易康三种面向对象的地物分类方法
eCognition8.9面向对象分类详细步骤
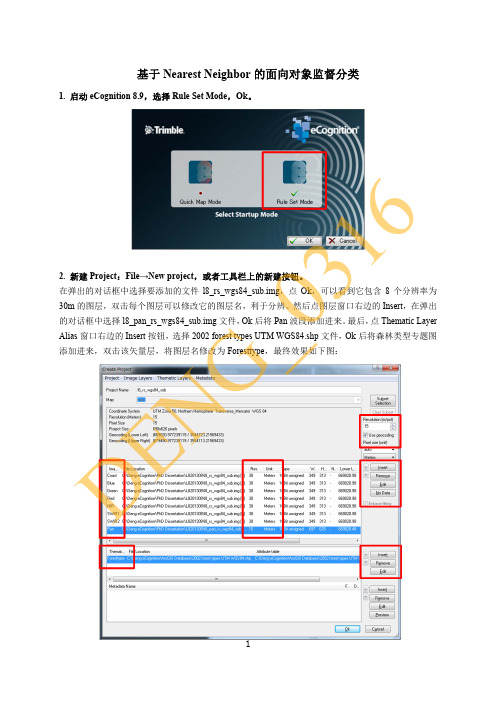
基于Nearest Neighbor 的面向对象监督分类1. 启动eCognition 8.9,选择Rule Set Mode ,Ok 。
2. 新建Project :File →New project ,或者工具栏上的新建按钮。
在弹出的对话框中选择要添加的文件l8_rs_wgs84_sub.img ,点Ok ,可以看到它包含8个分辨率为30m 的图层,双击每个图层可以修改它的图层名,利于分辨。
然后点图层窗口右边的Insert ,在弹出的对话框中选择l8_pan_rs_wgs84_sub.img 文件,Ok 后将Pan 波段添加进来。
最后,点Thematic Layer Alias 窗口右边的Insert 按钮,选择2002 forest types UTM WGS84.shp 文件,Ok 后将森林类型专题图添加进来,双击该矢量层,将图层名修改为Foresttype ,最终效果如下图:D E NG _0316Project Name 等按默认,点Ok ,回到主界面,图像按前3个波段RGB 显示,如下图:为了更好的辨别地物类型,点击工具栏上的图层显示编辑按钮,在弹出的对话框中点击修改RGB 为NIR ,Green ,Blue 显示:D E N G _0316如果取消勾选左下角No layer weights ,还可以设置不同波段的比重,在调整不同波段的比重时,在数值上左击鼠标增加比重,右击鼠标减少比重,如下图:点Ok 进行波段显示调整后的效果如下,然后保存这个Project 为l8_rs_wgs84_sub.dpr 。
D E N G _03163. 将图像分解为基本对象:首先,在Process Tree 窗口(如果没有,菜单栏View →Windows →Process Tree 调出),右击,选择Append New ,将Name 改为Segmentation ,其他按默认,然后点击Ok :其次,在Process Tree 窗口,右击Segmentation 这个新建规则(Rule),选Insert Child(插入子规则),Name 勾选自动,Algorithm 下拉菜单选择multiresolution segmentation (最常用的分割算法),在右边的参数窗口,找到Scale parameter 并将其设置为150,其他默认,然后点Execute(立即实行)或者Ok(稍后实行)。
eCognition应用
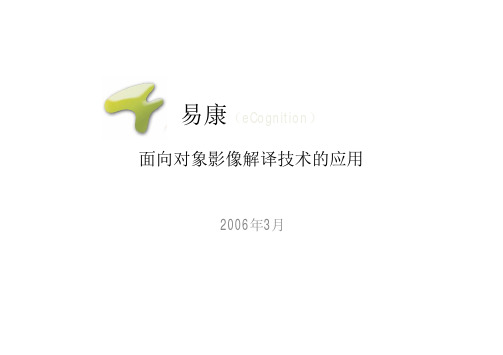
Hyperspectral CASI
7、5不同尺度分类结果统计信息集成
ID 针叶林面积
2 3 4 6 7 8
落叶林面积
950 29296 14081 16263 12432
7669
干针叶林面积
15479 12428
1722 3948 12791 29058
采伐区面积
0 350
0 631 648 2048
• 2)矢量数据:
• 外业检验并经目视解译的土地利用分类图 • 1:1万的土地利用现状图
• 3)其它资料:
与调查有关的行政区划、农、林等方面的文献资料
• 4)土地利用分类等级体系选择:
结合本次实验的目的及实验区特点,以农用地为主,选择 分类体系为2001年国土资源部发布《土地分类》试行版本, 该分类体系采用三级分类体系,其中一级地类3个、二级 地类15个、三级地类71个。
非植被 植被
Multiresolution Segmentation
不同尺度分割
像素精级影粗中分像分分割对割割象
多尺度分割——形成对象层次与邻域关系
a 对象等级结构
a 对象等级结构
b 对象层次
b 对象层次
易康的特点
• 德国易康eCognition软件面向对象的遥感影像解译思想朝 更接近人类思维模式的方向又迈进了一步,而且其解译的 精度和效率通过下面介绍的一些影像解译实际应用项目得 到了较系统客观的检验与验证;
所占百分比(%) 9.75 2.95 1.53 1.21 0.45 0.33 48.12 5.4 19.08 5.38 5.8 100
4、1 QuickBird影像城市土地利用分类
西安煤航宝鸡快鸟数据城市土地利用分类
面向对象分类法arcgis
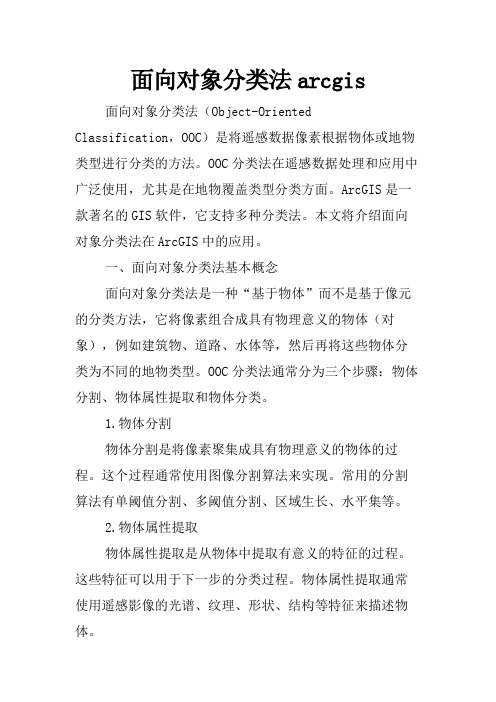
面向对象分类法arcgis 面向对象分类法(Object-Oriented Classification,OOC)是将遥感数据像素根据物体或地物类型进行分类的方法。
OOC分类法在遥感数据处理和应用中广泛使用,尤其是在地物覆盖类型分类方面。
ArcGIS是一款著名的GIS软件,它支持多种分类法。
本文将介绍面向对象分类法在ArcGIS中的应用。
一、面向对象分类法基本概念面向对象分类法是一种“基于物体”而不是基于像元的分类方法,它将像素组合成具有物理意义的物体(对象),例如建筑物、道路、水体等,然后再将这些物体分类为不同的地物类型。
OOC分类法通常分为三个步骤:物体分割、物体属性提取和物体分类。
1.物体分割物体分割是将像素聚集成具有物理意义的物体的过程。
这个过程通常使用图像分割算法来实现。
常用的分割算法有单阈值分割、多阈值分割、区域生长、水平集等。
2.物体属性提取物体属性提取是从物体中提取有意义的特征的过程。
这些特征可以用于下一步的分类过程。
物体属性提取通常使用遥感影像的光谱、纹理、形状、结构等特征来描述物体。
3.物体分类物体分类是将物体按照它们的物理意义分类的过程。
这个过程通常使用基于强分类器的机器学习方法来实现,例如支持向量机、随机森林等。
二、面向对象分类法在ArcGIS中的应用ArcGIS是一款功能强大的GIS软件,它支持多种遥感数据分类方法,包括像元分类、基于物体分类和混合分类等。
其中基于物体的分类法就是面向对象分类法。
使用ArcGIS进行面向对象分类法分析的步骤如下:1.数据准备首先需要准备一幅高分辨率的遥感影像,这个影像最好是多光谱遥感影像,因为多光谱遥感影像包含了丰富的地物信息,可以提高面向对象分类的精度。
其次需要准备一个数字高程模型(Digital Elevation Model,DEM),这个DEM可以用于去除地形效应,提高分类的精度。
2.物体分割在ArcGIS中实现物体分割是通过“物体识别工具”来实现的。
eCognition的概论 1
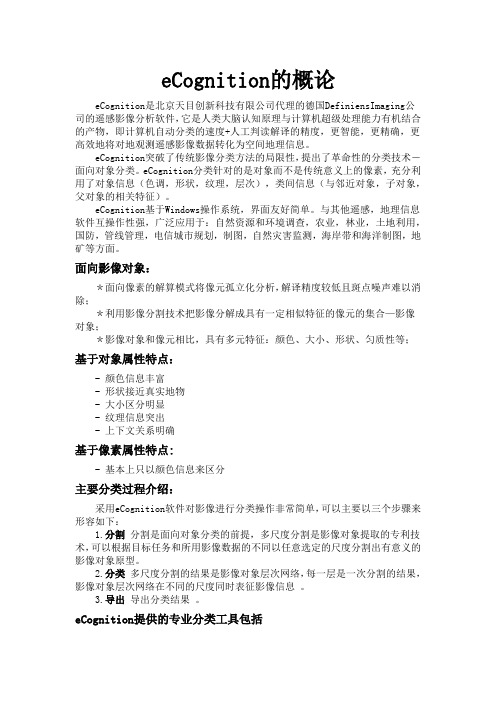
eCognition的概论eCognition是北京天目创新科技有限公司代理的德国DefiniensImaging公司的遥感影像分析软件,它是人类大脑认知原理与计算机超级处理能力有机结合的产物,即计算机自动分类的速度+人工判读解译的精度,更智能,更精确,更高效地将对地观测遥感影像数据转化为空间地理信息。
eCognition突破了传统影像分类方法的局限性,提出了革命性的分类技术-面向对象分类。
eCognition分类针对的是对象而不是传统意义上的像素,充分利用了对象信息(色调,形状,纹理,层次),类间信息(与邻近对象,子对象,父对象的相关特征)。
eCognition基于Windows操作系统,界面友好简单。
与其他遥感,地理信息软件互操作性强,广泛应用于:自然资源和环境调查,农业,林业,土地利用,国防,管线管理,电信城市规划,制图,自然灾害监测,海岸带和海洋制图,地矿等方面。
面向影像对象:*面向像素的解算模式将像元孤立化分析,解译精度较低且斑点噪声难以消除;*利用影像分割技术把影像分解成具有一定相似特征的像元的集合—影像对象;*影像对象和像元相比,具有多元特征:颜色、大小、形状、匀质性等;基于对象属性特点:- 颜色信息丰富- 形状接近真实地物- 大小区分明显- 纹理信息突出- 上下文关系明确基于像素属性特点:- 基本上只以颜色信息来区分主要分类过程介绍:采用eCognition软件对影像进行分类操作非常简单,可以主要以三个步骤来形容如下:1.分割分割是面向对象分类的前提,多尺度分割是影像对象提取的专利技术,可以根据目标任务和所用影像数据的不同以任意选定的尺度分割出有意义的影像对象原型。
2.分类多尺度分割的结果是影像对象层次网络,每一层是一次分割的结果,影像对象层次网络在不同的尺度同时表征影像信息。
3.导出导出分类结果。
eCognition提供的专业分类工具包括* 多源数据融合* 多尺度分割* 基于样本的监督分类* 基于知识的模糊分类* 人工分类* 自动分类多源数据融合工具:可用来融合不同分辨率的对地观测影像数据和GIS数据,如Landsat,Spot,IRS,IKONOS, QuickBird,SAR,航空影像,LIDAR等,不同类型的影像数据和矢量数据同时参与分类。
面向对象影像分析简要介绍--以eCognition为例

面向对象影像分析简要介绍——以eCognition软件为例前言遥感影像的光谱,空间,时间分辨率不断提高,为开展各类遥感应用提供各种数据。
但在遥感数据获取能力增强的同时,也使得丰富的影像数据得不到充分利用和挖掘,从而出现“数据丰富,信息贫乏”的困境。
如何快速自动准确地从遥感影像中提取出能满足某种应用的专题信息,是我们亟待要解决的问题。
随着面向对象思想的风行以及面向对象影像分析技术的不断成熟,使得我们从高分影像中提取专题信息变得更加便捷。
尤其是一些商业的面向对象影像分析软件的出现,如eCognition,Feature Analysis。
eCognition软件的口号就是“Exploring the soul of imagery(发掘影像最大潜能)”。
本论文旨在从eCognition软件了解面向对象影像分析的相关思想和技术。
希望通过探究eCognition软件背后的思想以及技术原理,如面向对象,多尺度分割,模糊分类等,为高分辨率遥感影像的特征描述以及建模带来一些启发。
1.面向对象面向对象的思想是针对具体应用,将问题处理对象(逻辑概念上或物理概念上)划分为合适粒度(即对象)来进行处理,并封装其相应的属性以及行为,同时为了更好的复用以及扩展,维护更新,使其具有继承,多态,聚合等特性。
1.1对象对象是指状态和行为的集合体,在物理实现上表现为数据和操作的集合,逻辑上表现为有职能的实体。
它是用来描述现实世界中的物理概念或逻辑概念上的物体。
比如人就是一个对象,它有性别,年龄,姓名等属性,人有吃饭睡觉等行为。
武汉大学也是一个对象,它有名称,学院机构,学校历史等属性,也有教学科研等行为。
不同的是人是物理概念上的对象,武汉大学是逻辑概念上的对象。
1.2抽象性,封装性,继承性抽象是抽取出我们所感兴趣的部分,用这些少量特征来描述一个事物。
封装性是对事物的数据和操作进行封装,即对其状态和行为进行封装。
继承特性是对事物属性和行为的继承。
遥感图像解译eCognition软件实习报告
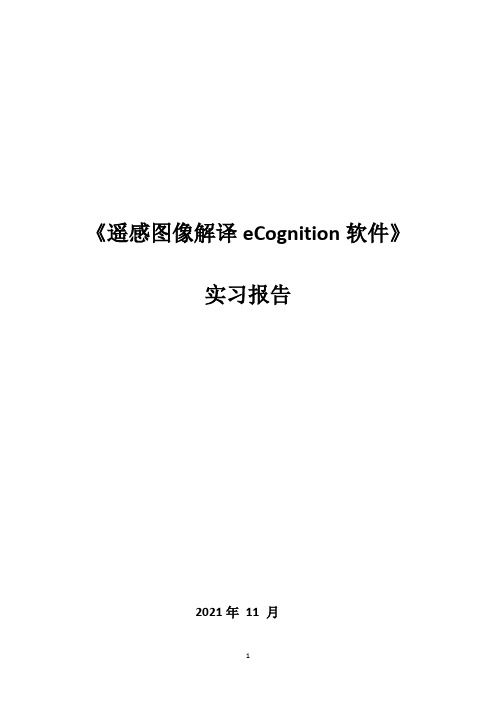
《遥感图像解译eCognition软件》实习报告2021年11 月eCognition软件数据处理报告目录目录 (1)1实习原理 (2)2实习目的 (2)3实习步骤 (2)3.1导入数据,进行预处理 (3)3.2影像分割 (5)3.2.1棋盘分割 (5)3.2.2四叉树分割 (6)3.2.3多尺度分割 (7)3.2.4波谱差异分割 (9)3.3建立分类体系 (10)3.4样区选择与特征空间的构建 (11)3.4.1样区选择 (11)3.4.2特征空间构建 (12)3.5执行分类 (15)3.3结果输出 (16)4实习心得 (17)正文一.实习原理随着遥感技术的不断发展,遥感信息的现势性、宏观性、成图周期短、多时性和立体覆盖能力的优势,让其在土地利用信息获取方面发挥着越来越重要的作用。
利用遥感影像对地物进行分类,并根据分类结果影像编制专题地图,也已经成为了土地利用,监测方面不可缺少手段。
而遥感影像分类的精度,直接影响着遥感数据可利用性和专题地图的精度。
因此利用相关软件或者算法提高遥感影像分类的精度,成为了提升遥感数据使用价值中刻不容缓的任务。
eCognition系列软件作为面向对象影像分析技术的专业软件,与传统的ERDAS/ENVI/PCI等有明显的不同,虽然ERDAS和ENVI里也有相应的面向对象分类模块,但其对高分辨率影像的信息提取效果,及高分辨率影像涉及的各个行业的应用范围无法与eCognition软件相比。
eCognition软件最大的特色采用面向对象的遥感影像分析。
首先将影像按照一定尺度分割成一个个对象,然后对每一个对象封装其光谱、形状、纹理等特性并且建立该对象与其相邻对象、父对象、子对象之间的关系。
其中主要包括分割与分类两个步骤。
分割——是指依据某种同质性或者异质性标准,将影像划分成很多小块对象的过程;是分类的前提。
分类——是指依据小块对象的形状、颜色、纹理、空间关系、隶属关系等属性来识别所属类别的过程。
易康分类特征介绍
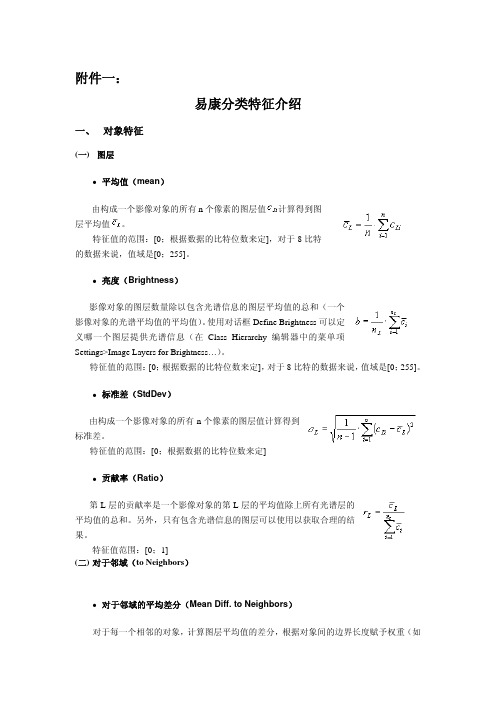
附件一:易康分类特征介绍一、 对象特征(一)图层z平均值(mean)由构成一个影像对象的所有n个像素的图层值计算得到图层平均值。
特征值的范围:[0;根据数据的比特位数来定],对于8比特的数据来说,值域是[0;255]。
z亮度(Brightness)影像对象的图层数量除以包含光谱信息的图层平均值的总和(一个影像对象的光谱平均值的平均值)。
使用对话框Define Brightness可以定义哪一个图层提供光谱信息(在Class Hierarchy编辑器中的菜单项Settings>Image Layers for Brightness…)。
特征值的范围:[0;根据数据的比特位数来定],对于8比特的数据来说,值域是[0;255]。
z标准差(StdDev)由构成一个影像对象的所有n个像素的图层值计算得到标准差。
特征值的范围:[0;根据数据的比特位数来定]z贡献率(Ratio)第L层的贡献率是一个影像对象的第L层的平均值除上所有光谱层的平均值的总和。
另外,只有包含光谱信息的图层可以使用以获取合理的结果。
特征值范围:[0;1](二)对于邻域(to Neighbors)z对于邻域的平均差分(Mean Diff. to Neighbors)对于每一个相邻的对象,计算图层平均值的差分,根据对象间的边界长度赋予权重(如果它们是直接相邻的,特征距离=0)或者根据相邻对象的面积赋予权重(如果被讨论的影像对象周围的邻域已用某一范围(像素级)来定义,特征距离>0)。
对于直接相邻对象的平均差分如下计算:所关心的影像对象的边界长度与第i个直接相邻对象共同的边界长度所关心的影像对象的图层平均值第i个相邻对象的图层平均值相邻对象的数量如果你用某一个范围内的对象来定义领域(参见特征距离(feature distance),平均差分则计算如下:所有领域对象的总面积第i领域对象的面积所关心的影像对象的图层平均值第i领域对象的图层平均值相邻对象的数量特征值的范围:[0;根据数据的比特位数来定]z对于邻域的平均差分(绝对值)(Mean Diff. to Neighbors(abs)和对于邻域的平均差分相同,不同是差分使用的是绝对值。
eCognition Developer软件常用算法与特征.
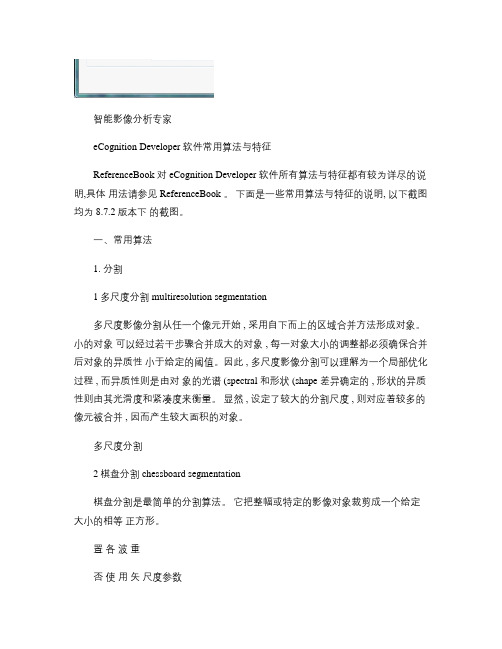
智能影像分析专家eCognition Developer 软件常用算法与特征ReferenceBook 对 eCognition Developer 软件所有算法与特征都有较为详尽的说明,具体用法请参见 ReferenceBook 。
下面是一些常用算法与特征的说明, 以下截图均为 8.7.2版本下的截图。
一、常用算法1. 分割1多尺度分割 multiresolution segmentation多尺度影像分割从任一个像元开始 , 采用自下而上的区域合并方法形成对象。
小的对象可以经过若干步骤合并成大的对象 , 每一对象大小的调整都必须确保合并后对象的异质性小于给定的阈值。
因此 , 多尺度影像分割可以理解为一个局部优化过程 , 而异质性则是由对象的光谱 (spectral 和形状 (shape 差异确定的 , 形状的异质性则由其光滑度和紧凑度来衡量。
显然 , 设定了较大的分割尺度 , 则对应着较多的像元被合并 , 因而产生较大面积的对象。
多尺度分割2棋盘分割 chessboard segmentation棋盘分割是最简单的分割算法。
它把整幅或特定的影像对象裁剪成一个给定大小的相等正方形。
置各波重否使用矢尺度参数形状因子紧致度因子智能影像分析专家棋盘分割因为棋盘分割算法产生简单的正方形对象,所以它经常用来细分影像与影像对象。
3四叉树分割四叉树分割与棋盘分割类似,但是它要创建出不同大小的正方形。
您可以使用Scale Parameter 定义每个正方形内的颜色差异上限。
在裁剪出一个初始的正方形网格后,四叉树分割继续如下:如果不符合同质性标准, 那么把每个正方形裁剪成四个较小的正方形。
例如:正方形对象中最大的颜色差异要比定义的尺度值大。
重复以上过程直到在每个正方形中都符合同质性标准。
四叉树分割尺度参数是否使用矢量参与分割2. 分类1 assign Class 根据限制条件将对象分为指定类别,一次只能分一类。
易康培训教程
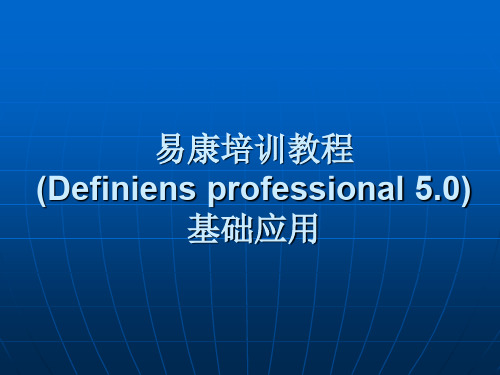
3. 确保以下工具被打开
规则树(process tree):在主菜单中选择Process>Process Tree或 选择工具按钮
类层(Class Hierarchy):在主菜单中选择Classification>Class Hierarchy或选择工具按钮 影像对象信息(Image Object Information):在主菜单中选择Image Objects>Image Object Information或选择工具按钮
算法图:应用提供的算法可以定义各种重要影像分析的操作
流程,以下是软件提供的各种算法的目录。
算法目录
1.8 影像对象域(Image Object Domain )
影像对象域所描述的是在影像层组中应用算 法所执行规则的某一区域,影像对象域由所对应 子集的结构描述来定义。下例为一个影像对象域 的整个影像、对象层、对象分类。
1.4 .2全局特征
全局特征一般来说描述的是当前影像对 象的层次结构情况。 例如,给定的影像层 均值度,影像对象的层数或类组中包含的 对象数,全局特征也描述了所输入数据的 附加的元数据信息。
如图所示,特征窗口中某层的均值特征
1.5类和分类
分类是把具有相近关系的影像对象归为一类的过程。 一个类所描述的是在层次结构中具有相同语义的影像对象, 所有类都是来自隶属层次结构层中的影像对象,它们所构 成的关系结构就称为类层次结构。
上图为Definiens Professional打开的两个窗口的用户界面,左窗显示为分 类结果,右窗显示的是影像数据,最高处为菜单栏和工具条,在最右侧为规 则树和类层窗口,以及特征窗口和影像对象信息窗口都被隐藏了
2 .1 Definiens工程文件
面向对象分类
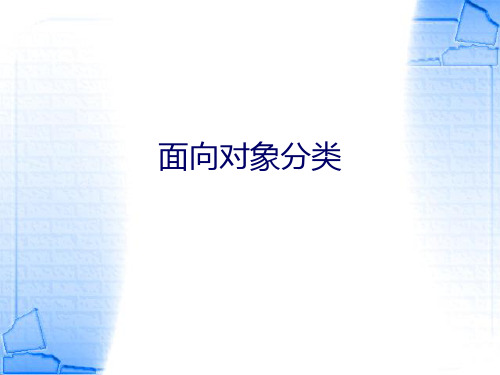
指定分类的算法参数
注释: 对于一个规则,您也可以添加自己的标注,您可以在打开编辑对话窗口中点击 注释图标 通过插入注释可以使规则变得容易理解和输入一些必须的信息。
规则可以包含任意数量的子规则,它们所显示的结果是 影像分析所定义的结构和流量控制图,规则包含很多不同类 型算法,允许用户建立一个连续图像分析流程。
4、应用分类规则:选择菜单“Classification -> Nearest Neighbor -> Apply Standard NN to Classes”把它插入到类描 述中,选择左边框中的类,单击,即可将该类加入到右边的框 中,如下图:
面向对象影像分类步骤(基于样本)
点击OK后,在Class Hierarchy中双击一个类,如草地,可 以看出分类特征已经添加到该类中,如下图:
循环次数
算法参数
在这个项中您能读到您在规则树中所设置的所有参量。
如图:自动命名的规则 上述例子命名解释:
。 所有对象的Mean nir特征值若小于200在第一层级将被分类为水体
算法(Algorithm):
从下拉菜单中选择您想要进行的算法,依据所选择的算法,在编 辑对话框右侧部分的规则集合设置的算法参数也将发生变化并显示。
➢ 影像分割是对遥感影像进行进一步面向对象分析、理解 和识别的基础,是高分辨率遥感影像应用领域的关键技 术之一。
影像分割
主要利用光谱特征,形状特征调整地块边界
Smoothness量小说明该对象边界比较光滑 Compactness表示对象紧凑程度。
基于区域合并的多尺度分割
分割
eCognition 中的分割算法
面向对象分类
易康简介
(Definiens professional 8.0) 基础应用
基于eCognition和Google earth影像的土地利用分类
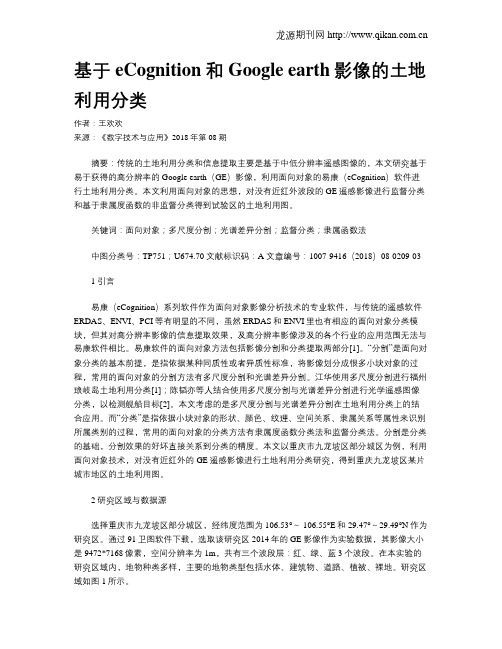
基于eCognition和Google earth影像的土地利用分类作者:王欢欢来源:《数字技术与应用》2018年第08期摘要:传统的土地利用分类和信息提取主要是基于中低分辨率遥感图像的,本文研究基于易于获得的高分辨率的Google earth(GE)影像,利用面向对象的易康(eCognition)软件进行土地利用分类。
本文利用面向对象的思想,对没有近红外波段的GE遥感影像进行监督分类和基于隶属度函数的非监督分类得到试验区的土地利用图。
关键词:面向对象;多尺度分割;光谱差异分割;监督分类;隶属函数法中图分类号:TP751;U674.70 文献标识码:A 文章编号:1007-9416(2018)08-0209-031 引言易康(eCognition)系列软件作为面向对象影像分析技术的专业软件,与传统的遥感软件ERDAS、ENVI、PCI等有明显的不同,虽然ERDAS和ENVI里也有相应的面向对象分类模块,但其对高分辨率影像的信息提取效果,及高分辨率影像涉及的各个行业的应用范围无法与易康软件相比。
易康软件的面向对象方法包括影像分割和分类提取两部分[1]。
“分割”是面向对象分类的基本前提,是指依据某种同质性或者异质性标准,将影像划分成很多小块对象的过程,常用的面向对象的分割方法有多尺度分割和光谱差异分割。
江华使用多尺度分割进行福州琅岐岛土地利用分类[1];陈韬亦等人结合使用多尺度分割与光谱差异分割进行光学遥感图像分类,以检测舰船目标[2]。
本文考虑的是多尺度分割与光谱差异分割在土地利用分类上的结合应用。
而“分类”是指依据小块对象的形状、颜色、纹理、空间关系、隶属关系等属性来识别所属类别的过程,常用的面向对象的分类方法有隶属度函数分类法和监督分类法。
分割是分类的基础,分割效果的好坏直接关系到分类的精度。
本文以重庆市九龙坡区部分城区为例,利用面向对象技术,对没有近红外的GE遥感影像进行土地利用分类研究,得到重庆九龙坡区某片城市地区的土地利用图。
基于卫星遥感影像的城市建筑物分类提取研究

基于卫星遥感影像的城市建筑物分类提取研究摘要遥感技术是根据电磁波的理论,应用各种传感仪器对远距离目标所辐射和反射的电磁波信息,进行收集、处理,并最后成像,从而对地面各种景物进行探测和识别的一种综合技术。
作为空间地理信息系统建设的一种有效方法,在智慧城市建设中发挥着重要作用。
文章通过应用遥感影像进行城市建筑物自动化分类提取方法的研究,为相关技术应用于智慧城市建设提供参考。
关键词:智慧城市;遥感影像;建筑物;自动化提取1.研究背景随着经济社会的高速发展,城市面貌也在发生着巨大的变化,为了更科学有效的进行城市规划、建设和管理,城市建设管理部门需要及时掌握城市建设变化情况。
在远程遥感观测技术出现之前,人们主要通过工程测量等方法来收集城市信息,进行城市规划与管理。
卫星遥感技术以其大范围、快速准确获取地面信息的优越性,越来越广泛应用于土地利用、城市规划与管理等领域。
在智慧城市建设中,对于建筑物的识别提取是极其重要的,如何识别建筑物从而更好的分类和提取也直接关系到建筑物提取的自动化程度。
此外,由于城市建设的不断发展,城市建筑物不断更新变化,因此,对建筑物进行有效的识别与提取是一个非常关键的问题,找到一种准确高效且自动化的高分遥感建筑物提取方法来替代人工方式具有重要意义。
2.研究现状目前的建筑物提取主要是通过利用图像特征信息来进行图像建筑物的识别以及提取。
这些年来,国内外的很多学者都专注于对遥感影像建筑物进行精确识别,以及在影像上提取出建筑物等信息的研究,并且提出了很多的方法和理论,也取得了一定的成果。
比如利用建筑物的位置关系对相似的建筑物进行过滤,最后通过图割算法实现对建筑物的精确提取;过Mask R-CNN算法来实现对建筑物的矢量提取;通过人工提取的方法对房屋进行提取,从而实现对建筑物房屋位置、范围等的初步自动化提取等。
在国外,Janja Avbelj and Rupert Muller提出了一种新的彩色高分辨率遥感图像建筑物提取方法。
易康-ETM+影像土地覆盖分类详细介绍

Hands on Exercise Using eCognition DeveloperGo the Windows Start menu and Click Start > All Programs> eCognition Developer 8.0> eCognition DeveloperUpon launching Definiens eCognition Developer 8, the following dialog appears:Figure: eCognition 8 launch screenFigure : The default display eCognition 81.Create a New Project• To Create New Project do the following:• Choose File > New Project on the main menu bar.• Navigate the folder C:\GISRS_Trn\Definiens• Select Image.img > Open (Here is image file Landsat ETM+, R136/P44)• Then select from the appropriate file in the files type.To open some specific file formats orstructures, you have to proceed as follows:• First select the correct driver inthe Files of type drop-down list box•Double-Click on Image Layer AliasRename the all layers name• Click>OK• Click> Insert > Select DEM.imgand Slope> Open• Double-Click on Layer Alias Rename the all the layers name Layer 1 (Blue),Layer 2 (Green), Layer 3 (Red), Layer 4 (Near IR), Layer 5 (Mid-IR), Layer 7 (Mid-IR), Layer 8 (DEM), Layer 8 (Slope)• Click > File> Save Project > Test.dpr1.1Subset SelectionNormally, image files are large in size and difficult to process. So we will be working with a smaller area to manage easily, which will take less memory and time. You can crop your image on the fly in the viewer by using Subset option without changing your original image file. You can create a "subset selection"when you start a project or during modification.To open the Subset Selection dialog box, do the following:• After importing image layers press the Subset Selection button.• Click on the image and Drag to select a subset area in the image viewer.• Alternatively, you may enter the subset coordinates. You can modify the coordinates by typing.• Confirm with OK to return to the superordinate dialog box.• You can clear the subset selection by Click ing Clear Subset in the superordinate dialog box.1.2Insert Thematic LayerGeographic representations are organized in a series of data themes, which are known as thematic layers. During the image classification with eCognition, you can insert shape file as a thematic layer and you can also use it in the process of image classification (if required).During the new project creating or modifying time, Shape files or other vector files can be inserted to viewer. To insert a thematic layer, do the followings:• Click the Insert button• Choose Thematic Layers > Insert on the menu bar of the dialog box.• Right-Click inside the thematic layer list and choose Insert from the context menu.The Import Thematic Layer dialog box opens, which is similar to the Import Image Layers dialog box.1.2.1Modify a ProjectUsing Modify a Project you can add/remove more image or thematic layer or you can rename project. Modify a selected project by exchanging or renaming image layers or through other operations.To modify a project, do the followingOpen a project and choose File > Modify Open Project on the main menu bar.• The Modify Project dialog box opens.• Modify the necessary things• Click OK to modify the project• Save a ProjectSave the currently open project to a project file (extension .dpr ).To save a project, do the following:• Choose File > Save Project on the main menu bar.• Choose File > Save Project As… on the main menu bar. The Save Project dialog box opens. Select a folder and enter a name for the project file (.dpr ). Click the Save button to store the file.2. Image Objects by SegmentationThe fundamental step of any eCognitionimage analysis is to do segmentation of ascene— representing an image—into imageobject primitives. Thus, initial segmentation isthe subdivision of an image into separatedregions represented by basic unclassifiedimage objects called image object primitives.For successful and accurate image analysis,defining object primitives of suitable size andshape is of utmost importance. As a rule ofthumb, good object primitives are as large aspossible, yet small enough to be used as building blocks for the objects to be detectedin the image. Pixel is the smallest possible building block of an image, however it has mixture of information. To get larger buildingblocks, different segmentation methods areavailable to form contiguous clusters of pixelsthat have larger property space.Commonly, in image processing,segmentation is the subdivision of a digitalimage into smaller partitions according togiven criteria. Different to this, within theeCognition technology, each operation thatcreates new image objects is calledsegmentation, no matter if the change isalgorithms provide several methods ofcreating of image object primitives.The new image objects created bysegmentation are stored in a new imageobject level. Each image object is definedby a contiguous set of pixels, where eachpixel belongs to exactly one image object.Each of the subsequent image objectrelated operations like classification,reshaping, re-segmentation, andobject levels serve as internal working areasof the image analysis.1.3 Classification of Land Cover Using Landsat ETM+ ImageImage Classification is a process of sorting pixels into a number of data categories based on their data file values and reducing images to information classes. Similar features will have similar spectral responses. The spectral response of a feature is unique with respect to all other features of interest. If we quantify the spectral response of a known feature in an image, we can use this information to find all occurrences of that feature throughout the image.1.3.1 Display the Image or Edit the Image Layer MixingDisplay the Image or Edit the Image Layer Mixing is one kind of band combination process. Often an image contains valuable information about vegetation or land features that is not easily visible until viewed in the right way. For this reason, in eCognition, you have to use Display the Image or Edit the Image Layer Mixing . The most fundamental of these techniques is to change the arrangement of the bands of light used to make the image display. In order to display an image in eCognition, assigns one or RGB color to each of up to three bands of reflected visible or non-visible light.You have the possibility to change the display of the loaded data using the ‘Edit Layer Mixing’ dialog box. This enables you to display the individual channels of a combination.• To open the ‘Edit Image Layer Mixing’, do one of the following:• From the View menu, select Image Layer Mixing• Click View > Image Layer Mixing on the main menu bar.Or Click on the Edit Image Layer Mixing button in the View Settings toolbar.Figure: Edit Image Layer Mixing dialog box. Changing the layer mixing and equalizing options affects the display of the image onlyChoose a layer mixing preset:∙(Clear): All assignments and weighting are removed from the Image Layer table ∙One Layer Gray displays one image layer in grayscale mode with the red, green and blue together∙False Color (Hot Metal) is recommended for single image layers with large intensity ranges to display in a color range from black over red to white. Use this preset for image data created with positron emission tomography (PET) ∙False Color (Rainbow) is recommended for single image layers to display a visualization in rainbow colors. Here, the regular color range is converted to acolor range between blue for darker pixel intensity values and red for brighterpixel intensity values∙Three Layer Mix displays layer one in the red channel, layer two in green and layer three in blue∙Six Layer Mix displays additional layers.∙For current exercise change the band combinations (B7, B2, and B1) and Equalizing Histrogram any others∙Click> OK1.4Create Image ObjectsThe fundamental step of any eCognition image analysis is a segmentation of a scene—representing an image—into image objects. Thus, initial segmentation is the subdivision of an image into separated regions represented by basic unclassified image objects called ‘Image Object Primitives’.1.5View Settings ToolbarThe View Settings Toolbar buttons, numbered from one to four, allow you to switch between the four window layouts. These are Load and Manage Data, Configure Analysis, Review Results and Develop Rule Sets. As much of the User Guide centers around writing rule sets – which organize and modify image analysis algorithms – the view activated by button number four, Develop Rule Sets, is most commonly usedIn the ‘View Settings’ toolbar there are 4 predefined View Setting s available, specific to the different phases of a Rule Set development workflow.View Settings toolbar with the 4 predefined View Setting buttons: Load and Manage Data, Configure Analysis, Review Results, Develop Rule Sets.Select the predefined View Setting number 4 ‘Develop Rulesets’ from the ‘View Settings’ toolbar.For the ‘Develop Rulesets’ view, per default one viewer window for the image data is open, as well as the ’Process Tree’ and the ‘Image Object Information’ window, the ‘Feature View’ and the ‘Class Hierarchy1.6Insert Rule for Object CreationThis is the first step of image classification in eCognition. This is a kind of assigning condition/s. Based on this, it will create image objects or segments. Within the rule sets, you can use variables in different ways. While developing rule sets, you commonly use scene and object variables for storing your dedicated fine-tuning tools for reuse within similar projects.1.6.1Insert a Process1.6.1.1Insert a Parent ProcessA parent process is used for grouping child processes together in a hierarchy level. The typical algorithm of the parent process is "Execute child process".• To open the Process Tree window Click Process> Process Tree• Go to the Process Tree window, which might be empty since you did not putany process yet.1.6.1.2Insert a Segmentation Parent Process• Right-Click in the ‘Process Tree’ window and select ‘Append New’ from thecontext menu.New Dialog box (Edit Process) will be appeared.In the ‘Name’ field enter the name ‘Segmentation’ and confirm with ‘OK’. It will be your Parents of Segmentation.1.6.1.2.1Insert a Child Process ( Multiresolution Segmentation)The child processes algorithm in conjunction with the no image object domain to structure to your process tree. A process with this setting serves as a container for a sequence of function related processes.The first crucial decision you have to make is which algorithm to be used for creating objects. The initial objects you create will be the basis for all further analysis. Multiresolution Segmentation creates groups of areas of similar pixel values into objects. Consequently, homogeneous areas result in larger objects, heterogeneous areas in smaller ones.• Select the inserted Segmentation Process and Right-Click on it. Choose ‘Insert Child’ form the context menu.• Click Algorithm > Select Multiresolution Segmentations• Give the level name (Level-1)• Change the image layer weights• Change the scale parameter and etc.• Click > OKWhich layers to be used for creating Objects?The basis of creating image objects is the input-data. According to the data and the algorithm you use, objects results in different shapes. The first thing you have to evaluate, which layers contain the important information. For example, we have two types of image data, the Image and the DEM. In most Segmentation algorithms you can choose whether you want to use all data available or only specific layer. It depends on where the important information is contained. In our case, we want to use VIS and NIR band for image object creation.Which Scale Parameter to be set?The ‘Scale parameter’ is an abstract term. It is the restricting parameter to stop the objects from getting too heterogenity. For the ‘Scale parameter’ there is no definite rule, you have to use trial and error to find out which ‘Scale parameter’ results in the objects is useful for your further classification.• Right-Click one the process and select execute to execute the MultiresolutionSegmentation process.1.7Create Relational Feature• To open the Relational Feature window, Click Tools> Feature ViewFeature View will be appeared.• Double-Click on Create new ‘Arithmetic Feature’, Edit Customize Feature will be appeared• Assign the Feature name > NDVIThe Normalized Difference Vegetation Index (NDVI)is a simple numerical indicator that can be used to analyze remote sensing measurements. NDVI is related to vegetation, where healthy vegetation reflects very well in the near infrared part of the spectrum. Index values can range from -1.0 to 1.0, but vegetation values typically range between 0.1 and 0.7.Free standing water (ocean, sea, lake, river, etc.) gives a rather low reflectance in both spectral bands and thus result in very low positive or even slightly negative NDVI values.Soils which generally exhibit a near-infrared spectral reflectance somewhat larger than the red, and thus tend to also generate rather small positive NDVI values (say 0.1 to 0.2).NDVI = (NIR - red) / (NIR + red)NDVI (ETM+) = (Band 4 - Band 3) / (Band 4 + Band 3)• Double-Click on Layer Values and then Mean Layer appear• Double-Click on Landsat ETM+ band and complete the formula for NDVIFor NDVI = ([Mean Layer 4 (Near IR)]-[Mean Layer 3 (Red)])/([Mean Layer 4(Near IR)]+[Mean Layer 3 (Red)])• Click >OKLand & Water Mask (LWM)Land and Water Mask index is a very useful tool to differentiate between land and water. This is very important variable to classify all type of waterbodies. Index values can range from 0 to 255, but water values typically range between 0 and 50.Water Mask = (MIR) / (Green) * 100• Assign the Feature name > Land & Water MaskLand & Water Mask (LWM) = [Mean Layer 5 (Mid-IR)]/([Mean Layer 2(Green)])*100Click > OK1.8Insert the Class/Class HierarchyNew Dialog box will be appear• On the Class Hierarchy Right-Click and Choose ‘Insert Class’ form the context menu and Class description dialog Box will be appeared,• On the Class description, give the class name Deep To Medium Deep Perennial Natural Waterbodies and Click> OK1.9Insert a Classification Parent Process• Right-Click in the ‘Process Tree’ window and select ‘Append New’ from the context menu.New Dialog box will be appeared.In the ‘Name’ field enter the name ‘Classification’ and confirm with ‘OK’. It will be your parents of Classification• Select the inserted Classification Process and Right-Click on it. Choose ‘Insert Child’ form the context menu.• In the ‘Name’ field, enter the name Perennial Natural Waterbodies and confirm with ‘OK’. It will be your Parents Class for a particular class (in this case, for Deep to Medium Perennial Natural Waterbodies Class).1.9.1Assign Class AlgorithmThe Assign Class algorithm is the most simple classification algorithm. It determines by means of a threshold condition whether the image object is a member of the class or not.This algorithm is used when one threshold condition is sufficient to assign an Image Object to a Class.Classify the Deep To Medium Deep Perennial Natural Waterbodies• Select the inserted Classification Process and Right-Click on it. Choose ‘Insert Child’ form the context menu and Assign Class Algorithm• In the Edit Process dialog box, select assign class from the Algorithm list.• In the algorithm parameter Use class, select Deep To Medium Deep Perennial Natural Waterbodies.• In the Image Object Domain group Click > Select image object level• In the Image Object Domain group set the Parameter Click on Level> Select Level-1• In the Class Filter dialog box, Select unclassified from the classification list.• In the Image Object Domain (Parameter) group Click the Threshold condition; it is labeled … if condition is not selected yet.• From the Select Single Feature box’s Double-Click on Land & Water Mask (LWM) assign the threshold <= 20 Click > OK to apply your settings• Right-Click one the process and select execute to execute the Perennial Natural Waterbodies process or Using F5 Execute the Process.2.5 Classify the Lake• Select the inserted Classification Process and Right-Click on it. Choose ‘Insert Child’ form the context menu.• In the ‘Name’ field, enter the name Lake and confirm with ‘OK’1.9.2Assign Class Algorithm for LakeThe Assign Class algorithm is the most simple classification algorithm. It determines by means of a threshold condition whether the image object is a member of the class or not.This algorithm is used when one threshold condition is sufficient to assign an Image Object to a Class.Classify the Lake• Select the inserted Classification Process and Right-Click on it. Choose ‘Insert Child’ form the context menu and Assign Class Algorithm• In the Edit Process dialog box, select assign class from the Algorithm list.• In the algorithm parameter Use class, select Lake.• In the Image Object Domain group Click > Select image object level• In the Image Object Domain group set the Parameter Click on Level> Select Level-1• In the Class Filter dialog box, Select unclassified from the classification list. • In the Image Object Domain (Parameter) group Click the Threshold condition; it is labeled … if condition is not selected yet.• From the Select Single Feature box’s Double-Click on Land & Water Mask (LWM) assign the threshold <= 52 Click > OK to apply your settings• Right-Click one the process and select execute to execute the Lake process or Using F5 Execute the Process.*Note: Based on the LWM algorithm others land cover area has been classified as Lake. So you have to use few more conditions for refining the Lake area.• In the Edit Process dialog box, select merge region from the Algorithm list and Fusion super objects Yes• In the Image Object Domain Select Level-1 and In the Class filter Select > Lake > OK• Using F5 Execute the algorithm• In the Edit Process dialog box, select assign class from the Algorithm list and Use class unclassified• In the Image Object Domain select image object level and parameter Level > Level-1, Class> Lake• In the parameter Click on Threshold condition and to apply your bellow settingsFeature select Area and Threshold <= 3600000• Using F5 Execute the Lake algorithmFigure Classified lake area2.6 Classify the River• Select the inserted Classification Process and Right-Click on it. Choose ‘Insert Child’ form the context menu.• In the ‘Name’ field, enter the name River and confirm with ‘OK’1.9.3Assign Class Algorithm for RiverThe Assign Class algorithm is the most simple classification algorithm. It determines by means of a threshold condition whether the image object is a member of the class or not.This algorithm is used when one threshold condition is sufficient to assign an Image Object to a Class.Classify the River• Select the inserted Classification Process and Right-Click on it. Choose ‘Insert Child’ form the context menu and Assign Class Algorithm• In the Edit Process dialog box, select assign class from the Algorithm list. • In the algorithm parameter Use class, select River.• In the Image Object Domain group Click > Select image object level• In the Image Object Domain group set the Parameter Click on Level> Select Level-1• In the Class Filter dialog box, Select unclassified from the classification list. • In the Image Object Domain (Parameter) group Click the Threshold condition; it is labeled … if condition is not selected yet.• From the Select Single Feature box’s Double-Click on Land & Water Mask (LWM) assign the threshold <= 34 Click > OK to apply your settings• Right-Click one the process and select execute to execute the River process orUsing F5 Execute the Process.*Note: Based on the LWM algorithm others land cover area has been classified as River. So you have to use few more conditions for refining the River area.• In the Edit Process dialog box, select assign class from the Algorithm list and Use class unclassified• In the Image Object Domain select image object level and parameter Level > Level-1, Class> River• In the parameter Click on Threshold condition and to apply your bellow settingsFeature select Length/Area and Threshold <= 1.6Similar way add following condition for river and • Using F5 Execute the Lake algorithm2.7 Classify the Broadleaved Tree Crop• Select the inserted Classification Process and right-click on it. Choose ‘Insert Child’ form the context menu.New Dialog box will be appearIn the ‘Name’ field enter the name ‘Broadleaved Tree Crop’ and confirm with‘OK’. It will be your parents of Classification• In the Edit Process dialog box, select assign class from the Algorithm list.Classify the Broadleaved Tree Crop• Select the inserted Classification Process and Right-Click on it. Choose ‘Insert Child’ form the context menu and Assign Class Algorithm• In the Edit Process dialog box, select assign class from the Algorithm list. • In the algorithm parameter Use class, select Broadleaved Tree Crop.• In the Image Object Domain group Click > Select image object level• In the Image Object Domain group set the Parameter Click on Level> Select Level-1• In the Class Filter dialog box, Select unclassified from the classification list. • In the Image Object Domain (Parameter) group Click the Threshold condition; it is labeled … if condition is not selected yet.• From the Select Single Feature box’s Double-Click on NDVI assign the threshold => 0.35 Click > OK to apply your settings• Right-Click one the process and select execute to execute the Broadleaved Tree Crop process or Using F5 Execute the Process.*Note: Based on the LWM algorithm others land cover area has been classified as Broadleaved Tree Crop. So you have to use few more conditions for refining the Broadleaved Tree Crop area.Similar way add other condition for Broadleaved Tree Crop and • Using F5 Execute the Broadleaved Tree Crop algorithmPlease set following condition for others land coverBare Soil in seasonally flooded areaBare SoilUrban andIndustrial AreasIrrigated Herbaceous CropRainfed Herbaceous CropClosed toOpenRoot ed ForbClosed to OpenGrasslandSmallHerbaceousCropsin sloping landClosed to Open Seasonally Flooded ShrubsClosed to Open ShrublandSmall Sized Field Of Tree CropBroadleaved Tree CropBroadleaved Open ForestBroadleaved Closed ForestClassified Land cover*Note The entire classification process shown base on single variable. For better results more variable need to use.2.8 Manual EditingManual editing of image objects and thematic objects allows you to manually influence the result of an image analysis. The main manual editing tools are Merge Objects Manually, Classify Image Objects Manually and Cut an Object Manually.While manual editing is not commonly used in automated image analysis, it can be applied to highlight or reclassify certain objects or to quickly improve the analysis result without adjusting the applied rule set.To open the Manual Editing toolbar choose View > Toolbars > Manual Editing on the main menu.Change Editing ModeThe Change Editing Mode drop-down list on the Manual Editing toolbar is set to Image Object Editing by default. If you work with thematic layers and want to edit them by hand, choose Thematic editing from the drop-down list.Selection ToolsObjects to be fused or classified can be selected from the Manual Editing toolbar in one of the following ways:1 Single Selection Mode selects one object. Select the object with a single click.2 Polygon Selection selects all objects that lie within or touch the border of a polygon.Set vertices of the polygon with a single click. Right-click and choose Close Polygon to close the polygon.3 Line Selection selects all objects along a line. Set vertices of the line with a singleclick. A line can also be closed to form a polygon by right-clicking and choosing Close Polygon. All objects that touch the line are selected.4 Rectangle Selection selects all objects within or touching the border of a rectangle.Drag a rectangle to select the image objects.Merge Objects ManuallyThe manual editing tool Merge Objects is used to manually merge selectedneighboring image or thematic objects.Note: Manual object merging operates only on the current image object level.Tools > Manual Editing > Merge Objects from the main menu bar or press the Merge Objects Manually button on the Manual Editing toolbar to activate the input mode. Or you can use right click.Note: You should have at list two objects.2.9 Classify Image Objects ManuallyThe manual editing tool Classify Image Objects allows easy class assignment of selected image objects.Manual image object classification can be used for the following purposes:• Manual correction of previous classification results including classification ofpreviously unclassified objects.• Classification without rule sets (in case the creation of an appropriate rule set ismore time-consuming), using the initial segmentation run for automated digitizing.Precondition: To classify image objects manually, the project has to contain at leastone image object level and one class in the Class Hierarchy.To perform a manual classification, do one of the following:• Choose Tools > Manual Editing > Classify Image Objects from the menu bar.• Click the Classify Image Objects button on the Manual Editing toolbar to activatethe manual classification input mode.In the Select Class for Manual Classification drop-down listbox, select the class to which you want to manually assignobjects. Note that selecting a class in the Legend window or inthe Class Hierarchy window (if available) will not determine theclass for manual editing; the class has to be selected from thebefore-mentioned drop-down list.Now objects can be classified manually with a single mouse-click. To classify objects, do one of the following:• Select the Classify Image Objects button and the Class for ManualClassification. Click the image objects to be classified.• Select the image object(s) you want to classify first. Select the Class for Manual Classification and press the Classify Image Objects button to classify all selected objects.• Select one or more image objects, right-click into the image object(s) and select Classify Selection from the context menu.When the object is classified, it is painted in the color of the respective class.If no class is selected, a mouse-click deletes the previous class assignment; the image object becomes unclassified.To undo a manual classification on a previously unclassified object, simply click the object a second time. If the object was previously classified, then clicking again does not restore the former classification; instead, the object becomes unclassified.。
基于面向对象分类的密云县城区地面不透水程度分析

M iu r a ra fC ia S On e in b s do be to in e t o t a d a y n u b nae so h n ’ igr go a e no jc—re tdmeh dwi L n st Be h TM aai d t n 2 0 .Th p r ah man yiv le sa l h n ni g be t irrh o s tn f h e v l o 06 ea p o c il ov det bi iga n s ma eo jc e ac yc n i igo r el es f h s t e
基 于 面 向对 象 分 类 的 密 云 县 城 区地 面 不 透 水 程 度 分 析
谭 衢 霖 , 东彪 徐
( 京 交 通 大 学 土 木 建 筑 工 程 学 院 , 京 10 4 ) 北 北 0 04
摘
要 : 用 L n st 利 a da 影像 数 据 , TM 试验 了一种基 于面 向对 象分 类分析 的城 区地 面不透 水 程度 分
于像 素 的遥感影 像分类 方法 难 以令 人满意 .
基 于 ( 面向 ) 或 对象 影像 分类分 析方法 中影像 分 类 的基本 单元不 是像素 , 而是 影像 对象或 区域 . 对 相 于基 于像素 的遥 感影 像 分 类 方法 , 向对象 影 像 分 面 类 的最大优 点是 基于影 像对 象生成 了大量 可用 的新
o jc-re tdca sf ain:A c s td b t in e lsi c t e o i o aesu y
T AN l Qu i n.XU n ba Do g io
( c o l f iiE gn eig e i i tn i r t , e i 0 0 4 C i ) S h o o vl n i r ,B i gJ oo g Unv s y B in 1 0 4 , hn C e n j n a ei jg a
基于面向对象的无人机正射影像地物分类

基于面向对象的无人机正射影像地物分类宋雪莲;阮玺睿;张威;张文;丁磊磊;雷霞;谢彩云;陈伟;王志伟【期刊名称】《测绘科学技术》【年(卷),期】2018(006)003【摘要】无人机航拍能够快速准确获取地表的高分辨率影像,已经成为遥感数据获取的重要手段之一。
采用eCognition软件面向对象分类方法,对无人机影像进行地物分类研究。
通过ENVI OneButton生成无人机正射镶嵌影像,选择合适的分割参数对实验区影像进行多尺度分割,找出最优的分割尺度。
利用eCognition特征优化功能选择最优对象特征组合,进行最近邻分类。
结果表明,分类的总体精度达到83%,Kappa达到0.8,采用eCognition面向对象的分类方法能够较为准确地得到地物覆盖信息。
利用无人机技术和eCognition面向对象分类方法,可充分利用影像的光谱信息和形状、纹理等空间信息,能够实现地物信息的快速、准确提取。
【总页数】9页(P165-173)【作者】宋雪莲;阮玺睿;张威;张文;丁磊磊;雷霞;谢彩云;陈伟;王志伟【作者单位】[1]贵州省农业科学院草业研究所,贵州贵阳;[1]贵州省农业科学院草业研究所,贵州贵阳;[2]贵州省水利水电勘测设计研究院,贵州贵阳;[1]贵州省农业科学院草业研究所,贵州贵阳;[3]贵州阳光草业科技有限责任公司,贵州贵阳;[1]贵州省农业科学院草业研究所,贵州贵阳;[1]贵州省农业科学院草业研究所,贵州贵阳;[1]贵州省农业科学院草业研究所,贵州贵阳;[1]贵州省农业科学院草业研究所,贵州贵阳;[1]贵州省农业科学院草业研究所,贵州贵阳【正文语种】中文【中图分类】TP39【相关文献】1.基于面向对象的QUICKBIRD数据和SAR数据融合的地物分类 [J], 王宇航;范文义;刘超逸2.基于面向对象分类方法在SPOT影像中的地物信息提取 [J], 祖琪;袁希平;莫源富;袁磊3.基于无人机遥感和面向对象法的简单地物分类研究 [J], 袁慧洁4.面向对象的无人机影像地物分类 [J], 马飞虎;徐发东;孙翠羽5.基于Wordview-3数据的面向对象地物分类研究 [J], 叶蕾;蒋永泉;沈润;罗琪因版权原因,仅展示原文概要,查看原文内容请购买。