核函数选择方法研究
svr目标表达式的解释
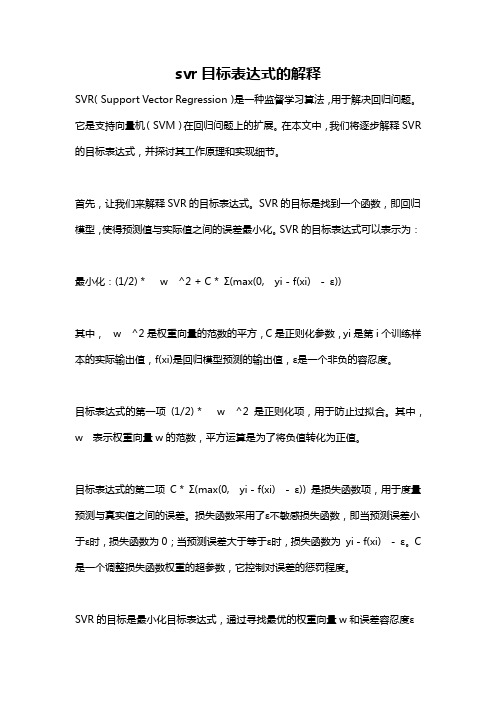
svr目标表达式的解释SVR(Support Vector Regression)是一种监督学习算法,用于解决回归问题。
它是支持向量机(SVM)在回归问题上的扩展。
在本文中,我们将逐步解释SVR 的目标表达式,并探讨其工作原理和实现细节。
首先,让我们来解释SVR的目标表达式。
SVR的目标是找到一个函数,即回归模型,使得预测值与实际值之间的误差最小化。
SVR的目标表达式可以表示为:最小化:(1/2) * w ^2 + C * Σ(max(0, yi - f(xi) - ε))其中,w ^2是权重向量的范数的平方,C是正则化参数,yi是第i个训练样本的实际输出值,f(xi)是回归模型预测的输出值,ε是一个非负的容忍度。
目标表达式的第一项(1/2) * w ^2 是正则化项,用于防止过拟合。
其中,w 表示权重向量w的范数,平方运算是为了将负值转化为正值。
目标表达式的第二项C * Σ(max(0, yi - f(xi) - ε)) 是损失函数项,用于度量预测与真实值之间的误差。
损失函数采用了ε不敏感损失函数,即当预测误差小于ε时,损失函数为0;当预测误差大于等于ε时,损失函数为yi - f(xi) - ε。
C 是一个调整损失函数权重的超参数,它控制对误差的惩罚程度。
SVR的目标是最小化目标表达式,通过寻找最优的权重向量w和误差容忍度ε来求解回归问题。
接下来,我们将逐步回答SVR算法的工作原理和实现细节。
1. 数据预处理:首先,我们需要对训练数据进行预处理,包括特征选择、特征缩放和数据标准化等。
这些步骤有助于提高算法的性能和收敛速度。
2. 核函数选择:SVR算法使用核函数来映射输入空间到高维特征空间,以处理非线性问题。
常用的核函数包括线性核、多项式核和高斯核等。
选择合适的核函数对算法的性能至关重要。
3. 优化求解:SVR目标表达式是一个凸二次规划问题,可以通过优化算法求解。
常用的优化方法包括序列最小最优化(SMO)算法、梯度下降算法和牛顿法等。
非线性回归模型中的核函数选择问题研究论文素材
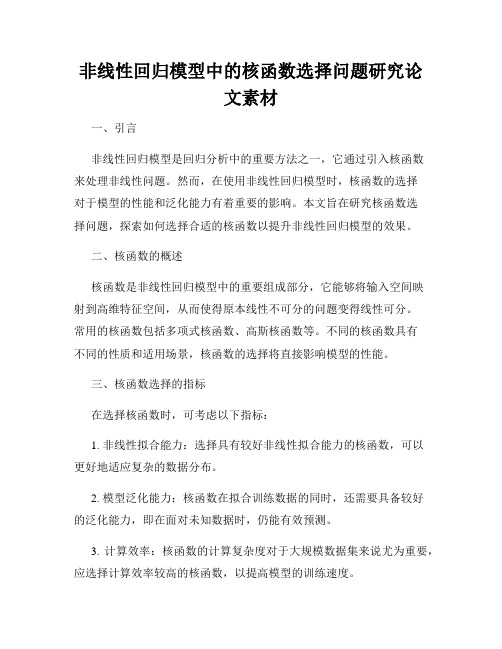
非线性回归模型中的核函数选择问题研究论文素材一、引言非线性回归模型是回归分析中的重要方法之一,它通过引入核函数来处理非线性问题。
然而,在使用非线性回归模型时,核函数的选择对于模型的性能和泛化能力有着重要的影响。
本文旨在研究核函数选择问题,探索如何选择合适的核函数以提升非线性回归模型的效果。
二、核函数的概述核函数是非线性回归模型中的重要组成部分,它能够将输入空间映射到高维特征空间,从而使得原本线性不可分的问题变得线性可分。
常用的核函数包括多项式核函数、高斯核函数等。
不同的核函数具有不同的性质和适用场景,核函数的选择将直接影响模型的性能。
三、核函数选择的指标在选择核函数时,可考虑以下指标:1. 非线性拟合能力:选择具有较好非线性拟合能力的核函数,可以更好地适应复杂的数据分布。
2. 模型泛化能力:核函数在拟合训练数据的同时,还需要具备较好的泛化能力,即在面对未知数据时,仍能有效预测。
3. 计算效率:核函数的计算复杂度对于大规模数据集来说尤为重要,应选择计算效率较高的核函数,以提高模型的训练速度。
四、常用的核函数及其特点以下是几种常用的核函数及其特点:1. 多项式核函数:多项式核函数是一种常用的核函数,具有较强的非线性拟合能力。
它的形式为K(x, y) = (x^T y + c)^d,其中c为常数,d为多项式的阶数。
2. 高斯核函数:高斯核函数是一种常用的平滑函数,具有较好的非线性拟合能力和泛化能力。
它的形式为K(x, y) = exp(-||x - y||^2 / (2 * sigma^2)),其中sigma为高斯核函数的带宽参数。
3. Sigmoid核函数:Sigmoid核函数具有非常好的非线性拟合能力,可用于解决二分类问题。
它的形式为K(x, y) = tanh(alpha * x^T y + c),其中alpha和c为常数。
五、核函数选择的方法在实际应用中,选择合适的核函数是一个复杂的问题。
以下是一些常用的核函数选择方法:1. 经验法则:根据先验经验选择常用的核函数,如高斯核函数在实际问题中通常表现较好。
基于样本分布特征的核函数选择方法研究
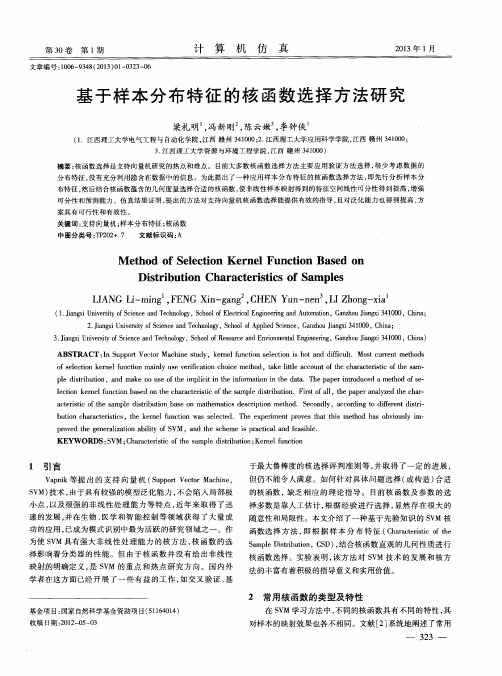
3 . J i a n g x i U n i v e s r i t y o f S c i e n c e a l l d T e c h n o l o g y , S c h o o l o f R e s o u r c e a n d E n v i o n m e n t a l E n g i n e e i r n g , G a n z h o u J i a n g x i 3 4 1 0 0 0 , C h i n a )
2 . J i a n g x i Un i v e r s i t y o f S c i e n c e a n d T e c h n o l o g y ,S c h o o l o f A p p l i e d S c i e n c e ,G a n z h o u J i a n g x i 3 4 1 0 0 0 ,C h i n a ;
第3 0 卷 第1 期
文章编号 : 1 0 0 6 — 9 3 4 8 ( 2 0 1 3 ) O 1 - 0 3 2 3 - 0 6
计
算
机
仿
真
2 0 1 3 年1 月
基 于 样 本 分 布 特 征 的核 函 数 选 择 方 法 研 究
梁礼 明 , 冯新 刚 , 陈云嫩 , 李钟侠
( 1 .江西理工大学 电气工程与 自动化学院 , 江西 赣州 3 4 1 0 0 0; 2 . 江西理工 大学应用科学学院 , 江西 赣州 3 4 1 0 0 0 ; 3 . 江西理工大学资源与环境工程学院 , 江西 赣 州 3 4 1 0 0 0 ) 摘要 : 核 函数选择是支持 向量机研究 的热点 和难点 。目前大多数核 函数选择方法 主要应用验证方 法选择 , 很少考 虑数据 的 分布特征 , 没有充 分利用 隐含在数据 中的信息 。为此提 出了一种应 用样本分布 特征的核 函数选择 方法 , 即先行分 析样本分
如何选择支持向量机的核函数
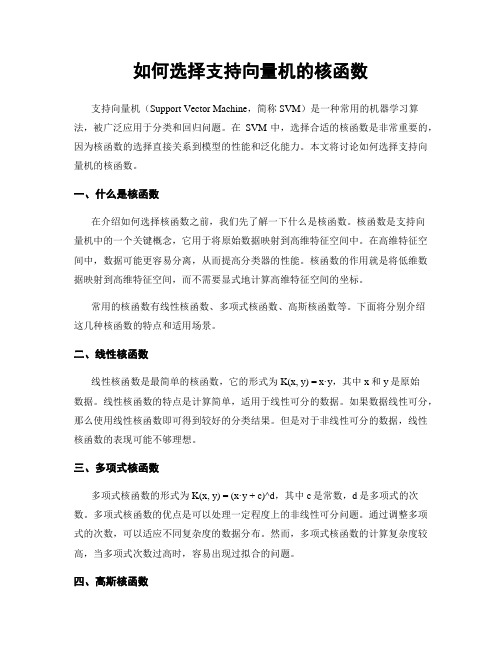
如何选择支持向量机的核函数支持向量机(Support Vector Machine,简称SVM)是一种常用的机器学习算法,被广泛应用于分类和回归问题。
在SVM中,选择合适的核函数是非常重要的,因为核函数的选择直接关系到模型的性能和泛化能力。
本文将讨论如何选择支持向量机的核函数。
一、什么是核函数在介绍如何选择核函数之前,我们先了解一下什么是核函数。
核函数是支持向量机中的一个关键概念,它用于将原始数据映射到高维特征空间中。
在高维特征空间中,数据可能更容易分离,从而提高分类器的性能。
核函数的作用就是将低维数据映射到高维特征空间,而不需要显式地计算高维特征空间的坐标。
常用的核函数有线性核函数、多项式核函数、高斯核函数等。
下面将分别介绍这几种核函数的特点和适用场景。
二、线性核函数线性核函数是最简单的核函数,它的形式为K(x, y) = x·y,其中x和y是原始数据。
线性核函数的特点是计算简单,适用于线性可分的数据。
如果数据线性可分,那么使用线性核函数即可得到较好的分类结果。
但是对于非线性可分的数据,线性核函数的表现可能不够理想。
三、多项式核函数多项式核函数的形式为K(x, y) = (x·y + c)^d,其中c是常数,d是多项式的次数。
多项式核函数的优点是可以处理一定程度上的非线性可分问题。
通过调整多项式的次数,可以适应不同复杂度的数据分布。
然而,多项式核函数的计算复杂度较高,当多项式次数过高时,容易出现过拟合的问题。
四、高斯核函数高斯核函数也称为径向基函数(Radial Basis Function,简称RBF),它的形式为K(x, y) = exp(-||x-y||^2 / (2σ^2)),其中σ是高斯核函数的宽度参数。
高斯核函数的特点是能够处理非线性可分问题,并且具有较好的泛化能力。
通过调整高斯核函数的宽度参数,可以控制支持向量机的决策边界的平滑程度。
五、选择核函数的方法在选择核函数时,需要考虑以下几个因素:1. 数据特点:首先要分析数据的特点,判断数据是否线性可分,是否存在复杂的非线性关系。
基于优化算法的核函数参数选择的研究

预先经验地指定 R F的参数的选取 范围, B 依然可 以优化获得最优 的参数。通过 对头部组 织 电导 率估算 问题进行 对 比研 究, 得 了 取
良好 的效 果 , 证 了该 方 法 的 有 效 性 。 验
关键词
支持 向量机
核 函数 参 数选择
优化算法 分段 函数
oN PARAM ETER SELECTI oN oF KERNEL FUNCTI N o BAS ED oN PTI I o M SATI oN ALGoRI THM
算法进行参数选取 , 也需要预先经验地获得核 函数 的参 数的选取 范 围。在介绍结构风险最小化原则及支持 向量机算 法的基础 上, 但 给 出了基于优化算法 的支持 向量机参 数选取 的一般性算法。 由于径 向基核 函数( B ) R F 的参数取 值大小 的不 同, 可导致其 性质 和作
用不 同, 为此 提 出 了 一 种分 段 函 数 对 R F的 参 数 进 行 选 择 的方 法 , 方 法 使 得 R F 的 参 数 取 大 值 和 小 值 的概 率 均 等 。 由 此 可 不 必 B 该 B
p o ris, O we p o o e a p e e s un to t o rs l ci h a a t rv l fRBF, c ke q a o a iiyi e e tn i — r pete S r p s ic wie f c in me h d f e e tngt e p r me e aueo o whih ma se u pr b blt n s lc ig b g l g ro mal rRBF pa a trv l e. nc h ee to a g fRBF。 r mee sc u d n tbee e rs l e r mee a u He e t e s lci n r n e o pa a tr o l o mpi c ly a p i td bu h ptmu paa e i r a l p o ne tt e o i m r m —
支持向量机

支持向量机支持向量机模型选择研究摘要: 统计学习理论为系统地研究有限样本情况下的机器学习问题提供了一套比较完整的理论体系。
支持向量机(suPportvectorMachine,SVM) 是在该理论体系下产生的一种新的机器学习方法它能较好地解决小样本、非线性、维数灾难和局部极小等问题, 具有很强的泛化能力。
支持向量机目前已经广泛地应用于模式识别、回归估计、概率密度估计等各个领域。
不仅如此, 支持向量机的出现推动了基于核的学习方法(Kernel-based Learning Methods)的迅速发展, 该方法使得研究人员能够高效地分析非线性关系, 而这种高效率原先只有线性算法才能得到。
目前, 以支持向量机为主要代表的核方法是机器学习领域研究的焦点课题之一。
众所周知, 支持向量机的性能主要取决于两个因素:(1) 核函数的选择;(2) 惩罚系数(正则化参数)C的选择。
对于具体的问题,如何确定SVM中的核函数与惩罚系数就是所谓的模型选择问题。
模型选择,尤其是核函数的选择是支持向量机研究的中心内容之一。
本文针对模型选择问题,特别是核函数的选择问题进行了较为深入的研究。
其中主要的内容如下:1. 系统地归纳总结了统计学习理论、核函数特征空间和支持向量机的有关理论与算法。
2. 研究了SVM参数的基本语义,指出数据集中的不同特征和不同样本对分类结果的影响可以分别由核参数和惩罚系数来刻画,从而样木重要性和特征重要性的考察可以归结到SVM的模型选择问题来研究。
在对样本加权svM莫型(例如模糊SVM分析的基础上,运用了特征加权svM莫型, 即FWSVM本质上就是SVM与特征加权的结合。
3,在系统归纳总结SVM莫型选择。
尤其是核函数参数选择的常用方法(例如交叉验证技术、最小化LOO M差及其上界、优化核评估标准)。
关键词:机器学习;莫式分类;支持向量机;莫型选择;核函数; 核函数评估支持向量机基础引言机器学习的科学基础之一是统计学。
支持向量机的核函数选择技巧
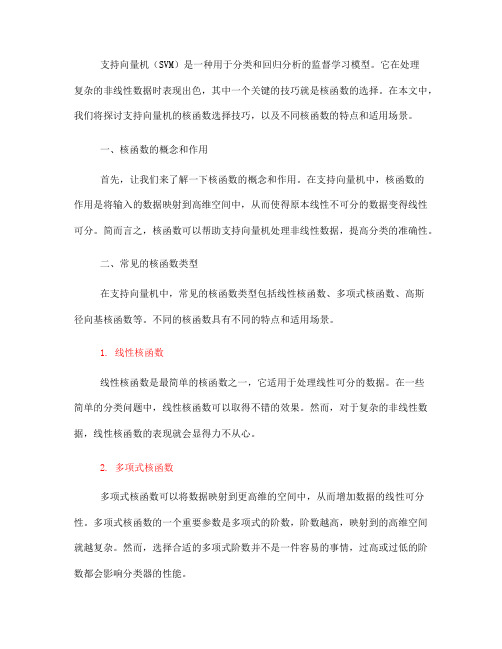
支持向量机(SVM)是一种用于分类和回归分析的监督学习模型。
它在处理复杂的非线性数据时表现出色,其中一个关键的技巧就是核函数的选择。
在本文中,我们将探讨支持向量机的核函数选择技巧,以及不同核函数的特点和适用场景。
一、核函数的概念和作用首先,让我们来了解一下核函数的概念和作用。
在支持向量机中,核函数的作用是将输入的数据映射到高维空间中,从而使得原本线性不可分的数据变得线性可分。
简而言之,核函数可以帮助支持向量机处理非线性数据,提高分类的准确性。
二、常见的核函数类型在支持向量机中,常见的核函数类型包括线性核函数、多项式核函数、高斯径向基核函数等。
不同的核函数具有不同的特点和适用场景。
1. 线性核函数线性核函数是最简单的核函数之一,它适用于处理线性可分的数据。
在一些简单的分类问题中,线性核函数可以取得不错的效果。
然而,对于复杂的非线性数据,线性核函数的表现就会显得力不从心。
2. 多项式核函数多项式核函数可以将数据映射到更高维的空间中,从而增加数据的线性可分性。
多项式核函数的一个重要参数是多项式的阶数,阶数越高,映射到的高维空间就越复杂。
然而,选择合适的多项式阶数并不是一件容易的事情,过高或过低的阶数都会影响分类器的性能。
3. 高斯径向基核函数高斯径向基核函数是支持向量机中最常用的核函数之一,也被称为RBF核函数。
它具有良好的非线性拟合能力,适用于处理复杂的非线性数据。
高斯径向基核函数有一个重要的参数σ,控制了数据映射到高维空间后的分布情况。
选择合适的σ值对支持向量机的性能影响巨大。
三、核函数选择的技巧在实际应用中,选择合适的核函数是非常关键的。
以下是一些核函数选择的技巧:1. 根据数据特点选择核函数在选择核函数时,需要根据数据的特点来进行选择。
如果数据是线性可分的,可以选择线性核函数;如果数据是非线性的,可以考虑使用多项式核函数或高斯径向基核函数。
2. 调参优化在使用多项式核函数或高斯径向基核函数时,需要对核函数的参数进行调参优化。
SVM算法核函数的选择
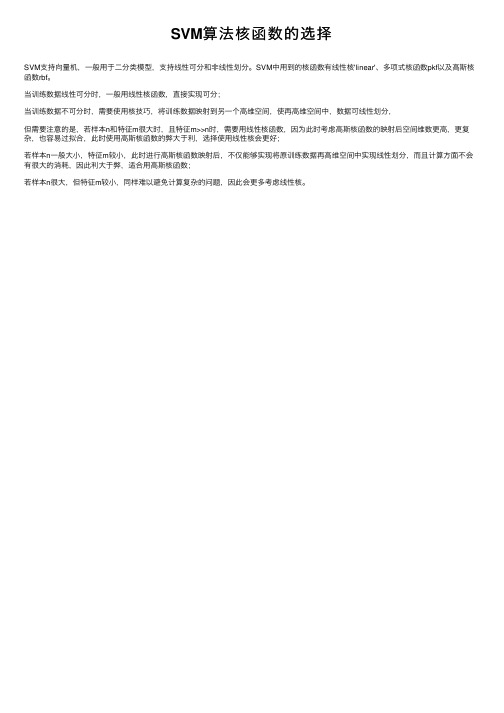
SVM算法核函数的选择
SVM⽀持向量机,⼀般⽤于⼆分类模型,⽀持线性可分和⾮线性划分。
SVM中⽤到的核函数有线性核'linear'、多项式核函数pkf以及⾼斯核函数rbf。
当训练数据线性可分时,⼀般⽤线性核函数,直接实现可分;
当训练数据不可分时,需要使⽤核技巧,将训练数据映射到另⼀个⾼维空间,使再⾼维空间中,数据可线性划分,
但需要注意的是,若样本n和特征m很⼤时,且特征m>>n时,需要⽤线性核函数,因为此时考虑⾼斯核函数的映射后空间维数更⾼,更复杂,也容易过拟合,此时使⽤⾼斯核函数的弊⼤于利,选择使⽤线性核会更好;
若样本n⼀般⼤⼩,特征m较⼩,此时进⾏⾼斯核函数映射后,不仅能够实现将原训练数据再⾼维空间中实现线性划分,⽽且计算⽅⾯不会有很⼤的消耗,因此利⼤于弊,适合⽤⾼斯核函数;
若样本n很⼤,但特征m较⼩,同样难以避免计算复杂的问题,因此会更多考虑线性核。
rbf核函数g取值范围问题

rbf核函数g取值范围问题【主题】rbf核函数g取值范围问题【导言】在机器学习领域,支持向量机(Support Vector Machine,简称SVM)是一种常用且强大的学习算法。
SVM通过核函数将非线性问题映射到高维特征空间,并通过找到最优分割超平面来解决分类问题。
在SVM中,径向基函数核函数(Radial Basis Function Kernel,简称RBF核函数)是一种常用的核函数。
然而,在使用RBF核函数时,我们需要关注它的参数g(gamma)的取值范围,以保证模型能够正确地学习和泛化。
本文将深入探讨RBF核函数g的取值范围问题,帮助读者更好地理解和应用SVM模型。
【正文】1. RBF核函数简介RBF核函数是SVM中最常用的核函数之一。
它的定义是一个关于特征空间中的两个向量之间距离的非线性函数。
在SVM中,RBF核函数的表达式为:K(x, y) = exp(-g * ||x - y||^2)其中,x和y是输入向量,在特征空间中表示样本数据的特征,||x - y||^2表示输入向量x与y之间的欧氏距离的平方,g是RBF核函数的一个参数,也称为gamma。
2. 参数g的作用与影响参数g在RBF核函数中起着重要的作用,它决定了样本点对分类器的影响程度。
参数g越大,每个样本点对分类器的影响越小,决策边界将会更加平滑;参数g越小,每个样本点对分类器的影响越大,决策边界将会更加复杂。
选取合适的参数g对于SVM模型的性能和泛化能力至关重要。
3. 参数g的取值范围在实际应用中,选取合适的参数g并不是一件容易的事情。
通常,我们可以尝试不同的取值范围,并通过交叉验证的方法来选择最优的参数。
在具体操作时,可以考虑以下几种策略:3.1 根据数据的分布情况选取g的初始范围我们可以通过观察数据的分布情况来初步确定参数g的取值范围。
如果数据具有明显的簇状结构,可以选择较小的g值,以保证决策边界可以更好地适应数据的密度变化。
支持向量机的核函数选择技巧(Ⅰ)

支持向量机(Support Vector Machine,SVM)是一种常用的机器学习算法,它在数据分类和回归问题上表现出色。
在SVM中,核函数的选择对算法的性能至关重要。
本文将讨论核函数选择的技巧,以帮助读者更好地理解SVM算法,并在实际应用中取得更好的效果。
一、核函数的作用在支持向量机中,核函数的作用是将输入数据从原始空间映射到更高维的特征空间,使得数据在新的空间中更容易分离。
核函数将数据进行非线性映射,从而允许SVM在非线性问题上进行分类。
常用的核函数包括线性核、多项式核、高斯核等。
二、核函数的选择原则在实际应用中,选择合适的核函数对SVM算法的性能至关重要。
下面是一些核函数选择的原则:1. 数据特征分布:在选择核函数时,需要考虑数据在原始空间中的分布情况。
如果数据在原始空间中是线性可分的,那么可以选择线性核函数。
如果数据在原始空间中是非线性可分的,那么需要选择非线性核函数。
2. 数据维度:数据维度越高,对核函数的选择就越敏感。
在高维数据上,多项式核函数和高斯核函数通常表现更好。
3. 计算复杂度:不同的核函数对应着不同的计算复杂度。
在实际应用中,需要根据数据规模和计算资源来选择合适的核函数,以兼顾算法的性能和计算效率。
三、常用核函数介绍1. 线性核函数线性核函数是最简单的核函数之一,它将数据直接映射到高维空间中。
线性核函数通常适用于数据在原始空间中是线性可分的情况,计算复杂度低,训练速度快。
然而,在处理非线性问题时,线性核函数的表现往往不理想。
2. 多项式核函数多项式核函数将数据映射到更高维的空间,通过多项式函数来处理非线性问题。
多项式核函数的一个重要参数是指数,它控制了数据在新空间中的曲率。
在实际应用中,需要根据数据的特性和非线性程度来选择合适的指数。
3. 高斯核函数高斯核函数是一种常用的非线性核函数,它将数据映射到无穷维的特征空间中。
高斯核函数的一个重要参数是带宽,它控制了数据在新空间中的分布。
kriging代理模型的高斯核函数 指数核函数

kriging代理模型的高斯核函数指数核函数在克里金(Kriging)代理模型中,核函数是评估样本点之间相关性的重要工具。
常见的核函数包括高斯核函数和指数核函数。
本文将重点介绍这两种核函数及其在克里金代理模型中的应用。
高斯核函数是一种常见的克里金核函数,也被称为径向基函数(Radial basis function)。
高斯核函数的形式如下:K(x, x') = exp(-||x - x'||^2 / (2 * h^2))其中,x和x'表示样本点,||x - x'||表示样本点之间的欧式距离,h是平滑参数。
高斯核函数的特点是中心对称,距离样本点越远,相关性越低。
指数核函数也是一种常见的克里金核函数,其形式如下:K(x, x') = exp(-||x - x'|| / (2 * h^2))指数核函数也具有中心对称性,但和高斯核函数不同的是,它的相关性随距离指数级递减。
克里金代理模型根据核函数来评估样本点之间的相关性,并用于预测未知位置上的数值。
首先,通过拟合已知数据点,构建一个适当的函数模型,然后利用该模型对未知数据点进行预测。
核函数在克里金模型中起到了连接已知数据点和未知数据点的重要作用。
其中,高斯核函数通过平滑参数h来控制相关性的程度。
当h 的值较大时,样本点之间的相关性较强,反之则较弱。
在实际应用中,根据具体问题的需求,可以通过交叉验证等方法来确定最合适的h的值。
指数核函数中的平滑参数h同样也用于控制相关性。
不同于高斯核函数,指数核函数的相关性随距离的增加呈指数级递减。
因此,指数核函数适用于那些样本点之间的关联距离较近,且距离增加会导致相关性迅速下降的情况。
选择合适的核函数是克里金模型成功应用的关键。
需要根据实际问题的特点来决定使用哪种核函数以及合适的平滑参数。
在选择核函数时,需要考虑到数据的空间相关性、平稳性、光滑性等因素。
一般来说,核函数的选择应尽可能符合样本数据的特点。
硕士研究生教学实践教案(3篇)

第1篇课程名称:高级数据挖掘与机器学习授课对象:硕士研究生授课时间:4学时授课地点:多媒体教室教学目标:1. 理解高级数据挖掘与机器学习的基本概念和原理。
2. 掌握常用的数据挖掘和机器学习算法,如支持向量机、决策树、聚类算法等。
3. 能够运用所学知识解决实际问题,如数据预处理、特征选择、模型训练与评估等。
4. 培养学生的团队合作能力和独立思考能力。
教学内容:一、绪论1. 数据挖掘与机器学习的定义和发展历程2. 数据挖掘与机器学习在各个领域的应用3. 本课程的学习目标和内容安排二、数据预处理1. 数据清洗:缺失值处理、异常值处理、重复值处理2. 数据集成:数据合并、数据转换3. 数据规约:数据采样、特征选择、特征提取三、特征选择1. 特征选择的重要性2. 常用特征选择方法:过滤式、包裹式、嵌入式3. 特征选择实例分析四、分类算法1. 决策树:ID3、C4.5、CART2. 支持向量机:SVM原理、核函数选择3. 随机森林:原理、应用五、聚类算法1. K-means算法:原理、优缺点2. 层次聚类:原理、算法3. 密度聚类:DBSCAN算法六、关联规则挖掘1. 关联规则挖掘的基本概念2. Apriori算法:原理、优缺点3. FP-growth算法:原理、应用七、实例分析与讨论1. 实例介绍:数据集、问题背景2. 数据预处理3. 特征选择与提取4. 模型训练与评估5. 结果分析与讨论教学过程:第一课时1. 导入:通过实例引入数据挖掘与机器学习的概念,激发学生的学习兴趣。
2. 讲解:详细讲解数据挖掘与机器学习的基本概念和发展历程,介绍其在各个领域的应用。
3. 讨论:引导学生思考数据挖掘与机器学习在实际生活中的应用,激发学生的思考。
4. 实践:布置课后作业,要求学生查找相关资料,了解数据挖掘与机器学习的一个具体应用案例。
第二课时1. 讲解:详细介绍数据预处理的方法,包括数据清洗、数据集成、数据规约。
2. 实践:提供一组数据集,要求学生进行数据预处理,并展示预处理后的数据。
支持向量机的核函数选择技巧(六)

支持向量机(Support Vector Machine,SVM)是一种常用的监督学习算法,广泛应用于分类和回归问题。
在实际应用中,选择合适的核函数是支持向量机模型中非常重要的一环。
本文将从数学原理和实际应用两个角度,探讨支持向量机的核函数选择技巧。
一、核函数的数学原理支持向量机的核函数在数学原理上是非常有深度的。
核函数的本质是将非线性可分的问题映射到高维空间中,使得原本线性不可分的问题在高维空间中变成线性可分的。
这样一来,支持向量机就可以通过寻找最优超平面来进行分类或回归。
在支持向量机中,常用的核函数包括线性核函数、多项式核函数、高斯核函数等。
不同的核函数具有不同的特点和适用范围。
例如,线性核函数适用于线性可分的问题;多项式核函数适用于中等复杂度的非线性问题;高斯核函数则适用于复杂的非线性问题。
在实际应用中,选择合适的核函数需要综合考虑问题的特点、数据的分布以及算法的性能。
下面将从实际应用的角度,继续探讨核函数选择的技巧。
二、核函数的选择技巧1. 理解问题的特点在选择核函数时,首先需要充分理解问题的特点。
如果问题是线性可分的,那么直接选择线性核函数即可;如果问题是非线性的,就需要考虑使用多项式核函数或高斯核函数。
举个例子,假设我们要解决一个图像识别的问题,图像数据通常是非线性的,这时就需要考虑使用多项式核函数或高斯核函数来处理。
2. 考虑数据的分布数据的分布对核函数的选择也有很大的影响。
如果数据集是均匀分布的,那么可以考虑使用多项式核函数;如果数据集呈现出明显的聚集现象,就需要考虑使用高斯核函数。
此外,数据的维度也会对核函数的选择产生影响。
在高维空间中,线性核函数的效果可能会比较好;而在低维空间中,需要考虑使用多项式核函数或高斯核函数。
3. 算法性能的考量在实际选择核函数时,还需要考虑算法的性能。
不同的核函数会对算法的计算复杂度和收敛速度产生不同的影响。
因此,需要综合考虑问题的复杂度和算法的性能,选择合适的核函数。
核密度估计h选取
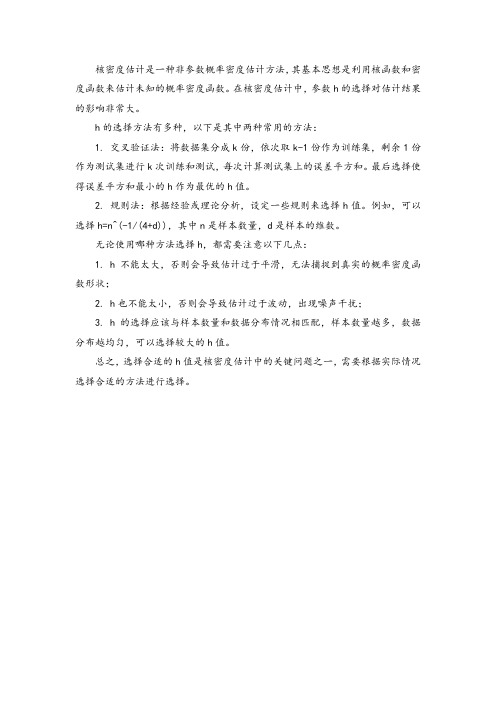
核密度估计是一种非参数概率密度估计方法,其基本思想是利用核函数和密度函数来估计未知的概率密度函数。
在核密度估计中,参数h的选择对估计结果的影响非常大。
h的选择方法有多种,以下是其中两种常用的方法:
1. 交叉验证法:将数据集分成k份,依次取k-1份作为训练集,剩余1份作为测试集进行k次训练和测试,每次计算测试集上的误差平方和。
最后选择使得误差平方和最小的h作为最优的h值。
2. 规则法:根据经验或理论分析,设定一些规则来选择h值。
例如,可以选择h=n^(-1/(4+d)),其中n是样本数量,d是样本的维数。
无论使用哪种方法选择h,都需要注意以下几点:
1. h不能太大,否则会导致估计过于平滑,无法捕捉到真实的概率密度函数形状;
2. h也不能太小,否则会导致估计过于波动,出现噪声干扰;
3. h的选择应该与样本数量和数据分布情况相匹配,样本数量越多,数据分布越均匀,可以选择较大的h值。
总之,选择合适的h值是核密度估计中的关键问题之一,需要根据实际情况选择合适的方法进行选择。
基于SVR组合模型的短期电力负荷预测
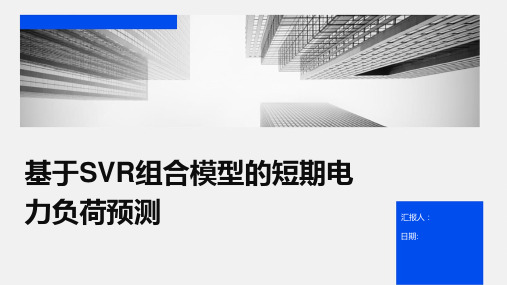
• SVR组合模型能够充分利用支持向量回归在处理小样本 和非线性问题上的优势,提高预测精度。
• 通过组合多个单一模型,可以降低单一模型的风险, 提高整体预测的稳定性。
局限性
• SVR组合模型对参数选择较为敏感,不同的参数组合可 能导致性能波动较大。
• 在处理大规模数据集时,SVR组合模型的训练时间和计 算资源消耗可能较高。因此,在实际应用中需要权衡 模型性能和计算成本。
考虑多种因素的组合模型构建策略
基于时间序列的组合模型
在构建组合模型时,可以充分考虑电力负荷 时间序列的特性,结合ARIMA等时间序列 模型,构建基于时间序列的组合模型,提高 预测的稳定性。
多尺度组合模型
针对不同时间尺度的负荷预测需求,可以构 建多尺度组合模型,综合考虑长期趋势、季 节变化、日周期等多种因素,提高预测的准
考虑新能源和智能电网的影响
随着新能源的大规模接入和智能电网的建设,电力负荷特性和影响因素将发生变化。未来研究需要关注 这些新变化,对现有预测方法进行适应性改进。
THANK YOU
正则化参数C:正则化参数C 控制模型的复杂度与误差之 间的权衡。较大的C值可能 导致过拟合,较小的C值可 能导致欠拟合。需要通过网 格搜索、交叉验证等方法寻 找最佳C值。
核函数参数:对于非线性核 函数,如多项式核和径向基 核,还需要确定相应的核函 数参数。这些参数同样可以 通过交叉验证、网格搜索等 方法进行优化。
数据来源
本研究所使用的数据来源于某地区电力系统的历史负荷数据,包括天气、节假 日、电价等多种影响因素。
数据预处理
对原始数据进行清洗、归一化和特征提取等预处理操作,以提高模型的训练效 率和预测精度。例如,处理缺失值、异常值,对数据进行标准化处理,以及提 取与负荷相关的特征等。
支持向量机中常用的核函数及选择技巧
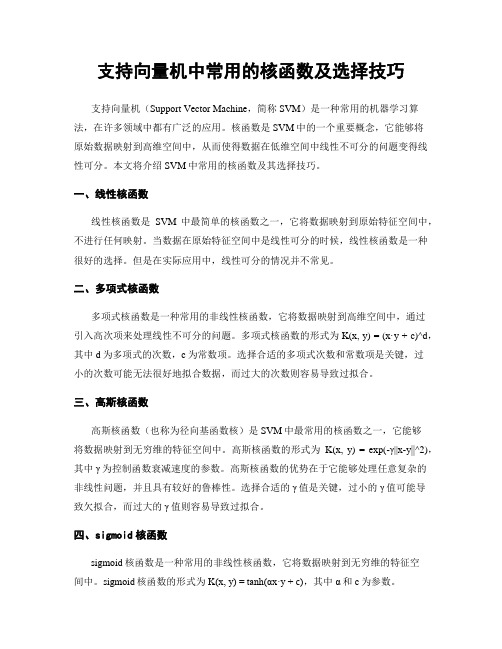
支持向量机中常用的核函数及选择技巧支持向量机(Support Vector Machine,简称SVM)是一种常用的机器学习算法,在许多领域中都有广泛的应用。
核函数是SVM中的一个重要概念,它能够将原始数据映射到高维空间中,从而使得数据在低维空间中线性不可分的问题变得线性可分。
本文将介绍SVM中常用的核函数及其选择技巧。
一、线性核函数线性核函数是SVM中最简单的核函数之一,它将数据映射到原始特征空间中,不进行任何映射。
当数据在原始特征空间中是线性可分的时候,线性核函数是一种很好的选择。
但是在实际应用中,线性可分的情况并不常见。
二、多项式核函数多项式核函数是一种常用的非线性核函数,它将数据映射到高维空间中,通过引入高次项来处理线性不可分的问题。
多项式核函数的形式为K(x, y) = (x·y + c)^d,其中d为多项式的次数,c为常数项。
选择合适的多项式次数和常数项是关键,过小的次数可能无法很好地拟合数据,而过大的次数则容易导致过拟合。
三、高斯核函数高斯核函数(也称为径向基函数核)是SVM中最常用的核函数之一,它能够将数据映射到无穷维的特征空间中。
高斯核函数的形式为K(x, y) = exp(-γ||x-y||^2),其中γ为控制函数衰减速度的参数。
高斯核函数的优势在于它能够处理任意复杂的非线性问题,并且具有较好的鲁棒性。
选择合适的γ值是关键,过小的γ值可能导致欠拟合,而过大的γ值则容易导致过拟合。
四、sigmoid核函数sigmoid核函数是一种常用的非线性核函数,它将数据映射到无穷维的特征空间中。
sigmoid核函数的形式为K(x, y) = tanh(αx·y + c),其中α和c为参数。
sigmoid核函数在某些情况下可以取得不错的效果,但是相对于高斯核函数来说,它的性能较差。
因此,在实际应用中,一般更倾向于使用高斯核函数。
在选择核函数时,需要根据具体的问题和数据集的特点来进行选择。
支持向量机的核函数选择指南
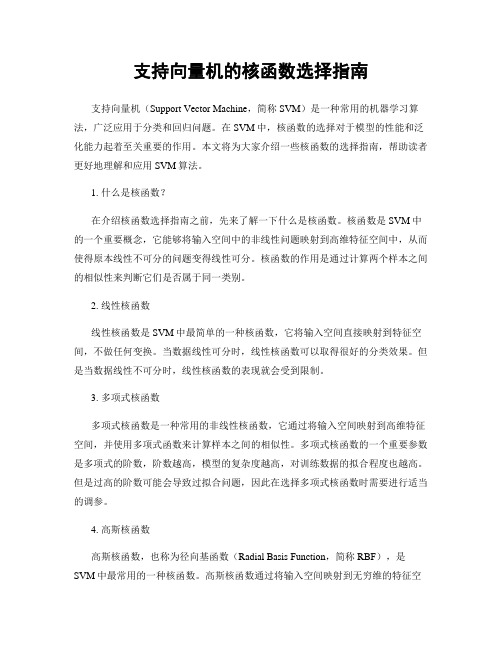
支持向量机的核函数选择指南支持向量机(Support Vector Machine,简称SVM)是一种常用的机器学习算法,广泛应用于分类和回归问题。
在SVM中,核函数的选择对于模型的性能和泛化能力起着至关重要的作用。
本文将为大家介绍一些核函数的选择指南,帮助读者更好地理解和应用SVM算法。
1. 什么是核函数?在介绍核函数选择指南之前,先来了解一下什么是核函数。
核函数是SVM中的一个重要概念,它能够将输入空间中的非线性问题映射到高维特征空间中,从而使得原本线性不可分的问题变得线性可分。
核函数的作用是通过计算两个样本之间的相似性来判断它们是否属于同一类别。
2. 线性核函数线性核函数是SVM中最简单的一种核函数,它将输入空间直接映射到特征空间,不做任何变换。
当数据线性可分时,线性核函数可以取得很好的分类效果。
但是当数据线性不可分时,线性核函数的表现就会受到限制。
3. 多项式核函数多项式核函数是一种常用的非线性核函数,它通过将输入空间映射到高维特征空间,并使用多项式函数来计算样本之间的相似性。
多项式核函数的一个重要参数是多项式的阶数,阶数越高,模型的复杂度越高,对训练数据的拟合程度也越高。
但是过高的阶数可能会导致过拟合问题,因此在选择多项式核函数时需要进行适当的调参。
4. 高斯核函数高斯核函数,也称为径向基函数(Radial Basis Function,简称RBF),是SVM中最常用的一种核函数。
高斯核函数通过将输入空间映射到无穷维的特征空间,并使用高斯函数来计算样本之间的相似性。
高斯核函数有一个重要的参数σ,控制着高斯函数的宽度。
较小的σ值会使高斯核函数更加陡峭,模型更加复杂,可能导致过拟合;较大的σ值则会使高斯核函数更加平缓,模型更加简单,可能导致欠拟合。
因此,在选择高斯核函数时,需要根据实际问题进行适当的调参。
5. 其他核函数除了线性核函数、多项式核函数和高斯核函数之外,还有一些其他的核函数可供选择,如Sigmoid核函数和拉普拉斯核函数等。
支持向量机的核函数选择技巧(Ⅲ)

支持向量机(Support Vector Machine,SVM)是一种经典的机器学习算法,被广泛应用于分类和回归问题。
在SVM中,核函数的选择对于模型的性能有着至关重要的影响。
本文将从理论和实践两个角度出发,探讨支持向量机的核函数选择技巧。
首先,我们来了解一下核函数在支持向量机中的作用。
核函数是SVM中的一个关键概念,它可以将输入空间映射到高维特征空间,从而使得原本线性不可分的数据在高维空间中变得线性可分。
这样一来,我们就可以使用线性分类器来对数据进行划分,从而实现对非线性数据的分类。
常见的核函数包括线性核、多项式核、高斯径向基核等。
不同的核函数适用于不同类型的数据,因此在选择核函数时需要根据具体问题和数据特点进行合理的选择。
其次,我们来探讨核函数选择的一些技巧和经验。
首先要考虑的是数据的特征,如果数据特征较为简单,可以尝试使用线性核函数进行建模,这样可以减少模型的复杂度,提高训练和预测的效率。
对于复杂的非线性数据,可以尝试使用多项式核函数或高斯径向基核函数,这些核函数能够更好地捕捉数据之间的复杂关系,提高模型的预测性能。
另外,还可以结合交叉验证的方法来选择最合适的核函数。
通过将数据集划分为训练集和验证集,可以在不同的核函数下进行模型训练和验证,最终选择表现最好的核函数。
这样可以避免在选择核函数时出现过拟合或欠拟合的情况,提高模型的泛化能力。
除了以上方法之外,还可以考虑使用核函数组合的方式。
有时候单一的核函数可能无法很好地满足数据的特点,因此可以尝试将多个核函数进行组合,从而得到更好的拟合效果。
例如,可以使用核函数的加法、乘法或者线性组合的方式来获得新的核函数,这样可以更好地适应各种类型的数据。
在实际应用中,选择合适的核函数需要综合考虑数据特点、问题需求和计算资源等因素。
因此,建议在选择核函数时要充分理解数据特点,结合实际问题进行多方面的考量,最终选择最适合的核函数。
最后,需要指出的是,核函数的选择并不是一成不变的。
支持向量机中核函数选择的指南
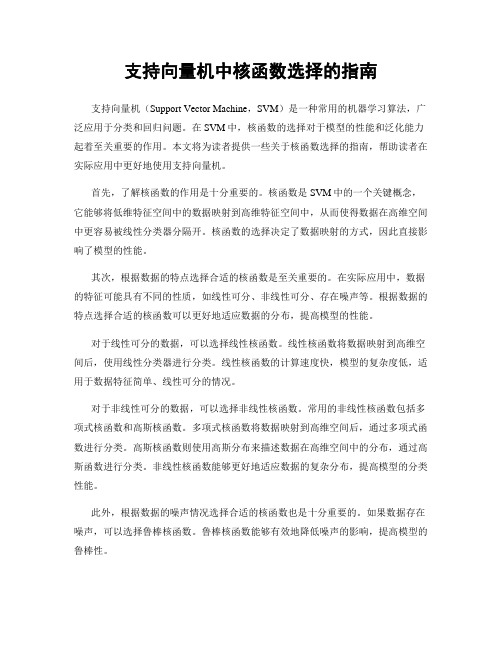
支持向量机中核函数选择的指南支持向量机(Support Vector Machine,SVM)是一种常用的机器学习算法,广泛应用于分类和回归问题。
在SVM中,核函数的选择对于模型的性能和泛化能力起着至关重要的作用。
本文将为读者提供一些关于核函数选择的指南,帮助读者在实际应用中更好地使用支持向量机。
首先,了解核函数的作用是十分重要的。
核函数是SVM中的一个关键概念,它能够将低维特征空间中的数据映射到高维特征空间中,从而使得数据在高维空间中更容易被线性分类器分隔开。
核函数的选择决定了数据映射的方式,因此直接影响了模型的性能。
其次,根据数据的特点选择合适的核函数是至关重要的。
在实际应用中,数据的特征可能具有不同的性质,如线性可分、非线性可分、存在噪声等。
根据数据的特点选择合适的核函数可以更好地适应数据的分布,提高模型的性能。
对于线性可分的数据,可以选择线性核函数。
线性核函数将数据映射到高维空间后,使用线性分类器进行分类。
线性核函数的计算速度快,模型的复杂度低,适用于数据特征简单、线性可分的情况。
对于非线性可分的数据,可以选择非线性核函数。
常用的非线性核函数包括多项式核函数和高斯核函数。
多项式核函数将数据映射到高维空间后,通过多项式函数进行分类。
高斯核函数则使用高斯分布来描述数据在高维空间中的分布,通过高斯函数进行分类。
非线性核函数能够更好地适应数据的复杂分布,提高模型的分类性能。
此外,根据数据的噪声情况选择合适的核函数也是十分重要的。
如果数据存在噪声,可以选择鲁棒核函数。
鲁棒核函数能够有效地降低噪声的影响,提高模型的鲁棒性。
最后,通过交叉验证选择最优的核函数参数也是核函数选择的重要步骤。
在实际应用中,核函数通常具有一些参数,如多项式核函数的次数、高斯核函数的带宽等。
通过交叉验证,可以选择最优的核函数参数,提高模型的泛化能力。
综上所述,核函数的选择对于支持向量机的性能和泛化能力至关重要。
根据数据的特点选择合适的核函数,可以更好地适应数据的分布,提高模型的分类性能。
基于Gram-Schmidt正交化和HSIC的核函数选择方法

基于Gram-Schmidt正交化和HSIC的核函数选择方法高雅田;贾斯淇
【期刊名称】《计算机技术与发展》
【年(卷),期】2024(34)6
【摘要】核方法是一种解决非线性、异构数据的有效方法,核函数的选择问题是核方法中的一个重要课题,对于不同的应用问题,如何选择合适的核函数还没有足够的理论基础,不适当的核函数选取会降低核方法的性能。
由此,提出了一种基于Gram-Schmidt正交化(GSO)和Hilbert-Schmidt独立准则的核选择方法(HSIC-GSO),该方法考虑了核函数选择过程中存在的不相关冗余信息。
首先,利用GSO消除核函数之间的冗余信息;然后,使用HSIC度量核函数与理想核之间的相似性;最后,得到一组判别能力强、多样性大的基核函数。
实验结果表明,HSIC-GSO方法选择的核函数泛化性好,并且提高了MKL的分类性能,验证了所提方法的有效性。
【总页数】7页(P148-154)
【作者】高雅田;贾斯淇
【作者单位】东北石油大学计算机与信息技术学院
【正文语种】中文
【中图分类】TP301
【相关文献】
1.基于Gram-Schmidt正交化法的串行干扰消除算法
2.基于Gram-Schmidt正交化的线阵方向图综合
3.基于树型正交前向选择方法的可调核函数模型
4.基于
Gauss分布和Gram-Schmidt正交化的朴素贝叶斯分类算法5.单边核磁共振磁体梯度磁场设计的Gram-Schmidt正交化拟合方法
因版权原因,仅展示原文概要,查看原文内容请购买。
- 1、下载文档前请自行甄别文档内容的完整性,平台不提供额外的编辑、内容补充、找答案等附加服务。
- 2、"仅部分预览"的文档,不可在线预览部分如存在完整性等问题,可反馈申请退款(可完整预览的文档不适用该条件!)。
- 3、如文档侵犯您的权益,请联系客服反馈,我们会尽快为您处理(人工客服工作时间:9:00-18:30)。
龙源期刊网
核函数选择方法研究
作者:王振武何关瑶
来源:《湖南大学学报·自然科学版》2018年第10期
摘要:核函数的选择对支持向量机的分类结果有着重要的影响,为了提高核函数选择的客观性,提出了一种以错分实例到支持向量所在界面的距离来表示错分程度,并基于此进行秩和检验的核函数选择方法.通过与K折交叉验证、配对t测试等参数检验的统计方法进行对比
分析,对9种常用核函数的分类能力在15个数据集进行了定量研究.与参数检验方法不同,秩和检验并未假定数据的分布情况(很多情况下数据并不满足假定的分布),而且数据实验证明,秩和检验不但能够对核函数的分类能力进行客观评估,而且在某些数据集上还能产生更好的核函数选择效果.
关键词:核函数;支持向量机;秩和检验; K折交叉验证;配对t测试
中图分类号:TP301.6 文献标志码:A
Abstract:The selection of kernel functions has an important influence on the classification results of support vector machines. This paper proposed a kernel functions selection method based on rank sum test in order to enhance the selection objectivity, where the error degree adopted in the rank sum test was represented by the distance between the error instance and the interface of support vectors. By comparing with other statistical methods, such as Kfolding cross validation and paired t test, the classification abilities of nine common kernel functions were quantitatively studied based on 15 datasets. Different from parameter test methods, the rank sum test does not assume the data distribution(in some cases data cannot satisfy the assumed distribution), the experimental data proves that the rank sum test not only can objectively evaluate the classification abilities of kernel functions, but also can produce better selection results on some data sets.
Key words:kernel function; support vector machines; rank sum test; K folding cross validation; paired t test
支持向量機(Support Vector Machine,SVM)[1]的使用与核函数的正确选择是密不可分的,核函数技术巧妙地解决了在高维特征空间中计算的“维数灾难”等问题,直接决定了SVM 的非线性处理能力[2].当前对核函数选择方法的研究主要集中在构造新的核函数[3-7]、核函数参数选择[8-13]以及核函数的评估[1,14-16]上.由于在使用SVM进行分类的过程中只定义了核函数(并不显式地定义映射函数),所以在同一分类问题上选择不同的核函数对分类效果影响较大,另外映射函数的类型是多变的,在没有先验知识的情况下人们更多地是凭借主观经验进行核函数的选择,具有较大的随意性.。