近似动态规划相关的外文文献及翻译
本科毕业论文外文翻译【范本模板】
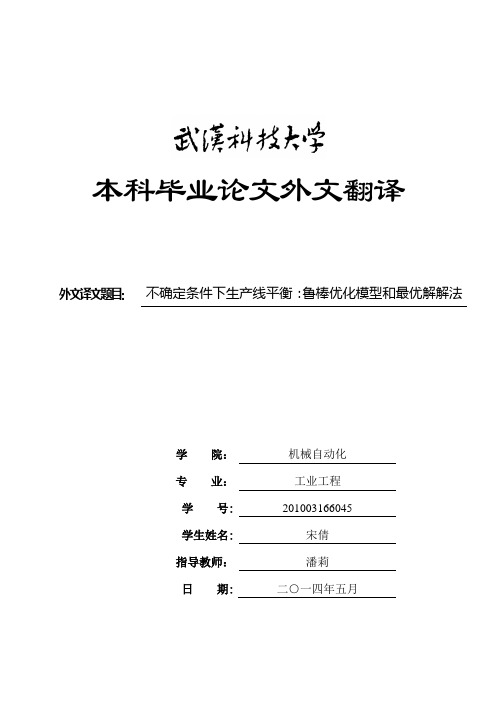
本科毕业论文外文翻译外文译文题目:不确定条件下生产线平衡:鲁棒优化模型和最优解解法学院:机械自动化专业:工业工程学号: 201003166045学生姓名: 宋倩指导教师:潘莉日期: 二○一四年五月Assembly line balancing under uncertainty: Robust optimization modelsand exact solution methodÖncü Hazır , Alexandre DolguiComputers &Industrial Engineering,2013,65:261–267不确定条件下生产线平衡:鲁棒优化模型和最优解解法安库·汉泽,亚历山大·多桂计算机与工业工程,2013,65:261–267摘要这项研究涉及在不确定条件下的生产线平衡,并提出两个鲁棒优化模型。
假设了不确定性区间运行的时间。
该方法提出了生成线设计方法,使其免受混乱的破坏。
基于分解的算法开发出来并与增强策略结合起来解决大规模优化实例.该算法的效率已被测试,实验结果也已经发表。
本文的理论贡献在于文中提出的模型和基于分解的精确算法的开发.另外,基于我们的算法设计出的基于不确定性整合的生产线的产出率会更高,因此也更具有实际意义。
此外,这是一个在装配线平衡问题上的开创性工作,并应该作为一个决策支持系统的基础。
关键字:装配线平衡;不确定性; 鲁棒优化;组合优化;精确算法1.简介装配线就是包括一系列在车间中进行连续操作的生产系统。
零部件依次向下移动直到完工。
它们通常被使用在高效地生产大量地标准件的工业行业之中。
在这方面,建模和解决生产线平衡问题也鉴于工业对于效率的追求变得日益重要。
生产线平衡处理的是分配作业到工作站来优化一些预定义的目标函数。
那些定义操作顺序的优先关系都是要被考虑的,同时也要对能力或基于成本的目标函数进行优化。
就生产(绍尔,1999)产品型号的数量来说,装配线可分为三类:单一模型(SALBP),混合模型(MALBP)和多模式(MMALBP)。
自然语言处理及计算语言学相关术语中英对译表
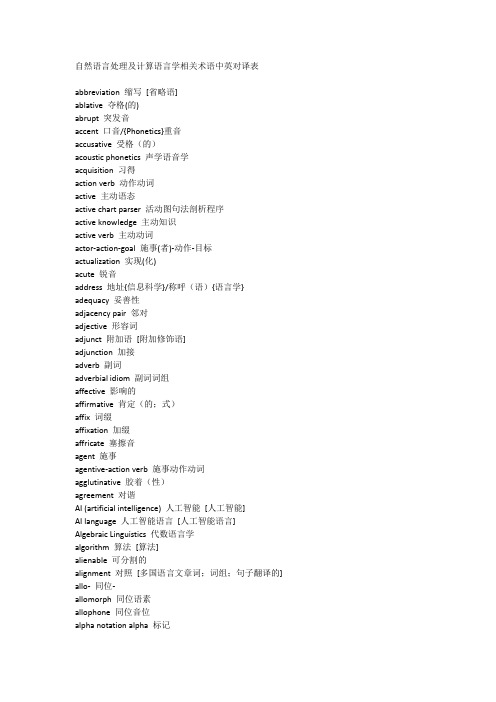
自然语言处理及计算语言学相关术语中英对译表abbreviation 缩写[省略语]ablative 夺格(的)abrupt 突发音accent 口音/{Phonetics}重音accusative 受格(的)acoustic phonetics 声学语音学acquisition 习得action verb 动作动词active 主动语态active chart parser 活动图句法剖析程序active knowledge 主动知识active verb 主动动词actor-action-goal 施事(者)-动作-目标actualization 实现(化)acute 锐音address 地址{信息科学}/称呼(语){语言学} adequacy 妥善性adjacency pair 邻对adjective 形容词adjunct 附加语[附加修饰语]adjunction 加接adverb 副词adverbial idiom 副词词组affective 影响的affirmative 肯定(的;式)affix 词缀affixation 加缀affricate 塞擦音agent 施事agentive-action verb 施事动作动词agglutinative 胶着(性)agreement 对谐AI (artificial intelligence) 人工智能[人工智能]AI language 人工智能语言[人工智能语言]Algebraic Linguistics 代数语言学algorithm 算法[算法]alienable 可分割的alignment 对照[多国语言文章词;词组;句子翻译的] allo- 同位-allomorph 同位语素allophone 同位音位alpha notation alpha 标记alphabetic writing 拼音文字alternation 交替alveolar 齿龈音ambiguity 歧义ambiguity resolution 歧义消解ambiguous 歧义American structuralism 美国结构主义analogy 类推analyzable 可分析的anaphor 照应语[前方照应词]animate 有生的A-not-A question 正反问句antecedent 先行词anterior 舌前音anticipation 预期(音变)antonym 反义词antonymy 反义A-over-A A-上-A 原则apposition 同位语appositive construction 同位结构appropriate 恰当的approximant 无擦通音approximate match 近似匹配arbitrariness 任意性archiphoneme 大音位argument 论元[变元]argument structure 论元结构[变元结构] arrangement 配列array 数组articulatory configuration 发音结构articulatory phonetics 发音语音学artificial intelligence (AI) 人工智能[人工智能] artificial language 人工语言ASCII 美国标准信息交换码aspect 态[体]aspirant 气音aspiration 送气assign 指派assimilation 同化association 关联associative phrase 联想词组asterisk 标星号ATN (augmented transition network) 扩充转移网络attested 经证实的attribute 属性attributive 属性auditory phonetics 听觉语音学augmented transition network 扩充转移网络automatic document classification 自动文件分类automatic indexing 自动索引automatic segmentation 自动切分automatic training 自动训练automatic word segmentation 自动分词automaton 自动机autonomous 自主的auxiliary 助动词axiom 公理baby-talk 儿语back-formation 逆生构词(法)backtrack 回溯Backus-Naur Form 巴科斯诺尔形式[巴科斯诺尔范式] backward deletion 逆向删略ba-construction 把─字句balanced corpus 平衡语料库base 词基Bayesian learning 贝式学习Bayesian statistics 贝式统计behaviorism 行为主义belief system 信念系统benefactive 受益(格;的)best first parser 最佳优先句法剖析器bidirectional linked list 双向串行bigram 双连词bilabial 双唇音bilateral 双边的bilingual concordancer 双语关键词前后文排序程序binary feature 双向特征[二分征性]binding 约束bit 位[二进制制;比特]biuniqueness 双向唯一性blade 舌叶blend 省并词block 封阻[封杀]Bloomfieldian 布隆菲尔德(学派)的body language 肢体语言Boolean lattice 布尔网格[布尔网格]borrow 借移Bottom-up 由下而上bottom-up parsing 由下而上剖析bound 附着(的)bound morpheme 附着语素[黏着语素]boundary marker 界线标记boundary symbol 界线符号bracketing 方括号法branching 分枝法breadth-first search 广度优先搜寻[宽度优先搜索]breath group 换气单位breathy 气息音的buffer 缓冲区byte 字节CAI (Computer Assisted Instruction) 计算机辅助教学CALL (computer assisted language learning) 计算机辅助语言学习canonical 典范的capacity 能力cardinal 基数的cardinal vowels 基本元音case 格位case frame 格位框架Case Grammar 格位语法case marking 格位标志CAT (computer assisted translation) 计算机辅助翻译cataphora 下指Categorial Grammar 范畴语法Categorial Unification Grammar 范畴连并语法[范畴合一语法]causative 使动causative verb 使役动词causativity 使役性centralization 央元音化chain 炼chart parsing 表式剖析[图表句法分析]checked 受阻的checking 验证Chinese character code 中文编码[汉字代码]Chinese character code for information interchange 中文信息交换码[汉字交换码] Chinese character coding input method 中文输入法[汉字编码输入]choice 选择Chomsky hierarchy 杭士基阶层[Chomsky 层次结构]citation form 基本形式CKY algorithm (Cocke-Kasami-Younger) CKY 算法classifier 类别词cleft sentence 分裂句click 啧音clitic 附着词closed world assumption 封闭世界假说cluster 音群Cocke-Kasami-Younger algorithm CKY 算法coda 音节尾code conversion 代码变换cognate 同源(的;词)Cognitive Linguistics 认知语言学coherence 一致性cohesion 凝结性[黏着性;结合力]collapse 合并collective 集合的collocation 连用语[同现;搭配]combinatorial construction 合并结构combinatorial insertion 合并中插combinatorial word 合并词Combinatory Categorial Grammar 组合范畴语法comment 评论commissive 许诺[语行]common sense semantics 常识语意学Communication Theory 通讯理论[通讯论;信息论] Comparative Linguistics 比较语言学comparison 比较competence 语言知能compiler 编译器complement 补语complementary 互补complementary distribution 互补分布complementizer 补语标记complex predicate 复杂谓语complex stative construction 复杂状态结构complex symbol 复杂符号complexity 复杂度component 成分compositionality 语意合成性[合成性] compound word 复合词Computational Lexical Semantics 计算词汇语意学Computational Lexicography 计算词典编纂学Computational Linguistics 计算语言学Computational Phonetics 计算语音学Computational Phonology 计算声韵学Computational Pragmatics 计算语用学Computational Semantics 计算语意学Computational Syntax 计算句法学computer language 计算器语言computer-aided translation 计算机辅助翻译[计算器辅助翻译]computer-assisted instruction (CAI) 计算机辅助教学computer-assisted language learning 计算机辅助语言学习[计算器辅助语言学习] concatenation 串联concept classification 概念分类concept dependency 概念依存conceptual hierarchy 概念阶层concord 谐和concordance 关键词(前后文) 排序concordancer 关键词(前后文) 排序的程序concurrent parsing 并行句法剖析conditional decision 条件决定[条件决策]conjoin 连接conjunction 连接词(合取;逻辑积;"与";连词)conjunctive 连接的connected speech 连续语言Connectionist model 类神经网络模型Connectionist model for natural language 自然语言类神经网络模型[自然语言连接模型] connotation 隐涵意义consonant 子音[辅音]constituent 成分constituent structure tree 词组结构树constraint 限制constraint propagation 限制条件的传递[限定因素增殖]constraint-based grammar formalism 限制为本的语法形式Construct Grammar 句构语法content word 实词context 语境context-free language 语境自由语言[上下文无关语言]context-sensitive language 语境限定语言[上下文有关语言;上下文敏感语言] continuant 连续音continuous speech recognition 连续语音识别contraction 缩约control agreement principle 控制一致原理control structure 控制结构control theory 控制论convention 约定俗成[规约]convergence 收敛[趋同现象]conversational implicature 会话含义converse 相反(词;的)cooccurrence relation 共现关系[同现关系]co-operative principle 合作原则coordination 对称连接词[同等;并列连接]copula 系词co-reference 同指涉[互指]co-referential 同指涉coronal 前舌音corpora 语料库corpus 语料库Corpus Linguistics 语料库语言学corpus-based learning 语料库为本的学习correlation 相关性counter-intuitive 违反语感的courseware 课程软件[课件]coverb 动介词C-structure 成分结构data compression 数据压缩[数据压缩]data driven analysis 数据驱动型分析[数据驱动型分析]data structure 数据结构[数据结构]database 数据库[数据库]database knowledge representation 数据库知识表示[数据库知识表示] data-driven 数据驱动[数据驱动]dative 与格declarative knowledge 陈述性知识decomposition 分解deductive database 演译数据库[演译数据库]default 默认值[默认;缺省]definite 定指Definite Clause Grammar 确定子句语法definite state automaton 有限状态自动机Definite State Grammar 有限状态语法definiteness 定指degree adverb 程度副词degree of freedom 自由度deixis 指示delimiter 定界符号[定界符]denotation 外延denotic logic 符号逻辑dependency 依存关系Dependency Grammar 依存关系语法dependency relation 依存关系depth-first search 深度优先搜寻derivation 派生derivational bound morpheme 派生性附着语素Descriptive Grammar 描述型语法[描写语法]Descriptive Linguistics 描述语言学[描写语言学]desiderative 意愿的determiner 限定词deterministic algorithm 决定型算法[确定性算法] deterministic finite state automaton 决定型有限状态机deterministic parser 决定型语法剖析器[确定性句法剖析程序] developmental psychology 发展心理学Diachronic Linguistics 历时语言学diacritic 附加符号dialectology 方言学dictionary database 辞典数据库[词点数据库]dictionary entry 辞典条目digital processing 数字处理[数值处理]diglossia 双言digraph 二合字母diminutive 指小词diphone 双连音directed acyclic graph 有向非循环图disambiguation 消除歧义[歧义消除]discourse 篇章discourse analysis 篇章分析[言谈分析]discourse planning 篇章规划Discourse Representation Theory 篇章表征理论[言谈表示理论] discourse strategy 言谈策略discourse structure 言谈结构discrete 离散的disjunction 选言dissimilation 异化distributed 分布式的distributed cooperative reasoning 分布协调型推理distributed text parsing 分布式文本剖析disyllabic 双音节的ditransitive verb 双宾动词[双宾语动词;双及物动词] divergence 扩散[分化]D-M (Determiner-Measure) construction 定量结构D-N (determiner-noun) construction 定名结构document retrieval system 文件检索系统[文献检索系统] domain dependency 领域依存性[领域依存关系]double insertion 交互中插double-base 双基downgrading 降级dummy 虚位duration 音长{语音学}/时段{语法学/语意学}dynamic programming 动态规划Earley algorithm Earley 算法echo 回声句egressive 呼气音ejective 紧喉音electronic dictionary 电子词典elementary string 基本字符串[基本单词串]ellipsis 省略EM algorithm EM算法embedding 崁入emic 功能关系的empiricism 经验论Empty Category Principle 虚范畴原则[空范畴原理]empty word 虚词enclitics 后接成份end user 终端用户[最终用户]endocentric 同心的endophora 语境照应entailment 蕴涵entity 实体entropy 熵entry 条目episodic memory 情节性记忆epistemological network 认识论网络ergative verb 作格动词ergativity 作格性Esperando 世界语etic 无功能关系etymology 词源学event 事件event driven control 事件驱动型控制example-based machine translation 以例句为本的机器翻译exclamation 感叹exclusive disjunction 排它性逻辑“或”experiencer case 经验者格expert system 专家系统extension 外延external argument 域外论元extraposition 移外变形[外置转换]facility value 易度值feature 特征feature bundle 特征束feature co-occurrence restriction 特征同现限制[特性同现限制] feature instantiation 特征体现feature structure 特征结构[特性结构]feature unification 特征连并[特性合一]feedback 回馈felicity condition 妥适条件file structure 档案结构finite automaton 有限状态机[有限自动机]finite state 有限状态Finite State Morphology 有限状态构词法[有限状态词法]finite-state automata 有限状态自动机finite-state language 有限状态语言finite-state machine 有限状态机finite-state transducer 有限状态置换器flap 闪音flat 降音foreground information 前景讯息[前景信息]Formal Language Theory 形式语言理论Formal Linguistics 形式语言学Formal Semantics 形式语意学forward inference 前向推理[向前推理]forward-backward algorithm 前前后后算法frame 框架frame based knowledge representation 框架型知识表示Frame Theory 框架理论free morpheme 自由语素Fregean principle Fregean 原则fricative 擦音F-structure 功能结构full text searching 全文检索function word 功能词Functional Grammar 功能语法functional programming 函数型程序设计[函数型程序设计]functional sentence perspective 功能句子观functional structure 功能结构functional unification 功能连并[功能合一]functor 功能符fundamental frequency 基频garden path sentence 花园路径句GB (Government and Binding) 管辖约束geminate 重迭音gender 性Generalized Phrase Structure Grammar 概化词组结构语法[广义短语结构语法] Generative Grammar 衍生语法Generative Linguistics 衍生语言学[生成语言学]generic 泛指genetic epistemology 发生认识论genetive marker 属格标记genitive 属格gerund 动名词Government and Binding Theory 管辖约束理论GPSG (Generalized Phrase Structure Grammar) 概化词组结构语法[广义短语结构语法] gradability 可分级性grammar checker 文法检查器grammatical affix 语法词缀grammatical category 语法范畴grammatical function 语法功能grammatical inference 文法推论grammatical relation 语法关系grapheme 字素haplology 类音删略head 中心语head driven phrase structure 中心语驱动词组结构[中心词驱动词组结构]head feature convention 中心语特征继承原理[中心词特性继承原理]Head-Driven Phrase Structure Grammar 中心语驱动词组结构律heteronym 同形heuristic parsing 经验式句法剖析Heuristics 经验知识hidden Markov model 隐式马可夫模型hierarchical structure 阶层结构[层次结构]holophrase 单词句homograph 同形异义词homonym 同音异义词homophone 同音词homophony 同音异义homorganic 同部位音的Horn clause Horn 子句HPSG (Head-Driven Phrase Structure Grammar) 中心语驱动词组结构语法human-machine interface 人机界面hypernym 上位词hypertext 超文件[超文本]hyponym 下位词hypotactic 主从结构的IC (immediate constituent) 直接成份ICG (Information-based Case Grammar) 讯息为本的格位语法idiom 成语[熟语]idiosyncrasy 特异性illocutionary 施为性immediate constituent 直接成份imperative 祈使句implicative predicate 蕴含谓词implicature 含意indexical 标引的indirect object 间接宾语indirect speech act 间接言谈行动[间接言语行为]Indo-European language 印欧语言inductional inference 归纳推理inference machine 推理机器infinitive 不定词[to 不定式]infix 中缀inflection/inflexion 屈折变化inflectional affix 屈折词缀information extraction 信息撷取information processing 信息处理[信息处理]information retrieval 信息检索Information Science 信息科学[信息科学; 情报科学]Information Theory 信息论[信息论]inherent feature 固有特征inherit 继承inheritance 继承inheritance hierarchy 继承阶层[继承层次]inheritance of attribute 属性继承innateness position 语法天生假说insertion 中插inside-outside algorithm 里里外外算法instantiation 体现instrumental (case) 工具格integrated parser 集成句法剖析程序integrated theory of discourse analysis 篇章分析综合理论[言谈分析综合理论] intelligence intensive production 知识密集型生产intensifier 加强成分intensional logic 内含逻辑Intensional Semantics 内涵语意学intensional type 内含类型interjection/exclamation 感叹词inter-level 中间成分interlingua 中介语言interlingual 中介语(的)interlocutor 对话者internalise 内化International Phonetic Association (IPA) 国际语音学会internet 因特网Interpretive Semantics 诠释性语意学intonation 语调intonation unit (IU) 语调单位IPA (International Phonetic Association) 国际语音学会IR (information retrieval) 信息检索IS-A relation IS-A 关系isomorphism 同形现象IU (intonation unit) 语调单位junction 连接keyword in context 上下文中关键词[上下文内关键词] kinesics 体势学knowledge acquisition 知识习得knowledge base 知识库knowledge based machine translation 知识为本之机器翻译knowledge extraction 知识撷取[知识题取]knowledge representation 知识表示KWIC (keyword in context) 关键词前后文[上下文内关键词] label 标签labial 唇音labio-dental 唇齿音labio-velar 软颚唇音LAD (language acquisition device) 语言习得装置lag 发声延迟language acquisition 语言习得language acquisition device 语言习得装置language engineering 语言工程language generation 语言生成language intuition 语感language model 语言模型language technology 语言科技left-corner parsing 左角落剖析[左角句法剖析]lemma 词元lenis 弱辅音letter-to-phone 字转音lexeme 词汇单位lexical ambiguity 词汇歧义lexical category 词类lexical conceptual structure 词汇概念结构lexical entry 词项lexical entry selection standard 选词标准lexical integrity 词语完整性Lexical Semantics 词汇语意学Lexical-Functional Grammar 词汇功能语法Lexicography 词典学Lexicology 词汇学lexicon 词汇库[词典;词库]lexis 词汇层LF (logical form) 逻辑形式LFG (Lexical-Functional Grammar) 词汇功能语法liaison 连音linear bounded automaton 线性有限自主机linear precedence 线性次序lingua franca 共通语linguistic decoding 语言译码linguistic unit 语言单位linked list 串行loan 外来语local 局部的localism 方位主义localizer 方位词locus model 轨迹模型locution 惯用语logic 逻辑logic array network 逻辑数组网络logic programming 逻辑程序设计[逻辑程序设计] logical form 逻辑形式logical operator 逻辑算子[逻辑算符]Logic-Based Grammar 逻辑为本语法[基于逻辑的语法] long term memory 长期记忆longest match principle 最长匹配原则[最长一致法] LR (left-right) parsing LR 剖析machine dictionary 机器词典machine language 机器语言machine learning 机器学习machine translation 机器翻译machine-readable dictionary (MRD) 机读辞典Macrolinguistics 宏观语言学Markov chart 马可夫图Mathematical Linguistics 数理语言学maximum entropy 最大熵M-D (modifier-head) construction 偏正结构mean length of utterance (MLU) 语句平均长度measure of information 讯习测度[信息测度] memory based 根据记忆的mental lexicon 心理词汇库mental model 心理模型mental process 心理过程[智力过程;智力处理] metalanguage 超语言metaphor 隐喻metaphorical extension 隐喻扩展metarule 律上律[元规则]metathesis 语音易位Microlinguistics 微观语言学middle structure 中间式结构minimal pair 最小对Minimalist Program 微言主义MLU (mean length of utterance) 语句平均长度modal 情态词modal auxiliary 情态助动词modal logic 情态逻辑modifier 修饰语Modular Logic Grammar 模块化逻辑语法modular parsing system 模块化句法剖析系统modularity 模块性(理论)module 模块monophthong 单元音monotonic 单调monotonicity 单调性Montague Grammar 蒙泰究语法[蒙塔格语法] mood 语气morpheme 词素morphological affix 构词词缀morphological decomposition 语素分解morphological pattern 词型morphological processing 词素处理morphological rule 构词律[词法规则] morphological segmentation 语素切分Morphology 构词学Morphophonemics 词音学[形态音位学;语素音位学] morphophonological rule 形态音位规则Morphosyntax 词句法Motor Theory 肌动理论movement 移位MRD (machine-readable dictionary) 机读辞典MT (machine translation) 机器翻译multilingual processing system 多语讯息处理系统multilingual translation 多语翻译multimedia 多媒体multi-media communication 多媒体通讯multiple inheritance 多重继承multistate logic 多态逻辑mutation 语音转换mutual exclusion 互斥mutual information 相互讯息nativist position 语法天生假说natural language 自然语言natural language processing (NLP) 自然语言处理natural language understanding 自然语言理解negation 否定negative sentence 否定句neologism 新词语nested structure 崁套结构network 网络neural network 类神经网络Neurolinguistics 神经语言学neutralization 中立化n-gram n-连词n-gram modeling n-连词模型NLP (natural language processing) 自然语言处理node 节点nominalization 名物化nonce 暂用的non-finite 非限定non-finite clause 非限定式子句non-monotonic reasoning 非单调推理normal distribution 常态分布noun 名词noun phrase 名词组NP (noun phrase) completeness 名词组完全性object 宾语{语言学}/对象{信息科学}object oriented programming 对象导向程序设计[面向对向的程序设计] official language 官方语言one-place predicate 一元述语on-line dictionary 在线查询词典[联机词点]onomatopoeia 拟声词onset 节首音ontogeny 个体发生Ontology 本体论open set 开放集operand 操作数[操作对象]optimization 最佳化[最优化]overgeneralization 过度概化overgeneration 过度衍生paradigmatic relation 聚合关系paralanguage 附语言parallel construction 并列结构Parallel Corpus 平行语料库parallel distributed processing (PDP) 平行分布处理paraphrase 转述[释意;意译;同意互训]parole 言语parser 剖析器[句法剖析程序]parsing 剖析part of speech (POS) 词类particle 语助词PART-OF relation PART-OF 关系part-of-speech tagging 词类标注pattern recognition 型样识别P-C (predicate-complement) insertion 述补中插PDP (parallel distributed processing) 平行分布处理perception 知觉perceptron 感觉器[感知器]perceptual strategy 感知策略performative 行为句periphrasis 用独立词表达perlocutionary 语效性的permutation 移位Petri Net Grammar Petri 网语法philology 语文学phone 语音phoneme 音素phonemic analysis 因素分析phonemic stratum 音素层Phonetics 语音学phonogram 音标Phonology 声韵学[音位学;广义语音学] Phonotactics 音位排列理论phrasal verb 词组动词[短语动词]phrase 词组[短语]phrase marker 词组标记[短语标记]pitch 音调pitch contour 调形变化Pivot Grammar 枢轴语法pivotal construction 承轴结构plausibility function 可能性函数PM (phrase marker) 词组标记[短语标记] polysemy 多义性POS-tagging 词类标记postposition 方位词PP (preposition phrase) attachment 介词依附Pragmatics 语用学Precedence Grammar 优先级语法precision 精确度predicate 述词predicate calculus 述词计算predicate logic 述词逻辑[谓词逻辑]predicate-argument structure 述词论元结构prefix 前缀premodification 前置修饰preposition 介词Prescriptive Linguistics 规定语言学[规范语言学]presentative sentence 引介句presupposition 前提Principle of Compositionality 语意合成性原理privative 二元对立的probabilistic parser 概率句法剖析程序problem solving 解决问题program 程序programming language 程序设计语言[程序设计语言]proofreading system 校对系统proper name 专有名词prosody 节律prototype 原型pseudo-cleft sentence 准分裂句Psycholinguistics 心理语言学punctuation 标点符号pushdown automata 下推自动机pushdown transducer 下推转换器qualification 后置修饰quantification 量化quantifier 范域词Quantitative Linguistics 计量语言学question answering system 问答系统queue 队列radical 字根[词干;词根;部首;偏旁]radix of tuple 元组数基random access 随机存取rationalism 理性论rationalist (position) 理性论立场[唯理论观点]reading laboratory 阅读实验室real time 实时real time control 实时控制[实时控制]recursive transition network 递归转移网络reduplication 重迭词[重复]reference 指涉referent 指称对象referential indices 指标referring expression 指涉词[指示短语]register 缓存器[寄存器]{信息科学}/调高{语音学}/语言的场合层级{社会语言学} regular language 正规语言[正则语言]relational database 关系型数据库[关系数据库] relative clause 关系子句relaxation method 松弛法relevance 相关性Restricted Logic Grammar 受限逻辑语法resumptive pronouns 复指代词retroactive inhibition 逆抑制rewriting rule 重写规则rheme 述位rhetorical structure 修辞结构rhetorics 修辞学robust 强健性robust processing 强健性处理robustness 强健性schema 基朴school grammar 教学语法scope 范域[作用域;范围]script 脚本search mechanism 检索机制search space 检索空间searching route 检索路径[搜索路径]second order predicate 二阶述词segmentation 分词segmentation marker 分段标志selectional restriction 选择限制semantic field 语意场semantic frame 语意架构semantic network 语意网络semantic representation 语意表征[语义表示] semantic representation language 语意表征语言semantic restriction 语意限制semantic structure 语意结构Semantics 语意学sememe 意素Semiotics 符号学sender 发送者sensorimotor stage 感觉运动期sensory information 感官讯息[感觉信息] sentence 句子sentence generator 句子产生器[句子生成程序] sentence pattern 句型separation of homonyms 同音词区分sequence 序列serial order learning 顺序学习serial verb construction 连动结构set oriented semantic network 集合导向型语意网络[面向集合型语意网络] SGML (Standard Generalized Markup Language) 结构化通用标记语言shift-reduce parsing 替换简化式剖析short term memory 短程记忆sign 信号signal processing technology 信号处理技术simple word 单纯词situation 情境Situation Semantics 情境语意学situational type 情境类型social context 社会环境sociolinguistics 社会语言学software engineering 软件工程[软件工程]sort 排序speaker-independent speech recognition 非特定语者语音识别spectrum 频谱speech 口语speech act assignment 言语行为指定speech continuum 言语连续体speech disorder 语言失序[言语缺失]speech recognition 语音辨识speech retrieval 语音检索speech situation 言谈情境[言语情境]speech synthesis 语音合成speech translation system 语音翻译系统speech understanding system 语音理解系统spreading activation model 扩散激发模型standard deviation 标准差Standard Generalized Markup Language 标准通用标示语言start-bound complement 接头词state of affairs algebra 事态代数state transition diagram 状态转移图statement kernel 句核static attribute list 静态属性表statistical analysis 统计分析Statistical Linguistics 统计语言学statistical significance 统计意义stem 词干stimulus-response theory 刺激反应理论stochastic approach to parsing 概率式句法剖析[句法剖析的随机方法] stop 爆破音Stratificational Grammar 阶层语法[层级语法]string 字符串[串;字符串]string manipulation language 字符串操作语言string matching 字符串匹配[字符串] structural ambiguity 结构歧义Structural Linguistics 结构语言学structural relation 结构关系structural transfer 结构转换structuralism 结构主义structure 结构structure sharing representation 结构共享表征subcategorization 次类划分[下位范畴化] subjunctive 假设的sublanguage 子语言subordinate 从属关系subordinate clause 从属子句[从句;子句] subordination 从属substitution rule 代换规则[置换规则] substrate 底层语言suffix 后缀superordinate 上位的superstratum 上层语言suppletion 异型[不规则词型变化] suprasegmental 超音段的syllabification 音节划分syllable 音节syllable structure constraint 音节结构限制symbolization and verbalization 符号化与字句化synchronic 同步的synonym 同义词syntactic category 句法类别syntactic constituent 句法成分syntactic rule 语法规律[句法规则] Syntactic Semantics 句法语意学syntagm 句段syntagmatic 组合关系[结构段的;组合的] Syntax 句法Systemic Grammar 系统语法tag 标记target language 目标语言[目标语言]task sharing 课题分享[任务共享]tautology 套套逻辑[恒真式;重言式;同义反复] taxonomical hierarchy 分类阶层[分类层次] telescopic compound 套装合并template 模板temporal inference 循序推理[时序推理]temporal logic 时间逻辑[时序逻辑]temporal marker 时貌标记tense 时态terminology 术语text 文本text analyzing 文本分析text coherence 文本一致性text generation 文本生成[篇章生成]Text Linguistics 文本语言学text planning 文本规划text proofreading 文本校对text retrieval 文本检索text structure 文本结构[篇章结构]text summarization 文本自动摘要[篇章摘要] text understanding 文本理解text-to-speech 文本转语音thematic role 题旨角色thematic structure 题旨结构theorem 定理thesaurus 同义词辞典theta role 题旨角色theta-grid 题旨网格token 实类[标记项]tone 音调tone language 音调语言tone sandhi 连调变换top-down 由上而下[自顶向下]topic 主题topicalization 主题化[话题化]trace 痕迹Trace Theory 痕迹理论training 训练transaction 异动[处理单位]transcription 转写[抄写;速记翻译] transducer 转换器transfer 转移transfer approach 转换方法transfer framework 转换框架transformation 变形[转换] Transformational Grammar 变形语法[转换语法] transitional state term set 转移状态项集合transitivity 及物性translation 翻译translation equivalence 翻译等值性translation memory 翻译记忆transparency 透明性tree 树状结构[树]Tree Adjoining Grammar 树形加接语法[树连接语法] treebank 树图数据库[语法关系树库]trigram 三连词t-score t-数turing machine 杜林机[图灵机]turing test 杜林测试[图灵试验]type 类型type/token node 标记类型/实类节点type-feature structure 类型特征结构typology 类型学ultimate constituent 终端成分unbounded dependency 无界限依存underlying form 基底型式underlying structure 基底结构unification 连并[合一]Unification-based Grammar 连并为本的语法[基于合一的语法] Universal Grammar 普遍性语法universal instantiation 普遍例式universal quantifier 全称范域词unknown word 未知词[未定义词]unrestricted grammar 非限制型语法usage flag 使用旗标user interface 使用者界面[用户界面]Valence Grammar 结合价语法Valence Theory 结合价理论valency 结合价variance 变异数[方差]verb 动词verb phrase 动词组[动词短语]verb resultative compound 动补复合词verbal association 词语联想verbal phrase 动词组verbal production 言语生成vernacular 本地话V-O construction (verb-object) 动宾结构vocabulary 字汇vocabulary entry 词条vocal track 声道vocative 呼格voice recognition 声音辨识[语音识别]vowel 元音vowel harmony 元音和谐[元音和谐]waveform 波形weak verb 弱化动词Whorfian hypothesis Whorfian 假说word 词word frequency 词频word frequency distribution 词频分布word order 词序word segmentation 分词word segmentation standard for Chinese 中文分词规范word segmentation unit 分词单位[切词单位]word set 词集working memory 工作记忆[工作存储区]world knowledge 世界知识writing system 书写系统X-Bar Theory X标杠理论["x"阶理论]Zipf's Law 利夫规律[齐普夫定律]。
外文文献翻译译稿和原文
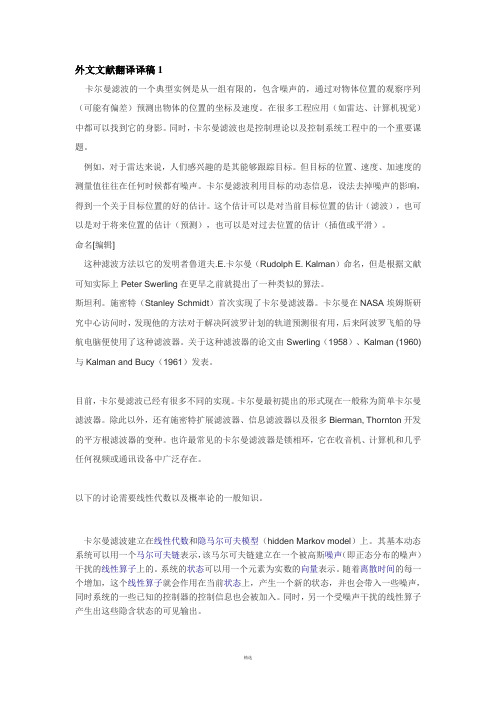
外文文献翻译译稿1卡尔曼滤波的一个典型实例是从一组有限的,包含噪声的,通过对物体位置的观察序列(可能有偏差)预测出物体的位置的坐标及速度。
在很多工程应用(如雷达、计算机视觉)中都可以找到它的身影。
同时,卡尔曼滤波也是控制理论以及控制系统工程中的一个重要课题。
例如,对于雷达来说,人们感兴趣的是其能够跟踪目标。
但目标的位置、速度、加速度的测量值往往在任何时候都有噪声。
卡尔曼滤波利用目标的动态信息,设法去掉噪声的影响,得到一个关于目标位置的好的估计。
这个估计可以是对当前目标位置的估计(滤波),也可以是对于将来位置的估计(预测),也可以是对过去位置的估计(插值或平滑)。
命名[编辑]这种滤波方法以它的发明者鲁道夫.E.卡尔曼(Rudolph E. Kalman)命名,但是根据文献可知实际上Peter Swerling在更早之前就提出了一种类似的算法。
斯坦利。
施密特(Stanley Schmidt)首次实现了卡尔曼滤波器。
卡尔曼在NASA埃姆斯研究中心访问时,发现他的方法对于解决阿波罗计划的轨道预测很有用,后来阿波罗飞船的导航电脑便使用了这种滤波器。
关于这种滤波器的论文由Swerling(1958)、Kalman (1960)与Kalman and Bucy(1961)发表。
目前,卡尔曼滤波已经有很多不同的实现。
卡尔曼最初提出的形式现在一般称为简单卡尔曼滤波器。
除此以外,还有施密特扩展滤波器、信息滤波器以及很多Bierman, Thornton开发的平方根滤波器的变种。
也许最常见的卡尔曼滤波器是锁相环,它在收音机、计算机和几乎任何视频或通讯设备中广泛存在。
以下的讨论需要线性代数以及概率论的一般知识。
卡尔曼滤波建立在线性代数和隐马尔可夫模型(hidden Markov model)上。
其基本动态系统可以用一个马尔可夫链表示,该马尔可夫链建立在一个被高斯噪声(即正态分布的噪声)干扰的线性算子上的。
系统的状态可以用一个元素为实数的向量表示。
近似动态规划相关的外文文献及翻译
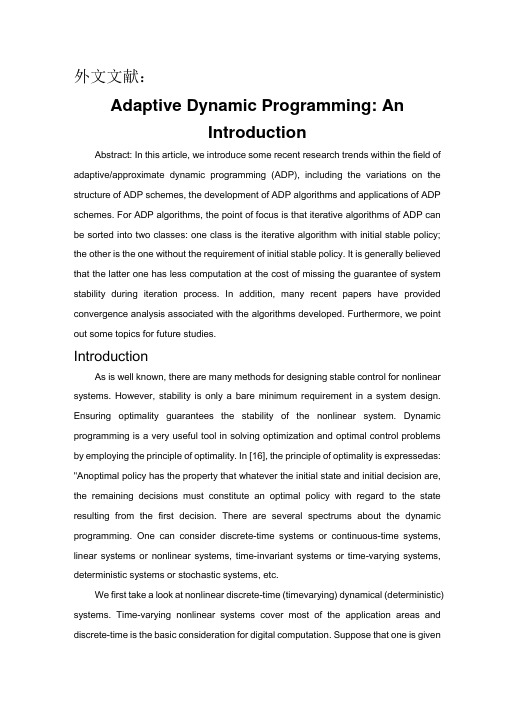
外文文献:Adaptive Dynamic Programming: AnIntroductionAbstract: In this article, we introduce some recent research trends within the field of adaptive/approximate dynamic programming (ADP), including the variations on the structure of ADP schemes, the development of ADP algorithms and applications of ADP schemes. For ADP algorithms, the point of focus is that iterative algorithms of ADP can be sorted into two classes: one class is the iterative algorithm with initial stable policy; the other is the one without the requirement of initial stable policy. It is generally believed that the latter one has less computation at the cost of missing the guarantee of system stability during iteration process. In addition, many recent papers have provided convergence analysis associated with the algorithms developed. Furthermore, we point out some topics for future studies.IntroductionAs is well known, there are many methods for designing stable control for nonlinear systems. However, stability is only a bare minimum requirement in a system design. Ensuring optimality guarantees the stability of the nonlinear system. Dynamic programming is a very useful tool in solving optimization and optimal control problems by employing the principle of optimality. In [16], the principle of optimality is expressedas: "Anoptimal policy has the property that whatever the initial state and initial decision are, the remaining decisions must constitute an optimal policy with regard to the state resulting from the first decision. There are several spectrums about the dynamic programming. One can consider discrete-time systems or continuous-time systems, linear systems or nonlinear systems, time-invariant systems or time-varying systems, deterministic systems or stochastic systems, etc.We first take a look at nonlinear discrete-time (timevarying) dynamical (deterministic) systems. Time-varying nonlinear systems cover most of the application areas and discrete-time is the basic consideration for digital computation. Suppose that one is givena discrete-time nonlinear (timevarying) dynamical system+ 1) = 侬),= 0,1T■■- (1)where x R n represents the state vector of the system and u R m denotes the control action and F is the system function. Suppose that one associateswith this system the performance index (or cost)J(x(i)a i) = 声(幻M) (2)k=iwhere U is called the utility function and g is the discount factor with 0 , g # 1. Note that the function J is dependent on the initial time i and the initial state x( i ), and it is referred to as the cost-to-go of state x( i ). The objective of dynamic programming problem is to choose a control sequence u(k), k5i, i11,c, so that the function J (i.e., the cost) in (2) is minimized. According to Bellman, the optimal cost from time k is equal to心))+ 刃侦1 1))}.⑶ 思!)The optimal control u* 1k2 at time k is the u1k2 which achieves this minimum, i.e., «*(fe) = arg min|U(x(fe), «(fe)) + yj*(x(k+ 1))}_ (4)Equation (3) is the principle of optimality for discrete-time systems. Its importance lies in the fact that it allows one to optimize over only one control vector at a time by working backward in time.In nonlinear continuous-time case, the system can be described by x(t)=F[x(t), r]" N b (5)The cost in this case is defined as7(^(0) = 口3(了),心))打. (6)For continuous-time systems, Bellman ' s principle of optimofitybe applied, too. The optimal cost J*(x0)5min J(x0, u(t)) will satisfy the Hamilton-Jacobi-Bellman EquationQt =孰 “(W).MW) + TFS(£),叩)")}=Z(x(f),/(£),£)+ (" \Ox(tEquations (3) and (7) are called the optimality equations of dynamic programming which are the basis for implementation of dynamic programming. In the above, if the function F in (1) or (5) and the cost function J in (2) or (6) are known, the solution of u(k ) becomes a simple optimization problem. If the system is modeled by linear dynamics and the cost function to be minimized is quadratic in the state and control, then the optimal control is a linear feedback of the states, where the gains are obtained by solving a standard Riccati equation [47]. On the other hand, if the system is modeled by nonlinear dynamics or the cost function is nonquadratic, the optimal state feedback control will depend upon solutions to the Hamilton-Jacobi-Bellman (HJB) equation [48] which is generally a nonlinear partial differential equation or difference equation. However, it is often computationally untenable to run true dynamic programming due to the backward numerical process required for its solutions, i.e., as a result of the well- known “curse of dimensionality [16], [28]. In [69], three curses are displayed in resource management and control problems to show the cost function J , which is the theoretical solution of the Hamilton-Jacobi- Bellman equation, is very difficult to obtain, except for systems satisfying some very good conditions. Over the years, progress has been made to circumventhe “curse of dimensionality by building a system, called “critic to , approximate the cost function in dynamic programming (cf. [10], [60], [61], [63], [70], [78], [92], [94],[95]). The idea is to approximate dynamic programming solutions by using a function approximation structure such as neural networks to approximate the cost function.In recent years, adaptive/approximate dynamic programming (ADP) has gaineddrTt)The asic Structures of ADPmuch attention from many researchers in order to obtain approximate solutions of the HJB equation, cf. [2], [3], [5], [8], [11] 03], [21], [22], [25], [30], [31], [34], [35],[40], [46], [49], [52], [54], [55], [63], [70], [76], [80], [83], [95], [96], [99], [100]. In 1977, Werbos [91] introduced an approach for ADP that was later called adaptive critic designs (ACDs). ACDs were proposed in [91], [94], [97] as a way for solving dynamic programming problems forward-in-time. In the literature, there are several synonyms used for "Adaptive CDticsigns ” [10], [24], [39], [43], [54], [70], [71], [87], including "Approximate Dynamic Programming ” [69], [82], [95]Asymptotic Dynamic Programming" [75], “Adaptive Dynamic Programming" [63], [64],“Heuristic Dynamic Programming"[9国6], “NedDpnamic Programming " [17],“Neural DynamiProgramming ” [82], [101], and "Reinforcement Learning ” [84].Bertsekas and Tsitsiklis gave an overview of the neurodynamic programming in their book [17]. They provided the background, gave a detailed introduction to dynamic programming, discussed the neural network architectures and methods for training them, and developed general convergence theorems for stochastic approximation methods as the foundation for analysis of various neuro-dynamic programming algorithms. They provided the core neuro-dynamic programming methodology, including many mathematical results and methodological insights. They suggested many useful methodologies for applications to neurodynamic programming, like Monte Carlo simulation, on-line and off-line temporal difference methods, Q-learning algorithm, optimistic policy iteration methods, Bellman error methods, approximate linear programming, approximate dynamic programming with cost-to-go function, etc. A particularly impressive success that greatly motivated subsequent research, was the development of a backgammon playing program by Tesauro [85]. Here a neural network was trained to approximate the optimal cost-to-go function of the game of backgammon by using simulation, that is, by letting the program play against itself. Unlike chess programs, this program did not use lookahead of many steps, so its successcan be attributed primarily to the use of a properly trained approximation of the optimal cost-to-go function.To implement the ADP algorithm, Werbos [95] proposed a means to get aroundthis numerical complexity by using “ approximate dynamic programming " formulations . His methods approximate the original problem with a discrete formulation. Solution to the ADP formulation is obtained through neural network based adaptive critic approach. The main idea of ADP is shown in Fig. 1. i Dynamic ; SystemAgent ') ------------- ------StateFIGURE 1 Learn from the environment*He proposed two basic versions which are heuristic dynamic programming (HDP)and dual heuristic programming (DHP).HDP is the most basic and widely applied structure of ADP [13], [38], [72], [79],[90], [93], [104], [106]. The structure of HDP is shown in Fig. 2. HDP is a method for estimating the cost function. Estimating the cost function for a given policy only requires samples from the instantaneous utility function U, while models of the environment and the instantaneous reward are needed to find the cost function corresponding to the optimal policy.Critica PerformanceIndex Function iReward/PenaltyActionControlFIGURE 2Ihe HDP structure.In HDP, the output of the critic network is J A, which is the estimate of J in equation (2). This is done by minimizing the following error measure over time 1101 =2^)= [火)-侦A) -寸侬+ 1 (s)h—土where JA(k)5JA 3x(k), u(k), k, WC4 and WC represents the parameters of the critic network. When Eh50 for all k, (8) implies thatj(Q = u(Q +刃筷+ 1) (9)J(k) =which is the same as (2) i=kDual heuristic programming is a method for estimating the gradient of the cost function, rather than J itself. To do this, a function is needed to describe the gradient of the instantaneous cost function with respect to the state of the system. In the DHP structure, the action network remains the same as the one for HDP, but for the second network, which is called the critic network, with the costate as its output and the state variables as its inputs.The critic network ' s training is more complicated thianHtBat since we need to take into account all relevant pathways of backpropagation.This is done by minimizing the following error measure over timed /、 I _ r 3/(t ) 屁|| =衬0=衣[丽(to ) where 'J A 1k2 /'x1k2 5'J A 3x1k2, u1k2, k, WC4/'x1k2 and WC represents theparameters of the critic network. When Eh50 for all k, (10) implies that a/(fe) a/(fe+ i)3x(/?) '—3x( fe)—*2. Theoretical DevelopmentsIn [82], Si et al summarizes the cross-disciplinary theoretical developments of ADP and overviews DP and ADP; and discusses their relations to artificial intelligence, approximation theory, control theory, operations research, and statistics.In [69], Powell shows how ADP, when coupled with mathematical programming, can solve (approximately) deterministic or stochastic optimization problems that are far larger than anything that could be solved using existing techniques and shows the improvement directions of ADP.In [95], Werbos further gave two other versions called namely, ADHDP (also known as Q-learning [89]) and ADDHP. In the two ADPstructures, the control is also the input of the critic networks. In 1997,Prokhorov and Wunsch [70] presented more algorithms according to ACDs.They discussed the design families of HDP, DHP, and globalized dual heuristic programming (GDHP). They suggested some new improvements to the original GDHP design. They promised to be useful for many engineering applications in the areas of optimization and optimal control. Based on one of these modifications, they present a unified approach to all ACDs. This leads to a generalized training procedure for ACDs. In [26], a realization of ADHDP was suggested:a least squares support vector machine (SVM) regressor has been used for generating the control actions, while an SVM-based tree-type neural network (NN) is used as the critic. The GDHP or ADGDHP structure minimizes the error with respect to both the cost and its derivatives. While it is more complex to do this simultaneously, the resulting behavior is expected to be superior. So in [102], GDHP serves as a reconfigurable controller to deal with both abrupt and incipient changesin the plant dynamics due to faults. A novel fault tolerant control (FTC) supervisor is combined with GDHP for the purpose of improving the performance of dU(k) dx( k) 所筷+ 1) 7 i)x(k)catt0ndep endentGDHP for fault tolerant control. When the plant is affected by a known abrupt fault, the new initial conditions of GDHP are loaded from dynamic model bank (DMB). On the other hand, if the fault is incipient, the reconfigurable controller maintains performance by continuously modifying itself without supervisor intervention. It is noted that the training of three networks used to implement the GDHP is in an online fashion by utilizing two distinct networks to implement the critic. The first critic network is trained at every iterations while the second one is updated with a copy of the first one at a given period of iterations.All the ADP structures can realize the same function that is to obtain the optimal control policy while the computation precision and running time are different from each other. Generally speaking, the computation burden of HDP is low but the computation precision is also low; while GDHP has better precision but the computation process will take longer time and the detailed comparison can be seen in [70]. In [30], [33] and [83], the schematic of direct heuristic dynamic programming is developed. Using the approach of [83], the model network in Fig. 1 is not needed anymore. Reference [101] makes significant contributions to model-free adaptive critic designs. Several practical examples are included in [101] for demonstration which include single inverted pendulum and triple inverted pendulum. A reinforcement learning-based controller design for nonlinear discrete-time systems with input constraints is presentedby [36], where the nonlinear tracking control is implemented with filtered tracking error using direct HDP designs. Similar works also see [37]. Reference [54] is also about model-free adaptive critic designs. Two approaches for the training of critic network are provided in [54]: A forward-in-time approach and a backward-in-time approach. Fig. 4 shows the diagram of forward-intimeapproach. In this approach, we view J A(k) in (8) as the output of the critic network to be trained and choose U(k)1gJA(k11) as the training target. Note that JA(k) and JA(k11) are obtained using state variables at different time instances. Fig. 5shows the diagram of backward-in-time approach. In this approach, we view J A(k11) in (8) as the output of the critic network to be trained and choose ( J,(k)2U(k))/g as the training target. The training ap proach of [101] can be considered as a backward-in-time ap proach. In Fig. 4 and Fig. 5, x(k11) is the output of the model network.FIGURE 3 The DHP structure.泌(丽糖做+ 1)) a雄 +1)FIGURE 4 Forward-in-time approach.FIGURE 5 Backward-in-time approach.An improvement and modification to the two network architecture, which is called the “single network adaptive crftNAC)” was presented in [65], [66]. This approach eliminates the action network. As a consequence,the SNAC architecture offers three potential advantages: a simpler architecture, lesser computational load (about half of the dual network algorithms), and no approximate error due to the fact that the action network is eliminated. The SNAC approach is applicable to a wide class of nonlinear systems where the optimal control (stationary) equation can be explicitly expressed in terms of the state and the costate variables. Most of the problems in aerospace, automobile, robotics, and other engineering disciplines can be characterized by the nonlinear control-affine equations that yield such a relation. SNAC-based controllers yield excellent tracking performances in applications to microelectronic mechanical systems, chemical reactor, and high-speed reentry problems. Padhi et al. [65] have proved that for linear systems (where the mapping between the costate at stage k11 and the state at stage k is linear), the solution obtained by the algorithm based on the SNAC structure converges to the solution of discrete Riccati equation.译文:自适应动态规划综述摘要:自适应动态规划(Adaptive dynamic programming, ADP)是最优控制领域新兴起的一种近似最优方法,是当前国际最优化领域的研究热点.ADP方法利用函数近似结构来近似哈密顿{雅可比{贝尔曼(Hamilton-Jacobi-Bellman, HJB)方程的解,采用离线迭代或者在线更新的方法,来获得系统的近似最优控制策略,从而能够有效地解决非线性系统的优化控制问题.本文按照ADP的结构变化、算法的发展和应用三个方面介绍ADP方法.对目前ADP方法的研究成果加以总结,并对这一研究领域仍需解决的问题和未来的发展方向作了进一步的展望。
数据分析外文文献+翻译
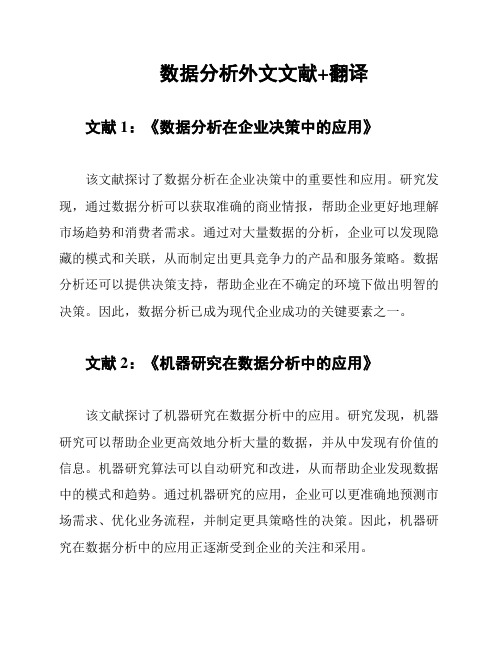
数据分析外文文献+翻译文献1:《数据分析在企业决策中的应用》该文献探讨了数据分析在企业决策中的重要性和应用。
研究发现,通过数据分析可以获取准确的商业情报,帮助企业更好地理解市场趋势和消费者需求。
通过对大量数据的分析,企业可以发现隐藏的模式和关联,从而制定出更具竞争力的产品和服务策略。
数据分析还可以提供决策支持,帮助企业在不确定的环境下做出明智的决策。
因此,数据分析已成为现代企业成功的关键要素之一。
文献2:《机器研究在数据分析中的应用》该文献探讨了机器研究在数据分析中的应用。
研究发现,机器研究可以帮助企业更高效地分析大量的数据,并从中发现有价值的信息。
机器研究算法可以自动研究和改进,从而帮助企业发现数据中的模式和趋势。
通过机器研究的应用,企业可以更准确地预测市场需求、优化业务流程,并制定更具策略性的决策。
因此,机器研究在数据分析中的应用正逐渐受到企业的关注和采用。
文献3:《数据可视化在数据分析中的应用》该文献探讨了数据可视化在数据分析中的重要性和应用。
研究发现,通过数据可视化可以更直观地呈现复杂的数据关系和趋势。
可视化可以帮助企业更好地理解数据,发现数据中的模式和规律。
数据可视化还可以帮助企业进行数据交互和决策共享,提升决策的效率和准确性。
因此,数据可视化在数据分析中扮演着非常重要的角色。
翻译文献1标题: The Application of Data Analysis in Business Decision-making The Application of Data Analysis in Business Decision-making文献2标题: The Application of Machine Learning in Data Analysis The Application of Machine Learning in Data Analysis文献3标题: The Application of Data Visualization in Data Analysis The Application of Data Visualization in Data Analysis翻译摘要:本文献研究了数据分析在企业决策中的应用,以及机器研究和数据可视化在数据分析中的作用。
DDS外文翻译
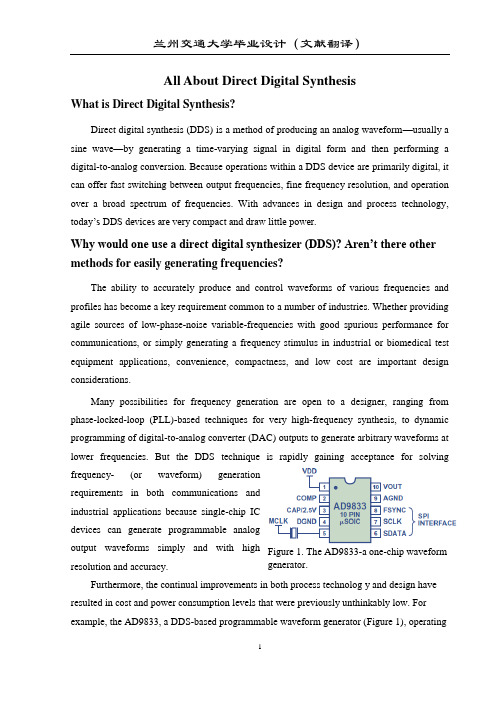
All About Direct Digital SynthesisWhat is Direct Digital Synthesis?Direct digital synthesis (DDS) is a method of producing an analog waveform —usually a sine wave —by generating a time-varying signal in digital form and then performing a digital-to-analog conversion. Because operations within a DDS device are primarily digital, it can offer fast switching between output frequencies, fine frequency resolution, and operation over a broad spectrum of frequencies. With advances in design and process technology, today’s DDS devices are very compact and draw little power.Why would one use a direct digital synthesizer (DDS)? Aren’t there other methods for easily generating frequencies?The ability to accurately produce and control waveforms of various frequencies and profiles has become a key requirement common to a number of industries. Whether providing agile sources of low-phase-noise variable-frequencies with good spurious performance for communications, or simply generating a frequency stimulus in industrial or biomedical test equipment applications, convenience, compactness, and low cost are important design considerations.Many possibilities for frequency generation are open to a designer, ranging from phase-locked-loop (PLL)-based techniques for very high-frequency synthesis, to dynamic programming of digital-to-analog converter (DAC) outputs to generate arbitrary waveforms at lower frequencies. But the DDS technique is rapidly gaining acceptance for solving frequency- (or waveform) generationrequirements in both communications andindustrial applications because single-chip ICdevices can generate programmable analogoutput waveforms simply and with high resolution and accuracy.Furthermore, the continual improvements in both process technolog y and design have resulted in cost and power consumption levels that were previously unthinkably low. For example, the AD9833, a DDS-based programmable waveform generator (Figure 1), operatingFigure 1. The AD9833-a one-chip waveformgenerator.at 5.5 V with a 25-MHz clock, consumes a maximum power of 30 milliwatts.What are the main benefits of using a DDS?DDS devices like the AD9833 are programmed through a high speed serial peripheral-interface (SPI), and need only an external clock to generate simple sine waves. DDS devices are now available that can generate frequencies from less than 1 Hz up to 400 MHz (based on a 1-GHz clock). The benefits of their low power, low cost, and single small package, combined with their inherent excellent performance and the ability to digitally program (and re-program) the output waveform, make DDS devices an extremely attractive solution—preferable to less-flexible solutions comprising aggregations of discrete elements. What kind of outputs can I generate with a typical DDS device?DDS devices are not limited to purelysinusoidal outputs. Figure 2 shows thesquare-, triangular-, and sinusoidal outputsavailable from an AD9833.How does a DDS device create asine wave?Here’s a breakdown of the internalcircuitry of a DDS device: its maincomponents are a phase accumulator, ameans of phase-to-amplitude conversion(often a sine look-up table), and a DAC. These blocks are represented in Figure 3.A DDS produces a sine waveat a given frequency. The frequency depends on two variables, the reference-clock frequency and the binar y number programmed into the Figure 2. Square-, triangular-, and sinusoidal outputs from a DDS.Figure 3. Components of a direct digital synthesizer.frequency register (tuning word).The binary number in the frequency register provides the main input to the phase accumulator. If a sine look-up table is used, the phase accumulator computes a phase (angle) address for the look-up table, which outputs the digital value of amplitude—corresponding to the sine of that phase angle—to the DAC. The DAC, in turn, converts that number to a corresponding value of analog voltage or current. To generate a fixed-frequency sine wave, a constant value (the phase increment—which is determined by the binary number) is added to the phase accumulator with each clock cycle. If the phase increment is large, the phase accumulator will step quickly through the sine look-up table and thus generate a high frequency sine wave. If the phase increment is small, the phase accumulator will take many more steps, accordingly generating a slower waveform.What do you mean by a complete DDS?The integration of a D/A converter and a DDS onto a single chip is commonly known as a complete DDS solution, a property common to all DDS devices from ADI.Let’s talk some more about the phase accumulator. How does it work?Continuous-time sinusoidal signals have a repetitive angular phase range of 0 to 2.The digital implementation is no different. The counter’scarry function allows the phase accumulator to act asa phase wheel in the DDS implementation.To understand this basic function, visualize thesine-wave oscillation as a vector rotating around aphase circle (see Figure 4). Each designated point onthe phase wheel corresponds to the equivalent pointon a cycle of a sine wave. As the vector rotatesaround the wheel, visualize that the sine of the anglegenerates a corresponding output sine wave. OneFigure 4. Digital phase wheel. revolution of the vector around the phase wheel, at aconstant speed, results in one complete cycle of the output sine wave. The phase accumulator provides the equally spaced angular values accompanying the vector’s linear rotation aroundthe phase wheel. The contents of the phase accumulator correspond to the points on the cycle of the output sine wave.The phase accumulator is actually a modulo- M counter that increments its stored number each time it receives a clock pulse. The magnitude of the increment is determined by the binary-coded input word (M). This word forms the phase step size between reference-clock updates; it effectively sets how many points to skip around the phase wheel. The larger the jump size, the faster the phase accumulator overflows and completes its equivalent of a sine-wave cycle. The number of discrete phase points contained in the wheel is determined by the resolution of the phase accumulator (n), which determines the tuning resolution of the DDS. For an n = 28-bit phase accumulator, an M value of 0000...0001 would result in the phase accumulator overflowing after 28 reference-clock cycles (increments). If the M value is changed to 0111...1111, the phase accumulator will overflow after only 2 reference-clock cycles (the minimum required by Nyquist). This relationship is found in the basic tuning equation for DDS architecture:n C out f M f 2⨯= where:fOUT = output frequency of the DDSM = binary tuning wordfC = internal reference clock frequency (system clock)n = length of the phase accumulator, in bitsChanges to the value of M result in immediate and phase-continuous changes in the output frequency. No loop settling time is incurred as in the case of a phase-locked loop.As the output frequency is increased, the number of samples per cycle decreases. Since sampling theory dictates that at least two samples per cycle are required to reconstruct the output waveform, the maximum fundamental output frequency of a DDS is fC/2. However, for practical applications, the output frequency is limited to somewhat less than that, improving the quality of the reconstructed waveform and permitting filtering on the output.When generating a constant frequency, the output of the phase accumulator increaseslinearly, so the analog waveform it generates is inherently a ramp.Then how is that linear output translated into a sine wave?A phase -to - amplitude lookup table is used to convert the phase-accumulator’sinstantaneous output value (28 bits for AD9833)—with unneeded less-significant bits eliminated by truncation—into the sine-wave amplitude information that is presented to the (10 -bit) D/A converter. The DDS architecture exploits the symmetrical nature of a sine wave and utilizes mapping logic to synthesize a complete sine wave from one-quarter-cycle of data from the phase accumulator. The phase-to- amplitude lookup table generates the remainingdata by reading forward then back through the lookup table. This is shown pictorially in Figure 5.Figure 5. Signal flow through the DDS architecture.关于直接数字频率合成器什么是直接数字频率合成器?直接数字频率合成器(DDS)是一种通过产生一个以数字形式时变的信号,然后执行由数字至模拟转换的方法。
关于动态规划的相关论文

旅行商问题动态规划旅行商问题的几种求解算法比较作者:(xxx学校)摘要:TSP问题是组合优化领域的经典问题之一,吸引了许多不同领域的研究工作者,包括数学,运筹学,物理,生物和人工智能等领域,他是目前优化领域里的热点.本文从动态规划法,分支界限法,回溯法分别来实现这个题目,并比较哪种更优越,来探索这个经典的NP(Nondeterministic Polynomial)难题.关键词:旅行商问题求解算法比较一.引言旅行商问题(Travelling Salesman Problem),是计算机算法中的一个经典的难解问题,已归为NP一完备问题类.围绕着这个问题有各种不同的求解方法,已有的算法如动态规划法,分支限界法,回溯法等,这些精确式方法都是指数级(2n)[2,3]的,根本无法解决目前的实际问题,贪心法是近似方法,而启发式算法不能保证得到的解是最优解,甚至是较好的解释.所以我认为很多问题有快速的算法(多项式算法),但是,也有很多问题是无法用算法解决的.事实上,已经证明很多问题不可能在多项式时间内解决出来.但是,有很多很重要的问题他们的解虽然很难求解出来,但是他们的值却是很容易求可以算出来的.这种事实导致了NP完全问题.NP表示非确定的多项式,意思是这个问题的解可以用非确定性的算法"猜"出来.如果我们有一个可以猜想的机器,我们就可以在合理的时间内找到一个比较好的解.NP-完全问题学习的简单与否,取决于问题的难易程度.因为有很多问题,它们的输出极其复杂,比如说人们早就提出的一类被称作NP-难题的问题.这类问题不像NP-完全问题那样时间有限的.因为NP-问题由上述那些特征,所以很容易想到一些简单的算法――把全部的可行解算一遍.但是这种算法太慢了(通常时间复杂度为O(2^n))在很多情况下是不可行的.现在,没有知道有没有那种精确的算法存在.证明存在或者不存在那种精确的算法这个沉重的担子就留给了新的研究者了,或许你就是成功者.本篇论文就是想用几种方法来就一个销售商从几个城市中的某一城市出发,不重复地走完其余N—1个城市,并回到原出发点,在所有可能的路径中求出路径长度最短的一条,比较是否是最优化,哪种结果好.二.求解策略及优化算法动态规划法解TSP问题我们将具有明显的阶段划分和状态转移方程的规划称为动态规划,这种动态规划是在研究多阶段决策问题时推导出来的,具有严格的数学形式,适合用于理论上的分析.在实际应用中,许多问题的阶段划分并不明显,这时如果刻意地划分阶段法反而麻烦.一般来说,只要该问题可以划分成规模更小的子问题,并且原问题的最优解中包含了子问题的最优解(即满足最优子化原理),则可以考虑用动态规划解决.所以动态规划的实质是分治思想和解决冗余,因此,动态规划是一种将问题实例分解为更小的,相似的子问题,并存储子问题的解而避免计算重复的子问题,以解决最优化问题的算法策略.旅行商问题(TSP问题)其实就是一个最优化问题,这类问题会有多种可能的解,每个解都有一个值,而动态规划找出其中最优(最大或最小)值的解.若存在若干个取最优值的解的话,它只取其中的一个.在求解过程中,该方法也是通过求解局部子问题的解达到全局最优解,但与分治法和贪心法不同的是,动态规划允许这些子问题不独立,(亦即各子问题可包含公共的子子问题)也允许其通过自身子问题的解作出选择,该方法对每一个子问题只解一次,并将结果保存起来,避免每次碰到时都要重复计算.关于旅行商的问题,状态变量是gk(i,S),表示从0出发经过k个城市到达i的最短距离,S为包含k个城市的可能集合,动态规划的递推关系为:gk(i,S)=min[gk-1(j,S\{j})+dji] j属于S,dji表示j-i的距离.或者我们可以用:f(S,v)表示从v出发,经过S中每个城市一次且一次,最短的路径.f(S,v)=min { f(S-{u},u)+dist(v,u) }u in Sf(V,1)即为所求2.分支限界法解TSP问题旅行商问题的解空间是一个排列树,与在子集树中进行最大收益和最小耗费分枝定界搜索类似,使用一个优先队列,队列中的每个元素中都包含到达根的路径.假设我们要寻找的是最小耗费的旅行路径,那可以使用最小耗费分枝定界法.在实现过程中,使用一个最小优先队列来记录活节点,队列中每个节点的类型为M i n H e ap N o d e.每个节点包括如下区域: x(从1到n的整数排列,其中x [ 0 ] = 1 ),s(一个整数,使得从排列树的根节点到当前节点的路径定义了旅行路径的前缀x[0:s], 而剩余待访问的节点是x [ s + 1 : n - 1 ]),c c(旅行路径前缀,即解空间树中从根节点到当前节点的耗费),l c o s t(该节点子树中任意叶节点中的最小耗费), rc o s t(从顶点x [ s : n - 1 ]出发的所有边的最小耗费之和).当类型为M i n He a p N o d e ( T )的数据被转换成为类型T时,其结果即为l c o s t的值.分枝定界算法的代码见程序.程序首先生成一个容量为1 0 0 0的最小堆,用来表示活节点的最小优先队列.活节点按其l c o s t值从最小堆中取出.接下来,计算有向图中从每个顶点出发的边中耗费最小的边所具有的耗费M i n O u t.如果某些顶点没有出边,则有向图中没有旅行路径,搜索终止.如果所有的顶点都有出边,则可以启动最小耗费分枝定界搜索.根的孩子(图1 6 - 5的节点B)作为第一个E-节点,在此节点上,所生成的旅行路径前缀只有一个顶点1,因此s=0, x[0]=1, x[1:n-1]是剩余的顶点(即顶点2 , 3 ,., n ).旅行路径前缀1 的开销为0 ,即c c = 0 ,并且,r c o st=n i=1M i n O u t .在程序中,bestc 给出了当前能找到的最少的耗费值.初始时,由于没有找到任何旅行路径,因此b e s t c的值被设为N o E d g e.程序旅行商问题的最小耗费分枝定界算法templateT AdjacencyWDigraph::BBTSP(int v[]){// 旅行商问题的最小耗费分枝定界算法// 定义一个最多可容纳1 0 0 0个活节点的最小堆MinHeap > H(1000);T *MinOut = new T [n+1];// 计算MinOut = 离开顶点i的最小耗费边的耗费T MinSum = 0; // 离开顶点i的最小耗费边的数目for (int i = 1; i <= n; i++) {T Min = NoEdge;for (int j = 1; j <= n; j++)if (a[j] != NoEdge &&(a[j] < Min || Min == NoEdge))Min = a[j];if (Min == NoEdge) return NoEdge; // 此路不通MinOut = Min;MinSum += Min;}// 把E-节点初始化为树根MinHeapNode E;E.x = new int [n];for (i = 0; i < n; i++)E.x = i + 1;E.s = 0; // 局部旅行路径为x [ 1 : 0 ] = 0; // 其耗费为0E.rcost = MinSum;T bestc = NoEdge; // 目前没有找到旅行路径// 搜索排列树while (E.s < n - 1) {// 不是叶子if (E.s == n - 2) {// 叶子的父节点// 通过添加两条边来完成旅行// 检查新的旅行路径是不是更好if (a[E.x[n-2]][E.x[n-1]] != NoEdge && a[E.x[n-1]][1] != NoEdge && ( + a[E.x[n-2]][E.x[n-1]] + a[E.x[n-1]][1] < bestc || bestc == NoEdge)) {// 找到更优的旅行路径bestc = + a[E.x[n-2]][E.x[n-1]] + a[E.x[n-1]][1]; = bestc;E.lcost = bestc;E . s + + ;H . I n s e r t ( E ) ; }else delete [] E.x;}else {// 产生孩子for (int i = E.s + 1; i < n; i++)if (a[E.x[E.s]][E.x] != NoEdge) {// 可行的孩子, 限定了路径的耗费T cc = + a[E.x[E.s]][E.x];T rcost = E.rcost - MinOut[E.x[E.s]];T b = cc + rcost; //下限if (b < bestc || bestc == NoEdge) {// 子树可能有更好的叶子// 把根保存到最大堆中MinHeapNode N;N.x = new int [n];for (int j = 0; j < n; j++)N.x[j] = E.x[j];N.x[E.s+1] = E.x;N.x = E.x[E.s+1]; = cc;N.s = E.s + 1;N.lcost = b;N.rcost = rcost;H . I n s e r t ( N ) ; }} // 结束可行的孩子delete [] E.x;} // 对本节点的处理结束try {H.DeleteMin(E);} // 取下一个E-节点catch (OutOfBounds) {break;} // 没有未处理的节点}if (bestc == NoEdge) return NoEdge; // 没有旅行路径// 将最优路径复制到v[1:n] 中for (i = 0; i < n; i++)v[i+1] = E.x;while (true) {//释放最小堆中的所有节点delete [] E.x;try {H.DeleteMin(E);}catch (OutOfBounds) {break;}}return bestc;}while 循环不断地展开E-节点,直到找到一个叶节点.当s = n - 1时即可说明找到了一个叶节点.旅行路径前缀是x [ 0 : n - 1 ],这个前缀中包含了有向图中所有的n个顶点.因此s = n - 1的活节点即为一个叶节点.由于算法本身的性质,在叶节点上lco st 和cc 恰好等于叶节点对应的旅行路径的耗费.由于所有剩余的活节点的lcost 值都大于等于从最小堆中取出的第一个叶节点的lcost 值,所以它们并不能帮助我们找到更好的叶节点,因此,当某个叶节点成为E-节点后,搜索过程即终止.while 循环体被分别按两种情况处理,一种是处理s = n - 2的E-节点,这时,E-节点是某个单独叶节点的父节点.如果这个叶节点对应的是一个可行的旅行路径,并且此旅行路径的耗费小于当前所能找到的最小耗费,则此叶节点被插入最小堆中,否则叶节点被删除,并开始处理下一个E-节点.其余的E-节点都放在while 循环的第二种情况中处理.首先,为每个E-节点生成它的两个子节点,由于每个E-节点代表着一条可行的路径x [ 0 : s ],因此当且仅当是有向图的边且x [ i ]是路径x [ s + 1 : n - 1 ]上的顶点时,它的子节点可行.对于每个可行的孩子节点,将边的耗费加上 即可得到此孩子节点的路径前缀( x [ 0 : s ],x) 的耗费c c.由于每个包含此前缀的旅行路径都必须包含离开每个剩余顶点的出边,因此任何叶节点对应的耗费都不可能小于cc 加上离开各剩余顶点的出边耗费的最小值之和,因而可以把这个下限值作为E-节点所生成孩子的lcost 值.如果新生成孩子的lcost 值小于目前找到的最优旅行路径的耗费b e s t c,则把新生成的孩子加入活节点队列(即最小堆)中.如果有向图没有旅行路径,程序返回N o E d g e;否则,返回最优旅行路径的耗费,而最优旅行路径的顶点序列存储在数组v 中.3.回朔法解TSP问题回朔法有"通用解题法"之称,它采用深度优先方式系统地搜索问题的所有解,基本思路是:确定解空间的组织结构之后,从根结点出发,即第一个活结点和第一个扩展结点向纵深方向转移至一个新结点,这个结点成为新的活结点,并成为当前扩展结点.如果在当前扩展结点处不能再向纵深方向转移,则当前扩展结点成为死结点.此时,回溯到最近的活结点处,并使其成为当前扩展结点,回溯到以这种工作方式递归地在解空间中搜索,直到找到所求解空间中已经无活结点为止.旅行商问题的解空间是一棵排列树.对于排列树的回溯搜索与生成1,2,……, n的所有排列的递归算法Perm类似.设开始时x=[ 1,2,… n ],则相应的排列树由x[ 1:n ]的所有排列构成.旅行商问题的回溯算法找旅行商回路的回溯算法Backtrack是类Treveling的私有成员函数,TSP是Treveling的友员.TSP(v)返回旅行售货员回路最小费用.整型数组v返回相应的回路.如果所给的图G不含旅行售货员回路,则返回NoEdge.函数TSP所作的工作主要是为调用Backtrack所需要变量初始化.由TSP调用Backtrack(2)搜索整个解空间.在递归函数Backtrack中,当i = n时,当前扩展结点是排列树的叶结点的父结点.此时,算法检测图G是否存在一条从顶点x[ n-1 ]到顶点x[ n ]的边和一条从顶点x[ n ]到顶点1的边.如果这两条边都存在,则找一条旅行售货员回路.此时,算法还需判断这条回路的费用是否优于已找到的当前最优回路的费用best.如果是,则必须更新当前最优值bestc和当前最优解bestx. 当i < n时,当前扩展结点位于排列树的第i–1 层.图G中存在从顶点x[ i-1 ]到顶点x[ i ]的边时,x[ 1:i ]构成图G的一条路径,且当x[ 1:i ]的费用小于当前最优值时,算法进入排列树的第I 层.否则将剪去相应的子树.算法中用变量cc记录当前路径x[ 1:i ]的费用.解旅行商售货员问题的回溯法可描述如下:templateclass Traveling {friend Type TSP(int * *,int [],Type);private:void Backtrack(int i);int n, //图G的顶点数* x, //当前解*bestx; //当前最优解Type * *a, //图G的邻接矩阵cc, //当前费用bestc, //当前最优值NoEdge; //无边际记};templatevode Traveling::Backtrack(int i){if(I==n){if(a[x[n-1]][x[n]]! = NoEdge &&a[x[n]][1]!= NoEdge &&(cc + a[x[n-1]][x[n]]+a[x[n]][1]bestc== NoEdge) ){for(int j=1;j<=n;j++)bestx[j]=x[j];bestc =cc + a[x[n-1]][x[n]]+ a[x[n]][1];}}else {for(int j=I; j<=n;j++)//是否可进入x[j]子树if(a[x[i-1]][x[j]]! = NoEdge &&(cc + a[x[i-1]][x[i]]< bestc||bestc == NoEdge//搜索子数Swap(x[i],x[j]);cc += a[x[i-1]][x[i]];Backtrack(I+1);cc -= a[x[i-1]][x[i]];Swap(x[i],x[j]);}}}templateType TSP(Type * *a,int v[],int n,Type NoEdge){Traveling Y;//初始化YY.x = new int[n+1];// 置x为单位排列for(int i=1;i<=n;i++)Y.x[i] = I;Y.a=a;Y.n=n;Y.bestc = NoEdge;Y.bestc = v; = 0;Y. NoEdge = NoEdge;//搜索x[2:n]的全排列Y.Backtrack(2);Delete[] Y.x;三.三种方法的比较1.动态规划法和回朔法的比较:这本来就是两个完全不同的领域,一个是算法领域,一个是数据结构问题.但两者又交叉,又有区别.从本质上讲就是算法与数据结构的本质区别,回朔是一个具体的算法,动态规划是数据结构中的一个概念.动态规划讲究的是状态的转化,以状态为基准,确定算法,动态规划法所针对的问题有一个显著的特征,即它所对应的子问题树中的子问题呈现大量的重复.动态规划法的关键就在于,对于重复出现的子问题,只在第一次遇到时加以求解,并把答案保存起来,让以后再遇到时直接引用,不必重新求解.简单的说就是:动态规划法是从小单元开始积累计算结果.回朔讲究过程的推进与反还,随数据的搜索,标记,确定下一步的行进方向,回朔是去搜索. 如果想要搜索时,发现有很多重复计算,就应该想到用动态规划了.动态规划和搜索都可以解决具有最优子结构的问题,然而动态规划在解决子问题的时候不重复计算已经计算过的子问题,对每个子问题只计算一次;而简单的搜索则递归地计算所有遇到的的子问题.比如一个问题的搜索树具有如下形式:........A......./.......B...C...../.\./.....D...E...F如果使用一般深度优先的搜索,依次搜索的顺序是A-B-D-E-C-E-F,注意其中节点E被重复搜索了两次;如果每个节点看作是一个子问题的话,节点E所代表的子问题就被重复计算了两次; 但是如果是用动态规划,按照树的层次划分阶段,按照自底向上的顺序,则在第一阶段计算D,E,F;第二阶段计算B,C;第三阶段计算A;这样就没有重复计算子问题E.搜索法的优点是实现方便,缺点是在子问题有大量的重复的时候要重复计算子问题,效率较低;动态规划虽然效率高,但是阶段的划分和状态的表示比较复杂,另外,搜索的时候只要保存单前的结点;而动态规划则至少要保存上一个阶段的所有节点,比如在动态规划进行到第2阶段的时候,必须把第三阶段的D,E,F三个节点全部保存起来,所以动态规划是用空间换时间. 另外,有一种折衷的办法,就是备忘录法,这是动态规划的一种变形.该方法的思想是:按照一般的搜索算法解决子问题,但是用一个表将所有解决过的子问题保存起来,遇到一个子问题的时候,先查表看是否是已经解决过的,如果已解决过了就不用重复计算.比如搜索上面那棵树,在A-B-D-E的时候,已经将E记录在表里了,等到了A-B-D-E-C的时候,发现E已经被搜索过,就不再搜索E,而直接搜索F,因此备忘录法的搜索顺序是A-B-D-E-C-(跳过E)-F自底向上的动态规划还有一个缺点,比如对于下面的树:........A......./.......B...C......G...../.\./.\..../.....D...E...F..H...I如用自底向上的动态规划,各个阶段搜索的节点依次是:D,E,F,H,IB,C,GAA才是我们最终要解决的问题,可以看到,G,H,I根本与问题A无关,但是动态规划还是将它们也解决了一遍,这就造成了效率降低.而备忘录法则可以避免这种问题,按照备忘录法,搜索的次序仍然是:A-B-D-E-C-(跳过E)-F.备忘录法的优点是实现简单,且在子问题空间中存在大量冗余子问题的时候效率较高;但是要占用较大的内存空间(需要开一个很大的表来记录已经解决的子问题),而且如果用递归实现的话递归压栈出栈也会影响效率;而自底向上的动态规划一般用for循环就可以了.值得一提的是,用动态规划法来计算旅行商的时间复杂度是指数型的.2. 分支限界法和回朔法的比较:分支限界法类似于回溯法,也是一种在问题的解空间树T上搜索问题解的算法.但在一般情况下,分支限界与回溯法的求解目标不同.回溯法的求解目标是找出T中满足约束条件的所有解,而分支限界法的求解目标则是找出满足约束条件的一个解,或是在满足约束条件的解中找出使某一目标函数值达到极大或极小的解,即在某种意义下的最优解.我们先看一个列子:设G=(V,E)是一个带权图.图1中各边的费用(权)为一正数.图中的一条周游线是包括V中的每个顶点在内的一条回路.一条周游路线的费用是这条路线上所有边的费用之和.所谓旅行售货员问题就是要在图G中找出一条有最小费用的周游路线.给定一个有n个顶点的带权图G,旅行售货员问题要找出图G的费用(权)最小的周游路线.图1是一个4顶点无向带权图.顶点序列1,2,4,3,1;1,3,2,4,1和1,4,3,2,1是该图中3条不同的周游路线.301 256 1043 20 4图1 4顶点带权图该问题的解空间可以组织成一棵树,从树的根结点到任一叶结点的路径定义了图G的一条周游路线.图1是当n=4时这种树结构的示例.其中从根结点A到叶结点L的路径上边的标号组成一条周游路线1,2,3,4,1.而从根结点到叶结点O的路径则表示周游路线1,3,4,2,1.图G的每一条周游路线都恰好对应解空间树中一条从根结点到叶结点的路径.因此,解空间树中叶结点个数为(n-1)!.A1B2 3 4C D E3 24 2 3F G H I J K4 3 4 2 3 2L M N O P Q图2 旅行售货员问题的解空间树对于图1中的图G,用回溯法找最小费用周游路线时,从解空间树的根结点A出发,搜索至B,C,F,L.在叶结点L处记录找到的周游路线1,2,3,4,1,该周游路线的费用为59.从叶结点L返回至最近活动结点F处.由于F处已没有可扩展结点,算法又返回到结点C处.结点C成为新扩展结点,由新扩展结点,算法再移至结点G 后又移至结点M,得到周游路线1,2,4,3,1,其费用为66.这个费用不比已有周游路线1,2,3,4,1的费用小.因此,舍弃该结点.算法有依次返回至结点G,C,B.从结点B,算法继续搜索至结点D,H,N.在叶结点N算法返回至结点H,D,然后再从结点D开始继续向纵深搜索至结点O.依次方式算法继续搜索遍整个解空间,最终得到1,3,2,4,1是一条最小费用周游路线.以上便是回溯法找最小费用周游路线的实列,但如果我们用分支限界法来解的话,会更适合.由于求解目标不同,导致分支限界法与回溯法在解空间树T上的搜索方式也不相同.回溯法以深度优先的方式搜索解空间树T,而分支限界法则以广度优先或以最小消耗优先的方式搜索解空间树T.分支限界法的搜索策略是,在扩展结点处,先生成所有的儿子结点(分支),然后再从当前的活动点表中选择下一个扩展结点.为了有效地选择下一扩展结点,以加速搜索的进程,在每一活结点处,计算一个函数值(限界),并根据这些已计算出的函数值,从当前活结点表中选择一个最有利的结点作为扩展结点,使搜索朝着解空间树上的最优解的分支推进,以便尽快的找出一个最优解.四.结论:参考文献:。
动态规划文献
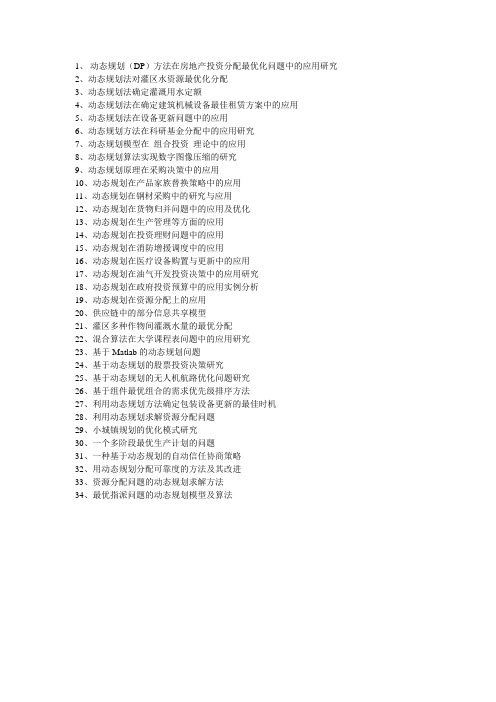
1、动态规划(DP)方法在房地产投资分配最优化问题中的应用研究
2、动态规划法对灌区水资源最优化分配
3、动态规划法确定灌溉用水定额
4、动态规划法在确定建筑机械设备最佳租赁方案中的应用
5、动态规划法在设备更新问题中的应用
6、动态规划方法在科研基金分配中的应用研究
7、动态规划模型在_组合投资_理论中的应用
8、动态规划算法实现数字图像压缩的研究
9、动态规划原理在采购决策中的应用
10、动态规划在产品家族替换策略中的应用
11、动态规划在钢材采购中的研究与应用
12、动态规划在货物归并问题中的应用及优化
13、动态规划在生产管理等方面的应用
14、动态规划在投资理财问题中的应用
15、动态规划在消防增援调度中的应用
16、动态规划在医疗设备购置与更新中的应用
17、动态规划在油气开发投资决策中的应用研究
18、动态规划在政府投资预算中的应用实例分析
19、动态规划在资源分配上的应用
20、供应链中的部分信息共享模型
21、灌区多种作物间灌溉水量的最优分配
22、混合算法在大学课程表问题中的应用研究
23、基于Matlab的动态规划问题
24、基于动态规划的股票投资决策研究
25、基于动态规划的无人机航路优化问题研究
26、基于组件最优组合的需求优先级排序方法
27、利用动态规划方法确定包装设备更新的最佳时机
28、利用动态规划求解资源分配问题
29、小城镇规划的优化模式研究
30、一个多阶段最优生产计划的问题
31、一种基于动态规划的自动信任协商策略
32、用动态规划分配可靠度的方法及其改进
33、资源分配问题的动态规划求解方法
34、最优指派问题的动态规划模型及算法。
关于运筹学的文献资料
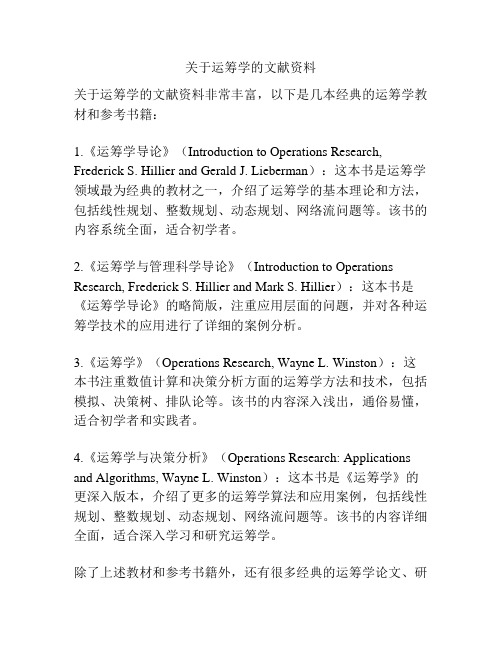
关于运筹学的文献资料关于运筹学的文献资料非常丰富,以下是几本经典的运筹学教材和参考书籍:1.《运筹学导论》(Introduction to Operations Research, Frederick S. Hillier and Gerald J. Lieberman):这本书是运筹学领域最为经典的教材之一,介绍了运筹学的基本理论和方法,包括线性规划、整数规划、动态规划、网络流问题等。
该书的内容系统全面,适合初学者。
2.《运筹学与管理科学导论》(Introduction to Operations Research, Frederick S. Hillier and Mark S. Hillier):这本书是《运筹学导论》的略简版,注重应用层面的问题,并对各种运筹学技术的应用进行了详细的案例分析。
3.《运筹学》(Operations Research, Wayne L. Winston):这本书注重数值计算和决策分析方面的运筹学方法和技术,包括模拟、决策树、排队论等。
该书的内容深入浅出,通俗易懂,适合初学者和实践者。
4.《运筹学与决策分析》(Operations Research: Applications and Algorithms, Wayne L. Winston):这本书是《运筹学》的更深入版本,介绍了更多的运筹学算法和应用案例,包括线性规划、整数规划、动态规划、网络流问题等。
该书的内容详细全面,适合深入学习和研究运筹学。
除了上述教材和参考书籍外,还有很多经典的运筹学论文、研究报告和学术期刊供学者和研究者参考。
一些知名的运筹学期刊包括《Operations Research》、《Management Science》和《INFORMS Journal on Computing》等。
值得一提的是,随着运筹学领域的发展,新的研究成果不断涌现,因此从学术期刊和学术会议等渠道获取最新的文献资料也是非常重要的。
project based learning外国文献

project based learning外国文献以下是一些关于project based learning(项目学习)的外国文献:1. Thomas, J. W. (2000). A review of research on project-based learning. San Rafael, CA: Autodesk Foundation.这篇文献是对项目学习研究进行综述的一篇重要文献,提供了项目学习的定义、特点和实施指导,以及项目学习对学生学业成就和技能发展的影响等方面的综合评估。
2. Blumenfeld, P. C., Soloway, E., Marx, R. W., Krajcik, J. S., Guzdial, M., & Palincsar, A. (1991). Motivating project-based learning: Sustaining the doing, supporting the learning. Educational psychologist, 26(3-4), 369-398.这篇文献探讨了项目学习的动机因素和实施过程中的支持措施,从社会认知理论和动机理论的视角分析了如何提高学生在项目学习中的积极参与和学习成果。
3. Hung, W. (2006). The 9-step problem design process for problem-based learning: application of the 3C3R model. Educational research review, 1(1), 27-40.这篇文献介绍了一个适用于问题驱动学习的设计过程模型,通过“3C3R”模型(Challenge、Concepts、Cases以及Reflection、Reconstruction、Review)指导教师在项目学习中的问题设计和课程设计。
nlp有重要意义的三篇文献
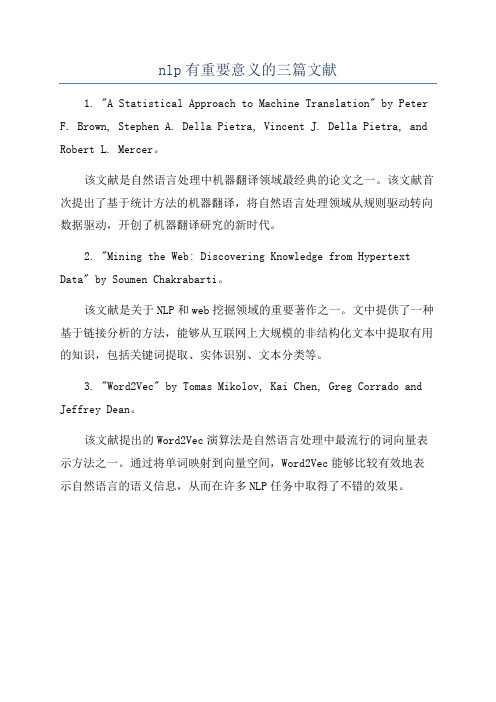
nlp有重要意义的三篇文献
1. "A Statistical Approach to Machine Translation" by Peter
F. Brown, Stephen A. Della Pietra, Vincent J. Della Pietra, and Robert L. Mercer。
该文献是自然语言处理中机器翻译领域最经典的论文之一。
该文献首次提出了基于统计方法的机器翻译,将自然语言处理领域从规则驱动转向数据驱动,开创了机器翻译研究的新时代。
2. "Mining the Web: Discovering Knowledge from Hypertext Data" by Soumen Chakrabarti。
该文献是关于NLP和web挖掘领域的重要著作之一。
文中提供了一种基于链接分析的方法,能够从互联网上大规模的非结构化文本中提取有用的知识,包括关键词提取、实体识别、文本分类等。
3. "Word2Vec" by Tomas Mikolov, Kai Chen, Greg Corrado and Jeffrey Dean。
该文献提出的Word2Vec演算法是自然语言处理中最流行的词向量表示方法之一。
通过将单词映射到向量空间,Word2Vec能够比较有效地表示自然语言的语义信息,从而在许多NLP任务中取得了不错的效果。
英文文献翻译
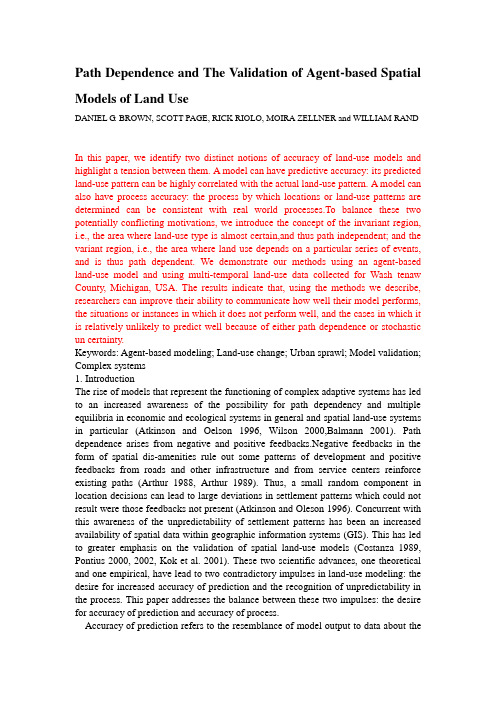
Path Dependence and The Validation of Agent-based Spatial Models of Land UseDANIEL G. BROWN, SCOTT PAGE, RICK RIOLO, MOIRA ZELLNER and WILLIAM RAND In this paper, we identify two distinct notions of accuracy of land-use models and highlight a tension between them. A model can have predictive accuracy: its predicted land-use pattern can be highly correlated with the actual land-use pattern. A model can also have process accuracy: the process by which locations or land-use patterns are determined can be consistent with real world processes.To balance these two potentially conflicting motivations, we introduce the concept of the invariant region, i.e., the area where land-use type is almost certain,and thus path independent; and the variant region, i.e., the area where land use depends on a particular series of events, and is thus path dependent. We demonstrate our methods using an agent-based land-use model and using multi-temporal land-use data collected for Wash tenaw County, Michigan, USA. The results indicate that, using the methods we describe, researchers can improve their ability to communicate how well their model performs, the situations or instances in which it does not perform well, and the cases in which it is relatively unlikely to predict well because of either path dependence or stochastic un certainty.Keywords: Agent-based modeling; Land-use change; Urban sprawl; Model validation; Complex systems1.IntroductionThe rise of models that represent the functioning of complex adaptive systems has led to an increased awareness of the possibility for path dependency and multiple equilibria in economic and ecological systems in general and spatial land-use systems in particular (Atkinson and Oelson 1996, Wilson 2000,Balmann 2001). Path dependence arises from negative and positive feedbacks.Negative feedbacks in the form of spatial dis-amenities rule out some patterns of development and positive feedbacks from roads and other infrastructure and from service centers reinforce existing paths (Arthur 1988, Arthur 1989). Thus, a small random component in location decisions can lead to large deviations in settlement patterns which could not result were those feedbacks not present (Atkinson and Oleson 1996). Concurrent with this awareness of the unpredictability of settlement patterns has been an increased availability of spatial data within geographic information systems (GIS). This has led to greater emphasis on the validation of spatial land-use models (Costanza 1989, Pontius 2000, 2002, Kok et al. 2001). These two scientific advances, one theoretical and one empirical, have lead to two contradictory impulses in land-use modeling: the desire for increased accuracy of prediction and the recognition of unpredictability in the process. This paper addresses the balance between these two impulses: the desire for accuracy of prediction and accuracy of process.Accuracy of prediction refers to the resemblance of model output to data about theenvironments and regions they are meant to describe, usually measured as either aggregate similarity and spatial similarity. Aggregate similarity refers to similarities in statistics that describe the mapped pattern of land use such as the distributions of sizes of developed clusters, the functional relationship between distance to city center and density (Batty and Longley 1994, Makse et al. 1998; Andersson et al.2002, Rand et al. 2003), or landscape pattern metrics developed within the landscape ecology literature (e.g., McGarigal and Marks 1995) to measure the degree of fragmentation in the landscape (Parker and Meretsky 2004).Spatial similarity refers to the degree of match between land-use maps and a single run or summary of multiple runs of a land-use model. The most common approaches build on the basic error matrix approach (Congalton 1991), by which agreement can be summarized using the kappa statistic (Cohen 1960). Pontius(2000) has developed map comparison methods for model validation that partitions total errors into those due to the amounts of each land-use type and those due to their locations. Because models rely on generalizations of reality, spatial similarity measures must be considered in light of their scale; the coarser the partition, the easier the matching task becomes (Constanza 1989, Kok et al. 2001, Pontius 2002and Hagen 2003).Because spatial patterns contain more information than can be captured bya handful of aggregate statistics, validation using spatial similarity raises the empirical bar over aggregate similarity. However, as we shall demonstrate in this paper, demanding that modelers get the locations right may be asking too much.Human decision-making is rarely deterministic, and land-use models commonly include stochastic processes as a result (e.g., in the use of random utility theory;Irwin and Geoghegan 2001). Many models, therefore, produce varying results because of stochastic uncertainty in their processes. Further, to represent the feedback processes, land-use modelers are making increasing use of cellular automata (Batty and Xie 1994, Clarke et al. 1996, White and Engelen 1997) and agent-based simulation (Balmann 2001, Rand et al. 2002, Parker and Meretsky2004). These and other modeling approaches that can represent feedbacks can exhibit spatial path dependence, i.e., the spatial patterns that result can be very sensitive to slight differences in processes or initial conditions. How sensitive depends upon specific attributes of the model. Given the presence of path dependence and the effect it can have on magnifying uncertainties in land-use models, any model that consistently returns spatial patterns in which the locations of land uses are similar to the real world could be overfit, i.e., it may represent the outcomes of a particular case well but the description of the process may not be generalizable.We believe that the situation creates an imposing challenge: to make accurate predictions, but to admit the inability to be completely accurate owing to path dependence and stochastic uncertainty. If we pursue only the first part of the dictumat the expense of the second part, we encourage a tendency towards over fitting, in which the model is constrained by more and more information such that its ability to run in the absence of data (e.g., in the future) or to predict surprising results is reduced. If we emphasize the latter, then we abandon hope of predicting those spatial properties that are path or state invariant. Though it is reasonable to ask,‘‘can themodel predict past behavior?’’the answer to this question depends as muchon the dynamic feedbacks and non-linearities of the system itself as on the accuracy of the model. Therefore, the more important question is ‘‘are the mechanisms and parameters of the model correct?’’In this paper we describe and demonstrate an approach to model validation that acknowledges path dependence in land-use models. The invariant-variant method enables us to determine what we know and what we don’t know spatially. Although we can only make limited interpretations about the amount of path dependence that we see for any one model applied to a particular landscape, comparing across a wide range of models and landscape patterns should allow us to understand if a model contains an appropriate level of path dependence and/or stochasticity.2.An agent-based model of land developmentThe model we use to illustrate the validation methods was developed in Swarm(), a multipurpose agent-based modeling platform. In the model,agents choose locations on a heterogeneous two-dimensional landscape. The spatial patterns of development are the result of agent behaviors.We developed this simple model for the purposes of experimentation and pedagogy, and present it as a means to illustrate the validation methods. These concerns created an incentive for simplicity. We needed to be able to accomplish hundreds, if not thousands of runs, in a reasonable time period and to be able tounder stand the driving forces under different assumptions. We could not have a model with dynamics that were so complicated that neither we nor our readers could understand them intuitively. Thus, the modeling decisions have tended to err, ifany thing, on the side of parsimony. We describe each of the three primary parts of the model: the environment, the agents, and the agent’s interaction with the environment.Each location on the landscape (i.e., a lattice) has three characteristics: a score for aesthetic quality scaled to the interval [0, 1], the presence or absence of initial service centers, and an average distance to services, which is updated at each step. On our artificial landscapes we calculate service-center distance as Euclidean distance.When we are working with a real landscape, we incorporate the road network in to the distance calculation. We simplified the calculation of road distance by calculating, first, the straight-line distance to the nearest point on the nearest road,then the straight-line distance from that point to the nearest service center. This approach is likely to underestimate the true road distance, but provides a reasonable approximation that is much quicker to calculate and incorporates the most salient features of road networks.3.Validation methodsThis section describes the two primary approaches to validation that we demonstrate in this paper: aggregate validation with pattern metrics and thein variant-variant method. Each method is used to compare the agent-based model with a reference map and is demonstrated for several cases, which are described in Section 4.To perform statistical validation we make use of landscape pattern metrics,originally developed for landscape ecological investigations. These metrics are included for comparison with our new method. The primary appeal of landscapepattern metrics in validation is that they can characterize several different aspects of the global patterns that emerge from the model (Parker and Meretsky 2004), and they describe the patterns in a way that relates them to the ecological impacts of land-use change.In our approach, we distinguish between those locations that the model always predicts as developed or undeveloped –the invariant region –and those locations that sometimes get developed and sometimes do not –the variant region. Before describing how we construct these regions and their usefulness, we first describe a more standard approach to measuring spatial similarity in a restricted case.Suppose a run of a model locates a land-use type (e.g., development) at M sites among N possible sites, where M is also the number of sites at which the land use is found in the reference map. We could ask how accurately that model run predicted the exact locations. First count the number of the M developed locations predicted by the model that are correct (C). M - C locations that the model predicts are,therefore, incorrect. We can also partition the M developed locations in there ference map into two types: those predicted correctly (C) and those predicted incorrectly (M - C), and calculate user’s and producer’s accuracies for the developed class, which are identical in this situation, as C/M.4.Demonstrations of model validation methodsWe ran multiple experiments with our agent-based model to illustrate both the importance of path dependence and the utility of the validation methods. First, we created artificial landscapes as experimental situations in which the ‘‘true’’process and outcome are known perfectly, which is not possible using real-world data. Next,we used data on land-use change collected and analyzed over Washtenaw County,Michigan, which contains Ann Arbor and is immediately west of Detroit. The primary goal of the latter demonstration was to analyze the effects of different starting times on path dependence and model accuracy using real data.Our initial demonstrations, Cases 1.1 through 1.5 below, were designed to test for two influences on path dependence: agent behavior and the environmental features.For all of these demonstrations, we randomly selected a single run of the model as the reference map. We, therefore, compared the model to a reference map that, by definition, was generated by exactly the same process, i.e., a 100% correct model.Any differences between the model runs and the reference map were, therefore,indicative of inherent unpredictability of the system, due to either stochastic uncertainty or path dependence, and not of any flaw or weakness in the model.The next demonstrations (Cases 2.1 and 2.2) were intended to illustrate how too much focus on getting a strong spatial similarity between model patterns of land use and the reference map can lead one to construct an over fitted model. For both of these cases, we used the landscape with variable aesthetic quality in two peaks described above, and the parameter values listed in Table 1. In each case 10 residents entered per time step, with one new service center per 20 residents. Each run resulted in highly path dependent development, i.e., almost all development is on one peak or another, depending only on the choice of early settlers. We selected one run of this model as the reference map, deliberately choosing a run in which the peak of qx,y tothe northwest was developed. This selected run we designated as the ‘‘true history’’against which we wished to validate our model. The first comparison with this reference map (Case 2.1) was to assume, as before, that we knew the actual process generating the true history, i.e., we ran the same model multiple times with different random seeds.5.ResultsThe results from the first five cases (with parameters set as in table 1) indicated that the degree of predictability in the models was affected by both the behavior of the agents and the pattern of environmental variability (table 3). The landscape pattern metric values from any given case were never significantly different from the reference map, with the possible exception of MNN in Case 1.1. One striking result,however, given that the reference maps were created by the same models, is that the overall prediction accuracies were as low as 22 percent (Case 1.4), a result of the strongly path-dependent development exhibited in some of these cases. This accuracy level would probably be too low to convince referees or policy analysts to accept the model and yet the model is perfectly accurate.The overall prediction accuracy, and the size and accuracy of the invariant region,increased both when positive feedbacks were added to encourage development near existing development (Case 1.2) and when, in addition, the agents were responding to a variable pattern of aesthetic quality (Case 1.3). In addition to improving the size and predictability within the invariant regions, these changes had the effect of increasing the predictive ability of the model in the variant region as well (i.e., VC/VRD). This means that, where the model was less consistent in its prediction, it still made increasingly better predictions than random.6.Discussion and conclusionsIn this paper, we have introduced the invariant-variant method to assess the accuracy and variability of outcomes of spatial agent-based land-use models. This method advances existing techniques that measure spatial similarity. Most importantly, it helps us come to terms with a fundamental tension in land-use modeling –the emphasis on accurate prediction of location and the recognition of path dependence and stochastic uncertainty. The methods described here should apply to any land-use models that have the potential to generate multiple outcomes.They would not apply to models that are deterministic, and therefore make a single prediction of settlement patterns. By definition, deterministic models can not generate path dependence unless one considers the impact of interventions. In that case, our approach would be applicable with the invariant region being that portion of the region that is developed regardless of the policy intervention.Our proposed distinction between invariant and variant regions is a crude measure, but one that allows researchers to better understand the processes that lead to accurate (or inaccurate) predictions by their models. With it we can distinguish between models that always get something right, and those that always get different things right. And that difference matters. It may be possible to further develop the statistical properties of the most useful of these and similar measures. Such measures will enable us to categorize environments and actors who create systems for which anyaccurate model will have low predictive accuracy and those who create systems for which we should demand high accuracy.We expect that, over time and by comparing across models, we can understand what landscape attributes and behavioral characteristics lead to greater or lesser predictability as captured by the relative size of the invariant region. For example,homogeneity in the environment increases unpredictability because the number of paths becomes unwieldy. Admittedly, size of the invariant region is not the only possible measure of predictability, but it is a useful one. A large invariant region suggests a predictable settlement pattern. A small invariant region implies that history or even single events matter.Our analysis emphasized path dependence as opposed to stochastic uncertainty because of our interest, and that of many land-use modelers, in policy intervention.Stochastic uncertainty, like the weather, is something we can all complain about but not affect. Path dependence, at least in theory, offers the opportunity for intervention. If we know that two paths of development patterns are possible, then we might be able to influence the process, through policy and the use of what Holland called ‘‘lever points,’’such that the most desirable path, on some measure,emerges (Holland 1995, Glad well 2000). Path dependence makes fitting a model more difficult and may tempt modelers to overfit the data, since often the one actual path of development depends on specific details that influence the choices of early settlers. On the other hand, path dependence creates the possibility of policy leverage.The results of running the model from multiple starting times in the history (and pseudo-history) of Washtenaw County, Michigan, seem somewhat counter intuitive at first, in that the overall match of the locations of newly settled agents with those in the 1995 map decreased with increasing information (i.e., later starting times).However, the additional metrics tell more of the story. The model actually improved with later starting times when the matches were compared with the numbers that would be expected at random. Fewer agents entering the landscape at the later times means relatively more possible combinations of places they can locate in the undeveloped part of the map. The model does reasonably well at predicting the aggregate patterns, matching three of the four metrics, partially because much of the aggregate pattern is predetermined in the initial maps. The fact that three of the mean pattern-metric values were statistically indistinguishable from the 1995 values when starting at even the earliest dates, however, suggests that the match is not only due to the initial map information. The size of the invariant developed region declined with later starting times, but became more accurate., When we located fewer residents, we were much less likely to see them locate consistently, rightly or wrongly. Further, within the variant region, the model located residents less well than would be expected with simply random location. This suggests that some features were missing or structurally wrong in our model. Two possibilities are that our map of aesthetic quality in the outlying areas does not accurately reflect preferences, or that soil qualities or some other willingness-to-sell characteristic of locations contributes to where settlements occur.The comparison between Cases 2.1 and 2.2 highlights the importance of recognizing path dependence in land-use change processes and the dangers of over fitting the model to data in the modeling processes. This danger, i.e., that the model will match the outcome of a particular case well but misrepresent the process,is endemic to land-use change models. Many models of land-use change are developed through calibration and statistical fitting to observed changes, derived from remotely sensed and GIS data sets. This rather extreme example makes the point that, even though the outcomes of the model may match the reference map in meaningful ways, e.g., both statistically and spatially, we cannot necessarily conclude that the processes contained in the model are correct. If the processes are not well represented, of course, then we possess limited ability to evaluate policy outcomes, for example by changing incentives or creating zones that limit certain activities on the landscape.In the context of the foregoing discussion, it is useful to reflect on how to proceed with model development. If we use the results from Washtenaw County as an indication of the validity of the model and wish to improve its validity, what should be our next steps? There are a number of factors that we did not include in our model that could be included in agent decision-making. These include the price of land, zoning, the different kinds of residential, commercial, and industrial developments, a different representation of roads and distances, and the presence of areas restricted for development (like parks). Any of these factors could be included in the model in a way that would improve the fit of the output to the 1995map. But, each new factor we add will have associated with it parameters that need to be set. As soon as we start fitting these parameters according to the values that produce outputs that best fit the data, we run the risk of losing control of the process-based understanding that models of this sort helps us grapple with. As we proceed, the question becomes: are we interested in fitting the data or understanding the process?基于空间模型使用的路径依赖研究作者:丹尼尔·g·布朗;斯科特·佩奇;里克·瑞路;莫伊拉;威廉·兰德在本文的研究中,我们通过两个明确的、却截然不同的概念模型,准确而明显的凸显了这两者之间的紧张关系。
cfd英文文献
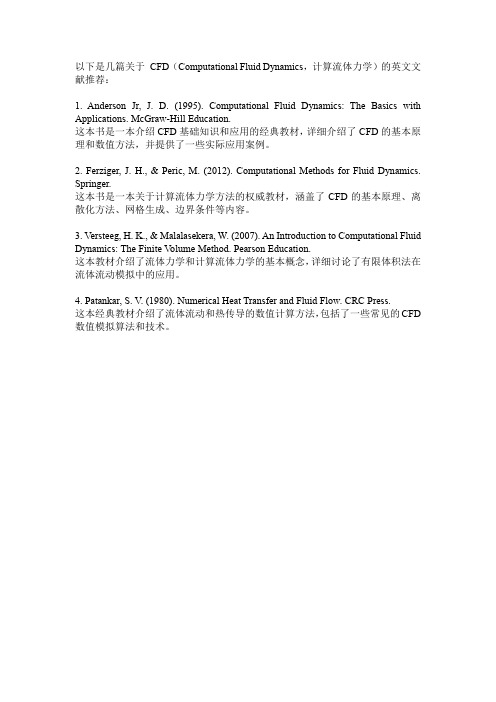
以下是几篇关于CFD(Computational Fluid Dynamics,计算流体力学)的英文文献推荐:
1. Anderson Jr, J. D. (1995). Computational Fluid Dynamics: The Basics with Applications. McGraw-Hill Education.
这本书是一本介绍CFD基础知识和应用的经典教材,详细介绍了CFD的基本原理和数值方法,并提供了一些实际应用案例。
2. Ferziger, J. H., & Peric, M. (2012). Computational Methods for Fluid Dynamics. Springer.
这本书是一本关于计算流体力学方法的权威教材,涵盖了CFD的基本原理、离散化方法、网格生成、边界条件等内容。
3. Versteeg, H. K., & Malalasekera, W. (2007). An Introduction to Computational Fluid Dynamics: The Finite V olume Method. Pearson Education.
这本教材介绍了流体力学和计算流体力学的基本概念,详细讨论了有限体积法在流体流动模拟中的应用。
4. Patankar, S. V. (1980). Numerical Heat Transfer and Fluid Flow. CRC Press.
这本经典教材介绍了流体流动和热传导的数值计算方法,包括了一些常见的CFD 数值模拟算法和技术。
reinforcement learning文献
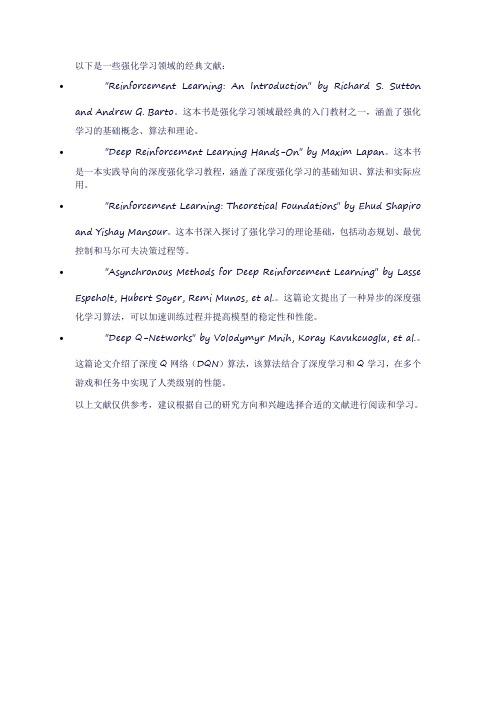
以下是一些强化学习领域的经典文献:•"Reinforcement Learning: An Introduction" by Richard S. Sutton and Andrew G. Barto。
这本书是强化学习领域最经典的入门教材之一,涵盖了强化学习的基础概念、算法和理论。
•"Deep Reinforcement Learning Hands-On" by Maxim Lapan。
这本书是一本实践导向的深度强化学习教程,涵盖了深度强化学习的基础知识、算法和实际应用。
•"Reinforcement Learning: Theoretical Foundations" by Ehud Shapiro and Yishay Mansour。
这本书深入探讨了强化学习的理论基础,包括动态规划、最优控制和马尔可夫决策过程等。
•"Asynchronous Methods for Deep Reinforcement Learning" by Lasse Espeholt, Hubert Soyer, Remi Munos, et al.。
这篇论文提出了一种异步的深度强化学习算法,可以加速训练过程并提高模型的稳定性和性能。
•"Deep Q-Networks" by Volodymyr Mnih, Koray Kavukcuoglu, et al.。
这篇论文介绍了深度Q网络(DQN)算法,该算法结合了深度学习和Q学习,在多个游戏和任务中实现了人类级别的性能。
以上文献仅供参考,建议根据自己的研究方向和兴趣选择合适的文献进行阅读和学习。
外文文献翻译原文+译文

外文文献翻译原文Analysis of Con tin uous Prestressed Concrete BeamsChris BurgoyneMarch 26, 20051、IntroductionThis conference is devoted to the development of structural analysis rather than the strength of materials, but the effective use of prestressed concrete relies on an appropriate combination of structural analysis techniques with knowledge of the material behaviour. Design of prestressed concrete structures is usually left to specialists; the unwary will either make mistakes or spend inordinate time trying to extract a solution from the various equations.There are a number of fundamental differences between the behaviour of prestressed concrete and that of other materials. Structures are not unstressed when unloaded; the design space of feasible solutions is totally bounded;in hyperstatic structures, various states of self-stress can be induced by altering the cable profile, and all of these factors get influenced by creep and thermal effects. How were these problems recognised and how have they been tackled?Ever since the development of reinforced concrete by Hennebique at the end of the 19th century (Cusack 1984), it was recognised that steel and concrete could be more effectively combined if the steel was pretensioned, putting the concrete into compression. Cracking could be reduced, if not prevented altogether, which would increase stiffness and improve durability. Early attempts all failed because the initial prestress soon vanished, leaving the structure to be- have as though it was reinforced; good descriptions of these attempts are given by Leonhardt (1964) and Abeles (1964).It was Freyssineti’s observations of the sagging of the shallow arches on three bridges that he had just completed in 1927 over the River Allier near Vichy which led directly to prestressed concrete (Freyssinet 1956). Only the bridge at Boutiron survived WWII (Fig 1). Hitherto, it had been assumed that concrete had a Young’s modulus which remained fixed, but he recognised that the de- ferred strains due to creep explained why the prestress had been lost in the early trials. Freyssinet (Fig. 2) also correctly reasoned that high tensile steel had to be used, so that some prestress would remain after the creep had occurred, and alsothat high quality concrete should be used, since this minimised the total amount of creep. The history of Freyssineti’s early prestressed concrete work is written elsewhereFigure1:Boutiron Bridge,Vic h yFigure 2: Eugen FreyssinetAt about the same time work was underway on creep at the BRE laboratory in England ((Glanville 1930) and (1933)). It is debatable which man should be given credit for the discovery of creep but Freyssinet clearly gets the credit for successfully using the knowledge to prestress concrete.There are still problems associated with understanding how prestressed concrete works, partly because there is more than one way of thinking about it. These different philosophies are to some extent contradictory, and certainly confusing to the young engineer. It is also reflected, to a certain extent, in the various codes of practice.Permissible stress design philosophy sees prestressed concrete as a way of avoiding cracking by eliminating tensile stresses; the objective is for sufficient compression to remain after creep losses. Untensionedreinforcement, which attracts prestress due to creep, is anathema. This philosophy derives directly from Freyssinet’s logic and is primarily a working stress concept.Ultimate strength philosophy sees prestressing as a way of utilising high tensile steel as reinforcement. High strength steels have high elastic strain capacity, which could not be utilised when used as reinforcement; if the steel is pretensioned, much of that strain capacity is taken out before bonding the steel to the concrete. Structures designed this way are normally designed to be in compression everywhere under permanent loads, but allowed to crack under high live load. The idea derives directly from the work of Dischinger (1936) and his work on the bridge at Aue in 1939 (Schonberg and Fichter 1939), as well as that of Finsterwalder (1939). It is primarily an ultimate load concept. The idea of partial prestressing derives from these ideas.The Load-Balancing philosophy, introduced by T.Y. Lin, uses prestressing to counter the effect of the permanent loads (Lin 1963). The sag of the cables causes an upward force on the beam, which counteracts the load on the beam. Clearly, only one load can be balanced, but if this is taken as the total dead weight, then under that load the beam will perceive only the net axial prestress and will have no tendency to creep up or down.These three philosophies all have their champions, and heated debates take place between them as to which is the most fundamental.2、Section designFrom the outset it was recognised that prestressed concrete has to be checked at both the working load and the ultimate load. For steel structures, and those made from reinforced concrete, there is a fairly direct relationship between the load capacity under an allowable stress design, and that at the ultimate load under an ultimate strength design. Older codes were based on permissible stresses at the working load; new codes use moment capacities at the ultimate load. Different load factors are used in the two codes, but a structure which passes one code is likely to be acceptable under the other.For prestressed concrete, those ideas do not hold, since the structure is highly stressed, even when unloaded. A small increase of load can cause some stress limits to be breached, while a large increase in load might be needed to cross other limits. The designer has considerable freedom to vary both the working load and ultimate load capacities independently; both need to be checked.A designer normally has to check the tensile and compressive stresses, in both the top and bottom fibre of the section, for every load case. The critical sections are normally, but not always, the mid-span and the sections over piers but other sections may become critical ,when the cable profile has to be determined.The stresses at any position are made up of three components, one of which normally has a different sign from the other two; consistency of sign convention is essential.If P is the prestressing force and e its eccentricity, A and Z are the area of the cross-section and its elastic section modulus, while M is the applied moment, then where ft and fc are the permissible stresses in tension and compression.c e t f ZM Z P A P f ≤-+≤Thus, for any combination of P and M , the designer already has four in- equalities to deal with.The prestressing force differs over time, due to creep losses, and a designer isusually faced with at least three combinations of prestressing force and moment;• the applied moment at the time the prestress is first applied, before creep losses occur,• the maximum applied moment after creep losses, and• the minimum applied moment after creep losses.Figure 4: Gustave MagnelOther combinations may be needed in more complex cases. There are at least twelve inequalities that have to be satisfied at any cross-section, but since an I-section can be defined by six variables, and two are needed to define the prestress, the problem is over-specified and it is not immediately obvious which conditions are superfluous. In the hands of inexperienced engineers, the design process can be very long-winded. However, it is possible to separate out the design of the cross-section from the design of the prestress. By considering pairs of stress limits on the same fibre, but for different load cases, the effects of the prestress can be eliminated, leaving expressions of the form:rangestress e Perm issibl Range Mom entZ These inequalities, which can be evaluated exhaustively with little difficulty, allow the minimum size of the cross-section to be determined.Once a suitable cross-section has been found, the prestress can be designed using a construction due to Magnel (Fig.4). The stress limits can all be rearranged into the form:()M fZ PA Z e ++-≤1 By plotting these on a diagram of eccentricity versus the reciprocal of the prestressing force, a series of bound lines will be formed. Provided the inequalities (2) are satisfied, these bound lines will always leave a zone showing all feasible combinations of P and e. The most economical design, using the minimum prestress, usually lies on the right hand side of the diagram, where the design is limited by the permissible tensile stresses.Plotting the eccentricity on the vertical axis allows direct comparison with the crosssection, as shown in Fig. 5. Inequalities (3) make no reference to the physical dimensions of the structure, but these practical cover limits can be shown as wellA good designer knows how changes to the design and the loadings alter the Magnel diagram. Changing both the maximum andminimum bending moments, but keeping the range the same, raises and lowers the feasible region. If the moments become more sagging the feasible region gets lower in the beam.In general, as spans increase, the dead load moments increase in proportion to the live load. A stage will be reached where the economic point (A on Fig.5) moves outside the physical limits of the beam; Guyon (1951a) denoted the limiting condition as the critical span. Shorter spans will be governed by tensile stresses in the two extreme fibres, while longer spans will be governed by the limiting eccentricity and tensile stresses in the bottom fibre. However, it does not take a large increase in moment ,at which point compressive stresses will govern in the bottom fibre under maximum moment.Only when much longer spans are required, and the feasible region moves as far down as possible, does the structure become governed by compressive stresses in both fibres.3、Continuous beamsThe design of statically determinate beams is relatively straightforward; the engineer can work on the basis of the design of individual cross-sections, as outlined above. A number of complications arise when the structure is indeterminate which means that the designer has to consider, not only a critical section,but also the behaviour of the beam as a whole. These are due to the interaction of a number of factors, such as Creep, Temperature effects and Construction Sequence effects. It is the development of these ideas whichforms the core of this paper. The problems of continuity were addressed at a conference in London (Andrew and Witt 1951). The basic principles, and nomenclature, were already in use, but to modern eyes concentration on hand analysis techniques was unusual, and one of the principle concerns seems to have been the difficulty of estimating losses of prestressing force.3.1 Secondary MomentsA prestressing cable in a beam causes the structure to deflect. Unlike the statically determinate beam, where this motion is unrestrained, the movement causes a redistribution of the support reactions which in turn induces additional moments. These are often termed Secondary Moments, but they are not always small, or Parasitic Moments, but they are not always bad.Freyssinet’s bridge across the Marne at Luzancy, started in 1941 but not completed until 1946, is often thought of as a simply supported beam, but it was actually built as a two-hinged arch (Harris 1986), with support reactions adjusted by means of flat jacks and wedges which were later grouted-in (Fig.6). The same principles were applied in the later and larger beams built over the same river.Magnel built the first indeterminate beam bridge at Sclayn, in Belgium (Fig.7) in 1946. The cables are virtually straight, but he adjusted the deck profile so that the cables were close to the soffit near mid-span. Even with straight cables the sagging secondary momentsare large; about 50% of the hogging moment at the central support caused by dead and live load.The secondary moments cannot be found until the profile is known but the cablecannot be designed until the secondary moments are known. Guyon (1951b) introduced the concept of the concordant profile, which is a profile that causes no secondary moments; es and ep thus coincide. Any line of thrust is itself a concordant profile.The designer is then faced with a slightly simpler problem; a cable profile has to be chosen which not only satisfies the eccentricity limits (3) but is also concordant. That in itself is not a trivial operation, but is helped by the fact that the bending moment diagram that results from any load applied to a beam will itself be a concordant profile for a cable of constant force. Such loads are termed notional loads to distinguish them from the real loads on the structure. Superposition can be used to progressively build up a set of notional loads whose bending moment diagram gives the desired concordant profile.3.2 Temperature effectsTemperature variations apply to all structures but the effect on prestressed concrete beams can be more pronounced than in other structures. The temperature profile through the depth of a beam (Emerson 1973) can be split into three components for the purposes of calculation (Hambly 1991). The first causes a longitudinal expansion, which is normally released by the articulation of the structure; the second causes curvature which leads to deflection in all beams and reactant moments in continuous beams, while the third causes a set of self-equilibrating set of stresses across the cross-section.The reactant moments can be calculated and allowed-for, but it is the self- equilibrating stresses that cause the main problems for prestressed concrete beams. These beams normally have high thermal mass which means that daily temperature variations do not penetrate to the core of the structure. The result is a very non-uniform temperature distribution across the depth which in turn leads to significant self-equilibrating stresses. If the core of the structure is warm, while the surface is cool, such as at night, then quite large tensile stresses can be developed on the top and bottom surfaces. However, they only penetrate a very short distance into the concrete and the potential crack width is very small. It can be very expensive to overcome the tensile stress by changing the section or the prestress。
工程管理外文文献
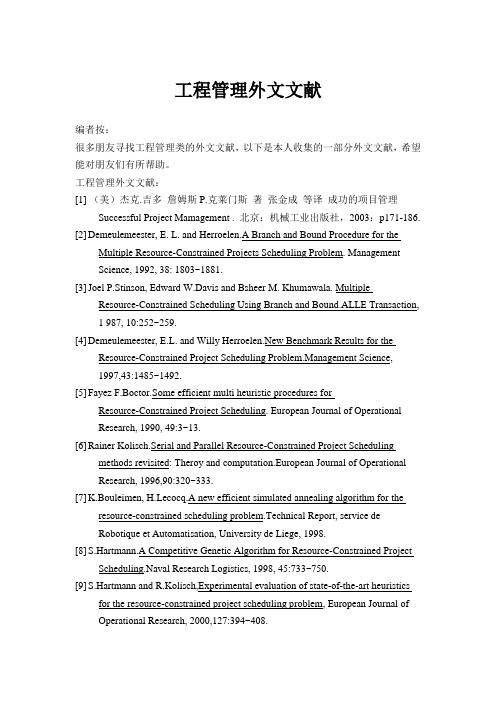
工程管理外文文献编者按:很多朋友寻找工程管理类的外文文献,以下是本人收集的一部分外文文献,希望能对朋友们有所帮助。
工程管理外文文献:[1](美)杰克.吉多詹姆斯P.克莱门斯著张金成等译成功的项目管理Successful Project Mamagement . 北京:机械工业出版社,2003:p171-186.[2]Demeulemeester, E. L. and Herroelen.A Branch and Bound Procedure for theMultiple Resource-Constrained Projects Scheduling Problem. ManagementScience, 1992, 38: 1803~1881.[3]Joel P.Stinson, Edward W.Davis and Bsheer M. Khumawala. MultipleResource-Constrained Scheduling Using Branch and Bound.ALLE Transaction,1 987, 10:252~259.[4]Demeulemeester, E.L. and Willy Herroelen.New Benchmark Results for theResource-Constrained Project Scheduling Problem.Management Science,1997,43:1485~1492.[5]Fayez F.Boctor.Some efficient multi heuristic procedures forResource-Constrained Project Scheduling. European Journal of OperationalResearch, 1990, 49:3~13.[6]Rainer Kolisch.Serial and Parallel Resource-Constrained Project Schedulingmethods revisited: Theroy and computation.European Journal of OperationalResearch, 1996,90:320~333.[7]K.Bouleimen, H.Lecocq.A new efficient simulated annealing algorithm for theresource-constrained scheduling problem.Technical Report, service deRobotique et Automatisation, University de Liege, 1998.[8]S.Hartmann.A Competitive Genetic Algorithm for Resource-Constrained ProjectScheduling.Naval Research Logistics, 1998, 45:733~750.[9]S.Hartmann and R.Kolisch,Experimental evaluation of state-of-the-art heuristicsfor the resource-constrained project scheduling problem, European Journal of Operational Research, 2000,127:394~408.[10]Fendley, L.G.Towards the Development of a Complete Multi-project SchedulingSystem. Journal of Industrial Engineering, 1968, 12:505~515.[11]Kurtulus.I, E.W.Davis. Multi-Project Scheduling: Categorization of HeuristicRules Performance.Management Science, 1982, 2:25~31.[12]Shigeru Tsubakitani, Richard F.Deckro. A heuristic for multi-project schedulingwith limited resources in the housing industry.European Journal of Operational Research, 1990, 49:80~91.[13]Soo-Young Kim, Robert C. Leachman.Multi-Project Scheduling with ExplicitLateness Costs. IIE Transactions, 1993, 25:34~43.[14]Paul C .Dinsmore,Winning in Business With Enterprise Project Management,PMI,1999.[15]Leach L P. Critical chain project management [M]. London: Artech House Inc,2000, 236~257[16]鲍伯,弗斯特.IS09001: 2000质量管理体系.中国标准出版社.2001:P.22-P.283.[17](美)杰克.吉多詹姆斯P.克莱门斯著张金成等译成功的项目管理Successful Project Mamagement . 北京:机械工业出版社,2003:p171-186.[18]项目管理知识体系(PMBOK, Project Management Body ofKnowledge) 是美国项目管理学会(PMI, Project ManagementInstitute)开发的一个关于项目管理的标准。
一些学习经济学用到的的数学书
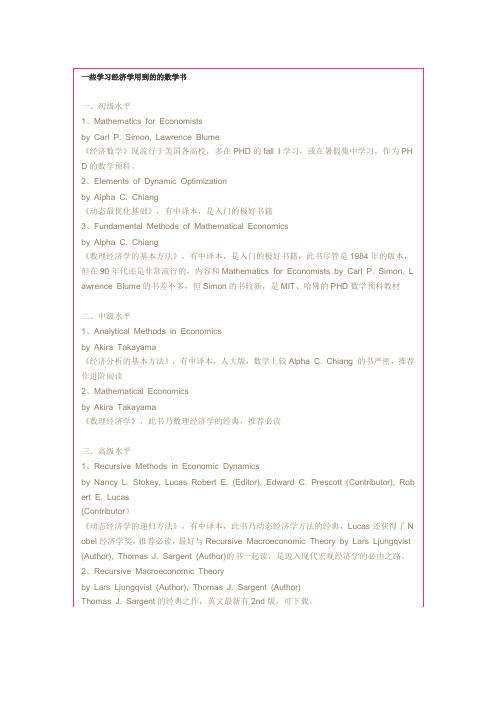
1、Analytical Methods in Economics
by Akira Takayama
《经济分析的基本方法》,有中译本,人大版,数学上较Alpha C. Chiang的书严密,推荐作进阶阅读
2、Mathematical Economics
by Akira Takayama
《数理经济学》,此书乃数理经济学的经典,推荐必读
《动态规划》,此书是动态规划领域的经典;
4、Stochastic Optimal Control: The Discrete Time Case
by Dimitri P. Bertsekas, Steven E. Shreve
《随机最优控制》,推荐阅读
5、Dynamic Programming and Optimal Control: 2nd Edition (Volumes 1 and 2)
一些学习经济学用到的的数学书
一、初级水平
1、Mathematics for Economists
by Carl P. Simon, Lawrence Blume
《经济数学》现流行于美国各高校,多在PHD的fall I学习,或在暑假集中学习,作为PHD的数学预科。
2、Elements of Dynamic Optimization
3、阅读高级水平的书需要一点实分析和泛函分析、随机过程、高等概率论的知识,只求应用者只需稍加学习,想进一步深入研究者最好选修数学系相关课程。
2、Recursive Macroeconomic Theory
by Lars Ljungqvist (Author), Thomas J. Sargent (Author)
Thomas J. Sargent的经典之作,英文最新有2nd版,可下载。
基于近似动态规划算法研究
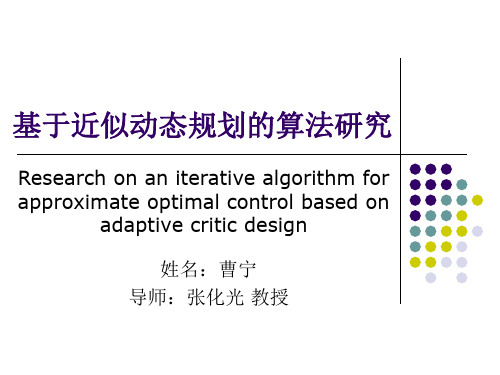
u(t) Action Network
x(t)
u(t) Action Network
x(t)
HDP评论网的训练
J (t) ktU[x(k),u(k), k] k t
Eh Eh (t)
t
1 [Jˆ(t) U (t) Jˆ(t 1)]2 2t
Jˆ(t) U (t) Jˆ(t 1) U (t) [U (t 1) Jˆ(t 2)]
-0.2 0
120
100
80
60
40
20
0 0
50
100
150
200
250
300
Time step
50
100
150
200
250
300
Time step
State trajecteory x2
The control
0.3 0.25
0.2 0.15
0.1 0.05
0 -0.05
0
4.5 4
3.5 3
2.5 2
输出 a f (n)
Inputs Multiple-input Neuron ouputs
p1
w1,1
p2
∑n f a
••
••
••
b
pR
w1,R 1
a=f(Wp+b)
其中 n w1,1 p1 w1,2 p2 w1,R pR b
神经网络模型(Network architectures)
w1i,j
线性系统HJB方程的解) ➢ 5.Neural Network Modeling(神经网络建模)
1. Introduction
动态规划及贝尔曼最优性原理
城市规划_从终极蓝图到动态规划_动态规划实践与理论_王富海
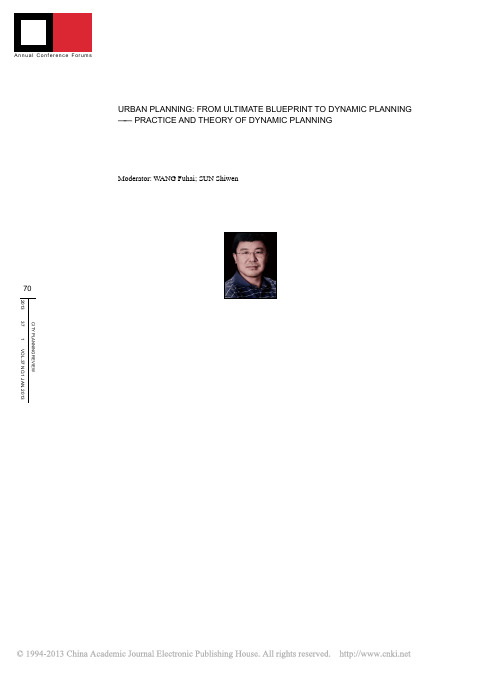
A n n u a l C o n f 城市规划 CITY PLANNING REVIEW2013年 第37卷 第1期 VOL.37 NO.1 JAN. 201370【修改日期】2013-01-06【文章编号】1002-1329 (2013)01-0070-06【中图分类号】TU984【文献标识码】C 王富海(中国城市规划学会理事,深圳市蕾奥城市规划设计咨询有限公司董事长,同济大学兼职教授,教授级高级城市规划师):欢迎大家来分享本次自由论坛:从终极蓝图到动态规划,这是一个关于规划理论的讨论。
规划面临转型,但转型的方向会有不同的角度,我从动态规划理论角度切入。
规划原理以静态规划理论为主线,对城市的认识是简化的,现状是丑化的,愿景是神化的,目标是美化的,作用方式是教化,对现实问题只能淡化,理想丰满而现实骨感。
针对蓝图式规划的种种弊端,国外早已出现了一系列关于动态规划理论与实践的成果,系统规划理论、连续性规划理论、行动规划模型等等,与传统规划相比,它把规划看成一个过程,而不是结果,既注重建设行为的协调性,更注重运用政策杠杆,更加关注近期的需要并强调灵活性。
规划不再是被动的蓝图,而成为改善城市的主动而具体的工具。
在10年前的中国规划界,也许普遍认为西方的规划演进与我们关系不大,但经过城镇化逐步成为国家主题、城市扩张成为地方施政核心的这10年,规划的工具作用更加明显,变革的需求越发迫切。
在今天论坛探讨的话题下,我们探讨的话题可以延伸为:存不存在中国的动态规划?即便有,时机到没到?动态规划有哪些实践基础?动态规划有哪些理论基础?动态规划理论的核心要点是什么?如何形成动态规划理论?动态规划理论怎样应用,宏观与微观层面如何实现?行动规划是新的规划品种吗?现阶段动态规划应侧重理论突破还是经验推广?静态规划理论的关键误区在于对城市的认知,流于对城市物质形态进行概括和分解组合,即便考虑社会经济要素,也是宏观的、断面的,不探究城市的运行,不清楚影响城市的要素,不考虑规划对城市的作用和反作用,培养出来的规划师很可能是浮于表面而不深入现实的。
- 1、下载文档前请自行甄别文档内容的完整性,平台不提供额外的编辑、内容补充、找答案等附加服务。
- 2、"仅部分预览"的文档,不可在线预览部分如存在完整性等问题,可反馈申请退款(可完整预览的文档不适用该条件!)。
- 3、如文档侵犯您的权益,请联系客服反馈,我们会尽快为您处理(人工客服工作时间:9:00-18:30)。
外文文献:Adaptive Dynamic Programming: AnIntroductionAbstract: In this article, we introduce some recent research trends within the field of adaptive/approximate dynamic programming (ADP), including the variations on the structure of ADP schemes, the development of ADP algorithms and applications of ADP schemes. For ADP algorithms, the point of focus is that iterative algorithms of ADP can be sorted into two classes: one class is the iterative algorithm with initial stable policy; the other is the one without the requirement of initial stable policy. It is generally believed that the latter one has less computation at the cost of missing the guarantee of system stability during iteration process. In addition, many recent papers have provided convergence analysis associated with the algorithms developed. Furthermore, we point out some topics for future studies.IntroductionAs is well known, there are many methods for designing stable control for nonlinear systems. However, stability is only a bare minimum requirement in a system design. Ensuring optimality guarantees the stability of the nonlinear system. Dynamic programming is a very useful tool in solving optimization and optimal control problems by employing the principle of optimality. In [16], the principle of optimality is expressed as: “An optimal policy has the property that whatever the initial state and initial decision are, the remaining decisions must constitute an optimal policy with regard to the state resulting from the first decision.” There are several spectrums about the dynamic programming. One can consider discrete-time systems or continuous-time systems, linear systems or nonlinear systems, time-invariant systems or time-varying systems, deterministic systems or stochastic systems, etc.We first take a look at nonlinear discrete-time (timevarying) dynamical (deterministic) systems. Time-varying nonlinear systems cover most of the application areas and discrete-time is the basic consideration for digital computation. Supposethat one is given a discrete-time nonlinear (timevarying) dynamical systemwhere nu R∈denotes the ∈represents the state vector of the system and mx Rcontrol action and F is the system function. Suppose that one associates with this system the performance index (or cost)where U is called the utility function and g is the discount factor with 0 , g # 1. Note that the function J is dependent on the initial time i and the initial state x( i ), and it is referred to as the cost-to-go of state x( i ). The objective of dynamic programming problem is to choose a control sequence u(k), k5i, i11,c, so that the function J (i.e., the cost) in (2) is minimized. According to Bellman, the optimal cost from time k is equal toThe optimal control u* 1k2 at time k is the u1k2 which achieves this minimum, i.e.,Equation (3) is the principle of optimality for discrete-time systems. Its importance lies in the fact that it allows one to optimize over only one control vector at a time by working backward in time.In nonlinear continuous-time case, the system can be described byThe cost in this case is defined asFor continuous-time systems, Bellman’s principle of optimality can be applied, too. The optimal cost J*(x0)5min J(x0, u(t)) will satisfy the Hamilton-Jacobi-Bellman EquationEquations (3) and (7) are called the optimality equations of dynamic programming which are the basis for implementation of dynamic programming. In the above, if the function F in (1) or (5) and the cost function J in (2) or (6) are known, the solution of u(k ) becomes a simple optimization problem. If the system is modeled by linear dynamics and the cost function to be minimized is quadratic in the state and control, then the optimal control is a linear feedback of the states, where the gains are obtained by solving a standard Riccati equation [47]. On the other hand, if the system is modeled by nonlinear dynamics or the cost function is nonquadratic, the optimal state feedback control will depend upon solutions to the Hamilton-Jacobi-Bellman (HJB) equation [48] which is generally a nonlinear partial differential equation or difference equation. However, it is often computationally untenable to run true dynamic programming due to the backward numerical process required for its solutions, i.e., as a result of the well-known “curse of dimensionality” [16], [28]. In [69], three curses are displayed in resource management and control problems to show the cost function J , which is the theoretical solution of the Hamilton-Jacobi- Bellman equation, is very difficult to obtain, except for systems satisfying some very good conditions. Over the years, progress has been made to circumvent the “curse of dimensionality” by building a system, called“critic”, to approximate the cost function in dynamic programming (cf. [10], [60], [61], [63], [70], [78], [92], [94], [95]). The idea is to approximate dynamic programming solutions by using a function approximation structure such as neural networks to approximate the cost function. The Basic Structures of ADPIn recent years, adaptive/approximate dynamic programming (ADP) has gainedmuch attention from many researchers in order to obtain approximate solutions of the HJB equation,cf. [2], [3], [5], [8], [11]–[13], [21], [22], [25], [30], [31], [34], [35], [40], [46], [49], [52], [54], [55], [63], [70], [76], [80], [83], [95], [96], [99], [100]. In 1977, Werbos [91] introduced an approach for ADP that was later called adaptive critic designs (ACDs). ACDs were proposed in [91], [94], [97] as a way for solving dynamic programming problems forward-in-time. In the literature, there are several synonyms used for “Adaptive Critic Designs” [10], [24], [39], [43], [54], [70], [71], [87], including “Approximate Dynamic Programming” [69], [82], [95], “Asymptotic Dynamic Programming” [75], “Adaptive Dynamic Programming”[63], [64], “Heuristic Dynamic Programming” [46],[93], “Neuro-Dynamic Programming” [17], “Neural Dynamic Programming” [82], [101], and “Reinforcement Learning” [84].Bertsekas and Tsitsiklis gave an overview of the neurodynamic programming in their book [17]. They provided the background, gave a detailed introduction to dynamic programming, discussed the neural network architectures and methods for training them, and developed general convergence theorems for stochastic approximation methods as the foundation for analysis of various neuro-dynamic programming algorithms. They provided the core neuro-dynamic programming methodology, including many mathematical results and methodological insights. They suggested many useful methodologies for applications to neurodynamic programming, like Monte Carlo simulation, on-line and off-line temporal difference methods, Q-learning algorithm, optimistic policy iteration methods, Bellman error methods, approximate linear programming, approximate dynamic programming with cost-to-go function, etc. A particularly impressive success that greatly motivated subsequent research, was the development of a backgammon playing program by Tesauro [85]. Here a neural network was trained to approximate the optimal cost-to-go function of the game of backgammon by using simulation, that is, by letting the program play against itself. Unlike chess programs, this program did not use lookahead of many steps, so its success can be attributed primarily to the use of a properly trained approximation of the optimal cost-to-go function.To implement the ADP algorithm, Werbos [95] proposed a means to get aroundthis numerical complexity by using “approximate dynamic programming” formulations. His methods approximate the original problem with a discrete formulation. Solution to the ADP formulation is obtained through neural network based adaptive critic approach. The main idea of ADP is shown in Fig. 1.He proposed two basic versions which are heuristic dynamic programming (HDP) and dual heuristic programming (DHP).HDP is the most basic and widely applied structure of ADP [13], [38], [72], [79], [90], [93], [104], [106]. The structure of HDP is shown in Fig. 2. HDP is a method for estimating the cost function. Estimating the cost function for a given policy only requires samples from the instantaneous utility function U, while models of the environment and the instantaneous reward are needed to find the cost function corresponding to the optimal policy.In HDP, the output of the critic network is J^, which is the estimate of J in equation (2). This is done by minimizing the following error measure over timewhere J^(k)5J^ 3x(k), u(k), k, WC4 and WC represents the parameters of the critic network. When Eh50 for all k, (8) implies thatDual heuristic programming is a method for estimating the gradient of the cost function, rather than J itself. To do this, a function is needed to describe the gradient of the instantaneous cost function with respect to the state of the system. In the DHP structure, the action network remains the same as the one for HDP, but for the second network, which is called the critic network, with the costate as its output and the state variables as its inputs.The critic network’s training is more complicated than that in HDP since we need to take into account all relevant pathways of backpropagation.This is done by minimizing the following error measure over timewhere 'J^ 1k2 /'x1k2 5'J^ 3x1k2, u1k2, k, WC4/'x1k2 and WC represents theparameters of the critic network. When Eh50 for all k, (10) implies that2. Theoretical DevelopmentsIn [82], Si et al summarizes the cross-disciplinary theoretical developments of ADP and overviews DP and ADP; and discusses their relations to artificial intelligence, approximation theory, control theory, operations research, and statistics.In [69], Powell shows how ADP, when coupled with mathematical programming, can solve (approximately) deterministic or stochastic optimization problems that are far larger than anything that could be solved using existing techniques and shows the improvement directions of ADP.In [95], Werbos further gave two other versions called “actiondependent critics,” namely, ADHDP (also known as Q-learning [89]) and ADDHP. In the two ADP structures, the control is also the input of the critic networks. In 1997, Prokhorov and Wunsch [70] presented more algorithms according to ACDs.They discussed the design families of HDP, DHP, and globalized dual heuristic programming (GDHP). They suggested some new improvements to the original GDHP design. They promised to be useful for many engineering applications in the areas of optimization and optimal control. Based on one of these modifications, they present a unified approach to all ACDs. This leads to a generalized training procedure for ACDs. In [26], a realization of ADHDP was suggested: a least squares support vector machine (SVM) regressor has been used for generating the control actions, while an SVM-based tree-type neural network (NN) is used as the critic. The GDHP or ADGDHP structure minimizes the error with respect to both the cost and its derivatives. While it is more complex to do this simultaneously, the resulting behavioris expected to be superior. So in [102], GDHP serves as a reconfigurable controller to deal with both abrupt and incipient changes in the plant dynamics due to faults. A novel fault tolerant control (FTC) supervisor is combined with GDHP for the purpose of improving the performance of GDHP for fault tolerant control. When the plant is affected by a known abrupt fault, the new initial conditions of GDHP are loaded from dynamic model bank (DMB). On the other hand, if the fault is incipient, the reconfigurable controller maintains performance by continuously modifying itself without supervisor intervention. It is noted that the training of three networks used to implement the GDHP is in an online fashion by utilizing two distinct networks to implement the critic. The first critic network is trained at every iterations while the second one is updated with a copy of the first one at a given period of iterations.All the ADP structures can realize the same function that is to obtain the optimal control policy while the computation precision and running time are different from each other. Generally speaking, the computation burden of HDP is low but the computation precision is also low; while GDHP has better precision but the computation process will take longer time and the detailed comparison can be seen in [70]. In [30], [33] and [83], the schematic of direct heuristic dynamic programming is developed. Using the approach of [83], the model network in Fig. 1 is not needed anymore. Reference [101] makes significant contributions to model-free adaptive critic designs. Several practical examples are included in [101] for demonstration which include single inverted pendulum and triple inverted pendulum. A reinforcement learning-based controller design for nonlinear discrete-time systems with input constraints is presented by [36], where the nonlinear tracking control is implemented with filtered tracking error using direct HDP designs. Similar works also see [37]. Reference [54] is also about model-free adaptive critic designs. Two approaches for the training of critic network are provided in [54]: A forward-in-time approach and a backward-in-time approach. Fig. 4 shows the diagram of forward-intimeapproach. In this approach, we view J^(k) in (8) as the output of the critic network to be trained and choose U(k)1gJ^(k11) as the training target. Note that J^(k) and J^(k11) are obtained using state variables at different time instances. Fig. 5shows the diagram of backward-in-time approach. In this approach, we view J^(k11) in (8) as the output of the critic network to be trained and choose ( J^(k)2U(k))/g as the training target. The training ap proach of [101] can be considered as a backward- in-time ap proach. In Fig. 4 and Fig. 5, x(k11) is the output of the model network.An improvement and modification to the two network architecture, which is called the “single network adaptive critic(SNAC)” was presented in [65], [66]. This approach eliminates the action network. As a consequence, the SNAC architecture offers three potential advantages: a simpler architecture, lesser computational load (about half of the dual network algorithms), and no approximate error due to the fact that the action network is eliminated. The SNAC approach is applicable to a wide class of nonlinear systems where the optimal control (stationary) equation can be explicitly expressed in terms of the state and the costate variables. Most of the problems in aerospace, automobile, robotics, and other engineering disciplines can be characterized by the nonlinear control-affine equations that yield such a relation. SNAC-based controllers yield excellent tracking performances in applications to microelectronic mechanical systems, chemical reactor, and high-speed reentry problems. Padhi et al. [65] have proved that for linear systems (where the mapping between the costate at stage k11 and the state at stage k is linear), the solution obtained by the algorithm based on the SNAC structure converges to the solution of discrete Riccati equation.译文:自适应动态规划综述摘要:自适应动态规划(Adaptive dynamic programming, ADP) 是最优控制领域新兴起的一种近似最优方法, 是当前国际最优化领域的研究热点. ADP 方法利用函数近似结构来近似哈密顿{ 雅可比{ 贝尔曼(Hamilton-Jacobi-Bellman, HJB)方程的解, 采用离线迭代或者在线更新的方法, 来获得系统的近似最优控制策略, 从而能够有效地解决非线性系统的优化控制问题. 本文按照ADP 的结构变化、算法的发展和应用三个方面介绍ADP 方法. 对目前ADP 方法的研究成果加以总结, 并对这一研究领域仍需解决的问题和未来的发展方向作了进一步的展望。