随机微分方程在数理金融中的应用硕士学位
随机微分方程在数理金融中的应用硕士学位论文 精品
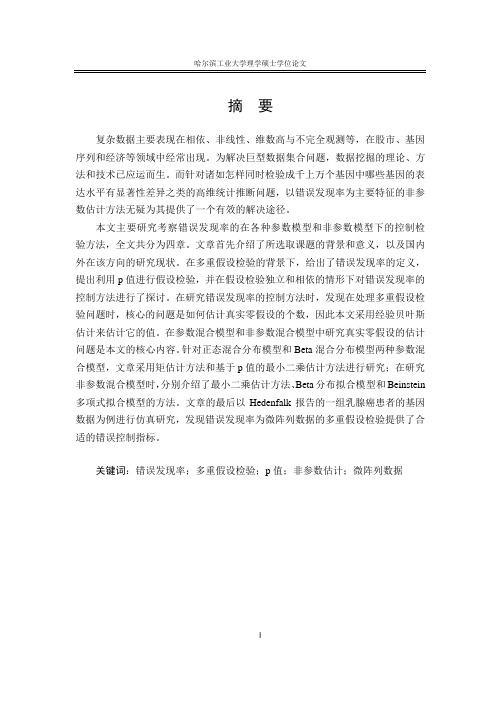
摘要复杂数据主要表现在相依、非线性、维数高与不完全观测等,在股市、基因序列和经济等领域中经常出现。
为解决巨型数据集合问题,数据挖掘的理论、方法和技术已应运而生。
而针对诸如怎样同时检验成千上万个基因中哪些基因的表达水平有显著性差异之类的高维统计推断问题,以错误发现率为主要特征的非参数估计方法无疑为其提供了一个有效的解决途径。
本文主要研究考察错误发现率的在各种参数模型和非参数模型下的控制检验方法,全文共分为四章。
文章首先介绍了所选取课题的背景和意义,以及国内外在该方向的研究现状。
在多重假设检验的背景下,给出了错误发现率的定义,提出利用p值进行假设检验,并在假设检验独立和相依的情形下对错误发现率的控制方法进行了探讨。
在研究错误发现率的控制方法时,发现在处理多重假设检验问题时,核心的问题是如何估计真实零假设的个数,因此本文采用经验贝叶斯估计来估计它的值。
在参数混合模型和非参数混合模型中研究真实零假设的估计问题是本文的核心内容。
针对正态混合分布模型和Beta混合分布模型两种参数混合模型,文章采用矩估计方法和基于p值的最小二乘估计方法进行研究;在研究非参数混合模型时,分别介绍了最小二乘估计方法、Beta分布拟合模型和Beinstein 多项式拟合模型的方法。
文章的最后以Hedenfalk报告的一组乳腺癌患者的基因数据为例进行仿真研究,发现错误发现率为微阵列数据的多重假设检验提供了合适的错误控制指标。
关键词:错误发现率;多重假设检验;p值;非参数估计;微阵列数据- I -AbstractComplex data always appear in the stock market, gene sequences, economic and other fields, which mainly show the characteristic of dependent, nonlinear, high dimension and incomplete observations. In order to solve the problem of huge data collection, the theories, methods and techniques of data mining are proposed. While how to examine the high-dimensional statistical inference problem, such as the significant differences of expression levels in thousands of genes, the non-parametric estimation of false discovery rate provide an effective solution.This paper mainly investigate the test method based on the false discovery rate of various parametric model and non-parametric model, which is divided into four chapters. Firstly, this paper introduce the background and significance of the topic, and the current studies in this direction at home and abroad. Under the background of multiple hypotheses testing, the paper describe the definition of the false discovery rate, propose using the p-value to test the hypothesis testing, and discuss the controlling method of the false discovery rate when the hypotheses testing is independent or dependent. When we investigate the controlling method of the false discovery rate and studied the multiple hypothesis testing problem, we find that the central problem is how to estimate the number of true null hypothesis, so this paper use the empirical Bayes estimation to estimate its value. Investigating the estimation of true null hypothesis in the mixing parametric model and non-parametric model is core of the dissertation. Aiming at the mixed normal distribution model and Beta mixture distribution model, This paper use the method of moment estimation and least squares estimation method based on the p-value to estimate its value; On studying thenon-parametric mixture model, the paper introduce the least square estimation method, Beta distribution fitting model method and the Beinstein polynomial fitting model method. Finally, the paper conduct the simulation research based on a group of patients with breast cancer gene data by Hedenfalk, and find that the false discovery rate is able to provide a suitable error control targets for the multiple hypothesis testing of microarray data.Keywords: false discovery rate, multiple hypotheses testing, p-value, non-parametric estimation, microarray data- II -- III -目 录摘 要 ..................................................................................................................... I Abstract ................................................................................................................... I I第1章 绪 论 (1)1.1 课题研究的背景及意义 (1)1.2 国内外在该方向的研究现状 (1)1.2.1 国外对错误发现率的研究现状 (1)1.2.2 国内研究现状 (3)1.3 本文拟研究的主要内容 (3)1.4 创新点 (3)第2章 错误发现率的多重检验方法 (5)2.1 多重假设检验的错误测度 (5)2.2 P 值的定义、性质和计算方法 (6)2.3 独立情形下基于FDR 控制的检验方法 (7)2.4 相依情形下基于FDR 控制的检验方法 (8)2.5 真实零假设的个数0m 或比值0π的估计 (9)2.5.1 -λ估计 (9)2.5.2 经验贝叶斯估计 (11)2.6 本章小结 (12)第3章 参数混合模型和非参数混合模型的估计 (13)3.1 引言 (13)3.2 正态分布混合模型 (13)3.3 Beta 分布混合模型 (17)3.4 非参数混合模型的估计 (21)3.4.1 最小二乘估计 (22)3.4.2 Beta 分布拟合模型 (23)3.4.3 Beinstein 多项式拟合模型 (25)3.5 本章小结 (26)第4章 错误发现率的估计方法的应用 (27)4.1 引言 (27)4.2 微阵列数据实例研究 (27)4.3 本章小结 (28)结论 (30)参考文献 (31)哈尔滨工业大学学位论文原创性声明和使用权限 .......... 错误!未定义书签。
金融数学专业硕士培养方案(优选.)
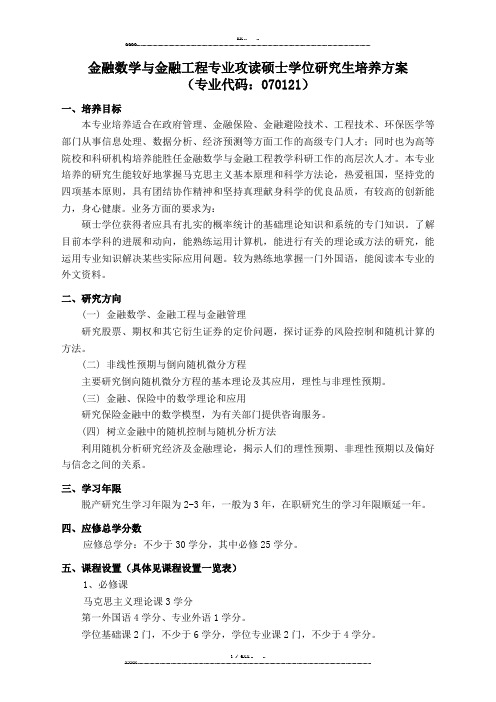
金融数学与金融工程专业攻读硕士学位研究生培养方案(专业代码:070121)一、培养目标本专业培养适合在政府管理、金融保险、金融避险技术、工程技术、环保医学等部门从事信息处理、数据分析、经济预测等方面工作的高级专门人才;同时也为高等院校和科研机构培养能胜任金融数学与金融工程教学科研工作的高层次人才。
本专业培养的研究生能较好地掌握马克思主义基本原理和科学方法论,热爱祖国,坚持党的四项基本原则,具有团结协作精神和坚持真理献身科学的优良品质,有较高的创新能力,身心健康。
业务方面的要求为:硕士学位获得者应具有扎实的概率统计的基础理论知识和系统的专门知识。
了解目前本学科的进展和动向,能熟练运用计算机,能进行有关的理论或方法的研究,能运用专业知识解决某些实际应用问题。
较为熟练地掌握一门外国语,能阅读本专业的外文资料。
二、研究方向(一)金融数学、金融工程与金融管理研究股票、期权和其它衍生证券的定价问题,探讨证券的风险控制和随机计算的方法。
(二)非线性预期与倒向随机微分方程主要研究倒向随机微分方程的基本理论及其应用,理性与非理性预期。
(三)金融、保险中的数学理论和应用研究保险金融中的数学模型,为有关部门提供咨询服务。
(四)树立金融中的随机控制与随机分析方法利用随机分析研究经济及金融理论,揭示人们的理性预期、非理性预期以及偏好与信念之间的关系。
三、学习年限脱产研究生学习年限为2-3年,一般为3年,在职研究生的学习年限顺延一年。
四、应修总学分数应修总学分:不少于30学分,其中必修25学分。
五、课程设置(具体见课程设置一览表)1、必修课马克思主义理论课3学分第一外国语4学分、专业外语1学分。
学位基础课2门,不少于6学分,学位专业课2门,不少于4学分。
前沿讲座2学分:①讲座的目的和内容前沿讲座旨在使硕士生了解本学科和本研究方向的重要学术问题、前沿性问题及这些问题的最新研究方法、技术及进展状况,提高学生参与学术研究的兴趣和学术交流能力。
随机过程及其在金融领域中的应用

高等学校教材随机过程及其在金融领域中的应用王 军 王 娟 编著清华大学出版社北京交通大学出版社·北京·内容简介本书主要包括两部分内容:一部分是概率空间、随机过程的基本概念、Poisson过程、更新过程、Markov链、Brown运动、鞅、随机微分方程等;另一部分是数理金融学的基本概念和基本知识、金融领域中的数学模型、期权定价理论、Black桘Scholes公式、随机过程的一些理论在金融领域中的应用等。
本书适用于应用数学、金融(金融工程,金融数学等)、管理科学、经济学,以及高等院校高年级学生与研究生的教学,也可供有关专业技术人员参考。
本书封面贴有清华大学出版社防伪标签,无标签者不得销售。
版权所有,侵权必究。
侵权举报电话:01062782989 13501256678 13801310933 图书在版编目(CIP)数据 随机过程及其在金融领域中的应用/王军,王娟编著.—北京:清华大学出版社;北京交通大学出版社,2007畅4(2009畅7重印) ISBN9787810829571 Ⅰ畅随… Ⅱ畅①王… ②王… Ⅲ畅随机过程应用金融学高等学校教材 Ⅳ畅F830 中国版本图书馆CIP数据核字(2007)第023616号责任编辑:黎 丹 出版发行:清华大学出版社 邮编:100084 电话:01062776969北京交通大学出版社 邮编:100044 电话:01051686414印刷者:北京瑞达方舟印务有限公司经销:全国新华书店开本:185×230 印张:17 字数:378千字版次:2007年4月第1版 2009年7月第2次印刷书号:ISBN9787810829571/F·217印数:4001~6000册 定价:26畅00元本书如有质量问题,请向北京交通大学出版社质监组反映。
对您的意见和批评,我们表示欢迎和感谢。
投诉电话:01051686043,51686008;传真:01062225406;E桘mail:press@bjtu畅edu畅cn。
概率论与数理统计专业攻读硕士学位研究生学术型培养方案专业代码070103

概率论与数理统计专业攻读硕士学位研究生(学术型)培养方案(专业代码:070103)一、培养目标旨在培养我国概率统计领域从事应用研究的专门人才。
具体要求如下:1、掌握当代社会主义优秀理论成果,热爱祖国,遵纪守法,品德高尚,有志于投身社会主义建设事业。
2、具有比较扎实的概率统计学理论基础,在概率统计学某研究方向上有系统的学习与研究,并能够利用概率统计理论解决实际应用问题。
3、掌握一门外国语,并能运用该门外国语比较熟练的阅读本专业的外文资料。
4、具有健康的体格和心理素质。
二、研究方向1、应用概率统计2、倒向随机微分方程与非线性期望3、量化金融与风险度量4、参数与非参数统计三、学习年限全日制硕士研究生的学制为3年,在校学习期限为2-3年。
原则上不提前毕业,对于特别优秀者,最多可提前一年。
提前毕业的硕士研究生除完成培养方案规定的课程外,必须有一篇以上SCI/CSSCI论文发表,并须经学位委员会审核通过。
所取得的科研成果均要求研究生为第一作者,作者单位需为山东大学。
四、培养方式根据宽口径、厚基础的原则,提倡按一级学科培养硕士研究生;充分利用校内外优质教育资源,鼓励研究生进行“三种经历”,实行双导师合作培养。
五、应修满的总学分数应修总学分:30,其中必修24学分(含前沿讲座与社会实践),选修6学分。
六、课程的类别及设置硕士研究生课程分为必修课与选修课两大类。
1.必修课是为达到培养目标要求,保证研究生培养质量而必须学习的课程。
必修课分学位公共课、学位基础课和学位专业课。
学位基础课一般按一级学科进行设置,学位专业课一般按二级学科设置。
选修课必须含有2门专业课。
经学校批准建设的全英语教学课程要纳入培养方案的课程体系中。
(1)思想政治理论,计3学分;(2)第一外国语,计3学分。
由学科开设的专业必修课包括:(1)专业外语,计2学分,学院考核。
重在培养研究生的学术论文外语写作和国际学术交流能力。
如学习2门及以上全英语专业必修课程(各专业培养计划课程表中所标注的全英语课程,是我院全英语系列课程项目建设中的全英语课程,但是否已开始全英语授课,需经学院审核批准后,以授课语言全英语为准),可免修专业外语,并通过申请可直接获得相应学分;(2)学位基础课2门,不少于6学分(测度与概率、高等数理统计引论、泛函分析基础、近世代数引论,四选二);(3)学位专业课2门,不少于6学分(随机过程及其应用、倒向随机微分方程及其应用、线性统计模型理论及其应用,三选二);(4)前沿讲座,计2学分;前沿讲座旨在使研究生熟悉本学科的重要学术理论和前沿性成果,提高硕士研究生参与学术活动的兴趣和学术交流能力。
届金融数学毕的业论文题目
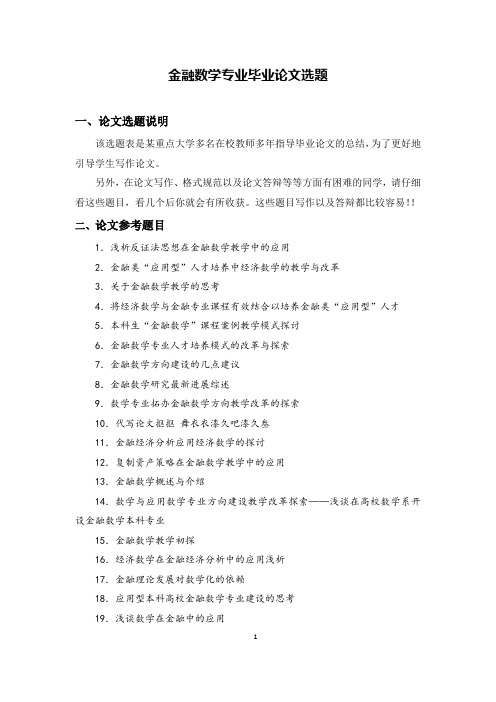
金融数学专业毕业论文选题一、论文选题说明该选题表是某重点大学多名在校教师多年指导毕业论文的总结,为了更好地引导学生写作论文。
另外,在论文写作、格式规范以及论文答辩等等方面有困难的同学,请仔细看这些题目,看几个后你就会有所收获。
这些题目写作以及答辩都比较容易!!二、论文参考题目1.浅析反证法思想在金融数学教学中的应用2.金融类“应用型”人才培养中经济数学的教学与改革3.关于金融数学教学的思考4.将经济数学与金融专业课程有效结合以培养金融类“应用型”人才5.本科生“金融数学”课程案例教学模式探讨6.金融数学专业人才培养模式的改革与探索7.金融数学方向建设的几点建议8.金融数学研究最新进展综述9.数学专业拓办金融数学方向教学改革的探索10.代写论文抠抠舞衣衣漆久吧漆久叁11.金融经济分析应用经济数学的探讨12.复制资产策略在金融数学教学中的应用13.金融数学概述与介绍14.数学与应用数学专业方向建设教学改革探索——浅谈在高校数学系开设金融数学本科专业15.金融数学教学初探16.经济数学在金融经济分析中的应用浅析17.金融理论发展对数学化的依赖18.应用型本科高校金融数学专业建设的思考19.浅谈数学在金融中的应用20.高校金融数学专业建设新探21.金融数学在西部高校的融合式教学发展研究22.金融数学专业“概率论”课程教学例题选题研究23.金融数学专业课程设置与人才培养质量分析24.金融类“应用型”人才培养中经济数学的教学与改革25.金融数学模型26.浅谈金融专业数学教学的改革27.金融类院校开设数学建模课程应解决的几个问题28.案例教学法在金融数学教学中的应用29.金融数学研究综述及其前景展望30.“金融数学”探究式教学的探索与实践31.金融数学金融工程和金融电子化32.浅析金融经济分析中经济数学的应用33.金融数学中的若干前沿问题34.金融数学与金融工程专业介绍及其发展前景35.浅析数学建模教育在金融人才培养中的作用及对策36.针对金融数学专业进行金融工程学课程教学改革的探索37.金融危机中企业受波及的数学模型38.财经院校金融数学高层次人才培养模式研究39.当前行为金融研究中数学建模应用的价值分析40.地方院校金融数学专业(方向)的课程设置41.高校金融数学专业实验课程的设置42.以辩证的观点浅析数学金融研究43.金融数学概述及其展望44.金融数学研究综述与展望45.金融数学概述46.浅谈金融与数学47.金融数学的教学与研究48.浅析数学方法在金融领域的应用49.金融数学:历史与现状50.金融数学教学方法改革的探讨与实践51.以就业为导向的金融数学课程设置与教学改革研究52.对“金融数学”专业人才培养的探索与实践53.金融数学研究前景展望54.金融危机与金融数学55.高校数学系金融数学实验教学模式的探讨56.金融类院校经济数学与现代信息技术深度融合探究57.浅谈数学建模教学与金融人才的培养58.金融中数学模型对实践的影响:过去、现在和未来59.金融数学方向《随机过程》课程建设的研究与实践60.论数学模型在金融领域中的应用61.浅谈数学模型在金融市场中的应用62.论金融经济学的数学化63.比较教学法在金融数学教学中的应用64.金融数学的发展及其在证券投资组合中的应用65.金融数学本科专业教学现状及对策分析66.刍议金融工程与金融数学专业的培养方案67.一类金融数学方程解的适定性研究68.金融数学课程设置与专业建设的一些体会69.数学在金融领域中的适用性和局限性70.金融数学的起源和发展及金融工程简介71.金融数学研究进展与展望72.我国金融数学的发展及前景73.谈如何运用金融数学技巧进行期权定价74.20世纪金融数学的若干进展及前瞻75.金融数学介绍76.结合学科特色的高等数学课程教学改革研究——以金融院校为例77.基于数学模型的金融系统分析研究78.数学金融中的经验与洞察79.我国金融数学教学工作改进分析80.计算机技术在金融数学课程教学中的运用81.数学建模教育与金融人才培养82.金融数学专业会计课程设置及实验教学思考83.金融专科生提高数学素养的思考84.金融数学的研究与进展85.金融数学及金融工程学──公司理财和金融风险防范的高新技术86.金融数学模型概述87.谈谈成人学校金融专业数学教学内容改革88.金融数学引论研究性教学探讨89.向应用型高校转型形势下的本科金融数学专业课程设置初探90.新建地方本科院校应用型金融数学人才培养的思考91.金融数学中两个基于高等数学的证明92.金融数学专业数学分析课程教学探索与实践93.地方高师院校金融数学教学模式初探94.金融数学教学方法的探索与实践95.关于金融数学深入认识的几点思考96.中职学校金融类专业数学选择性教学的实践研究97.应用型本科院校金融数学专业学生培养研究98.地方高师院校金融数学专业实验课程体系建设探索99.对金融数学专业教学改革问题的思考100.金融市场收益率离散数学模型及其定性分析101.对金融数学专业会计教学改革的思考102.成人金融院校数学教学改革初探103.金融对数学方法运用的探讨104.金融数学教育与实用型金融人才的培养105.“第六届全国金融数学与金融工程学科建设与学术研讨会”综述106.金融工程学的数学模型与方法107.非线性数学期望在金融风险中的应用108.论现代金融风险监管体系的数学模型109.数学与现代金融投资理论110.非线性数学期望111.金融数学介绍112.金融定量分析中的数学方法113.金融数学114.关于新升本金融类院校高等数学课程教学方法的研究115.提高数学教学质量适应现代金融事业发展116.西部新建地方本科院校金融数学教学模式初探117.浅谈数学在金融中的应用118.金融类院校经济数学教学现状及对策119.数学建模在现代行为金融研究领域的应用120.论金融风险监管中的数学模型方法121.金融工程学视角下的数学模型与应用122.金融数学发展综述123.应重视金融数学在外汇收支统计分析中的应用124.金融类院校数学建模课程设置的实践研究125.彭实戈:中国金融数学奠基人126.十年来我国金融数学的回顾和前景127.数学金融的分数次Black-Scholes模型及应用128.数学专业拓办统计与金融数学方向的教学改革129.一种借贷关系分析的数学方法和金融风险防范130.数学方法的金融应用初探131.数学建模思想在高职金融数学课程上的应用实践——以房贷按揭问题为例132.金融数学专业课程体系分析133.市场经济体制下金融机制及其数学建模机理的可拓性分析134.金融数学的发展及其在证券投资组合中的应用135.高校教学模式改革的有益探索——兼论金融数学专业实验教学的改革与完善136.数学建模教育与金融学科人才培养137.金融理论研究中的数学方法138.数学方法在金融投资风险分析中的应用139.21世纪应用型人才培养模式研究探索——湖南人文科技学院《应用数学(数理金融)本科专业人才培养计划》解读140.金融数学专业实变函数教学方法探析141.金融风暴下的数学专业142.金融数学本科专业人才培养模式的研究——以新疆财经大学为例143.“3+1”培养模式下《金融数学》课程实践教学改革的研究与实践144.《金融数学》课程对大学人才培养的作用145.金融数学培养方向实验项目资源建设的几点建议146.在《金融数学》教学中培养大学生的学习兴趣147.金融数学课程案例教学的探讨148."金融数学专业设计性实验的教学安排149.数学在经济学研究中的角色:基于金融危机视角的思考150.概率论和金融学的结合——金融数学的现代发展综述151.金融数学的研究与进展152.金融衍生品和信用风险定价的数学模型153.山东大学“金融数学与金融工程基地班”人才培养模式探索154.独立学院数学与应用数学专业(金融证券方向)人才培养研究155.金融危机内在成因的数学建模研究156.案例教学法在金融数学专业数学分析教学中的应用157.地方院校金融数学专业“三模块”课程体系改革的探讨158.基于ADDIE模型的金融工程和金融数学专业实践性教学环节教学模式研究159.第九届全国微分方程暨金融数学学术会议在延边大学召开160.北京师范大学数学科学学院(统计与金融数学系)承办“3+X统计学及其应用Workshop 2011”161.提高金融院校大学生的数学素养是数学教学的根本任务162.金融危机发生时资金运作的数学模型研究163.多媒体技术在金融数学课堂教学中的应用研究164.改革金融数学基础课程解析几何考试模式培养实践能力165.经济类院校经济数学分层次教学改革探讨——以山东轻工业学院财政与金融学院为例166.浅谈金融类院校高等数学分层教学的评价策略167.金融机构社会责任评价的数学模型168.浅谈金融数学169.试论数学分析在金融研究中的作用170.金融投资收益与风险的数学模型及其应用171.金融数学专业高等代数与解析几何教学探讨172.泛系资源泛通论:交通·通信·金融·数学——计算机·网络·智能·科技史新论识173.2007年全国金融数学学术研讨会会议纪要174.基于神经网络的金融相关比率(FIR)数学模型的建立175.期权如何定价?──金融数学拾零176.浅析金融数学模型177.金融类院校中经济数学对学生职业能力培养的研究178.金融数学模型及其非参数估计问题179.风险与回报:银行业中的数学(上)180.中国金融数学的先行者——金融数学领域彭实戈侧记181.金融系统数学模型的机理分析与控制182.金融数学中的欧式期权定价方法183.非线性数学期望,模糊下的最优停时原理及其在金融中的应用184.开展金融数学研究为金融事业决策服务185.关于地方院校新办金融数学专业课程体系构建的思考——以乐山师范学院为例186.金融工程:久期模型及其数学分析187.基于金融数学模型方法的电力衍生产品的定价研究188.国际金融法研究的切入点与数学方法189.期权类衍生金融工具的多期二项式定价数学模型190.非线性数学期望及其在金融中的应用191.谈金融专业学校数学教学的改革192.金融数学拓荒人——记著名金融数学家、山东大学数学研究所所长彭实戈教授193.非线性数学期望的性质及其在金融风险中的应用194.大数据时代金融专业数学的发展趋势195.浅议金融工作者数学素养的培育196.企业受金融危机影响的数学模型197.破产理论研究及其在金融数学中的应用198.数学在21世纪的金融中必将发挥更大的作用199.开展金融数学金融工程和金融管理研究200.金融经济学中的组合数学问题201.在金融危机中企业受波击的数学模型202.转变点在经济、金融、计量经济学中的数学建模203.卓越金融本科人才指标体系构建与评估——运用模糊数学的方法204.金融危机中企业受波及的数学模型的定性分析205.金融数学的崛起206.金融数学本科生多元统计分析课程教学的改革与实践207.Brown运动首达时在金融数学中的应用208.经济与金融中的“数学显微镜”209.基于数学规划模型的金融资源配置测算分析210.浅谈影响新建本科人才培养与有效教学的主要因素——以哈尔滨金融学院数学教学为例211.评《金融衍生产品定价的数学模型与案例分析》212.浅谈数学在金融领域的发展及应用213.基于正规金融信贷选择的一个数学博弈分析214.金融投资类线性规划及其数学模型的MATLAB求解215.马克思主义认识论的数学描述及其在金融经济学中的一个应用216.模糊数学在金融管理中的应用217.金融数学专业概率统计研究性教学的探索218.期权定价—数学在金融行业中的应用浅议219.金融和金融数学研究220.新兴的交叉学科——金融数学221.数学工具处理金融问题222.在金融写作中要注意正确运用数学概念223.最优控制的若干问题及其在金融数学中的应用224.浅谈数学金融学的变革与发展225.浅论数学金融学中关于期权定价的问题226.美国的金融风暴,源自美国失败的数学教育?227.金融控股集团资本金重复计算问题的数学分析228.一个有关咨信公司在金融市场中作用的数学分析229.数学模型在商业银行管理领域中的应用230.Knight不确定金融投资决策与风险度量研究231.“金融大厦”离不开数学支撑232.浅议数学在金融事务专业课程教学的影响与作用233.金融投资中的数学方法234.倒向随机微分方程和金融数学235.芝加哥大学数学系的金融数学学位236."多维球面模型及其在股市分析中的应用——金融数学的新思考237.在金融院校高数教学中运用网络资源的研究238.金融数学第一人——访山东省科学技术最高奖获得者彭实戈239.民族地区金融数学专业常微分方程教学改革与实践240.有趣的金融数学241.金融数学的现在和未来242.金融数学帮您钱生钱243.经济数学与信息技术深度融合探究244.地方高校金融专业教学中数理分析能力的强化与培养245.重视金融数学研究的现实意义246.结合模糊数学与信息扩散法的Logit模型在信用评级中的应用247.金融中的数学——读《数学与金融》248.地方高校金融人才数理分析能力的强化与培养249.连续时间证券投资组合250.彭实戈:中国金融数学第一推动人251.随机理论在连续时间金融市场模型中的应用252.信用风险分类评级数学模型的研究253.非线性数学期望的性质254.等比数列在金融领域中的一个应用255.研究突发事件:数学金融学的重要课题256.当代金融技术发展的趋势257.不相关金融投资收益与风险优化模型探讨258.我国金融危机预警模型的构建与实证研究259.中国“入世”对金融服务业影响的模糊数学模型分析260.有限离散时间金融市场模型261.金融数学中的若干极限定理262.容度极限理论和非线性数学期望在金融中的应用263.港鲁两校在数学领域的合作264.企业金融资产管理数学模型265.金融,也是科学和数学的事业──由1997年诺贝尔经济学奖引发的思考266.投资选择及资产定价数学模型研究267.陕西财经学院1981年硕士研究生入学数学试题(金融专业用)268.陕西财经学院1982年攻读硕士研究生入学数学试题(金融专业用)269.碳排放权交易的实物期权定价方法与数学模型270.开放教育金融专科“经济数学基础”教与学模式271.基于模糊层次分析法的互联网金融风险评估研究272.经济全球化背景下中国银行业税收问题研究273.非线性数字期望274.基于模糊数学中S型隶属函数的风险度量VaR275.股票投资风险管理的数学模型研究276.关于数学系列课程的教学建议277.论经济危机、金融危机的形成原因与遏制278.数学金融学与微分对策(英文)279.关于柱形H-半鞅的算子值随机积分及其在金融上的应用280.数学在经济学研究中的角色:基于金融危机视角的思考281.金融市场预测中数学的使用、误用和滥用282.威尔士斯旺西大学283.基于仓单质押的物流金融风险管理与控制研究284.山西票号金融稽核创新与研究285.金融模拟实验课程的建设与实践286.金融市场风险测量模型—VaR及基于VaR的证券组合选择287.探索数理之美构建艺术化金融教学模式288.基于过度自信的金融市场委托-代理模型研究289.资本监管标准与金融安全机理探讨290.基于经济增长偏好的地方政府金融行为研究291.在经济数学课程中实施参与型教学法的研究292.正倒向随机微分方程的数值方法及其在金融与双曲型方程柯西问题中的应用293.“中国商业经济学会经济数学研究分会第七次年会”综述294.随机利率情况下期权定价问题研究及应用295.分层目标教学法在经济数学教学中的应用296.“摧毁”华尔街的数学公式297.我国农村金融体系协调性及其测度298.PPR数学模型在通胀成因定量分析中的应用299.现代金融理论的进展综述300.浅析数学方法在金融学中的应用301.中国工业化进程中的金融先导战略研究302.复杂适应系统软件平台SWARM在金融体系中的博弈仿真研究303.高师院校数学类各本科专业应用型人才培养的思考304.从股票期权看数学科学305.金融衍生证券定价数值估计的理论分析306.金融专科学校高等数学课内容设置的构想307.基于分形的期权定价及风险价值计算308.静态利率期限结构的数学模型与算法的研究309.基于跳跃——扩散过程的最优消费投资组合问题研究310.金融统计教学的创新与实践311.20世纪经济数学的若干进展312.经济学向何处去——金融危机以来的经济学反思313.数学概率统计在实际生活重要领域的应用314.吉林大学金融学院315.上市金融企业内部控制有效性的研究316.金融经济学的现代进展317.银行业数学化探讨318.一种基于高阶矩的金融危机预测方法319.物流金融业务风险评价方法研究320.采用自学教学法是金融教育必由之路321.数学模型在商业银行管理领域中的应用322.欧式看涨期权定价微分方程的有限差分求解方法323.金融机构专利权质押贷款风险评估研究324.金融工程教学改革的研究与实践325.风险的测度研究──对偶方法326.数理统计与现代金融关系评论327.数字是经济管理的支柱328.用模糊数学评判信用社经营效益的初步研究329.组合投资数学模型发展的研究330.封闭方程组约束下的国际金融琼斯模型331.地方本科大学数学专业人才培养模式的探索332.经济数学教学提高职业能力培养创新人才模式的探究333.中国利率市场化若干问题研究334.金融计划简易概率网络模型335.金融工程学教学方法新探336.伊藤过程理论及其在金融中的应用337.外汇期权定价的数学模型分析338.试用数学方法研究储蓄339.在非线性情形下的一些大偏差结果以及在金融中的应用340.运用模糊数学方法统筹构建货币流通的模型341.试建一个金融资金流向流量优化模型342.金融分析师之路343.分数布朗运动环境下的欧式与美式期权定价研究344.股票价格的期权定价模型345.三中全会后金融改革趋势展望346.一类扩散过程的最优停止347.金融企业内部控制评价体系的思考与实践348.一类基于MATLAB程序的线性规划及数学模型的求解349.浅谈金融学中的数学350.委托-代理关系的数学描述及应用分析351.市场易变性与期权理论定价数学模型的比较352.金融市场化测度与中国金融市场化过程研究353.数学金融学中的期权定价问题354.跳跃点统计检测的小波方法及其在金融汇率中的应用355.进化金融及中国股市实证研究356.信用风险管理应避免滥用数学公式357.具脉冲影响的商品定价决策与金融调控问题的动力学模型研究358.泊松过程理论在地震灾害金融风险管理中的应用359.投资者有限理性与证券价格行为研究360.商业银行小微企业金融服务研究361.期权的定价与应用362.基于JSP技术平台下银行金融信息系统开发风险管理研究363.金融复杂性与中国金融效率364.期权定价理论的起源:巴夏里埃365.股票价格为跳跃扩散过程的期权定价的研究与应用366.证券选择的多元化问题研究367.基于指数方差伽玛模型的金融衍生品定价368.中国金融结构制度变迁及动因分析369.非线性跟踪—微分器在VaR中的应用研究370.中国农村金融供给创新的路径选择371.基于随机微分方程模型的金融时间序列预测的研究372.湖南省农村金融产品持续创新动力评价研究373.中国金融制度的风险机理研究374.基于多Agent模型的连续双向拍卖金融市场仿真实验研究375.经济心理与金融行为376.规范场理论和金融市场模型377.从学科交叉看金融工程学的发展378.首次穿过边界概率及其在金融中的应用(英文)379.分数布朗运动环境下可换债券定价模型380.“金融和保险领域中非线性复杂系统的研究”青年科研创新团队介绍381.群体模型下的金融市场和资产定价研究382.金融衍生产品中美式与亚式期权定价的数值方法研究383.几类奇异期权的风险VaR度量384.Rijndael算法硬件实现的优化设计及应用385.金融发展对城乡居民收入差距的影响386.金融保险中的大偏差问题387.随机控制理论在金融和保险中的应用388.后金融危机时代资源枯竭型城市产业结构与主导产业选择研究389.价差期权定价方法的研究390.电力系统商业化运营优化模式的分析与研究391.分形维数的数学基础及对上海股票市场混沌、分形特性的实证分析392.实际利率法应用中关键数据逻辑关系分析——以应付债券后续计量为例393.经济与金融:最“人文”的经济394.随机微分方程在金融中的若干应用395.金融时间序列隐含模式挖掘方法及其应用研究396.区域金融结构和金融发展理论与实证研究397.非正常金融环境下金融机构的VaR对比研究398.南京港物流发展研究399.我国农村微型金融服务及风险防范研究400.金融泡沫运行与控制研究401.金融混业经营及其风险管理研究402.金融企业应用管理信息系统的绩效评价研究403.甘肃省金融发展规模、结构、效率的协调性测度研究404.我国农村金融供求失衡深层机理研究405.中国政策性金融促进自主创新的有效性研究406.中国农村合作金融制度变迁研究407.中国区域金融协调发展研究408.辽宁城乡金融发展差异对城乡经济增长影响的实证研究409.衍生金融工具风险监控问题探析410.金融危机之信用失衡411.基于西部金融中心建设目标的成都金融人才需求预测研究。
中山大学岭南学院-金融系简介
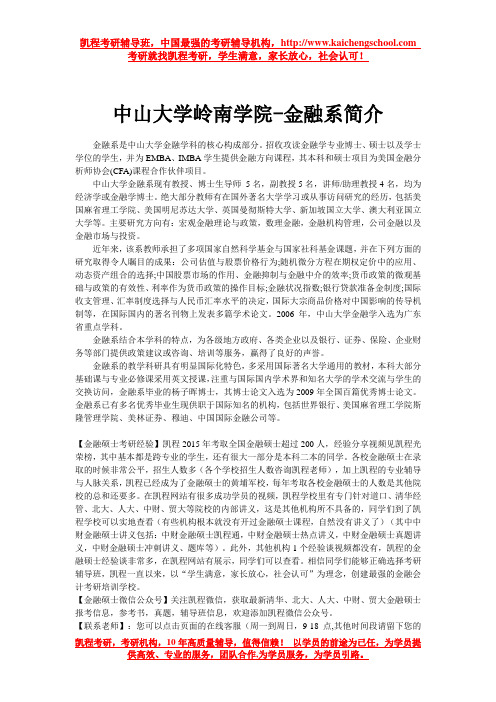
中山大学岭南学院-金融系简介金融系是中山大学金融学科的核心构成部分。
招收攻读金融学专业博士、硕士以及学士学位的学生,并为EMBA、IMBA学生提供金融方向课程,其本科和硕士项目为美国金融分析师协会(CFA)课程合作伙伴项目。
中山大学金融系现有教授、博士生导师5名,副教授5名,讲师/助理教授4名,均为经济学或金融学博士。
绝大部分教师有在国外著名大学学习或从事访问研究的经历,包括美国麻省理工学院、美国明尼苏达大学、英国曼彻斯特大学、新加坡国立大学、澳大利亚国立大学等。
主要研究方向有:宏观金融理论与政策,数理金融,金融机构管理,公司金融以及金融市场与投资。
近年来,该系教师承担了多项国家自然科学基金与国家社科基金课题,并在下列方面的研究取得令人瞩目的成果:公司估值与股票价格行为;随机微分方程在期权定价中的应用、动态资产组合的选择;中国股票市场的作用、金融抑制与金融中介的效率;货币政策的微观基础与政策的有效性、利率作为货币政策的操作目标;金融状况指数;银行贷款准备金制度;国际收支管理、汇率制度选择与人民币汇率水平的决定,国际大宗商品价格对中国影响的传导机制等,在国际国内的著名刊物上发表多篇学术论文。
2006年,中山大学金融学入选为广东省重点学科。
金融系结合本学科的特点,为各级地方政府、各类企业以及银行、证券、保险、企业财务等部门提供政策建议或咨询、培训等服务,赢得了良好的声誉。
金融系的教学科研具有明显国际化特色,多采用国际著名大学通用的教材,本科大部分基础课与专业必修课采用英文授课,注重与国际国内学术界和知名大学的学术交流与学生的交换访问,金融系毕业的杨子晖博士,其博士论文入选为2009年全国百篇优秀博士论文。
金融系已有多名优秀毕业生现供职于国际知名的机构,包括世界银行、美国麻省理工学院斯隆管理学院、美林证券、穆迪、中国国际金融公司等。
【金融硕士考研经验】凯程2015年考取全国金融硕士超过200人,经验分享视频见凯程光荣榜,其中基本都是跨专业的学生,还有很大一部分是本科二本的同学。
随机微分方程在经济学中的应用

§5.3 经济学中的SDE 模型经济学是SDE 的主要应用领域之一.考虑到经济运行中充满了不确定性,而这种不确定性乃是大量随机因素干扰的结果,在对经济过程的数学描述中运用SDE 应当是不可避免的.早在20世纪70年代,著名经济学家Merton 等人就将SDE 应用于经济与金融中,且获得了令人瞩目的结果.近年来,SDE 在经济学中的运用更为普遍与深入.用SDE 构建的经济模型已如此之多,此处根本不可能作什么概括.下面仅介绍三个典型的经济模型,它们在现代动态宏观经济理论中起着基本的作用.用这些模型作为解释SDE 方法的例子,应该说是很具启发性的.考虑到不可避免地要用到一些经济学的术语与记号,下面作一最低限度的介绍.设想我们考虑的是某个独立运行的经济系统,与其相关的经济变量都是随机过程.分别以K,H,A,L,Y 记该经济中物质资本、人力资本、技术、劳动力与产出的总量;以k=K/L 记物质资本的人均量,h=H/L 仿此.假定K,H,A,L,的增长服从如下微分方程:[][]12d d ,d d ,d d d (),d d d (),K H A K K t H H t K H A A t a w t L L n t b w t ψψψ⎧==⎪=+⎨⎪=+⎩5.3.1a 5.3.1b 5.3.1c)()()(其中K ψ与H ψ本别为K 与H 的增长路率,A ψ与n 分别为A 与L 的期望增长率,假定n 是常数.A 与L 的增长分别受到Brown 运动1()w t 与2()w t 的扰动,其扰动强度分别为a b 与,假定12,0,a b w w >与相互独立.对于K 与H 不考虑其自身的扰动.以上所做的种种假设不免有些理想化,但这能使下面建立的模型较为简单.5.3.1 Solow 模型Solow 模型是现代经济增长理论中具有奠基意义的模型,在SDE 形式下,它可表成[]d ()d d (),k sf k k t bk w t μ=-+ 5.3.2)(其中0s >表示储蓄率; ()y f k =是生产函数,假定满足如下条件:()0,()0,()0,()(),0(0)0(),(0)()f k f k f k f k kf k k f f f f ''''>><>>⎧⎨''==∞=∞=∞⎩5.3.3)(222,,,,n b w w n b w μ=-=依式 5.3.1c)(.方程 5.3.2)(的推导并不难:假定d d ,(,)K sY t Y F K L ==是一次齐次的生产函数,记()(,1),f k F k =则d d d d =d 1K K K L L k L L K L L ⎛⎫⎛⎫⎛⎫=-- ⎪ ⎪ ⎪⎝⎭⎝⎭⎝⎭ (用(1.3.31)) 22d d d (1d d )K Yk s t n t b w n t b w L L ⎛⎫=------ ⎪⎝⎭(用(1.3.1c ))2()()d d ()sf k n b t bk w t ⎡⎤=--+⎣⎦ 下面分析方程 5.3.2)(.方程 5.3.2)(显然有零解.其次,因()f k 在(0,)∞内是局部Lipschitz 的,对每个00k >,方程 5.3.2)(必存在局部解0(,)k t k (依定理3.1.4).我们关心的是,0(,)k t k 是否为整体解且保持为正?这由以下定理解决.定理5.3.1 设22b n <,则对任何00k >,方程 5.3.2)(的解是0()(,)k t k t k =定义于[)0,∞上,且a.s.地有()0(0)k t t >∀≥.证 采用定理5.2.2的证法.定义停时{}1inf 0:()(),,l t k t k t l l l τ-⎡⎤=≥∈∈⎣⎦有定义且N.只要证2()0(,0).2,l P t l t b n τ≤→→∞><因故可取0,λ>使得22/(1)b n λ<+.定义()ln (0),V k k k k λλ=->则(0)(),()(1)0.V V V k V +=∞=∞≥>设L 是结合方程5.3.2)(的微分算子,则[]221122()()()(1)2b k V k kksf k k k k λλλλμλ----⎡⎤=--+-+⎣⎦L 22()(1)()()22sf k b sf k b k k k k λλλλλμλμϕ⎡⎤-=---++⎢⎥⎣⎦利用条件 5.3.3)(易算出()()lim(0),lim()0.k k f k f k f f kk→→∞''==∞=∞=其次,用22/(1)b n λ<+得222(1)2(1)0b n b μλλ+-=-+>利用以上事实立得(0)(),ϕϕ=-∞=∞因此必()(0),k c k c ϕ≤∀>是某个正常数.于是0001E (())()E (())d ().l tl V k t V k LV k s sV k ct c ττ∧∧=+≤+⎰另一方面,当l t τ≤时有1(=l k l l τ-)或,于是 11E (())()()(),()(),.l l l c V k t P t V l V l V l V l l ττ--⎡⎤≥∧≥≤∧⎣⎦∧→∞→∞这推出()0()l P t l τ≤→→∞,如所要证.条件22b n <意味着与劳动力的增长比较,劳动力的随机波动是偏小的.定理5.3.1表明,在此条件下,经济一旦启动,就将一直运行下去,绝不会在有限时间内因崩溃或过度膨胀而终止.下面保持22b n <这一条件而分析方程 5.3.2)(的解的渐近状态.我们特别关心的是:能否求出经济增长率的一个下限?试用§2.3中的方法,取Liapunov 函数2()V k k=,今估计γαβ=-(依(2.3.31)):[]2222122()1222()(2)k sf k k b kk bk kk sk f k b μγμ--+⋅⎛⎫=-⎪⎝⎭=-+注意到2d ()()()0d f k kf k f k k k k '-⎡⎤=<⎢⎥⎣⎦, (用(5.3.3)) 即()/f k k 单调下降,有222()inf 2lim(2)(2)2k k f k s b kb b nγγμμ>→∞=-+=-+=-这就得到(参见定理2.3.9):00,k ∀>有120lim ln (,)/2,a.s..t tk t k b n -→∞≥- (5.3.4)近似地可以说,式(5.3.4)表明0(,)k t k 的增长率至少为2/2b n -.因已设22b n <,式(5.3.4)并不能保证0(,)k t k 有正增长,但可保证绝不能有过大的负增长.若撤去条件22b n <,则可能出现三种结局:(ⅰ)在有限时间内经济因0(,)0k t k →而中止; (ⅱ)在有限时间内经济因无界增长而爆炸; (ⅲ)至少以2/2(0)b n -≥为增长率持久地增长.5.3.2 人力资本模型在同时考虑物质资本K 与H 人力资本的情况下,假定经济依据如下生产函数运行:1Y K H Lαβαβ--= (5.3.5)其中0,1/2.αβ<<与solow 模型中的d d K sY t =相对照,现在设12d d ,d d K s Y t H s Y t ==,其中,12,(0,1)s s ∈分别为产出用于物质资本与人力资本投资的份额,于是1d d d d 1s YL L k k t K L L ⎛⎫⎛⎫=--⎪ ⎪⎝⎭⎝⎭ (用(1.3.31)) 1()d d ,s y k t bk μω=-+ (用(1.3.1),(5.3.5))其中222,,d d ,,,y k h n b n b αβμωωω==-=-依式(5.3.1c ).对d h 可求出类似的式子,于是得到关于(,)k h 的如下SDE:12d ()d d (),d ()d d (),k s y k t bk t h s y h t bh t μωμω=-+⎧⎨=-+⎩(5.3.6) 或写成矩阵形式:12d d d ()s y k k k t b t s y h h h μωμ-⎡⎤⎡⎤⎡⎤=+⎢⎥⎢⎥⎢⎥-⎣⎦⎣⎦⎣⎦5.3.6'() 对于方程(5.3.6)有类似于定理5.3.1的以下结果.定理5.3.2 设以下条件满足:22/(2),b n αβ<-∧ (5.3.7)则对任何初值200(,)k h ++∈R ,方程(5.3.6)的解((),())k t h t 定义于区间[)0,∞上,且a.s.地保持为正.证 仍用定理4.6.1的证法.定义停时{}11inf 0:()(),(),l t k t k t l l h t l l τ--⎡⎤⎡⎤=≥∈∈⎣⎦⎣⎦有定义且或, 只要证()0(,0)l P t l t τ≤→→∞>.约定1,1ααββ''=-=-.定义12(,)(ln )(ln )()()V k h kk hh V k V h αβαβ''''=-+-+ ,则12(,)()()V k h V k V h =+L L L2211222221(2)22(2)222(2)2b b k s h s k h b b hs ks k hn b k s h αβαββααβαβααμααααμββμββββμααα''-''-''''''=-++-++'''''-++-++''⎡⎤≤---+⎣⎦222(2)const 2n b h s k βαβββ'''⎡⎤---++⎣⎦ (5.3.8)由条件(5.3.7)推出222(2)0,2(2)0n b n b αβ-->-->由,1/2αβ<推出,ααββ''<<.这就由(5.3.8)得到(,),V k h c c ≤L 是某个正常数.于是000001E ((),()(,)E ((),()d (,)l l l tV k t h t V k h LV k s h s sV k h ct c τττ∧∧∧=+≤+⎰另一方面,当l t τ≤时以下四种情况之一必发生:11(),(),(),()l l l l k l k l h l h lττττ--====于是1111122E ((),()()()()()()()()l l l l c V k t h t P t V l V l V l V l P t U l ττττ--≥∧∧⎡⎤≥≤∧∧∧⎣⎦≤显然()()U l l →∞→∞,故得()0l P t τ≤→,如所要证.类似于solow 模型,若令22(,)V k h k h =+,则22T22221222221222(,)(,)(,)(,)2(,)2()2()()22()22,,0V k h b V k h k h V k h V k h k s y k h s y h b k h bk hk h s k s h b n b n k h k hαβγμμ⎡⎤∇-⎢⎥⎣⎦-+-++=-++=+-≥->+L因此,若(),()k t h t 是方程(5.3.6)的整体解且a.s.保证为正,则1222lim ln ()()2,a.s.t tk t h t b n -→∞⎡⎤+≥-⎣⎦(5.3.9)于是得出经济可能的三种结局是:(ⅰ)因在有限时间内()k t 或()h t 趋于零而中止;(ⅱ)在有限时间内()k t 或()h t 无界增长; (ⅲ)经济持久地运行且增长率不低于22b n -.若条件(5.3.7)满足,则仅有情况(ⅲ)出现,以上事实与solow 模型非常类似.5.3.3 R &D 模型前面两个模型都不涉及技术因素,因而不能解释技术进步对于经济增长的作用.今考虑将技术水平A 纳入模型之内.设A 依式(5.3.1b)增长,1,()AA Y C K AL Y C KAL ξθηβαψ--==, (5.3.10)其中,,,,,,A Y C C ξθηαβ均为正参数.A ψ的表达式表明,技术增长率A ψ与物质资本及劳动力的投入正相关,而与现有技术水平负相关;后者意味着,技术水平愈高,其增长就愈不容易.为记号简单起见,约定A x ψ=,K y ψ=且设(/)K s Y K ψ=.于是22212d d d d (1)d (1)d 22(1)d d d d d d d 2()d d +d x K A L K A x K A LK A L K A K L A L L K A K L A L p x y t a w b w ξξθθξθηηηξθξηθηθξθη-+⎛⎫⎛⎫=-+-+ ⎪ ⎪⎝⎭⎝⎭-⎛⎫--+- ⎪⎝⎭=-+- (用(1.3.28))其中221(1)(1)2p n a b ηθθηη⎡⎤=++--⎣⎦. (5.3.11a)类似地求出1d y y -,因而得到关于(,)x y 的如下SDE:[][]1212d ()d d +d d ()d d +d x x p x y t a w b w y y q x y t a w b w θξθηαβαα⎧=-+-⎪⎨=+--⎪⎩ (5.3.12) 其中22(1)()2q n a b ααα-=-+ (5.3.11b)(5.3.12)可写成如下矩阵形式:12d d diag (,)d d w x p x a b x y t w y q y a b θξθηαβαα⎧⎫⎛⎫--⎡⎤⎡⎤⎡⎤⎡⎤⎡⎤⎡⎤⎪⎪=++⎨⎪⎬⎢⎥⎢⎥⎢⎥⎢⎥⎢⎥⎢⎥⎣⎦⎣⎦⎣⎦⎣⎦⎣⎦⎣⎦⎪⎪⎝⎭⎩⎭,这正是在§5.2中讨论过的Lotka-Volterra 系统.由(5.3.12)表示的模型就是随机形式下的R &D 模型,它是现代经济增长理论中的主要模型之一. 鉴于R &D 模型是一个LV 系统,此处正是应用上节结果的地方.虽然方程(5.3.12)的扰动部分与(5.2.41)略有不同,但仍可应用定理5.2.13得到定理5.3.3 设以下条件满足:,ξθαβ<<, (5.3.13)则对任何初值200(,)x y ++∈R ,方程(5.3.12)存在唯一以00(,)x y 为初值的正整体解((),())x t y t ,且对任何0p >成立22limE ()()pt x t y t K →∞⎡⎤+≤⎣⎦ (5.3.14) 其中K 是与初值无关(但可能与p 有关)的正常数.特别,()x t 与()y t 都是最终一致均方有界的.直观上,定理5.3.3意味着在条件(5.3.13)之下,经济一旦启动,则无论资本与技术均将持久地保持正增长.至于条件(5.3.13)的直观意义,粗略地说就是:高的技术水平对于技术继续增长的负面影响明显地强于资本对技术进步的正面影响,而生产规模收益递降(11)βα-+<.这些因素都对经济的爆发性扩张起抑制作用.一般认为,条件(5.3.13)是现实合理的.命题5.2.8亦可用于方程(5.3.12),得出如下渐近轨道估计结果. 定理5.3.4 设存在常数12,0c c >,使得矩阵11212222c c c c c c θξαξαβ-+⎡⎤⎢⎥+-⎣⎦负定,即212124()c c c c βθξα>+. (5.3.15)则对任何200(,)x y ++∈R ,方程(5.3.12)存在唯一以00(,)x y 为初值的正整体解((),())x t y t ,且满足22()()lim0ln t x t y t t→∞+≤,a.s.. (5.3.16)若取121c c ==,则条件(5.3.15)简化为24()βθαξ>+ (5.3.17)条件(5.3.13)与(5.3.17)并不可相互比较.不过容易看出,满足条件(5.3.17)的参数组(,,,)αβξθ甚多,其中具有现实意义者亦不少.。
数学一级学科研究生课程设置一览表
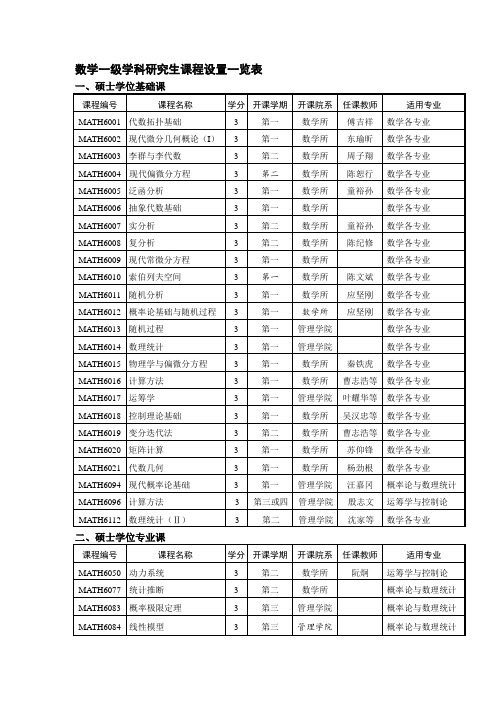
四、硕士跨一级学科课程:物理学、力学、应用经济学等一级学科课程。 硕士跨一级学科课程: 五、博士学位专业课
课程编号 MATH8029 MATH8030 MATH8031 MATH8032 MATH8033 MATH8034 MATH8035 MATH8036 课程名称 现代统计方法 随机过程极限定理 过程统计 分布参数系统最优 控制理论 最优化理论 微分对策理论 数学金融学 变分分析 3 3 3 3 3 3 学分 开课学期 3 3 3 第二 第二 第二 第二 第一 第一 第二 第二 第一 开课院系 管理学院 管理学院 管理学院 数学所 管理学院 数学所 数学所 管理学院 管理学院 任课教师 适用专业 概率论与数理统计 概率论与数理统计 概率论与数理统计 雍炯敏等 运筹学与控制论 朱道立 运筹学与控制论
雍炯敏等 运筹学与控制论 雍炯敏等 运筹学与控制论 朱道立 芮明杰等 运筹学与控制论 概率论与数理统计、 运筹学与控制论
MANA8028 管理学进展
六、博士专业选修课
课程编号 课程名称 学分 开课学期 3 第三 第三 第三 第三 第三 第三 第三 第三至五 第一、二 第一、二 第四或五 第一或二 开课院系 管理学院 管理学院 管理学院 管理学院 数学所 数学所 数学所 数学所 管理学院 管理学院 管理学院 管理学院 赵学雷 任课教师 适用专业 概率论与数理统计 概率论与数理统计 概率论与数理统计 概率论与数理统计 概率论与数理统计 概率论与数理统计 雍炯敏等 运筹学与控制论 导师 朱道立 数学各专业 运筹学与控制论 MATH8067 现代统计方法(续)
第三或四 第四 第三至五 第三至五 第三至五 第三至五 第三至五 第四 第四 第四 第四 第三至五 第四 第四 第四或五
数学所 数学所 数学所 数学所 数学所 数学所 数学所 管理学院 管理学院 管理学院 管理学院 数学所 数学所 数学所 管理学院
金融数学毕业论文题目(698个)之欧阳家百创编
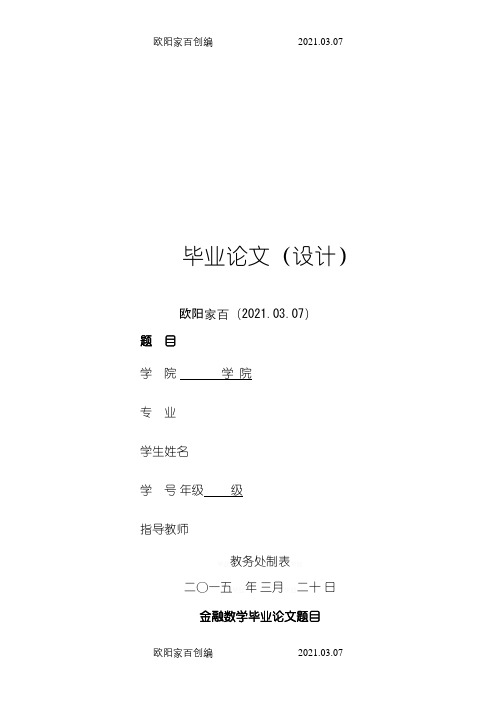
毕业论文(设计)欧阳家百(2021.03.07)题目学院学院专业学生姓名学号年级级指导教师毕业教务处制表毕业二〇一五毕业年三月毕业二十日金融数学毕业论文题目一、论文说明本团队长期从事论文写作与论文发表服务,擅长案例分析、编程仿真、图表绘制、理论分析等,专科本科论文300起,具体信息联系二、论文参考题目浅析反证法思想在金融数学教学中的应用金融类“应用型”人才培养中经济数学的教学与改革关于金融数学教学的思考将经济数学与金融专业课程有效结合以培养金融类“应用型”人才本科生“金融数学”课程案例教学模式探讨金融数学专业人才培养模式的改革与探索金融数学方向建设的几点建议金融数学研究最新进展综述数学专业拓办金融数学方向教学改革的探索新建地方院校金融数学专业本科人才培养探讨金融经济分析应用经济数学的探讨复制资产策略在金融数学教学中的应用金融数学介绍金融数学概述数学与应用数学专业方向建设教学改革探索——浅谈在高校数学系开设金融数学本科专业金融数学教学初探经济数学在金融经济分析中的应用浅析金融理论发展对数学化的依赖应用型本科高校金融数学专业建设的思考浅谈数学在金融中的应用高校金融数学专业建设新探金融数学在西部高校的融合式教学发展研究金融数学专业“概率论”课程教学例题选题研究金融数学专业课程设置与人才培养质量分析金融类“应用型”人才培养中经济数学的教学与改革金融数学模型浅谈金融专业数学教学的改革金融类院校开设数学建模课程应解决的几个问题案例教学法在金融数学教学中的应用金融数学研究综述及其前景展望“金融数学”探究式教学的探索与实践金融数学金融工程和金融电子化浅析金融经济分析中经济数学的应用金融数学中的若干前沿问题金融数学与金融工程专业介绍及其发展前景浅析数学建模教育在金融人才培养中的作用及对策针对金融数学专业进行金融工程学课程教学改革的探索金融危机中企业受波及的数学模型金融数学财经院校金融数学高层次人才培养模式研究当前行为金融研究中数学建模应用的价值分析地方院校金融数学专业(方向)的课程设置高校金融数学专业实验课程的设置以辩证的观点浅析数学金融研究金融数学概述及其展望金融数学研究综述与展望金融数学概述浅谈金融与数学金融数学的教学与研究浅析数学方法在金融领域的应用金融数学:历史与现状金融数学教学方法改革的探讨与实践以就业为导向的金融数学课程设置与教学改革研究对“金融数学”专业人才培养的探索与实践金融数学研究前景展望金融危机与金融数学高校数学系金融数学实验教学模式的探讨金融类院校经济数学与现代信息技术深度融合探究浅谈数学建模教学与金融人才的培养金融中数学模型对实践的影响:过去、现在和未来金融数学方向《随机过程》课程建设的研究与实践论数学模型在金融领域中的应用浅谈数学模型在金融市场中的应用论金融经济学的数学化比较教学法在金融数学教学中的应用金融数学的发展及其在证券投资组合中的应用金融数学本科专业教学现状及对策分析刍议金融工程与金融数学专业的培养方案一类金融数学方程解的适定性研究金融数学课程设置与专业建设的一些体会数学在金融领域中的适用性和局限性金融数学的起源和发展及金融工程简介金融数学研究进展与展望我国金融数学的发展及前景谈如何运用金融数学技巧进行期权定价20世纪金融数学的若干进展及前瞻金融数学介绍结合学科特色的高等数学课程教学改革研究——以金融院校为例基于数学模型的金融系统分析研究数学金融中的经验与洞察我国金融数学教学工作改进分析计算机技术在金融数学课程教学中的运用数学建模教育与金融人才培养金融数学专业会计课程设置及实验教学思考金融专科生提高数学素养的思考金融数学的研究与进展金融数学及金融工程学──公司理财和金融风险防范的高新技术金融数学模型概述谈谈成人学校金融专业数学教学内容改革金融数学引论研究性教学探讨向应用型高校转型形势下的本科金融数学专业课程设置初探新建地方本科院校应用型金融数学人才培养的思考金融数学中两个基于高等数学的证明金融数学专业数学分析课程教学探索与实践地方高师院校金融数学教学模式初探金融数学教学方法的探索与实践关于金融数学深入认识的几点思考中职学校金融类专业数学选择性教学的实践研究应用型本科院校金融数学专业学生培养研究地方高师院校金融数学专业实验课程体系建设探索对金融数学专业教学改革问题的思考金融市场收益率离散数学模型及其定性分析对金融数学专业会计教学改革的思考成人金融院校数学教学改革初探金融对数学方法运用的探讨金融数学教育与实用型金融人才的培养“第六届全国金融数学与金融工程学科建设与学术研讨会”综述金融工程学的数学模型与方法非线性数学期望在金融风险中的应用论现代金融风险监管体系的数学模型数学与现代金融投资理论非线性数学期望金融数学介绍金融定量分析中的数学方法金融数学关于新升本金融类院校高等数学课程教学方法的研究提高数学教学质量适应现代金融事业发展西部新建地方本科院校金融数学教学模式初探浅谈数学在金融中的应用金融类院校经济数学教学现状及对策数学建模在现代行为金融研究领域的应用论金融风险监管中的数学模型方法金融工程学视角下的数学模型与应用金融数学发展综述应重视金融数学在外汇收支统计分析中的应用金融类院校数学建模课程设置的实践研究彭实戈:中国金融数学奠基人十年来我国金融数学的回顾和前景数学金融的分数次Black-Scholes模型及应用数学专业拓办统计与金融数学方向的教学改革一种借贷关系分析的数学方法和金融风险防范数学方法的金融应用初探数学建模思想在高职金融数学课程上的应用实践——以房贷按揭问题为例金融数学专业课程体系分析市场经济体制下金融机制及其数学建模机理的可拓性分析金融数学的发展及其在证券投资组合中的应用高校教学模式改革的有益探索——兼论金融数学专业实验教学的改革与完善数学建模教育与金融学科人才培养金融理论研究中的数学方法数学方法在金融投资风险分析中的应用21世纪应用型人才培养模式研究探索——湖南人文科技学院《应用数学(数理金融)本科专业人才培养计划》解读金融数学专业实变函数教学方法探析金融风暴下的数学专业金融数学本科专业人才培养模式的研究——以新疆财经大学为例“3+1”培养模式下《金融数学》课程实践教学改革的研究与实践《金融数学》课程对大学人才培养的作用金融数学培养方向实验项目资源建设的几点建议在《金融数学》教学中培养大学生的学习兴趣金融数学课程案例教学的探讨"金融数学专业设计性实验的教学安排数学在经济学研究中的角色:基于金融危机视角的思考概率论和金融学的结合——金融数学的现代发展综述金融数学的研究与进展金融衍生品和信用风险定价的数学模型山东大学“金融数学与金融工程基地班”人才培养模式探索独立学院数学与应用数学专业(金融证券方向)人才培养研究金融危机内在成因的数学建模研究案例教学法在金融数学专业数学分析教学中的应用地方院校金融数学专业“三模块”课程体系改革的探讨基于ADDIE模型的金融工程和金融数学专业实践性教学环节教学模式研究第九届全国微分方程暨金融数学学术会议在延边大学召开北京师范大学数学科学学院(统计与金融数学系)承办“3+X统计学及其应用Workshop 2011”提高金融院校大学生的数学素养是数学教学的根本任务<ahref=""/yxdetail.aspx?filename=PPTT20150 6020AQ&dbname=CAPJ2015"" target=""_blank"">向应用型高校转型形势下的本科金融数学专业课程设置初探"金融危机发生时资金运作的数学模型研究多媒体技术在金融数学课堂教学中的应用研究改革金融数学基础课程解析几何考试模式培养实践能力经济类院校经济数学分层次教学改革探讨——以山东轻工业学院财政与金融学院为例浅谈金融类院校高等数学分层教学的评价策略金融机构社会责任评价的数学模型浅谈金融数学试论数学分析在金融研究中的作用金融投资收益与风险的数学模型及其应用金融数学专业高等代数与解析几何教学探讨泛系资源泛通论:交通·通信·金融·数学——计算机·网络·智能·科技史新论识2007年全国金融数学学术研讨会会议纪要基于神经网络的金融相关比率(FIR)数学模型的建立期权如何定价?──金融数学拾零浅析金融数学模型金融类院校中经济数学对学生职业能力培养的研究金融数学模型及其非参数估计问题风险与回报:银行业中的数学(上)中国金融数学的先行者——金融数学领域彭实戈侧记金融系统数学模型的机理分析与控制金融数学中的欧式期权定价方法非线性数学期望,模糊下的最优停时原理及其在金融中的应用开展金融数学研究为金融事业决策服务关于地方院校新办金融数学专业课程体系构建的思考——以乐山师范学院为例金融工程:久期模型及其数学分析基于金融数学模型方法的电力衍生产品的定价研究国际金融法研究的切入点与数学方法期权类衍生金融工具的多期二项式定价数学模型非线性数学期望及其在金融中的应用谈金融专业学校数学教学的改革金融数学拓荒人——记著名金融数学家、山东大学数学研究所所长彭实戈教授非线性数学期望的性质及其在金融风险中的应用大数据时代金融专业数学的发展趋势浅议金融工作者数学素养的培育企业受金融危机影响的数学模型破产理论研究及其在金融数学中的应用数学在21世纪的金融中必将发挥更大的作用开展金融数学金融工程和金融管理研究金融经济学中的组合数学问题在金融危机中企业受波击的数学模型转变点在经济、金融、计量经济学中的数学建模卓越金融本科人才指标体系构建与评估——运用模糊数学的方法金融危机中企业受波及的数学模型的定性分析金融数学的崛起金融数学本科生多元统计分析课程教学的改革与实践Brown运动首达时在金融数学中的应用经济与金融中的“数学显微镜”基于数学规划模型的金融资源配置测算分析浅谈影响新建本科人才培养与有效教学的主要因素——以哈尔滨金融学院数学教学为例评《金融衍生产品定价的数学模型与案例分析》浅谈数学在金融领域的发展及应用基于正规金融信贷选择的一个数学博弈分析金融投资类线性规划及其数学模型的MATLAB求解马克思主义认识论的数学描述及其在金融经济学中的一个应用模糊数学在金融管理中的应用金融数学专业概率统计研究性教学的探索期权定价—数学在金融行业中的应用浅议金融和金融数学研究新兴的交叉学科——金融数学数学工具处理金融问题在金融写作中要注意正确运用数学概念最优控制的若干问题及其在金融数学中的应用浅谈数学金融学的变革与发展浅论数学金融学中关于期权定价的问题美国的金融风暴,源自美国失败的数学教育?金融控股集团资本金重复计算问题的数学分析一个有关咨信公司在金融市场中作用的数学分析数学模型在商业银行管理领域中的应用Knight不确定金融投资决策与风险度量研究“金融大厦”离不开数学支撑浅议数学在金融事务专业课程教学的影响与作用金融投资中的数学方法倒向随机微分方程和金融数学芝加哥大学数学系的金融数学学位"多维球面模型及其在股市分析中的应用——金融数学的新思考在金融院校高数教学中运用网络资源的研究金融数学第一人——访山东省科学技术最高奖获得者彭实戈民族地区金融数学专业常微分方程教学改革与实践有趣的金融数学金融数学的现在和未来金融数学帮您钱生钱经济数学与信息技术深度融合探究地方高校金融专业教学中数理分析能力的强化与培养重视金融数学研究的现实意义结合模糊数学与信息扩散法的Logit模型在信用评级中的应用金融中的数学——读《数学与金融》地方高校金融人才数理分析能力的强化与培养连续时间证券投资组合<ahref=""/yxdetail.aspx?filename=ZXDB2015 060902Q&dbname=CAPJ2015"" target=""_blank"">金融数学专业概率统计研究性教学的探索"彭实戈:中国金融数学第一推动人随机理论在连续时间金融市场模型中的应用信用风险分类评级数学模型的研究非线性数学期望的性质等比数列在金融领域中的一个应用研究突发事件:数学金融学的重要课题当代金融技术发展的趋势不相关金融投资收益与风险优化模型探讨我国金融危机预警模型的构建与实证研究中国“入世”对金融服务业影响的模糊数学模型分析有限离散时间金融市场模型金融数学中的若干极限定理容度极限理论和非线性数学期望在金融中的应用港鲁两校在数学领域的合作企业金融资产管理数学模型金融,也是科学和数学的事业──由1997年诺贝尔经济学奖引发的思考投资选择及资产定价数学模型研究陕西财经学院1981年硕士研究生入学数学试题(金融专业用)陕西财经学院1982年攻读硕士研究生入学数学试题(金融专业用)碳排放权交易的实物期权定价方法与数学模型开放教育金融专科“经济数学基础”教与学模式基于模糊层次分析法的互联网金融风险评估研究经济全球化背景下中国银行业税收问题研究非线性数字期望基于模糊数学中S型隶属函数的风险度量VaR股票投资风险管理的数学模型研究关于数学系列课程的教学建议论经济危机、金融危机的形成原因与遏制数学金融学与微分对策(英文)关于柱形H-半鞅的算子值随机积分及其在金融上的应用数学在经济学研究中的角色:基于金融危机视角的思考金融市场预测中数学的使用、误用和滥用威尔士斯旺西大学基于仓单质押的物流金融风险管理与控制研究山西票号金融稽核创新与研究金融模拟实验课程的建设与实践金融市场风险测量模型—VaR及基于VaR的证券组合选择探索数理之美构建艺术化金融教学模式基于过度自信的金融市场委托-代理模型研究资本监管标准与金融安全机理探讨基于经济增长偏好的地方政府金融行为研究在经济数学课程中实施参与型教学法的研究正倒向随机微分方程的数值方法及其在金融与双曲型方程柯西问题中的应用“中国商业经济学会经济数学研究分会第七次年会”综述随机利率情况下期权定价问题研究及应用分层目标教学法在经济数学教学中的应用“摧毁”华尔街的数学公式我国农村金融体系协调性及其测度PPR数学模型在通胀成因定量分析中的应用现代金融理论的进展综述浅析数学方法在金融学中的应用中国工业化进程中的金融先导战略研究复杂适应系统软件平台SWARM在金融体系中的博弈仿真研究高师院校数学类各本科专业应用型人才培养的思考从股票期权看数学科学金融衍生证券定价数值估计的理论分析金融专科学校高等数学课内容设置的构想基于分形的期权定价及风险价值计算静态利率期限结构的数学模型与算法的研究基于跳跃——扩散过程的最优消费投资组合问题研究金融统计教学的创新与实践20世纪经济数学的若干进展经济学向何处去——金融危机以来的经济学反思数学概率统计在实际生活重要领域的应用吉林大学金融学院上市金融企业内部控制有效性的研究金融经济学的现代进展银行业数学化探讨一种基于高阶矩的金融危机预测方法物流金融业务风险评价方法研究采用自学教学法是金融教育必由之路数学模型在商业银行管理领域中的应用欧式看涨期权定价微分方程的有限差分求解方法金融机构专利权质押贷款风险评估研究金融工程教学改革的研究与实践风险的测度研究──对偶方法数理统计与现代金融关系评论数字是经济管理的支柱用模糊数学评判信用社经营效益的初步研究组合投资数学模型发展的研究封闭方程组约束下的国际金融琼斯模型地方本科大学数学专业人才培养模式的探索经济数学教学提高职业能力培养创新人才模式的探究中国利率市场化若干问题研究金融计划简易概率网络模型金融工程学教学方法新探伊藤过程理论及其在金融中的应用外汇期权定价的数学模型分析试用数学方法研究储蓄在非线性情形下的一些大偏差结果以及在金融中的应用运用模糊数学方法统筹构建货币流通的模型试建一个金融资金流向流量优化模型金融分析师之路分数布朗运动环境下的欧式与美式期权定价研究股票价格的期权定价模型三中全会后金融改革趋势展望一类扩散过程的最优停止金融企业内部控制评价体系的思考与实践一类基于MATLAB程序的线性规划及数学模型的求解浅谈金融学中的数学委托-代理关系的数学描述及应用分析市场易变性与期权理论定价数学模型的比较金融市场化测度与中国金融市场化过程研究数学金融学中的期权定价问题跳跃点统计检测的小波方法及其在金融汇率中的应用进化金融及中国股市实证研究信用风险管理应避免滥用数学公式具脉冲影响的商品定价决策与金融调控问题的动力学模型研究泊松过程理论在地震灾害金融风险管理中的应用投资者有限理性与证券价格行为研究商业银行小微企业金融服务研究期权的定价与应用基于JSP技术平台下银行金融信息系统开发风险管理研究金融复杂性与中国金融效率期权定价理论的起源:巴夏里埃股票价格为跳跃扩散过程的期权定价的研究与应用证券选择的多元化问题研究基于指数方差伽玛模型的金融衍生品定价中国金融结构制度变迁及动因分析非线性跟踪—微分器在VaR中的应用研究中国农村金融供给创新的路径选择基于随机微分方程模型的金融时间序列预测的研究湖南省农村金融产品持续创新动力评价研究中国金融制度的风险机理研究基于多Agent模型的连续双向拍卖金融市场仿真实验研究经济心理与金融行为规范场理论和金融市场模型从学科交叉看金融工程学的发展首次穿过边界概率及其在金融中的应用(英文)分数布朗运动环境下可换债券定价模型“金融和保险领域中非线性复杂系统的研究”青年科研创新团队介绍群体模型下的金融市场和资产定价研究金融衍生产品中美式与亚式期权定价的数值方法研究几类奇异期权的风险VaR度量Rijndael算法硬件实现的优化设计及应用金融发展对城乡居民收入差距的影响金融保险中的大偏差问题随机控制理论在金融和保险中的应用后金融危机时代资源枯竭型城市产业结构与主导产业选择研究价差期权定价方法的研究电力系统商业化运营优化模式的分析与研究分形维数的数学基础及对上海股票市场混沌、分形特性的实证分析实际利率法应用中关键数据逻辑关系分析——以应付债券后续计量为例经济与金融:最“人文”的经济随机微分方程在金融中的若干应用金融时间序列隐含模式挖掘方法及其应用研究区域金融结构和金融发展理论与实证研究非正常金融环境下金融机构的VaR对比研究南京港物流发展研究我国农村微型金融服务及风险防范研究金融泡沫运行与控制研究金融混业经营及其风险管理研究金融企业应用管理信息系统的绩效评价研究甘肃省金融发展规模、结构、效率的协调性测度研究我国农村金融供求失衡深层机理研究中国政策性金融促进自主创新的有效性研究。
专业数理经济专业代码99j2授予理学博士学位.doc
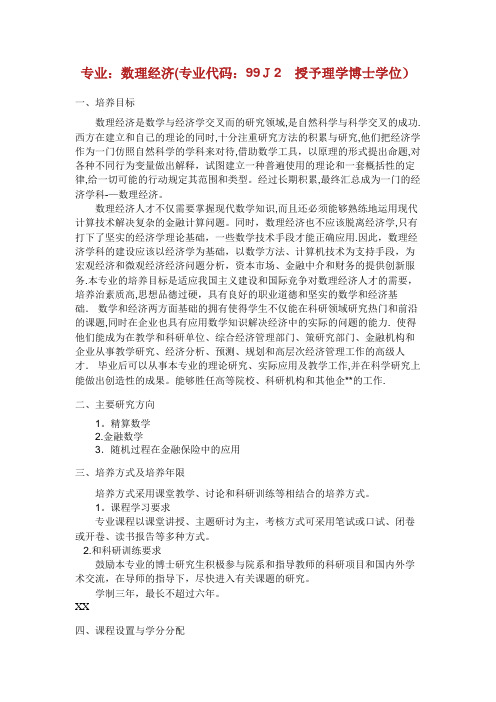
专业:数理经济(专业代码:99J2授予理学博士学位)一、培养目标数理经济是数学与经济学交叉而的研究领域,是自然科学与科学交叉的成功.西方在建立和自己的理论的同时,十分注重研究方法的积累与研究,他们把经济学作为一门仿照自然科学的学科来对待,借助数学工具,以原理的形式提出命题,对各种不同行为变量做出解释,试图建立一种普遍使用的理论和一套概括性的定律,给一切可能的行动规定其范围和类型。
经过长期积累,最终汇总成为一门的经济学科-—数理经济。
数理经济人才不仅需要掌握现代数学知识,而且还必须能够熟练地运用现代计算技术解决复杂的金融计算问题。
同时,数理经济也不应该脱离经济学,只有打下了坚实的经济学理论基础,一些数学技术手段才能正确应用.因此,数理经济学科的建设应该以经济学为基础,以数学方法、计算机技术为支持手段,为宏观经济和微观经济经济问题分析,资本市场、金融中介和财务的提供创新服务.本专业的培养目标是适应我国主义建设和国际竞争对数理经济人才的需要,培养治素质高,思想品德过硬,具有良好的职业道德和坚实的数学和经济基础.数学和经济两方面基础的拥有使得学生不仅能在科研领域研究热门和前沿的课题,同时在企业也具有应用数学知识解决经济中的实际的问题的能力. 使得他们能成为在教学和科研单位、综合经济管理部门、策研究部门、金融机构和企业从事教学研究、经济分析、预测、规划和高层次经济管理工作的高级人才.毕业后可以从事本专业的理论研究、实际应用及教学工作,并在科学研究上能做出创造性的成果。
能够胜任高等院校、科研机构和其他企**的工作.二、主要研究方向1。
精算数学2.金融数学3.随机过程在金融保险中的应用三、培养方式及培养年限培养方式采用课堂教学、讨论和科研训练等相结合的培养方式。
1。
课程学习要求专业课程以课堂讲授、主题研讨为主,考核方式可采用笔试或口试、闭卷或开卷、读书报告等多种方式。
2.和科研训练要求鼓励本专业的博士研究生积极参与院系和指导教师的科研项目和国内外学术交流,在导师的指导下,尽快进入有关课题的研究。
2019南京理工理学院硕士培养方案
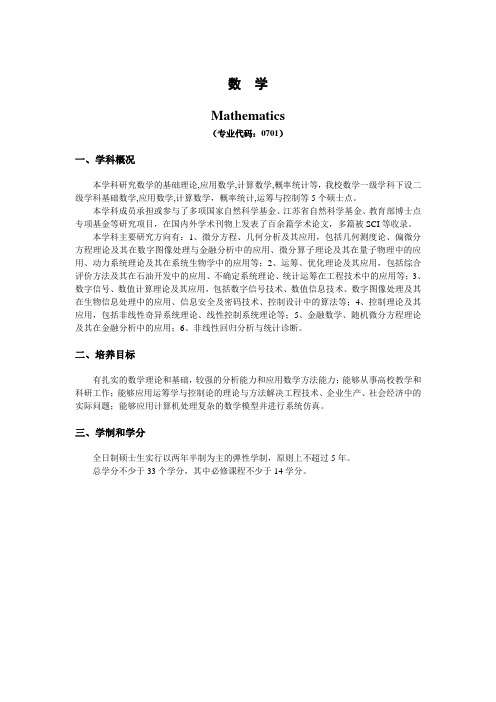
数学Mathematics(专业代码:0701)一、学科概况本学科研究数学的基础理论,应用数学,计算数学,概率统计等,我校数学一级学科下设二级学科基础数学,应用数学,计算数学,概率统计,运筹与控制等5个硕士点。
本学科成员承担或参与了多项国家自然科学基金、江苏省自然科学基金、教育部博士点专项基金等研究项目,在国内外学术刊物上发表了百余篇学术论文,多篇被SCI等收录。
本学科主要研究方向有:1、微分方程、几何分析及其应用,包括几何测度论、偏微分方程理论及其在数字图像处理与金融分析中的应用、微分算子理论及其在量子物理中的应用、动力系统理论及其在系统生物学中的应用等;2、运筹、优化理论及其应用,包括综合评价方法及其在石油开发中的应用、不确定系统理论、统计运筹在工程技术中的应用等;3、数字信号、数值计算理论及其应用,包括数字信号技术、数值信息技术、数字图像处理及其在生物信息处理中的应用、信息安全及密码技术、控制设计中的算法等;4、控制理论及其应用,包括非线性奇异系统理论、线性控制系统理论等;5、金融数学、随机微分方程理论及其在金融分析中的应用;6、非线性回归分析与统计诊断。
二、培养目标有扎实的数学理论和基础,较强的分析能力和应用数学方法能力;能够从事高校教学和科研工作;能够应用运筹学与控制论的理论与方法解决工程技术、企业生产、社会经济中的实际问题;能够应用计算机处理复杂的数学模型并进行系统仿真。
三、学制和学分全日制硕士生实行以两年半制为主的弹性学制,原则上不超过5年。
总学分不少于33个学分,其中必修课程不少于14学分。
四、课程设置五、科研能力与水平本专业主要是培养学生数学素质、数学应用和科学计算能力。
数学素质是指在研究生学习期间必须掌握现代数学基础,为研究生深入学习和研究培养必要的数学素养。
在科学研究方面则要求学生具备如下能力:应用现代信息资源的能力如图书馆文献检索、因特网上信息搜索、电子文档检索等;掌握科研论文的写作能力;具有初步的专题研究、科学计算和数值模拟能力。
HJB偏微分方程的数值计算
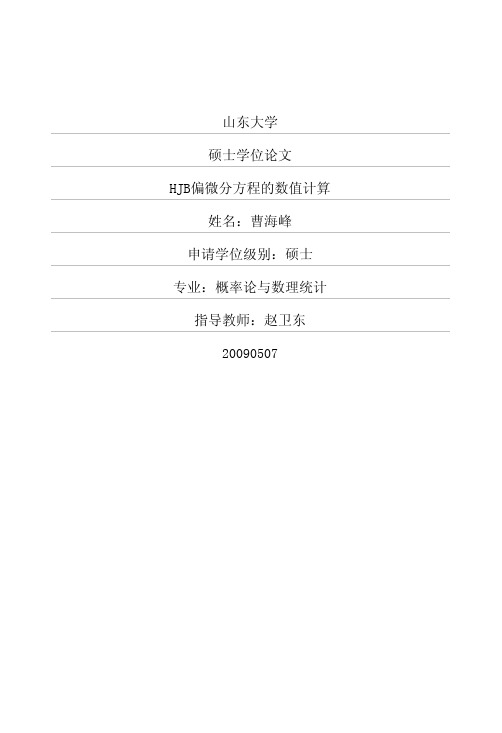
山东大学硕士学位论文HJB偏微分方程的数值计算姓名:曹海峰申请学位级别:硕士专业:概率论与数理统计指导教师:赵卫东20090507HJB偏微分方程的数值计算作者:曹海峰学位授予单位:山东大学1.学位论文吕峰推广的Lipschitz条件下的倒向随机微分方程及g—期望2004该文主要讨论了在一类推广的Lipschitz条件下的倒向随机微分方程和g期望及其相关性质.这个限制使得我们无法将倒向随机微分方程的相关理论应用于一个更广的范围.该文中,我们假设g满足如下形式的一类推广的Lipschitz条件:|g(t,y<,1>,z<,1>)-g(t,y<,2>,z<,2>)|≤r<,t>|y<,1>-y<,2>|+μ<,t>|z<,1>-z<,2>|在此假设之下,得到了倒向随机微分方程解的存在唯一性定理,相对于经典情况而言,在这种情形中,该文的结果对于解的可积性有一个更严格的要求;进一步的,该文还得到了在此条件之下的比较定理.以此为基础,类似于经典情形,定义了相应的g-期望,并以此为工具,得到了在此假设之下的倒向随机微分方程的逆比较定理.2.学位论文杨维强倒向随机微分方程和非线性期望在金融中的应用:风险度量,定价机制的估计以及期权定价2006倒向随机微分方程(BSDE)的线性形式首先由Bismut(1973)在引入,1990年Pardoux&Peng(1990)研究了Lipschitz条件下非线性倒向随机微分方程解的存在唯一性定理。
Duffie&Epstein(1992b)在研究随机微分效用过程中也独立地引进了一类倒向随机微分方程。
倒向随机微分方程在随机控制、偏微分方程、数理金融、经济等领域都有着广泛的应用。
经典的期望是一个线性泛函,在线性期望和可加测度之间存在一一对应的关系。
但是这种一一对应的关系在非线性情形下并不成立,一般地,给定一个非线性期望,我们仍然可以导出一个非可加概率测度,但是却存在无穷多的非线性期望满足这一关系。
华中科技大学数学与统计学院研究生

数学与统计学院华中科技大学数学与统计学院始建于1953年,经历了由数学教研室、数学系到数学与统计学院的发展历程。
上世纪50年代初,著名数理统计学家林少宫先生从美国Illinois大学归来与熊庆来先生的弟子戴良谟等数学界老前辈一起主持了本学科的初创工作。
上世纪70年代末,徐利治教授亲任系主任主持了数学系的创建工作,并于1981年获得应用数学硕士点,随后形成了应用数学、计算数学与运筹学协调发展的良好局面,在全国率先举办了数理逻辑、模糊数学等专题讨论班,创建了《数学评论与研究》和《模糊数学》学术期刊,并培养了国际著名数理经济学家田国强、艾春荣,美国Purdue大学教授蔡智强等一批杰出学者。
八十年代中期,陈庆益教授加盟我校进一步推动和加速了本学科的发展,其主持创建了《应用数学》杂志,并培养了长江特聘教授方复全等一大批数学人才。
九十年代初,黄志远教授调来我校创建了随机分析研究中心,其与杰出青年基金获得者、长江特聘教授任佳刚一起推动了我校在概率统计领域的研究,并使我校获得了概率论与数理统计博士点。
经过几十年的艰苦努力,目前数学与统计学院拥有数学博士后流动站、数学一级学科博士点和数学一级学科硕士点、统计学一级学科博士点,以及应用统计专业硕士点。
在学位授权点建设的同时,数学与统计学院也先后建立了数学与应用数学、信息与计算科学、统计学三个本科专业。
学院现有在校学生648人,其中本科生446人、硕士生156人、博士生46人、硕博连读生所占比例为60%左右。
数学与统计学院现有教职工103人,在编教师87人,其中博士生导师13人,教授19人,副教授37人,教师中有博士学位者占61%,有“长江讲座教授”1名、“新世纪百千万人才工程”入选者1名、教育部“(跨)新世纪优秀人才支持计划”入选者5名、“高校青年教师奖”获得者1名、“湖北省有突出贡献的中青年专家”3名、“湖北省楚天学者”1名以及“宝钢优秀教师奖”获得者4名。
近五年来,数学与统计学院的学术团队共获科研经费890万元,其中国家自然科学基金27项,联合获批国家自然科学基金重大、重点项目2项,;发表SCI收录论文260余篇;出版各类专著、教材30余本。
随机微分方程在金融定价中的应用

随机微分方程在金融定价中的应用摘要随机微分方程是描述随机演化过程的数学模型,在金融学中广泛应用于期权定价、风险度量和投资组合管理等领域。
本文将介绍随机微分方程的概念和基本形式,重点讨论了随机波动率模型和随机跳跃模型在期权定价中的应用。
我们还将给出一些实证研究的案例,通过对实证结果的分析,来进一步验证随机微分方程在金融定价中的应用价值。
随机微分方程的基本概念随机微分方程是随机演化过程的数学模型,它是微分方程的一个扩展。
将随机变量的随机性纳入微分方程的描述中,可以更准确地描述复杂的随机演化过程。
随机微分方程的基本形式如下:du t=a(u t,t)dt+b(u t,t)dW t+c(u t,t)dN t其中,dW t是标准布朗运动的随机微分形式,dN t是泊松流的随机微分形式。
a(u t,t),b(u t,t)和c(u t,t)是随机过程。
当b(u t,t)和c(u t,t)均为0时,随机微分方程就变成了普通的微分方程。
随机微分方程在期权定价中的应用随机波动率模型随机波动率模型是一种期权定价模型,它可以更好地解释实际市场中的波动率裂口现象。
随机波动率模型基于以下假设:1.股票价格服从几何布朗运动。
2.股票波动率是一个随机过程,它的演化遵循某个随机微分方程模型,例如,CIR模型。
根据上述假设,随机波动率模型可以被表示为:$$\\frac{dS_t}{S_t}=r dt+\\sqrt{v_t} dW_t$$其中,S t是股票价格,r是固定无风险利率,v t是波动率,dW t是标准布朗运动。
根据此模型,可以计算出欧式看涨期权(European Call Option)的价格:C(S0,v0,K,T,r)=S0N(d1)−Ke−rT N(d2)其中,S0表示股票当前价格,v0表示股票当前波动率,K是期权行权价,T是期权到期时间,N(x)是标准正态分布的累积分布函数。
d1和d2是带有期权隐含波动率的标准正态分布的分位数,可以通过Black-Scholes方程求解得到。
随机微分方程在金融领域的应用研究
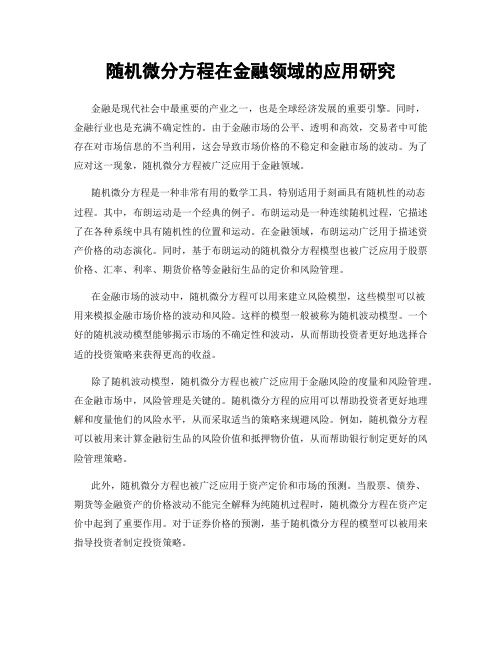
随机微分方程在金融领域的应用研究金融是现代社会中最重要的产业之一,也是全球经济发展的重要引擎。
同时,金融行业也是充满不确定性的。
由于金融市场的公平、透明和高效,交易者中可能存在对市场信息的不当利用,这会导致市场价格的不稳定和金融市场的波动。
为了应对这一现象,随机微分方程被广泛应用于金融领域。
随机微分方程是一种非常有用的数学工具,特别适用于刻画具有随机性的动态过程。
其中,布朗运动是一个经典的例子。
布朗运动是一种连续随机过程,它描述了在各种系统中具有随机性的位置和运动。
在金融领域,布朗运动广泛用于描述资产价格的动态演化。
同时,基于布朗运动的随机微分方程模型也被广泛应用于股票价格、汇率、利率、期货价格等金融衍生品的定价和风险管理。
在金融市场的波动中,随机微分方程可以用来建立风险模型,这些模型可以被用来模拟金融市场价格的波动和风险。
这样的模型一般被称为随机波动模型。
一个好的随机波动模型能够揭示市场的不确定性和波动,从而帮助投资者更好地选择合适的投资策略来获得更高的收益。
除了随机波动模型,随机微分方程也被广泛应用于金融风险的度量和风险管理。
在金融市场中,风险管理是关键的。
随机微分方程的应用可以帮助投资者更好地理解和度量他们的风险水平,从而采取适当的策略来规避风险。
例如,随机微分方程可以被用来计算金融衍生品的风险价值和抵押物价值,从而帮助银行制定更好的风险管理策略。
此外,随机微分方程也被广泛应用于资产定价和市场的预测。
当股票、债券、期货等金融资产的价格波动不能完全解释为纯随机过程时,随机微分方程在资产定价中起到了重要作用。
对于证券价格的预测,基于随机微分方程的模型可以被用来指导投资者制定投资策略。
综上所述,随机微分方程在金融领域中起到了非常重要的作用。
它为金融市场的波动、风险管理、资产定价和市场预测提供了有效的数学方法和工具。
无论是在金融机构还是个人投资者中,应用随机微分方程对于制定投资策略和管理风险都非常重要。
金融数学与金融工程专业攻读硕士学位研究生培养方案 (专业代码
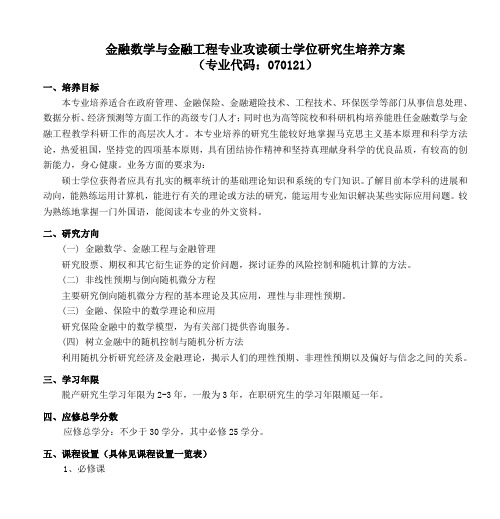
金融数学与金融工程专业攻读硕士学位研究生培养方案(专业代码:070121)一、培养目标本专业培养适合在政府管理、金融保险、金融避险技术、工程技术、环保医学等部门从事信息处理、数据分析、经济预测等方面工作的高级专门人才;同时也为高等院校和科研机构培养能胜任金融数学与金融工程教学科研工作的高层次人才。
本专业培养的研究生能较好地掌握马克思主义基本原理和科学方法论,热爱祖国,坚持党的四项基本原则,具有团结协作精神和坚持真理献身科学的优良品质,有较高的创新能力,身心健康。
业务方面的要求为:硕士学位获得者应具有扎实的概率统计的基础理论知识和系统的专门知识。
了解目前本学科的进展和动向,能熟练运用计算机,能进行有关的理论或方法的研究,能运用专业知识解决某些实际应用问题。
较为熟练地掌握一门外国语,能阅读本专业的外文资料。
二、研究方向(一)金融数学、金融工程与金融管理研究股票、期权和其它衍生证券的定价问题,探讨证券的风险控制和随机计算的方法。
(二)非线性预期与倒向随机微分方程主要研究倒向随机微分方程的基本理论及其应用,理性与非理性预期。
(三)金融、保险中的数学理论和应用研究保险金融中的数学模型,为有关部门提供咨询服务。
(四)树立金融中的随机控制与随机分析方法利用随机分析研究经济及金融理论,揭示人们的理性预期、非理性预期以及偏好与信念之间的关系。
三、学习年限脱产研究生学习年限为2-3年,一般为3年,在职研究生的学习年限顺延一年。
四、应修总学分数应修总学分:不少于30学分,其中必修25学分。
五、课程设置(具体见课程设置一览表)1、必修课马克思主义理论课3学分第一外国语4学分、专业外语1学分。
学位基础课2门,不少于6学分,学位专业课2门,不少于4学分。
前沿讲座2学分:(1)讲座课的内容:数学学科及其下属的二级学科组织的综合或专题报告会(2)每学期至少参加3次讲座,以书面报告的形式进行考核。
要求对数学中若干重要方向的发展有所了解。
统计学一级学科硕士研究生培养方案

统计学一级学科硕士研究生培养方案(专业代码:071400)一、培养目标为适应教育面向现代化、面向世界、面向未来的目标,培养社会主义建设事业需要的高层次专门人才,要求统计专业的硕士研究生:1.应具有较扎实的统计学理论基础;2.应系统地掌握本专业基本理论、基本研究方法和技巧;3.应具有较强的学术沟通能力和良好的团队协作精神;4.应具备创新意识和独立科研能力;5.应该熟练掌握一门外语,具有阅读外文资料和用外文写作论文的能力;6.应具有熟练地使用计算机进行科学计算以及借助互联网查阅专业资料的能力;7.身心健康,德才兼备。
二、培养方式与学习年限1.培养方式采用导师指导为主,导师与指导小组集体培养相结合的模式,通过课堂授课、专题讨论班、专家讲学、课题研究、参加学术报告(会议)等培养方式,使学生成为有学习积极性、主动性和创造性的高层次专门人才。
2.学习年限本专业的硕士研究生学制为三年。
三、研究方向试验设计,非参数估计,金融统计,风险管理。
四、课程设置五、学习要求与考核方式1.课程学习要求要求每位研究生至少修满35学分,其中学科基础课至少修满6学分,专业主干课至少修满6学分。
考核分为考试与考查。
必修课进行考试,选修课进行考试或考查。
考试成绩按百分制计分,考查成绩采用五级记分制。
2.实践环节要求实践内容包括教学实践(为本科生授课、辅导、批改作业、指导大学生毕业论文等)与科研实践(参与具体的科研项目、科研咨询、课题调研,参加学术报告或学术会议等)。
相关的要求见本培养方案有关条目。
3.科研成果数量要求本专业的硕士研究生在学习期间至少发表(含录用)1篇专业学术论文(除导师外,申请者须排名第一)。
特殊情况下,经导师同意并经学院学术委员会认定达到毕业水平者,可以不要求有学术论文在毕业前被发表或录用。
六、中期考核课程学习阶段完成后,学生最迟在入学后的第四学期末之前,参加学院组织的中期考核。
中期考核办法参照“硕士学位研究生中期考核规定”进行。
简析应用数学与金融学的关系
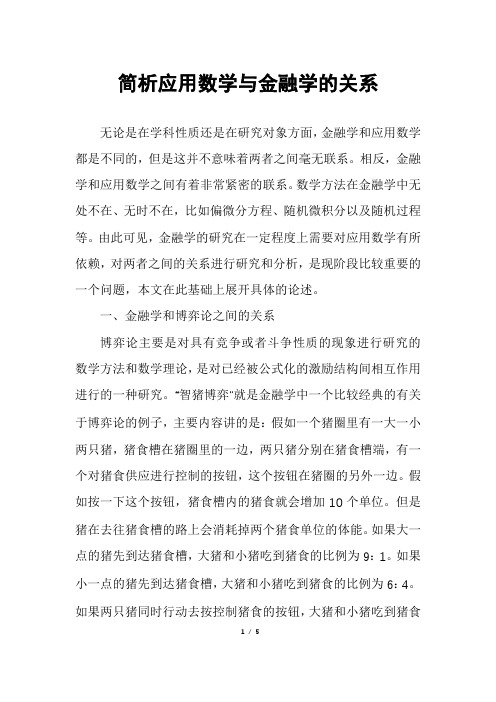
简析应用数学与金融学的关系无论是在学科性质还是在研究对象方面,金融学和应用数学都是不同的,但是这并不意味着两者之间毫无联系。
相反,金融学和应用数学之间有着非常紧密的联系。
数学方法在金融学中无处不在、无时不在,比如偏微分方程、随机微积分以及随机过程等。
由此可见,金融学的研究在一定程度上需要对应用数学有所依赖,对两者之间的关系进行研究和分析,是现阶段比较重要的一个问题,本文在此基础上展开具体的论述。
一、金融学和博弈论之间的关系博弈论主要是对具有竞争或者斗争性质的现象进行研究的数学方法和数学理论,是对已经被公式化的激励结构间相互作用进行的一种研究。
“智猪博弈”就是金融学中一个比较经典的有关于博弈论的例子,主要内容讲的是:假如一个猪圈里有一大一小两只猪,猪食槽在猪圈里的一边,两只猪分别在猪食槽端,有一个对猪食供应进行控制的按钮,这个按钮在猪圈的另外一边。
假如按一下这个按钮,猪食槽内的猪食就会增加10个单位。
但是猪在去往猪食槽的路上会消耗掉两个猪食单位的体能。
如果大一点的猪先到达猪食槽,大猪和小猪吃到猪食的比例为9:1。
如果小一点的猪先到达猪食槽,大猪和小猪吃到猪食的比例为6:4。
如果两只猪同时行动去按控制猪食的按钮,大猪和小猪吃到猪食1/ 5的比例为7:3。
如果这两头猪都有一定的智慧,最终的结果是,小一点的猪一定会选择等待。
利用博弈论中的支付矩阵就可以计算出,小一点的猪如果选择等待,最坏的情况就是获得0收益,最好的情况可以获得+4的收益,如果小一点的猪选择行动,最好的情况可以获得1收益,而且这个时候还有-1收益的风险。
所以,如果小猪有智慧,一定会选择等待。
这就是著名的“智猪博弈”,这个经典案例以及结论可以运用到今天的金融学当中。
例如,一个小的,在必要的时候可以选择沉住气去等待,让大的率先去开发市场,这个时候的不作为就可以为将来的有所为做铺垫。
小的企业是选择等待,无论是在研究还是在观察上,都能节约很多一些不必要的费用,这样就可以让企业的发展和管理上升到一个新的阶段。
- 1、下载文档前请自行甄别文档内容的完整性,平台不提供额外的编辑、内容补充、找答案等附加服务。
- 2、"仅部分预览"的文档,不可在线预览部分如存在完整性等问题,可反馈申请退款(可完整预览的文档不适用该条件!)。
- 3、如文档侵犯您的权益,请联系客服反馈,我们会尽快为您处理(人工客服工作时间:9:00-18:30)。
随机微分方程在数理金融中的应用硕士学位摘要复杂数据主要表现在相依、非线性、维数高与不完全观测等,在股市、基因序列和经济等领域中经常出现。
为解决巨型数据集合问题,数据挖掘的理论、方法和技术已应运而生。
而针对诸如怎样同时检验成千上万个基因中哪些基因的表达水平有显著性差异之类的高维统计推断问题,以错误发现率为主要特征的非参数估计方法无疑为其提供了一个有效的解决途径。
本文主要研究考察错误发现率的在各种参数模型和非参数模型下的控制检验方法,全文共分为四章。
文章首先介绍了所选取课题的背景和意义,以及国内外在该方向的研究现状。
在多重假设检验的背景下,给出了错误发现率的定义,提出利用p值进行假设检验,并在假设检验独立和相依的情形下对错误发现率的控制方法进行了探讨。
在研究错误发现率的控制方法时,发现在处理多重假设检验问题时,核心的问题是如何估计真实零假设的个数,因此本文采用经验贝叶斯估计来估计它的值。
在参数混合模型和非参数混合模型中研究真实零假设的估计问题是本文的核心内容。
针对正态混合分布模型和Beta混合分布模型两种参数混合模型,文章采用矩估计方法和基于p值的最小二乘估计方法进行研究;在研究非参数混合模型时,分别介绍了最小二乘估计方法、Beta分布拟合模型和Beinstein多项式拟合模型的方法。
文章的最后以Hedenfalk报告的一组乳腺癌患者的基因数据为例进行仿真研究,发现错误发现率为微阵列数据的多重假设检验提供了合适的错误控制指标。
关键词:错误发现率;多重假设检验;p值;非参数估计;微阵列数据AbstractComplex data always appear in the stock market, gene sequences, economic and other fields, which mainly show the characteristic of dependent, nonlinear, high dimension and incomplete observations. In order to solve the problem of huge data collection, the theories, methods and techniques of data mining are proposed. While how to examine the high-dimensional statistical inference problem, such as the significant differences of expression levels in thousands of genes, the non-parametric estimation of false discovery rate provide an effective solution.This paper mainly investigate the test method based on the false discovery rate of various parametric model and non-parametric model, which is divided into four chapters. Firstly, this paper introduce the background and significance of the topic, and the current studies in this direction at home and abroad. Under the background of multiple hypotheses testing, the paper describe the definition of the false discovery rate, propose using the p-value to test the hypothesis testing, and discuss the controlling method of the false discovery rate when the hypotheses testing is independent or dependent. When we investigate the controlling method of the false discovery rate and studied the multiple hypothesis testing problem, we find that the central problem is how to estimate the number of true null hypothesis, so this paper use the empirical Bayes estimation to estimate its value. Investigating the estimation of true null hypothesis in the mixing parametric model and non-parametric model is core of the dissertation. Aiming at the mixed normal distribution model and Beta mixture distribution model, This paper use the method of moment estimation and least squares estimation method based on the p-value to estimate its value; On studying the non-parametric mixture model, the paper introduce the least square estimation method, Beta distribution fitting model method and the Beinstein polynomial fitting model method. Finally, the paper conduct the simulation research based on a group of patients with breast cancer gene data by Hedenfalk, and find that the false discovery rate is able to provide a suitable error control targets for the multiple hypothesis testing of microarray data.Keywords: false discovery rate, multiple hypotheses testing, p-value, non-parametric estimation, microarray data目 录摘 要 ..................................................................................................................... I Abstract . (II)第1章 绪 论 (1)1.1 课题研究的背景及意义 (1)1.2 国内外在该方向的研究现状 (1)1.2.1 国外对错误发现率的研究现状 (1)1.2.2 国内研究现状 (3)1.3 本文拟研究的主要内容 (3)1.4 创新点 (3)第2章 错误发现率的多重检验方法 (5)2.1 多重假设检验的错误测度 (5)2.2 P 值的定义、性质和计算方法 (6)2.3 独立情形下基于FDR 控制的检验方法 (7)2.4 相依情形下基于FDR 控制的检验方法 (8)2.5 真实零假设的个数0m 或比值0π的估计 (9)2.5.1 -λ估计 (9)2.5.2 经验贝叶斯估计 (11)2.6 本章小结 (12)第3章 参数混合模型和非参数混合模型的估计 (13)3.1 引言 (13)3.2 正态分布混合模型 (13)3.3 Beta 分布混合模型 (17)3.4 非参数混合模型的估计 (21)3.4.1 最小二乘估计 (22)3.4.2 Beta 分布拟合模型 (24)3.4.3 Beinstein 多项式拟合模型 (25)3.5 本章小结 (26)第4章 错误发现率的估计方法的应用 (27)4.1 引言 (27)4.2 微阵列数据实例研究 (27)4.3 本章小结 (29)结论 (30)参考文献 (31)哈尔滨工业大学学位论文原创性声明和使用权限............. 错误!未定义书签。
致谢 ..................................................................................... 错误!未定义书签。
第1章绪论1.1 课题研究的背景及意义复杂数据主要表现在相依、维数高、非线性与不完全观测等,经常出现在股市、基因序列和经济等领域中。
在研究处理低维的简单数据时,采用传统的数理统计方法是有效的,但在研究比较复杂的数据时,就会变得比较困难。
因此,“复杂数据的统计推断问题”已被列为我国统计学研究的重点课题。
随着科学技术的不断发展,在实际的统计研究过程中,出现了越来越多的大型数据集合问题。
在研究巨型数据的高维统计推断问题时,以错误发现率为主要特征的非参数估计方法为其提供了一个有效地解决途径。
在巨型数据问题的统计分析中,错误发现率( false discovery rate, FDR)有着非常重要的作用,现已被越来越多地应用在微阵列(Microarray)数据研究和功能磁共振成像(Functional magnetic resonance imaging, fMRI)等领域。
以微阵列数据研究和功能磁共振成像(fMRI)为代表的现代生物技术已经给医学界的研究带来了很大的影响。
由于错误发现率可以为大规模数据多重检验中的错误控制提供一个合适的测量标准,因此在微阵列数据的研究中,研究者通常采用错误发现率(FDR)来控制多重假设检验的错误率。
例如在研究基因表达的差异性试验中,假设我们挑选了R个差异表达的基因,其中有S个是真正有差异表达的,另外有V个其实是没有差异表达的,也就是说是假阳性的。