis in the light of various estimates condition, the cost of water diverted into the Yellow River is 31~7.0m yuan c =The cost-benefit rate ()85.8~2.61∈=rc ω (11) 3.2.2 DesalinizationThough diversion project can balance water supply between places one has enough water and the other has water shortage, the costs will higher than desalinization when the distance more than 40 kilometers.Desalinization and comprehensive utilization of the work are increasingly taking centre stage on the problem of solving freshwater scarcity. Many countries and areas devote to optimize an effective way by enhancing the development of science and technology.According to the International Desalination Association, in 2009,14,451 desalination plants operated worldwide, producing 59.9 million cubic meters per day, a year-on-year increase of 12.3%.[6] The production was 68 million 3m in 2010, and expected to reach 120million 3m by 2020; some 40 million 3m is planned for the Middle East.[7]China has built more than 70 sets of sea water desalinization device with the design capacity of 600,000m3 and an average annual growth rate of more than 60%; technology with independent intellectual property rights of a breakthrough in the reverse osmosis seawater membranes, high pressure pumps, devices for energy recovery achieved significant progress, the desalinization rate raises from 99.2% to 99.7%; conditions of industrial development and the desalination market has been basically formed.MethodsDe-salinization refers to any of several processes that remove some amount of salt and other minerals from saline water. More generally, desalination may also refer to the removal of salts and minerals.[8] Most of the modern interest in desalination is focused on developing cost-effective ways of providing fresh water for human use.There are two main methods of desalinization:1. Extract freshwater from saline water: Distillation (Multi-stage flash distillation, Vapor compression distillation, Low temperature multi-effect distillation), Reverse osmosis, Hydrate formation process, Solvent extraction, Freezing.2. Remove salt from saline water: Ion exchange process, Pressure infiltration method, Electroosmosis demolition method.For desalination, energy consumption directly determines the level of the cost of the key. Among the above methods, reverse osmosis is more cost-effective than the other ways of providing fresh water for human use. So, reverse osmosis technology has become the dominant technology in international desalinization of seawater.The following two figures show the working principle diagram of a reverse osmosis system.Figure7 working principle diagram of a reverse osmosis systemCost and benefits analysisTable 12 general costs for a reverse osmosis systemItem Unitprice(yuan/t)Chemicals cost 0.391electric charge 2.85Wages 0.034 Labor costWelfare 0.04 Administrative expenses 0.0008maintenance costs 0.23Membrane replacement cost 0.923Depreciationexpense Fixed assets depreciation0.97expenseTotal costs 5.446Table 13 general benefit for a reverse osmosis systemItem ValueHourly output(t) 10Working hours/day24 Daily output(t)240 Working days/year 365 Yearly output(t)87600 Yearly other benefits(yuan)310980 Unit water other benefits3.55 Water Price(yuan/t)8 Unit water total benefits11.55 Unit water total benefit 55.11=rWater cost-benefit ratio 4715.055.11446.52===r c w (12) 3.2.3 Sewage TreatmentSewage treatment is an important process of water pollution treatment. It uses physical, chemical, and biological ways removing contaminants from water . Its objective is to relief the environment impact of water pollution.This diagram shows a typical sewage treatment process.Figure 8 Sewage treatment flow mapTake Sewage Treatment Plant in east china as an example to analysis the cost and benefit of sewage treatment.Suppose:Sewage treatment scale d t x 100001=,The Sewage Treatment Plant workdays in a year 300=d ,Concession period is twenty to thirty years, generally 251=t years, Construction period is one to three years, generally 32=t years.Operation period = Concession period - Construction period.Cost estimation Table 14 fixed investment estimate c1(ten thousand Yuan)number project ConstructioninvestmentEquipment investment 1 Preprocessing stage38 27 2Biological treatment section 42 134 3End-product stage 11 44 4 Sludge treatment section 6323 5 accessory equipment 456 Line instrument 687 Construction investment 3008 Unexpected expense 809 Other expense 10010 Total investment975 Table 15 Operating expense estimate c2 (ten thousand Yuan)[9]number project expenses1 maintenance expenses 6.52 wages 103 Power Consumption 404Agent cost 10 5 Small meter operating cost 66.56 Amortization of intangibles 127Amortization of Construction 6.6 8Amortization of Equipment 19.8 9Annual total cost 104.9 10 Tons of water operation cost 0.29Annual total investment 15022213=+÷=c c c ten thousand YuanAnnual amount of sewage treatment t x x 3000000100003003001=×=×= Unit sewage investment t yuan t yuan x c c 5.03000000150000034=÷=÷= Benefit analysisSewage mainly comes from domestic sewage(40%), industrial sewage(30%), and the others(including stormwater , 30%)Sewage treatment price: domestic sewage is about t yuan 8.0, industrialsewage is about t yuan 5.1, and other is about t yuan 5.2.Unit sewage treatment approximate price t yuan t yuan t yuan tyuan p 52.1%302%305.1%408.01=×+×+×=Unit Sewage treatment benefit:t yuan p p r 52.321=+= Cost-benefit ratio 142.052.35.043===r c ω (13) 3.2.4 Conservation of water resourcesTo realize the sustainable development of water resources, one of the important aspects is the conservation of water resources. Saving water is thekey of conservation, so, we the construction of water-saving society is the keyof water resources conservation strategy.To construct the water-saving society, we give more concern about two aspects:agricultural water saving and life water saving. Finally, we analysis the cost andbenefit about water-saving society by building model.3.2.4.1 Agricultural water savingStrategic suggestions of water-saving agriculture1. Strengthen the government policies and public finance support2. Mobilizing all social forces to promote water-saving agriculture development3. Innovating enterprises to improve the science and technology4. Suggesting countries to regard water saving as a basic state policy5. Implement the strategy of science and technology innovationwater saving function product research and development as the key point, the research and development of a batch of suitable for high efficiency and low energy consumption, low investment, multi-function water saving and high efficient agriculture key technology and major equipment. Micro sprinkler irrigation water saving technology and equipment is the typical technology.[10] Typical analysis: drip irrigation technologyIrrigation uniformity DU and field irrigation water utilization αE can be expressed as the technical elements of the function :[11]),,,,,,(01co c in t F I S n L q f DU α=),,,,,,,(0SMD t F I S n L q f E co c in αα=RD SMD fc )(θθ−=in q is single discharge into earth,L is (channel) long,n is manning coefficient,0S is tiny terrain conditions,c I is soil infiltration parameters,αF is (channel) cross-sectional parameters,co t is irrigation water supply time,SMD is irrigation soil water deficit value,fc θ is the soil field capacity,θis the soil moisture content,RD is the root zone depth.According to the study we found that the use of modern surface irrigation technology such as sprinkler irrigation, micro spray irrigation and pressure irrigation system, can improve the utilization rate of water to 95%, better than common ground water saving irrigation mode, more than 1/2 ~ 2/3 of water-saving irrigation mode, therefore, advanced water saving technology is very important. 3.2.4.2 Life water saving China is a country with a large population and scarce water , so we should use water more reasonably and effectively.Tiered water pricing modelThe model is for all types of users in certain period to regulate basic water consumption, in the basic consumption, we collect fees by the basic price standard, when actual consumption beyond basic consumption, the beyond part will introduce penalty factor: the more water exceed, the higher punishment rate will be. For actual consumption is less than basic consumption, the user can get additional incentives, encouraging people to save water .[12] Three ladder water price modelWe assume that urban resident’s basic water consumption is 1q , the first stage water price is 1P , the second stage water price is 2P , by analogy, q P is used to express the water price in stage q , model formula is()()⎪⎪⎩⎪⎪⎨⎧−++−+−+=−)(11211121111m m q q q p q q p q p q q p q p q p p L L L (14) From the equation (14), that in the tiered water pricing system, as more price levers are divided, it will be more able to reflect the city water supply’s public property and public welfare, be much beneficial to motive users to save water . On the other hand, much more price levers will be bound to increase the transaction cost of both the water supplier and the water user . Seeing from practical application effects of the current step water price model , Three ladder water price model much meets the actual functional requirements of urban water supply system in our country, the specific pricing method see Figure 9.Figure 9 Taking three step level water price model, can to some extent, Contain people waste the limited water resources , promote enterprises into taking all kinds of advanced technologies to improve the Comprehensive utilization of water resources, and realize the goal of urban water conservation and limited water resources Sustainable and high-efficiency using and saving. In conclude, it’s an effective and feasible strategy at present.Cost-Benefit Analysis of water-saving society construction1. Cost-Benefit Analyses ModelThe benefit of the water-saving society construction n s B B B −= (15) :s B water use benefit of the whole society in Water-saving condition。
- 1、下载文档前请自行甄别文档内容的完整性,平台不提供额外的编辑、内容补充、找答案等附加服务。
- 2、"仅部分预览"的文档,不可在线预览部分如存在完整性等问题,可反馈申请退款(可完整预览的文档不适用该条件!)。
- 3、如文档侵犯您的权益,请联系客服反馈,我们会尽快为您处理(人工客服工作时间:9:00-18:30)。
For office use only T1 ________________ T2 ________________ T3 ________________ T4 ________________ Team Control Number11111Problem ChosenABCDFor office use onlyF1 ________________F2 ________________F3 ________________F4 ________________ 2015Mathematical Contest in Modeling (MCM/ICM) Summary Sheet In order to evaluate the performance of a coach, we describe metrics in five aspects:historical record, game gold content, playoff performance, honors and contribution to the sports. Moreover, each aspect is subdivided into several secondary metrics. Take playoff performance as example, we collect postseason result (Sweet Sixteen, Final Four, etc.) per year from NCAA official website, Wikimedia and so on.First, ****grade.To eval*** , in turn, are John Wooden, Mike Krzyzewski, Adolph Rupp, Dean Smith and Bob Knight.Time line horizon does make a difference. According to turning points in NCAA history, we divide the previous century into six periods with different time weights which lead to the change of ranking.We conduct sensitivity analysis on FSE to find best membership function and calculation rule. Sensitivity analysis on aggregation weight is also performed. It proves AM performs better than single model. As a creative use, top 3 presidents(U.S.) are picked out: Abraham Lincoln, George Washington, Franklin D. Roosevelt.At last, the strength and weakness of our mode are discussed, non-technical explanation is presented and the future work is pointed as well.Key words: Ebola virus disease; Epidemiology; West Africa; ******ContentsI. Introduction (2)1.1 (2)1.2 (2)1.3 (2)1.4 (2)1.5 (2)1.6 (2)II. The Description of the Problem (2)2.1 How do we approximate the whole course of paying toll? (2)2.2 How do we define the optimal configuration? (2)2.3 The local optimization and the overall optimization (3)2.4 The differences in weights and sizes of vehicles (3)2.5 What if there is no data available? (3)III. Models (3)3.1 Basic Model (3)3.1.1 Terms, Definitions and Symbols (3)3.1.2 Assumptions (3)3.1.3 The Foundation of Model (4)3.1.4 Solution and Result (4)3.1.5 Analysis of the Result (4)3.1.6 Strength and Weakness (4)3.2 Improved Model (4)3.2.1 Extra Symbols (5)3.2.2 Additional Assumptions (5)3.2.3 The Foundation of Model (5)3.2.4 Solution and Result (5)3.2.5 Analysis of the Result (6)3.2.6 Strength and Weakness (6)IV. Conclusions (6)4.1 Conclusions of the problem (6)4.2 Methods used in our models (6)4.3 Applications of our models (6)V. Future Work (6)5.1 Another model (6)5.1.1 The limitations of queuing theory (6)5.1.2 (7)5.1.3 (7)5.1.4 (7)5.2 Another layout of toll plaza (7)5.3 The newly- adopted charging methods (7)VI. References (8)VII. Appendix (8)I. IntroductionIn order to indicate the origin of the toll way problems, the following background is worth mentioning.1.11.21.31.41.51.6II. The Description of the Problem2.1 How do we approximate the whole course of paying toll?●●●●2.2 How do we define the optimal configuration?1) From the perspective of motorist:2) From the perspective of the toll plaza:3) Compromise:2.3 The local optimization and the overall optimization●●●Virtually:2.4 The differences in weights and sizes of vehicles2.5 What if there is no data available?III. Models3.1 Basic Model3.1.1 Terms, Definitions and SymbolsThe signs and definitions are mostly generated from queuing theory.●●●●●3.1.2 Assumptions●●●●●3.1.3 The Foundation of Model1) The utility function●The cost of toll plaza:●The loss of motorist:●The weight of each aspect:●Compromise:2) The integer programmingAccording to queuing theory, we can calculate the statistical properties as follows.3)The overall optimization and the local optimization●The overall optimization:●The local optimization:●The optimal number of tollbooths:3.1.4 Solution and Result1) The solution of the integer programming:2) Results:3.1.5 Analysis of the Result●Local optimization and overall optimization:●Sensitivity: The result is quite sensitive to the change of the threeparameters●Trend:●Comparison:3.1.6 Strength and Weakness●Strength: In despite of this, the model has proved that . Moreover,we have drawn some useful conclusions about . The model is fit for,such as●Weakness: This model just applies to . As we have stated, . That’sjust what we should do in the improved model.3.2 Improved Model3.2.1 Extra SymbolsSigns and definitions indicated above are still valid. Here are some extra signs and definitions.●●●●3.2.2 Additional Assumptions●●●Assumptions concerning the anterior process are the same as the Basic Model.3.2.3 The Foundation of Model1) How do we determine the optimal number?As we have concluded from the Basic Model,3.2.4 Solution and Result1) Simulation algorithmBased on the analysis above, we design our simulation arithmetic as follows.●Step1:●Step2:●Step3:●Step4:●Step5:●Step6:●Step7:●Step8:●Step9:2) Flow chartThe figure below is the flow chart of the simulation.3) Solution3.2.5 Analysis of the Result3.2.6 Strength and Weakness●Strength: The Improved Model aims to make up for the neglect of .The result seems to declare that this model is more reasonable than the Basic Model and much more effective than the existing design.●Weakness: . Thus the model is still an approximate on a large scale.This has doomed to limit the applications of it.IV. Conclusions4.1 Conclusions of the problem●●●4.2 Methods used in our models●●●4.3 Applications of our models●●●V. Future Work5.1 Another model5.1.1 The limitations of queuing theory5.1.25.1.35.1.41)●●●●2)●●●3)●●●4)5.2 Another layout of toll plaza5.3 The newly- adopted charging methodsVI. References[1][2][3][4]VII. Appendix欢迎您的下载,资料仅供参考!致力为企业和个人提供合同协议,策划案计划书,学习资料等等打造全网一站式需求。